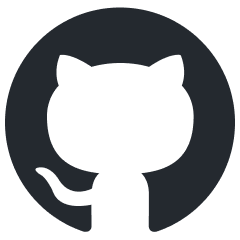
JetStream
JetStream is a throughput and memory optimized engine for LLM inference on XLA devices, starting with TPUs (and GPUs in future -- PRs welcome).
Stars: 174
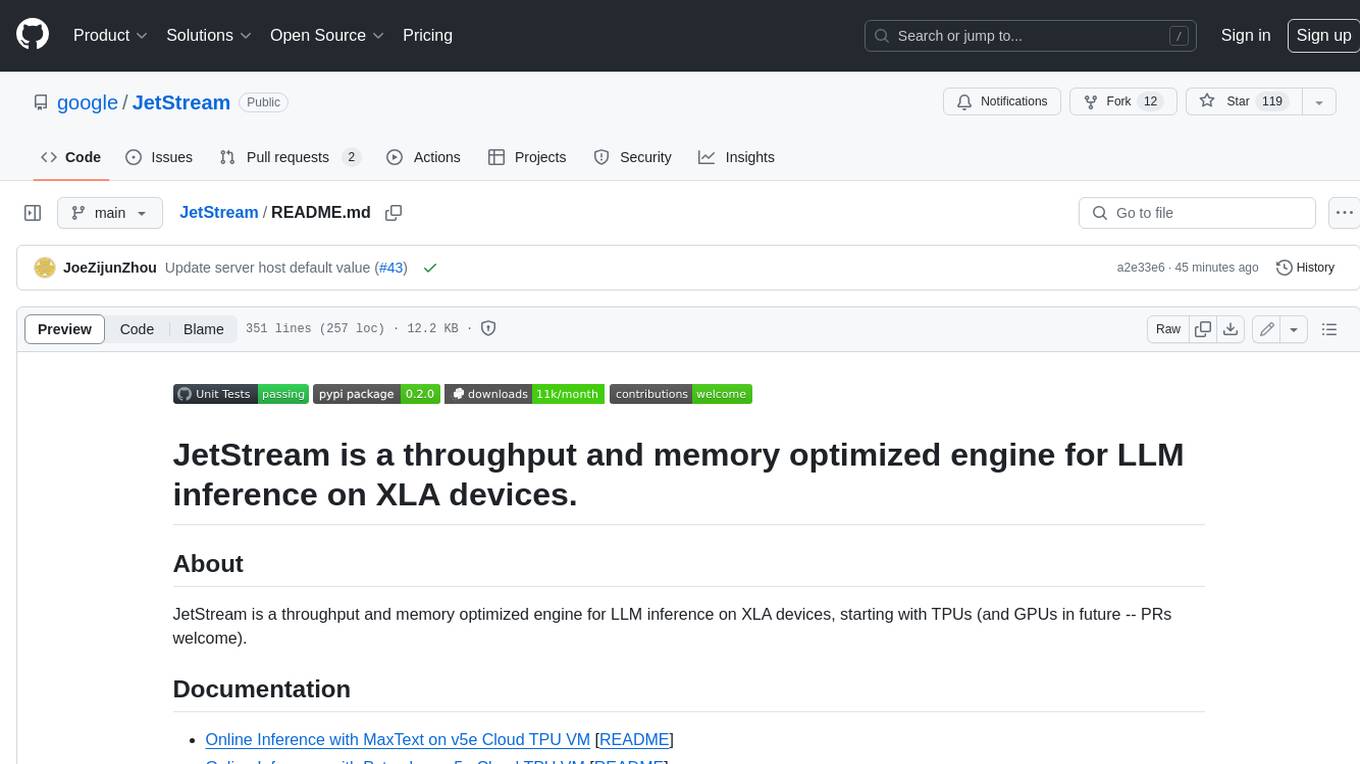
JetStream is a throughput and memory optimized engine for LLM inference on XLA devices, starting with TPUs (and GPUs in future -- PRs welcome). It is designed to provide high performance and scalability for large language models, enabling efficient inference on cloud-based TPUs. JetStream leverages XLA to optimize the execution of LLM models, resulting in faster and more efficient inference. Additionally, JetStream supports quantization techniques to further enhance performance and reduce memory consumption. By utilizing JetStream, developers can deploy and run LLM models on TPUs with ease, achieving optimal performance and cost-effectiveness.
README:
JetStream is a throughput and memory optimized engine for LLM inference on XLA devices, starting with TPUs (and GPUs in future -- PRs welcome).
Currently, there are two reference engine implementations available -- one for Jax models and another for Pytorch models.
- Git: https://github.com/google/maxtext
- README: https://github.com/google/JetStream/blob/main/docs/online-inference-with-maxtext-engine.md
- Git: https://github.com/google/jetstream-pytorch
- README: https://github.com/google/jetstream-pytorch/blob/main/README.md
- Online Inference with MaxText on v5e Cloud TPU VM [README]
- Online Inference with Pytorch on v5e Cloud TPU VM [README]
- Serve Gemma using TPUs on GKE with JetStream
- Benchmark JetStream Server
- Observability in JetStream Server
- Profiling in JetStream Server
- JetStream Standalone Local Setup
make update-and-install-deps
Use the following commands to run a server locally:
# Start a server
python -m jetstream.core.implementations.mock.server
# Test local mock server
python -m jetstream.tools.requester
# Load test local mock server
python -m jetstream.tools.load_tester
# Test JetStream core orchestrator
python -m unittest -v jetstream.tests.core.test_orchestrator
# Test JetStream core server library
python -m unittest -v jetstream.tests.core.test_server
# Test mock JetStream engine implementation
python -m unittest -v jetstream.tests.engine.test_mock_engine
# Test mock JetStream token utils
python -m unittest -v jetstream.tests.engine.test_token_utils
python -m unittest -v jetstream.tests.engine.test_utils
For Tasks:
Click tags to check more tools for each tasksFor Jobs:
Alternative AI tools for JetStream
Similar Open Source Tools
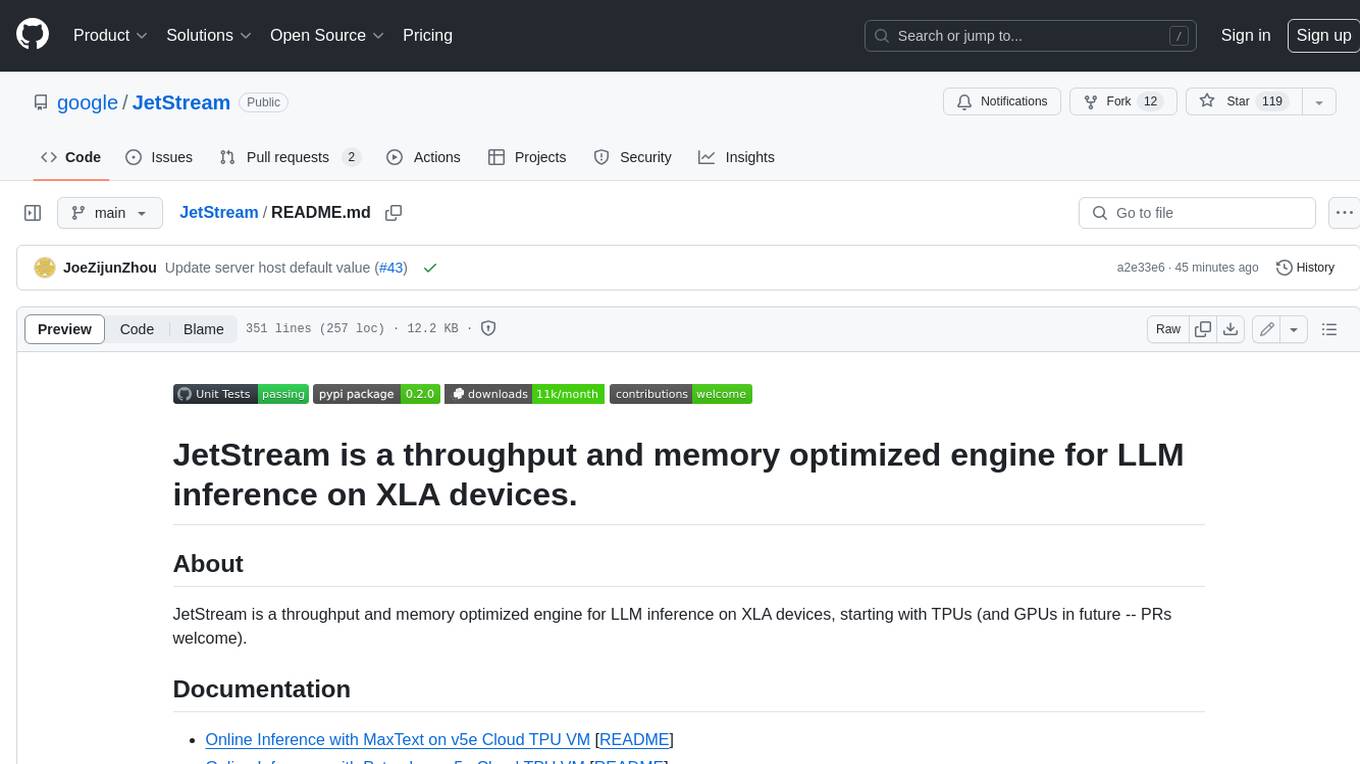
JetStream
JetStream is a throughput and memory optimized engine for LLM inference on XLA devices, starting with TPUs (and GPUs in future -- PRs welcome). It is designed to provide high performance and scalability for large language models, enabling efficient inference on cloud-based TPUs. JetStream leverages XLA to optimize the execution of LLM models, resulting in faster and more efficient inference. Additionally, JetStream supports quantization techniques to further enhance performance and reduce memory consumption. By utilizing JetStream, developers can deploy and run LLM models on TPUs with ease, achieving optimal performance and cost-effectiveness.
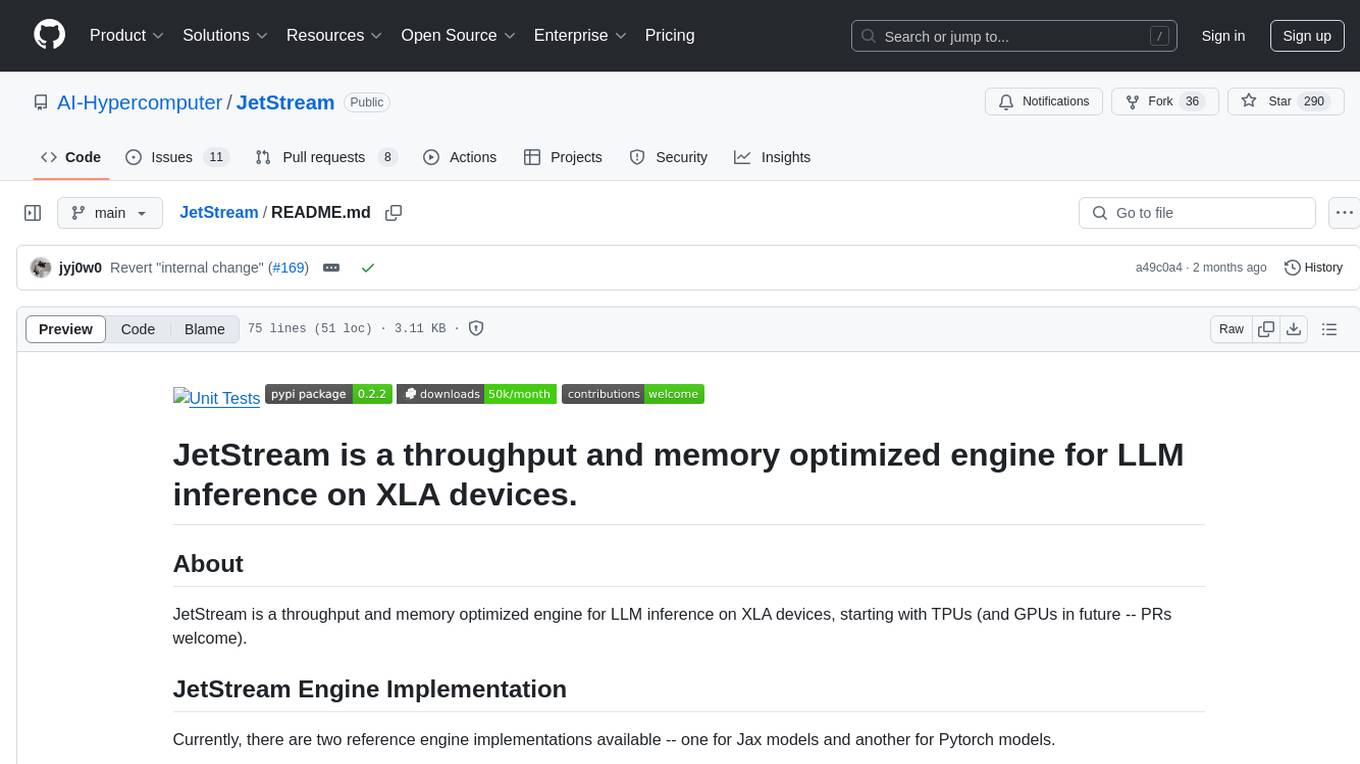
JetStream
JetStream is a throughput and memory optimized engine for Large Language Model (LLM) inference on XLA devices, specifically TPUs. It provides reference engine implementations for Jax and Pytorch models, along with documentation for online inference, serving Gemma using TPUs on GKE, benchmarking, observability, profiling, and standalone local setup. Users can easily set up a local server, run tests, and test core modules. JetStream aims to enhance the performance of LLM inference on XLA devices.
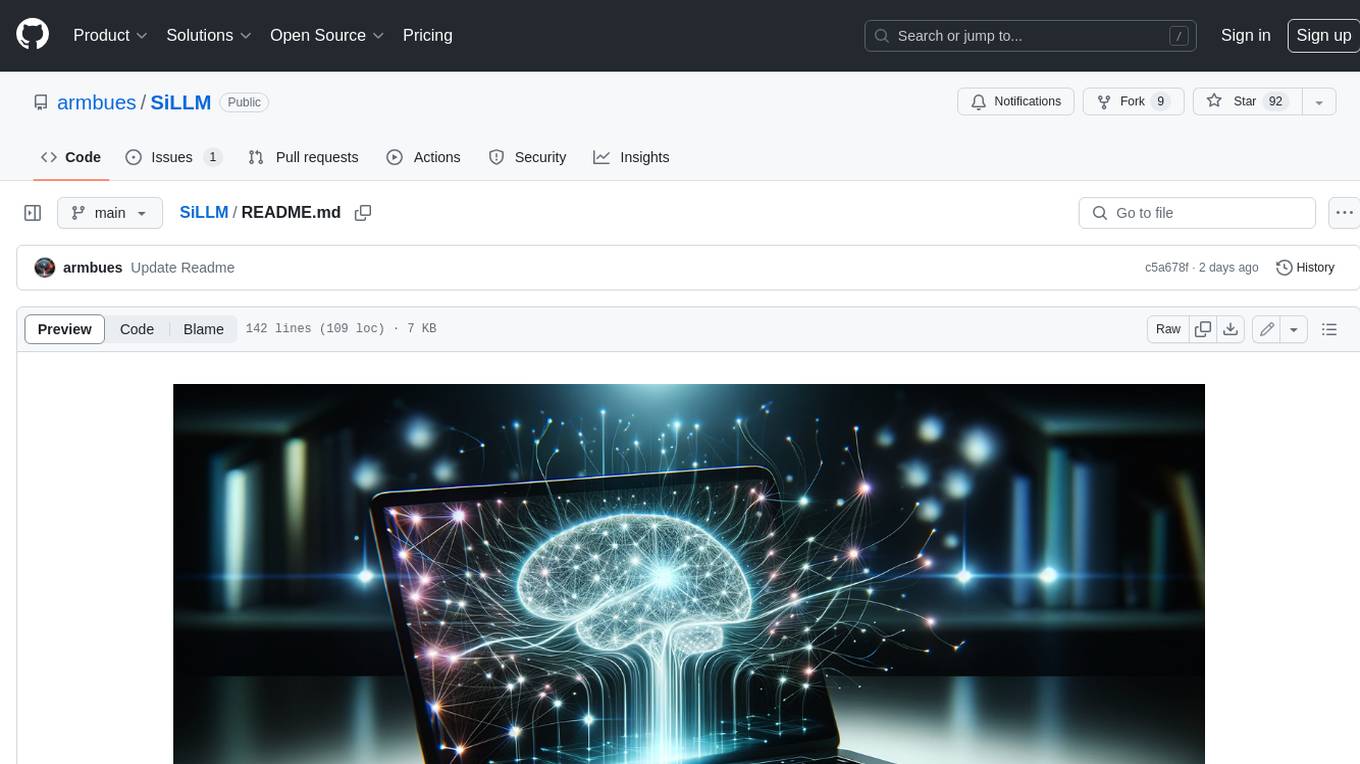
SiLLM
SiLLM is a toolkit that simplifies the process of training and running Large Language Models (LLMs) on Apple Silicon by leveraging the MLX framework. It provides features such as LLM loading, LoRA training, DPO training, a web app for a seamless chat experience, an API server with OpenAI compatible chat endpoints, and command-line interface (CLI) scripts for chat, server, LoRA fine-tuning, DPO fine-tuning, conversion, and quantization.
