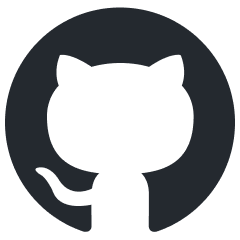
VideoLLaMA2
VideoLLaMA 2: Advancing Spatial-Temporal Modeling and Audio Understanding in Video-LLMs
Stars: 630
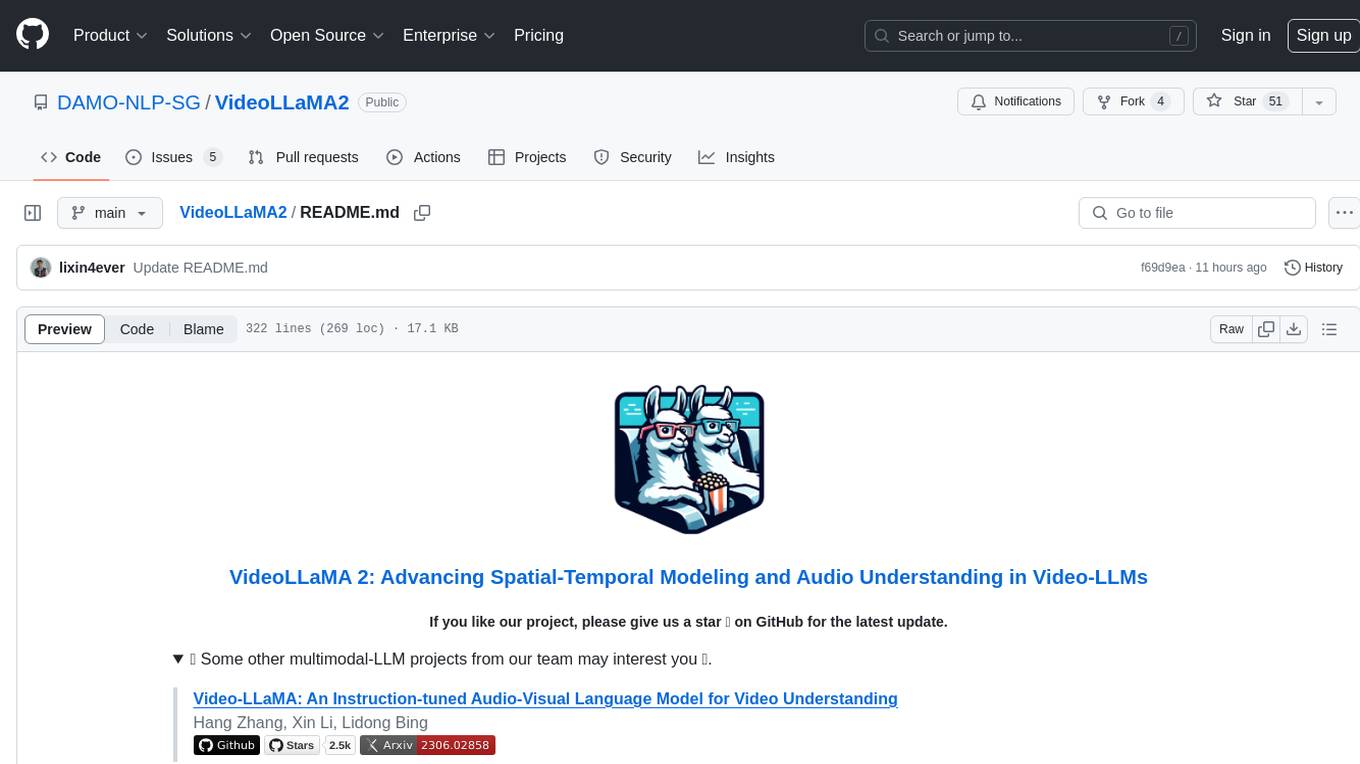
VideoLLaMA 2 is a project focused on advancing spatial-temporal modeling and audio understanding in video-LLMs. It provides tools for multi-choice video QA, open-ended video QA, and video captioning. The project offers model zoo with different configurations for visual encoder and language decoder. It includes training and evaluation guides, as well as inference capabilities for video and image processing. The project also features a demo setup for running a video-based Large Language Model web demonstration.
README:
๐ก Some other multimodal-LLM projects from our team may interest you โจ.
Video-LLaMA: An Instruction-tuned Audio-Visual Language Model for Video Understanding
Hang Zhang, Xin Li, Lidong Bing
![]()
![]()
![]()
VCD: Mitigating Object Hallucinations in Large Vision-Language Models through Visual Contrastive Decoding
Sicong Leng, Hang Zhang, Guanzheng Chen, Xin Li, Shijian Lu, Chunyan Miao, Lidong Bing
![]()
![]()
![]()
- [2024.07.30] Release checkpoints of VideoLLaMA2-8x7B-Base and VideoLLaMA2-8x7B.
- [2024.06.25] ๐ฅ๐ฅ As of Jun 25, our VideoLLaMA2-7B-16F is the Top-1 ~7B-sized VideoLLM on the MLVU Leaderboard.
- [2024.06.18] ๐ฅ๐ฅ As of Jun 18, our VideoLLaMA2-7B-16F is the Top-1 ~7B-sized VideoLLM on the VideoMME Leaderboard.
- [2024.06.17] ๐๐ Update technical report with the latest results and the missing references. If you have works closely related to VideoLLaMA 2 but not mentioned in the paper, feel free to let us know.
- [2024.06.14] ๐ฅ๐ฅ Online Demo is available.
- [2024.06.03] Release training, evaluation, and serving codes of VideoLLaMA 2.
Basic Dependencies:
- Python >= 3.8
- Pytorch >= 2.2.0
- CUDA Version >= 11.8
- transformers >= 4.41.2 (for mistral tokenizer)
- tokenizers >= 0.19.1 (for mistral tokenizer)
[Online Mode] Install required packages (better for development):
git clone https://github.com/DAMO-NLP-SG/VideoLLaMA2
cd VideoLLaMA2
pip install -r requirements.txt
pip install flash-attn==2.5.8 --no-build-isolation
[Offline Mode] Install VideoLLaMA2 as a Python package (better for direct use):
git clone https://github.com/DAMO-NLP-SG/VideoLLaMA2
cd VideoLLaMA2
pip install --upgrade pip # enable PEP 660 support
pip install -e .
pip install flash-attn==2.5.8 --no-build-isolation
Model Name | Model Type | Visual Encoder | Language Decoder | # Training Frames |
---|---|---|---|---|
VideoLLaMA2-7B-Base | Base | clip-vit-large-patch14-336 | Mistral-7B-Instruct-v0.2 | 8 |
VideoLLaMA2-7B | Chat | clip-vit-large-patch14-336 | Mistral-7B-Instruct-v0.2 | 8 |
VideoLLaMA2-7B-16F-Base | Base | clip-vit-large-patch14-336 | Mistral-7B-Instruct-v0.2 | 16 |
VideoLLaMA2-7B-16F | Chat | clip-vit-large-patch14-336 | Mistral-7B-Instruct-v0.2 | 16 |
VideoLLaMA2-8x7B-Base | Base | clip-vit-large-patch14-336 | Mixtral-8x7B-Instruct-v0.1 | 8 |
VideoLLaMA2-8x7B | Chat | clip-vit-large-patch14-336 | Mixtral-8x7B-Instruct-v0.1 | 8 |
VideoLLaMA2-72B-Base | Base | clip-vit-large-patch14-336 | Qwen2-72B-Instruct | 8 |
VideoLLaMA2-72B | Chat | clip-vit-large-patch14-336 | Qwen2-72B-Instruct | 8 |
It is highly recommended to try our online demo first.
To run a video-based LLM (Large Language Model) web demonstration on your device, you will first need to ensure that you have the necessary model checkpoints prepared, followed by adhering to the steps outlined to successfully launch the demo.
- Launch a gradio app directly (VideoLLaMA2-7B is adopted by default):
python videollama2/serve/gradio_web_server_adhoc.py
- Launch a global controller
cd /path/to/VideoLLaMA2
python -m videollama2.serve.controller --host 0.0.0.0 --port 10000
- Launch a gradio webserver
python -m videollama2.serve.gradio_web_server --controller http://localhost:10000 --model-list-mode reload
- Launch one or multiple model workers
# export HF_ENDPOINT=https://hf-mirror.com # If you are unable to access Hugging Face, try to uncomment this line.
python -m videollama2.serve.model_worker --host 0.0.0.0 --controller http://localhost:10000 --port 40000 --worker http://localhost:40000 --model-path /PATH/TO/MODEL1
python -m videollama2.serve.model_worker --host 0.0.0.0 --controller http://localhost:10000 --port 40001 --worker http://localhost:40001 --model-path /PATH/TO/MODEL2
python -m videollama2.serve.model_worker --host 0.0.0.0 --controller http://localhost:10000 --port 40002 --worker http://localhost:40002 --model-path /PATH/TO/MODEL3
...
To facilitate further development on top of our codebase, we provide a quick-start guide on how to train a customized VideoLLaMA2 with VideoLLaVA dataset and evaluate the trained model on the mainstream video-llm benchmarks.
- Training Data Structure:
VideoLLaMA2
โโโ datasets
โ โโโ videollava_pt
| | โโโ llava_image/ # Available at: https://pan.baidu.com/s/17GYcE69FcJjjUM0e4Gad2w?pwd=9ga3 or https://drive.google.com/drive/folders/1QmFj2FcMAoWNCUyiUtdcW0-IOhLbOBcf?usp=drive_link
| | โโโ valley/ # Available at: https://pan.baidu.com/s/1jluOimE7mmihEBfnpwwCew?pwd=jyjz or https://drive.google.com/drive/folders/1QmFj2FcMAoWNCUyiUtdcW0-IOhLbOBcf?usp=drive_link
| | โโโ valley_llavaimage.json # Available at: https://drive.google.com/file/d/1zGRyVSUMoczGq6cjQFmT0prH67bu2wXD/view, including 703K video-text and 558K image-text pairs
โ โโโ videollava_sft
| | โโโ llava_image_tune/ # Available at: https://pan.baidu.com/s/1l-jT6t_DlN5DTklwArsqGw?pwd=o6ko
| | โโโ videochatgpt_tune/ # Available at: https://pan.baidu.com/s/10hJ_U7wVmYTUo75YHc_n8g?pwd=g1hf
| | โโโ videochatgpt_llavaimage_tune.json # Available at: https://drive.google.com/file/d/1zGRyVSUMoczGq6cjQFmT0prH67bu2wXD/view, including 100K video-centric, 625K image-centric and 40K text-only conversations
- Command:
# VideoLLaMA2-vllava pretraining
bash scripts/vllava/pretrain.sh
# VideoLLaMA2-vllava finetuning
bash scripts/vllava/finetune.sh
- Evaluation Data Structure:
VideoLLaMA2
โโโ eval
โ โโโ egoschema # Official website: https://github.com/egoschema/EgoSchema
| | โโโ good_clips_git/ # Available at: https://drive.google.com/drive/folders/1SS0VVz8rML1e5gWq7D7VtP1oxE2UtmhQ
| | โโโ questions.json # Available at: https://github.com/egoschema/EgoSchema/blob/main/questions.json
โ โโโ mvbench # Official website: https://huggingface.co/datasets/OpenGVLab/MVBench
| | โโโ video/
| | | โโโ clever/
| | | โโโ ...
| | โโโ json/
| | | โโโ action_antonym.json
| | | โโโ ...
โ โโโ perception_test_mcqa # Official website: https://huggingface.co/datasets/OpenGVLab/MVBench
| | โโโ videos/ # Available at: https://storage.googleapis.com/dm-perception-test/zip_data/test_videos.zip
| | โโโ mc_question_test.json # Download from https://storage.googleapis.com/dm-perception-test/zip_data/mc_question_test_annotations.zip
โ โโโ videomme # Official website: https://video-mme.github.io/home_page.html#leaderboard
| | โโโ test-00000-of-00001.parquet
| | โโโ videos/
| | โโโ subtitles/
โ โโโ Activitynet_Zero_Shot_QA # Official website: https://github.com/MILVLG/activitynet-qa
| | โโโ all_test/ # Available at: https://mbzuaiac-my.sharepoint.com/:u:/g/personal/hanoona_bangalath_mbzuai_ac_ae/EatOpE7j68tLm2XAd0u6b8ABGGdVAwLMN6rqlDGM_DwhVA?e=90WIuW
| | โโโ test_q.json # Available at: https://github.com/MILVLG/activitynet-qa/tree/master/dataset
| | โโโ test_a.json # Available at: https://github.com/MILVLG/activitynet-qa/tree/master/dataset
โ โโโ MSVD_Zero_Shot_QA # Official website: https://github.com/xudejing/video-question-answering
| | โโโ videos/
| | โโโ test_q.json
| | โโโ test_a.json
โ โโโ videochatgpt_gen # Official website: https://github.com/mbzuai-oryx/Video-ChatGPT/tree/main/quantitative_evaluation
| | โโโ Test_Videos/ # Available at: https://mbzuaiac-my.sharepoint.com/:u:/g/personal/hanoona_bangalath_mbzuai_ac_ae/EatOpE7j68tLm2XAd0u6b8ABGGdVAwLMN6rqlDGM_DwhVA?e=90WIuW
| | โโโ Test_Human_Annotated_Captions/ # Available at: https://mbzuaiac-my.sharepoint.com/personal/hanoona_bangalath_mbzuai_ac_ae/_layouts/15/onedrive.aspx?id=%2Fpersonal%2Fhanoona%5Fbangalath%5Fmbzuai%5Fac%5Fae%2FDocuments%2FVideo%2DChatGPT%2FData%5FCode%5FModel%5FRelease%2FQuantitative%5FEvaluation%2Fbenchamarking%2FTest%5FHuman%5FAnnotated%5FCaptions%2Ezip&parent=%2Fpersonal%2Fhanoona%5Fbangalath%5Fmbzuai%5Fac%5Fae%2FDocuments%2FVideo%2DChatGPT%2FData%5FCode%5FModel%5FRelease%2FQuantitative%5FEvaluation%2Fbenchamarking&ga=1
| | โโโ generic_qa.json # These three json files available at: https://mbzuaiac-my.sharepoint.com/personal/hanoona_bangalath_mbzuai_ac_ae/_layouts/15/onedrive.aspx?id=%2Fpersonal%2Fhanoona%5Fbangalath%5Fmbzuai%5Fac%5Fae%2FDocuments%2FVideo%2DChatGPT%2FData%5FCode%5FModel%5FRelease%2FQuantitative%5FEvaluation%2Fbenchamarking%2FBenchmarking%5FQA&ga=1
| | โโโ temporal_qa.json
| | โโโ consistency_qa.json
- Command:
# mvbench evaluation
CUDA_VISIBLE_DEVICES=0,1,2,3,4,5,6,7 bash scripts/eval/eval_video_qa_mvbench.sh
# activitynet-qa evaluation (need to set azure openai key/endpoint/deployname)
CUDA_VISIBLE_DEVICES=0,1,2,3,4,5,6,7 bash scripts/eval/eval_video_qa_mvbench.sh
If you want to train a video-llm on your data, you need to follow the procedures below to prepare the video/image sft data:
- Suppose your data structure is like:
VideoLLaMA2
โโโ datasets
โ โโโ custom_sft
โ | โโโ images
โ | โโโ videos
| | โโโ custom.json
- Then you should re-organize the annotated video/image sft data according to the following format:
[
{
"id": 0,
"video": "images/xxx.jpg",
"conversations": [
{
"from": "human",
"value": "<image>\nWhat are the colors of the bus in the image?"
},
{
"from": "gpt",
"value": "The bus in the image is white and red."
},
...
],
}
{
"id": 1,
"video": "videos/xxx.mp4",
"conversations": [
{
"from": "human",
"value": "<video>\nWhat are the main activities that take place in the video?"
},
{
"from": "gpt",
"value": "The main activities that take place in the video are the preparation of camera equipment by a man, a group of men riding a helicopter, and a man sailing a boat through the water."
},
...
],
},
...
]
- Modify the
scripts/custom/finetune.sh
:
...
--data_path datasets/custom_sft/custom.json
--data_folder datasets/custom_sft/
--pretrain_mm_mlp_adapter CONNECTOR_DOWNLOAD_PATH (e.g., DAMO-NLP-SG/VideoLLaMA2-7B-Base)
...
Video/Image Inference:
import sys
sys.path.append('./')
from videollama2 import model_init, mm_infer
from videollama2.utils import disable_torch_init
def inference():
disable_torch_init()
# Video Inference
modal = 'video'
modal_path = 'assets/cat_and_chicken.mp4'
instruct = 'What animals are in the video, what are they doing, and how does the video feel?'
