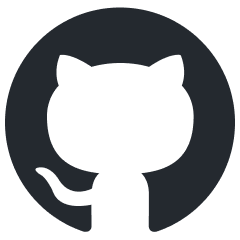
contoso-chat
This sample has the full End2End process of creating RAG application with Prompty and AI Studio. It includes GPT 3.5 Turbo LLM application code, evaluations, deployment automation with AZD CLI, GitHub actions for evaluation and deployment and intent mapping for multiple LLM task mapping.
Stars: 431
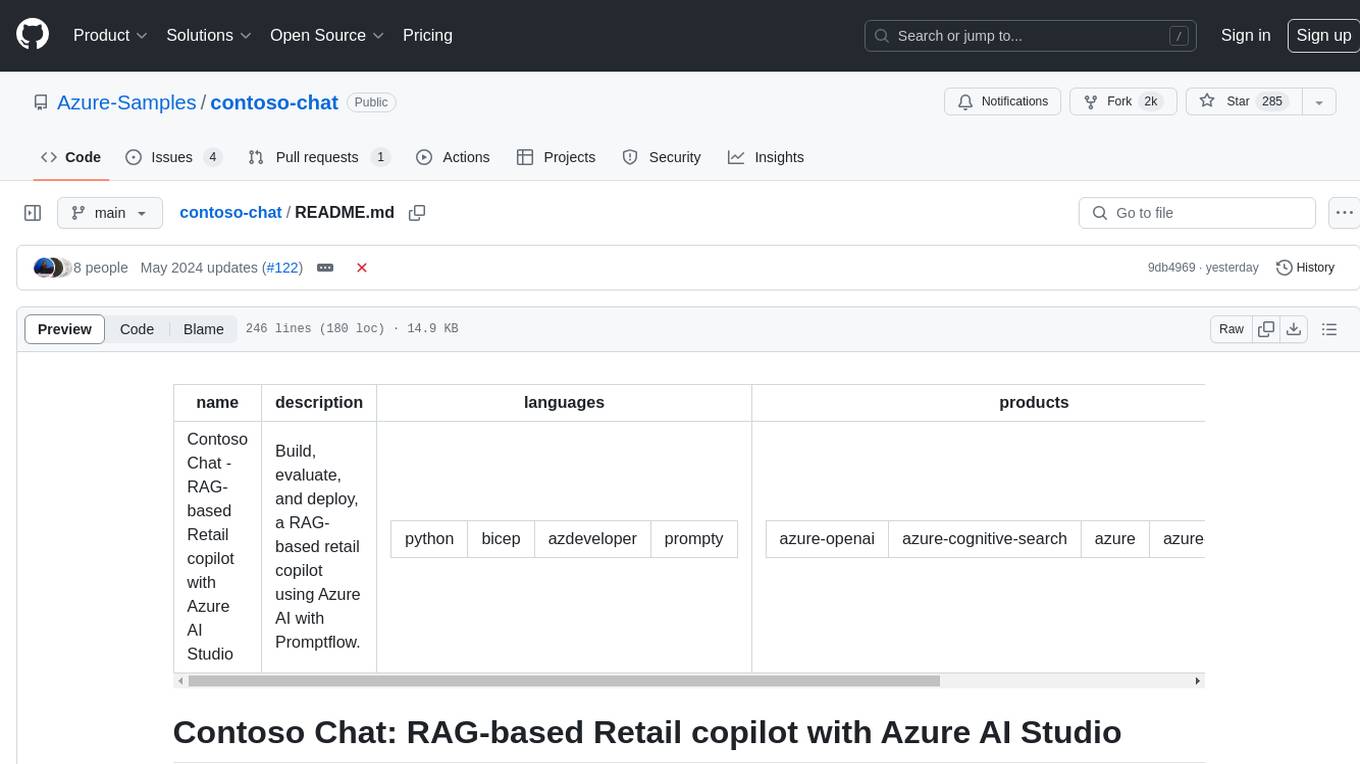
Contoso Chat is a Python sample demonstrating how to build, evaluate, and deploy a retail copilot application with Azure AI Studio using Promptflow with Prompty assets. The sample implements a Retrieval Augmented Generation approach to answer customer queries based on the company's product catalog and customer purchase history. It utilizes Azure AI Search, Azure Cosmos DB, Azure OpenAI, text-embeddings-ada-002, and GPT models for vectorizing user queries, AI-assisted evaluation, and generating chat responses. By exploring this sample, users can learn to build a retail copilot application, define prompts using Prompty, design, run & evaluate a copilot using Promptflow, provision and deploy the solution to Azure using the Azure Developer CLI, and understand Responsible AI practices for evaluation and content safety.
README:
page_type: sample languages:
- azdeveloper
- python
- bash
- bicep
- prompty products:
- azure
- azure-openai
- azure-cognitive-search
- azure-cosmos-db urlFragment: contoso-chat name: Contoso Chat - Retail RAG Copilot with Azure AI Studio and Prompty (Python Implementation) description: Build, evaluate, and deploy, a RAG-based retail copilot that responds to customer questions with responses grounded in the retailer's product and customer data.
[!WARNING]
This sample is being actively updated at present and make have breaking changes. We are refactoring the code to use new Azure AI platform features and moving deployment from Azure AI Studio to Azure Container Apps. We will remove this notice once the migration is complete. Till then, please pause on submitting new issues as codebase is changing.Some of the features used in this repository are in preview. Preview versions are provided without a service level agreement, and they are not recommended for production workloads. Certain features might not be supported or might have constrained capabilities. For more information, see Supplemental Terms of Use for Microsoft Azure Previews.**
- Overview
- Features
- Pre-Requisites
- Getting Started
- Development
- Testing
- Deployment
- Costs
- Security Guidelines
- Resources
- Code of Conduct
- Responsible AI Guidelines
Contoso Outdoor is an online retailer specializing in hiking and camping equipment for outdoor enthusiasts. The website offers an extensive catalog of products - resulting in customers needing product information and recommendations to assist them in making relevant purchases.
This sample implements Contoso Chat - a retail copilot solution for Contoso Outdoor that uses a retrieval augmented generation design pattern to ground chatbot responses in the retailer's product and customer data. Customers can now ask questions from the website in natural language, and get relevant responses along with potential recommendations based on their purchase history - with responsible AI practices to ensure response quality and safety.
The sample illustrates the end-to-end workflow (GenAIOps) for building a RAG-based copilot code-first with Azure AI and Prompty. By exploring and deploying this sample, you will learn to:
- Ideate and iterate rapidly on app prototypes using Prompty
- Deploy and use Azure OpenAI models for chat, embeddings and evaluation
- Use Azure AI Search (indexes) and Azure CosmosDB (databases) for your data
- Evaluate chat responses for quality using AI-assisted evaluation flows
- Host the application as a FastAPI endpoint deployed to Azure Container Apps
- Provision and deploy the solution using the Azure Developer CLI
- Support Responsible AI practices with content safety & assessments
The project template provides the following features:
- Azure OpenAI for embeddings, chat, and evaluation models
- Prompty for creating and managing prompts for rapid ideati
- Azure AI Search for performing semantic similarity search
- Azure CosmosDB for storing customer orders in a noSQL database
- Azure Container Apps for hosting the chat AI endpoint on Azure
It also comes with:
- Sample product and customer data for rapid prototyping
- Sample application code for chat and evaluation workflows
- Sample datasets and custom evaluators using prompty assets
(In Planning) - Get an intuitive sense for how simple it can be to go from template discovery, to codespaces launch, to application deployment with azd up
. Watch this space for a demo video.
The Contoso Chat sample has undergone numerous architecture and tooling changes since its first version back in 2023. The table below links to legacy versions for awareness only. We recommend all users start with the latest version to leverage the latest tools and practices.
Version Description v0 : #cc2e808 MSAITour 2023-24 (dag-flow, jnja template) - Skillable Lab v1 : msbuild-lab322 MSBuild 2024 (dag-flow, jnja template) - Skillable Lab v2 : raghack-24 RAG Hack 2024 (flex-flow, prompty asset) - AZD Template v3 : main 🆕 MSAITour 2024-25 (prompty asset, ACA)- AZD Template
To deploy and explore the sample, you will need:
- An active Azure subscription - Signup for a free account here
- An active GitHub account - Signup for a free account here
- Access to Azure OpenAI Services - Learn about Limited Access here
- Access to Azure AI Search - With Semantic Ranker (premiun feature)
- Available Quota for:
text-embedding-ada-002
,gpt-35-turbo
. andgpt-4
We recommend deployments to swedencentral
or francecentral
as regions that can support all these models. In addition to the above, you will also need the ability to:
- provision Azure Monitor (free tier)
- provision Azure Container Apps (free tier)
- provision Azure CosmosDB for noSQL (free tier)
From a tooling perspective, familiarity with the following is useful:
- Visual Studio Code (and extensions)
- GitHub Codespaces and dev containers
- Python and Jupyter Notebooks
- Azure CLI, Azure Developer CLI and commandline usage
You have three options for setting up your development environment:
- Use GitHub Codespaces - for a prebuilt dev environment in the cloud
- Use Docker Desktop - for a prebuilt dev environment on local device
- Use Manual Setup - for control over all aspects of local env setup
We recommend going with GitHub Codespaces for the fastest start and lowest maintenance overheads. Pick one option below - click to expand the section and view the details.
1️⃣ | Quickstart with GitHub Codespaces
- Fork this repository to your personal GitHub account
- Click the green
Code
button in your fork of the repo - Select the
Codespaces
tab and clickCreate new codespaces ...
- You should see: a new browser tab launch with a VS Code IDE
- Wait till Codespaces is ready - VS Code terminal has active cursor.
- ✅ | Congratulations! - Your Codespaces environment is ready!
2️⃣ | Get Started with Docker Desktop
- Install VS Code (with Dev Containers Extension) to your local device
- Install Docker Desktop to your local device - and start the daemon
- Fork this repository to your personal GitHub account
- Clone the fork to your local device - open with Visual Studio Code
- If Dev Containers Extension installed - you see: "Reopen in Container" prompt
- Else read Dev Containers Documentation - to launch it manually
- Wait till the Visual Studio Code environment is ready - cursor is active.
- ✅ | Congratulations! - Your Docker Desktop environment is ready!
3️⃣ | Get Started with Manual Setup
- Verify you have Python 3 installed on your machine
- Install dependencies with
pip install -r src/api/requirements.txt
- Install the Azure Developer CLI for your OS
- Install the Azure CLI for your OS
- Verify that all required tools are installed and active:
az version azd version prompty --version python --version
Once you have set up the development environment, it's time to get started with the development workflow by first provisioning the required Azure infrastructure, then deploying the application from the template.
Regardless of the setup route you chose, you should at this point have a Visual Studio Code IDE running, with the required tools and package dependencies met in the associated dev environment.
1️⃣ | Authenticate With Azure
-
Open a VS Code terminal and authenticate with Azure CLI. Use the
--use-device-code
option if authenticating from GitHub Codespaces. Complete the auth workflow as guided.az login --use-device-code
-
Now authenticate with Azure Developer CLI in the same terminal. Complete the auth workflow as guided. You should see: Logged in on Azure.
azd auth login
2️⃣ | Provision-Deploy with AZD
-
Run
azd up
to provision infrastructure and deploy the application, with one command. (You can also useazd provision
,azd deploy
separately if needed)azd up
-
The command will ask for an
environment name
, alocation
for deployment and thesubscription
you wish to use for this purpose.- The environment name maps to
rg-ENVNAME
as the resource group created - The location should be
swedencentral
orfrancecentral
for model quota - The subscription should be an active subscription meeting pre-requistes
- The environment name maps to
-
The
azd up
command can take 15-20 minutes to complete. Successful completion sees aSUCCESS: ...
messages posted to the console. We can now validate the outcomes.
3️⃣ | Validate the Infrastructure
- Visit the Azure Portal - look for the
rg-ENVNAME
resource group created above - Click the
Deployments
link in the Essentials section - wait till all are completed. - Return to
Overview
page - you should see: 35 deployments, 15 resources - Click on the
Azure CosmosDB resource
in the list- Visit the resource detail page - click "Data Explorer"
- Verify that it has created a
customers
database with data items in it
- Click on the
Azure AI Search
resource in the list- Visit the resource detail page - click "Search Explorer"
- Verify that it has created a
contoso-products
index with data items in it
- Click on the
Azure Container Apps
resource in the list- Visit the resource detail page - click
Application Url
- Verify that you see a hosted endpoint with a
Hello World
message on page
- Visit the resource detail page - click
- Next, visit the Azure AI Studio portal
- Sign in - you should be auto-logged in with existing Azure credential
- Click on
All Resources
- you should see anAIServices
andHub
resources - Click the hub resource - you should see an
AI Project
resource listed - Click the project resource - look at Deployments page to verify models
- ✅ | Congratulations! - Your Azure project infrastructure is ready!
4️⃣ | Validate the Deployment
- The
azd up
process also deploys the application as an Azure Container App - Visit the ACA resource page - click on
Application Url
to view endpoint - Add a
/docs
suffix to default deployed path - to get a Swagger API test page - Click
Try it out
to unlock inputs - you seequestion
,customer_id
,chat_history
- Enter
question
= "Tell me about the waterproof tents" - Enter
customer_id
= 2 - Enter
chat_history
= [] - Click Execute to see results: You should see a valid response with a list of matching tents from the product catalog with additional details.
- Enter
- ✅ | Congratulations! - Your Chat AI Deployment is working!
We can think about two levels of testing - manual validation and automated evaluation. The first is interactive, using a single test prompt to validate the prototype as we iterate. The second is code-driven, using a test prompt dataset to assess quality and safety of prototype responses for a diverse set of prompt inputs - and score them for criteria like coherence, fluency, relevance and groundedness based on built-in or custom evaluators.