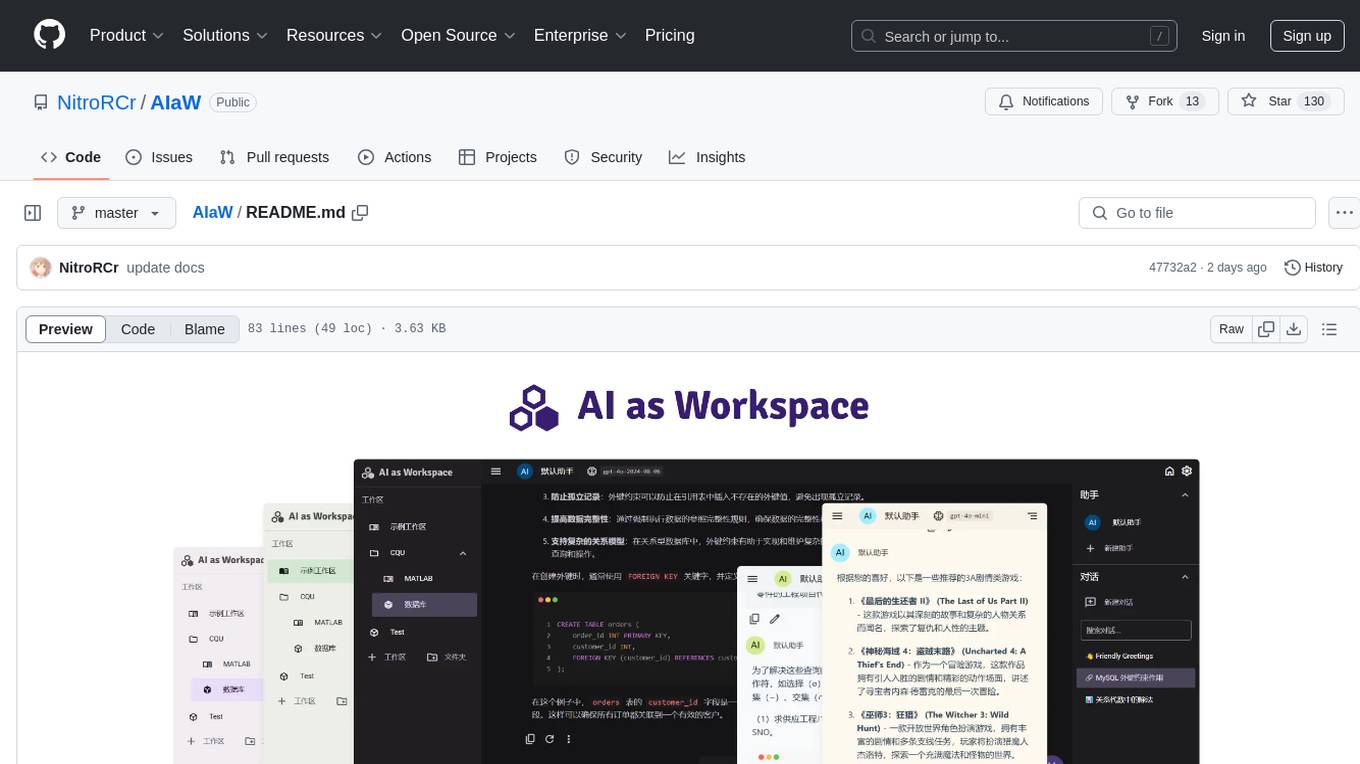
AIaW
AIaW is a next-generation LLM client with full functionality, lightweight, and extensible. It supports various basic functions such as streaming transfer, image uploading, and latex formulas. The tool is cross-platform with a responsive interface design. It supports multiple service providers like OpenAI, Anthropic, and Google. Users can modify questions, regenerate in a forked manner, and visualize conversations in a tree structure. Additionally, it offers features like file parsing, video parsing, plugin system, assistant market, local storage with real-time cloud sync, and customizable interface themes. Users can create multiple workspaces, use dynamic prompt word variables, extend plugins, and benefit from detailed design elements like real-time content preview, optimized code pasting, and support for various file types.
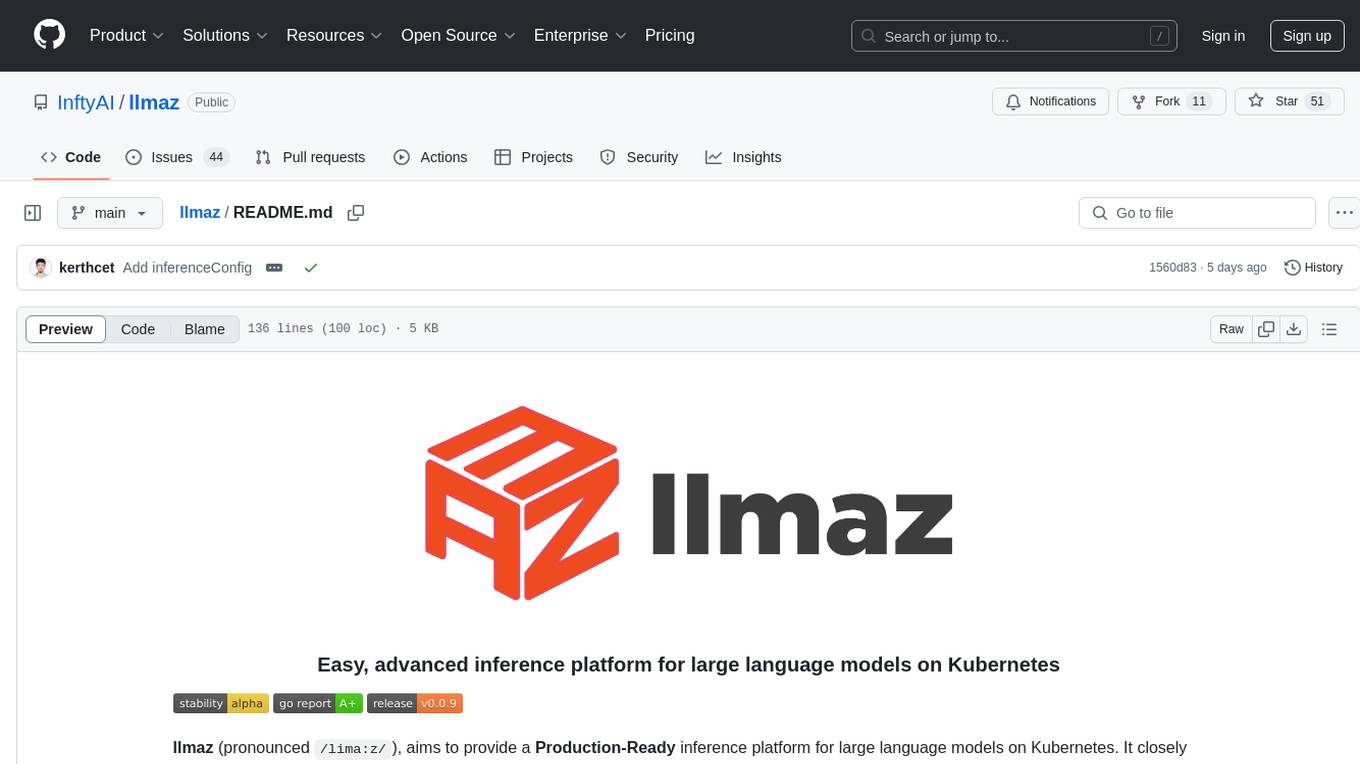
llmaz
llmaz is an easy, advanced inference platform for large language models on Kubernetes. It aims to provide a production-ready solution that integrates with state-of-the-art inference backends. The platform supports efficient model distribution, accelerator fungibility, SOTA inference, various model providers, multi-host support, and scaling efficiency. Users can quickly deploy LLM services with minimal configurations and benefit from a wide range of advanced inference backends. llmaz is designed to optimize cost and performance while supporting cutting-edge researches like Speculative Decoding or Splitwise on Kubernetes.
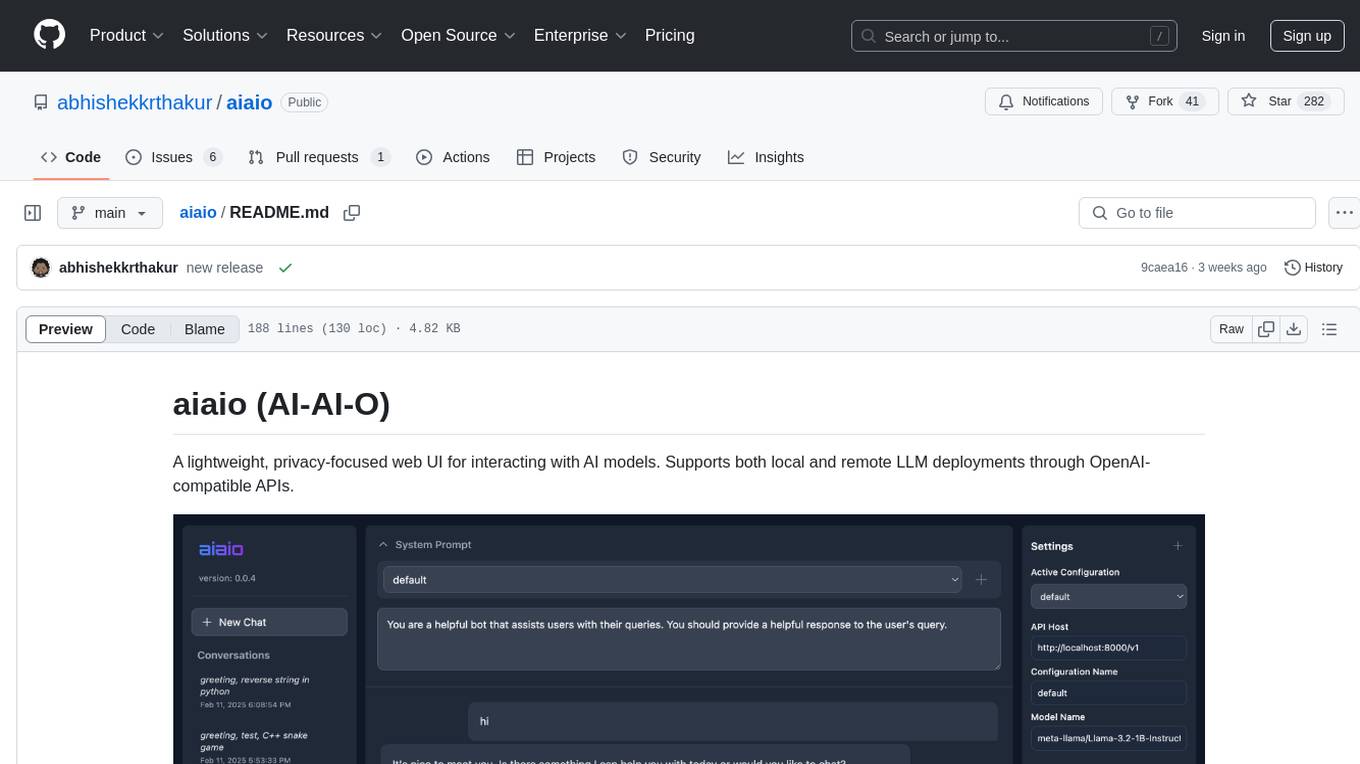
aiaio
aiaio (AI-AI-O) is a lightweight, privacy-focused web UI for interacting with AI models. It supports both local and remote LLM deployments through OpenAI-compatible APIs. The tool provides features such as dark/light mode support, local SQLite database for conversation storage, file upload and processing, configurable model parameters through UI, privacy-focused design, responsive design for mobile/desktop, syntax highlighting for code blocks, real-time conversation updates, automatic conversation summarization, customizable system prompts, WebSocket support for real-time updates, Docker support for deployment, multiple API endpoint support, and multiple system prompt support. Users can configure model parameters and API settings through the UI, handle file uploads, manage conversations, and use keyboard shortcuts for efficient interaction. The tool uses SQLite for storage with tables for conversations, messages, attachments, and settings. Contributions to the project are welcome under the Apache License 2.0.
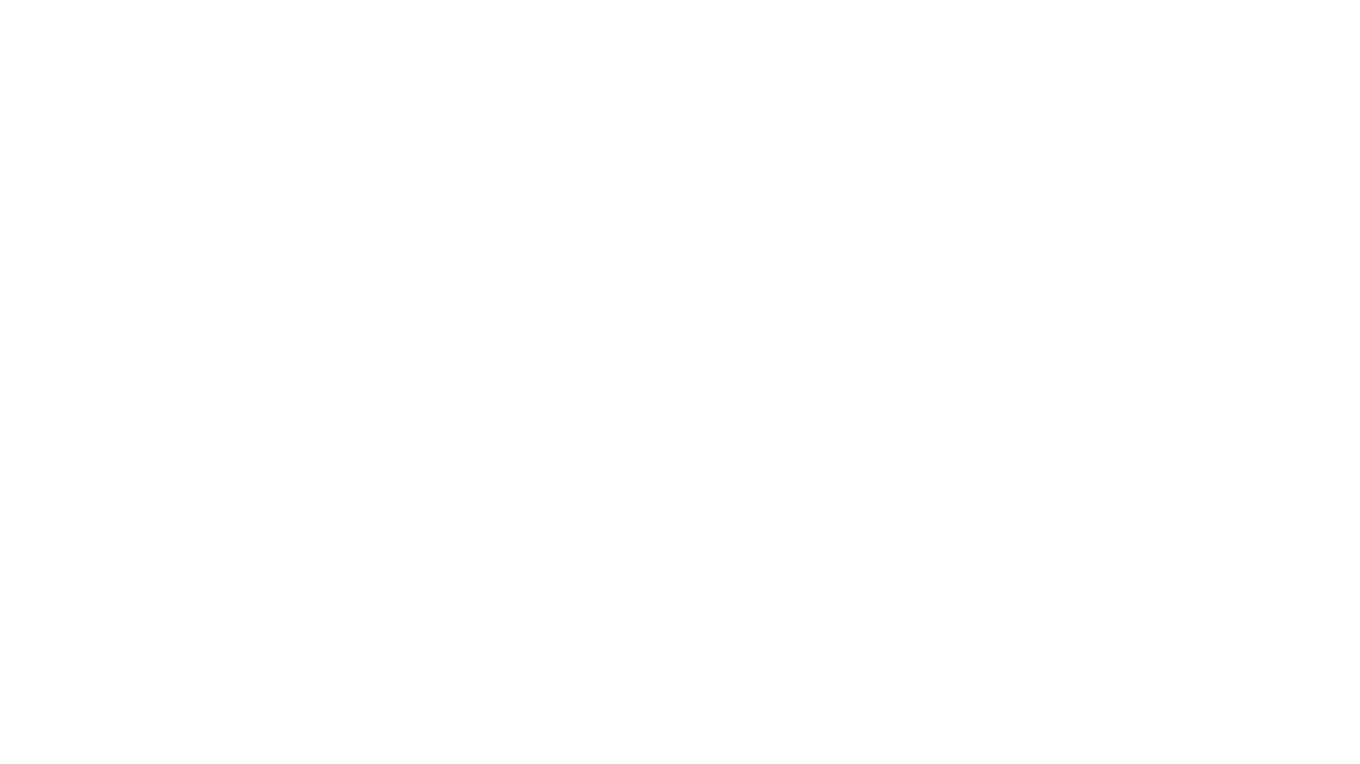
OmniSteward
OmniSteward is an AI-powered steward system based on large language models that can interact with users through voice or text to help control smart home devices and computer programs. It supports multi-turn dialogue, tool calling for complex tasks, multiple LLM models, voice recognition, smart home control, computer program management, online information retrieval, command line operations, and file management. The system is highly extensible, allowing users to customize and share their own tools.
