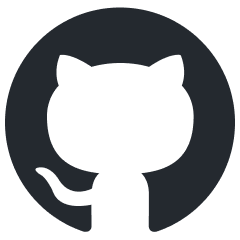
chatgpt-cli
ChatGPT CLI is a versatile tool for interacting with LLM models through OpenAI and Azure, as well as models from Perplexity AI and Llama. It supports prompts and history tracking for seamless, context-aware interactions. With extensive configuration options, it’s designed for both users and developers to create a customized GPT experience.
Stars: 661
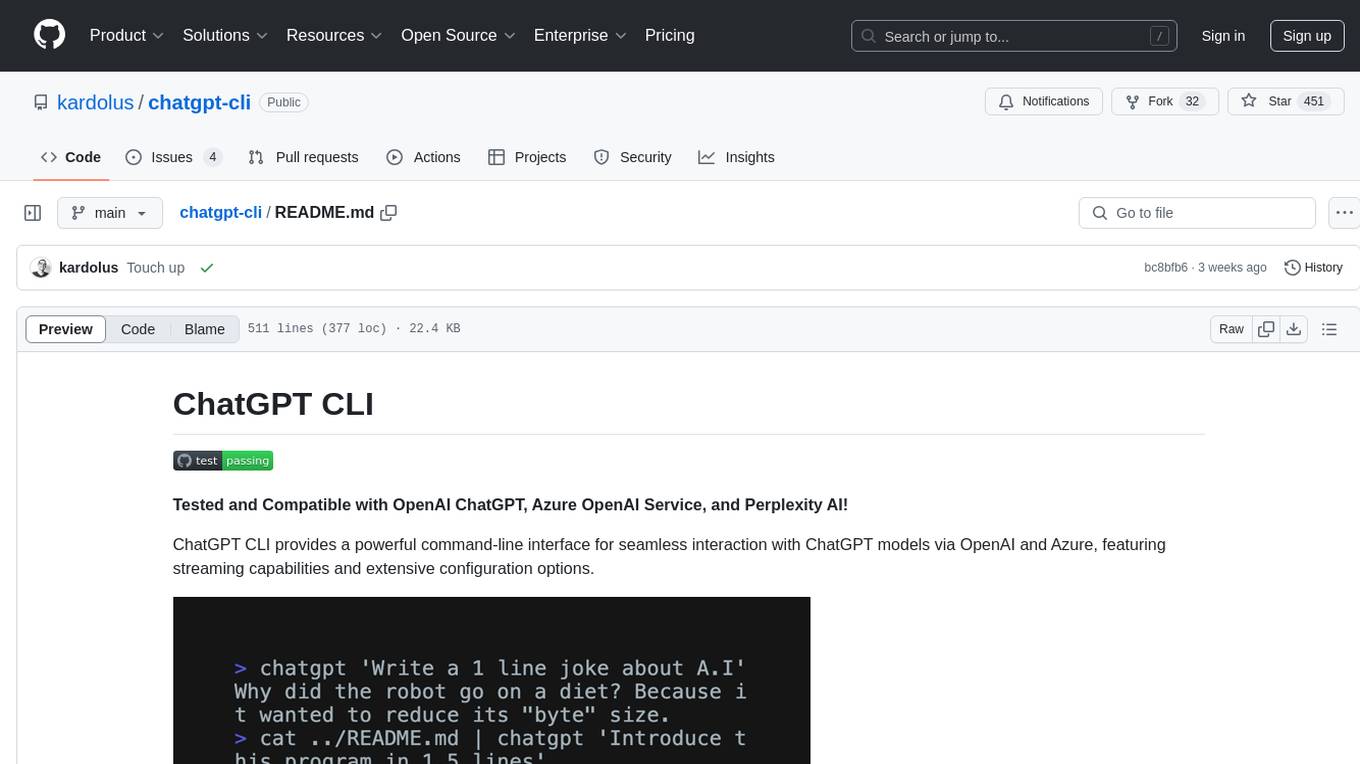
ChatGPT CLI provides a powerful command-line interface for seamless interaction with ChatGPT models via OpenAI and Azure. It features streaming capabilities, extensive configuration options, and supports various modes like streaming, query, and interactive mode. Users can manage thread-based context, sliding window history, and provide custom context from any source. The CLI also offers model and thread listing, advanced configuration options, and supports GPT-4, GPT-3.5-turbo, and Perplexity's models. Installation is available via Homebrew or direct download, and users can configure settings through default values, a config.yaml file, or environment variables.
