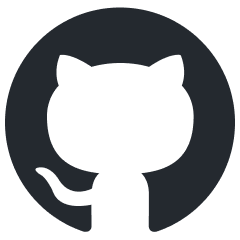
NeMo
A scalable generative AI framework built for researchers and developers working on Large Language Models, Multimodal, and Speech AI (Automatic Speech Recognition and Text-to-Speech)
Stars: 11842
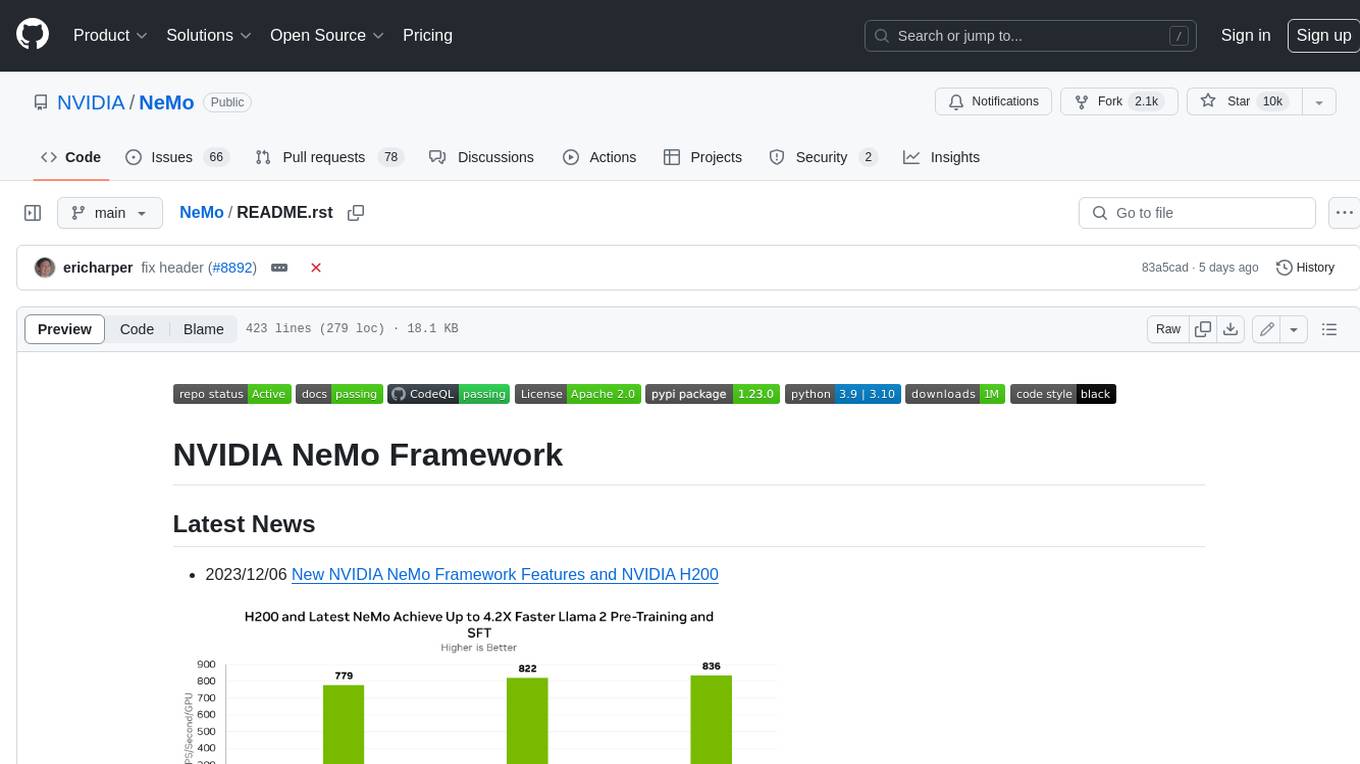
NeMo Framework is a generative AI framework built for researchers and pytorch developers working on large language models (LLMs), multimodal models (MM), automatic speech recognition (ASR), and text-to-speech synthesis (TTS). The primary objective of NeMo is to provide a scalable framework for researchers and developers from industry and academia to more easily implement and design new generative AI models by being able to leverage existing code and pretrained models.
README:
NeMo 2.0
We've released NeMo 2.0, an update on the NeMo Framework which prioritizes modularity and ease-of-use. Please refer to the NeMo Framework User Guide to get started.Large Language Models and Multimodal Models
New Llama 3.1 Support (2024-07-23)
The NeMo Framework now supports training and customizing the Llama 3.1 collection of LLMs from Meta.Accelerate your Generative AI Distributed Training Workloads with the NVIDIA NeMo Framework on Amazon EKS (2024-07-16)
NVIDIA NeMo Framework now runs distributed training workloads on an Amazon Elastic Kubernetes Service (Amazon EKS) cluster. For step-by-step instructions on creating an EKS cluster and running distributed training workloads with NeMo, see the GitHub repository here.NVIDIA NeMo Accelerates LLM Innovation with Hybrid State Space Model Support (2024/06/17)
NVIDIA NeMo and Megatron Core now support pre-training and fine-tuning of state space models (SSMs). NeMo also supports training models based on the Griffin architecture as described by Google DeepMind.NVIDIA releases 340B base, instruct, and reward models pretrained on a total of 9T tokens. (2024-06-18)
See documentation and tutorials for SFT, PEFT, and PTQ with Nemotron 340B in the NeMo Framework User Guide.NVIDIA sets new generative AI performance and scale records in MLPerf Training v4.0 (2024/06/12)
Using NVIDIA NeMo Framework and NVIDIA Hopper GPUs NVIDIA was able to scale to 11,616 H100 GPUs and achieve near-linear performance scaling on LLM pretraining. NVIDIA also achieved the highest LLM fine-tuning performance and raised the bar for text-to-image training.Accelerate your generative AI journey with NVIDIA NeMo Framework on GKE (2024/03/16)
An end-to-end walkthrough to train generative AI models on the Google Kubernetes Engine (GKE) using the NVIDIA NeMo Framework is available at https://github.com/GoogleCloudPlatform/nvidia-nemo-on-gke. The walkthrough includes detailed instructions on how to set up a Google Cloud Project and pre-train a GPT model using the NeMo Framework.Speech Recognition
Accelerating Leaderboard-Topping ASR Models 10x with NVIDIA NeMo (2024/09/24)
NVIDIA NeMo team released a number of inference optimizations for CTC, RNN-T, and TDT models that resulted in up to 10x inference speed-up. These models now exceed an inverse real-time factor (RTFx) of 2,000, with some reaching RTFx of even 6,000.New Standard for Speech Recognition and Translation from the NVIDIA NeMo Canary Model (2024/04/18)
The NeMo team just released Canary, a multilingual model that transcribes speech in English, Spanish, German, and French with punctuation and capitalization. Canary also provides bi-directional translation, between English and the three other supported languages.Pushing the Boundaries of Speech Recognition with NVIDIA NeMo Parakeet ASR Models (2024/04/18)
NVIDIA NeMo, an end-to-end platform for the development of multimodal generative AI models at scale anywhere—on any cloud and on-premises—released the Parakeet family of automatic speech recognition (ASR) models. These state-of-the-art ASR models, developed in collaboration with Suno.ai, transcribe spoken English with exceptional accuracy.Turbocharge ASR Accuracy and Speed with NVIDIA NeMo Parakeet-TDT (2024/04/18)
NVIDIA NeMo, an end-to-end platform for developing multimodal generative AI models at scale anywhere—on any cloud and on-premises—recently released Parakeet-TDT. This new addition to the NeMo ASR Parakeet model family boasts better accuracy and 64% greater speed over the previously best model, Parakeet-RNNT-1.1B.NVIDIA NeMo Framework is a scalable and cloud-native generative AI framework built for researchers and PyTorch developers working on Large Language Models (LLMs), Multimodal Models (MMs), Automatic Speech Recognition (ASR), Text to Speech (TTS), and Computer Vision (CV) domains. It is designed to help you efficiently create, customize, and deploy new generative AI models by leveraging existing code and pre-trained model checkpoints.
For technical documentation, please see the NeMo Framework User Guide.
NVIDIA NeMo 2.0 introduces several significant improvements over its predecessor, NeMo 1.0, enhancing flexibility, performance, and scalability.
-
Python-Based Configuration - NeMo 2.0 transitions from YAML files to a Python-based configuration, providing more flexibility and control. This shift makes it easier to extend and customize configurations programmatically.
-
Modular Abstractions - By adopting PyTorch Lightning’s modular abstractions, NeMo 2.0 simplifies adaptation and experimentation. This modular approach allows developers to more easily modify and experiment with different components of their models.
-
Scalability - NeMo 2.0 seamlessly scaling large-scale experiments across thousands of GPUs using NeMo-Run, a powerful tool designed to streamline the configuration, execution, and management of machine learning experiments across computing environments.
Overall, these enhancements make NeMo 2.0 a powerful, scalable, and user-friendly framework for AI model development.
[!IMPORTANT]
NeMo 2.0 is currently supported by the LLM (large language model) and VLM (vision language model) collections.
- Refer to the Quickstart for examples of using NeMo-Run to launch NeMo 2.0 experiments locally and on a slurm cluster.
- For more information about NeMo 2.0, see the NeMo Framework User Guide.
- NeMo 2.0 Recipes contains additional examples of launching large-scale runs using NeMo 2.0 and NeMo-Run.
- For an in-depth exploration of the main features of NeMo 2.0, see the Feature Guide.
- To transition from NeMo 1.0 to 2.0, see the Migration Guide for step-by-step instructions.
All NeMo models are trained with Lightning. Training is automatically scalable to 1000s of GPUs.
When applicable, NeMo models leverage cutting-edge distributed training techniques, incorporating parallelism strategies to enable efficient training of very large models. These techniques include Tensor Parallelism (TP), Pipeline Parallelism (PP), Fully Sharded Data Parallelism (FSDP), Mixture-of-Experts (MoE), and Mixed Precision Training with BFloat16 and FP8, as well as others.
NeMo Transformer-based LLMs and MMs utilize NVIDIA Transformer Engine for FP8 training on NVIDIA Hopper GPUs, while leveraging NVIDIA Megatron Core for scaling Transformer model training.
NeMo LLMs can be aligned with state-of-the-art methods such as SteerLM, Direct Preference Optimization (DPO), and Reinforcement Learning from Human Feedback (RLHF). See NVIDIA NeMo Aligner for more information.
In addition to supervised fine-tuning (SFT), NeMo also supports the latest parameter efficient fine-tuning (PEFT) techniques such as LoRA, P-Tuning, Adapters, and IA3. Refer to the NeMo Framework User Guide for the full list of supported models and techniques.
NeMo LLMs and MMs can be deployed and optimized with NVIDIA NeMo Microservices.
NeMo ASR and TTS models can be optimized for inference and deployed for production use cases with NVIDIA Riva.
[!IMPORTANT]
NeMo Framework Launcher is compatible with NeMo version 1.0 only. NeMo-Run is recommended for launching experiments using NeMo 2.0.
NeMo Framework Launcher is a cloud-native tool that streamlines the NeMo Framework experience. It is used for launching end-to-end NeMo Framework training jobs on CSPs and Slurm clusters.
The NeMo Framework Launcher includes extensive recipes, scripts, utilities, and documentation for training NeMo LLMs. It also includes the NeMo Framework Autoconfigurator, which is designed to find the optimal model parallel configuration for training on a specific cluster.
To get started quickly with the NeMo Framework Launcher, please see the NeMo Framework Playbooks. The NeMo Framework Launcher does not currently support ASR and TTS training, but it will soon.
Getting started with NeMo Framework is easy. State-of-the-art pretrained NeMo models are freely available on Hugging Face Hub and NVIDIA NGC. These models can be used to generate text or images, transcribe audio, and synthesize speech in just a few lines of code.
We have extensive tutorials that can be run on Google Colab or with our NGC NeMo Framework Container. We also have playbooks for users who want to train NeMo models with the NeMo Framework Launcher.
For advanced users who want to train NeMo models from scratch or fine-tune existing NeMo models, we have a full suite of example scripts that support multi-GPU/multi-node training.
- Python 3.10 or above
- Pytorch 1.13.1 or above
- NVIDIA GPU (if you intend to do model training)
Version | Status | Description |
---|---|---|
Latest | Documentation of the latest (i.e. main) branch. | |
Stable | Documentation of the stable (i.e. most recent release) |
The NeMo Framework can be installed in a variety of ways, depending on your needs. Depending on the domain, you may find one of the following installation methods more suitable.
- Conda / Pip - Refer to Conda and Pip for
installation instructions.
- This is the recommended method for ASR and TTS domains.
- When using a Nvidia PyTorch container as the base, this is the recommended method for all domains.
- Docker Containers - Refer to Docker containers
for installation instructions.
- NeMo Framework container - [nvcr.io/nvidia/nemo:24.05]{.title-ref}
- LLMs and MMs Dependencies - Refer to LLMs and MMs Dependencies for installation instructions.
Important: We strongly recommended that you start with a base NVIDIA PyTorch container: nvcr.io/nvidia/pytorch:24.02-py3.
Install NeMo in a fresh Conda environment:
conda create --name nemo python==3.10.12
conda activate nemo
Install PyTorch using their configurator:
conda install pytorch torchvision torchaudio pytorch-cuda=11.8 -c pytorch -c nvidia
The command to install PyTorch may depend on your system. Use the configurator linked above to find the right command for your system.
Then, install NeMo via Pip or from Source. We do not provide NeMo on the conda-forge or any other Conda channel.
To install the nemo_toolkit, use the following installation method:
apt-get update && apt-get install -y libsndfile1 ffmpeg
pip install Cython packaging
pip install nemo_toolkit['all']
Depending on the shell used, you may need to use the
"nemo_toolkit[all]"
specifier instead in the above command.
To install a specific domain of NeMo, you must first install the nemo_toolkit using the instructions listed above. Then, you run the following domain-specific commands:
pip install nemo_toolkit['asr']
pip install nemo_toolkit['nlp']
pip install nemo_toolkit['tts']
pip install nemo_toolkit['vision']
pip install nemo_toolkit['multimodal']
If you want to work with a specific version of NeMo from a particular GitHub branch (e.g main), use the following installation method:
apt-get update && apt-get install -y libsndfile1 ffmpeg
pip install Cython packaging
python -m pip install git+https://github.com/NVIDIA/NeMo.git@{BRANCH}#egg=nemo_toolkit[all]
If you want to clone the NeMo GitHub repository and contribute to NeMo open-source development work, use the following installation method:
apt-get update && apt-get install -y libsndfile1 ffmpeg
git clone https://github.com/NVIDIA/NeMo
cd NeMo
./reinstall.sh
If you only want the toolkit without the additional Conda-based
dependencies, you can replace reinstall.sh
with pip install -e .
when your PWD is the root of the NeMo repository.
To install NeMo on Mac computers with the Apple M-Series GPU, you need to create a new Conda environment, install PyTorch 2.0 or higher, and then install the nemo_toolkit.
Important: This method is only applicable to the ASR domain.
Run the following code:
# [optional] install mecab using Homebrew, to use sacrebleu for NLP collection
# you can install Homebrew here: https://brew.sh
brew install mecab
# [optional] install pynini using Conda, to use text normalization
conda install -c conda-forge pynini
# install Cython manually
pip install cython packaging
# clone the repo and install in development mode
git clone https://github.com/NVIDIA/NeMo
cd NeMo
pip install 'nemo_toolkit[all]'
# Note that only the ASR toolkit is guaranteed to work on MacBook - so for MacBook use pip install 'nemo_toolkit[asr]'
To install the Windows Subsystem for Linux (WSL), run the following code in PowerShell:
wsl --install
# [note] If you run wsl --install and see the WSL help text, it means WSL is already installed.
To learn more about installing WSL, refer to Microsoft's official documentation.
After installing your Linux distribution with WSL, two options are available:
Option 1: Open the distribution (Ubuntu by default) from the Start menu and follow the instructions.
Option 2: Launch the Terminal application. Download it from Microsoft's Windows Terminal page if not installed.
Next, follow the instructions for Linux systems, as provided above. For example:
apt-get update && apt-get install -y libsndfile1 ffmpeg
git clone https://github.com/NVIDIA/NeMo
cd NeMo
./reinstall.sh
For optimal performance of a Recurrent Neural Network Transducer (RNNT), install the Numba package from Conda.
Run the following code:
conda remove numba
pip uninstall numba
conda install -c conda-forge numba
If you work with the LLM and MM domains, three additional dependencies are required: NVIDIA Apex, NVIDIA Transformer Engine, and NVIDIA Megatron Core. When working with the [main]{.title-ref} branch, these dependencies may require a recent commit.
The most recent working versions of these dependencies are here:
export apex_commit=810ffae374a2b9cb4b5c5e28eaeca7d7998fca0c
export te_commit=bfe21c3d68b0a9951e5716fb520045db53419c5e
export mcore_commit=02871b4df8c69fac687ab6676c4246e936ce92d0
export nv_pytorch_tag=24.02-py3
When using a released version of NeMo, please refer to the Software Component Versions for the correct versions.
We recommended that you start with a base NVIDIA PyTorch container: nvcr.io/nvidia/pytorch:24.02-py3.
If starting with a base NVIDIA PyTorch container, you must first launch the container:
docker run \
--gpus all \
-it \
--rm \
--shm-size=16g \
--ulimit memlock=-1 \
--ulimit stack=67108864 \
nvcr.io/nvidia/pytorch:$nv_pytorch_tag
Next, you need to install the dependencies.
NVIDIA Apex is required for LLM and MM domains. Although Apex is pre-installed in the NVIDIA PyTorch container, you may need to update it to a newer version.
To install Apex, run the following code:
git clone https://github.com/NVIDIA/apex.git
cd apex
git checkout $apex_commit
pip install . -v --no-build-isolation --disable-pip-version-check --no-cache-dir --config-settings "--build-option=--cpp_ext --cuda_ext --fast_layer_norm --distributed_adam --deprecated_fused_adam --group_norm"
When attempting to install Apex separately from the NVIDIA PyTorch container, you might encounter an error if the CUDA version on your system is different from the one used to compile PyTorch. To bypass this error, you can comment out the relevant line in the setup file located in the Apex repository on GitHub here: https://github.com/NVIDIA/apex/blob/master/setup.py#L32.
cuda-nvprof is needed to install Apex. The version should match the CUDA version that you are using.
