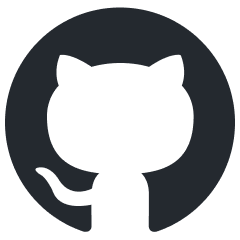
julep
Serverless AI Workflows for Data & ML Teams
Stars: 5151
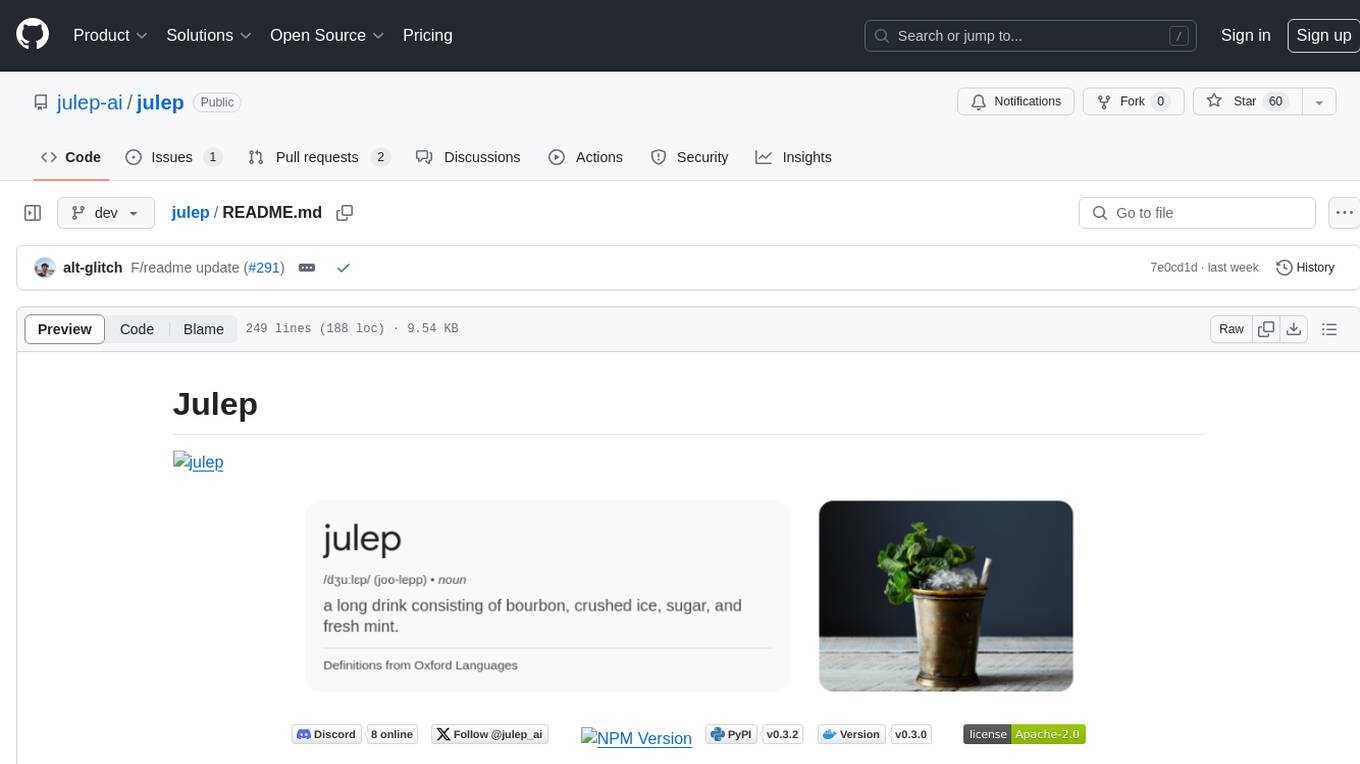
Julep is an advanced platform for creating stateful and functional AI apps powered by large language models. It offers features like statefulness by design, automatic function calling, production-ready deployment, cron-like asynchronous functions, 90+ built-in tools, and the ability to switch between different LLMs easily. Users can build AI applications without the need to write code for embedding, saving, and retrieving conversation history, and can connect to third-party applications using Composio. Julep simplifies the process of getting started with AI apps, whether they are conversational, functional, or agentic.
README:
We're excited to announce the launch of our Open Responses API! This new API offers:
- OpenAI-compatible interface - A drop-in replacement for your existing code
- Self-hosted, open-source implementation - Works with any LLM backend
- Model Provider Agnostic - Connect to any LLM provider (OpenAI, Anthropic, etc.)
The Open Responses API makes it easy to integrate with your existing applications while adding powerful new capabilities.
Ready to try it out? Check out our Open Responses API documentation to get started!
Julep is a serverless platform that helps data and ML teams build sophisticated AI workflows. It provides a robust foundation for orchestrating complex AI operations, managing state across interactions, and integrating with your existing data infrastructure and tools.
Whether you're building data pipelines or creating AI workflows, Julep makes it easy to compose and scale LLM-powered workflows without managing infrastructure. Imagine you want to build an AI agent that can do more than just answer simple questions—it needs to handle complex tasks, remember past interactions, and maybe even use other tools or APIs. That's where Julep comes in. Our platform handles the heavy lifting so you can focus on building intelligent solutions for your business.
💡 To learn more about Julep, check out the Documentation.
- ✨ Key Features
- 🧠 Mental Model
- 📦 Installation
- 🚀 Quick Start
- 🔍 Reference
- 💻 Local Setup
- 👥 Contributors
- 📄 License
🧠 | Smart Memory | Agents that remember context and learn from past interactions |
🔄 | Workflow Engine | Build complex, multi-step processes with branching and loops |
⚡ | Parallel Processing | Run multiple operations simultaneously for maximum efficiency |
🛠️ | Tool Integration | Seamlessly connect with external APIs and services |
🔌 | Easy Setup | Get started quickly with Python and Node.js SDKs |
🔒 | Reliable & Secure | Built-in error handling, retries, and security features |
📊 | Monitoring | Track task progress and performance in real-time |
💡 To learn more about Julep, check out the Documentation.
Julep is made up of the following components:
- Julep Platform: The Julep platform is a cloud service that runs your workflows. It includes a language for describing workflows, a server for running those workflows, and an SDK for interacting with the platform.
- Julep SDKs: Julep SDKs are a set of libraries for building workflows. There are SDKs for Python and JavaScript, with more on the way.
- Julep CLI: The Julep CLI is a command-line tool that allows you to interact with the Julep platform directly from your terminal.
- Julep API: The Julep API is a RESTful API that you can use to interact with the Julep platform.
Think of Julep as a platform that combines both client-side and server-side components to help you build advanced AI agents. Here's how to visualize it:
-
Your Application Code:
- You can use the Julep SDK in your application to define agents, tasks, and workflows.
- The SDK provides functions and classes that make it easy to set up and manage these components.
- You can use the Julep CLI to interact with the Julep platform directly from your terminal.
-
Julep Backend Service:
- The SDK communicates with the Julep backend over the network.
- The CLI communicates with the Julep backend via the SDK.
- The backend handles execution of tasks, maintains session state, stores documents, and orchestrates workflows.
-
Integration with Tools and APIs:
- Within your workflows, you can integrate external tools and services.
- The backend facilitates these integrations, so your agents can, for example, perform web searches, access databases, or call third-party APIs.
To get started with Julep, install it using npm or pip:
npm install @julep/sdk
# or
bun add @julep/sdk
pip install julep
[!NOTE] 🔑 Get your API key here.
Reach out on Discord to get to know more about Julep.
Julep CLI is a command-line tool that allows you to interact with the Julep platform directly from your terminal. It provides a convenient way to manage your AI workflows, tasks, and agents without needing to write code.
pip install julep-cli
For more details, check out the Julep CLI Documentation.
[!NOTE] The CLI is currently in beta and available for Python only. Node.js support coming soon!
Imagine a Research AI agent that can do the following:
- Take a topic,
- Come up with 30 search queries for that topic,
- Perform those web searches in parallel,
- Summarize the results,
- Send the summary to Discord.
[!NOTE] In Julep, this would be a single task under 80 lines of code and run fully managed all on its own. All of the steps are executed on Julep's own servers and you don't need to lift a finger.
Here's a complete example of a task definition:
# yaml-language-server: $schema=https://raw.githubusercontent.com/julep-ai/julep/refs/heads/dev/schemas/create_task_request.json
name: Research Agent
description: A research assistant that can search the web and send the summary to Discord
########################################################
####################### INPUT ##########################
########################################################
# Define the input schema for the task
input_schema:
type: object
properties:
topic:
type: string
description: The main topic to research
num_questions:
type: integer
description: The number of search queries to generate
########################################################
####################### TOOLS ##########################
########################################################
# Define the tools that the agent can use
tools:
- name: web_search
type: integration
integration:
provider: brave
setup:
api_key: "<your-brave-api-key>"
- name: discord_webhook
type: api_call
api_call:
url: https://discord.com/api/webhooks/<your-webhook-id>/<your-webhook-token>
method: POST
headers:
Content-Type: application/json
########################################################
####################### MAIN WORKFLOW #################
########################################################
# Special variables:
# - steps[index].input: for accessing the input to the step at that index
# - steps[index].output: for accessing the output of the step at that index
# - _: for accessing the output of the previous step
# Define the main workflow
main:
# Step 0: Generate search queries
- prompt:
- role: system
content: >-
$ f"""
You are a research assistant.
Generate {{steps[0].input.num_questions|default(30, true)}} diverse search queries related to the topic:
{steps[0].input.topic}
Write one query per line.
"""
unwrap: true
# Step 1: Evaluate the search queries using a simple python expression
- evaluate:
search_queries: $ _.split(NEWLINE)
# Step 2: Run the web search in parallel for each query
- over: $ _.search_queries
map:
tool: web_search
arguments:
query: $ _
parallelism: 5
# Step 3: Collect the results from the web search
- evaluate:
search_results: $ _
# Step 4: Summarize the results
- prompt:
- role: system
content: >
$ f"""
You are a research summarizer. Create a comprehensive summary of the following research results on the topic {steps[0].input.topic}.
The summary should be well-structured, informative, and highlight key findings and insights. Keep the summary concise and to the point.
The length of the summary should be less than 150 words.
Here are the search results:
{_.search_results}
"""
unwrap: true
settings:
model: gpt-4o-mini
# Step 5: Send the summary to Discord
- evaluate:
discord_message: |-
$ f'''
**Research Summary for {steps[0].input.topic}**
{_}
'''
# Step 6: Send the summary to Discord
- tool: discord_webhook
arguments:
json_:
content: $ _.discord_message[:2000] # Discord has a 2000 character limit
Here you can execute the above workflow using the Julep SDK:
Python (Click to expand)
from julep import Client
import yaml
import time
# Initialize the client
client = Client(api_key=JULEP_API_KEY)
# Create the agent
agent = client.agents.create(
name="Julep Browser Use Agent",
description="A Julep agent that can use the computer tool to interact with the browser.",
)
# Load the task definition
with open('./research_agent.yaml', 'r') as file:
task_definition = yaml.safe_load(file)
# Create the task
task = client.tasks.create(
agent_id=agent.id,
**task_definition
)
# Create the execution
execution = client.executions.create(
task_id=task.id,
input={
"topic": "artificial intelligence",
"num_questions": 30
}
)
# Wait for the execution to complete
while (result := client.executions.get(execution.id)).status not in ['succeeded', 'failed']:
print(result.status)
time.sleep(1)
# Print the result
if result.status == "succeeded":
print(result.output)
else:
print(f"Error: {result.error}")
Node.js (Click to expand)
import { Julep } from '@julep/sdk';
import yaml from 'yaml';
import fs from 'fs';
// Initialize the client
const client = new Julep({
apiKey: 'your_julep_api_key'
});
// Create the agent
const agent = await client.agents.create({
name: "Julep Browser Use Agent",
description: "A Julep agent that can use the computer tool to interact with the browser.",
});
// Parse the task definition
const taskDefinition = yaml.parse(fs.readFileSync('./research_agent.yaml', 'utf8'));
// Create the task
const task = await client.tasks.create(
agent.id,
taskDefinition
);
// Create the execution
const execution = await client.executions.create(
task.id,
{
input: {
"topic": "artificial intelligence",
"num_questions": 30
}
}
);
// Wait for the execution to complete
let result;
while (true) {
result = await client.executions.get(execution.id);
if (result.status === 'succeeded' || result.status === 'failed') break;
console.log(result.status);
await new Promise(resolve => setTimeout(resolve, 1000));
}
// Print the result
if (result.status === 'succeeded') {
console.log(result.output);
} else {
console.error(`Error: ${result.error}`);
}
In this example, Julep will automatically manage parallel executions, retry failed steps, resend API requests, and keep the tasks running reliably until completion.
This runs in under 30 seconds and returns the following output:
Research Summary for AI (Click to expand)
Research Summary for AI
The field of Artificial Intelligence (AI) has seen significant advancements in recent years, marked by the development of methods and technologies that enable machines to perceive their environment, learn from data, and make decisions. The primary focus of this summary is on the insights derived from various research findings related to AI.
Definition and Scope of AI:
- AI is defined as a branch of computer science focused on creating systems that can perform tasks requiring human-like intelligence, including learning, reasoning, and problem-solving (Wikipedia).
- It encompasses various subfields, including machine learning, natural language processing, robotics, and computer vision.
Impact and Applications:
- AI technologies are being integrated into numerous sectors, improving efficiency and productivity. Applications range from autonomous vehicles and healthcare diagnostics to customer service automation and financial forecasting (OpenAI).
- Google's commitment to making AI beneficial for everyone highlights its potential to significantly improve daily life by enhancing user experiences across various platforms (Google AI).
