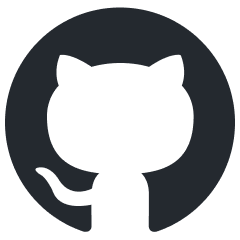
phoenix
AI Observability & Evaluation
Stars: 5270
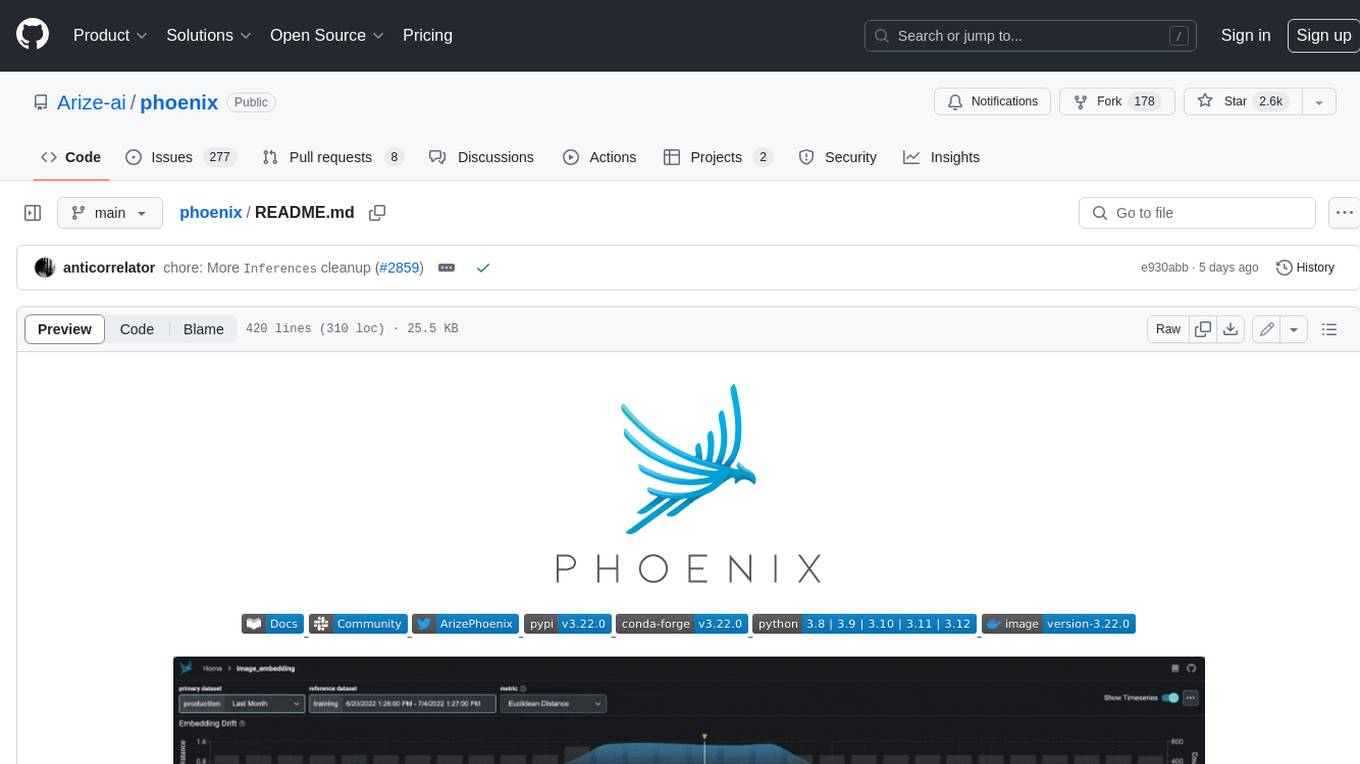
Phoenix is a tool that provides MLOps and LLMOps insights at lightning speed with zero-config observability. It offers a notebook-first experience for monitoring models and LLM Applications by providing LLM Traces, LLM Evals, Embedding Analysis, RAG Analysis, and Structured Data Analysis. Users can trace through the execution of LLM Applications, evaluate generative models, explore embedding point-clouds, visualize generative application's search and retrieval process, and statistically analyze structured data. Phoenix is designed to help users troubleshoot problems related to retrieval, tool execution, relevance, toxicity, drift, and performance degradation.
README:
Phoenix is an open-source AI observability platform designed for experimentation, evaluation, and troubleshooting. It provides:
- Tracing - Trace your LLM application's runtime using OpenTelemetry-based instrumentation.
- Evaluation - Leverage LLMs to benchmark your application's performance using response and retrieval evals.
- Datasets - Create versioned datasets of examples for experimentation, evaluation, and fine-tuning.
- Experiments - Track and evaluate changes to prompts, LLMs, and retrieval.
- Playground- Optimize prompts, compare models, adjust parameters, and replay traced LLM calls.
- Prompt Mangement- Manage and test prompt changes systematically using version control, tagging, and experimentation.
Phoenix is vendor and language agnostic with out-of-the-box support for popular frameworks (π¦LlamaIndex, π¦βLangChain, Haystack, π§©DSPy, π€smolagents) and LLM providers (OpenAI, Bedrock, MistralAI, VertexAI, LiteLLM, and more). For details on auto-instrumentation, check out the OpenInference project.
Phoenix runs practically anywhere, including your local machine, a Jupyter notebook, a containerized deployment, or in the cloud.
Install Phoenix via pip
or conda
pip install arize-phoenix
Phoenix container images are available via Docker Hub and can be deployed using Docker or Kubernetes.
Phoenix is built on top of OpenTelemetry and is vendor, language, and framework agnostic. For details about tracing integrations and example applications, see the OpenInference project.
Python Integrations
Integration | Package | Version Badge |
---|---|---|
OpenAI | openinference-instrumentation-openai |
|
OpenAI Agents | openinference-instrumentation-openai-agents |
|
LlamaIndex | openinference-instrumentation-llama-index |
|
DSPy | openinference-instrumentation-dspy |
|
AWS Bedrock | openinference-instrumentation-bedrock |
|
LangChain | openinference-instrumentation-langchain |
|
MistralAI | openinference-instrumentation-mistralai |
|
Guardrails | openinference-instrumentation-guardrails |
|
VertexAI | openinference-instrumentation-vertexai |
|
CrewAI | openinference-instrumentation-crewai |
|
Haystack | openinference-instrumentation-haystack |
|
LiteLLM | openinference-instrumentation-litellm |
|
Groq | openinference-instrumentation-groq |
|
Instructor | openinference-instrumentation-instructor |
|
Anthropic | openinference-instrumentation-anthropic |
|
Smolagents | openinference-instrumentation-smolagents |
Integration | Package | Version Badge |
---|---|---|
OpenAI | @arizeai/openinference-instrumentation-openai |
|
LangChain.js | @arizeai/openinference-instrumentation-langchain |
|
Vercel AI SDK | @arizeai/openinference-vercel |
|
BeeAI | @arizeai/openinference-instrumentation-beeai |
Phoenix has native integrations with LangFlow, LiteLLM Proxy, and BeeAI.
Join our community to connect with thousands of AI builders.
- π Join our Slack community.
- π Read our documentation.
- π‘ Ask questions and provide feedback in the #phoenix-support channel.
- π Leave a star on our GitHub.
- π Report bugs with GitHub Issues.
- π Follow us on π.
- πΊοΈ Check out our roadmap to see where we're heading next.
See the migration guide for a list of breaking changes.
Copyright 2025 Arize AI, Inc. All Rights Reserved.
Portions of this code are patent protected by one or more U.S. Patents. See the IP_NOTICE.
This software is licensed under the terms of the Elastic License 2.0 (ELv2). See LICENSE.
For Tasks:
Click tags to check more tools for each tasksFor Jobs:
Alternative AI tools for phoenix
Similar Open Source Tools
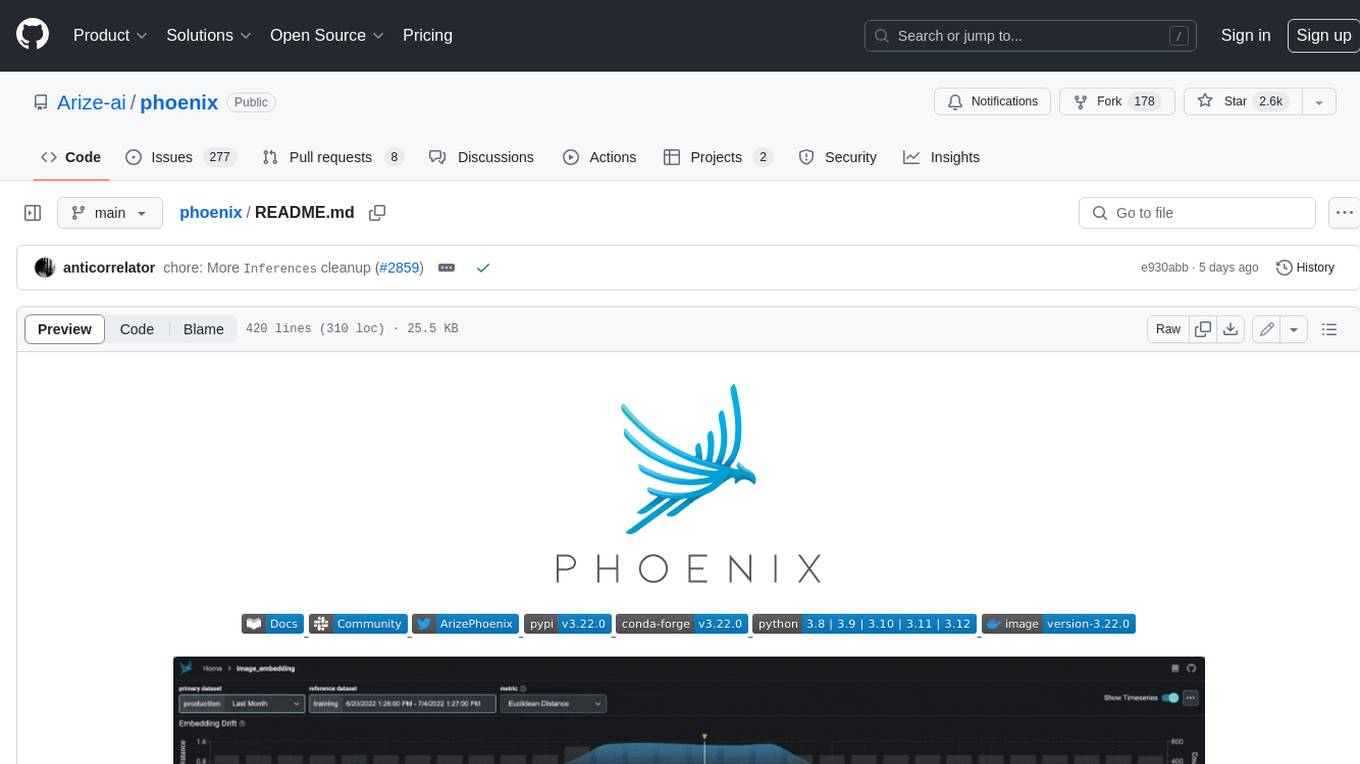
phoenix
Phoenix is a tool that provides MLOps and LLMOps insights at lightning speed with zero-config observability. It offers a notebook-first experience for monitoring models and LLM Applications by providing LLM Traces, LLM Evals, Embedding Analysis, RAG Analysis, and Structured Data Analysis. Users can trace through the execution of LLM Applications, evaluate generative models, explore embedding point-clouds, visualize generative application's search and retrieval process, and statistically analyze structured data. Phoenix is designed to help users troubleshoot problems related to retrieval, tool execution, relevance, toxicity, drift, and performance degradation.
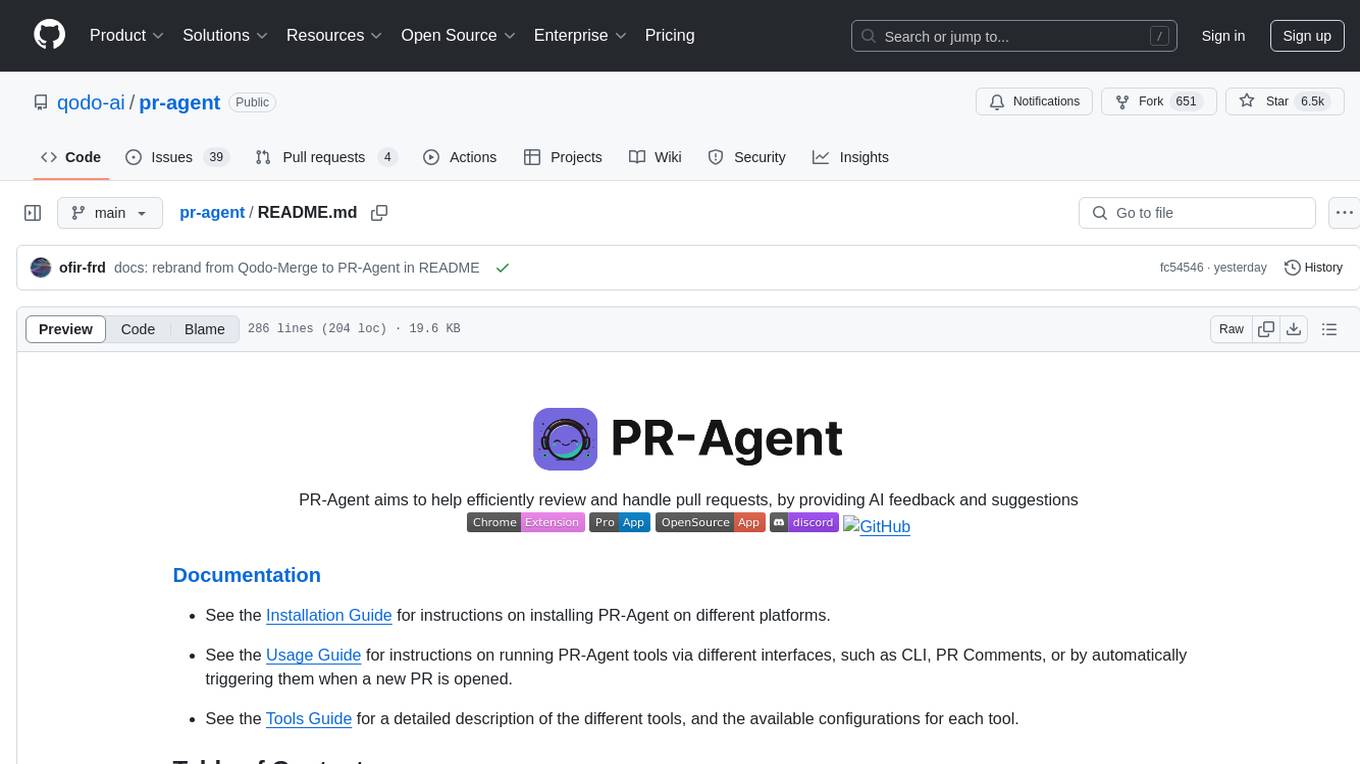
pr-agent
PR-Agent is a tool designed to assist in efficiently reviewing and handling pull requests by providing AI feedback and suggestions. It offers various tools such as Review, Describe, Improve, Ask, Update CHANGELOG, and more, with the ability to run them via different interfaces like CLI, PR Comments, or automatically triggering them when a new PR is opened. The tool supports multiple git platforms and models, emphasizing real-life practical usage and modular, customizable tools.