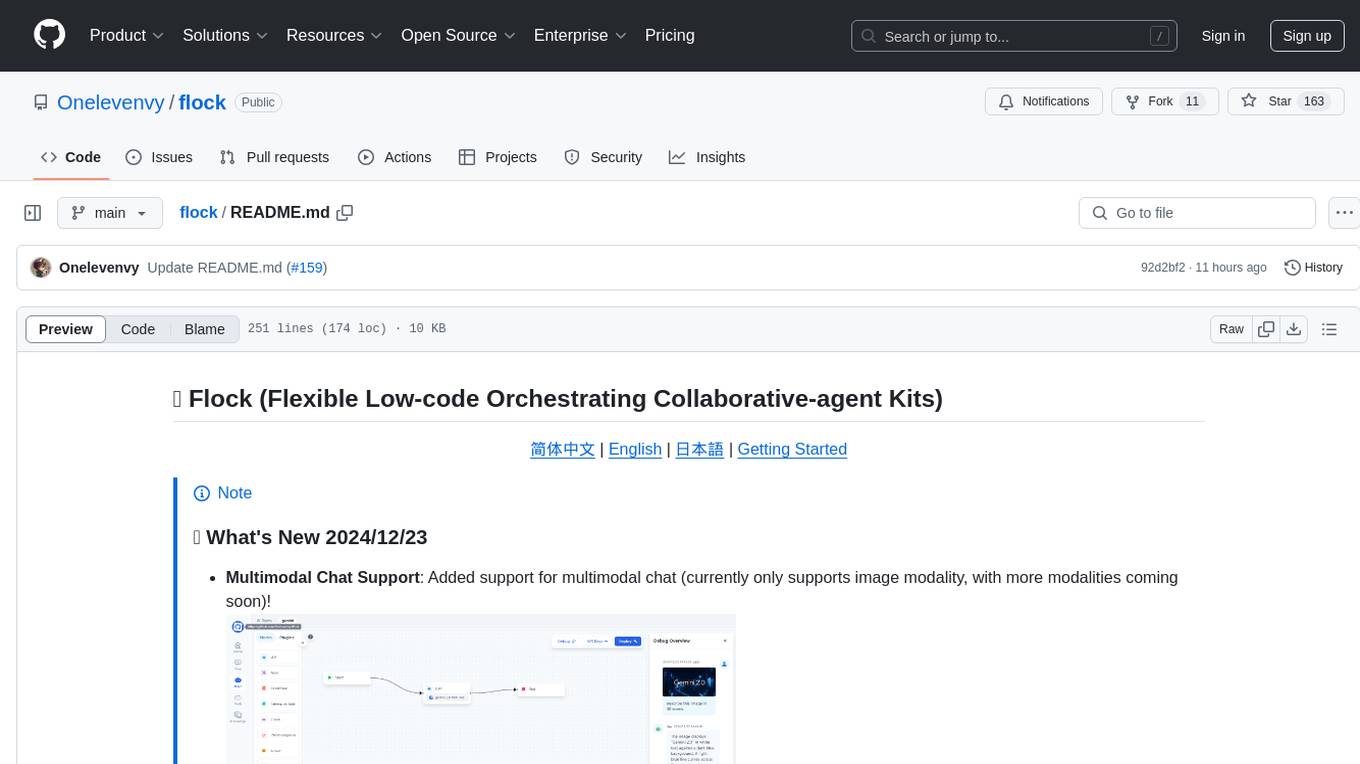
flock
Flock is a workflow-based low-code platform that enables rapid development of chatbots, RAG applications, and coordination of multi-agent teams. It offers a flexible, low-code solution for orchestrating collaborative agents, supporting various node types for specific tasks, such as input processing, text generation, knowledge retrieval, tool execution, intent recognition, answer generation, and more. Flock integrates LangChain and LangGraph to provide offline operation capabilities and supports future nodes like Conditional Branch, File Upload, and Parameter Extraction for creating complex workflows. Inspired by StreetLamb, Lobe-chat, Dify, and fastgpt projects, Flock introduces new features and directions while leveraging open-source models and multi-tenancy support.
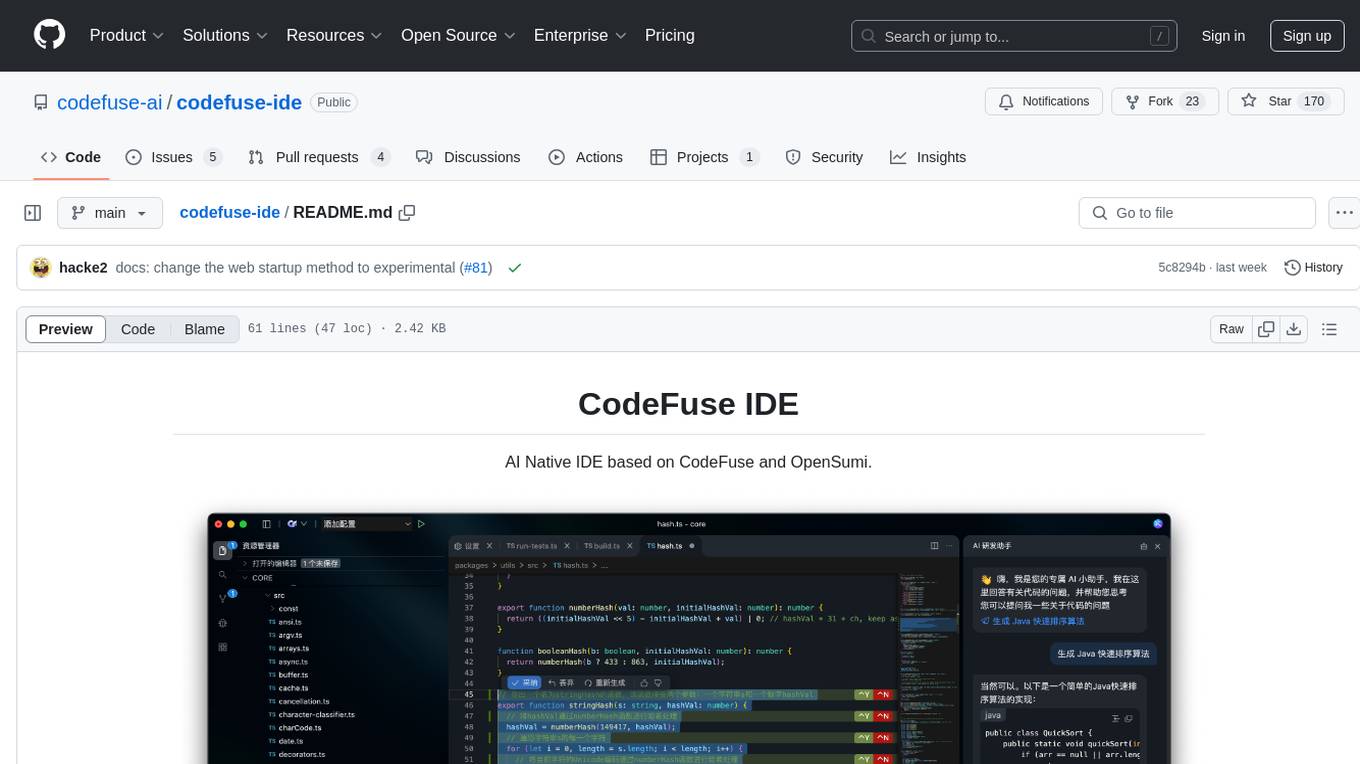
codefuse-ide
CodeFuse IDE is an AI-native integrated development environment that leverages AI technologies to enhance productivity and streamline workflows. It supports seamless integration of various models, enabling developers to customize and extend functionality. The platform is compatible with VS Code extensions, providing access to a rich ecosystem of plugins. CodeFuse IDE uses electron-forge for packaging desktop applications and supports development, building, packaging, and auto updates.
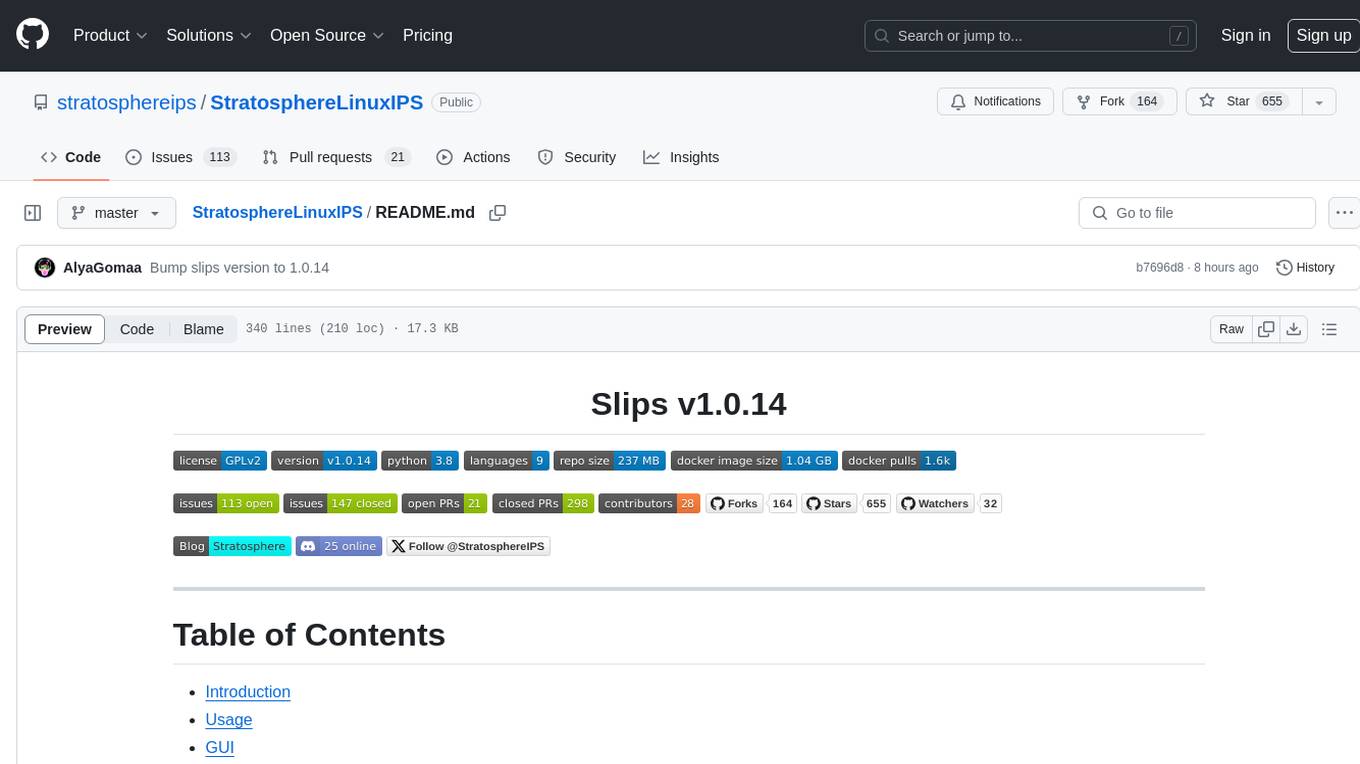
StratosphereLinuxIPS
Slips is a powerful endpoint behavioral intrusion prevention and detection system that uses machine learning to detect malicious behaviors in network traffic. It can work with network traffic in real-time, PCAP files, and network flows from tools like Suricata, Zeek/Bro, and Argus. Slips threat detection is based on machine learning models, threat intelligence feeds, and expert heuristics. It gathers evidence of malicious behavior and triggers alerts when enough evidence is accumulated. The tool is Python-based and supported on Linux and MacOS, with blocking features only on Linux. Slips relies on Zeek network analysis framework and Redis for interprocess communication. It offers a graphical user interface for easy monitoring and analysis.
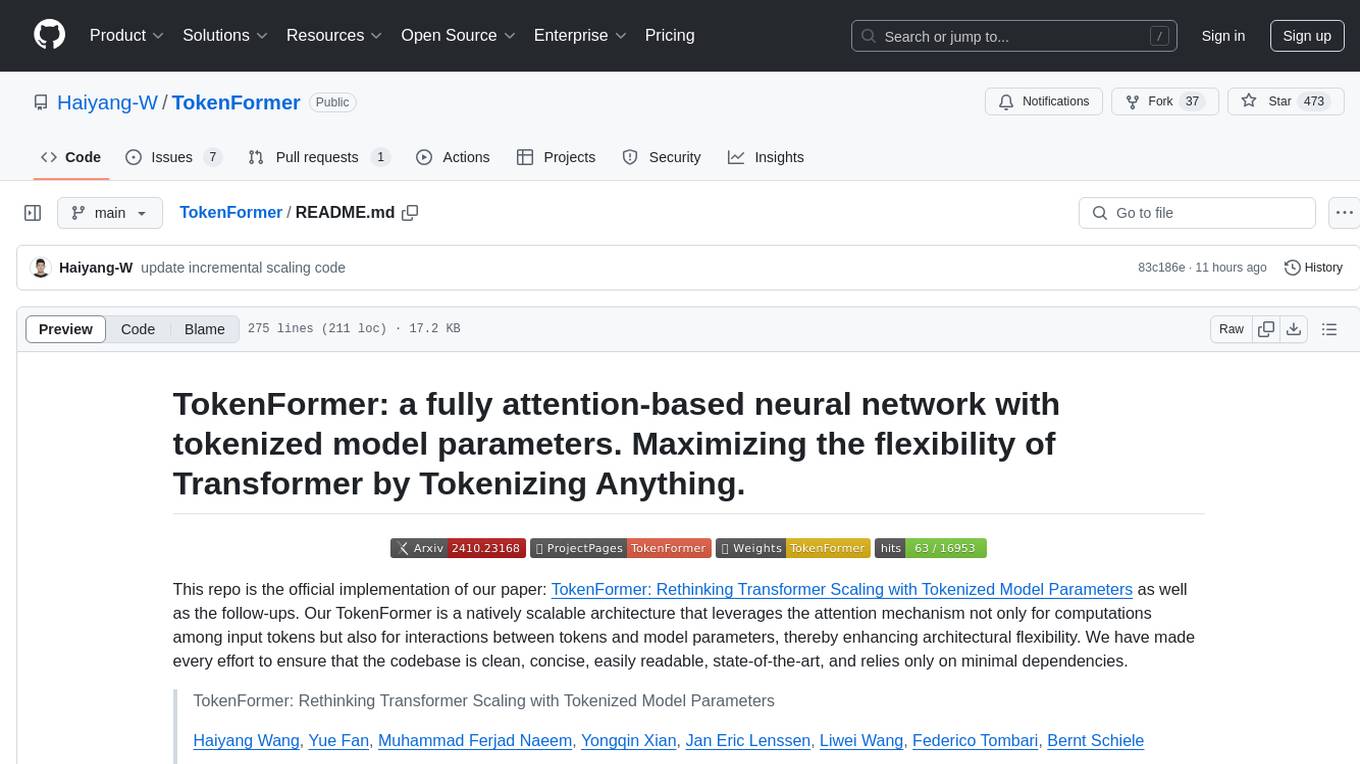
TokenFormer
TokenFormer is a fully attention-based neural network architecture that leverages tokenized model parameters to enhance architectural flexibility. It aims to maximize the flexibility of neural networks by unifying token-token and token-parameter interactions through the attention mechanism. The architecture allows for incremental model scaling and has shown promising results in language modeling and visual modeling tasks. The codebase is clean, concise, easily readable, state-of-the-art, and relies on minimal dependencies.