1️⃣ | Manual Testing (interactive)
The Contoso Chat application is implemented as a FastAPI application that can be deployed to a hosted endpoint in Azure Container Apps. The API implementation is defined in src/api/main.py
and currently exposes 2 routes:
-
/
- which shows the default "Hello World" message -
/api/create_request
- which is our chat AI endpoint for test prompts
To test locally, we run the FastAPI dev server, then use the Swagger endpoint at the /docs
route to test the locally-served endpoint in the same way we tested the deployed version/
- Change to the root folder of the repository
- Run
fastapi dev ./src/api/main.py
- it should launch a dev server - Click
Open in browser
to preview the dev server page in a new tab- You should see: "Hello, World" with route at
/
- You should see: "Hello, World" with route at
- Add
/docs
to the end of the path URL in the browser tab- You should see: "FASTAPI" page with 2 routes listed
- Click the
POST
route then clickTry it out
to unlock inputs
- Try a test input
- Enter
question
= "Tell me about the waterproof tents" - Enter
customer_id
= 2 - Enter
chat_history
= [] - Click Execute to see results: You should see a valid response with a list of matching tents from the product catalog with additional details.
- Enter
- ✅ | Congratulations! - You successfully tested the app locally
2️⃣ | AI-Assisted Evaluation (code-driven)
Testing a single prompt is good for rapid prototyping and ideation. But once we have our application designed, we want to validate the quality and safety of responses against diverse test prompts. The sample shows you how to do AI-Assisted Evaluation using custom evaluators implemented with Prompty.
- Visit the
src/api/evaluators/
folder - Open the
evaluate-chat-flow.ipynb
notebook - "Select Kernel" to activate - Clear inputs and then
Run all
- starts evaluaton flow withdata.jsonl
test dataset - Once evaluation completes (takes 10+ minutes), you should see
-
results.jsonl
= the chat model's responses to test inputs -
evaluated_results.jsonl
= the evaluation model's scoring of the responses - tabular results = coherence, fluency, relevance, groundedness scores
-
Want to get a better understanding of how custom evaluators work? Check out the src/api/evaluators/custom_evals
folder and explore the relevant Prompty assets and their template instructions.
The Prompty tooling also has support for built-in tracing for observability. Look for a .runs/
subfolder to be created during the evaluation run, with .tracy
files containing the trace data. Click one of them to get a trace-view display in Visual Studio Code to help you drill down or debug the interaction flow. This is a new feature so look for more updates in usage soon.
The solution is deployed using the Azure Developer CLI. The azd up
command effectively calls azd provision
and then azd deploy
- allowing you to provision infrastructure and deploy the application with a single command. Subsequent calls to azd up
(e.g., ,after making changes to the application) should be faster, re-deploying the application and updating infrastructure provisioning only if required. You can then test the deployed endpoint as described earlier.
Pricing for services may vary by region and usage and exact costs are hard to determine. You can estimate the cost of this project's architecture with Azure's pricing calculator with these services:
- Azure OpenAI - Standard tier, GPT-35-turbo and Ada models. See Pricing
- Azure AI Search - Basic tier, Semantic Ranker enabled. See Pricing
- Azure Cosmos DB for NoSQL - Serverless, Free Tier. See Pricing
- Azure Monitor - Serverless, Free Tier. See Pricing
- Azure Container Apps - Severless, Free Tier. See Pricing
This template uses Managed Identity for authentication with key Azure services including Azure OpenAI, Azure AI Search, and Azure Cosmos DB. Applications can use managed identities to obtain Microsoft Entra tokens without having to manage any credentials. This also removes the need for developers to manage these credentials themselves and reduces their complexity.
Additionally, we have added a GitHub Action tool that scans the infrastructure-as-code files and generates a report containing any detected issues. To ensure best practices we recommend anyone creating solutions based on our templates ensure that the Github secret scanning setting is enabled in your repo.
This project has adopted the Microsoft Open Source Code of Conduct. Learn more here:
- Microsoft Open Source Code of Conduct
- Microsoft Code of Conduct FAQ
- Contact [email protected] with questions or concerns
For more information see the Code of Conduct FAQ or contact [email protected] with any additional questions or comments.
This project follows below responsible AI guidelines and best practices, please review them before using this project:
- Microsoft Responsible AI Guidelines
- Responsible AI practices for Azure OpenAI models
- Safety evaluations transparency notes
For Tasks:
Click tags to check more tools for each tasksFor Jobs:
Alternative AI tools for contoso-chat
Similar Open Source Tools
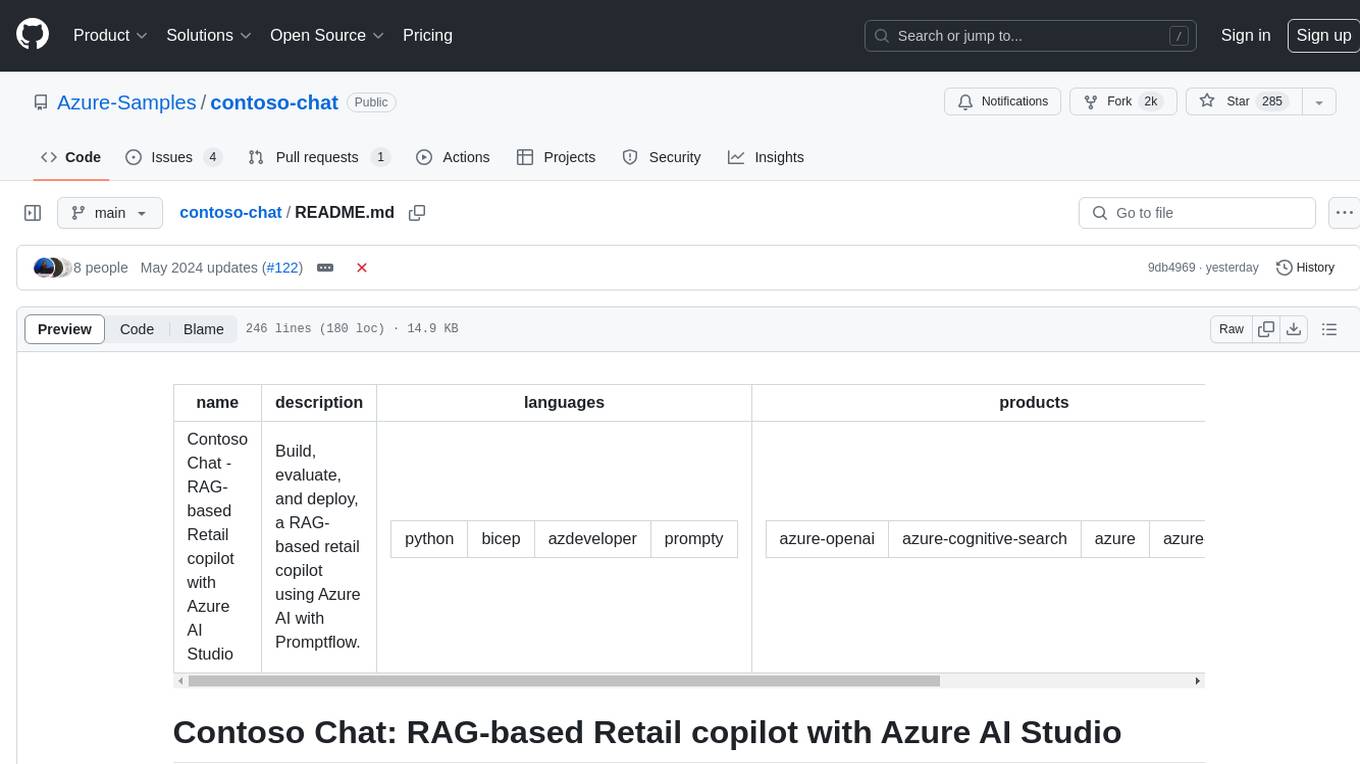
contoso-chat
Contoso Chat is a Python sample demonstrating how to build, evaluate, and deploy a retail copilot application with Azure AI Studio using Promptflow with Prompty assets. The sample implements a Retrieval Augmented Generation approach to answer customer queries based on the company's product catalog and customer purchase history. It utilizes Azure AI Search, Azure Cosmos DB, Azure OpenAI, text-embeddings-ada-002, and GPT models for vectorizing user queries, AI-assisted evaluation, and generating chat responses. By exploring this sample, users can learn to build a retail copilot application, define prompts using Prompty, design, run & evaluate a copilot using Promptflow, provision and deploy the solution to Azure using the Azure Developer CLI, and understand Responsible AI practices for evaluation and content safety.
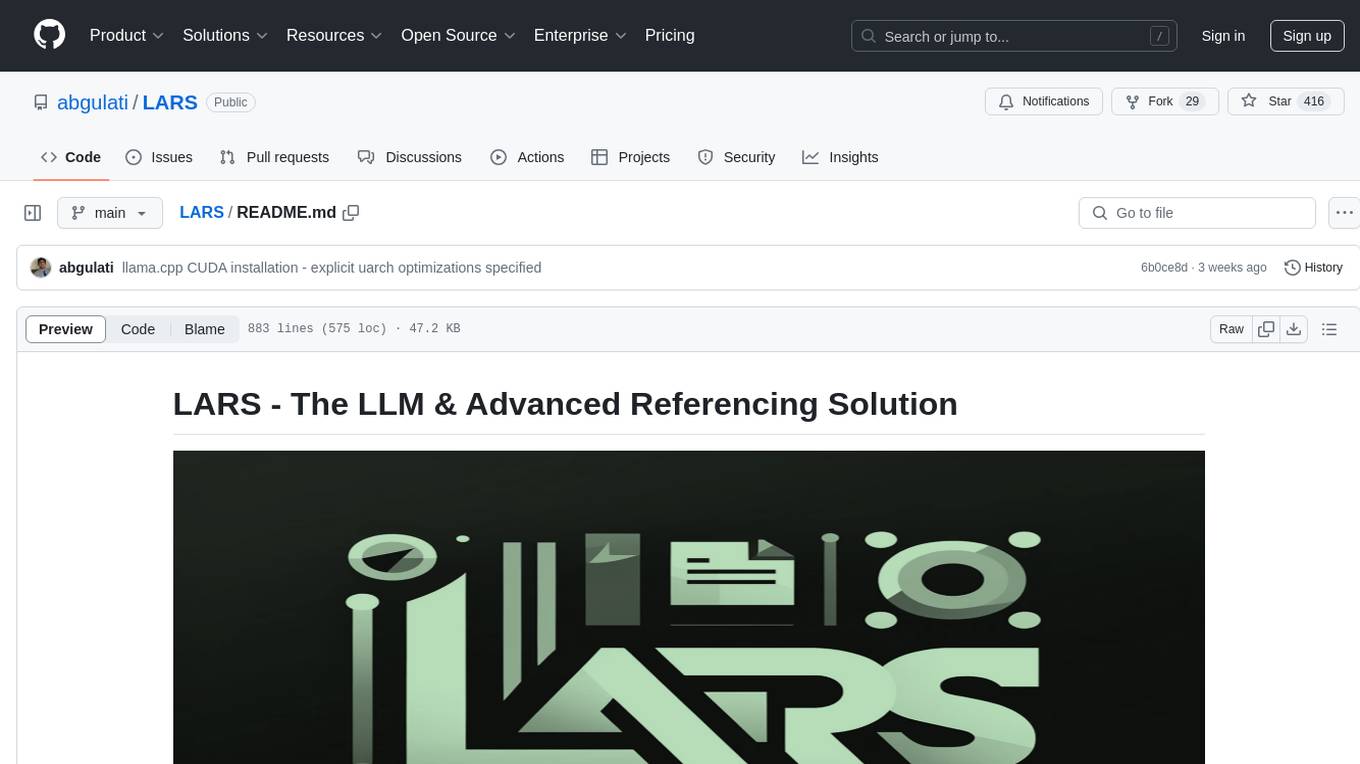
LARS
LARS is an application that enables users to run Large Language Models (LLMs) locally on their devices, upload their own documents, and engage in conversations where the LLM grounds its responses with the uploaded content. The application focuses on Retrieval Augmented Generation (RAG) to increase accuracy and reduce AI-generated inaccuracies. LARS provides advanced citations, supports various file formats, allows follow-up questions, provides full chat history, and offers customization options for LLM settings. Users can force enable or disable RAG, change system prompts, and tweak advanced LLM settings. The application also supports GPU-accelerated inferencing, multiple embedding models, and text extraction methods. LARS is open-source and aims to be the ultimate RAG-centric LLM application.
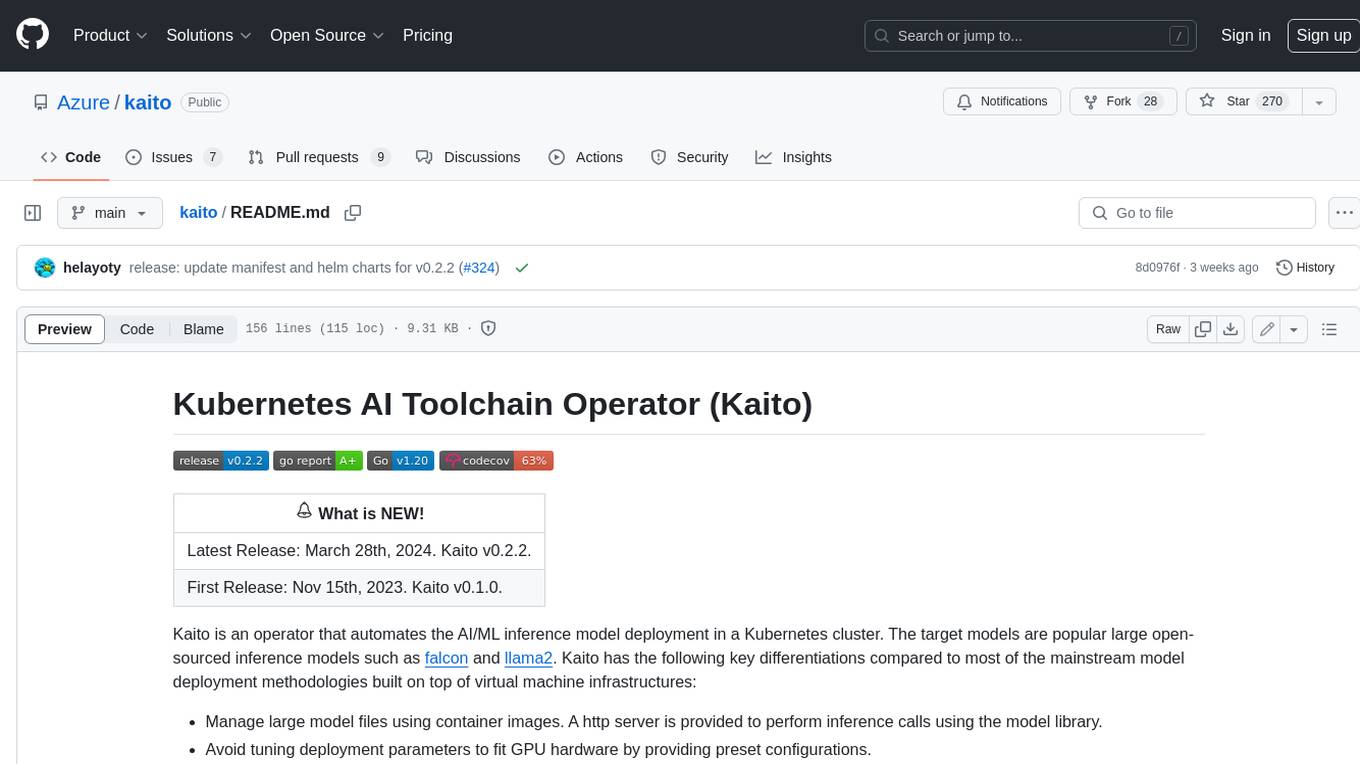
kaito
Kaito is an operator that automates the AI/ML inference model deployment in a Kubernetes cluster. It manages large model files using container images, avoids tuning deployment parameters to fit GPU hardware by providing preset configurations, auto-provisions GPU nodes based on model requirements, and hosts large model images in the public Microsoft Container Registry (MCR) if the license allows. Using Kaito, the workflow of onboarding large AI inference models in Kubernetes is largely simplified.