# Reply:
# The video features a kitten and a baby chick playing together. The kitten is seen laying on the floor while the baby chick hops around. The two animals interact playfully with each other, and the video has a cute and heartwarming feel to it.
# Image Inference
modal = 'image'
modal_path = 'assets/sora.png'
instruct = 'What is the woman wearing, what is she doing, and how does the image feel?'
# Reply:
# The woman in the image is wearing a black coat and sunglasses, and she is walking down a rain-soaked city street. The image feels vibrant and lively, with the bright city lights reflecting off the wet pavement, creating a visually appealing atmosphere. The woman's presence adds a sense of style and confidence to the scene, as she navigates the bustling urban environment.
model_path = 'DAMO-NLP-SG/VideoLLaMA2-7B'
# Base model inference (only need to replace model_path)
# model_path = 'DAMO-NLP-SG/VideoLLaMA2-7B-Base'
model, processor, tokenizer = model_init(model_path)
output = mm_infer(processor[modal](modal_path), instruct, model=model, tokenizer=tokenizer, do_sample=False, modal=modal)
print(output)
if __name__ == "__main__":
inference()
If you find VideoLLaMA useful for your research and applications, please cite using this BibTeX:
@article{damonlpsg2024videollama2,
title={VideoLLaMA 2: Advancing Spatial-Temporal Modeling and Audio Understanding in Video-LLMs},
author={Cheng, Zesen and Leng, Sicong and Zhang, Hang and Xin, Yifei and Li, Xin and Chen, Guanzheng and Zhu, Yongxin and Zhang, Wenqi and Luo, Ziyang and Zhao, Deli and Bing, Lidong},
journal={arXiv preprint arXiv:2406.07476},
year={2024},
url = {https://arxiv.org/abs/2406.07476}
}
@article{damonlpsg2023videollama,
title = {Video-LLaMA: An Instruction-tuned Audio-Visual Language Model for Video Understanding},
author = {Zhang, Hang and Li, Xin and Bing, Lidong},
journal = {arXiv preprint arXiv:2306.02858},
year = {2023},
url = {https://arxiv.org/abs/2306.02858}
}
The codebase of VideoLLaMA 2 is adapted from LLaVA 1.5 and FastChat. We are also grateful for the following projects our VideoLLaMA 2 arise from:
- LLaMA 2, Mistral-7B, OpenAI CLIP, Honeybee.
- Video-ChatGPT, Video-LLaVA.
- WebVid, Panda-70M, LanguageBind, InternVid.
- VideoChat2, Valley, VTimeLLM, ShareGPT4V.
This project is released under the Apache 2.0 license as found in the LICENSE file. The service is a research preview intended for non-commercial use ONLY, subject to the model Licenses of LLaMA and Mistral, Terms of Use of the data generated by OpenAI, and Privacy Practices of ShareGPT. Please get in touch with us if you find any potential violations.
For Tasks:
Click tags to check more tools for each tasksFor Jobs:
Alternative AI tools for VideoLLaMA2
Similar Open Source Tools
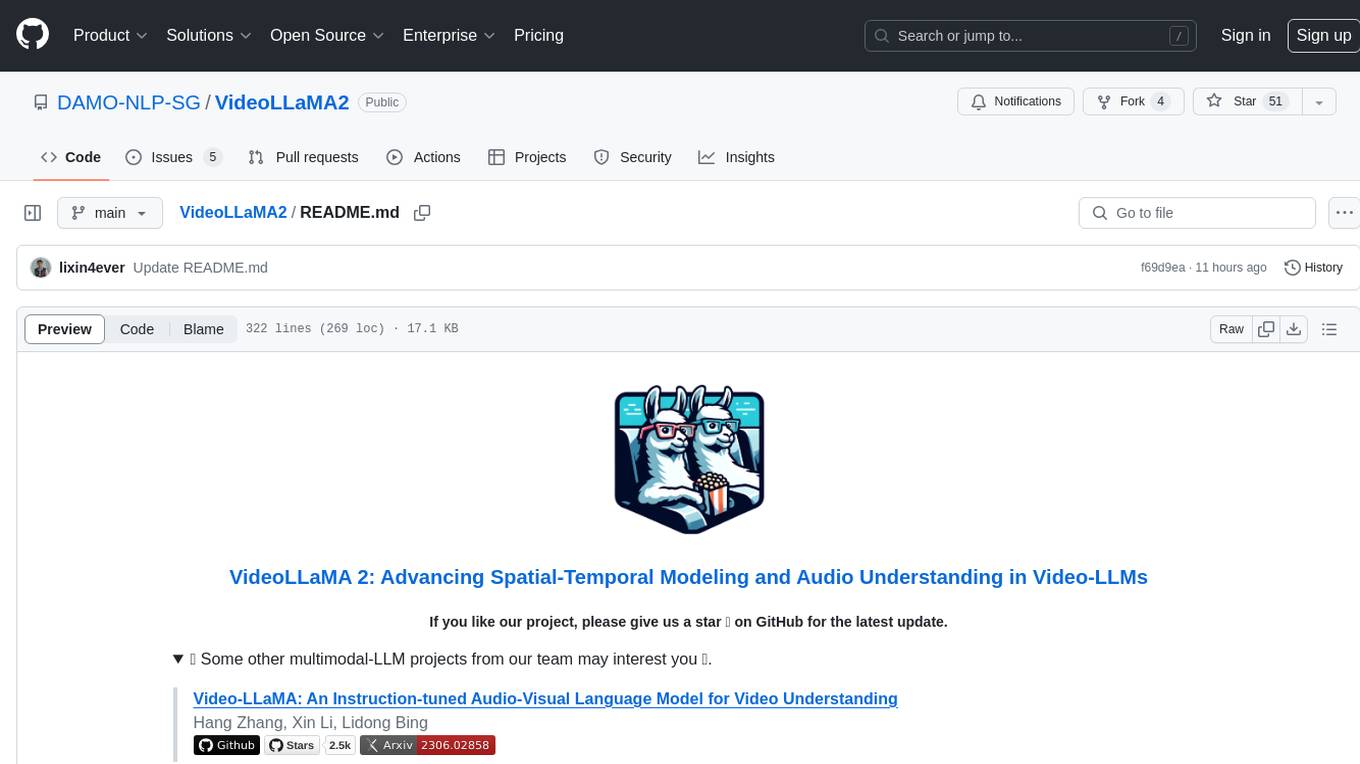
VideoLLaMA2
VideoLLaMA 2 is a project focused on advancing spatial-temporal modeling and audio understanding in video-LLMs. It provides tools for multi-choice video QA, open-ended video QA, and video captioning. The project offers model zoo with different configurations for visual encoder and language decoder. It includes training and evaluation guides, as well as inference capabilities for video and image processing. The project also features a demo setup for running a video-based Large Language Model web demonstration.
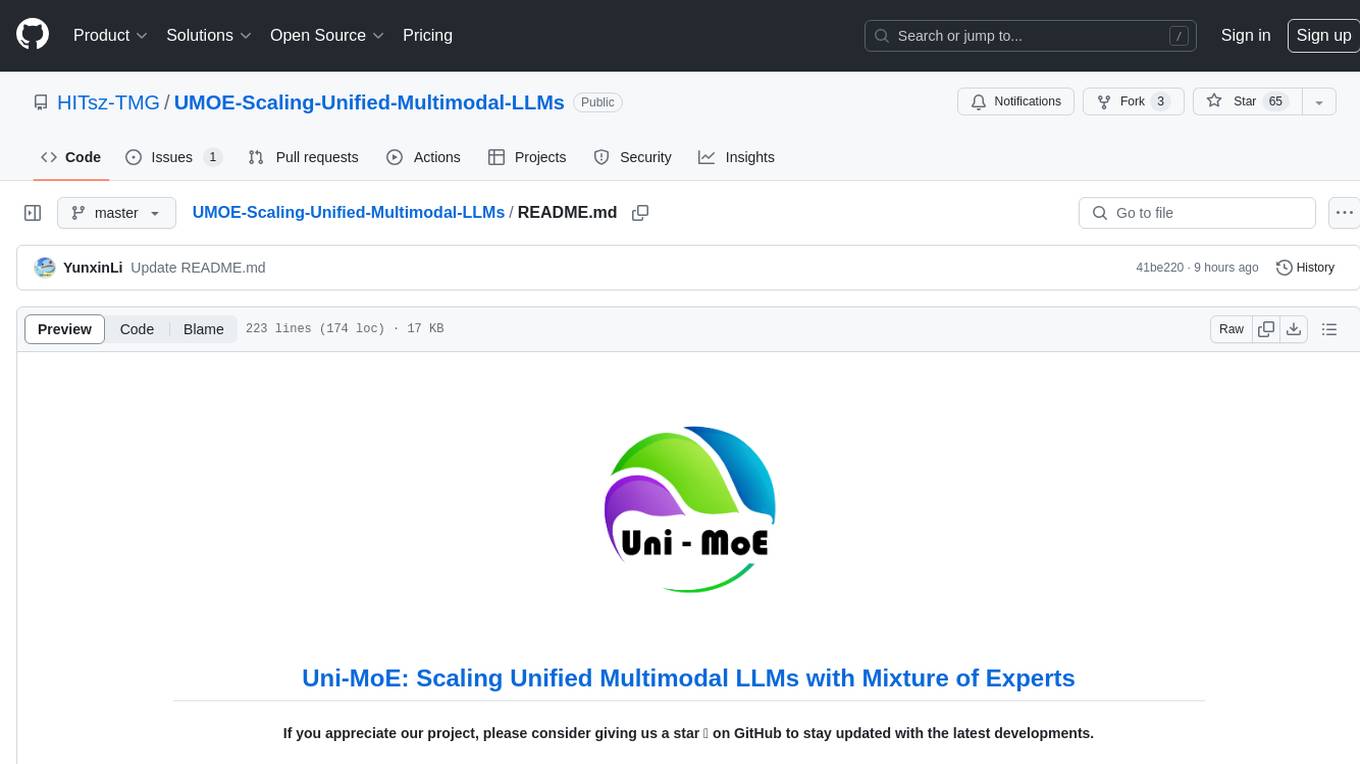
UMOE-Scaling-Unified-Multimodal-LLMs
Uni-MoE is a MoE-based unified multimodal model that can handle diverse modalities including audio, speech, image, text, and video. The project focuses on scaling Unified Multimodal LLMs with a Mixture of Experts framework. It offers enhanced functionality for training across multiple nodes and GPUs, as well as parallel processing at both the expert and modality levels. The model architecture involves three training stages: building connectors for multimodal understanding, developing modality-specific experts, and incorporating multiple trained experts into LLMs using the LoRA technique on mixed multimodal data. The tool provides instructions for installation, weights organization, inference, training, and evaluation on various datasets.
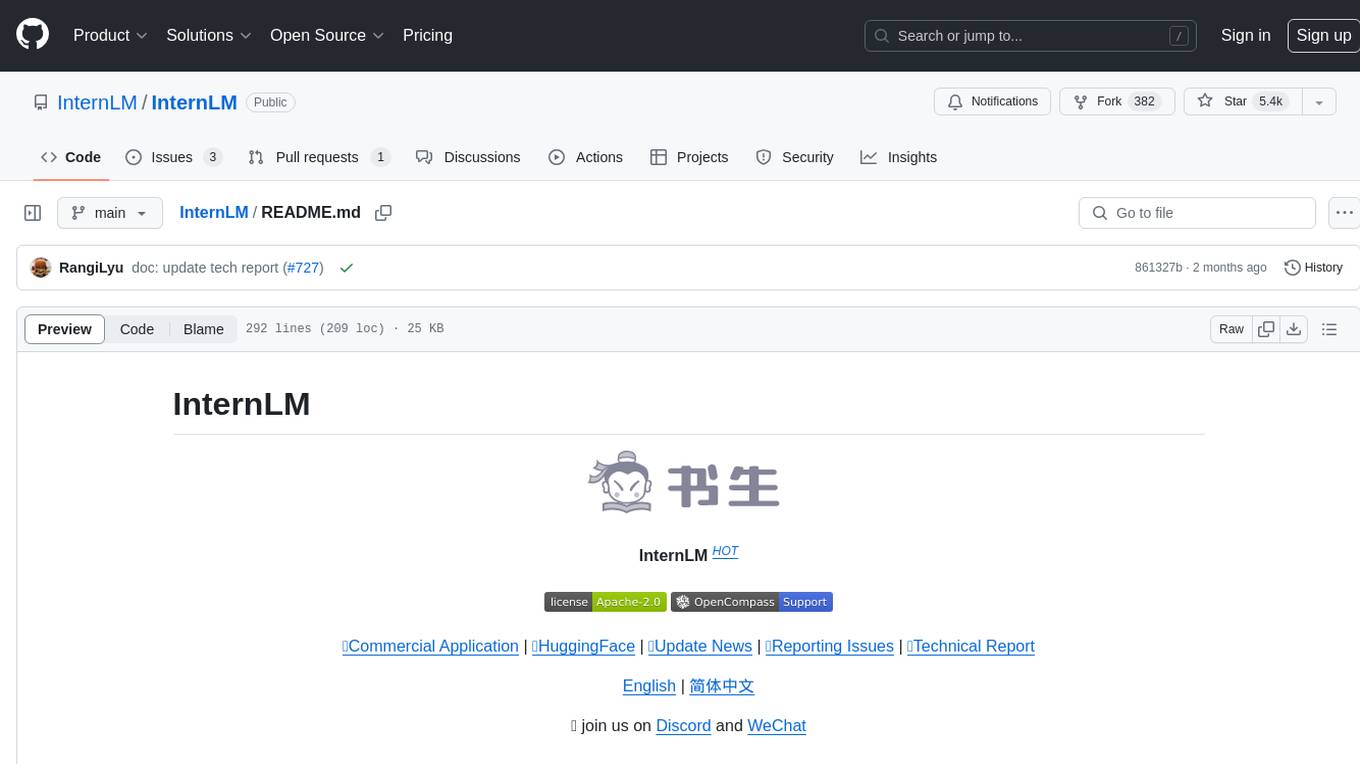
InternLM
InternLM is a powerful language model series with features such as 200K context window for long-context tasks, outstanding comprehensive performance in reasoning, math, code, chat experience, instruction following, and creative writing, code interpreter & data analysis capabilities, and stronger tool utilization capabilities. It offers models in sizes of 7B and 20B, suitable for research and complex scenarios. The models are recommended for various applications and exhibit better performance than previous generations. InternLM models may match or surpass other open-source models like ChatGPT. The tool has been evaluated on various datasets and has shown superior performance in multiple tasks. It requires Python >= 3.8, PyTorch >= 1.12.0, and Transformers >= 4.34 for usage. InternLM can be used for tasks like chat, agent applications, fine-tuning, deployment, and long-context inference.
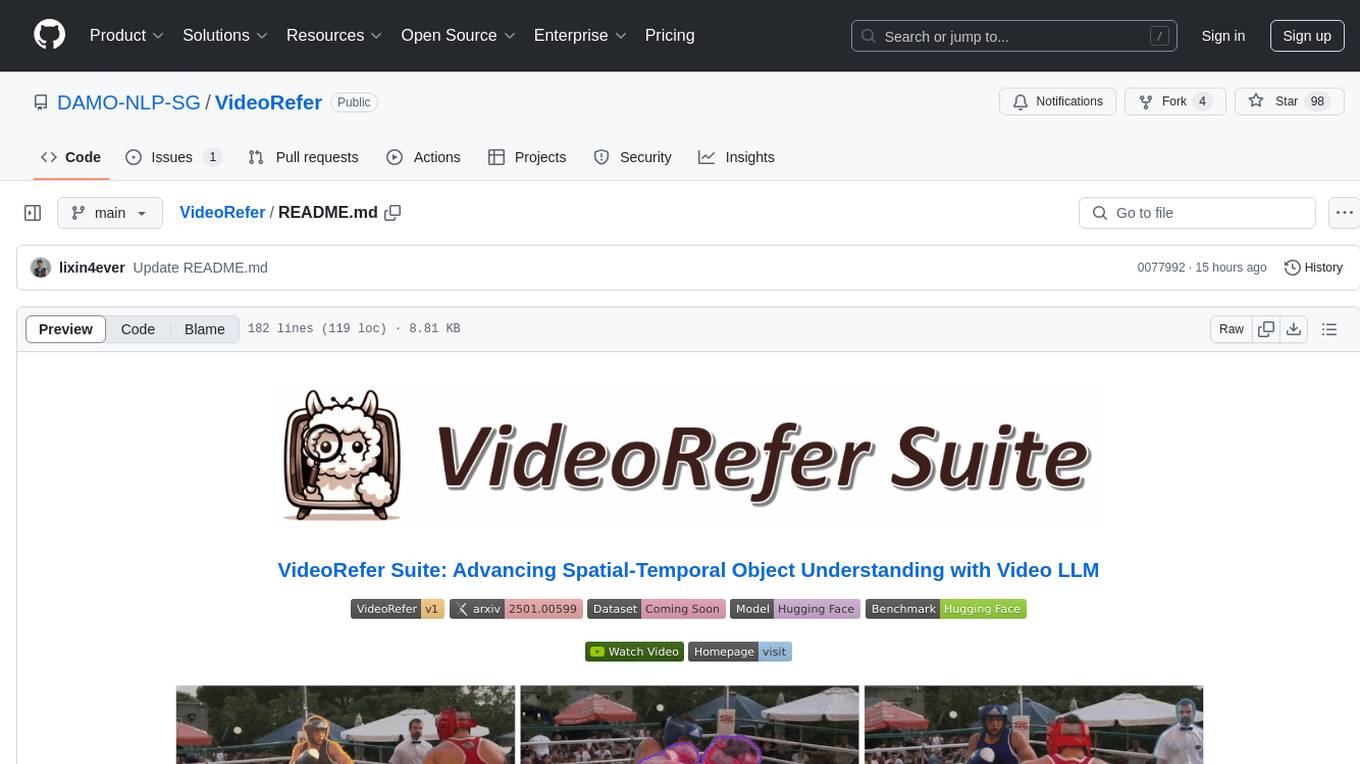
VideoRefer
VideoRefer Suite is a tool designed to enhance the fine-grained spatial-temporal understanding capabilities of Video Large Language Models (Video LLMs). It consists of three primary components: Model (VideoRefer) for perceiving, reasoning, and retrieval for user-defined regions at any specified timestamps, Dataset (VideoRefer-700K) for high-quality object-level video instruction data, and Benchmark (VideoRefer-Bench) to evaluate object-level video understanding capabilities. The tool can understand any object within a video.