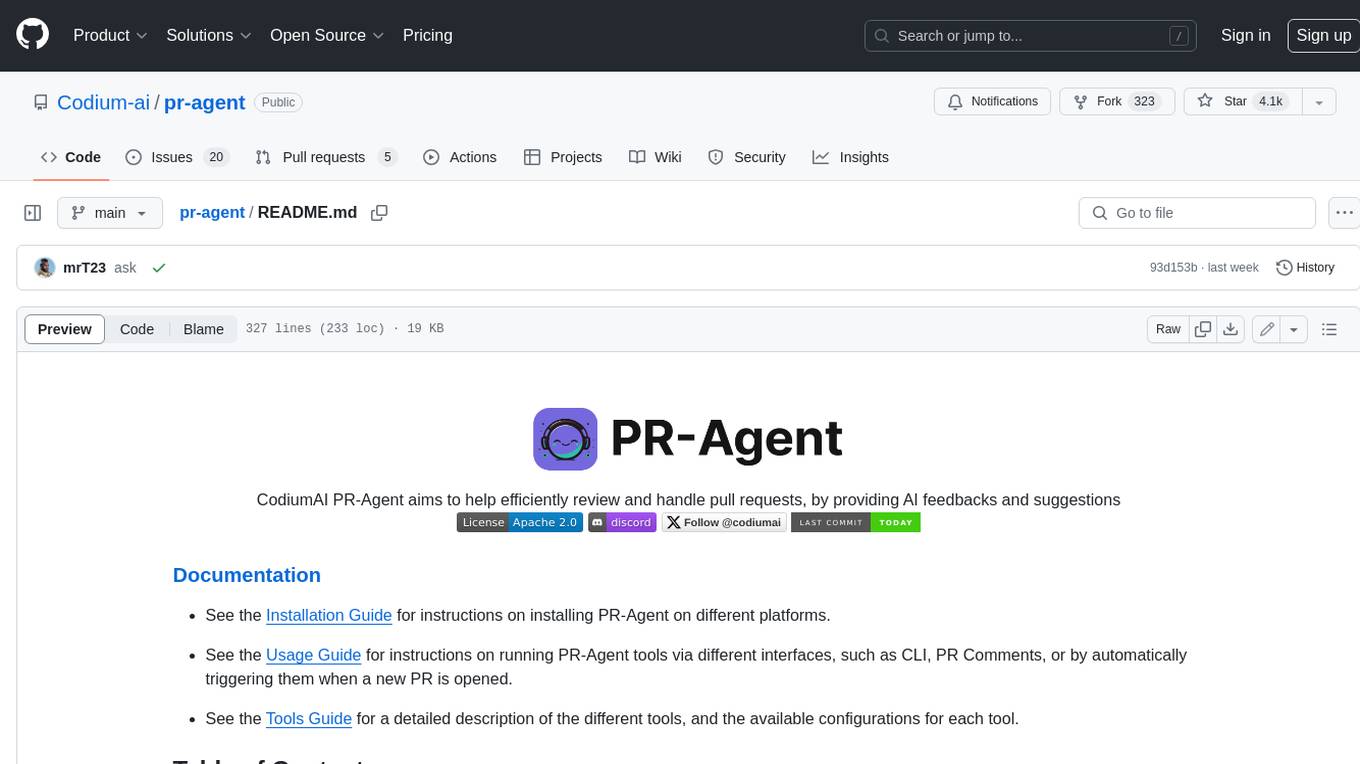
pr-agent
PR-Agent is a tool that helps to efficiently review and handle pull requests by providing AI feedbacks and suggestions. It supports various commands such as generating PR descriptions, providing code suggestions, answering questions about the PR, and updating the CHANGELOG.md file. PR-Agent can be used via CLI, GitHub Action, GitHub App, Docker, and supports multiple git providers and models. It emphasizes real-life practical usage, with each tool having a single GPT-4 call for quick and affordable responses. The PR Compression strategy enables effective handling of both short and long PRs, while the JSON prompting strategy allows for modular and customizable tools. PR-Agent Pro, the hosted version by CodiumAI, provides additional benefits such as full management, improved privacy, priority support, and extra features.
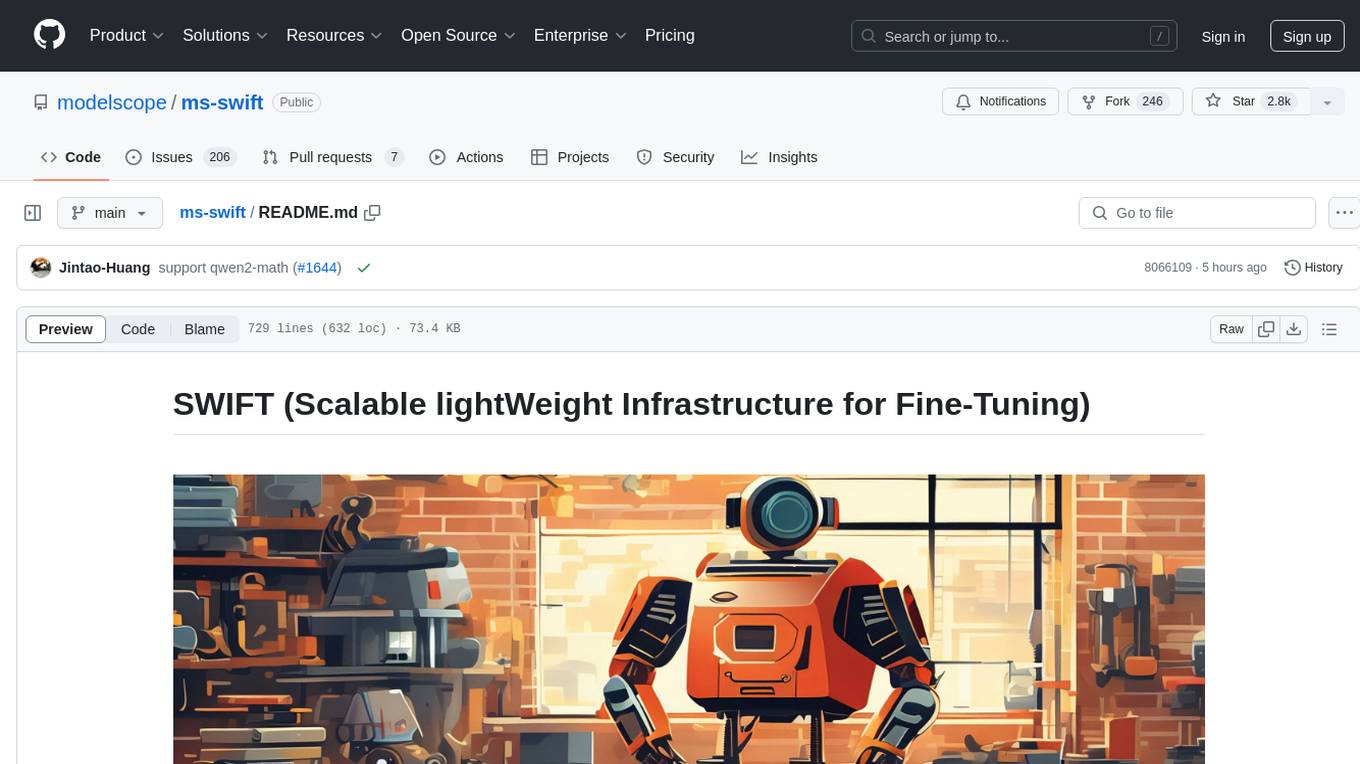
ms-swift
ms-swift is an official framework provided by the ModelScope community for fine-tuning and deploying large language models and multi-modal large models. It supports training, inference, evaluation, quantization, and deployment of over 400 large models and 100+ multi-modal large models. The framework includes various training technologies and accelerates inference, evaluation, and deployment modules. It offers a Gradio-based Web-UI interface and best practices for easy application of large models. ms-swift supports a wide range of model types, dataset types, hardware support, lightweight training methods, distributed training techniques, quantization training, RLHF training, multi-modal training, interface training, plugin and extension support, inference acceleration engines, model evaluation, and model quantization.
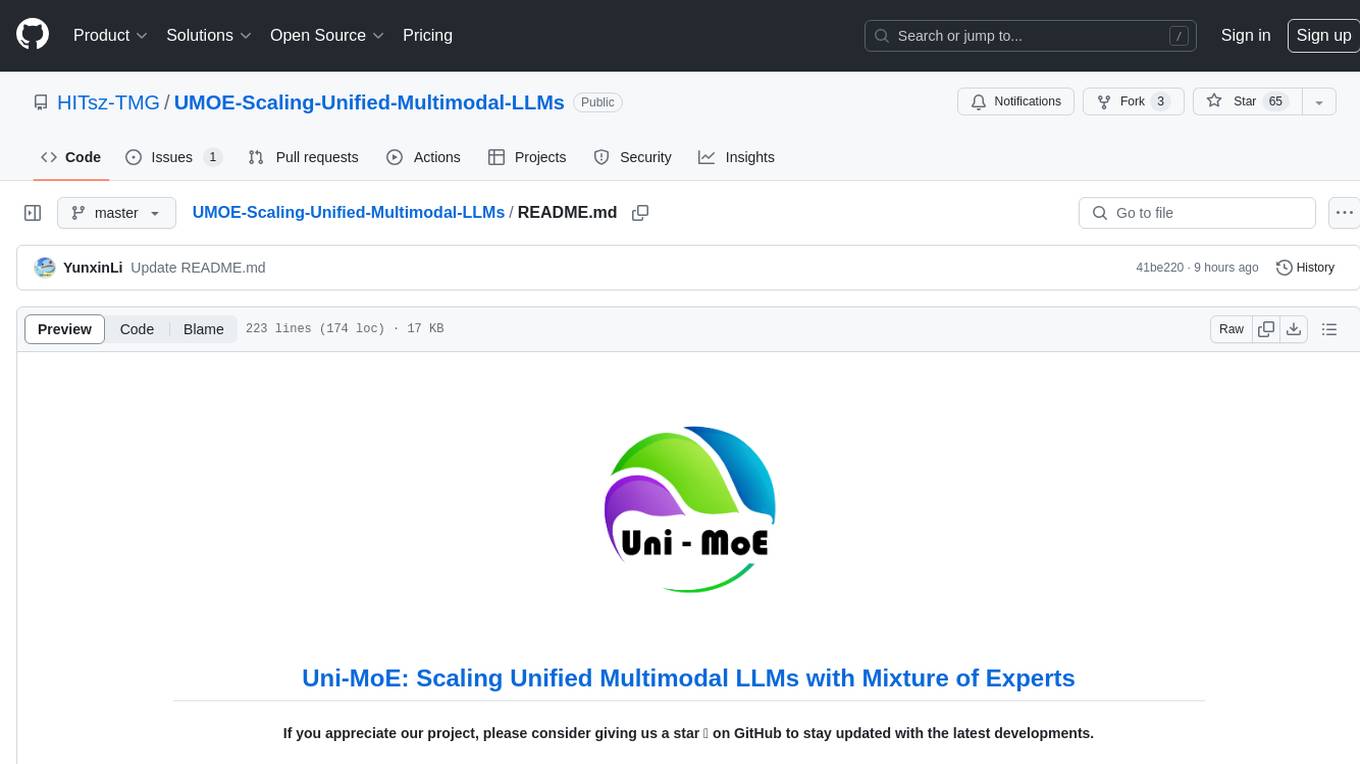
UMOE-Scaling-Unified-Multimodal-LLMs
Uni-MoE is a MoE-based unified multimodal model that can handle diverse modalities including audio, speech, image, text, and video. The project focuses on scaling Unified Multimodal LLMs with a Mixture of Experts framework. It offers enhanced functionality for training across multiple nodes and GPUs, as well as parallel processing at both the expert and modality levels. The model architecture involves three training stages: building connectors for multimodal understanding, developing modality-specific experts, and incorporating multiple trained experts into LLMs using the LoRA technique on mixed multimodal data. The tool provides instructions for installation, weights organization, inference, training, and evaluation on various datasets.
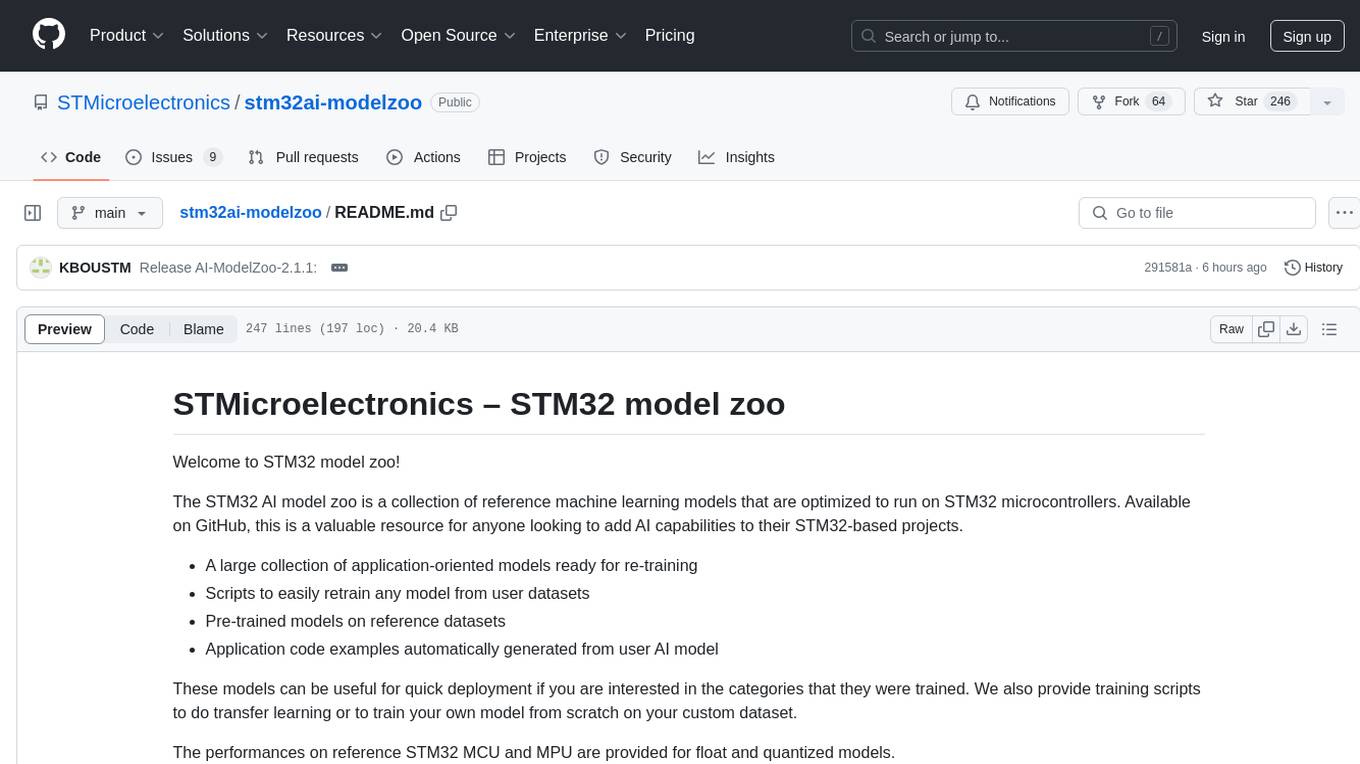
stm32ai-modelzoo
The STM32 AI model zoo is a collection of reference machine learning models optimized to run on STM32 microcontrollers. It provides a large collection of application-oriented models ready for re-training, scripts for easy retraining from user datasets, pre-trained models on reference datasets, and application code examples generated from user AI models. The project offers training scripts for transfer learning or training custom models from scratch. It includes performances on reference STM32 MCU and MPU for float and quantized models. The project is organized by application, providing step-by-step guides for training and deploying models.