README:
Tested and Compatible with OpenAI ChatGPT, Azure OpenAI Service, Perplexity AI and Llama!
ChatGPT CLI provides a powerful command-line interface for seamless interaction with ChatGPT models via OpenAI and Azure, featuring streaming capabilities and extensive configuration options.
- Features
- Installation
- Getting Started
- Configuration
- Markdown Rendering
- Development
- Reporting Issues and Contributing
- Uninstallation
- Useful Links
- Additional Resources
- Streaming mode: Real-time interaction with the GPT model.
- Query mode: Single input-output interactions with the GPT model.
- Interactive mode: The interactive mode allows for a more conversational experience with the model. Prints the token usage when combined with query mode.
- Thread-based context management: Enjoy seamless conversations with the GPT model with individualized context for each thread, much like your experience on the OpenAI website. Each unique thread has its own history, ensuring relevant and coherent responses across different chat instances.
-
Sliding window history: To stay within token limits, the chat history automatically trims while still preserving
the necessary context. The size of this window can be adjusted through the
context-window
setting. - Custom context from any source: You can provide the GPT model with a custom context during conversation. This context can be piped in from any source, such as local files, standard input, or even another program. This flexibility allows the model to adapt to a wide range of conversational scenarios.
-
Support for images: Upload an image or provide an image URL using the
--image
flag. Note that image support may not be available for all models. You can also pipe an image directly:pngpaste - | chatgpt "What is this photo?"
-
Model listing: Access a list of available models using the
-l
or--list-models
flag. -
Thread listing: Display a list of active threads using the
--list-threads
flag. -
Advanced configuration options: The CLI supports a layered configuration system where settings can be specified
through default values, a
config.yaml
file, and environment variables. For quick adjustments, various--set-<value>
flags are provided. To verify your current settings, use the--config
or-c
flag. - Availability Note: This CLI supports gpt-4, gpt-3.5-turbo, and Perplexity’s models (e.g., llama-3.1-sonar-small-128k-online). However, the specific ChatGPT model used on chat.openai.com may not be available via the OpenAI API.
We’re excited to introduce support for prompt files with the --prompt
flag in version 1.7.1! This feature
allows you to provide a rich and detailed context for your conversations directly from a file.
The --prompt
flag lets you specify a file containing the initial context or instructions for your ChatGPT
conversation. This is especially useful when you have detailed instructions or context that you want to reuse across
different conversations.
To use the --prompt
flag, pass the path of your prompt file like this:
chatgpt --prompt path/to/your/prompt.md "Use a pipe or provide a query here"
The contents of prompt.md
will be read and used as the initial context for the conversation, while the query you
provide directly will serve as the specific question or task you want to address.
Here’s a fun example where you can use the output of a git diff
command as a prompt:
git diff | chatgpt --prompt ../prompts/write_pull-request.md
In this example, the content from the write_pull-request.md
prompt file is used to guide the model's response based on
the diff data from git diff
.
For a variety of ready-to-use prompts, check out this awesome prompts repository. These can serve as great starting points or inspiration for your own custom prompts!
You can install chatgpt-cli using Homebrew:
brew tap kardolus/chatgpt-cli && brew install chatgpt-cli
For a quick and easy installation without compiling, you can directly download the pre-built binary for your operating system and architecture:
curl -L -o chatgpt https://github.com/kardolus/chatgpt-cli/releases/latest/download/chatgpt-darwin-arm64 && chmod +x chatgpt && sudo mv chatgpt /usr/local/bin/
curl -L -o chatgpt https://github.com/kardolus/chatgpt-cli/releases/latest/download/chatgpt-darwin-amd64 && chmod +x chatgpt && sudo mv chatgpt /usr/local/bin/
curl -L -o chatgpt https://github.com/kardolus/chatgpt-cli/releases/latest/download/chatgpt-linux-amd64 && chmod +x chatgpt && sudo mv chatgpt /usr/local/bin/
curl -L -o chatgpt https://github.com/kardolus/chatgpt-cli/releases/latest/download/chatgpt-linux-arm64 && chmod +x chatgpt && sudo mv chatgpt /usr/local/bin/
curl -L -o chatgpt https://github.com/kardolus/chatgpt-cli/releases/latest/download/chatgpt-linux-386 && chmod +x chatgpt && sudo mv chatgpt /usr/local/bin/
curl -L -o chatgpt https://github.com/kardolus/chatgpt-cli/releases/latest/download/chatgpt-freebsd-amd64 && chmod +x chatgpt && sudo mv chatgpt /usr/local/bin/
curl -L -o chatgpt https://github.com/kardolus/chatgpt-cli/releases/latest/download/chatgpt-freebsd-arm64 && chmod +x chatgpt && sudo mv chatgpt /usr/local/bin/
Download the binary from this link and add it to your PATH.
