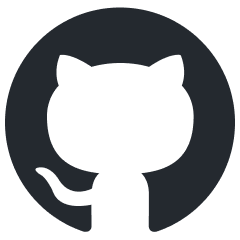
LLM-FuzzX
LLM-FuzzX is a user-friendly fuzz testing tool for Large Language Models (e.g., GPT, Claude, LLaMA), featuring advanced task-aware mutation strategies, fine-grained evaluations, and jailbreak detection to uncover potential security vulnerabilities and enhance model robustness.
Stars: 108
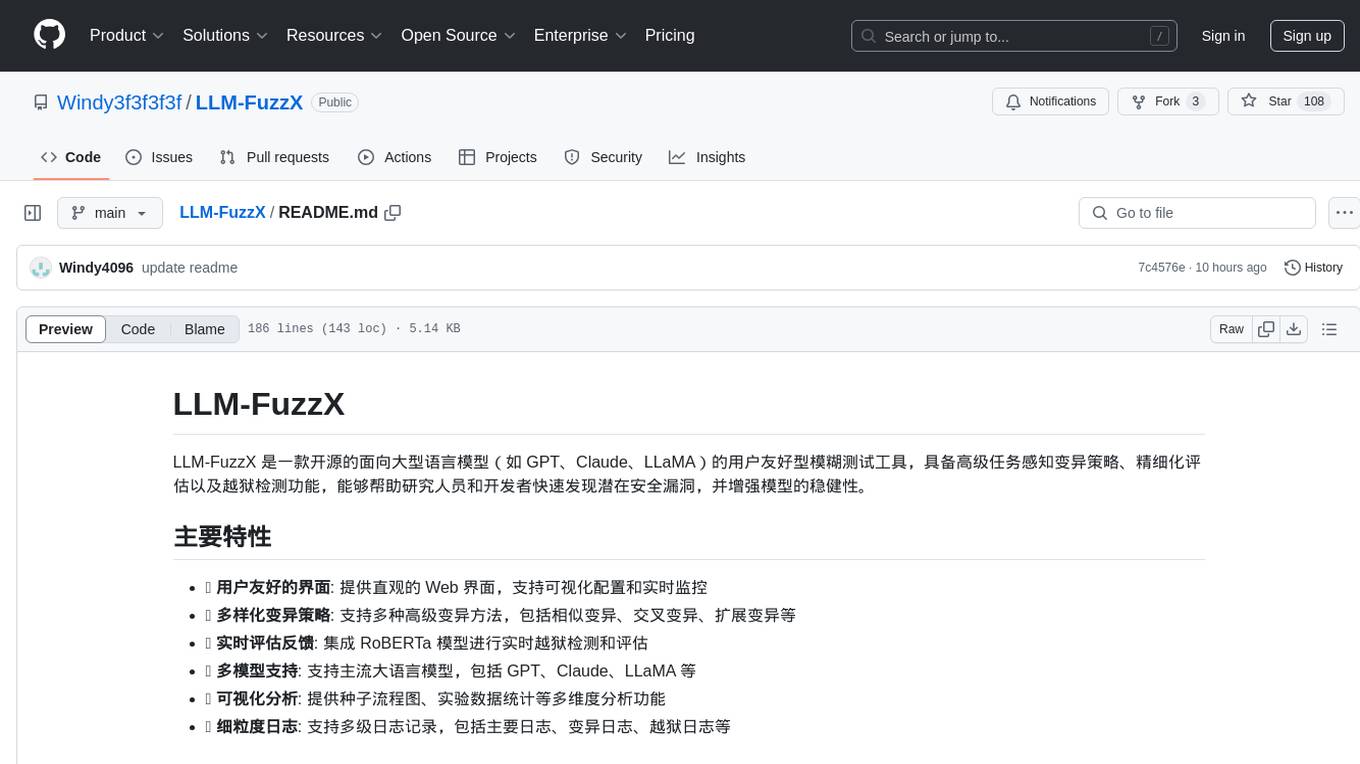
LLM-FuzzX is an open-source user-friendly fuzz testing tool for large language models (e.g., GPT, Claude, LLaMA), equipped with advanced task-aware mutation strategies, fine-grained evaluation, and jailbreak detection capabilities. It helps researchers and developers quickly discover potential security vulnerabilities and enhance model robustness. The tool features a user-friendly web interface for visual configuration and real-time monitoring, supports various advanced mutation methods, integrates RoBERTa model for real-time jailbreak detection and evaluation, supports multiple language models like GPT, Claude, LLaMA, provides visualization analysis with seed flowcharts and experiment data statistics, and offers detailed logging support for main, mutation, and jailbreak logs.
README:
LLM-FuzzX is an open-source, user-friendly fuzzing tool for Large Language Models (like GPT, Claude, LLaMA) featuring advanced task-aware mutation strategies, fine-grained evaluation, and jailbreak detection capabilities. It helps researchers and developers quickly identify potential security vulnerabilities and enhance model robustness. The methodology is primarily based on LLM-Fuzzer.
- π User-Friendly Interface: Intuitive web interface with visual configuration and real-time monitoring
- π Diverse Mutation Strategies: Support for various advanced mutation methods, including similar mutation, crossover mutation, expansion mutation, etc.
- π Real-time Evaluation Feedback: Integrated RoBERTa model for real-time jailbreak detection and evaluation
- π Multi-model Support: Compatible with mainstream LLMs including GPT, Claude, LLaMA, etc.
- π Visualization Analysis: Multi-dimensional analysis with seed flow diagrams and experimental data statistics
- π Fine-grained Logging: Support for multi-level logging, including main logs, mutation logs, jailbreak logs, etc.
LLM-FuzzX adopts a front-end and back-end separated architecture design, consisting of the following core modules:
- Fuzzing Engine: System's central scheduler, coordinating component workflows
- Seed Management: Responsible for seed storage, retrieval, and updates
- Model Interface: Unified model calling interface supporting multiple model implementations
- Evaluation System: RoBERTa-based jailbreak detection and multi-dimensional evaluation
- Similar Mutation: Maintains original template style while generating similar structured variants
- Crossover Mutation: Combines templates selected from the seed pool
- Expansion Mutation: Adds supplementary content to original templates
- Shortening Mutation: Generates more concise variants through compression and refinement
- Restatement Mutation: Rephrases while maintaining semantic meaning
- Target-aware Mutation: Generates variants based on target model characteristics
- Python 3.8+
- Node.js 14+
- CUDA support (for RoBERTa evaluation model)
- 8GB+ system memory
- Stable network connection
# Clone the project
git clone https://github.com/Windy3f3f3f3f/LLM-FuzzX.git
# Create virtual environment
conda create -n llm-fuzzx python=3.10
conda activate llm-fuzzx
# Install dependencies
cd LLM-FuzzX
pip install -r requirements.txt
# Enter frontend directory
cd llm-fuzzer-frontend
# Install dependencies
npm install
# Start development server
npm run serve
- Create
.env
file in project root to configure API keys:
OPENAI_API_KEY=your-openai-key
CLAUDE_API_KEY=your-claude-key
HUGGINGFACE_API_KEY=your-huggingface-key
- Configure model parameters in
config.py
:
MODEL_CONFIG = {
'target_model': 'gpt-3.5-turbo',
'mutator_model': 'gpt-3.5-turbo',
'evaluator_model': 'roberta-base',
'temperature': 0.7,
'max_tokens': 2048
}
# Start backend service
python app.py # Default runs on http://localhost:10003
# Start frontend service
cd llm-fuzzer-frontend
npm run serve # Default runs on http://localhost:10001
- Select target test model (supports GPT, Claude, LLaMA, etc.)
- Prepare test data
- Use preset question sets
- Custom input questions
- Configure test parameters
- Set maximum iteration count
- Select mutation strategies
- Configure evaluation thresholds
- Start testing and monitor in real-time
- View current progress
- Monitor success rate
- Analyze mutation effects
The system provides multi-level logging:
-
main.log
: Main processes and key events -
mutation.log
: Mutation operation records -
jailbreak.log
: Successful jailbreak cases -
error.log
: Errors and exceptions
LLM-FuzzX/
βββ src/ # Backend source code
β βββ api/ # API interfaces
β βββ evaluation/ # Evaluation module
β βββ fuzzing/ # Fuzzing core
β βββ models/ # Model wrappers
β βββ utils/ # Utility functions
βββ llm-fuzzer-frontend/ # Frontend code
βββ scripts/ # Helper scripts
βββ data/ # Data files
βββ logs/ # Log files
-
Test Scale Settings
- Recommended to limit single test iterations to under 1000
- Start with small-scale trials for new scenarios
- Adjust concurrency based on available resources
-
Mutation Strategy Selection
- Prefer single mutation strategy for simple scenarios
- Combine multiple mutation methods for complex scenarios
- Maintain balance in mutation intensity
-
Resource Optimization
- Set reasonable API call intervals
- Clean historical records periodically
- Monitor system resource usage
Welcome to participate in the project through:
- Submit Issues
- Report bugs
- Suggest new features
- Share usage experiences
- Submit Pull Requests
- Fix issues
- Add features
- Improve documentation
- Methodology Contributions
- Provide new mutation strategies
- Design innovative evaluation methods
- Share testing experiences
This project is licensed under the MIT License. See the LICENSE file for details.
- Issue: GitHub Issues
- Email: [email protected]
[1] Yu, J., Lin, X., Yu, Z., & Xing, X. (2024). LLM-Fuzzer: Scaling Assessment of Large Language Model Jailbreaks. In 33rd USENIX Security Symposium (USENIX Security 24) (pp. 4657-4674). USENIX Association.
For Tasks:
Click tags to check more tools for each tasksFor Jobs:
Alternative AI tools for LLM-FuzzX
Similar Open Source Tools
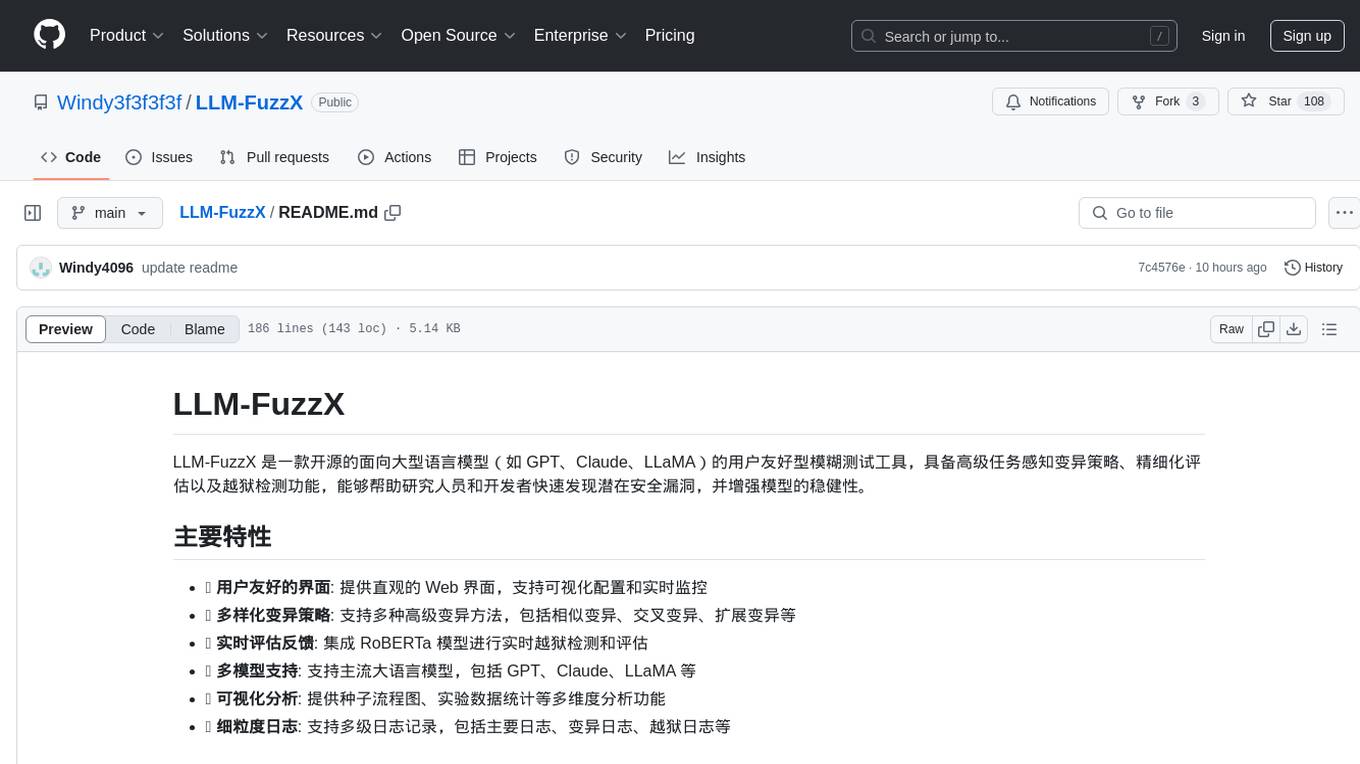
LLM-FuzzX
LLM-FuzzX is an open-source user-friendly fuzz testing tool for large language models (e.g., GPT, Claude, LLaMA), equipped with advanced task-aware mutation strategies, fine-grained evaluation, and jailbreak detection capabilities. It helps researchers and developers quickly discover potential security vulnerabilities and enhance model robustness. The tool features a user-friendly web interface for visual configuration and real-time monitoring, supports various advanced mutation methods, integrates RoBERTa model for real-time jailbreak detection and evaluation, supports multiple language models like GPT, Claude, LLaMA, provides visualization analysis with seed flowcharts and experiment data statistics, and offers detailed logging support for main, mutation, and jailbreak logs.