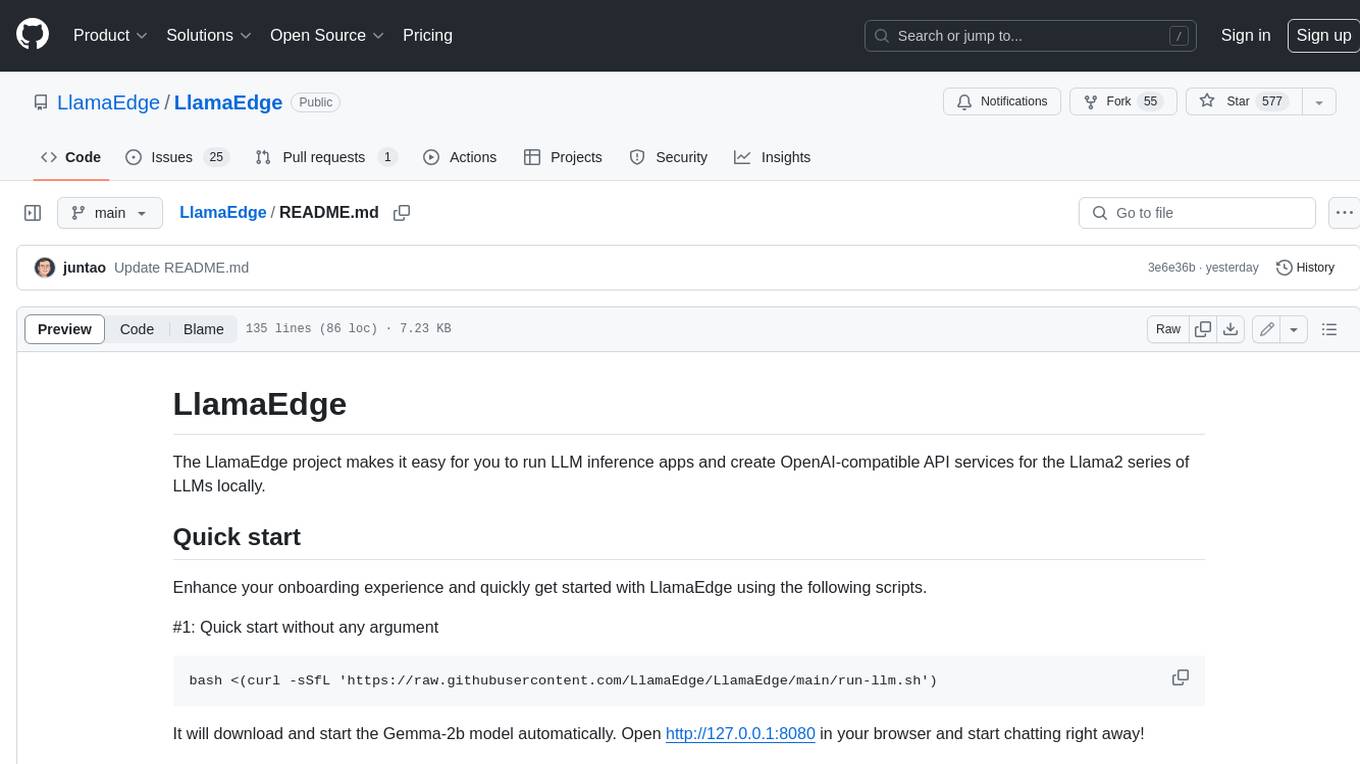
LlamaEdge
The LlamaEdge project makes it easy to run LLM inference apps and create OpenAI-compatible API services for the Llama2 series of LLMs locally. It provides a Rust+Wasm stack for fast, portable, and secure LLM inference on heterogeneous edge devices. The project includes source code for text generation, chatbot, and API server applications, supporting all LLMs based on the llama2 framework in the GGUF format. LlamaEdge is committed to continuously testing and validating new open-source models and offers a list of supported models with download links and startup commands. It is cross-platform, supporting various OSes, CPUs, and GPUs, and provides troubleshooting tips for common errors.
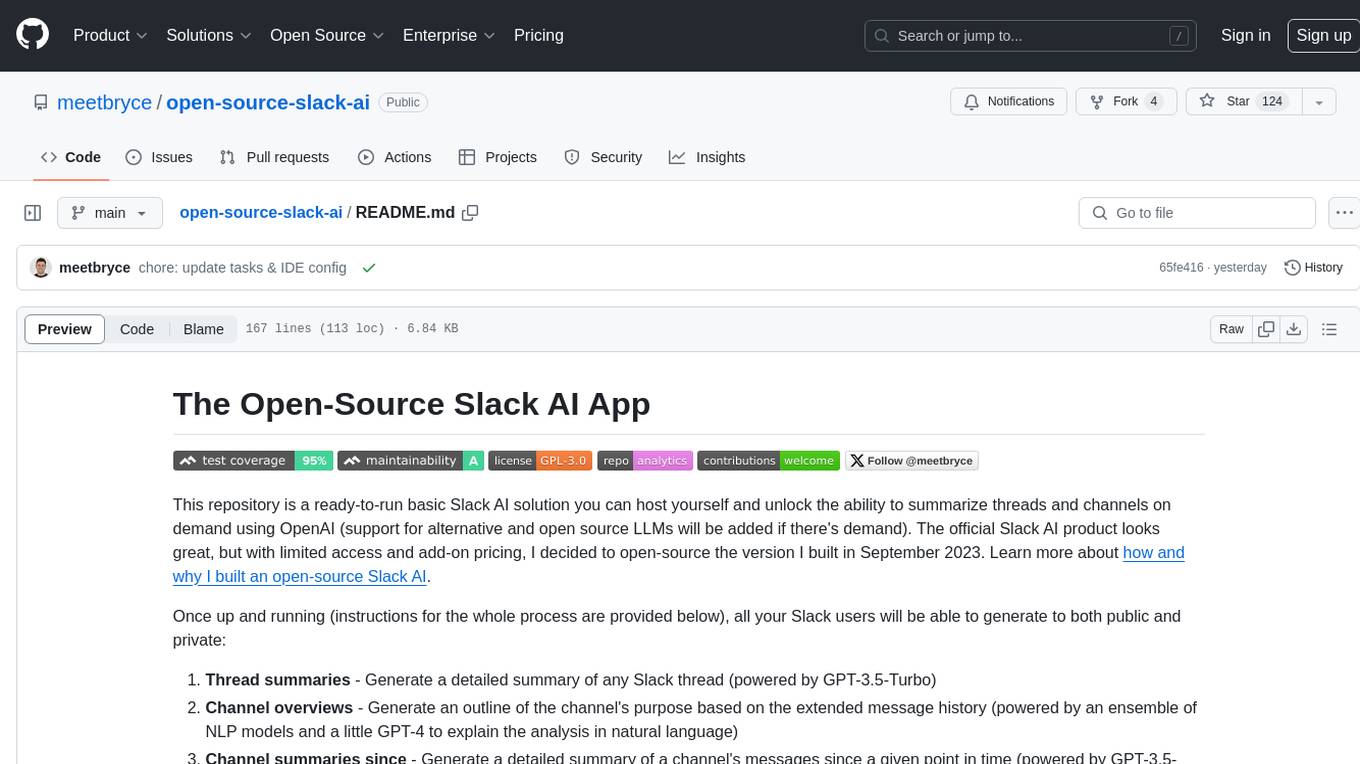
open-source-slack-ai
This repository provides a ready-to-run basic Slack AI solution that allows users to summarize threads and channels using OpenAI. Users can generate thread summaries, channel overviews, channel summaries since a specific time, and full channel summaries. The tool is powered by GPT-3.5-Turbo and an ensemble of NLP models. It requires Python 3.8 or higher, an OpenAI API key, Slack App with associated API tokens, Poetry package manager, and ngrok for local development. Users can customize channel and thread summaries, run tests with coverage using pytest, and contribute to the project for future enhancements.
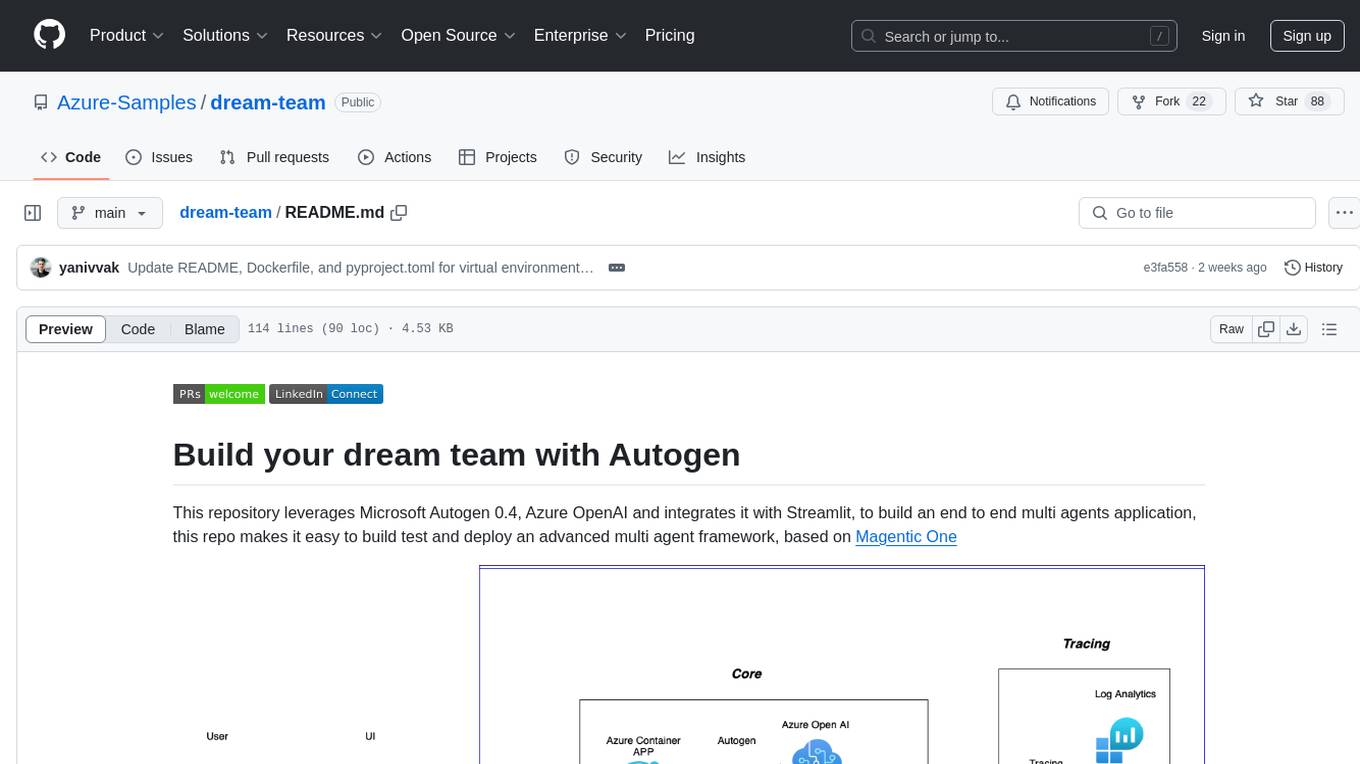
dream-team
Build your dream team with Autogen is a repository that leverages Microsoft Autogen 0.4, Azure OpenAI, and Streamlit to create an end-to-end multi-agent application. It provides an advanced multi-agent framework based on Magentic One, with features such as a friendly UI, single-line deployment, secure code execution, managed identities, and observability & debugging tools. Users can deploy Azure resources and the app with simple commands, work locally with virtual environments, install dependencies, update configurations, and run the application. The repository also offers resources for learning more about building applications with Autogen.
