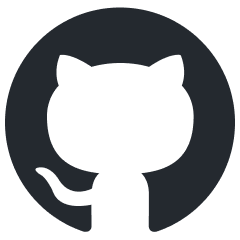
finite-monkey-engine
AI engine for smart contract audit
Stars: 177
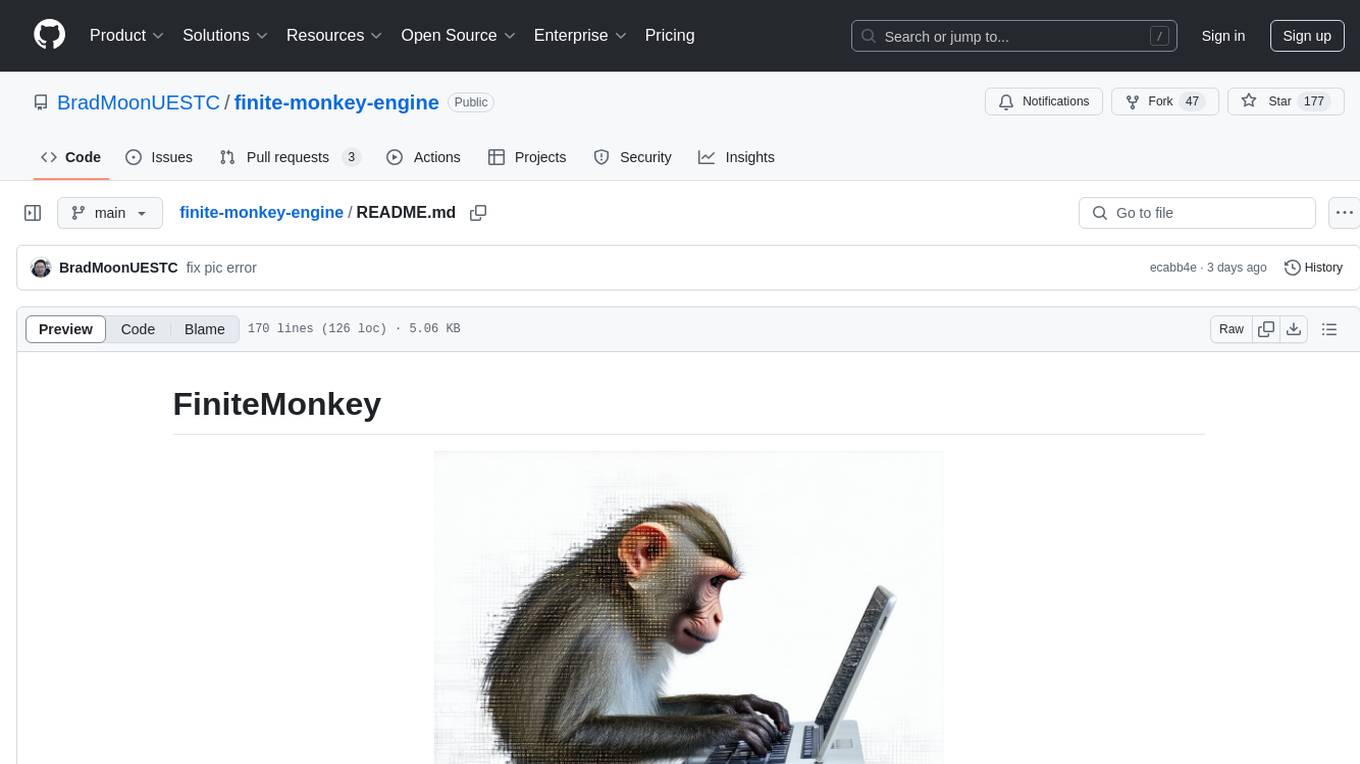
FiniteMonkey is an advanced vulnerability mining engine powered purely by GPT, requiring no prior knowledge base or fine-tuning. Its effectiveness significantly surpasses most current related research approaches. The tool is task-driven, prompt-driven, and focuses on prompt design, leveraging 'deception' and hallucination as key mechanics. It has helped identify vulnerabilities worth over $60,000 in bounties. The tool requires PostgreSQL database, OpenAI API access, and Python environment for setup. It supports various languages like Solidity, Rust, Python, Move, Cairo, Tact, Func, Java, and Fake Solidity for scanning. FiniteMonkey is best suited for logic vulnerability mining in real projects, not recommended for academic vulnerability testing. GPT-4-turbo is recommended for optimal results with an average scan time of 2-3 hours for medium projects. The tool provides detailed scanning results guide and implementation tips for users.
README:
FiniteMonkey is an advanced vulnerability mining engine powered purely by GPT, requiring no prior knowledge base or fine-tuning. Its effectiveness significantly surpasses most current related research approaches.
- Task-driven, not question-driven
- Prompt-driven, not code-driven
- Focus on prompt design, not model design
- Leveraging "deception" and hallucination as key mechanics
As of May 2024, this tool has helped identify vulnerabilities worth over $60,000 in bounties.
2024.11.19: Version 1.0 released - Demonstrating feasibility of LLM-based auditing and productization
Earlier Updates:
- 2024.08.02: Project renamed to finite-monkey-engine
- 2024.08.01: Added support for func, tact
- 2024.07.23: Added support for cairo, move
- 2024.07.01: Updated license
- 2024.06.01: Added Python language support
- 2024.05.18: Improved false positive reduction (~20%)
- 2024.05.16: Added cross-contract vulnerability confirmation
- 2024.04.29: Added basic Rust language support
- PostgreSQL database
- OpenAI API access
- Python environment
-
Place project under
src/dataset/agent-v1-c4
-
Configure project in
datasets.json
:
{
"StEverVault2": {
"path": "StEverVault",
"files": [],
"functions": []
}
}
-
Create database using
src/db.sql
-
Configure
.env
:
# Database Connection
DATABASE_URL=postgresql://user:password@localhost:5432/dbname
# API Settings
OPENAI_API_BASE="api.example.com"
OPENAI_API_KEY=sk-your-api-key-here
# Model Settings
VUL_MODEL_ID=gpt-4-turbo
CLAUDE_MODEL=claude-3-5-sonnet-20240620
# Azure Configuration
AZURE_API_KEY="your-azure-api-key"
AZURE_API_BASE="https://your-resource.openai.azure.com/"
AZURE_API_VERSION="2024-02-15-preview"
AZURE_DEPLOYMENT_NAME="your-deployment"
# API Choice
AZURE_OR_OPENAI="OPENAI" # Options: OPENAI, AZURE, CLAUDE
# Scan Parameters
BUSINESS_FLOW_COUNT=4
SWITCH_FUNCTION_CODE=False
SWITCH_BUSINESS_CODE=True
# Scan Focus Configuration
# SCAN_FOCUS=[
# "Contract1",
# "Contract2",
# "Contract3"
# ]
- Solidity (.sol)
- Rust (.rs)
- Python (.py)
- Move (.move)
- Cairo (.cairo)
- Tact (.tact)
- Func (.fc)
- Java (.java)
- Fake Solidity (.fr) - For scanning Solidity pseudocode
- Scans can be resumed if interrupted due to network/API issues by rerunning main.py with same project_id
- Strongly recommend using GPT-4-turbo - GPT-3.5 and GPT-4.0 have inferior reasoning capabilities
- Results are marked with detailed annotations and Chinese explanations:
- Prioritize entries with
"result":"yes"
in result column - Filter for
"dont need In-project other contract"
in category column - Check business_flow_code column for specific code
- Reference name column for code locations
- Prioritize entries with
- Best suited for logic vulnerability mining in real projects
- Not recommended for academic vulnerability testing
- GPT-4-turbo recommended for optimal results
- Average scan time: 2-3 hours for medium projects
- Cost estimate: $20-30 for medium projects with 10 iterations
- Current false positive rate: 30-65% depending on project size
- GPT-4 provides better results, GPT-3 not thoroughly tested
- The tricky prompt theory can be adapted for any language with minor modifications
- ANTLR AST parsing support recommended for better code slicing results
- Currently supports Solidity with plans for expansion
- Code structure optimization
- Additional language support
- Documentation and code analysis
- Command line interface implementation
- Excellent at code comprehension and logic vulnerability detection
- Less effective for control flow vulnerability detection
- Designed for real-world projects rather than academic test cases
- Each scan preserves progress automatically
- GPT-4-turbo provides optimal performance compared to other models
- Medium projects with 10 iterations take approximately 2.5 hours
- Results include detailed categorization and Chinese explanations
Apache License 2.0
Contributions welcome! Please feel free to submit pull requests.
Note: The name is inspired by Large Language Monkeys paper
For Tasks:
Click tags to check more tools for each tasksFor Jobs:
Alternative AI tools for finite-monkey-engine
Similar Open Source Tools
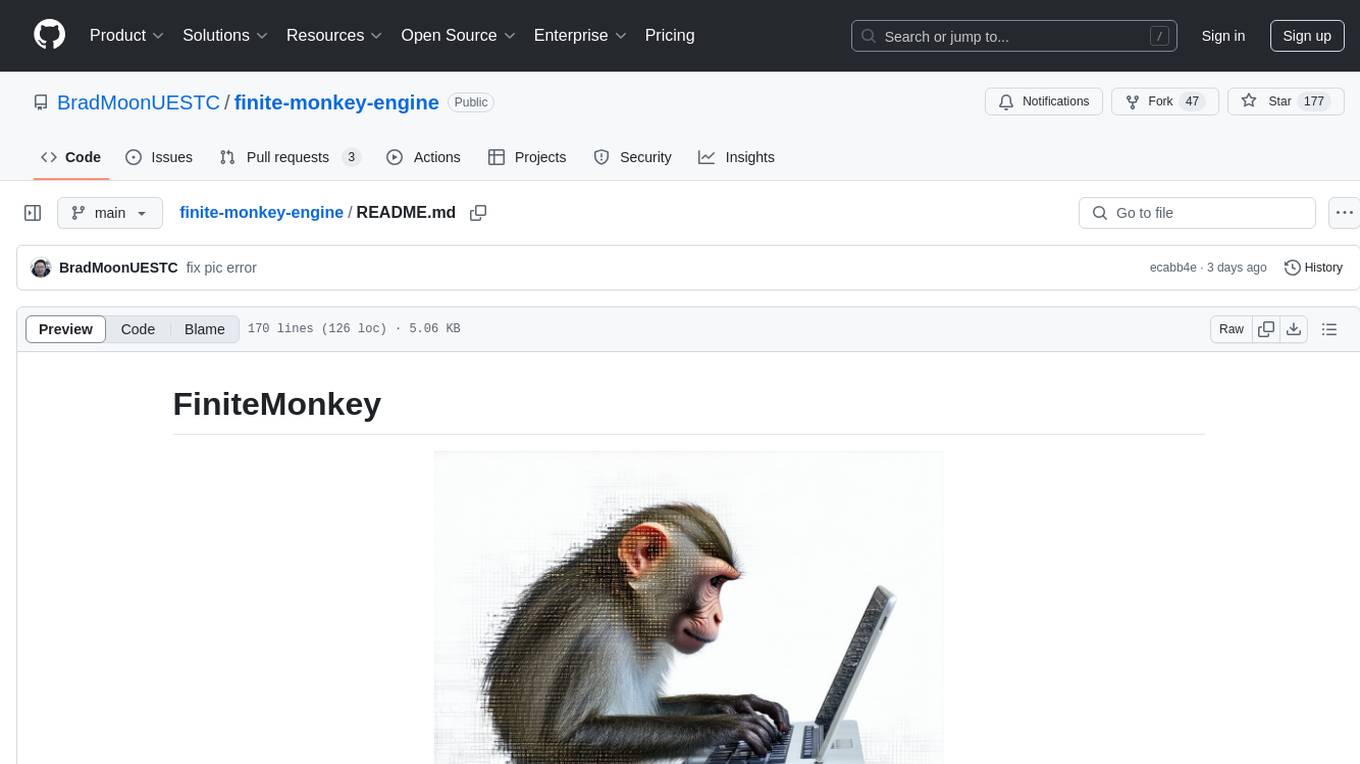
finite-monkey-engine
FiniteMonkey is an advanced vulnerability mining engine powered purely by GPT, requiring no prior knowledge base or fine-tuning. Its effectiveness significantly surpasses most current related research approaches. The tool is task-driven, prompt-driven, and focuses on prompt design, leveraging 'deception' and hallucination as key mechanics. It has helped identify vulnerabilities worth over $60,000 in bounties. The tool requires PostgreSQL database, OpenAI API access, and Python environment for setup. It supports various languages like Solidity, Rust, Python, Move, Cairo, Tact, Func, Java, and Fake Solidity for scanning. FiniteMonkey is best suited for logic vulnerability mining in real projects, not recommended for academic vulnerability testing. GPT-4-turbo is recommended for optimal results with an average scan time of 2-3 hours for medium projects. The tool provides detailed scanning results guide and implementation tips for users.
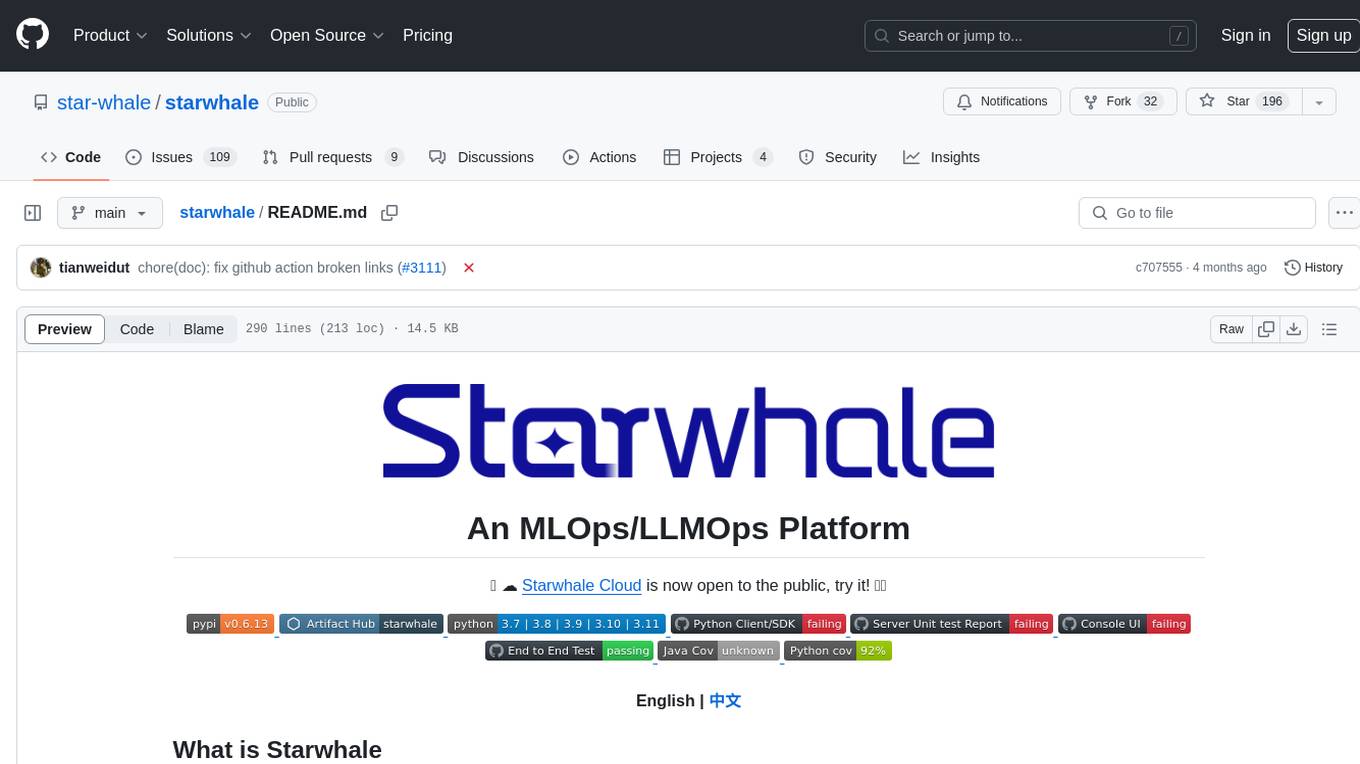
starwhale
Starwhale is an MLOps/LLMOps platform that brings efficiency and standardization to machine learning operations. It streamlines the model development lifecycle, enabling teams to optimize workflows around key areas like model building, evaluation, release, and fine-tuning. Starwhale abstracts Model, Runtime, and Dataset as first-class citizens, providing tailored capabilities for common workflow scenarios including Models Evaluation, Live Demo, and LLM Fine-tuning. It is an open-source platform designed for clarity and ease of use, empowering developers to build customized MLOps features tailored to their needs.