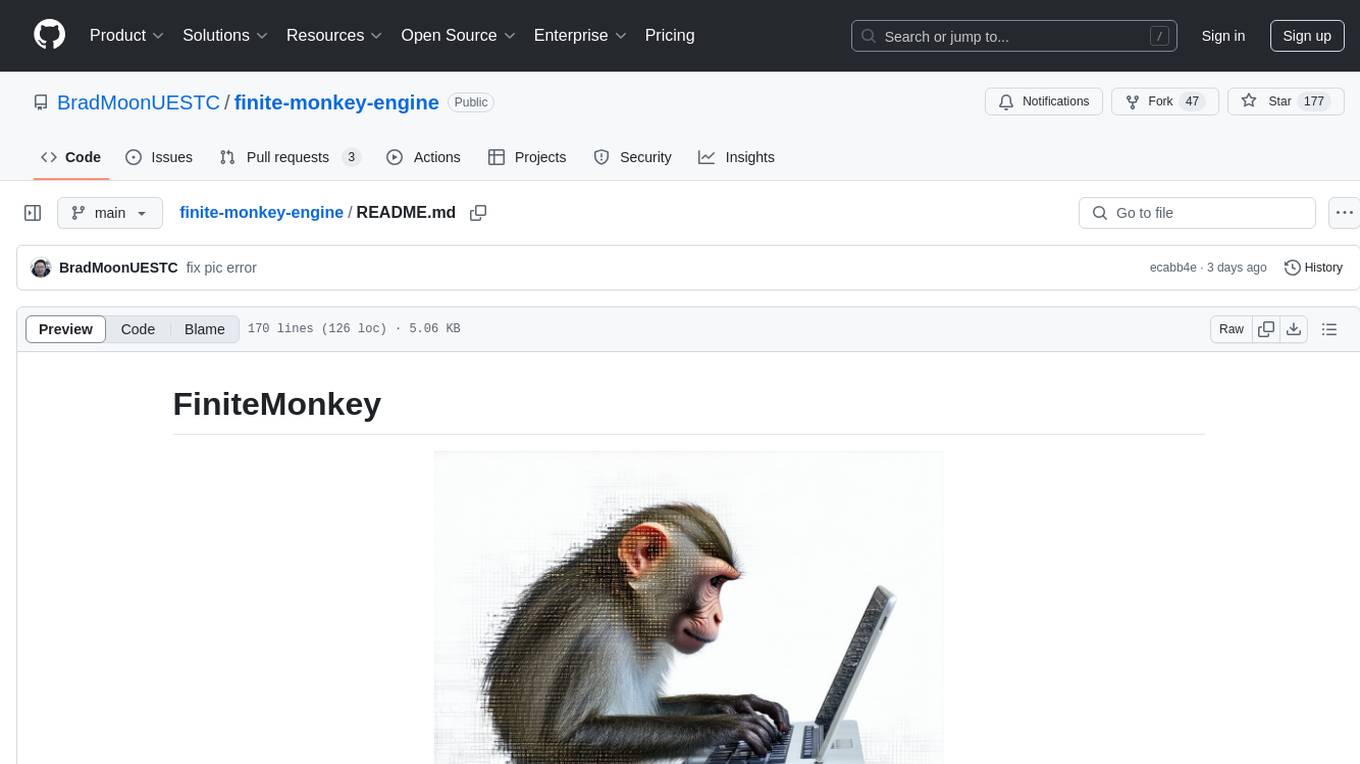
finite-monkey-engine
FiniteMonkey is an advanced vulnerability mining engine powered purely by GPT, requiring no prior knowledge base or fine-tuning. Its effectiveness significantly surpasses most current related research approaches. The tool is task-driven, prompt-driven, and focuses on prompt design, leveraging 'deception' and hallucination as key mechanics. It has helped identify vulnerabilities worth over $60,000 in bounties. The tool requires PostgreSQL database, OpenAI API access, and Python environment for setup. It supports various languages like Solidity, Rust, Python, Move, Cairo, Tact, Func, Java, and Fake Solidity for scanning. FiniteMonkey is best suited for logic vulnerability mining in real projects, not recommended for academic vulnerability testing. GPT-4-turbo is recommended for optimal results with an average scan time of 2-3 hours for medium projects. The tool provides detailed scanning results guide and implementation tips for users.
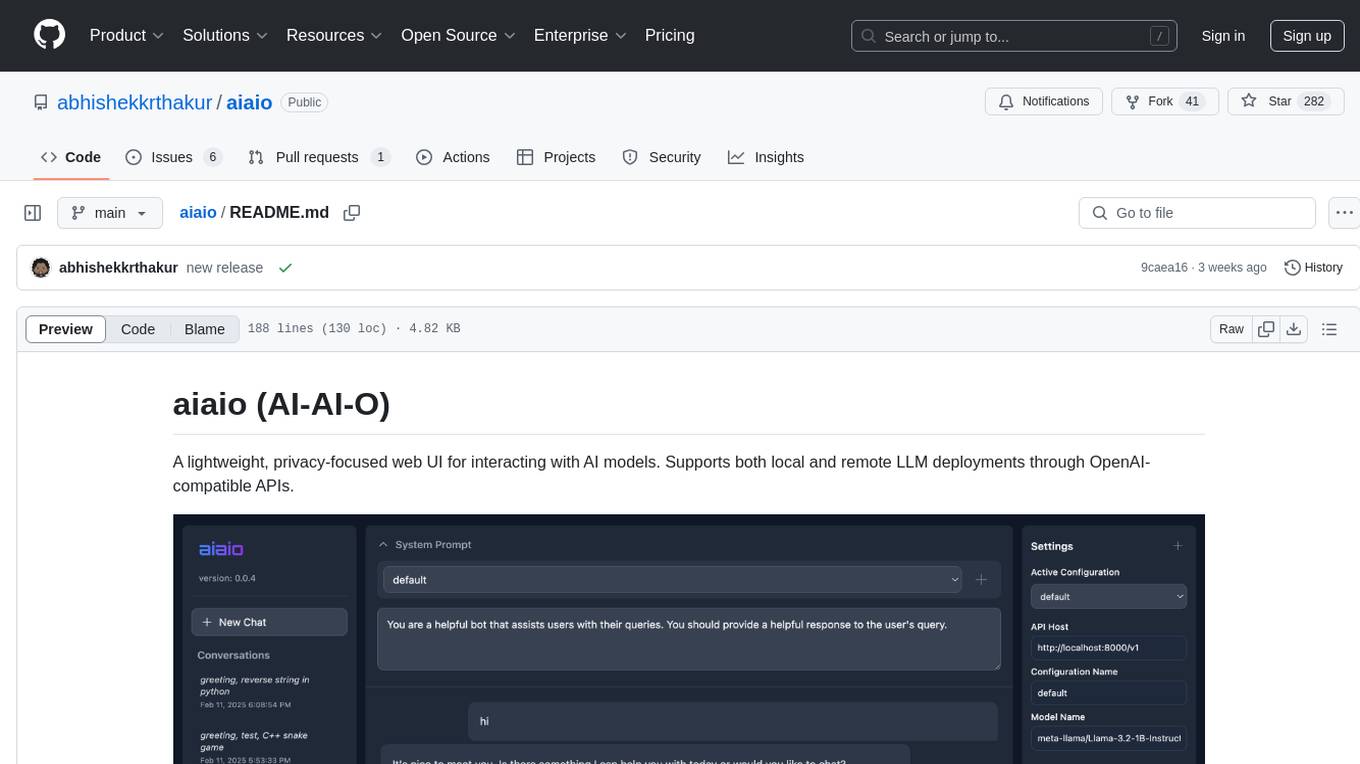
aiaio
aiaio (AI-AI-O) is a lightweight, privacy-focused web UI for interacting with AI models. It supports both local and remote LLM deployments through OpenAI-compatible APIs. The tool provides features such as dark/light mode support, local SQLite database for conversation storage, file upload and processing, configurable model parameters through UI, privacy-focused design, responsive design for mobile/desktop, syntax highlighting for code blocks, real-time conversation updates, automatic conversation summarization, customizable system prompts, WebSocket support for real-time updates, Docker support for deployment, multiple API endpoint support, and multiple system prompt support. Users can configure model parameters and API settings through the UI, handle file uploads, manage conversations, and use keyboard shortcuts for efficient interaction. The tool uses SQLite for storage with tables for conversations, messages, attachments, and settings. Contributions to the project are welcome under the Apache License 2.0.
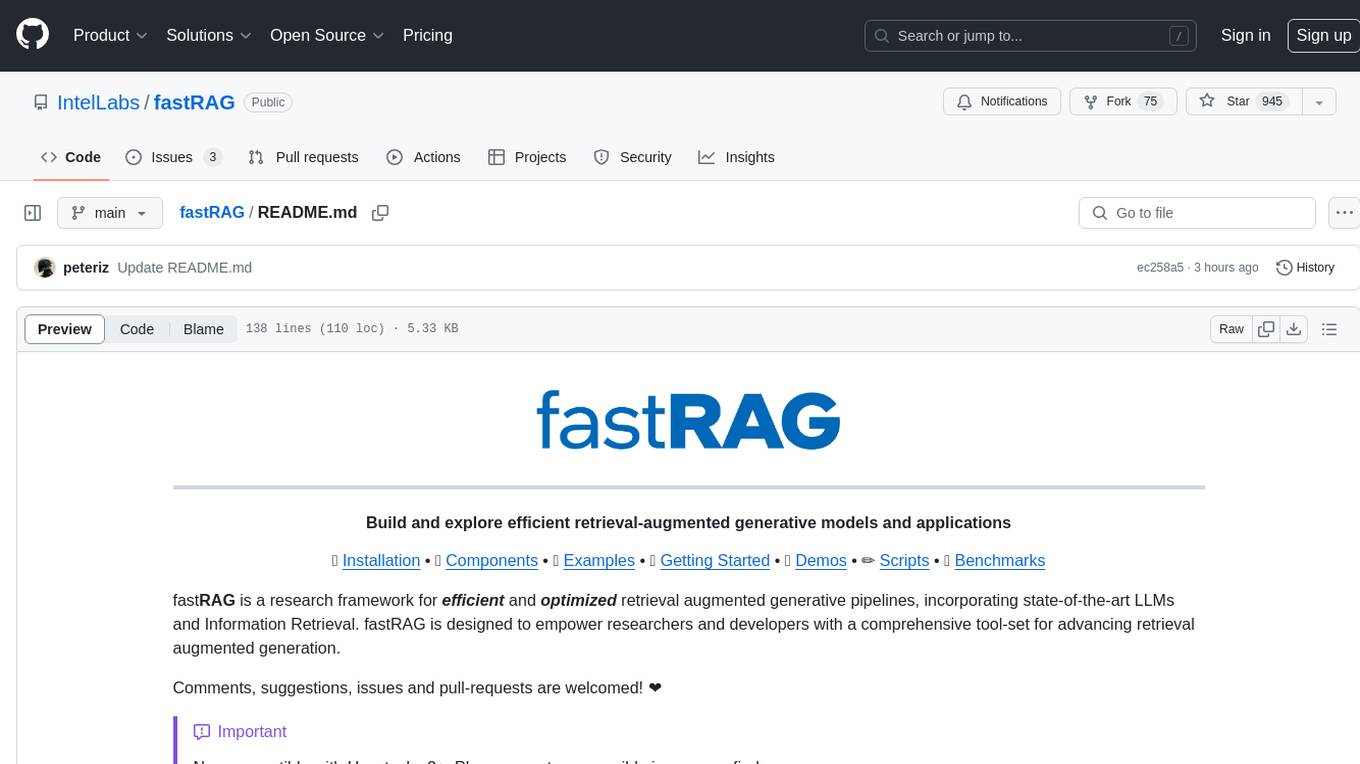
fastRAG
fastRAG is a research framework designed to build and explore efficient retrieval-augmented generative models. It incorporates state-of-the-art Large Language Models (LLMs) and Information Retrieval to empower researchers and developers with a comprehensive tool-set for advancing retrieval augmented generation. The framework is optimized for Intel hardware, customizable, and includes key features such as optimized RAG pipelines, efficient components, and RAG-efficient components like ColBERT and Fusion-in-Decoder (FiD). fastRAG supports various unique components and backends for running LLMs, making it a versatile tool for research and development in the field of retrieval-augmented generation.