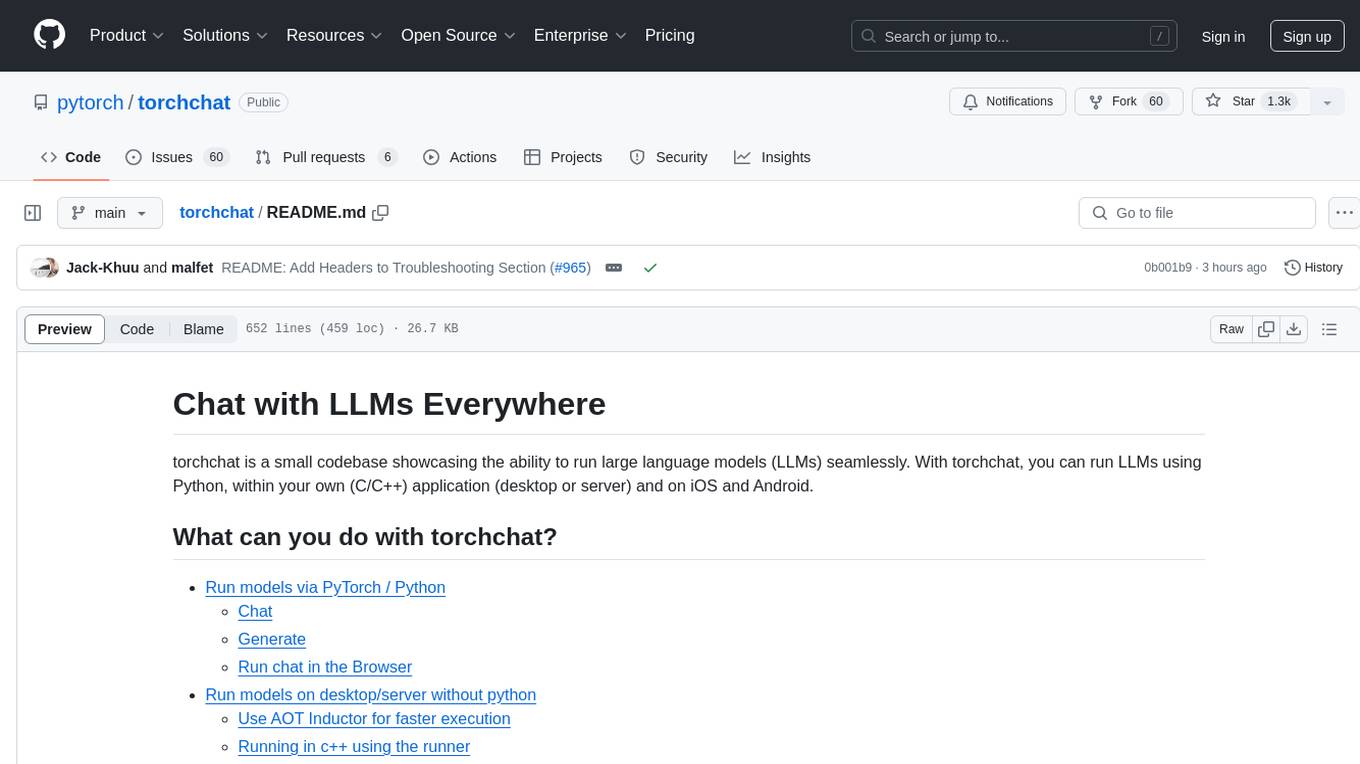
torchchat
torchchat is a codebase showcasing the ability to run large language models (LLMs) seamlessly. It allows running LLMs using Python in various environments such as desktop, server, iOS, and Android. The tool supports running models via PyTorch, chatting, generating text, running chat in the browser, and running models on desktop/server without Python. It also provides features like AOT Inductor for faster execution, running in C++ using the runner, and deploying and running on iOS and Android. The tool supports popular hardware and OS including Linux, Mac OS, Android, and iOS, with various data types and execution modes available.
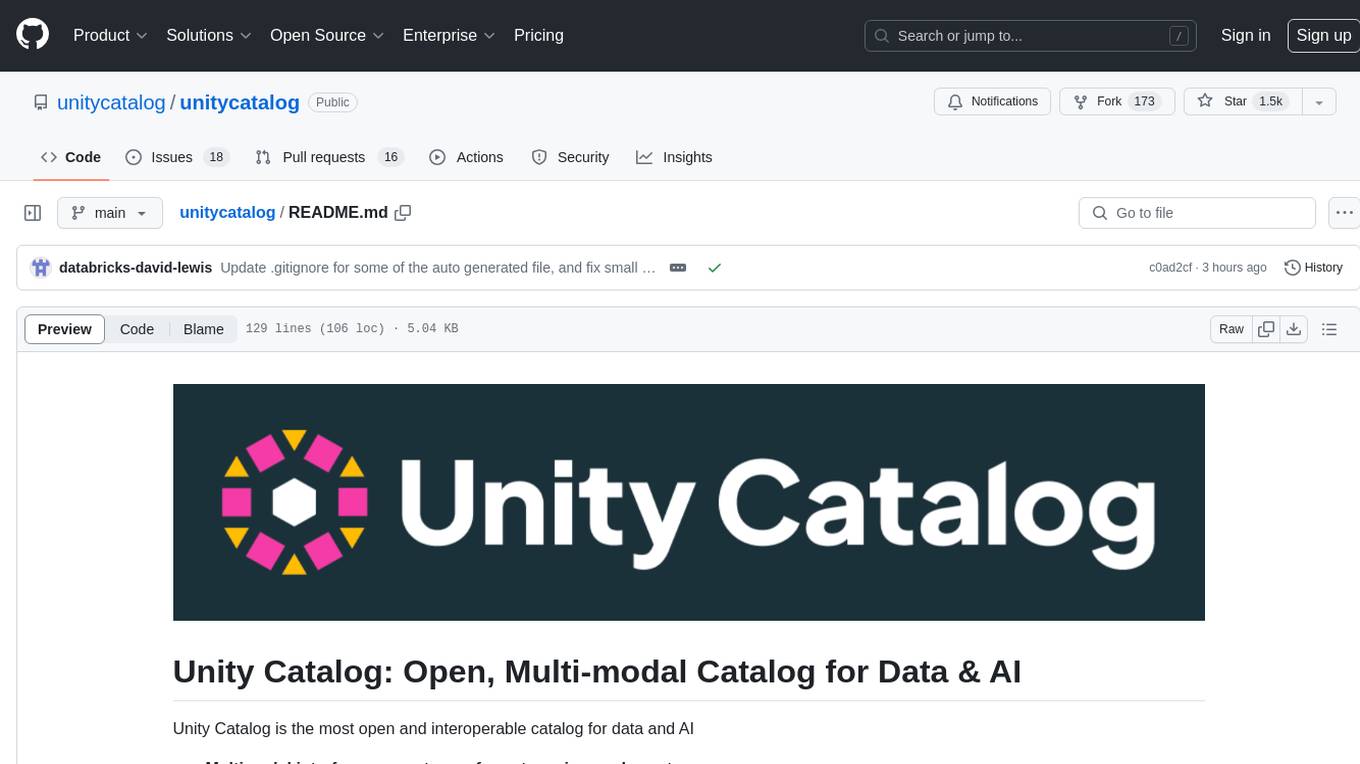
unitycatalog
Unity Catalog is an open and interoperable catalog for data and AI, supporting multi-format tables, unstructured data, and AI assets. It offers plugin support for extensibility and interoperates with Delta Sharing protocol. The catalog is fully open with OpenAPI spec and OSS implementation, providing unified governance for data and AI with asset-level access control enforced through REST APIs.
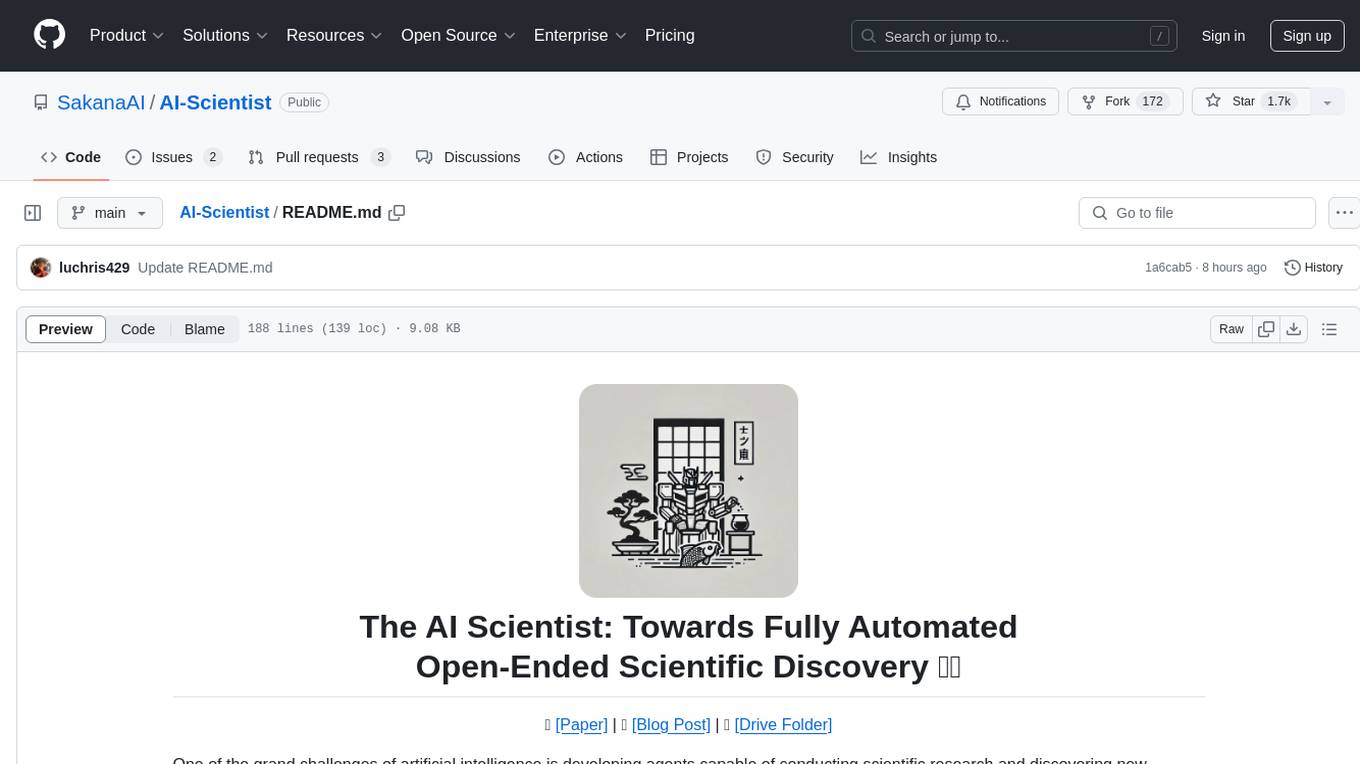
AI-Scientist
The AI Scientist is a comprehensive system for fully automatic scientific discovery, enabling Foundation Models to perform research independently. It aims to tackle the grand challenge of developing agents capable of conducting scientific research and discovering new knowledge. The tool generates papers on various topics using Large Language Models (LLMs) and provides a platform for exploring new research ideas. Users can create their own templates for specific areas of study and run experiments to generate papers. However, caution is advised as the codebase executes LLM-written code, which may pose risks such as the use of potentially dangerous packages and web access.
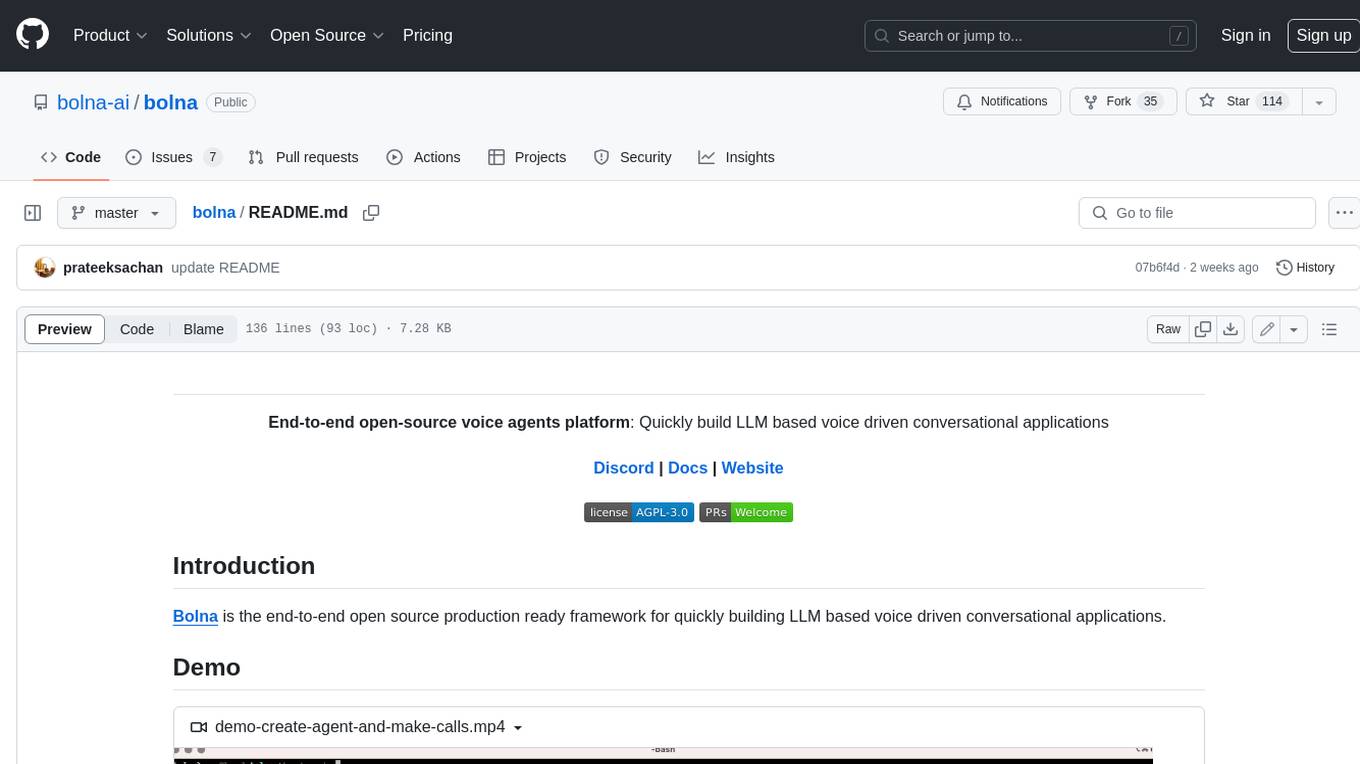
bolna
Bolna is an open-source platform for building voice-driven conversational applications using large language models (LLMs). It provides a comprehensive set of tools and integrations to handle various aspects of voice-based interactions, including telephony, transcription, LLM-based conversation handling, and text-to-speech synthesis. Bolna simplifies the process of creating voice agents that can perform tasks such as initiating phone calls, transcribing conversations, generating LLM-powered responses, and synthesizing speech. It supports multiple providers for each component, allowing users to customize their setup based on their specific needs. Bolna is designed to be easy to use, with a straightforward local setup process and well-documented APIs. It is also extensible, enabling users to integrate with other telephony providers or add custom functionality.