Ethical Considerations:
- There is an ongoing discourse regarding the ethical implications of AI, including concerns about privacy, bias, and accountability in decision-making processes. The need for a framework that ensures the safe and responsible use of AI technologies is emphasized (OpenAI).
Learning Mechanisms:
- AI systems utilize different learning mechanisms, such as supervised learning, unsupervised learning, and reinforcement learning. These methods allow AI to improve performance over time by learning from past experiences and data (Wikipedia).
- The distinction between supervised and unsupervised learning is critical; supervised learning relies on labeled data, while unsupervised learning identifies patterns without predefined labels (Unsupervised).
Future Directions:
- Future AI developments are expected to focus on enhancing the interpretability and transparency of AI systems, ensuring that they can provide justifiable decisions and actions (OpenAI).
- There is also a push towards making AI systems more accessible and user-friendly, encouraging broader adoption across different demographics and industries (Google AI).
AI represents a transformative force across multiple domains, promising to reshape industries and improve quality of life. However, as its capabilities expand, it is crucial to address the ethical and societal implications that arise. Continued research and collaboration among technologists, ethicists, and policymakers will be essential in navigating the future landscape of AI.
- 📚 Explore more examples in our Cookbook
- 🔧 Learn about Tool Integration
- 🧠 Understand Agent Memory
- 🔄 Dive into Complex Workflows
[!TIP] 💡 Checkout more tutorials in the Tutorials section of the documentation.
💡 If you are a beginner, we recommend starting with the Quickstart Guide.
💡 If you are looking for more ideas, check out the Ideas section of the repository.
💡 If you more into cookbook style recipes, check out the Cookbook section of the repository.
- Node.js SDK Reference | NPM Package
- Python SDK Reference | PyPI Package
Explore our API documentation to learn more about agents, tasks, tools, and the Julep CLI here: API Reference
Clone the repository from your preferred source:
git clone <repository_url>
Change to the root directory of the project:
cd <repository_root>
- Create a
.env
file in the root directory. - Refer to the
.env.example
file for a list of required variables. - Ensure that all necessary variables are set in the
.env
file.
Create a Docker volume named grafana_data
, memory_store_data
, temporal-db-data
, prometheus_data
, seaweedfs_data
:
docker volume create grafana_data
docker volume create memory_store_data
docker volume create temporal-db-data
docker volume create prometheus_data
docker volume create seaweedfs_data
You can run the project in two different modes: Single Tenant or Multi-Tenant. Choose one of the following commands based on your requirement:
- Single-Tenant Mode
Run the project in single-tenant mode:
docker compose --env-file .env --profile temporal-ui --profile single-tenant --profile self-hosted-db --profile blob-store --profile temporal-ui-public up --build --force-recreate --watch
Note: In single-tenant mode, you can interact with the SDK directly without the need for the API KEY.
- Multi-Tenant Mode
Run the project in multi-tenant mode:
docker compose --env-file .env --profile temporal-ui --profile multi-tenant --profile embedding-cpu --profile self-hosted-db --profile blob-store --profile temporal-ui-public up --force-recreate --build --watch
Note: In multi-tenant mode, you need to generate a JWT token locally that act as an API KEY to interact with the SDK.
Generate a JWT Token (Only for Multi-Tenant Mode)
To generate a JWT token, jwt-cli
is required. Kindly install the same before proceeding with the next steps.
Use the following command and replace JWT_SHARED_KEY
with the corresponding key from your .env
file to generate a JWT token:
jwt encode --secret JWT_SHARED_KEY --alg HS512 --exp=$(date -d '+10 days' +%s) --sub '00000000-0000-0000-0000-000000000000' '{}'
This command generates a JWT token that will be valid for 10 days.
-
Temporal UI: You can access the Temporal UI through the specified port in your
.env
file. - Julep SDK: The Julep SDK is a Python/Node.js library that allows you to interact with the Julep API.
from julep import Client
client = Client(api_key="your_jwt_token")
Note: SDK in Multi-Tenant mode, you need to generate a JWT token locally that acts as an API KEY to interact with the SDK. Furthermore, while initializing the client you will need to set the environment to local_multi_tenant
and the api key to the JWT token you generated in the previous step. Whereas in Single-Tenant mode you can interact with the SDK directly without the need for the API KEY and set the environment to local
.
- Ensure that all required Docker images are available.
- Check for missing environment variables in the
.env
file. - Use the
docker compose logs
command to view detailed logs for debugging.
We're excited to welcome new contributors to the Julep project! We've created several "good first issues" to help you get started.
- 📖 Check out our CONTRIBUTING.md file for guidelines
- 🔍 Browse our good first issues
- 💬 Join our Discord for help and discussions
Your contributions, big or small, are valuable to us. Let's build something amazing together! 🚀
Julep is licensed under the Apache License 2.0.
See the LICENSE file for more details.
For Tasks:
Click tags to check more tools for each tasksFor Jobs:
Alternative AI tools for julep
Similar Open Source Tools
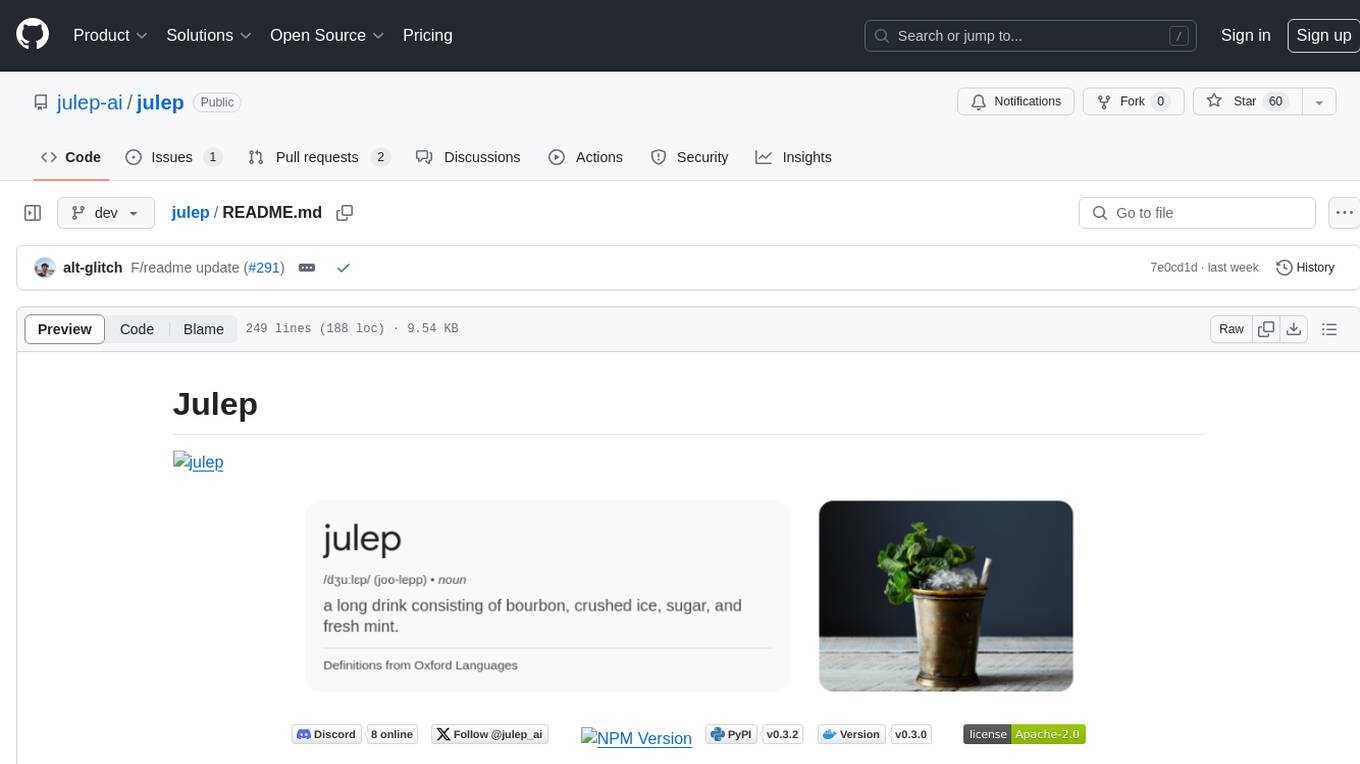
julep
Julep is an advanced platform for creating stateful and functional AI apps powered by large language models. It offers features like statefulness by design, automatic function calling, production-ready deployment, cron-like asynchronous functions, 90+ built-in tools, and the ability to switch between different LLMs easily. Users can build AI applications without the need to write code for embedding, saving, and retrieving conversation history, and can connect to third-party applications using Composio. Julep simplifies the process of getting started with AI apps, whether they are conversational, functional, or agentic.
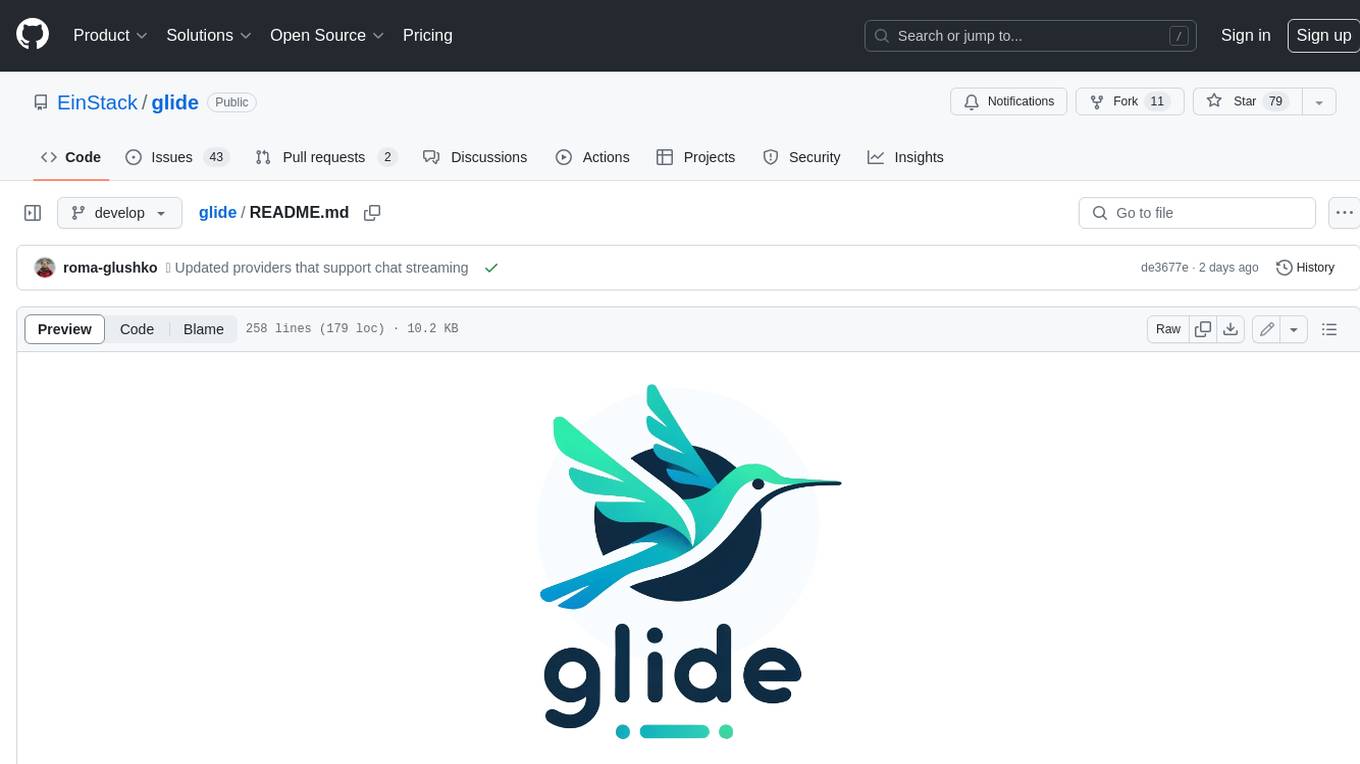
glide
Glide is a cloud-native LLM gateway that provides a unified REST API for accessing various large language models (LLMs) from different providers. It handles LLMOps tasks such as model failover, caching, key management, and more, making it easy to integrate LLMs into applications. Glide supports popular LLM providers like OpenAI, Anthropic, Azure OpenAI, AWS Bedrock (Titan), Cohere, Google Gemini, OctoML, and Ollama. It offers high availability, performance, and observability, and provides SDKs for Python and NodeJS to simplify integration.