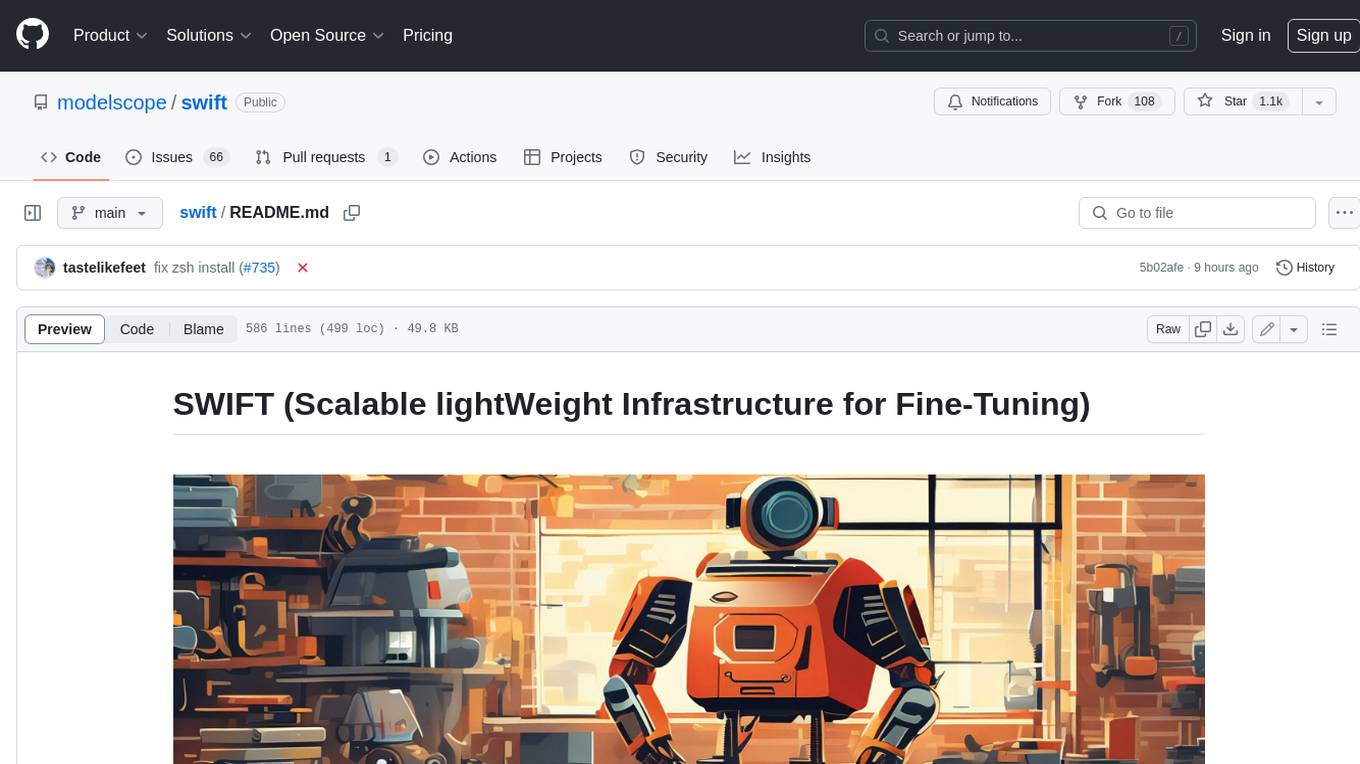
swift
SWIFT (Scalable lightWeight Infrastructure for Fine-Tuning) supports training, inference, evaluation and deployment of nearly **200 LLMs and MLLMs** (multimodal large models). Developers can directly apply our framework to their own research and production environments to realize the complete workflow from model training and evaluation to application. In addition to supporting the lightweight training solutions provided by [PEFT](https://github.com/huggingface/peft), we also provide a complete **Adapters library** to support the latest training techniques such as NEFTune, LoRA+, LLaMA-PRO, etc. This adapter library can be used directly in your own custom workflow without our training scripts. To facilitate use by users unfamiliar with deep learning, we provide a Gradio web-ui for controlling training and inference, as well as accompanying deep learning courses and best practices for beginners. Additionally, we are expanding capabilities for other modalities. Currently, we support full-parameter training and LoRA training for AnimateDiff.
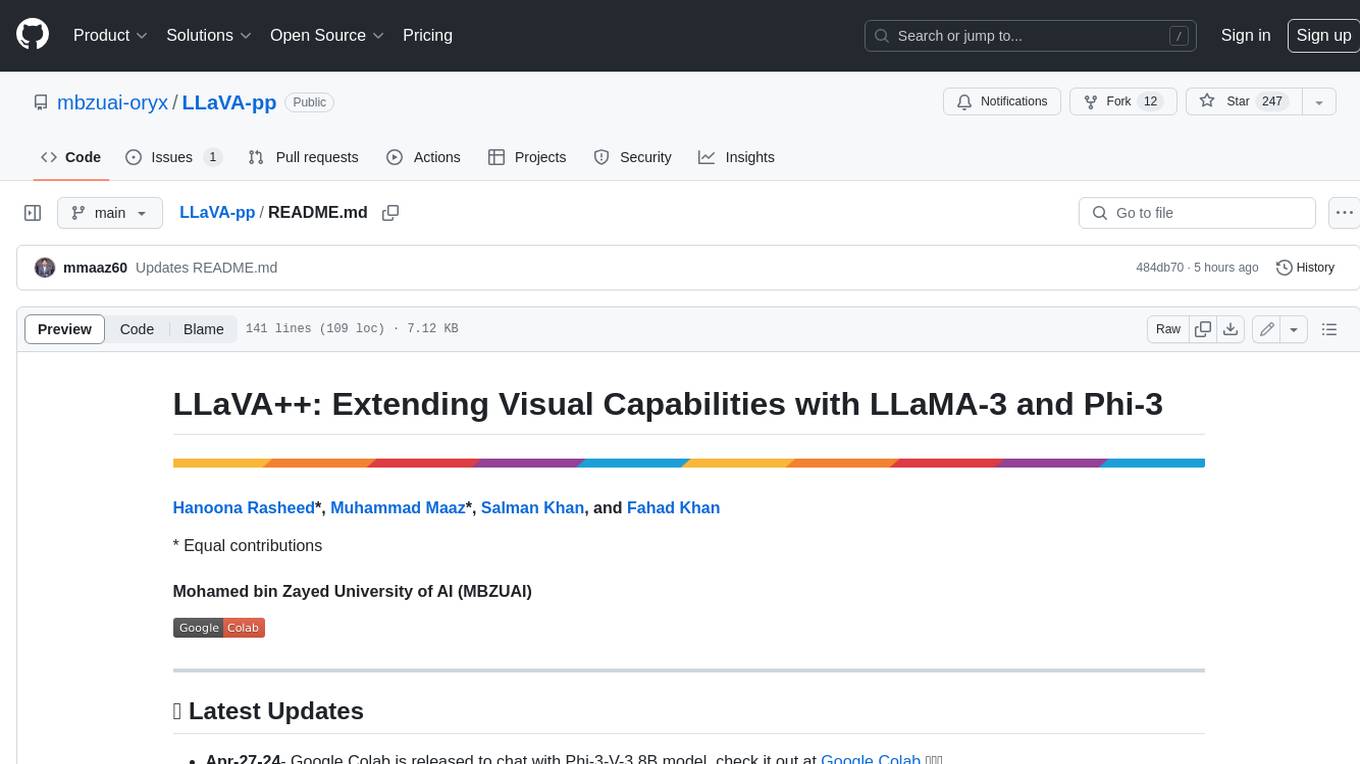
LLaVA-pp
This repository, LLaVA++, extends the visual capabilities of the LLaVA 1.5 model by incorporating the latest LLMs, Phi-3 Mini Instruct 3.8B, and LLaMA-3 Instruct 8B. It provides various models for instruction-following LMMS and academic-task-oriented datasets, along with training scripts for Phi-3-V and LLaMA-3-V. The repository also includes installation instructions and acknowledgments to related open-source contributions.
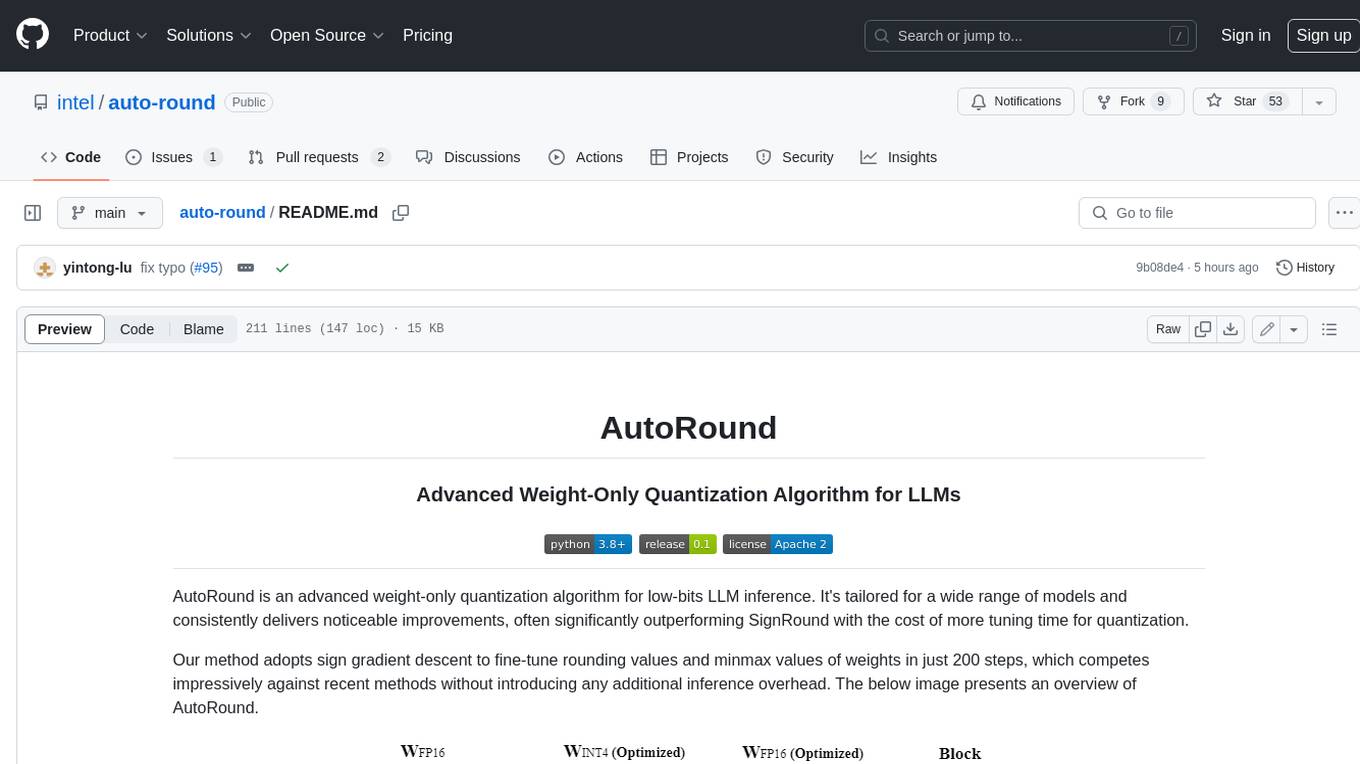
auto-round
AutoRound is an advanced weight-only quantization algorithm for low-bits LLM inference. It competes impressively against recent methods without introducing any additional inference overhead. The method adopts sign gradient descent to fine-tune rounding values and minmax values of weights in just 200 steps, often significantly outperforming SignRound with the cost of more tuning time for quantization. AutoRound is tailored for a wide range of models and consistently delivers noticeable improvements.
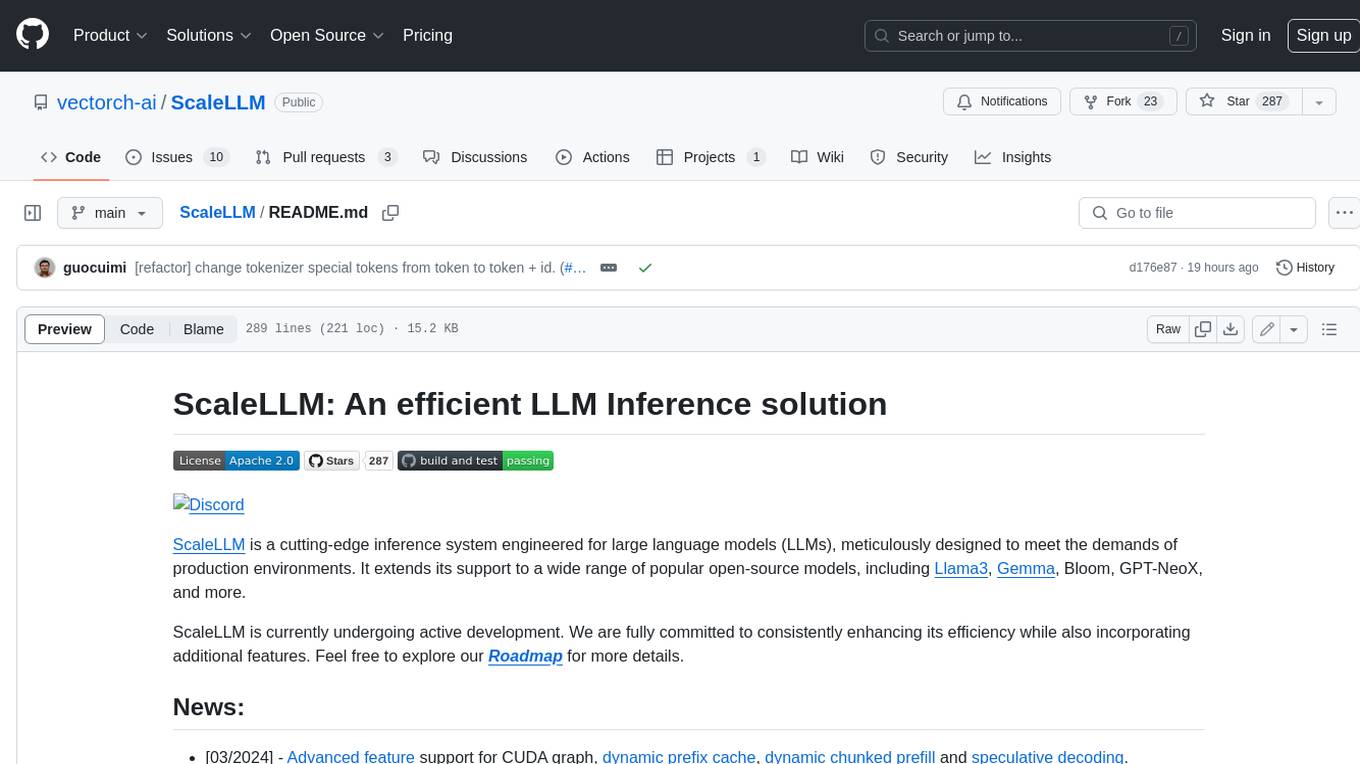
ScaleLLM
ScaleLLM is a cutting-edge inference system engineered for large language models (LLMs), meticulously designed to meet the demands of production environments. It extends its support to a wide range of popular open-source models, including Llama3, Gemma, Bloom, GPT-NeoX, and more. ScaleLLM is currently undergoing active development. We are fully committed to consistently enhancing its efficiency while also incorporating additional features. Feel free to explore our **_Roadmap_** for more details. ## Key Features * High Efficiency: Excels in high-performance LLM inference, leveraging state-of-the-art techniques and technologies like Flash Attention, Paged Attention, Continuous batching, and more. * Tensor Parallelism: Utilizes tensor parallelism for efficient model execution. * OpenAI-compatible API: An efficient golang rest api server that compatible with OpenAI. * Huggingface models: Seamless integration with most popular HF models, supporting safetensors. * Customizable: Offers flexibility for customization to meet your specific needs, and provides an easy way to add new models. * Production Ready: Engineered with production environments in mind, ScaleLLM is equipped with robust system monitoring and management features to ensure a seamless deployment experience.