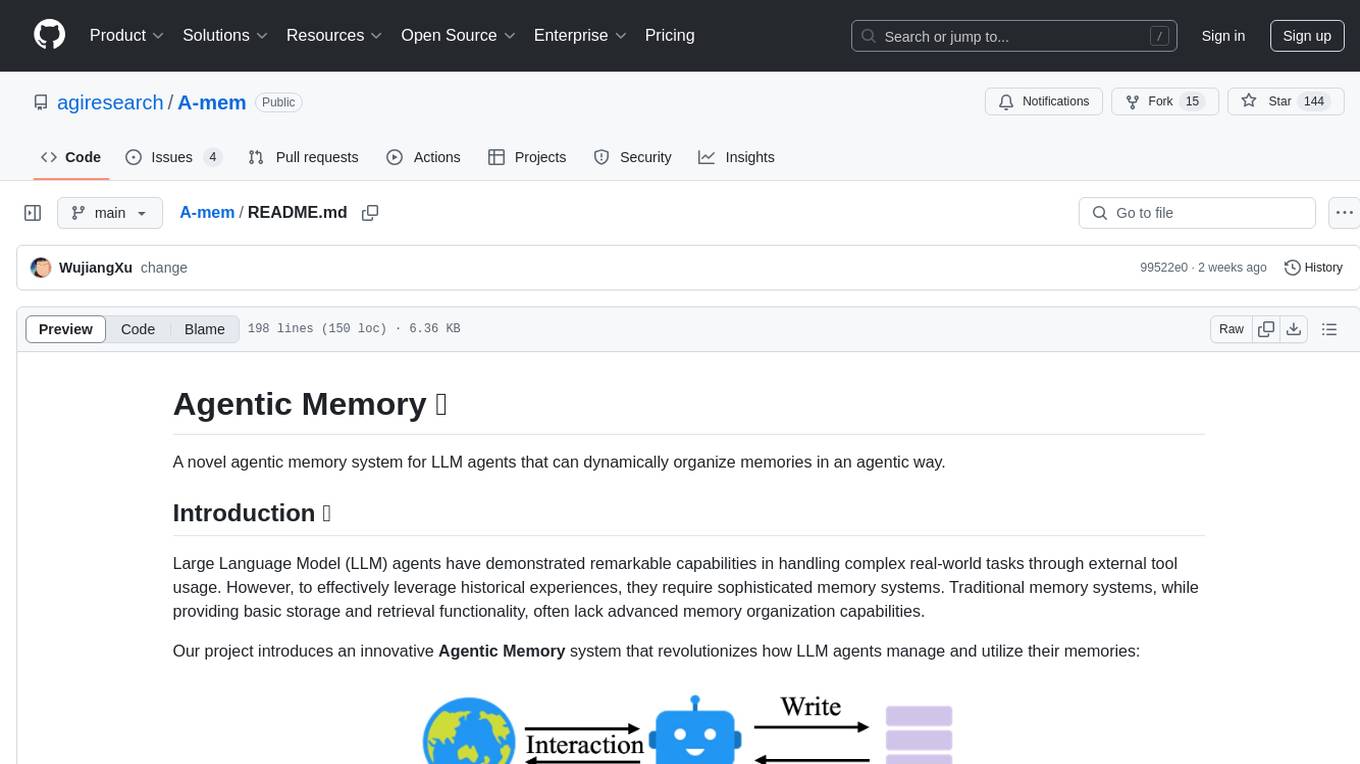
A-mem
A-MEM is a novel agentic memory system designed for Large Language Model (LLM) agents to dynamically organize memories in an agentic way. It introduces advanced memory organization capabilities, intelligent indexing, and linking of memories, comprehensive note generation, interconnected knowledge networks, continuous memory evolution, and agent-driven decision making for adaptive memory management. The system facilitates agent construction and enables dynamic memory operations and flexible agent-memory interactions.
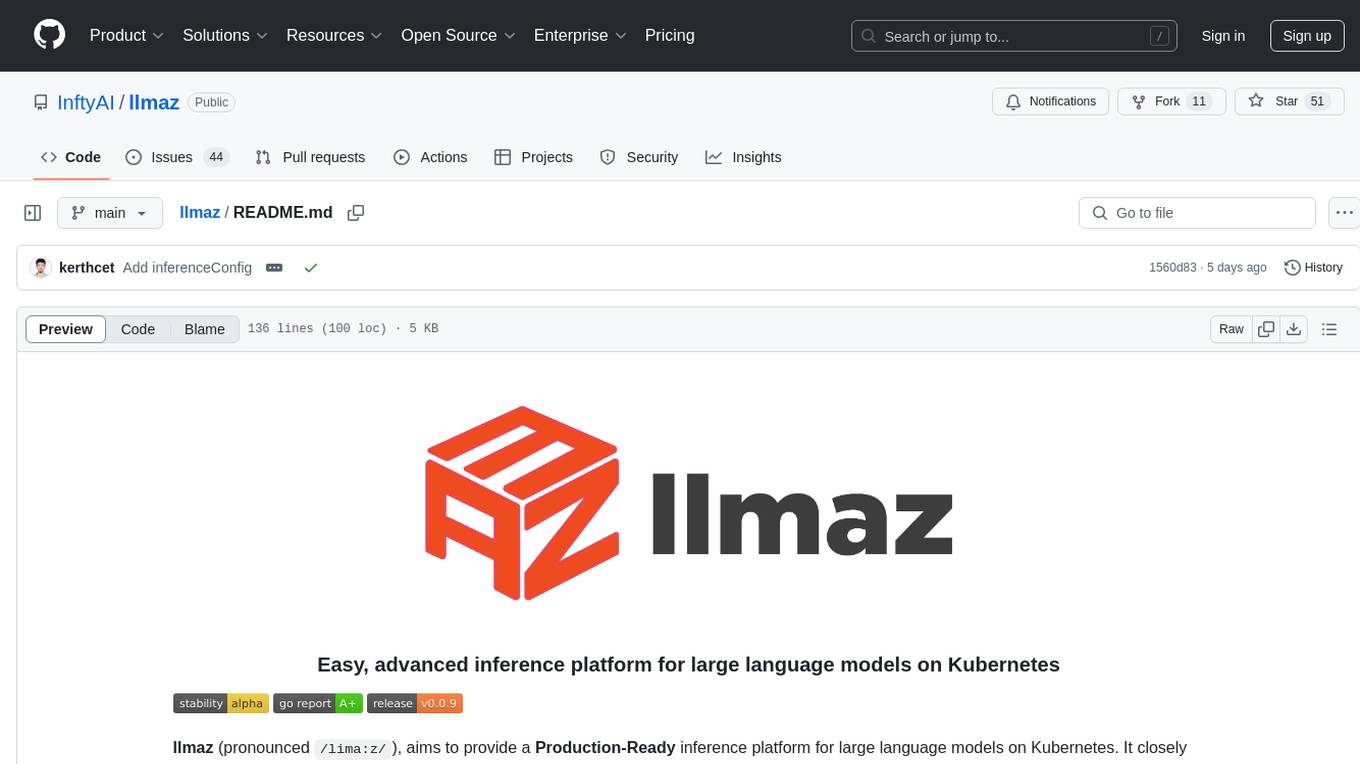
llmaz
llmaz is an easy, advanced inference platform for large language models on Kubernetes. It aims to provide a production-ready solution that integrates with state-of-the-art inference backends. The platform supports efficient model distribution, accelerator fungibility, SOTA inference, various model providers, multi-host support, and scaling efficiency. Users can quickly deploy LLM services with minimal configurations and benefit from a wide range of advanced inference backends. llmaz is designed to optimize cost and performance while supporting cutting-edge researches like Speculative Decoding or Splitwise on Kubernetes.
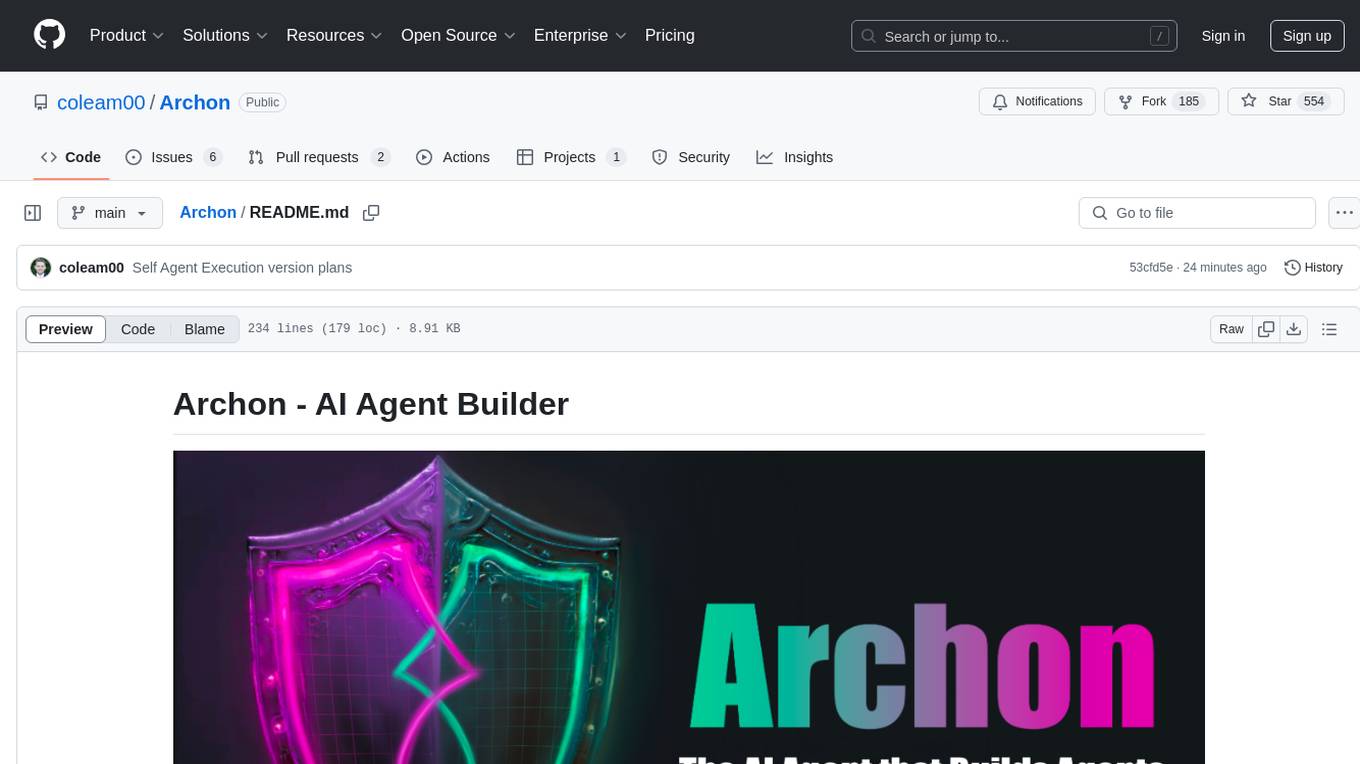
Archon
Archon is an AI meta-agent designed to autonomously build, refine, and optimize other AI agents. It serves as a practical tool for developers and an educational framework showcasing the evolution of agentic systems. Through iterative development, Archon demonstrates the power of planning, feedback loops, and domain-specific knowledge in creating robust AI agents.
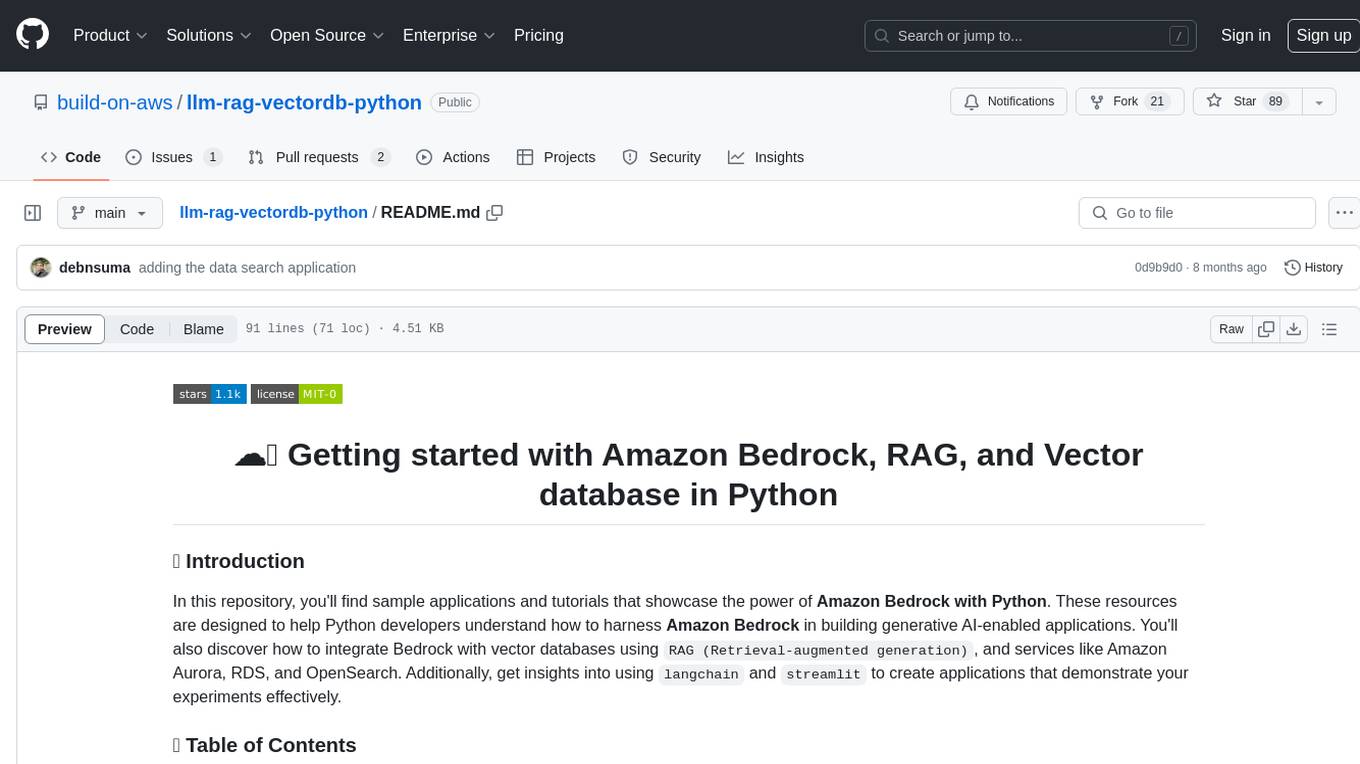
llm-rag-vectordb-python
This repository provides sample applications and tutorials to showcase the power of Amazon Bedrock with Python. It helps Python developers understand how to harness Amazon Bedrock in building generative AI-enabled applications. The resources also demonstrate integration with vector databases using RAG (Retrieval-augmented generation) and services like Amazon Aurora, RDS, and OpenSearch. Additionally, it explores using langchain and streamlit to create effective experimental applications.