To install cuda-nvprof, run the following code:
conda install -c nvidia cuda-nvprof=11.8
Finally, install the packaging:
pip install packaging
To install the most recent versions of Apex locally, it might be necessary to remove the [pyproject.toml]{.title-ref} file from the Apex directory.
NVIDIA Transformer Engine is required for LLM and MM domains. Although the Transformer Engine is pre-installed in the NVIDIA PyTorch container, you may need to update it to a newer version.
The Transformer Engine facilitates training with FP8 precision on NVIDIA Hopper GPUs and introduces many enhancements for the training of Transformer-based models. Refer to Transformer Engine for information.
To install Transformer Engine, run the following code:
git clone https://github.com/NVIDIA/TransformerEngine.git && \
cd TransformerEngine && \
git checkout $te_commit && \
git submodule init && git submodule update && \
NVTE_FRAMEWORK=pytorch NVTE_WITH_USERBUFFERS=1 MPI_HOME=/usr/local/mpi pip install .
Transformer Engine requires PyTorch to be built with at least CUDA 11.8.
Megatron Core is required for LLM and MM domains. Megatron Core is a library for scaling large Transformer-based models. NeMo LLMs and MMs leverage Megatron Core for model parallelism, transformer architectures, and optimized PyTorch datasets.
To install Megatron Core, run the following code:
git clone https://github.com/NVIDIA/Megatron-LM.git && \
cd Megatron-LM && \
git checkout $mcore_commit && \
pip install . && \
cd megatron/core/datasets && \
make
NeMo Text Processing, specifically Inverse Text Normalization, is now a separate repository. It is located here: https://github.com/NVIDIA/NeMo-text-processing.
NeMo containers are launched concurrently with NeMo version updates. NeMo Framework now supports LLMs, MMs, ASR, and TTS in a single consolidated Docker container. You can find additional information about released containers on the NeMo releases page.
To use a pre-built container, run the following code:
docker pull nvcr.io/nvidia/nemo:24.05
To build a nemo container with Dockerfile from a branch, run the following code:
DOCKER_BUILDKIT=1 docker build -f Dockerfile -t nemo:latest
If you choose to work with the main branch, we recommend using NVIDIA's PyTorch container version 23.10-py3 and then installing from GitHub.
docker run --gpus all -it --rm -v <nemo_github_folder>:/NeMo --shm-size=8g \
-p 8888:8888 -p 6006:6006 --ulimit memlock=-1 --ulimit \
stack=67108864 --device=/dev/snd nvcr.io/nvidia/pytorch:23.10-py3
The NeMo Framework Launcher does not currently support ASR and TTS training, but it will soon.
FAQ can be found on the NeMo Discussions board. You are welcome to ask questions or start discussions on the board.
We welcome community contributions! Please refer to CONTRIBUTING.md for the process.
We provide an ever-growing list of publications that utilize the NeMo Framework.
To contribute an article to the collection, please submit a pull request
to the gh-pages-src
branch of this repository. For detailed
information, please consult the README located at the gh-pages-src
branch.
Large Language Models and Multimodal Models
Bria Builds Responsible Generative AI for Enterprises Using NVIDIA NeMo, Picasso (2024/03/06)
Bria, a Tel Aviv startup at the forefront of visual generative AI for enterprises now leverages the NVIDIA NeMo Framework. The Bria.ai platform uses reference implementations from the NeMo Multimodal collection, trained on NVIDIA Tensor Core GPUs, to enable high-throughput and low-latency image generation. Bria has also adopted NVIDIA Picasso, a foundry for visual generative AI models, to run inference.New NVIDIA NeMo Framework Features and NVIDIA H200 (2023/12/06)
NVIDIA NeMo Framework now includes several optimizations and enhancements, including: 1) Fully Sharded Data Parallelism (FSDP) to improve the efficiency of training large-scale AI models, 2) Mix of Experts (MoE)-based LLM architectures with expert parallelism for efficient LLM training at scale, 3) Reinforcement Learning from Human Feedback (RLHF) with TensorRT-LLM for inference stage acceleration, and 4) up to 4.2x speedups for Llama 2 pre-training on NVIDIA H200 Tensor Core GPUs.
NVIDIA now powers training for Amazon Titan Foundation models (2023/11/28)
NVIDIA NeMo Framework now empowers the Amazon Titan foundation models (FM) with efficient training of large language models (LLMs). The Titan FMs form the basis of Amazon’s generative AI service, Amazon Bedrock. The NeMo Framework provides a versatile framework for building, customizing, and running LLMs.- NeMo GitHub Apache 2.0 license
- NeMo is licensed under the NVIDIA AI PRODUCT AGREEMENT. By pulling and using the container, you accept the terms and conditions of this license.
For Tasks:
Click tags to check more tools for each tasksFor Jobs:
Alternative AI tools for NeMo
Similar Open Source Tools
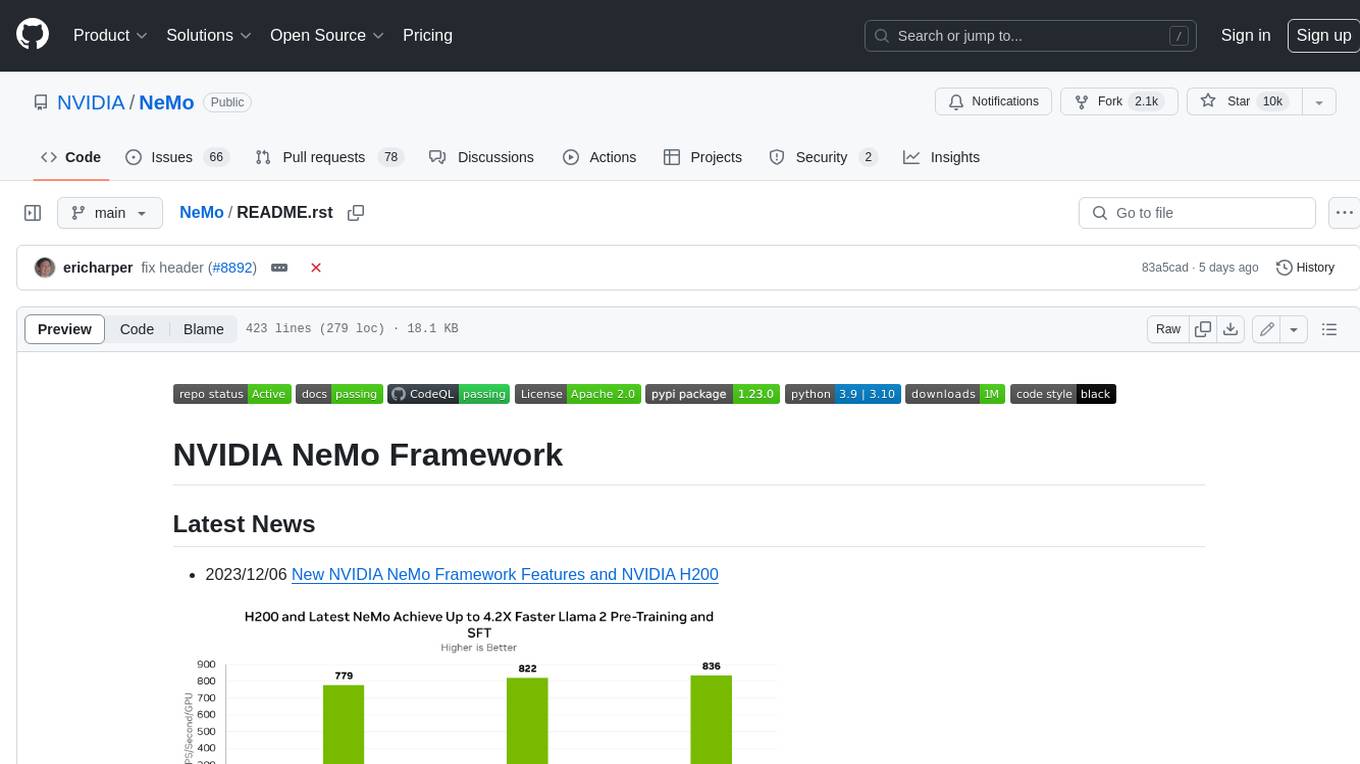
NeMo
NeMo Framework is a generative AI framework built for researchers and pytorch developers working on large language models (LLMs), multimodal models (MM), automatic speech recognition (ASR), and text-to-speech synthesis (TTS). The primary objective of NeMo is to provide a scalable framework for researchers and developers from industry and academia to more easily implement and design new generative AI models by being able to leverage existing code and pretrained models.
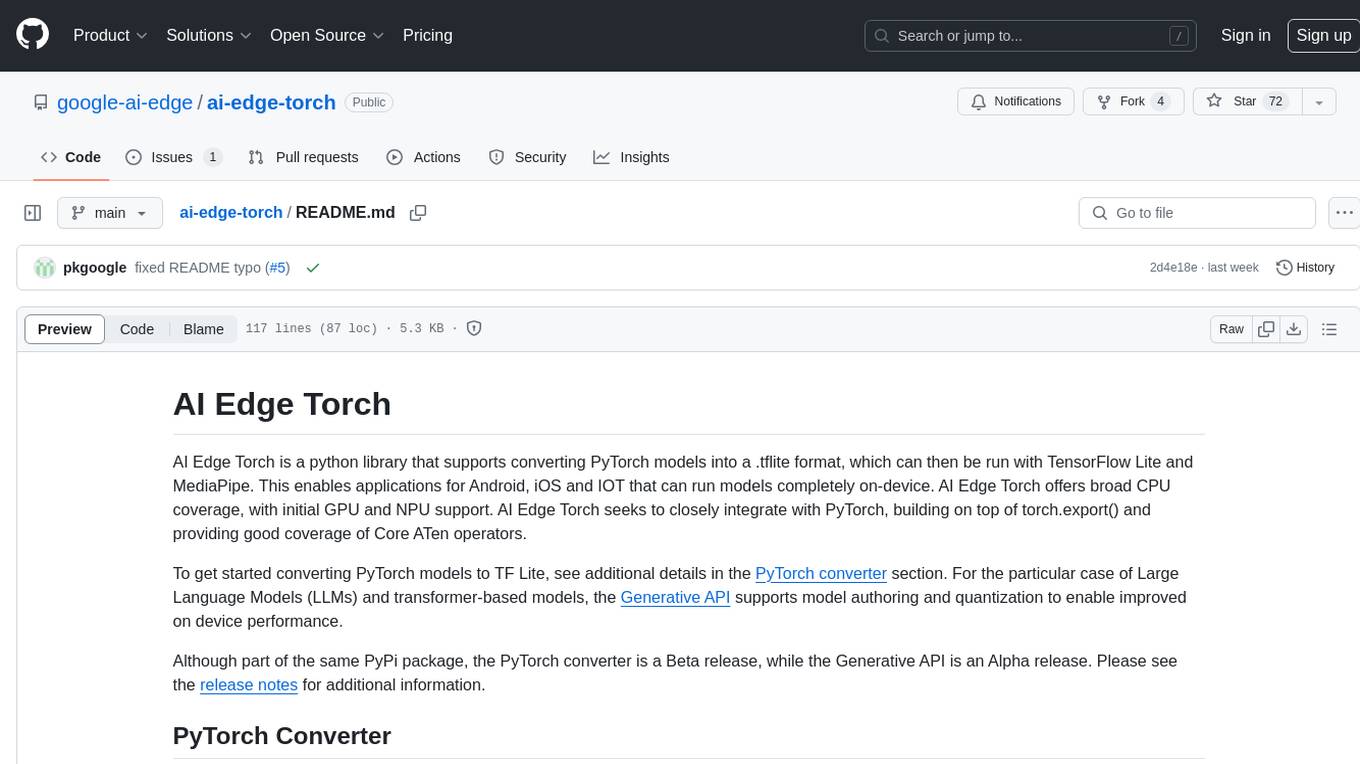
ai-edge-torch
AI Edge Torch is a Python library that supports converting PyTorch models into a .tflite format for on-device applications on Android, iOS, and IoT devices. It offers broad CPU coverage with initial GPU and NPU support, closely integrating with PyTorch and providing good coverage of Core ATen operators. The library includes a PyTorch converter for model conversion and a Generative API for authoring mobile-optimized PyTorch Transformer models, enabling easy deployment of Large Language Models (LLMs) on mobile devices.
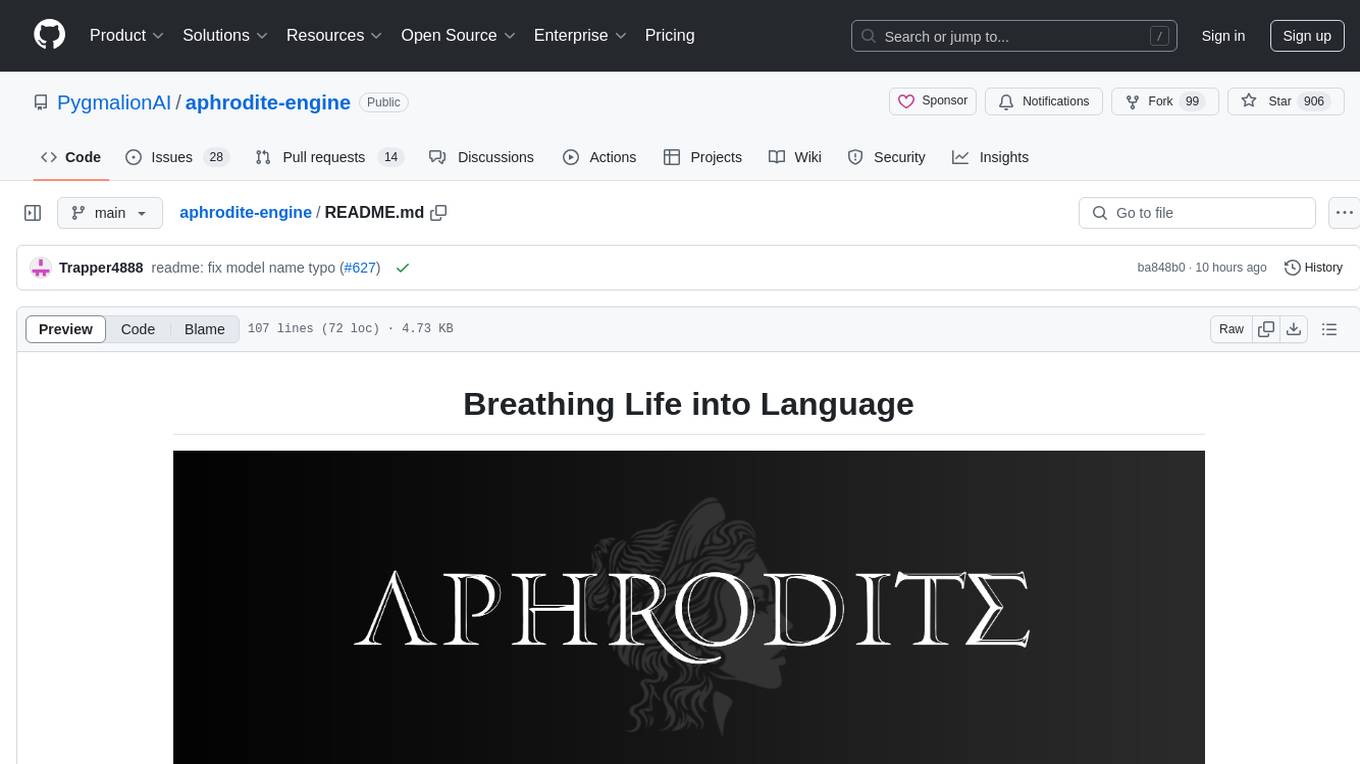
aphrodite-engine
Aphrodite is the official backend engine for PygmalionAI, serving as the inference endpoint for the website. It allows serving Hugging Face-compatible models with fast speeds. Features include continuous batching, efficient K/V management, optimized CUDA kernels, quantization support, distributed inference, and 8-bit KV Cache. The engine requires Linux OS and Python 3.8 to 3.12, with CUDA >= 11 for build requirements. It supports various GPUs, CPUs, TPUs, and Inferentia. Users can limit GPU memory utilization and access full commands via CLI.