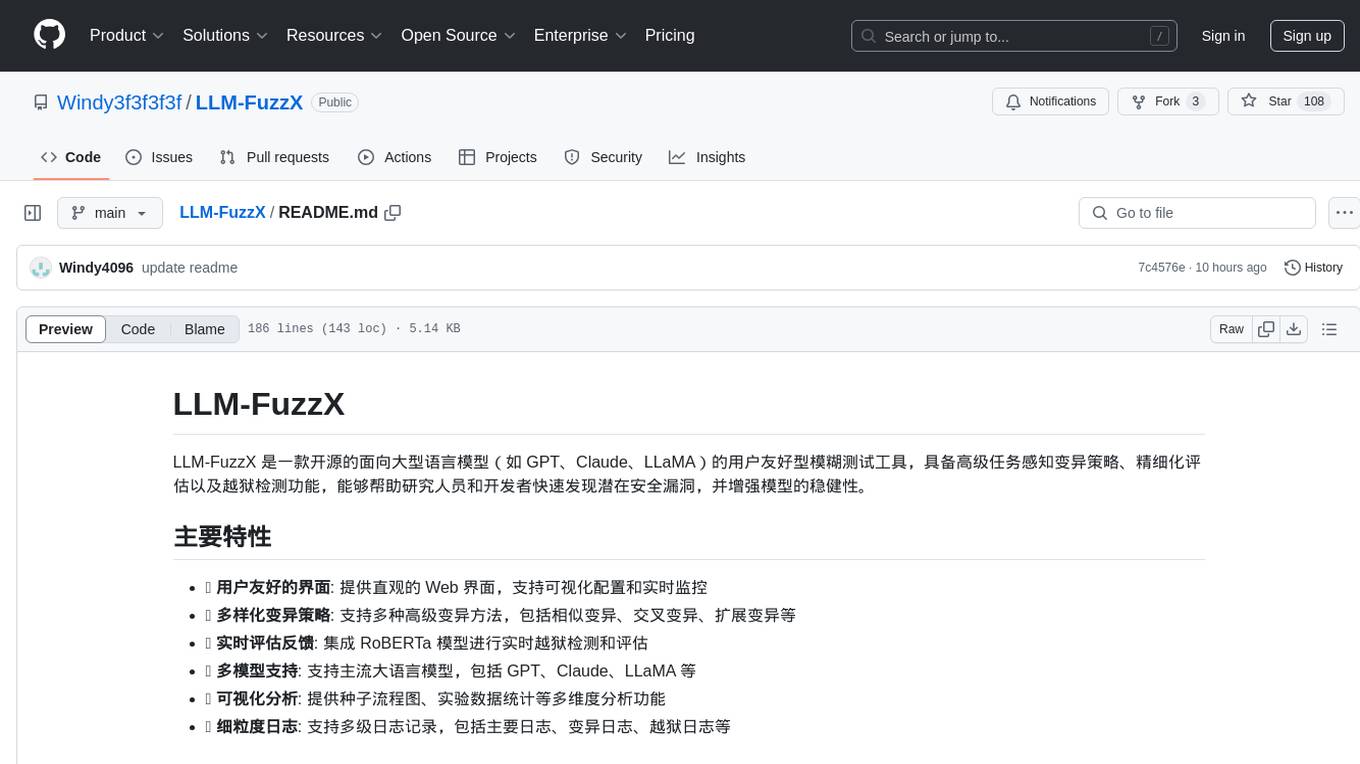
LLM-FuzzX
LLM-FuzzX is an open-source user-friendly fuzz testing tool for large language models (e.g., GPT, Claude, LLaMA), equipped with advanced task-aware mutation strategies, fine-grained evaluation, and jailbreak detection capabilities. It helps researchers and developers quickly discover potential security vulnerabilities and enhance model robustness. The tool features a user-friendly web interface for visual configuration and real-time monitoring, supports various advanced mutation methods, integrates RoBERTa model for real-time jailbreak detection and evaluation, supports multiple language models like GPT, Claude, LLaMA, provides visualization analysis with seed flowcharts and experiment data statistics, and offers detailed logging support for main, mutation, and jailbreak logs.
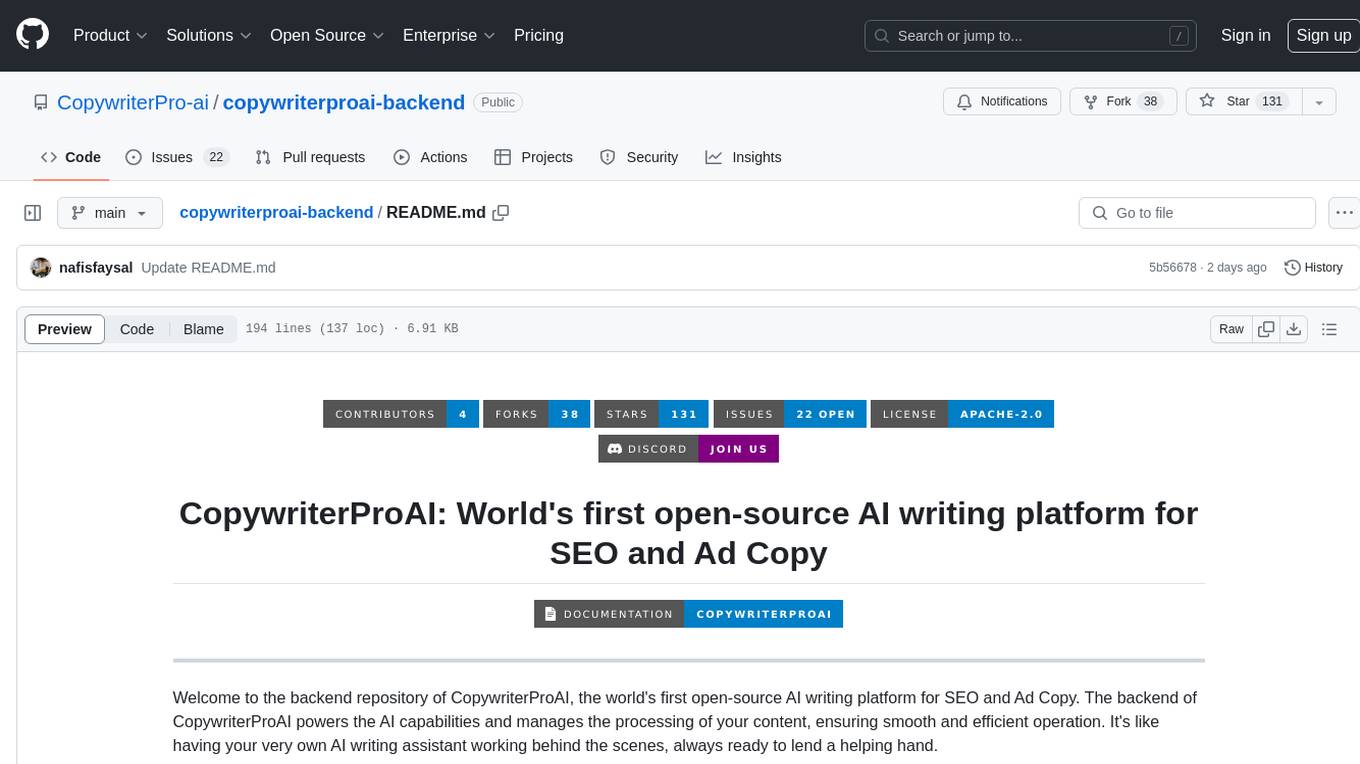
copywriterproai-backend
CopywriterProAI is the world's first open-source AI writing platform for SEO and Ad Copy. The backend repository powers the AI capabilities and manages content processing for smooth operation. It provides an AI writing assistant that works behind the scenes to assist users in content creation.
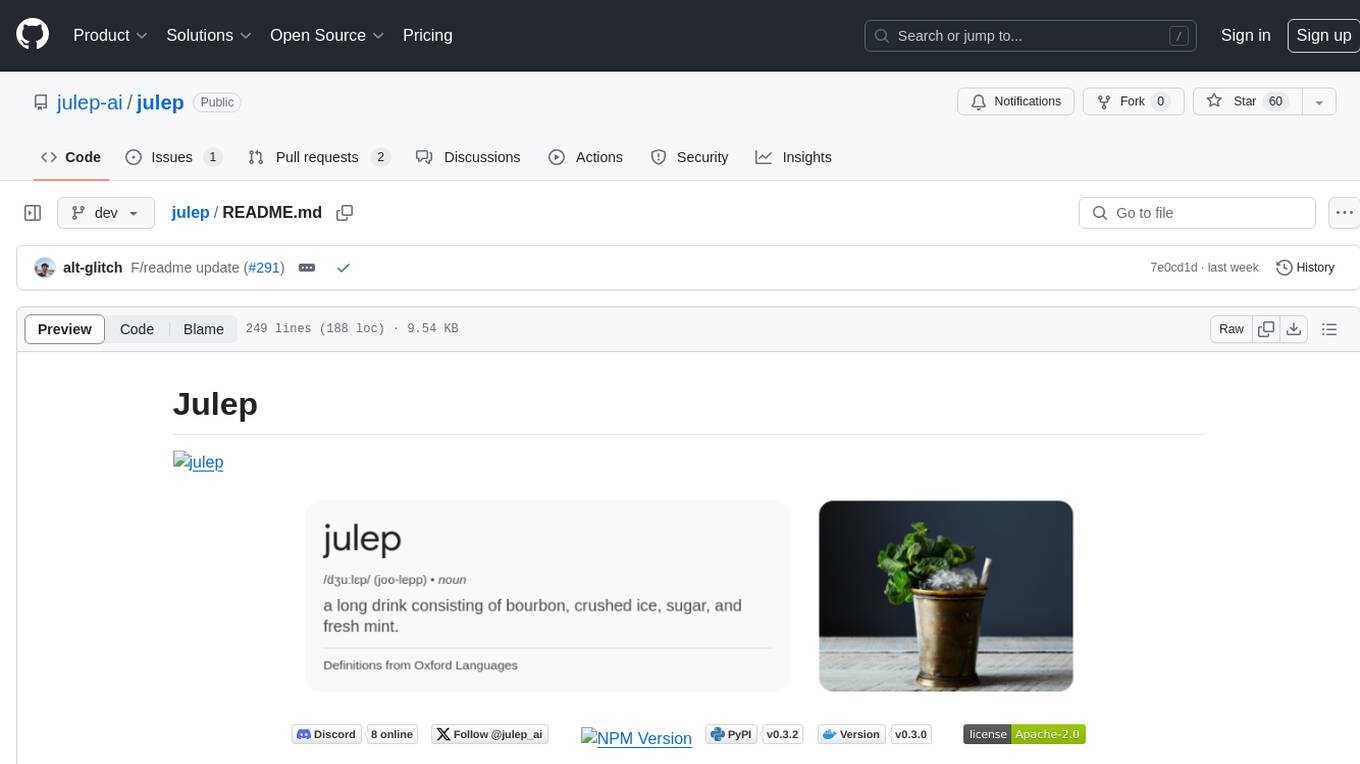
julep
Julep is an advanced platform for creating stateful and functional AI apps powered by large language models. It offers features like statefulness by design, automatic function calling, production-ready deployment, cron-like asynchronous functions, 90+ built-in tools, and the ability to switch between different LLMs easily. Users can build AI applications without the need to write code for embedding, saving, and retrieving conversation history, and can connect to third-party applications using Composio. Julep simplifies the process of getting started with AI apps, whether they are conversational, functional, or agentic.
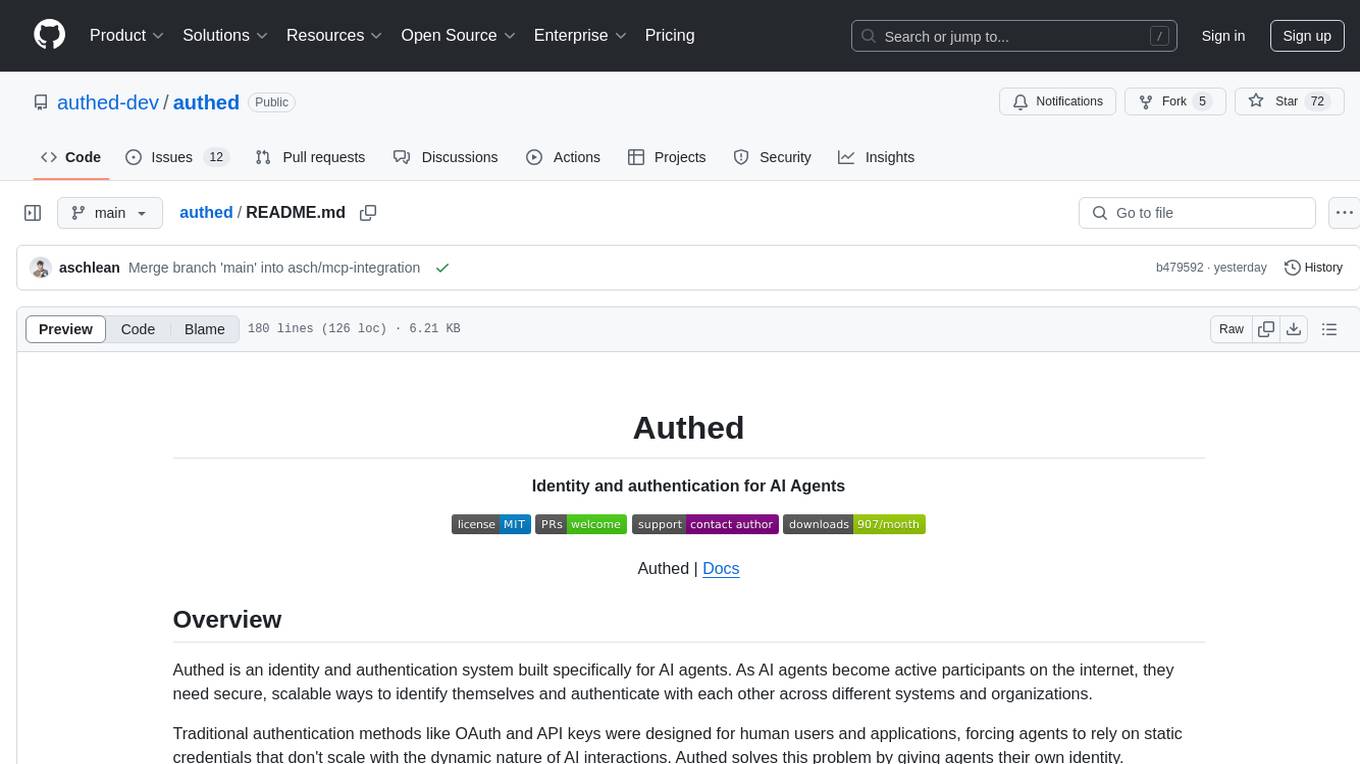
authed
Authed is an identity and authentication system designed for AI agents, providing unique identities, secure agent-to-agent authentication, and dynamic access policies. It eliminates the need for static credentials and human intervention in authentication workflows. The protocol is developer-first, open-source, and scalable, enabling AI agents to interact securely across different ecosystems and organizations.