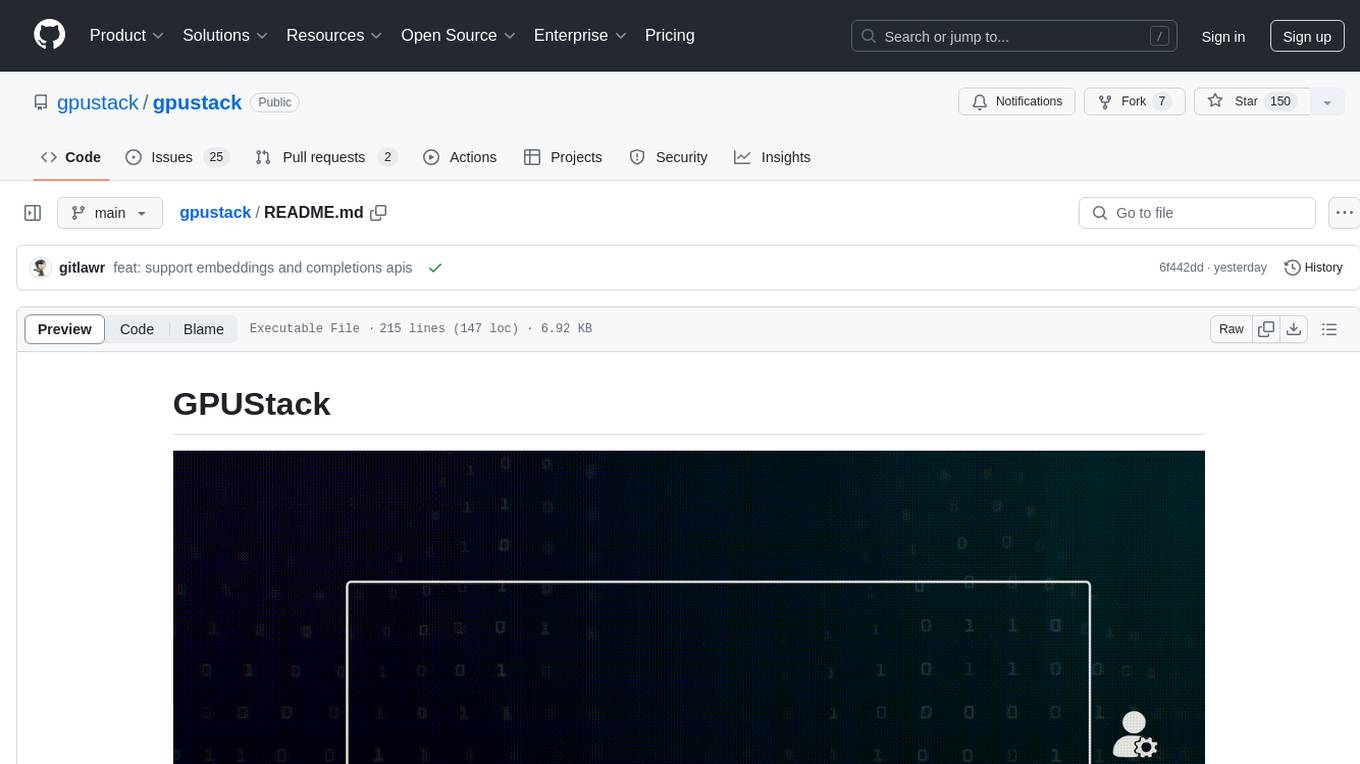
gpustack
GPUStack is an open-source GPU cluster manager designed for running large language models (LLMs). It supports a wide variety of hardware, scales with GPU inventory, offers lightweight Python package with minimal dependencies, provides OpenAI-compatible APIs, simplifies user and API key management, enables GPU metrics monitoring, and facilitates token usage and rate metrics tracking. The tool is suitable for managing GPU clusters efficiently and effectively.
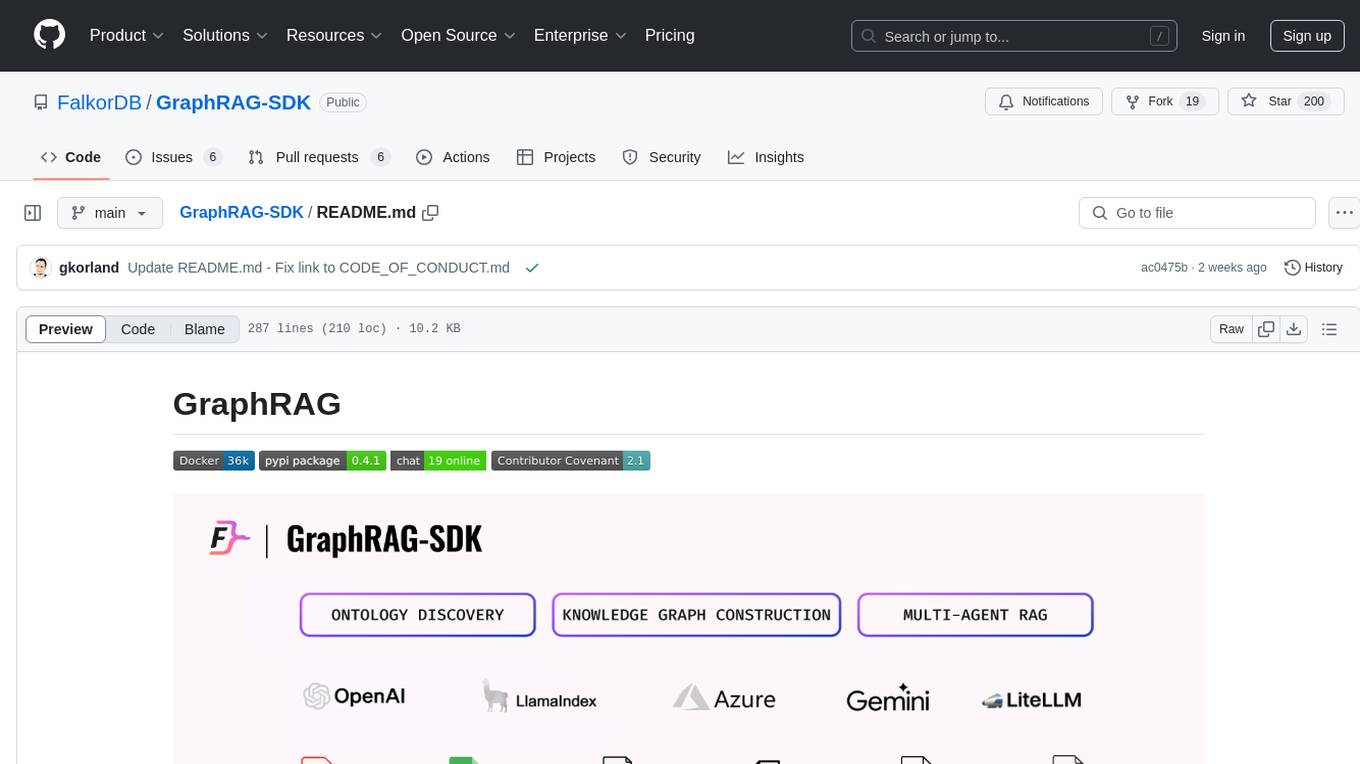
GraphRAG-SDK
Build fast and accurate GenAI applications with GraphRAG SDK, a specialized toolkit for building Graph Retrieval-Augmented Generation (GraphRAG) systems. It integrates knowledge graphs, ontology management, and state-of-the-art LLMs to deliver accurate, efficient, and customizable RAG workflows. The SDK simplifies the development process by automating ontology creation, knowledge graph agent creation, and query handling, enabling users to interact and query their knowledge graphs effectively. It supports multi-agent systems and orchestrates agents specialized in different domains. The SDK is optimized for FalkorDB, ensuring high performance and scalability for large-scale applications. By leveraging knowledge graphs, it enables semantic relationships and ontology-driven queries that go beyond standard vector similarity, enhancing retrieval-augmented generation capabilities.
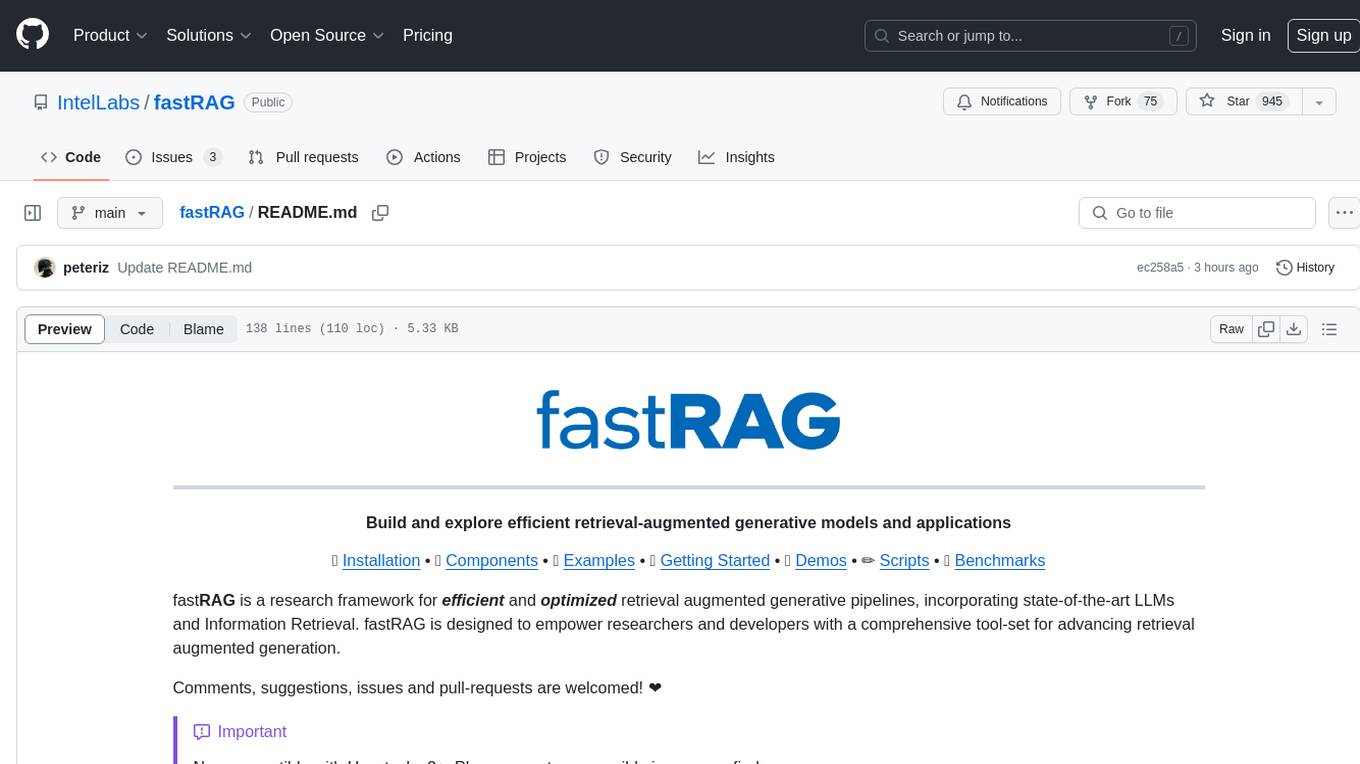
fastRAG
fastRAG is a research framework designed to build and explore efficient retrieval-augmented generative models. It incorporates state-of-the-art Large Language Models (LLMs) and Information Retrieval to empower researchers and developers with a comprehensive tool-set for advancing retrieval augmented generation. The framework is optimized for Intel hardware, customizable, and includes key features such as optimized RAG pipelines, efficient components, and RAG-efficient components like ColBERT and Fusion-in-Decoder (FiD). fastRAG supports various unique components and backends for running LLMs, making it a versatile tool for research and development in the field of retrieval-augmented generation.
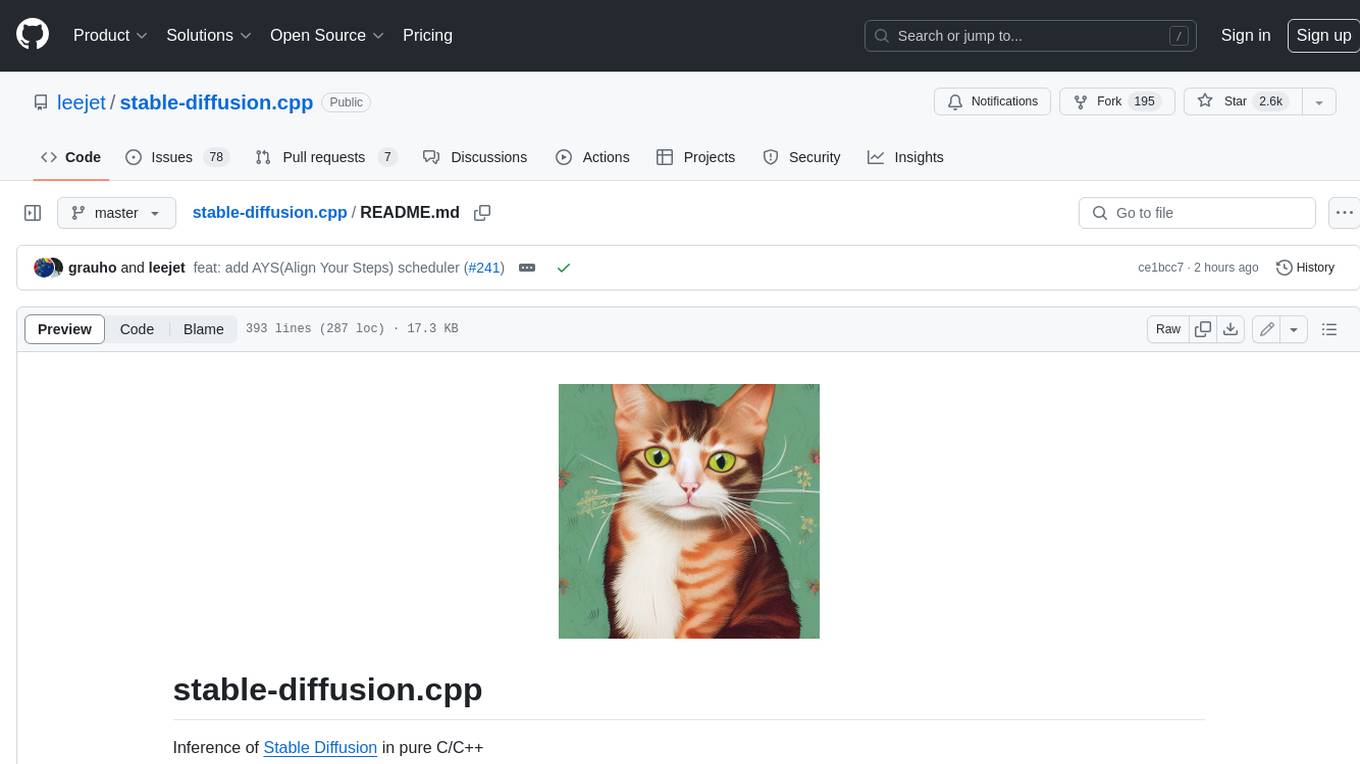
stable-diffusion.cpp
The stable-diffusion.cpp repository provides an implementation for inferring stable diffusion in pure C/C++. It offers features such as support for different versions of stable diffusion, lightweight and dependency-free implementation, various quantization support, memory-efficient CPU inference, GPU acceleration, and more. Users can download the built executable program or build it manually. The repository also includes instructions for downloading weights, building from scratch, using different acceleration methods, running the tool, converting weights, and utilizing various features like Flash Attention, ESRGAN upscaling, PhotoMaker support, and more. Additionally, it mentions future TODOs and provides information on memory requirements, bindings, UIs, contributors, and references.