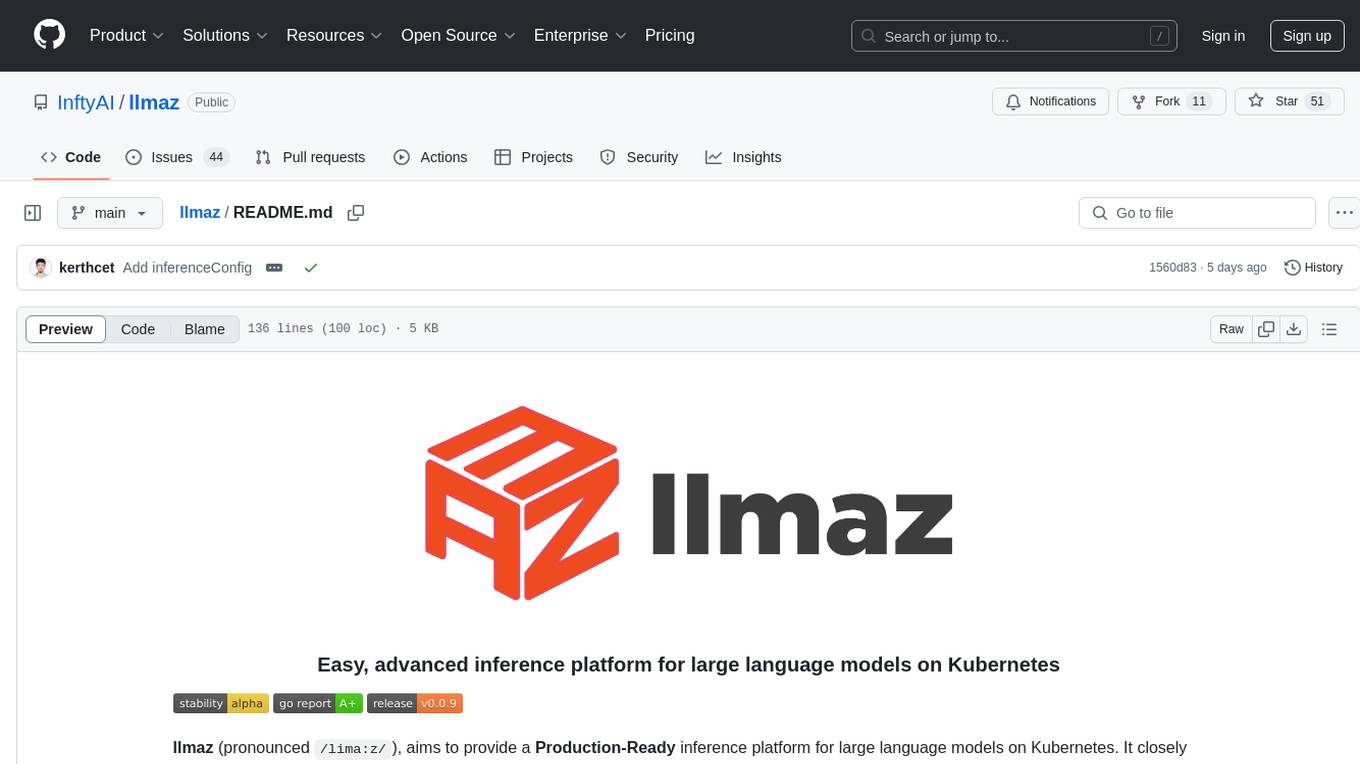
llmaz
llmaz is an easy, advanced inference platform for large language models on Kubernetes. It aims to provide a production-ready solution that integrates with state-of-the-art inference backends. The platform supports efficient model distribution, accelerator fungibility, SOTA inference, various model providers, multi-host support, and scaling efficiency. Users can quickly deploy LLM services with minimal configurations and benefit from a wide range of advanced inference backends. llmaz is designed to optimize cost and performance while supporting cutting-edge researches like Speculative Decoding or Splitwise on Kubernetes.
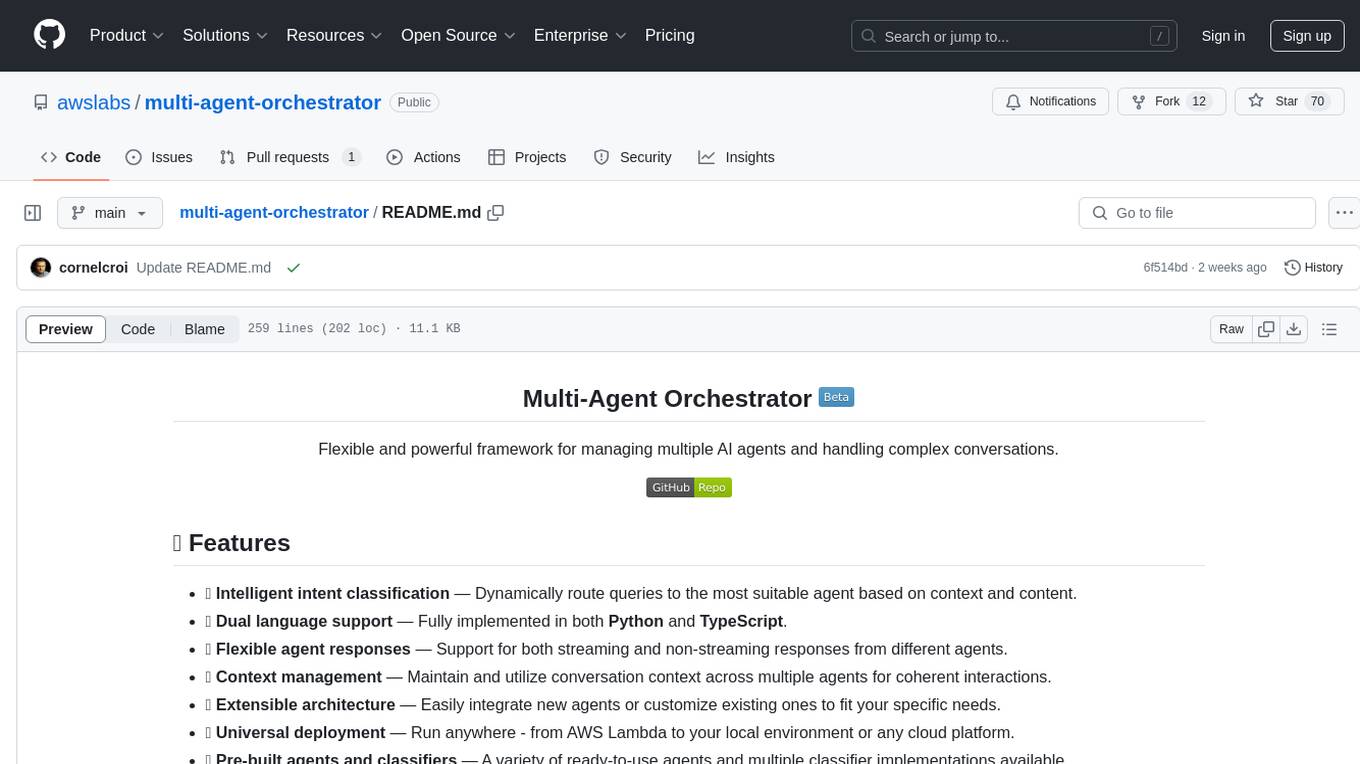
multi-agent-orchestrator
Multi-Agent Orchestrator is a flexible and powerful framework for managing multiple AI agents and handling complex conversations. It intelligently routes queries to the most suitable agent based on context and content, supports dual language implementation in Python and TypeScript, offers flexible agent responses, context management across agents, extensible architecture for customization, universal deployment options, and pre-built agents and classifiers. It is suitable for various applications, from simple chatbots to sophisticated AI systems, accommodating diverse requirements and scaling efficiently.
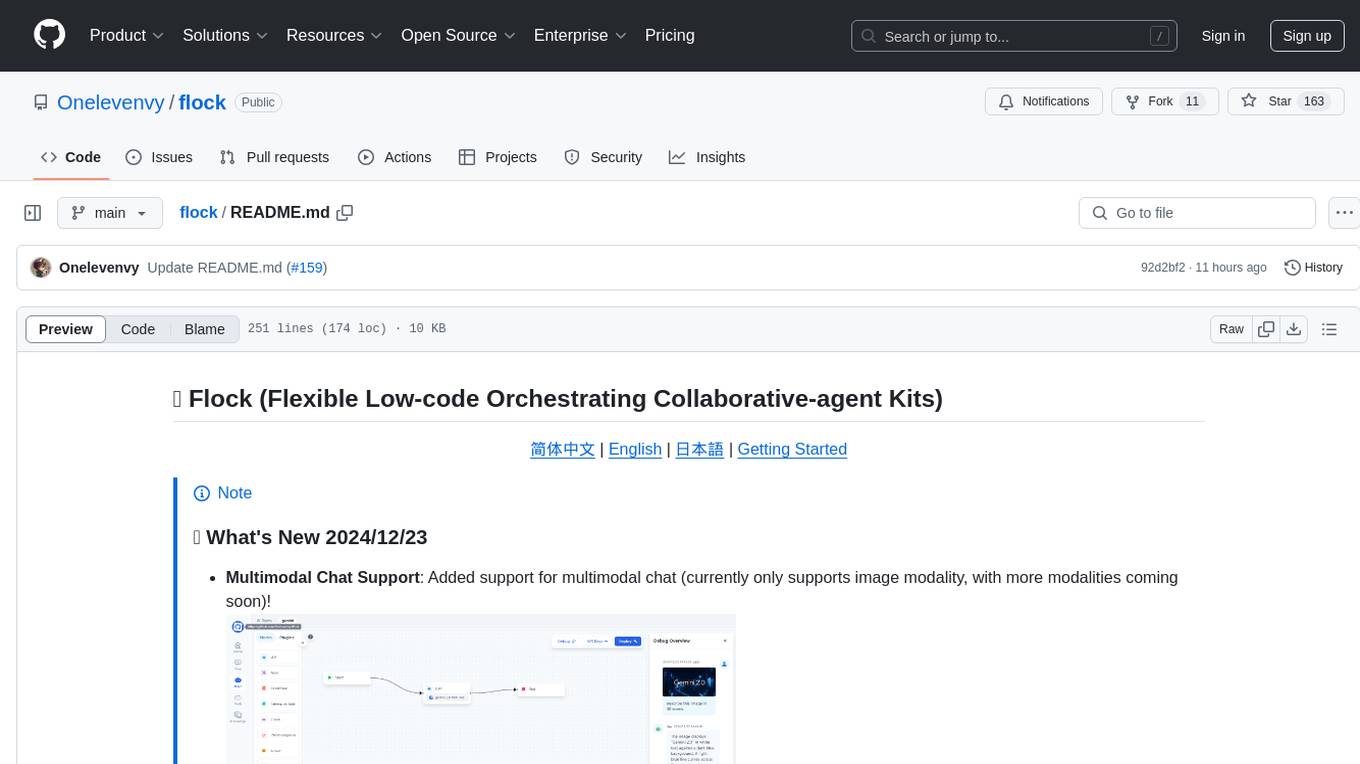
flock
Flock is a workflow-based low-code platform that enables rapid development of chatbots, RAG applications, and coordination of multi-agent teams. It offers a flexible, low-code solution for orchestrating collaborative agents, supporting various node types for specific tasks, such as input processing, text generation, knowledge retrieval, tool execution, intent recognition, answer generation, and more. Flock integrates LangChain and LangGraph to provide offline operation capabilities and supports future nodes like Conditional Branch, File Upload, and Parameter Extraction for creating complex workflows. Inspired by StreetLamb, Lobe-chat, Dify, and fastgpt projects, Flock introduces new features and directions while leveraging open-source models and multi-tenancy support.
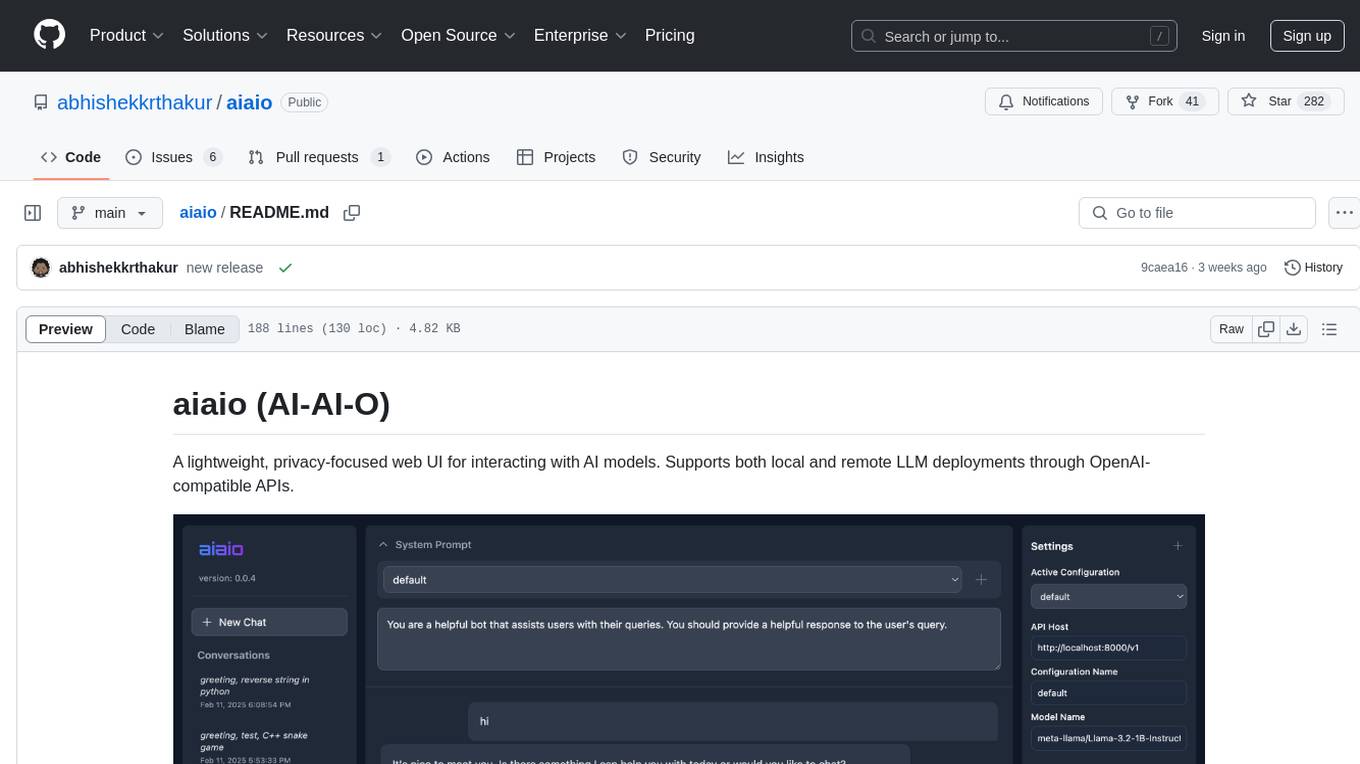
aiaio
aiaio (AI-AI-O) is a lightweight, privacy-focused web UI for interacting with AI models. It supports both local and remote LLM deployments through OpenAI-compatible APIs. The tool provides features such as dark/light mode support, local SQLite database for conversation storage, file upload and processing, configurable model parameters through UI, privacy-focused design, responsive design for mobile/desktop, syntax highlighting for code blocks, real-time conversation updates, automatic conversation summarization, customizable system prompts, WebSocket support for real-time updates, Docker support for deployment, multiple API endpoint support, and multiple system prompt support. Users can configure model parameters and API settings through the UI, handle file uploads, manage conversations, and use keyboard shortcuts for efficient interaction. The tool uses SQLite for storage with tables for conversations, messages, attachments, and settings. Contributions to the project are welcome under the Apache License 2.0.