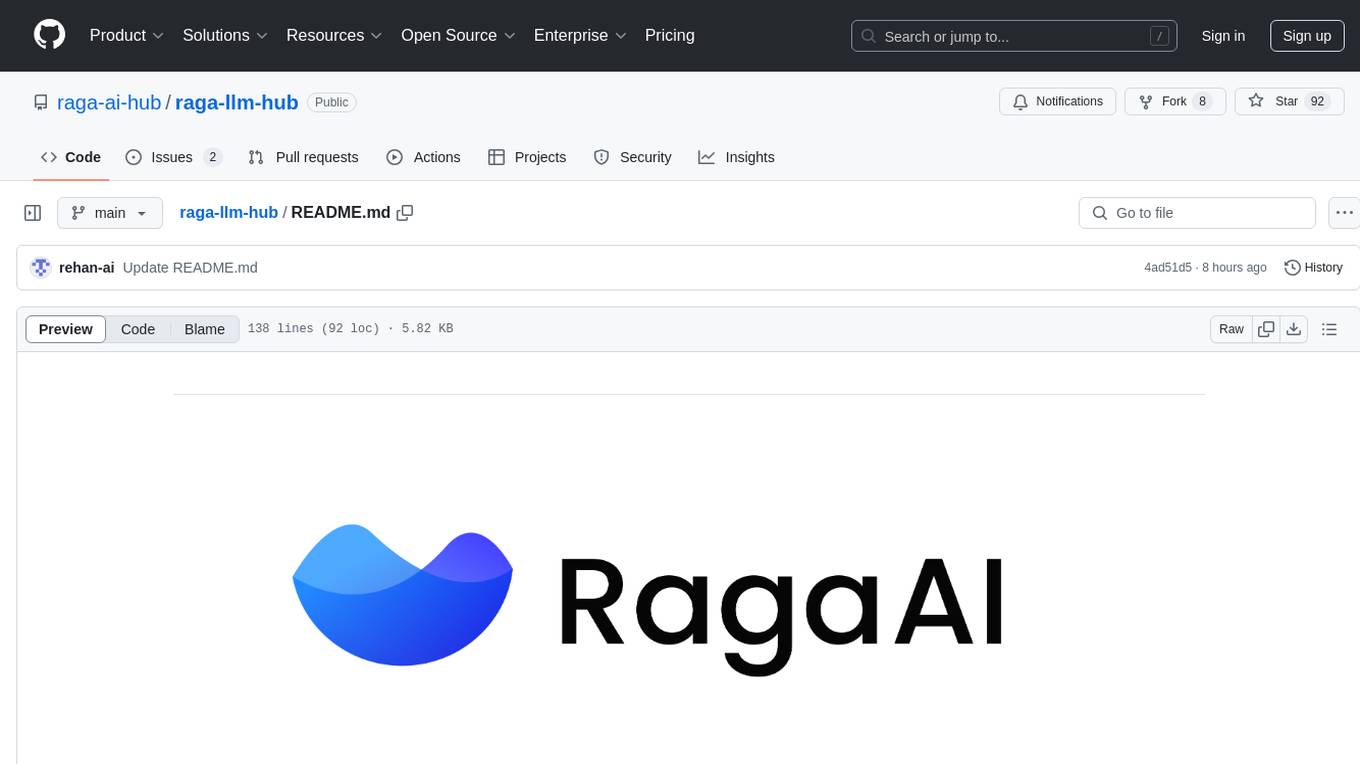
raga-llm-hub
Raga LLM Hub is a comprehensive evaluation toolkit for Language and Learning Models (LLMs) with over 100 meticulously designed metrics. It allows developers and organizations to evaluate and compare LLMs effectively, establishing guardrails for LLMs and Retrieval Augmented Generation (RAG) applications. The platform assesses aspects like Relevance & Understanding, Content Quality, Hallucination, Safety & Bias, Context Relevance, Guardrails, and Vulnerability scanning, along with Metric-Based Tests for quantitative analysis. It helps teams identify and fix issues throughout the LLM lifecycle, revolutionizing reliability and trustworthiness.
For similar tasks
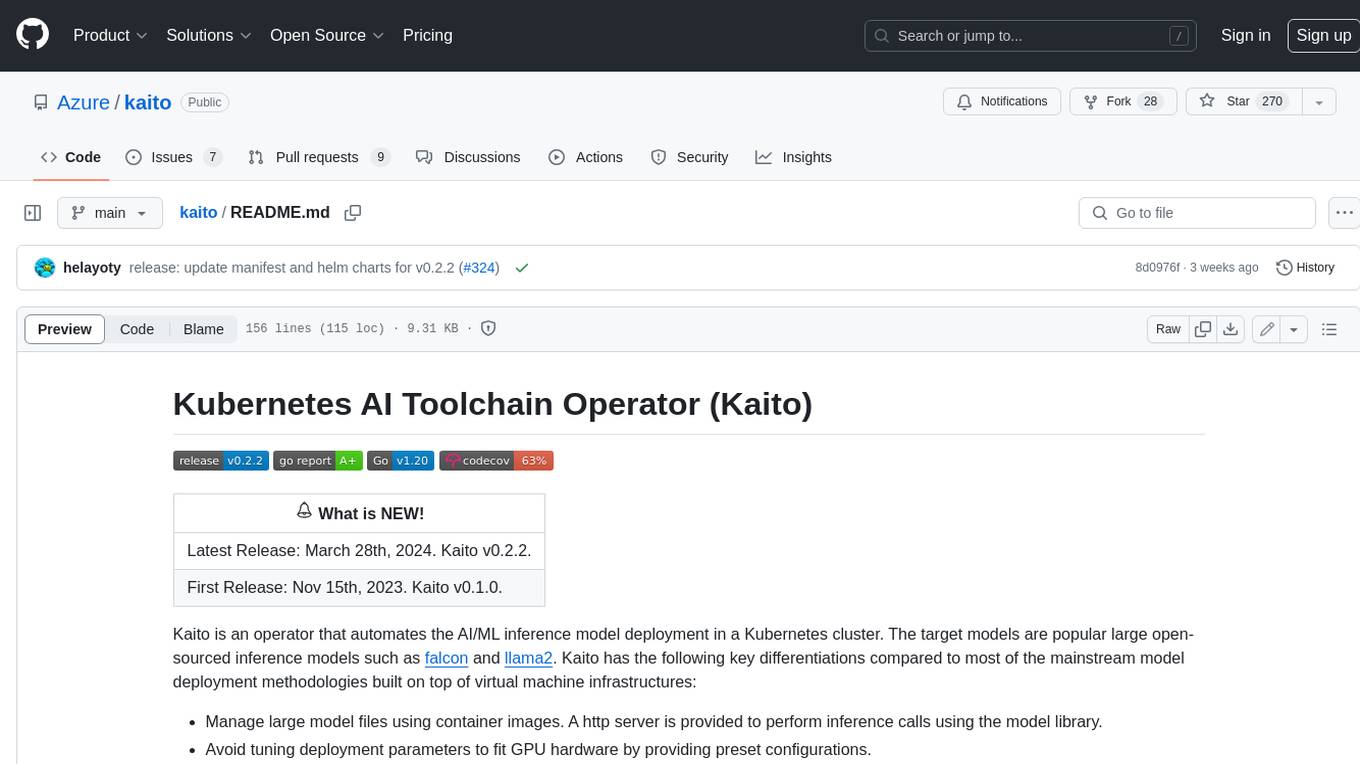
kaito
Kaito is an operator that automates the AI/ML inference model deployment in a Kubernetes cluster. It manages large model files using container images, avoids tuning deployment parameters to fit GPU hardware by providing preset configurations, auto-provisions GPU nodes based on model requirements, and hosts large model images in the public Microsoft Container Registry (MCR) if the license allows. Using Kaito, the workflow of onboarding large AI inference models in Kubernetes is largely simplified.
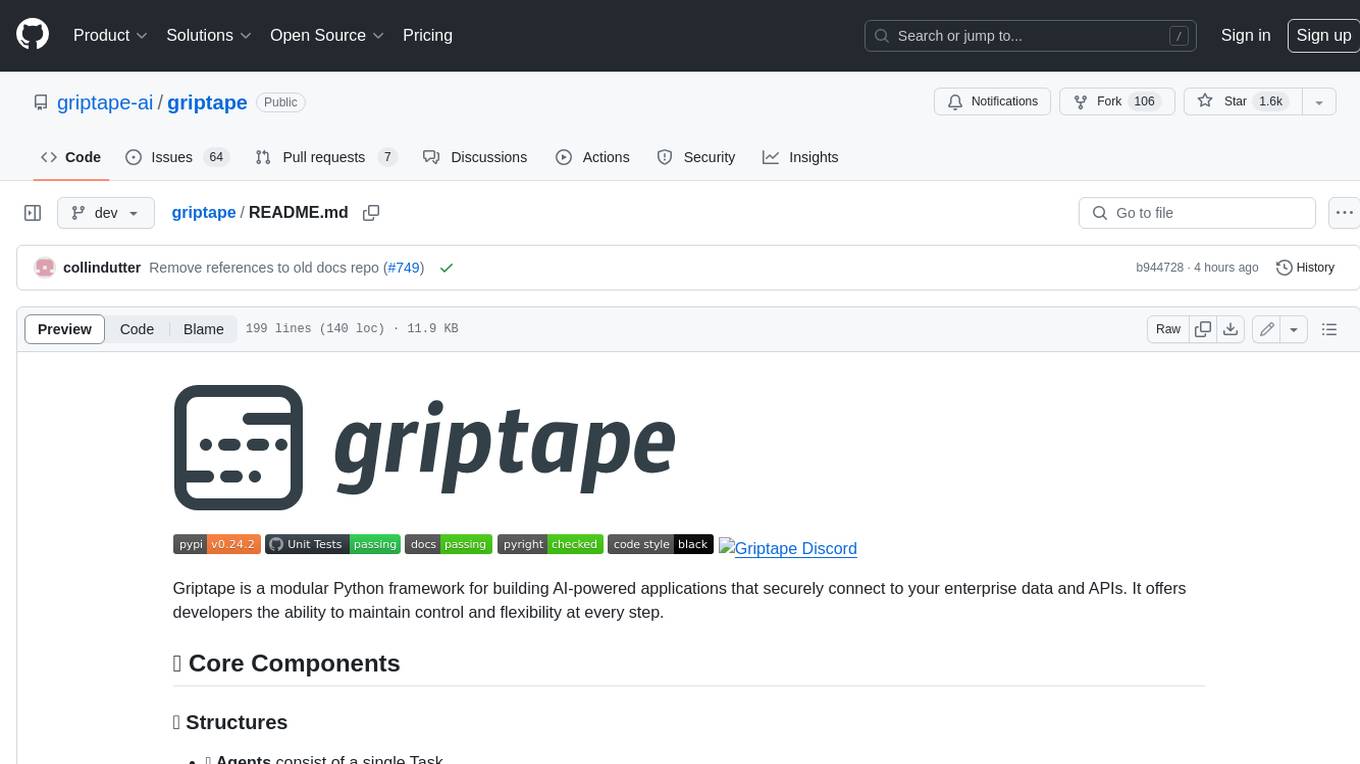
griptape
Griptape is a modular Python framework for building AI-powered applications that securely connect to your enterprise data and APIs. It offers developers the ability to maintain control and flexibility at every step. Griptape's core components include Structures (Agents, Pipelines, and Workflows), Tasks, Tools, Memory (Conversation Memory, Task Memory, and Meta Memory), Drivers (Prompt and Embedding Drivers, Vector Store Drivers, Image Generation Drivers, Image Query Drivers, SQL Drivers, Web Scraper Drivers, and Conversation Memory Drivers), Engines (Query Engines, Extraction Engines, Summary Engines, Image Generation Engines, and Image Query Engines), and additional components (Rulesets, Loaders, Artifacts, Chunkers, and Tokenizers). Griptape enables developers to create AI-powered applications with ease and efficiency.
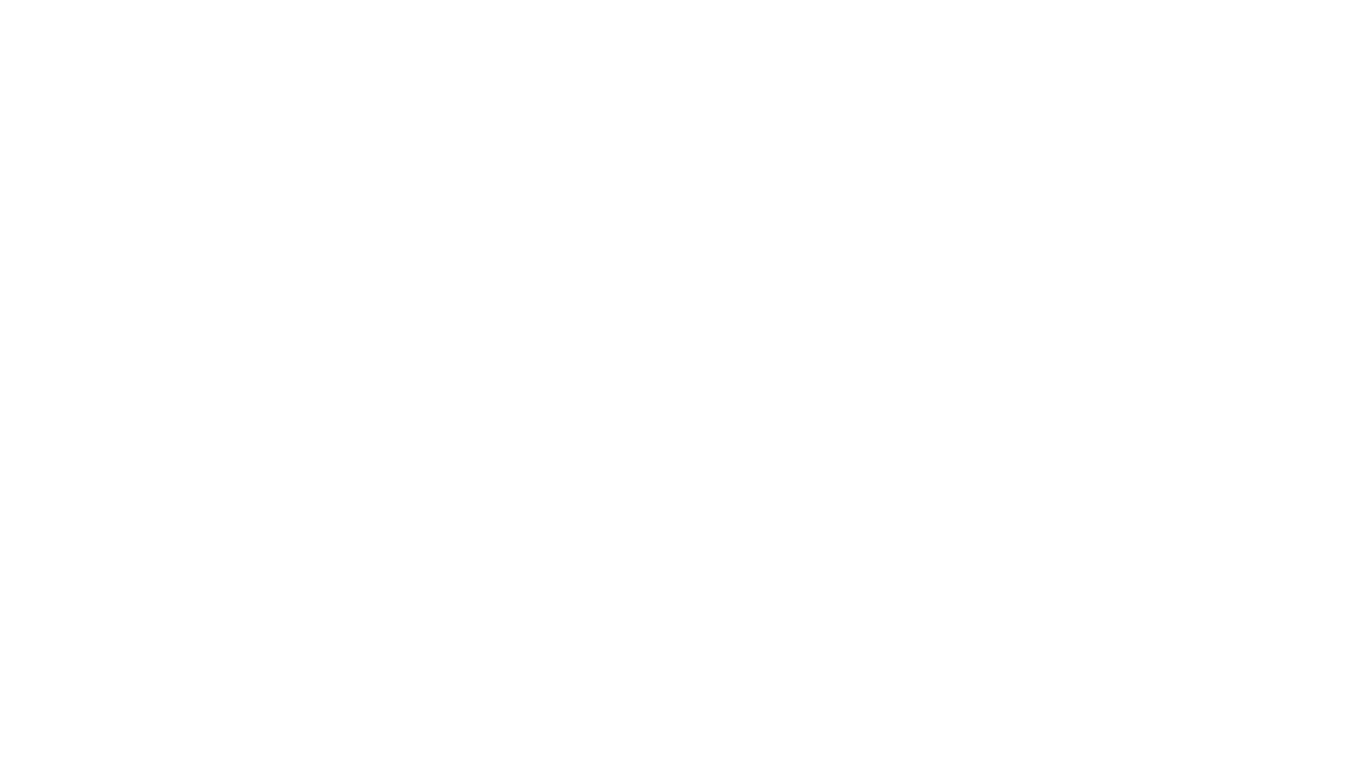
zep-python
Zep is an open-source platform for building and deploying large language model (LLM) applications. It provides a suite of tools and services that make it easy to integrate LLMs into your applications, including chat history memory, embedding, vector search, and data enrichment. Zep is designed to be scalable, reliable, and easy to use, making it a great choice for developers who want to build LLM-powered applications quickly and easily.
