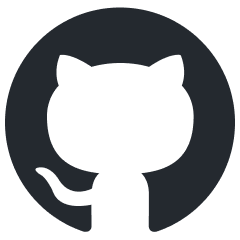
vertex-ai-samples
Notebooks, code samples, sample apps, and other resources that demonstrate how to use, develop and manage machine learning and generative AI workflows using Google Cloud Vertex AI.
Stars: 275
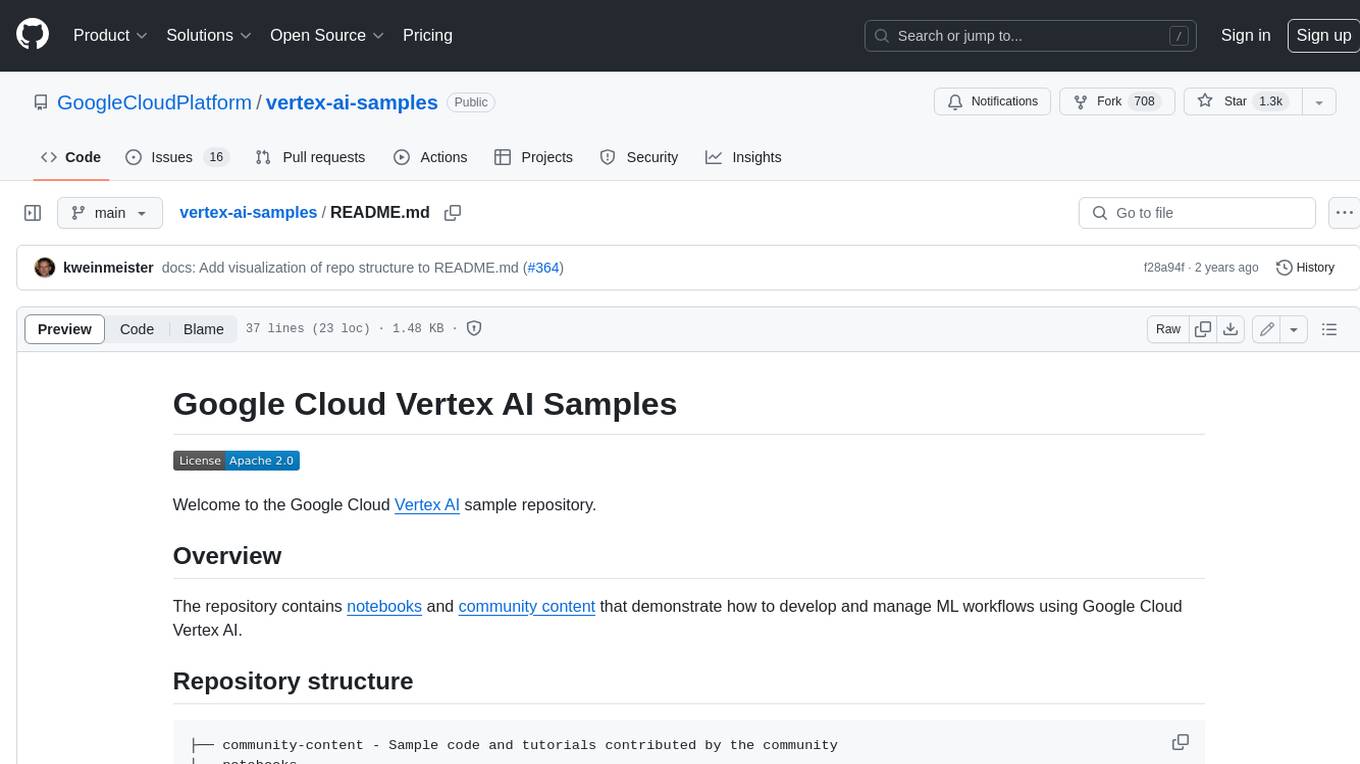
The Google Cloud Vertex AI sample repository contains notebooks and community content that demonstrate how to develop and manage ML workflows using Google Cloud Vertex AI.
README:
This repository contains notebooks, code samples, sample apps, and other resources that demonstrate how to use, develop and manage machine learning and generative AI workflows using Google Cloud Vertex AI.
Vertex AI is a fully-managed, unified AI development platform for building and using generative AI. This repository is designed to help you get started with Vertex AI. Whether you're new to Vertex AI or an experienced ML practitioner, you'll find valuable resources here.
For more Vertex AI Generative AI notebook samples, please visit the Vertex AI Generative AI GitHub repository.
You can explore, learn, and contribute to this repository to unleash the full potential of machine learning on Vertex AI!
Explore this repository, follow the links in the header section of each of the notebooks to -
Open and run the notebook in Colab
Open and run the notebook in Colab Enterprise
Open and run the notebook in Vertex AI Workbench
View the notebook on Github
See the Contributing Guide.
To get started using Vertex AI, you must have a Google Cloud project.
- If you don't have a Google Cloud project, you can learn and build on GCP for free using Free Trail.
- Once you have a Google Cloud project, you can learn more about setting up a project and a development environment.
├── notebooks
│ ├── official - Notebooks demonstrating use of each Vertex AI service
│ │ ├── automl
│ │ ├── custom
│ │ ├── ...
│ ├── community - Notebooks contributed by the community
│ │ ├── model_garden
│ │ ├── ...
├── community-content - Sample code and tutorials contributed by the community
Category | Product | Description |
---|---|---|
Model |
Model Garden/
|
Curated collection of first-party, open-source, and third-party models available on Vertex AI including Gemini, Gemma, Llama 3, Claude 3 and many more. |
Data |
Feature Store/
|
Set up and manage online serving using Vertex AI Feature Store. |
datasets/
|
Use BigQuery and Data Labeling service with Vertex AI. | |
Model development |
automl/
|
Train and make predictions on AutoML models |
custom/
|
Create, deploy and serve custom models on Vertex AI | |
ray_on_vertex_ai/
|
Use Colab Enterprise and Vertex AI SDK for Python to connect to the Ray Cluster. | |
Deploy and use |
prediction/
|
Build, train and deploy models using prebuilt containers for custom training and prediction. |
model_registry/
|
Use Model Registry to create and register a model. | |
Explainable AI/
|
Use Vertex Explainable AI's feature-based and example-based explanations to explain how or why a model produced a specific prediction. | |
ml_metadata/
|
Record the metadata and artifacts and query that metadata to help analyze, debug, and audit the performance of your ML system. | |
Tools |
Pipelines/
|
Use `Vertex AI Pipelines` and `Google Cloud Pipeline Components` to build, tune, or deploy a custom model. |
Please use the Issues page to provide feedback or submit a bug report.
This is not an officially supported Google product. The code in this repository is for demonstrative purposes only.
- Vertex AI Jupyter Notebook tutorials
- Vertex AI Generative AI GitHub repository
- Vertex AI documentaton
For Tasks:
Click tags to check more tools for each tasksFor Jobs:
Alternative AI tools for vertex-ai-samples
Similar Open Source Tools
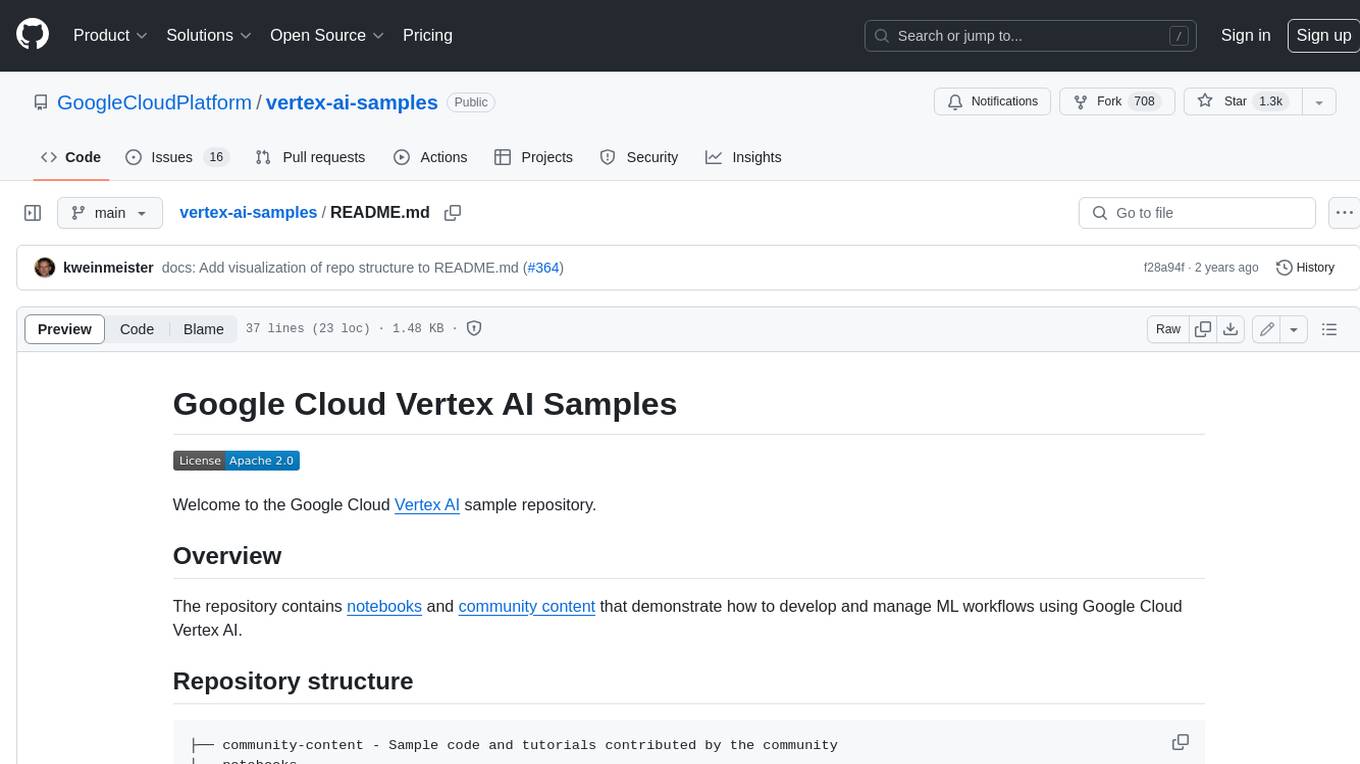
vertex-ai-samples
The Google Cloud Vertex AI sample repository contains notebooks and community content that demonstrate how to develop and manage ML workflows using Google Cloud Vertex AI.
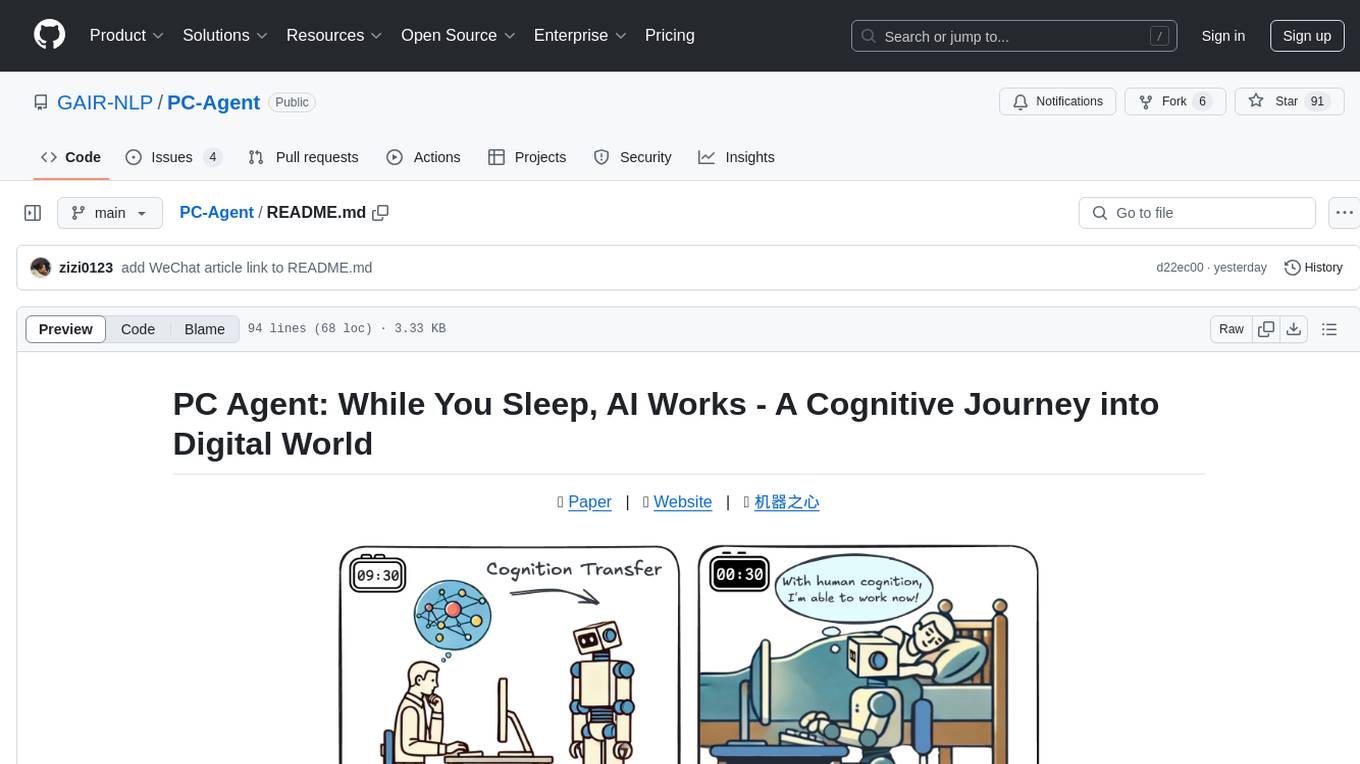
PC-Agent
PC Agent introduces a novel framework to empower autonomous digital agents through human cognition transfer. It consists of PC Tracker for data collection, Cognition Completion for transforming raw data, and a multi-agent system for decision-making and visual grounding. Users can set up the tool in Python environment, customize data collection with PC Tracker, process data into cognitive trajectories, and run the multi-agent system. The tool aims to enable AI to work autonomously while users sleep, providing a cognitive journey into the digital world.
