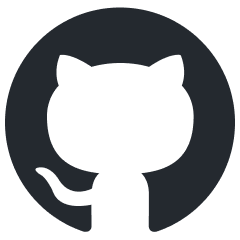
aphrodite-engine
Large-scale LLM inference engine
Stars: 1210
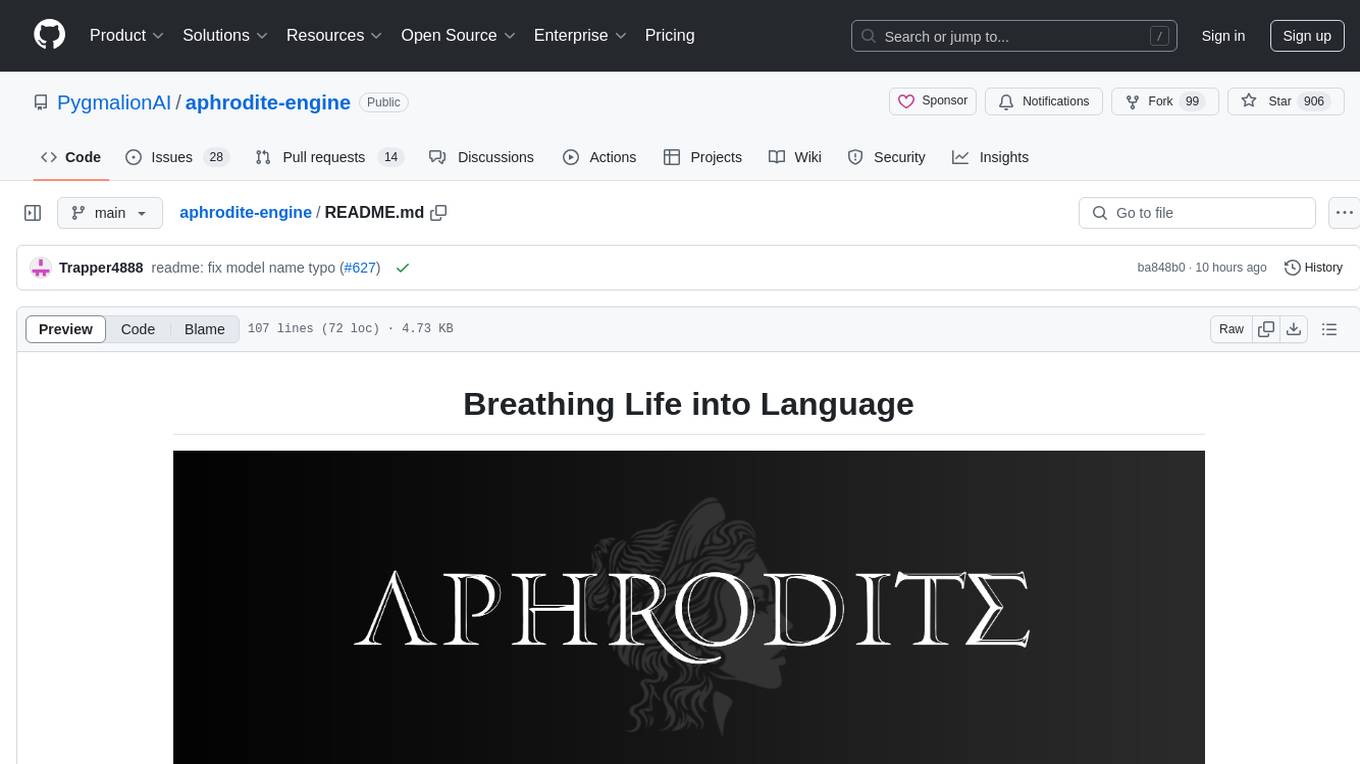
Aphrodite is the official backend engine for PygmalionAI, serving as the inference endpoint for the website. It allows serving Hugging Face-compatible models with fast speeds. Features include continuous batching, efficient K/V management, optimized CUDA kernels, quantization support, distributed inference, and 8-bit KV Cache. The engine requires Linux OS and Python 3.8 to 3.12, with CUDA >= 11 for build requirements. It supports various GPUs, CPUs, TPUs, and Inferentia. Users can limit GPU memory utilization and access full commands via CLI.
README:
Aphrodite is the official backend engine for PygmalionAI. It is designed to serve as the inference endpoint for the PygmalionAI website, and to allow serving Hugging Face-compatible models to a large number of users with blazing fast speeds (thanks to vLLM's Paged Attention).
Aphrodite builds upon and integrates the exceptional work from various projects, primarily vLLM.
Aphrodite is developed in collaboration with Ruliad.
(09/2024) v0.6.1 is here. You can now load FP16 models in FP2 to FP7 quant formats, to achieve extremely high throughput and save on memory.
(09/2024) v0.6.0 is released, with huge throughput improvements, many new quant formats (including fp8 and llm-compressor), asymmetric tensor parallel, pipeline parallel and more! Please check out the exhaustive documentation for the User and Developer guides.
- Continuous Batching
- Efficient K/V management with PagedAttention from vLLM
- Optimized CUDA kernels for improved inference
- Quantization support via AQLM, AWQ, Bitsandbytes, GGUF, GPTQ, QuIP#, Smoothquant+, SqueezeLLM, Marlin, FP2-FP12
- Distributed inference
- 8-bit KV Cache for higher context lengths and throughput, at both FP8 E5M3 and E4M3 formats.
Install the engine:
pip install -U aphrodite-engine
Then launch a model:
aphrodite run meta-llama/Meta-Llama-3.1-8B-Instruct
This will create a OpenAI-compatible API server that can be accessed at port 2242 of the localhost. You can plug in the API into a UI that supports OpenAI, such as SillyTavern.
Please refer to the documentation for the full list of arguments and flags you can pass to the engine.
You can play around with the engine in the demo here:
Additionally, we provide a Docker image for easy deployment. Here's a basic command to get you started:
docker run --runtime nvidia --gpus all \
-v ~/.cache/huggingface:/root/.cache/huggingface \
#--env "CUDA_VISIBLE_DEVICES=0,1,2,3,4,5,6,7" \
-p 2242:2242 \
--ipc=host \
alpindale/aphrodite-openai:latest \
--model NousResearch/Meta-Llama-3.1-8B-Instruct \
--tensor-parallel-size 8 \
--api-keys "sk-empty"
This will pull the Aphrodite Engine image (~8GiB download), and launch the engine with the Llama-3.1-8B-Instruct model at port 2242.
- Operating System: Linux, Windows (Needs building from source)
- Python: 3.8 to 3.12
- CUDA >= 11
For supported devices, see here. Generally speaking, all semi-modern GPUs are supported - down to Pascal (GTX 10xx, P40, etc.) We also support AMD GPUs, Intel CPUs and GPUs, Google TPU, and AWS Inferentia.
-
By design, Aphrodite takes up 90% of your GPU's VRAM. If you're not serving an LLM at scale, you may want to limit the amount of memory it takes up. You can do this in the API example by launching the server with the
--gpu-memory-utilization 0.6
(0.6 means 60%), or--single-user-mode
to only allocate as much memory as needed for a single sequence. -
You can view the full list of commands by running
aphrodite run --help
.
Aphrodite Engine would have not been possible without the phenomenal work of other open-source projects. Credits go to:
- vLLM (CacheFlow)
- TensorRT-LLM
- xFormers
- Flash Attention
- llama.cpp
- AutoAWQ
- AutoGPTQ
- SqueezeLLM
- Exllamav2
- TabbyAPI
- AQLM
- KoboldAI
- Text Generation WebUI
- Megatron-LM
- Ray
Everyone is welcome to contribute. You can support the project by opening Pull Requests for new features, fixes, or general UX improvements.
For Tasks:
Click tags to check more tools for each tasksFor Jobs:
Alternative AI tools for aphrodite-engine
Similar Open Source Tools
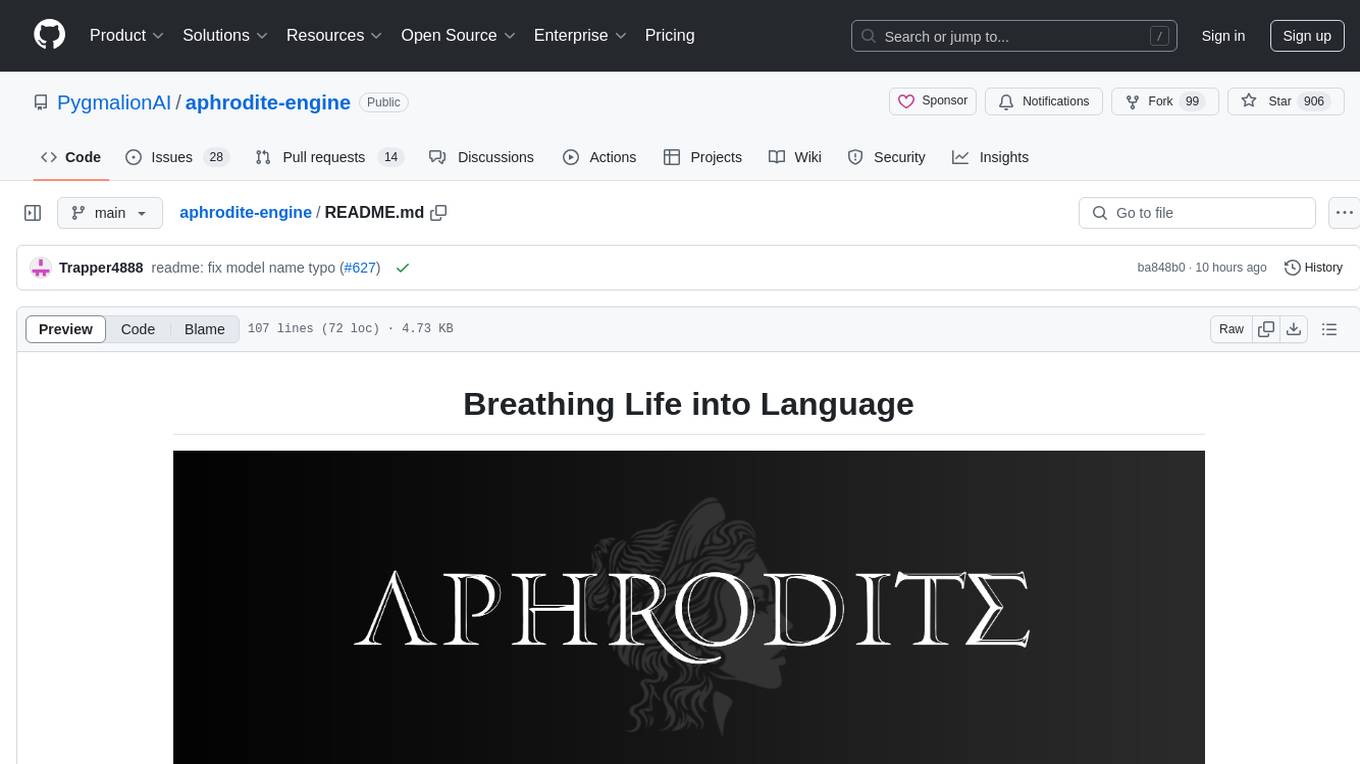
aphrodite-engine
Aphrodite is the official backend engine for PygmalionAI, serving as the inference endpoint for the website. It allows serving Hugging Face-compatible models with fast speeds. Features include continuous batching, efficient K/V management, optimized CUDA kernels, quantization support, distributed inference, and 8-bit KV Cache. The engine requires Linux OS and Python 3.8 to 3.12, with CUDA >= 11 for build requirements. It supports various GPUs, CPUs, TPUs, and Inferentia. Users can limit GPU memory utilization and access full commands via CLI.
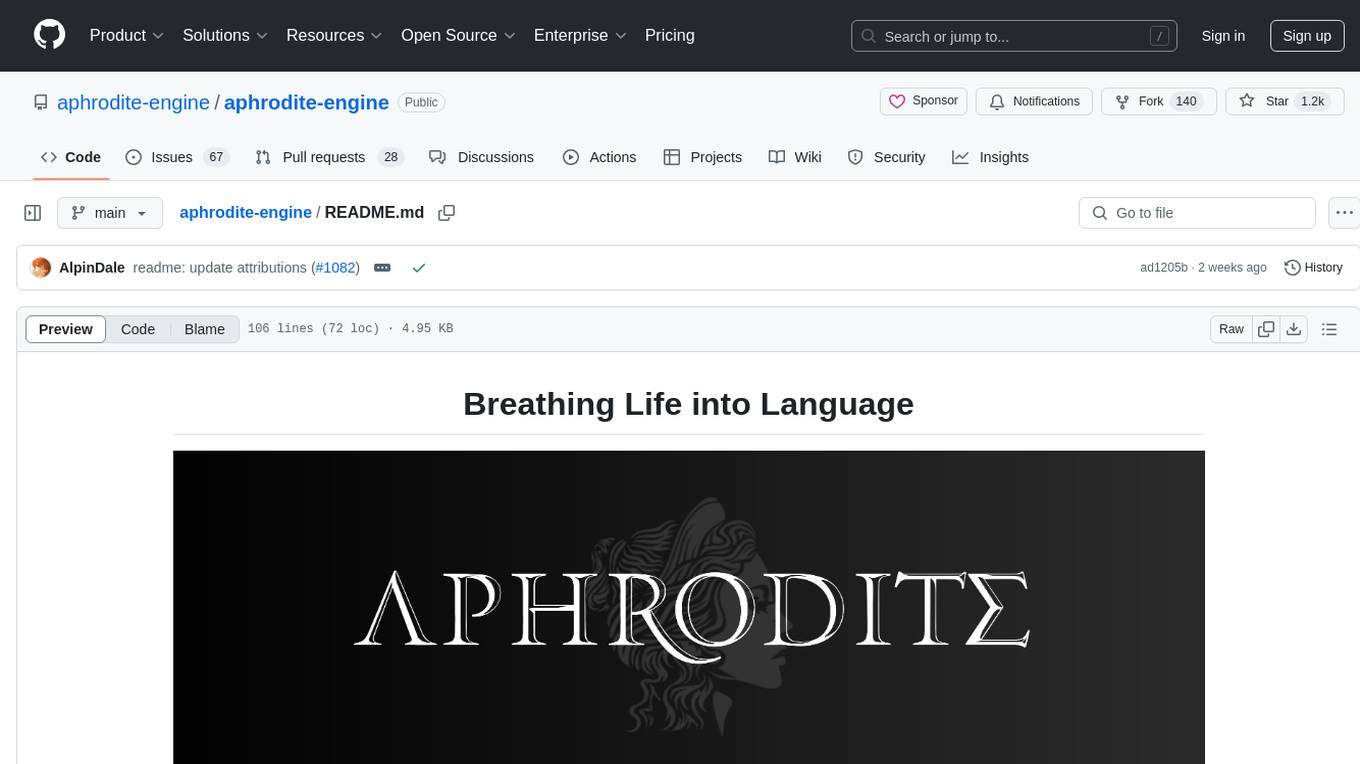
aphrodite-engine
Aphrodite is an inference engine optimized for serving HuggingFace-compatible models at scale. It leverages vLLM's Paged Attention technology to deliver high-performance model inference for multiple concurrent users. The engine supports continuous batching, efficient key/value management, optimized CUDA kernels, quantization support, distributed inference, and modern samplers. It can be easily installed and launched, with Docker support for deployment. Aphrodite requires Linux or Windows OS, Python 3.8 to 3.12, and CUDA >= 11. It is designed to utilize 90% of GPU VRAM but offers options to limit memory usage. Contributors are welcome to enhance the engine.