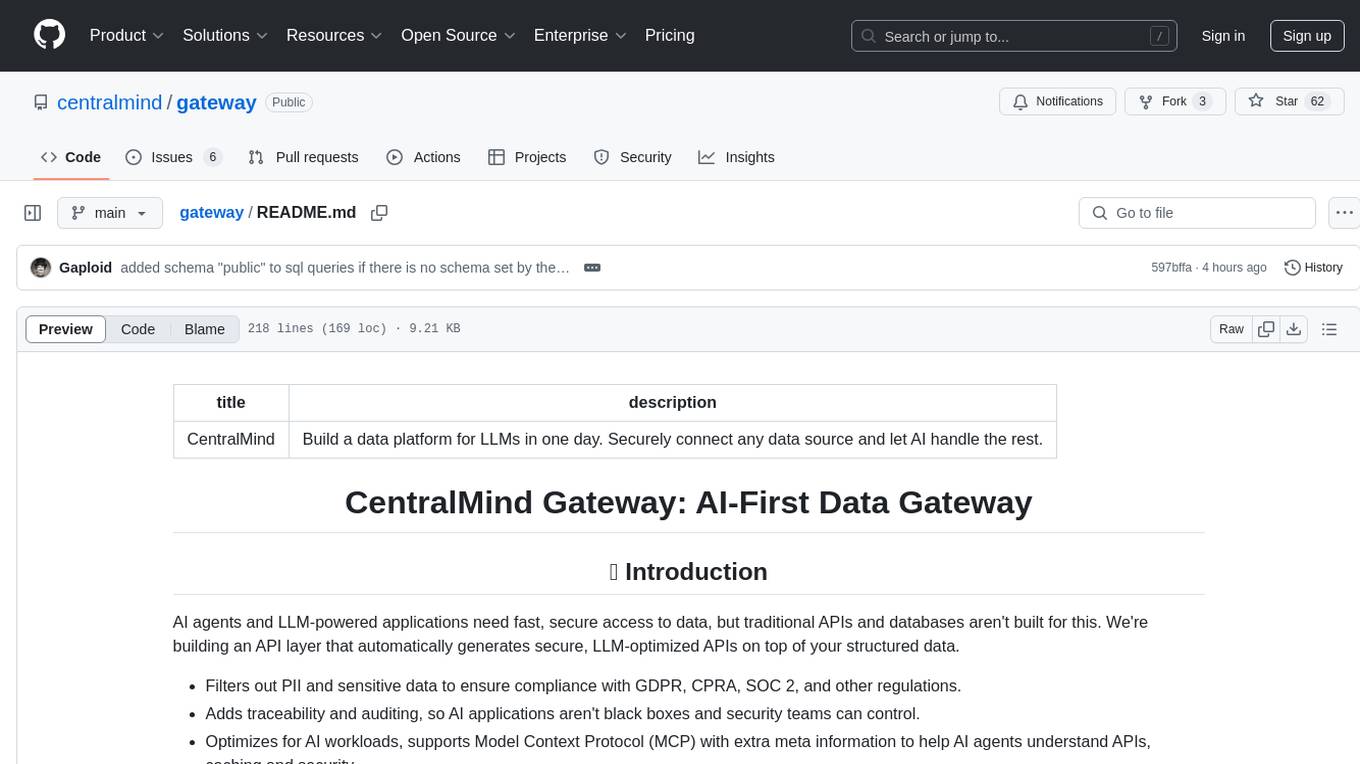
gateway
CentralMind Gateway is an AI-first data gateway that securely connects any data source and automatically generates secure, LLM-optimized APIs. It filters out sensitive data, adds traceability, and optimizes for AI workloads. Suitable for companies deploying AI agents for customer support and analytics.
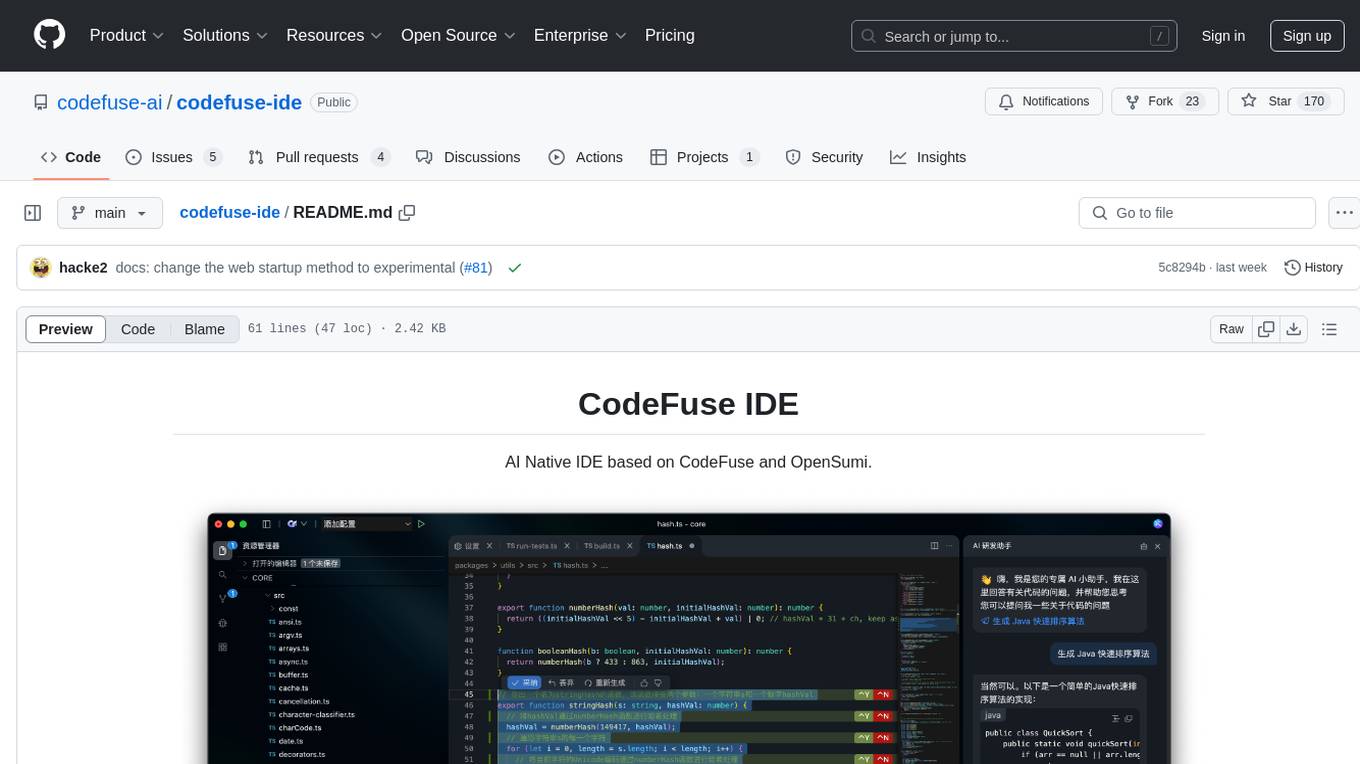
codefuse-ide
CodeFuse IDE is an AI-native integrated development environment that leverages AI technologies to enhance productivity and streamline workflows. It supports seamless integration of various models, enabling developers to customize and extend functionality. The platform is compatible with VS Code extensions, providing access to a rich ecosystem of plugins. CodeFuse IDE uses electron-forge for packaging desktop applications and supports development, building, packaging, and auto updates.
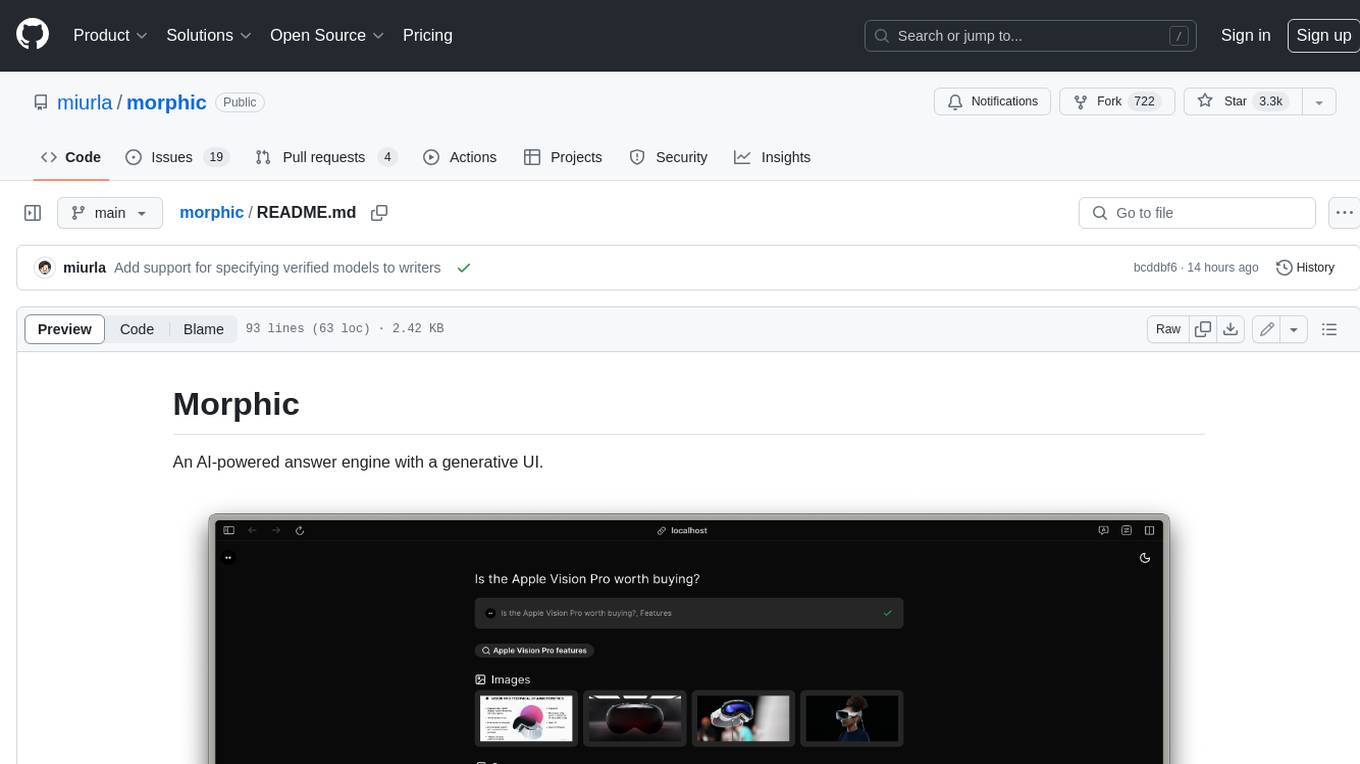
morphic
Morphic is an AI-powered answer engine with a generative UI. It utilizes a stack of Next.js, Vercel AI SDK, OpenAI, Tavily AI, shadcn/ui, Radix UI, and Tailwind CSS. To get started, fork and clone the repo, install dependencies, fill out secrets in the .env.local file, and run the app locally using 'bun dev'. You can also deploy your own live version of Morphic with Vercel. Verified models that can be specified to writers include Groq, LLaMA3 8b, and LLaMA3 70b.
