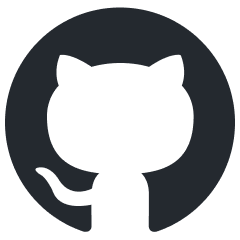
Steel-LLM
Train a 1B LLM with 1T tokens from scratch by personal
Stars: 560
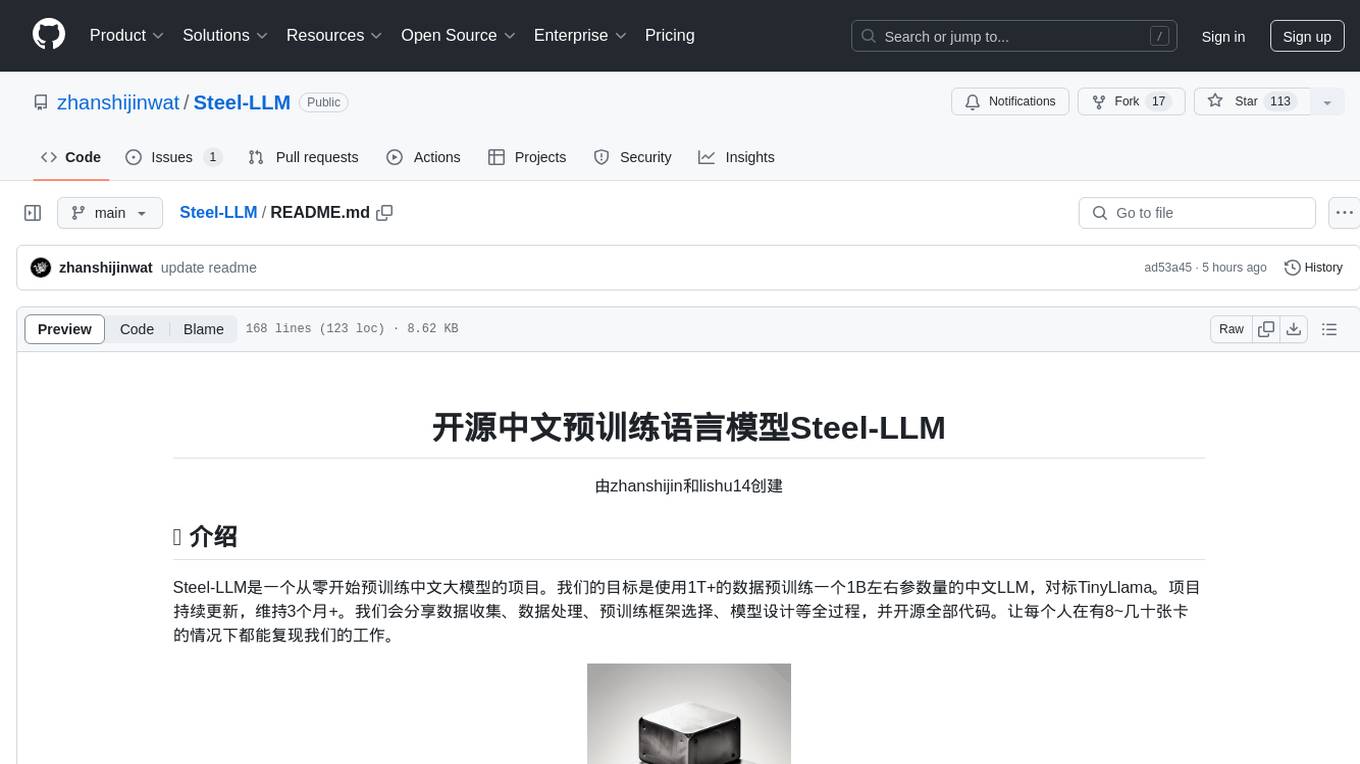
Steel-LLM is a project to pre-train a large Chinese language model from scratch using over 1T of data to achieve a parameter size of around 1B, similar to TinyLlama. The project aims to share the entire process including data collection, data processing, pre-training framework selection, model design, and open-source all the code. The goal is to enable reproducibility of the work even with limited resources. The name 'Steel' is inspired by a band '万能青年旅店' and signifies the desire to create a strong model despite limited conditions. The project involves continuous data collection of various cultural elements, trivia, lyrics, niche literature, and personal secrets to train the LLM. The ultimate aim is to fill the model with diverse data and leave room for individual input, fostering collaboration among users.
README:
[ 中文 | English ]
Steel-LLM是个人发起的从零预训练中文大模型项目。我们使用了1T token的数据预训练一个1B左右参数量的中文LLM。项目从开始到微调出第一版模型耗时了8个月。我们详细的分享了数据收集、数据处理、预训练框架选择、模型设计等全过程,并开源全部代码。让每个人在有8~几十张卡的情况下都能复现我们的工作。得益于开源中文数据,Steel-LLM在中文benchmark上表现优于机构早期发布的一些更大的模型,在ceval达到了42分,cmmlu达到了36分。
"Steel(钢)"取名灵感来源于华北平原一只优秀的乐队“万能青年旅店(万青)”。乐队在做一专的时候条件有限,自称是在“土法炼钢”,但却是一张神专。我们训练LLM的条件同样有限,但也希望能炼出好“钢”来。
后续会在数学能力、强化学习、复杂推理等方面进一步探索......
[2025/3/6] 🎉🎉🎉《Steel-LLM:From Scratch to Open Source -- A Personal Journey in Building a Chinese-Centric LLM》被ICLR 2025 workshop 接收
[2025/2/13] 上传了技术报告:https://arxiv.org/abs/2502.06635
[2025/1/17] 更新steel-LLM-chat-v2,微调时加入了英文数据,中英文数据比例和预训练保持一致,最终在ceval上由38分提高到了41.9分,cmmlu从33分提高到了36分。
[2024/11/13] 🔥发布一篇项目汇总文章《个人从零预训练1B LLM心路历程》:https://mp.weixin.qq.com/s/POUugkCNZTzmlKWZVVD1CQ🔥
[2024/10/28]更新了第一版chat模型,在ceval达到了38分,cmmlu达到了33分。
[2024/10/24]发布了Steel-LLM微调和评估的细节。微调时探索了cot、模型刷榜等实验。博客地址:https://mp.weixin.qq.com/s/KK0G0spNw0D9rPUESkHMew
[2024/9/2] HuggingFace更新了480k、660k、720k、980k、1060k(最后一个checkpoint)step的checkpoint。
[2024/8/18] 预训练已经完成,后续进行微调以及评测
[2024/7/18] 使用8*H800继续训练,wandb:https://api.wandb.ai/links/steel-llm-lab/vqf297nr
[2024/6/30] 放出预训练200k个step的checkpoint,huggingface链接
[2024/5/21] 模型开启正式训练,后续不定期放出checkpoint。
[2024/5/19] 基于Qwen1.5完成模型修改,模型大小1.12B:
- FFN层使用softmax moe,相同参数量下有更高的训练速度
- 使用双层的SwiGLU
相关博客:https://zhuanlan.zhihu.com/p/700395878
[2024/5/5] 预训练程序修改相关的博客:https://zhuanlan.zhihu.com/p/694223107
[2024/4/24] 完成训练程序改进:兼容Hugginface格式模型、支持数据断点续训、支持追加新的数据
[2024/4/14] 完成数据收集与处理,生成预训练程序所需要的bin文件。更新数据收集与处理相关的博客:https://zhuanlan.zhihu.com/p/687338497
欢迎加入交流群,人数已超过200,添加微信入群:a1843450905
使用的数据集和链接如下所示,更详细的介绍见此篇文章
- Skywork/Skypile-150B数据集
- wanjuan1.0(nlp部分)
- 中文维基过滤数据
- 百度百科数据
- 百度百科问答数据
- 知乎问答数据
- BELLE对话数据
- moss项目对话数据
- firefly1.1M
- starcoder
(详细内容见此篇文章)
- 源数据:针对4类数据进行格式统一的转化处理:
- 简单文本:百度百科(title和各段落需要手动合并)、中文维基
- 对话(含单轮与多轮):百度百科问答数据、BELLE对话数据(BELLE_3_5M)、moss项目对话数据、知乎问答数据、BELLE任务数据(BELLE_2_5M)、firefly1.1M
- 代码数据:starcode
- 其他数据:wanjuan和skypile数据集不用做单独处理
- 目标格式:
{"text": "asdfasdf..."}
,文件保存为.jsonl类型。 - 运行方式:
python data/pretrain_data_prepare/step1_data_process.py
-
运行方式:
sh data/pretrain_data_prepare/step2/run_step2.sh
-
具体使用的data juicer算子见此文档。
需要先在代码中修改filename_sets,指定数据路径,然后运行如下程序:
python pretrain_modify_from_TinyLlama/scripts/prepare_steel_llm_data.py
输入数据格式为:包含'text'字段的jsonl文件
不单独训练tokenizer,使用Qwen/Qwen1.5-MoE-A2.7B-Chat的tokenizer
(详细内容见此篇文章)
基于Qwen1.5模型,进行了如下改动:
- FFN层使用softmax moe,相同参数量下有更高的训练速度
- 使用双层的SwiGLU
(详细内容见此篇文章)
基于TinyLlama预训练程序进行如下改进:
- 兼容HuggingFace格式的模型
- 加载checkpoint时,完全恢复数据训练的进度
- 数据一致性检测
- 在不影响已训练数据的情况下,在数据集中追加新的数据
启动预训练:
python Steel-LLM/pretrain_modify_from_TinyLlama/pretrain/pretrain_steel_llm.py
(详细内容见此篇文章)
Steel-LLM在CEVAL、CMMLU上进行了测试。Steel-LLM旨在训练一个中文LLM,80%的训练数据都是中文,因此在英文benchmark并未做过多的测试。 其他模型的指标来自于CEVAL论文、MiniCPM技术报告、MAP-Neo技术报告等途径。更多模型的指标可查看之前的博客
CEVAL | CMMLU | |
---|---|---|
Steel-LLM-chat-v2 | 41.90 | 36.08 |
Steel-LLM-chat-v1 | 38.57 | 33.48 |
Tiny-Llama-1.1B | 25.02 | 24.03 |
Gemma-2b-it | 32.3 | 33.07 |
Phi2(2B) | 23.37 | 24.18 |
Deepseek-coder-1.3B-instruct | 28.33 | 27.75 |
CT-LLM-SFT-2B | 41.54 | 41.48 |
MiniCPM-2B-sft-fp32 | 49.14 | 51.0 |
Qwen1.5-1.8B-Chat | 56.84 | 54.11 |
ChatGLM-6B | 38.9 | - |
Moss | 33.1 | - |
LLAMA-65B | 34.7 | - |
Qwen-7B | 58.96 | 60.35 |
Gemma-7B | 42.57 | 44.20 |
OLMo-7B | 35.18 | 35.55 |
MAP-NEO-7B | 56.97 | 55.01 |
from modelscope import AutoModelForCausalLM, AutoTokenizer
model_name = "zhanshijin/Steel-LLM"
model = AutoModelForCausalLM.from_pretrained(
model_name,
torch_dtype="auto",
device_map="auto"
)
tokenizer = AutoTokenizer.from_pretrained(model_name)
prompt = "你是谁开发的"
messages = [
{"role": "user", "content": prompt}
]
text = tokenizer.apply_chat_template(
messages,
tokenize=False,
add_generation_prompt=True
)
model_inputs = tokenizer([text], return_tensors="pt").to(model.device)
generated_ids = model.generate(
**model_inputs,
max_new_tokens=512
)
generated_ids = [
output_ids[len(input_ids):] for input_ids, output_ids in zip(model_inputs.input_ids, generated_ids)
]
response = tokenizer.batch_decode(generated_ids, skip_special_tokens=True)[0]
print(response)
GPU:8* H800 80G(训练30天左右)
GPU:8* A100 80G(训练60天左右)
硬盘:4TB
BibTeX:
@article{gu2025steel,
title={Steel-LLM: From Scratch to Open Source--A Personal Journey in Building a Chinese-Centric LLM},
author={Gu, Qingshui and Li, Shu and Zheng, Tianyu and Zhang, Zhaoxiang},
journal={arXiv preprint arXiv:2502.06635},
year={2025}
}
For Tasks:
Click tags to check more tools for each tasksFor Jobs:
Alternative AI tools for Steel-LLM
Similar Open Source Tools
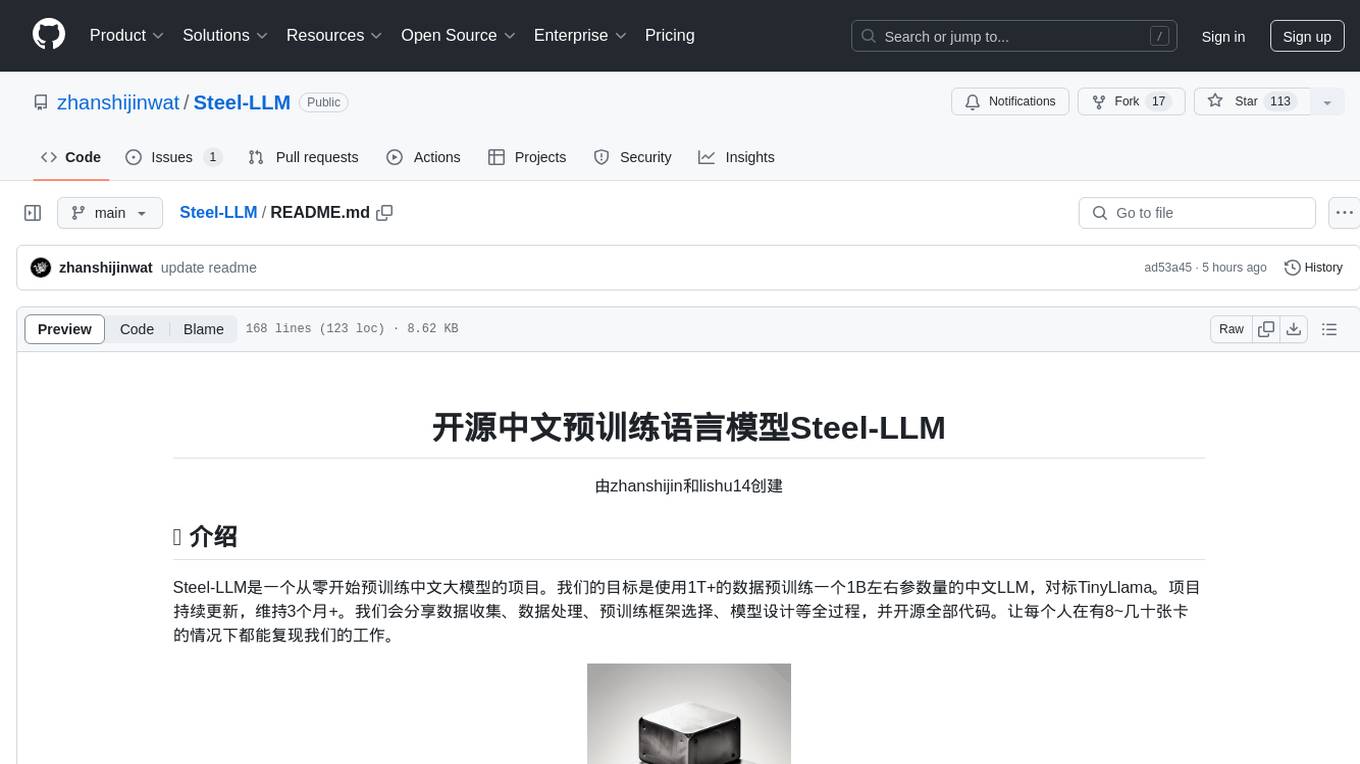
Steel-LLM
Steel-LLM is a project to pre-train a large Chinese language model from scratch using over 1T of data to achieve a parameter size of around 1B, similar to TinyLlama. The project aims to share the entire process including data collection, data processing, pre-training framework selection, model design, and open-source all the code. The goal is to enable reproducibility of the work even with limited resources. The name 'Steel' is inspired by a band '万能青年旅店' and signifies the desire to create a strong model despite limited conditions. The project involves continuous data collection of various cultural elements, trivia, lyrics, niche literature, and personal secrets to train the LLM. The ultimate aim is to fill the model with diverse data and leave room for individual input, fostering collaboration among users.