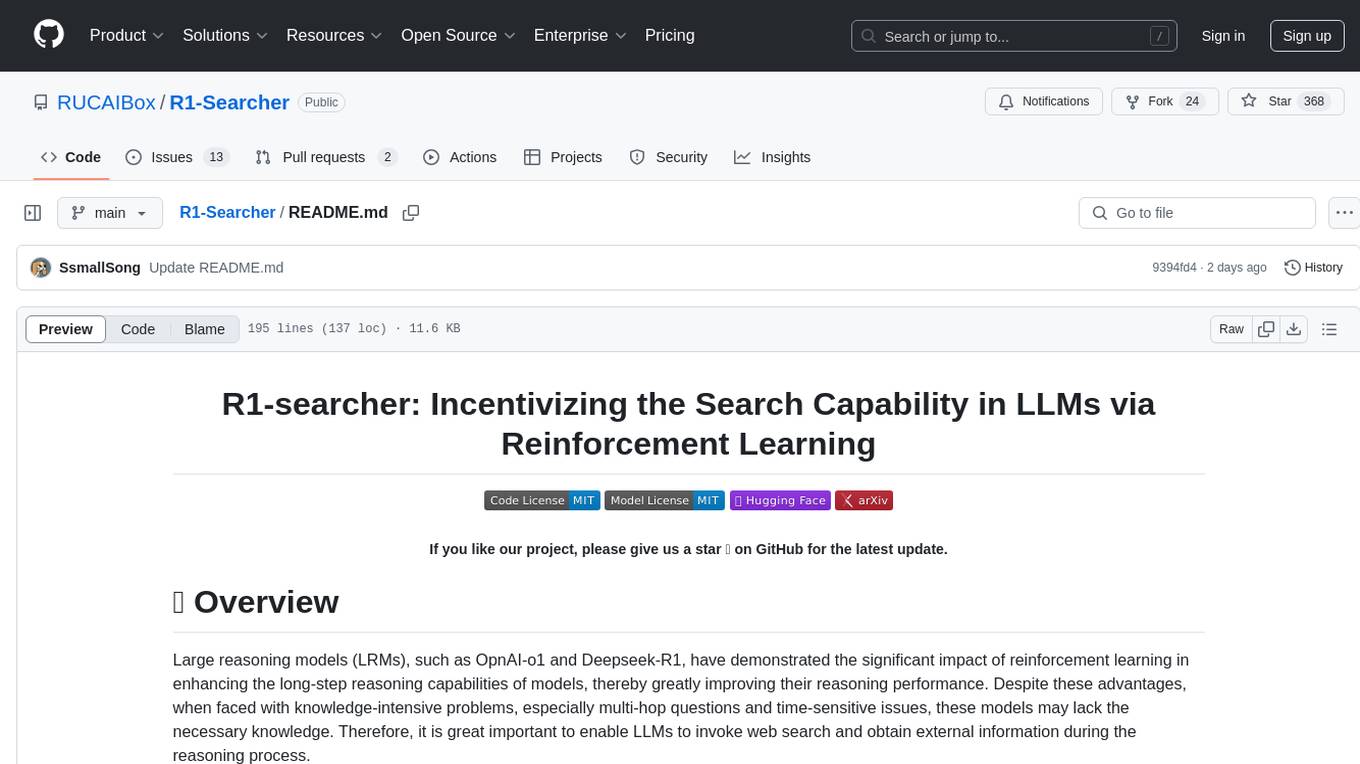
R1-Searcher
R1-searcher is a tool designed to incentivize the search capability in large reasoning models (LRMs) via reinforcement learning. It enables LRMs to invoke web search and obtain external information during the reasoning process by utilizing a two-stage outcome-supervision reinforcement learning approach. The tool does not require instruction fine-tuning for cold start and is compatible with existing Base LLMs or Chat LLMs. It includes training code, inference code, model checkpoints, and a detailed technical report.
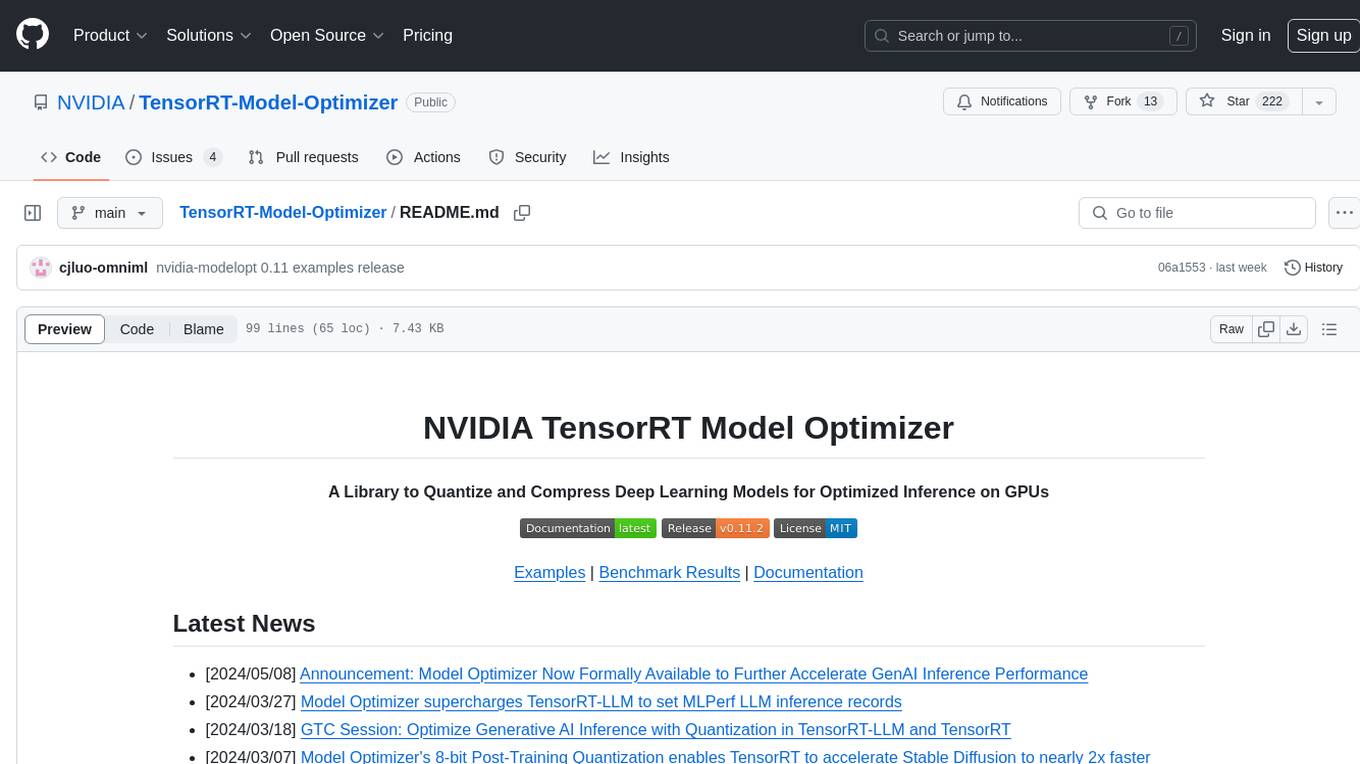
TensorRT-Model-Optimizer
The NVIDIA TensorRT Model Optimizer is a library designed to quantize and compress deep learning models for optimized inference on GPUs. It offers state-of-the-art model optimization techniques including quantization and sparsity to reduce inference costs for generative AI models. Users can easily stack different optimization techniques to produce quantized checkpoints from torch or ONNX models. The quantized checkpoints are ready for deployment in inference frameworks like TensorRT-LLM or TensorRT, with planned integrations for NVIDIA NeMo and Megatron-LM. The tool also supports 8-bit quantization with Stable Diffusion for enterprise users on NVIDIA NIM. Model Optimizer is available for free on NVIDIA PyPI, and this repository serves as a platform for sharing examples, GPU-optimized recipes, and collecting community feedback.
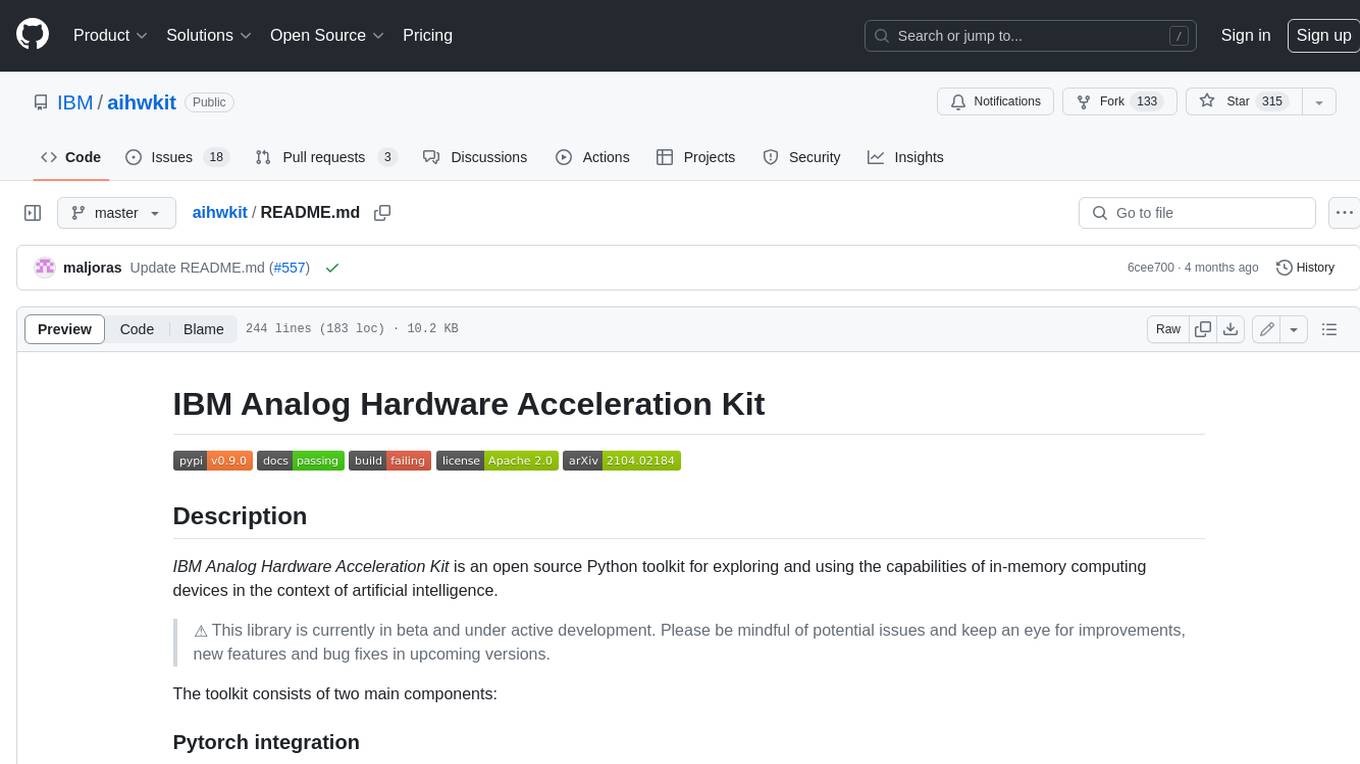
aihwkit
The IBM Analog Hardware Acceleration Kit is an open-source Python toolkit for exploring and using the capabilities of in-memory computing devices in the context of artificial intelligence. It consists of two main components: Pytorch integration and Analog devices simulator. The Pytorch integration provides a series of primitives and features that allow using the toolkit within PyTorch, including analog neural network modules, analog training using torch training workflow, and analog inference using torch inference workflow. The Analog devices simulator is a high-performant (CUDA-capable) C++ simulator that allows for simulating a wide range of analog devices and crossbar configurations by using abstract functional models of material characteristics with adjustable parameters. Along with the two main components, the toolkit includes other functionalities such as a library of device presets, a module for executing high-level use cases, a utility to automatically convert a downloaded model to its equivalent Analog model, and integration with the AIHW Composer platform. The toolkit is currently in beta and under active development, and users are advised to be mindful of potential issues and keep an eye for improvements, new features, and bug fixes in upcoming versions.
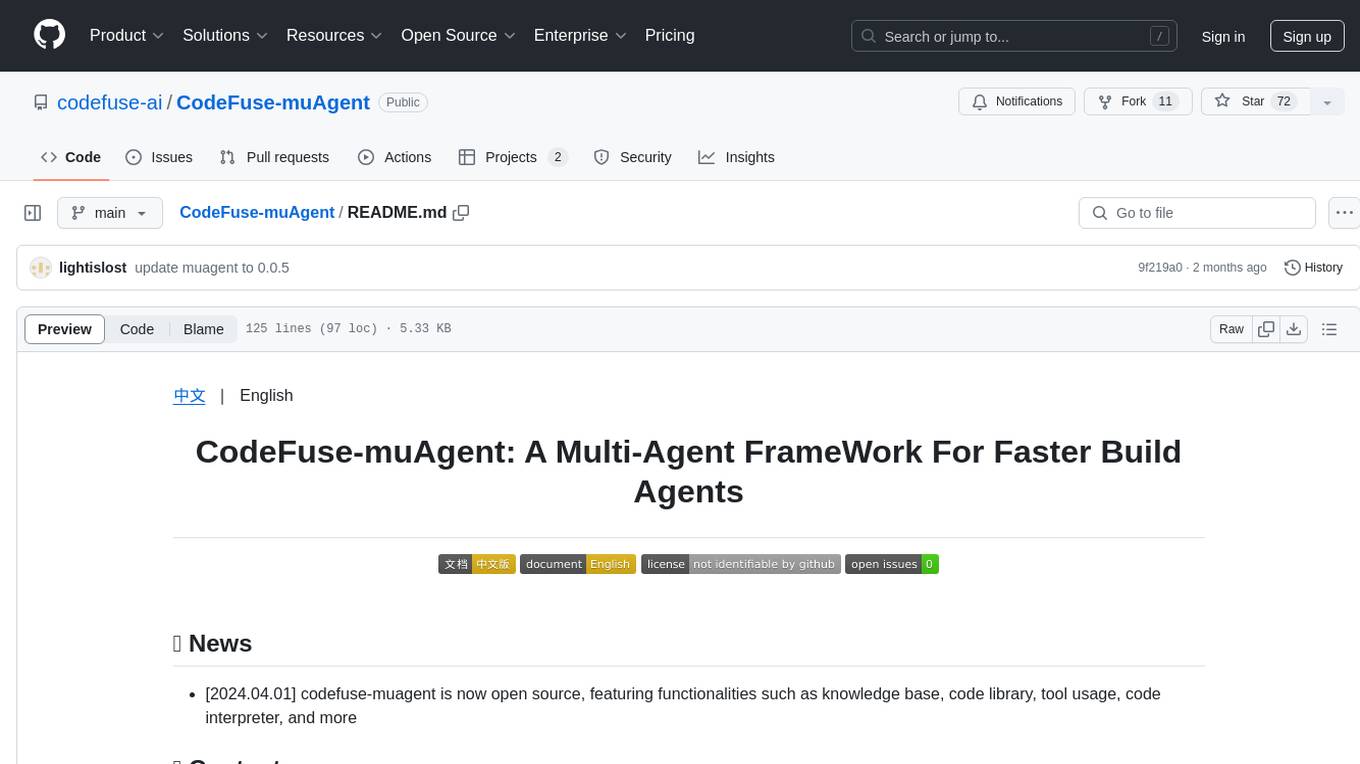
CodeFuse-muAgent
CodeFuse-muAgent is a Multi-Agent framework designed to streamline Standard Operating Procedure (SOP) orchestration for agents. It integrates toolkits, code libraries, knowledge bases, and sandbox environments for rapid construction of complex Multi-Agent interactive applications. The framework enables efficient execution and handling of multi-layered and multi-dimensional tasks.