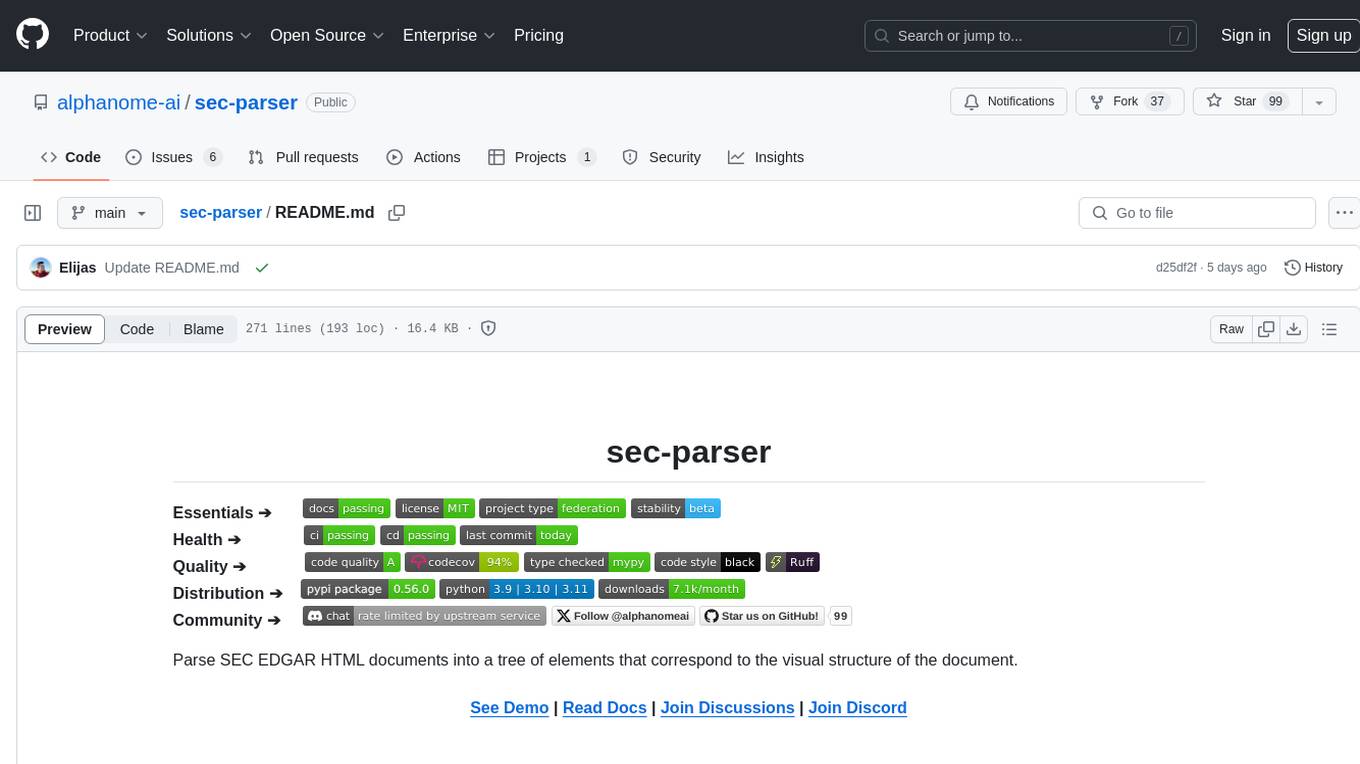
sec-parser
The `sec-parser` project simplifies extracting meaningful information from SEC EDGAR HTML documents by organizing them into semantic elements and a tree structure. It helps in parsing SEC filings for financial and regulatory analysis, analytics and data science, AI and machine learning, causal AI, and large language models. The tool is especially beneficial for AI, ML, and LLM applications by streamlining data pre-processing and feature extraction.
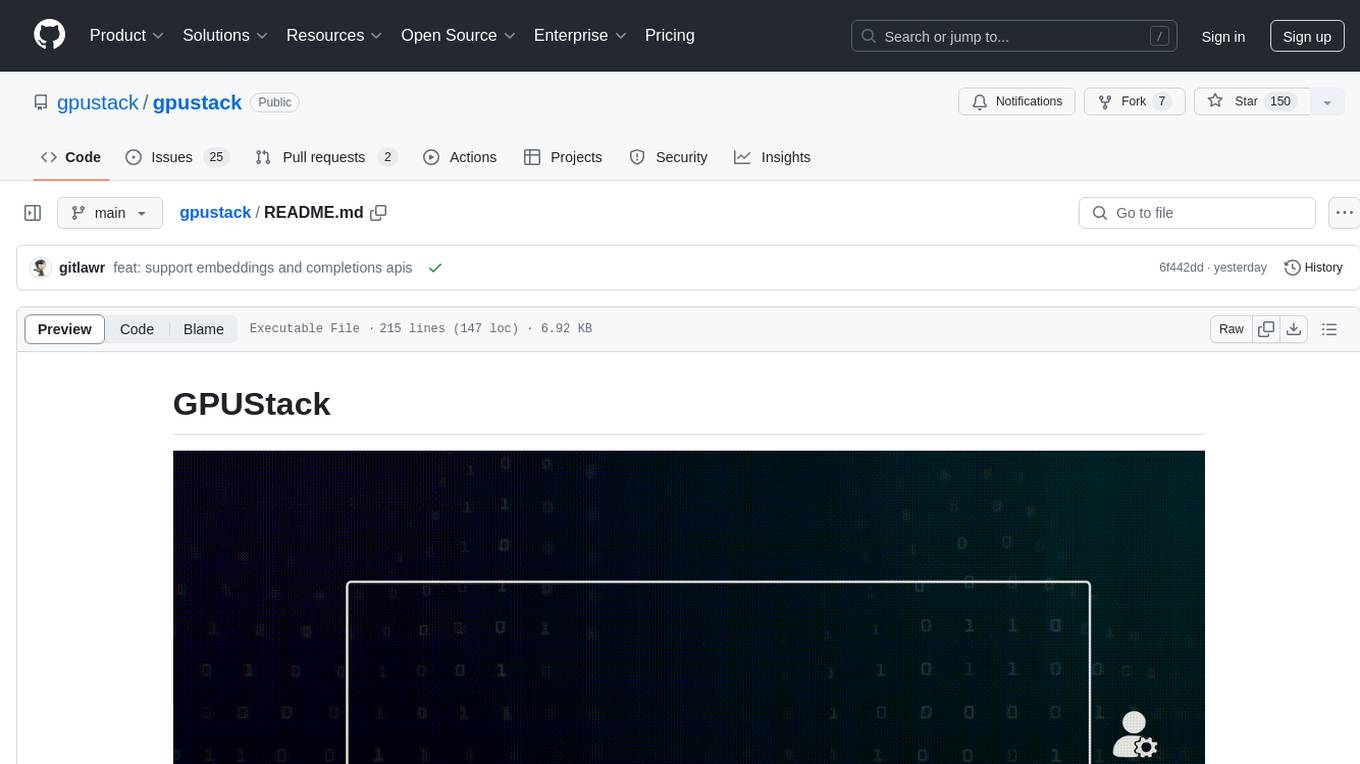
gpustack
GPUStack is an open-source GPU cluster manager designed for running large language models (LLMs). It supports a wide variety of hardware, scales with GPU inventory, offers lightweight Python package with minimal dependencies, provides OpenAI-compatible APIs, simplifies user and API key management, enables GPU metrics monitoring, and facilitates token usage and rate metrics tracking. The tool is suitable for managing GPU clusters efficiently and effectively.
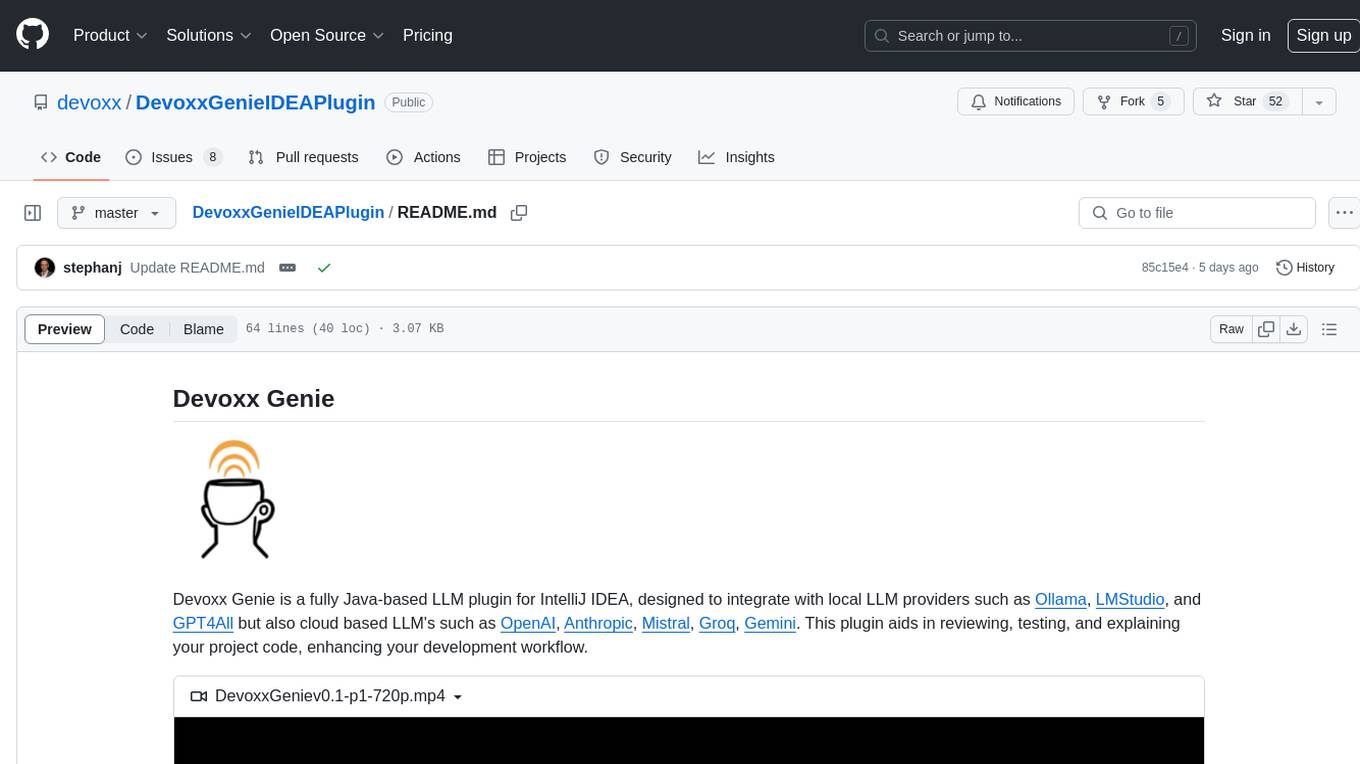
DevoxxGenieIDEAPlugin
Devoxx Genie is a Java-based IntelliJ IDEA plugin that integrates with local and cloud-based LLM providers to aid in reviewing, testing, and explaining project code. It supports features like code highlighting, chat conversations, and adding files/code snippets to context. Users can modify REST endpoints and LLM parameters in settings, including support for cloud-based LLMs. The plugin requires IntelliJ version 2023.3.4 and JDK 17. Building and publishing the plugin is done using Gradle tasks. Users can select an LLM provider, choose code, and use commands like review, explain, or generate unit tests for code analysis.