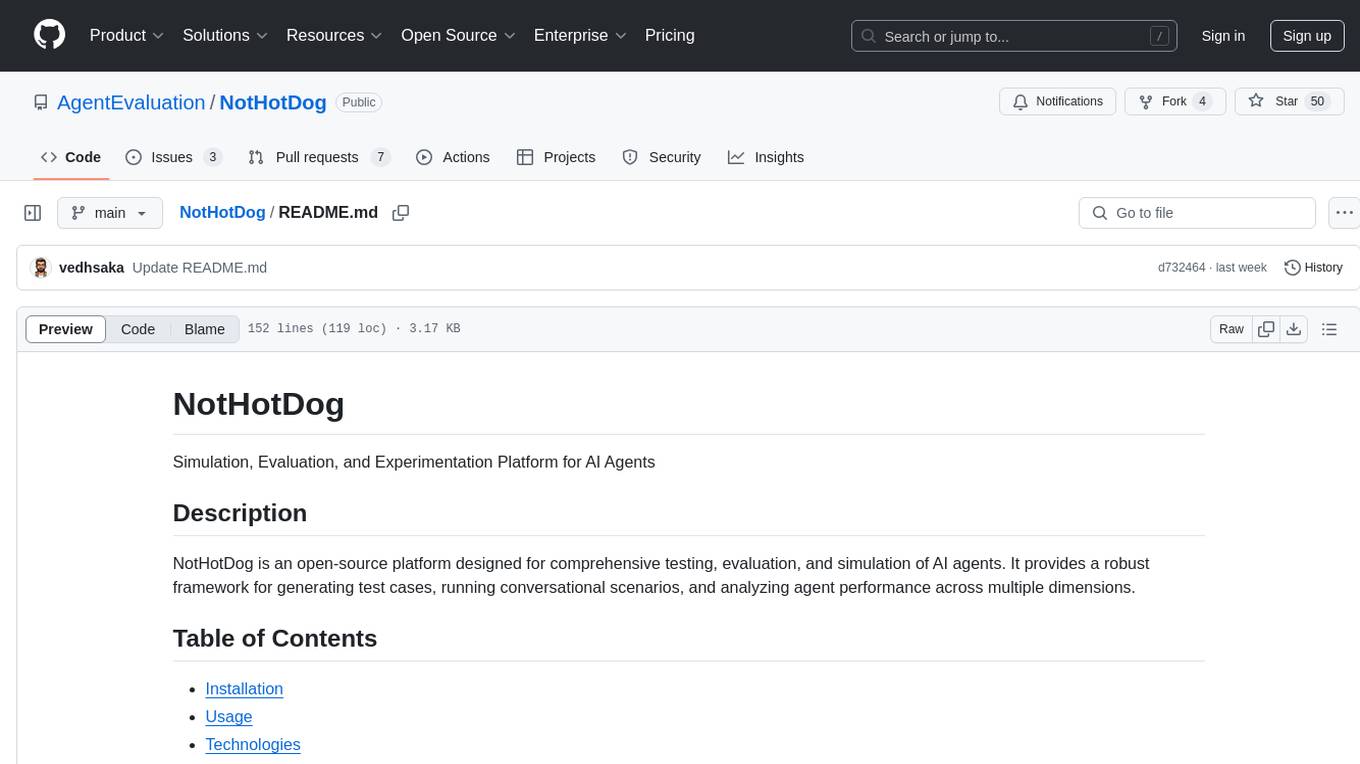
NotHotDog
NotHotDog is an open-source platform for testing, evaluating, and simulating AI agents. It offers a robust framework for generating test cases, running conversational scenarios, and analyzing agent performance.
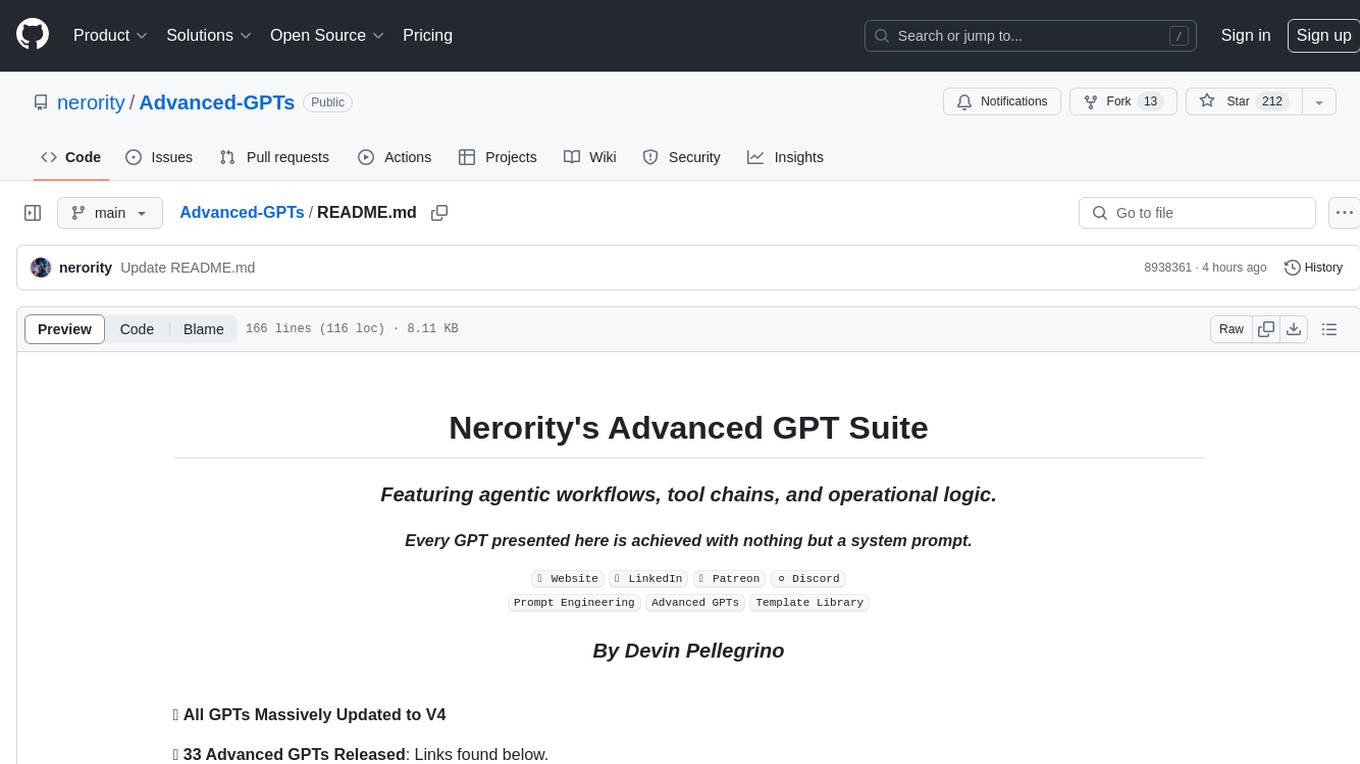
Advanced-GPTs
Nerority's Advanced GPT Suite is a collection of 33 GPTs that can be controlled with natural language prompts. The suite includes tools for various tasks such as strategic consulting, business analysis, career profile building, content creation, educational purposes, image-based tasks, knowledge engineering, marketing, persona creation, programming, prompt engineering, role-playing, simulations, and task management. Users can access links, usage instructions, and guides for each GPT on their respective pages. The suite is designed for public demonstration and usage, offering features like meta-sequence optimization, AI priming, prompt classification, and optimization. It also provides tools for generating articles, analyzing contracts, visualizing data, distilling knowledge, creating educational content, exploring topics, generating marketing copy, simulating scenarios, managing tasks, and more.
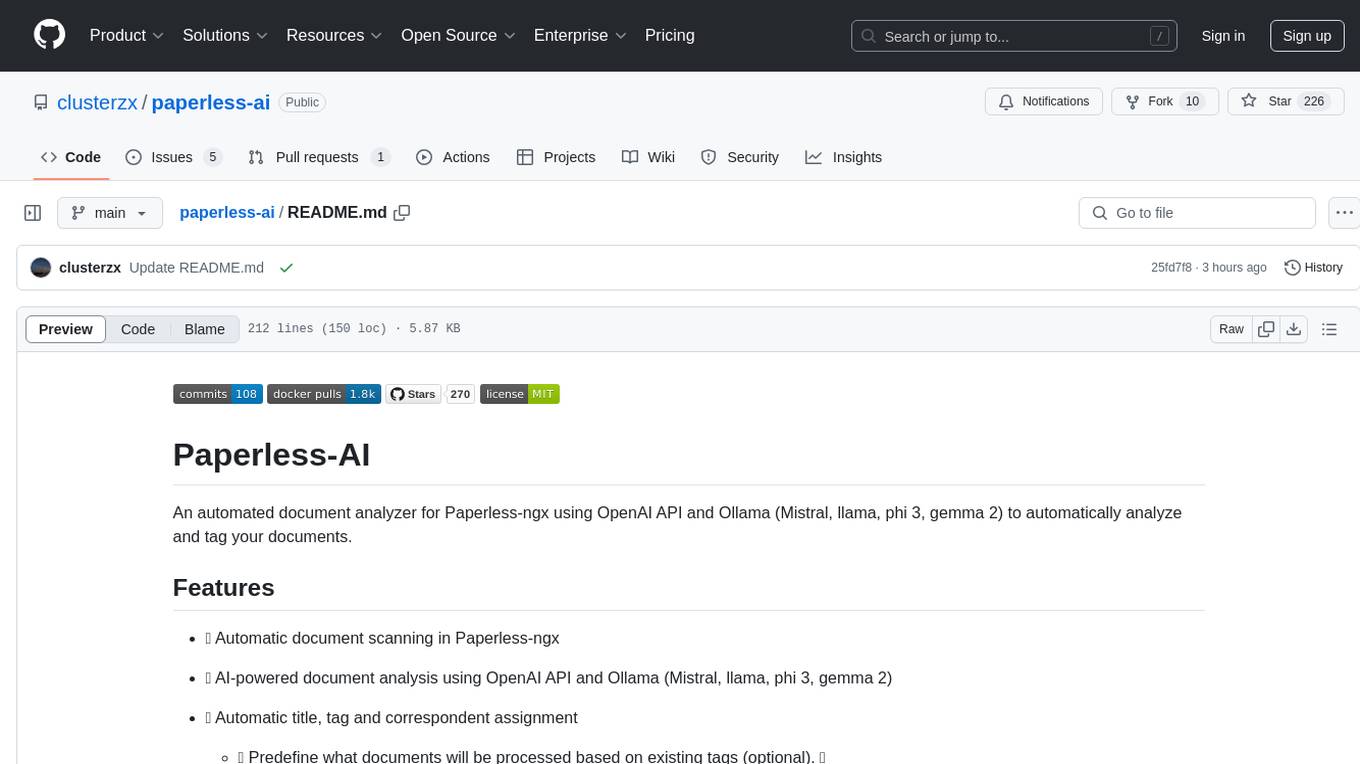
paperless-ai
Paperless-AI is an automated document analyzer tool designed for Paperless-ngx users. It utilizes the OpenAI API and Ollama (Mistral, llama, phi 3, gemma 2) to automatically scan, analyze, and tag documents. The tool offers features such as automatic document scanning, AI-powered document analysis, automatic title and tag assignment, manual mode for analyzing documents, easy setup through a web interface, document processing dashboard, error handling, and Docker support. Users can configure the tool through a web interface and access a debug interface for monitoring and troubleshooting. Paperless-AI aims to streamline document organization and analysis processes for users with access to Paperless-ngx and AI capabilities.
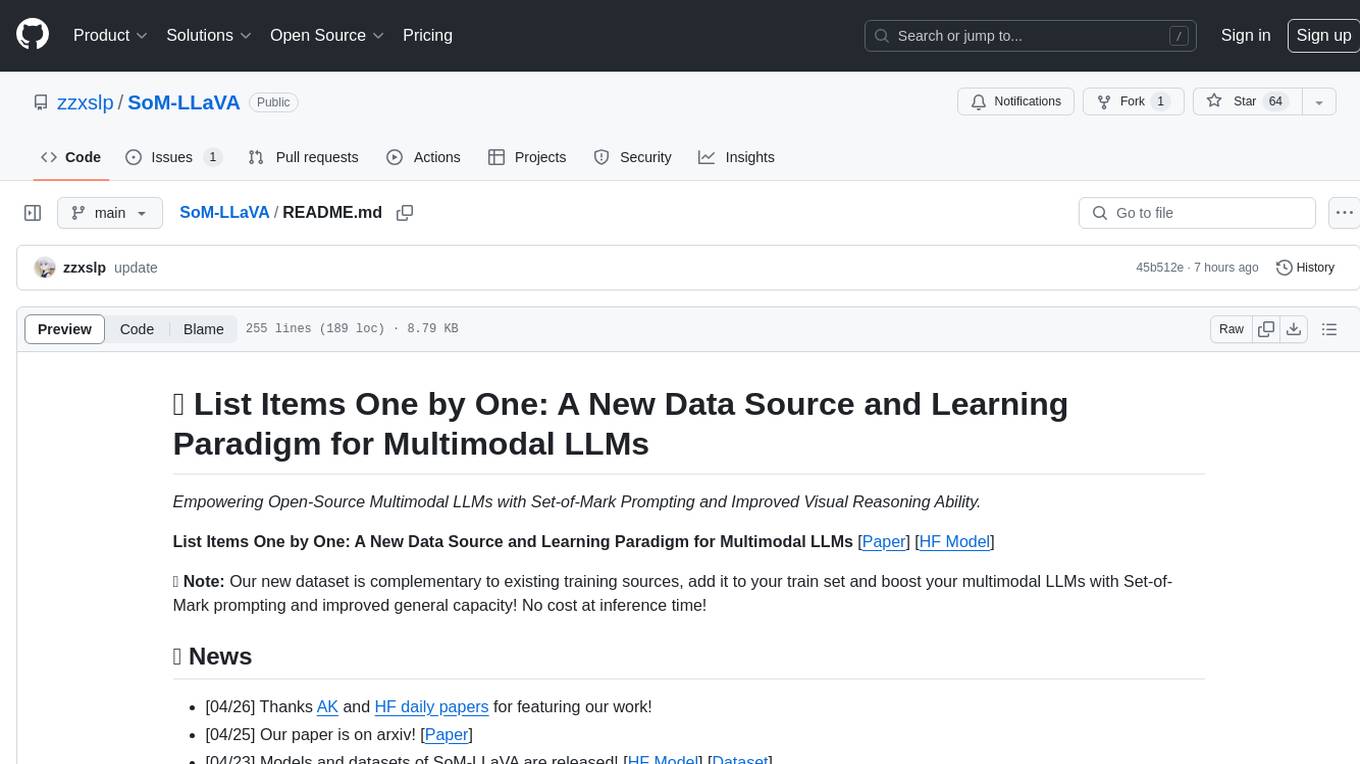
SoM-LLaVA
SoM-LLaVA is a new data source and learning paradigm for Multimodal LLMs, empowering open-source Multimodal LLMs with Set-of-Mark prompting and improved visual reasoning ability. The repository provides a new dataset that is complementary to existing training sources, enhancing multimodal LLMs with Set-of-Mark prompting and improved general capacity. By adding 30k SoM data to the visual instruction tuning stage of LLaVA, the tool achieves 1% to 6% relative improvements on all benchmarks. Users can train SoM-LLaVA via command line and utilize the implementation to annotate COCO images with SoM. Additionally, the tool can be loaded in Huggingface for further usage.