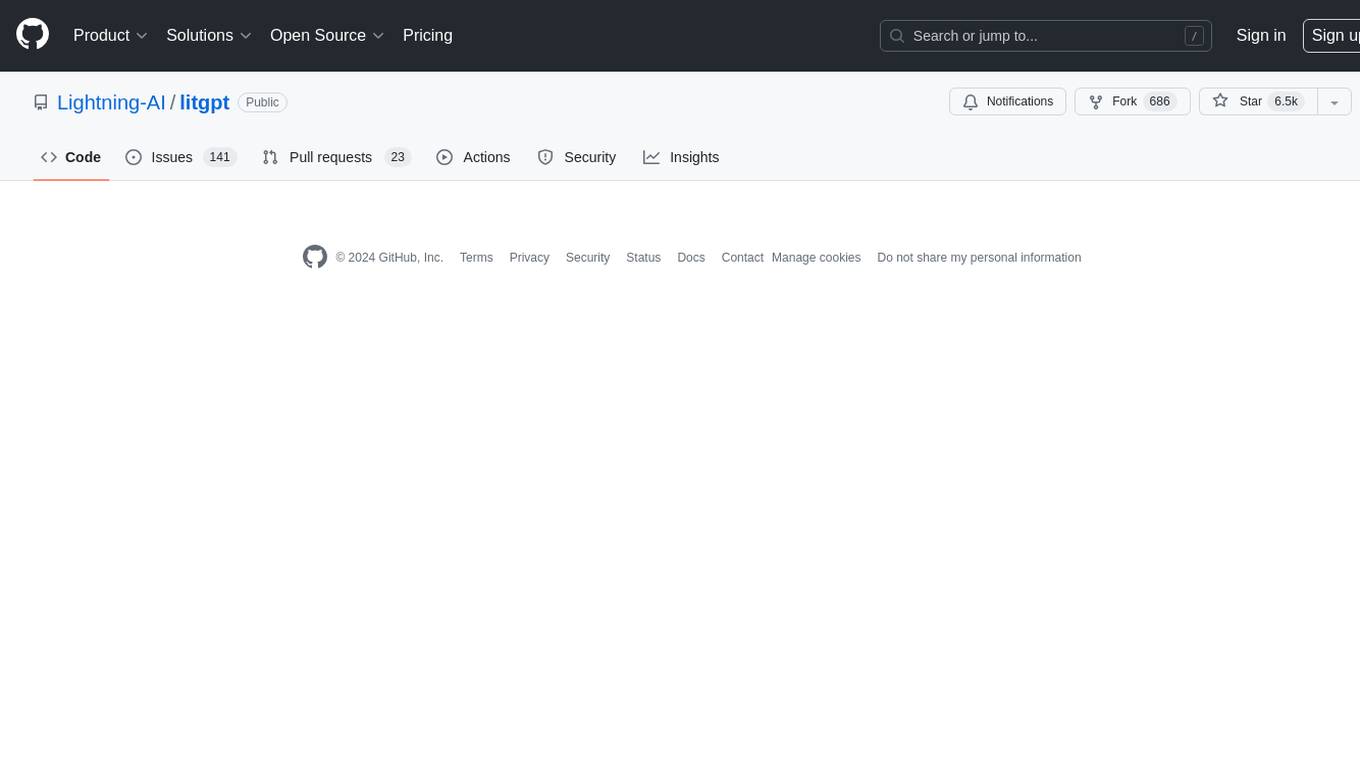
litgpt
LitGPT is a command-line tool designed to easily finetune, pretrain, evaluate, and deploy 20+ LLMs **on your own data**. It features highly-optimized training recipes for the world's most powerful open-source large-language-models (LLMs).
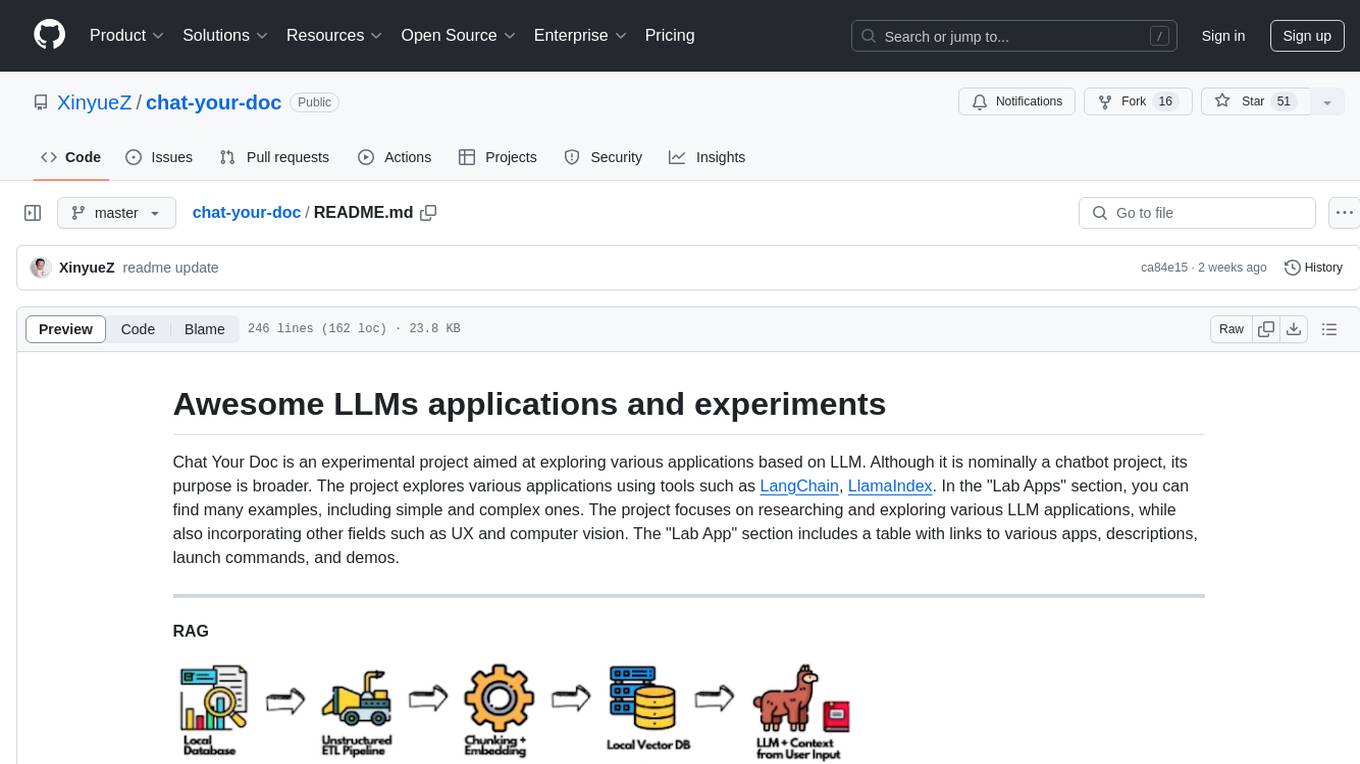
chat-your-doc
Chat Your Doc is an experimental project exploring various applications based on LLM technology. It goes beyond being just a chatbot project, focusing on researching LLM applications using tools like LangChain and LlamaIndex. The project delves into UX, computer vision, and offers a range of examples in the 'Lab Apps' section. It includes links to different apps, descriptions, launch commands, and demos, aiming to showcase the versatility and potential of LLM applications.
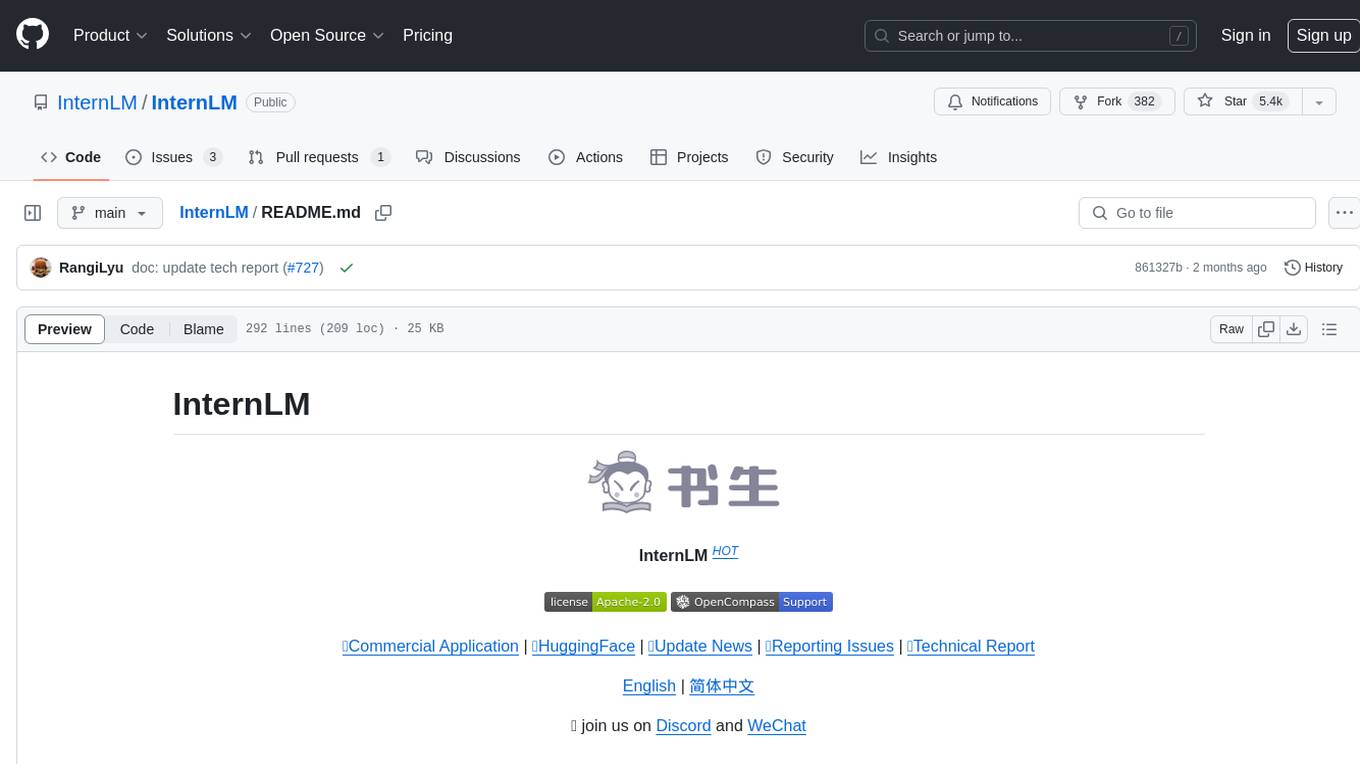
InternLM
InternLM is a powerful language model series with features such as 200K context window for long-context tasks, outstanding comprehensive performance in reasoning, math, code, chat experience, instruction following, and creative writing, code interpreter & data analysis capabilities, and stronger tool utilization capabilities. It offers models in sizes of 7B and 20B, suitable for research and complex scenarios. The models are recommended for various applications and exhibit better performance than previous generations. InternLM models may match or surpass other open-source models like ChatGPT. The tool has been evaluated on various datasets and has shown superior performance in multiple tasks. It requires Python >= 3.8, PyTorch >= 1.12.0, and Transformers >= 4.34 for usage. InternLM can be used for tasks like chat, agent applications, fine-tuning, deployment, and long-context inference.
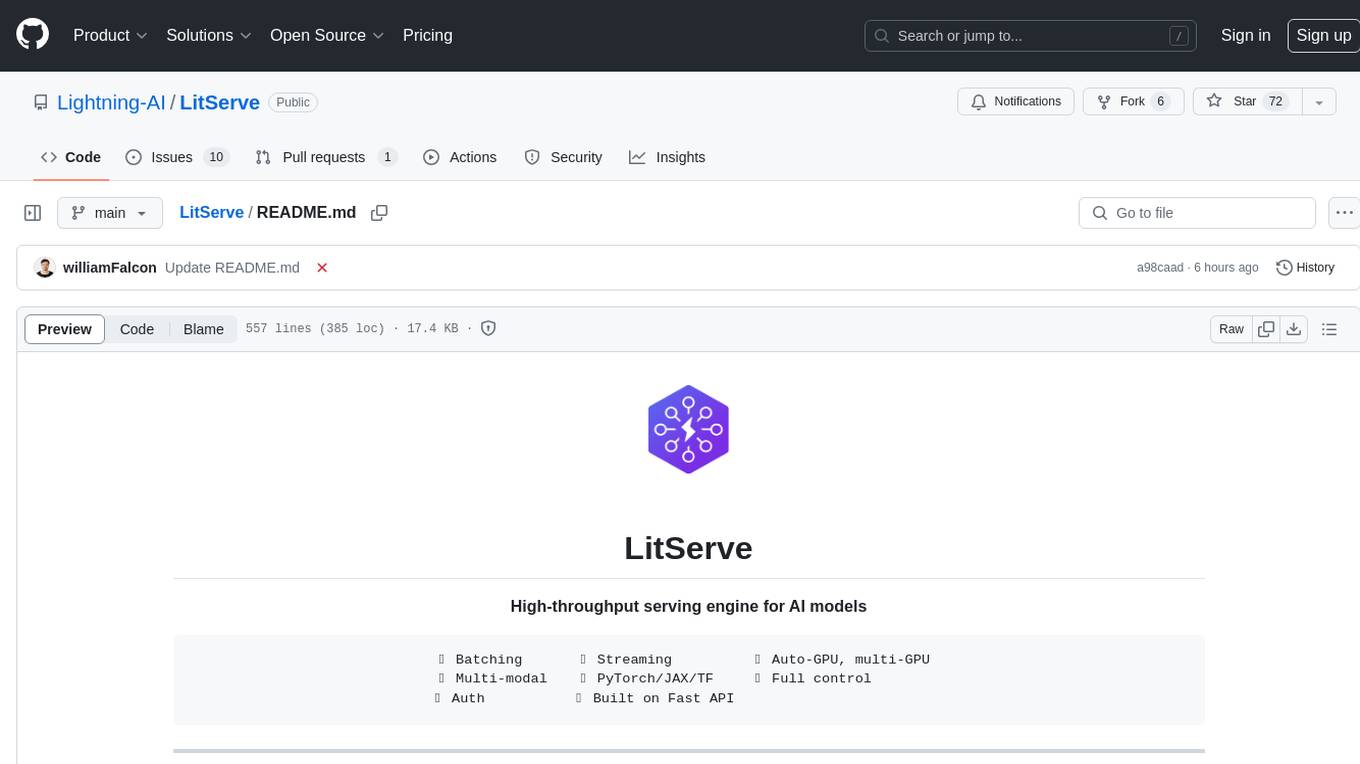
LitServe
LitServe is a high-throughput serving engine designed for deploying AI models at scale. It generates an API endpoint for models, handles batching, streaming, and autoscaling across CPU/GPUs. LitServe is built for enterprise scale with a focus on minimal, hackable code-base without bloat. It supports various model types like LLMs, vision, time-series, and works with frameworks like PyTorch, JAX, Tensorflow, and more. The tool allows users to focus on model performance rather than serving boilerplate, providing full control and flexibility.
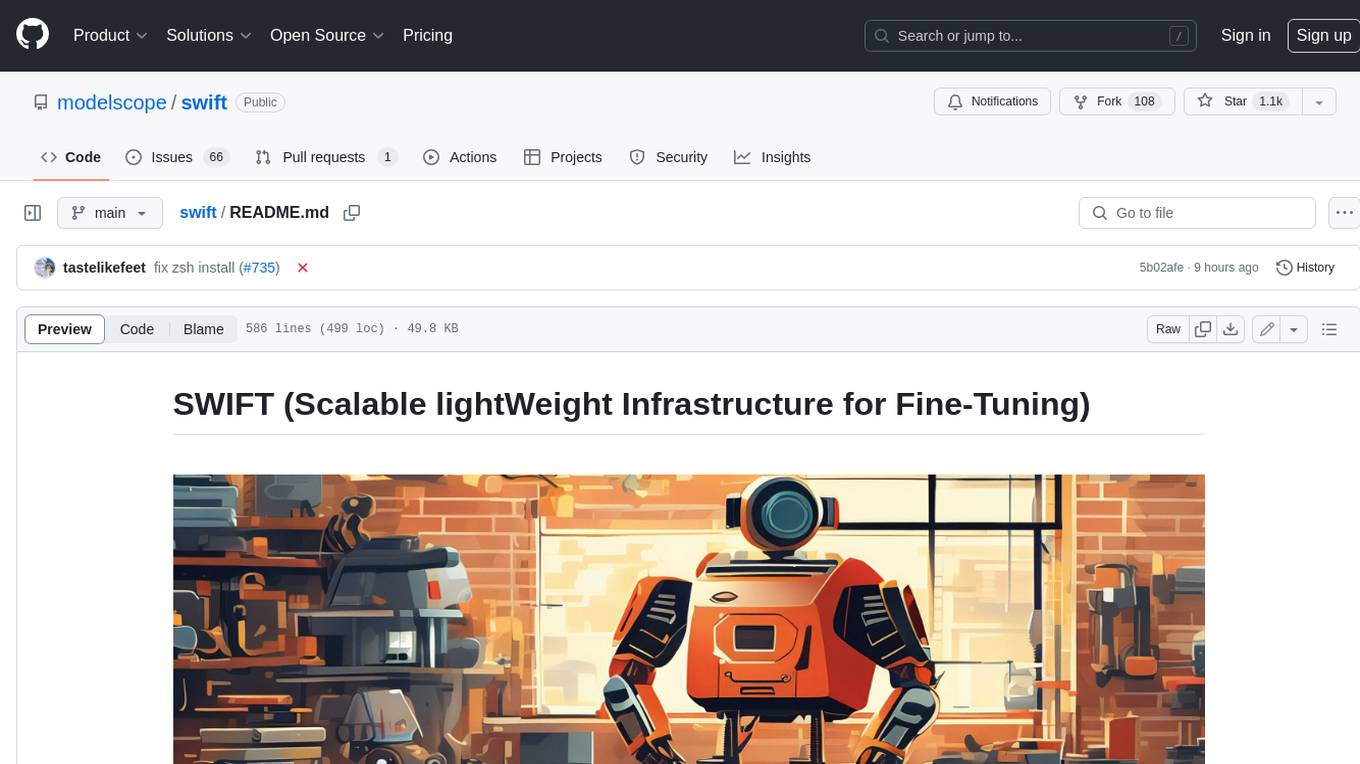
swift
SWIFT (Scalable lightWeight Infrastructure for Fine-Tuning) supports training, inference, evaluation and deployment of nearly **200 LLMs and MLLMs** (multimodal large models). Developers can directly apply our framework to their own research and production environments to realize the complete workflow from model training and evaluation to application. In addition to supporting the lightweight training solutions provided by [PEFT](https://github.com/huggingface/peft), we also provide a complete **Adapters library** to support the latest training techniques such as NEFTune, LoRA+, LLaMA-PRO, etc. This adapter library can be used directly in your own custom workflow without our training scripts. To facilitate use by users unfamiliar with deep learning, we provide a Gradio web-ui for controlling training and inference, as well as accompanying deep learning courses and best practices for beginners. Additionally, we are expanding capabilities for other modalities. Currently, we support full-parameter training and LoRA training for AnimateDiff.