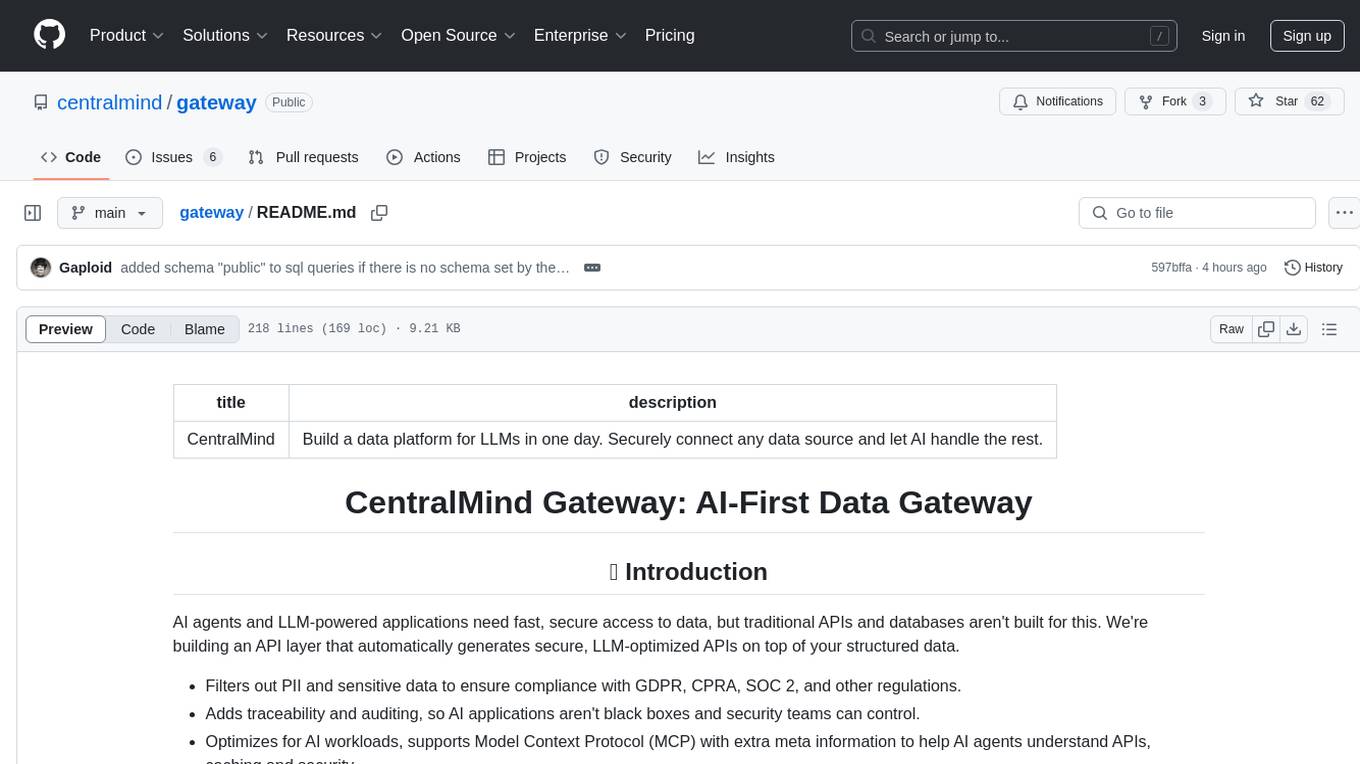
gateway
CentralMind Gateway is an AI-first data gateway that securely connects any data source and automatically generates secure, LLM-optimized APIs. It filters out sensitive data, adds traceability, and optimizes for AI workloads. Suitable for companies deploying AI agents for customer support and analytics.
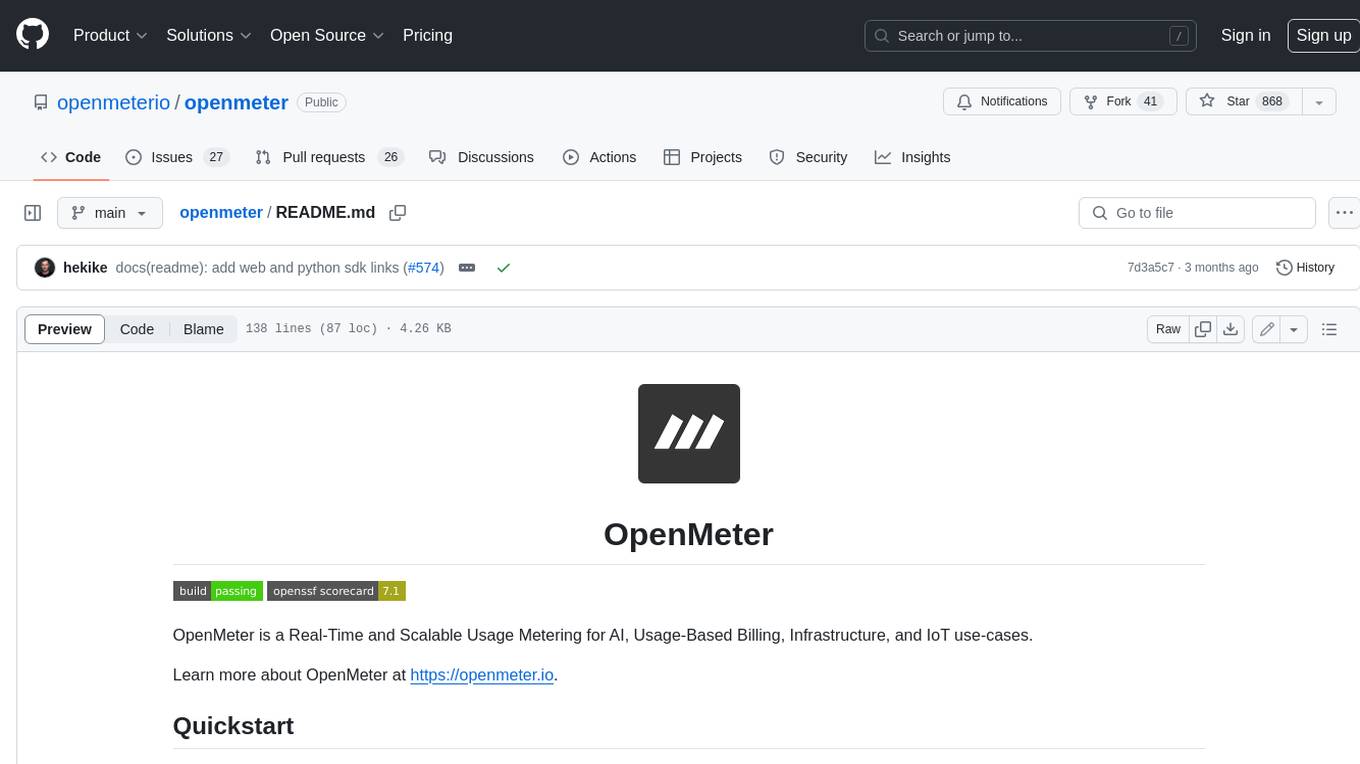
openmeter
OpenMeter is a real-time and scalable usage metering tool for AI, usage-based billing, infrastructure, and IoT use cases. It provides a REST API for integrations and offers client SDKs in Node.js, Python, Go, and Web. OpenMeter is licensed under the Apache 2.0 License.
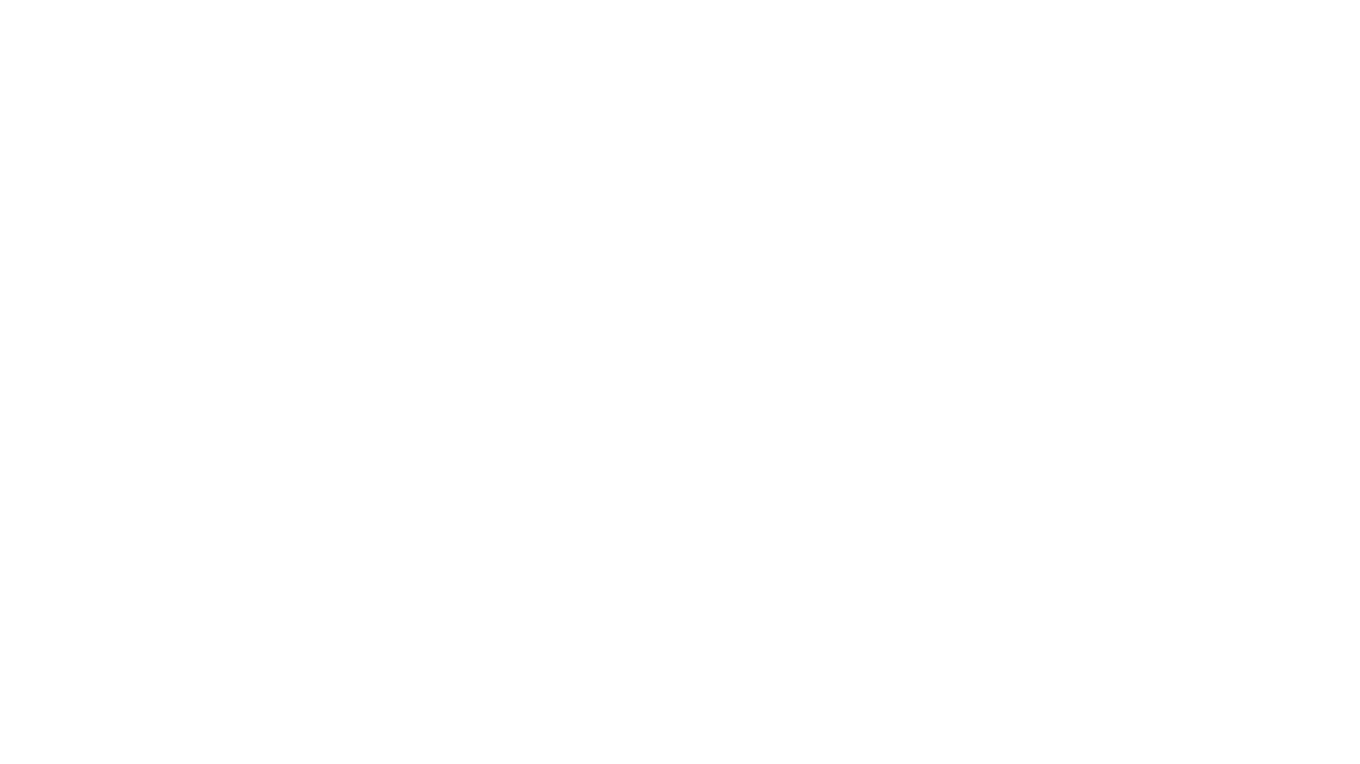
DevDocs
DevDocs is a platform designed to simplify the process of digesting technical documentation for software engineers and developers. It automates the extraction and conversion of web content into markdown format, making it easier for users to access and understand the information. By crawling through child pages of a given URL, DevDocs provides a streamlined approach to gathering relevant data and integrating it into various tools for software development. The tool aims to save time and effort by eliminating the need for manual research and content extraction, ultimately enhancing productivity and efficiency in the development process.
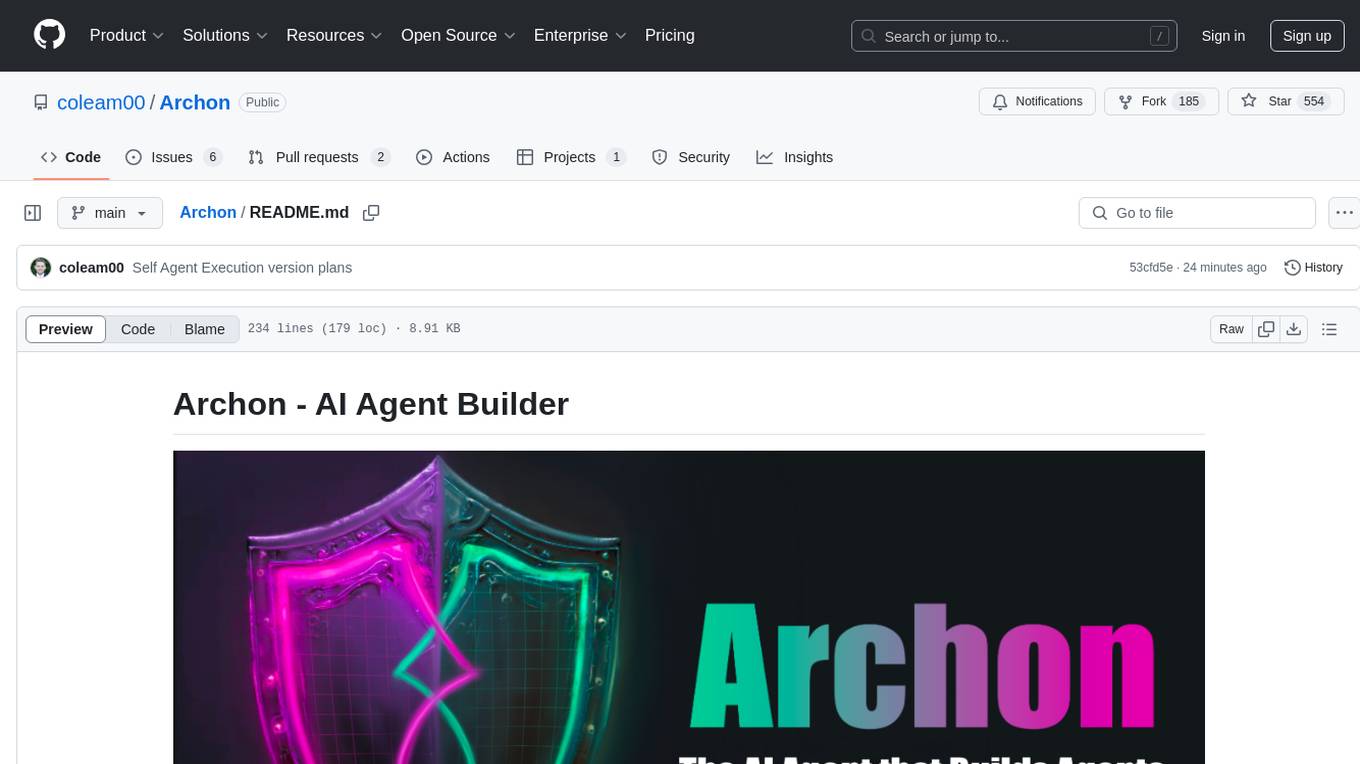
Archon
Archon is an AI meta-agent designed to autonomously build, refine, and optimize other AI agents. It serves as a practical tool for developers and an educational framework showcasing the evolution of agentic systems. Through iterative development, Archon demonstrates the power of planning, feedback loops, and domain-specific knowledge in creating robust AI agents.