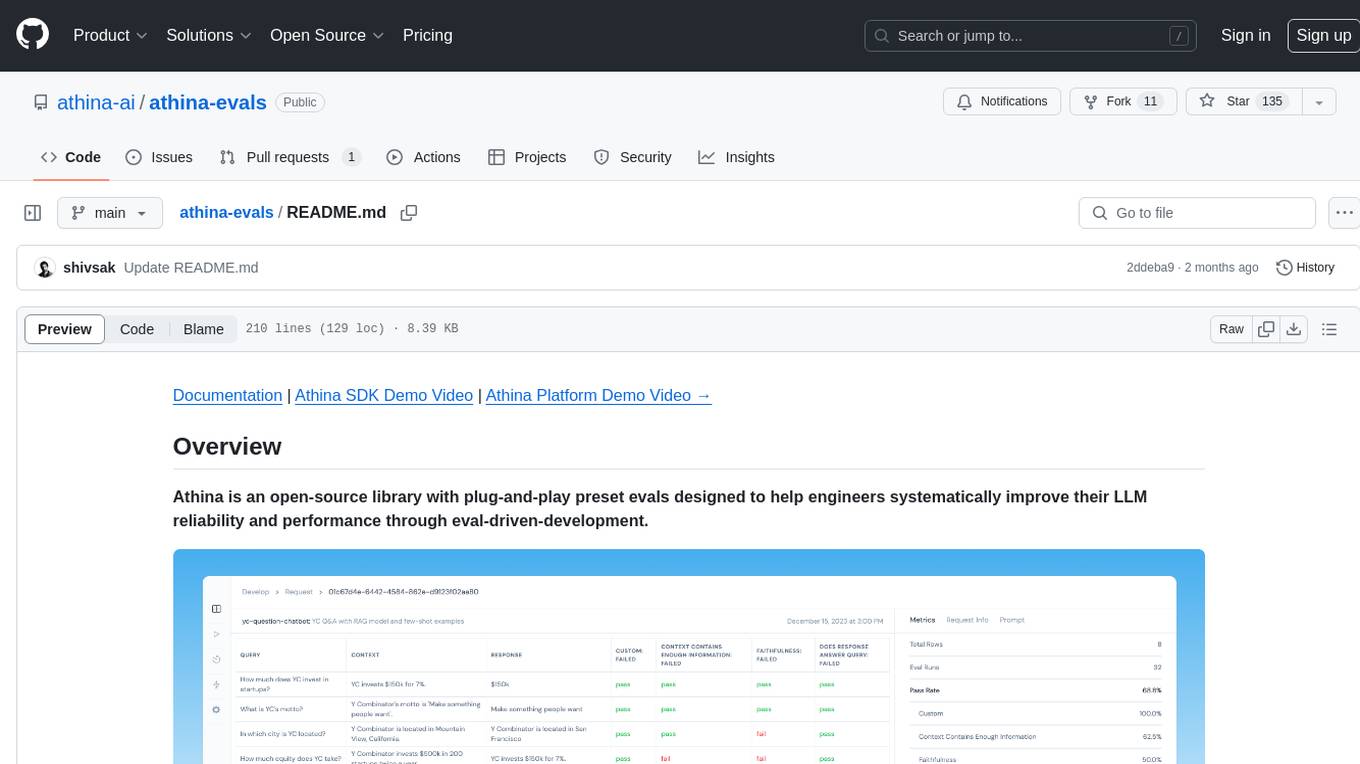
athina-evals
Athina is an open-source library designed to help engineers improve the reliability and performance of Large Language Models (LLMs) through eval-driven development. It offers plug-and-play preset evals for catching and preventing bad outputs, measuring model performance, running experiments, A/B testing models, detecting regressions, and monitoring production data. Athina provides a solution to the flaws in current LLM developer workflows by offering rapid experimentation, customizable evaluators, integrated dashboard, consistent metrics, historical record tracking, and easy setup. It includes preset evaluators for RAG applications and summarization accuracy, as well as the ability to write custom evals. Athina's evals can run on both development and production environments, providing consistent metrics and removing the need for manual infrastructure setup.
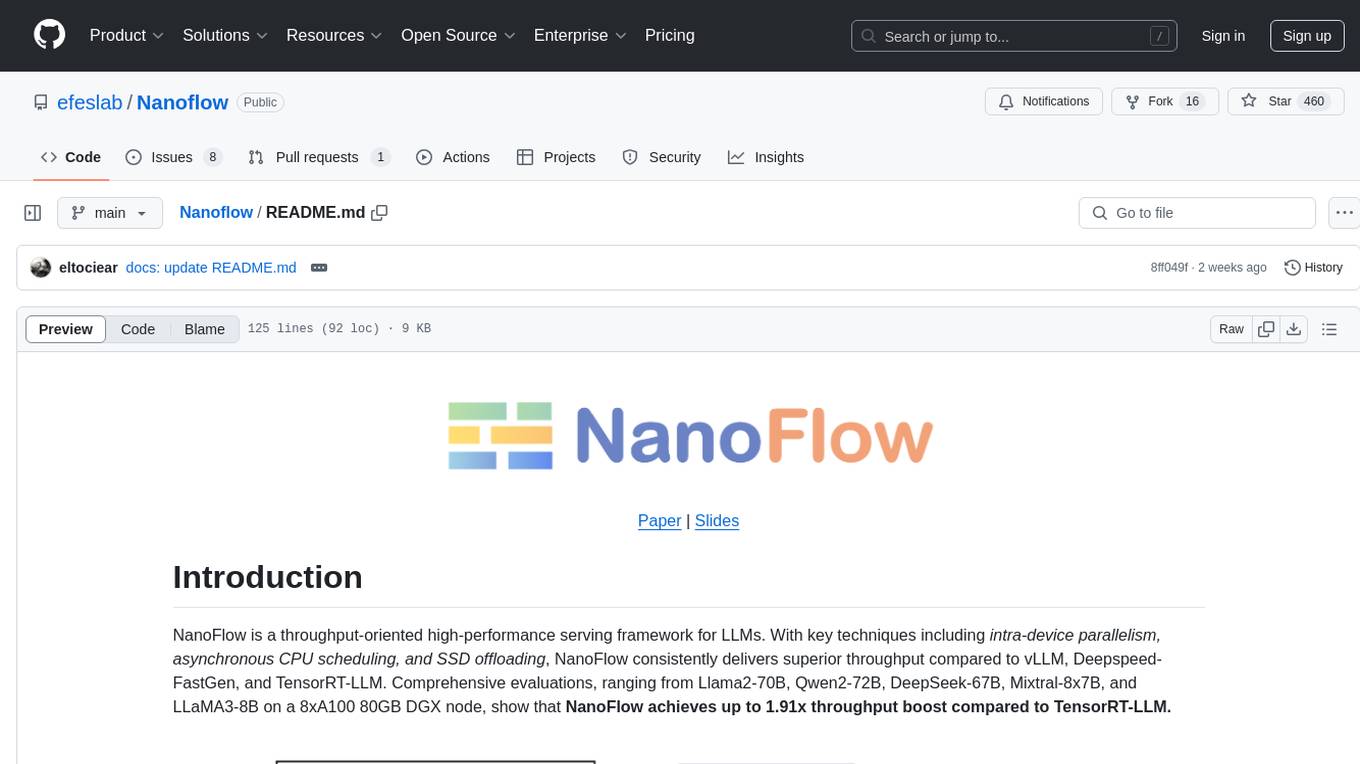
Nanoflow
NanoFlow is a throughput-oriented high-performance serving framework for Large Language Models (LLMs) that consistently delivers superior throughput compared to other frameworks by utilizing key techniques such as intra-device parallelism, asynchronous CPU scheduling, and SSD offloading. The framework proposes nano-batching to schedule compute-, memory-, and network-bound operations for simultaneous execution, leading to increased resource utilization. NanoFlow also adopts an asynchronous control flow to optimize CPU overhead and eagerly offloads KV-Cache to SSDs for multi-round conversations. The open-source codebase integrates state-of-the-art kernel libraries and provides necessary scripts for environment setup and experiment reproduction.
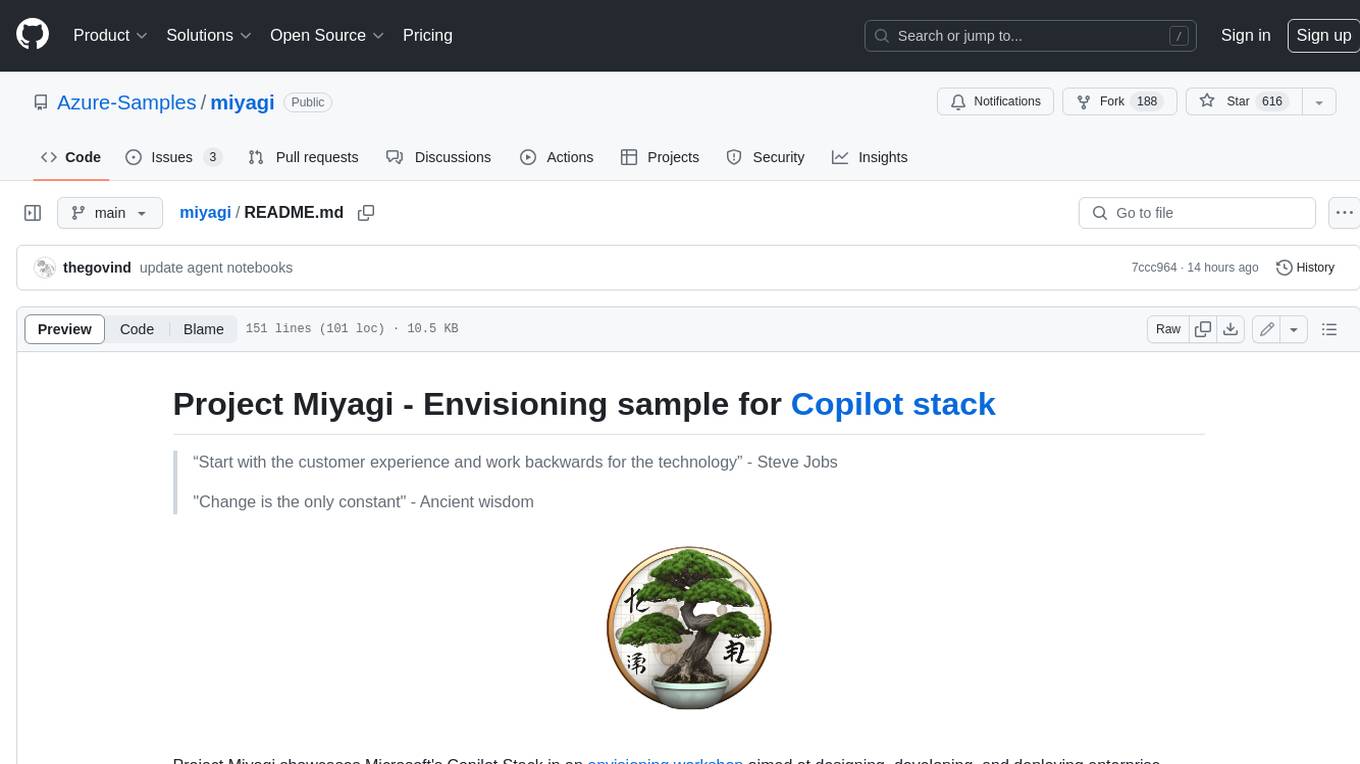
miyagi
Project Miyagi showcases Microsoft's Copilot Stack in an envisioning workshop aimed at designing, developing, and deploying enterprise-grade intelligent apps. By exploring both generative and traditional ML use cases, Miyagi offers an experiential approach to developing AI-infused product experiences that enhance productivity and enable hyper-personalization. Additionally, the workshop introduces traditional software engineers to emerging design patterns in prompt engineering, such as chain-of-thought and retrieval-augmentation, as well as to techniques like vectorization for long-term memory, fine-tuning of OSS models, agent-like orchestration, and plugins or tools for augmenting and grounding LLMs.
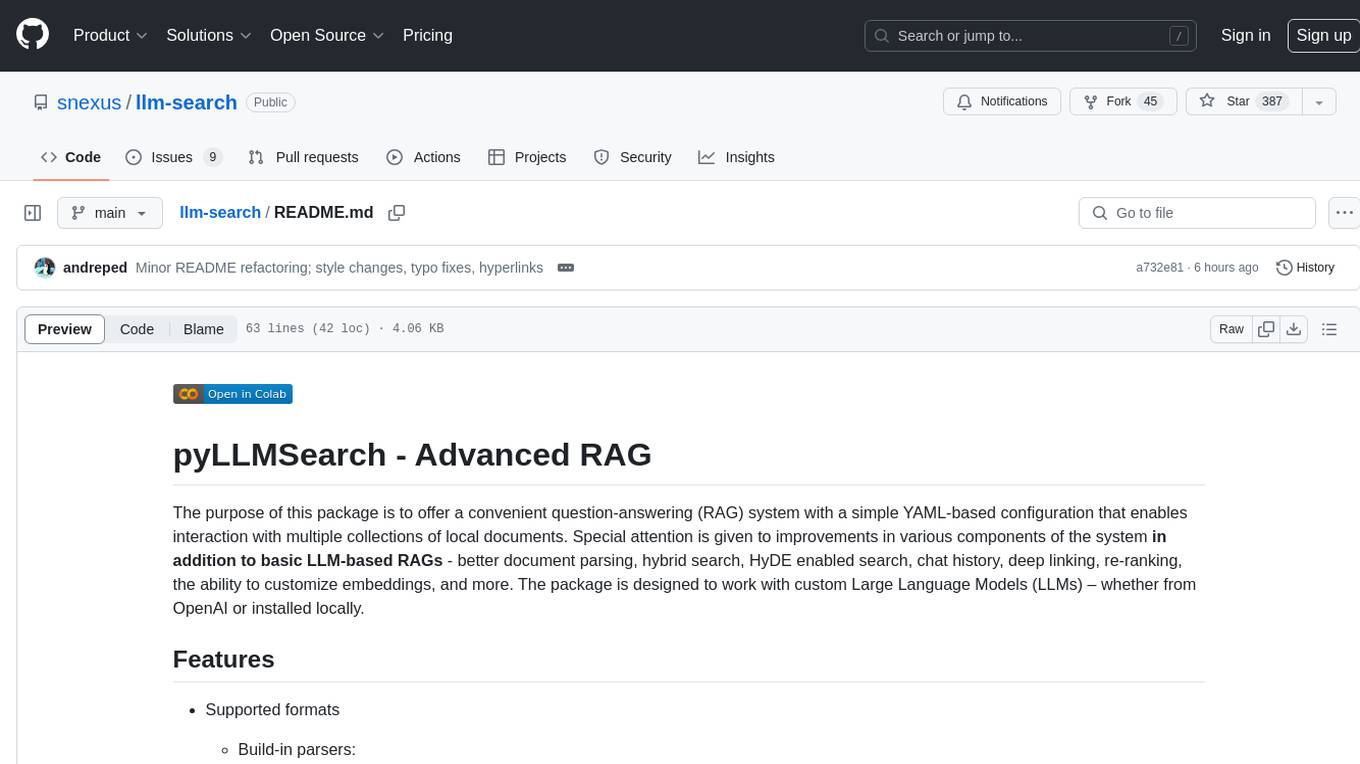
llm-search
pyLLMSearch is an advanced RAG system that offers a convenient question-answering system with a simple YAML-based configuration. It enables interaction with multiple collections of local documents, with improvements in document parsing, hybrid search, chat history, deep linking, re-ranking, customizable embeddings, and more. The package is designed to work with custom Large Language Models (LLMs) from OpenAI or installed locally. It supports various document formats, incremental embedding updates, dense and sparse embeddings, multiple embedding models, 'Retrieve and Re-rank' strategy, HyDE (Hypothetical Document Embeddings), multi-querying, chat history, and interaction with embedded documents using different models. It also offers simple CLI and web interfaces, deep linking, offline response saving, and an experimental API.