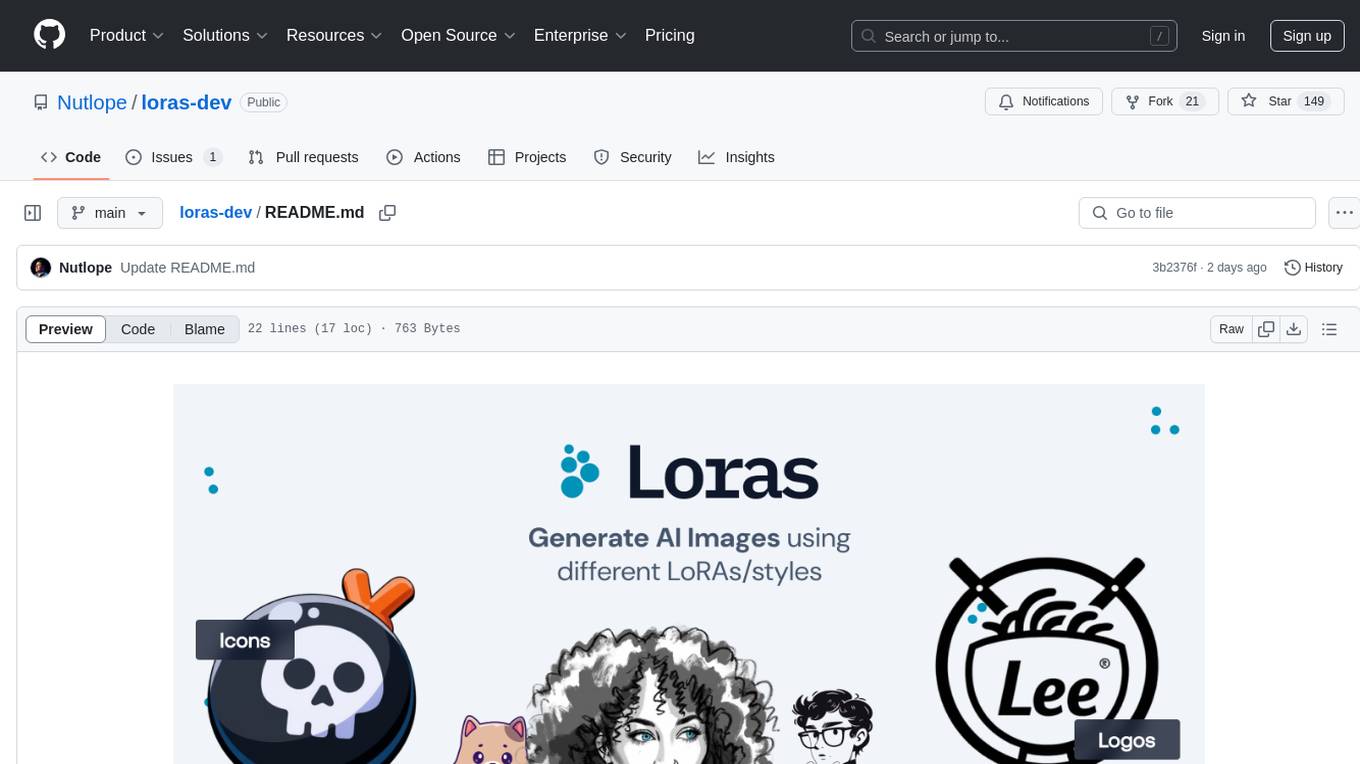
loras-dev
Loras is an open source real-time AI image generator powered by Flux through Together.ai. It utilizes Flux Dev from BFL for the image model, Together AI for inference, Next.js app router with Tailwind, Helicone for observability, and Plausible for website analytics. Users can clone the repository, add their Together AI API key to a .env.local file, install dependencies, and run the tool locally to generate AI images in real-time.
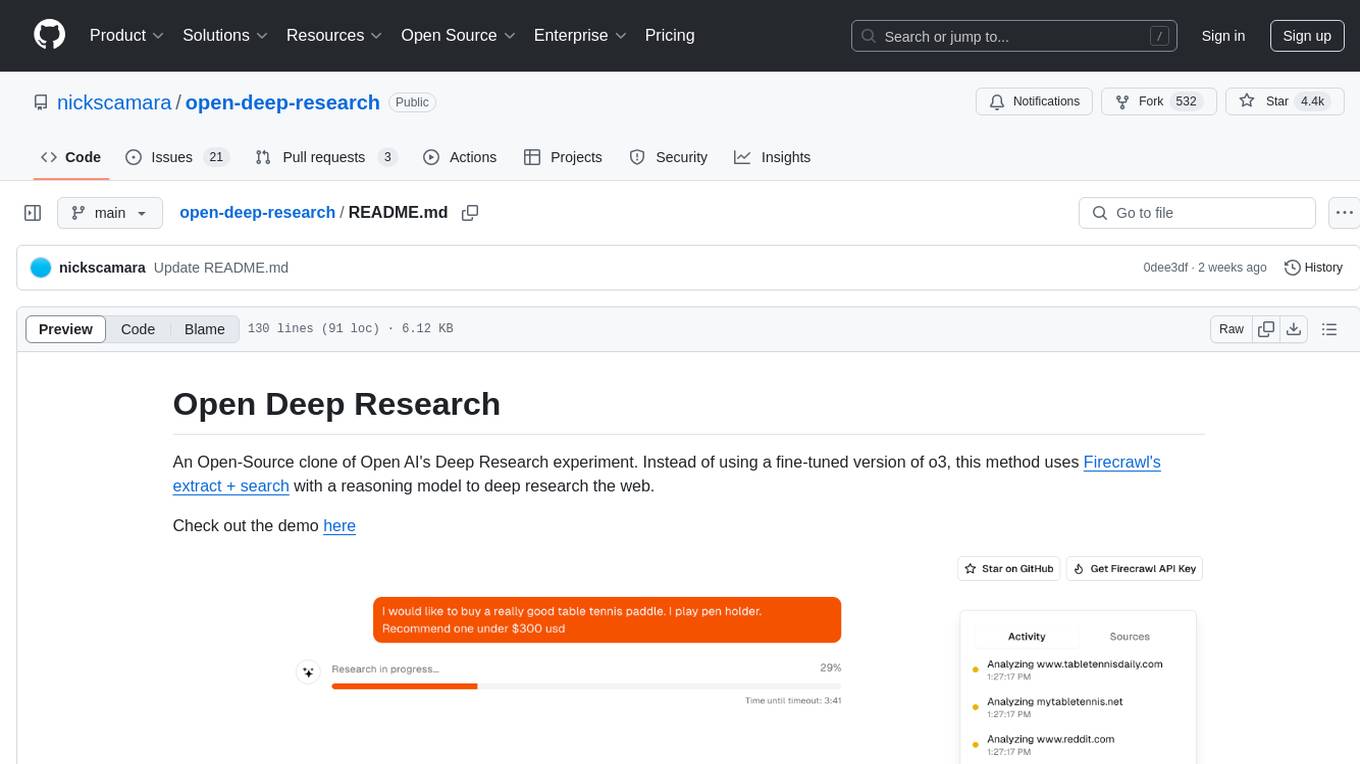
open-deep-research
Open Deep Research is an open-source project that serves as a clone of Open AI's Deep Research experiment. It utilizes Firecrawl's extract and search method along with a reasoning model to conduct in-depth research on the web. The project features Firecrawl Search + Extract, real-time data feeding to AI via search, structured data extraction from multiple websites, Next.js App Router for advanced routing, React Server Components and Server Actions for server-side rendering, AI SDK for generating text and structured objects, support for various model providers, styling with Tailwind CSS, data persistence with Vercel Postgres and Blob, and simple and secure authentication with NextAuth.js.
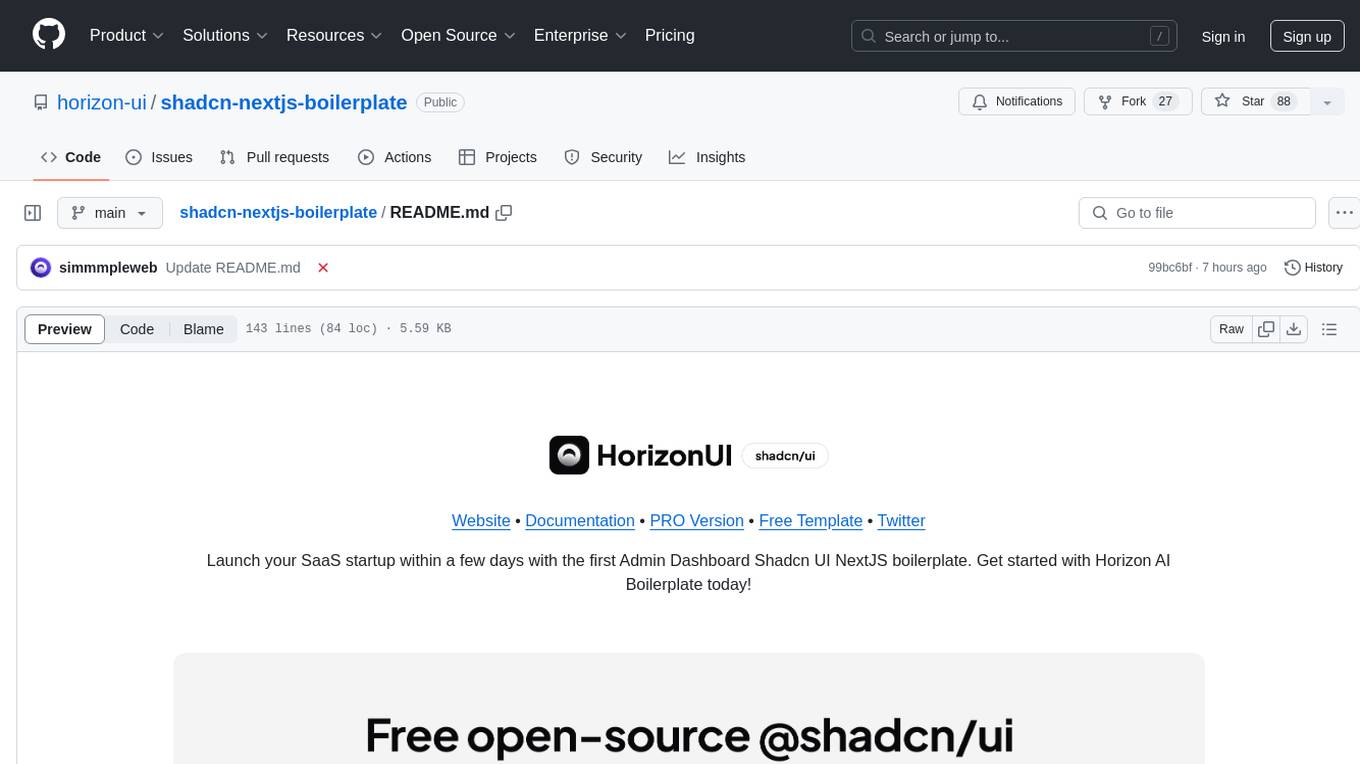
shadcn-nextjs-boilerplate
Horizon AI Boilerplate is an open-source Admin Dashboard template designed for Shadcn UI, NextJS, and Tailwind CSS. It provides over 30 dark/light frontend elements for creating Chat AI SaaS Apps quickly. The documentation is detailed and complex, guiding users through installation and usage. Users can start their local server with simple commands. The tool requires a valid OpenAI API key for ChatGPT functionality. Additionally, a Figma version is available for design purposes. The PRO version offers more components and pages. Users can report issues on GitHub and connect with the community via Discord. The tool credits open-source resources like Shadcn UI Library, NextJS Subscription Payments, and ChatBot UI by mckaywrigley.
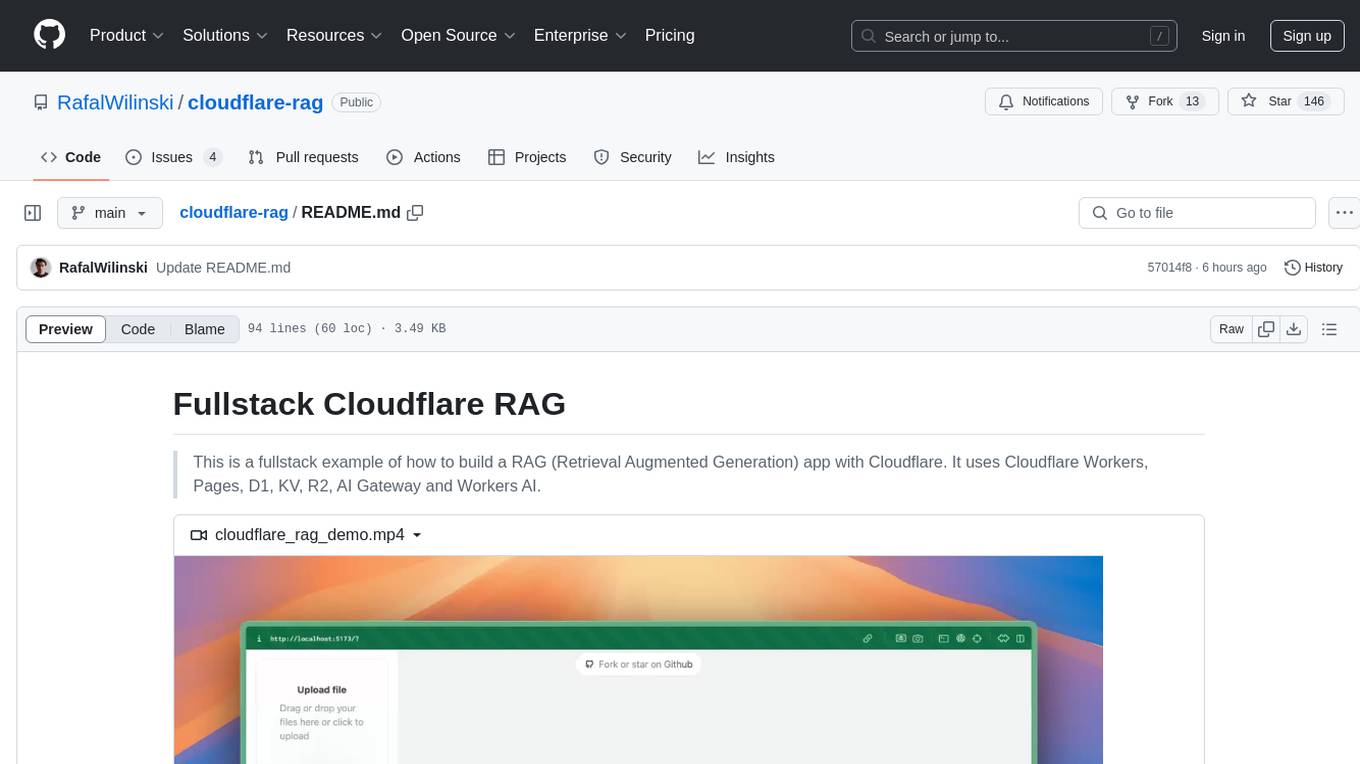
cloudflare-rag
This repository provides a fullstack example of building a Retrieval Augmented Generation (RAG) app with Cloudflare. It utilizes Cloudflare Workers, Pages, D1, KV, R2, AI Gateway, and Workers AI. The app features streaming interactions to the UI, hybrid RAG with Full-Text Search and Vector Search, switchable providers using AI Gateway, per-IP rate limiting with Cloudflare's KV, OCR within Cloudflare Worker, and Smart Placement for workload optimization. The development setup requires Node, pnpm, and wrangler CLI, along with setting up necessary primitives and API keys. Deployment involves setting up secrets and deploying the app to Cloudflare Pages. The project implements a Hybrid Search RAG approach combining Full Text Search against D1 and Hybrid Search with embeddings against Vectorize to enhance context for the LLM.
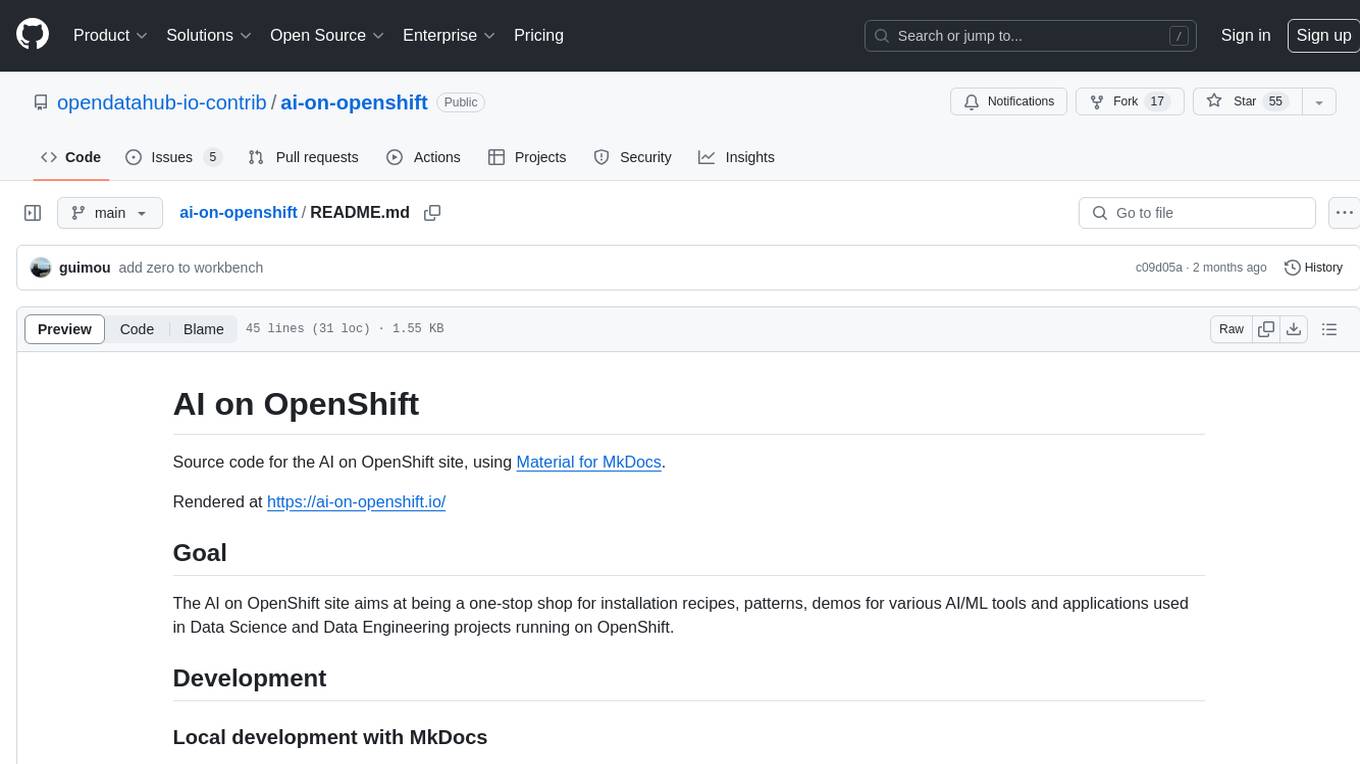
ai-on-openshift
AI on OpenShift is a site providing installation recipes, patterns, and demos for AI/ML tools and applications used in Data Science and Data Engineering projects running on OpenShift. It serves as a comprehensive resource for developers looking to deploy AI solutions on the OpenShift platform.