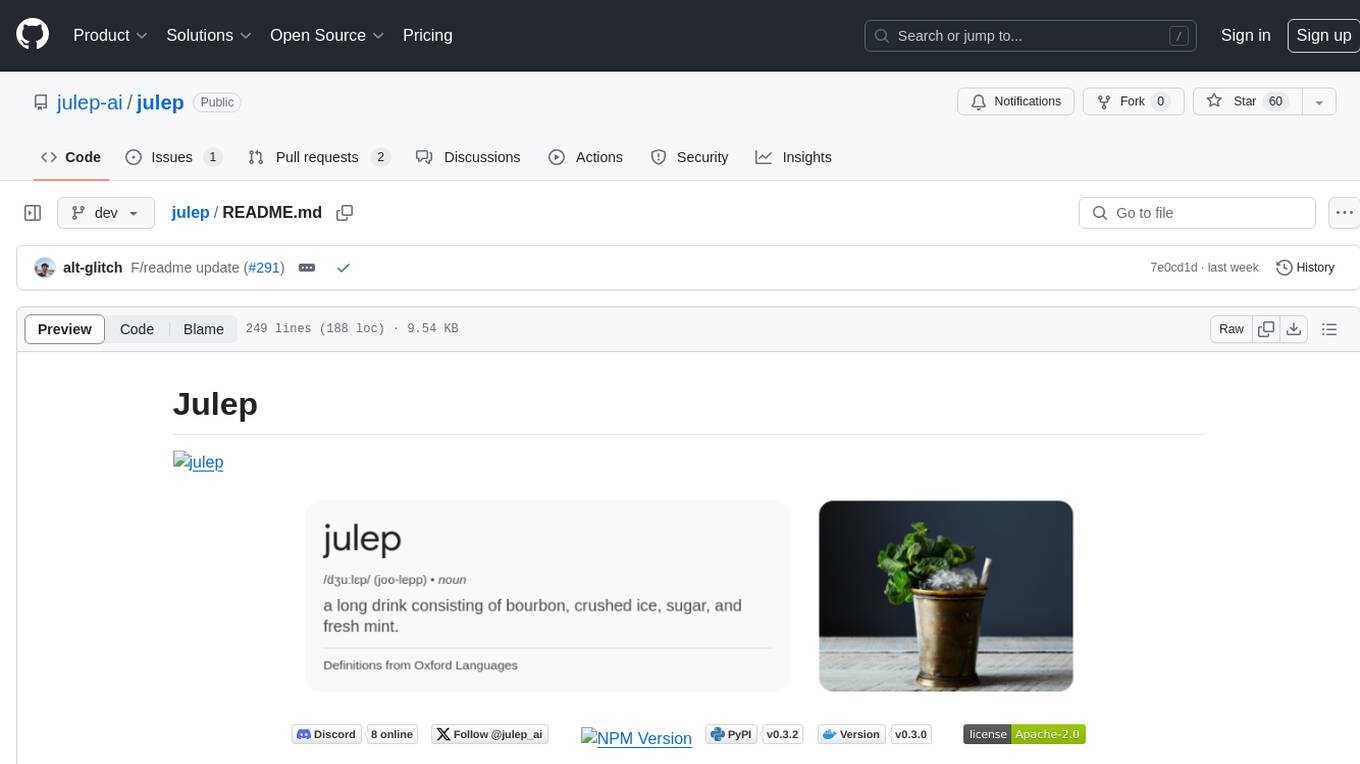
julep
Julep is an advanced platform for creating stateful and functional AI apps powered by large language models. It offers features like statefulness by design, automatic function calling, production-ready deployment, cron-like asynchronous functions, 90+ built-in tools, and the ability to switch between different LLMs easily. Users can build AI applications without the need to write code for embedding, saving, and retrieving conversation history, and can connect to third-party applications using Composio. Julep simplifies the process of getting started with AI apps, whether they are conversational, functional, or agentic.
For similar tasks
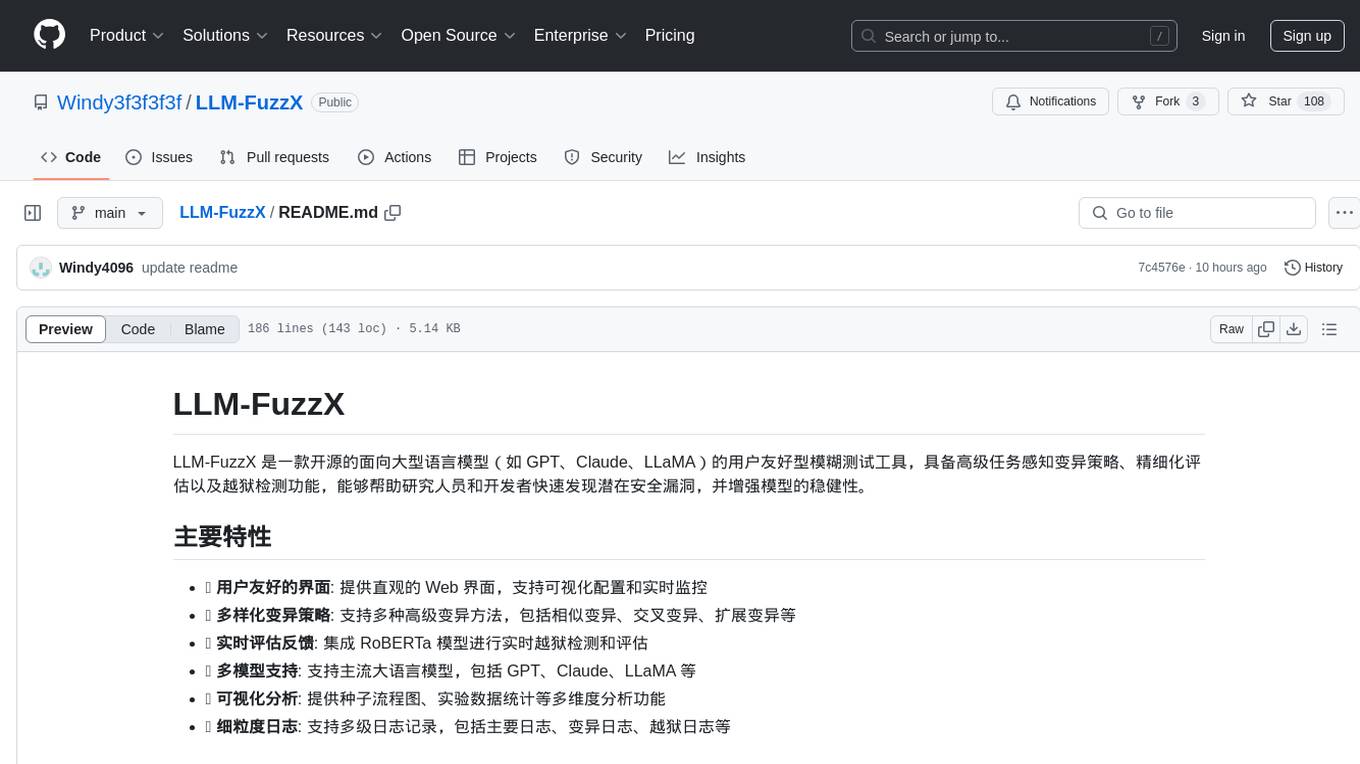
LLM-FuzzX
LLM-FuzzX is an open-source user-friendly fuzz testing tool for large language models (e.g., GPT, Claude, LLaMA), equipped with advanced task-aware mutation strategies, fine-grained evaluation, and jailbreak detection capabilities. It helps researchers and developers quickly discover potential security vulnerabilities and enhance model robustness. The tool features a user-friendly web interface for visual configuration and real-time monitoring, supports various advanced mutation methods, integrates RoBERTa model for real-time jailbreak detection and evaluation, supports multiple language models like GPT, Claude, LLaMA, provides visualization analysis with seed flowcharts and experiment data statistics, and offers detailed logging support for main, mutation, and jailbreak logs.
For similar jobs
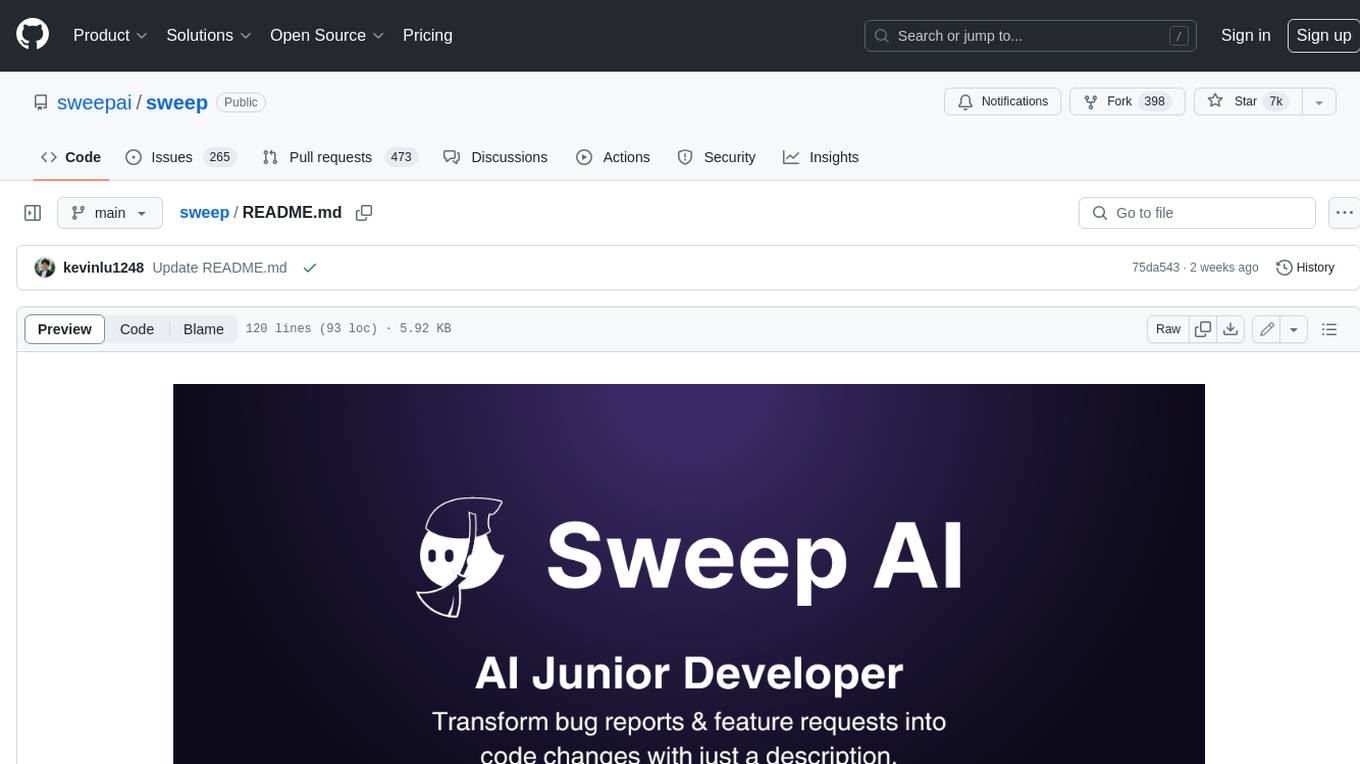
sweep
Sweep is an AI junior developer that turns bugs and feature requests into code changes. It automatically handles developer experience improvements like adding type hints and improving test coverage.