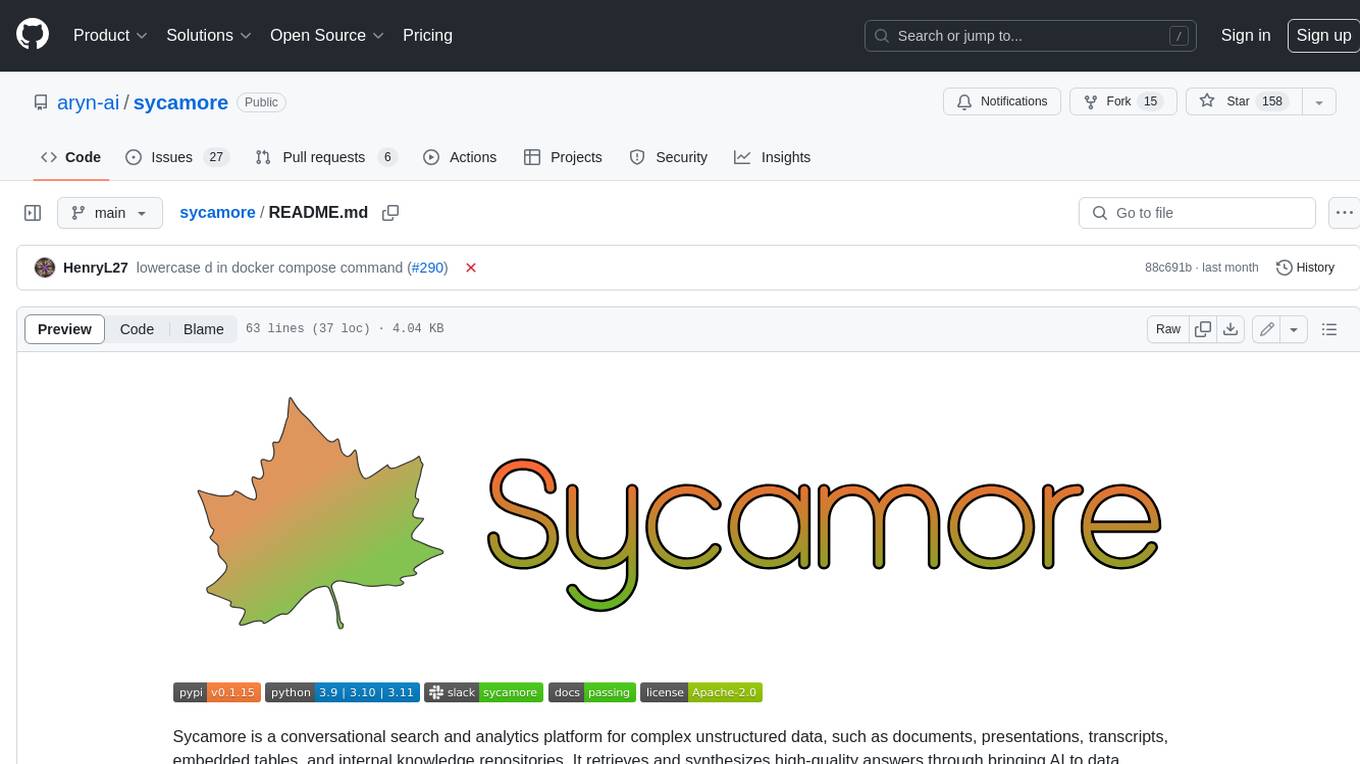
sycamore
Sycamore is a conversational search and analytics platform for complex unstructured data, such as documents, presentations, transcripts, embedded tables, and internal knowledge repositories. It retrieves and synthesizes high-quality answers through bringing AI to data preparation, indexing, and retrieval. Sycamore makes it easy to prepare unstructured data for search and analytics, providing a toolkit for data cleaning, information extraction, enrichment, summarization, and generation of vector embeddings that encapsulate the semantics of data. Sycamore uses your choice of generative AI models to make these operations simple and effective, and it enables quick experimentation and iteration. Additionally, Sycamore uses OpenSearch for indexing, enabling hybrid (vector + keyword) search, retrieval-augmented generation (RAG) pipelining, filtering, analytical functions, conversational memory, and other features to improve information retrieval.
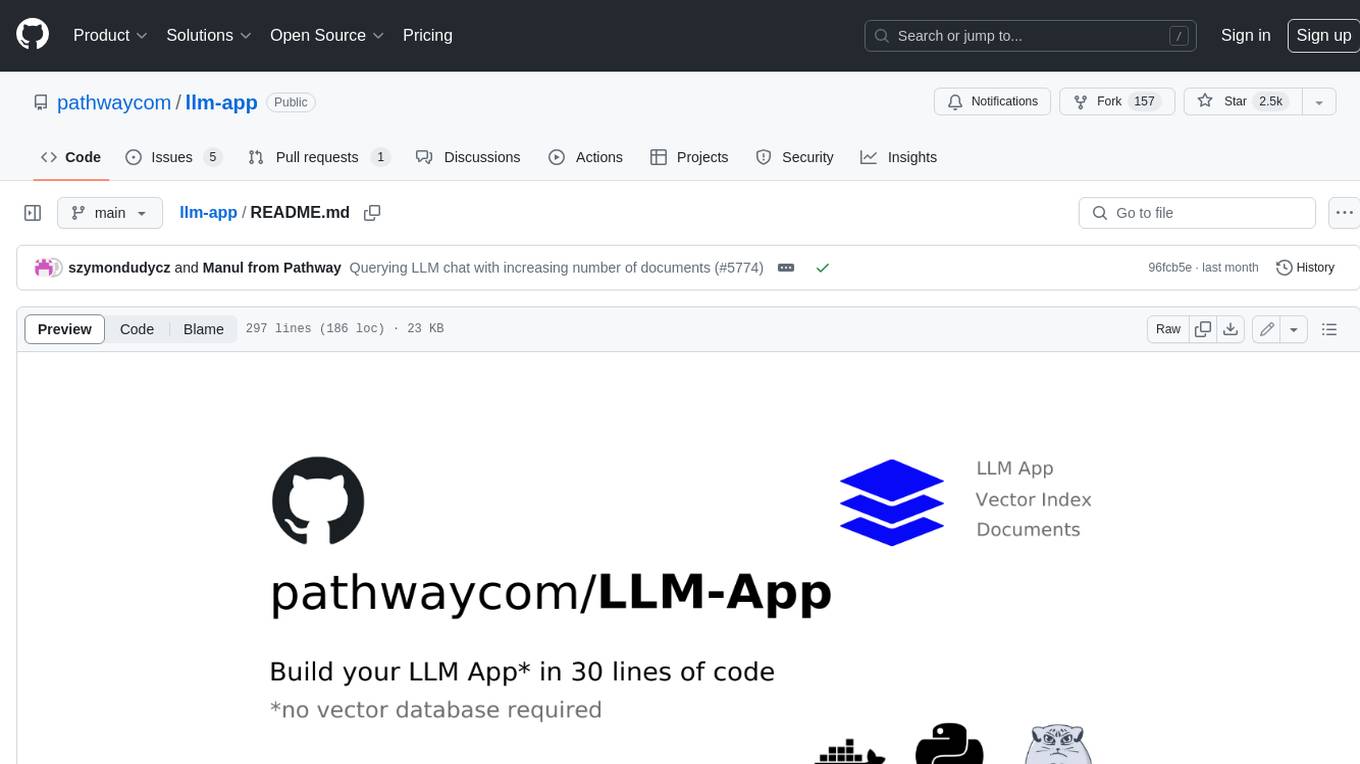
llm-app
Pathway's LLM (Large Language Model) Apps provide a platform to quickly deploy AI applications using the latest knowledge from data sources. The Python application examples in this repository are Docker-ready, exposing an HTTP API to the frontend. These apps utilize the Pathway framework for data synchronization, API serving, and low-latency data processing without the need for additional infrastructure dependencies. They connect to document data sources like S3, Google Drive, and Sharepoint, offering features like real-time data syncing, easy alert setup, scalability, monitoring, security, and unification of application logic.
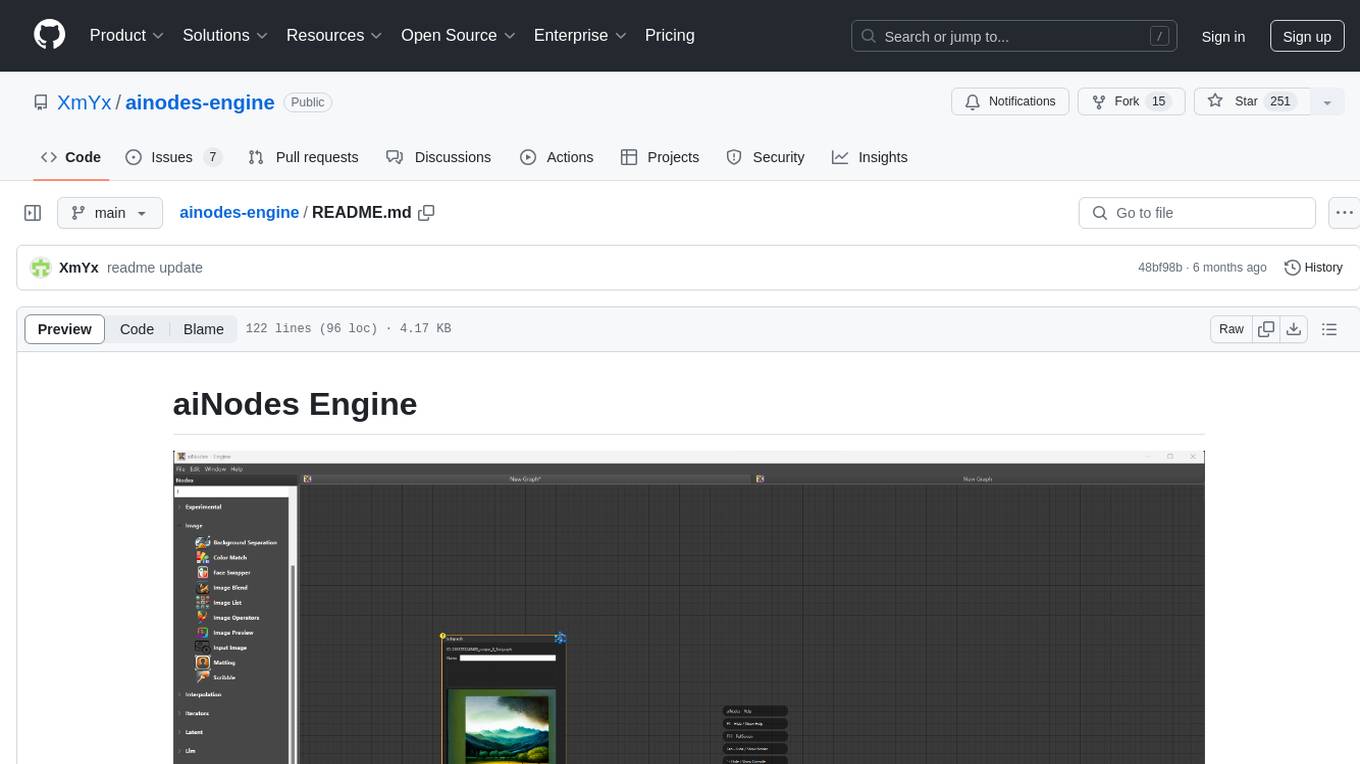
ainodes-engine
aiNodes Engine is a Python-based AI image/motion picture generator node engine with a live execution chain, python code editor node, and plug-in support. It offers full modularity, colored background drop, and easy node creation with IDE annotations. The project is officially supported by Deforum and incorporates various open-source projects like ComfyUI. It is designed to be flexible, with an Unreal-like execution chain, supporting features such as Deforum, Stable Diffusion, Upscalers, Kandinsky, ControlNet, and more. The engine allows for background separation, human matting/masking, compositing, drag and drop, subgraphs, and graph saving/loading from image metadata. It aims to provide a unique, controllable manner of working with a strict user-declared execution chain.
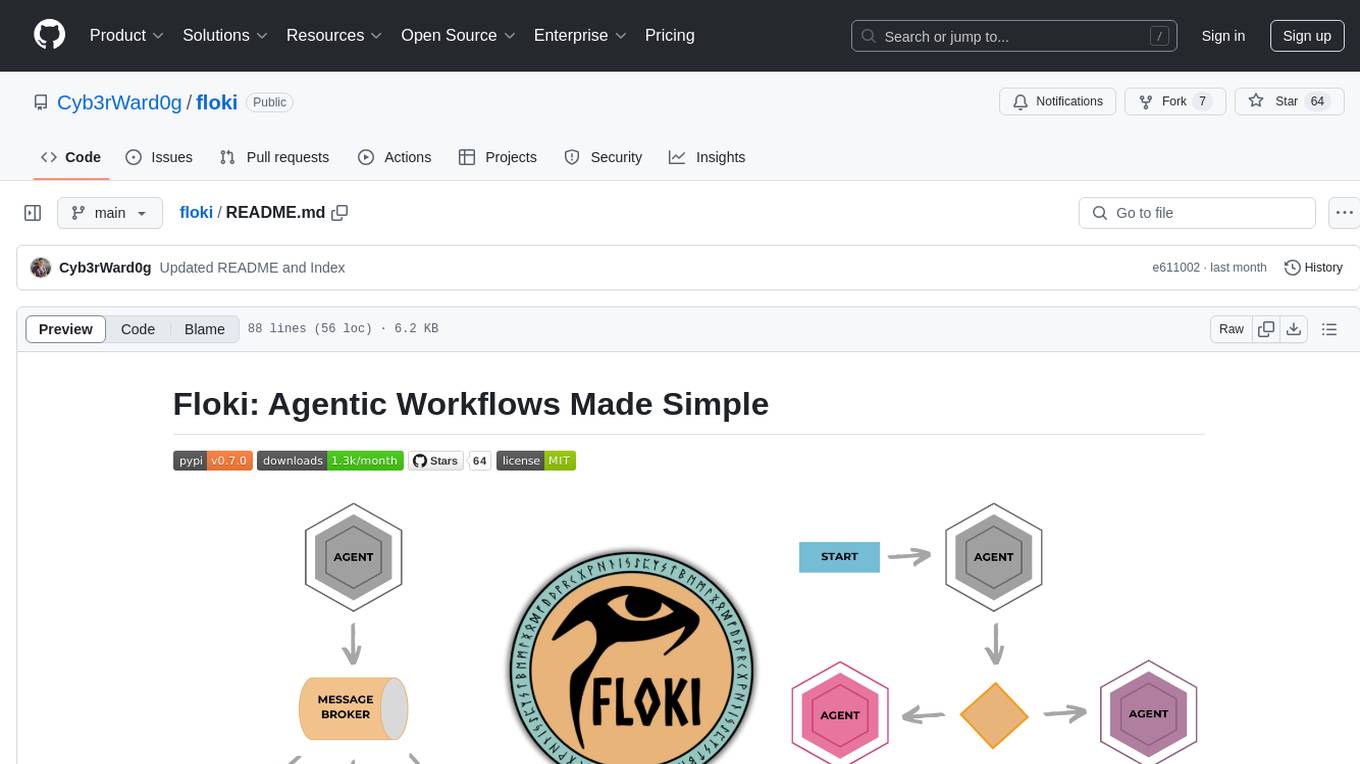
floki
Floki is an open-source framework for researchers and developers to experiment with LLM-based autonomous agents. It provides tools to create, orchestrate, and manage agents while seamlessly connecting to LLM inference APIs. Built on Dapr, Floki leverages a unified programming model that simplifies microservices and supports both deterministic workflows and event-driven interactions. By bringing together these features, Floki provides a powerful way to explore agentic workflows and the components that enable multi-agent systems to collaborate and scale, all powered by Dapr.