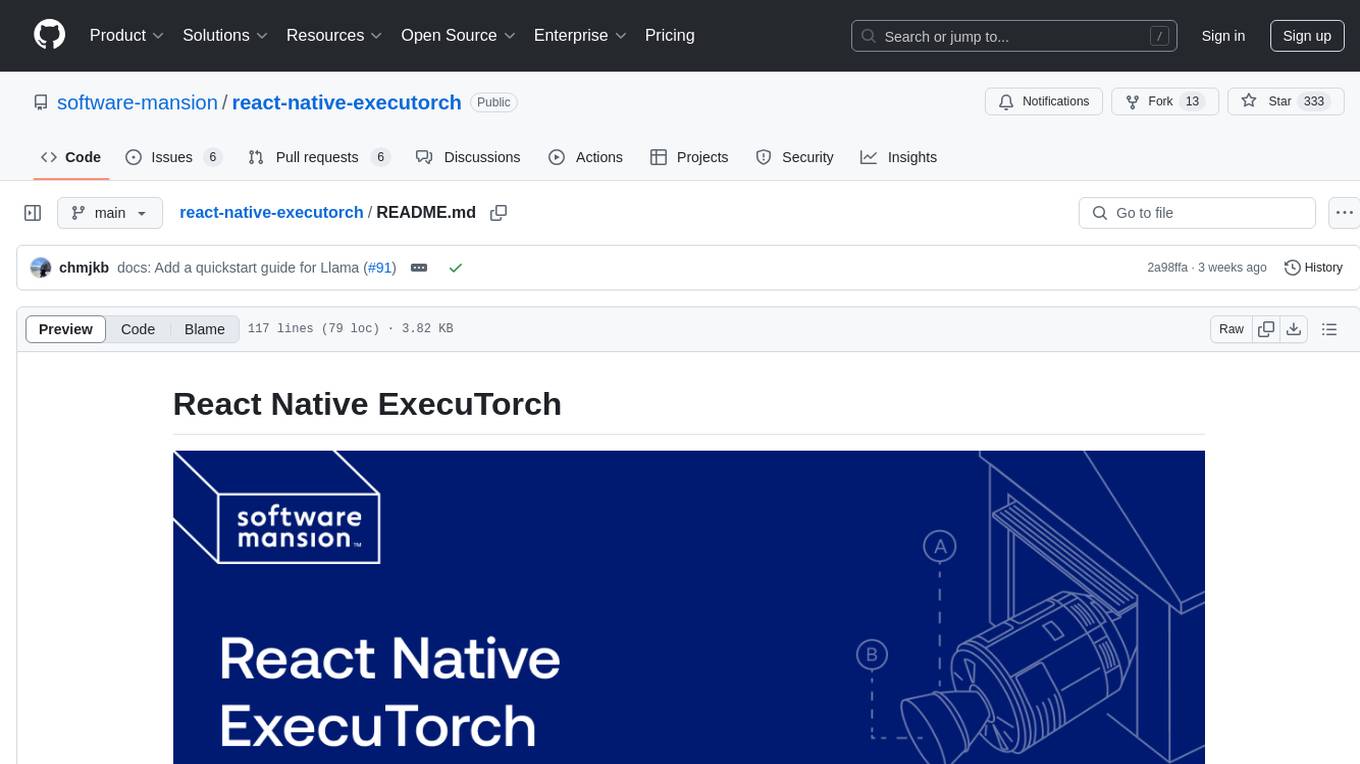
react-native-executorch
React Native ExecuTorch is a framework that allows developers to run AI models on mobile devices using React Native. It bridges the gap between React Native and native platform capabilities, providing high-performance AI model execution without requiring deep knowledge of native code or machine learning internals. The tool supports ready-made models in `.pte` format and offers a Python API for custom models. It is designed to simplify the integration of AI features into React Native apps.
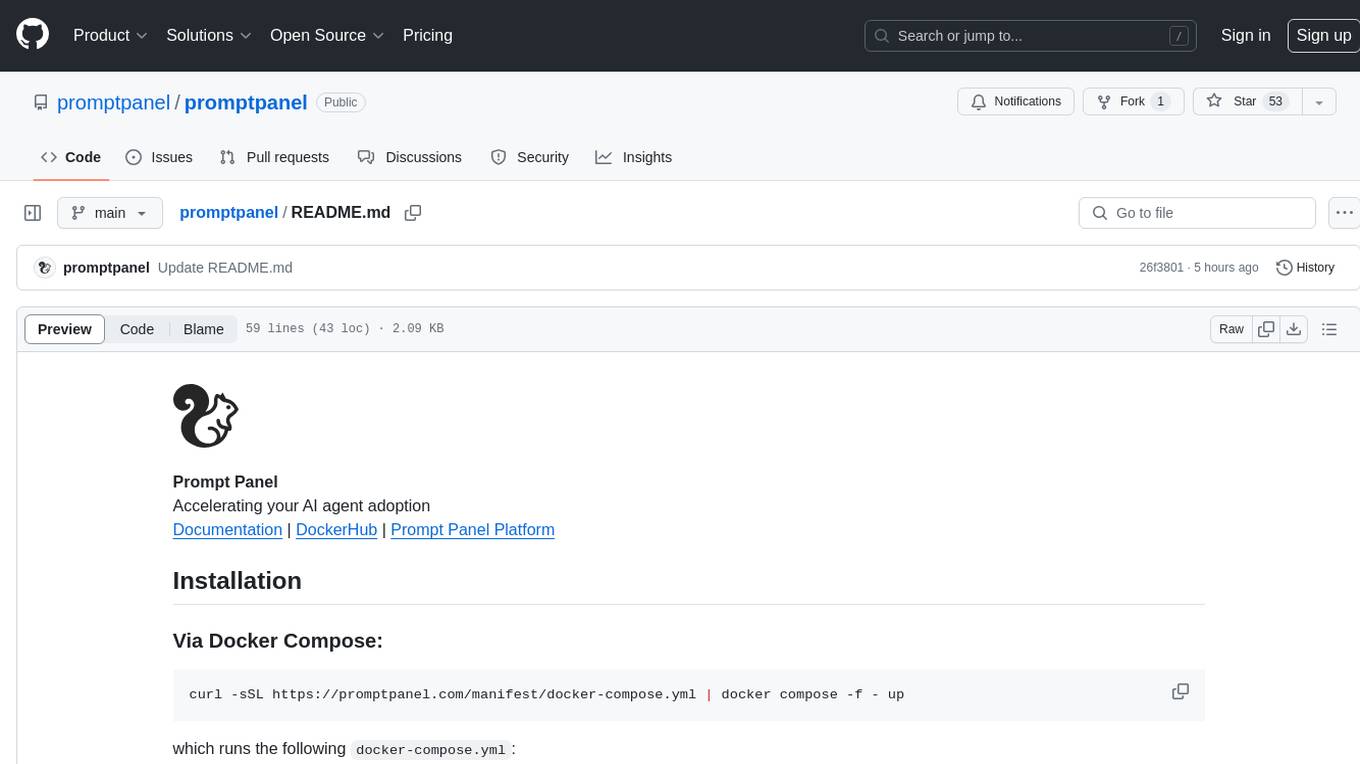
promptpanel
Prompt Panel is a tool designed to accelerate the adoption of AI agents by providing a platform where users can run large language models across any inference provider, create custom agent plugins, and use their own data safely. The tool allows users to break free from walled-gardens and have full control over their models, conversations, and logic. With Prompt Panel, users can pair their data with any language model, online or offline, and customize the system to meet their unique business needs without any restrictions.
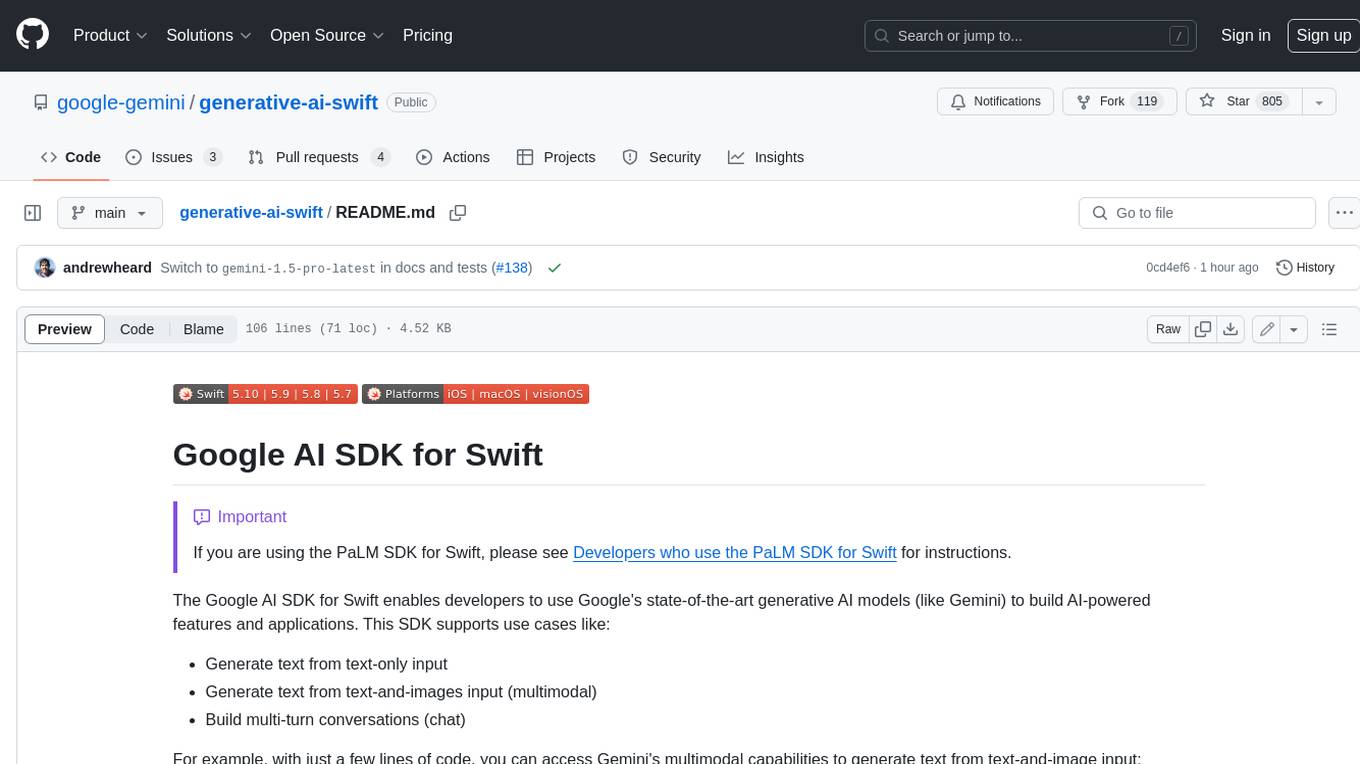
generative-ai-swift
The Google AI SDK for Swift enables developers to use Google's state-of-the-art generative AI models (like Gemini) to build AI-powered features and applications. This SDK supports use cases like: - Generate text from text-only input - Generate text from text-and-images input (multimodal) - Build multi-turn conversations (chat)
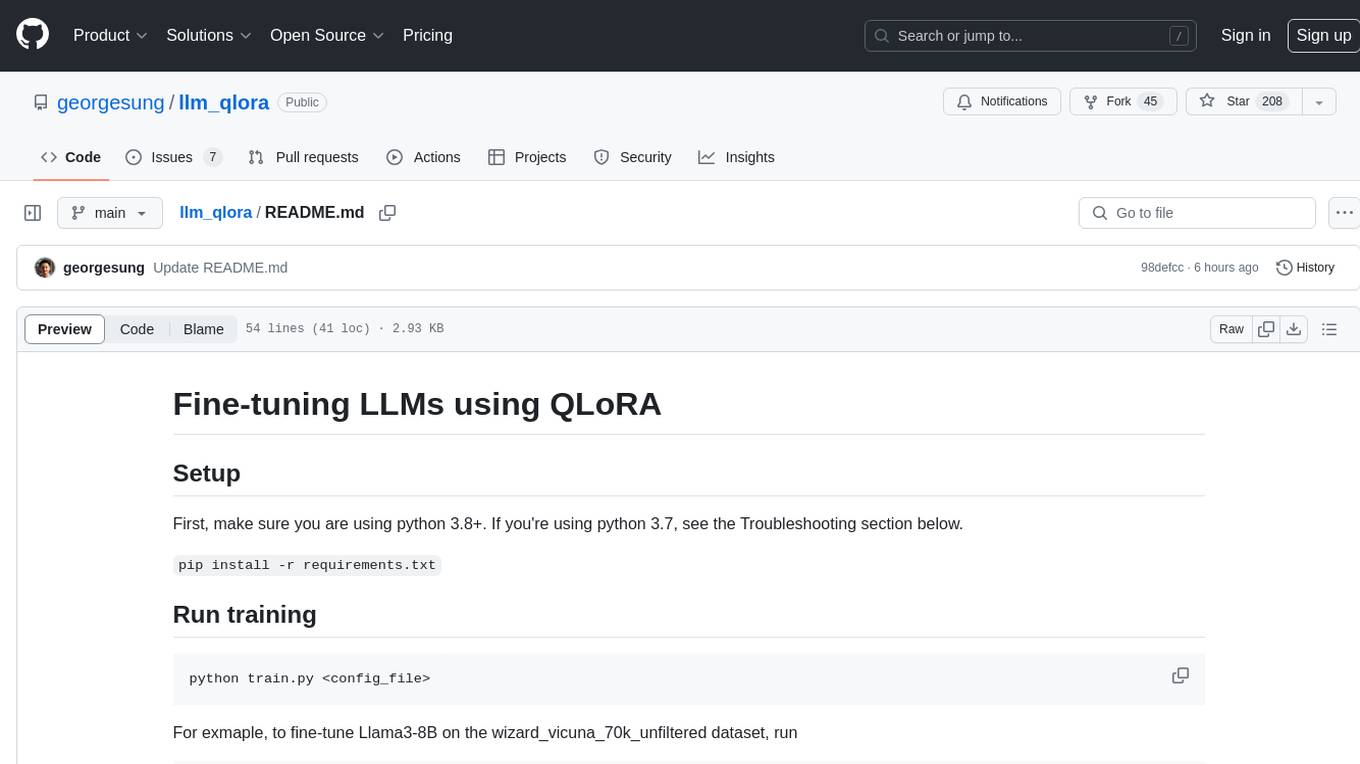
llm_qlora
LLM_QLoRA is a repository for fine-tuning Large Language Models (LLMs) using QLoRA methodology. It provides scripts for training LLMs on custom datasets, pushing models to HuggingFace Hub, and performing inference. Additionally, it includes models trained on HuggingFace Hub, a blog post detailing the QLoRA fine-tuning process, and instructions for converting and quantizing models. The repository also addresses troubleshooting issues related to Python versions and dependencies.