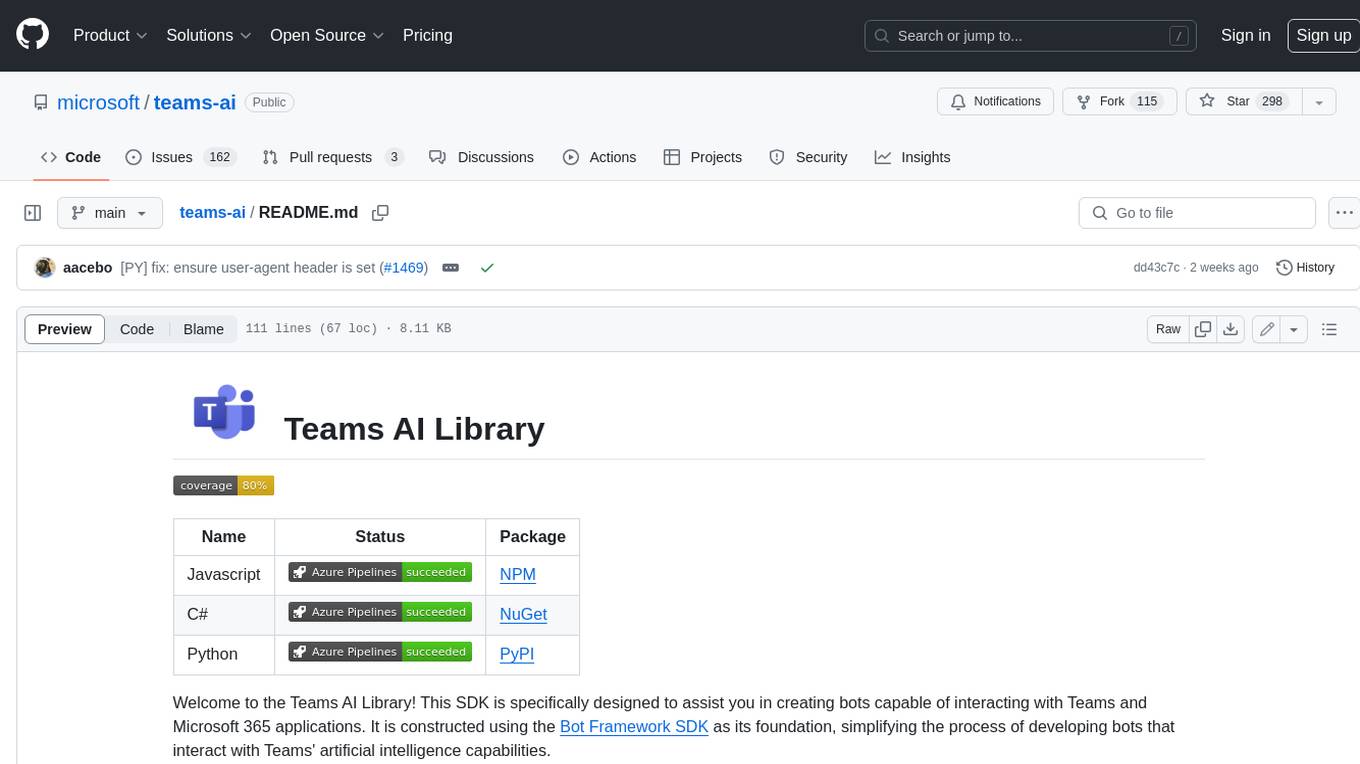
teams-ai
The Teams AI Library is a software development kit (SDK) that helps developers create bots that can interact with Teams and Microsoft 365 applications. It is built on top of the Bot Framework SDK and simplifies the process of developing bots that interact with Teams' artificial intelligence capabilities. The SDK is available for JavaScript/TypeScript, .NET, and Python.
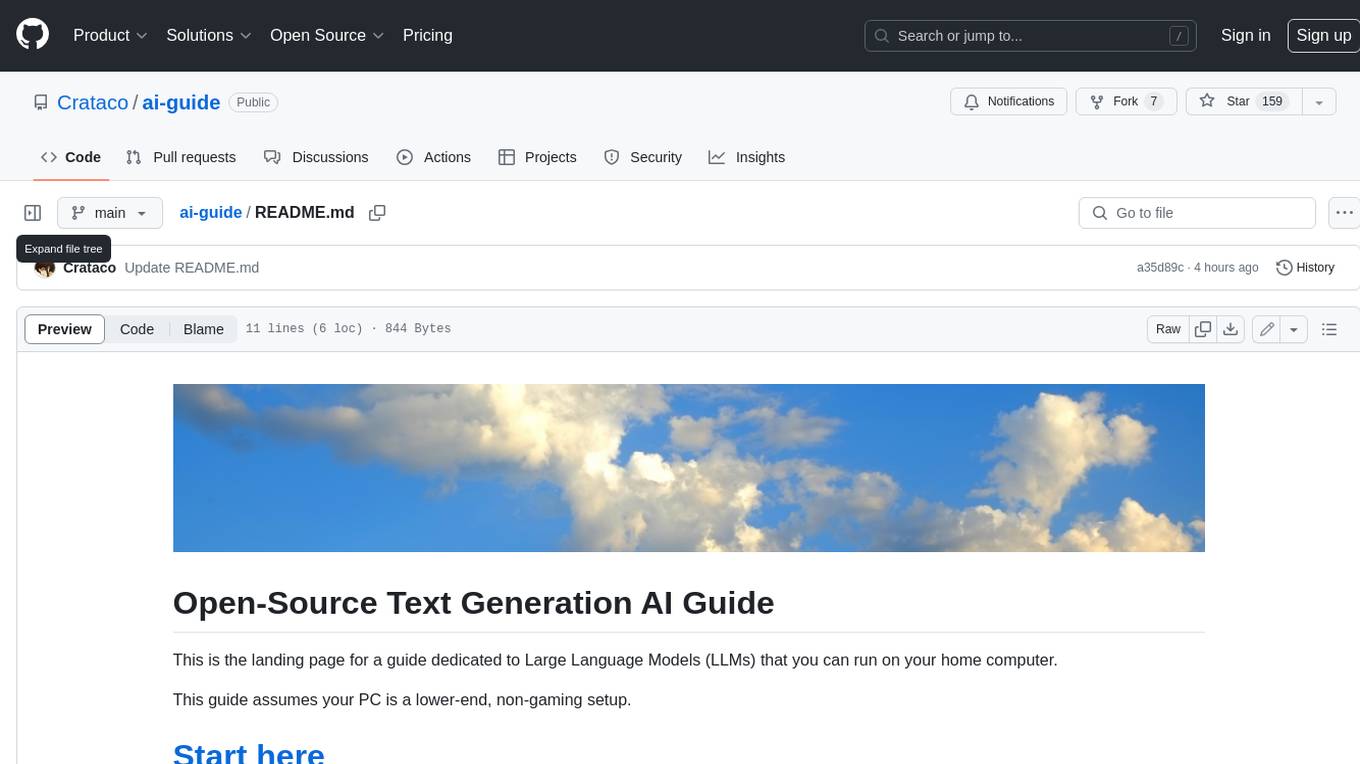
ai-guide
This guide is dedicated to Large Language Models (LLMs) that you can run on your home computer. It assumes your PC is a lower-end, non-gaming setup.
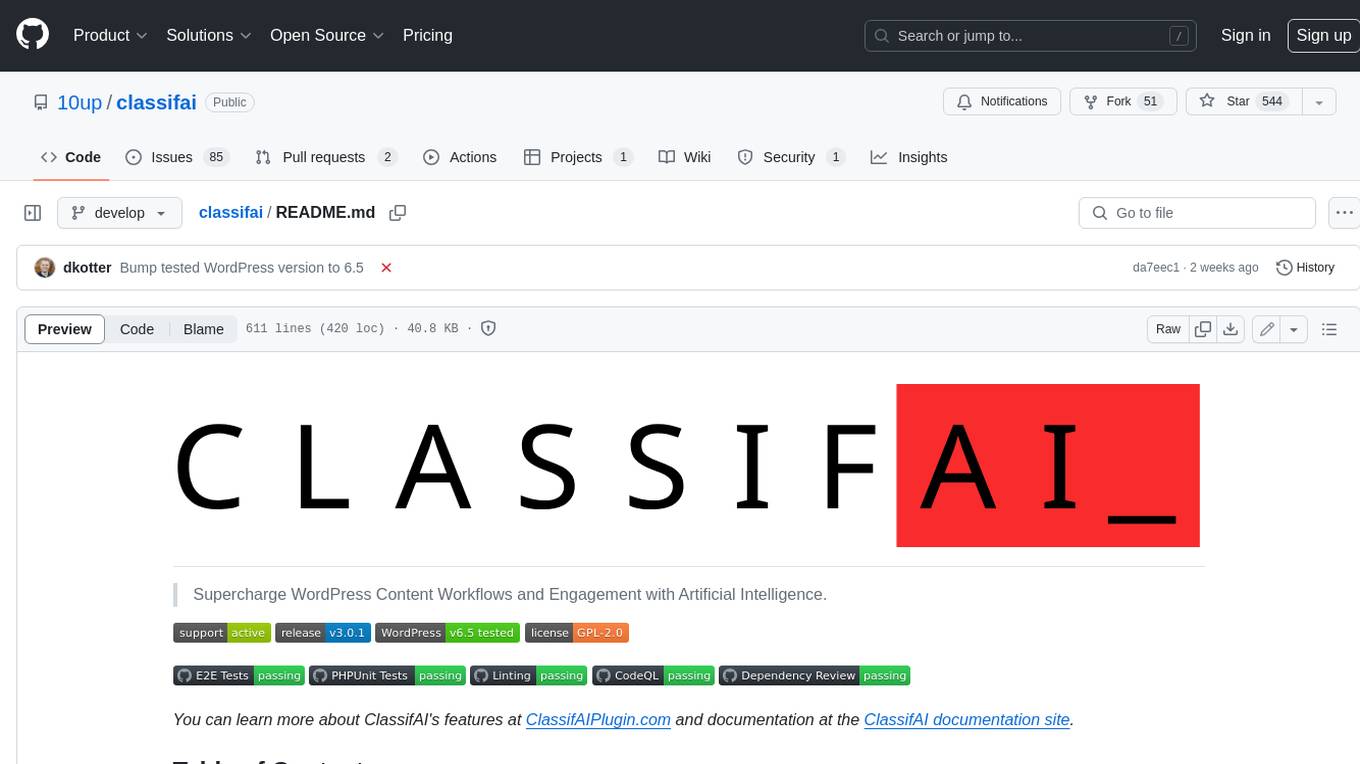
classifai
Supercharge WordPress Content Workflows and Engagement with Artificial Intelligence. Tap into leading cloud-based services like OpenAI, Microsoft Azure AI, Google Gemini and IBM Watson to augment your WordPress-powered websites. Publish content faster while improving SEO performance and increasing audience engagement. ClassifAI integrates Artificial Intelligence and Machine Learning technologies to lighten your workload and eliminate tedious tasks, giving you more time to create original content that matters.
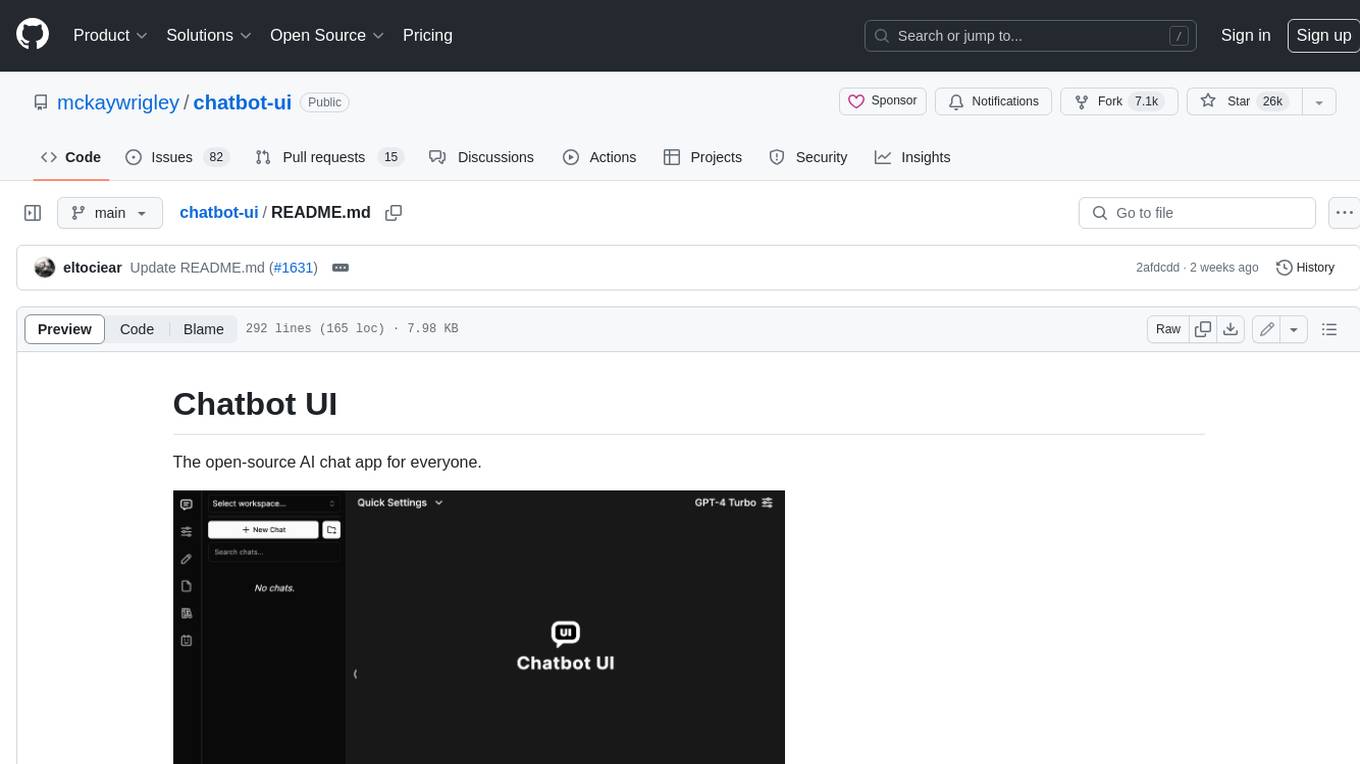
chatbot-ui
Chatbot UI is an open-source AI chat app that allows users to create and deploy their own AI chatbots. It is easy to use and can be customized to fit any need. Chatbot UI is perfect for businesses, developers, and anyone who wants to create a chatbot.