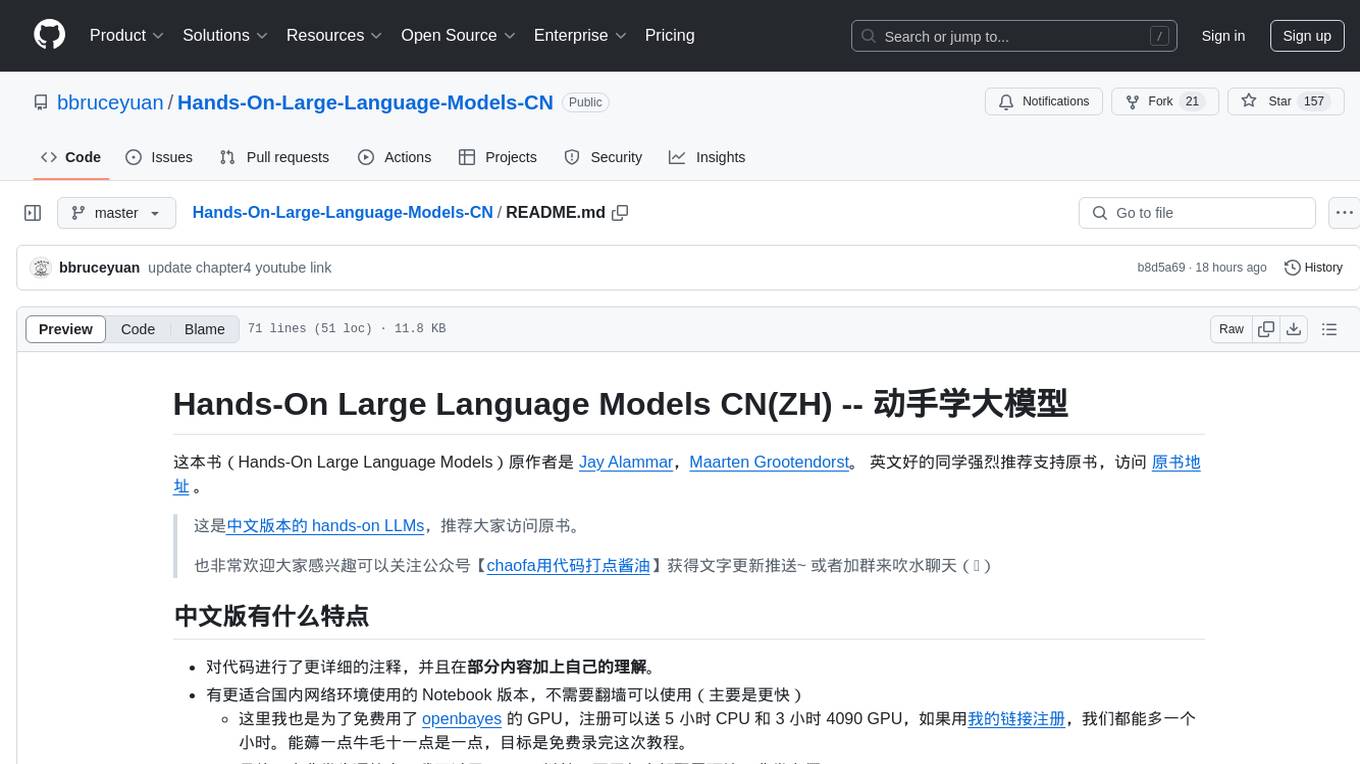
Hands-On-Large-Language-Models-CN
Hands-On Large Language Models CN(ZH) is a Chinese version of the book 'Hands-On Large Language Models' by Jay Alammar and Maarten Grootendorst. It provides detailed code annotations and additional insights, offers Notebook versions suitable for Chinese network environments, utilizes openbayes for free GPU access, allows convenient environment setup with vscode, and includes accompanying Chinese language videos on platforms like Bilibili and YouTube. The book covers various chapters on topics like Tokens and Embeddings, Transformer LLMs, Text Classification, Text Clustering, Prompt Engineering, Text Generation, Semantic Search, Multimodal LLMs, Text Embedding Models, Fine-tuning Models, and more.
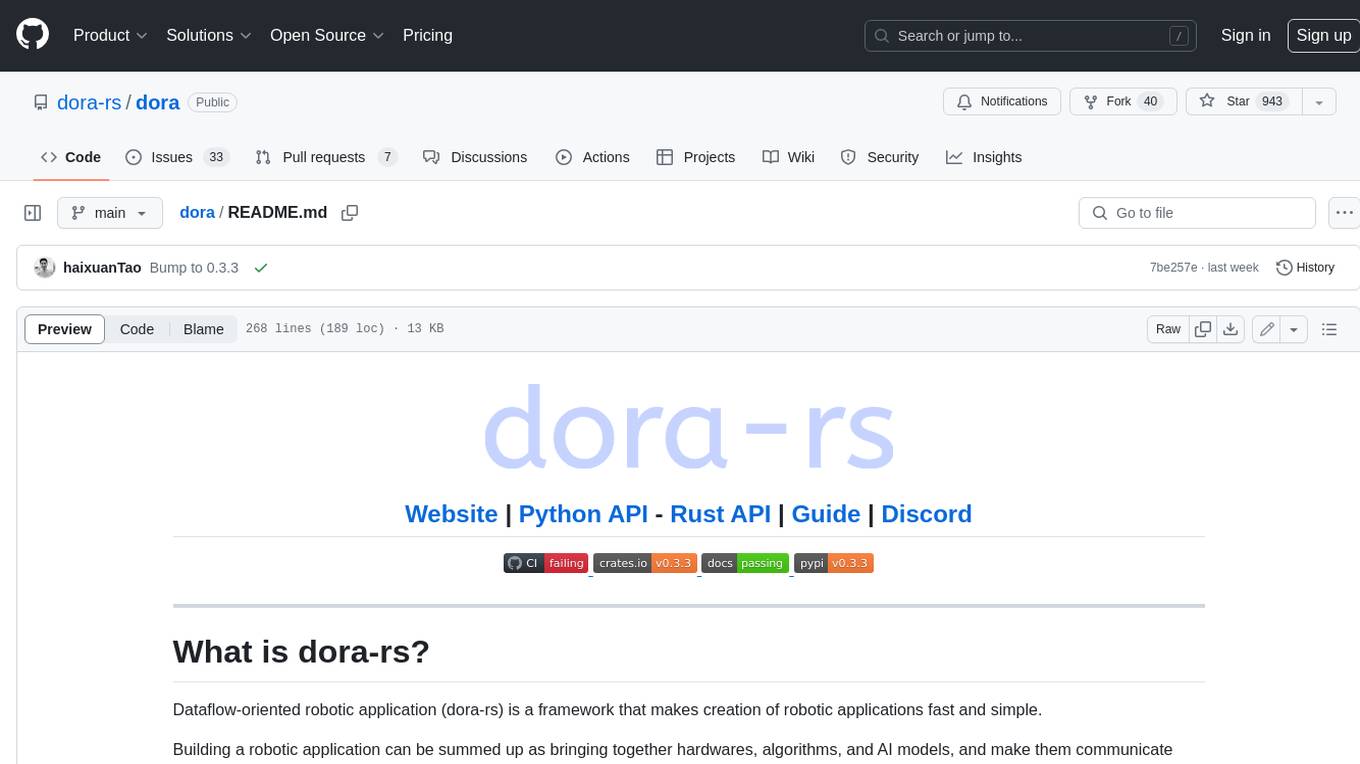
dora
Dataflow-oriented robotic application (dora-rs) is a framework that makes creation of robotic applications fast and simple. Building a robotic application can be summed up as bringing together hardwares, algorithms, and AI models, and make them communicate with each others. At dora-rs, we try to: make integration of hardware and software easy by supporting Python, C, C++, and also ROS2. make communication low latency by using zero-copy Arrow messages. dora-rs is still experimental and you might experience bugs, but we're working very hard to make it stable as possible.
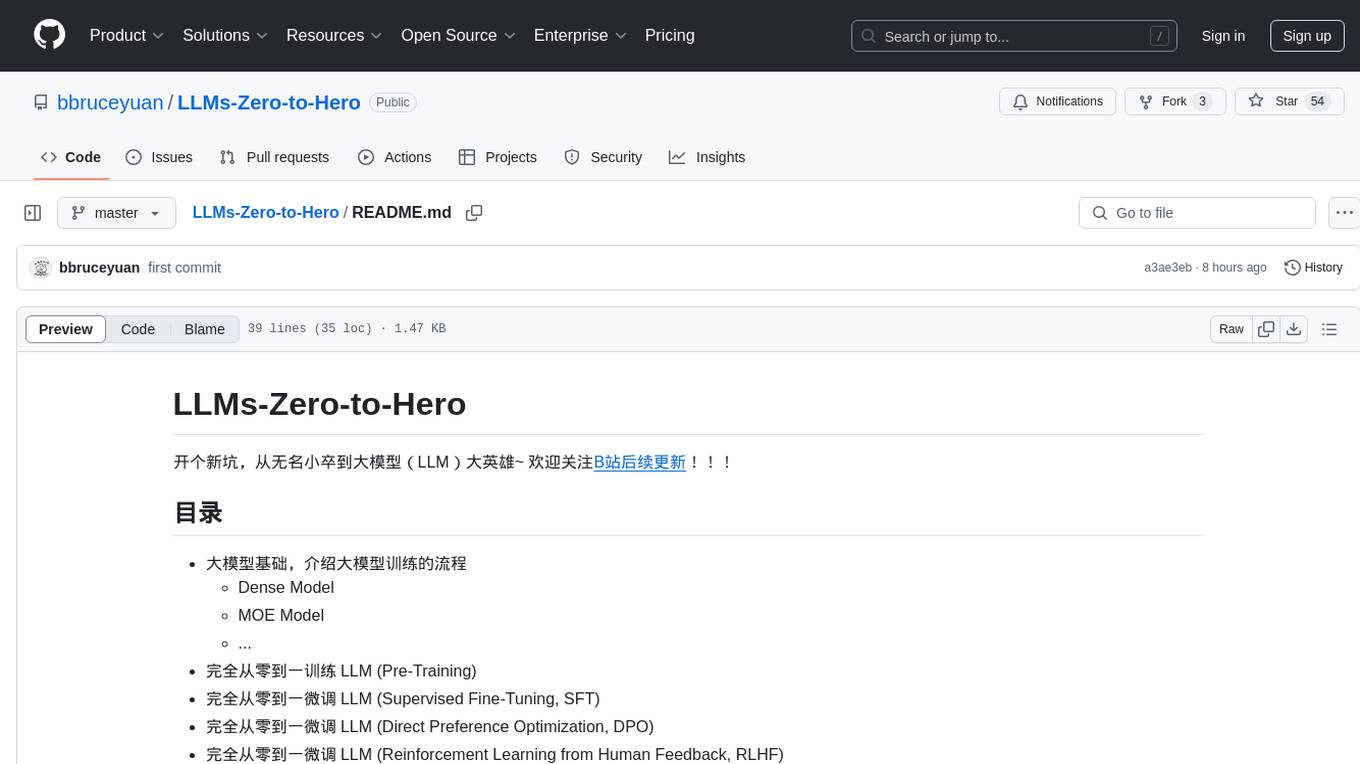
LLMs-Zero-to-Hero
LLMs-Zero-to-Hero is a repository dedicated to training large language models (LLMs) from scratch, covering topics such as dense models, MOE models, pre-training, supervised fine-tuning, direct preference optimization, reinforcement learning from human feedback, and deploying large models. The repository provides detailed learning notes for different chapters, code implementations, and resources for training and deploying LLMs. It aims to guide users from being beginners to proficient in building and deploying large language models.
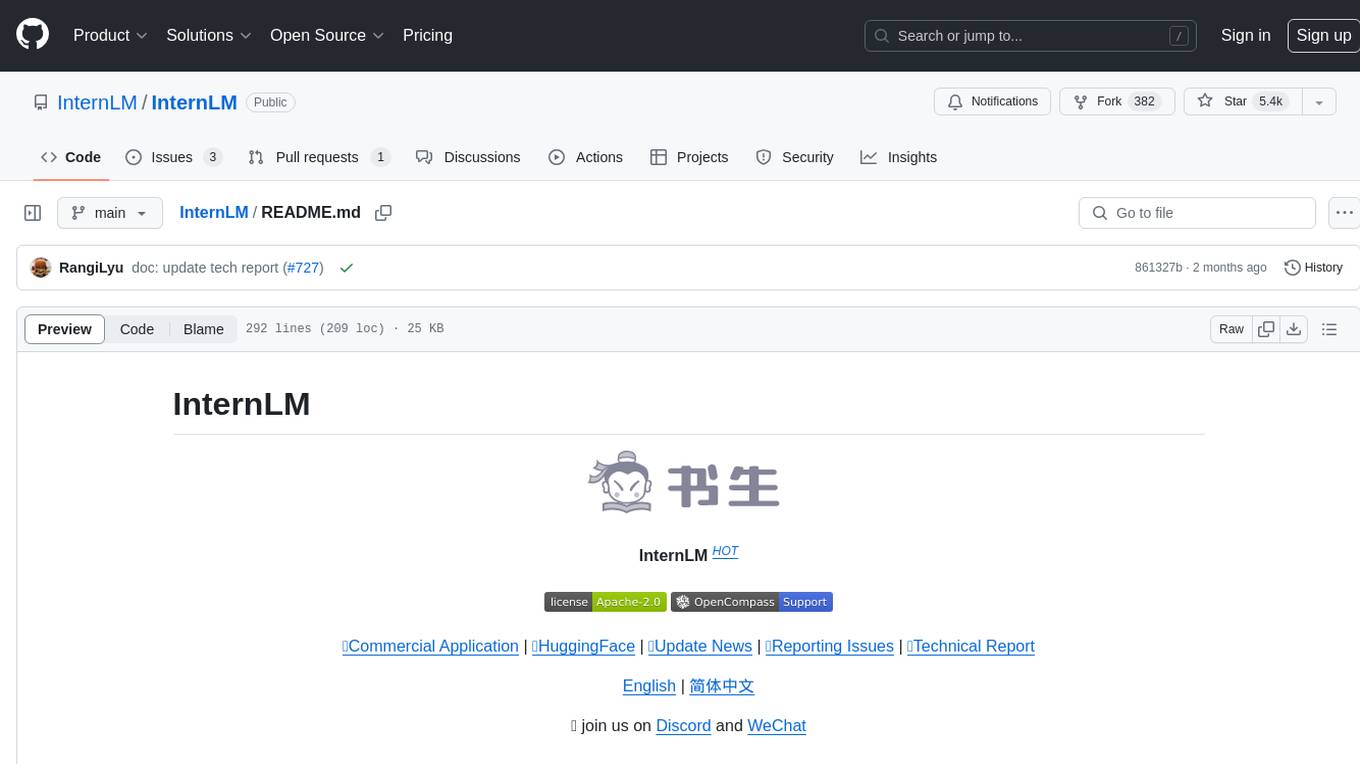
InternLM
InternLM is a powerful language model series with features such as 200K context window for long-context tasks, outstanding comprehensive performance in reasoning, math, code, chat experience, instruction following, and creative writing, code interpreter & data analysis capabilities, and stronger tool utilization capabilities. It offers models in sizes of 7B and 20B, suitable for research and complex scenarios. The models are recommended for various applications and exhibit better performance than previous generations. InternLM models may match or surpass other open-source models like ChatGPT. The tool has been evaluated on various datasets and has shown superior performance in multiple tasks. It requires Python >= 3.8, PyTorch >= 1.12.0, and Transformers >= 4.34 for usage. InternLM can be used for tasks like chat, agent applications, fine-tuning, deployment, and long-context inference.
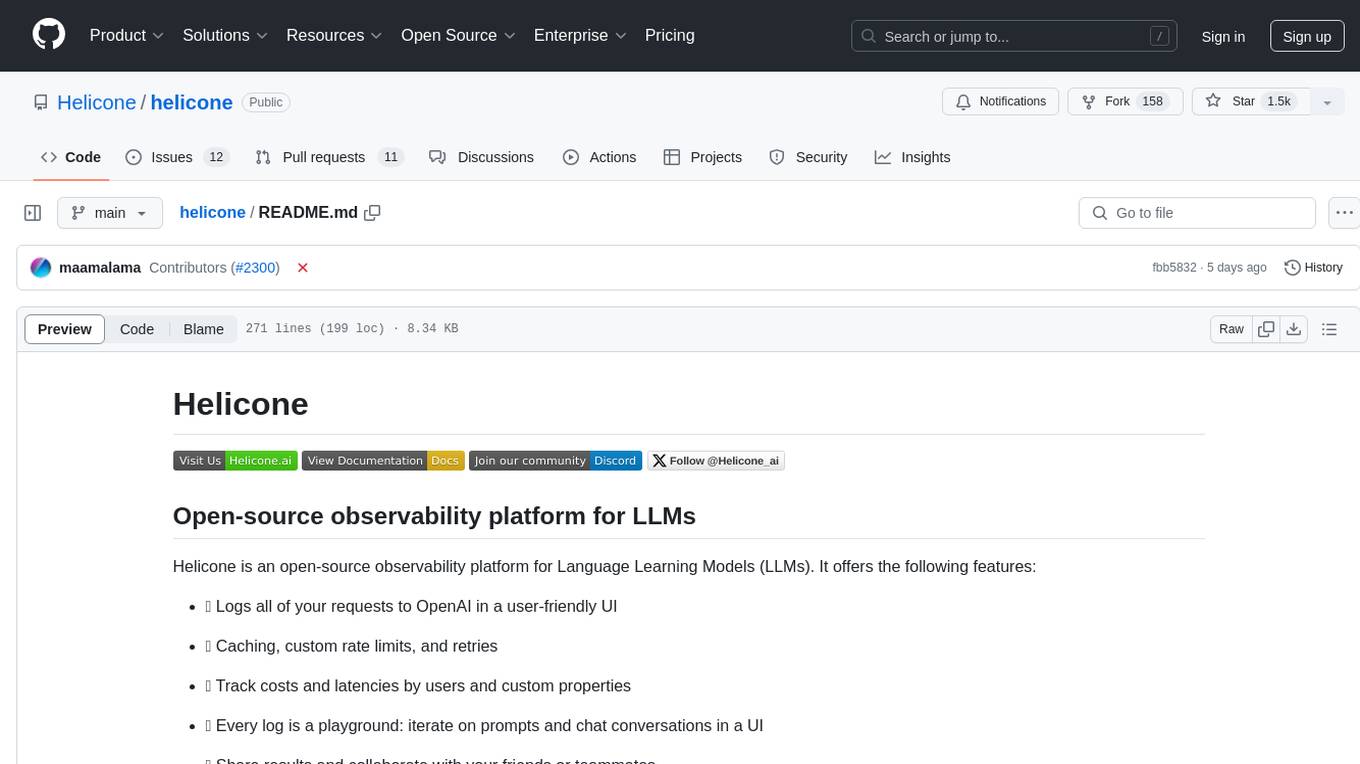
helicone
Helicone is an open-source observability platform designed for Language Learning Models (LLMs). It logs requests to OpenAI in a user-friendly UI, offers caching, rate limits, and retries, tracks costs and latencies, provides a playground for iterating on prompts and chat conversations, supports collaboration, and will soon have APIs for feedback and evaluation. The platform is deployed on Cloudflare and consists of services like Web (NextJs), Worker (Cloudflare Workers), Jawn (Express), Supabase, and ClickHouse. Users can interact with Helicone locally by setting up the required services and environment variables. The platform encourages contributions and provides resources for learning, documentation, and integrations.