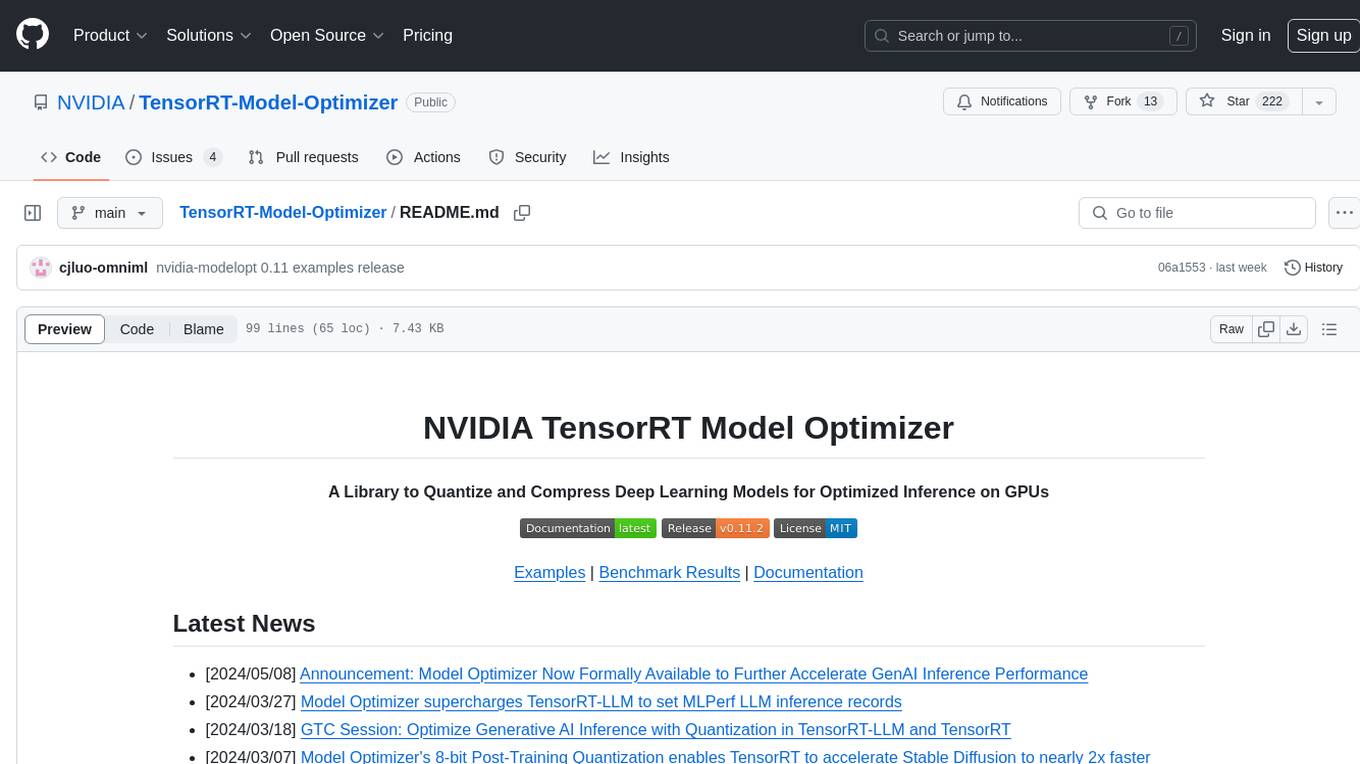
TensorRT-Model-Optimizer
The NVIDIA TensorRT Model Optimizer is a library designed to quantize and compress deep learning models for optimized inference on GPUs. It offers state-of-the-art model optimization techniques including quantization and sparsity to reduce inference costs for generative AI models. Users can easily stack different optimization techniques to produce quantized checkpoints from torch or ONNX models. The quantized checkpoints are ready for deployment in inference frameworks like TensorRT-LLM or TensorRT, with planned integrations for NVIDIA NeMo and Megatron-LM. The tool also supports 8-bit quantization with Stable Diffusion for enterprise users on NVIDIA NIM. Model Optimizer is available for free on NVIDIA PyPI, and this repository serves as a platform for sharing examples, GPU-optimized recipes, and collecting community feedback.
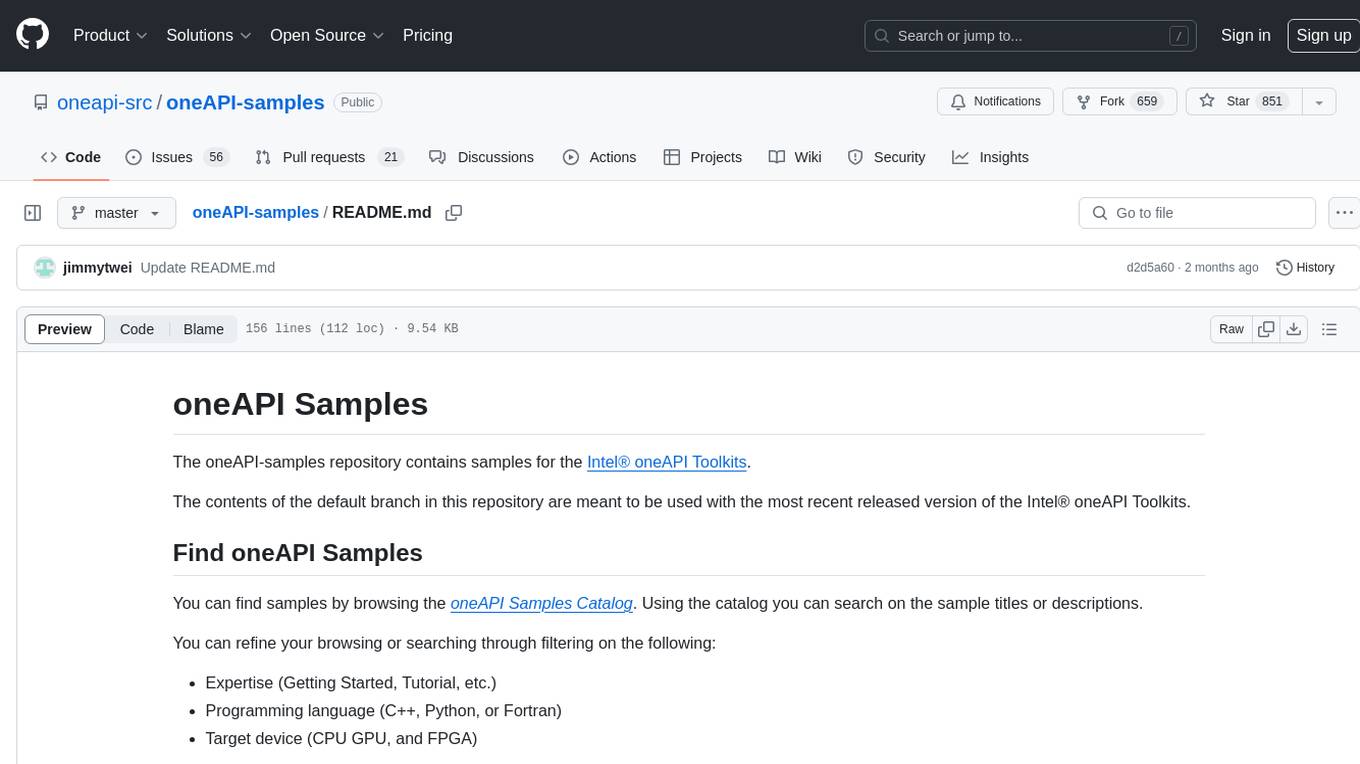
oneAPI-samples
The oneAPI-samples repository contains a collection of samples for the Intel oneAPI Toolkits. These samples cover various topics such as AI and analytics, end-to-end workloads, features and functionality, getting started samples, Jupyter notebooks, direct programming, C++, Fortran, libraries, publications, rendering toolkit, and tools. Users can find samples based on expertise, programming language, and target device. The repository structure is organized by high-level categories, and platform validation includes Ubuntu 22.04, Windows 11, and macOS. The repository provides instructions for getting samples, including cloning the repository or downloading specific tagged versions. Users can also use integrated development environments (IDEs) like Visual Studio Code. The code samples are licensed under the MIT license.
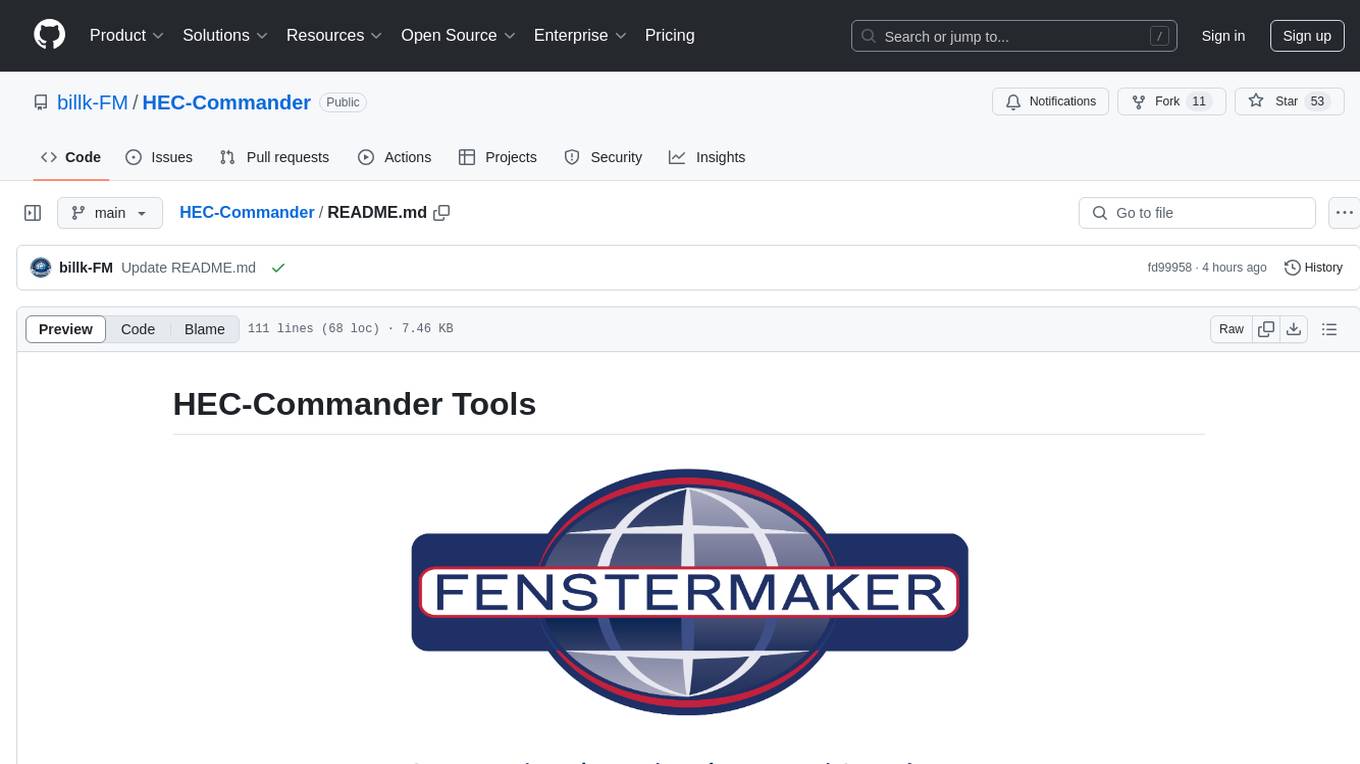
HEC-Commander
HEC-Commander Tools is a suite of python notebooks developed with AI assistance for water resource engineering workflows, providing automation for HEC-RAS and HEC-HMS through Jupyter Notebooks. It contains automation scripts for HEC-HMS, HEC-RAS, and DSS, along with miscellaneous tools. The repository also includes blog posts, ChatGPT assistants, and presentations related to H&H modeling and water resources workflows. Developed to support Region 4 of the Louisiana Watershed Initiative by Fenstermaker.
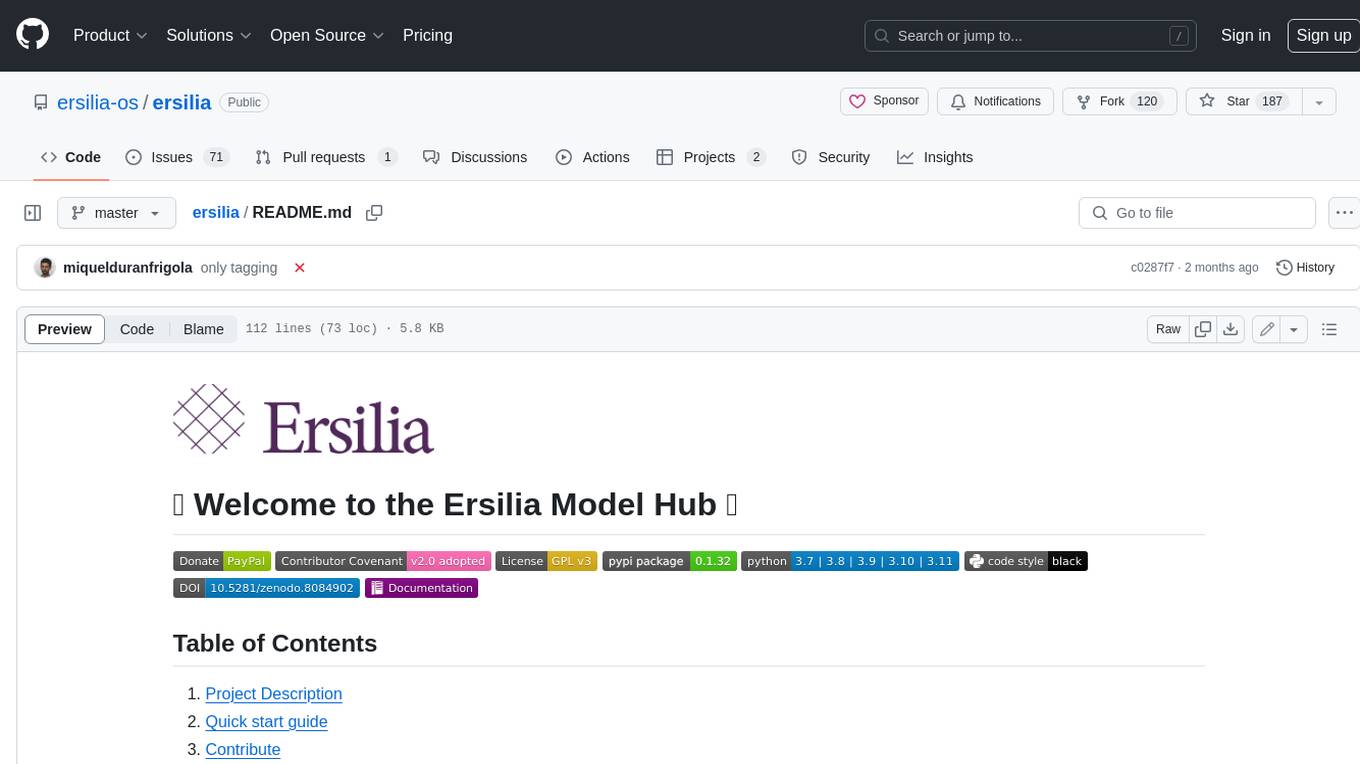
ersilia
The Ersilia Model Hub is a unified platform of pre-trained AI/ML models dedicated to infectious and neglected disease research. It offers an open-source, low-code solution that provides seamless access to AI/ML models for drug discovery. Models housed in the hub come from two sources: published models from literature (with due third-party acknowledgment) and custom models developed by the Ersilia team or contributors.
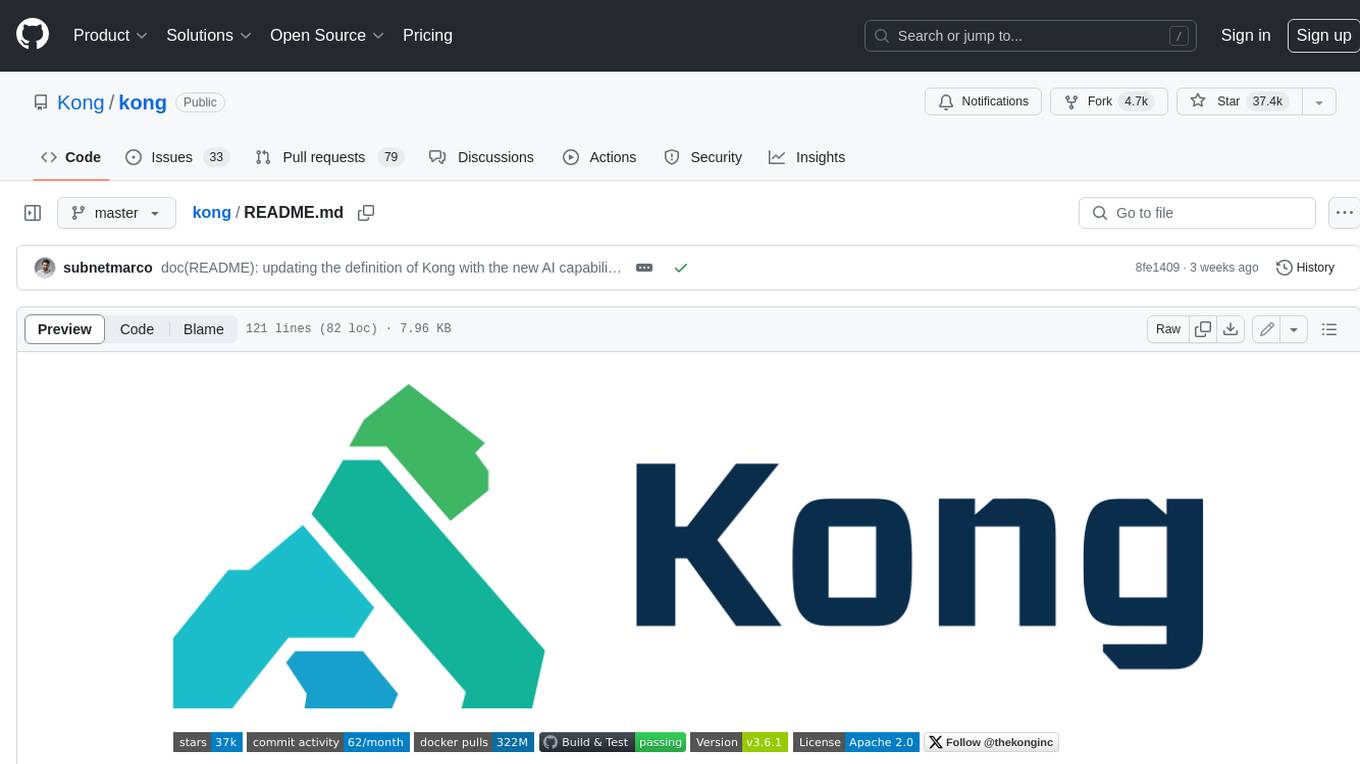
kong
Kong, or Kong API Gateway, is a cloud-native, platform-agnostic, scalable API Gateway distinguished for its high performance and extensibility via plugins. It also provides advanced AI capabilities with multi-LLM support. By providing functionality for proxying, routing, load balancing, health checking, authentication (and more), Kong serves as the central layer for orchestrating microservices or conventional API traffic with ease. Kong runs natively on Kubernetes thanks to its official Kubernetes Ingress Controller.