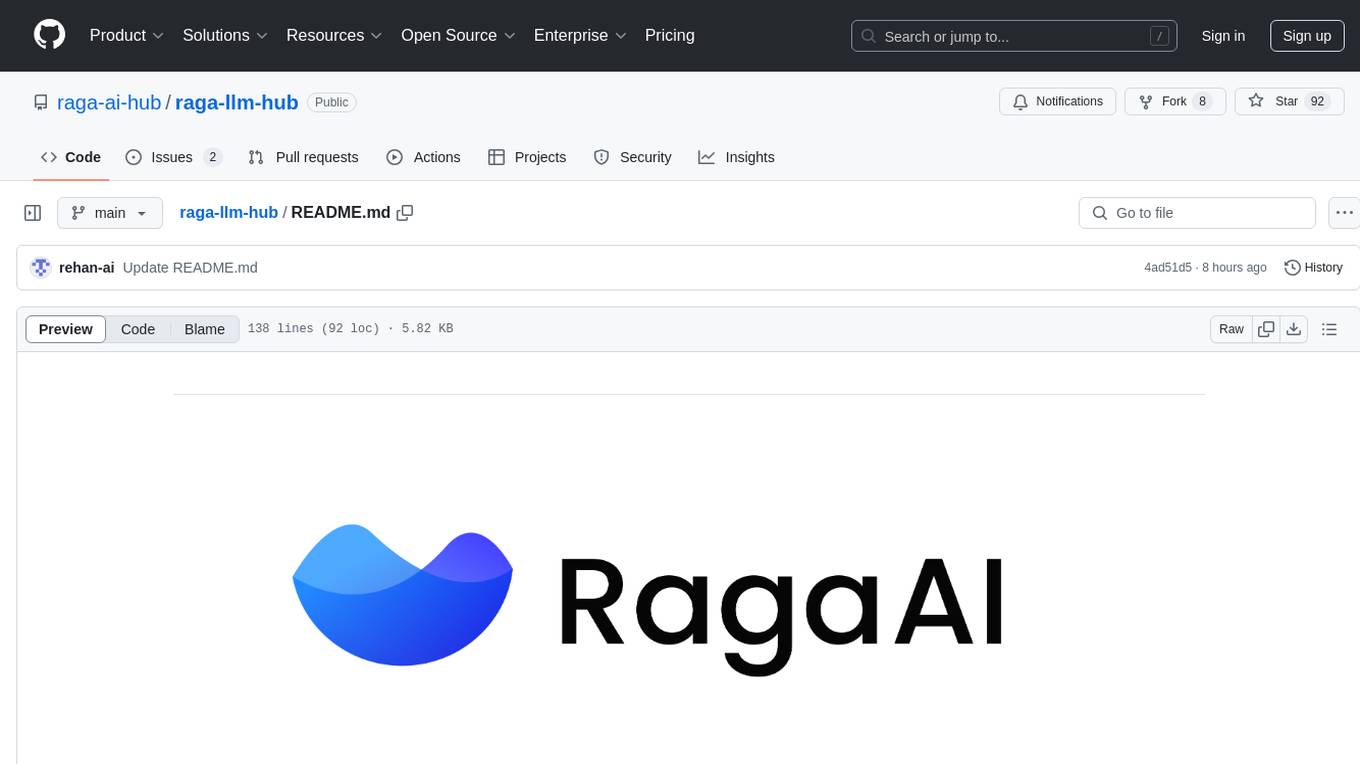
raga-llm-hub
Raga LLM Hub is a comprehensive evaluation toolkit for Language and Learning Models (LLMs) with over 100 meticulously designed metrics. It allows developers and organizations to evaluate and compare LLMs effectively, establishing guardrails for LLMs and Retrieval Augmented Generation (RAG) applications. The platform assesses aspects like Relevance & Understanding, Content Quality, Hallucination, Safety & Bias, Context Relevance, Guardrails, and Vulnerability scanning, along with Metric-Based Tests for quantitative analysis. It helps teams identify and fix issues throughout the LLM lifecycle, revolutionizing reliability and trustworthiness.
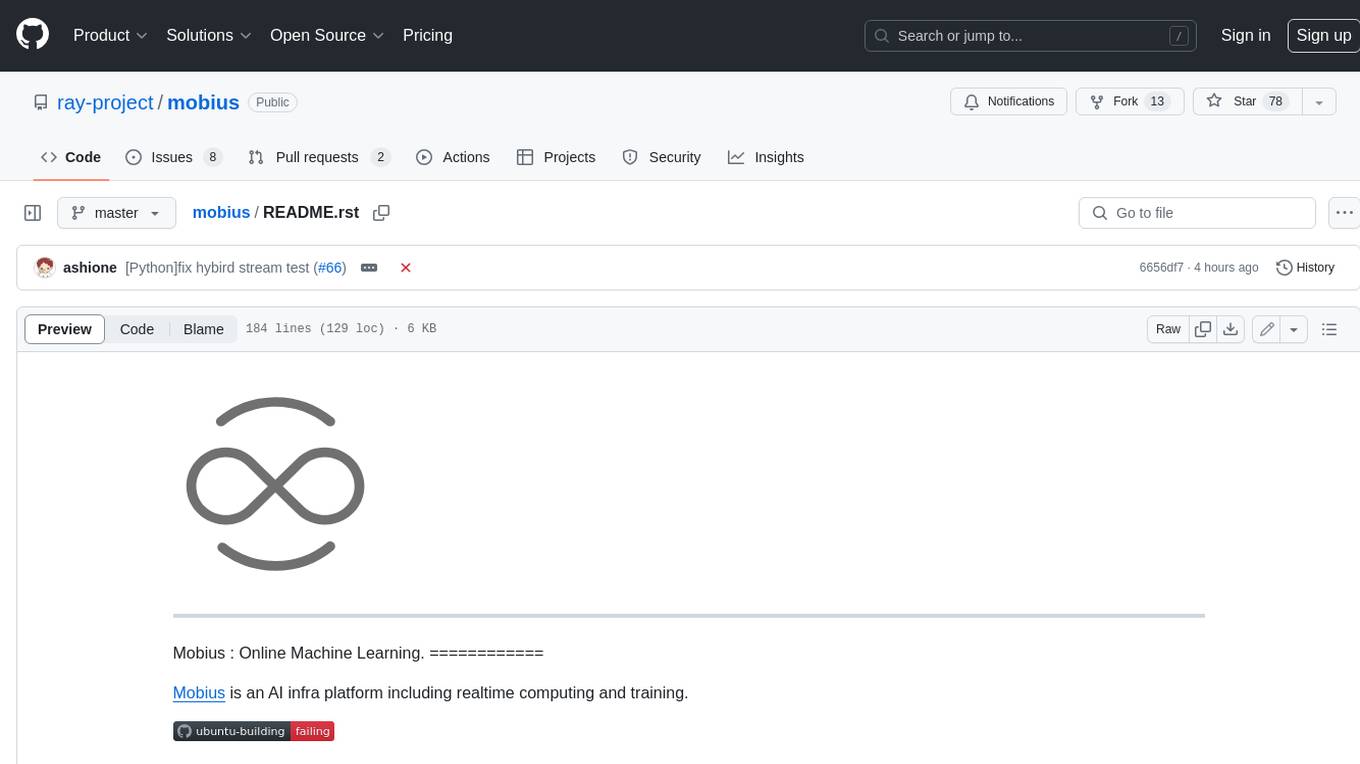
mobius
Mobius is an AI infra platform including realtime computing and training. It is built on Ray, a distributed computing framework, and provides a number of features that make it well-suited for online machine learning tasks. These features include: * **Cross Language**: Mobius can run in multiple languages (only Python and Java are supported currently) with high efficiency. You can implement your operator in different languages and run them in one job. * **Single Node Failover**: Mobius has a special failover mechanism that only needs to rollback the failed node itself, in most cases, to recover the job. This is a huge benefit if your job is sensitive about failure recovery time. * **AutoScaling**: Mobius can generate a new graph with different configurations in runtime without stopping the job. * **Fusion Training**: Mobius can combine TensorFlow/Pytorch and streaming, then building an e2e online machine learning pipeline. Mobius is still under development, but it has already been used to power a number of real-world applications, including: * A real-time recommendation system for a major e-commerce company * A fraud detection system for a large financial institution * A personalized news feed for a major news organization If you are interested in using Mobius for your own online machine learning projects, you can find more information in the documentation.
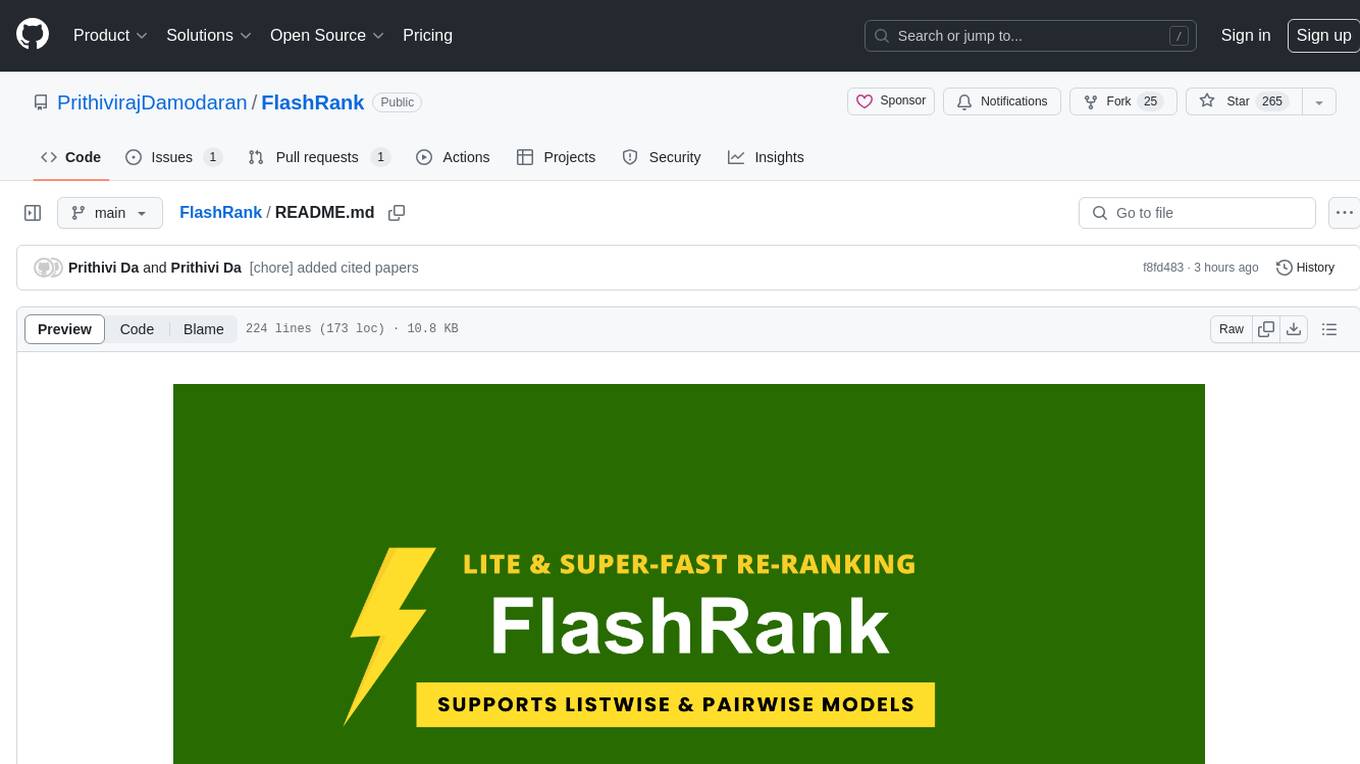
FlashRank
FlashRank is an ultra-lite and super-fast Python library designed to add re-ranking capabilities to existing search and retrieval pipelines. It is based on state-of-the-art Language Models (LLMs) and cross-encoders, offering support for pairwise/pointwise rerankers and listwise LLM-based rerankers. The library boasts the tiniest reranking model in the world (~4MB) and runs on CPU without the need for Torch or Transformers. FlashRank is cost-conscious, with a focus on low cost per invocation and smaller package size for efficient serverless deployments. It supports various models like ms-marco-TinyBERT, ms-marco-MiniLM, rank-T5-flan, ms-marco-MultiBERT, and more, with plans for future model additions. The tool is ideal for enhancing search precision and speed in scenarios where lightweight models with competitive performance are preferred.