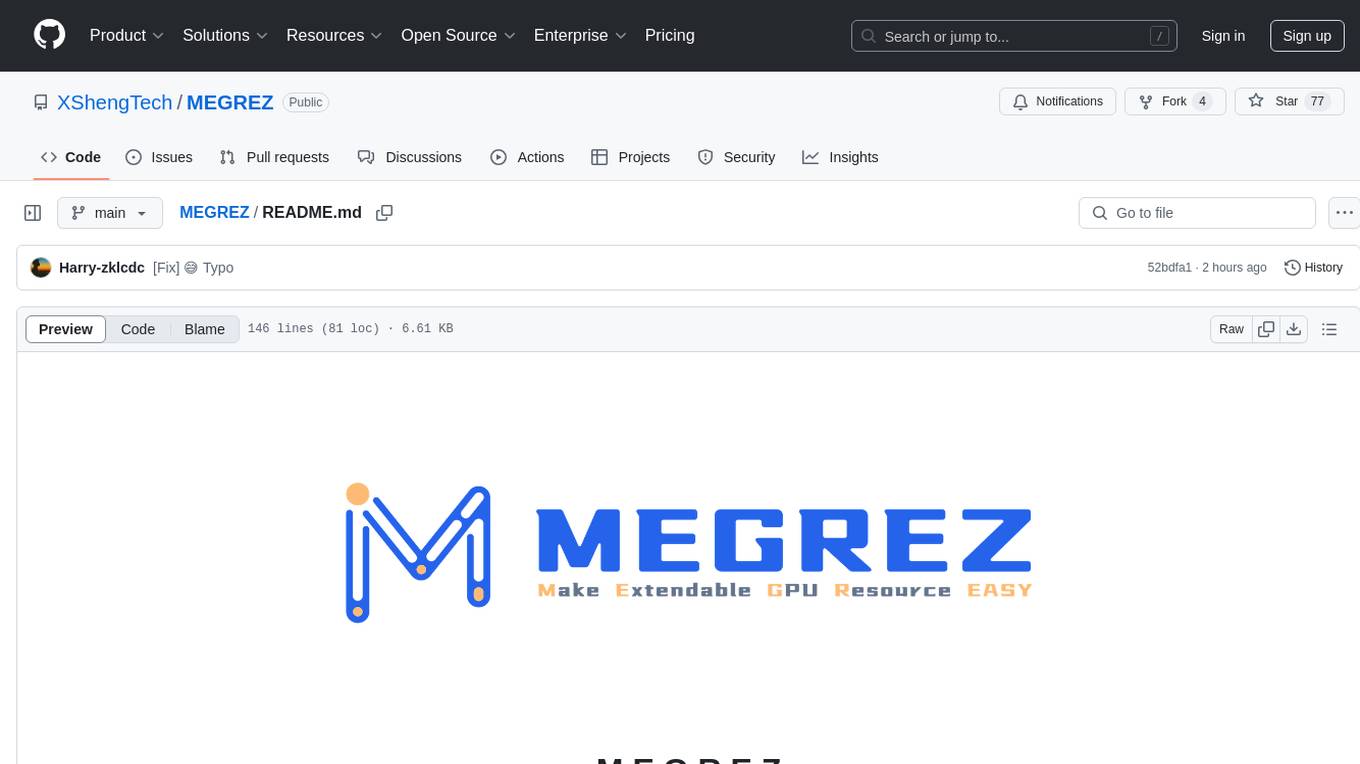
MEGREZ
MEGREZ is a modern and elegant open-source high-performance computing platform that efficiently manages GPU resources. It allows for easy container instance creation, supports multiple nodes/multiple GPUs, modern UI environment isolation, customizable performance configurations, and user data isolation. The platform also comes with pre-installed deep learning environments, supports multiple users, features a VSCode web version, resource performance monitoring dashboard, and Jupyter Notebook support.
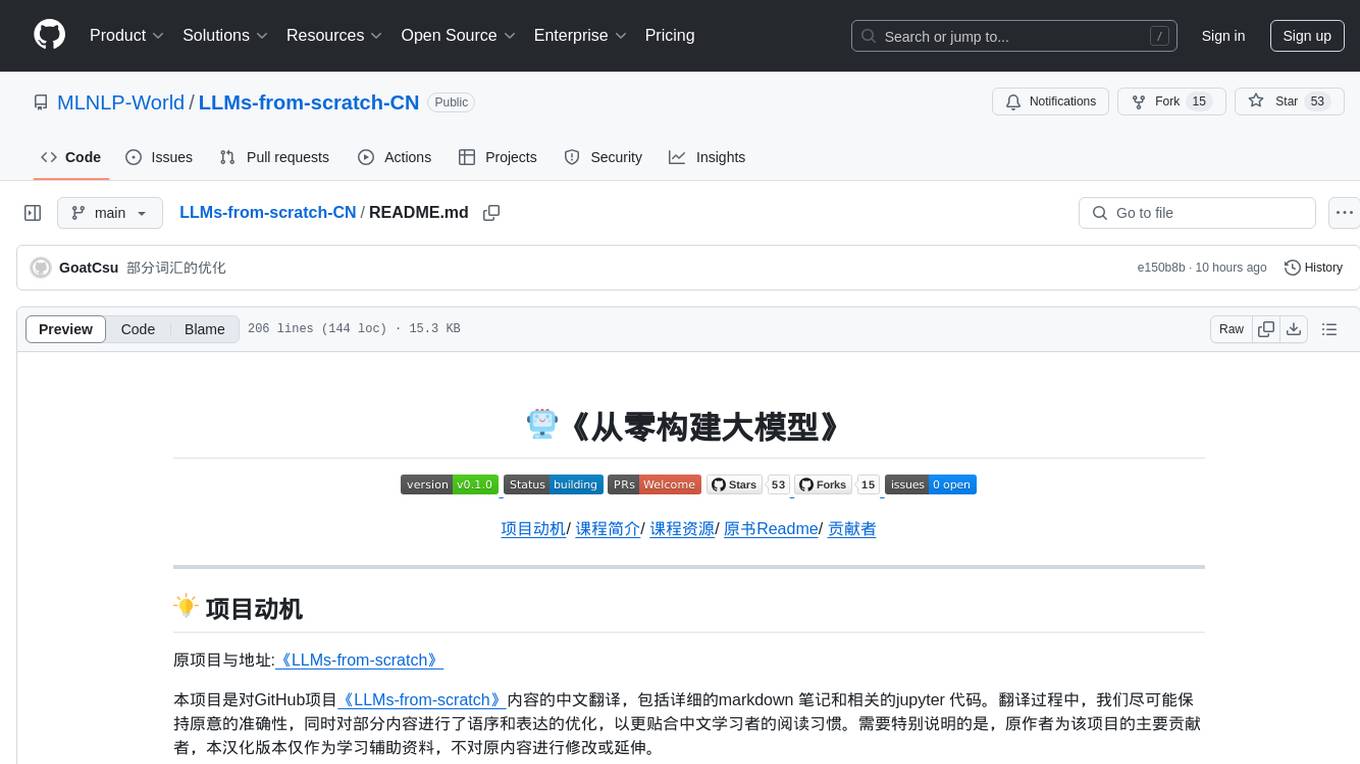
LLMs-from-scratch-CN
This repository is a Chinese translation of the GitHub project 'LLMs-from-scratch', including detailed markdown notes and related Jupyter code. The translation process aims to maintain the accuracy of the original content while optimizing the language and expression to better suit Chinese learners' reading habits. The repository features detailed Chinese annotations for all Jupyter code, aiding users in practical implementation. It also provides various supplementary materials to expand knowledge. The project focuses on building Large Language Models (LLMs) from scratch, covering fundamental constructions like Transformer architecture, sequence modeling, and delving into deep learning models such as GPT and BERT. Each part of the project includes detailed code implementations and learning resources to help users construct LLMs from scratch and master their core technologies.
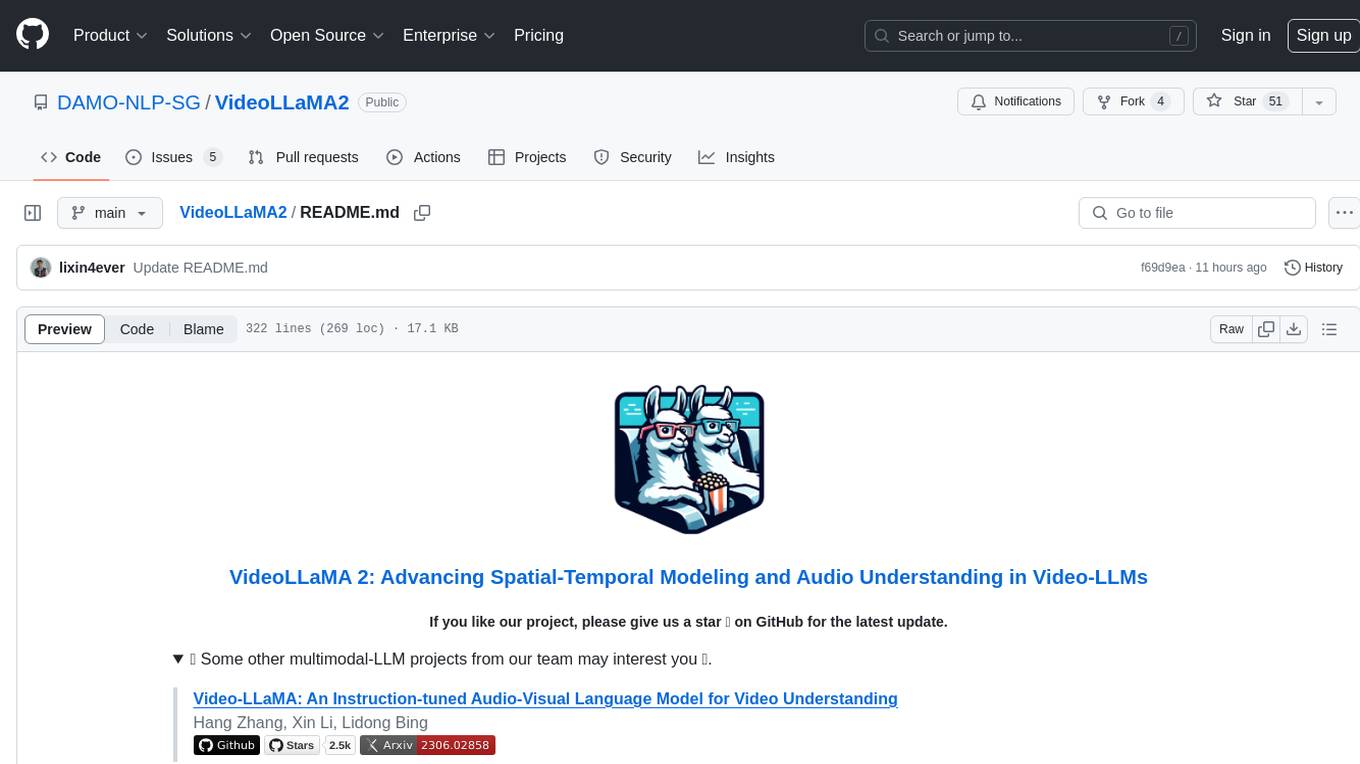
VideoLLaMA2
VideoLLaMA 2 is a project focused on advancing spatial-temporal modeling and audio understanding in video-LLMs. It provides tools for multi-choice video QA, open-ended video QA, and video captioning. The project offers model zoo with different configurations for visual encoder and language decoder. It includes training and evaluation guides, as well as inference capabilities for video and image processing. The project also features a demo setup for running a video-based Large Language Model web demonstration.
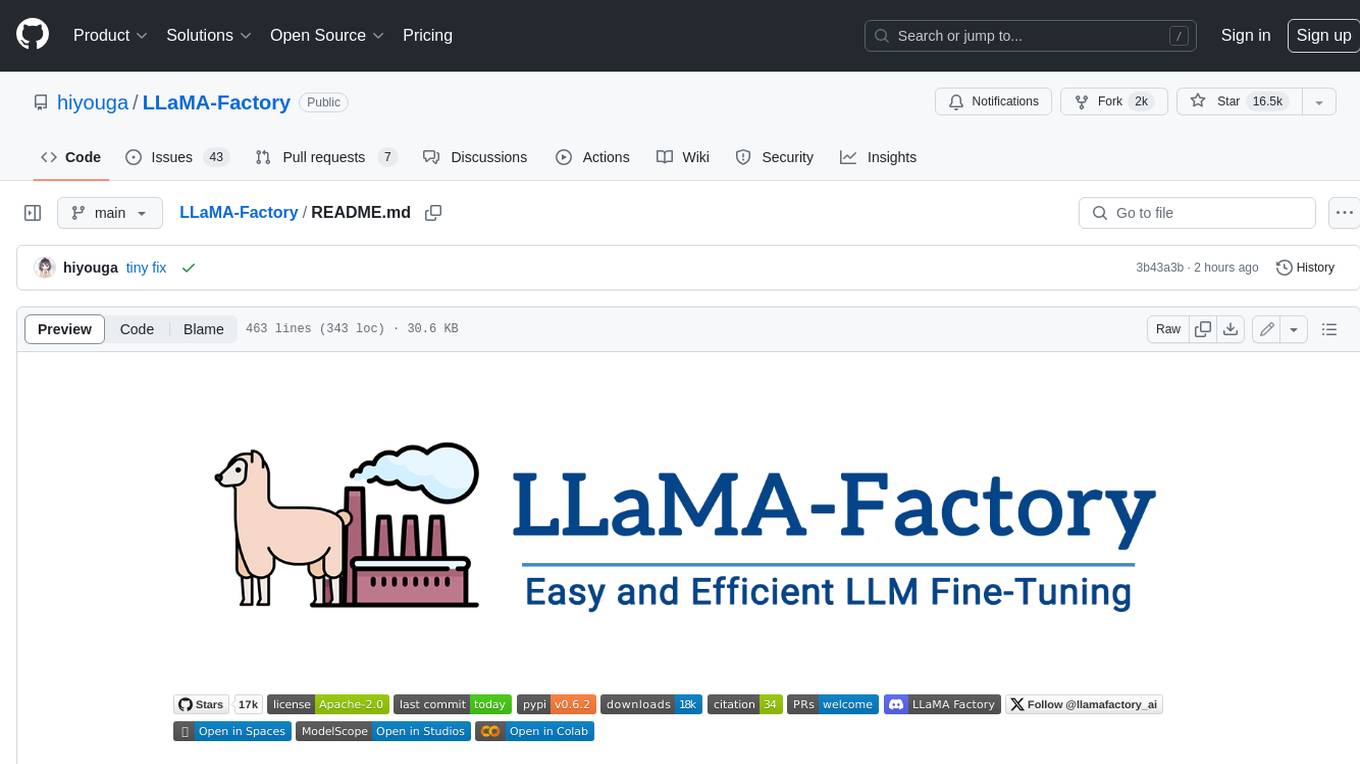
LLaMA-Factory
LLaMA Factory is a unified framework for fine-tuning 100+ large language models (LLMs) with various methods, including pre-training, supervised fine-tuning, reward modeling, PPO, DPO and ORPO. It features integrated algorithms like GaLore, BAdam, DoRA, LongLoRA, LLaMA Pro, LoRA+, LoftQ and Agent tuning, as well as practical tricks like FlashAttention-2, Unsloth, RoPE scaling, NEFTune and rsLoRA. LLaMA Factory provides experiment monitors like LlamaBoard, TensorBoard, Wandb, MLflow, etc., and supports faster inference with OpenAI-style API, Gradio UI and CLI with vLLM worker. Compared to ChatGLM's P-Tuning, LLaMA Factory's LoRA tuning offers up to 3.7 times faster training speed with a better Rouge score on the advertising text generation task. By leveraging 4-bit quantization technique, LLaMA Factory's QLoRA further improves the efficiency regarding the GPU memory.