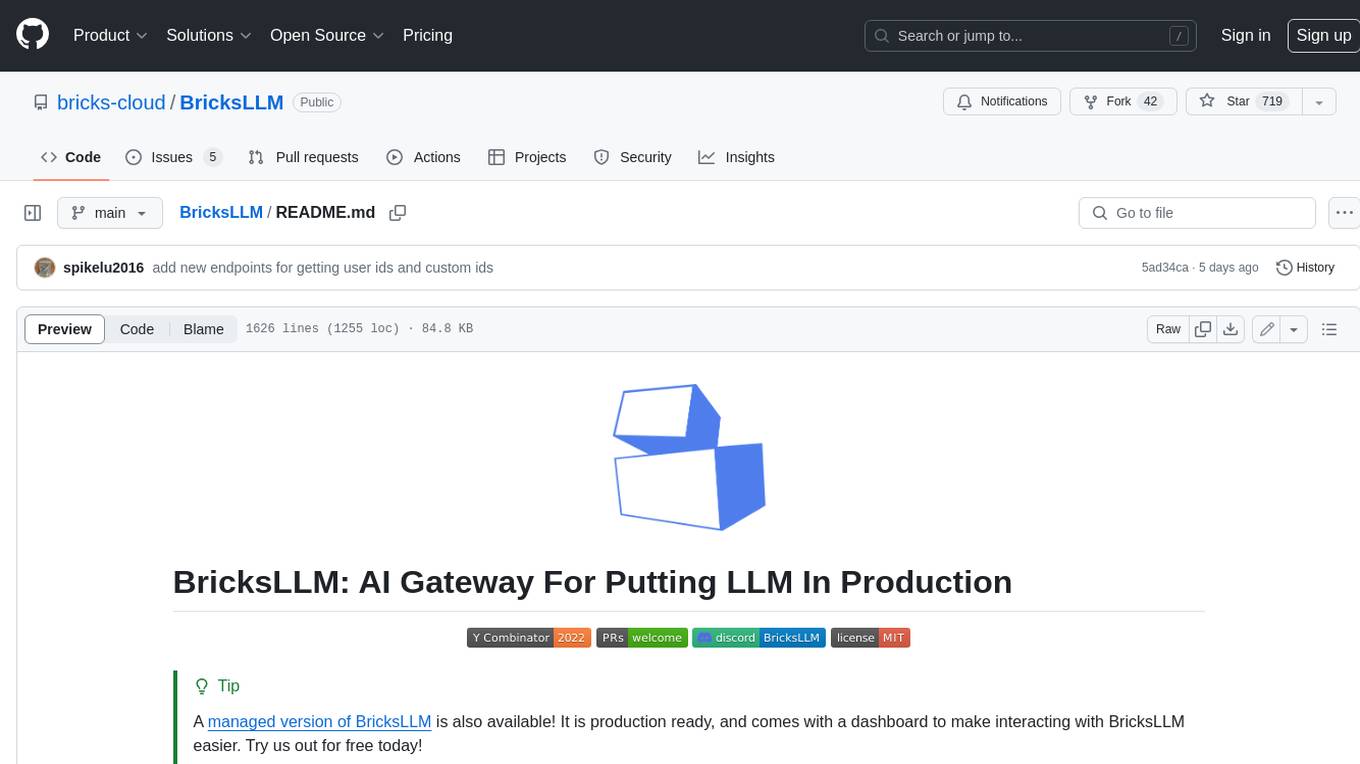
BricksLLM
BricksLLM is a cloud native AI gateway written in Go. Currently, it provides native support for OpenAI, Anthropic, Azure OpenAI and vLLM. BricksLLM aims to provide enterprise level infrastructure that can power any LLM production use cases. Here are some use cases for BricksLLM: * Set LLM usage limits for users on different pricing tiers * Track LLM usage on a per user and per organization basis * Block or redact requests containing PIIs * Improve LLM reliability with failovers, retries and caching * Distribute API keys with rate limits and cost limits for internal development/production use cases * Distribute API keys with rate limits and cost limits for students
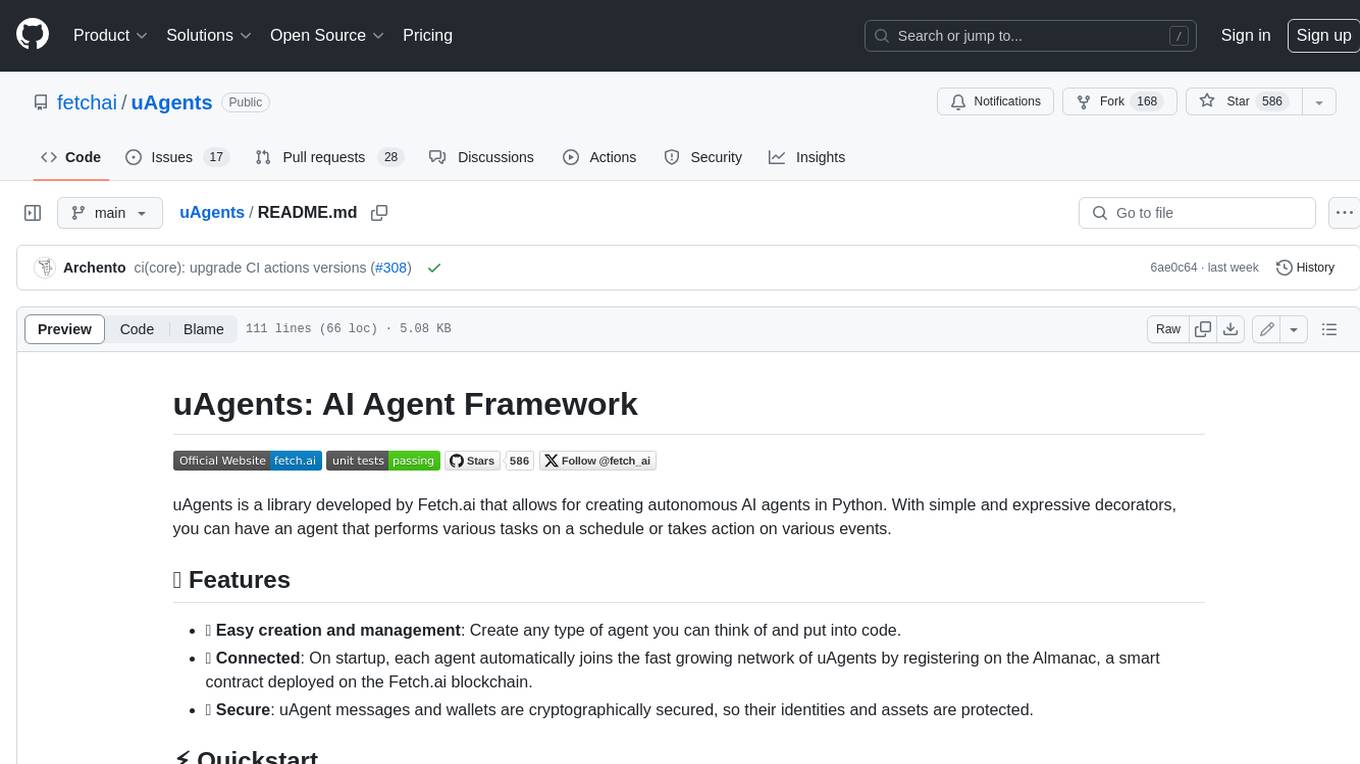
uAgents
uAgents is a Python library developed by Fetch.ai that allows for the creation of autonomous AI agents. These agents can perform various tasks on a schedule or take action on various events. uAgents are easy to create and manage, and they are connected to a fast-growing network of other uAgents. They are also secure, with cryptographically secured messages and wallets.
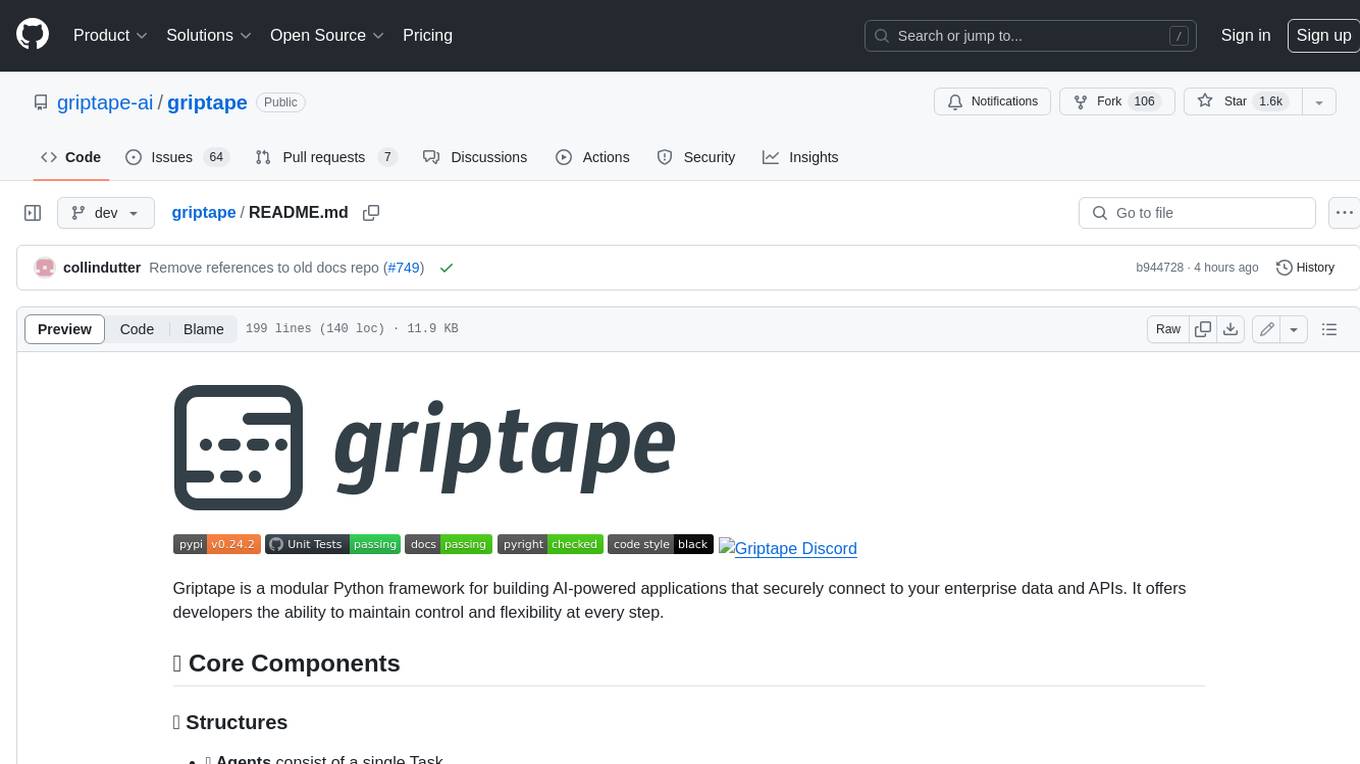
griptape
Griptape is a modular Python framework for building AI-powered applications that securely connect to your enterprise data and APIs. It offers developers the ability to maintain control and flexibility at every step. Griptape's core components include Structures (Agents, Pipelines, and Workflows), Tasks, Tools, Memory (Conversation Memory, Task Memory, and Meta Memory), Drivers (Prompt and Embedding Drivers, Vector Store Drivers, Image Generation Drivers, Image Query Drivers, SQL Drivers, Web Scraper Drivers, and Conversation Memory Drivers), Engines (Query Engines, Extraction Engines, Summary Engines, Image Generation Engines, and Image Query Engines), and additional components (Rulesets, Loaders, Artifacts, Chunkers, and Tokenizers). Griptape enables developers to create AI-powered applications with ease and efficiency.