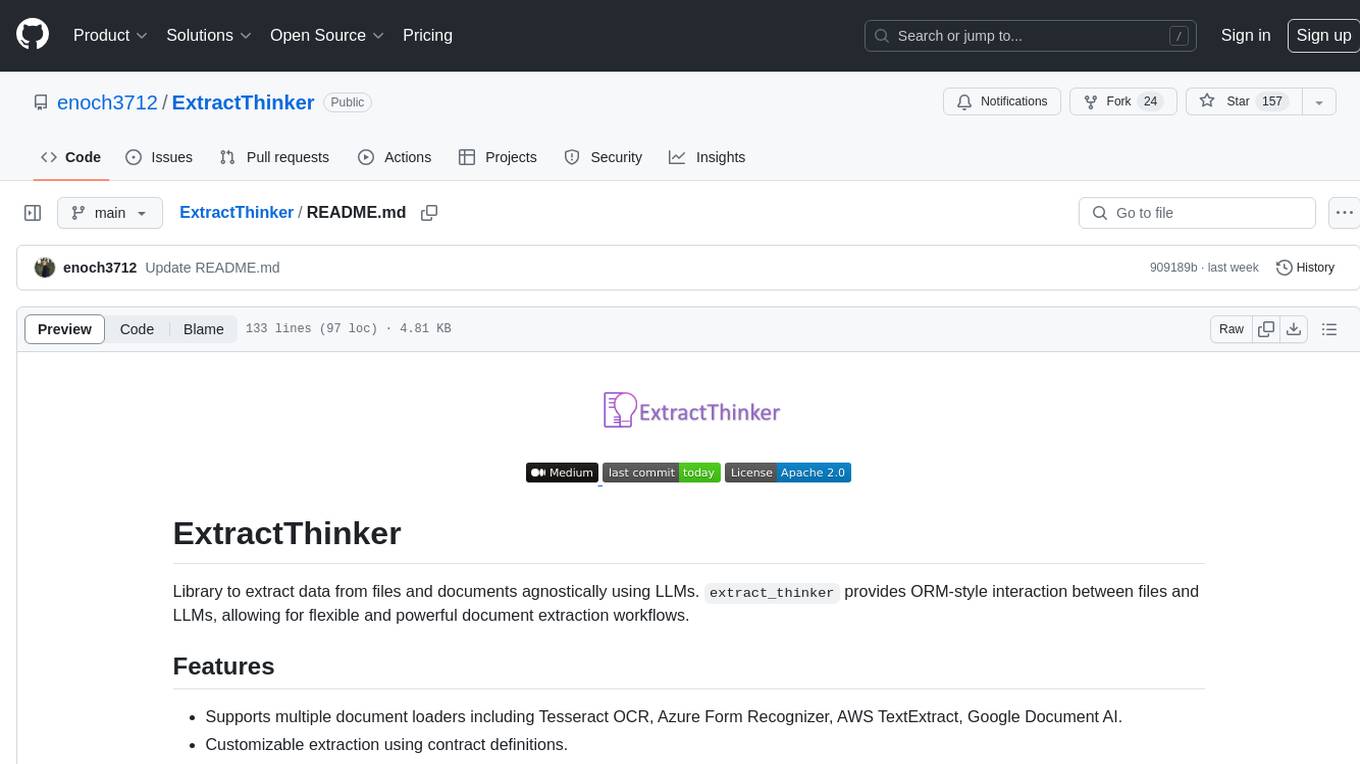
ExtractThinker
ExtractThinker is a library designed for extracting data from files and documents using Language Model Models (LLMs). It offers ORM-style interaction between files and LLMs, supporting multiple document loaders such as Tesseract OCR, Azure Form Recognizer, AWS TextExtract, and Google Document AI. Users can customize extraction using contract definitions, process documents asynchronously, handle various document formats efficiently, and split and process documents. The project is inspired by the LangChain ecosystem and focuses on Intelligent Document Processing (IDP) using LLMs to achieve high accuracy in document extraction tasks.
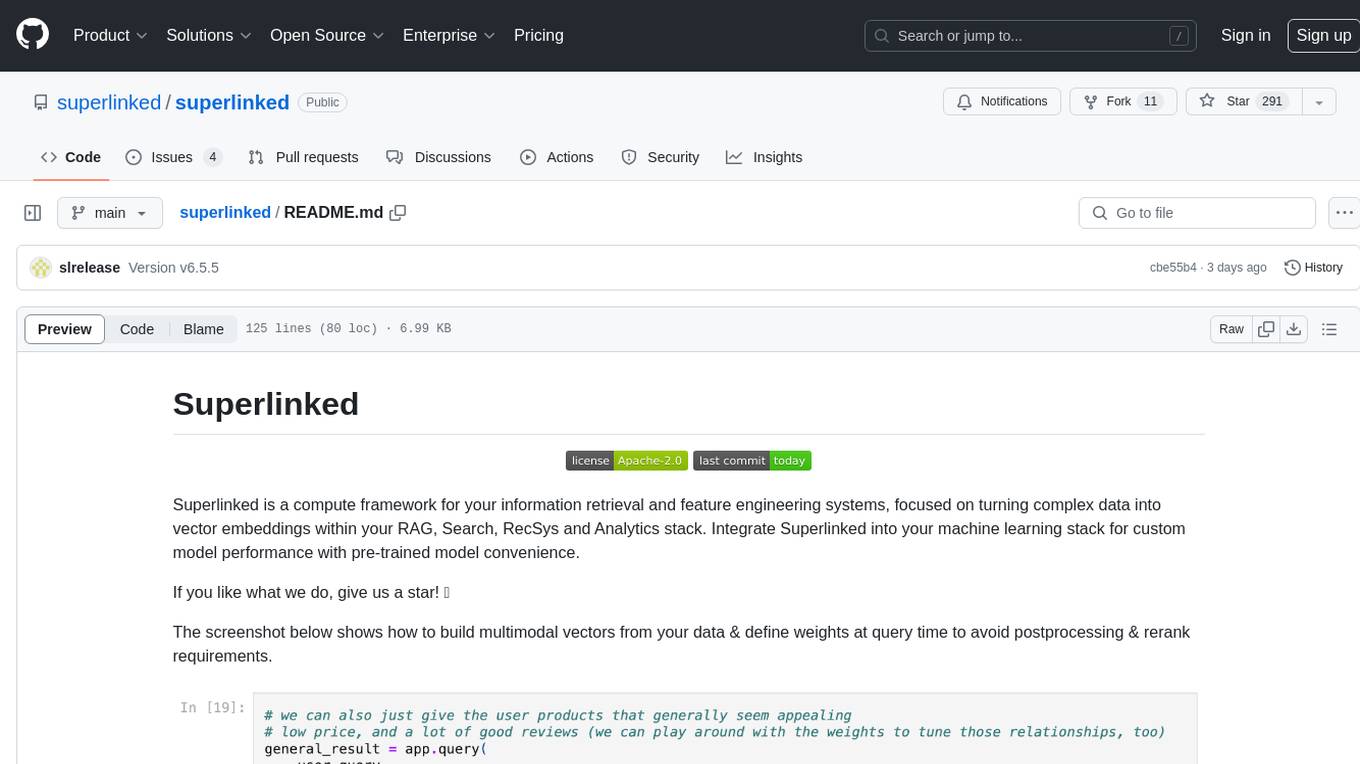
superlinked
Superlinked is a compute framework for information retrieval and feature engineering systems, focusing on converting complex data into vector embeddings for RAG, Search, RecSys, and Analytics stack integration. It enables custom model performance in machine learning with pre-trained model convenience. The tool allows users to build multimodal vectors, define weights at query time, and avoid postprocessing & rerank requirements. Users can explore the computational model through simple scripts and python notebooks, with a future release planned for production usage with built-in data infra and vector database integrations.
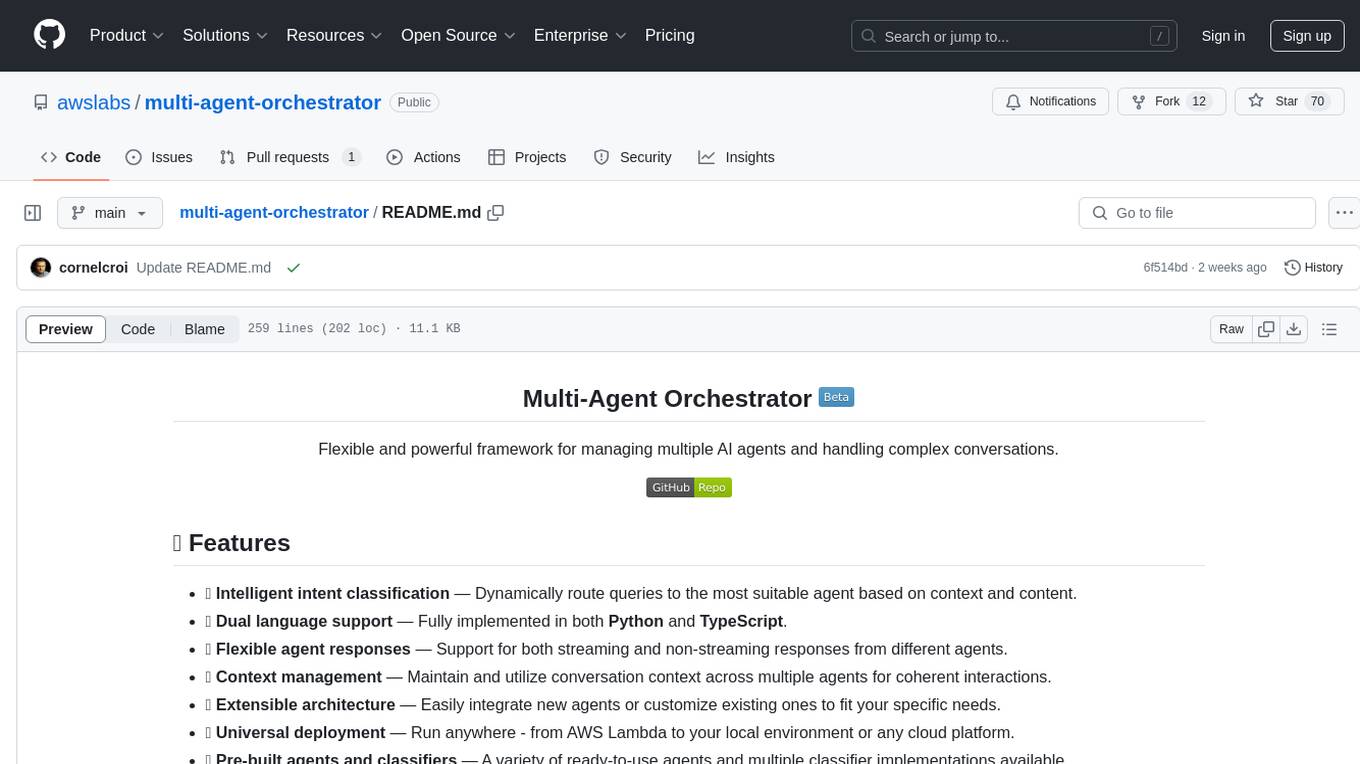
multi-agent-orchestrator
Multi-Agent Orchestrator is a flexible and powerful framework for managing multiple AI agents and handling complex conversations. It intelligently routes queries to the most suitable agent based on context and content, supports dual language implementation in Python and TypeScript, offers flexible agent responses, context management across agents, extensible architecture for customization, universal deployment options, and pre-built agents and classifiers. It is suitable for various applications, from simple chatbots to sophisticated AI systems, accommodating diverse requirements and scaling efficiently.
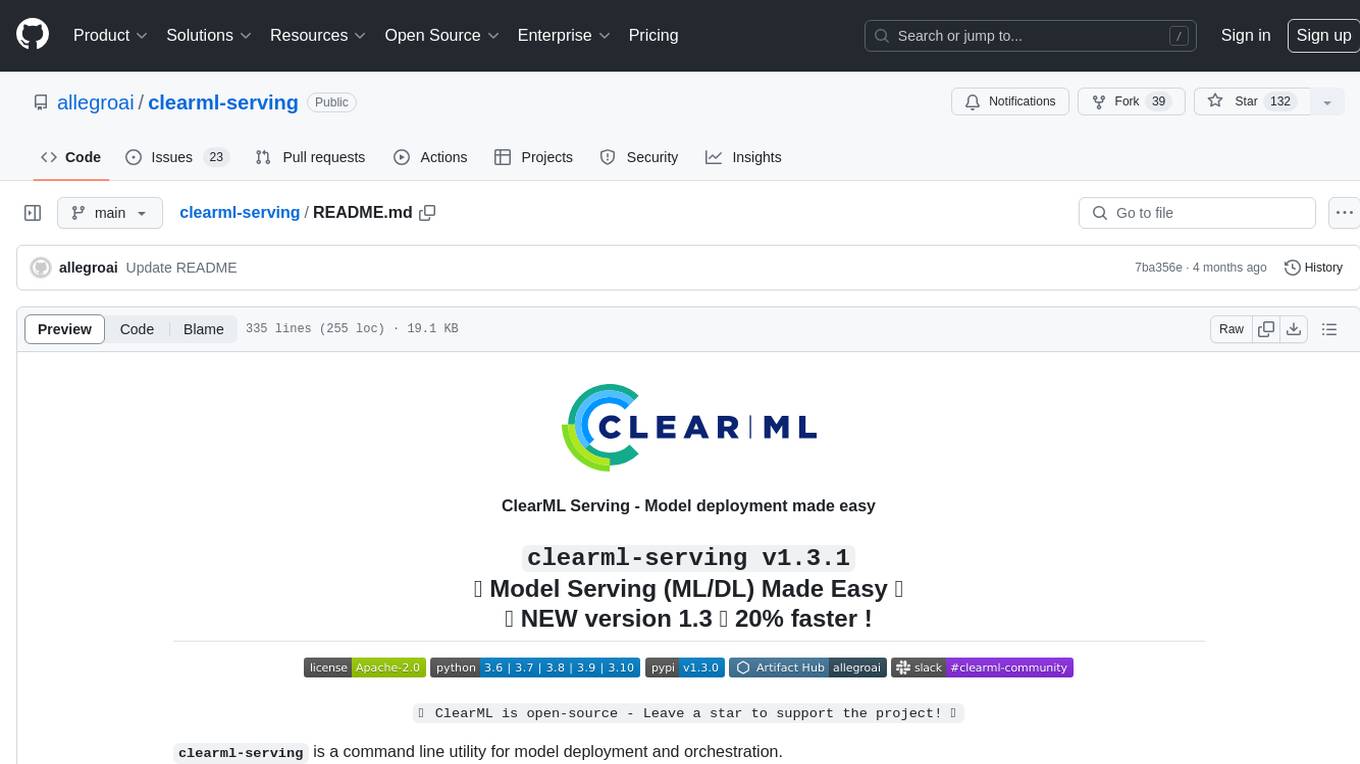
clearml-serving
ClearML Serving is a command line utility for model deployment and orchestration, enabling model deployment including serving and preprocessing code to a Kubernetes cluster or custom container based solution. It supports machine learning models like Scikit Learn, XGBoost, LightGBM, and deep learning models like TensorFlow, PyTorch, ONNX. It provides a customizable RestAPI for serving, online model deployment, scalable solutions, multi-model per container, automatic deployment, canary A/B deployment, model monitoring, usage metric reporting, metric dashboard, and model performance metrics. ClearML Serving is modular, scalable, flexible, customizable, and open source.