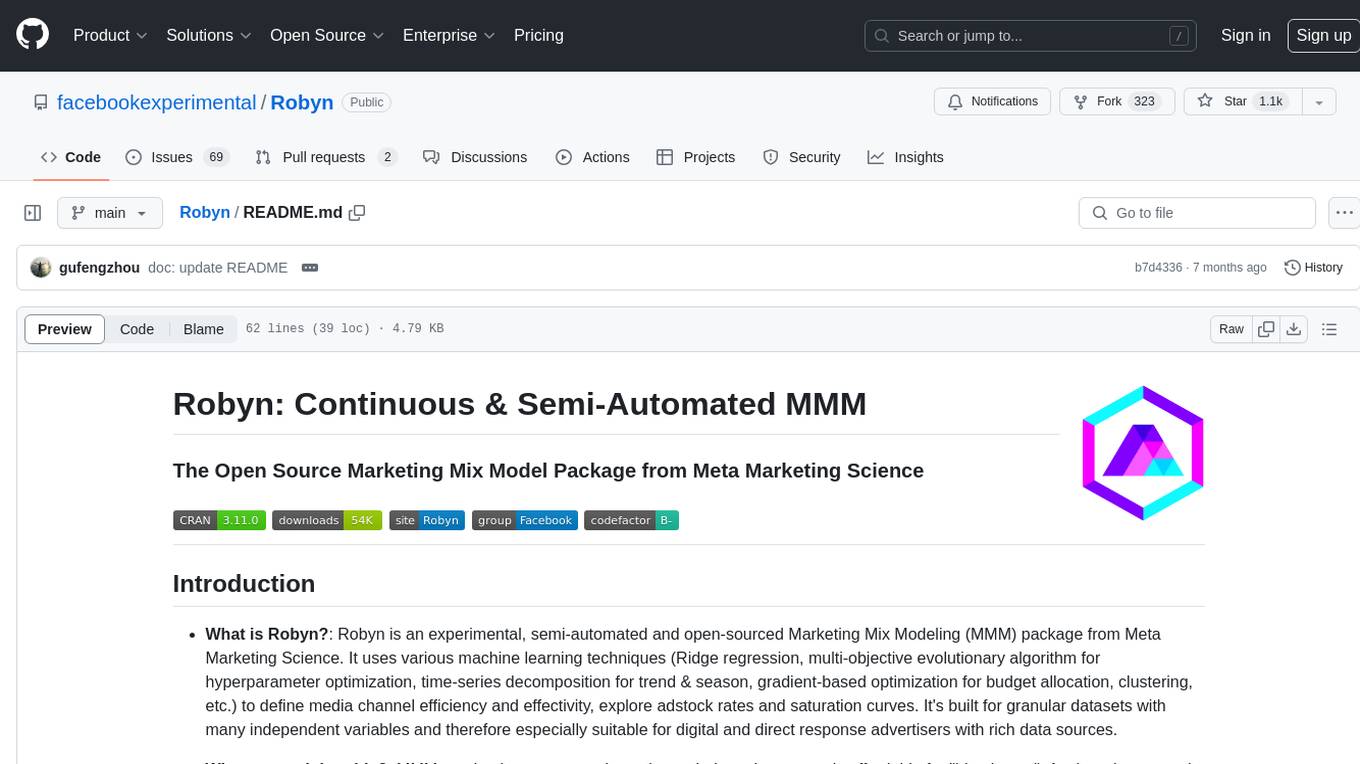
Robyn
Robyn is an experimental, semi-automated and open-sourced Marketing Mix Modeling (MMM) package from Meta Marketing Science. It uses various machine learning techniques to define media channel efficiency and effectivity, explore adstock rates and saturation curves. Built for granular datasets with many independent variables, especially suitable for digital and direct response advertisers with rich data sources. Aiming to democratize MMM, make it accessible for advertisers of all sizes, and contribute to the measurement landscape.
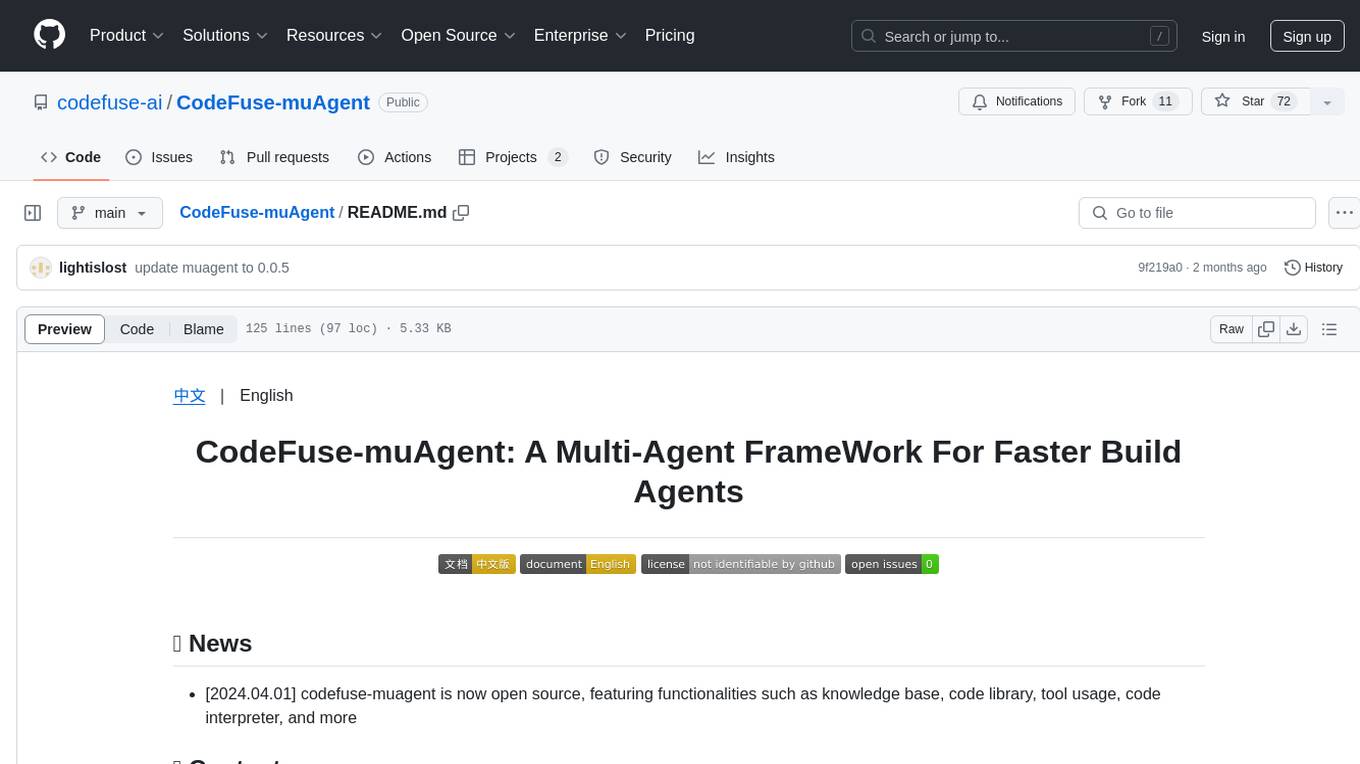
CodeFuse-muAgent
CodeFuse-muAgent is a Multi-Agent framework designed to streamline Standard Operating Procedure (SOP) orchestration for agents. It integrates toolkits, code libraries, knowledge bases, and sandbox environments for rapid construction of complex Multi-Agent interactive applications. The framework enables efficient execution and handling of multi-layered and multi-dimensional tasks.
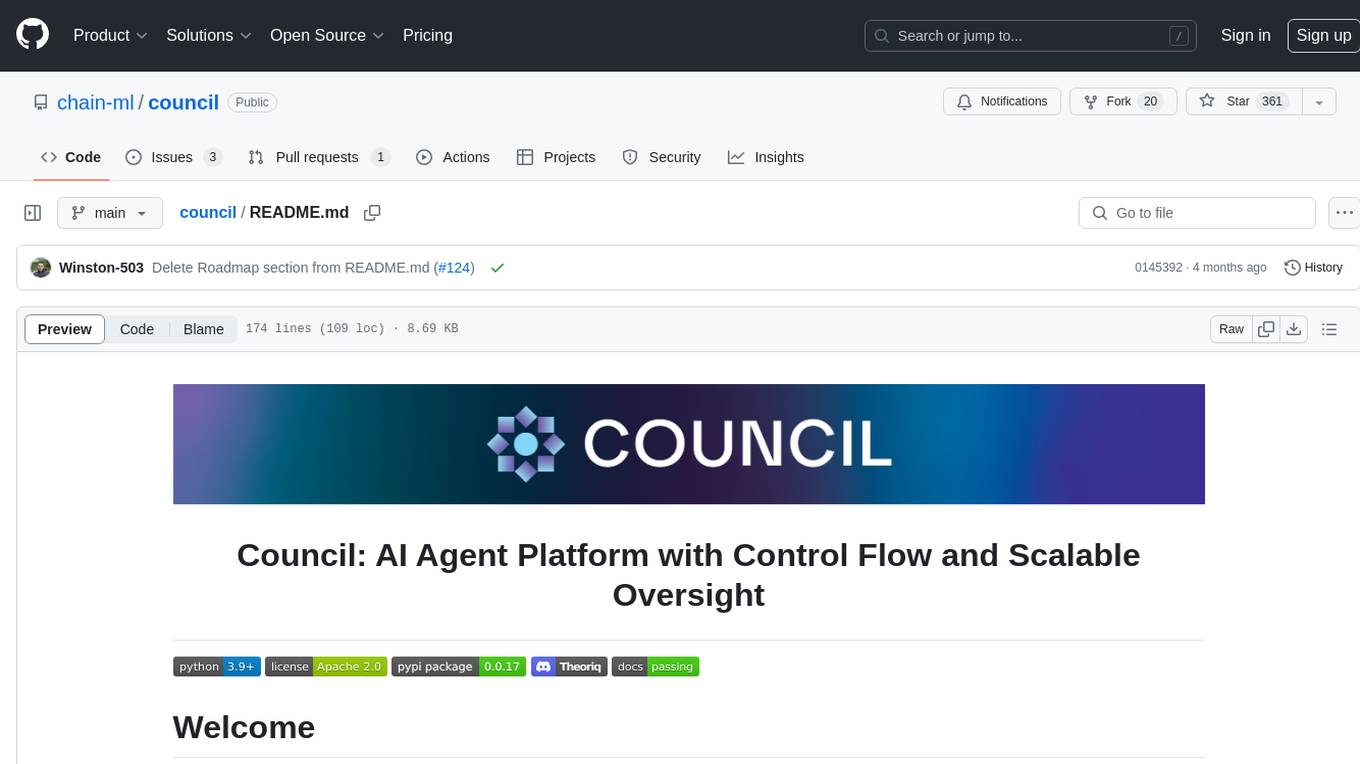
council
Council is an open-source platform designed for the rapid development and deployment of customized generative AI applications using teams of agents. It extends the LLM tool ecosystem by providing advanced control flow and scalable oversight for AI agents. Users can create sophisticated agents with predictable behavior by leveraging Council's powerful approach to control flow using Controllers, Filters, Evaluators, and Budgets. The framework allows for automated routing between agents, comparing, evaluating, and selecting the best results for a task. Council aims to facilitate packaging and deploying agents at scale on multiple platforms while enabling enterprise-grade monitoring and quality control.
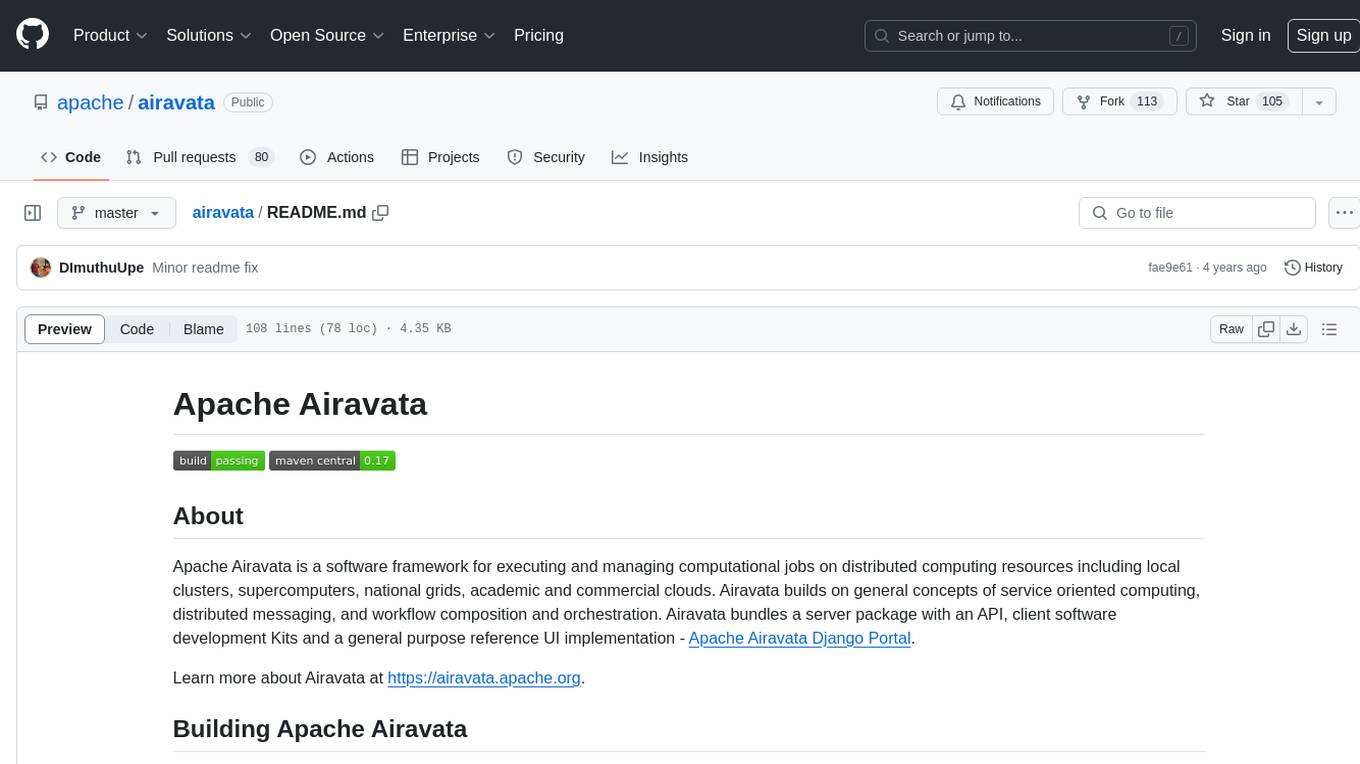
airavata
Apache Airavata is a software framework for executing and managing computational jobs on distributed computing resources. It supports local clusters, supercomputers, national grids, academic and commercial clouds. Airavata utilizes service-oriented computing, distributed messaging, and workflow composition. It includes a server package with an API, client SDKs, and a general-purpose UI implementation called Apache Airavata Django Portal.
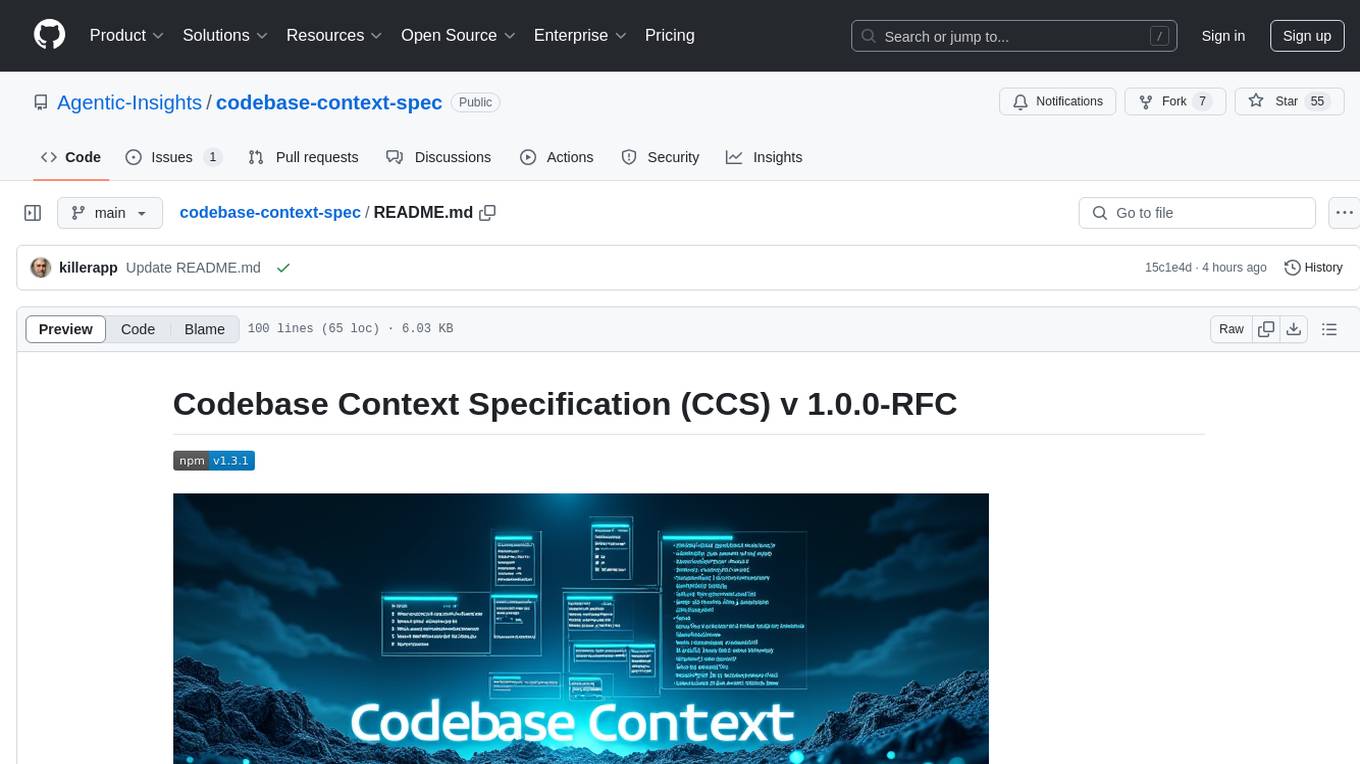
codebase-context-spec
The Codebase Context Specification (CCS) project aims to standardize embedding contextual information within codebases to enhance understanding for both AI and human developers. It introduces a convention similar to `.env` and `.editorconfig` files but focused on documenting code for both AI and humans. By providing structured contextual metadata, collaborative documentation guidelines, and standardized context files, developers can improve code comprehension, collaboration, and development efficiency. The project includes a linter for validating context files and provides guidelines for using the specification with AI assistants. Tooling recommendations suggest creating memory systems, IDE plugins, AI model integrations, and agents for context creation and utilization. Future directions include integration with existing documentation systems, dynamic context generation, and support for explicit context overriding.