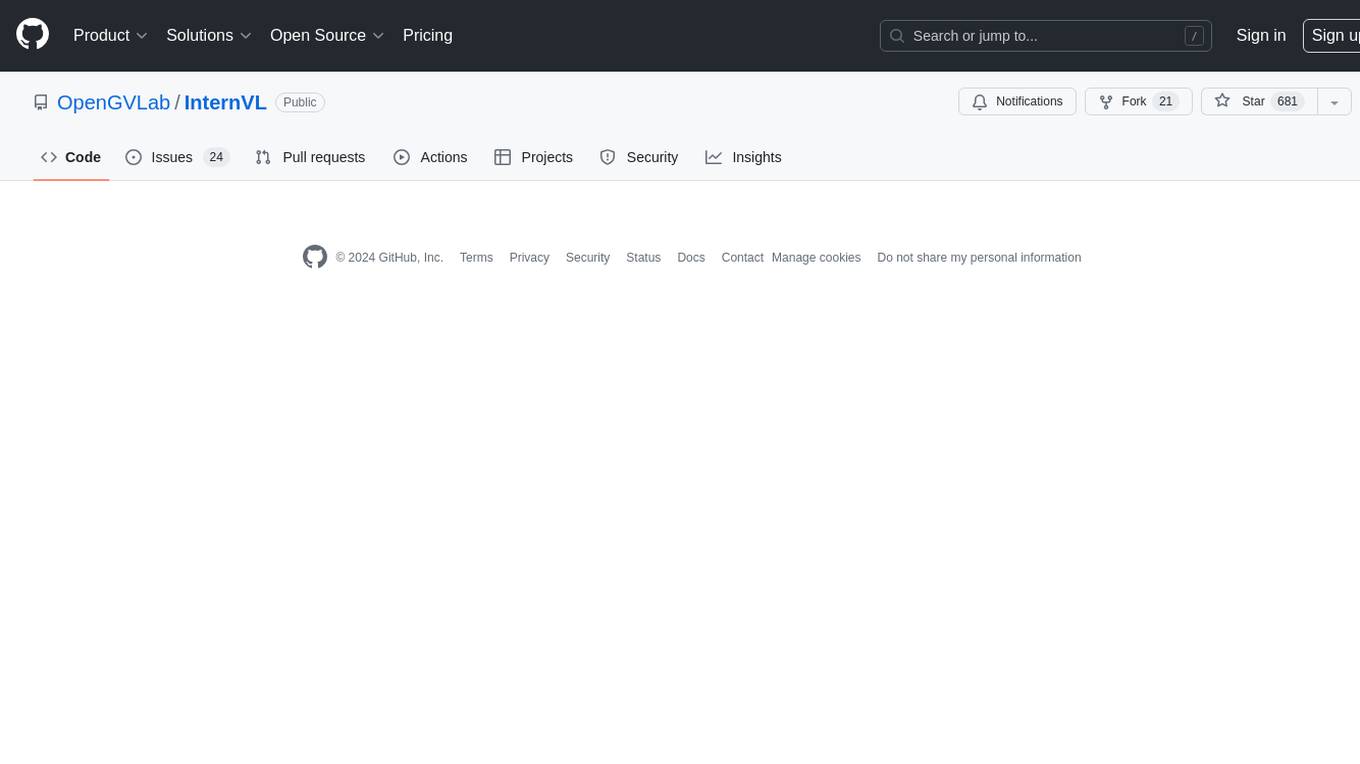
InternVL
InternVL scales up the ViT to _**6B parameters**_ and aligns it with LLM. It is a vision-language foundation model that can perform various tasks, including: **Visual Perception** - Linear-Probe Image Classification - Semantic Segmentation - Zero-Shot Image Classification - Multilingual Zero-Shot Image Classification - Zero-Shot Video Classification **Cross-Modal Retrieval** - English Zero-Shot Image-Text Retrieval - Chinese Zero-Shot Image-Text Retrieval - Multilingual Zero-Shot Image-Text Retrieval on XTD **Multimodal Dialogue** - Zero-Shot Image Captioning - Multimodal Benchmarks with Frozen LLM - Multimodal Benchmarks with Trainable LLM - Tiny LVLM InternVL has been shown to achieve state-of-the-art results on a variety of benchmarks. For example, on the MMMU image classification benchmark, InternVL achieves a top-1 accuracy of 51.6%, which is higher than GPT-4V and Gemini Pro. On the DocVQA question answering benchmark, InternVL achieves a score of 82.2%, which is also higher than GPT-4V and Gemini Pro. InternVL is open-sourced and available on Hugging Face. It can be used for a variety of applications, including image classification, object detection, semantic segmentation, image captioning, and question answering.
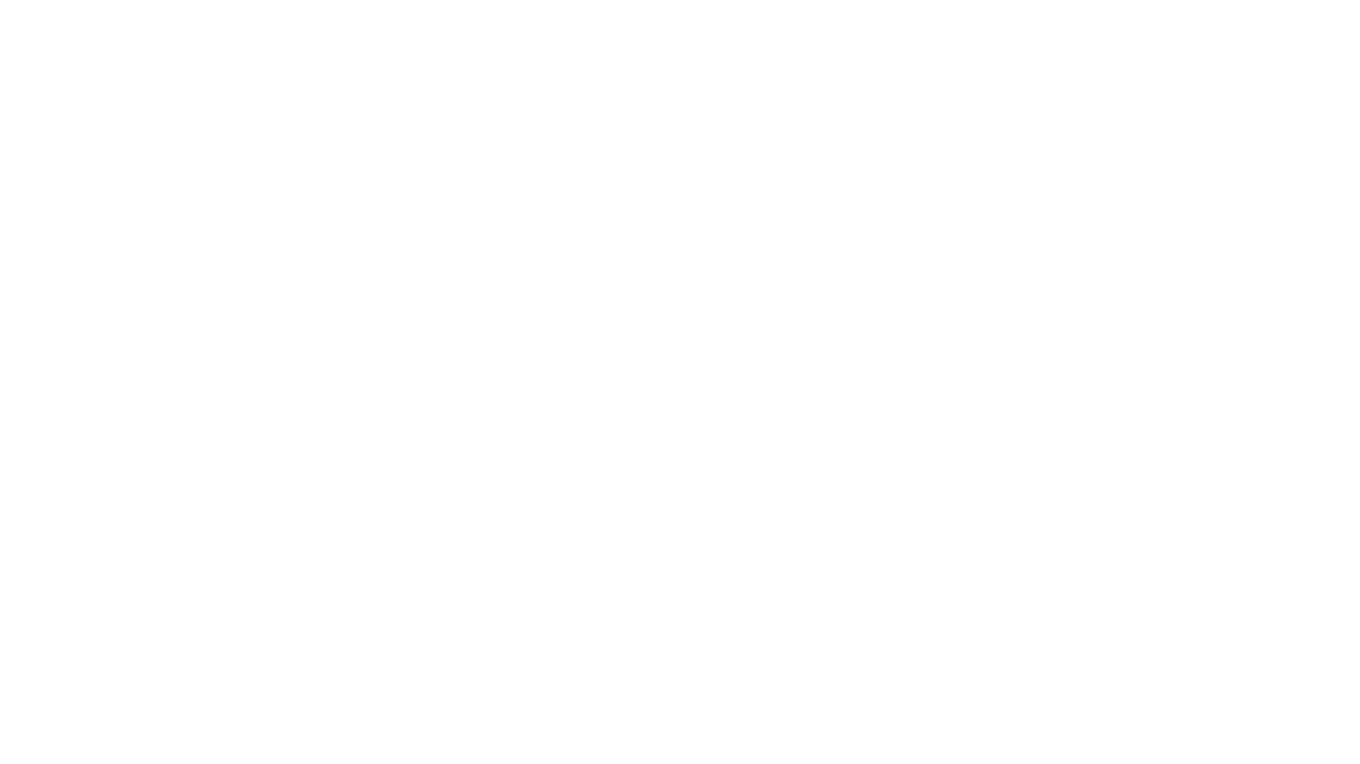
chatluna
Chatluna is a machine learning model plugin that provides chat services with large language models. It is highly extensible, supports multiple output formats, and offers features like custom conversation presets, rate limiting, and context awareness. Users can deploy Chatluna under Koishi without additional configuration. The plugin supports various models/platforms like OpenAI, Azure OpenAI, Google Gemini, and more. It also provides preset customization using YAML files and allows for easy forking and development within Koishi projects. However, the project lacks web UI, HTTP server, and project documentation, inviting contributions from the community.
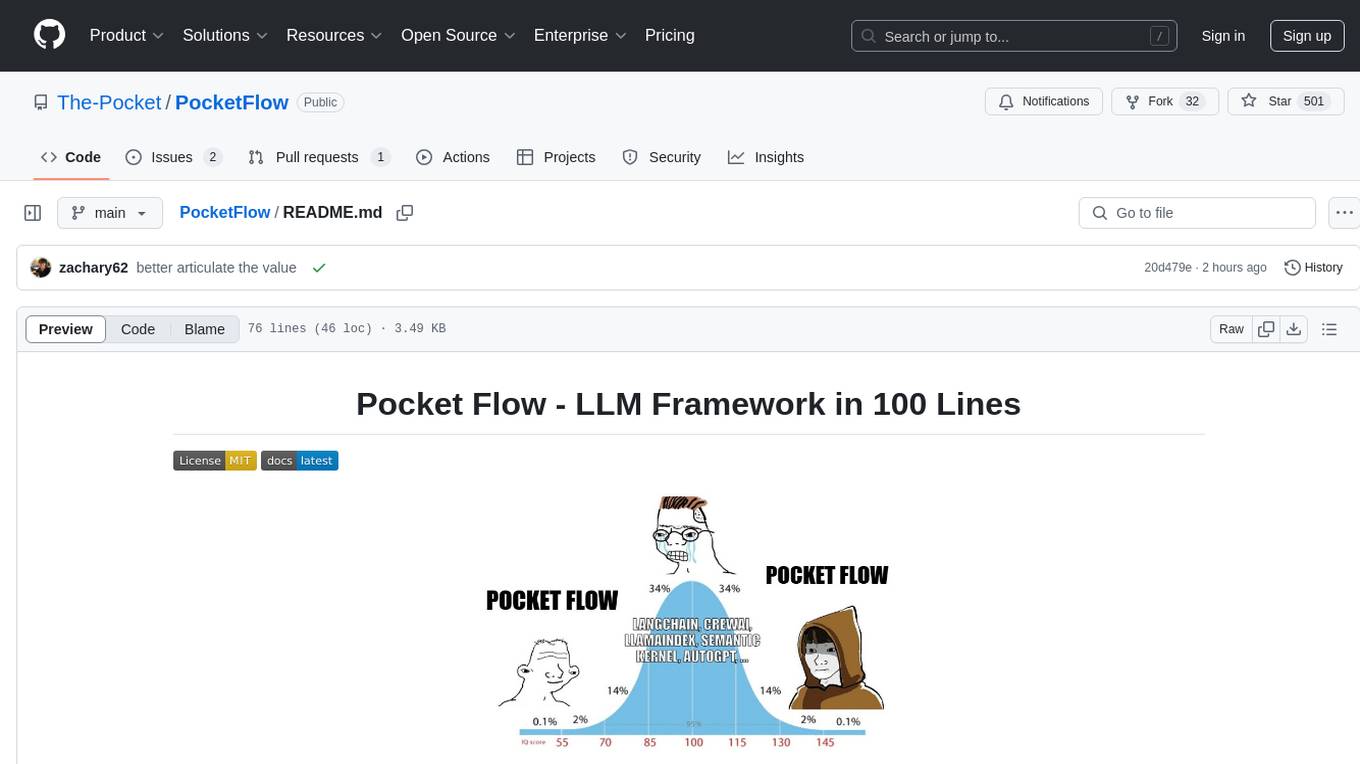
PocketFlow
Pocket Flow is a 100-line minimalist LLM framework designed for (Multi-)Agents, Workflow, RAG, etc. It provides a core abstraction for LLM projects by focusing on computation and communication through a graph structure and shared store. The framework aims to support the development of LLM Agents, such as Cursor AI, by offering a minimal and low-level approach that is well-suited for understanding and usage. Users can install Pocket Flow via pip or by copying the source code, and detailed documentation is available on the project website.
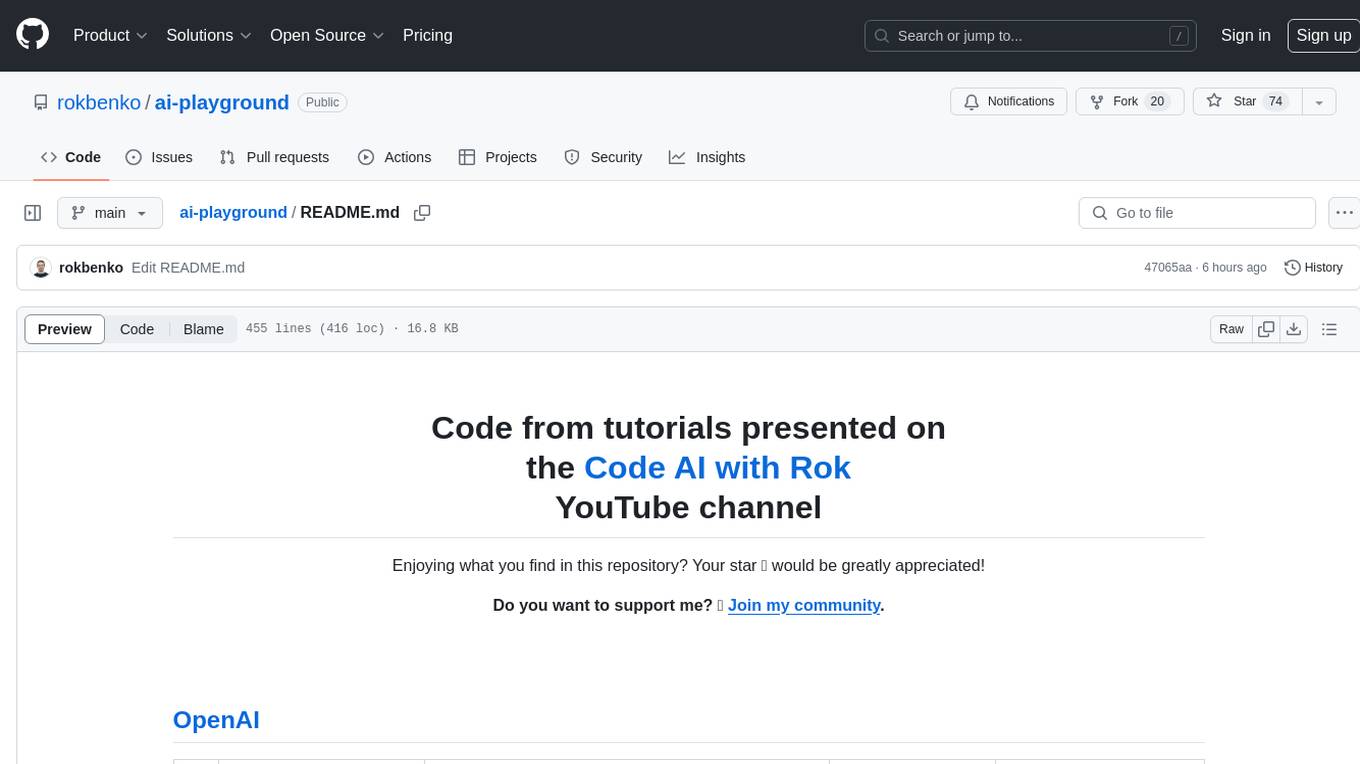
ai-playground
The ai-playground repository contains code from tutorials presented on the Code AI with Rok YouTube channel. It includes tutorials on using the OpenAI Assistants API v1 beta to build personal math tutors, customer support chatbots, and more. Additionally, there are tutorials on using Gemini Pro API, Snowflake Cortex LLM functions, LlamaIndex chat streaming app, Fetch.ai uAgents, Milvus Standalone, spaCy for NER, and more. The repository aims to provide practical examples and guides for developers interested in AI-related projects and tools.