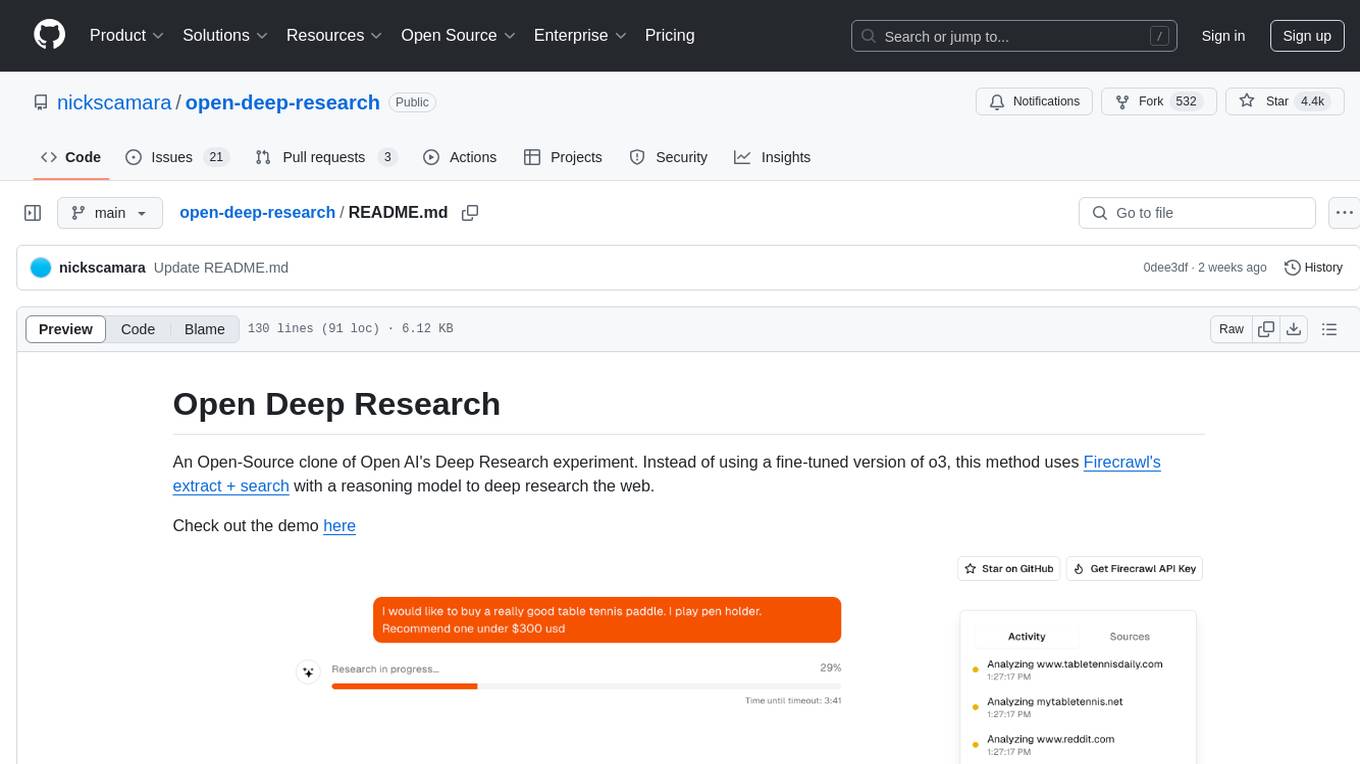
open-deep-research
Open Deep Research is an open-source project that serves as a clone of Open AI's Deep Research experiment. It utilizes Firecrawl's extract and search method along with a reasoning model to conduct in-depth research on the web. The project features Firecrawl Search + Extract, real-time data feeding to AI via search, structured data extraction from multiple websites, Next.js App Router for advanced routing, React Server Components and Server Actions for server-side rendering, AI SDK for generating text and structured objects, support for various model providers, styling with Tailwind CSS, data persistence with Vercel Postgres and Blob, and simple and secure authentication with NextAuth.js.
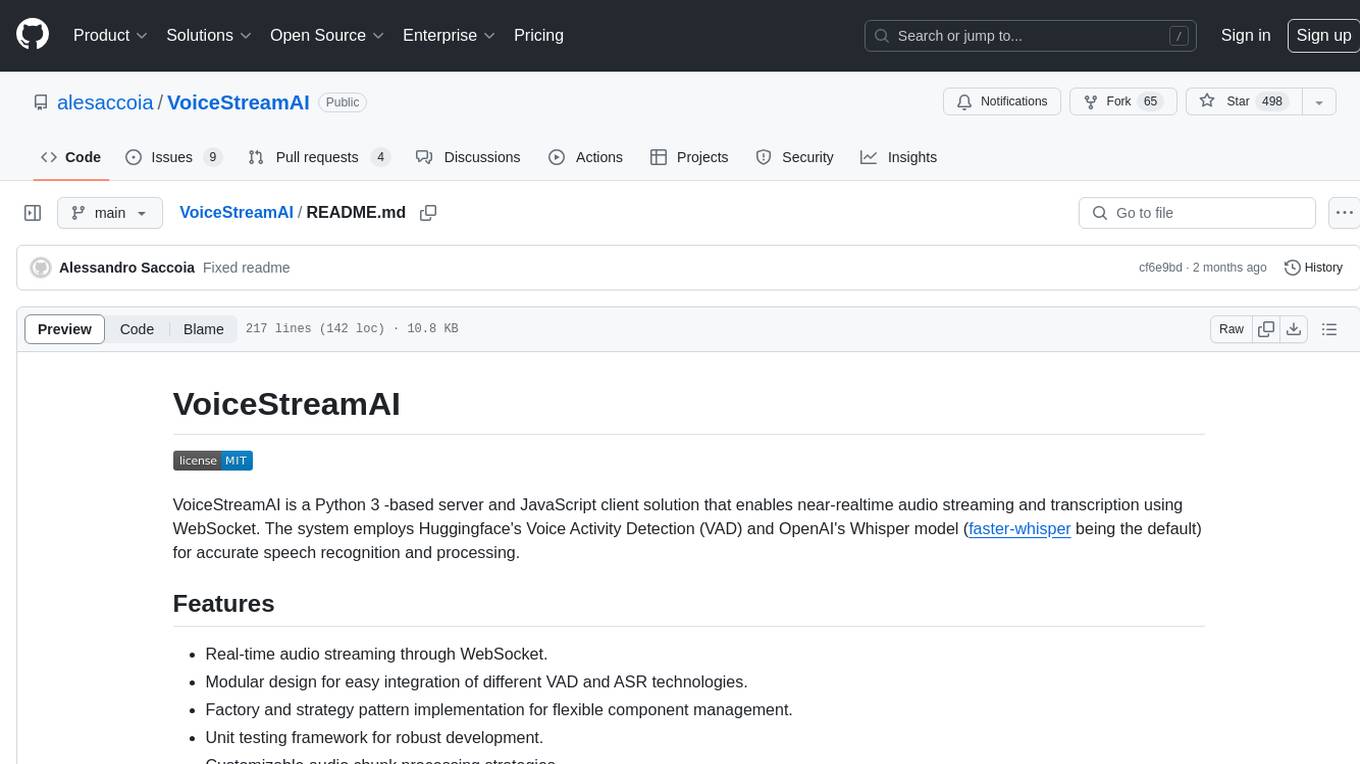
VoiceStreamAI
VoiceStreamAI is a Python 3-based server and JavaScript client solution for near-realtime audio streaming and transcription using WebSocket. It employs Huggingface's Voice Activity Detection (VAD) and OpenAI's Whisper model for accurate speech recognition. The system features real-time audio streaming, modular design for easy integration of VAD and ASR technologies, customizable audio chunk processing strategies, support for multilingual transcription, and secure sockets support. It uses a factory and strategy pattern implementation for flexible component management and provides a unit testing framework for robust development.
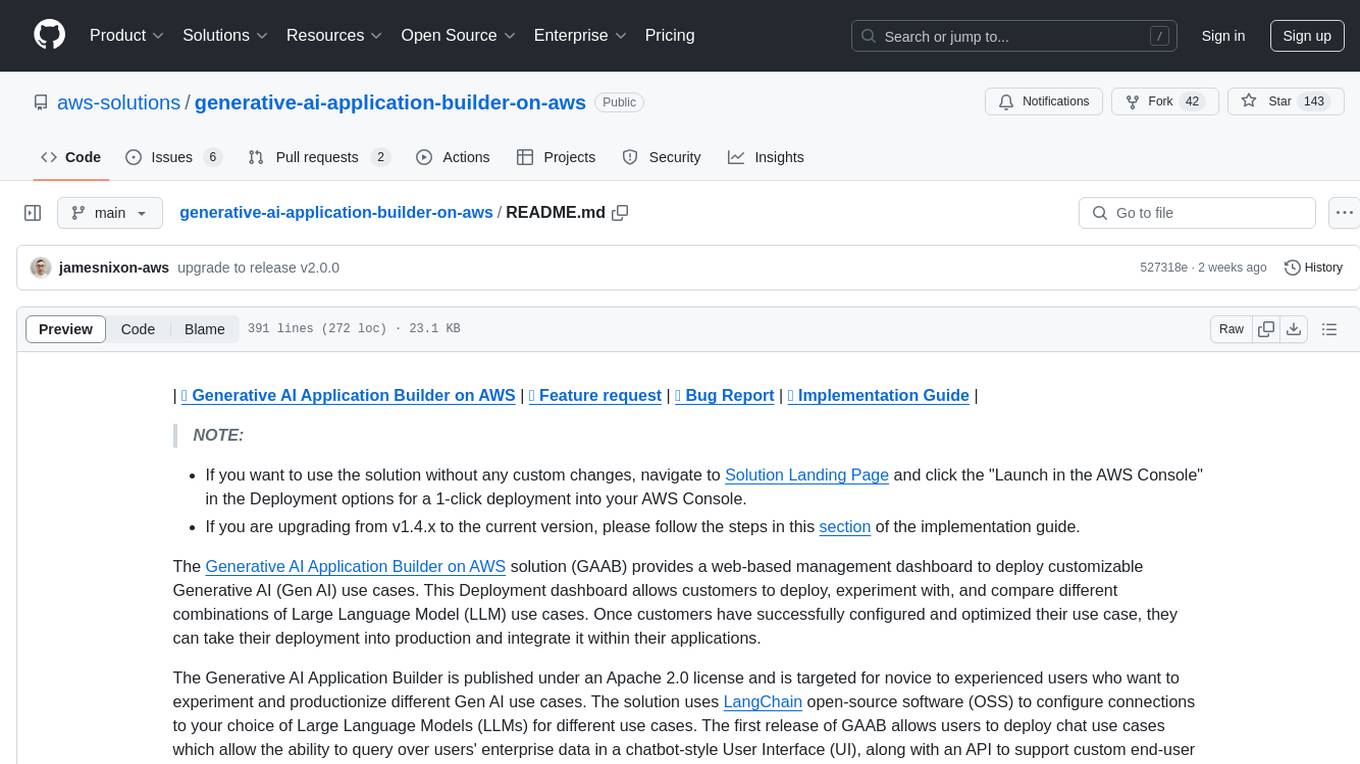
generative-ai-application-builder-on-aws
The Generative AI Application Builder on AWS (GAAB) is a solution that provides a web-based management dashboard for deploying customizable Generative AI (Gen AI) use cases. Users can experiment with and compare different combinations of Large Language Model (LLM) use cases, configure and optimize their use cases, and integrate them into their applications for production. The solution is targeted at novice to experienced users who want to experiment and productionize different Gen AI use cases. It uses LangChain open-source software to configure connections to Large Language Models (LLMs) for various use cases, with the ability to deploy chat use cases that allow querying over users' enterprise data in a chatbot-style User Interface (UI) and support custom end-user implementations through an API.