Choose the appropriate command for your system, which will download the binary, make it executable, and move it to your /usr/local/bin directory (or %PATH% on Windows) for easy access.
-
Set the
OPENAI_API_KEY
environment variable to your ChatGPT secret key. To set the environment variable, you can add the following line to your shell profile (e.g., ~/.bashrc, ~/.zshrc, or ~/.bash_profile), replacing your_api_key with your actual key:export OPENAI_API_KEY="your_api_key"
-
To enable history tracking across CLI calls, create a ~/.chatgpt-cli directory using the command:
mkdir -p ~/.chatgpt-cli
Once this directory is in place, the CLI automatically manages the message history for each "thread" you converse with. The history operates like a sliding window, maintaining context up to a configurable token maximum. This ensures a balance between maintaining conversation context and achieving optimal performance.
By default, if a specific thread is not provided by the user, the CLI uses the default thread and stores the history at
~/.chatgpt-cli/history/default.json
. You can find more details about how to configure thethread
parameter in the Configuration section of this document. -
Try it out:
chatgpt what is the capital of the Netherlands
-
To start interactive mode, use the
-i
or--interactive
flag:chatgpt --interactive
If you want the CLI to automatically create a new thread for each session, ensure that the
auto_create_new_thread
configuration variable is set totrue
. This will create a unique thread identifier for each interactive session. -
To use the pipe feature, create a text file containing some context. For example, create a file named context.txt with the following content:
Kya is a playful dog who loves swimming and playing fetch.
Then, use the pipe feature to provide this context to ChatGPT:
cat context.txt | chatgpt "What kind of toy would Kya enjoy?"
-
To list all available models, use the -l or --list-models flag:
chatgpt --list-models
-
For more options, see:
chatgpt --help
The ChatGPT CLI adopts a four-tier configuration strategy, with different levels of precedence assigned to flags, environment variables, a config.yaml file, and default values, in that respective order:
- Flags: Command-line flags have the highest precedence. Any value provided through a flag will override other configurations.
- Environment Variables: If a setting is not specified by a flag, the corresponding environment variable (prefixed with the name field from the config) will be checked.
- Config file (config.yaml): If neither a flag nor an environment variable is set, the value from the config.yaml file will be used.
- Default Values: If no value is specified through flags, config.yaml, or environment variables, the CLI will fall back to its built-in default values.
Variable | Description | Default |
---|---|---|
name |
The prefix for environment variable overrides. | 'openai' |
thread |
The name of the current chat thread. Each unique thread name has its own context. | 'default' |
omit_history |
If true, the chat history will not be used to provide context for the GPT model. | false |
command_prompt |
The command prompt in interactive mode. Should be single-quoted. | '[%datetime] [Q%counter]' |
output_prompt |
The output prompt in interactive mode. Should be single-quoted. | '' |
command_prompt_color |
The color of the command_prompt in interactive mode. Supported colors: "red", "green", "blue", "yellow", "magenta". | '' |
output_prompt_color |
The color of the output_prompt in interactive mode. Supported colors: "red", "green", "blue", "yellow", "magenta". | '' |
auto_create_new_thread |
If set to true , a new thread with a unique identifier (e.g., int_a1b2 ) will be created for each interactive session. If false , the CLI will use the thread specified by the thread parameter. |
false |
track_token_usage |
If set to true, displays the total token usage after each query in --query mode, helping you monitor API usage. | false |
debug |
If set to true, prints the raw request and response data during API calls, useful for debugging. | false |
skip_tls_verify |
If set to true, skips TLS certificate verification, allowing insecure HTTPS requests. | false |
multiline |
If set to true, enables multiline input mode in interactive sessions. | false |
Variable | Description | Default |
---|---|---|
api_key |
Your API key. | (none for security) |
model |
The GPT model used by the application. | 'gpt-3.5-turbo' |
max_tokens |
The maximum number of tokens that can be used in a single API call. | 4096 |
context_window |
The memory limit for how much of the conversation can be remembered at one time. | 8192 |
role |
The system role | 'You are a helpful assistant.' |
temperature |
What sampling temperature to use, between 0 and 2. Higher values make the output more random; lower values make it more focused and deterministic. | 1.0 |
frequency_penalty |
Number between -2.0 and 2.0. Positive values penalize new tokens based on their existing frequency in the text so far. | 0.0 |
top_p |
An alternative to sampling with temperature, called nucleus sampling, where the model considers the results of the tokens with top_p probability mass. | 1.0 |
presence_penalty |
Number between -2.0 and 2.0. Positive values penalize new tokens based on whether they appear in the text so far. | 0.0 |
seed |
Sets the seed for deterministic sampling (Beta). Repeated requests with the same seed and parameters aim to return the same result. | 0 |
url |
The base URL for the OpenAI API. | 'https://api.openai.com' |
completions_path |
The API endpoint for completions. | '/v1/chat/completions' |
models_path |
The API endpoint for accessing model information. | '/v1/models' |
auth_header |
The header used for authorization in API requests. | 'Authorization' |
auth_token_prefix |
The prefix to be added before the token in the auth_header . |
'Bearer ' |
By default, ChatGPT CLI stores configuration and history files in the ~/.chatgpt-cli
directory. However, you can
easily
override these locations by setting environment variables, allowing you to store configuration and history in custom
directories.