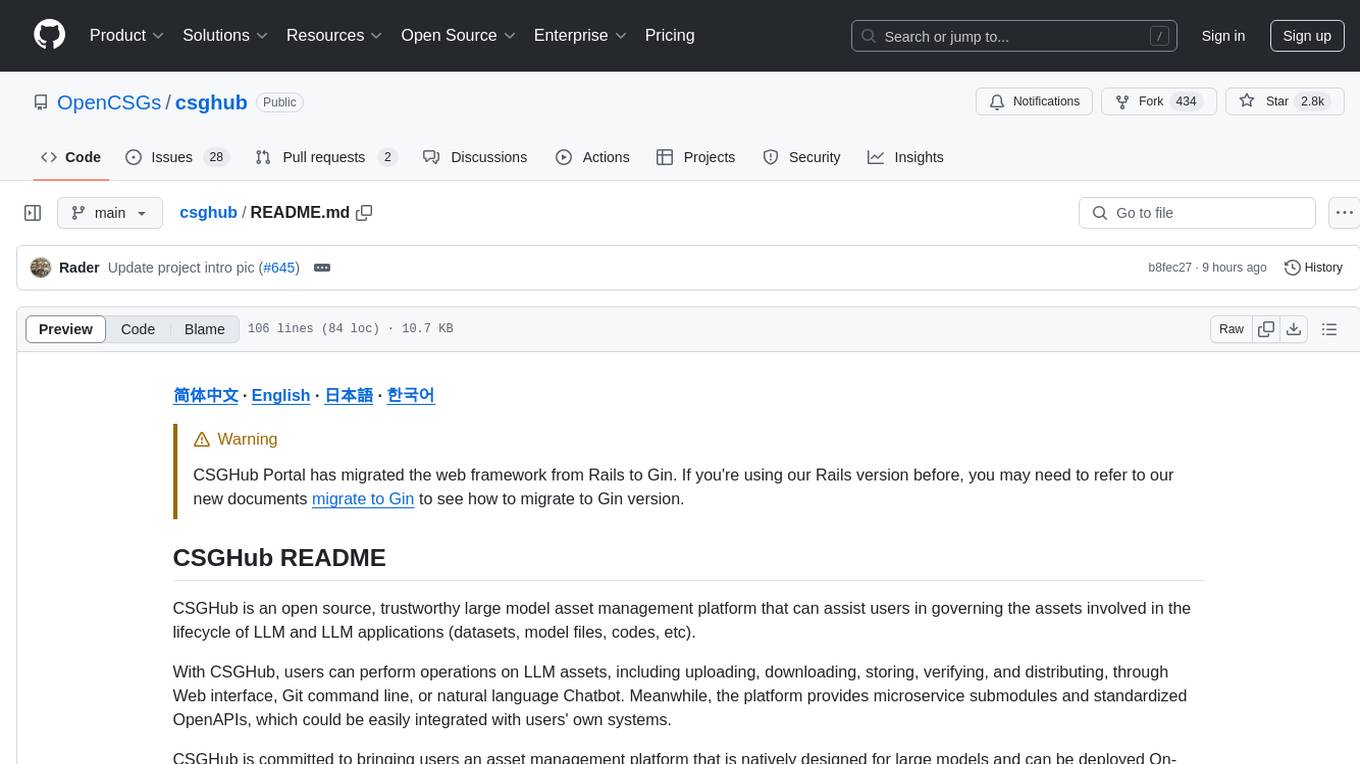
csghub
CSGHub is an open source platform for managing large model assets, including datasets, model files, and codes. It offers functionalities similar to a privatized Huggingface, managing assets in a manner akin to how OpenStack Glance manages virtual machine images. Users can perform operations such as uploading, downloading, storing, verifying, and distributing assets through various interfaces. The platform provides microservice submodules and standardized OpenAPIs for easy integration with users' systems. CSGHub is designed for large models and can be deployed On-Premise for offline operation.
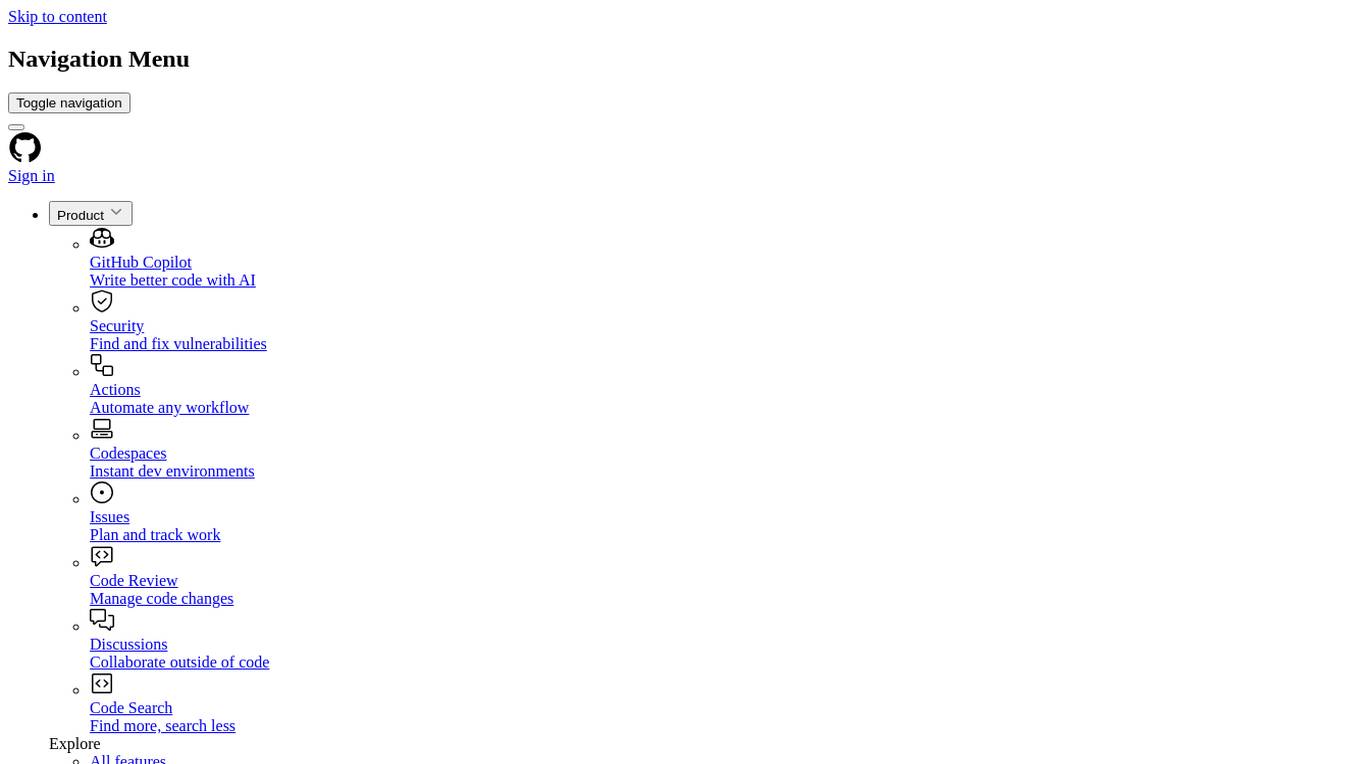
agentUniverse
agentUniverse is a multi-agent framework based on large language models, providing flexible capabilities for building individual agents. It focuses on collaborative pattern components to solve problems in various fields and integrates domain experience. The framework supports LLM model integration and offers various pattern components like PEER and DOE. Users can easily configure models and set up agents for tasks. agentUniverse aims to assist developers and enterprises in constructing domain-expert-level intelligent agents for seamless collaboration.
For similar tasks
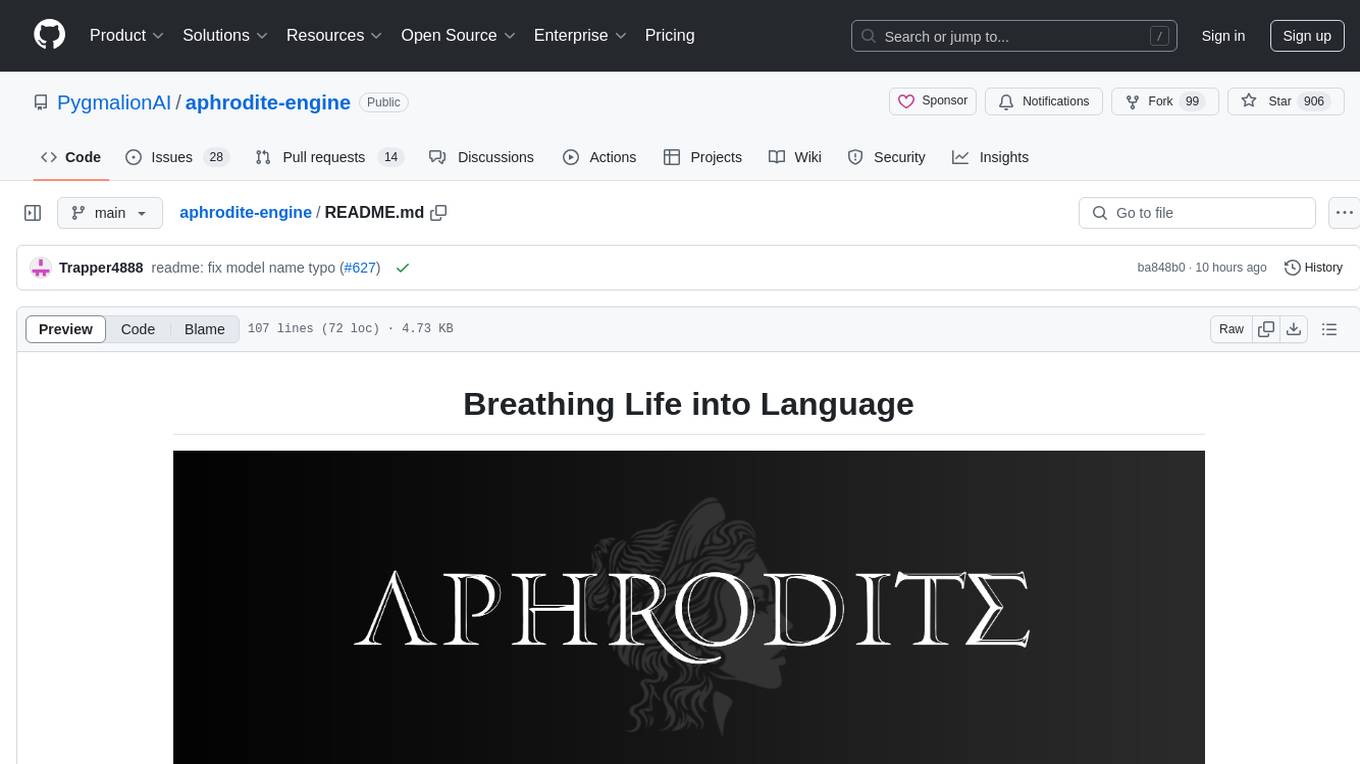
aphrodite-engine
Aphrodite is the official backend engine for PygmalionAI, serving as the inference endpoint for the website. It allows serving Hugging Face-compatible models with fast speeds. Features include continuous batching, efficient K/V management, optimized CUDA kernels, quantization support, distributed inference, and 8-bit KV Cache. The engine requires Linux OS and Python 3.8 to 3.12, with CUDA >= 11 for build requirements. It supports various GPUs, CPUs, TPUs, and Inferentia. Users can limit GPU memory utilization and access full commands via CLI.
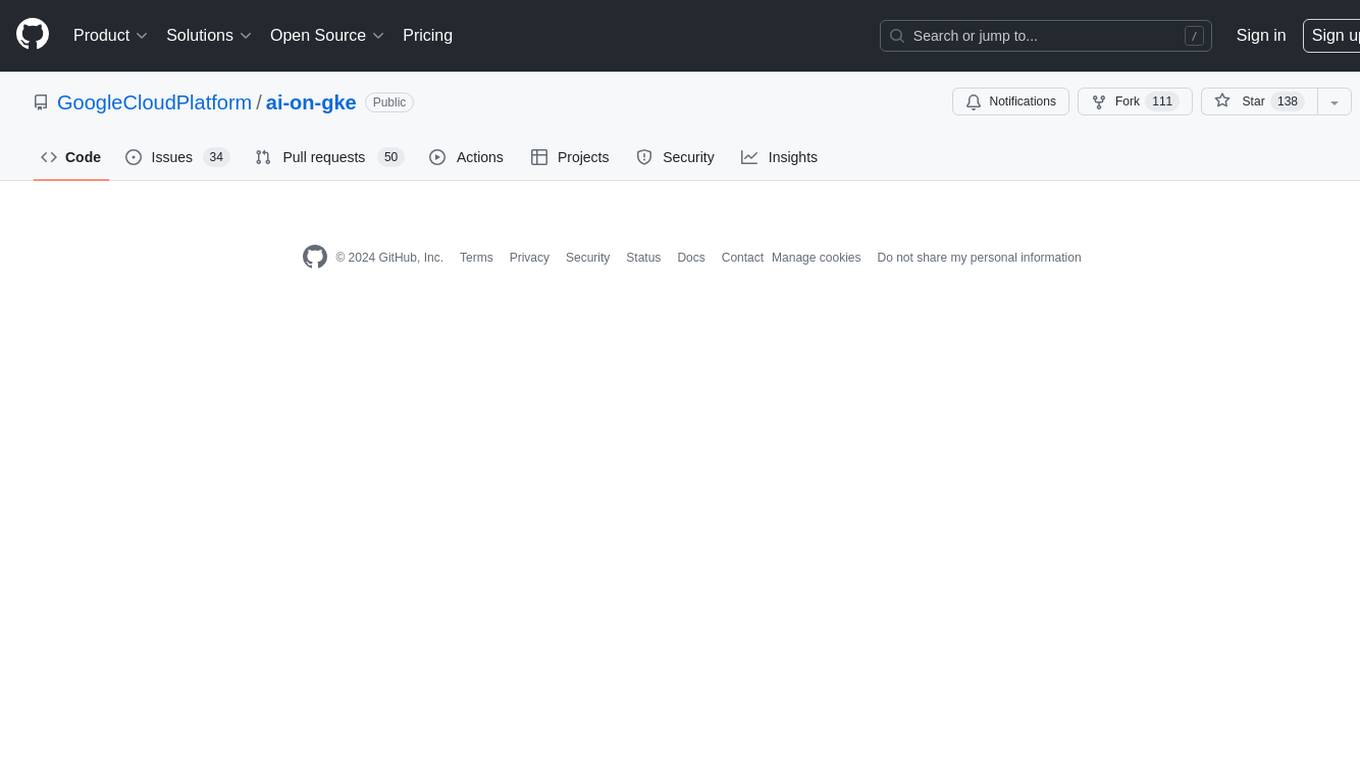
ai-on-gke
This repository contains assets related to AI/ML workloads on Google Kubernetes Engine (GKE). Run optimized AI/ML workloads with Google Kubernetes Engine (GKE) platform orchestration capabilities. A robust AI/ML platform considers the following layers: Infrastructure orchestration that support GPUs and TPUs for training and serving workloads at scale Flexible integration with distributed computing and data processing frameworks Support for multiple teams on the same infrastructure to maximize utilization of resources
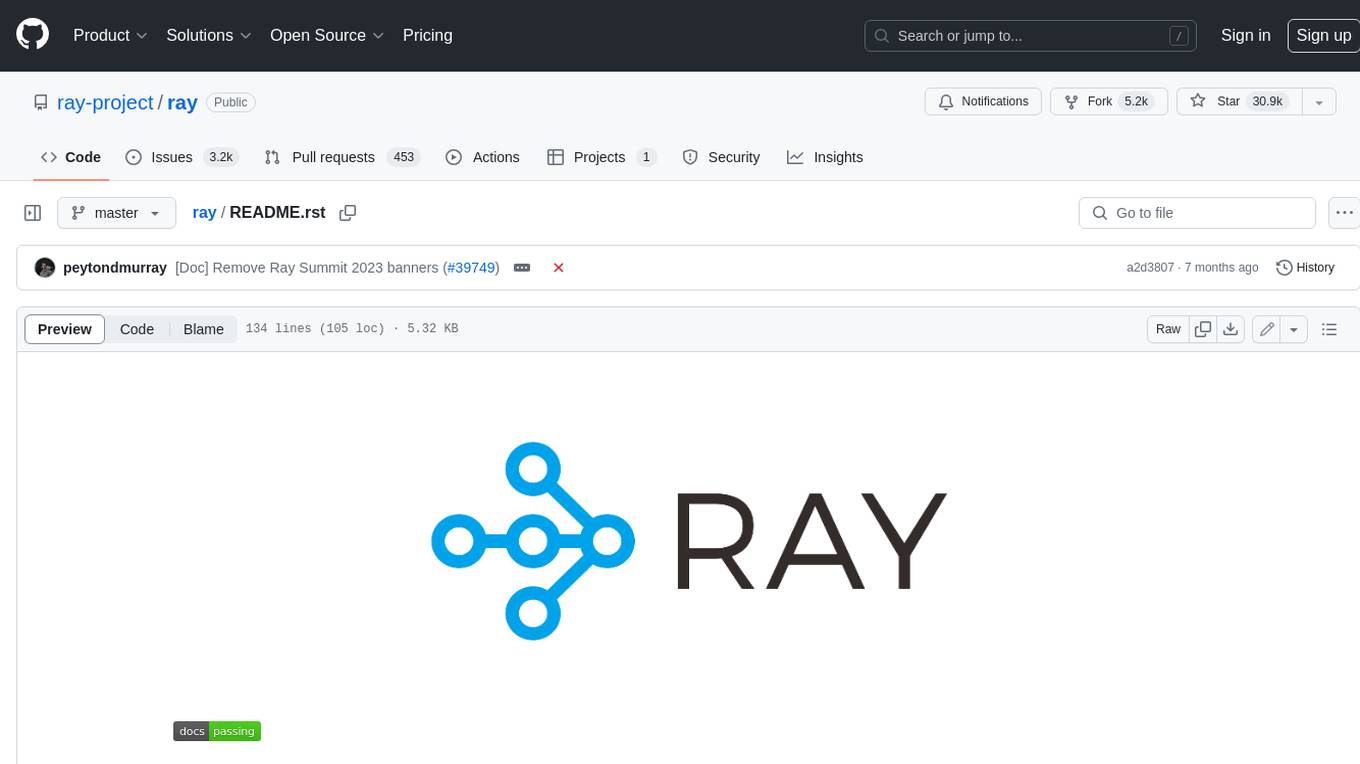
ray
Ray is a unified framework for scaling AI and Python applications. It consists of a core distributed runtime and a set of AI libraries for simplifying ML compute, including Data, Train, Tune, RLlib, and Serve. Ray runs on any machine, cluster, cloud provider, and Kubernetes, and features a growing ecosystem of community integrations. With Ray, you can seamlessly scale the same code from a laptop to a cluster, making it easy to meet the compute-intensive demands of modern ML workloads.