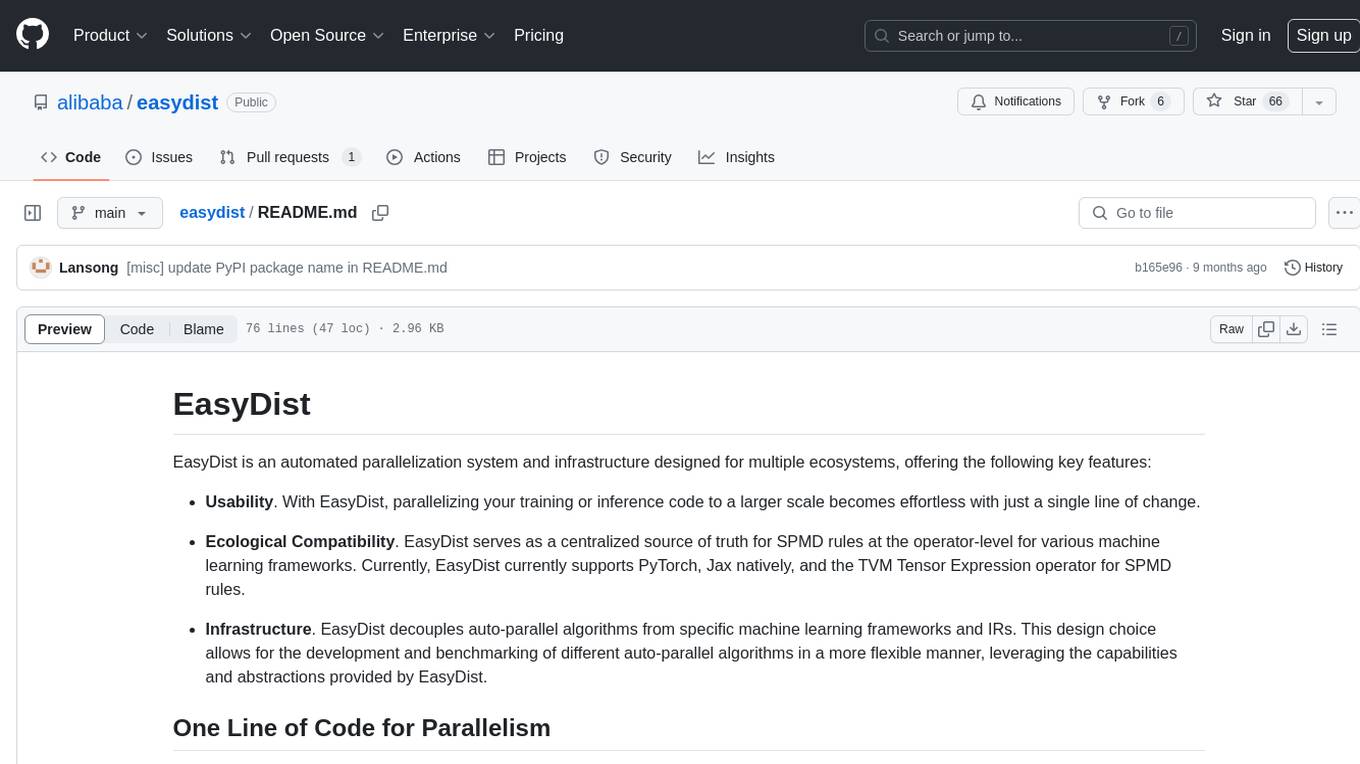
easydist
EasyDist is an automated parallelization system and infrastructure designed for multiple ecosystems. It offers usability by making parallelizing training or inference code effortless with just a single line of change. It ensures ecological compatibility by serving as a centralized source of truth for SPMD rules at the operator-level for various machine learning frameworks. EasyDist decouples auto-parallel algorithms from specific frameworks and IRs, allowing for the development and benchmarking of different auto-parallel algorithms in a flexible manner. The architecture includes MetaOp, MetaIR, and the ShardCombine Algorithm for SPMD sharding rules without manual annotations.
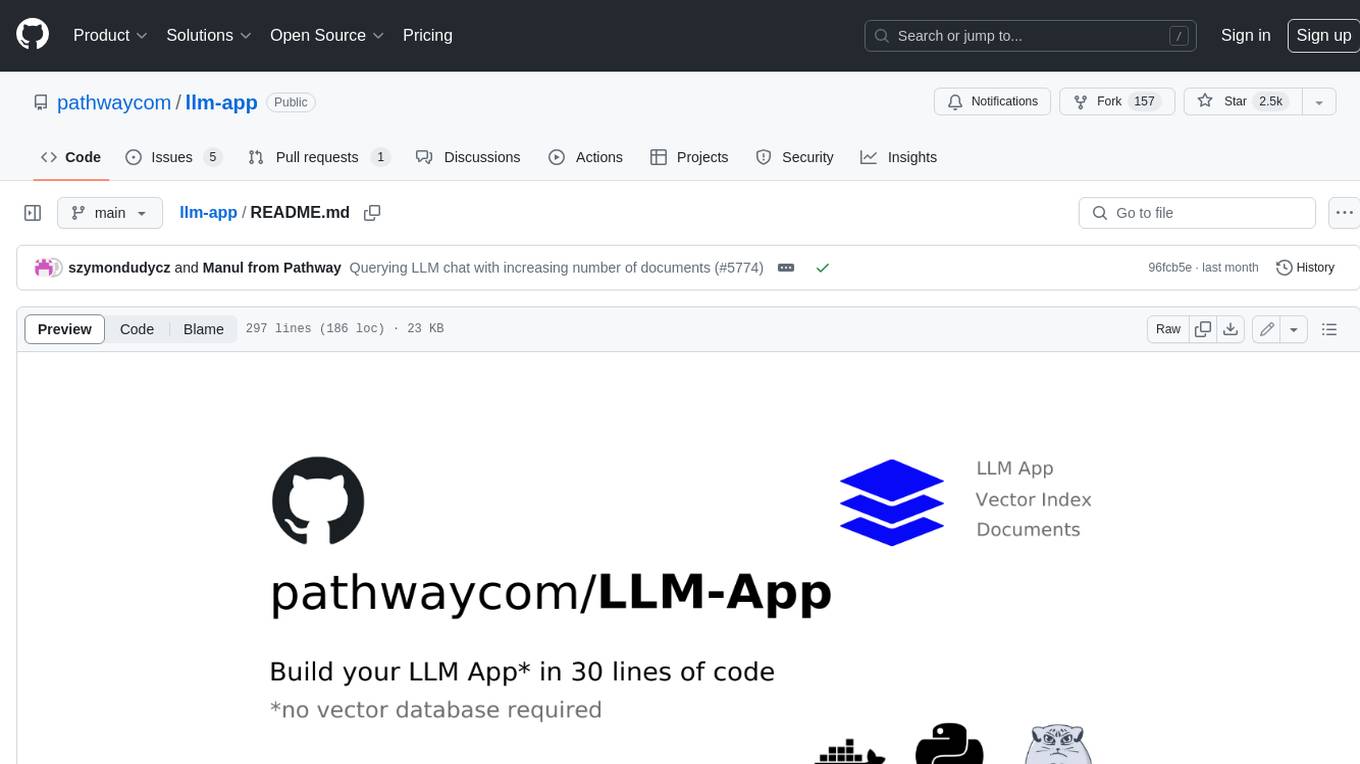
llm-app
Pathway's LLM (Large Language Model) Apps provide a platform to quickly deploy AI applications using the latest knowledge from data sources. The Python application examples in this repository are Docker-ready, exposing an HTTP API to the frontend. These apps utilize the Pathway framework for data synchronization, API serving, and low-latency data processing without the need for additional infrastructure dependencies. They connect to document data sources like S3, Google Drive, and Sharepoint, offering features like real-time data syncing, easy alert setup, scalability, monitoring, security, and unification of application logic.
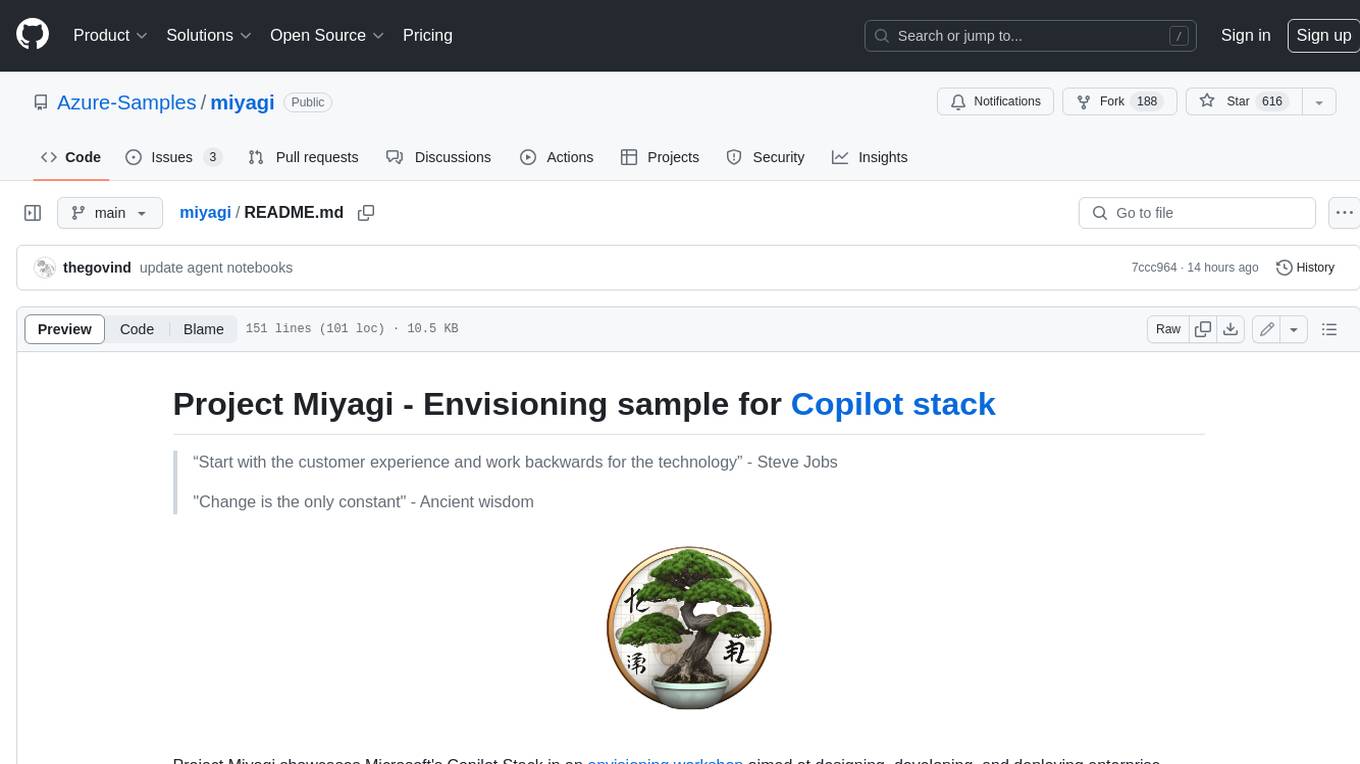
miyagi
Project Miyagi showcases Microsoft's Copilot Stack in an envisioning workshop aimed at designing, developing, and deploying enterprise-grade intelligent apps. By exploring both generative and traditional ML use cases, Miyagi offers an experiential approach to developing AI-infused product experiences that enhance productivity and enable hyper-personalization. Additionally, the workshop introduces traditional software engineers to emerging design patterns in prompt engineering, such as chain-of-thought and retrieval-augmentation, as well as to techniques like vectorization for long-term memory, fine-tuning of OSS models, agent-like orchestration, and plugins or tools for augmenting and grounding LLMs.
For similar tasks
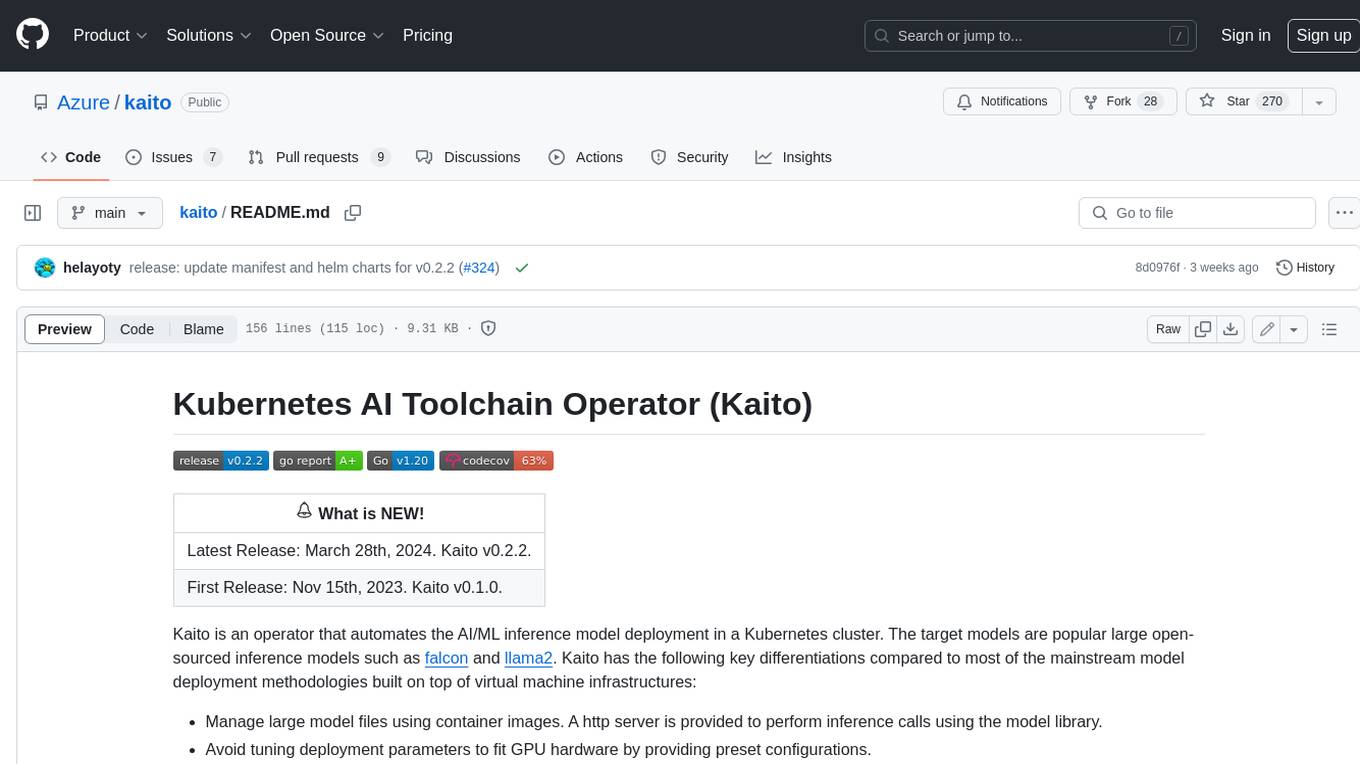
kaito
Kaito is an operator that automates the AI/ML inference model deployment in a Kubernetes cluster. It manages large model files using container images, avoids tuning deployment parameters to fit GPU hardware by providing preset configurations, auto-provisions GPU nodes based on model requirements, and hosts large model images in the public Microsoft Container Registry (MCR) if the license allows. Using Kaito, the workflow of onboarding large AI inference models in Kubernetes is largely simplified.
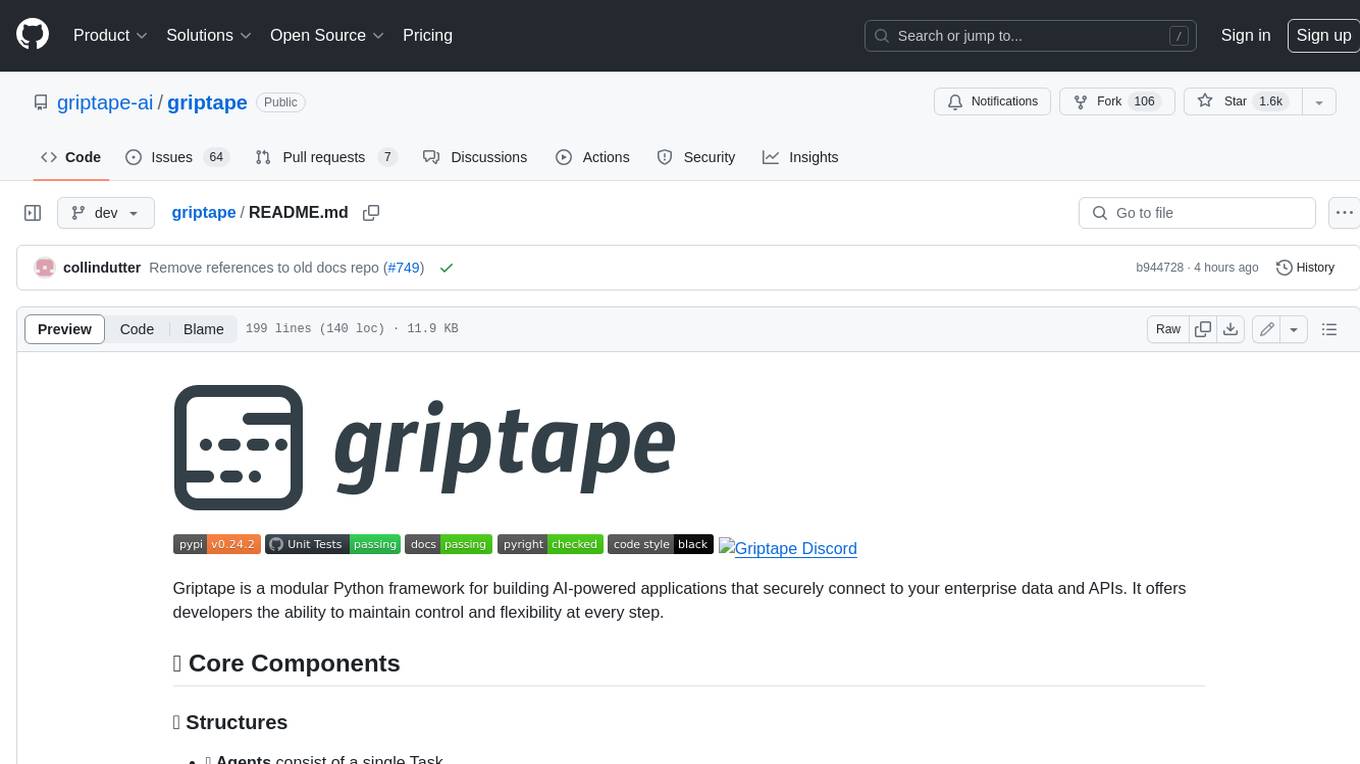
griptape
Griptape is a modular Python framework for building AI-powered applications that securely connect to your enterprise data and APIs. It offers developers the ability to maintain control and flexibility at every step. Griptape's core components include Structures (Agents, Pipelines, and Workflows), Tasks, Tools, Memory (Conversation Memory, Task Memory, and Meta Memory), Drivers (Prompt and Embedding Drivers, Vector Store Drivers, Image Generation Drivers, Image Query Drivers, SQL Drivers, Web Scraper Drivers, and Conversation Memory Drivers), Engines (Query Engines, Extraction Engines, Summary Engines, Image Generation Engines, and Image Query Engines), and additional components (Rulesets, Loaders, Artifacts, Chunkers, and Tokenizers). Griptape enables developers to create AI-powered applications with ease and efficiency.