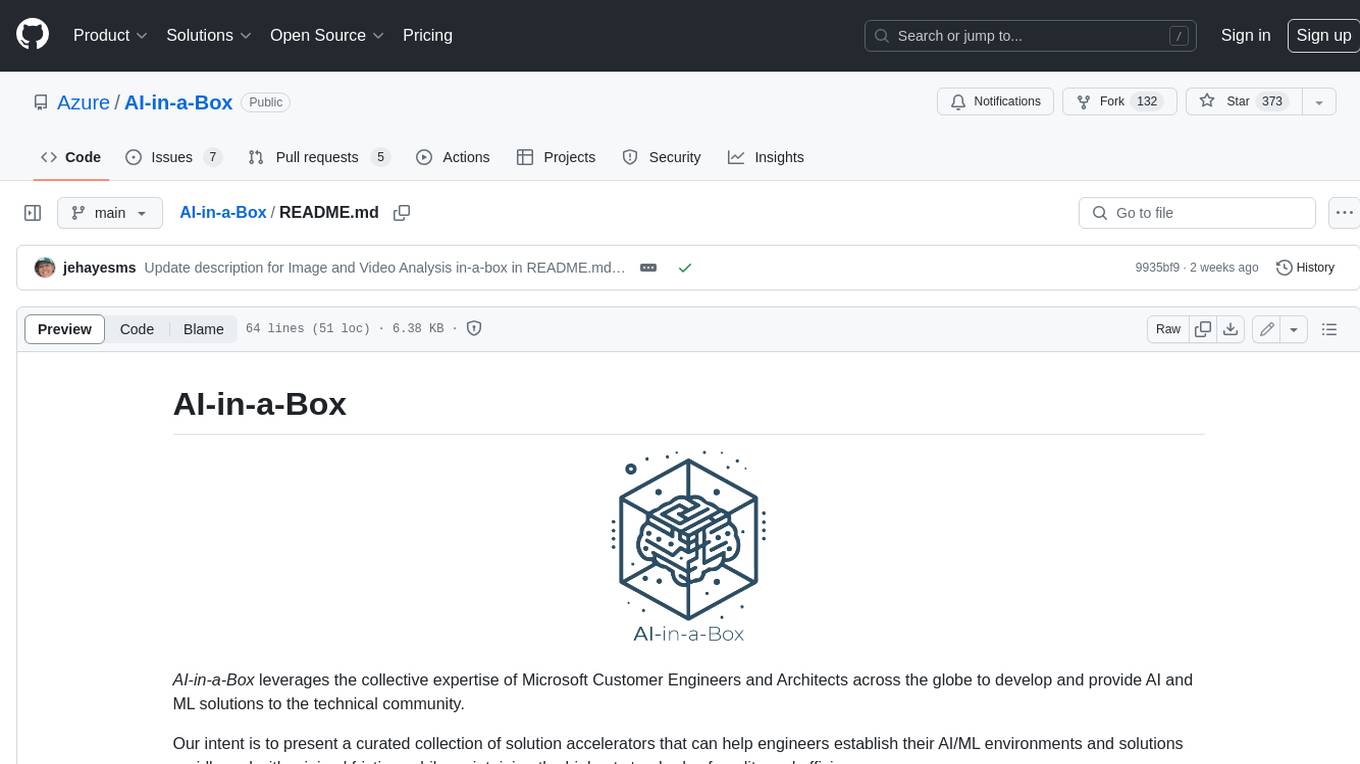
AI-in-a-Box
AI-in-a-Box is a curated collection of solution accelerators that can help engineers establish their AI/ML environments and solutions rapidly and with minimal friction, while maintaining the highest standards of quality and efficiency. It provides essential guidance on the responsible use of AI and LLM technologies, specific security guidance for Generative AI (GenAI) applications, and best practices for scaling OpenAI applications within Azure. The available accelerators include: Azure ML Operationalization in-a-box, Edge AI in-a-box, Doc Intelligence in-a-box, Image and Video Analysis in-a-box, Cognitive Services Landing Zone in-a-box, Semantic Kernel Bot in-a-box, NLP to SQL in-a-box, Assistants API in-a-box, and Assistants API Bot in-a-box.
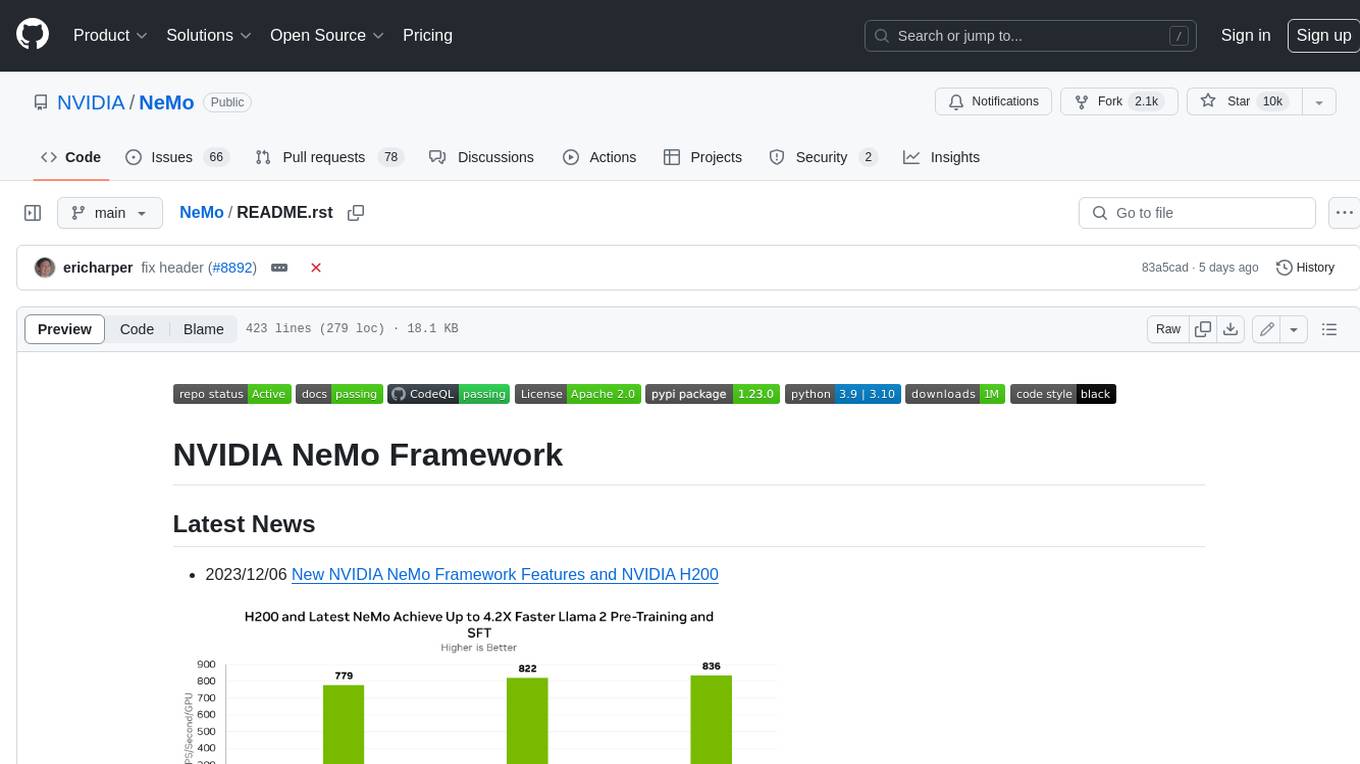
NeMo
NeMo Framework is a generative AI framework built for researchers and pytorch developers working on large language models (LLMs), multimodal models (MM), automatic speech recognition (ASR), and text-to-speech synthesis (TTS). The primary objective of NeMo is to provide a scalable framework for researchers and developers from industry and academia to more easily implement and design new generative AI models by being able to leverage existing code and pretrained models.
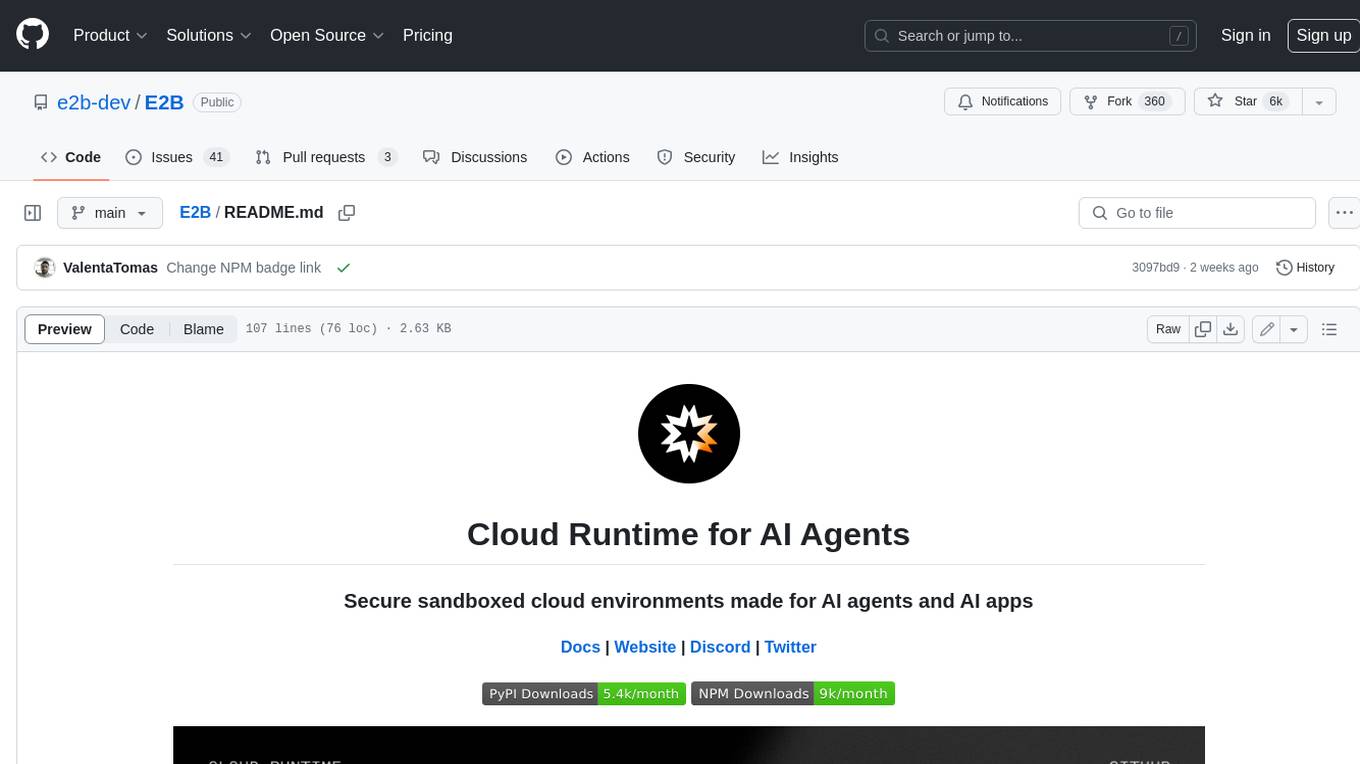
E2B
E2B Sandbox is a secure sandboxed cloud environment made for AI agents and AI apps. Sandboxes allow AI agents and apps to have long running cloud secure environments. In these environments, large language models can use the same tools as humans do. For example: * Cloud browsers * GitHub repositories and CLIs * Coding tools like linters, autocomplete, "go-to defintion" * Running LLM generated code * Audio & video editing The E2B sandbox can be connected to any LLM and any AI agent or app.
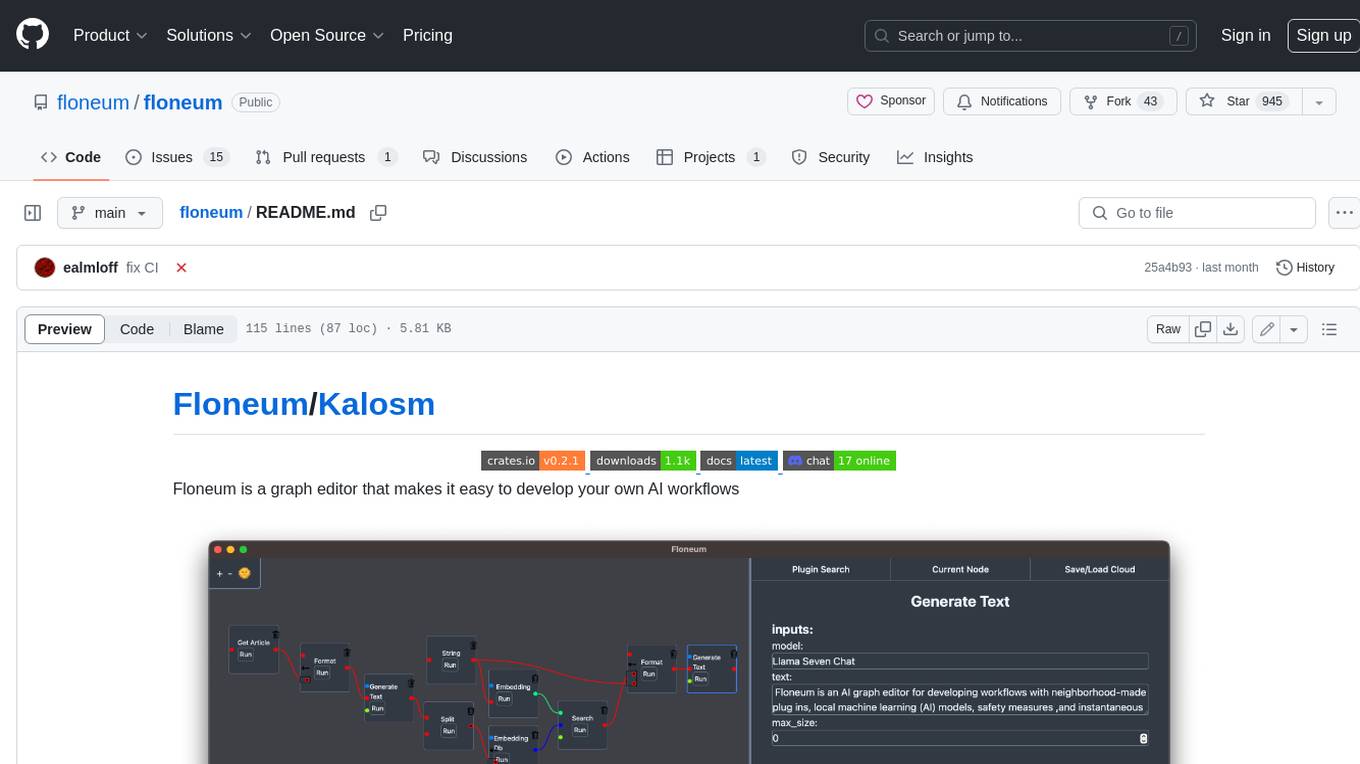
floneum
Floneum is a graph editor that makes it easy to develop your own AI workflows. It uses large language models (LLMs) to run AI models locally, without any external dependencies or even a GPU. This makes it easy to use LLMs with your own data, without worrying about privacy. Floneum also has a plugin system that allows you to improve the performance of LLMs and make them work better for your specific use case. Plugins can be used in any language that supports web assembly, and they can control the output of LLMs with a process similar to JSONformer or guidance.