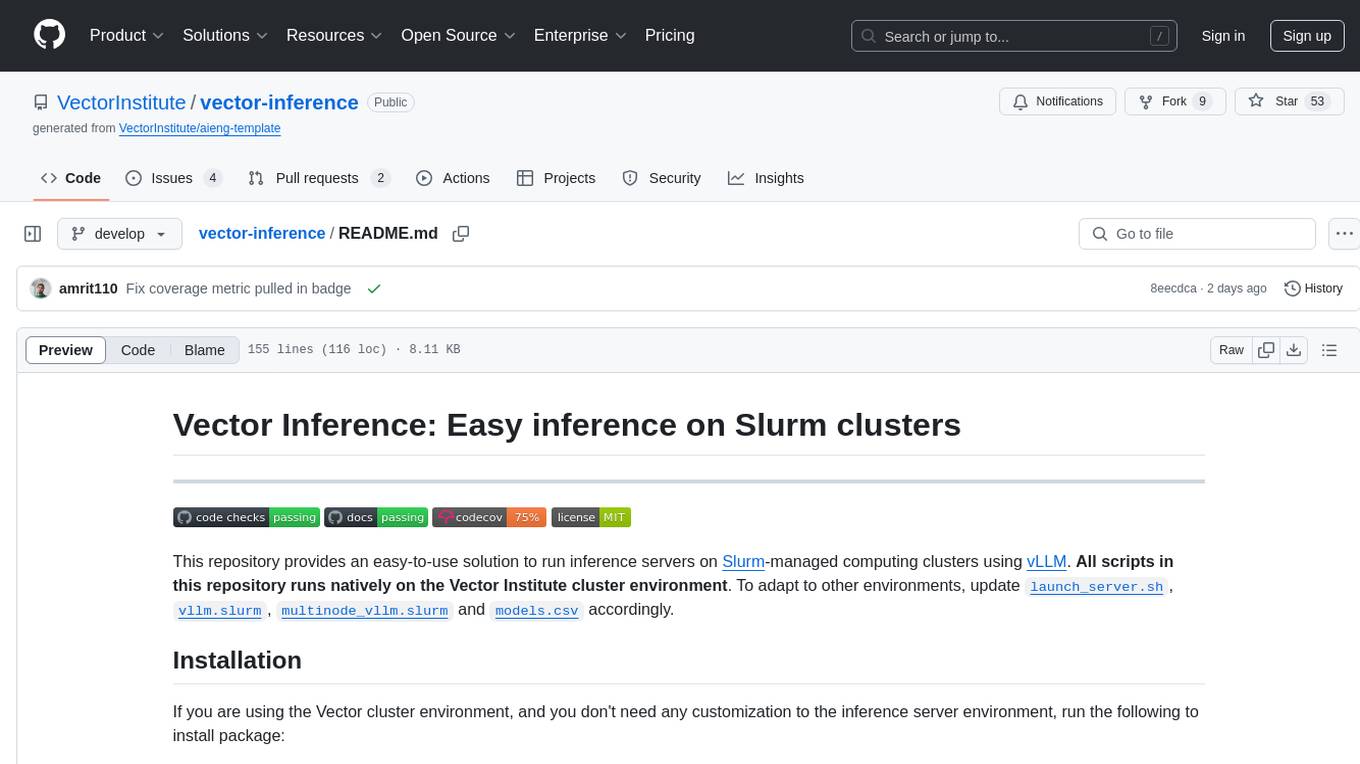
vector-inference
This repository provides an easy-to-use solution for running inference servers on Slurm-managed computing clusters using vLLM. All scripts in this repository run natively on the Vector Institute cluster environment. Users can deploy models as Slurm jobs, check server status and performance metrics, and shut down models. The repository also supports launching custom models with specific configurations. Additionally, users can send inference requests and set up an SSH tunnel to run inference from a local device.
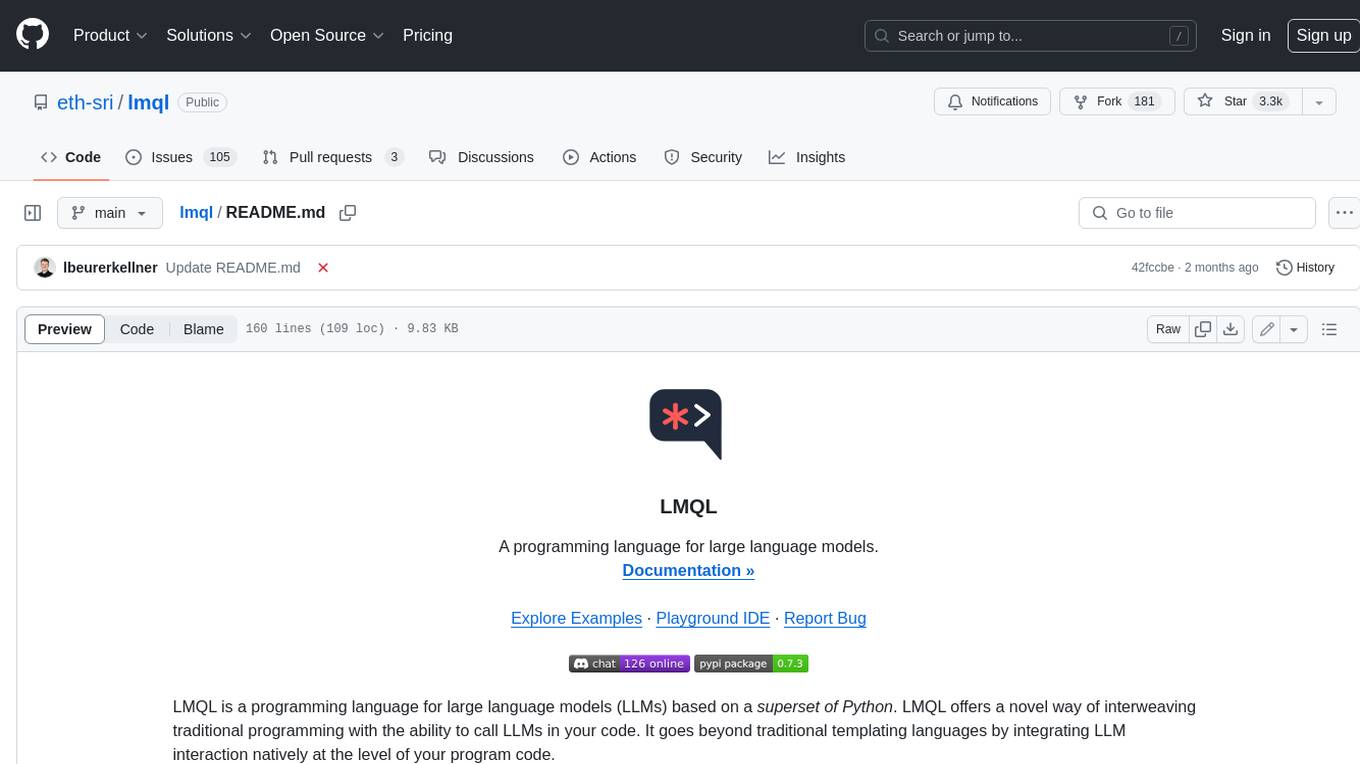
lmql
LMQL is a programming language designed for large language models (LLMs) that offers a unique way of integrating traditional programming with LLM interaction. It allows users to write programs that combine algorithmic logic with LLM calls, enabling model reasoning capabilities within the context of the program. LMQL provides features such as Python syntax integration, rich control-flow options, advanced decoding techniques, powerful constraints via logit masking, runtime optimization, sync and async API support, multi-model compatibility, and extensive applications like JSON decoding and interactive chat interfaces. The tool also offers library integration, flexible tooling, and output streaming options for easy model output handling.
For similar tasks
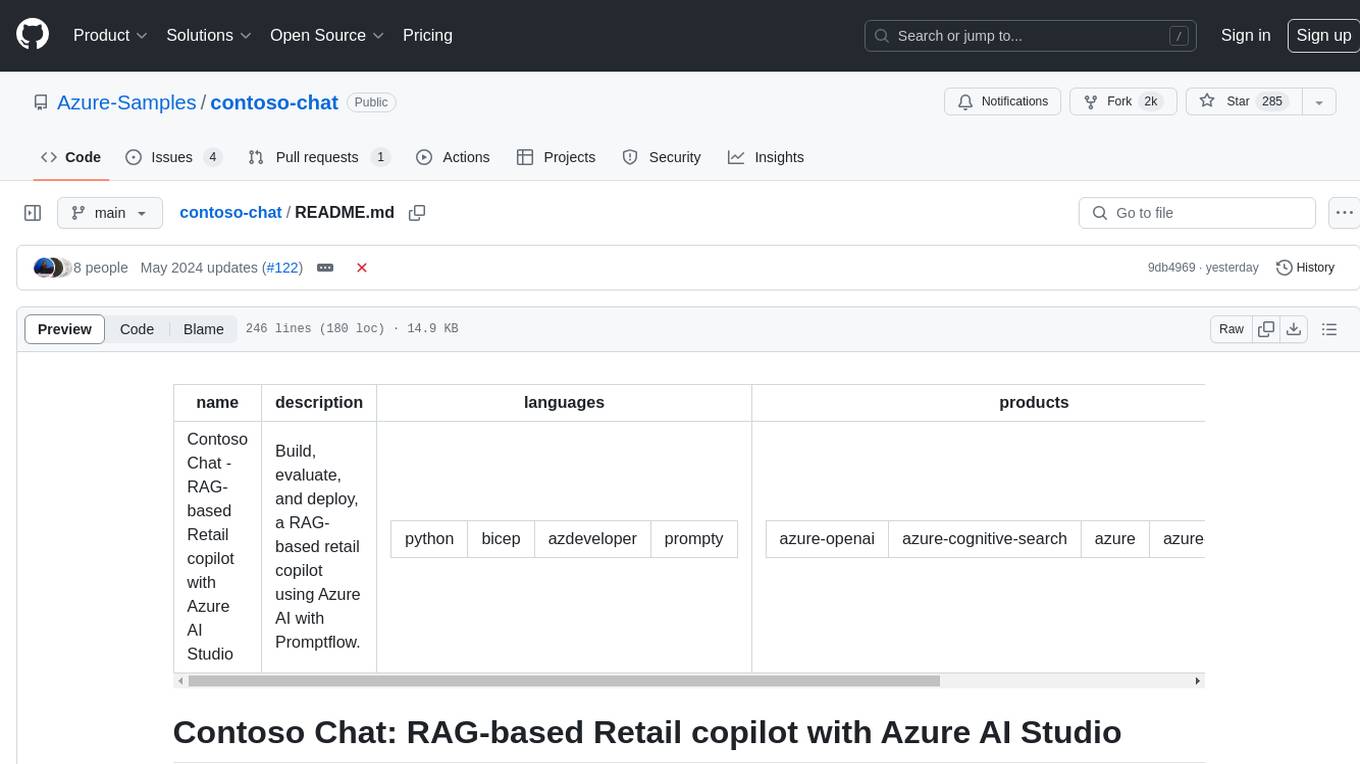
contoso-chat
Contoso Chat is a Python sample demonstrating how to build, evaluate, and deploy a retail copilot application with Azure AI Studio using Promptflow with Prompty assets. The sample implements a Retrieval Augmented Generation approach to answer customer queries based on the company's product catalog and customer purchase history. It utilizes Azure AI Search, Azure Cosmos DB, Azure OpenAI, text-embeddings-ada-002, and GPT models for vectorizing user queries, AI-assisted evaluation, and generating chat responses. By exploring this sample, users can learn to build a retail copilot application, define prompts using Prompty, design, run & evaluate a copilot using Promptflow, provision and deploy the solution to Azure using the Azure Developer CLI, and understand Responsible AI practices for evaluation and content safety.
For similar jobs
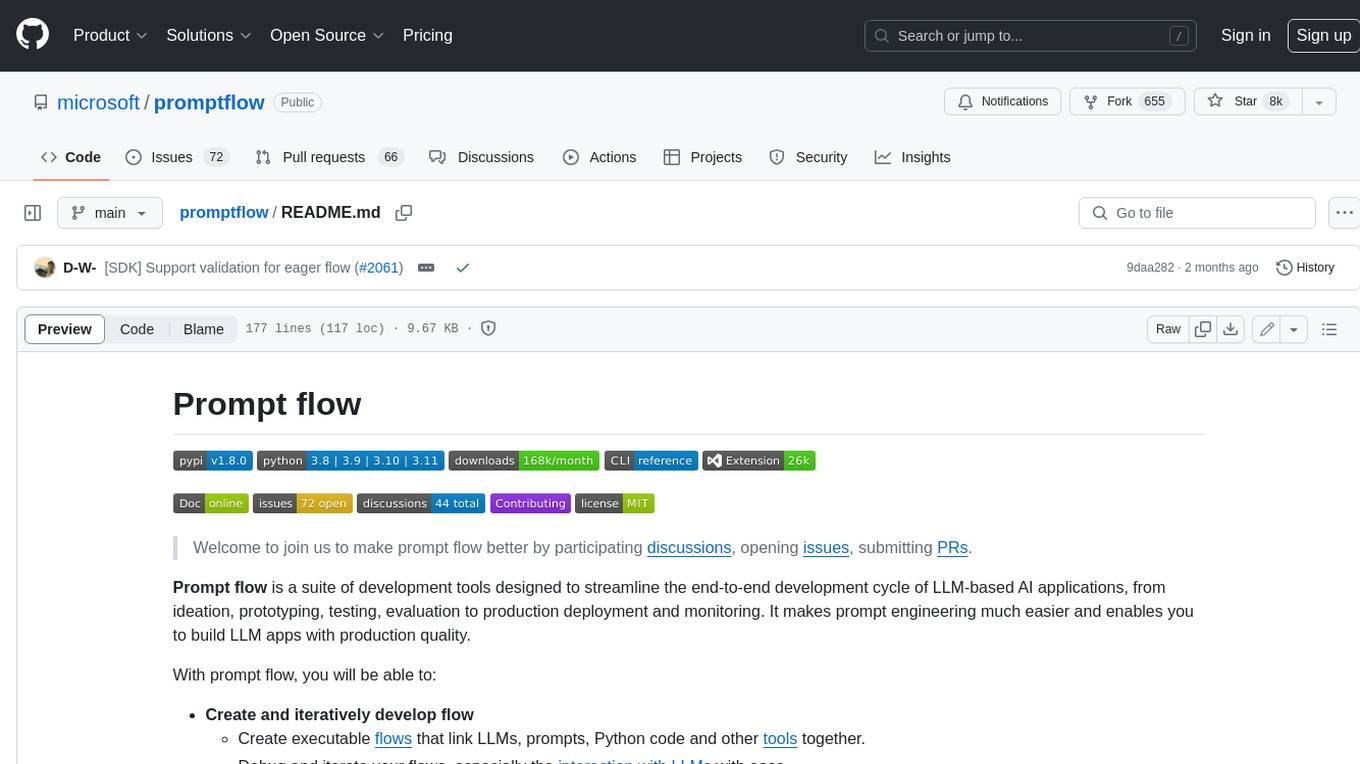
promptflow
**Prompt flow** is a suite of development tools designed to streamline the end-to-end development cycle of LLM-based AI applications, from ideation, prototyping, testing, evaluation to production deployment and monitoring. It makes prompt engineering much easier and enables you to build LLM apps with production quality.