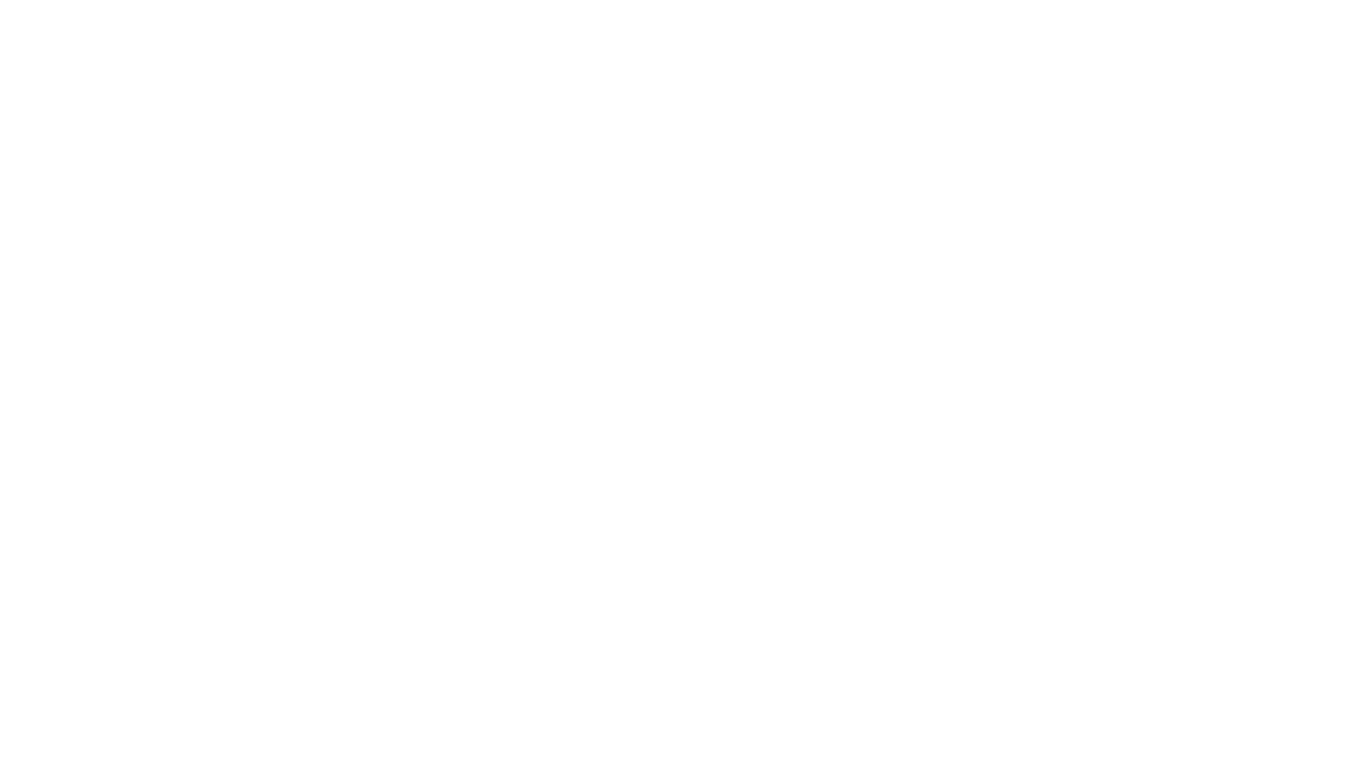
intel-extension-for-transformers
Intelยฎ Extension for Transformers is an innovative toolkit designed to accelerate GenAI/LLM everywhere with the optimal performance of Transformer-based models on various Intel platforms, including Intel Gaudi2, Intel CPU, and Intel GPU. The toolkit provides the below key features and examples: * Seamless user experience of model compressions on Transformer-based models by extending [Hugging Face transformers](https://github.com/huggingface/transformers) APIs and leveraging [Intelยฎ Neural Compressor](https://github.com/intel/neural-compressor) * Advanced software optimizations and unique compression-aware runtime (released with NeurIPS 2022's paper [Fast Distilbert on CPUs](https://arxiv.org/abs/2211.07715) and [QuaLA-MiniLM: a Quantized Length Adaptive MiniLM](https://arxiv.org/abs/2210.17114), and NeurIPS 2021's paper [Prune Once for All: Sparse Pre-Trained Language Models](https://arxiv.org/abs/2111.05754)) * Optimized Transformer-based model packages such as [Stable Diffusion](examples/huggingface/pytorch/text-to-image/deployment/stable_diffusion), [GPT-J-6B](examples/huggingface/pytorch/text-generation/deployment), [GPT-NEOX](examples/huggingface/pytorch/language-modeling/quantization#2-validated-model-list), [BLOOM-176B](examples/huggingface/pytorch/language-modeling/inference#BLOOM-176B), [T5](examples/huggingface/pytorch/summarization/quantization#2-validated-model-list), [Flan-T5](examples/huggingface/pytorch/summarization/quantization#2-validated-model-list), and end-to-end workflows such as [SetFit-based text classification](docs/tutorials/pytorch/text-classification/SetFit_model_compression_AGNews.ipynb) and [document level sentiment analysis (DLSA)](workflows/dlsa) * [NeuralChat](intel_extension_for_transformers/neural_chat), a customizable chatbot framework to create your own chatbot within minutes by leveraging a rich set of [plugins](https://github.com/intel/intel-extension-for-transformers/blob/main/intel_extension_for_transformers/neural_chat/docs/advanced_features.md) such as [Knowledge Retrieval](./intel_extension_for_transformers/neural_chat/pipeline/plugins/retrieval/README.md), [Speech Interaction](./intel_extension_for_transformers/neural_chat/pipeline/plugins/audio/README.md), [Query Caching](./intel_extension_for_transformers/neural_chat/pipeline/plugins/caching/README.md), and [Security Guardrail](./intel_extension_for_transformers/neural_chat/pipeline/plugins/security/README.md). This framework supports Intel Gaudi2/CPU/GPU. * [Inference](https://github.com/intel/neural-speed/tree/main) of Large Language Model (LLM) in pure C/C++ with weight-only quantization kernels for Intel CPU and Intel GPU (TBD), supporting [GPT-NEOX](https://github.com/intel/neural-speed/tree/main/neural_speed/models/gptneox), [LLAMA](https://github.com/intel/neural-speed/tree/main/neural_speed/models/llama), [MPT](https://github.com/intel/neural-speed/tree/main/neural_speed/models/mpt), [FALCON](https://github.com/intel/neural-speed/tree/main/neural_speed/models/falcon), [BLOOM-7B](https://github.com/intel/neural-speed/tree/main/neural_speed/models/bloom), [OPT](https://github.com/intel/neural-speed/tree/main/neural_speed/models/opt), [ChatGLM2-6B](https://github.com/intel/neural-speed/tree/main/neural_speed/models/chatglm), [GPT-J-6B](https://github.com/intel/neural-speed/tree/main/neural_speed/models/gptj), and [Dolly-v2-3B](https://github.com/intel/neural-speed/tree/main/neural_speed/models/gptneox). Support AMX, VNNI, AVX512F and AVX2 instruction set. We've boosted the performance of Intel CPUs, with a particular focus on the 4th generation Intel Xeon Scalable processor, codenamed [Sapphire Rapids](https://www.intel.com/content/www/us/en/products/docs/processors/xeon-accelerated/4th-gen-xeon-scalable-processors.html).
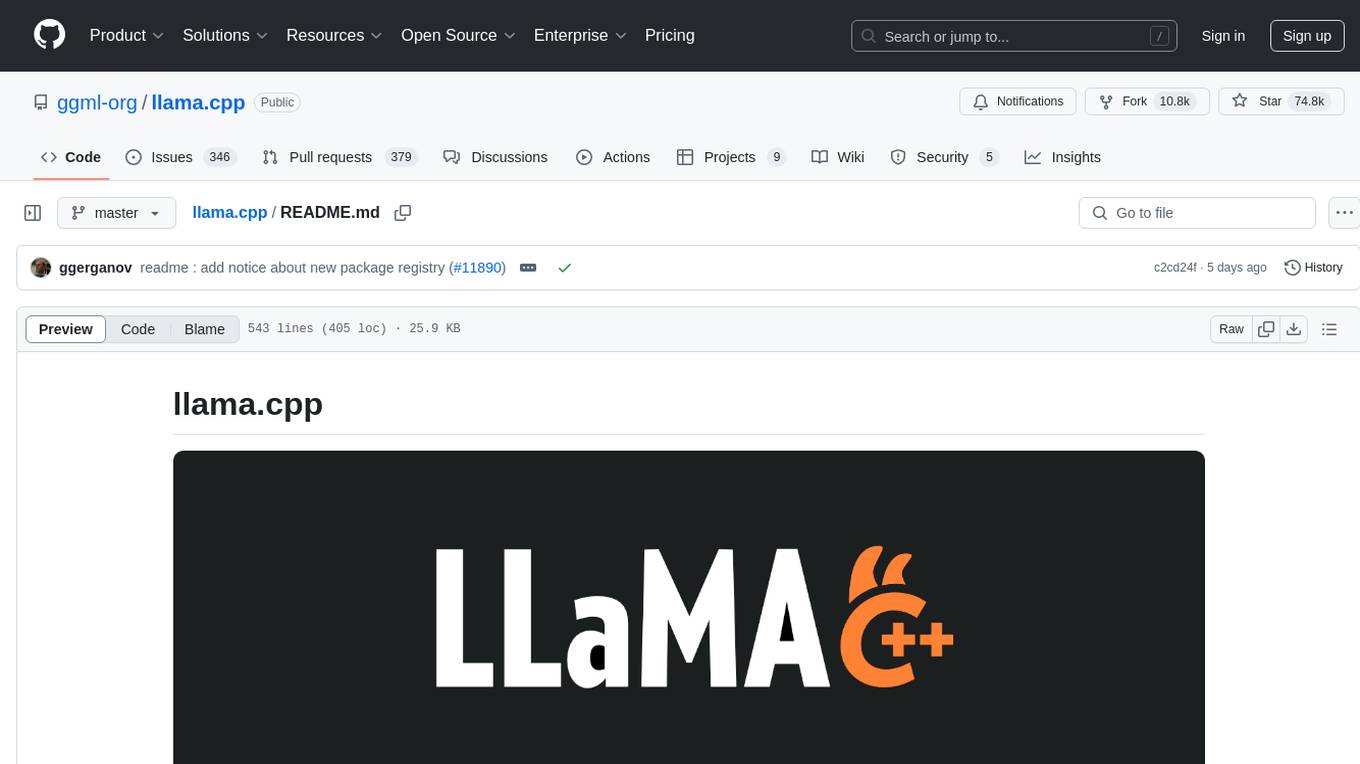
llama.cpp
The main goal of llama.cpp is to enable LLM inference with minimal setup and state-of-the-art performance on a wide range of hardware - locally and in the cloud. It provides a Plain C/C++ implementation without any dependencies, optimized for Apple silicon via ARM NEON, Accelerate and Metal frameworks, and supports various architectures like AVX, AVX2, AVX512, and AMX. It offers integer quantization for faster inference, custom CUDA kernels for NVIDIA GPUs, Vulkan and SYCL backend support, and CPU+GPU hybrid inference. llama.cpp is the main playground for developing new features for the ggml library, supporting various models and providing tools and infrastructure for LLM deployment.
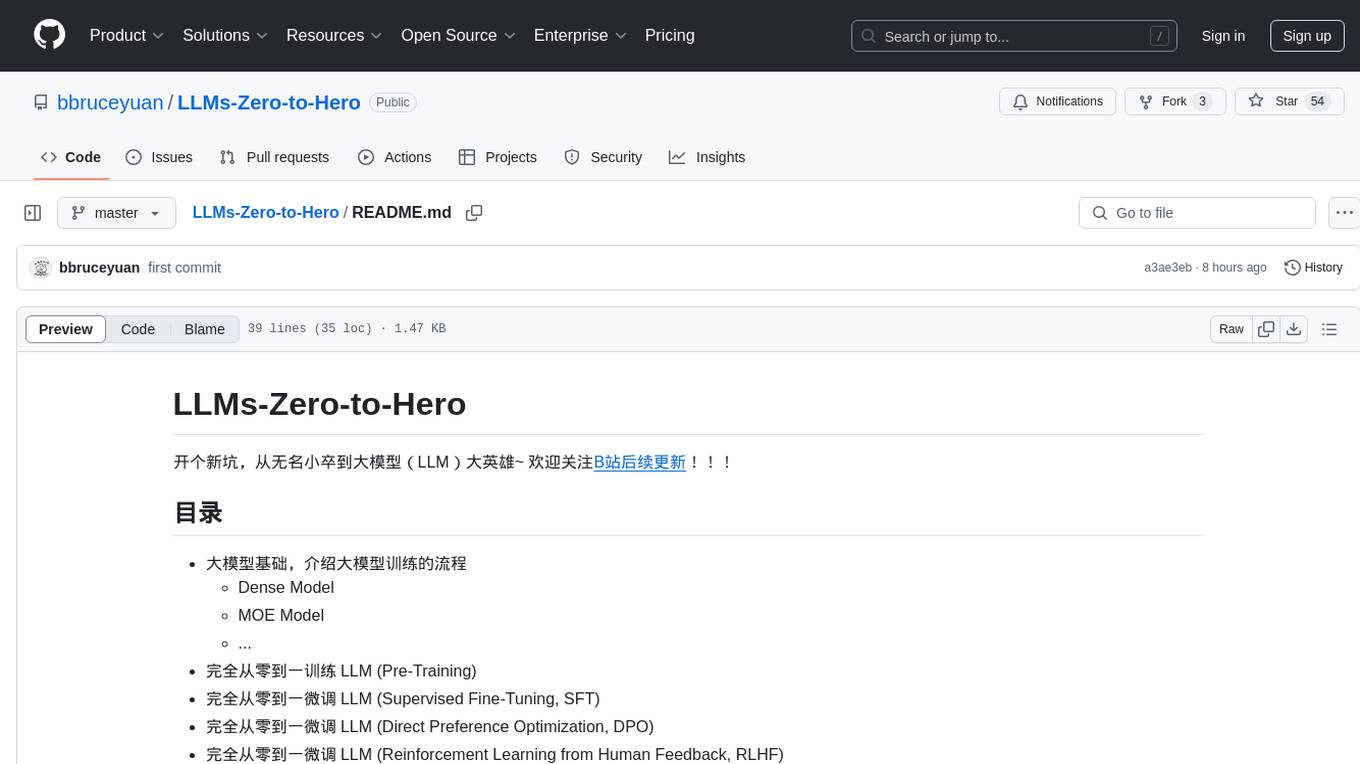
LLMs-Zero-to-Hero
LLMs-Zero-to-Hero is a repository dedicated to training large language models (LLMs) from scratch, covering topics such as dense models, MOE models, pre-training, supervised fine-tuning, direct preference optimization, reinforcement learning from human feedback, and deploying large models. The repository provides detailed learning notes for different chapters, code implementations, and resources for training and deploying LLMs. It aims to guide users from being beginners to proficient in building and deploying large language models.