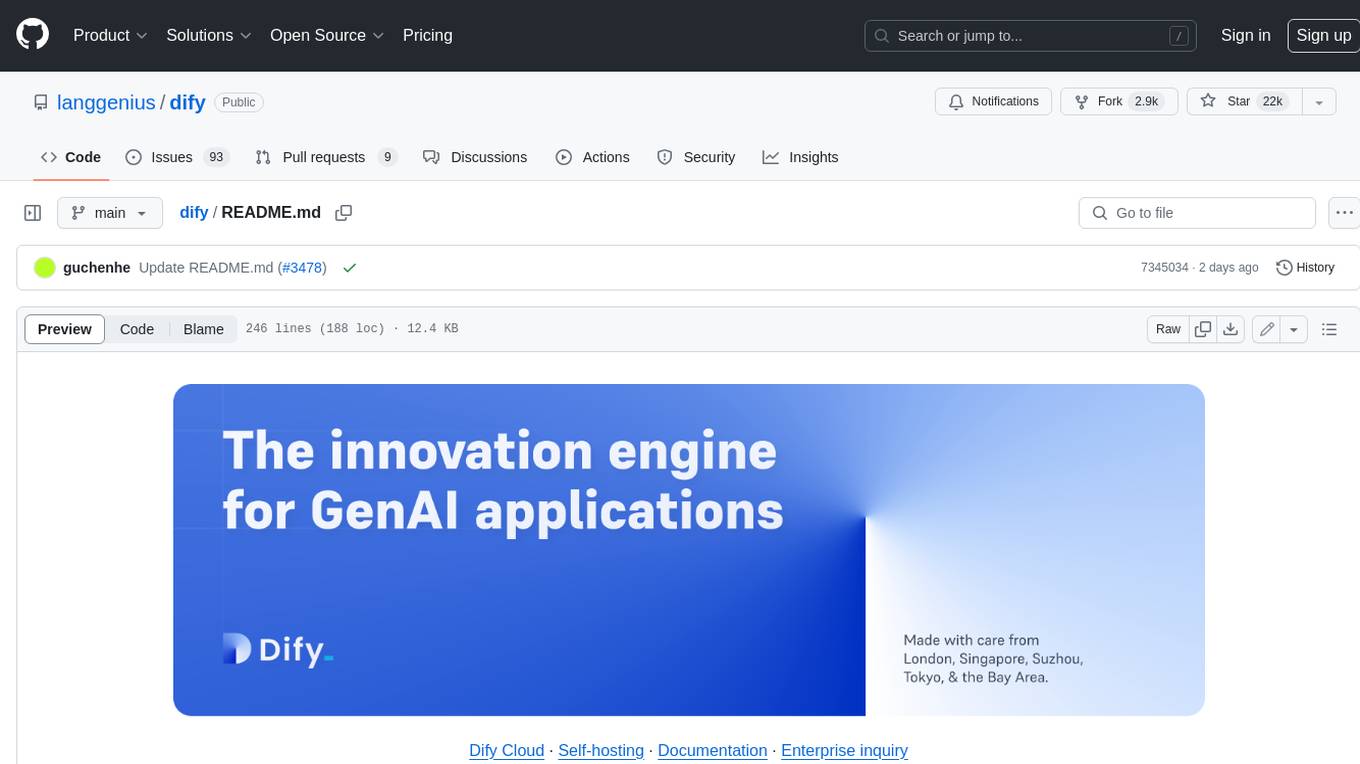
dify
Dify is an open-source LLM app development platform that combines AI workflow, RAG pipeline, agent capabilities, model management, observability features, and more. It allows users to quickly go from prototype to production. Key features include: 1. Workflow: Build and test powerful AI workflows on a visual canvas. 2. Comprehensive model support: Seamless integration with hundreds of proprietary / open-source LLMs from dozens of inference providers and self-hosted solutions. 3. Prompt IDE: Intuitive interface for crafting prompts, comparing model performance, and adding additional features. 4. RAG Pipeline: Extensive RAG capabilities that cover everything from document ingestion to retrieval. 5. Agent capabilities: Define agents based on LLM Function Calling or ReAct, and add pre-built or custom tools. 6. LLMOps: Monitor and analyze application logs and performance over time. 7. Backend-as-a-Service: All of Dify's offerings come with corresponding APIs for easy integration into your own business logic.
For similar jobs
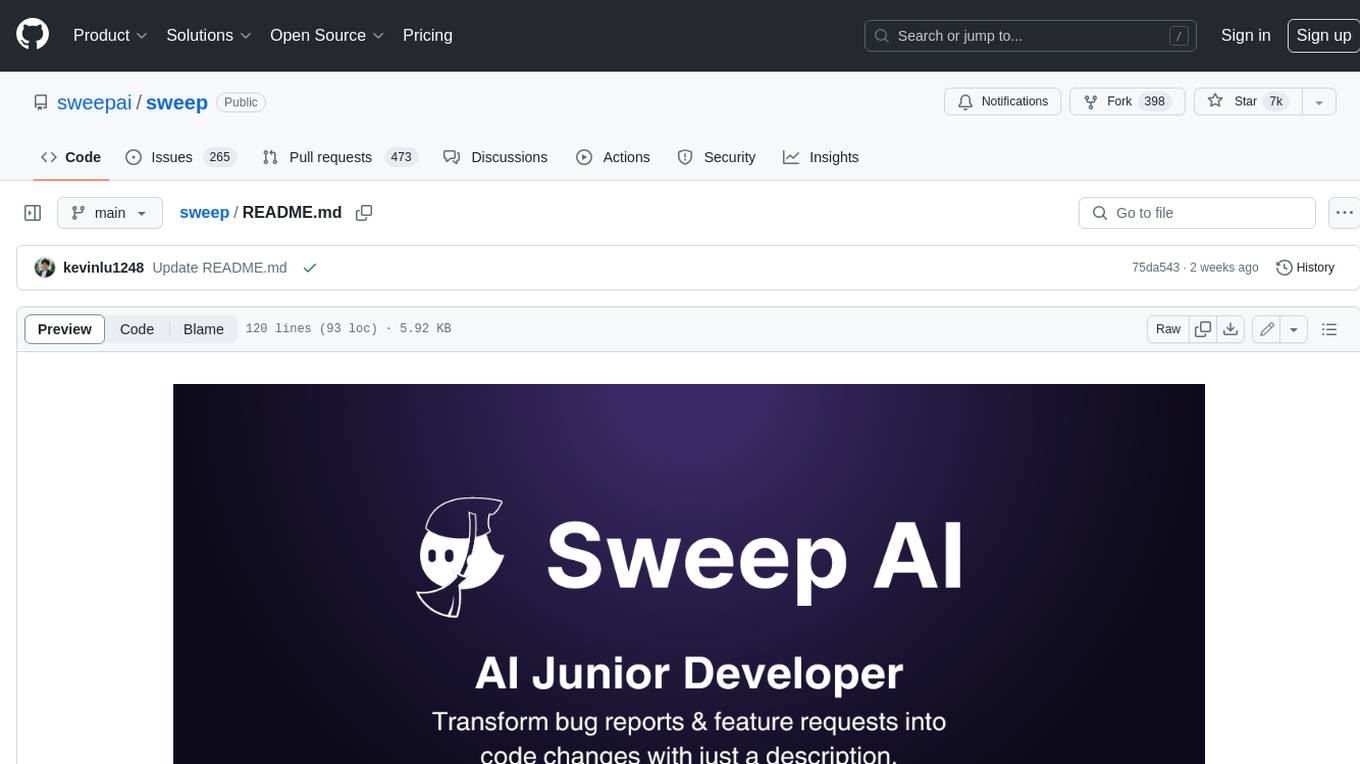
sweep
Sweep is an AI junior developer that turns bugs and feature requests into code changes. It automatically handles developer experience improvements like adding type hints and improving test coverage.
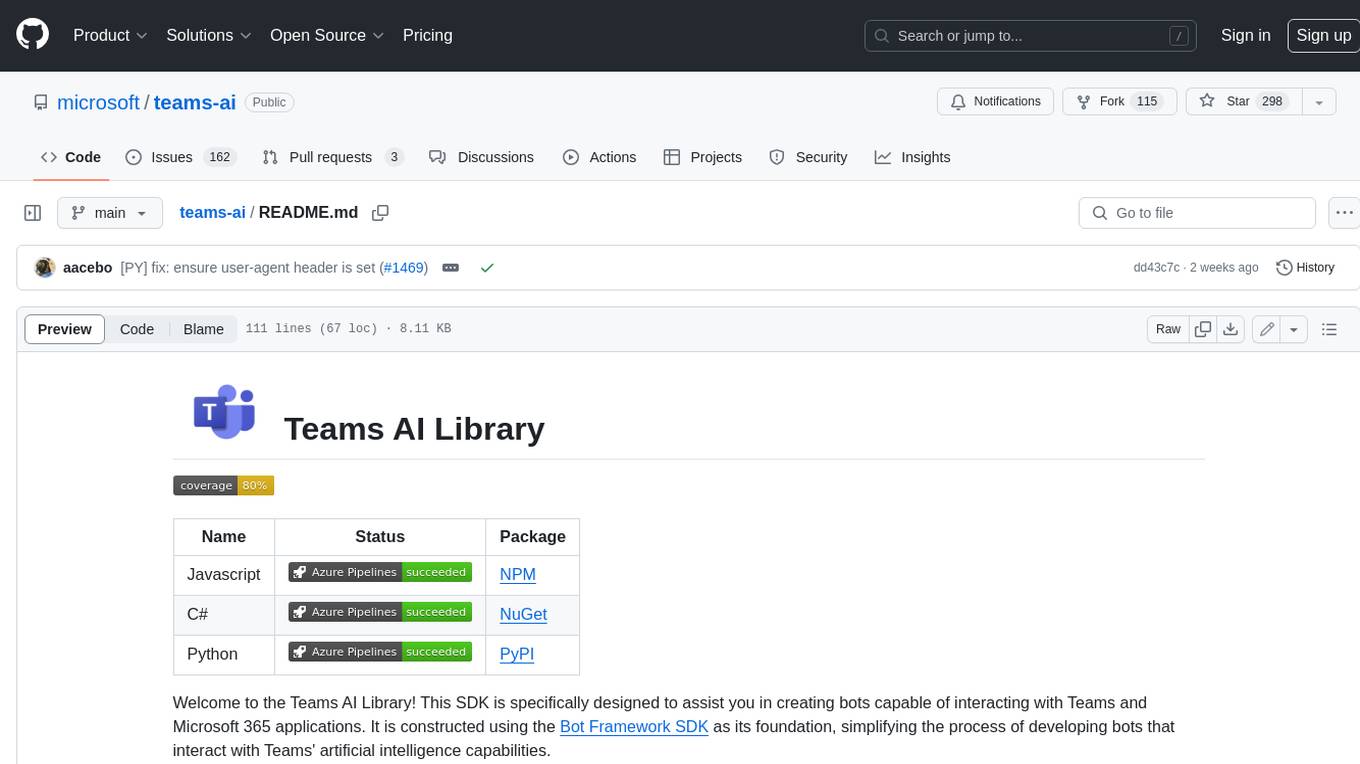
teams-ai
The Teams AI Library is a software development kit (SDK) that helps developers create bots that can interact with Teams and Microsoft 365 applications. It is built on top of the Bot Framework SDK and simplifies the process of developing bots that interact with Teams' artificial intelligence capabilities. The SDK is available for JavaScript/TypeScript, .NET, and Python.
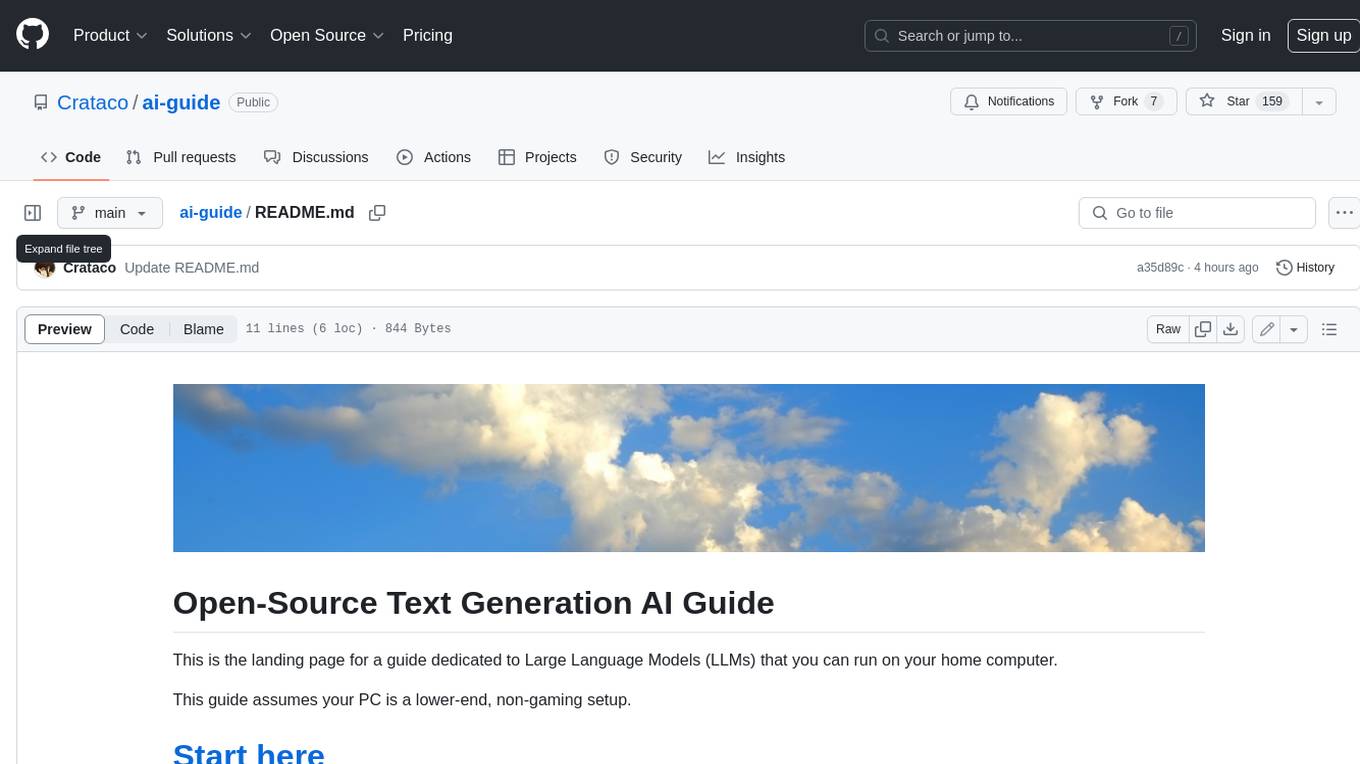
ai-guide
This guide is dedicated to Large Language Models (LLMs) that you can run on your home computer. It assumes your PC is a lower-end, non-gaming setup.
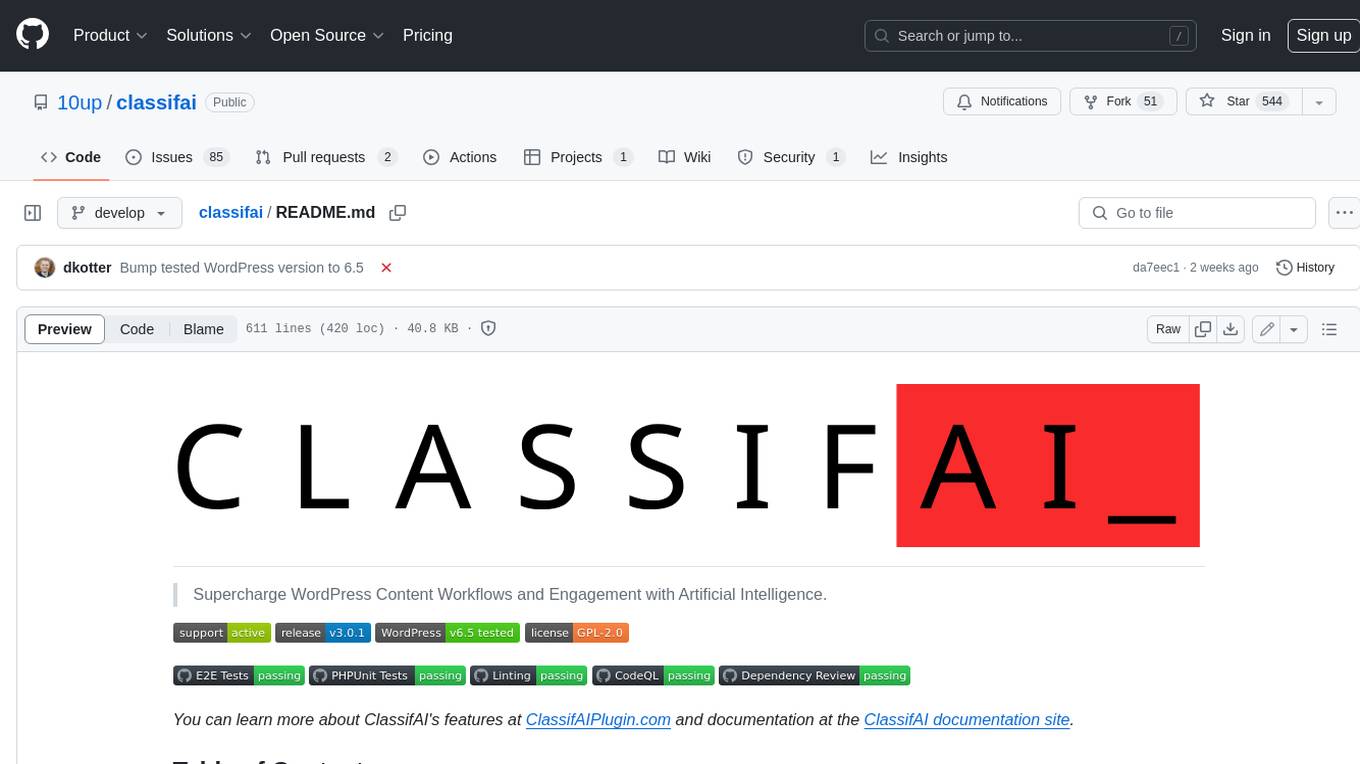
classifai
Supercharge WordPress Content Workflows and Engagement with Artificial Intelligence. Tap into leading cloud-based services like OpenAI, Microsoft Azure AI, Google Gemini and IBM Watson to augment your WordPress-powered websites. Publish content faster while improving SEO performance and increasing audience engagement. ClassifAI integrates Artificial Intelligence and Machine Learning technologies to lighten your workload and eliminate tedious tasks, giving you more time to create original content that matters.