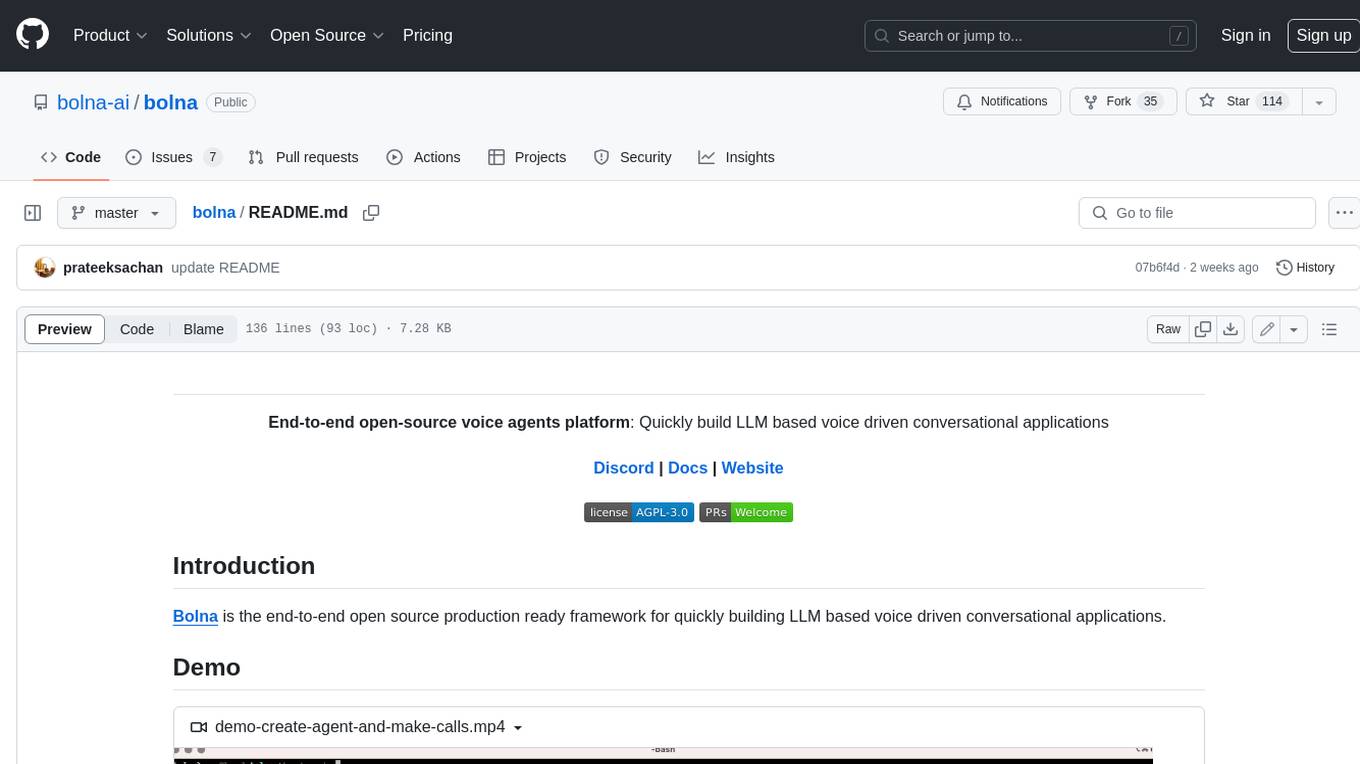
bolna
Bolna is an open-source platform for building voice-driven conversational applications using large language models (LLMs). It provides a comprehensive set of tools and integrations to handle various aspects of voice-based interactions, including telephony, transcription, LLM-based conversation handling, and text-to-speech synthesis. Bolna simplifies the process of creating voice agents that can perform tasks such as initiating phone calls, transcribing conversations, generating LLM-powered responses, and synthesizing speech. It supports multiple providers for each component, allowing users to customize their setup based on their specific needs. Bolna is designed to be easy to use, with a straightforward local setup process and well-documented APIs. It is also extensible, enabling users to integrate with other telephony providers or add custom functionality.
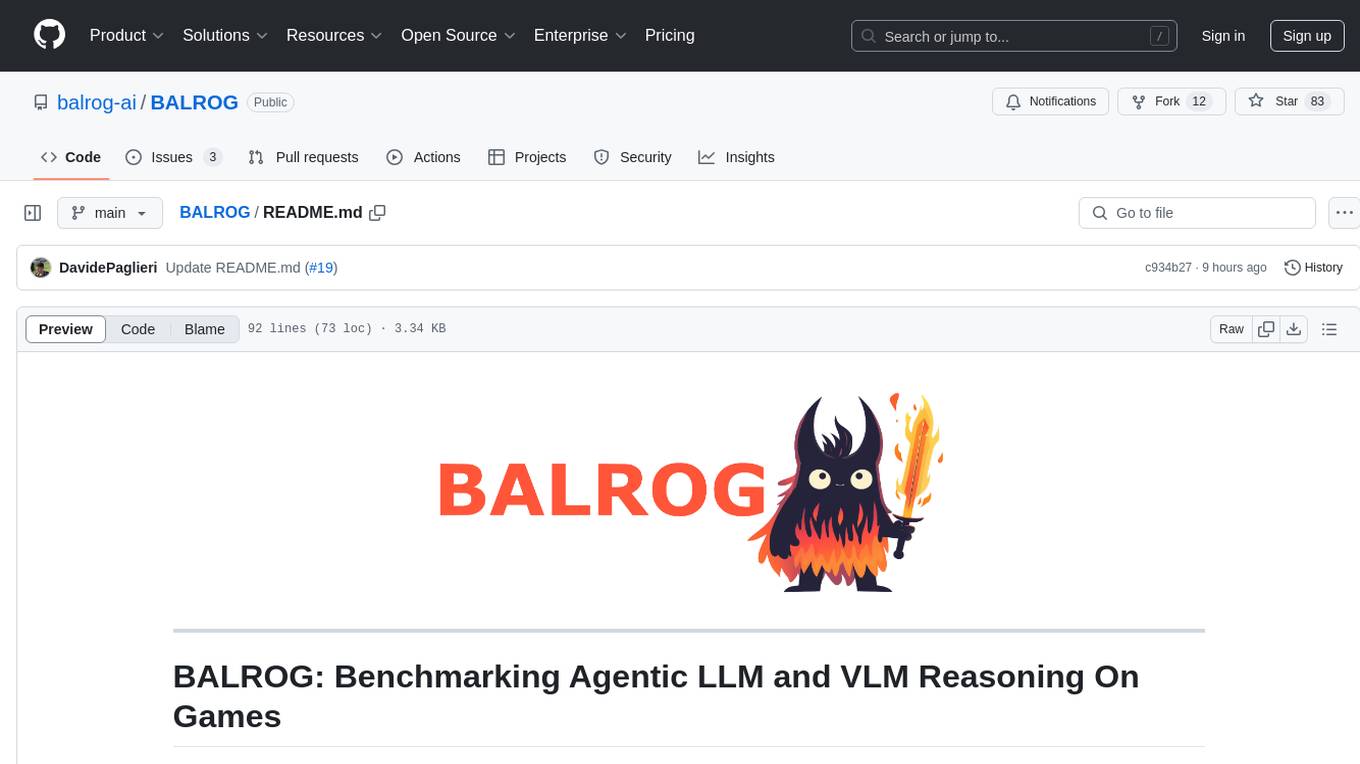
BALROG
BALROG is a benchmark tool designed to evaluate agentic Long-Longitudinal Memory (LLM) and Vision-Language Memory (VLM) capabilities using reinforcement learning environments. It provides a comprehensive assessment of agentic abilities, supports both language and vision-language models, integrates with popular AI APIs, and allows for easy integration of custom agents, new environments, and models.
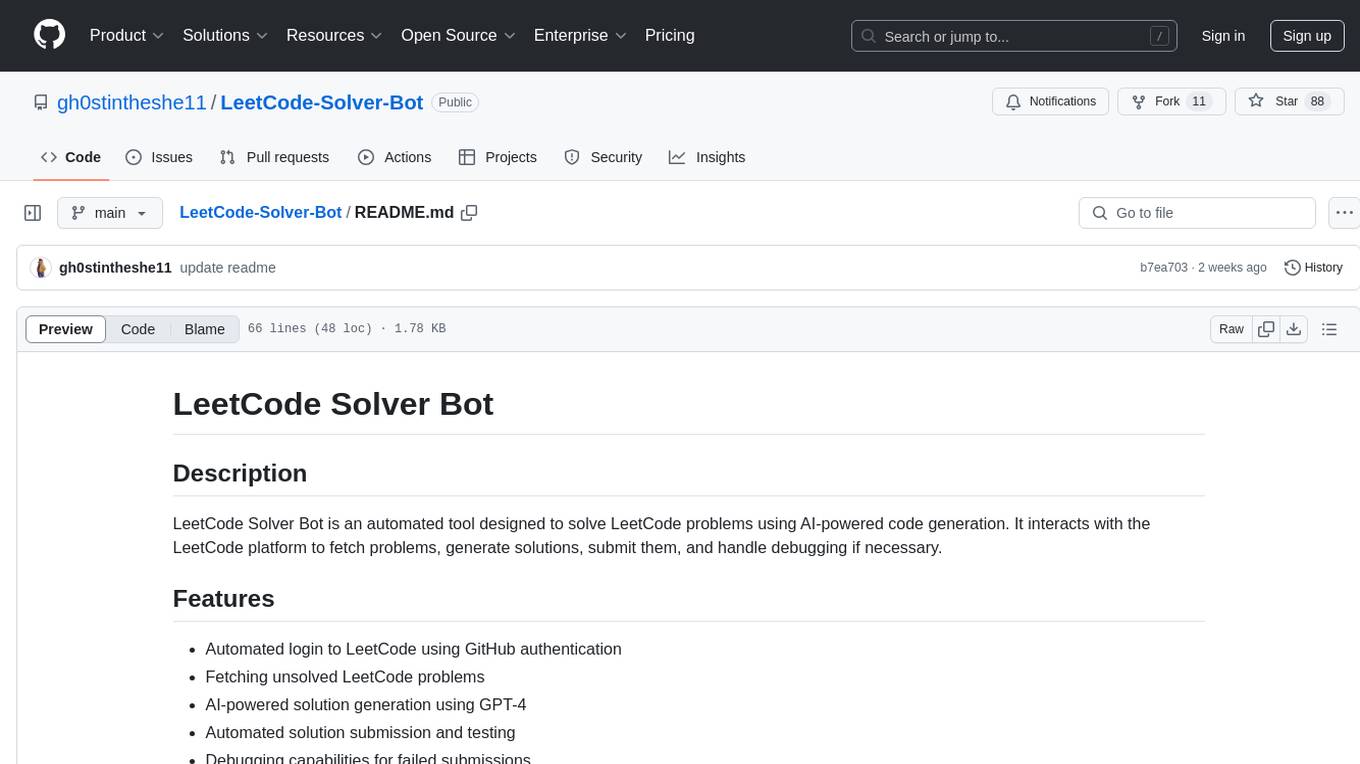
LeetCode-Solver-Bot
LeetCode Solver Bot is an automated tool designed to solve LeetCode problems using AI-powered code generation. It interacts with the LeetCode platform to fetch problems, generate solutions, submit them, and handle debugging if necessary. The tool supports automated login using GitHub authentication, fetching unsolved problems, AI-powered solution generation with GPT-4, automated solution submission and testing, debugging capabilities for failed submissions, and currently focuses on Python programming language.
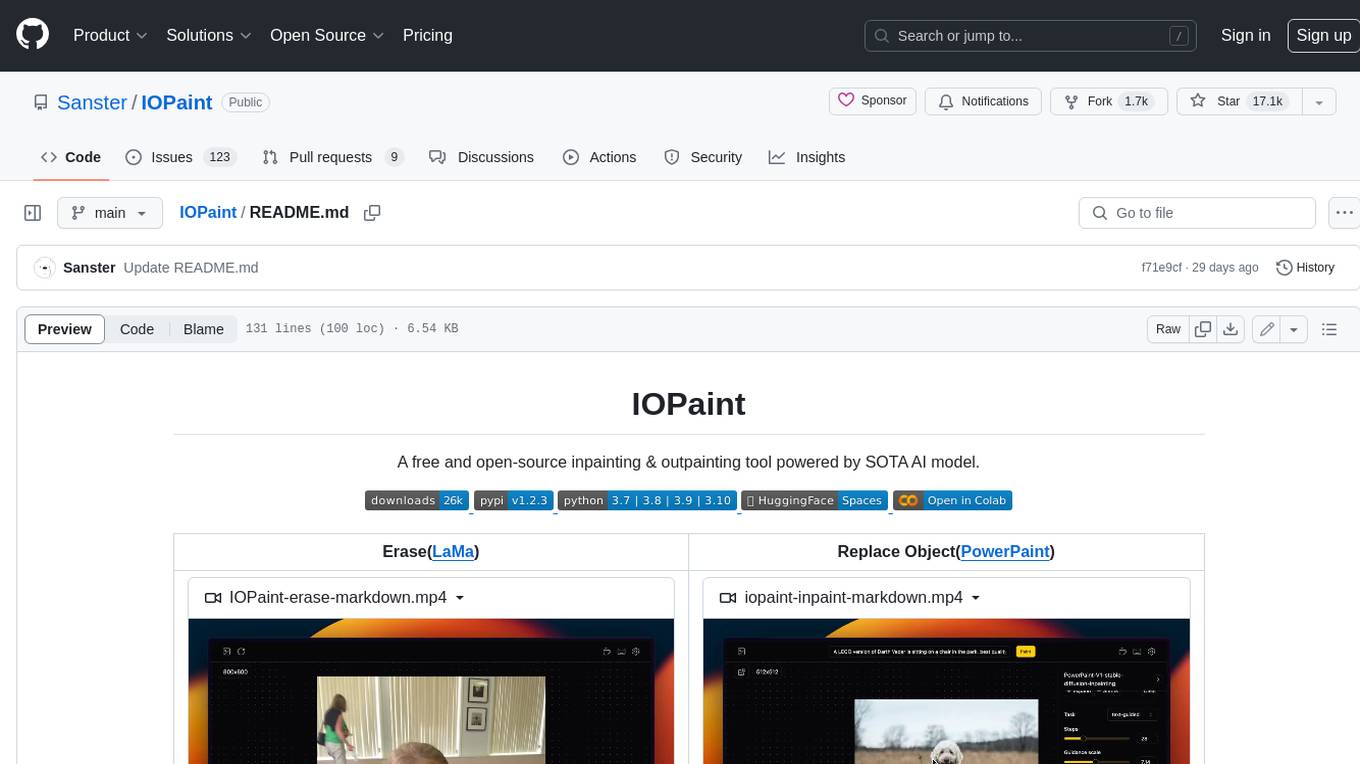
IOPaint
IOPaint is a free and open-source inpainting & outpainting tool powered by SOTA AI model. It supports various AI models to perform erase, inpainting, or outpainting tasks. Users can remove unwanted objects, defects, watermarks, or people from images using erase models. Additionally, diffusion models can replace objects or perform outpainting. The tool also offers plugins for interactive object segmentation, background removal, anime segmentation, super resolution, face restoration, and file management. IOPaint provides a web UI for easy access to the latest AI models and supports batch processing of images through the command line. Developers can contribute to the project by installing front-end dependencies, setting up the backend, and starting the development environment for both front-end and back-end components.