Environment Variable | Description | Default Location |
---|---|---|
OPENAI_CONFIG_HOME |
Overrides the default config directory path. | ~/.chatgpt-cli |
OPENAI_DATA_HOME |
Overrides the default data directory path. | ~/.chatgpt-cli/history |
To change the default configuration or data directories, set the appropriate environment variables:
export OPENAI_CONFIG_HOME="/custom/config/path"
export OPENAI_DATA_HOME="/custom/data/path"
If these environment variables are not set, the application defaults to ~/.chatgpt-cli for configuration files and ~ /.chatgpt-cli/history for history.
-
%date
: The current date in the formatYYYY-MM-DD
. -
%time
: The current time in the formatHH:MM:SS
. -
%datetime
: The current date and time in the formatYYYY-MM-DD HH:MM:SS
. -
%counter
: The total number of queries in the current session. -
%usage
: The usage in total tokens used (only works in query mode).
The defaults can be overridden by providing your own values in the user configuration file. The structure of this file
mirrors that of the default configuration. For instance, to override
the model
and max_tokens
parameters, your file might look like this:
model: gpt-3.5-turbo-16k
max_tokens: 4096
This alters the model
to gpt-3.5-turbo-16k
and adjusts max_tokens
to 4096
. All other options, such as url
, completions_path
, and models_path
, can similarly be modified. If the user configuration file cannot be accessed or
is missing, the application will resort to the default configuration.
Another way to adjust values without manually editing the configuration file is by using environment variables.
The name
attribute forms the prefix for these variables. As an example, the model
can be modified using
the OPENAI_MODEL
environment variable. Similarly, to disable history during the execution of a command, use:
OPENAI_OMIT_HISTORY=true chatgpt what is the capital of Denmark?
This approach is especially beneficial for temporary changes or for testing varying configurations.
Moreover, you can use the --config
or -c
flag to view the present configuration. This handy feature allows users to
swiftly verify their current settings without the need to manually inspect the configuration files.
chatgpt --config
Executing this command will display the active configuration, including any overrides instituted by environment variables or the user configuration file.
To facilitate convenient adjustments, the ChatGPT CLI provides flags for swiftly modifying the model
, thread
, context-window
and max_tokens
parameters in your user configured config.yaml
. These flags are --set-model
, --set-thread
, --set-context-window
and --set-max-tokens
.
For instance, to update the model, use the following command:
chatgpt --set-model gpt-3.5-turbo-16k
This feature allows for rapid changes to key configuration parameters, optimizing your experience with the ChatGPT CLI.