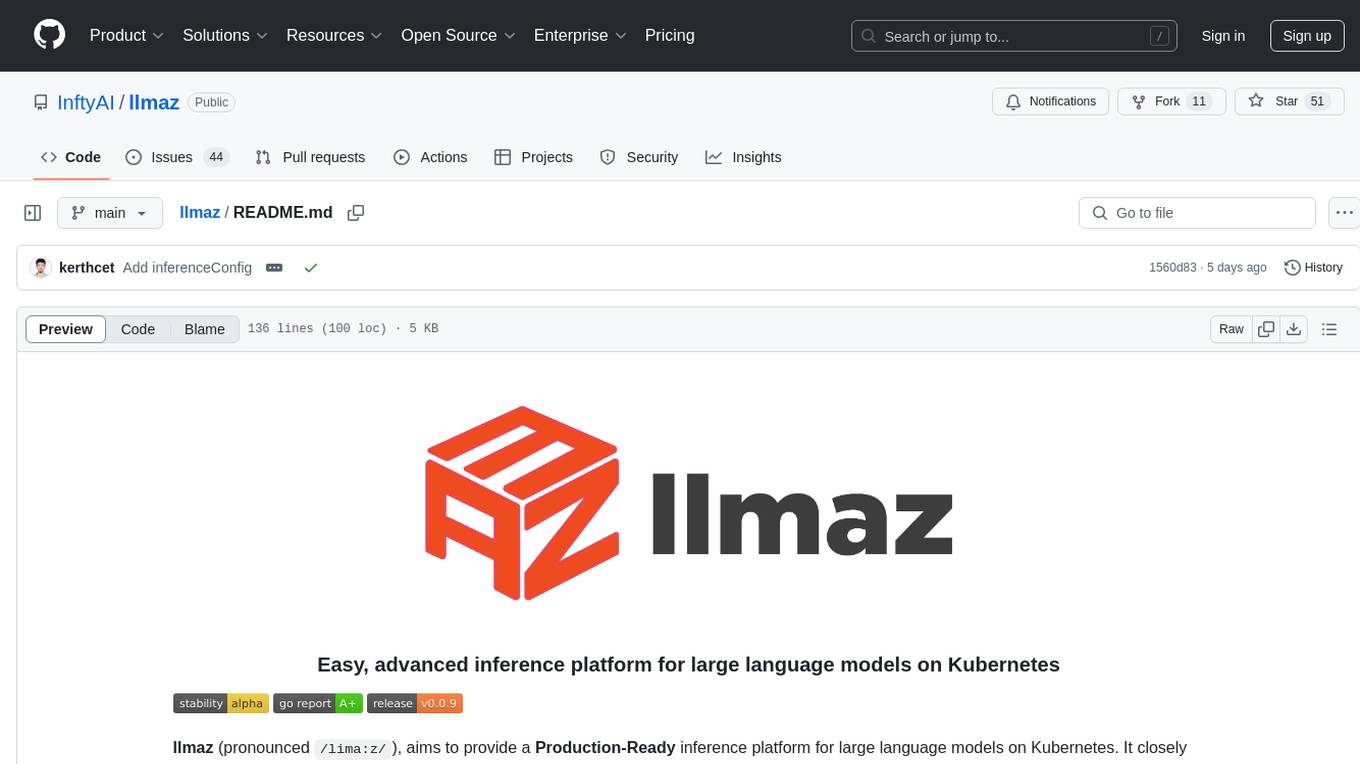
llmaz
llmaz is an easy, advanced inference platform for large language models on Kubernetes. It aims to provide a production-ready solution that integrates with state-of-the-art inference backends. The platform supports efficient model distribution, accelerator fungibility, SOTA inference, various model providers, multi-host support, and scaling efficiency. Users can quickly deploy LLM services with minimal configurations and benefit from a wide range of advanced inference backends. llmaz is designed to optimize cost and performance while supporting cutting-edge researches like Speculative Decoding or Splitwise on Kubernetes.
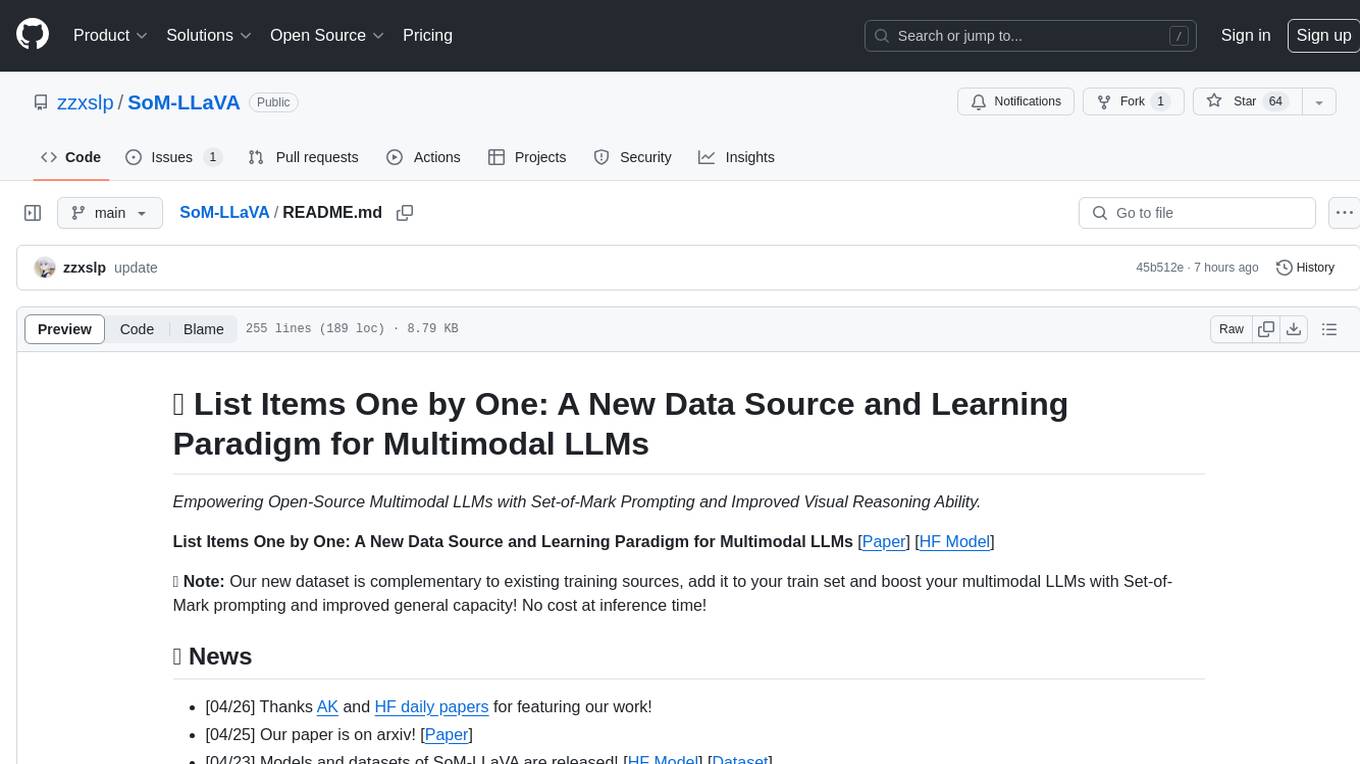
SoM-LLaVA
SoM-LLaVA is a new data source and learning paradigm for Multimodal LLMs, empowering open-source Multimodal LLMs with Set-of-Mark prompting and improved visual reasoning ability. The repository provides a new dataset that is complementary to existing training sources, enhancing multimodal LLMs with Set-of-Mark prompting and improved general capacity. By adding 30k SoM data to the visual instruction tuning stage of LLaVA, the tool achieves 1% to 6% relative improvements on all benchmarks. Users can train SoM-LLaVA via command line and utilize the implementation to annotate COCO images with SoM. Additionally, the tool can be loaded in Huggingface for further usage.
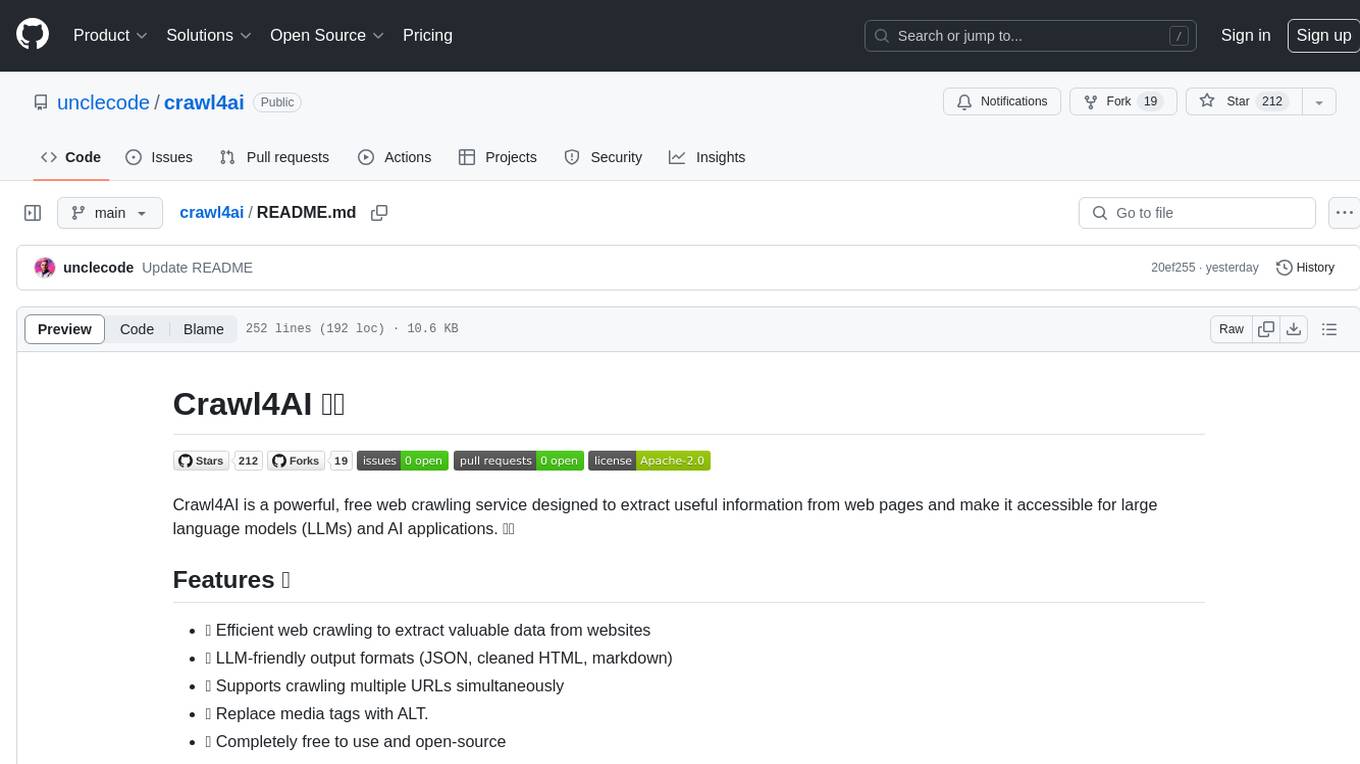
crawl4ai
Crawl4AI is a powerful and free web crawling service that extracts valuable data from websites and provides LLM-friendly output formats. It supports crawling multiple URLs simultaneously, replaces media tags with ALT, and is completely free to use and open-source. Users can integrate Crawl4AI into Python projects as a library or run it as a standalone local server. The tool allows users to crawl and extract data from specified URLs using different providers and models, with options to include raw HTML content, force fresh crawls, and extract meaningful text blocks. Configuration settings can be adjusted in the `crawler/config.py` file to customize providers, API keys, chunk processing, and word thresholds. Contributions to Crawl4AI are welcome from the open-source community to enhance its value for AI enthusiasts and developers.
For similar tasks
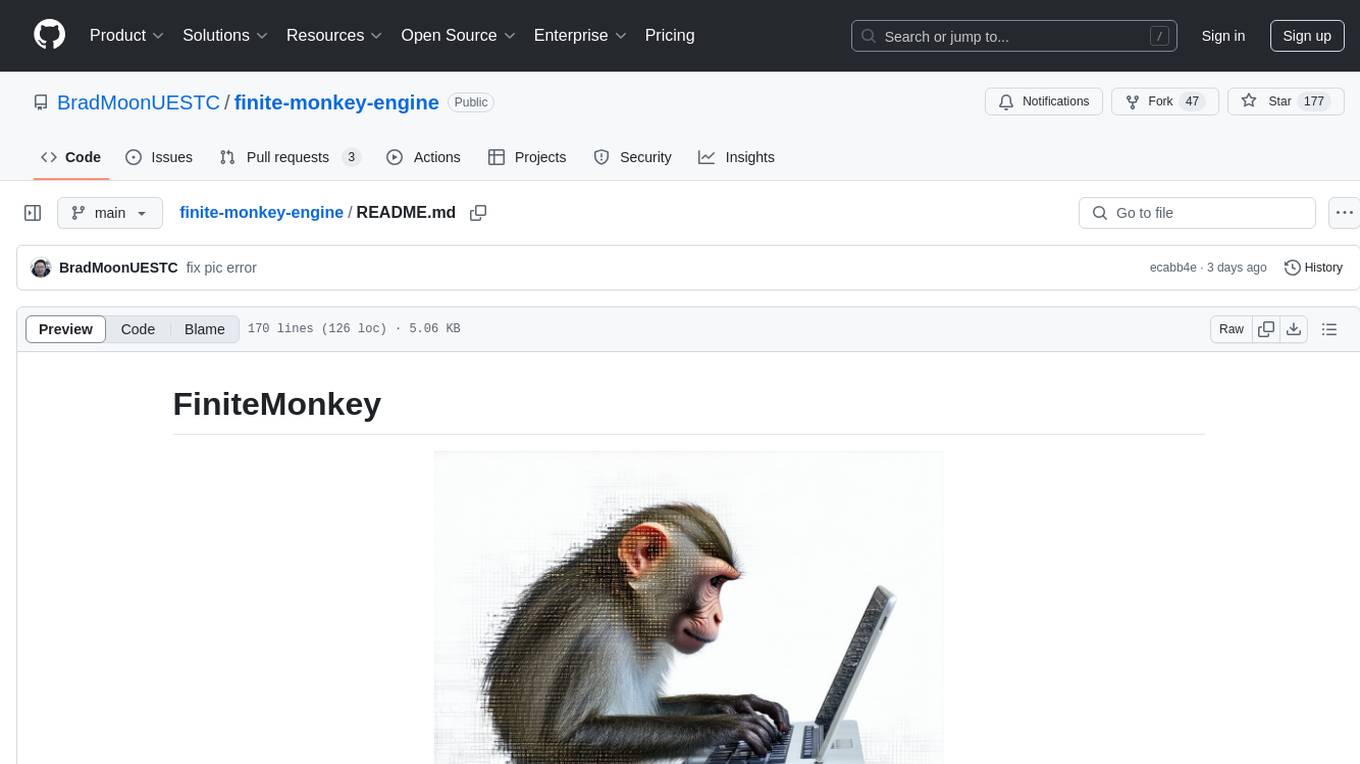
finite-monkey-engine
FiniteMonkey is an advanced vulnerability mining engine powered purely by GPT, requiring no prior knowledge base or fine-tuning. Its effectiveness significantly surpasses most current related research approaches. The tool is task-driven, prompt-driven, and focuses on prompt design, leveraging 'deception' and hallucination as key mechanics. It has helped identify vulnerabilities worth over $60,000 in bounties. The tool requires PostgreSQL database, OpenAI API access, and Python environment for setup. It supports various languages like Solidity, Rust, Python, Move, Cairo, Tact, Func, Java, and Fake Solidity for scanning. FiniteMonkey is best suited for logic vulnerability mining in real projects, not recommended for academic vulnerability testing. GPT-4-turbo is recommended for optimal results with an average scan time of 2-3 hours for medium projects. The tool provides detailed scanning results guide and implementation tips for users.