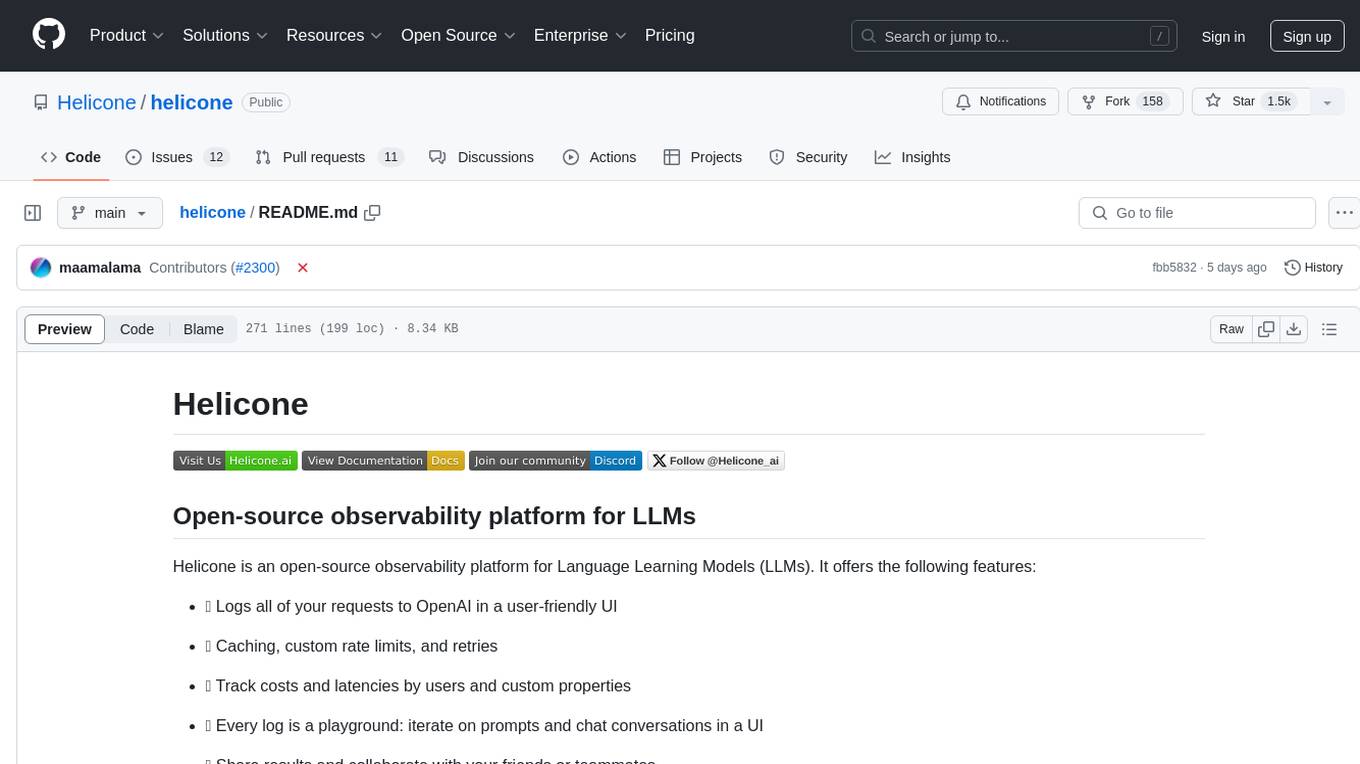
helicone
Helicone is an open-source observability platform designed for Language Learning Models (LLMs). It logs requests to OpenAI in a user-friendly UI, offers caching, rate limits, and retries, tracks costs and latencies, provides a playground for iterating on prompts and chat conversations, supports collaboration, and will soon have APIs for feedback and evaluation. The platform is deployed on Cloudflare and consists of services like Web (NextJs), Worker (Cloudflare Workers), Jawn (Express), Supabase, and ClickHouse. Users can interact with Helicone locally by setting up the required services and environment variables. The platform encourages contributions and provides resources for learning, documentation, and integrations.
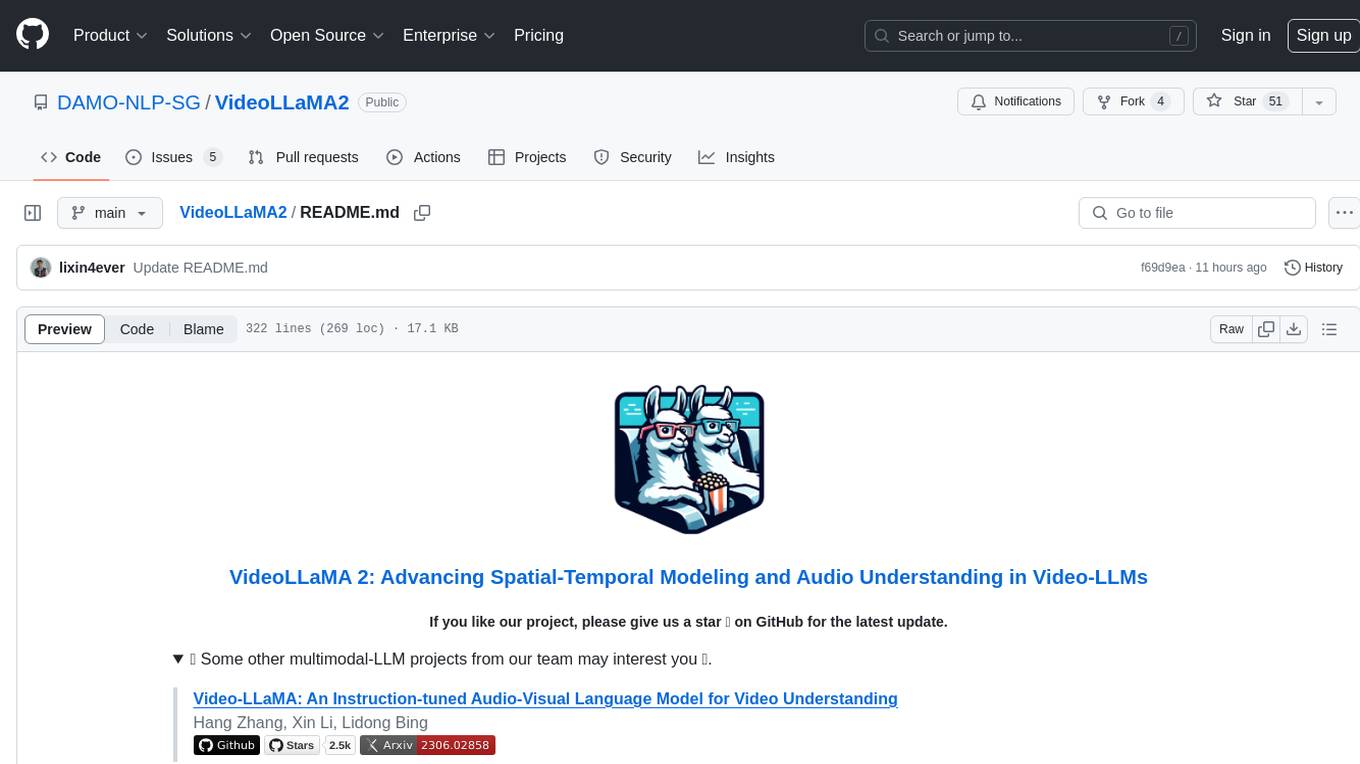
VideoLLaMA2
VideoLLaMA 2 is a project focused on advancing spatial-temporal modeling and audio understanding in video-LLMs. It provides tools for multi-choice video QA, open-ended video QA, and video captioning. The project offers model zoo with different configurations for visual encoder and language decoder. It includes training and evaluation guides, as well as inference capabilities for video and image processing. The project also features a demo setup for running a video-based Large Language Model web demonstration.
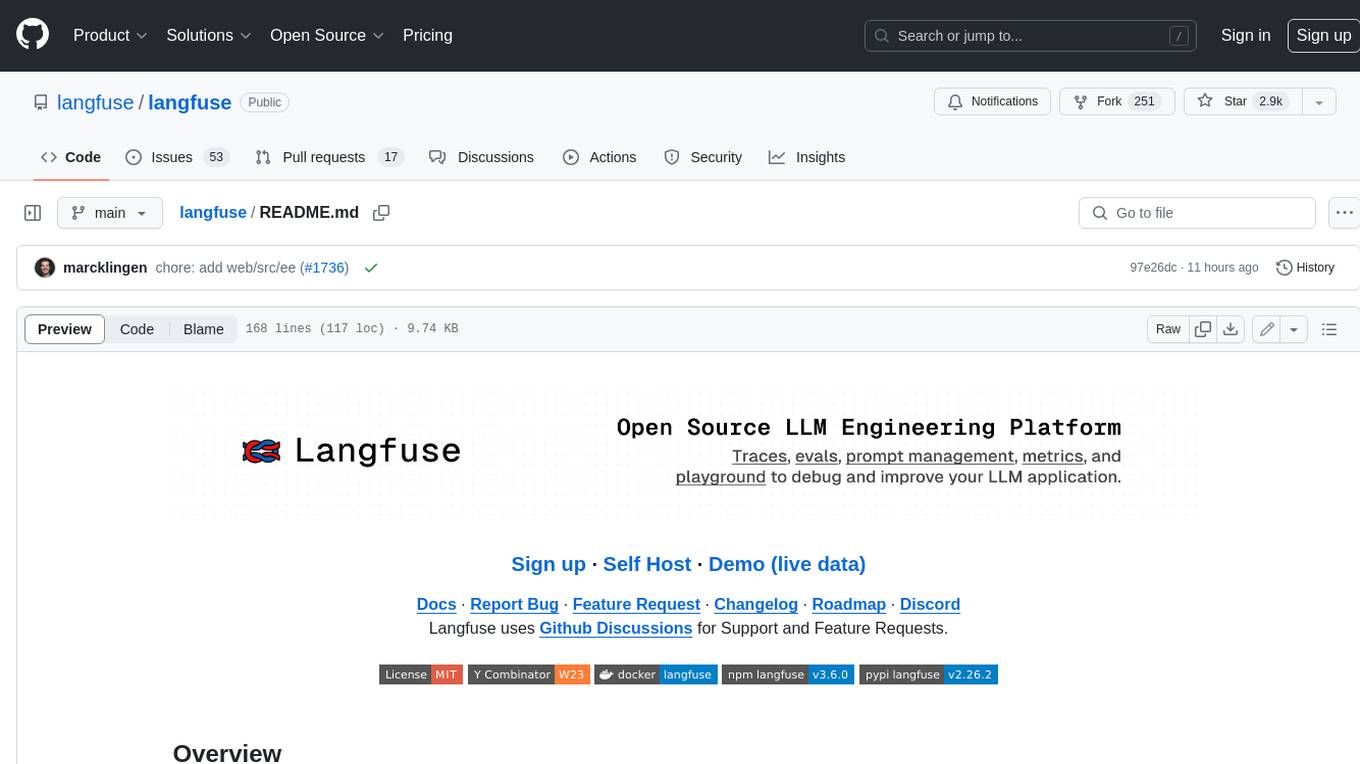
langfuse
Langfuse is a powerful tool that helps you develop, monitor, and test your LLM applications. With Langfuse, you can: * **Develop:** Instrument your app and start ingesting traces to Langfuse, inspect and debug complex logs, and manage, version, and deploy prompts from within Langfuse. * **Monitor:** Track metrics (cost, latency, quality) and gain insights from dashboards & data exports, collect and calculate scores for your LLM completions, run model-based evaluations, collect user feedback, and manually score observations in Langfuse. * **Test:** Track and test app behaviour before deploying a new version, test expected in and output pairs and benchmark performance before deploying, and track versions and releases in your application. Langfuse is easy to get started with and offers a generous free tier. You can sign up for Langfuse Cloud or deploy Langfuse locally or on your own infrastructure. Langfuse also offers a variety of integrations to make it easy to connect to your LLM applications.
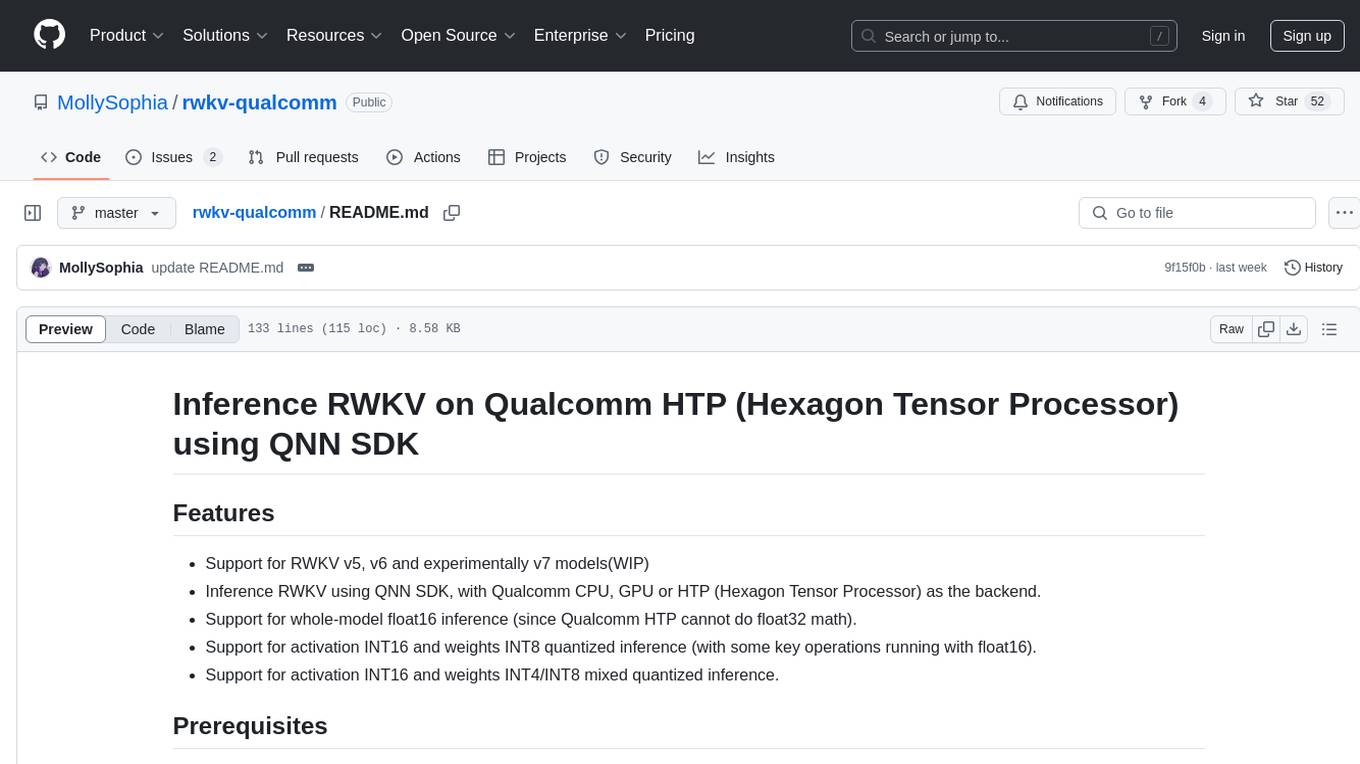
rwkv-qualcomm
This repository provides support for inference RWKV models on Qualcomm HTP (Hexagon Tensor Processor) using QNN SDK. It supports RWKV v5, v6, and experimentally v7 models, inference using Qualcomm CPU, GPU, or HTP as the backend, whole-model float16 inference, activation INT16 and weights INT8 quantized inference, and activation INT16 and weights INT4/INT8 mixed quantized inference. Users can convert model weights to QNN model library files, generate HTP context cache, and run inference on Qualcomm Snapdragon SM8650 with HTP v75. The project requires QNN SDK, AIMET toolkit, and specific hardware for verification.