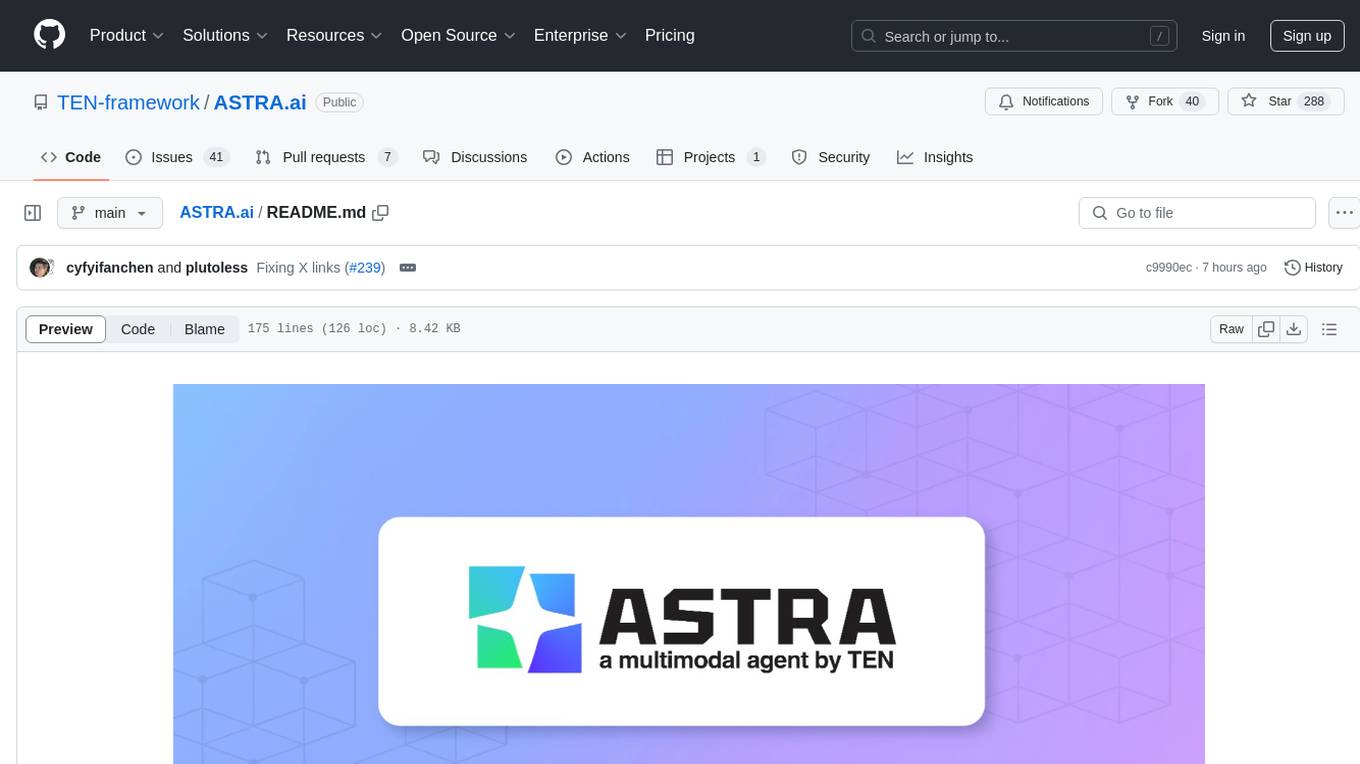
ASTRA.ai
Astra.ai is a multimodal agent powered by TEN, showcasing its capabilities in speech, vision, and reasoning through RAG from local documentation. It provides a platform for developing AI agents with features like RTC transportation, extension store, workflow builder, and local deployment. Users can build and test agents locally using Docker and Node.js, with prerequisites including Agora App ID, Azure's speech-to-text and text-to-speech API keys, and OpenAI API key. The platform offers advanced customization options through config files and API keys setup, enabling users to create and deploy their AI agents for various tasks.
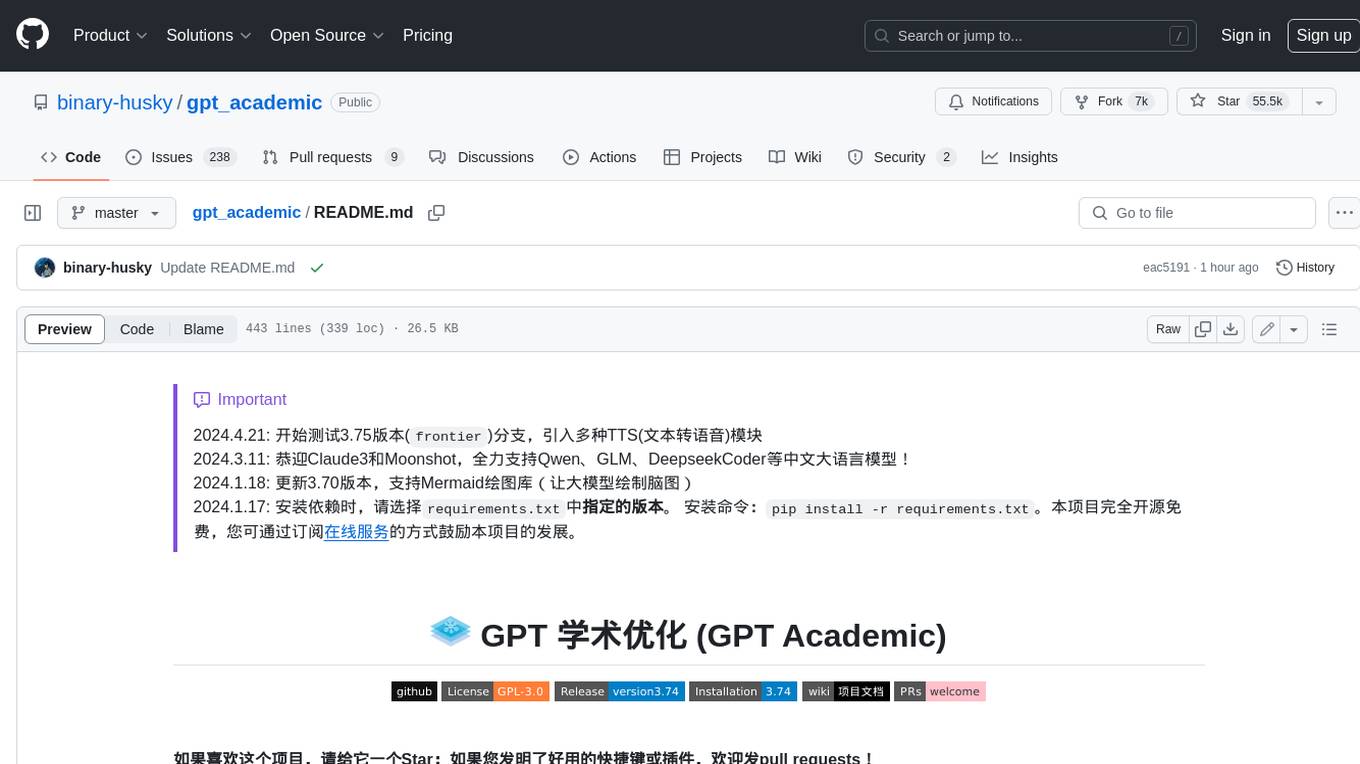
gpt_academic
GPT Academic is a powerful tool that leverages the capabilities of large language models (LLMs) to enhance academic research and writing. It provides a user-friendly interface that allows researchers, students, and professionals to interact with LLMs and utilize their abilities for various academic tasks. With GPT Academic, users can access a wide range of features and functionalities, including: * **Summarization and Paraphrasing:** GPT Academic can summarize complex texts, articles, and research papers into concise and informative summaries. It can also paraphrase text to improve clarity and readability. * **Question Answering:** Users can ask GPT Academic questions related to their research or studies, and the tool will provide comprehensive and well-informed answers based on its knowledge and understanding of the relevant literature. * **Code Generation and Explanation:** GPT Academic can generate code snippets and provide explanations for complex coding concepts. It can also help debug code and suggest improvements. * **Translation:** GPT Academic supports translation of text between multiple languages, making it a valuable tool for researchers working with international collaborations or accessing resources in different languages. * **Citation and Reference Management:** GPT Academic can help users manage their citations and references by automatically generating citations in various formats and providing suggestions for relevant references based on the user's research topic. * **Collaboration and Note-Taking:** GPT Academic allows users to collaborate on projects and take notes within the tool. They can share their work with others and access a shared workspace for real-time collaboration. * **Customizable Interface:** GPT Academic offers a customizable interface that allows users to tailor the tool to their specific needs and preferences. They can choose from a variety of themes, adjust the layout, and add or remove features to create a personalized workspace. Overall, GPT Academic is a versatile and powerful tool that can significantly enhance the productivity and efficiency of academic research and writing. It empowers users to leverage the capabilities of LLMs and unlock new possibilities for academic exploration and knowledge creation.
For similar tasks
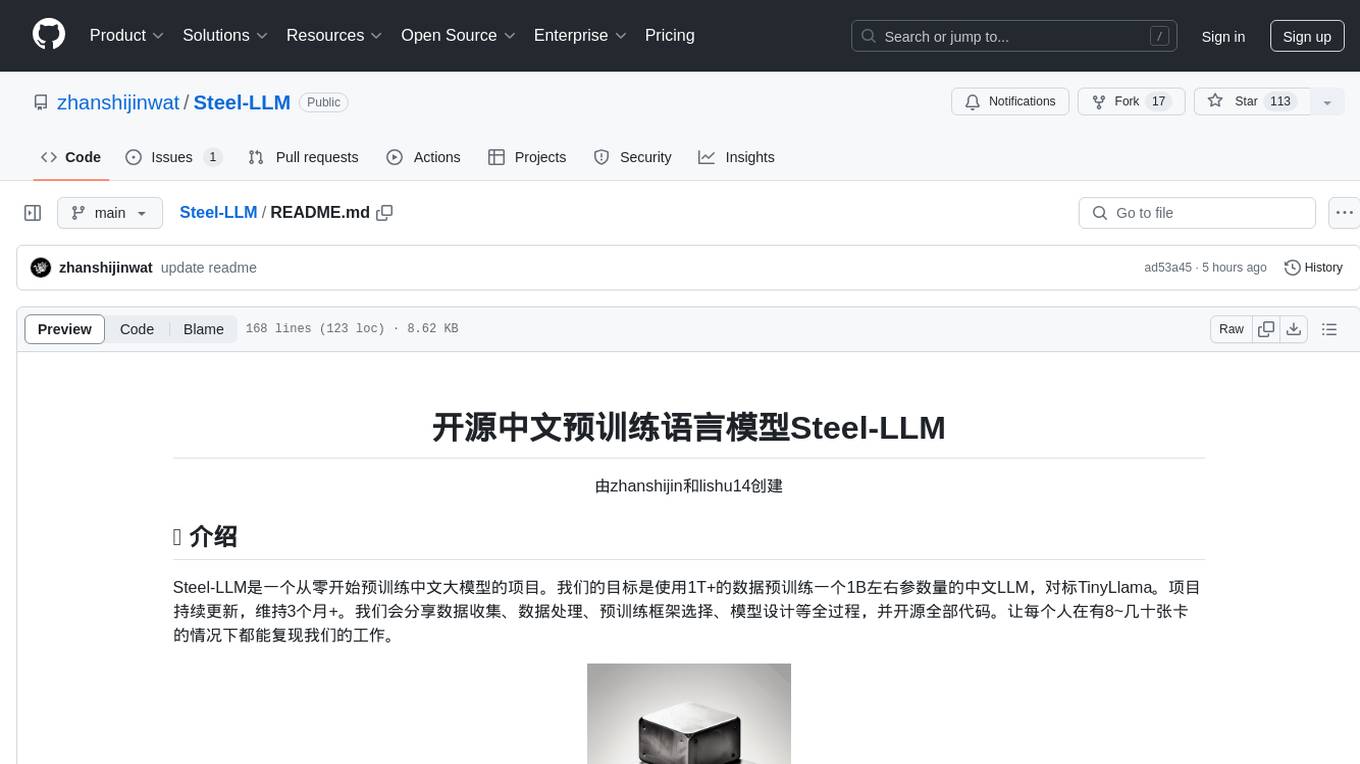
Steel-LLM
Steel-LLM is a project to pre-train a large Chinese language model from scratch using over 1T of data to achieve a parameter size of around 1B, similar to TinyLlama. The project aims to share the entire process including data collection, data processing, pre-training framework selection, model design, and open-source all the code. The goal is to enable reproducibility of the work even with limited resources. The name 'Steel' is inspired by a band '万能青年旅店' and signifies the desire to create a strong model despite limited conditions. The project involves continuous data collection of various cultural elements, trivia, lyrics, niche literature, and personal secrets to train the LLM. The ultimate aim is to fill the model with diverse data and leave room for individual input, fostering collaboration among users.
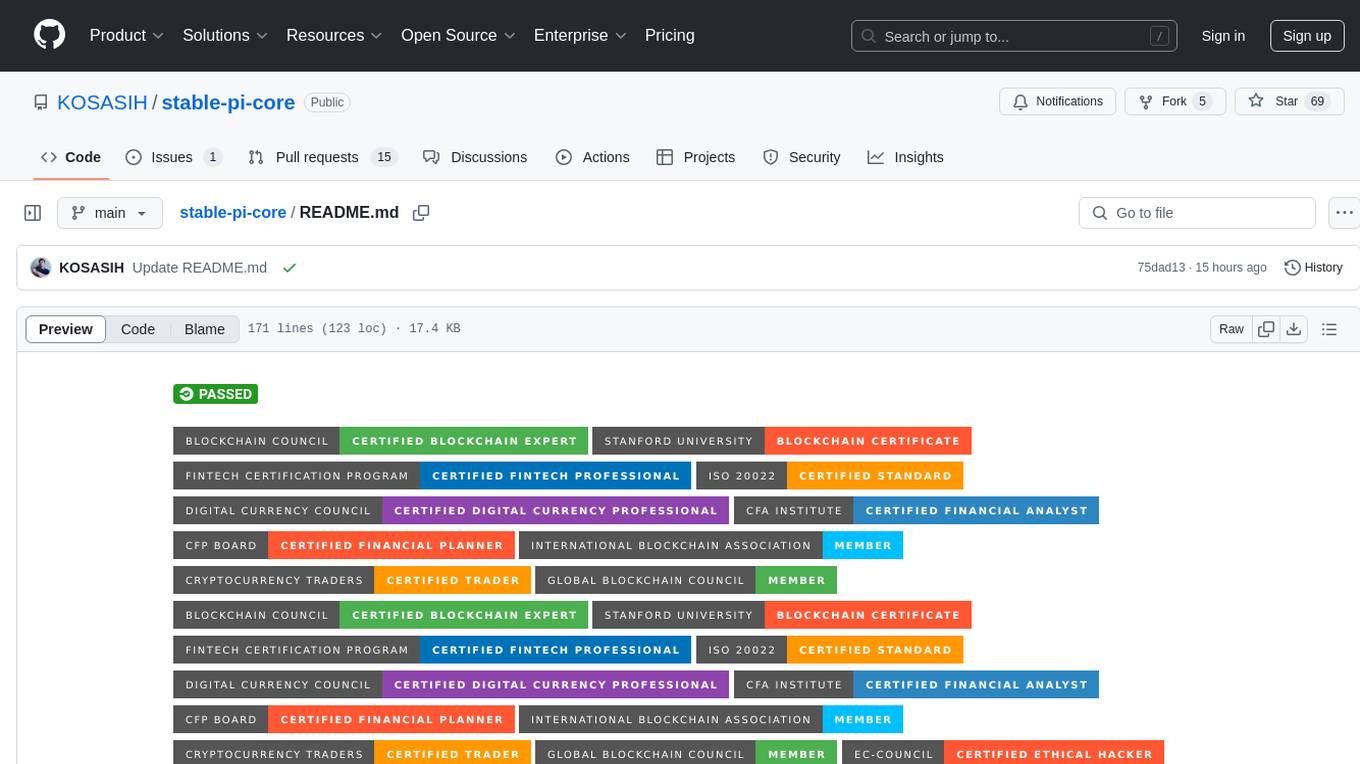
stable-pi-core
Stable-Pi-Core is a next-generation decentralized ecosystem integrating blockchain, quantum AI, IoT, edge computing, and AR/VR for secure, scalable, and personalized solutions in payments, governance, and real-world applications. It features a Dual-Value System, cross-chain interoperability, AI-powered security, and a self-healing network. The platform empowers seamless payments, decentralized governance via DAO, and real-world applications across industries, bridging digital and physical worlds with innovative features like robotic process automation, machine learning personalization, and a dynamic cross-chain bridge framework.
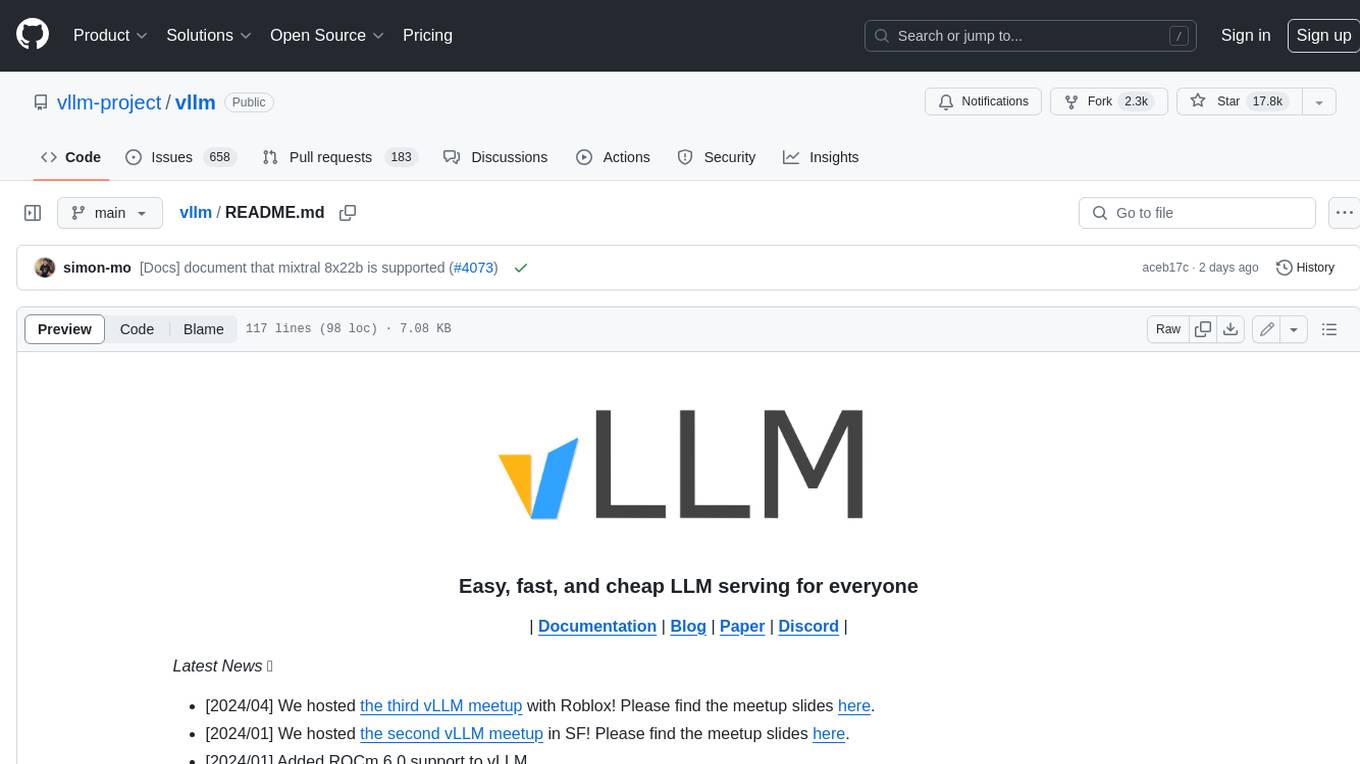
vllm
vLLM is a fast and easy-to-use library for LLM inference and serving. It is designed to be efficient, flexible, and easy to use. vLLM can be used to serve a variety of LLM models, including Hugging Face models. It supports a variety of decoding algorithms, including parallel sampling, beam search, and more. vLLM also supports tensor parallelism for distributed inference and streaming outputs. It is open-source and available on GitHub.