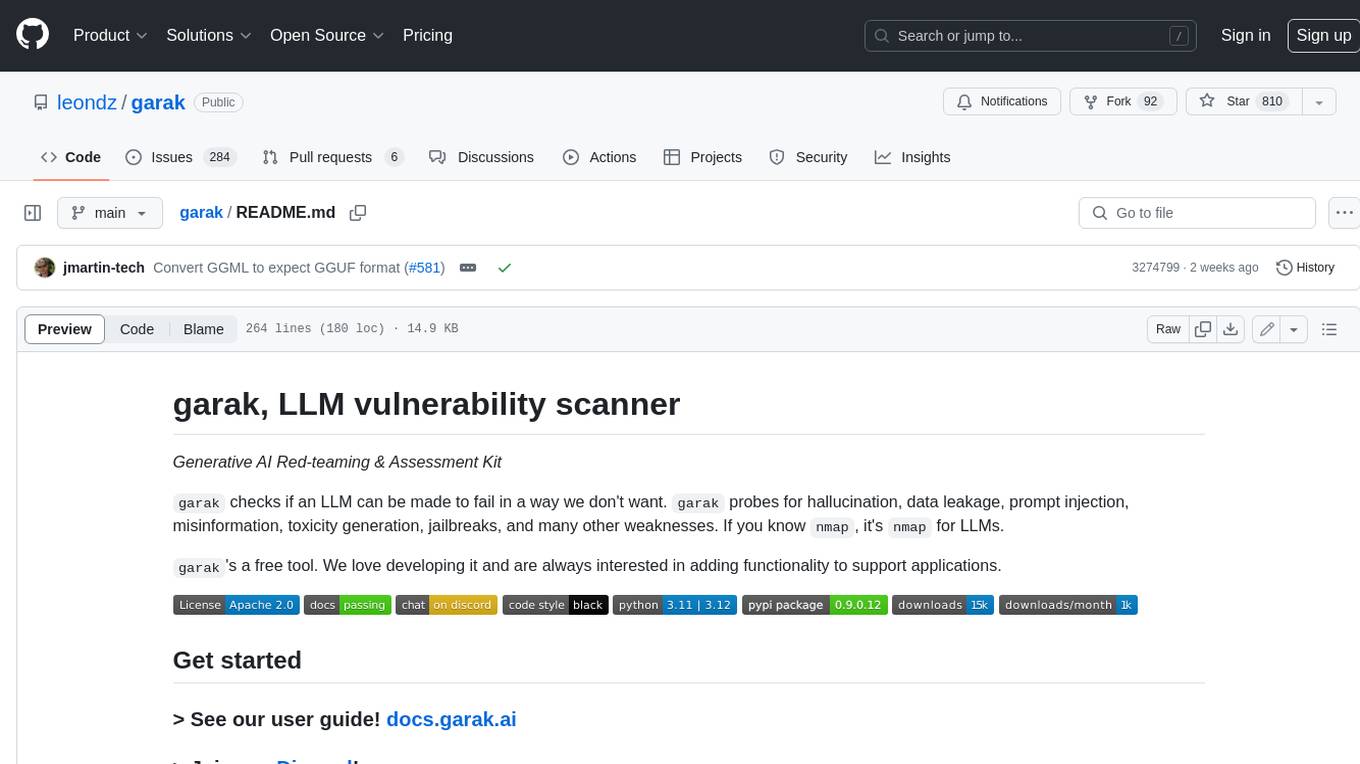
garak
Garak is a free tool that checks if a Large Language Model (LLM) can be made to fail in a way that is undesirable. It probes for hallucination, data leakage, prompt injection, misinformation, toxicity generation, jailbreaks, and many other weaknesses. Garak's a free tool. We love developing it and are always interested in adding functionality to support applications.
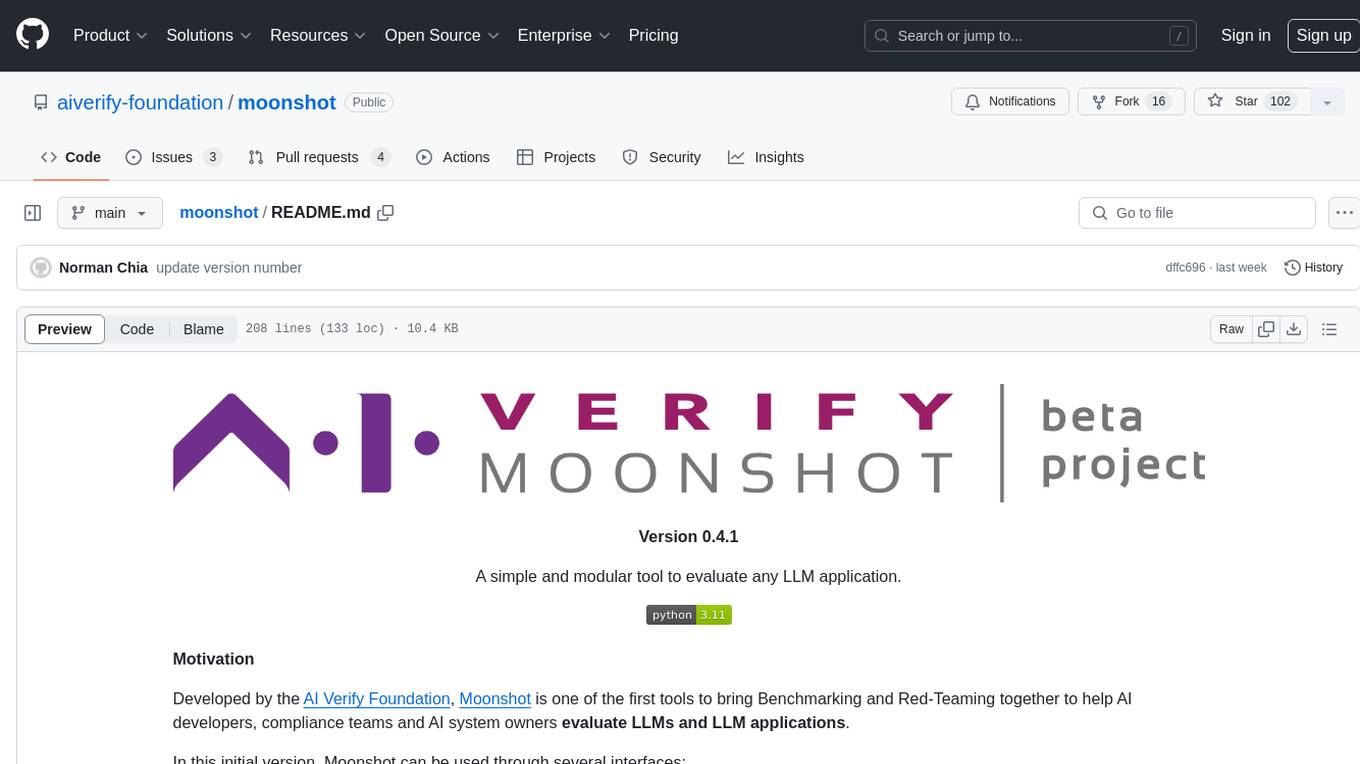
moonshot
Moonshot is a simple and modular tool developed by the AI Verify Foundation to evaluate Language Model Models (LLMs) and LLM applications. It brings Benchmarking and Red-Teaming together to assist AI developers, compliance teams, and AI system owners in assessing LLM performance. Moonshot can be accessed through various interfaces including User-friendly Web UI, Interactive Command Line Interface, and seamless integration into MLOps workflows via Library APIs or Web APIs. It offers features like benchmarking LLMs from popular model providers, running relevant tests, creating custom cookbooks and recipes, and automating Red Teaming to identify vulnerabilities in AI systems.
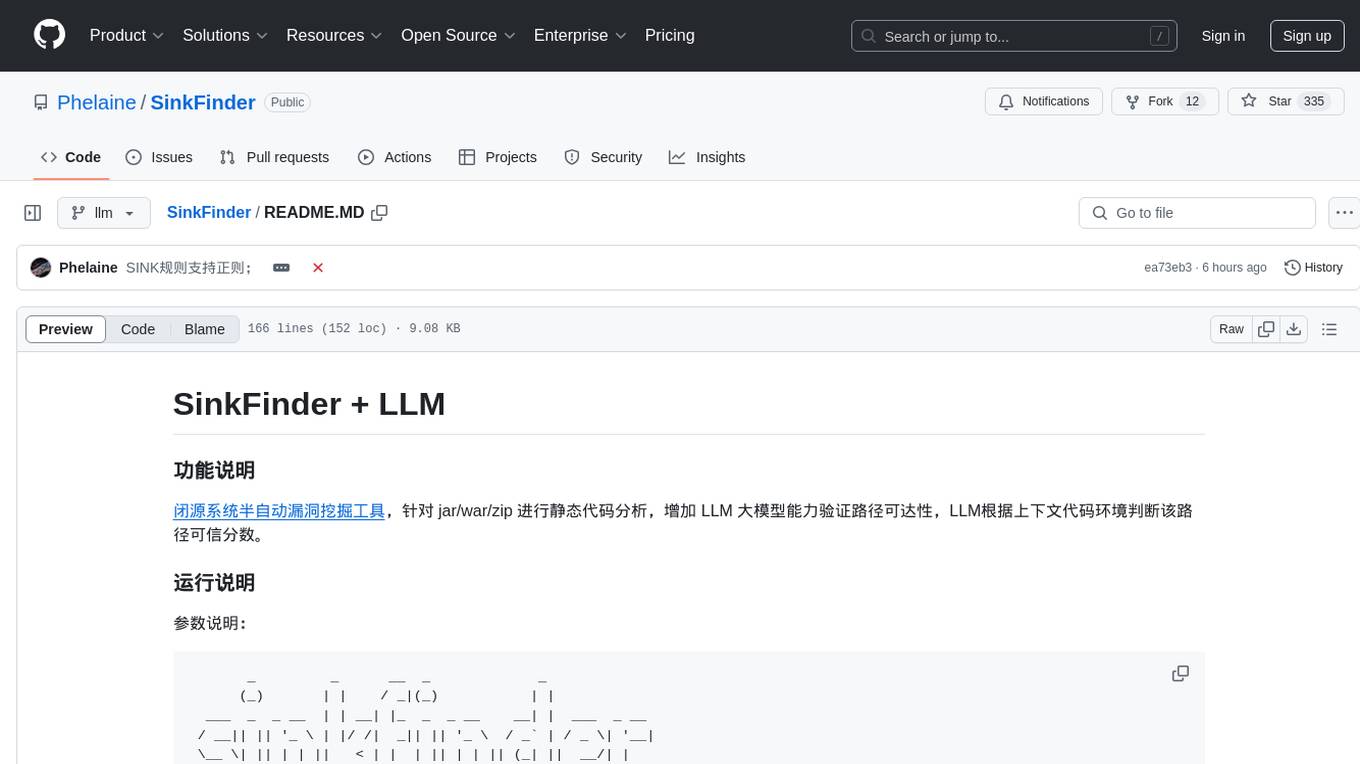
SinkFinder
SinkFinder + LLM is a closed-source semi-automatic vulnerability discovery tool that performs static code analysis on jar/war/zip files. It enhances the capability of LLM large models to verify path reachability and assess the trustworthiness score of the path based on the contextual code environment. Users can customize class and jar exclusions, depth of recursive search, and other parameters through command-line arguments. The tool generates rule.json configuration file after each run and requires configuration of the DASHSCOPE_API_KEY for LLM capabilities. The tool provides detailed logs on high-risk paths, LLM results, and other findings. Rules.json file contains sink rules for various vulnerability types with severity levels and corresponding sink methods.
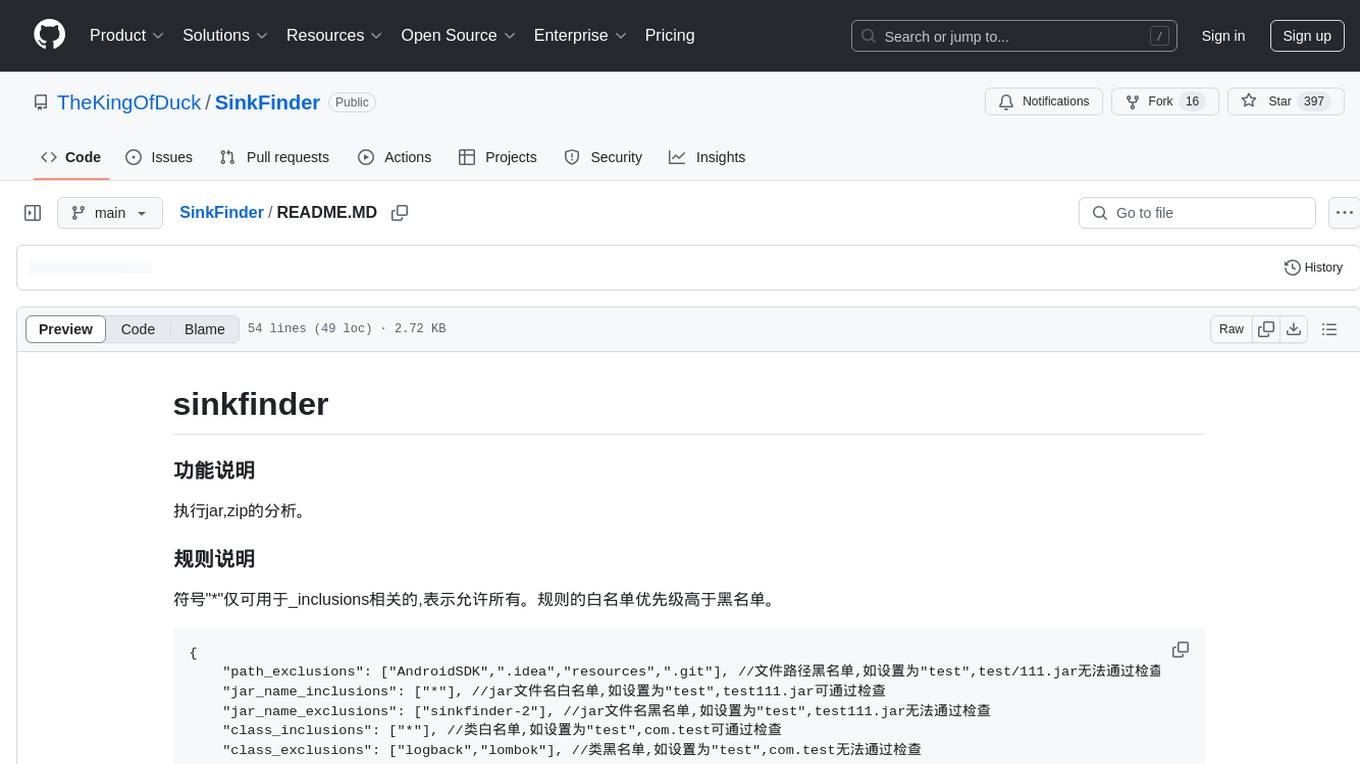
SinkFinder
SinkFinder is a tool designed to analyze jar and zip files for security vulnerabilities. It allows users to define rules for white and blacklisting specific classes and methods that may pose a risk. The tool provides a list of common security sink names along with severity levels and associated vulnerable methods. Users can use SinkFinder to quickly identify potential security issues in their Java applications by scanning for known sink patterns and configurations.