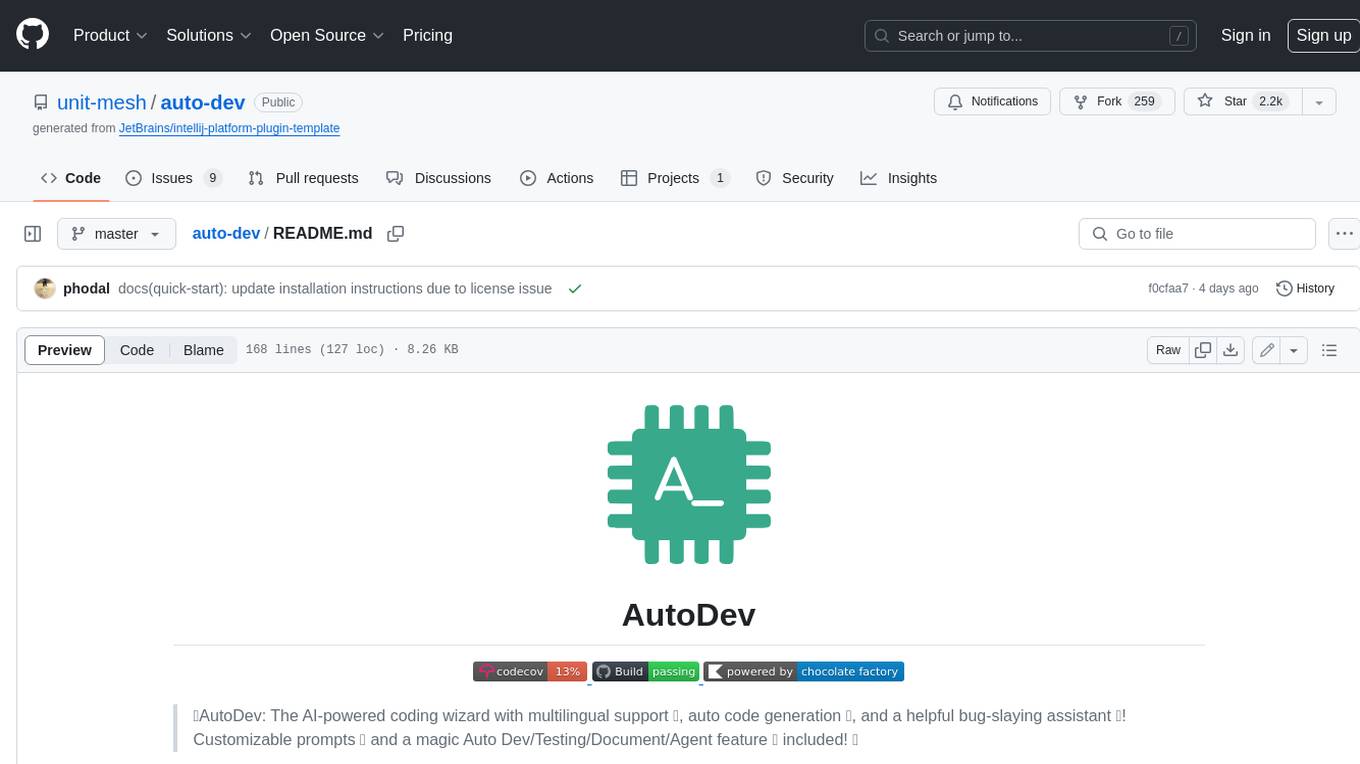
auto-dev
AutoDev is an AI-powered coding wizard that supports multiple languages, including Java, Kotlin, JavaScript/TypeScript, Rust, Python, Golang, C/C++/OC, and more. It offers a range of features, including auto development mode, copilot mode, chat with AI, customization options, SDLC support, custom AI agent integration, and language features such as language support, extensions, and a DevIns language for AI agent development. AutoDev is designed to assist developers with tasks such as auto code generation, bug detection, code explanation, exception tracing, commit message generation, code review content generation, smart refactoring, Dockerfile generation, CI/CD config file generation, and custom shell/command generation. It also provides a built-in LLM fine-tune model and supports UnitEval for LLM result evaluation and UnitGen for code-LLM fine-tune data generation.
For similar tasks
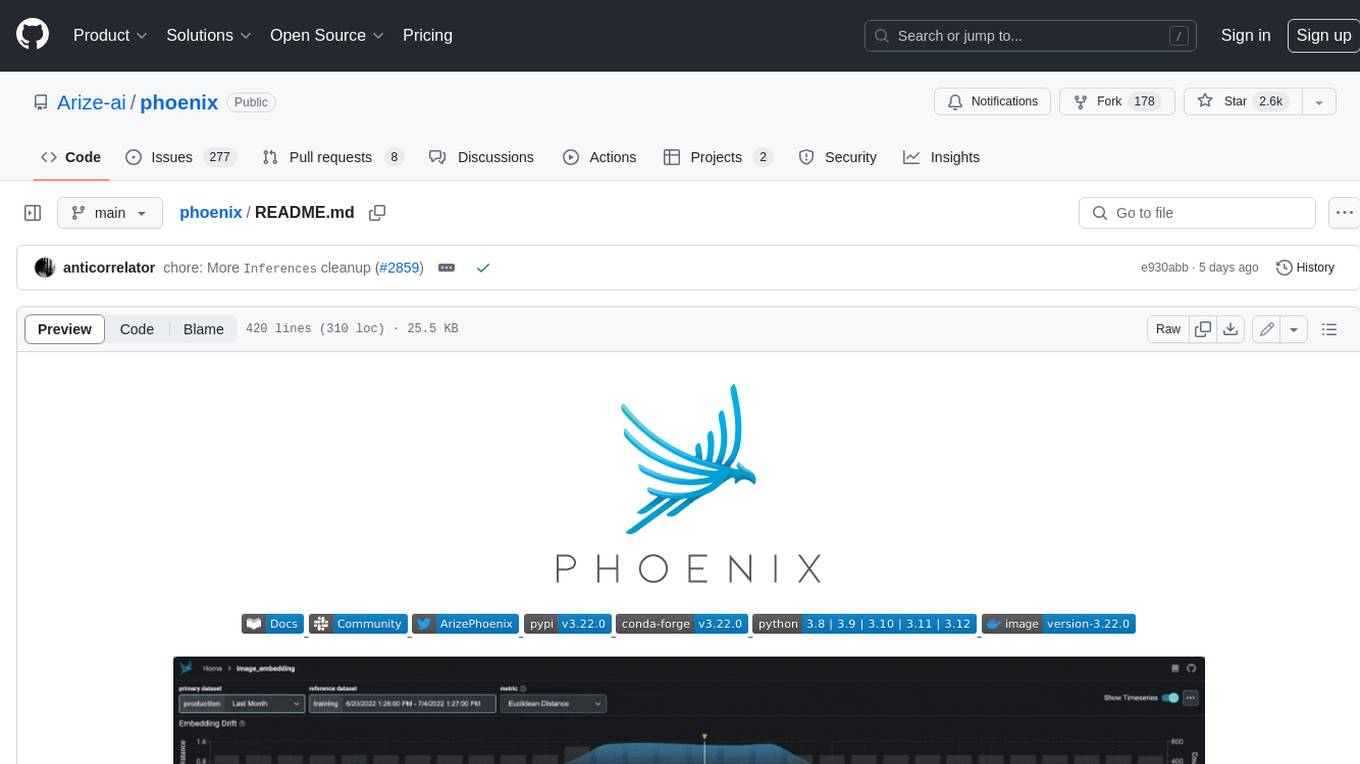
phoenix
Phoenix is a tool that provides MLOps and LLMOps insights at lightning speed with zero-config observability. It offers a notebook-first experience for monitoring models and LLM Applications by providing LLM Traces, LLM Evals, Embedding Analysis, RAG Analysis, and Structured Data Analysis. Users can trace through the execution of LLM Applications, evaluate generative models, explore embedding point-clouds, visualize generative application's search and retrieval process, and statistically analyze structured data. Phoenix is designed to help users troubleshoot problems related to retrieval, tool execution, relevance, toxicity, drift, and performance degradation.
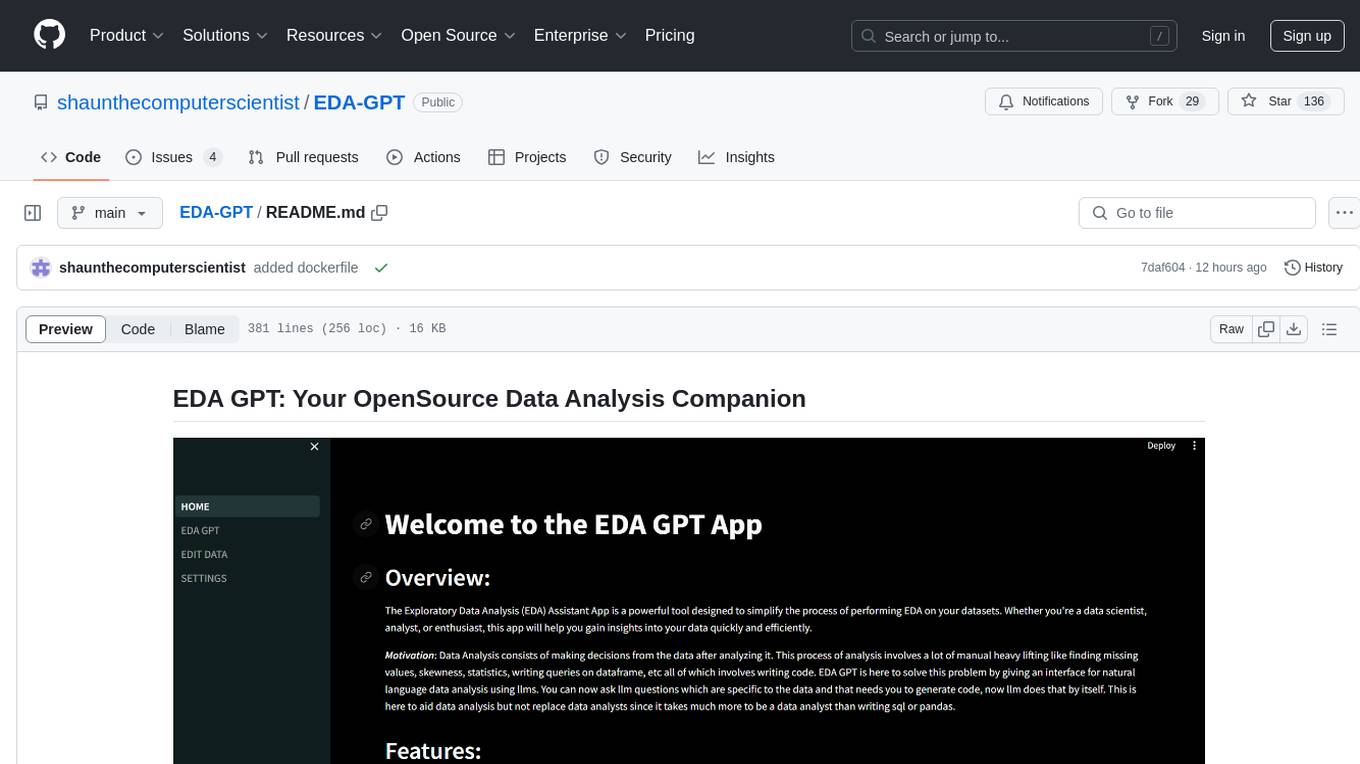
EDA-GPT
EDA GPT is an open-source data analysis companion that offers a comprehensive solution for structured and unstructured data analysis. It streamlines the data analysis process, empowering users to explore, visualize, and gain insights from their data. EDA GPT supports analyzing structured data in various formats like CSV, XLSX, and SQLite, generating graphs, and conducting in-depth analysis of unstructured data such as PDFs and images. It provides a user-friendly interface, powerful features, and capabilities like comparing performance with other tools, analyzing large language models, multimodal search, data cleaning, and editing. The tool is optimized for maximal parallel processing, searching internet and documents, and creating analysis reports from structured and unstructured data.
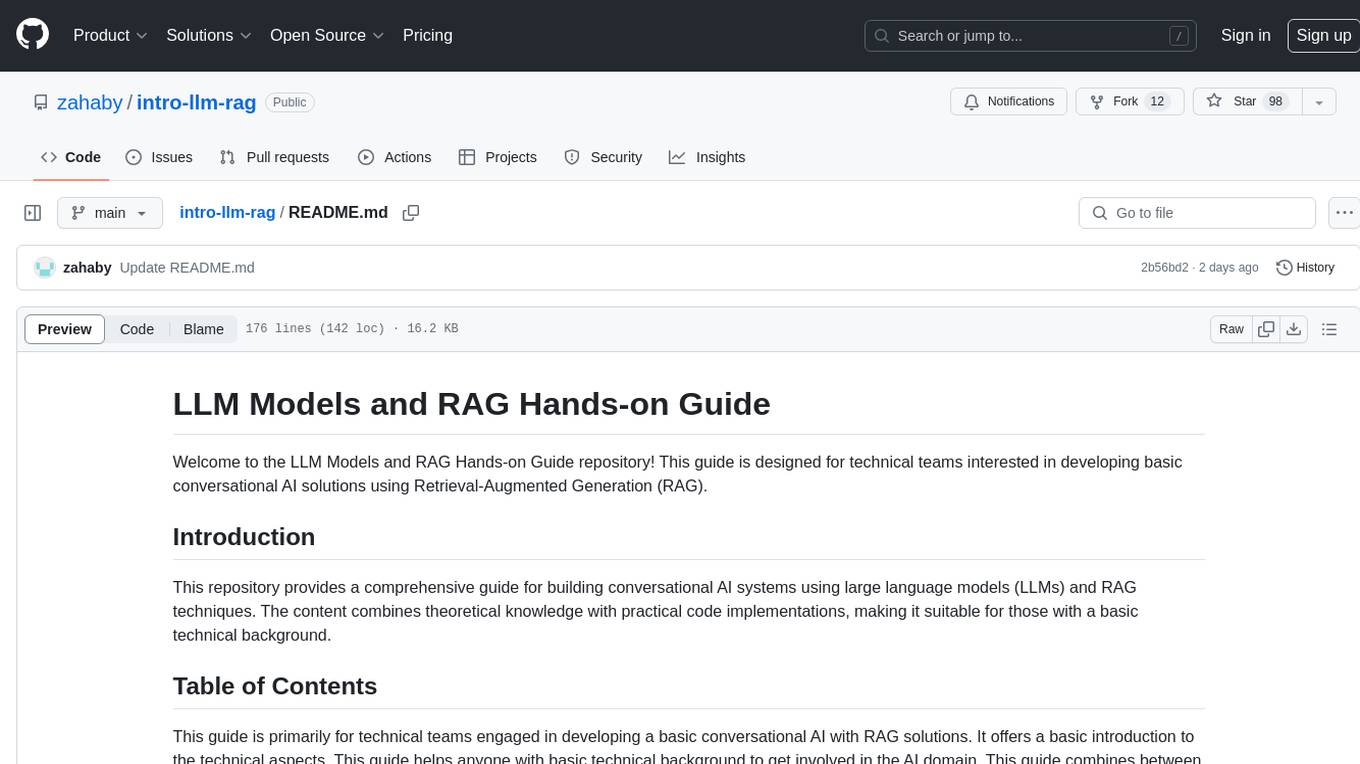
intro-llm-rag
This repository serves as a comprehensive guide for technical teams interested in developing conversational AI solutions using Retrieval-Augmented Generation (RAG) techniques. It covers theoretical knowledge and practical code implementations, making it suitable for individuals with a basic technical background. The content includes information on large language models (LLMs), transformers, prompt engineering, embeddings, vector stores, and various other key concepts related to conversational AI. The repository also provides hands-on examples for two different use cases, along with implementation details and performance analysis.