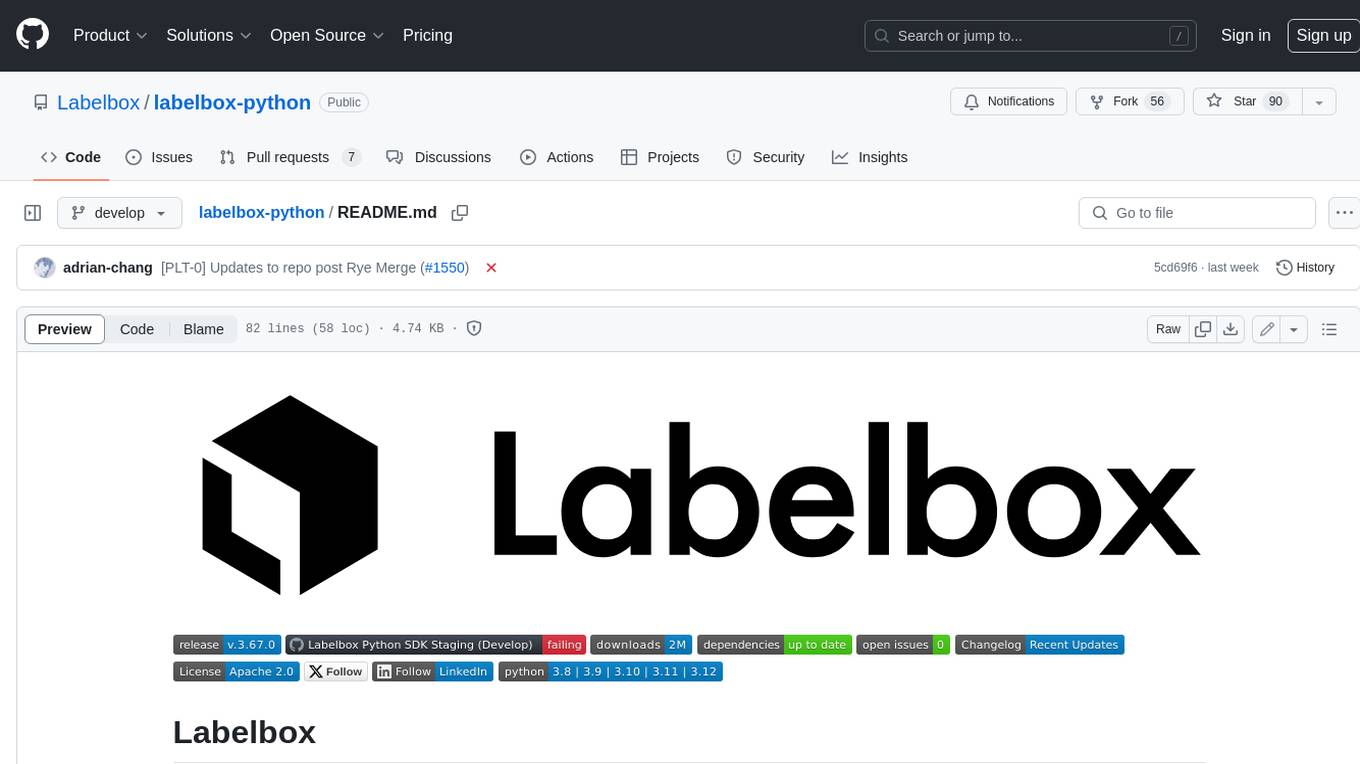
labelbox-python
Labelbox is a data-centric AI platform for enterprises to develop, optimize, and use AI to solve problems and power new products and services. Enterprises use Labelbox to curate data, generate high-quality human feedback data for computer vision and LLMs, evaluate model performance, and automate tasks by combining AI and human-centric workflows. The academic & research community uses Labelbox for cutting-edge AI research.
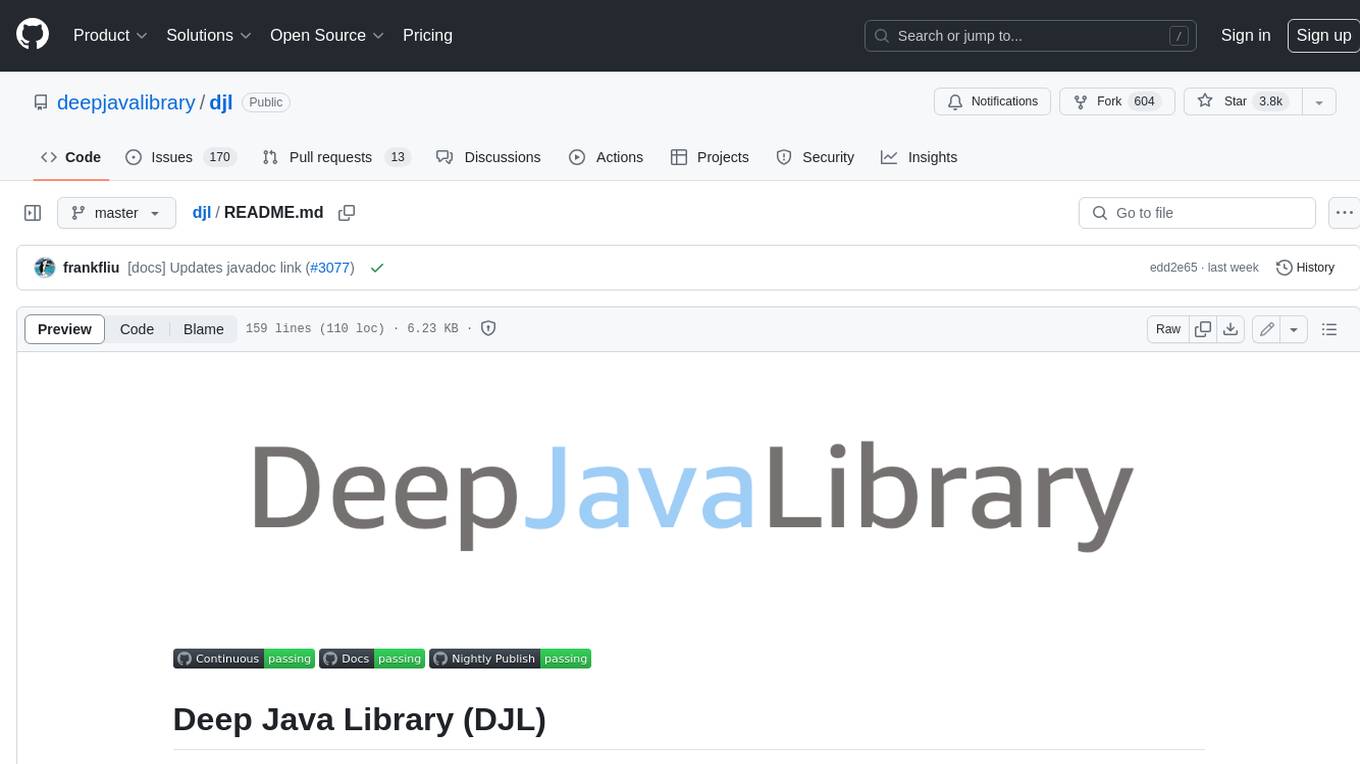
djl
Deep Java Library (DJL) is an open-source, high-level, engine-agnostic Java framework for deep learning. It is designed to be easy to get started with and simple to use for Java developers. DJL provides a native Java development experience and allows users to integrate machine learning and deep learning models with their Java applications. The framework is deep learning engine agnostic, enabling users to switch engines at any point for optimal performance. DJL's ergonomic API interface guides users with best practices to accomplish deep learning tasks, such as running inference and training neural networks.
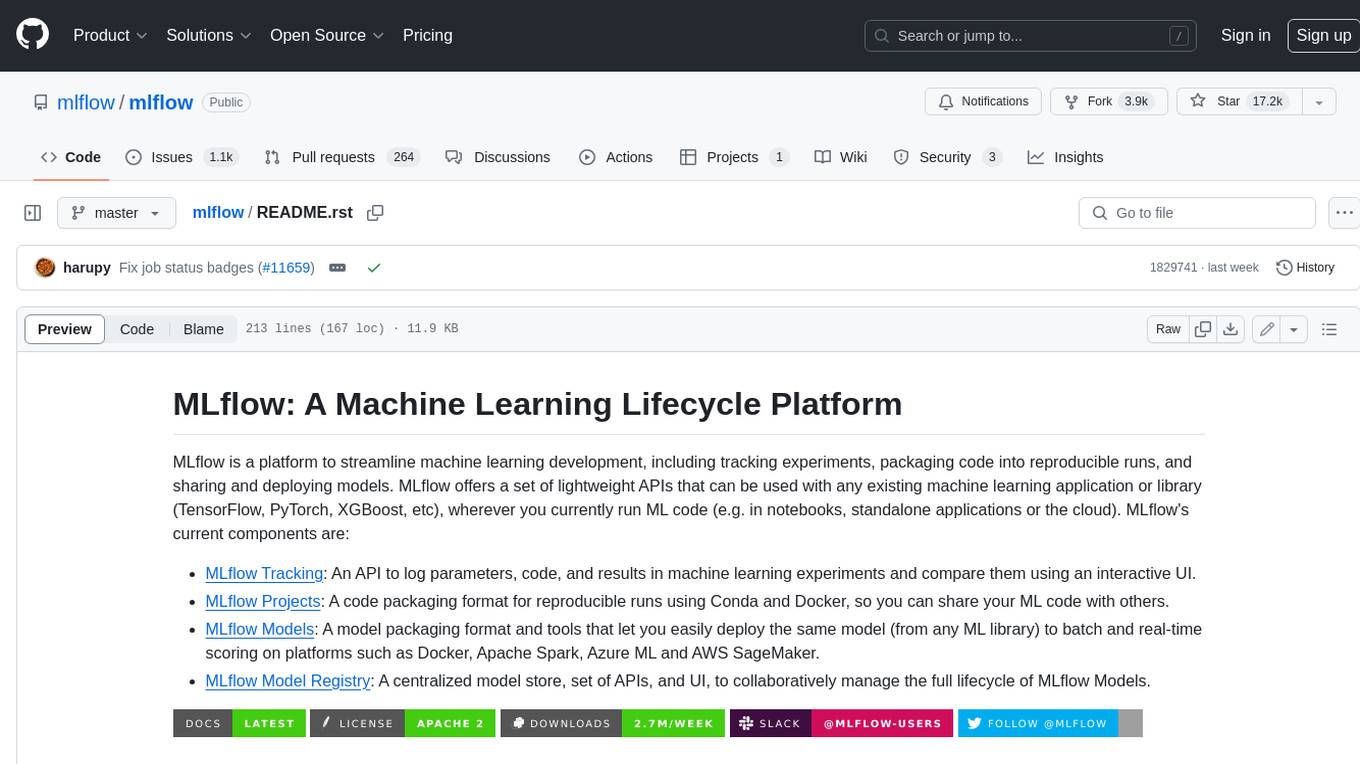
mlflow
MLflow is a platform to streamline machine learning development, including tracking experiments, packaging code into reproducible runs, and sharing and deploying models. MLflow offers a set of lightweight APIs that can be used with any existing machine learning application or library (TensorFlow, PyTorch, XGBoost, etc), wherever you currently run ML code (e.g. in notebooks, standalone applications or the cloud). MLflow's current components are:
* `MLflow Tracking
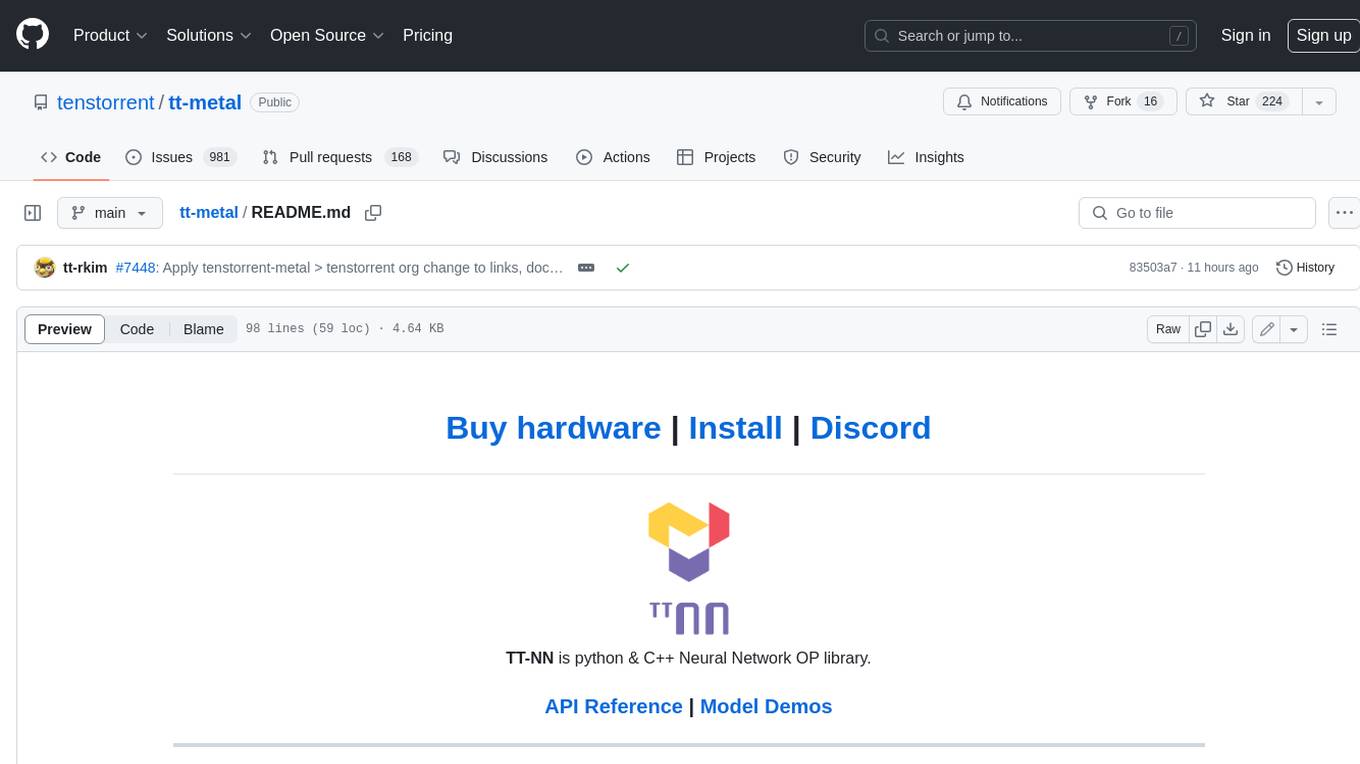
tt-metal
TT-NN is a python & C++ Neural Network OP library. It provides a low-level programming model, TT-Metalium, enabling kernel development for Tenstorrent hardware.