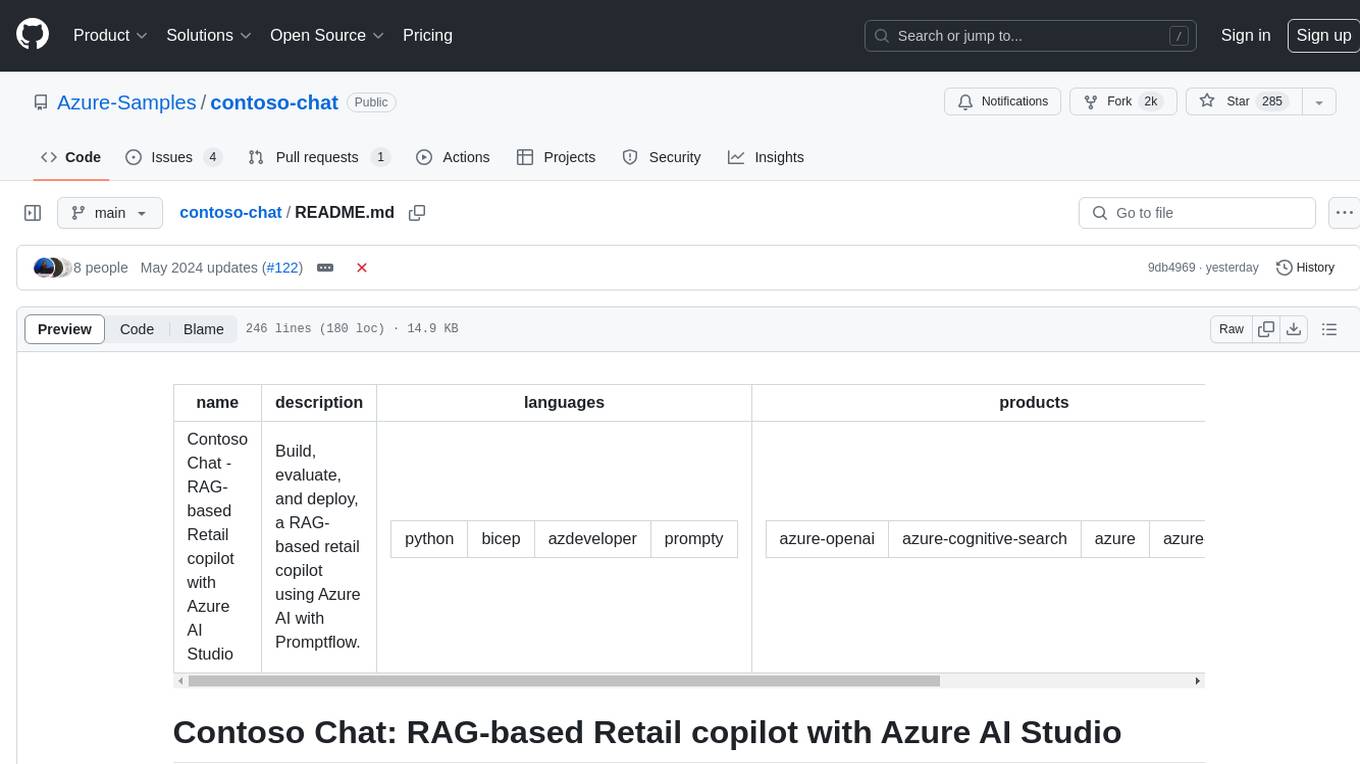
contoso-chat
Contoso Chat is a Python sample demonstrating how to build, evaluate, and deploy a retail copilot application with Azure AI Studio using Promptflow with Prompty assets. The sample implements a Retrieval Augmented Generation approach to answer customer queries based on the company's product catalog and customer purchase history. It utilizes Azure AI Search, Azure Cosmos DB, Azure OpenAI, text-embeddings-ada-002, and GPT models for vectorizing user queries, AI-assisted evaluation, and generating chat responses. By exploring this sample, users can learn to build a retail copilot application, define prompts using Prompty, design, run & evaluate a copilot using Promptflow, provision and deploy the solution to Azure using the Azure Developer CLI, and understand Responsible AI practices for evaluation and content safety.
For similar tasks
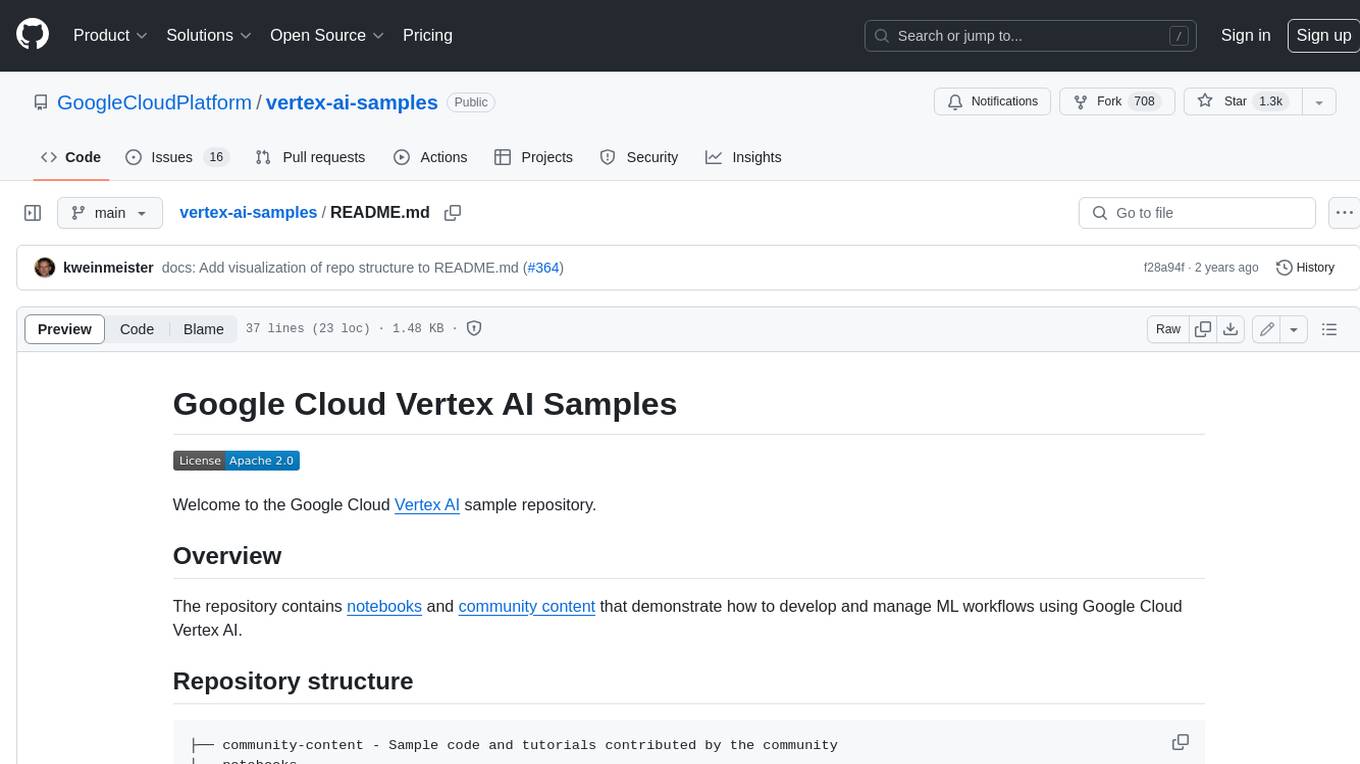
vertex-ai-samples
The Google Cloud Vertex AI sample repository contains notebooks and community content that demonstrate how to develop and manage ML workflows using Google Cloud Vertex AI.
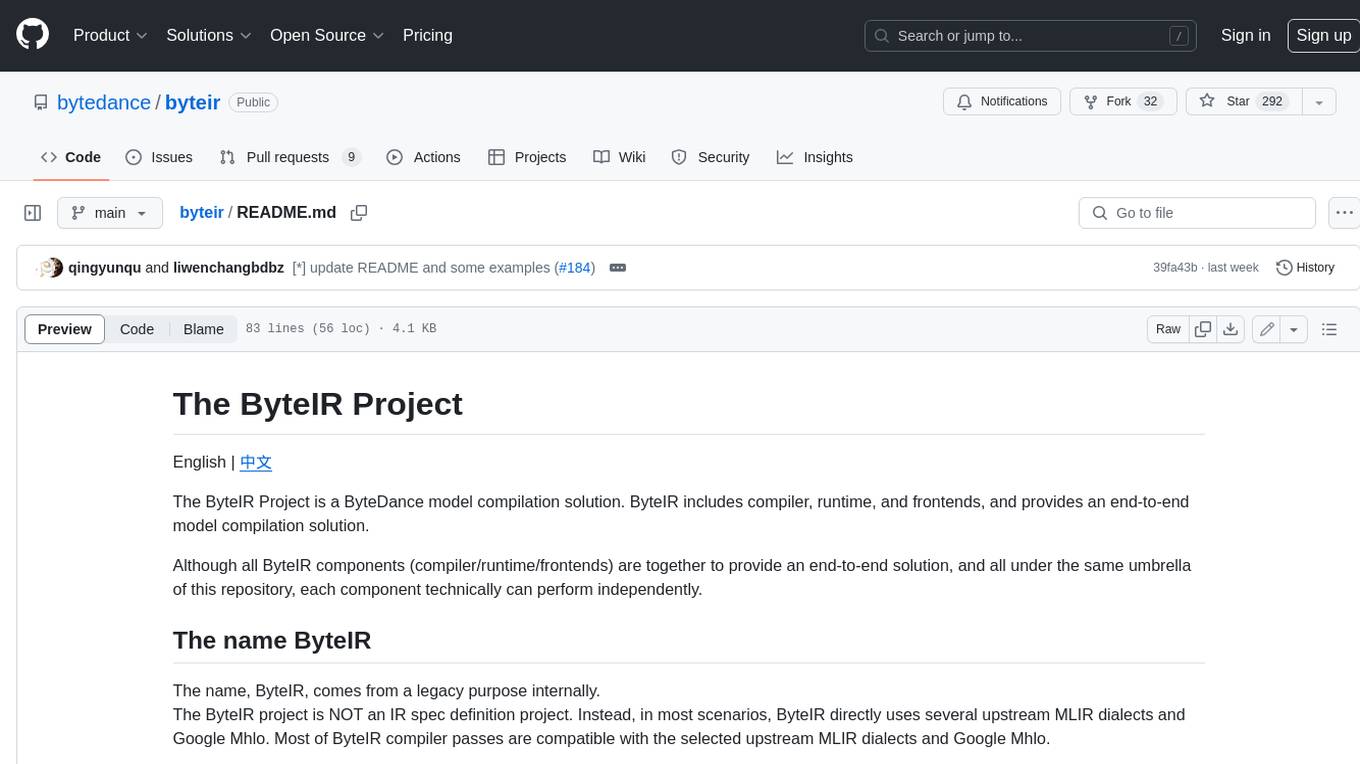
byteir
The ByteIR Project is a ByteDance model compilation solution. ByteIR includes compiler, runtime, and frontends, and provides an end-to-end model compilation solution. Although all ByteIR components (compiler/runtime/frontends) are together to provide an end-to-end solution, and all under the same umbrella of this repository, each component technically can perform independently. The name, ByteIR, comes from a legacy purpose internally. The ByteIR project is NOT an IR spec definition project. Instead, in most scenarios, ByteIR directly uses several upstream MLIR dialects and Google Mhlo. Most of ByteIR compiler passes are compatible with the selected upstream MLIR dialects and Google Mhlo.
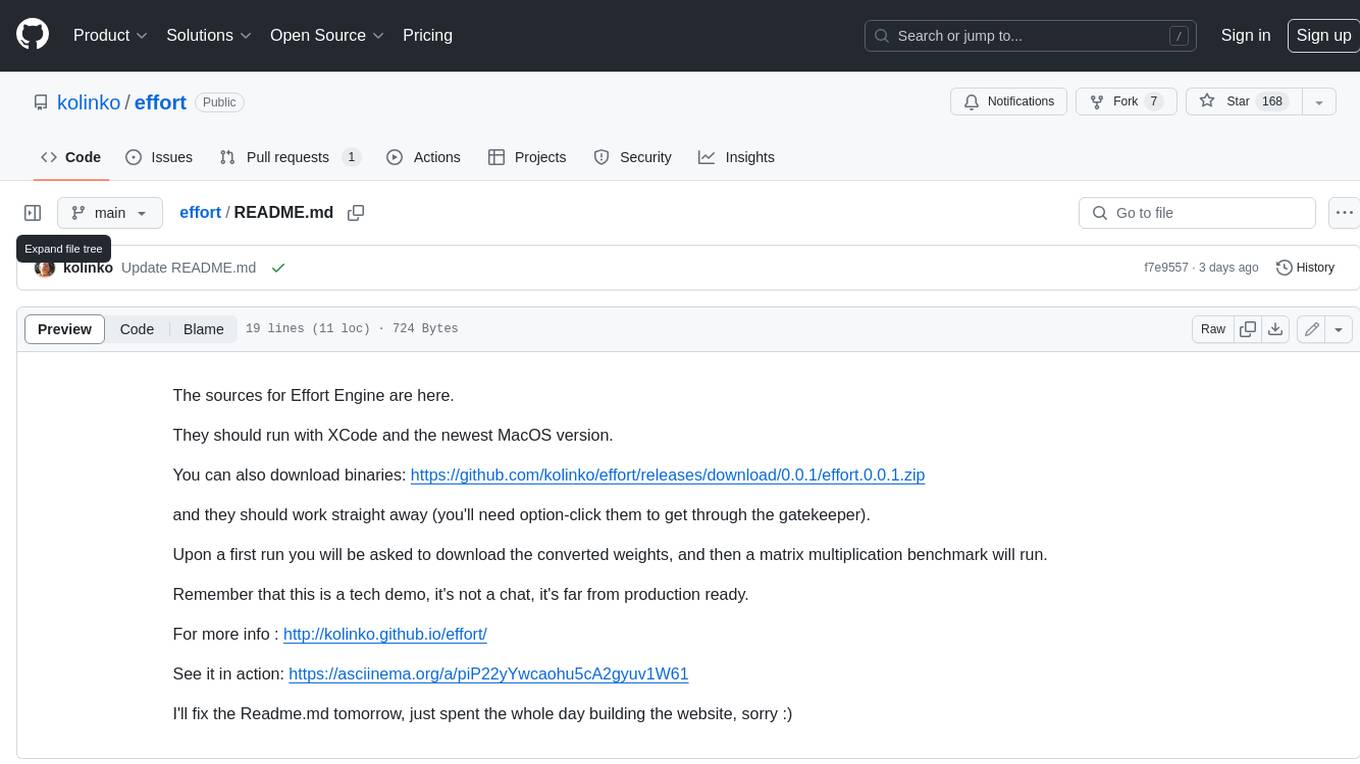
effort
Effort is an example implementation of the bucketMul algorithm, which allows for real-time adjustment of the number of calculations performed during inference of an LLM model. At 50% effort, it performs as fast as regular matrix multiplications on Apple Silicon chips; at 25% effort, it is twice as fast while still retaining most of the quality. Additionally, users have the option to skip loading the least important weights.