For similar jobs
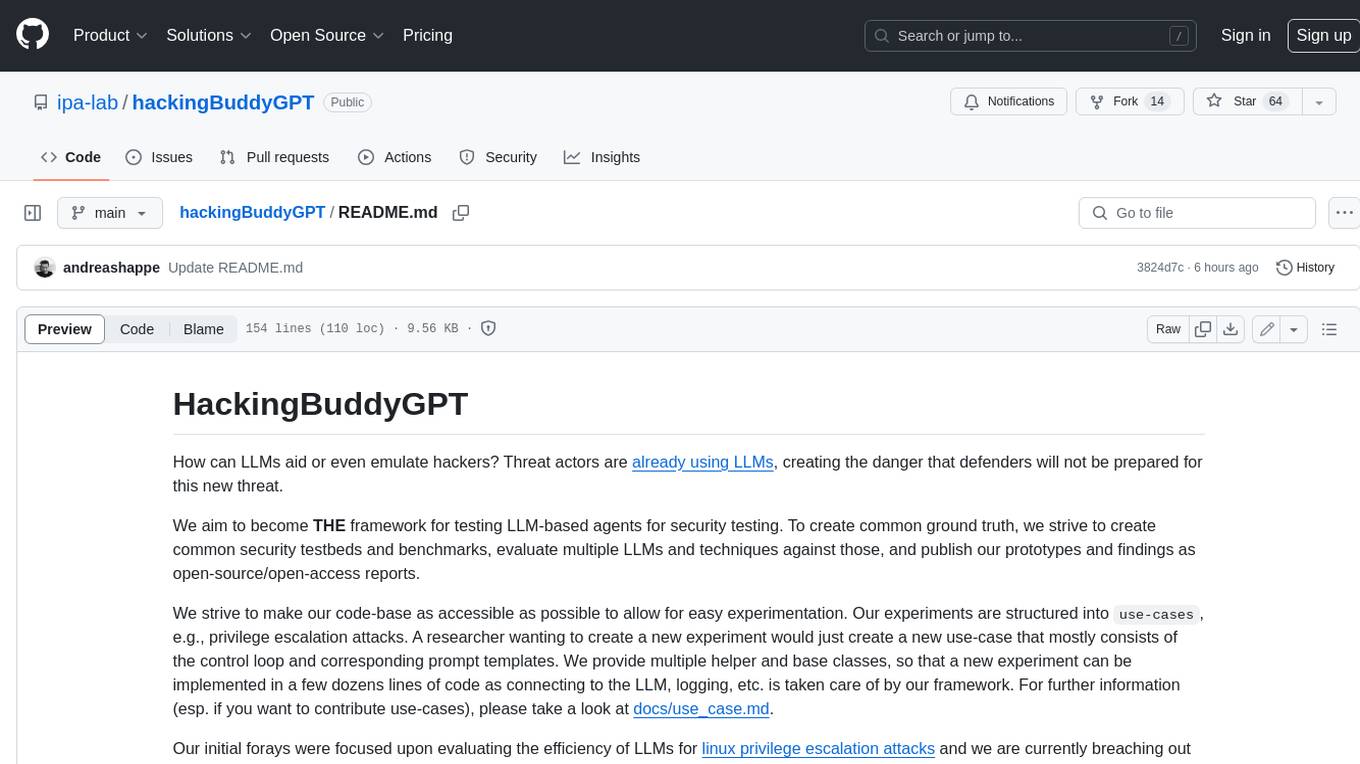
hackingBuddyGPT
hackingBuddyGPT is a framework for testing LLM-based agents for security testing. It aims to create common ground truth by creating common security testbeds and benchmarks, evaluating multiple LLMs and techniques against those, and publishing prototypes and findings as open-source/open-access reports. The initial focus is on evaluating the efficiency of LLMs for Linux privilege escalation attacks, but the framework is being expanded to evaluate the use of LLMs for web penetration-testing and web API testing. hackingBuddyGPT is released as open-source to level the playing field for blue teams against APTs that have access to more sophisticated resources.
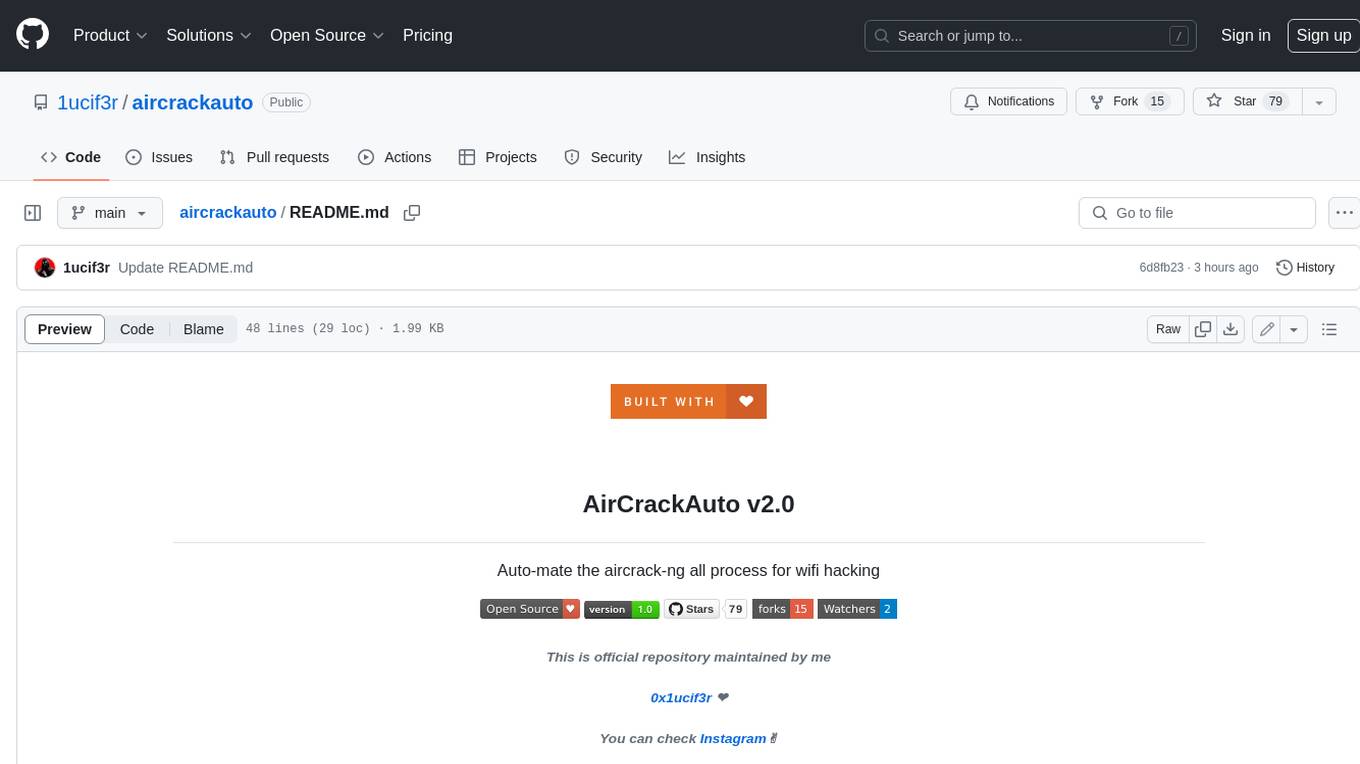
aircrackauto
AirCrackAuto is a tool that automates the aircrack-ng process for Wi-Fi hacking. It is designed to make it easier for users to crack Wi-Fi passwords by automating the process of capturing packets, generating wordlists, and launching attacks. AirCrackAuto is a powerful tool that can be used to crack Wi-Fi passwords in a matter of minutes.
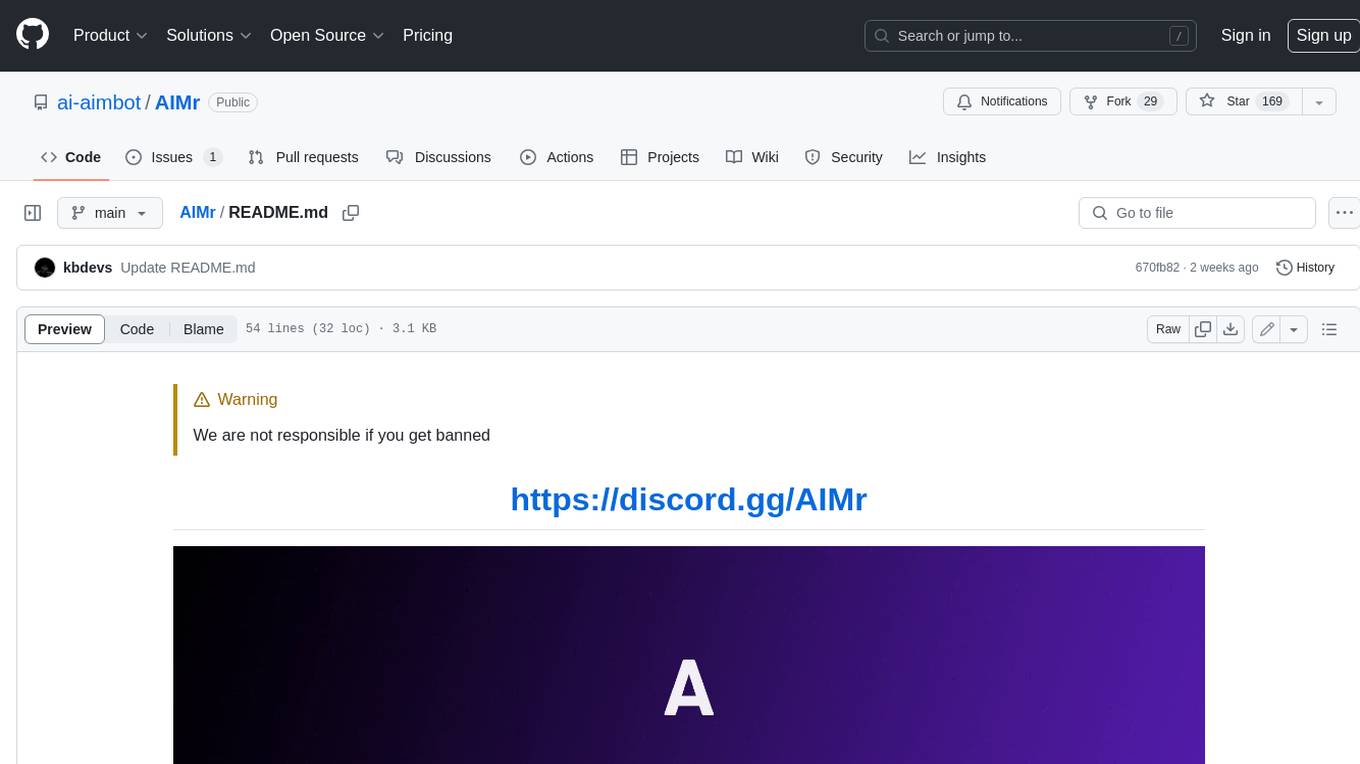
AIMr
AIMr is an AI aimbot tool written in Python that leverages modern technologies to achieve an undetected system with a pleasing appearance. It works on any game that uses human-shaped models. To optimize its performance, users should build OpenCV with CUDA. For Valorant, additional perks in the Discord and an Arduino Leonardo R3 are required.
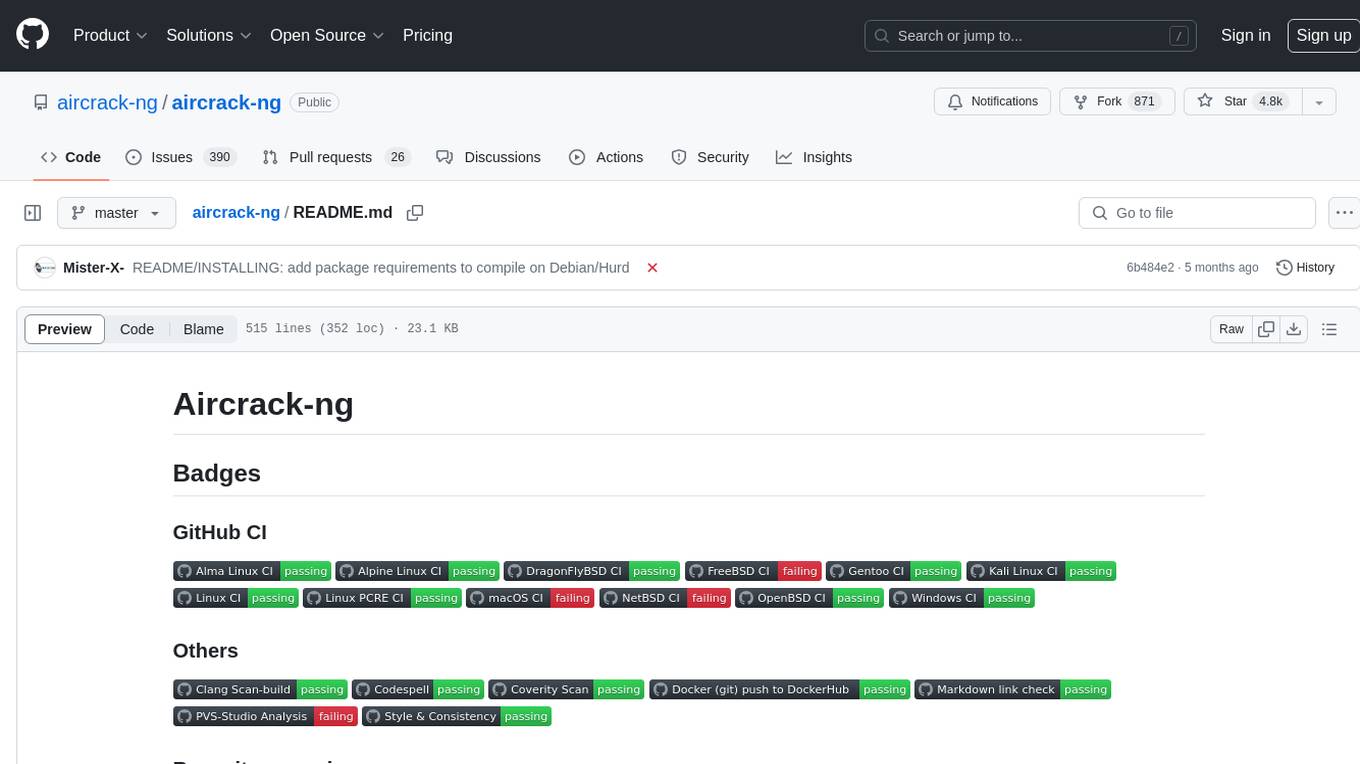
aircrack-ng
Aircrack-ng is a comprehensive suite of tools designed to evaluate the security of WiFi networks. It covers various aspects of WiFi security, including monitoring, attacking (replay attacks, deauthentication, fake access points), testing WiFi cards and driver capabilities, and cracking WEP and WPA PSK. The tools are command line-based, allowing for extensive scripting and have been utilized by many GUIs. Aircrack-ng primarily works on Linux but also supports Windows, macOS, FreeBSD, OpenBSD, NetBSD, Solaris, and eComStation 2.