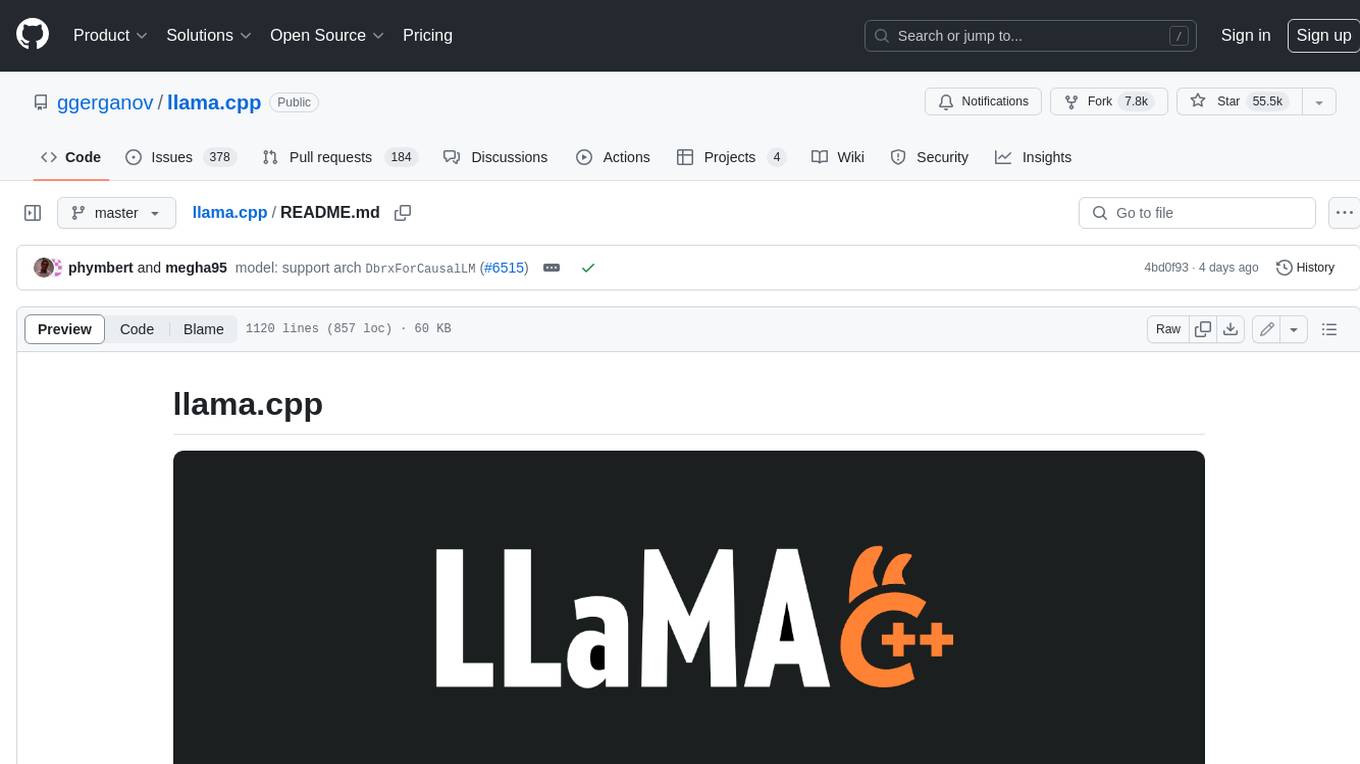
llama.cpp
llama.cpp is a C++ implementation of LLaMA, a large language model from Meta. It provides a command-line interface for inference and can be used for a variety of tasks, including text generation, translation, and question answering. llama.cpp is highly optimized for performance and can be run on a variety of hardware, including CPUs, GPUs, and TPUs.
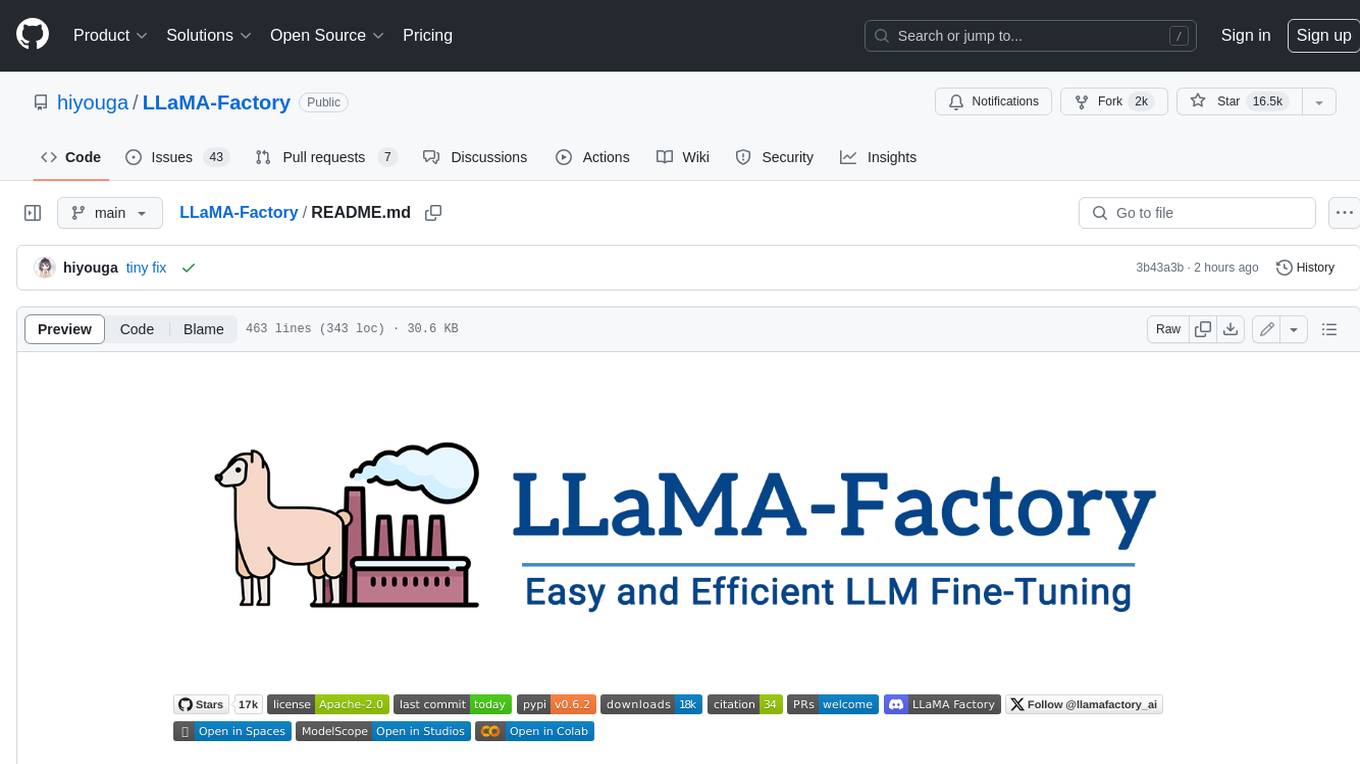
LLaMA-Factory
LLaMA Factory is a unified framework for fine-tuning 100+ large language models (LLMs) with various methods, including pre-training, supervised fine-tuning, reward modeling, PPO, DPO and ORPO. It features integrated algorithms like GaLore, BAdam, DoRA, LongLoRA, LLaMA Pro, LoRA+, LoftQ and Agent tuning, as well as practical tricks like FlashAttention-2, Unsloth, RoPE scaling, NEFTune and rsLoRA. LLaMA Factory provides experiment monitors like LlamaBoard, TensorBoard, Wandb, MLflow, etc., and supports faster inference with OpenAI-style API, Gradio UI and CLI with vLLM worker. Compared to ChatGLM's P-Tuning, LLaMA Factory's LoRA tuning offers up to 3.7 times faster training speed with a better Rouge score on the advertising text generation task. By leveraging 4-bit quantization technique, LLaMA Factory's QLoRA further improves the efficiency regarding the GPU memory.
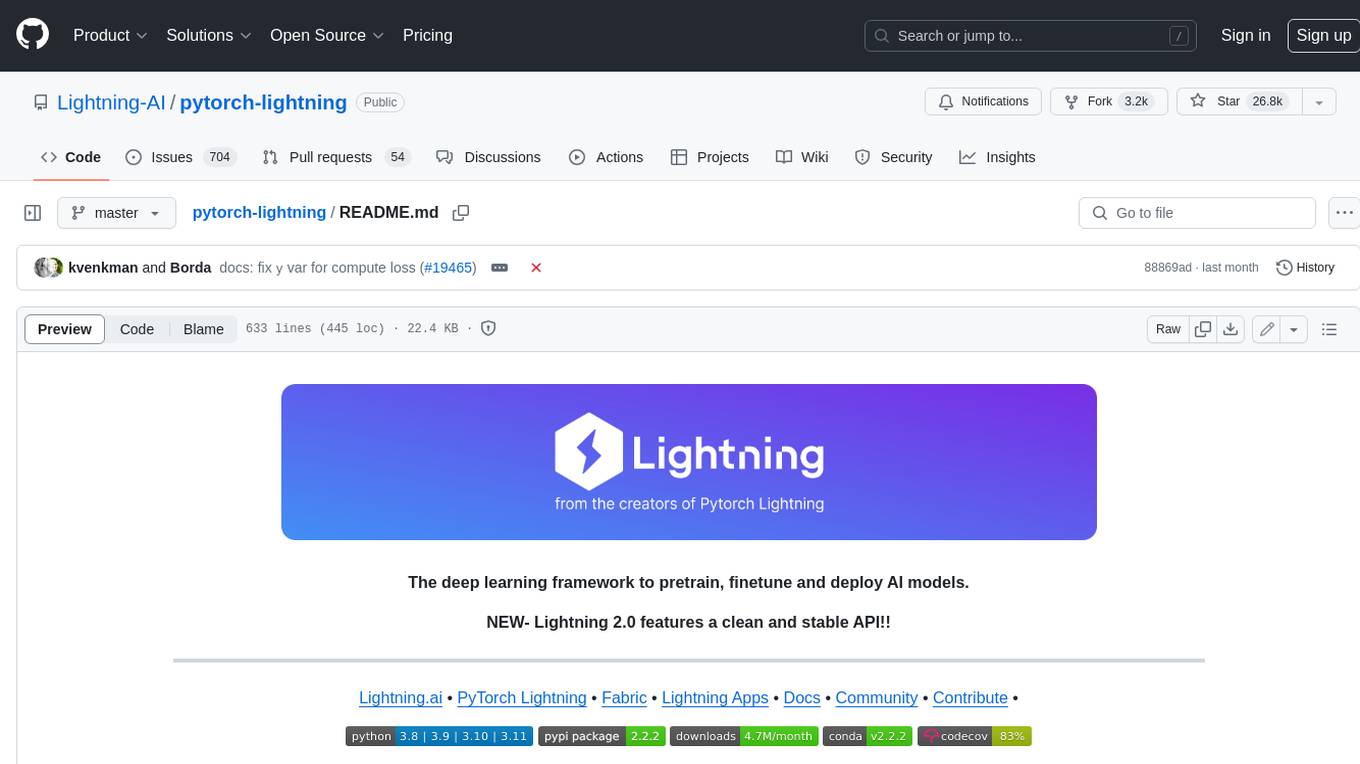
pytorch-lightning
PyTorch Lightning is a framework for training and deploying AI models. It provides a high-level API that abstracts away the low-level details of PyTorch, making it easier to write and maintain complex models. Lightning also includes a number of features that make it easy to train and deploy models on multiple GPUs or TPUs, and to track and visualize training progress. PyTorch Lightning is used by a wide range of organizations, including Google, Facebook, and Microsoft. It is also used by researchers at top universities around the world. Here are some of the benefits of using PyTorch Lightning: * **Increased productivity:** Lightning's high-level API makes it easy to write and maintain complex models. This can save you time and effort, and allow you to focus on the research or business problem you're trying to solve. * **Improved performance:** Lightning's optimized training loops and data loading pipelines can help you train models faster and with better performance. * **Easier deployment:** Lightning makes it easy to deploy models to a variety of platforms, including the cloud, on-premises servers, and mobile devices. * **Better reproducibility:** Lightning's logging and visualization tools make it easy to track and reproduce training results.
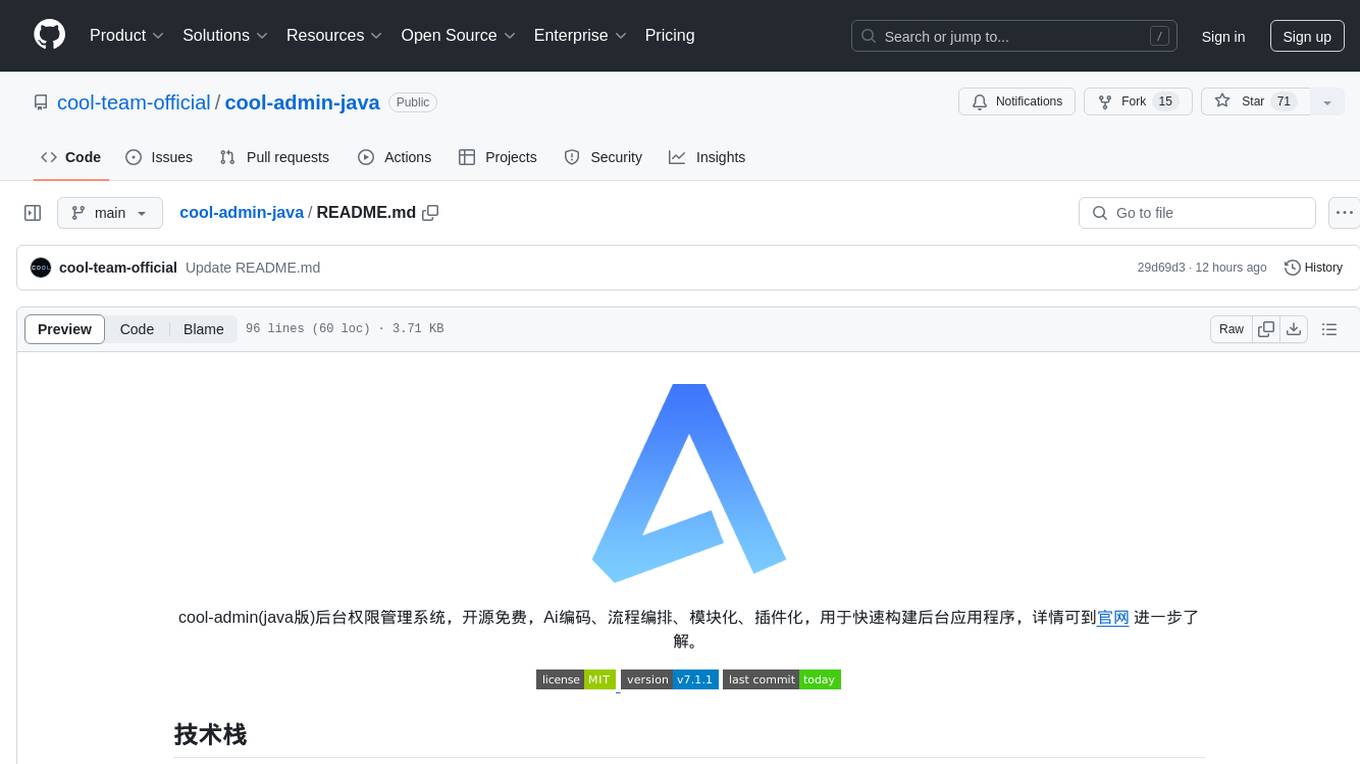
cool-admin-java
Cool-admin-java is an open-source backend permission management system with features like Ai coding, flow arrangement, modularity, and plugin support. It is used to quickly build backend applications. The system offers a modern development experience by providing functionalities such as one-click generation of API interfaces to frontend pages, drag-and-drop flow arrangement, modularized code for easy maintenance, and extensibility through plugin installation for features like payments, SMS, and emails.