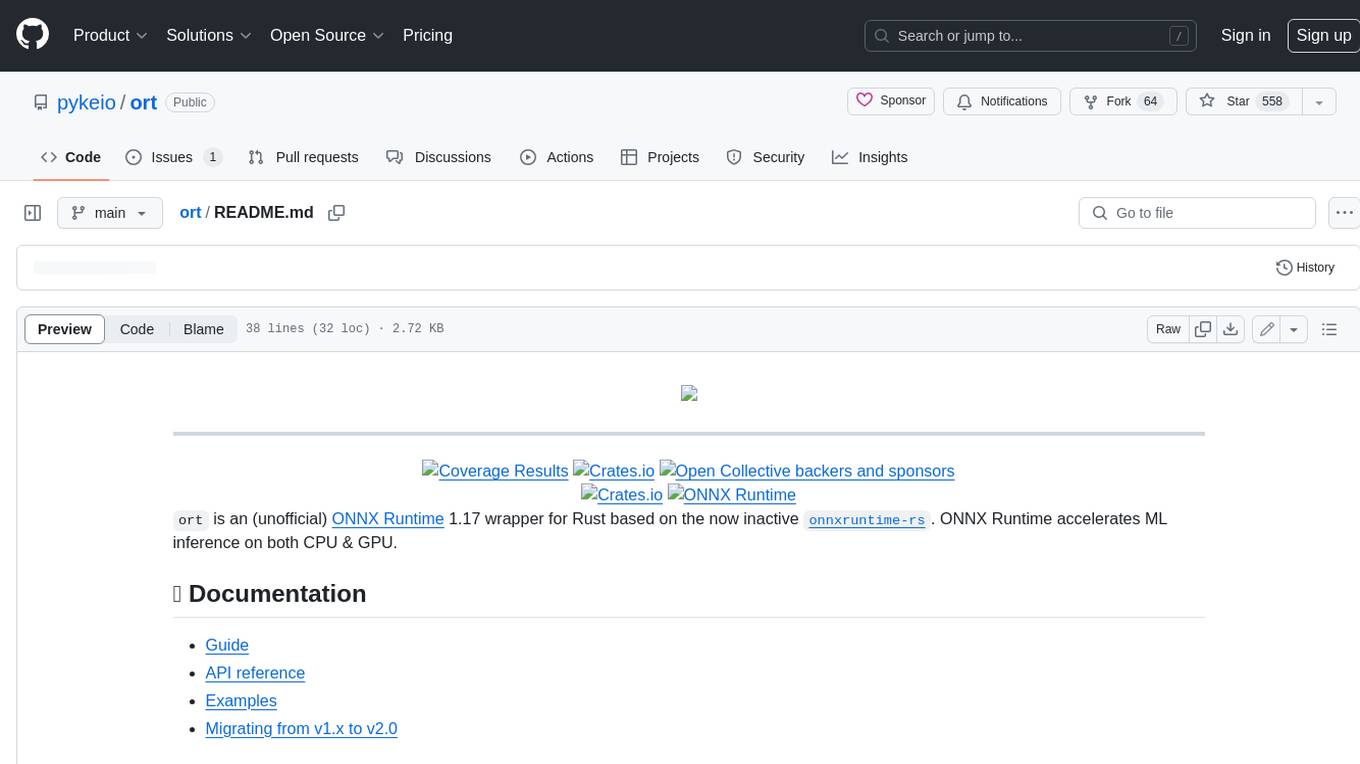
ort
Ort is an unofficial ONNX Runtime 1.17 wrapper for Rust based on the now inactive onnxruntime-rs. ONNX Runtime accelerates ML inference on both CPU and GPU.
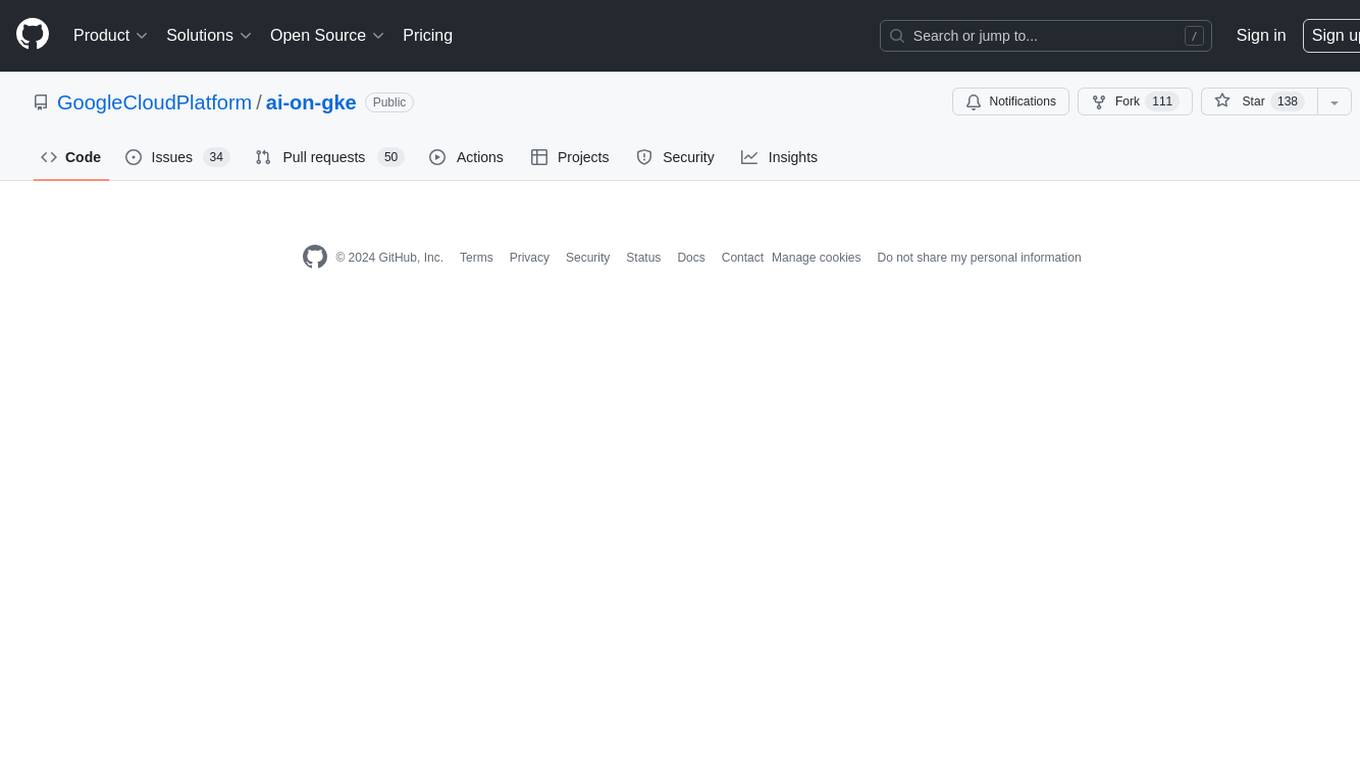
ai-on-gke
This repository contains assets related to AI/ML workloads on Google Kubernetes Engine (GKE). Run optimized AI/ML workloads with Google Kubernetes Engine (GKE) platform orchestration capabilities. A robust AI/ML platform considers the following layers: Infrastructure orchestration that support GPUs and TPUs for training and serving workloads at scale Flexible integration with distributed computing and data processing frameworks Support for multiple teams on the same infrastructure to maximize utilization of resources
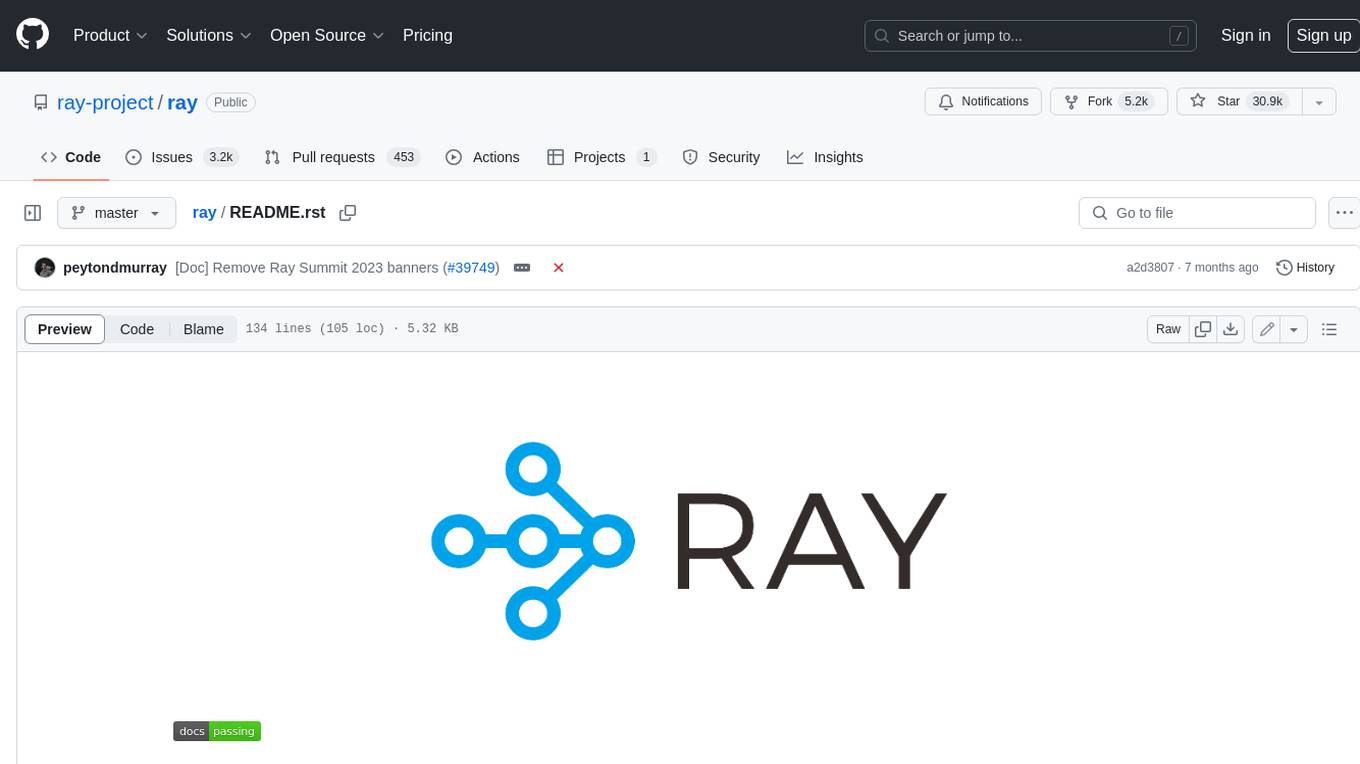
ray
Ray is a unified framework for scaling AI and Python applications. It consists of a core distributed runtime and a set of AI libraries for simplifying ML compute, including Data, Train, Tune, RLlib, and Serve. Ray runs on any machine, cluster, cloud provider, and Kubernetes, and features a growing ecosystem of community integrations. With Ray, you can seamlessly scale the same code from a laptop to a cluster, making it easy to meet the compute-intensive demands of modern ML workloads.
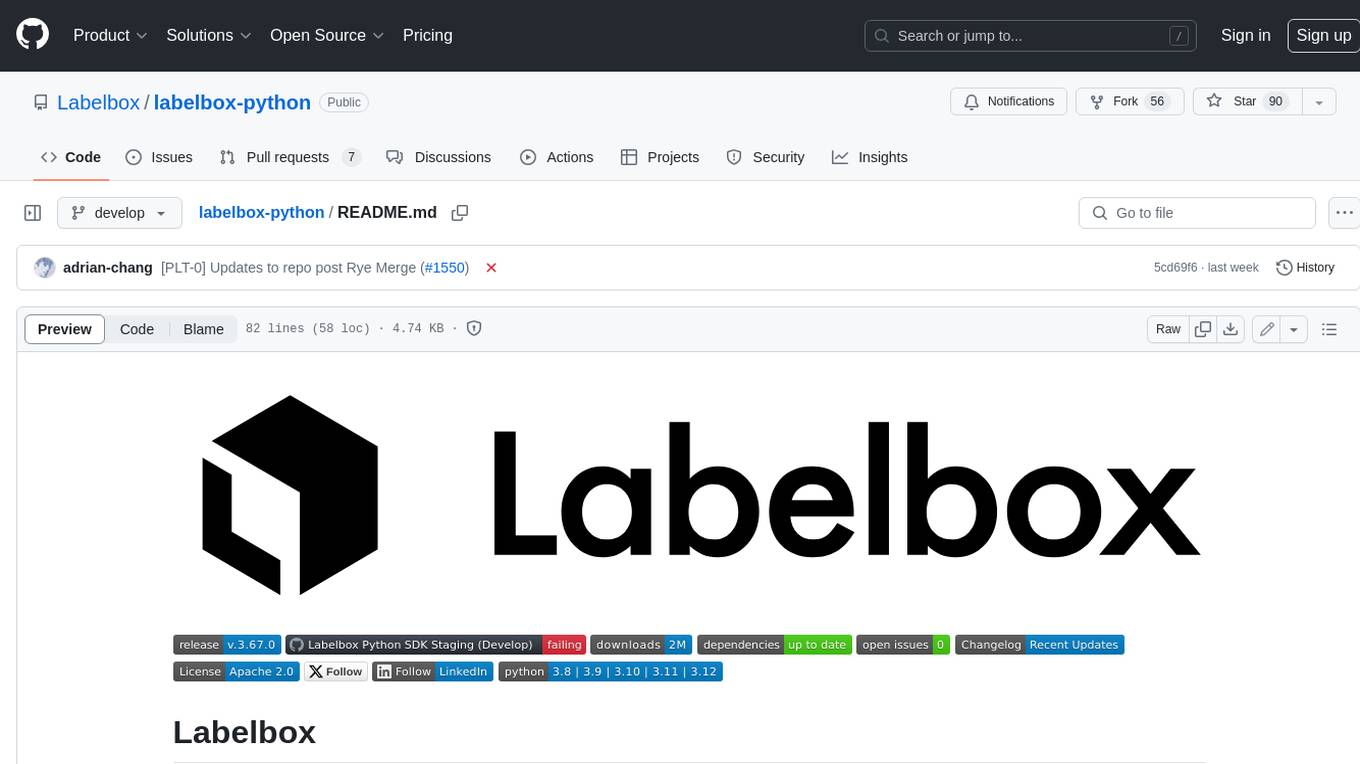
labelbox-python
Labelbox is a data-centric AI platform for enterprises to develop, optimize, and use AI to solve problems and power new products and services. Enterprises use Labelbox to curate data, generate high-quality human feedback data for computer vision and LLMs, evaluate model performance, and automate tasks by combining AI and human-centric workflows. The academic & research community uses Labelbox for cutting-edge AI research.