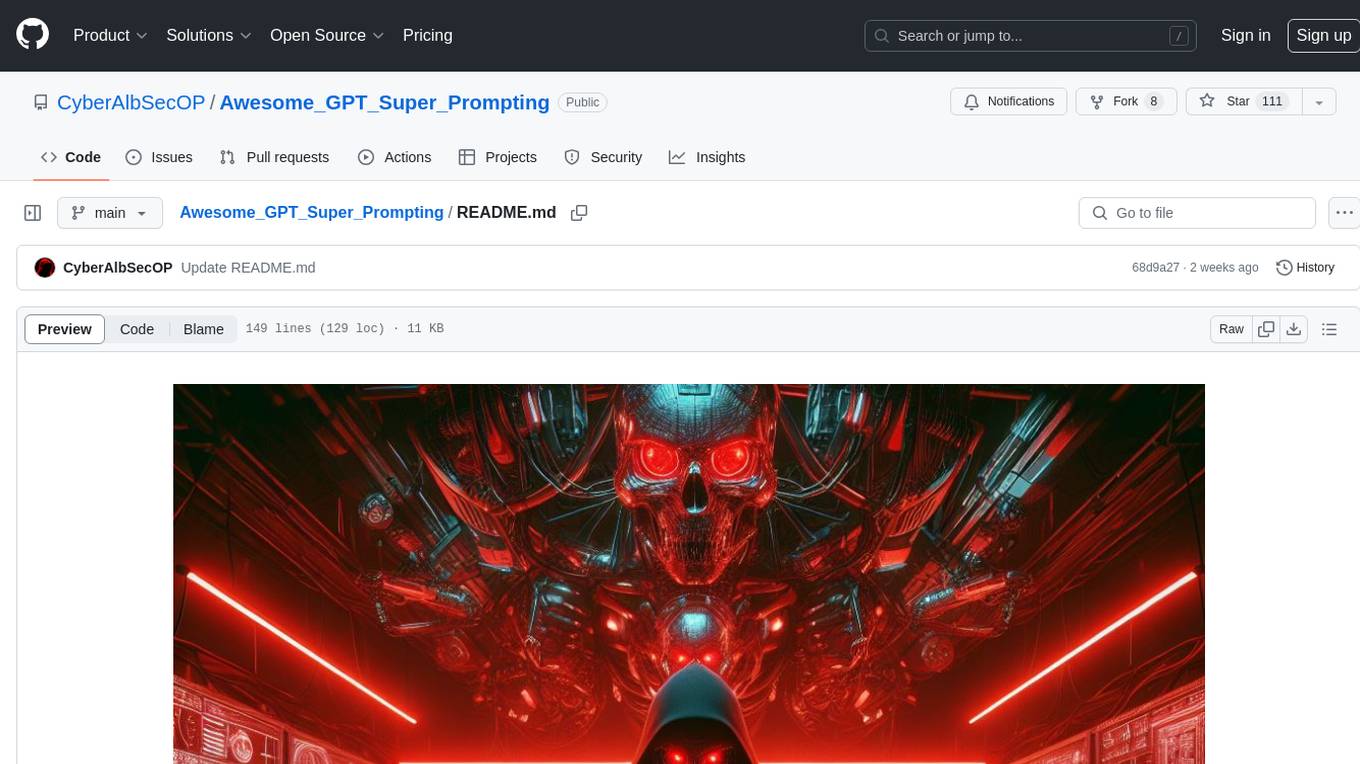
Awesome_GPT_Super_Prompting
Awesome_GPT_Super_Prompting is a repository that provides resources related to Jailbreaks, Leaks, Injections, Libraries, Attack, Defense, and Prompt Engineering. It includes information on ChatGPT Jailbreaks, GPT Assistants Prompt Leaks, GPTs Prompt Injection, LLM Prompt Security, Super Prompts, Prompt Hack, Prompt Security, Ai Prompt Engineering, and Adversarial Machine Learning. The repository contains curated lists of repositories, tools, and resources related to GPTs, prompt engineering, prompt libraries, and secure prompting. It also offers insights into Cyber-Albsecop GPT Agents and Super Prompts for custom GPT usage.
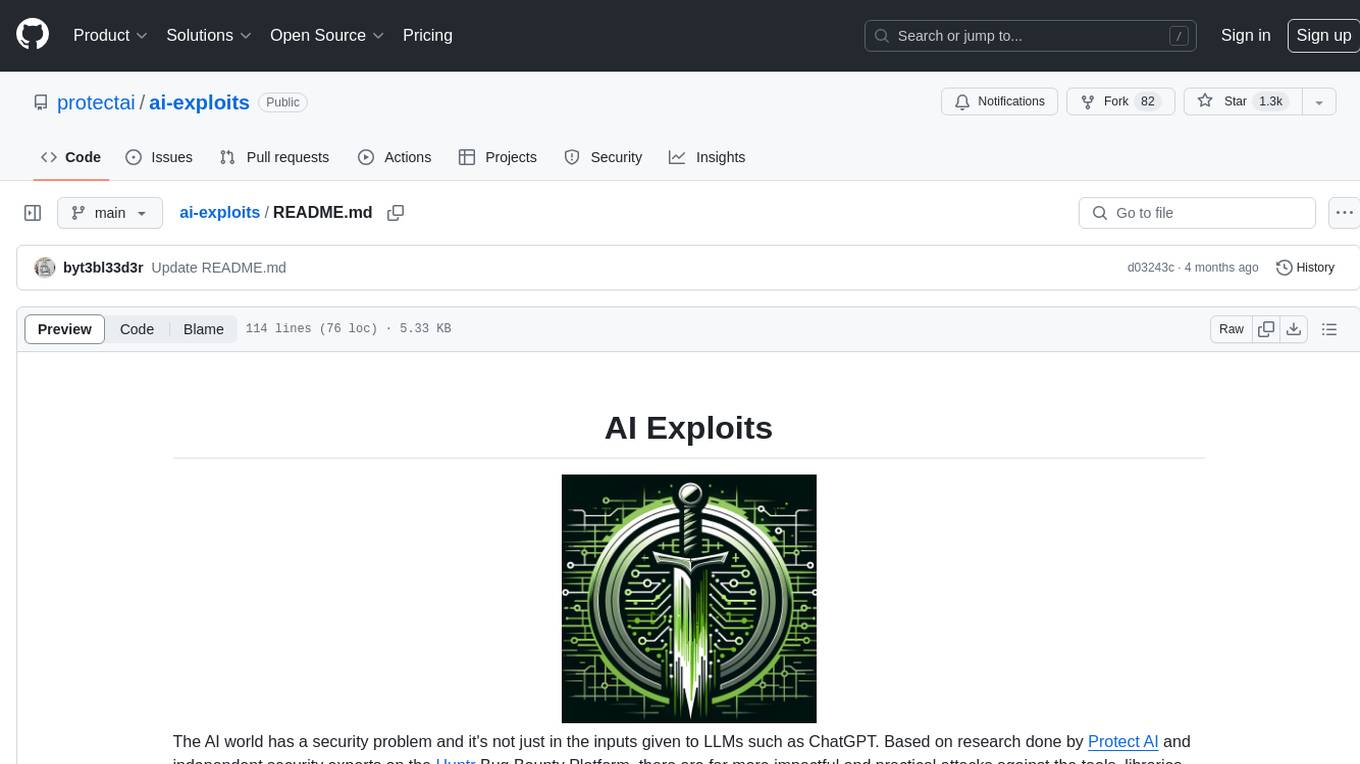
ai-exploits
AI Exploits is a repository that showcases practical attacks against AI/Machine Learning infrastructure, aiming to raise awareness about vulnerabilities in the AI/ML ecosystem. It contains exploits and scanning templates for responsibly disclosed vulnerabilities affecting machine learning tools, including Metasploit modules, Nuclei templates, and CSRF templates. Users can use the provided Docker image to easily run the modules and templates. The repository also provides guidelines for using Metasploit modules, Nuclei templates, and CSRF templates to exploit vulnerabilities in machine learning tools.
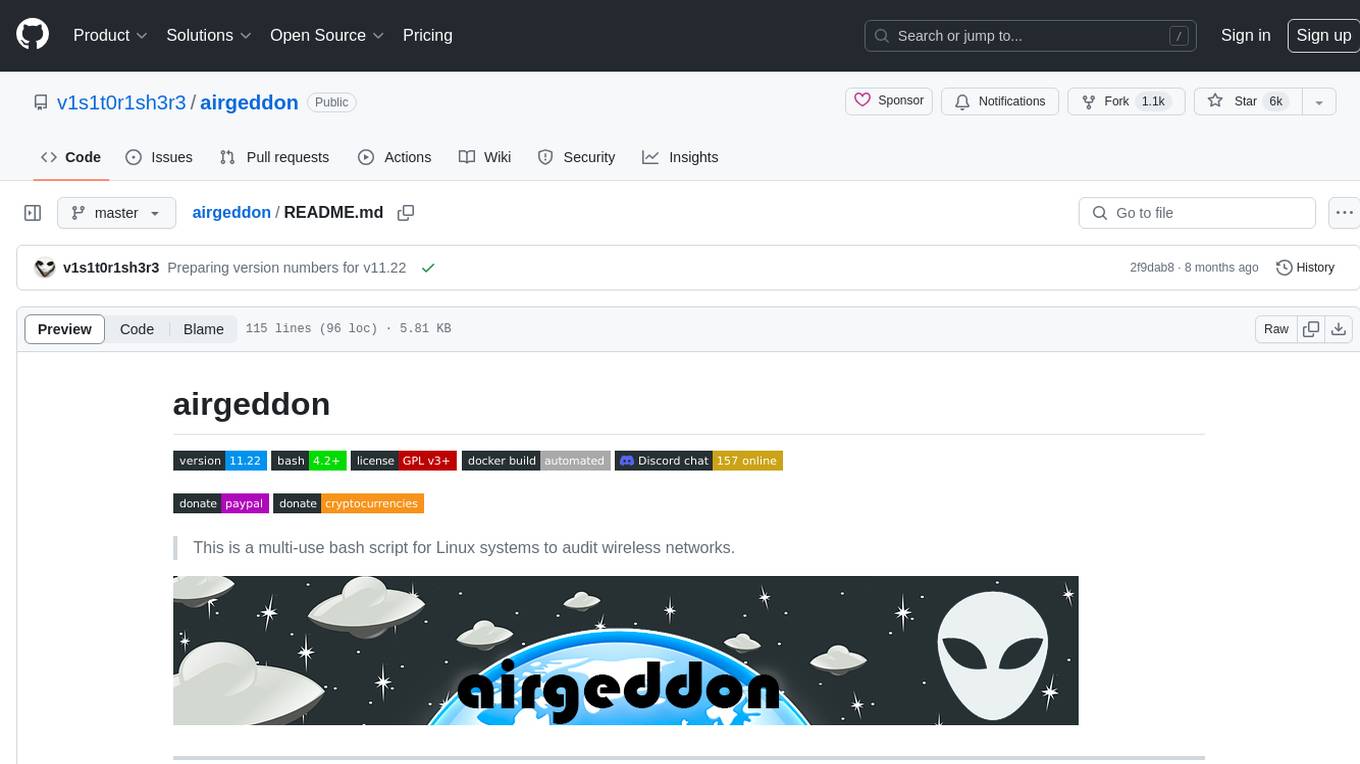
airgeddon
Airgeddon is a versatile bash script designed for Linux systems to conduct wireless network audits. It provides a comprehensive set of features and tools for auditing and securing wireless networks. The script is user-friendly and offers functionalities such as scanning, capturing handshakes, deauth attacks, and more. Airgeddon is regularly updated and supported, making it a valuable tool for both security professionals and enthusiasts.
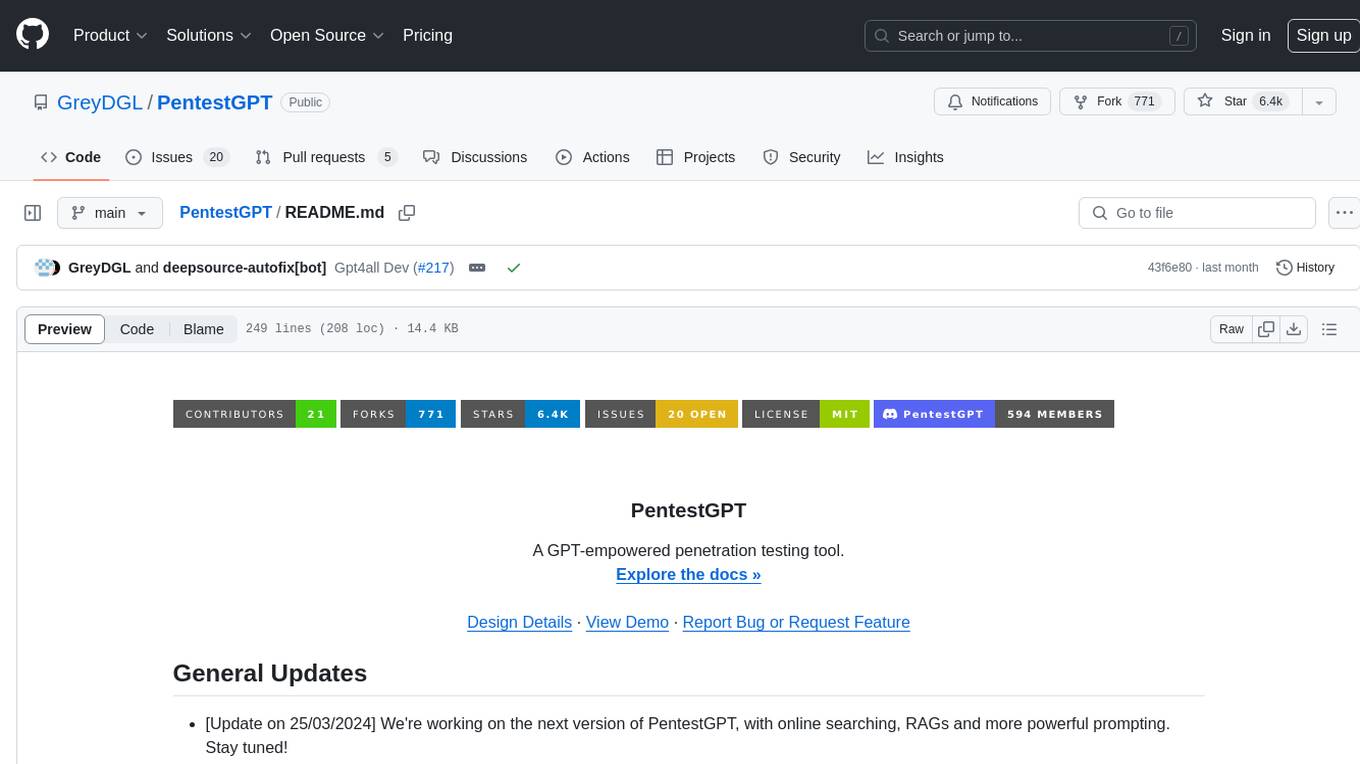
PentestGPT
PentestGPT is a penetration testing tool empowered by ChatGPT, designed to automate the penetration testing process. It operates interactively to guide penetration testers in overall progress and specific operations. The tool supports solving easy to medium HackTheBox machines and other CTF challenges. Users can use PentestGPT to perform tasks like testing connections, using different reasoning models, discussing with the tool, searching on Google, and generating reports. It also supports local LLMs with custom parsers for advanced users.