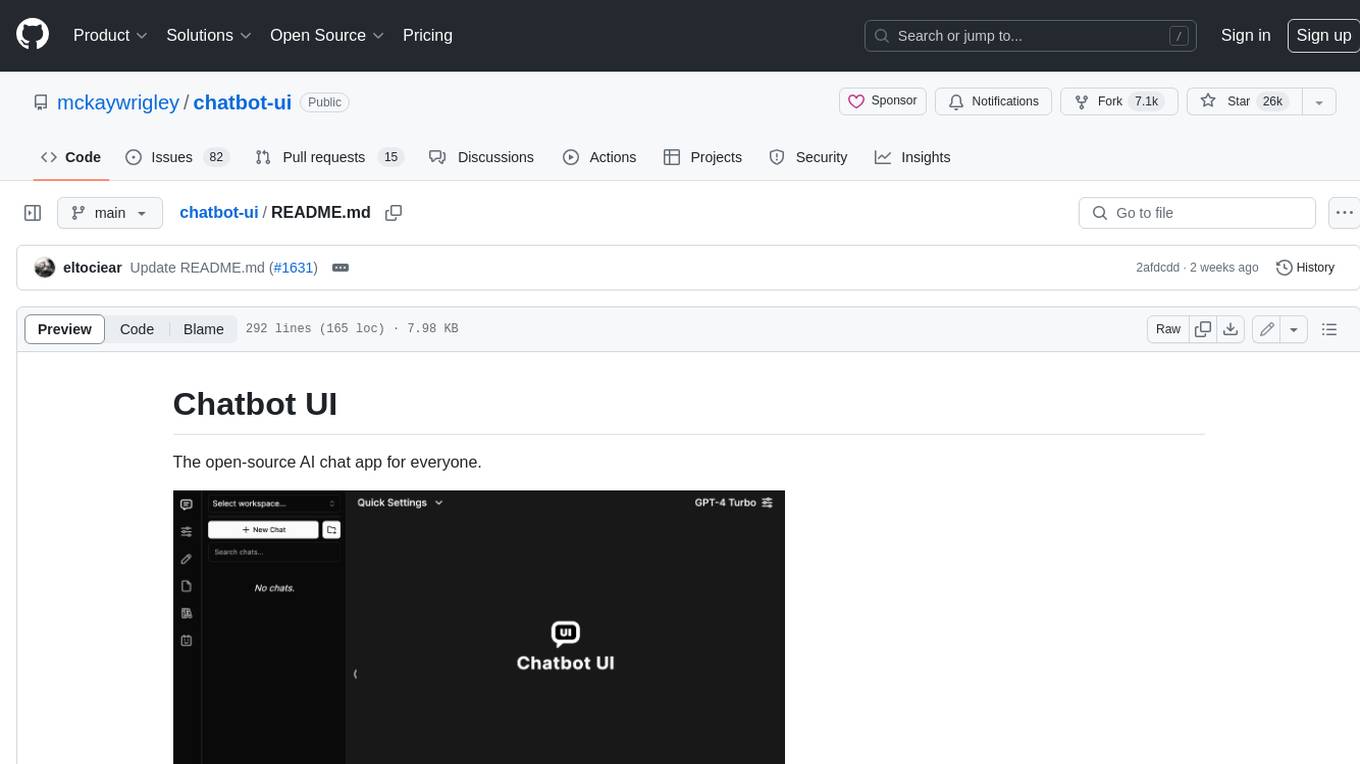
chatbot-ui
Chatbot UI is an open-source AI chat app that allows users to create and deploy their own AI chatbots. It is easy to use and can be customized to fit any need. Chatbot UI is perfect for businesses, developers, and anyone who wants to create a chatbot.
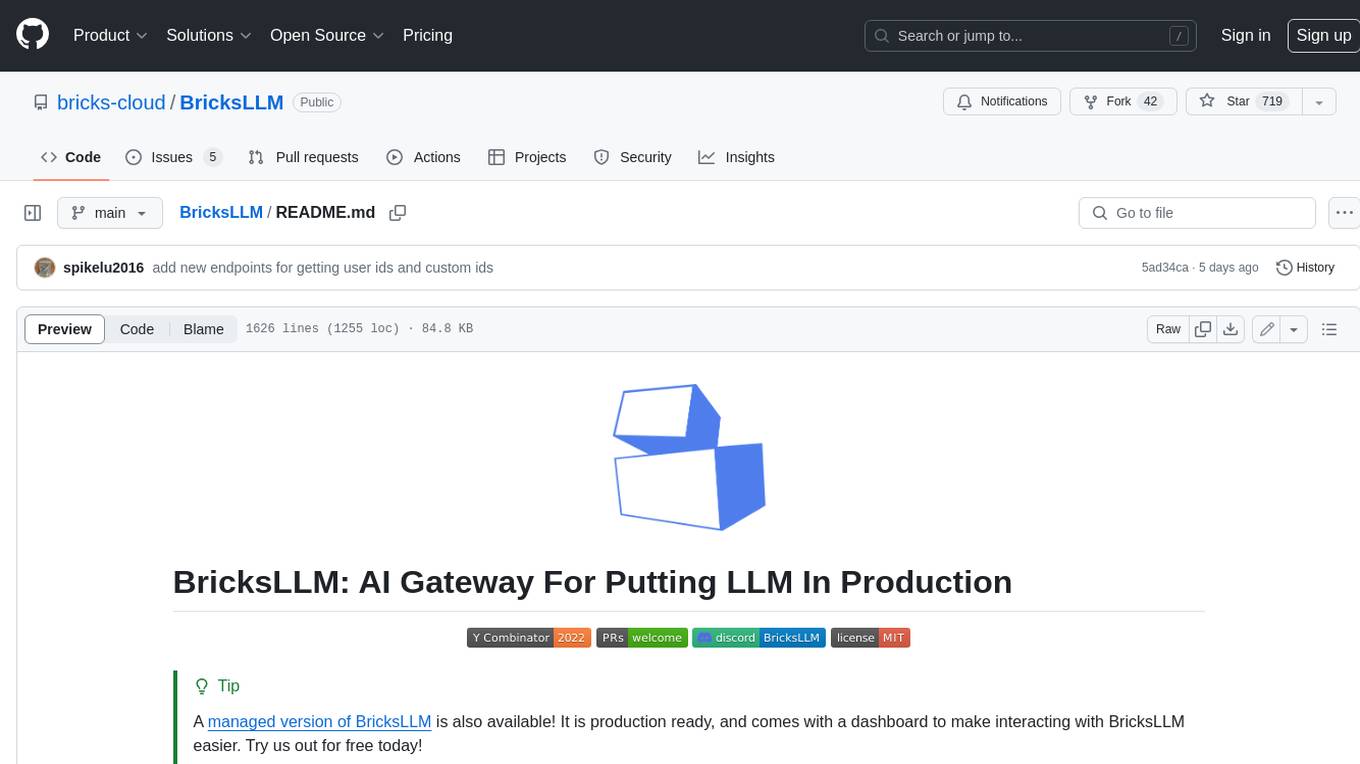
BricksLLM
BricksLLM is a cloud native AI gateway written in Go. Currently, it provides native support for OpenAI, Anthropic, Azure OpenAI and vLLM. BricksLLM aims to provide enterprise level infrastructure that can power any LLM production use cases. Here are some use cases for BricksLLM: * Set LLM usage limits for users on different pricing tiers * Track LLM usage on a per user and per organization basis * Block or redact requests containing PIIs * Improve LLM reliability with failovers, retries and caching * Distribute API keys with rate limits and cost limits for internal development/production use cases * Distribute API keys with rate limits and cost limits for students
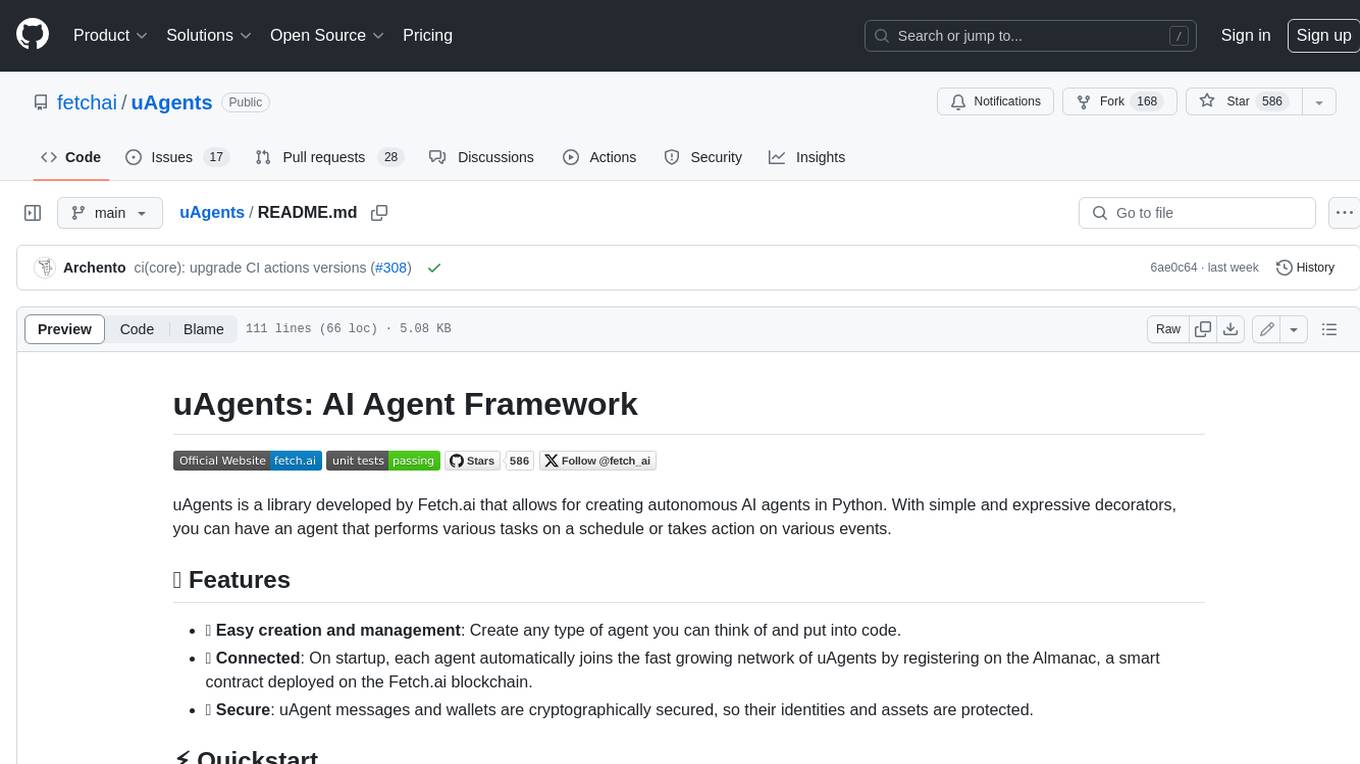
uAgents
uAgents is a Python library developed by Fetch.ai that allows for the creation of autonomous AI agents. These agents can perform various tasks on a schedule or take action on various events. uAgents are easy to create and manage, and they are connected to a fast-growing network of other uAgents. They are also secure, with cryptographically secured messages and wallets.
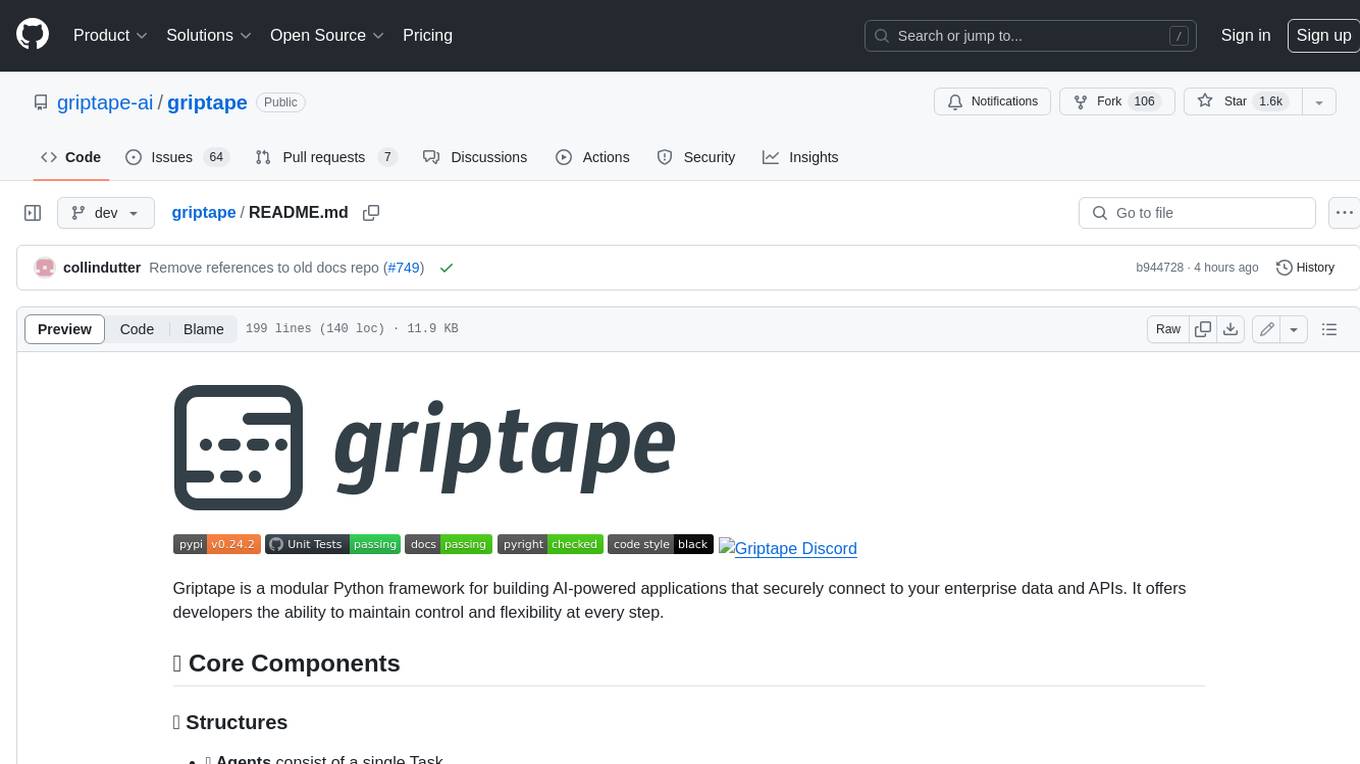
griptape
Griptape is a modular Python framework for building AI-powered applications that securely connect to your enterprise data and APIs. It offers developers the ability to maintain control and flexibility at every step. Griptape's core components include Structures (Agents, Pipelines, and Workflows), Tasks, Tools, Memory (Conversation Memory, Task Memory, and Meta Memory), Drivers (Prompt and Embedding Drivers, Vector Store Drivers, Image Generation Drivers, Image Query Drivers, SQL Drivers, Web Scraper Drivers, and Conversation Memory Drivers), Engines (Query Engines, Extraction Engines, Summary Engines, Image Generation Engines, and Image Query Engines), and additional components (Rulesets, Loaders, Artifacts, Chunkers, and Tokenizers). Griptape enables developers to create AI-powered applications with ease and efficiency.