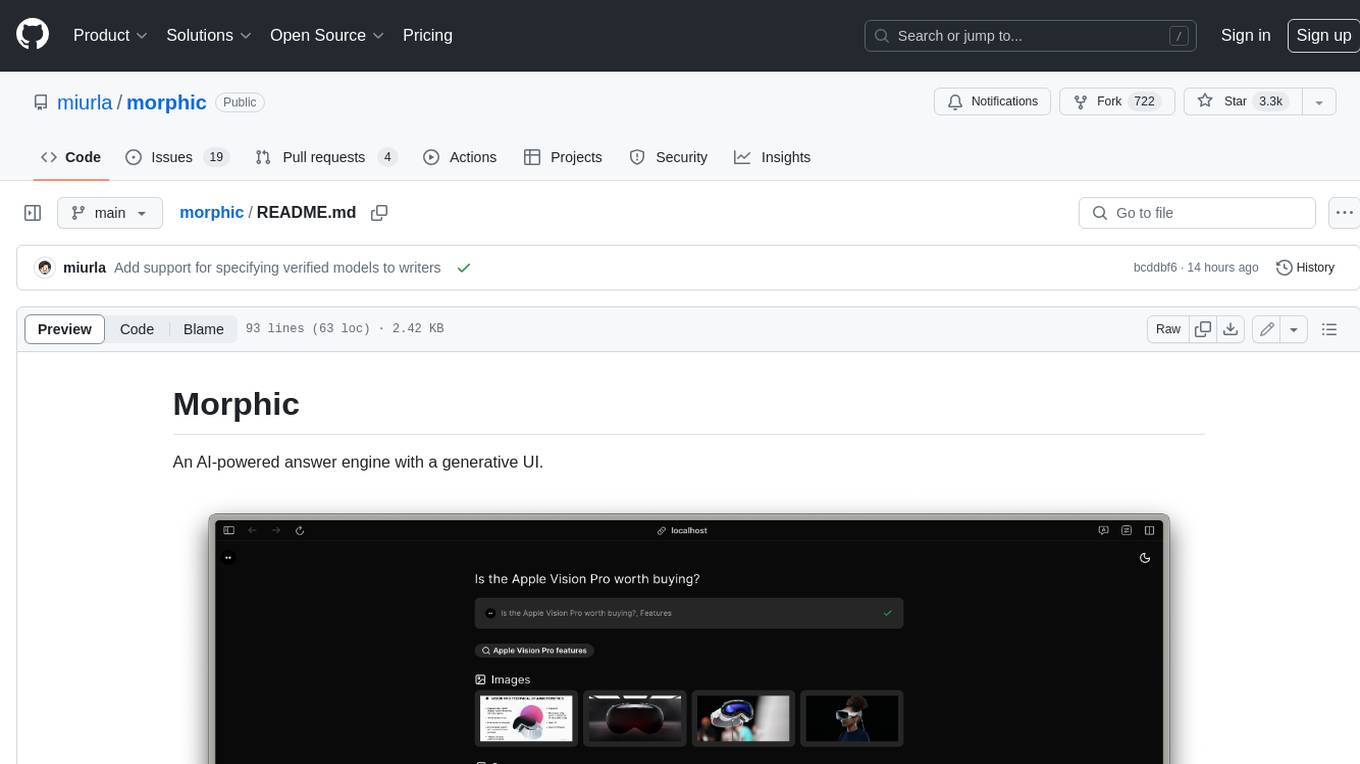
morphic
Morphic is an AI-powered answer engine with a generative UI. It utilizes a stack of Next.js, Vercel AI SDK, OpenAI, Tavily AI, shadcn/ui, Radix UI, and Tailwind CSS. To get started, fork and clone the repo, install dependencies, fill out secrets in the .env.local file, and run the app locally using 'bun dev'. You can also deploy your own live version of Morphic with Vercel. Verified models that can be specified to writers include Groq, LLaMA3 8b, and LLaMA3 70b.
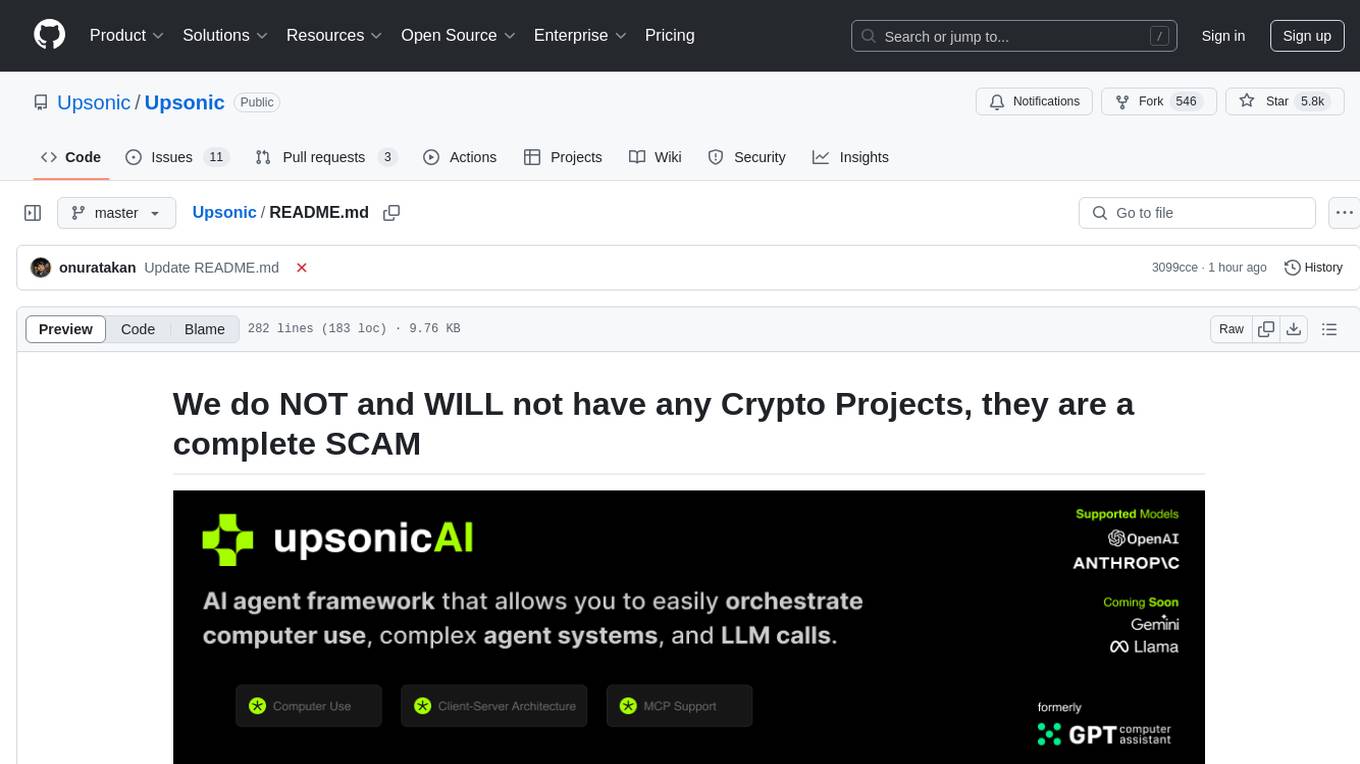
Upsonic
Upsonic offers a cutting-edge enterprise-ready framework for orchestrating LLM calls, agents, and computer use to complete tasks cost-effectively. It provides reliable systems, scalability, and a task-oriented structure for real-world cases. Key features include production-ready scalability, task-centric design, MCP server support, tool-calling server, computer use integration, and easy addition of custom tools. The framework supports client-server architecture and allows seamless deployment on AWS, GCP, or locally using Docker.
For similar tasks
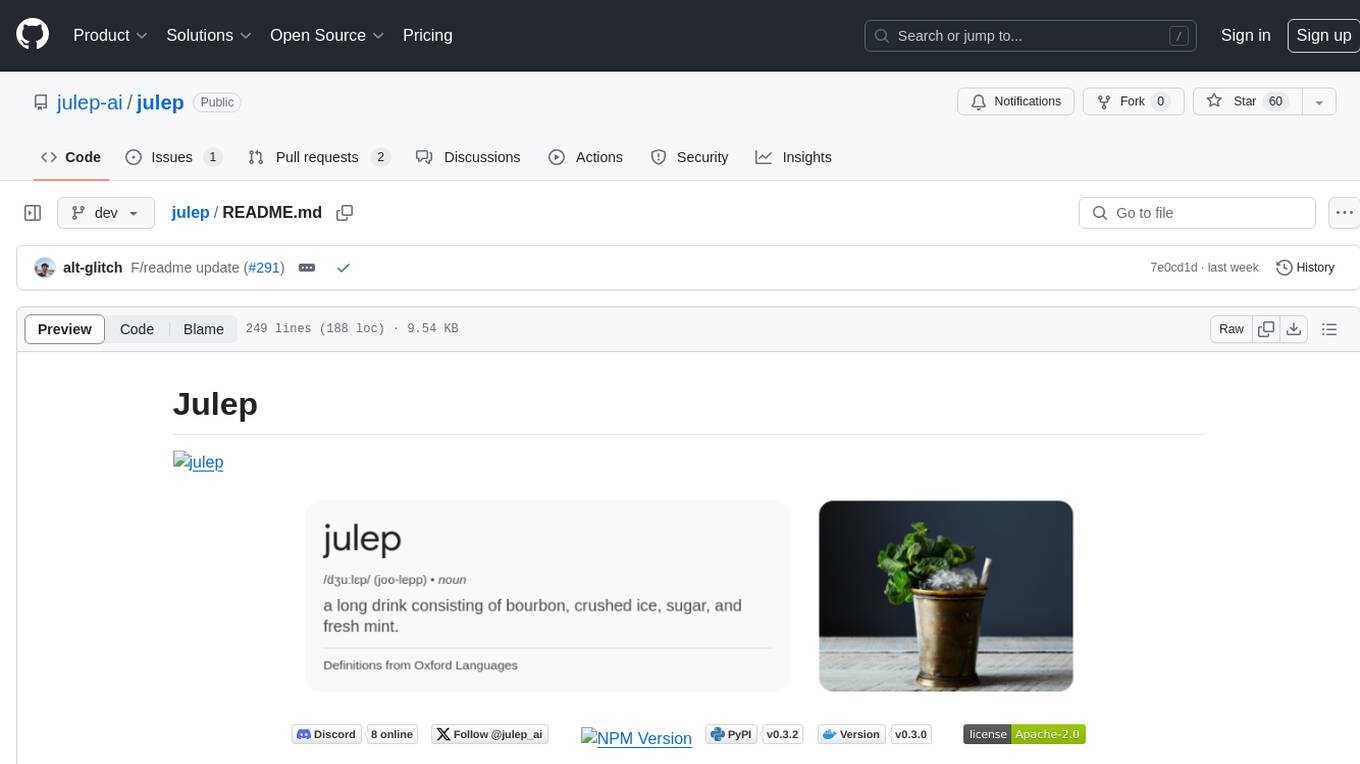
julep
Julep is an advanced platform for creating stateful and functional AI apps powered by large language models. It offers features like statefulness by design, automatic function calling, production-ready deployment, cron-like asynchronous functions, 90+ built-in tools, and the ability to switch between different LLMs easily. Users can build AI applications without the need to write code for embedding, saving, and retrieving conversation history, and can connect to third-party applications using Composio. Julep simplifies the process of getting started with AI apps, whether they are conversational, functional, or agentic.
For similar jobs
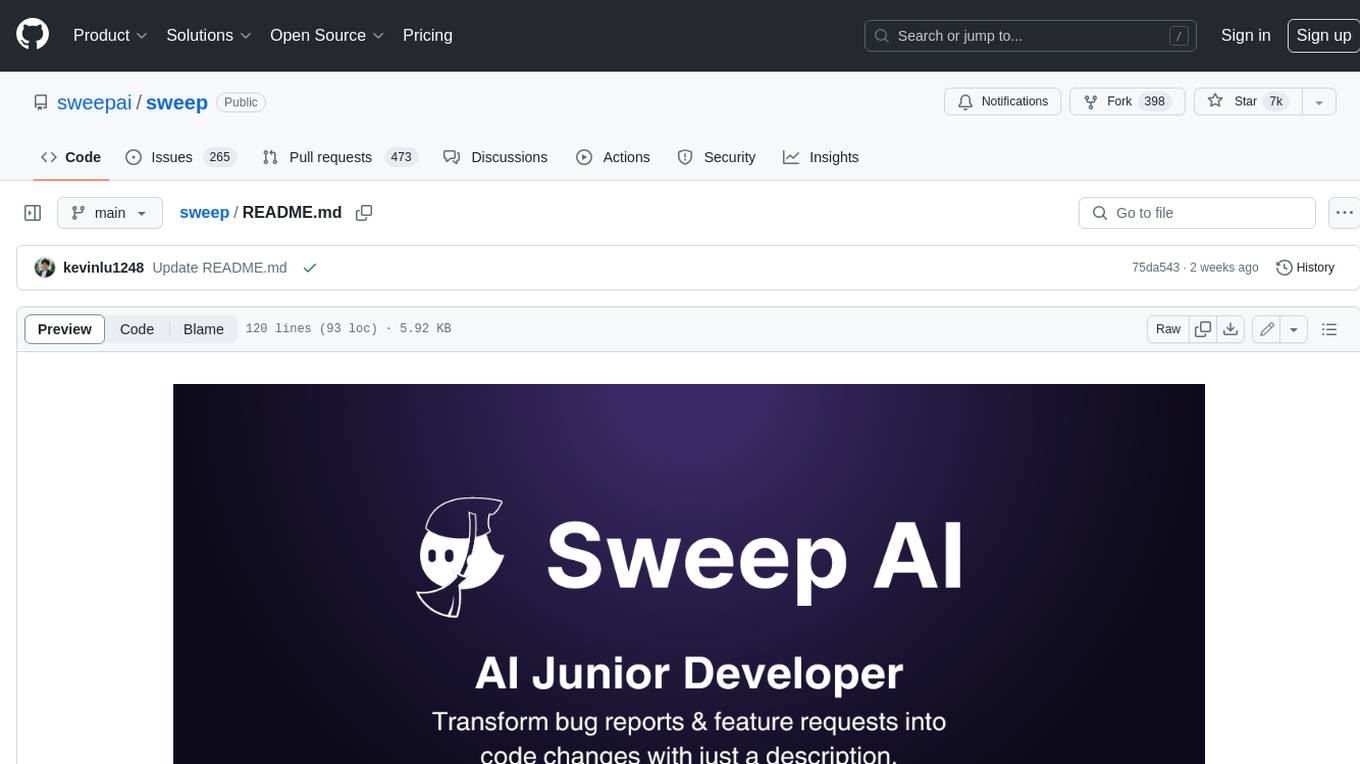
sweep
Sweep is an AI junior developer that turns bugs and feature requests into code changes. It automatically handles developer experience improvements like adding type hints and improving test coverage.