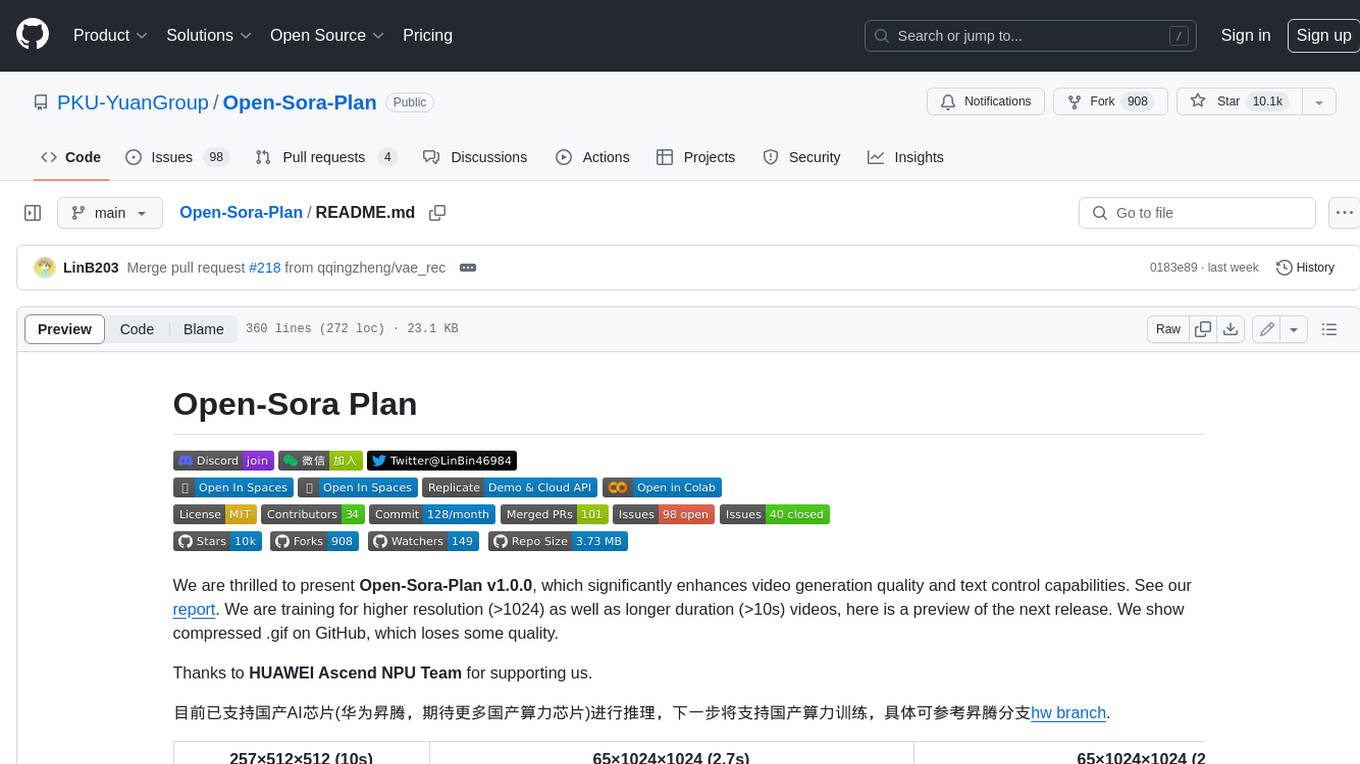
Open-Sora-Plan
Open-Sora-Plan is a project that aims to create a simple and scalable repo to reproduce Sora (OpenAI, but we prefer to call it "ClosedAI"). The project is still in its early stages, but the team is working hard to improve it and make it more accessible to the open-source community. The project is currently focused on training an unconditional model on a landscape dataset, but the team plans to expand the scope of the project in the future to include text2video experiments, training on video2text datasets, and controlling the model with more conditions.
For similar tasks
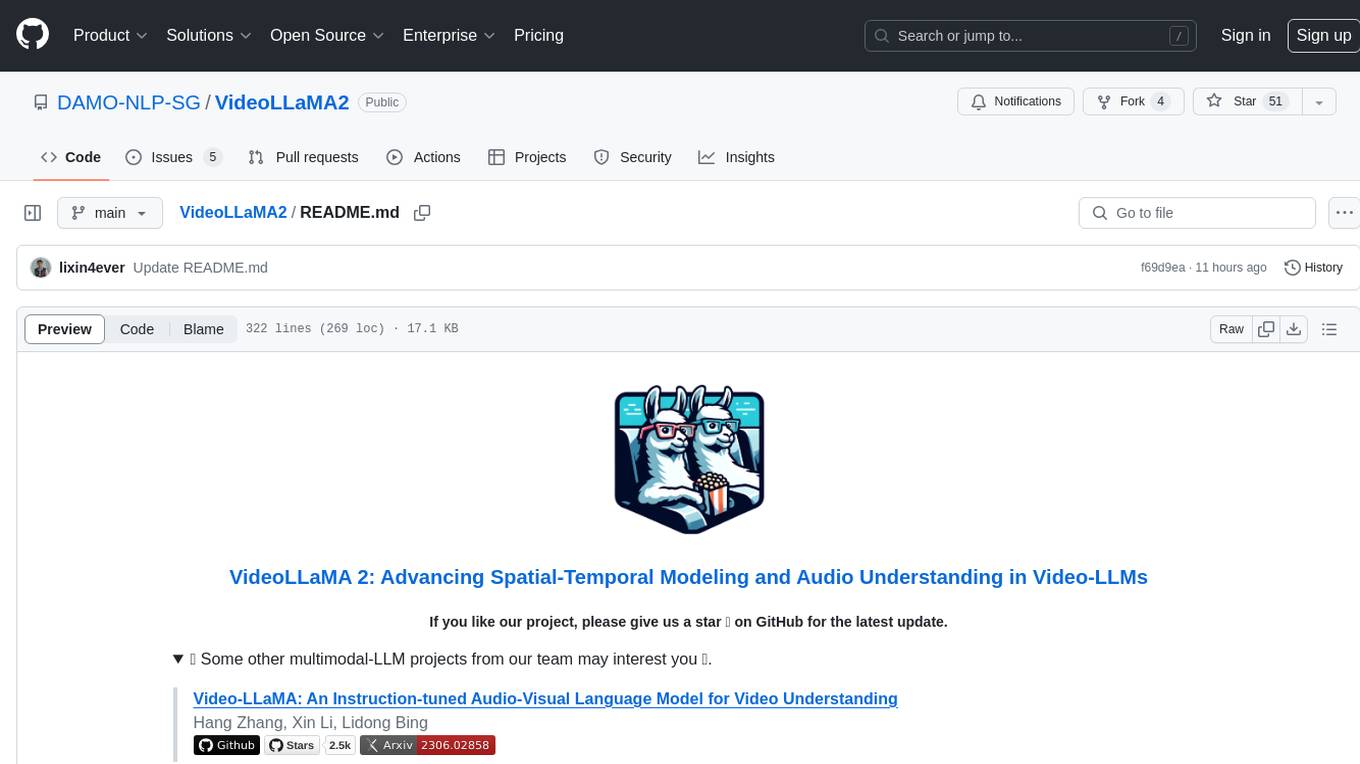
VideoLLaMA2
VideoLLaMA 2 is a project focused on advancing spatial-temporal modeling and audio understanding in video-LLMs. It provides tools for multi-choice video QA, open-ended video QA, and video captioning. The project offers model zoo with different configurations for visual encoder and language decoder. It includes training and evaluation guides, as well as inference capabilities for video and image processing. The project also features a demo setup for running a video-based Large Language Model web demonstration.
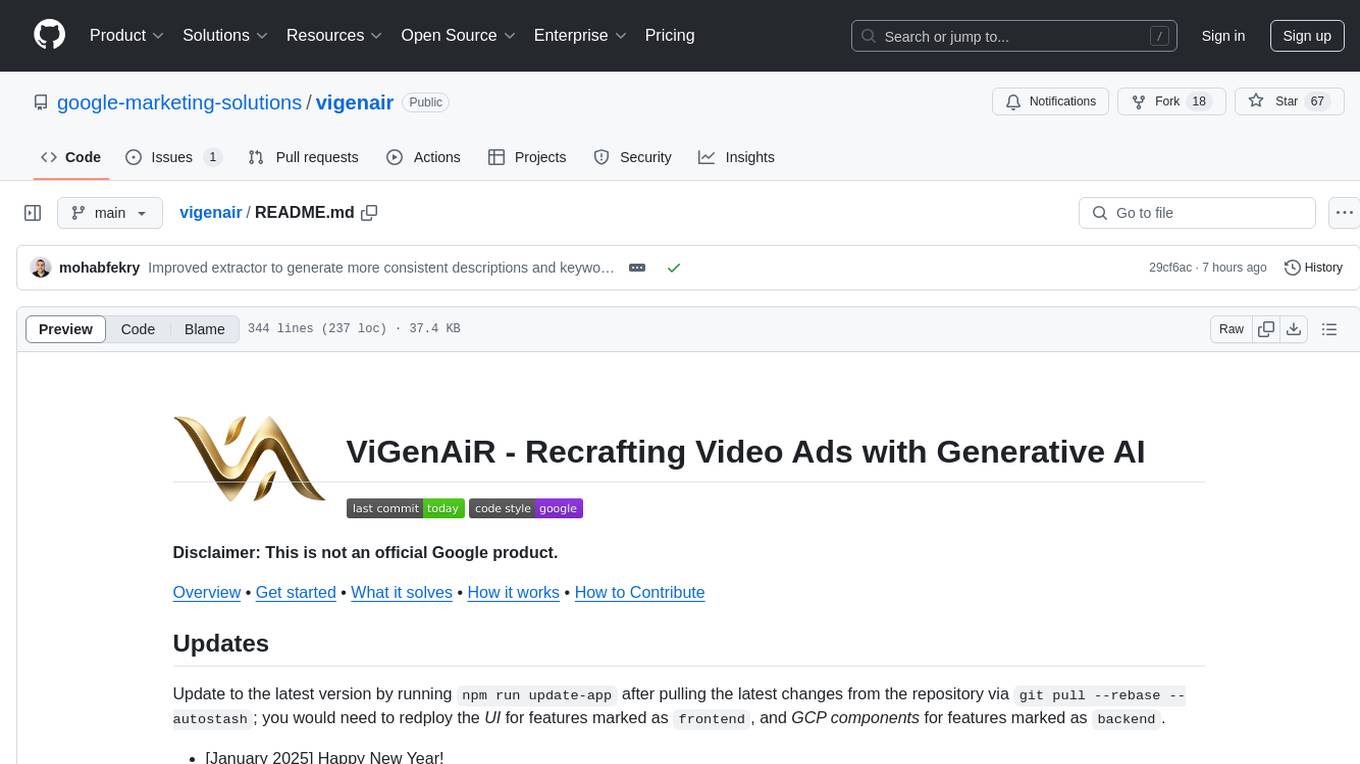
vigenair
ViGenAiR is a tool that harnesses the power of Generative AI models on Google Cloud Platform to automatically transform long-form Video Ads into shorter variants, targeting different audiences. It generates video, image, and text assets for Demand Gen and YouTube video campaigns. Users can steer the model towards generating desired videos, conduct A/B testing, and benefit from various creative features. The tool offers benefits like diverse inventory, compelling video ads, creative excellence, user control, and performance insights. ViGenAiR works by analyzing video content, splitting it into coherent segments, and generating variants following Google's best practices for effective ads.
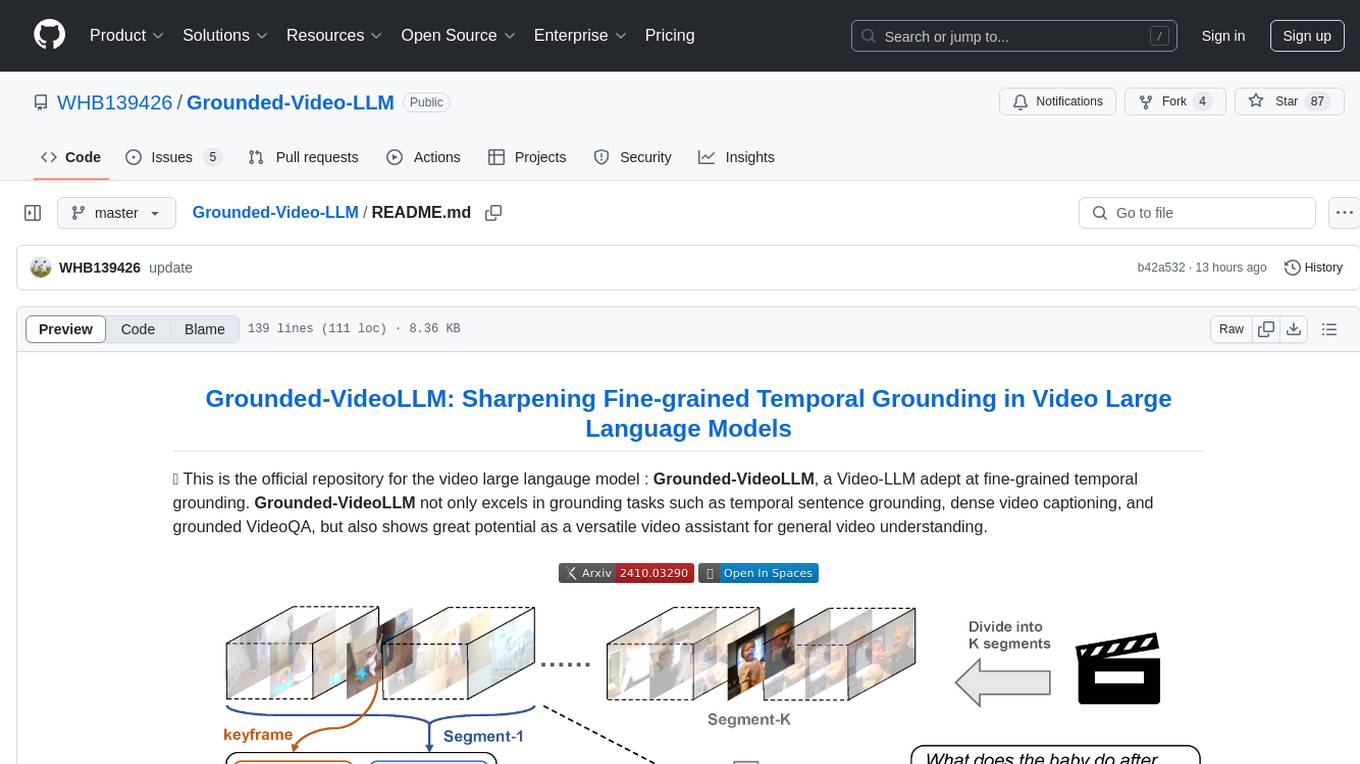
Grounded-Video-LLM
Grounded-VideoLLM is a Video Large Language Model specialized in fine-grained temporal grounding. It excels in tasks such as temporal sentence grounding, dense video captioning, and grounded VideoQA. The model incorporates an additional temporal stream, discrete temporal tokens with specific time knowledge, and a multi-stage training scheme. It shows potential as a versatile video assistant for general video understanding. The repository provides pretrained weights, inference scripts, and datasets for training. Users can run inference queries to get temporal information from videos and train the model from scratch.
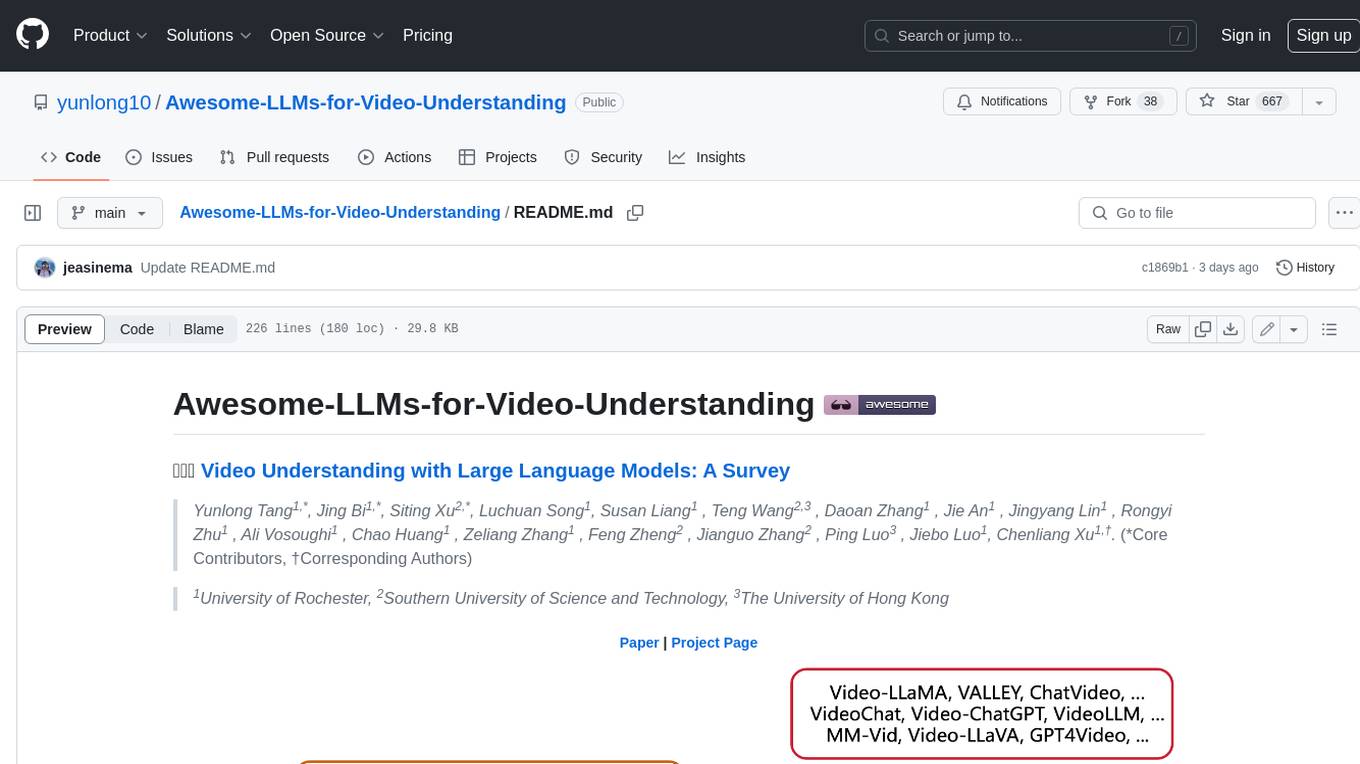
Awesome-LLMs-for-Video-Understanding
Awesome-LLMs-for-Video-Understanding is a repository dedicated to exploring Video Understanding with Large Language Models. It provides a comprehensive survey of the field, covering models, pretraining, instruction tuning, and hybrid methods. The repository also includes information on tasks, datasets, and benchmarks related to video understanding. Contributors are encouraged to add new papers, projects, and materials to enhance the repository.