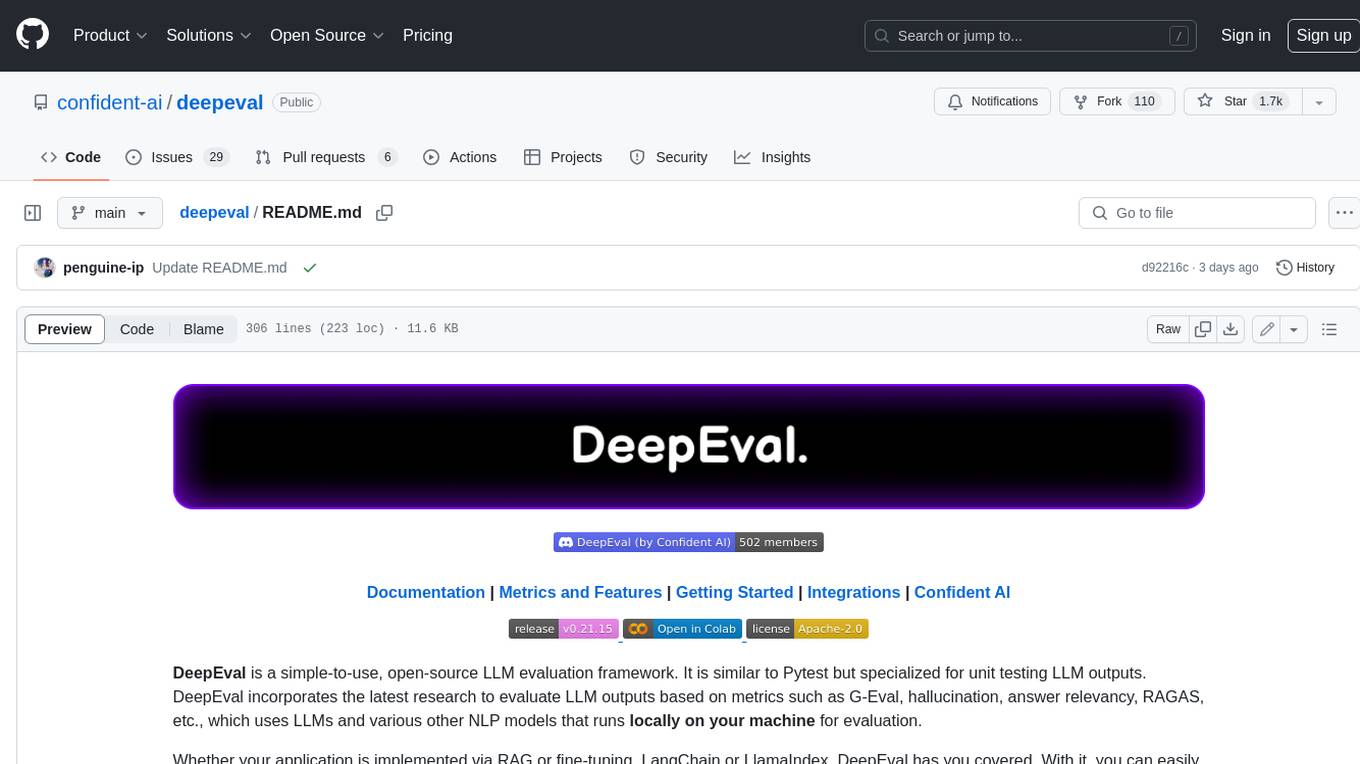
deepeval
DeepEval is a simple-to-use, open-source LLM evaluation framework specialized for unit testing LLM outputs. It incorporates various metrics such as G-Eval, hallucination, answer relevancy, RAGAS, etc., and runs locally on your machine for evaluation. It provides a wide range of ready-to-use evaluation metrics, allows for creating custom metrics, integrates with any CI/CD environment, and enables benchmarking LLMs on popular benchmarks. DeepEval is designed for evaluating RAG and fine-tuning applications, helping users optimize hyperparameters, prevent prompt drifting, and transition from OpenAI to hosting their own Llama2 with confidence.
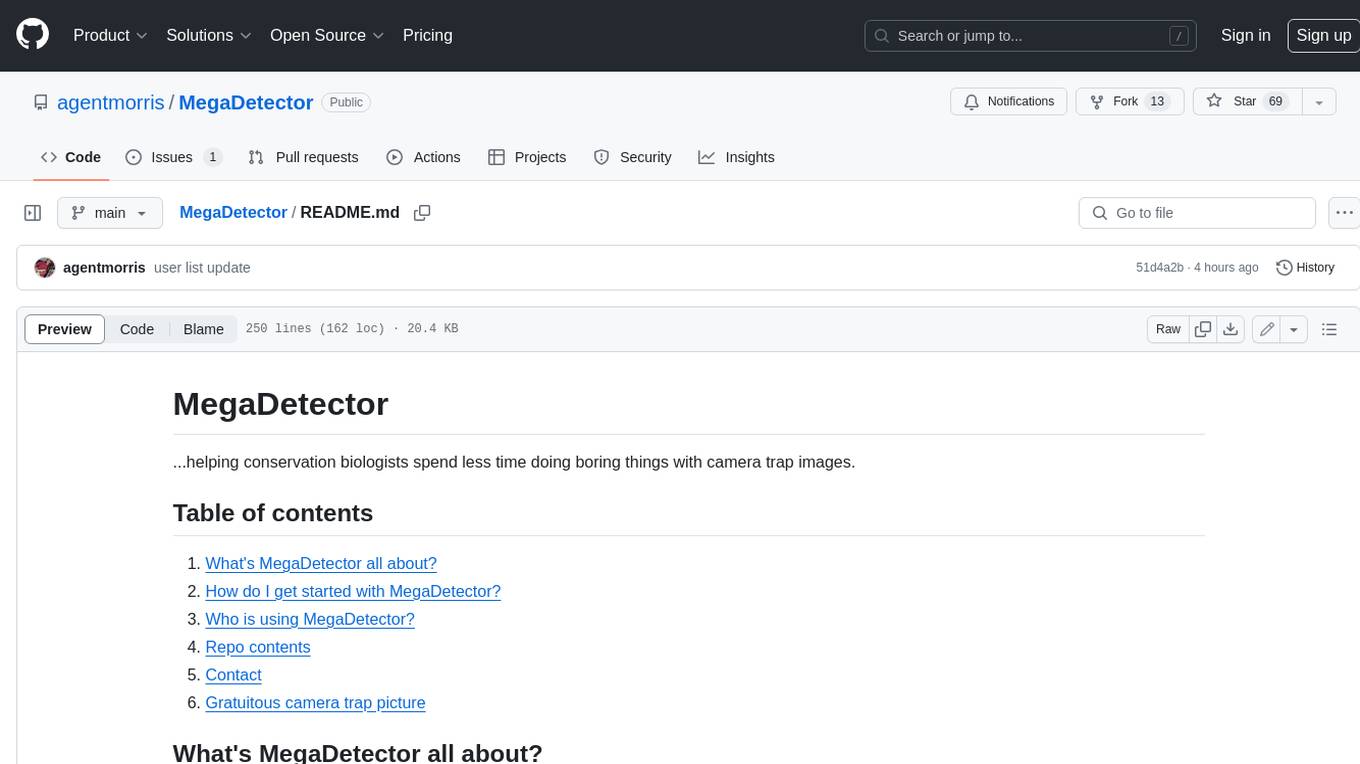
MegaDetector
MegaDetector is an AI model that identifies animals, people, and vehicles in camera trap images (which also makes it useful for eliminating blank images). This model is trained on several million images from a variety of ecosystems. MegaDetector is just one of many tools that aims to make conservation biologists more efficient with AI. If you want to learn about other ways to use AI to accelerate camera trap workflows, check out our of the field, affectionately titled "Everything I know about machine learning and camera traps".
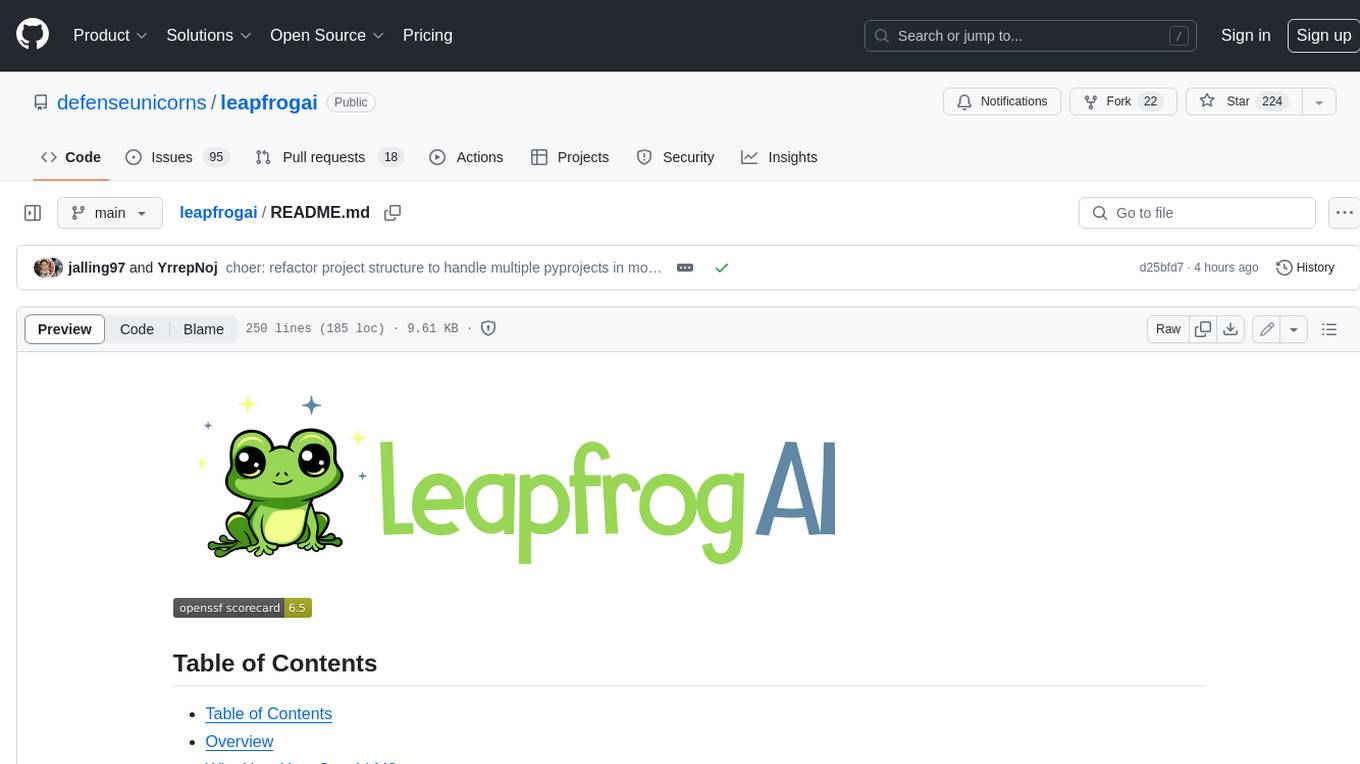
leapfrogai
LeapfrogAI is a self-hosted AI platform designed to be deployed in air-gapped resource-constrained environments. It brings sophisticated AI solutions to these environments by hosting all the necessary components of an AI stack, including vector databases, model backends, API, and UI. LeapfrogAI's API closely matches that of OpenAI, allowing tools built for OpenAI/ChatGPT to function seamlessly with a LeapfrogAI backend. It provides several backends for various use cases, including llama-cpp-python, whisper, text-embeddings, and vllm. LeapfrogAI leverages Chainguard's apko to harden base python images, ensuring the latest supported Python versions are used by the other components of the stack. The LeapfrogAI SDK provides a standard set of protobuffs and python utilities for implementing backends and gRPC. LeapfrogAI offers UI options for common use-cases like chat, summarization, and transcription. It can be deployed and run locally via UDS and Kubernetes, built out using Zarf packages. LeapfrogAI is supported by a community of users and contributors, including Defense Unicorns, Beast Code, Chainguard, Exovera, Hypergiant, Pulze, SOSi, United States Navy, United States Air Force, and United States Space Force.
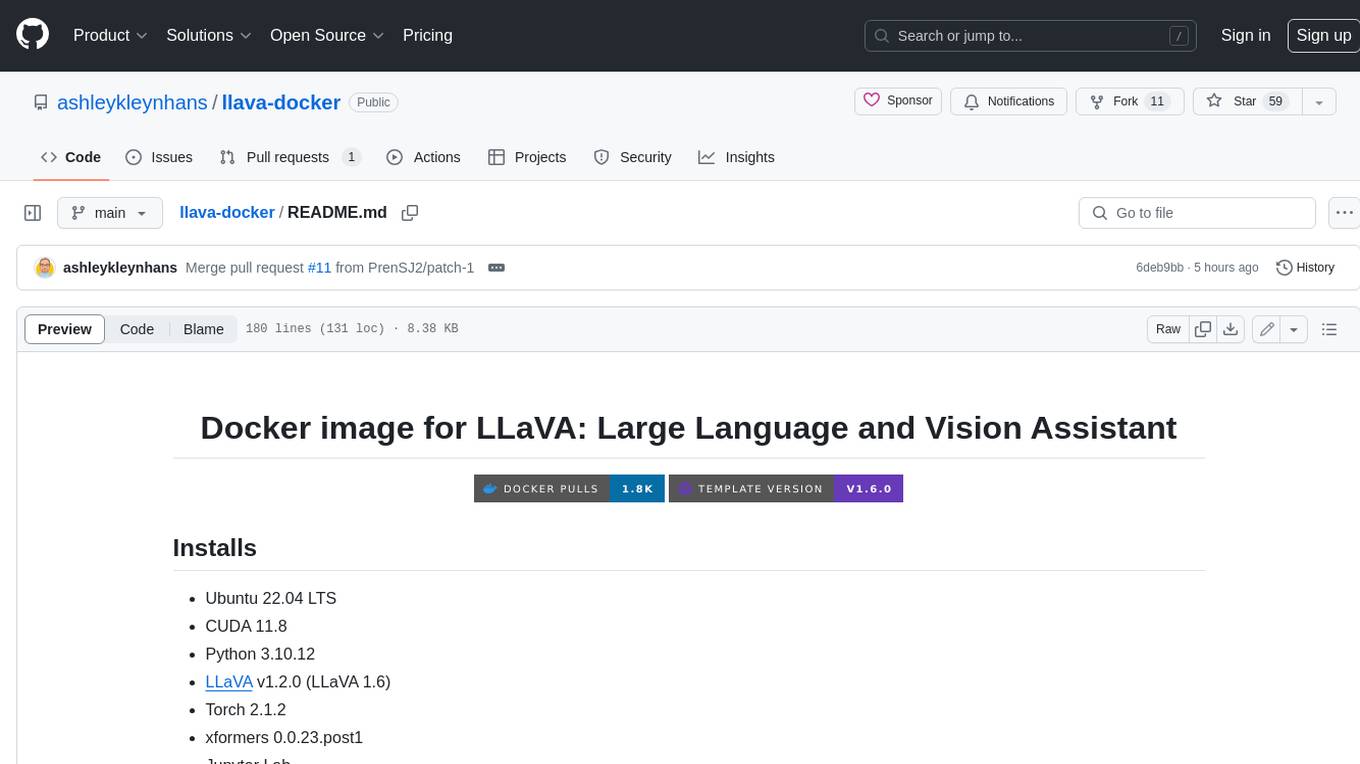
llava-docker
This Docker image for LLaVA (Large Language and Vision Assistant) provides a convenient way to run LLaVA locally or on RunPod. LLaVA is a powerful AI tool that combines natural language processing and computer vision capabilities. With this Docker image, you can easily access LLaVA's functionalities for various tasks, including image captioning, visual question answering, text summarization, and more. The image comes pre-installed with LLaVA v1.2.0, Torch 2.1.2, xformers 0.0.23.post1, and other necessary dependencies. You can customize the model used by setting the MODEL environment variable. The image also includes a Jupyter Lab environment for interactive development and exploration. Overall, this Docker image offers a comprehensive and user-friendly platform for leveraging LLaVA's capabilities.