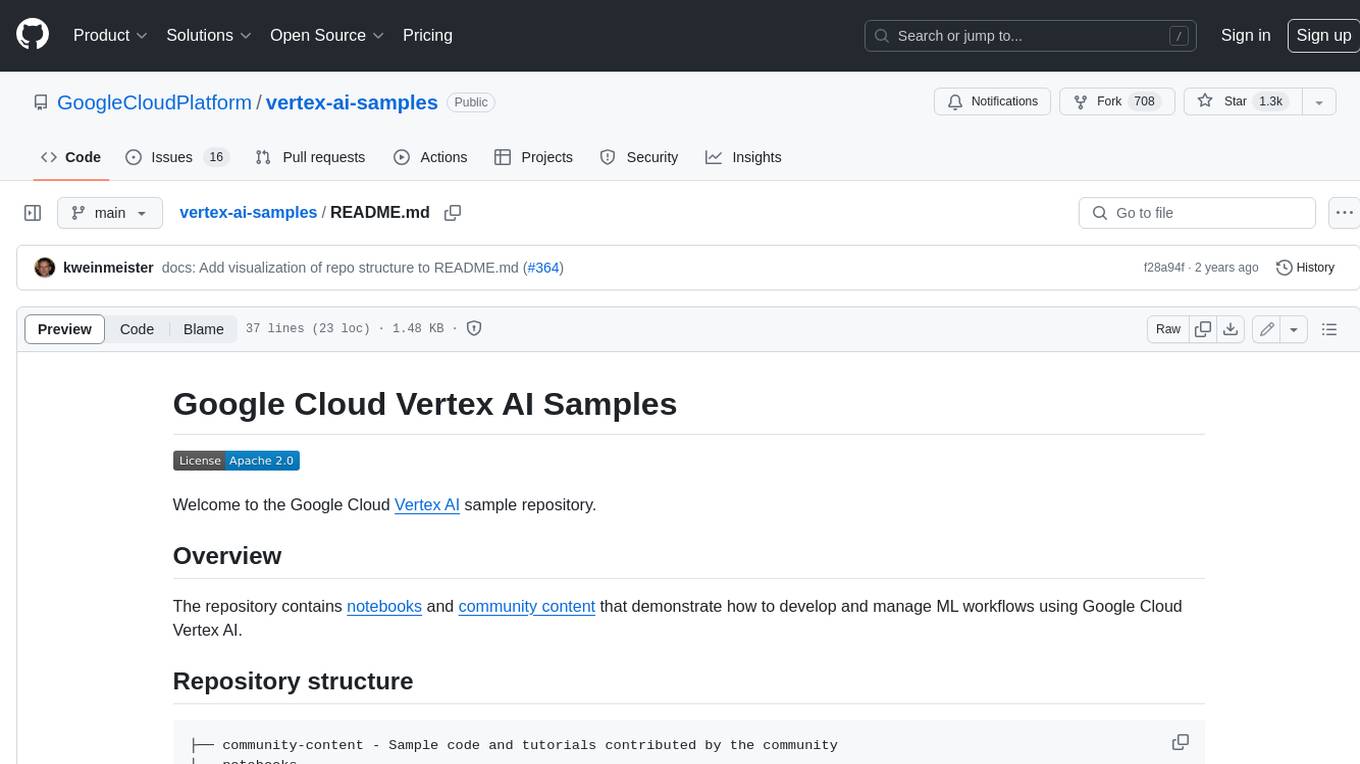
vertex-ai-samples
The Google Cloud Vertex AI sample repository contains notebooks and community content that demonstrate how to develop and manage ML workflows using Google Cloud Vertex AI.
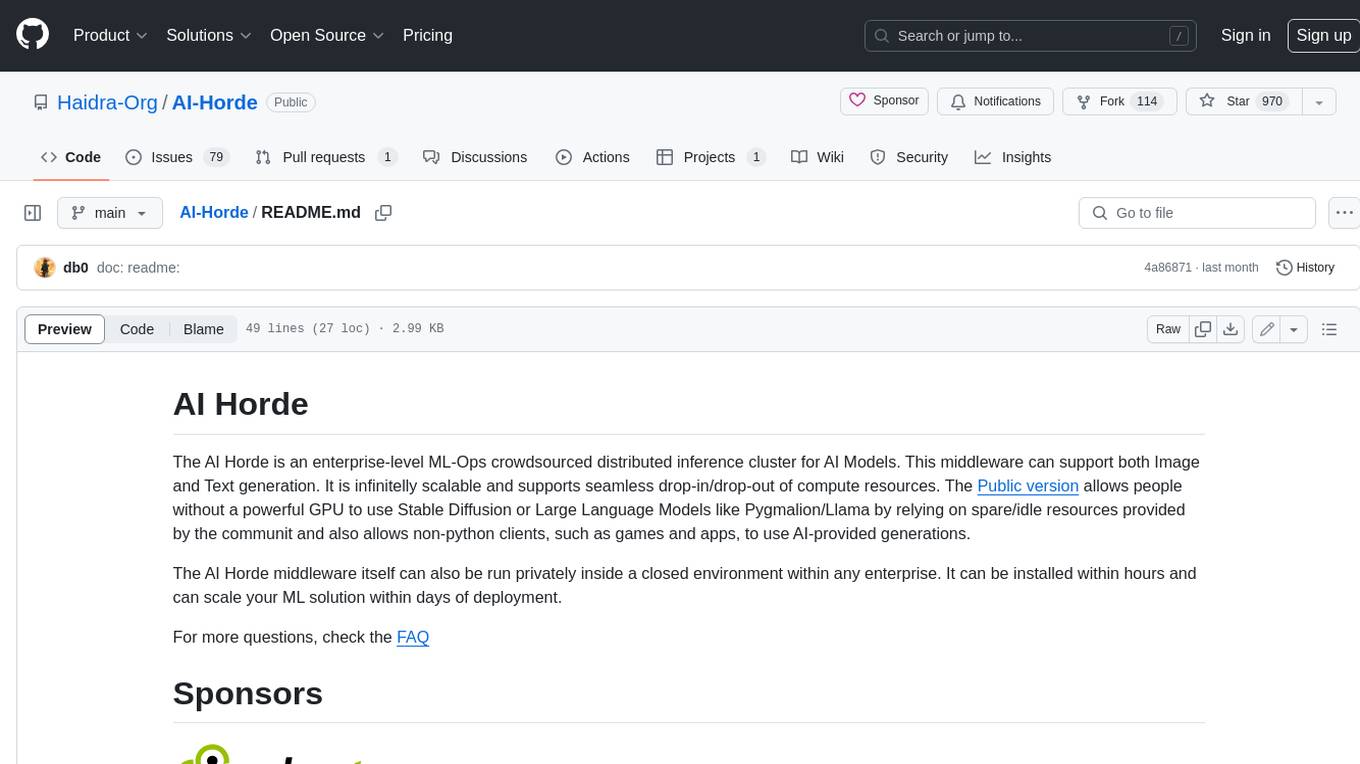
AI-Horde
The AI Horde is an enterprise-level ML-Ops crowdsourced distributed inference cluster for AI Models. This middleware can support both Image and Text generation. It is infinitely scalable and supports seamless drop-in/drop-out of compute resources. The Public version allows people without a powerful GPU to use Stable Diffusion or Large Language Models like Pygmalion/Llama by relying on spare/idle resources provided by the community and also allows non-python clients, such as games and apps, to use AI-provided generations.
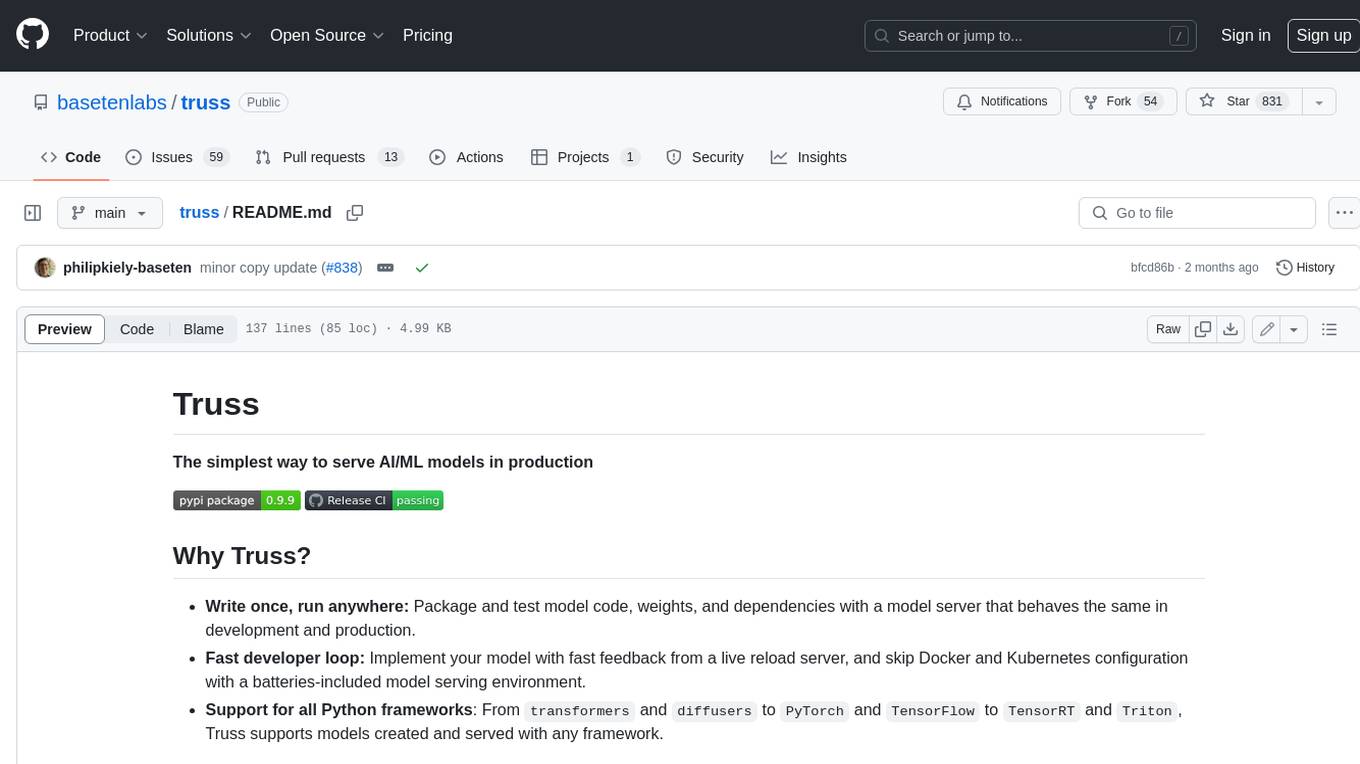
truss
Truss is a tool that simplifies the process of serving AI/ML models in production. It provides a consistent and easy-to-use interface for packaging, testing, and deploying models, regardless of the framework they were created with. Truss also includes a live reload server for fast feedback during development, and a batteries-included model serving environment that eliminates the need for Docker and Kubernetes configuration.
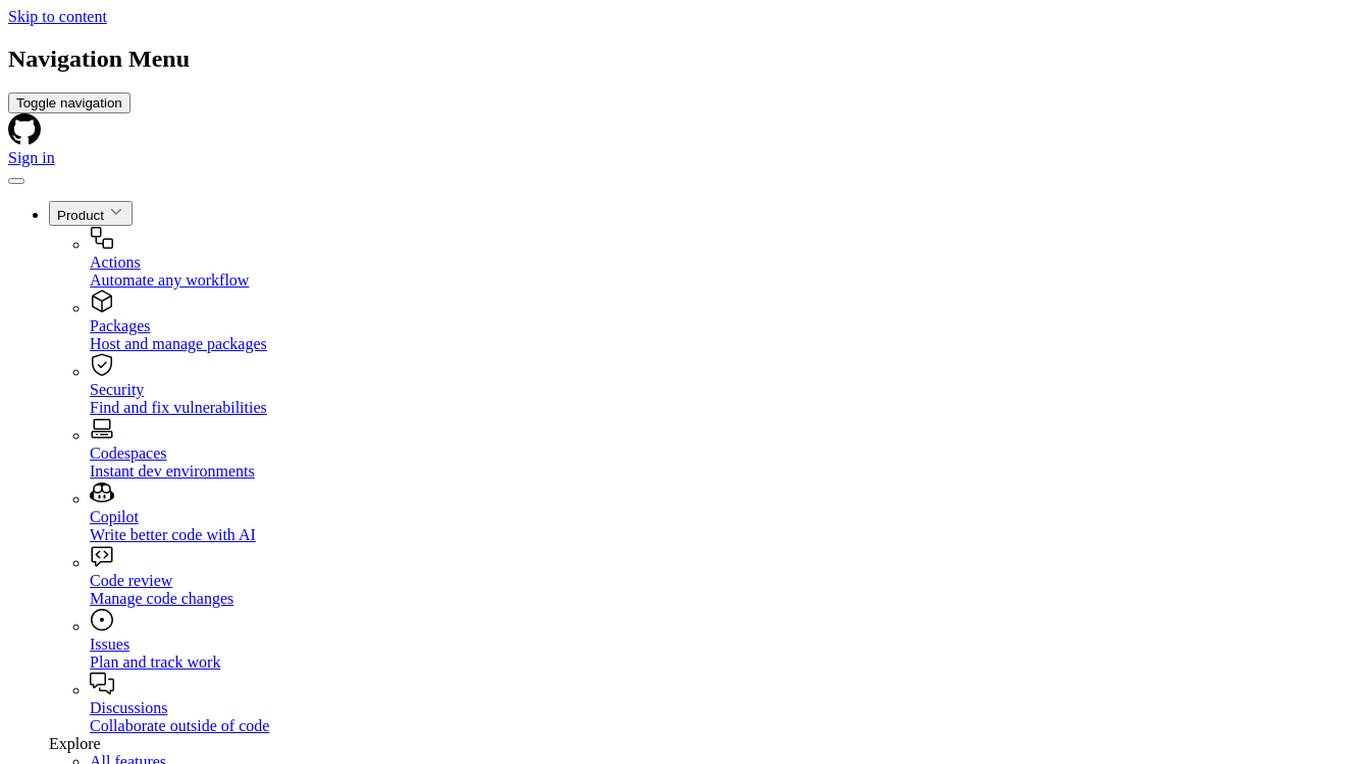
cyclops
Cyclops is a toolkit for facilitating research and deployment of ML models for healthcare. It provides a few high-level APIs namely: data - Create datasets for training, inference and evaluation. We use the popular π€ datasets to efficiently load and slice different modalities of data models - Use common model implementations using scikit-learn and PyTorch tasks - Use common ML task formulations such as binary classification or multi-label classification on tabular, time-series and image data evaluate - Evaluate models on clinical prediction tasks monitor - Detect dataset shift relevant for clinical use cases report - Create model report cards for clinical ML models
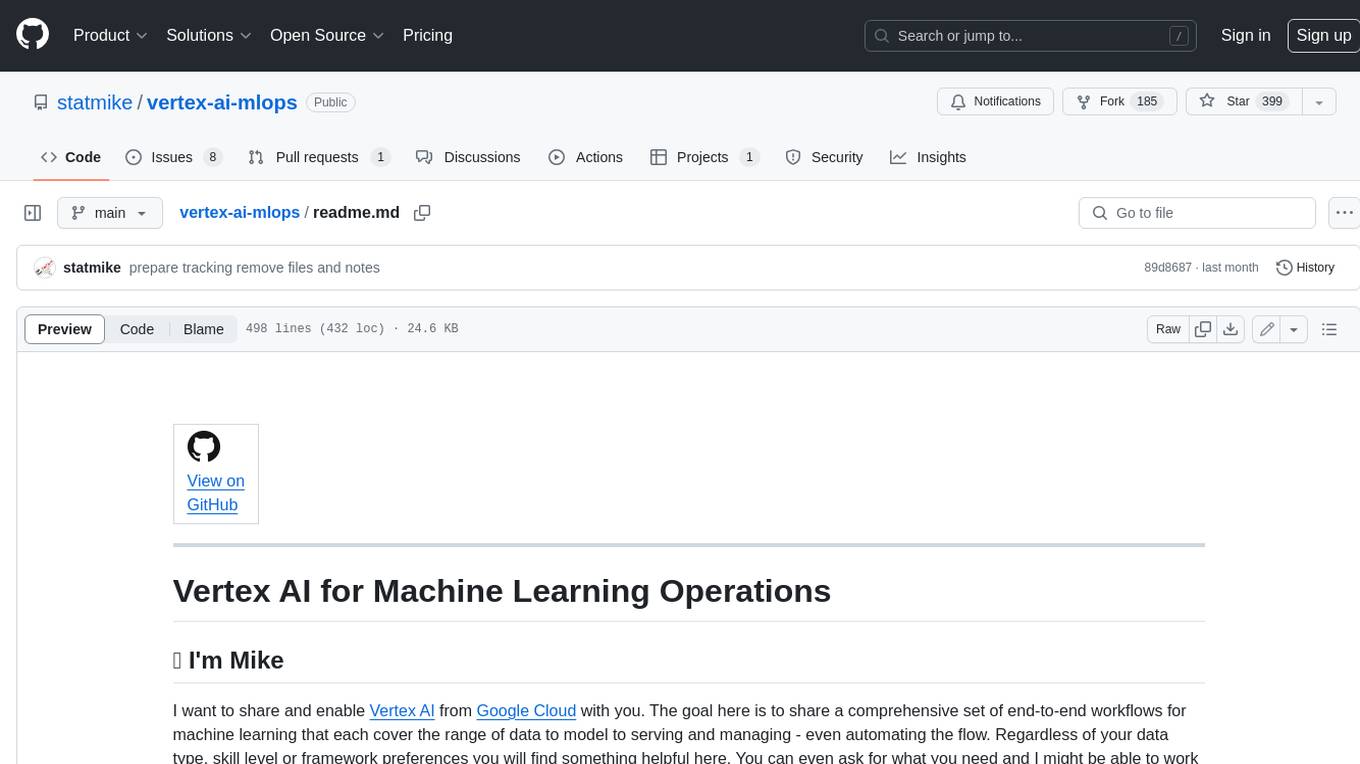
vertex-ai-mlops
Vertex AI is a platform for end-to-end model development. It consist of core components that make the processes of MLOps possible for design patterns of all types.