For Azure, use a configuration similar to:
name: azure
api_key: <your_key>
model: <not relevant, read from the completions path>
max_tokens: 4096
context_window: 8192
role: You are a helpful assistant.
temperature: 1
top_p: 1
frequency_penalty: 0
presence_penalty: 0
thread: default
omit_history: false
url: https://<your_resource>.openai.azure.com
completions_path: /openai/deployments/<your_deployment>/chat/completions?api-version=<your_api>
models_path: /v1/models
auth_header: api-key
auth_token_prefix: " "
command_prompt: '[%datetime] [Q%counter]'
auto_create_new_thread: false
track_token_usage: false
debug: false
You can set the API key either in the config.yaml file as shown above or export it as an environment variable:
export AZURE_API_KEY=<your_key>
For Perplexity, use a configuration similar to:
name: perplexity
api_key: ""
model: llama-3.1-sonar-small-128k-online
max_tokens: 4096
context_window: 8192
role: Be precise and concise.
temperature: 1
top_p: 1
frequency_penalty: 0
presence_penalty: 0
thread: test
omit_history: false
url: https://api.perplexity.ai
completions_path: /chat/completions
models_path: /models
auth_header: Authorization
auth_token_prefix: 'Bearer '
command_prompt: '[%datetime] [Q%counter] [%usage]'
auto_create_new_thread: true
track_token_usage: true
debug: false
You can set the API key either in the config.yaml file as shown above or export it as an environment variable:
export PERPLEXITY_API_KEY=<your_key>
You can set the API key either in the config.yaml
file as shown above or export it as an environment variable:
export AZURE_API_KEY=<your_key>
Enhance your CLI experience with our new autocompletion feature for command flags!
Autocompletion is currently supported for the following shells: Bash, Zsh, Fish, and PowerShell. To activate flag completion in your current shell session, execute the appropriate command based on your shell:
-
Bash
. <(chatgpt --set-completions bash)
-
Zsh
. <(chatgpt --set-completions zsh)
-
Fish
chatgpt --set-completions fish | source
-
PowerShell
chatgpt --set-completions powershell | Out-String | Invoke-Expression
For added convenience, you can make autocompletion persist across all new shell sessions by adding the appropriate sourcing command to your shell's startup file. Here are the files typically used for each shell:
-
Bash: Add to
.bashrc
or.bash_profile
-
Zsh: Add to
.zshrc
-
Fish: Add to
config.fish
- PowerShell: Add to your PowerShell profile script
For example, for Bash, you would add the following line to your .bashrc
file:
. <(chatgpt --set-completions bash)
This ensures that command flag autocompletion is enabled automatically every time you open a new terminal window.
You can render markdown in real-time using the mdrender.sh
script, located here. You'll first
need to
install glow.
Example:
chatgpt write a hello world program in Java | ./scripts/mdrender.sh
To start developing, set the OPENAI_API_KEY
environment variable to
your ChatGPT secret key.
The Makefile simplifies development tasks by providing several targets for testing, building, and deployment.
-
all-tests: Run all tests, including linting, formatting, and go mod tidy.
make all-tests
-
binaries: Build binaries for multiple platforms.
make binaries
-
shipit: Run the release process, create binaries, and generate release notes.
make shipit
-
updatedeps: Update dependencies and commit any changes.
make updatedeps
For more available commands, use:
make help
.\scripts\install.ps1
-
After a successful build, test the application with the following command:
./bin/chatgpt what type of dog is a Jack Russel?
-
As mentioned previously, the ChatGPT CLI supports tracking conversation history across CLI calls. This feature creates a seamless and conversational experience with the GPT model, as the history is utilized as context in subsequent interactions.
To enable this feature, you need to create a
~/.chatgpt-cli
directory using the command:mkdir -p ~/.chatgpt-cli
If you encounter any issues or have suggestions for improvements, please submit an issue on GitHub. We appreciate your feedback and contributions to help make this project better.
If for any reason you wish to uninstall the ChatGPT CLI application from your system, you can do so by following these steps:
If you installed the CLI using Homebrew you can do:
brew uninstall chatgpt-cli
And to remove the tap:
brew untap kardolus/chatgpt-cli
If you installed the binary directly, follow these steps:
-
Remove the binary:
sudo rm /usr/local/bin/chatgpt
-
Optionally, if you wish to remove the history tracking directory, you can also delete the
~/.chatgpt-cli
directory:rm -rf ~/.chatgpt-cli
-
Navigate to the location of the
chatgpt
binary in your system, which should be in your PATH. -
Delete the
chatgpt
binary. -
Optionally, if you wish to remove the history tracking, navigate to the
~/.chatgpt-cli
directory (where~
refers to your user's home directory) and delete it.
Please note that the history tracking directory ~/.chatgpt-cli
only contains conversation history and no personal
data. If you have any concerns about this, please feel free to delete this directory during uninstallation.
- Amazing Prompts
- OpenAI API Reference
- OpenAI Key Usage Dashboard
- OpenAI Pricing Page
- Perplexity API Reference
- Perplexity Key Usage Dashboard
- Perplexity Models
- "Summarize any text instantly with a single shortcut" on Medium: Dive deep into the capabilities of this CLI tool with this detailed walkthrough.