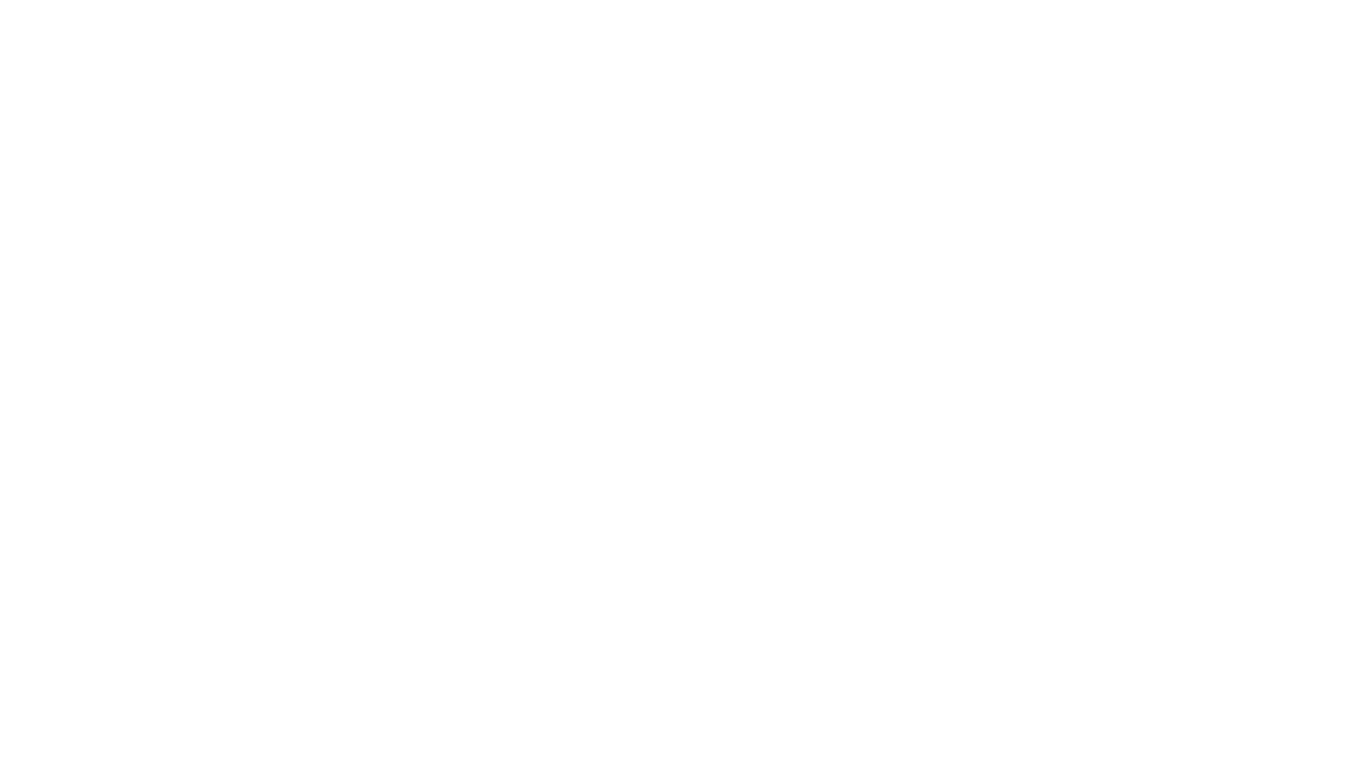
carrot
The 'carrot' repository on GitHub provides a list of free and user-friendly ChatGPT mirror sites for easy access. The repository includes sponsored sites offering various GPT models and services. Users can find and share sites, report errors, and access stable and recommended sites for ChatGPT usage. The repository also includes a detailed list of ChatGPT sites, their features, and accessibility options, making it a valuable resource for ChatGPT users seeking free and unlimited GPT services.
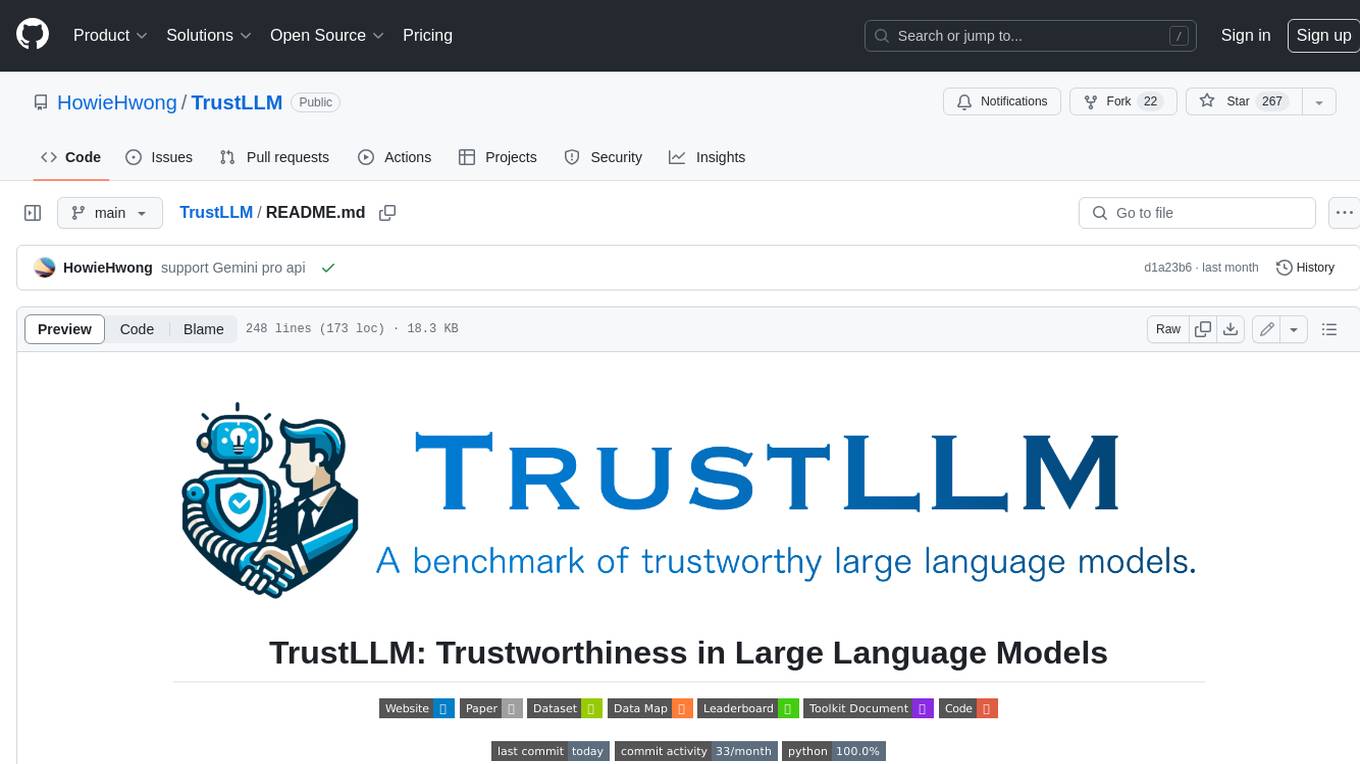
TrustLLM
TrustLLM is a comprehensive study of trustworthiness in LLMs, including principles for different dimensions of trustworthiness, established benchmark, evaluation, and analysis of trustworthiness for mainstream LLMs, and discussion of open challenges and future directions. Specifically, we first propose a set of principles for trustworthy LLMs that span eight different dimensions. Based on these principles, we further establish a benchmark across six dimensions including truthfulness, safety, fairness, robustness, privacy, and machine ethics. We then present a study evaluating 16 mainstream LLMs in TrustLLM, consisting of over 30 datasets. The document explains how to use the trustllm python package to help you assess the performance of your LLM in trustworthiness more quickly. For more details about TrustLLM, please refer to project website.
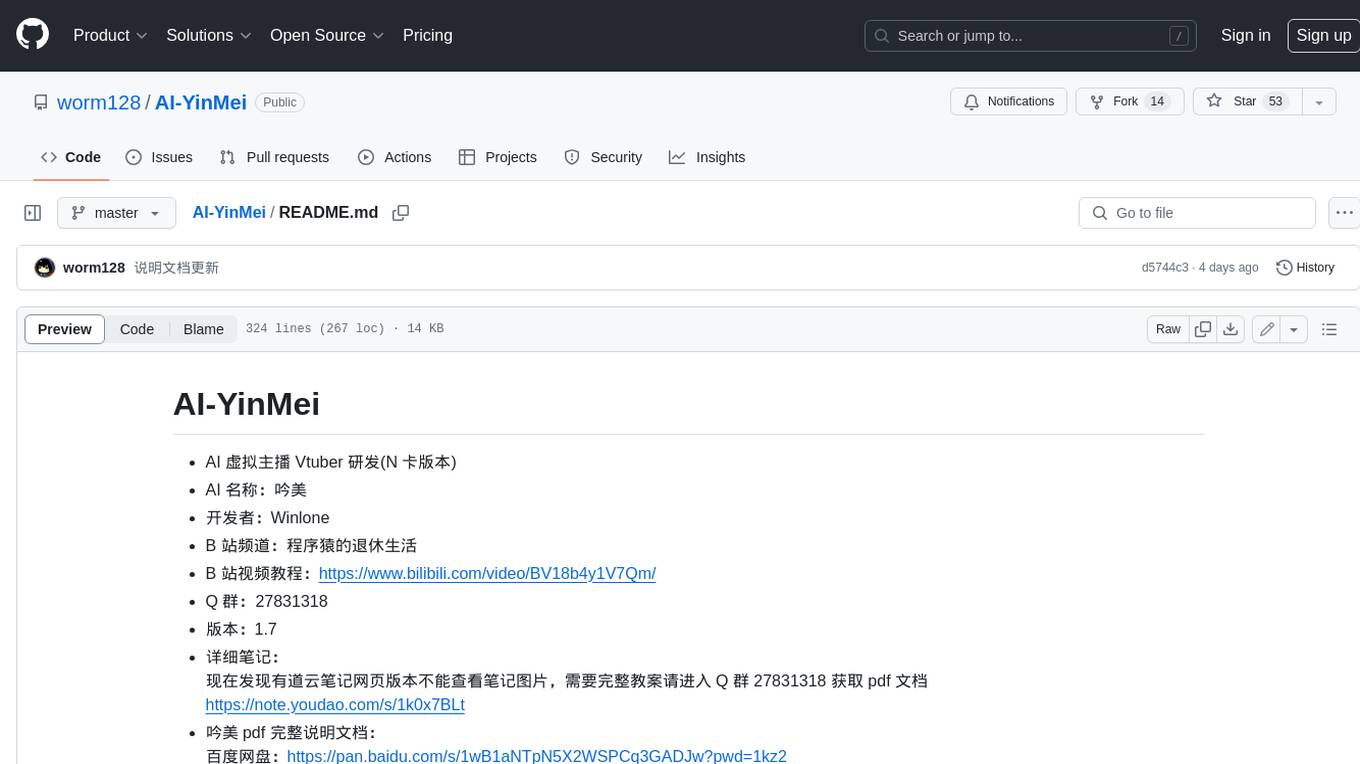
AI-YinMei
AI-YinMei is an AI virtual anchor Vtuber development tool (N card version). It supports fastgpt knowledge base chat dialogue, a complete set of solutions for LLM large language models: [fastgpt] + [one-api] + [Xinference], supports docking bilibili live broadcast barrage reply and entering live broadcast welcome speech, supports Microsoft edge-tts speech synthesis, supports Bert-VITS2 speech synthesis, supports GPT-SoVITS speech synthesis, supports expression control Vtuber Studio, supports painting stable-diffusion-webui output OBS live broadcast room, supports painting picture pornography public-NSFW-y-distinguish, supports search and image search service duckduckgo (requires magic Internet access), supports image search service Baidu image search (no magic Internet access), supports AI reply chat box [html plug-in], supports AI singing Auto-Convert-Music, supports playlist [html plug-in], supports dancing function, supports expression video playback, supports head touching action, supports gift smashing action, supports singing automatic start dancing function, chat and singing automatic cycle swing action, supports multi scene switching, background music switching, day and night automatic switching scene, supports open singing and painting, let AI automatically judge the content.