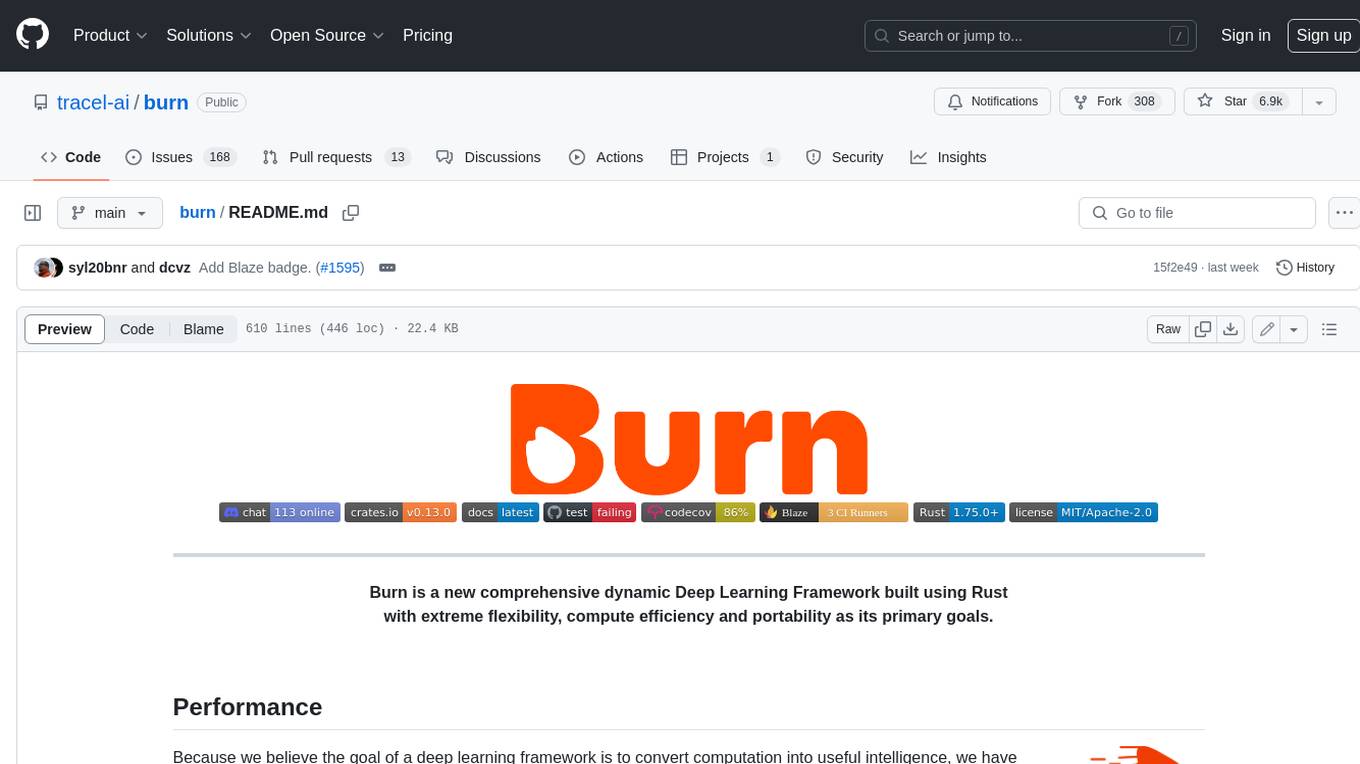
burn
Burn is a new comprehensive dynamic Deep Learning Framework built using Rust with extreme flexibility, compute efficiency and portability as its primary goals.
For similar jobs
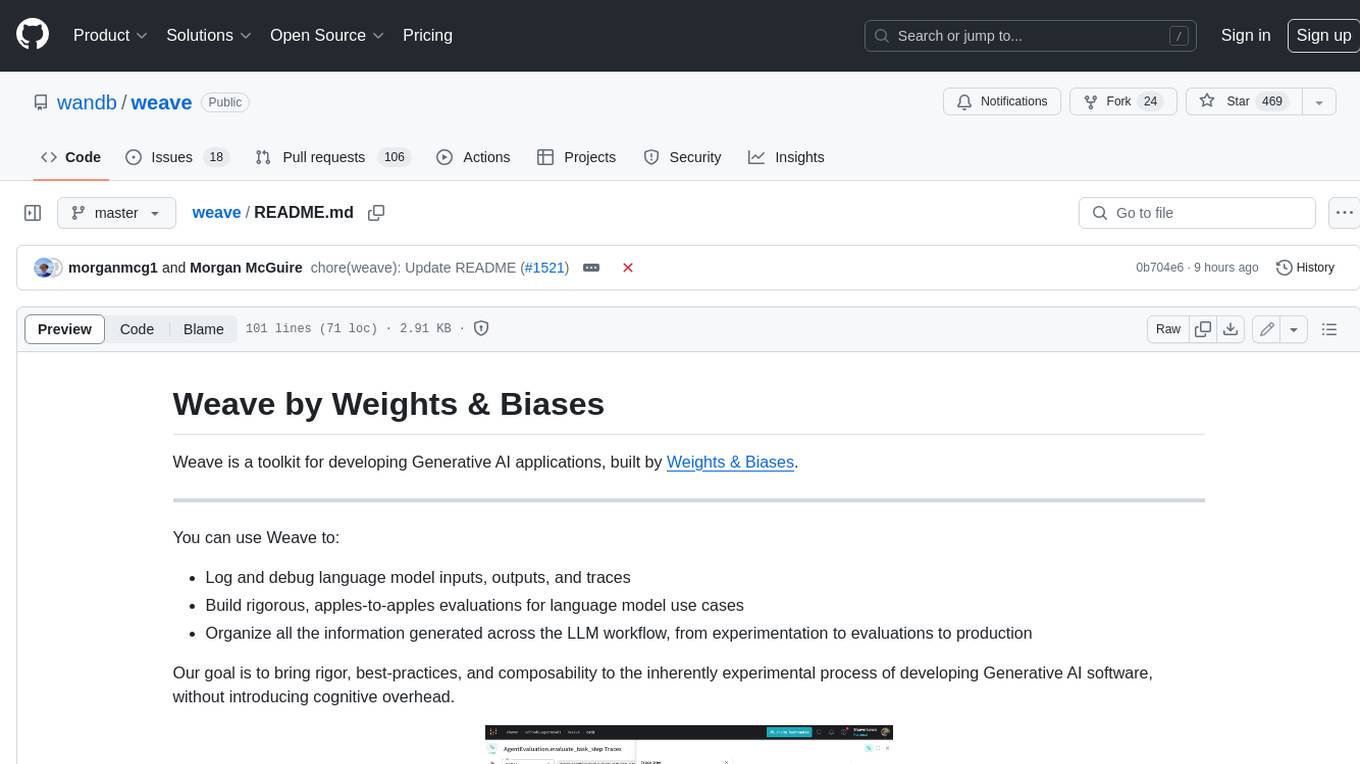
weave
Weave is a toolkit for developing Generative AI applications, built by Weights & Biases. With Weave, you can log and debug language model inputs, outputs, and traces; build rigorous, apples-to-apples evaluations for language model use cases; and organize all the information generated across the LLM workflow, from experimentation to evaluations to production. Weave aims to bring rigor, best-practices, and composability to the inherently experimental process of developing Generative AI software, without introducing cognitive overhead.
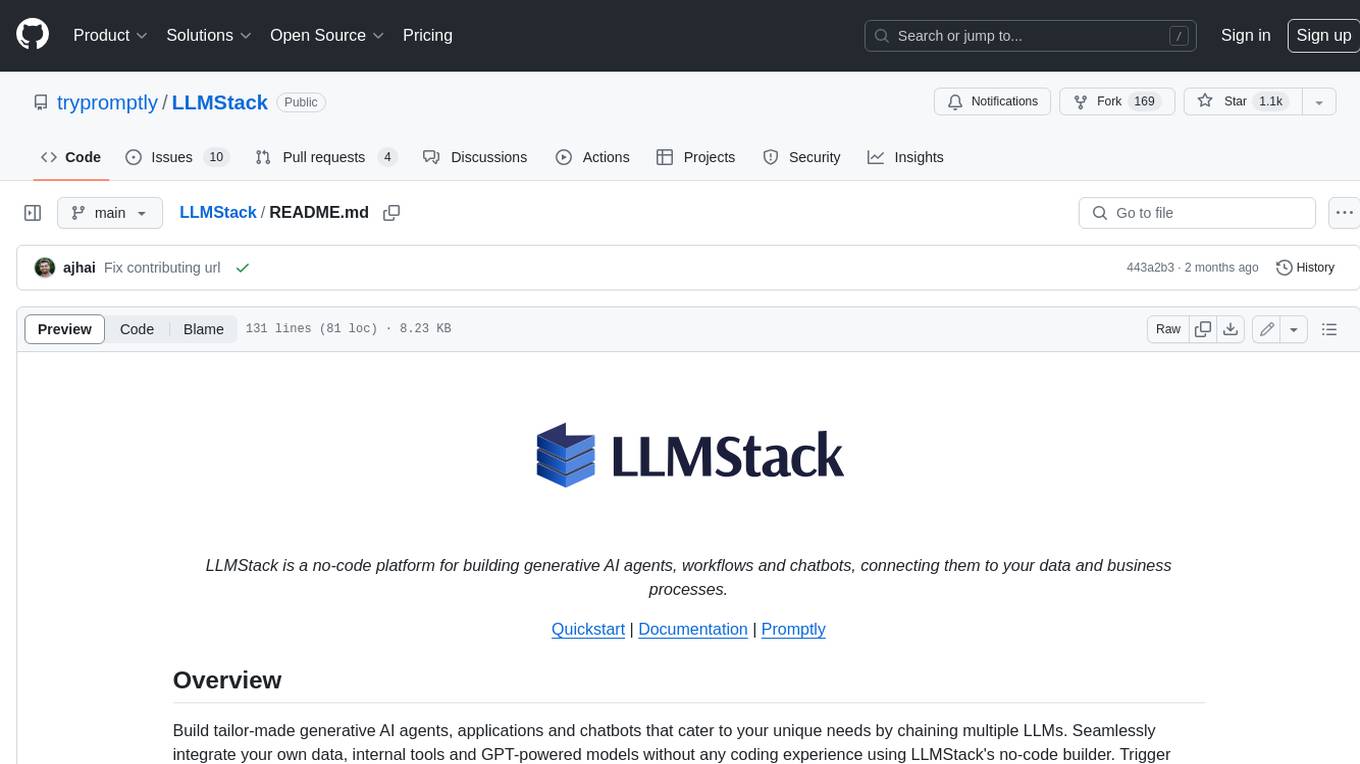
LLMStack
LLMStack is a no-code platform for building generative AI agents, workflows, and chatbots. It allows users to connect their own data, internal tools, and GPT-powered models without any coding experience. LLMStack can be deployed to the cloud or on-premise and can be accessed via HTTP API or triggered from Slack or Discord.
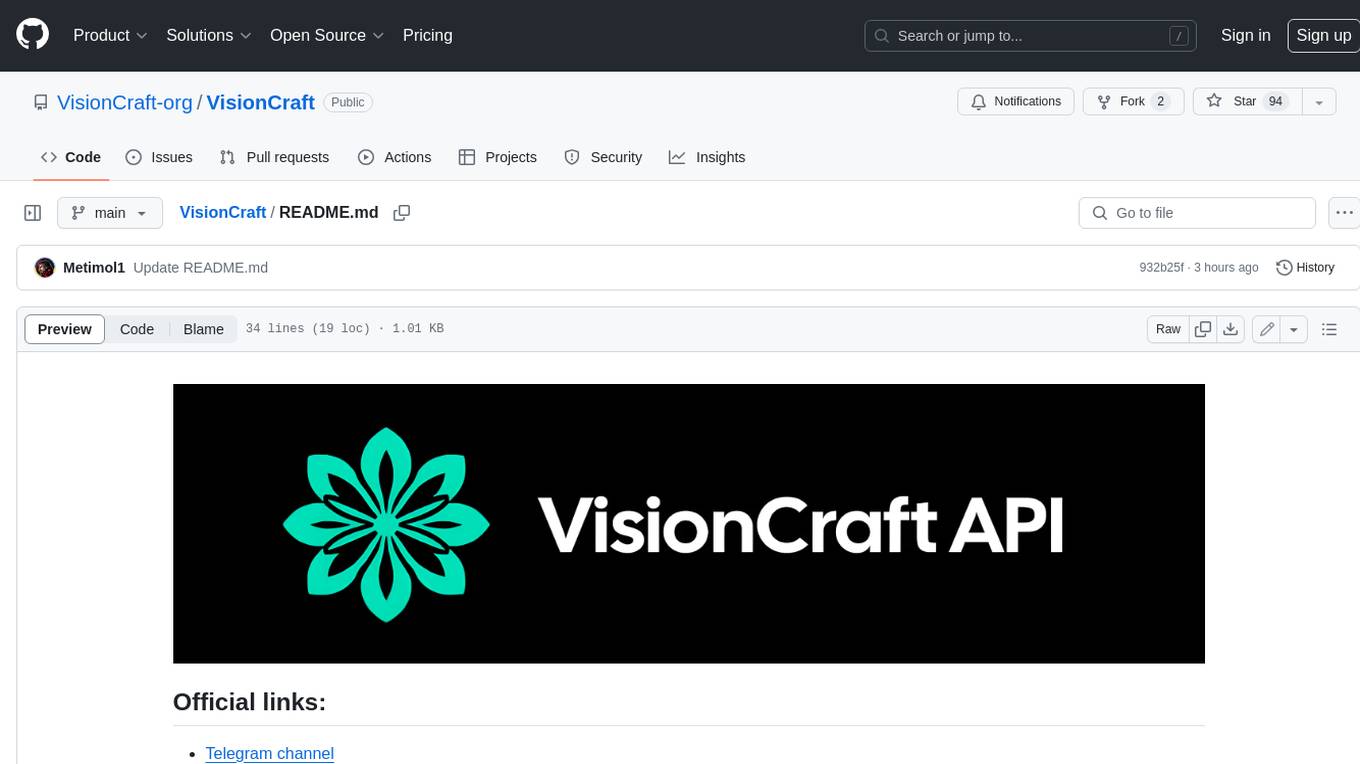
VisionCraft
The VisionCraft API is a free API for using over 100 different AI models. From images to sound.
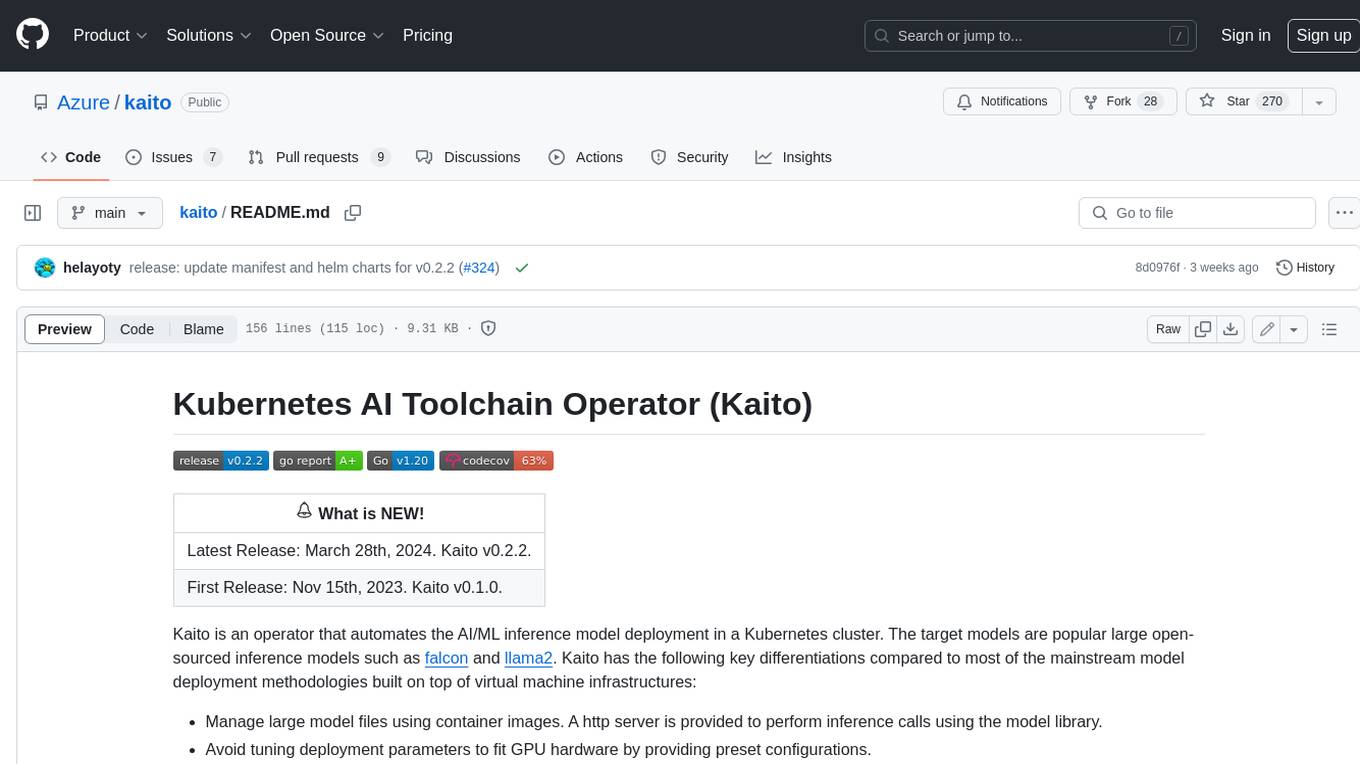
kaito
Kaito is an operator that automates the AI/ML inference model deployment in a Kubernetes cluster. It manages large model files using container images, avoids tuning deployment parameters to fit GPU hardware by providing preset configurations, auto-provisions GPU nodes based on model requirements, and hosts large model images in the public Microsoft Container Registry (MCR) if the license allows. Using Kaito, the workflow of onboarding large AI inference models in Kubernetes is largely simplified.