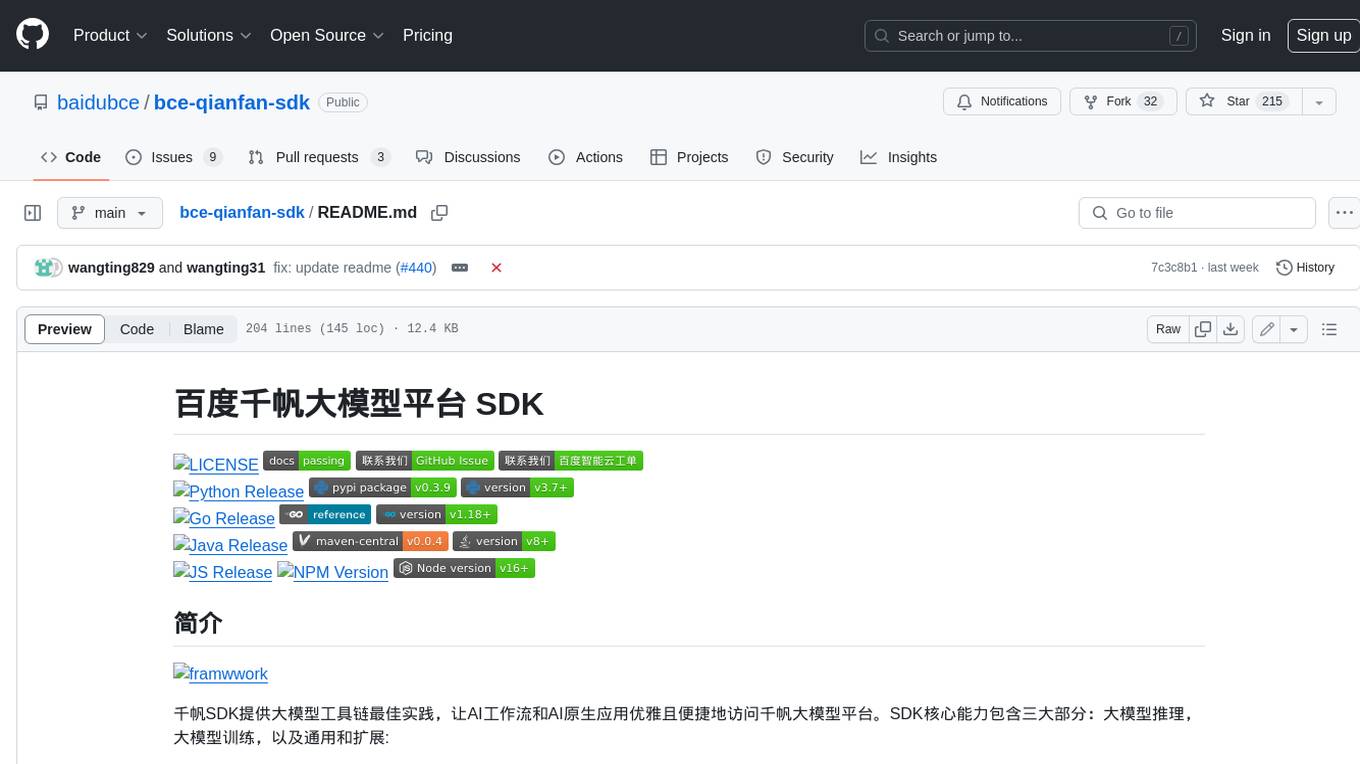
bce-qianfan-sdk
The Qianfan SDK provides best practices for large model toolchains, allowing AI workflows and AI-native applications to access the Qianfan large model platform elegantly and conveniently. The core capabilities of the SDK include three parts: large model reasoning, large model training, and general and extension: * `Large model reasoning`: Implements interface encapsulation for reasoning of Yuyan (ERNIE-Bot) series, open source large models, etc., supporting dialogue, completion, Embedding, etc. * `Large model training`: Based on platform capabilities, it supports end-to-end large model training process, including training data, fine-tuning/pre-training, and model services. * `General and extension`: General capabilities include common AI development tools such as Prompt/Debug/Client. The extension capability is based on the characteristics of Qianfan to adapt to common middleware frameworks.
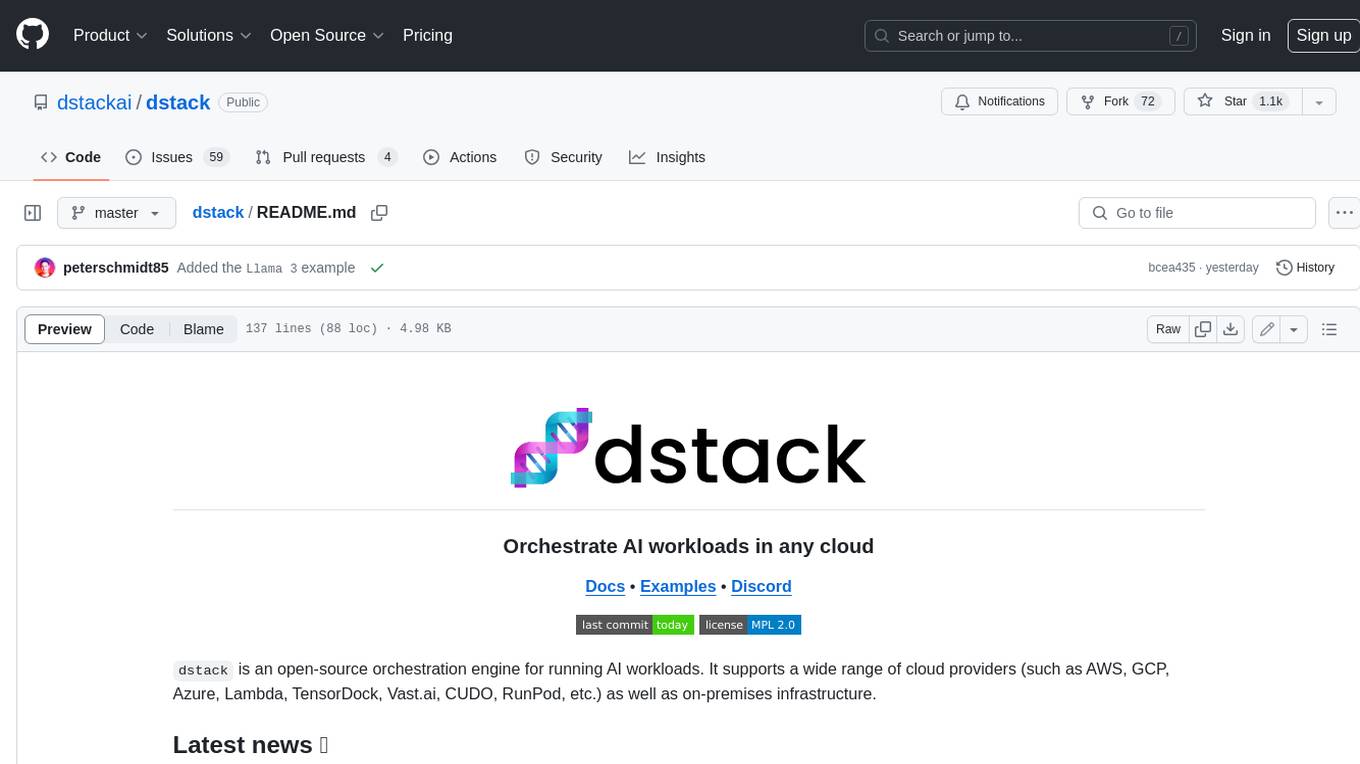
dstack
Dstack is an open-source orchestration engine for running AI workloads in any cloud. It supports a wide range of cloud providers (such as AWS, GCP, Azure, Lambda, TensorDock, Vast.ai, CUDO, RunPod, etc.) as well as on-premises infrastructure. With Dstack, you can easily set up and manage dev environments, tasks, services, and pools for your AI workloads.
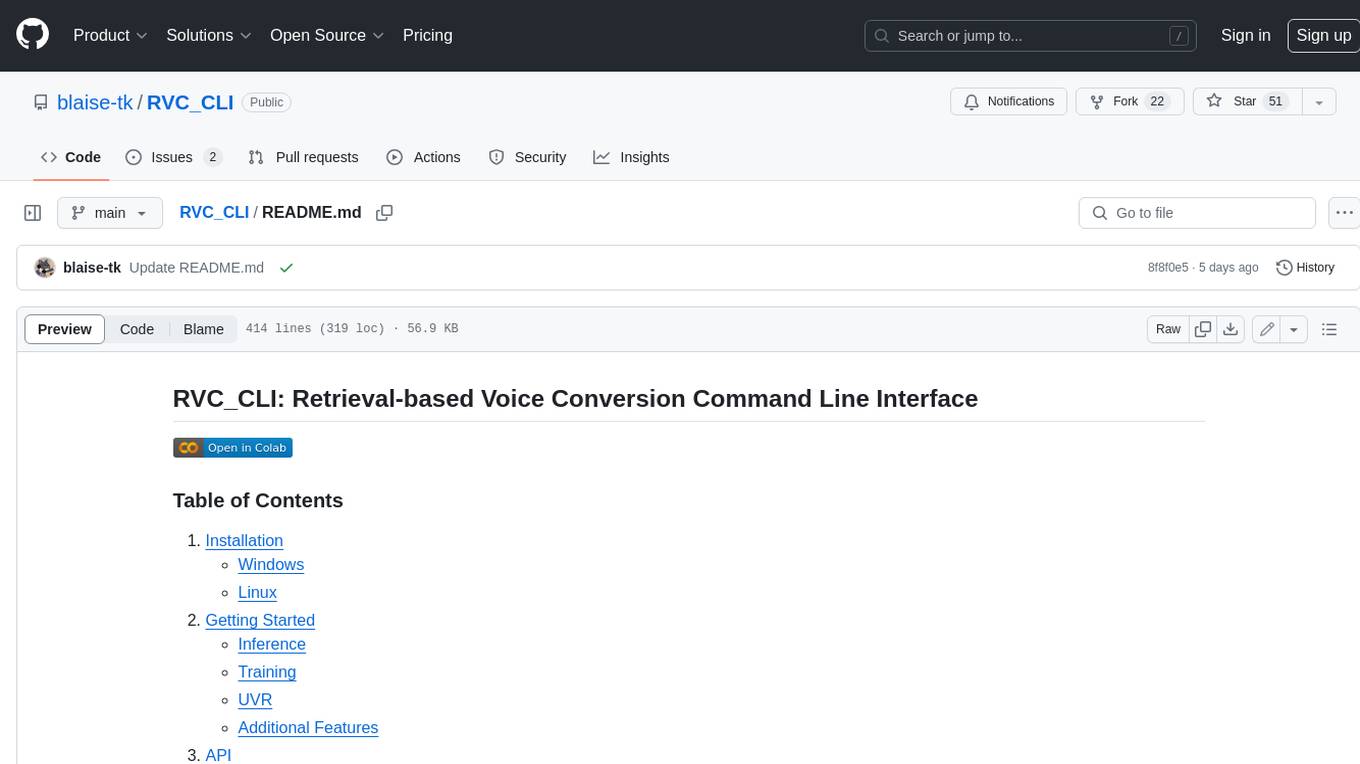
RVC_CLI
**RVC_CLI: Retrieval-based Voice Conversion Command Line Interface** This command-line interface (CLI) provides a comprehensive set of tools for voice conversion, enabling you to modify the pitch, timbre, and other characteristics of audio recordings. It leverages advanced machine learning models to achieve realistic and high-quality voice conversions. **Key Features:** * **Inference:** Convert the pitch and timbre of audio in real-time or process audio files in batch mode. * **TTS Inference:** Synthesize speech from text using a variety of voices and apply voice conversion techniques. * **Training:** Train custom voice conversion models to meet specific requirements. * **Model Management:** Extract, blend, and analyze models to fine-tune and optimize performance. * **Audio Analysis:** Inspect audio files to gain insights into their characteristics. * **API:** Integrate the CLI's functionality into your own applications or workflows. **Applications:** The RVC_CLI finds applications in various domains, including: * **Music Production:** Create unique vocal effects, harmonies, and backing vocals. * **Voiceovers:** Generate voiceovers with different accents, emotions, and styles. * **Audio Editing:** Enhance or modify audio recordings for podcasts, audiobooks, and other content. * **Research and Development:** Explore and advance the field of voice conversion technology. **For Jobs:** * Audio Engineer * Music Producer * Voiceover Artist * Audio Editor * Machine Learning Engineer **AI Keywords:** * Voice Conversion * Pitch Shifting * Timbre Modification * Machine Learning * Audio Processing **For Tasks:** * Convert Pitch * Change Timbre * Synthesize Speech * Train Model * Analyze Audio
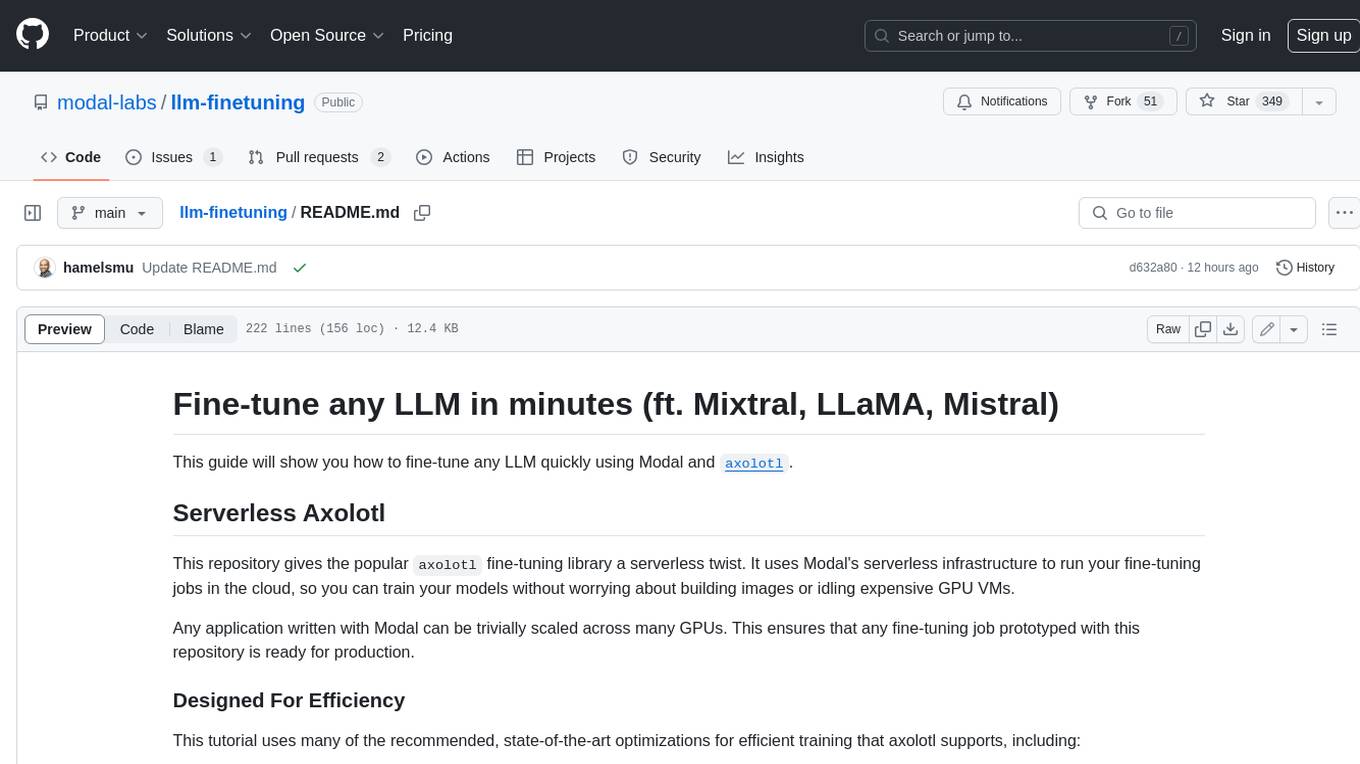
llm-finetuning
llm-finetuning is a repository that provides a serverless twist to the popular axolotl fine-tuning library using Modal's serverless infrastructure. It allows users to quickly fine-tune any LLM model with state-of-the-art optimizations like Deepspeed ZeRO, LoRA adapters, Flash attention, and Gradient checkpointing. The repository simplifies the fine-tuning process by not exposing all CLI arguments, instead allowing users to specify options in a config file. It supports efficient training and scaling across multiple GPUs, making it suitable for production-ready fine-tuning jobs.