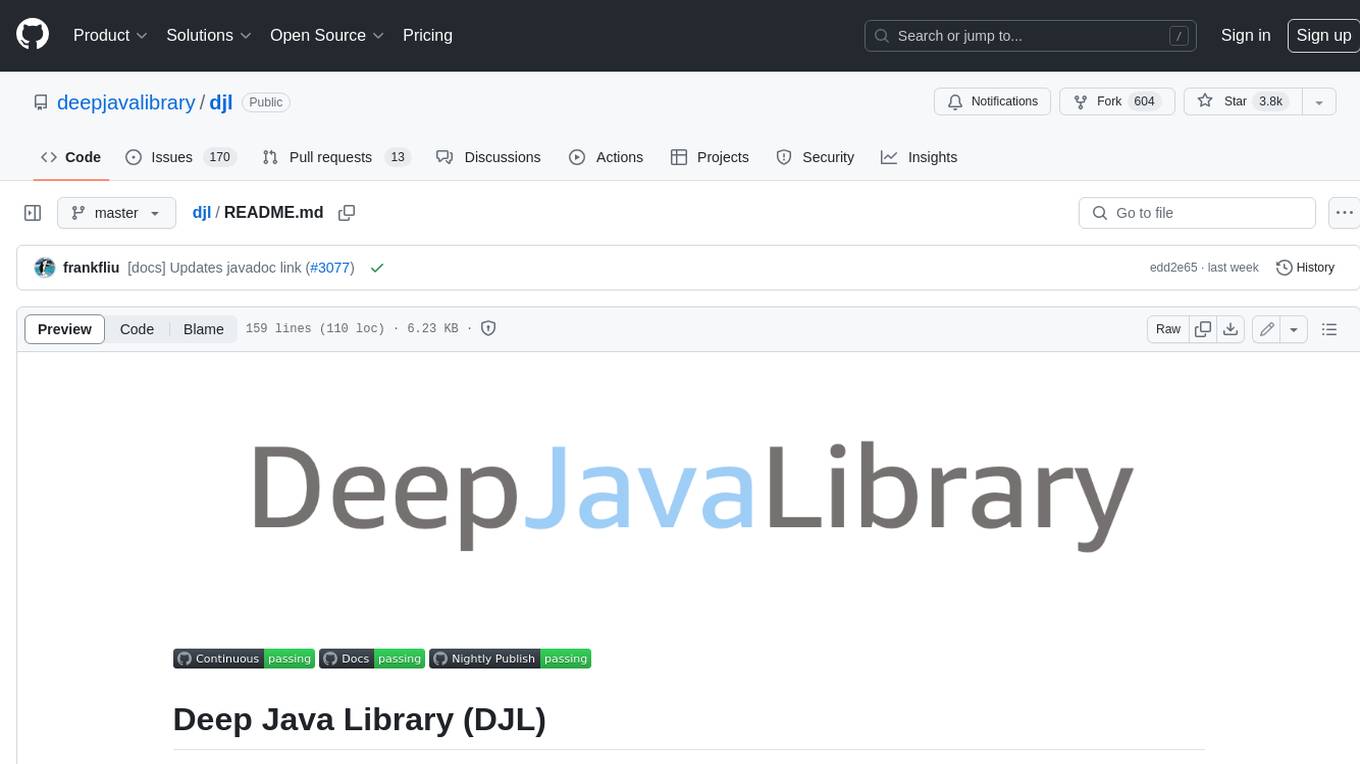
djl
Deep Java Library (DJL) is an open-source, high-level, engine-agnostic Java framework for deep learning. It is designed to be easy to get started with and simple to use for Java developers. DJL provides a native Java development experience and allows users to integrate machine learning and deep learning models with their Java applications. The framework is deep learning engine agnostic, enabling users to switch engines at any point for optimal performance. DJL's ergonomic API interface guides users with best practices to accomplish deep learning tasks, such as running inference and training neural networks.
For similar jobs
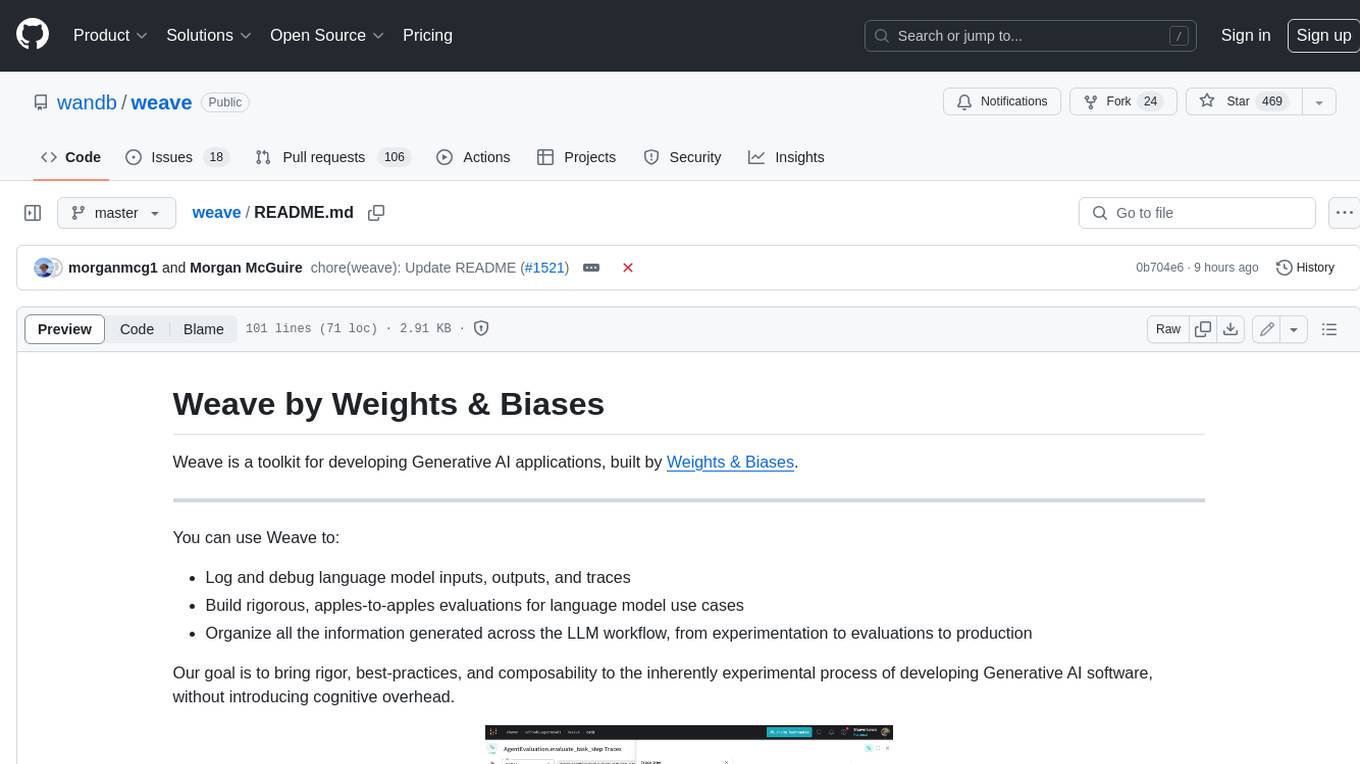
weave
Weave is a toolkit for developing Generative AI applications, built by Weights & Biases. With Weave, you can log and debug language model inputs, outputs, and traces; build rigorous, apples-to-apples evaluations for language model use cases; and organize all the information generated across the LLM workflow, from experimentation to evaluations to production. Weave aims to bring rigor, best-practices, and composability to the inherently experimental process of developing Generative AI software, without introducing cognitive overhead.
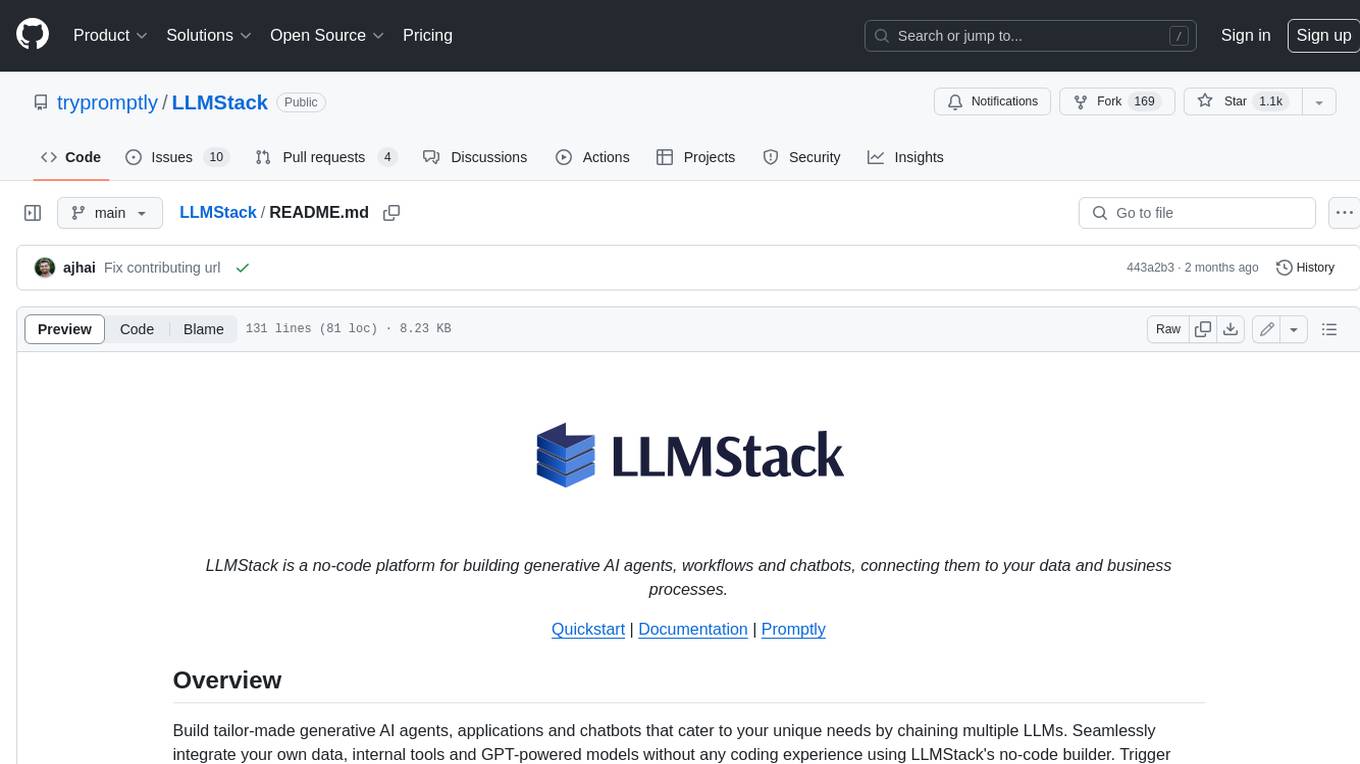
LLMStack
LLMStack is a no-code platform for building generative AI agents, workflows, and chatbots. It allows users to connect their own data, internal tools, and GPT-powered models without any coding experience. LLMStack can be deployed to the cloud or on-premise and can be accessed via HTTP API or triggered from Slack or Discord.
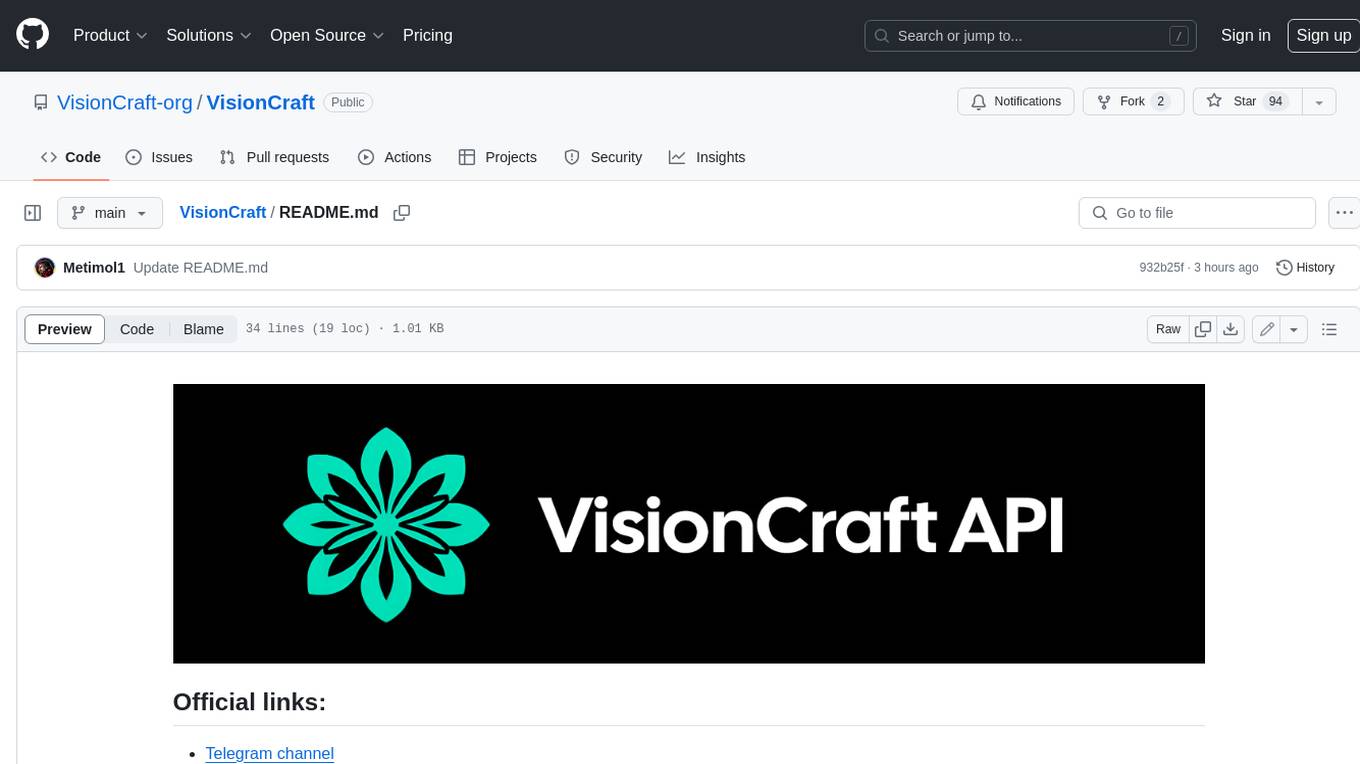
VisionCraft
The VisionCraft API is a free API for using over 100 different AI models. From images to sound.
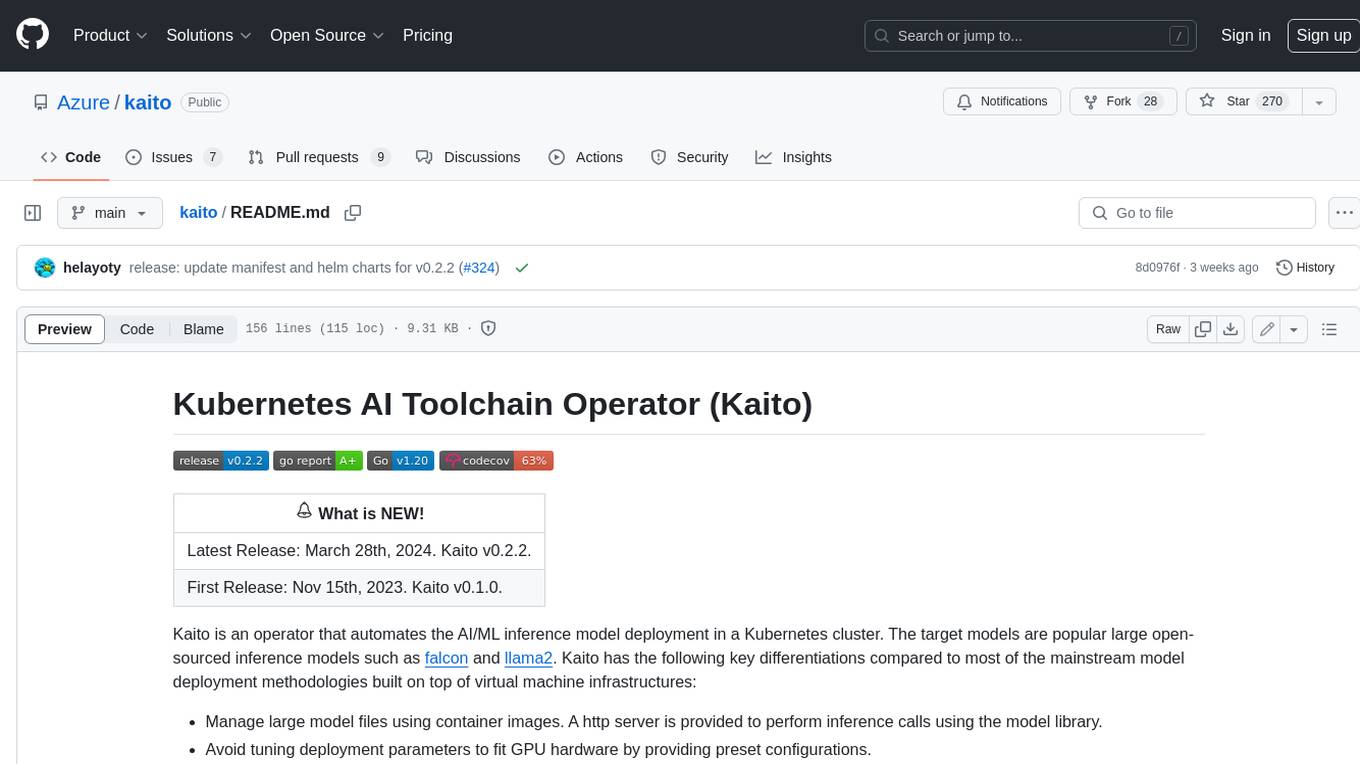
kaito
Kaito is an operator that automates the AI/ML inference model deployment in a Kubernetes cluster. It manages large model files using container images, avoids tuning deployment parameters to fit GPU hardware by providing preset configurations, auto-provisions GPU nodes based on model requirements, and hosts large model images in the public Microsoft Container Registry (MCR) if the license allows. Using Kaito, the workflow of onboarding large AI inference models in Kubernetes is largely simplified.