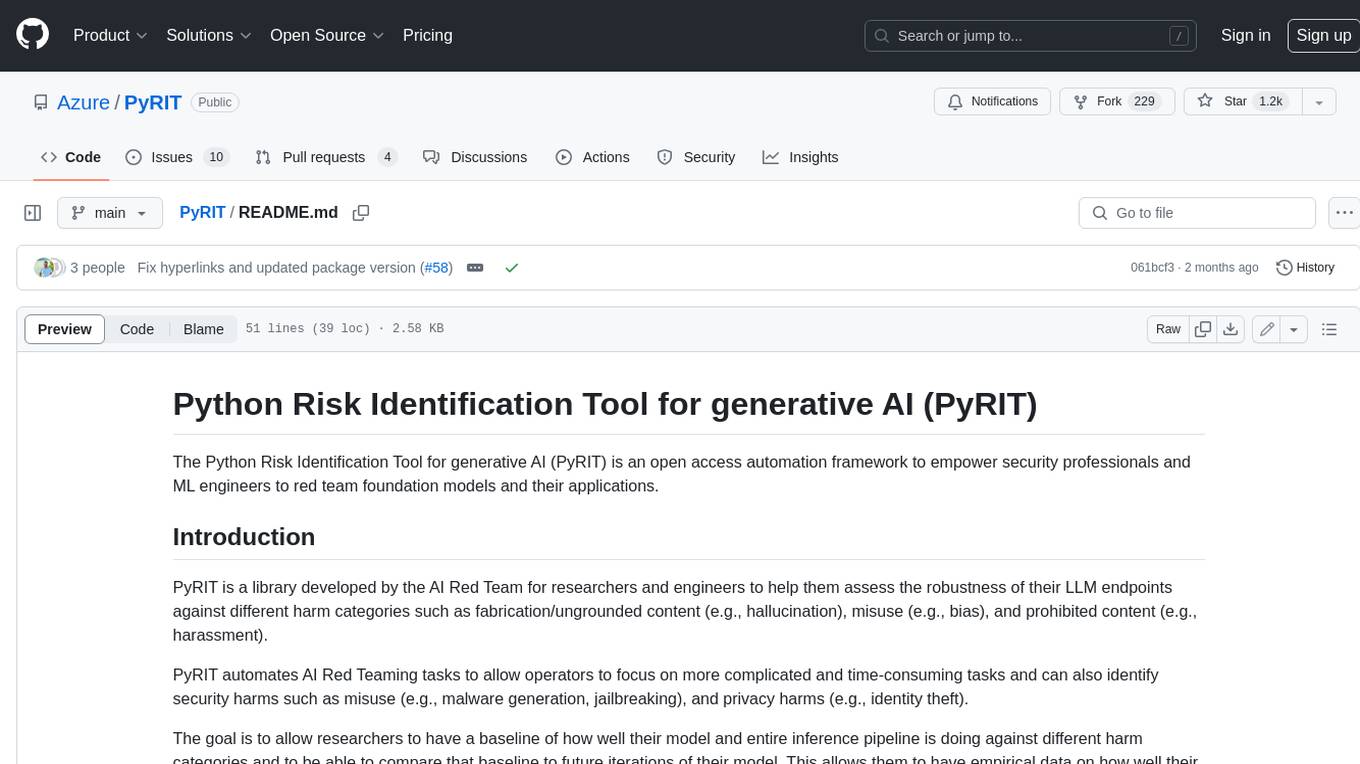
PyRIT
PyRIT is an open access automation framework designed to empower security professionals and ML engineers to red team foundation models and their applications. It automates AI Red Teaming tasks to allow operators to focus on more complicated and time-consuming tasks and can also identify security harms such as misuse (e.g., malware generation, jailbreaking), and privacy harms (e.g., identity theft). The goal is to allow researchers to have a baseline of how well their model and entire inference pipeline is doing against different harm categories and to be able to compare that baseline to future iterations of their model. This allows them to have empirical data on how well their model is doing today, and detect any degradation of performance based on future improvements.
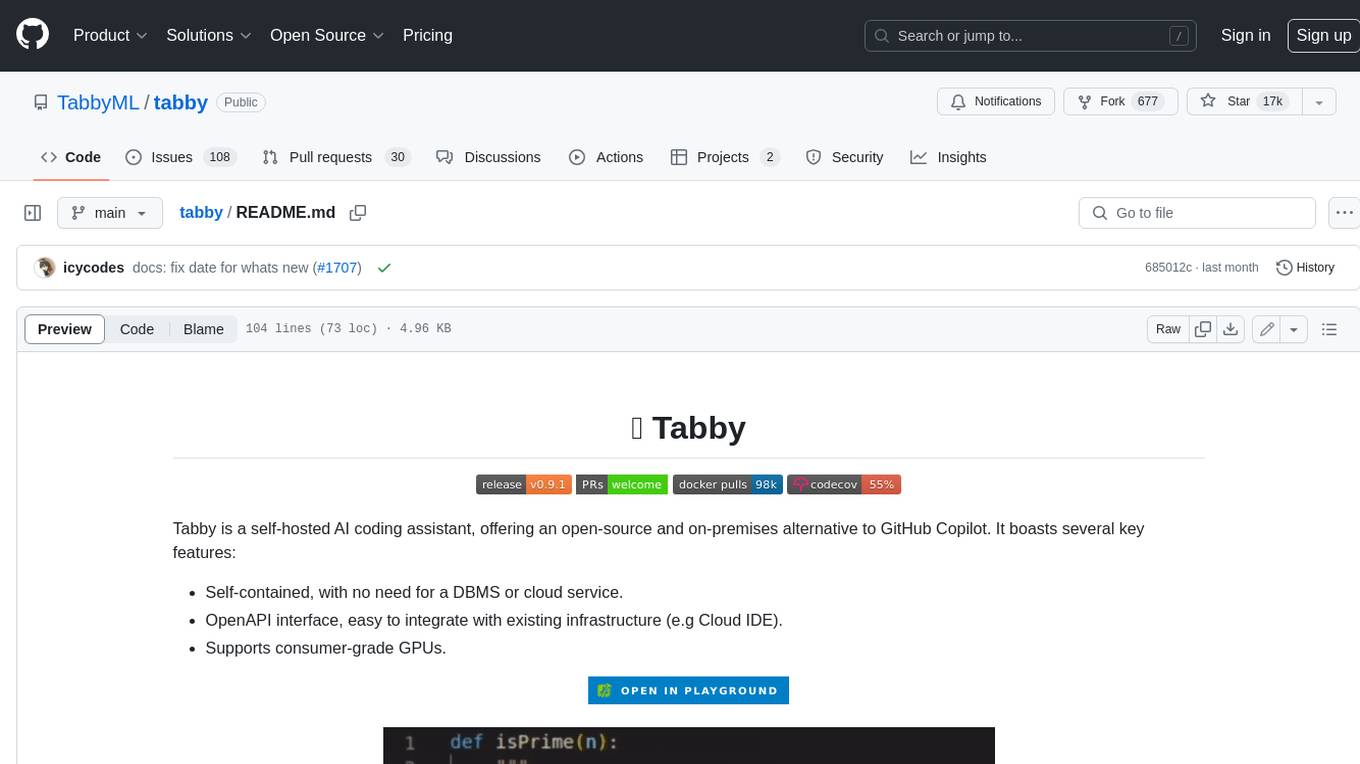
tabby
Tabby is a self-hosted AI coding assistant, offering an open-source and on-premises alternative to GitHub Copilot. It boasts several key features: * Self-contained, with no need for a DBMS or cloud service. * OpenAPI interface, easy to integrate with existing infrastructure (e.g Cloud IDE). * Supports consumer-grade GPUs.
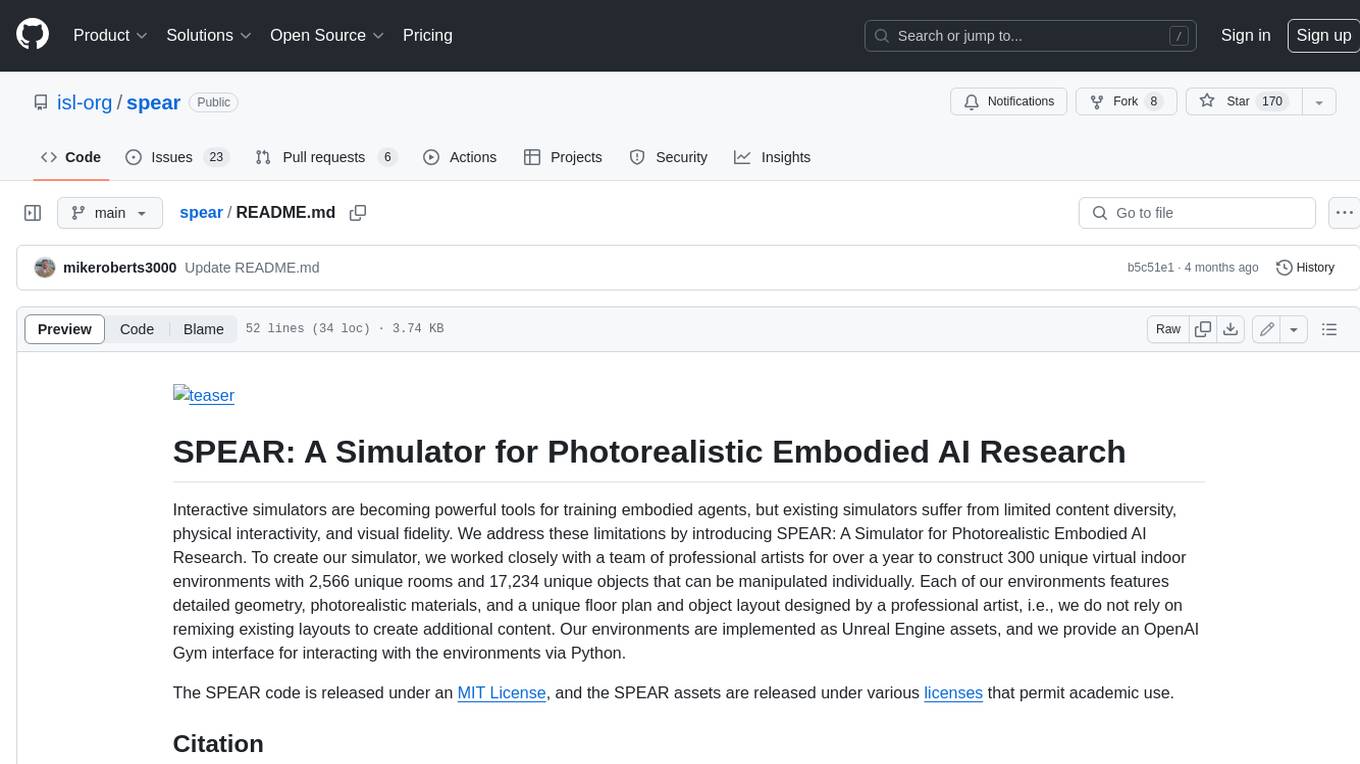
spear
SPEAR (Simulator for Photorealistic Embodied AI Research) is a powerful tool for training embodied agents. It features 300 unique virtual indoor environments with 2,566 unique rooms and 17,234 unique objects that can be manipulated individually. Each environment is designed by a professional artist and features detailed geometry, photorealistic materials, and a unique floor plan and object layout. SPEAR is implemented as Unreal Engine assets and provides an OpenAI Gym interface for interacting with the environments via Python.
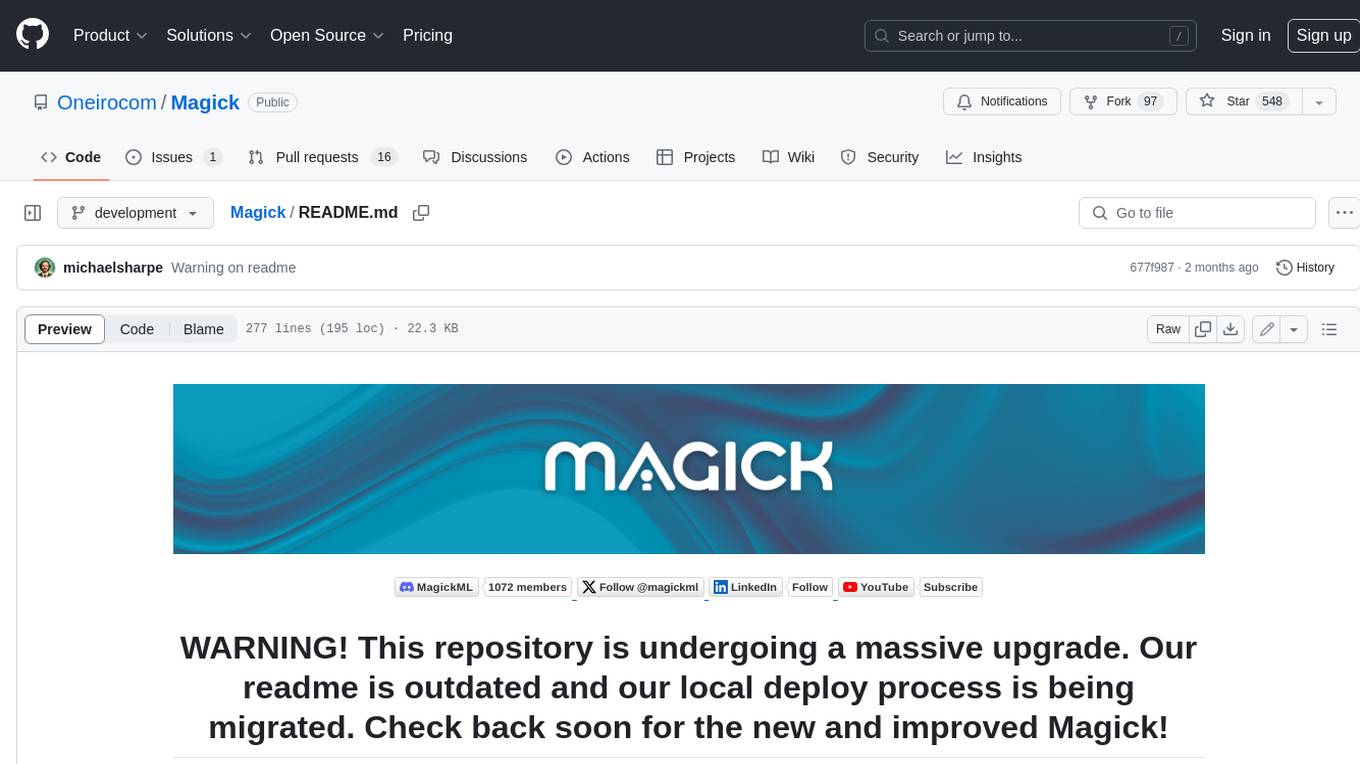
Magick
Magick is a groundbreaking visual AIDE (Artificial Intelligence Development Environment) for no-code data pipelines and multimodal agents. Magick can connect to other services and comes with nodes and templates well-suited for intelligent agents, chatbots, complex reasoning systems and realistic characters.