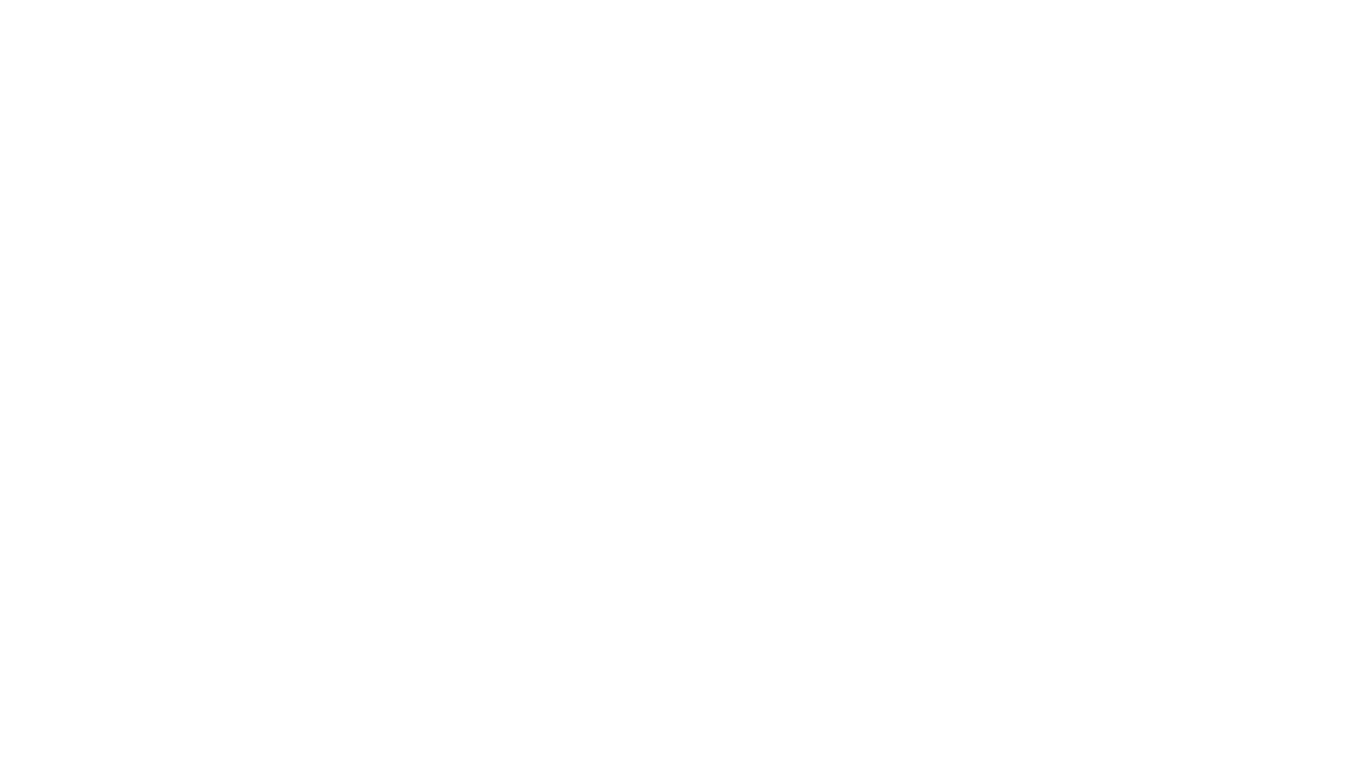
zep-python
Zep is an open-source platform for building and deploying large language model (LLM) applications. It provides a suite of tools and services that make it easy to integrate LLMs into your applications, including chat history memory, embedding, vector search, and data enrichment. Zep is designed to be scalable, reliable, and easy to use, making it a great choice for developers who want to build LLM-powered applications quickly and easily.
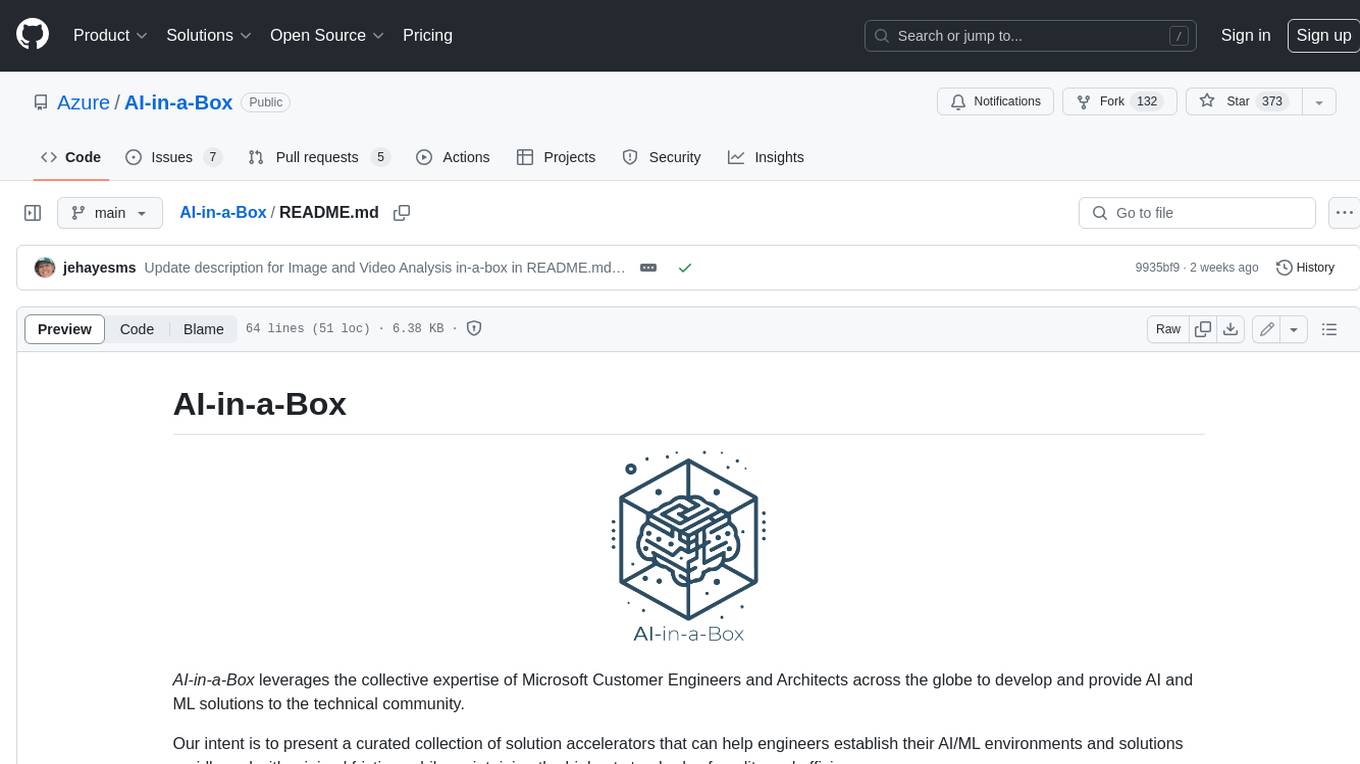
AI-in-a-Box
AI-in-a-Box is a curated collection of solution accelerators that can help engineers establish their AI/ML environments and solutions rapidly and with minimal friction, while maintaining the highest standards of quality and efficiency. It provides essential guidance on the responsible use of AI and LLM technologies, specific security guidance for Generative AI (GenAI) applications, and best practices for scaling OpenAI applications within Azure. The available accelerators include: Azure ML Operationalization in-a-box, Edge AI in-a-box, Doc Intelligence in-a-box, Image and Video Analysis in-a-box, Cognitive Services Landing Zone in-a-box, Semantic Kernel Bot in-a-box, NLP to SQL in-a-box, Assistants API in-a-box, and Assistants API Bot in-a-box.
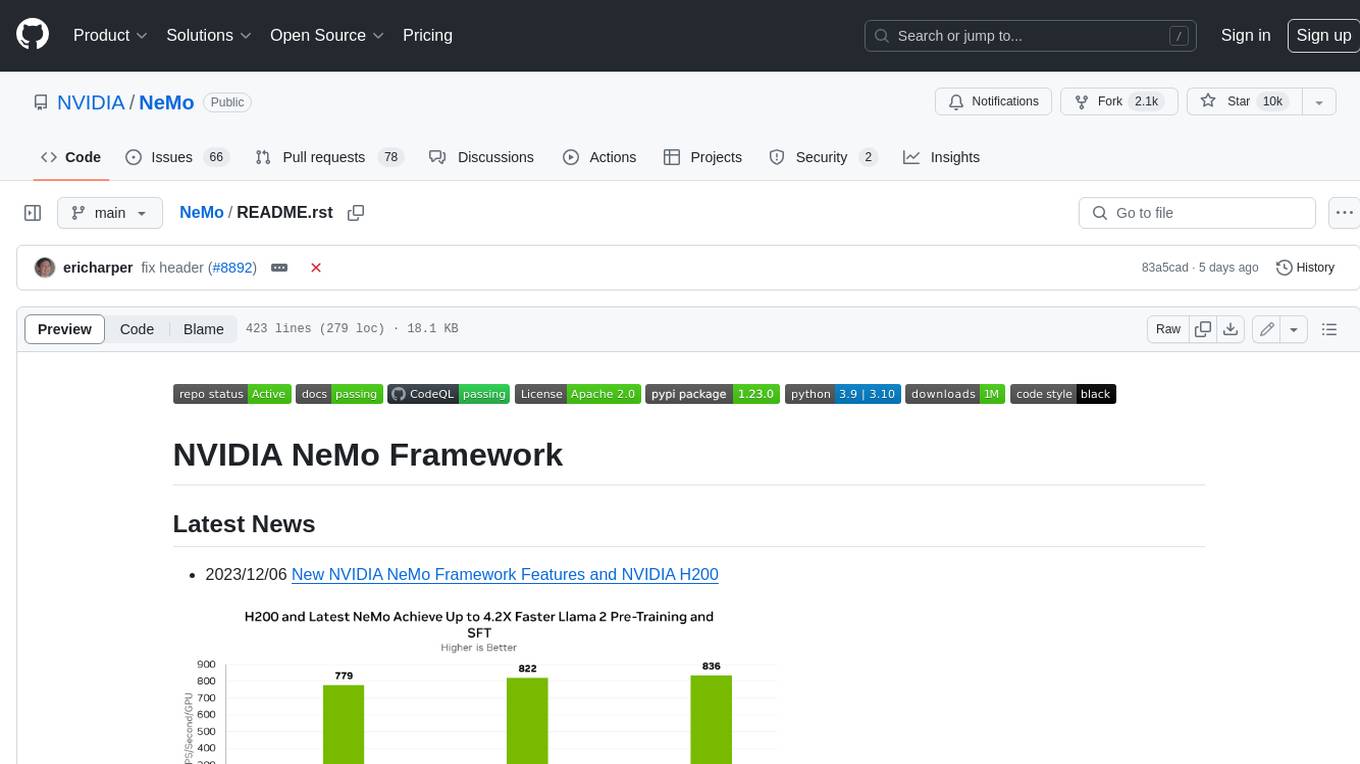
NeMo
NeMo Framework is a generative AI framework built for researchers and pytorch developers working on large language models (LLMs), multimodal models (MM), automatic speech recognition (ASR), and text-to-speech synthesis (TTS). The primary objective of NeMo is to provide a scalable framework for researchers and developers from industry and academia to more easily implement and design new generative AI models by being able to leverage existing code and pretrained models.
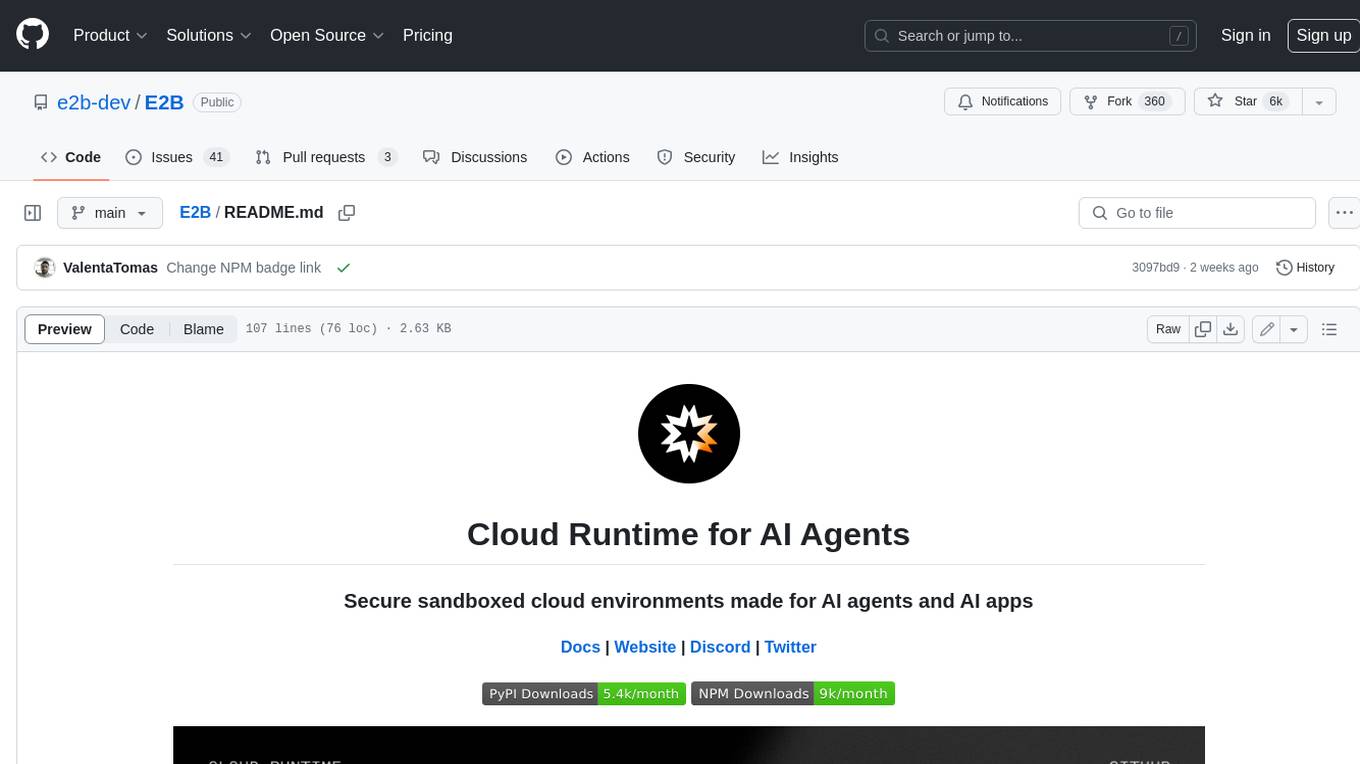
E2B
E2B Sandbox is a secure sandboxed cloud environment made for AI agents and AI apps. Sandboxes allow AI agents and apps to have long running cloud secure environments. In these environments, large language models can use the same tools as humans do. For example: * Cloud browsers * GitHub repositories and CLIs * Coding tools like linters, autocomplete, "go-to defintion" * Running LLM generated code * Audio & video editing The E2B sandbox can be connected to any LLM and any AI agent or app.