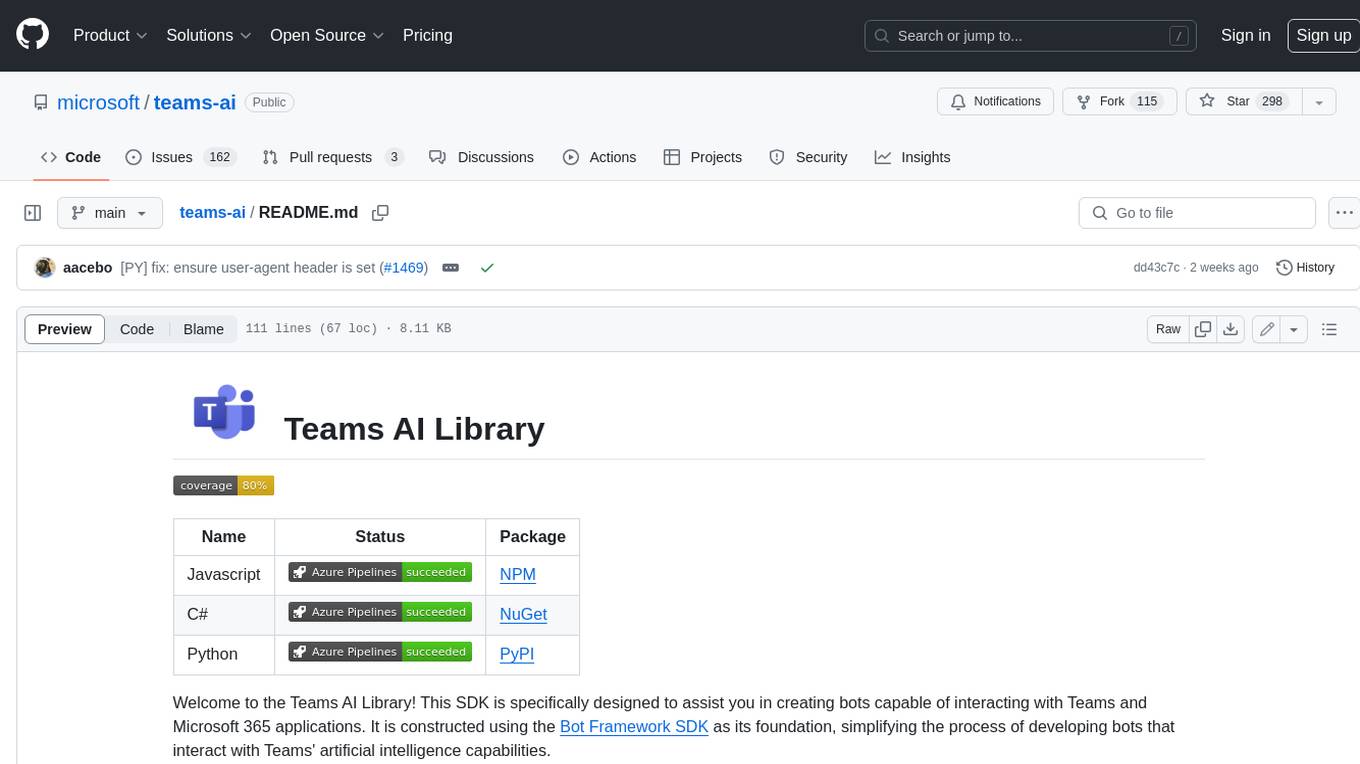
teams-ai
The Teams AI Library is a software development kit (SDK) that helps developers create bots that can interact with Teams and Microsoft 365 applications. It is built on top of the Bot Framework SDK and simplifies the process of developing bots that interact with Teams' artificial intelligence capabilities. The SDK is available for JavaScript/TypeScript, .NET, and Python.
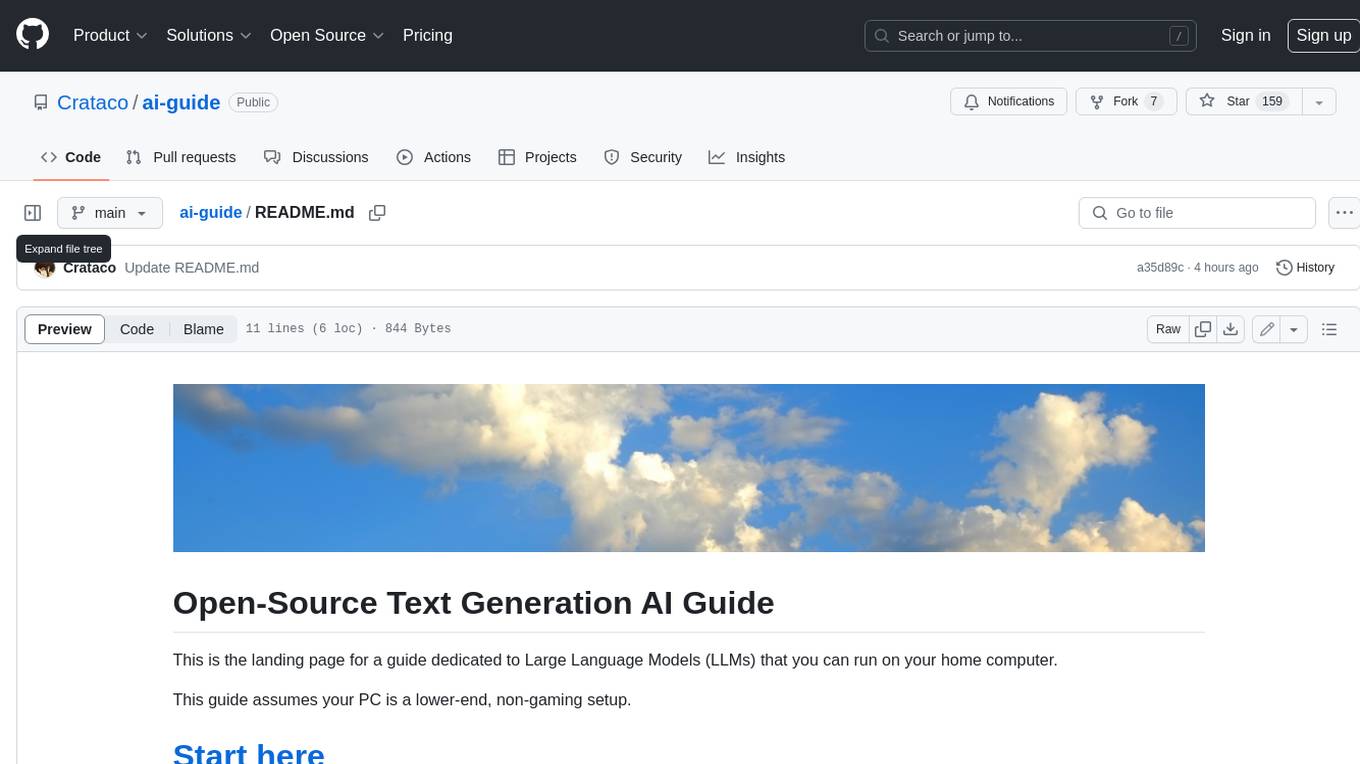
ai-guide
This guide is dedicated to Large Language Models (LLMs) that you can run on your home computer. It assumes your PC is a lower-end, non-gaming setup.
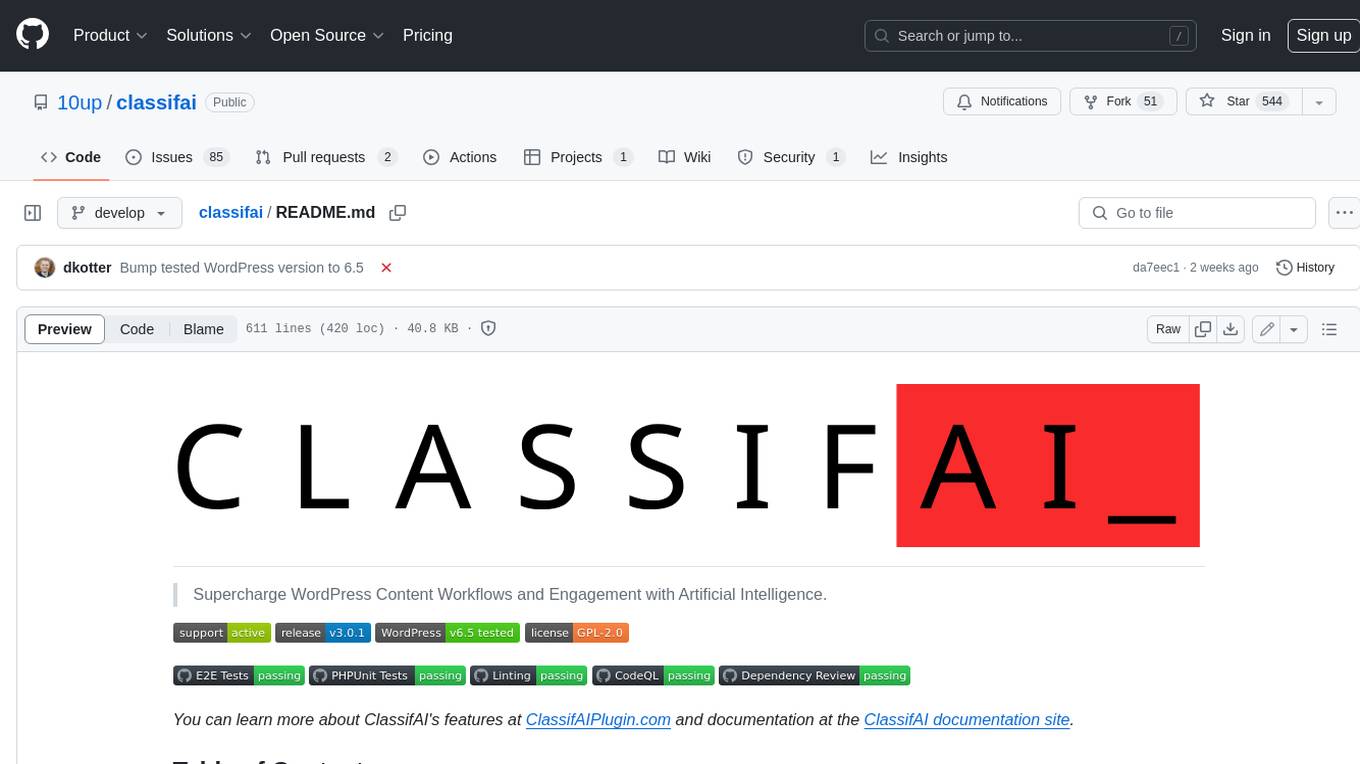
classifai
Supercharge WordPress Content Workflows and Engagement with Artificial Intelligence. Tap into leading cloud-based services like OpenAI, Microsoft Azure AI, Google Gemini and IBM Watson to augment your WordPress-powered websites. Publish content faster while improving SEO performance and increasing audience engagement. ClassifAI integrates Artificial Intelligence and Machine Learning technologies to lighten your workload and eliminate tedious tasks, giving you more time to create original content that matters.