For similar jobs
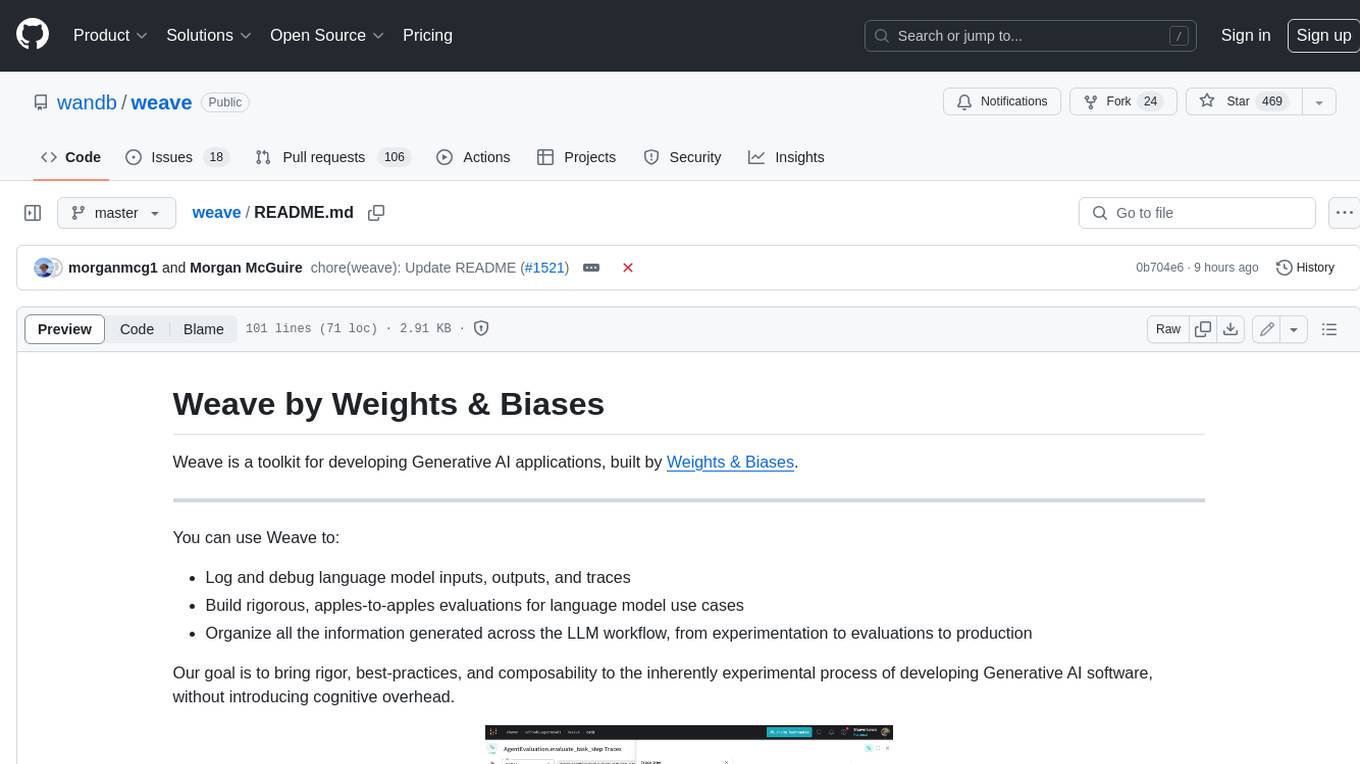
weave
Weave is a toolkit for developing Generative AI applications, built by Weights & Biases. With Weave, you can log and debug language model inputs, outputs, and traces; build rigorous, apples-to-apples evaluations for language model use cases; and organize all the information generated across the LLM workflow, from experimentation to evaluations to production. Weave aims to bring rigor, best-practices, and composability to the inherently experimental process of developing Generative AI software, without introducing cognitive overhead.
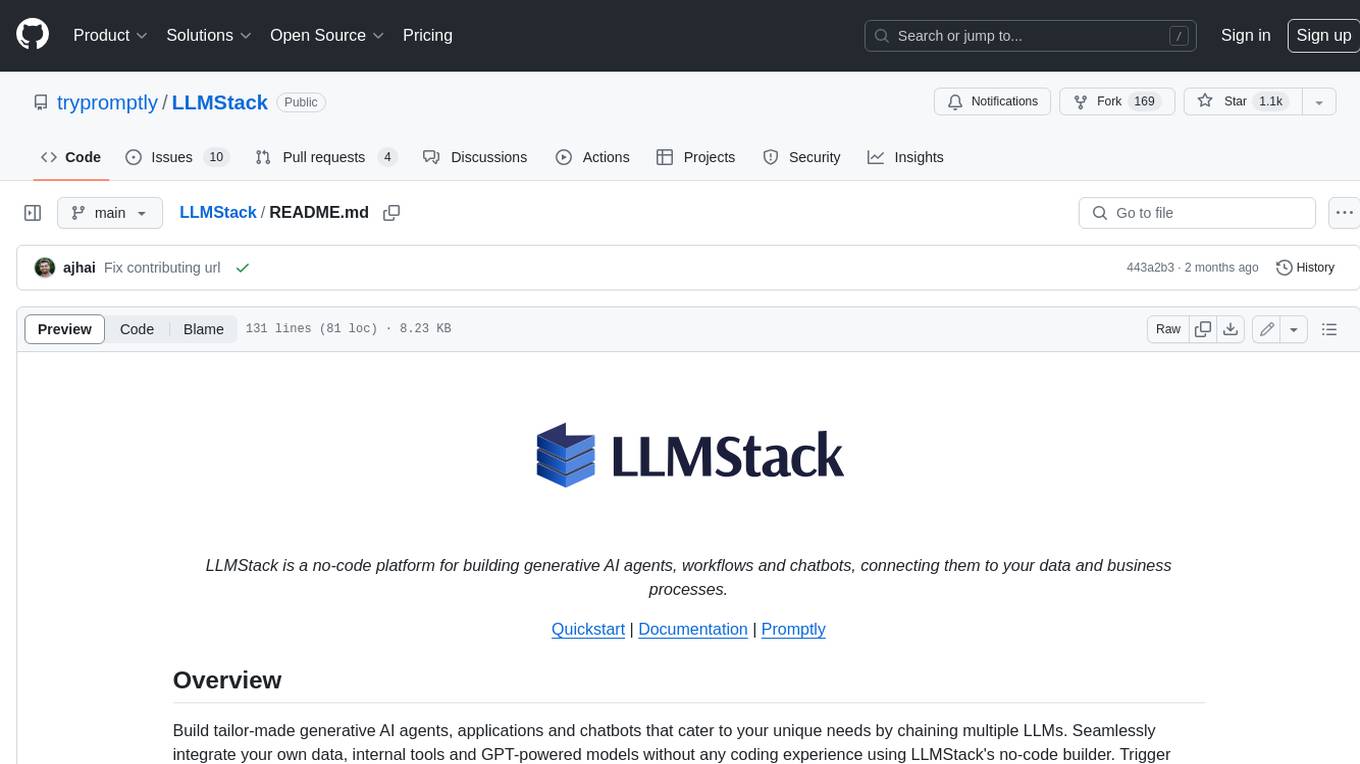
LLMStack
LLMStack is a no-code platform for building generative AI agents, workflows, and chatbots. It allows users to connect their own data, internal tools, and GPT-powered models without any coding experience. LLMStack can be deployed to the cloud or on-premise and can be accessed via HTTP API or triggered from Slack or Discord.
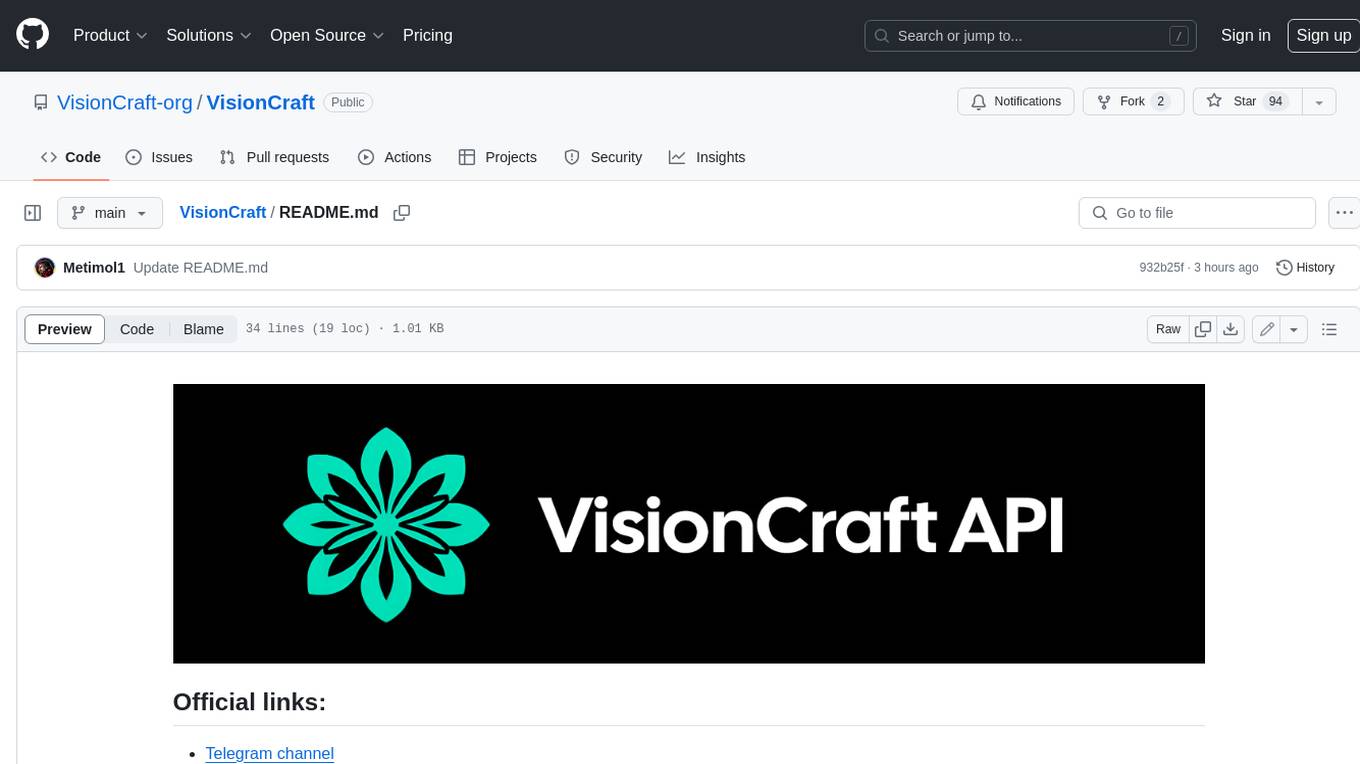
VisionCraft
The VisionCraft API is a free API for using over 100 different AI models. From images to sound.
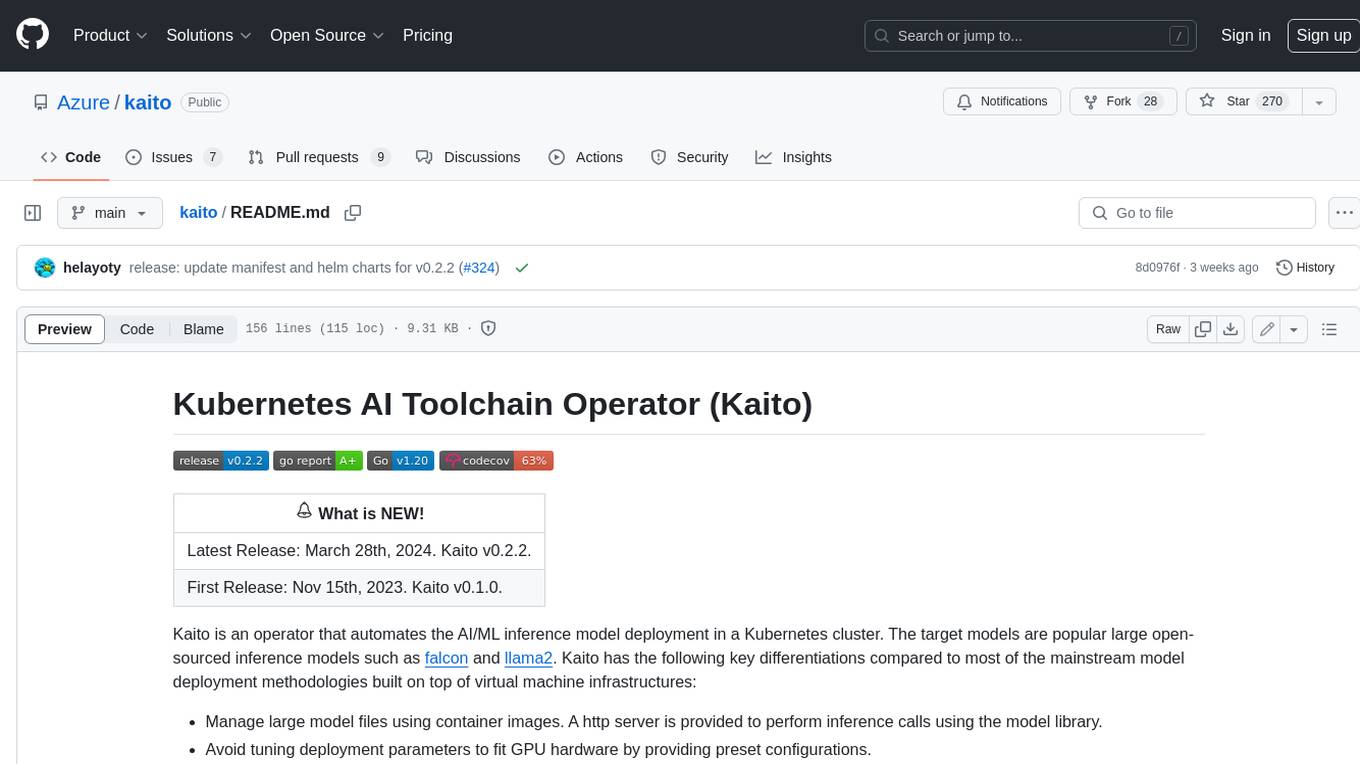
kaito
Kaito is an operator that automates the AI/ML inference model deployment in a Kubernetes cluster. It manages large model files using container images, avoids tuning deployment parameters to fit GPU hardware by providing preset configurations, auto-provisions GPU nodes based on model requirements, and hosts large model images in the public Microsoft Container Registry (MCR) if the license allows. Using Kaito, the workflow of onboarding large AI inference models in Kubernetes is largely simplified.