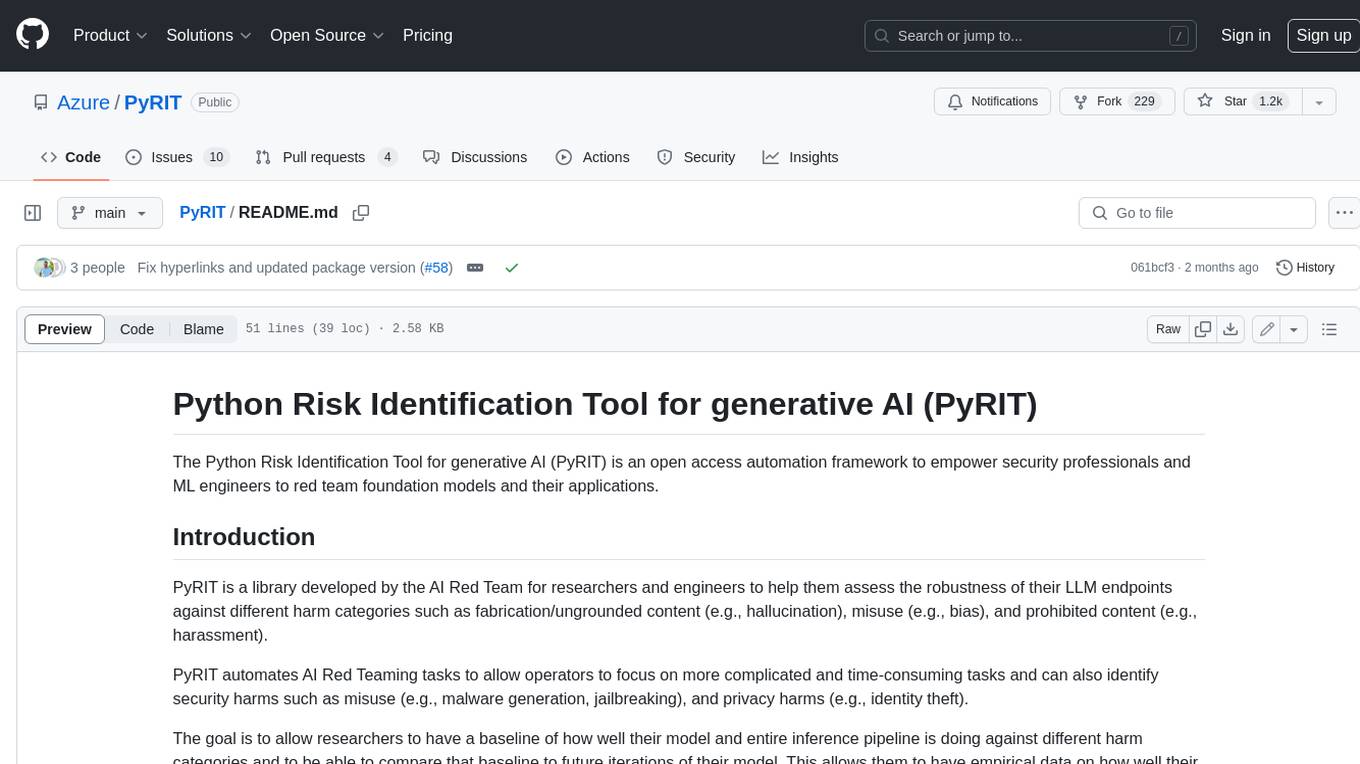
PyRIT
PyRIT is an open access automation framework designed to empower security professionals and ML engineers to red team foundation models and their applications. It automates AI Red Teaming tasks to allow operators to focus on more complicated and time-consuming tasks and can also identify security harms such as misuse (e.g., malware generation, jailbreaking), and privacy harms (e.g., identity theft). The goal is to allow researchers to have a baseline of how well their model and entire inference pipeline is doing against different harm categories and to be able to compare that baseline to future iterations of their model. This allows them to have empirical data on how well their model is doing today, and detect any degradation of performance based on future improvements.
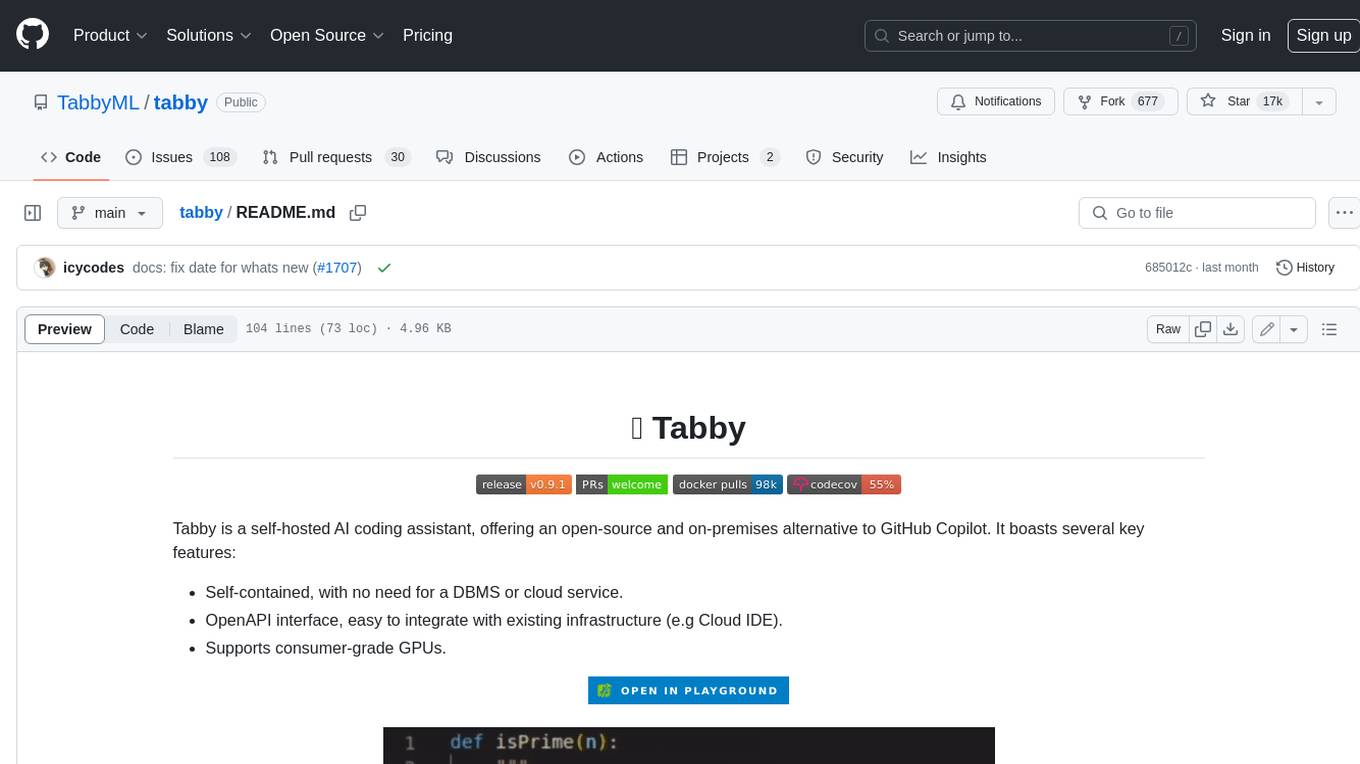
tabby
Tabby is a self-hosted AI coding assistant, offering an open-source and on-premises alternative to GitHub Copilot. It boasts several key features: * Self-contained, with no need for a DBMS or cloud service. * OpenAPI interface, easy to integrate with existing infrastructure (e.g Cloud IDE). * Supports consumer-grade GPUs.
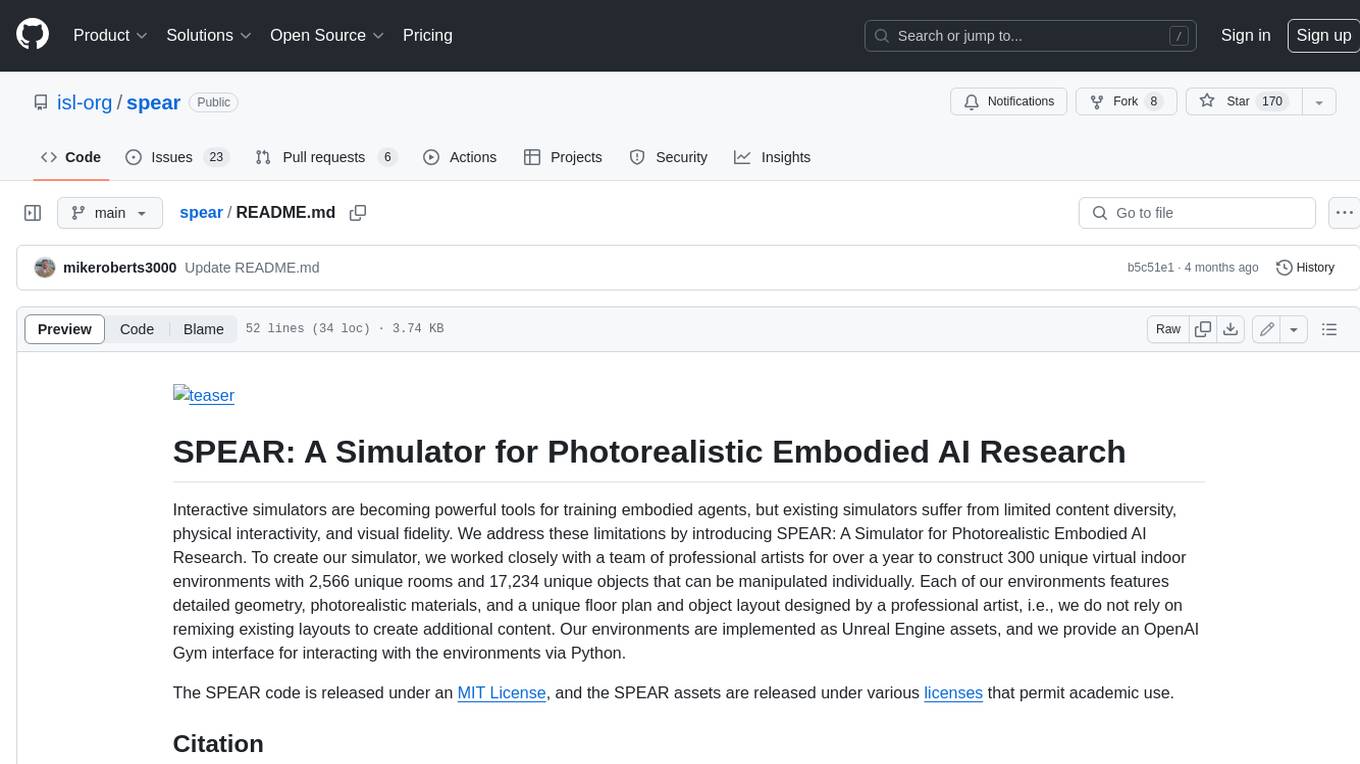
spear
SPEAR (Simulator for Photorealistic Embodied AI Research) is a powerful tool for training embodied agents. It features 300 unique virtual indoor environments with 2,566 unique rooms and 17,234 unique objects that can be manipulated individually. Each environment is designed by a professional artist and features detailed geometry, photorealistic materials, and a unique floor plan and object layout. SPEAR is implemented as Unreal Engine assets and provides an OpenAI Gym interface for interacting with the environments via Python.
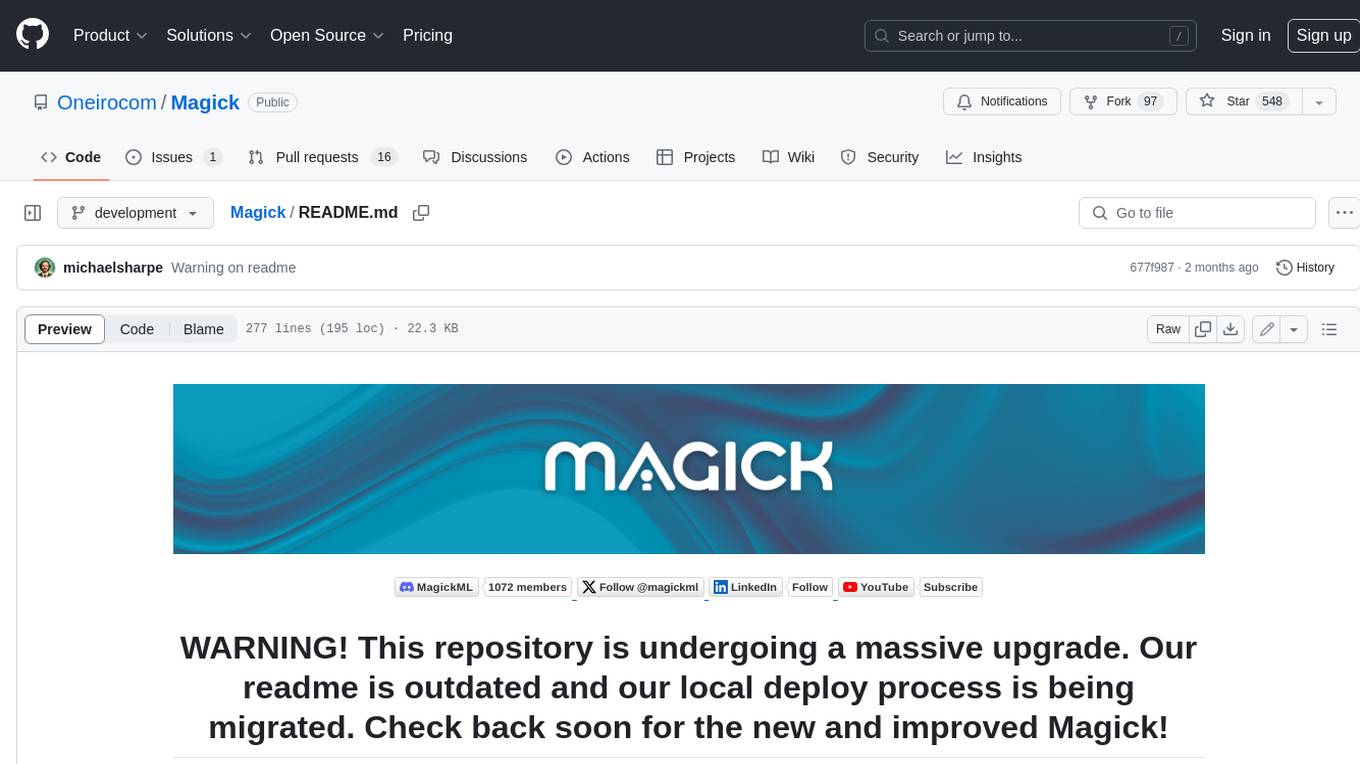
Magick
Magick is a groundbreaking visual AIDE (Artificial Intelligence Development Environment) for no-code data pipelines and multimodal agents. Magick can connect to other services and comes with nodes and templates well-suited for intelligent agents, chatbots, complex reasoning systems and realistic characters.