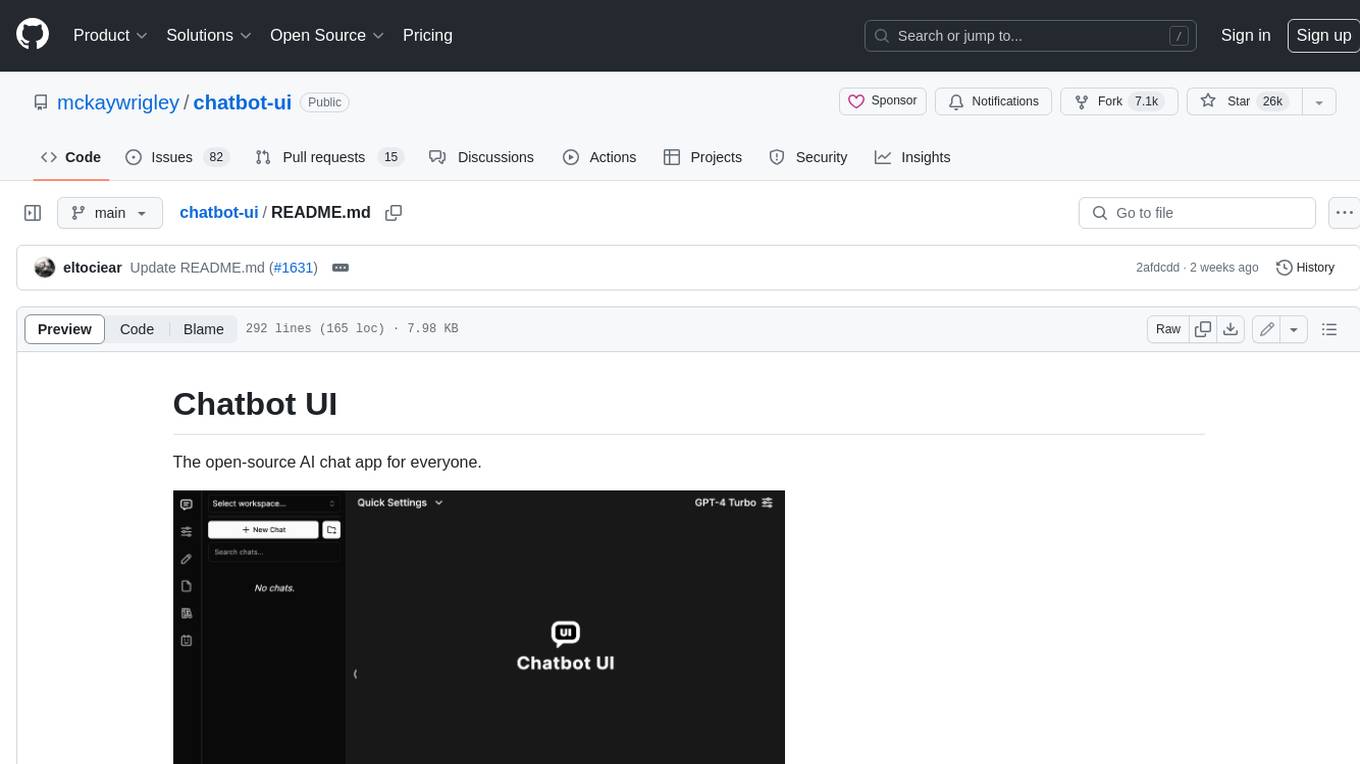
chatbot-ui
Chatbot UI is an open-source AI chat app that allows users to create and deploy their own AI chatbots. It is easy to use and can be customized to fit any need. Chatbot UI is perfect for businesses, developers, and anyone who wants to create a chatbot.
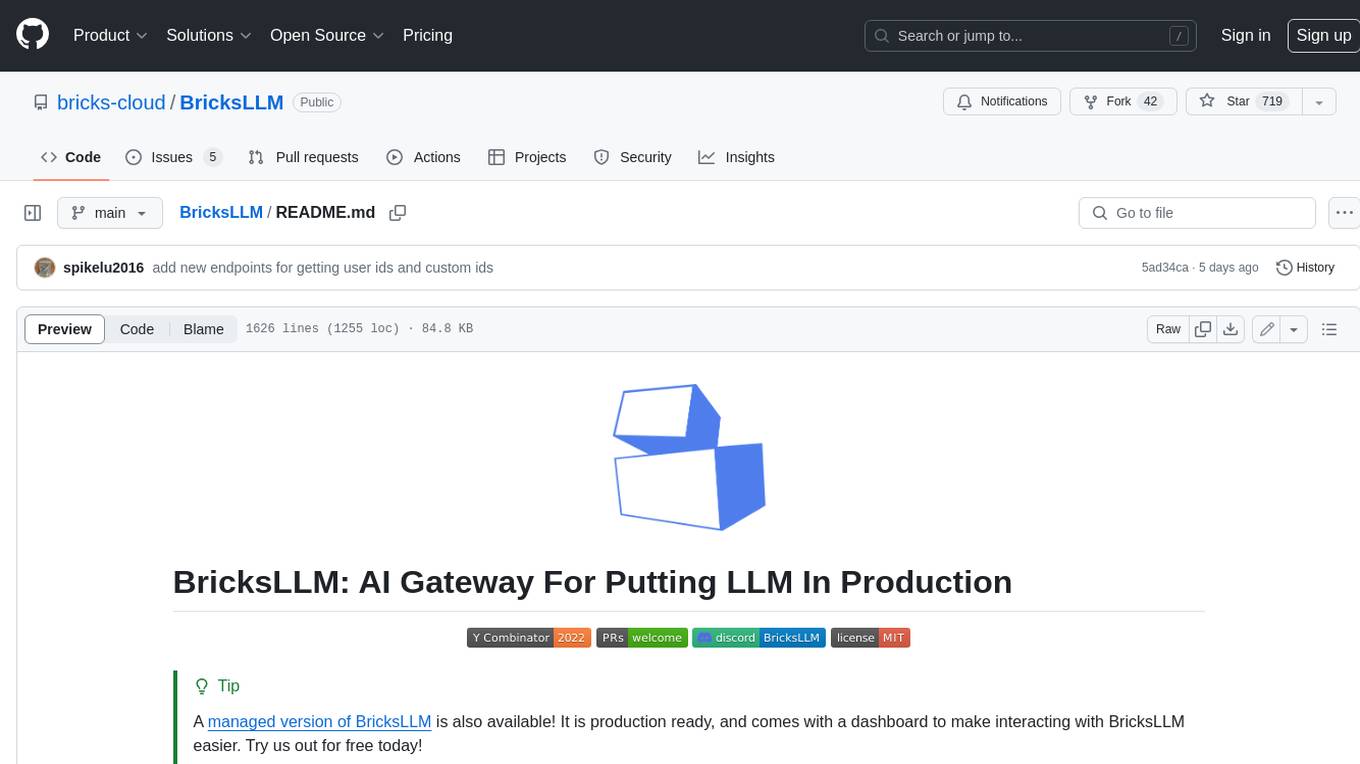
BricksLLM
BricksLLM is a cloud native AI gateway written in Go. Currently, it provides native support for OpenAI, Anthropic, Azure OpenAI and vLLM. BricksLLM aims to provide enterprise level infrastructure that can power any LLM production use cases. Here are some use cases for BricksLLM: * Set LLM usage limits for users on different pricing tiers * Track LLM usage on a per user and per organization basis * Block or redact requests containing PIIs * Improve LLM reliability with failovers, retries and caching * Distribute API keys with rate limits and cost limits for internal development/production use cases * Distribute API keys with rate limits and cost limits for students
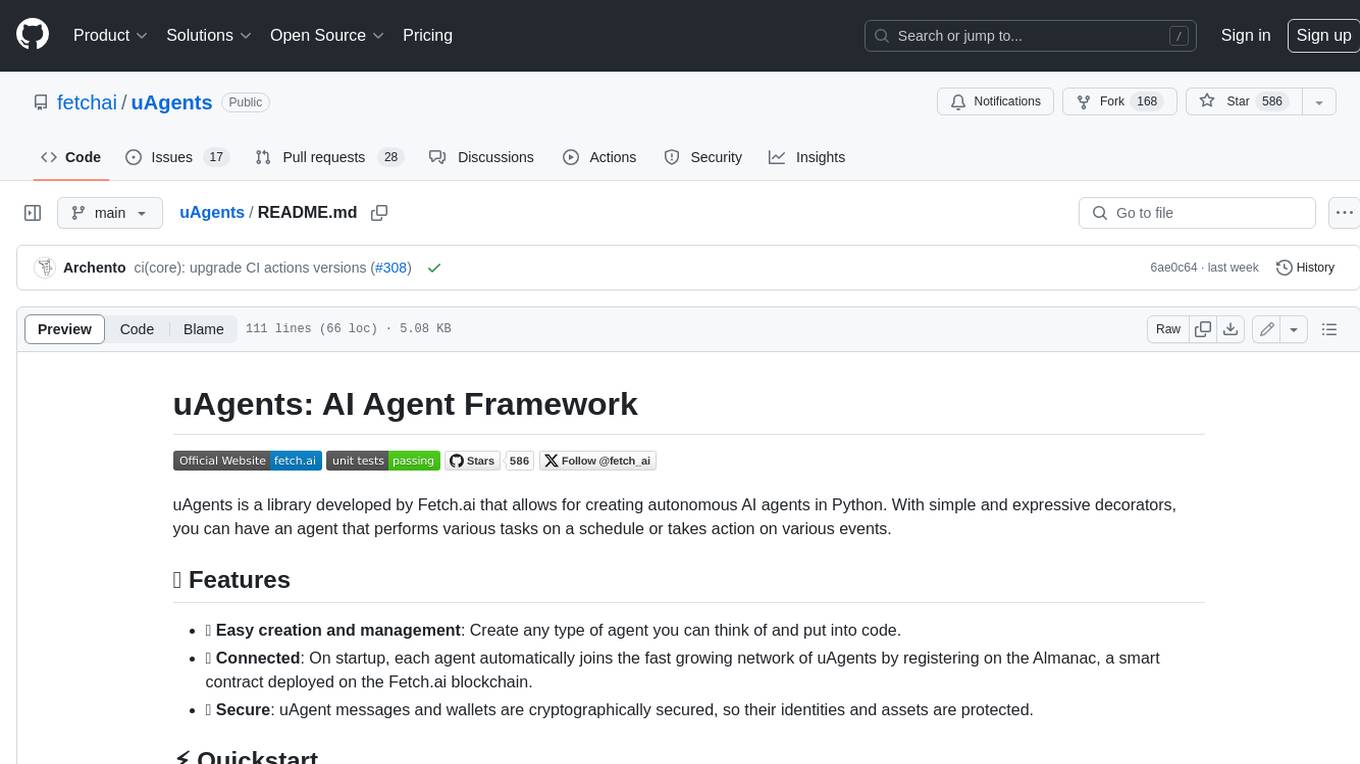
uAgents
uAgents is a Python library developed by Fetch.ai that allows for the creation of autonomous AI agents. These agents can perform various tasks on a schedule or take action on various events. uAgents are easy to create and manage, and they are connected to a fast-growing network of other uAgents. They are also secure, with cryptographically secured messages and wallets.
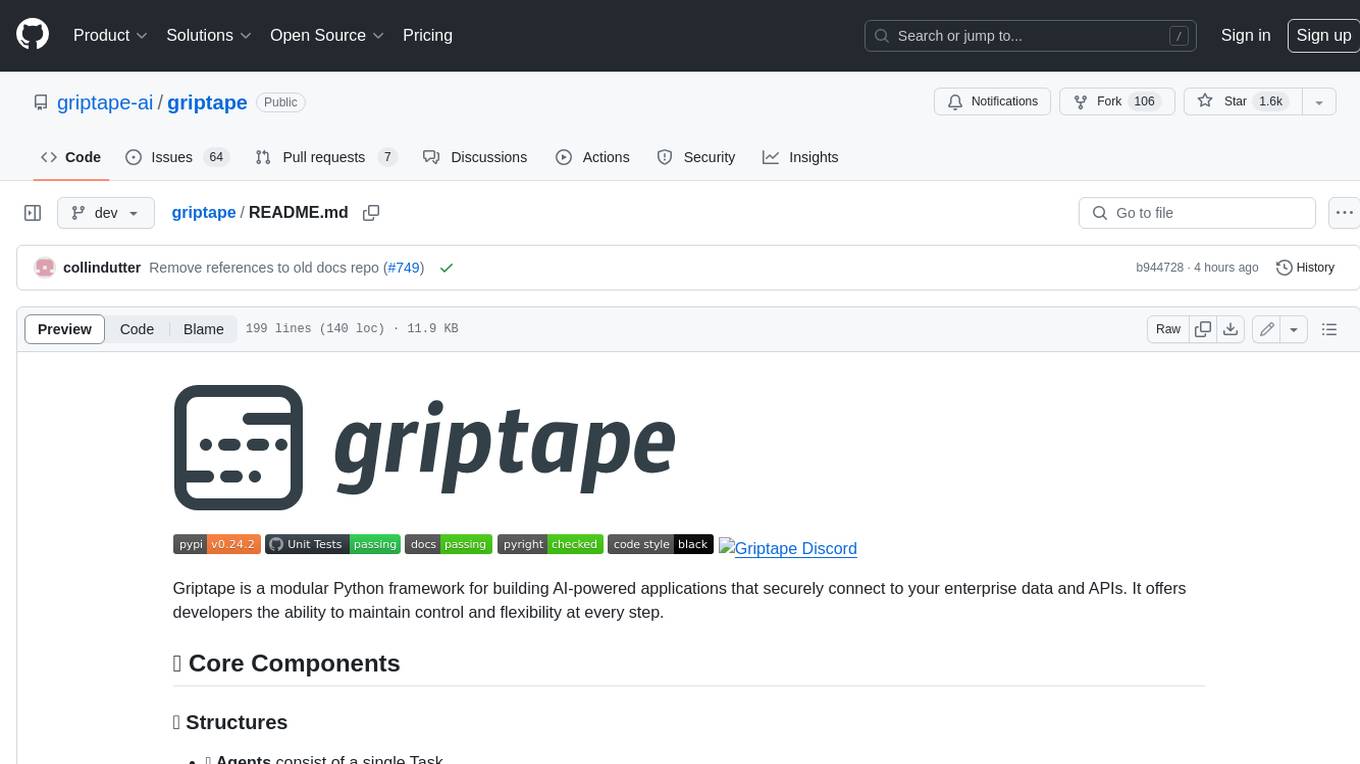
griptape
Griptape is a modular Python framework for building AI-powered applications that securely connect to your enterprise data and APIs. It offers developers the ability to maintain control and flexibility at every step. Griptape's core components include Structures (Agents, Pipelines, and Workflows), Tasks, Tools, Memory (Conversation Memory, Task Memory, and Meta Memory), Drivers (Prompt and Embedding Drivers, Vector Store Drivers, Image Generation Drivers, Image Query Drivers, SQL Drivers, Web Scraper Drivers, and Conversation Memory Drivers), Engines (Query Engines, Extraction Engines, Summary Engines, Image Generation Engines, and Image Query Engines), and additional components (Rulesets, Loaders, Artifacts, Chunkers, and Tokenizers). Griptape enables developers to create AI-powered applications with ease and efficiency.