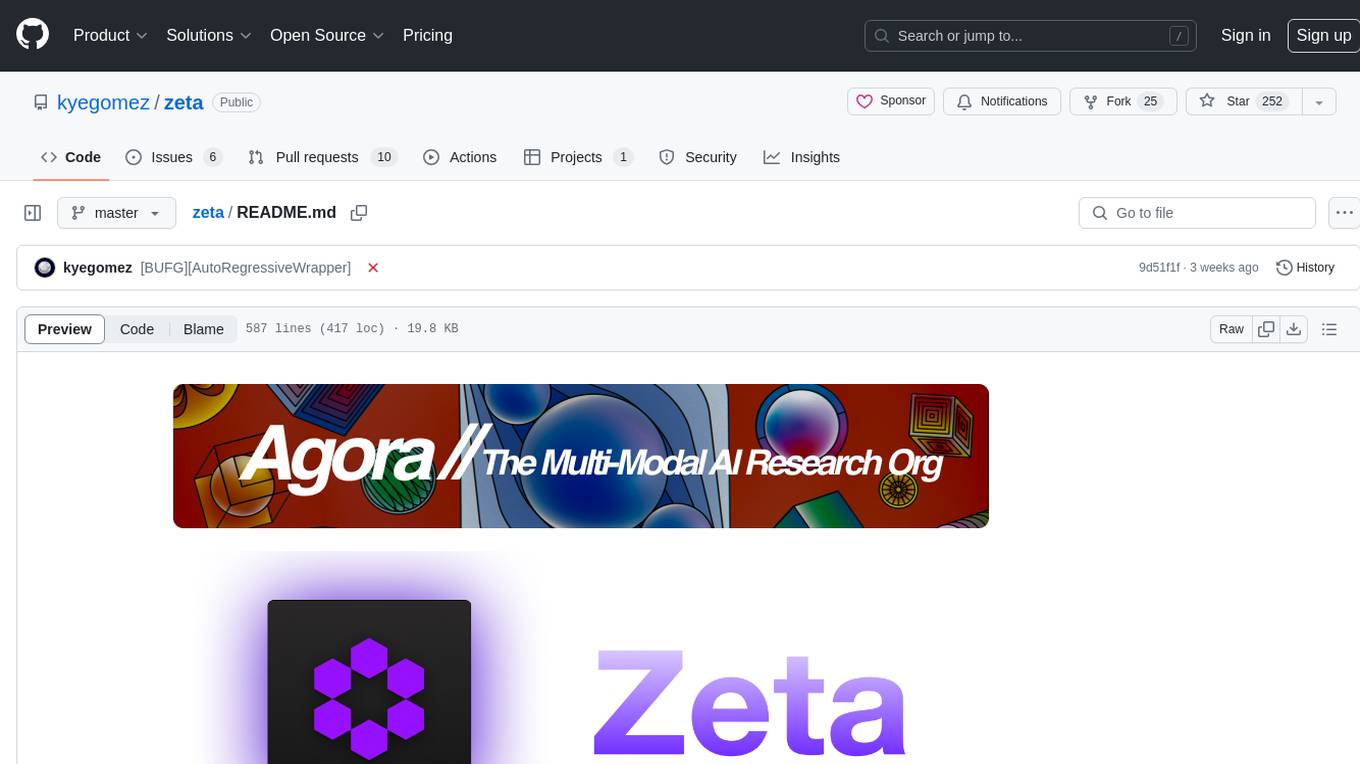
zeta
Zeta is a tool designed to build state-of-the-art AI models faster by providing modular, high-performance, and scalable building blocks. It addresses the common issues faced while working with neural nets, such as chaotic codebases, lack of modularity, and low performance modules. Zeta emphasizes usability, modularity, and performance, and is currently used in hundreds of models across various GitHub repositories. It enables users to prototype, train, optimize, and deploy the latest SOTA neural nets into production. The tool offers various modules like FlashAttention, SwiGLUStacked, RelativePositionBias, FeedForward, BitLinear, PalmE, Unet, VisionEmbeddings, niva, FusedDenseGELUDense, FusedDropoutLayerNorm, MambaBlock, Film, hyper_optimize, DPO, and ZetaCloud for different tasks in AI model development.
For similar jobs
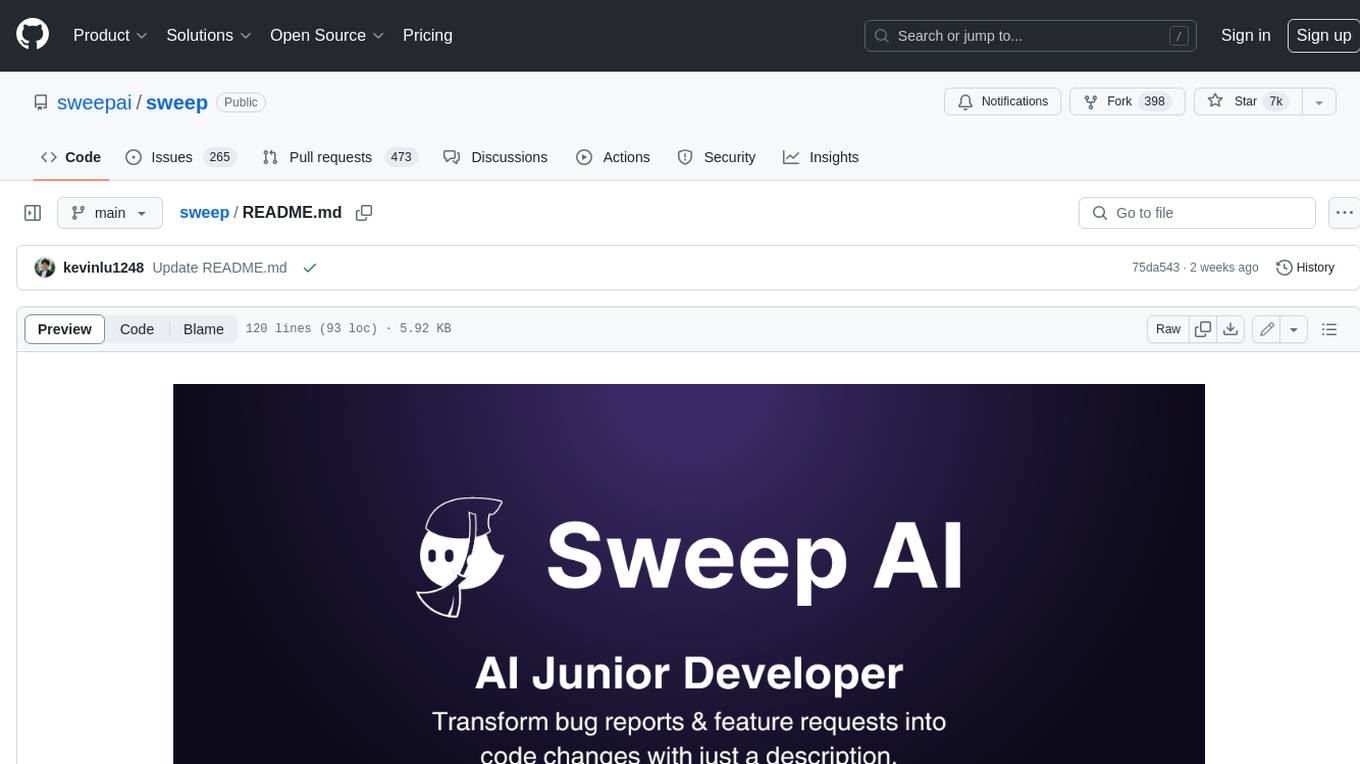
sweep
Sweep is an AI junior developer that turns bugs and feature requests into code changes. It automatically handles developer experience improvements like adding type hints and improving test coverage.
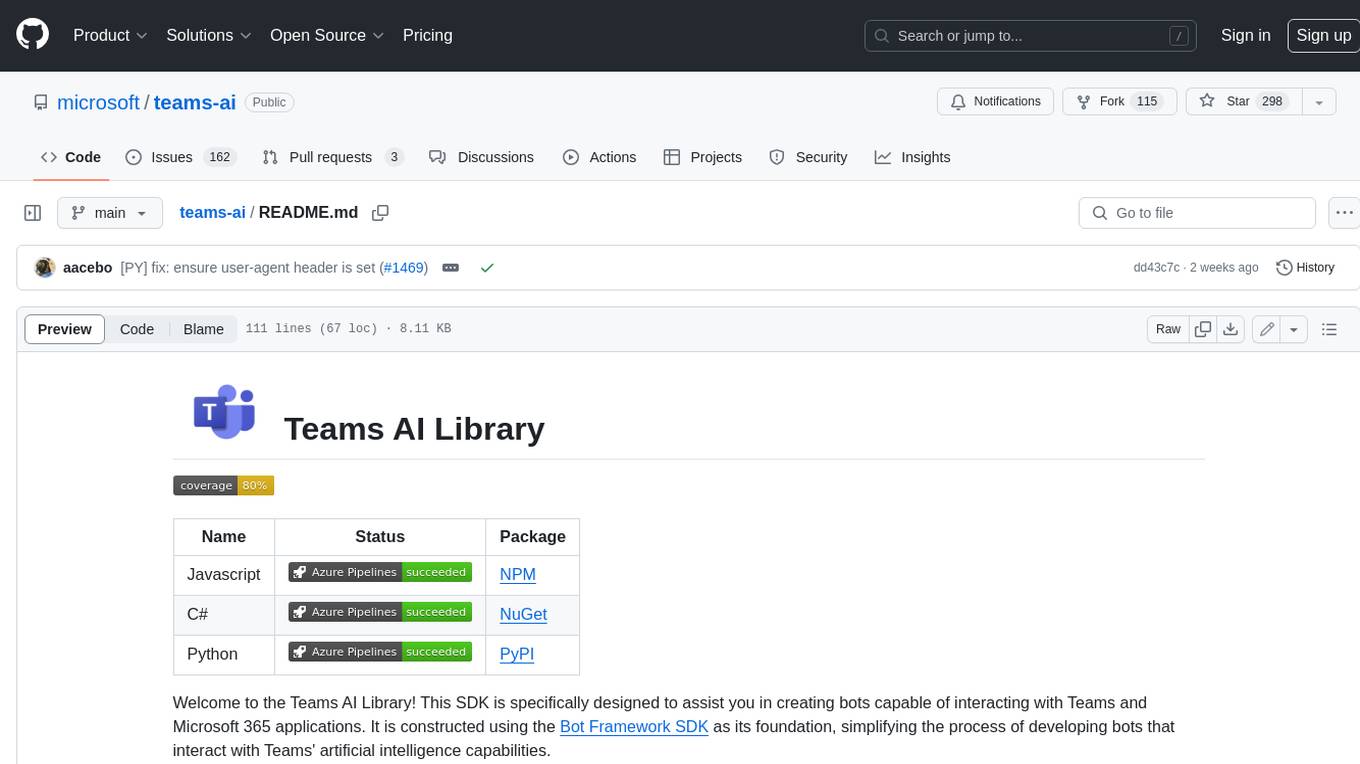
teams-ai
The Teams AI Library is a software development kit (SDK) that helps developers create bots that can interact with Teams and Microsoft 365 applications. It is built on top of the Bot Framework SDK and simplifies the process of developing bots that interact with Teams' artificial intelligence capabilities. The SDK is available for JavaScript/TypeScript, .NET, and Python.
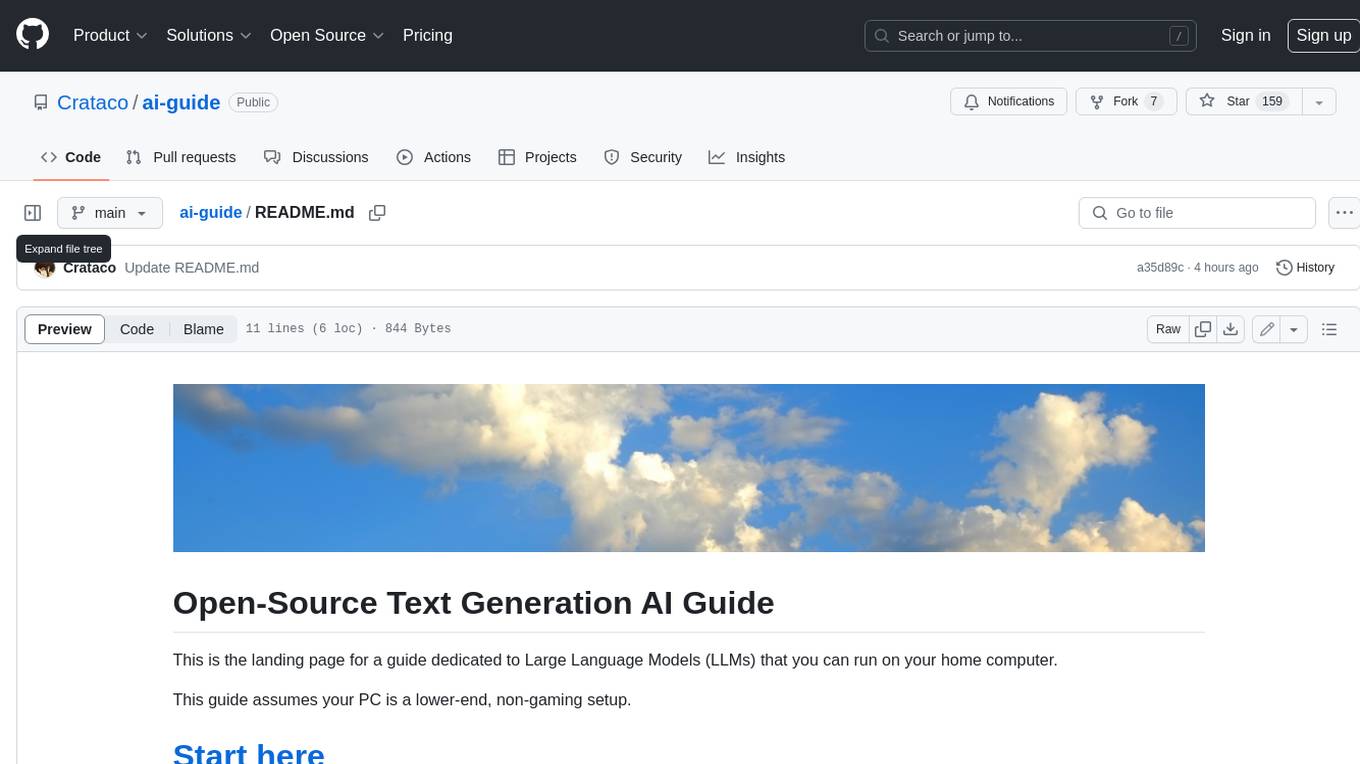
ai-guide
This guide is dedicated to Large Language Models (LLMs) that you can run on your home computer. It assumes your PC is a lower-end, non-gaming setup.