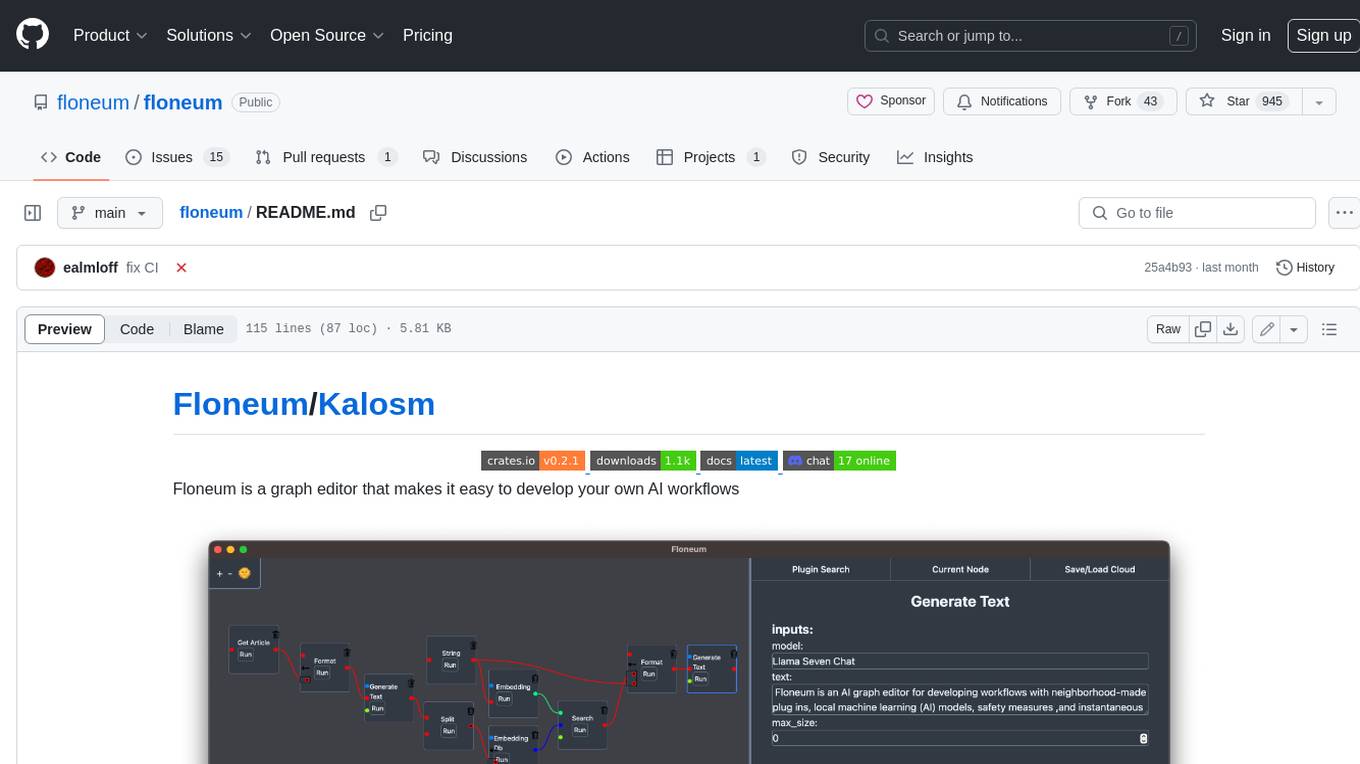
floneum
Floneum is a graph editor that makes it easy to develop your own AI workflows. It uses large language models (LLMs) to run AI models locally, without any external dependencies or even a GPU. This makes it easy to use LLMs with your own data, without worrying about privacy. Floneum also has a plugin system that allows you to improve the performance of LLMs and make them work better for your specific use case. Plugins can be used in any language that supports web assembly, and they can control the output of LLMs with a process similar to JSONformer or guidance.
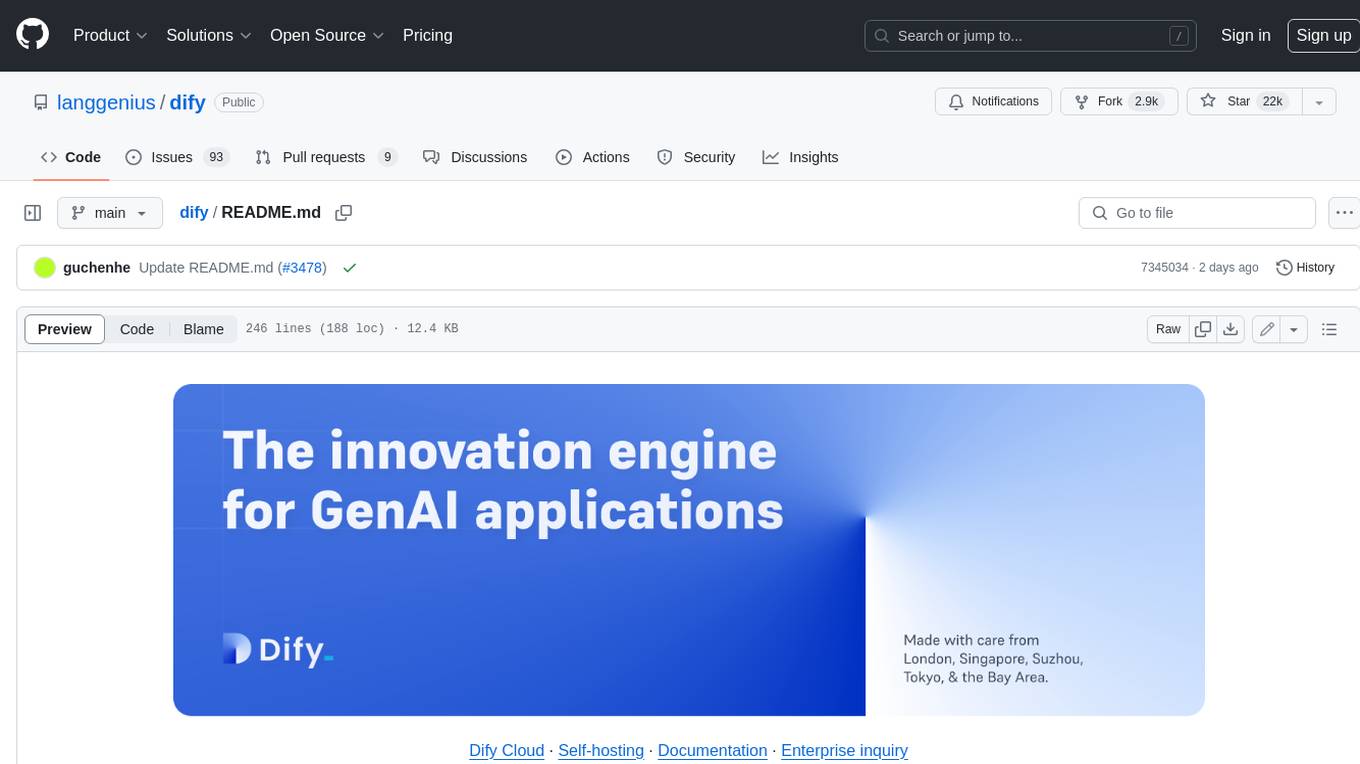
dify
Dify is an open-source LLM app development platform that combines AI workflow, RAG pipeline, agent capabilities, model management, observability features, and more. It allows users to quickly go from prototype to production. Key features include: 1. Workflow: Build and test powerful AI workflows on a visual canvas. 2. Comprehensive model support: Seamless integration with hundreds of proprietary / open-source LLMs from dozens of inference providers and self-hosted solutions. 3. Prompt IDE: Intuitive interface for crafting prompts, comparing model performance, and adding additional features. 4. RAG Pipeline: Extensive RAG capabilities that cover everything from document ingestion to retrieval. 5. Agent capabilities: Define agents based on LLM Function Calling or ReAct, and add pre-built or custom tools. 6. LLMOps: Monitor and analyze application logs and performance over time. 7. Backend-as-a-Service: All of Dify's offerings come with corresponding APIs for easy integration into your own business logic.
For similar jobs
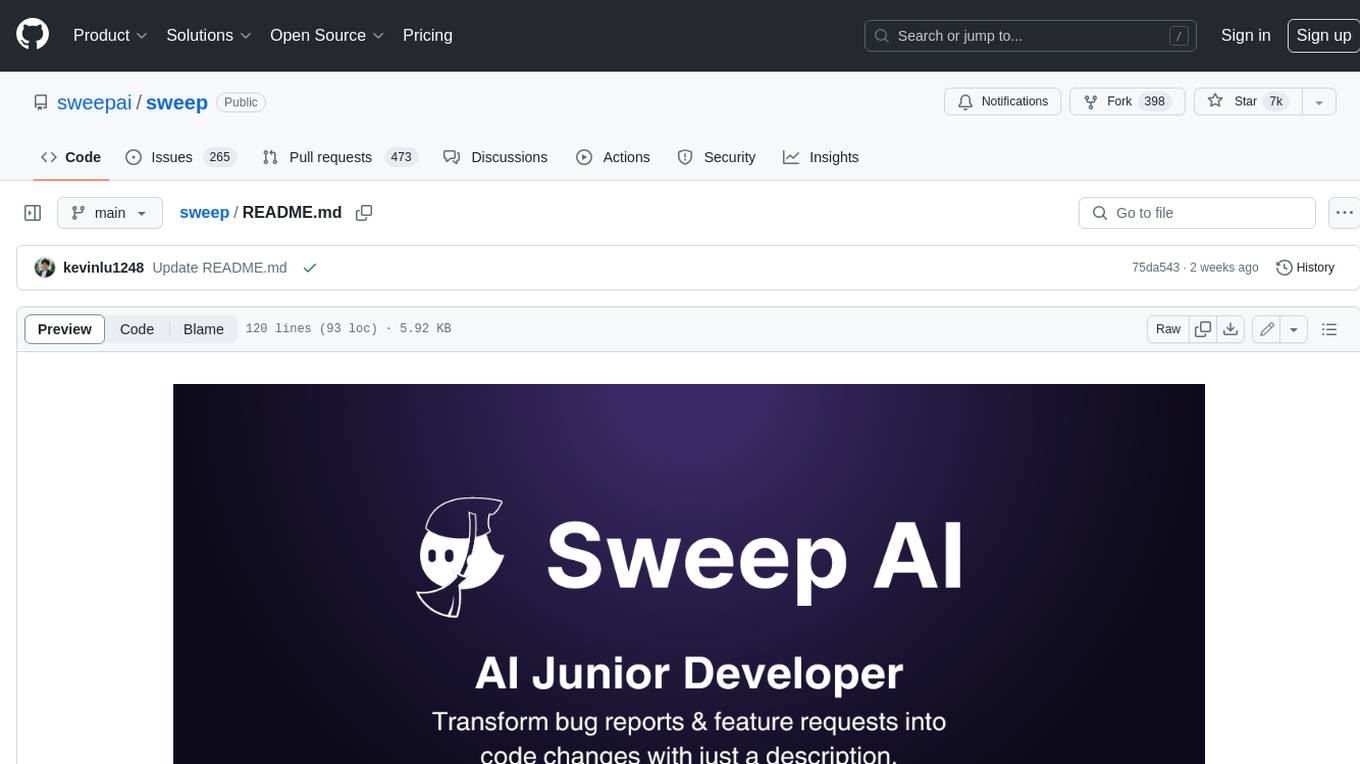
sweep
Sweep is an AI junior developer that turns bugs and feature requests into code changes. It automatically handles developer experience improvements like adding type hints and improving test coverage.
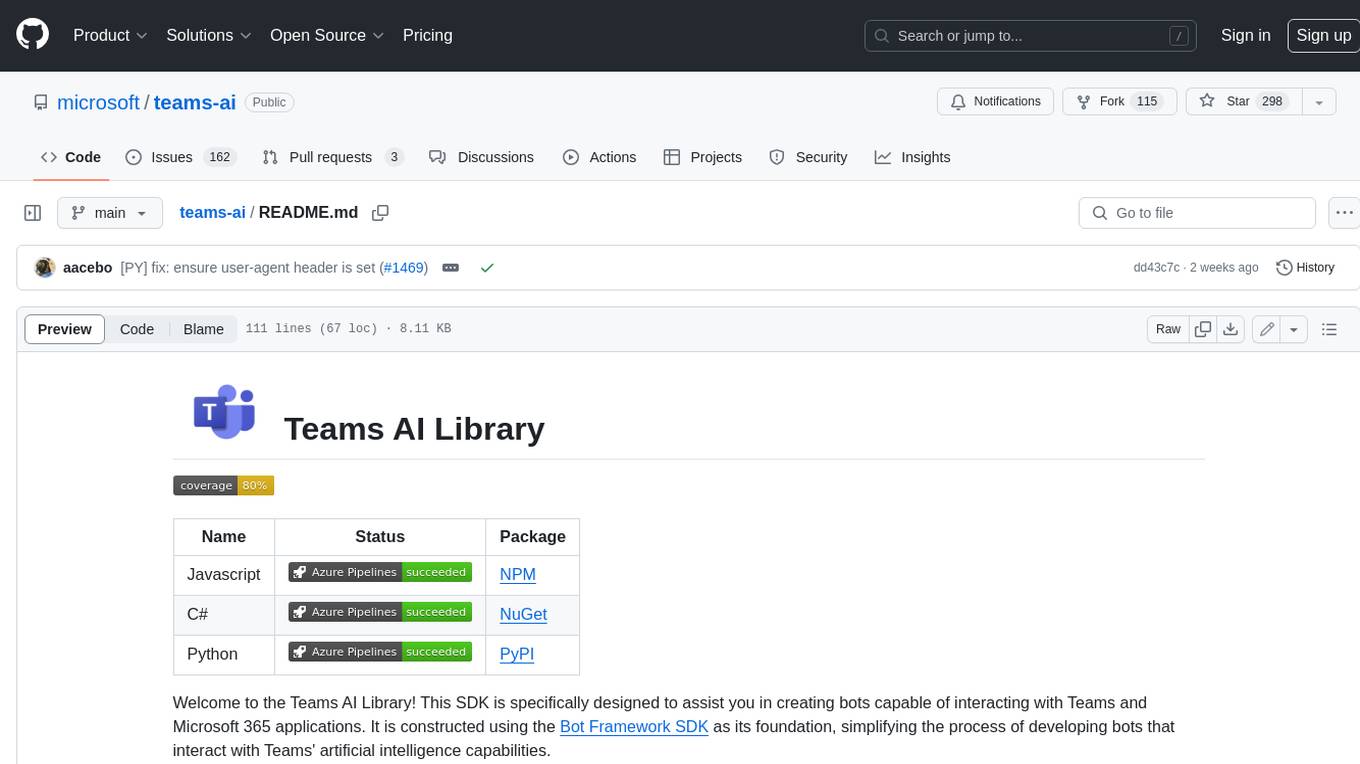
teams-ai
The Teams AI Library is a software development kit (SDK) that helps developers create bots that can interact with Teams and Microsoft 365 applications. It is built on top of the Bot Framework SDK and simplifies the process of developing bots that interact with Teams' artificial intelligence capabilities. The SDK is available for JavaScript/TypeScript, .NET, and Python.
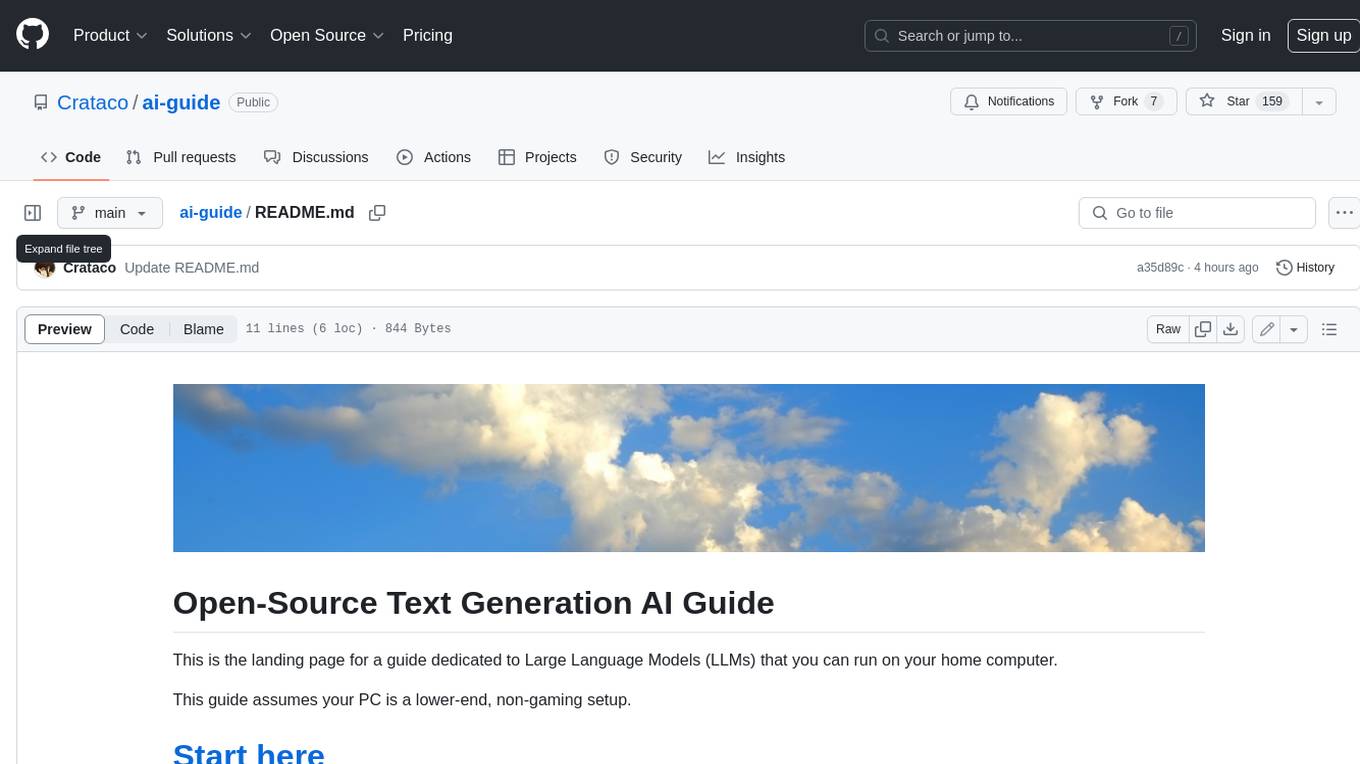
ai-guide
This guide is dedicated to Large Language Models (LLMs) that you can run on your home computer. It assumes your PC is a lower-end, non-gaming setup.