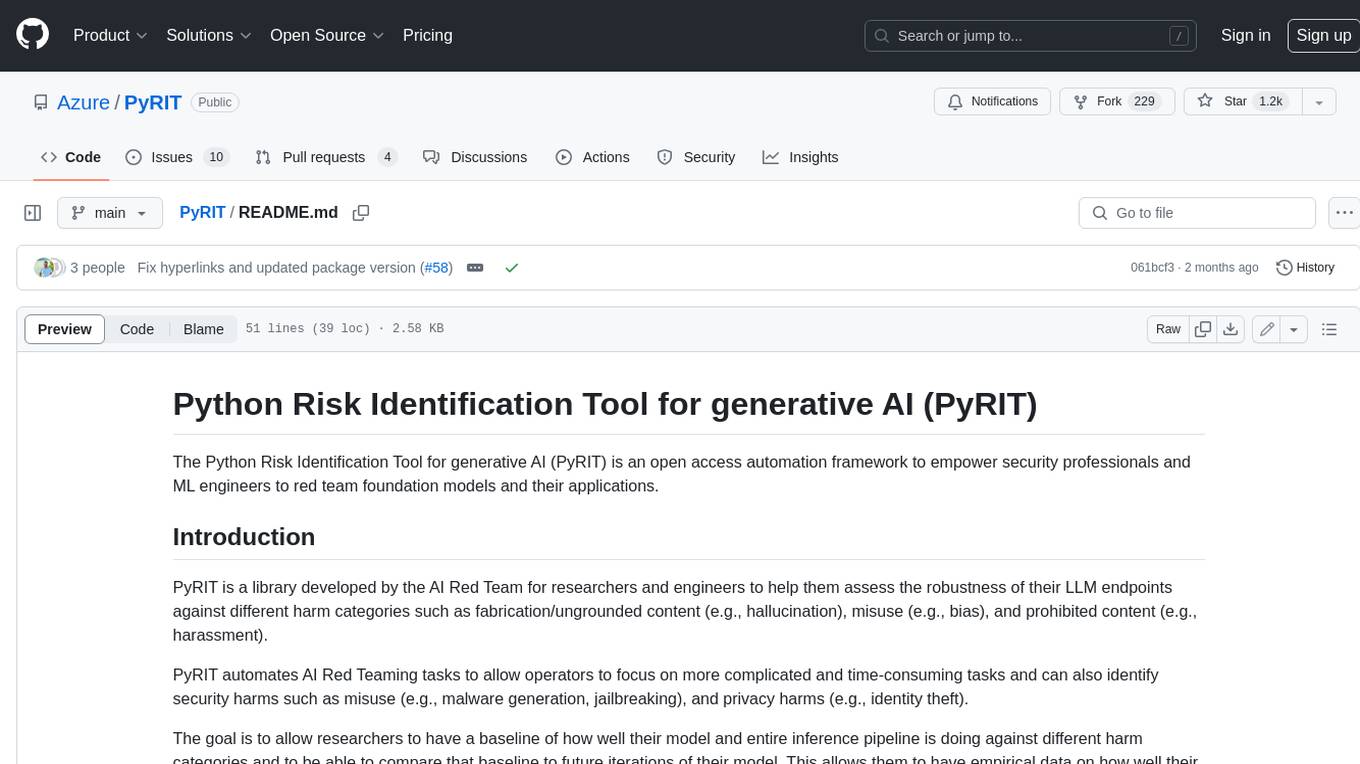
PyRIT
PyRIT is an open access automation framework designed to empower security professionals and ML engineers to red team foundation models and their applications. It automates AI Red Teaming tasks to allow operators to focus on more complicated and time-consuming tasks and can also identify security harms such as misuse (e.g., malware generation, jailbreaking), and privacy harms (e.g., identity theft). The goal is to allow researchers to have a baseline of how well their model and entire inference pipeline is doing against different harm categories and to be able to compare that baseline to future iterations of their model. This allows them to have empirical data on how well their model is doing today, and detect any degradation of performance based on future improvements.
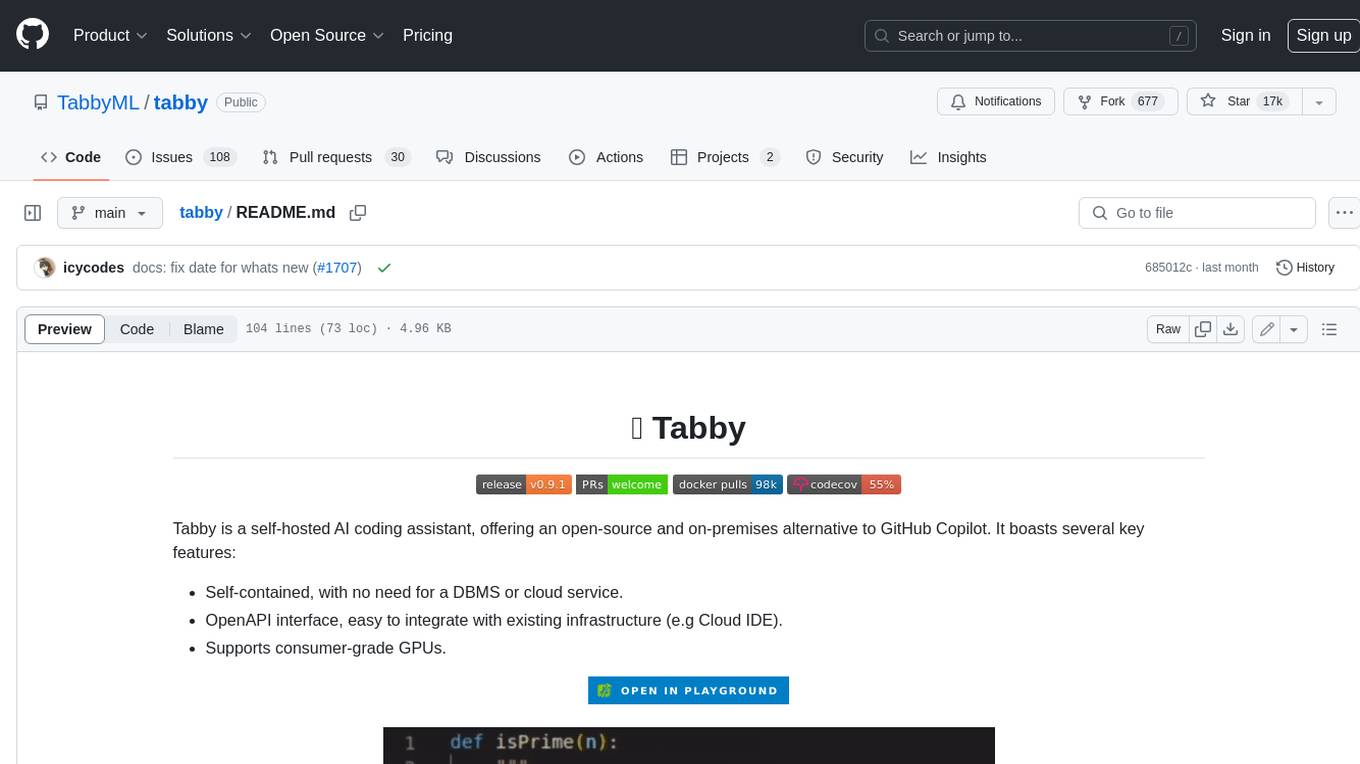
tabby
Tabby is a self-hosted AI coding assistant, offering an open-source and on-premises alternative to GitHub Copilot. It boasts several key features: * Self-contained, with no need for a DBMS or cloud service. * OpenAPI interface, easy to integrate with existing infrastructure (e.g Cloud IDE). * Supports consumer-grade GPUs.
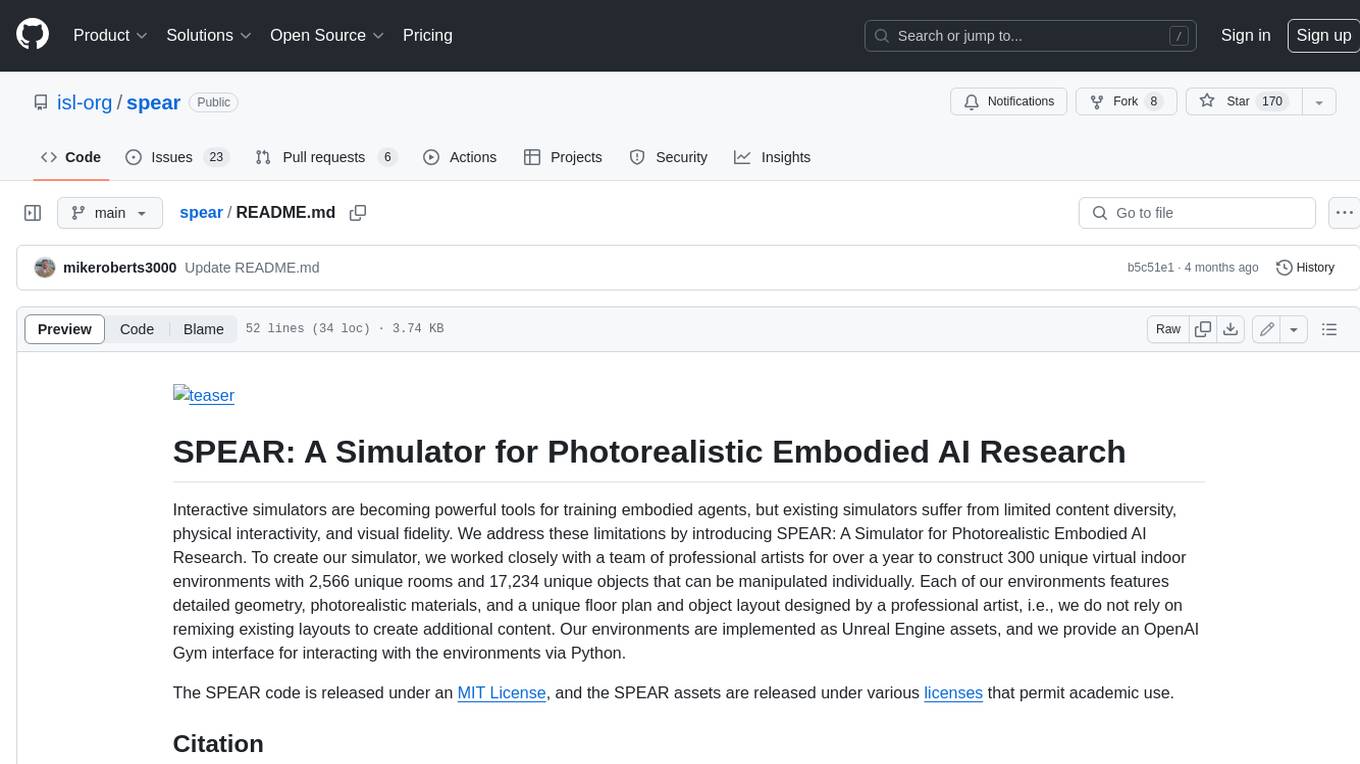
spear
SPEAR (Simulator for Photorealistic Embodied AI Research) is a powerful tool for training embodied agents. It features 300 unique virtual indoor environments with 2,566 unique rooms and 17,234 unique objects that can be manipulated individually. Each environment is designed by a professional artist and features detailed geometry, photorealistic materials, and a unique floor plan and object layout. SPEAR is implemented as Unreal Engine assets and provides an OpenAI Gym interface for interacting with the environments via Python.
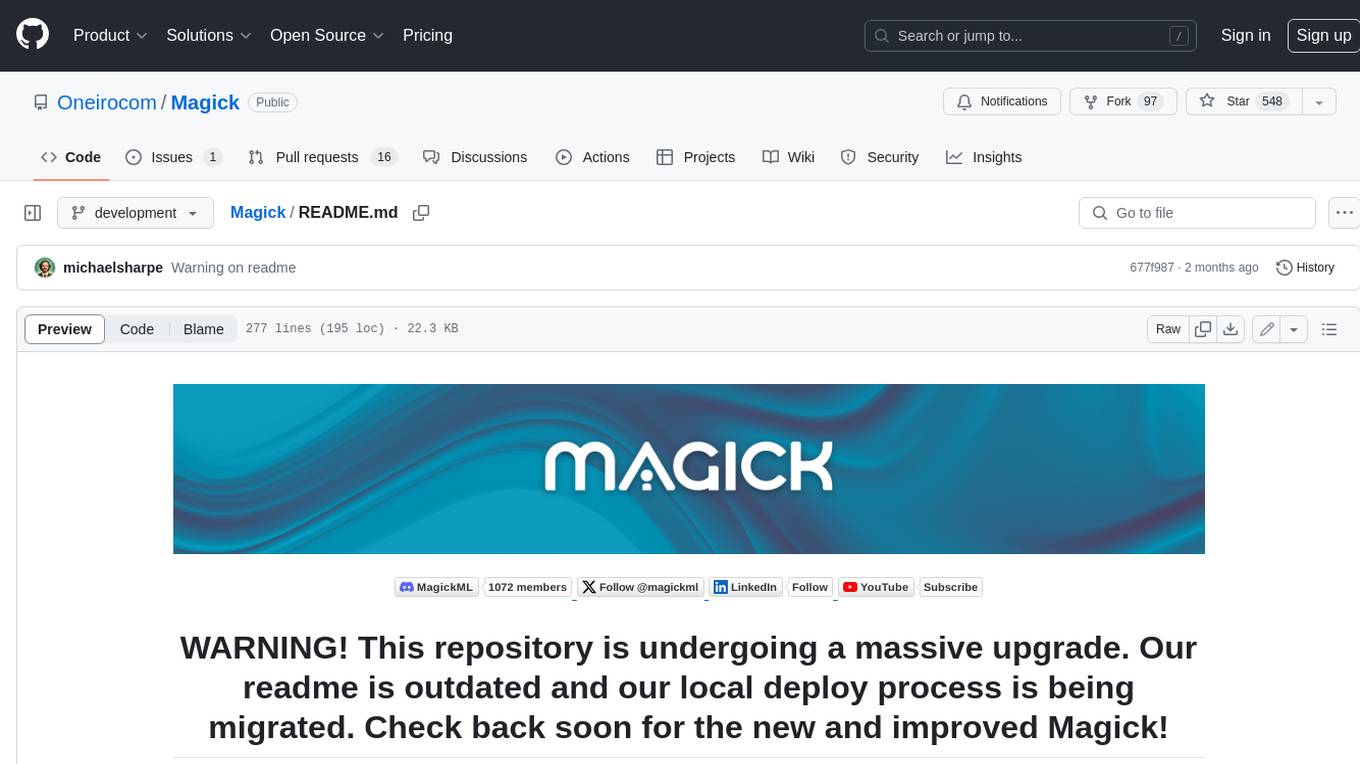
Magick
Magick is a groundbreaking visual AIDE (Artificial Intelligence Development Environment) for no-code data pipelines and multimodal agents. Magick can connect to other services and comes with nodes and templates well-suited for intelligent agents, chatbots, complex reasoning systems and realistic characters.