For similar jobs
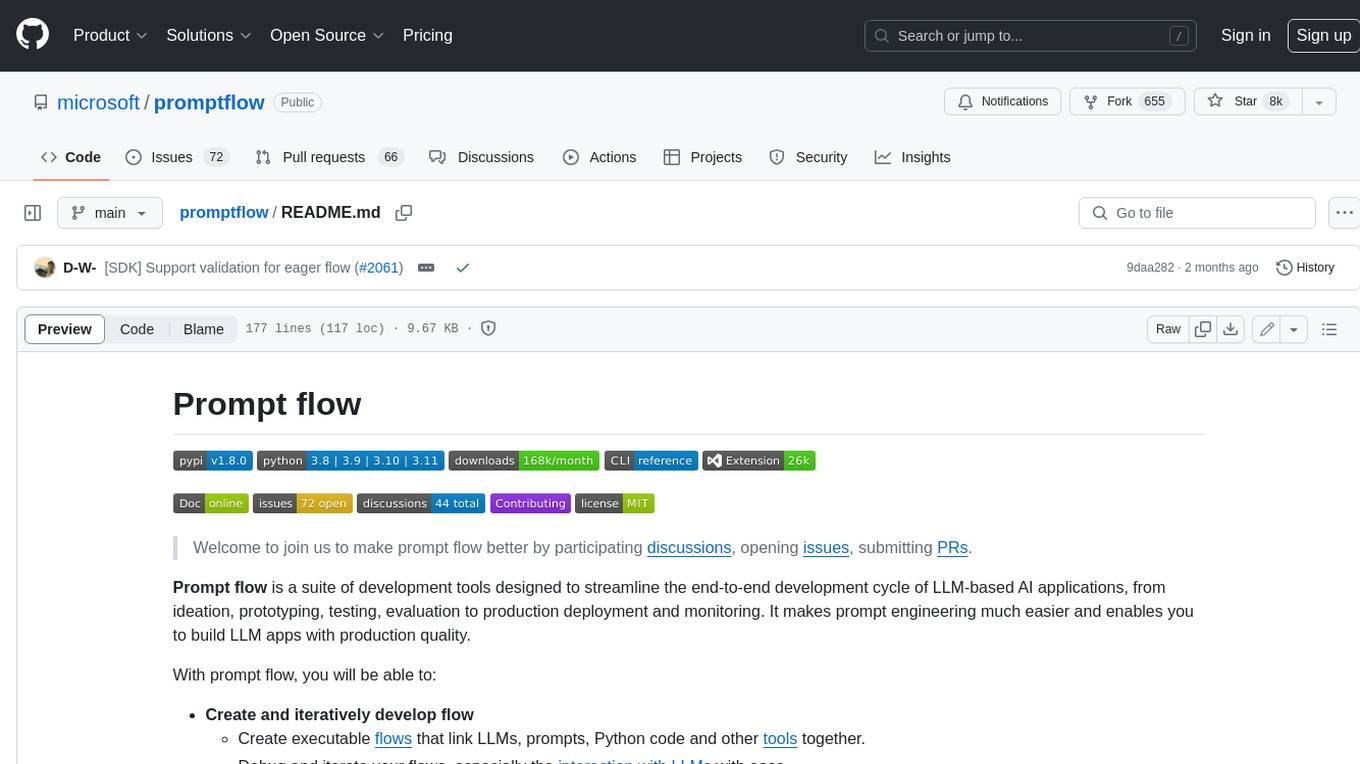
promptflow
**Prompt flow** is a suite of development tools designed to streamline the end-to-end development cycle of LLM-based AI applications, from ideation, prototyping, testing, evaluation to production deployment and monitoring. It makes prompt engineering much easier and enables you to build LLM apps with production quality.
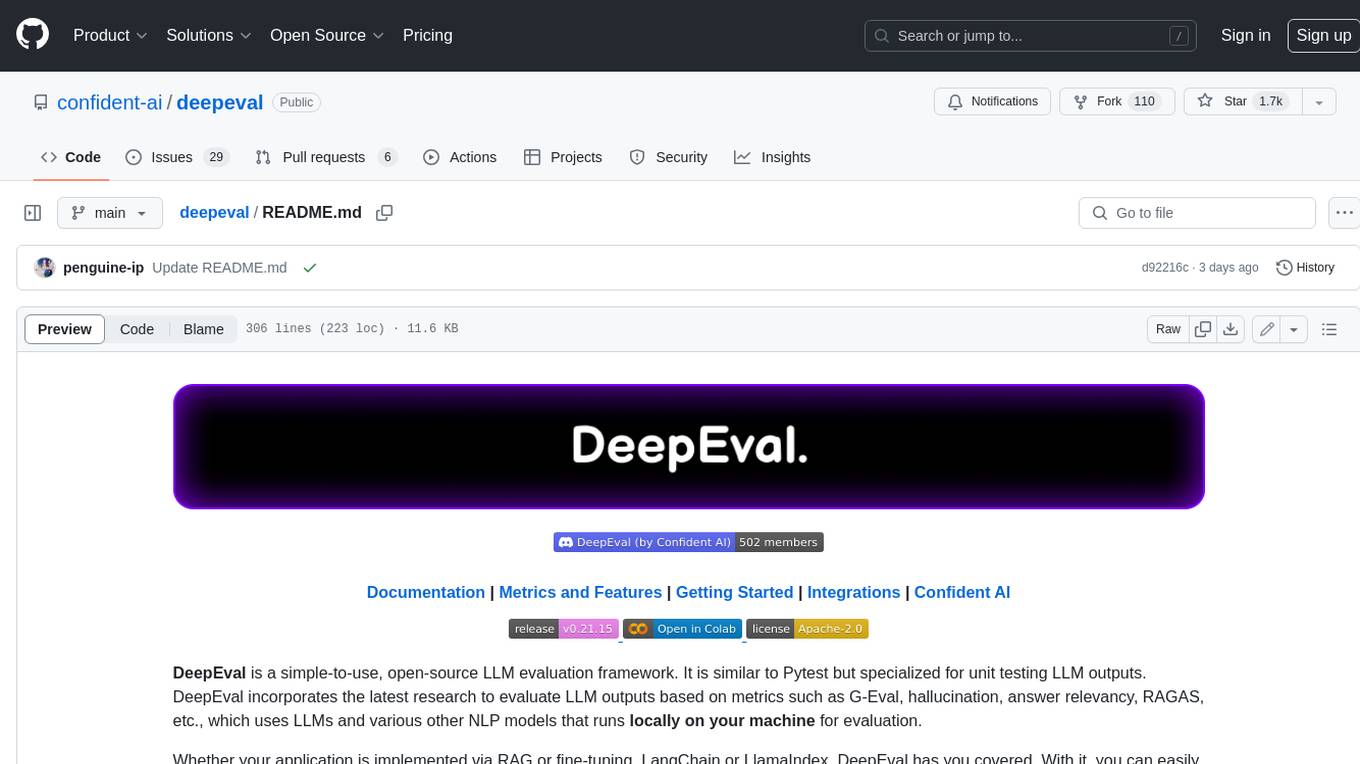
deepeval
DeepEval is a simple-to-use, open-source LLM evaluation framework specialized for unit testing LLM outputs. It incorporates various metrics such as G-Eval, hallucination, answer relevancy, RAGAS, etc., and runs locally on your machine for evaluation. It provides a wide range of ready-to-use evaluation metrics, allows for creating custom metrics, integrates with any CI/CD environment, and enables benchmarking LLMs on popular benchmarks. DeepEval is designed for evaluating RAG and fine-tuning applications, helping users optimize hyperparameters, prevent prompt drifting, and transition from OpenAI to hosting their own Llama2 with confidence.
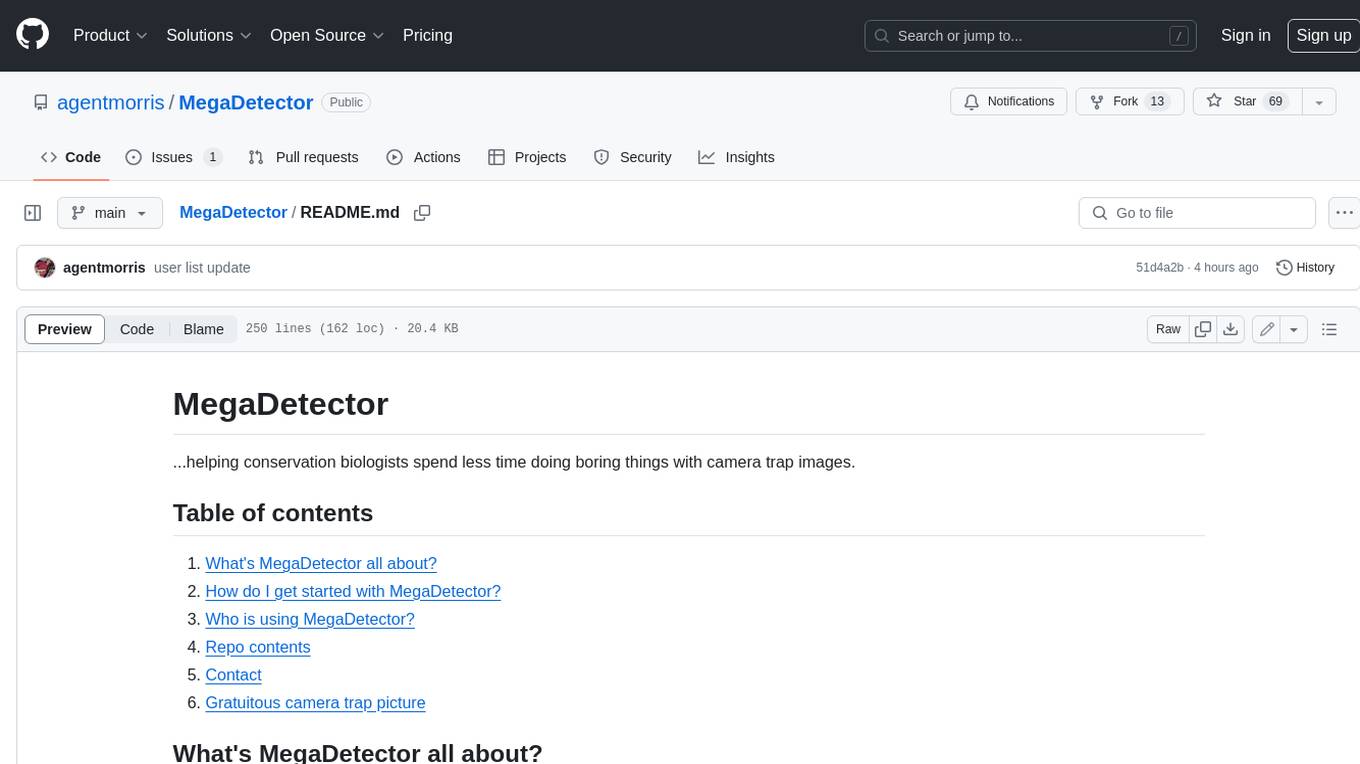
MegaDetector
MegaDetector is an AI model that identifies animals, people, and vehicles in camera trap images (which also makes it useful for eliminating blank images). This model is trained on several million images from a variety of ecosystems. MegaDetector is just one of many tools that aims to make conservation biologists more efficient with AI. If you want to learn about other ways to use AI to accelerate camera trap workflows, check out our of the field, affectionately titled "Everything I know about machine learning and camera traps".
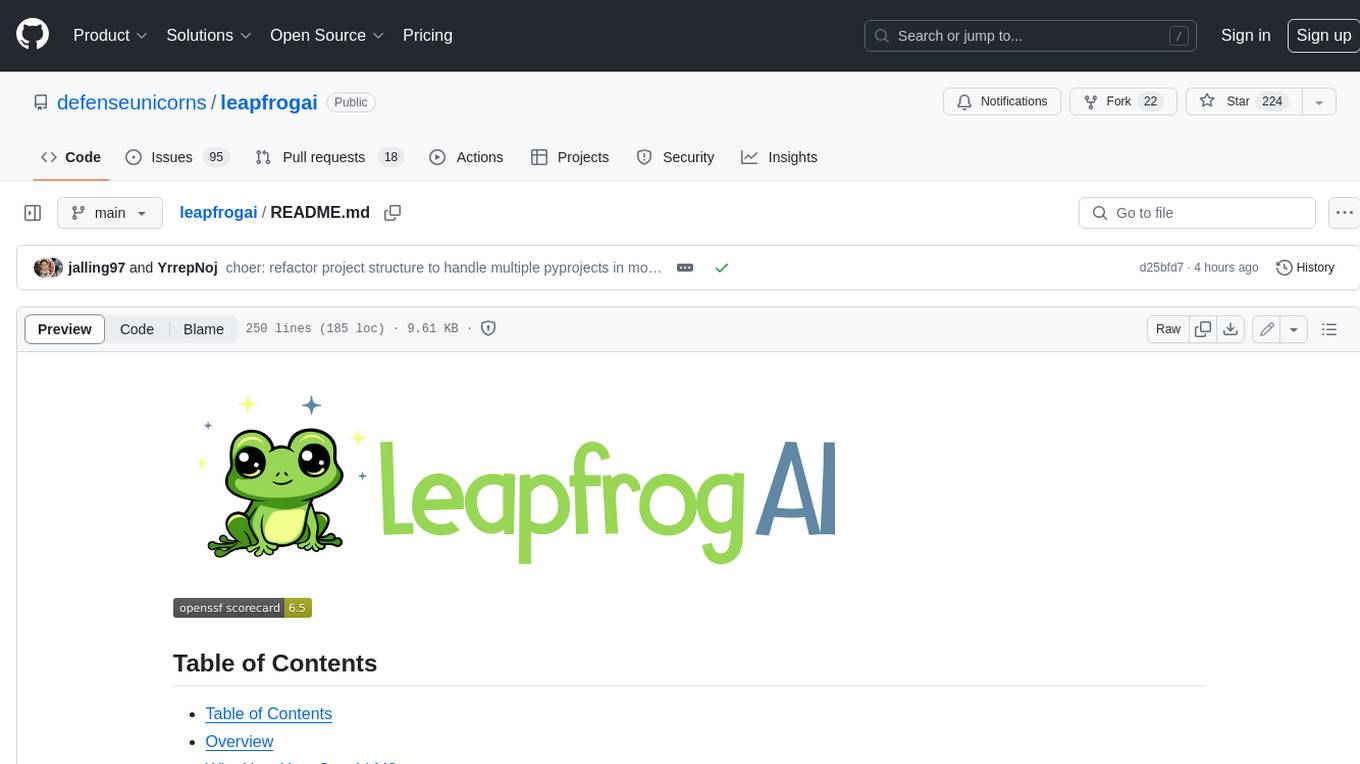
leapfrogai
LeapfrogAI is a self-hosted AI platform designed to be deployed in air-gapped resource-constrained environments. It brings sophisticated AI solutions to these environments by hosting all the necessary components of an AI stack, including vector databases, model backends, API, and UI. LeapfrogAI's API closely matches that of OpenAI, allowing tools built for OpenAI/ChatGPT to function seamlessly with a LeapfrogAI backend. It provides several backends for various use cases, including llama-cpp-python, whisper, text-embeddings, and vllm. LeapfrogAI leverages Chainguard's apko to harden base python images, ensuring the latest supported Python versions are used by the other components of the stack. The LeapfrogAI SDK provides a standard set of protobuffs and python utilities for implementing backends and gRPC. LeapfrogAI offers UI options for common use-cases like chat, summarization, and transcription. It can be deployed and run locally via UDS and Kubernetes, built out using Zarf packages. LeapfrogAI is supported by a community of users and contributors, including Defense Unicorns, Beast Code, Chainguard, Exovera, Hypergiant, Pulze, SOSi, United States Navy, United States Air Force, and United States Space Force.