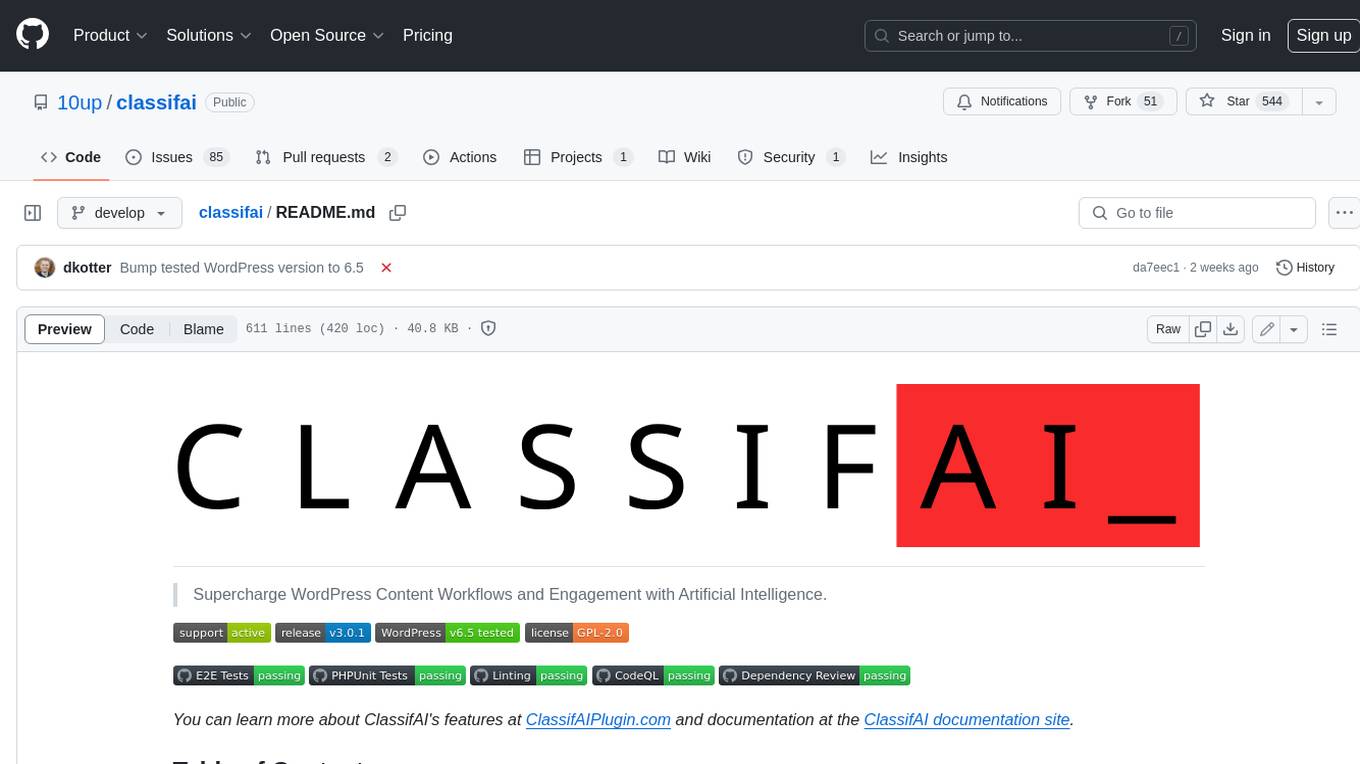
classifai
Supercharge WordPress Content Workflows and Engagement with Artificial Intelligence. Tap into leading cloud-based services like OpenAI, Microsoft Azure AI, Google Gemini and IBM Watson to augment your WordPress-powered websites. Publish content faster while improving SEO performance and increasing audience engagement. ClassifAI integrates Artificial Intelligence and Machine Learning technologies to lighten your workload and eliminate tedious tasks, giving you more time to create original content that matters.
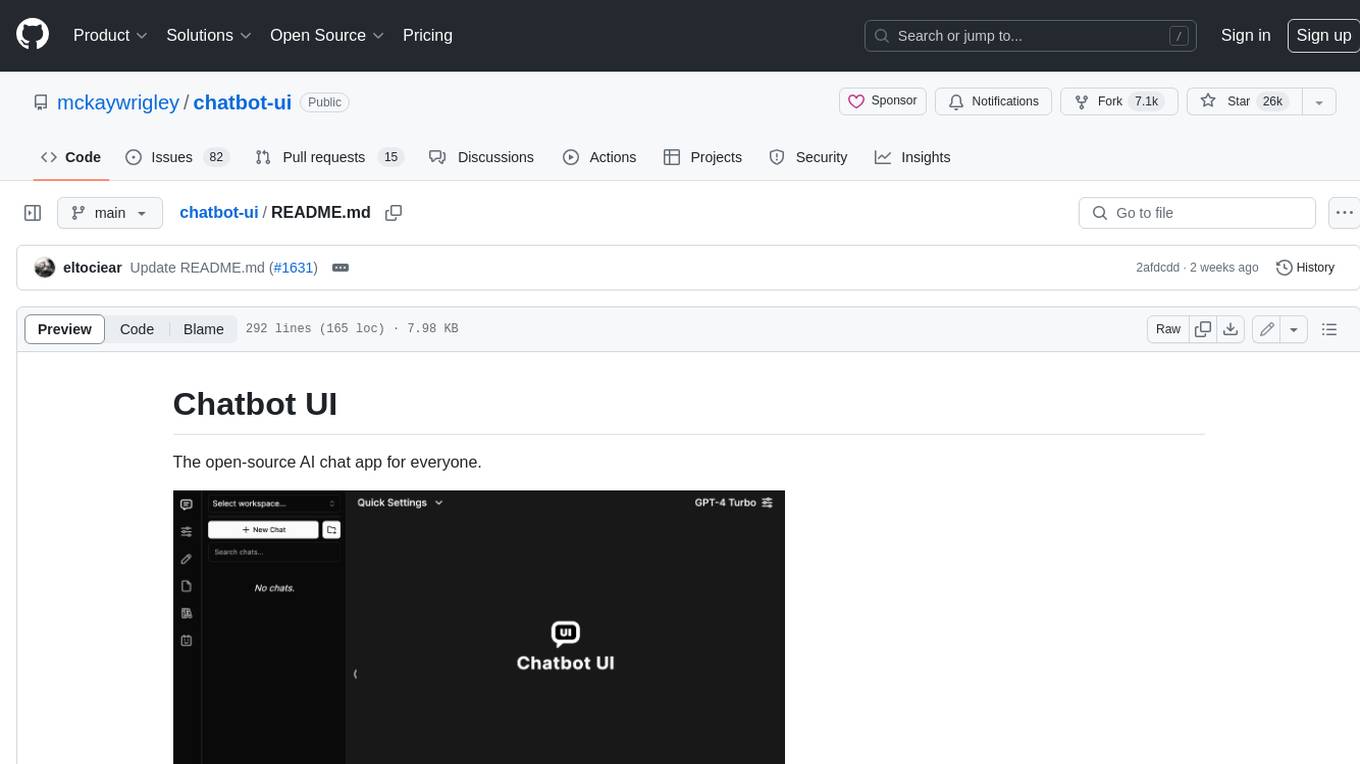
chatbot-ui
Chatbot UI is an open-source AI chat app that allows users to create and deploy their own AI chatbots. It is easy to use and can be customized to fit any need. Chatbot UI is perfect for businesses, developers, and anyone who wants to create a chatbot.
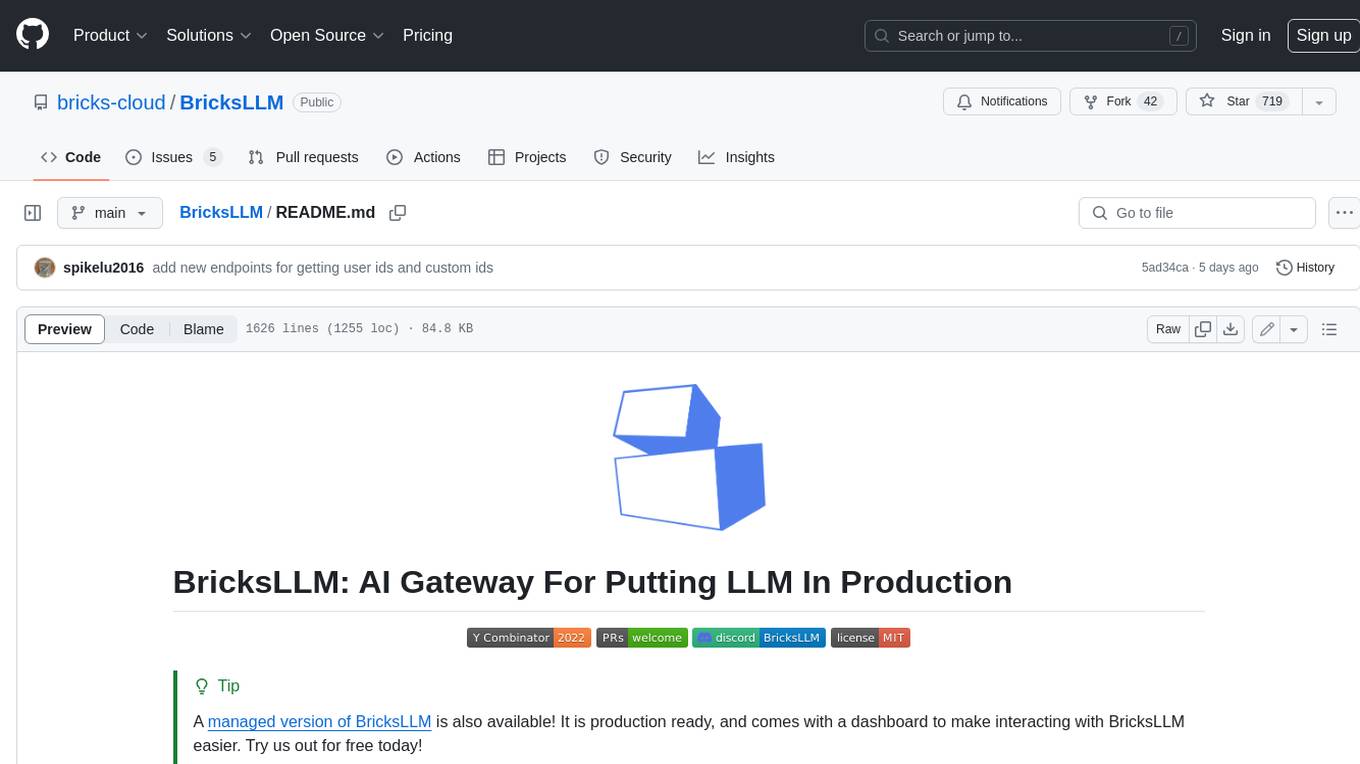
BricksLLM
BricksLLM is a cloud native AI gateway written in Go. Currently, it provides native support for OpenAI, Anthropic, Azure OpenAI and vLLM. BricksLLM aims to provide enterprise level infrastructure that can power any LLM production use cases. Here are some use cases for BricksLLM: * Set LLM usage limits for users on different pricing tiers * Track LLM usage on a per user and per organization basis * Block or redact requests containing PIIs * Improve LLM reliability with failovers, retries and caching * Distribute API keys with rate limits and cost limits for internal development/production use cases * Distribute API keys with rate limits and cost limits for students
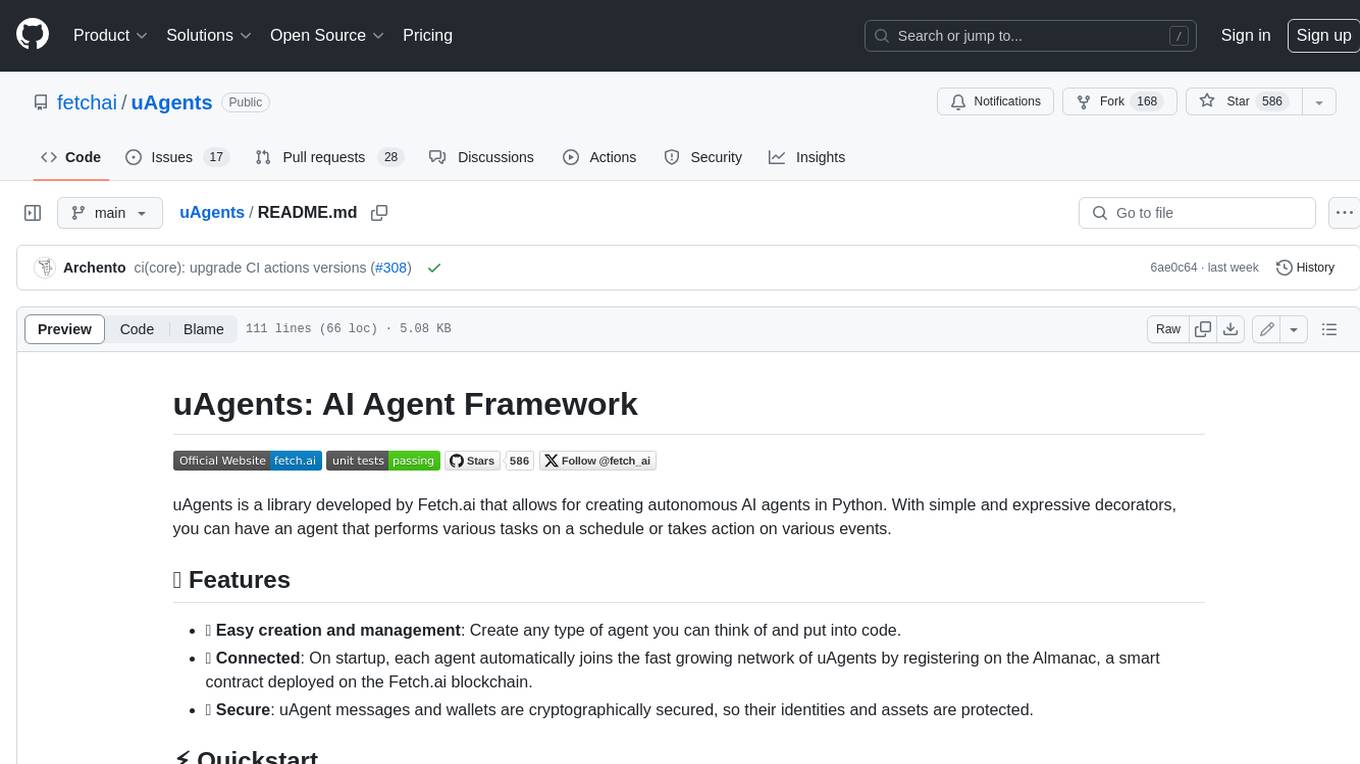
uAgents
uAgents is a Python library developed by Fetch.ai that allows for the creation of autonomous AI agents. These agents can perform various tasks on a schedule or take action on various events. uAgents are easy to create and manage, and they are connected to a fast-growing network of other uAgents. They are also secure, with cryptographically secured messages and wallets.
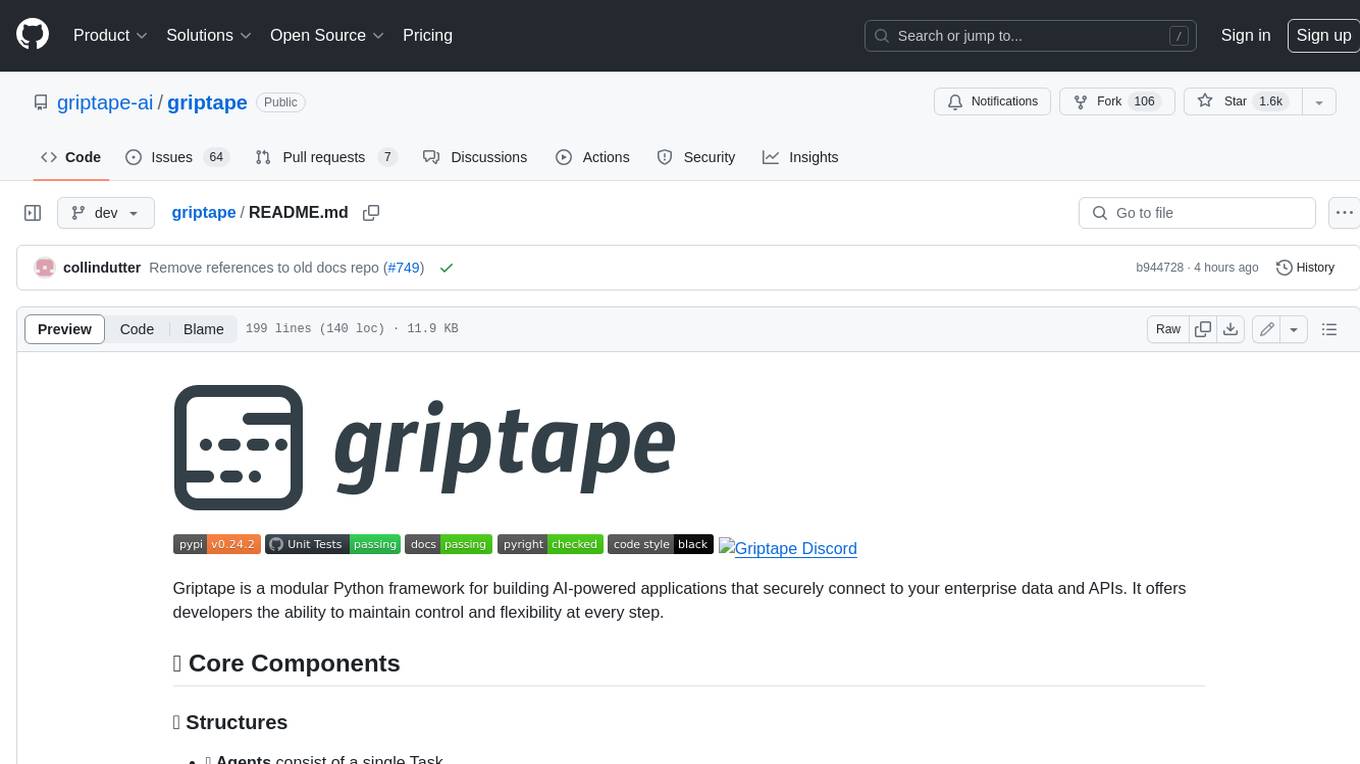
griptape
Griptape is a modular Python framework for building AI-powered applications that securely connect to your enterprise data and APIs. It offers developers the ability to maintain control and flexibility at every step. Griptape's core components include Structures (Agents, Pipelines, and Workflows), Tasks, Tools, Memory (Conversation Memory, Task Memory, and Meta Memory), Drivers (Prompt and Embedding Drivers, Vector Store Drivers, Image Generation Drivers, Image Query Drivers, SQL Drivers, Web Scraper Drivers, and Conversation Memory Drivers), Engines (Query Engines, Extraction Engines, Summary Engines, Image Generation Engines, and Image Query Engines), and additional components (Rulesets, Loaders, Artifacts, Chunkers, and Tokenizers). Griptape enables developers to create AI-powered applications with ease and efficiency.