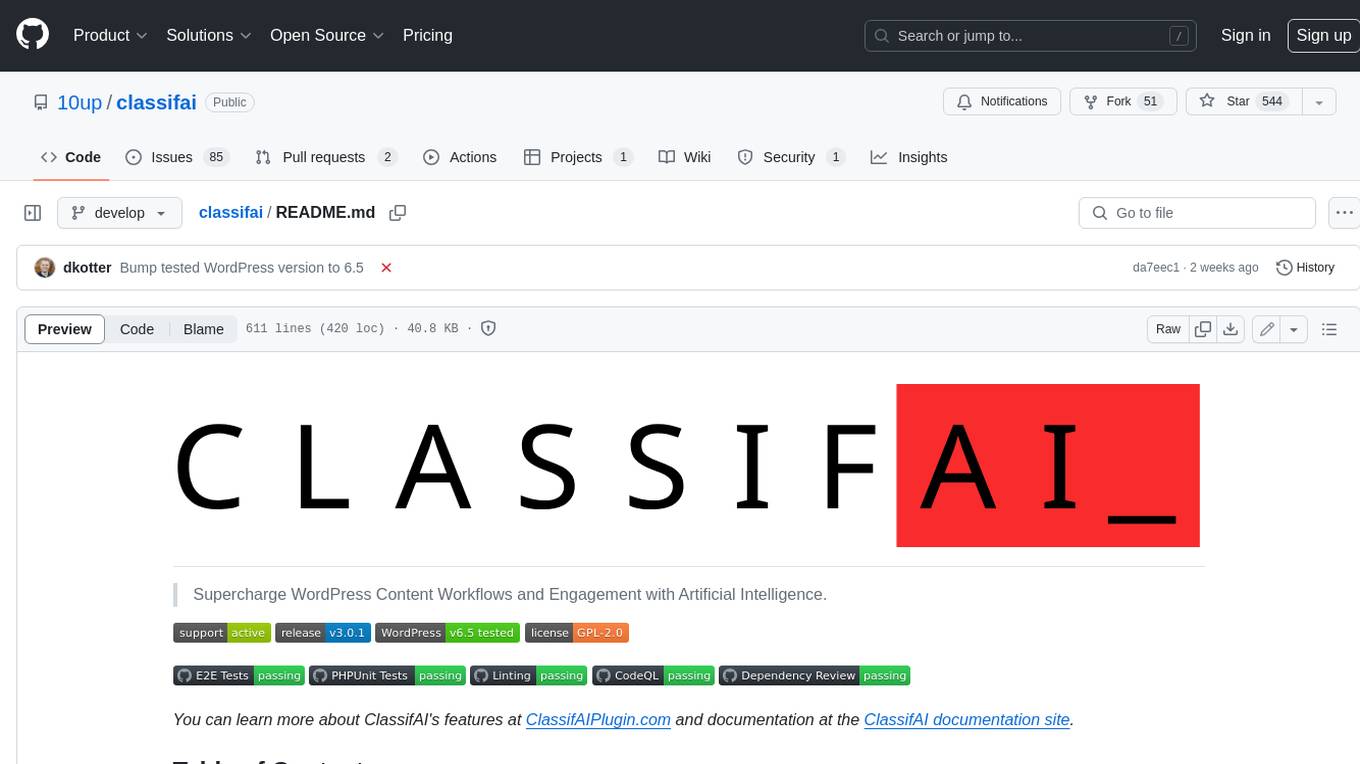
classifai
Supercharge WordPress Content Workflows and Engagement with Artificial Intelligence. Tap into leading cloud-based services like OpenAI, Microsoft Azure AI, Google Gemini and IBM Watson to augment your WordPress-powered websites. Publish content faster while improving SEO performance and increasing audience engagement. ClassifAI integrates Artificial Intelligence and Machine Learning technologies to lighten your workload and eliminate tedious tasks, giving you more time to create original content that matters.
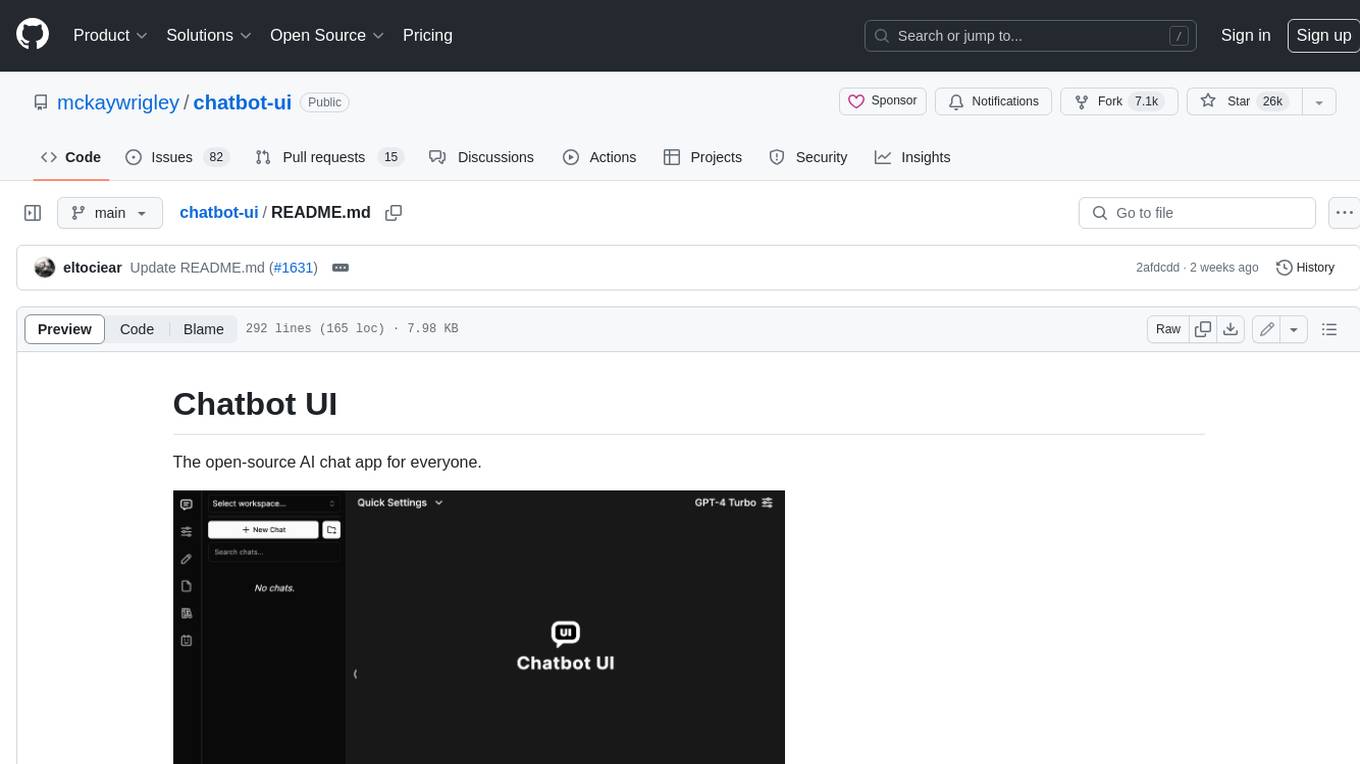
chatbot-ui
Chatbot UI is an open-source AI chat app that allows users to create and deploy their own AI chatbots. It is easy to use and can be customized to fit any need. Chatbot UI is perfect for businesses, developers, and anyone who wants to create a chatbot.
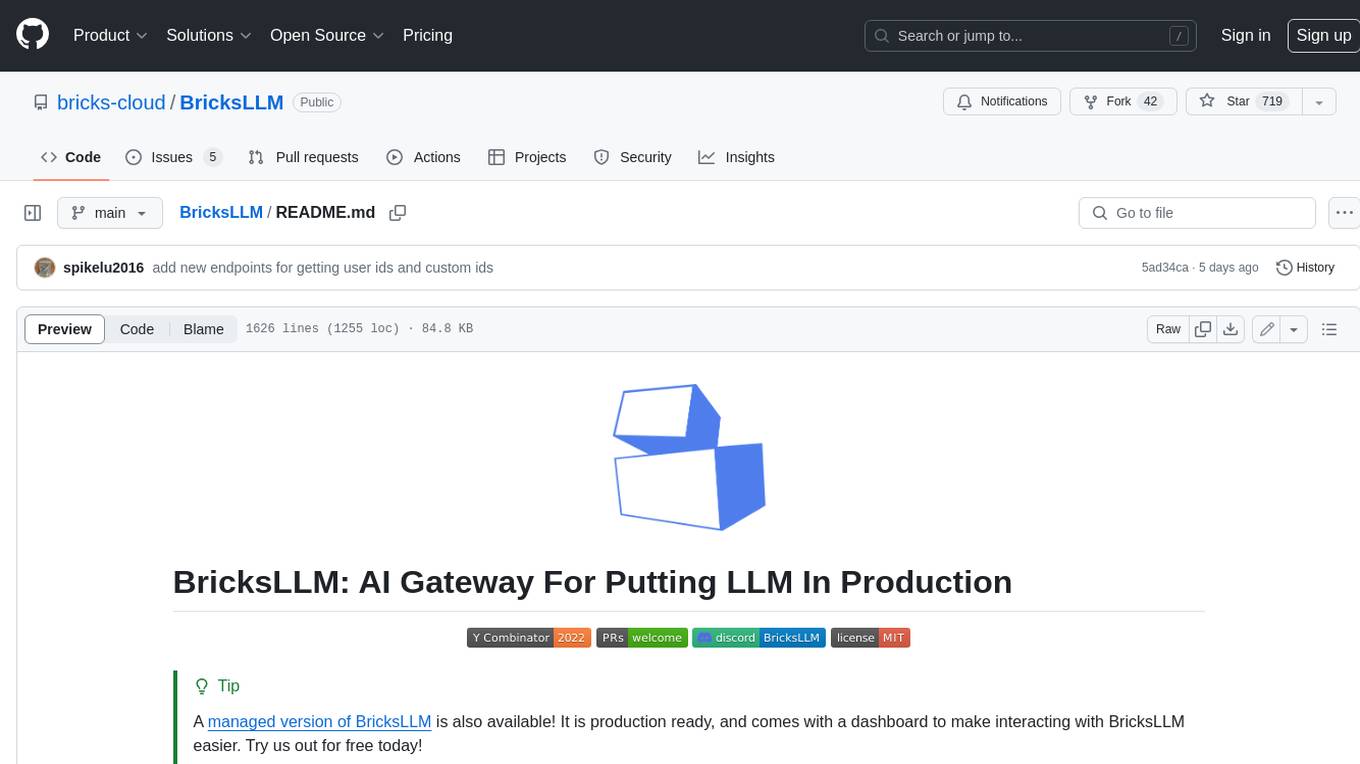
BricksLLM
BricksLLM is a cloud native AI gateway written in Go. Currently, it provides native support for OpenAI, Anthropic, Azure OpenAI and vLLM. BricksLLM aims to provide enterprise level infrastructure that can power any LLM production use cases. Here are some use cases for BricksLLM: * Set LLM usage limits for users on different pricing tiers * Track LLM usage on a per user and per organization basis * Block or redact requests containing PIIs * Improve LLM reliability with failovers, retries and caching * Distribute API keys with rate limits and cost limits for internal development/production use cases * Distribute API keys with rate limits and cost limits for students
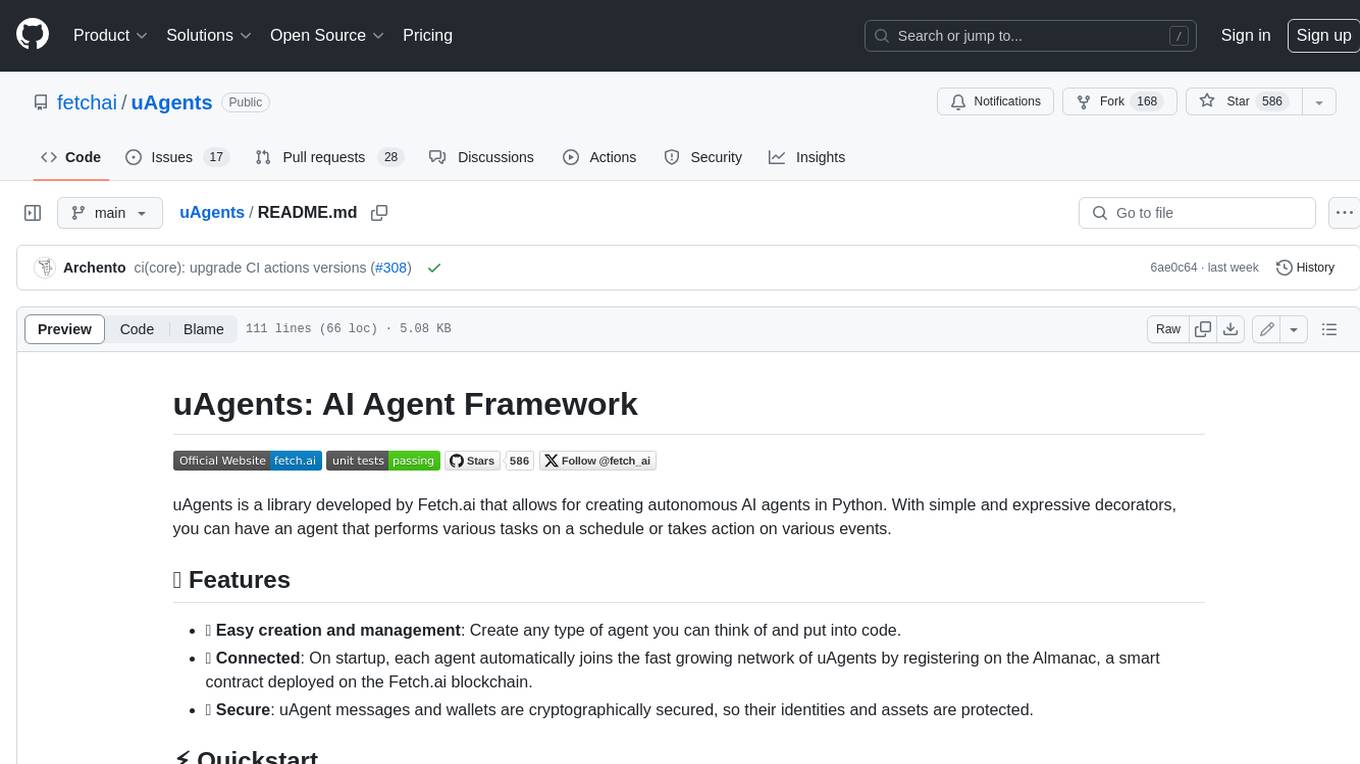
uAgents
uAgents is a Python library developed by Fetch.ai that allows for the creation of autonomous AI agents. These agents can perform various tasks on a schedule or take action on various events. uAgents are easy to create and manage, and they are connected to a fast-growing network of other uAgents. They are also secure, with cryptographically secured messages and wallets.
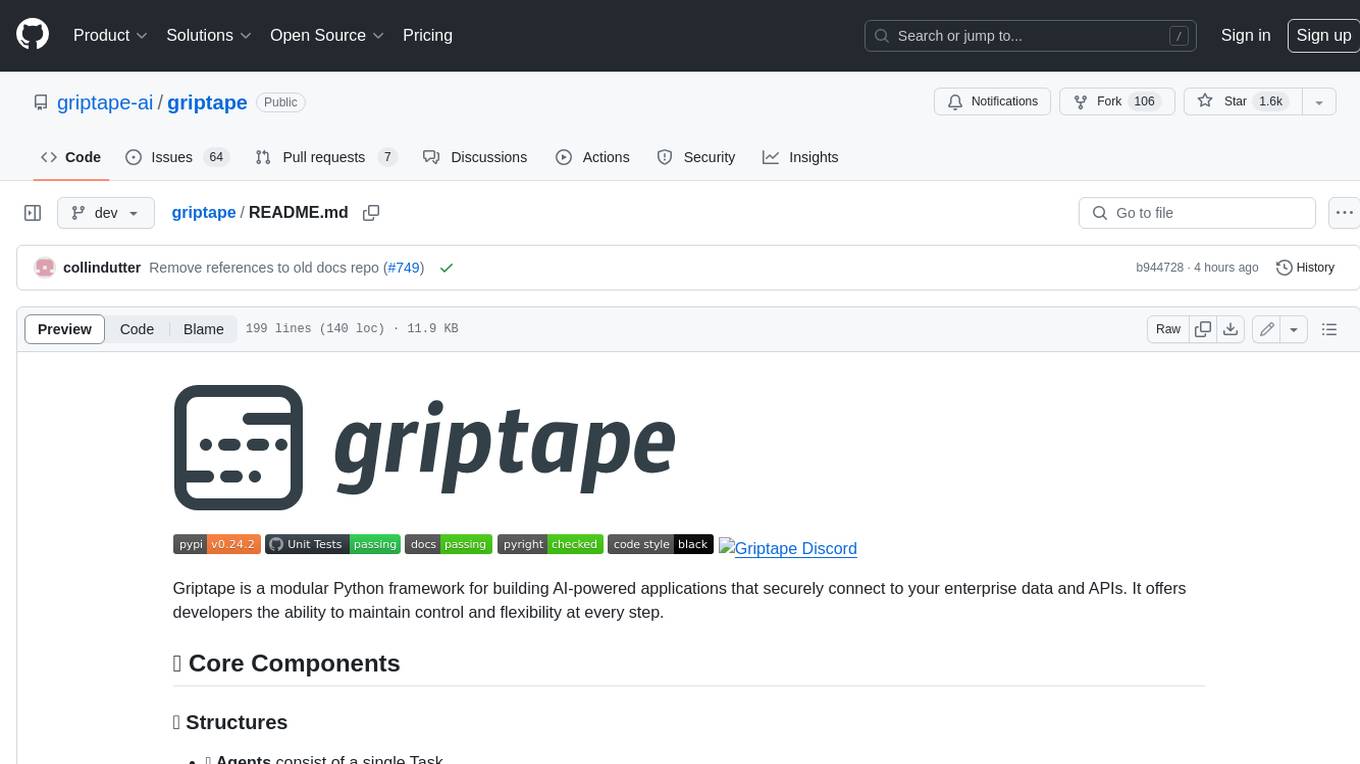
griptape
Griptape is a modular Python framework for building AI-powered applications that securely connect to your enterprise data and APIs. It offers developers the ability to maintain control and flexibility at every step. Griptape's core components include Structures (Agents, Pipelines, and Workflows), Tasks, Tools, Memory (Conversation Memory, Task Memory, and Meta Memory), Drivers (Prompt and Embedding Drivers, Vector Store Drivers, Image Generation Drivers, Image Query Drivers, SQL Drivers, Web Scraper Drivers, and Conversation Memory Drivers), Engines (Query Engines, Extraction Engines, Summary Engines, Image Generation Engines, and Image Query Engines), and additional components (Rulesets, Loaders, Artifacts, Chunkers, and Tokenizers). Griptape enables developers to create AI-powered applications with ease and efficiency.