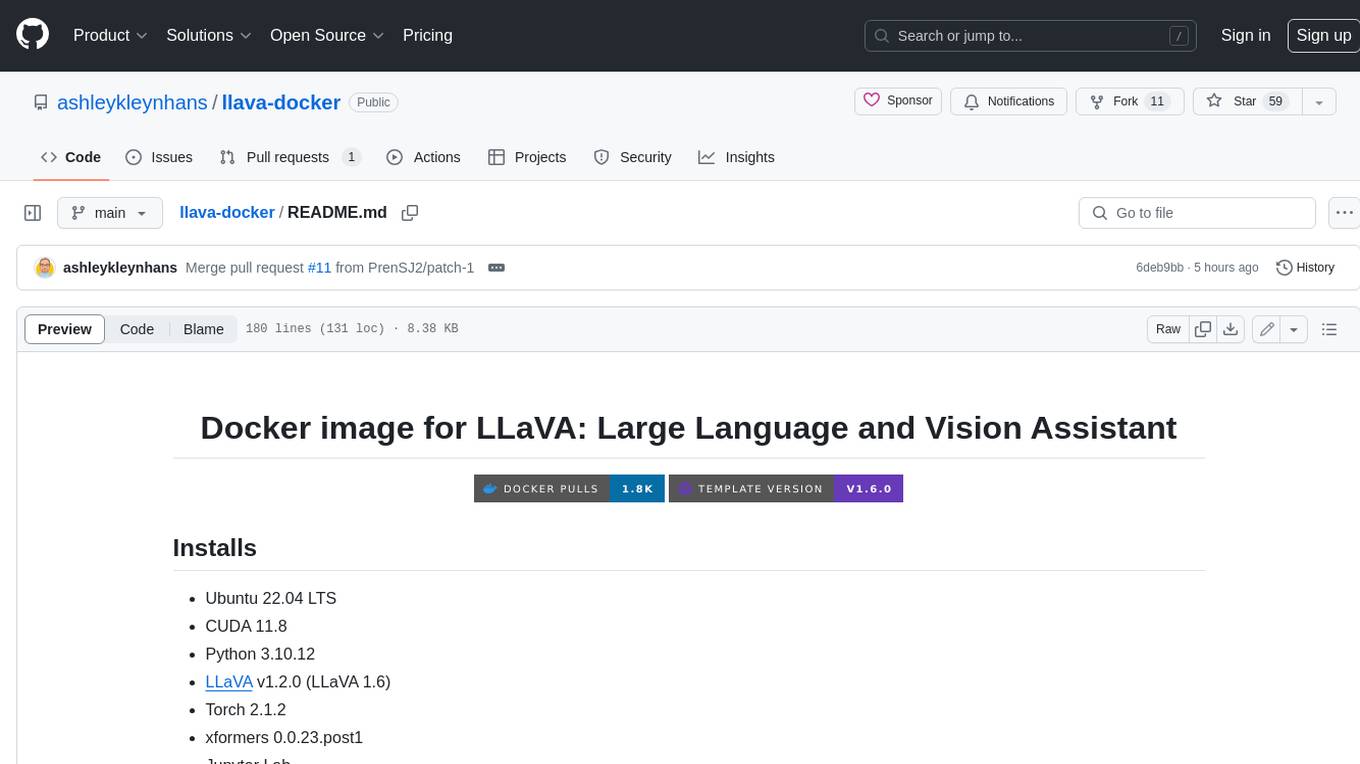
llava-docker
This Docker image for LLaVA (Large Language and Vision Assistant) provides a convenient way to run LLaVA locally or on RunPod. LLaVA is a powerful AI tool that combines natural language processing and computer vision capabilities. With this Docker image, you can easily access LLaVA's functionalities for various tasks, including image captioning, visual question answering, text summarization, and more. The image comes pre-installed with LLaVA v1.2.0, Torch 2.1.2, xformers 0.0.23.post1, and other necessary dependencies. You can customize the model used by setting the MODEL environment variable. The image also includes a Jupyter Lab environment for interactive development and exploration. Overall, this Docker image offers a comprehensive and user-friendly platform for leveraging LLaVA's capabilities.
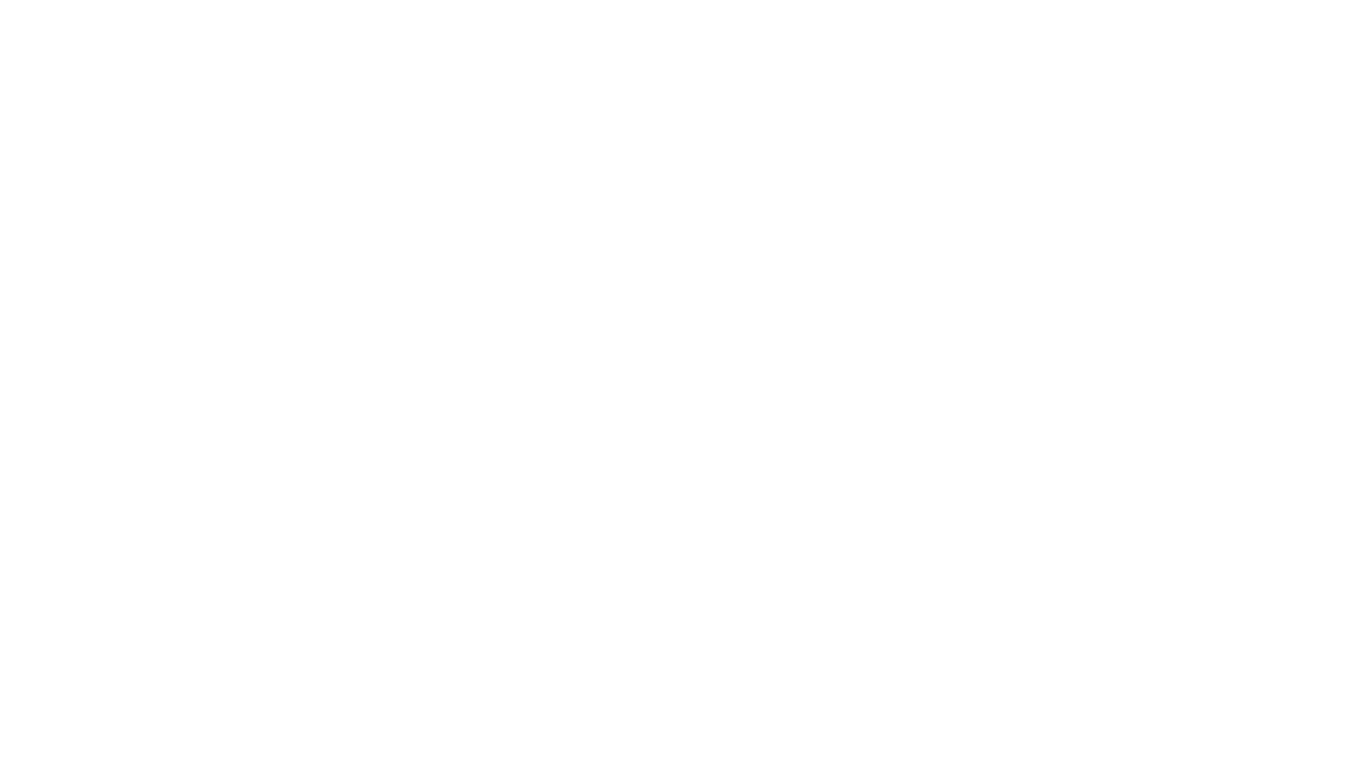
carrot
The 'carrot' repository on GitHub provides a list of free and user-friendly ChatGPT mirror sites for easy access. The repository includes sponsored sites offering various GPT models and services. Users can find and share sites, report errors, and access stable and recommended sites for ChatGPT usage. The repository also includes a detailed list of ChatGPT sites, their features, and accessibility options, making it a valuable resource for ChatGPT users seeking free and unlimited GPT services.
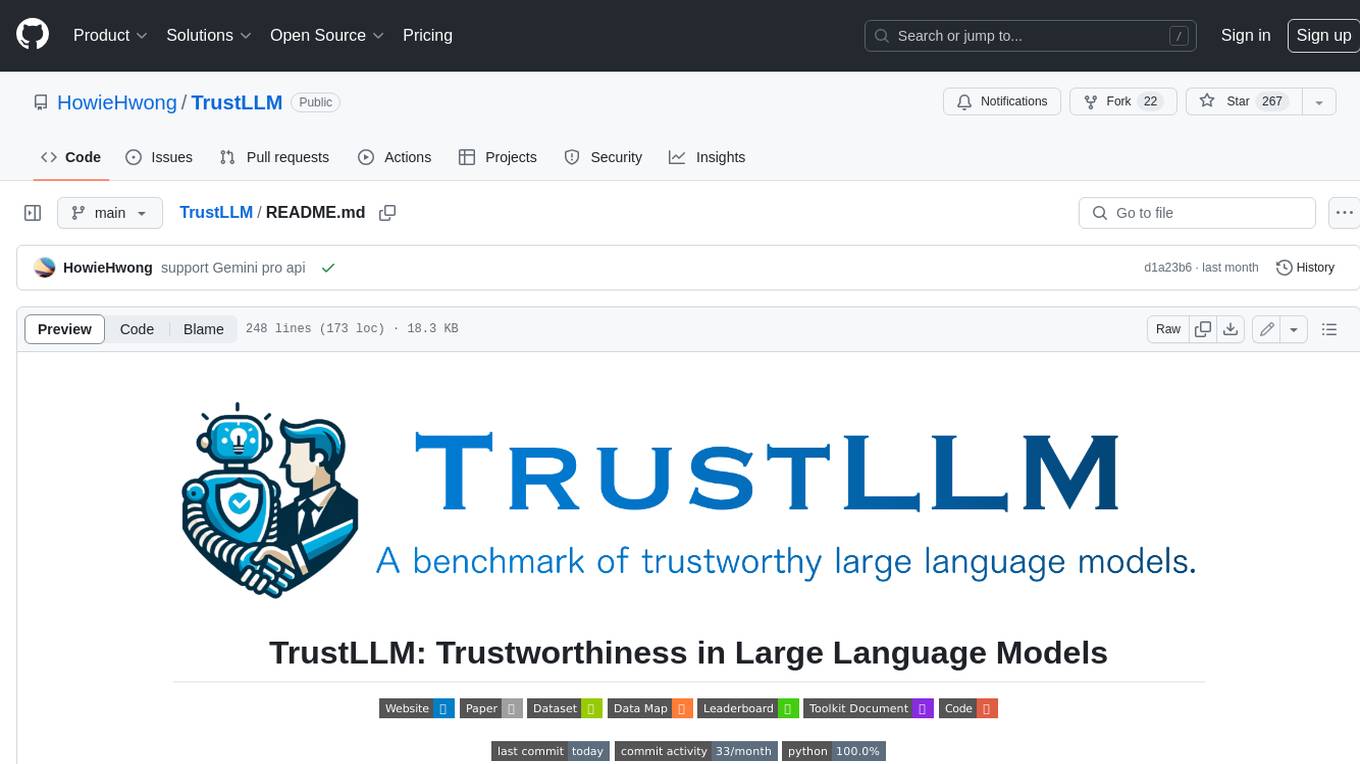
TrustLLM
TrustLLM is a comprehensive study of trustworthiness in LLMs, including principles for different dimensions of trustworthiness, established benchmark, evaluation, and analysis of trustworthiness for mainstream LLMs, and discussion of open challenges and future directions. Specifically, we first propose a set of principles for trustworthy LLMs that span eight different dimensions. Based on these principles, we further establish a benchmark across six dimensions including truthfulness, safety, fairness, robustness, privacy, and machine ethics. We then present a study evaluating 16 mainstream LLMs in TrustLLM, consisting of over 30 datasets. The document explains how to use the trustllm python package to help you assess the performance of your LLM in trustworthiness more quickly. For more details about TrustLLM, please refer to project website.
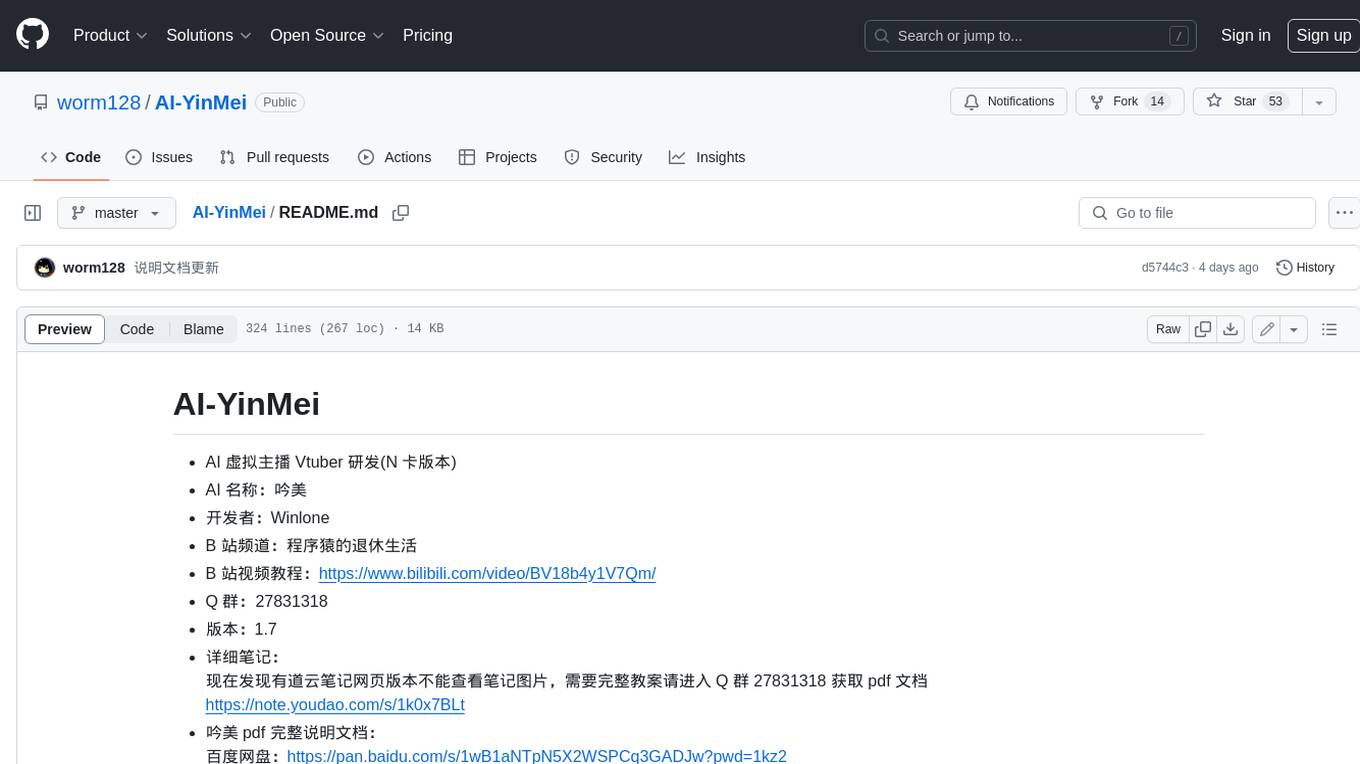
AI-YinMei
AI-YinMei is an AI virtual anchor Vtuber development tool (N card version). It supports fastgpt knowledge base chat dialogue, a complete set of solutions for LLM large language models: [fastgpt] + [one-api] + [Xinference], supports docking bilibili live broadcast barrage reply and entering live broadcast welcome speech, supports Microsoft edge-tts speech synthesis, supports Bert-VITS2 speech synthesis, supports GPT-SoVITS speech synthesis, supports expression control Vtuber Studio, supports painting stable-diffusion-webui output OBS live broadcast room, supports painting picture pornography public-NSFW-y-distinguish, supports search and image search service duckduckgo (requires magic Internet access), supports image search service Baidu image search (no magic Internet access), supports AI reply chat box [html plug-in], supports AI singing Auto-Convert-Music, supports playlist [html plug-in], supports dancing function, supports expression video playback, supports head touching action, supports gift smashing action, supports singing automatic start dancing function, chat and singing automatic cycle swing action, supports multi scene switching, background music switching, day and night automatic switching scene, supports open singing and painting, let AI automatically judge the content.