- Join the conversation on Reddit: Discuss the tool, ask questions, and share your experiences with our growing community.
Thank you for using ChatGPT CLI!
For Tasks:
Click tags to check more tools for each tasksFor Jobs:
Alternative AI tools for chatgpt-cli
Similar Open Source Tools
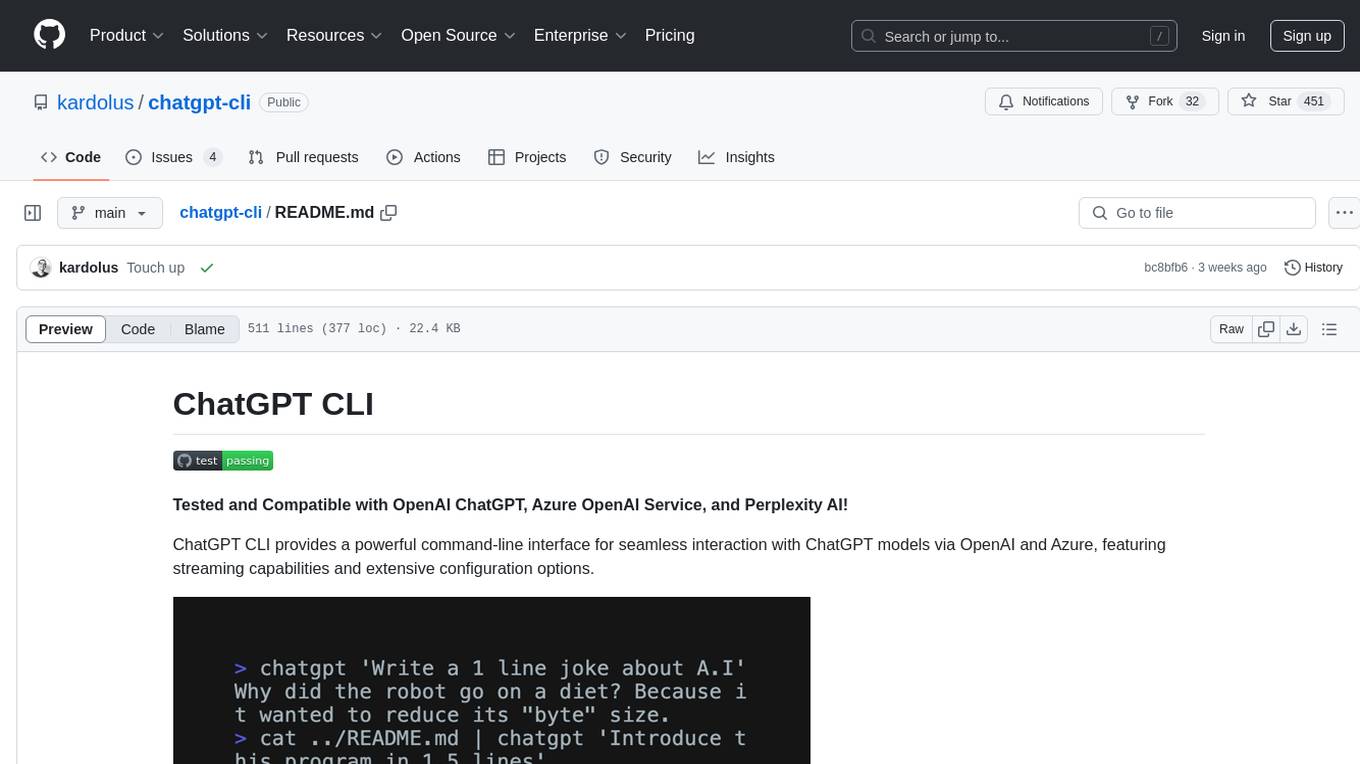
chatgpt-cli
ChatGPT CLI provides a powerful command-line interface for seamless interaction with ChatGPT models via OpenAI and Azure. It features streaming capabilities, extensive configuration options, and supports various modes like streaming, query, and interactive mode. Users can manage thread-based context, sliding window history, and provide custom context from any source. The CLI also offers model and thread listing, advanced configuration options, and supports GPT-4, GPT-3.5-turbo, and Perplexity's models. Installation is available via Homebrew or direct download, and users can configure settings through default values, a config.yaml file, or environment variables.
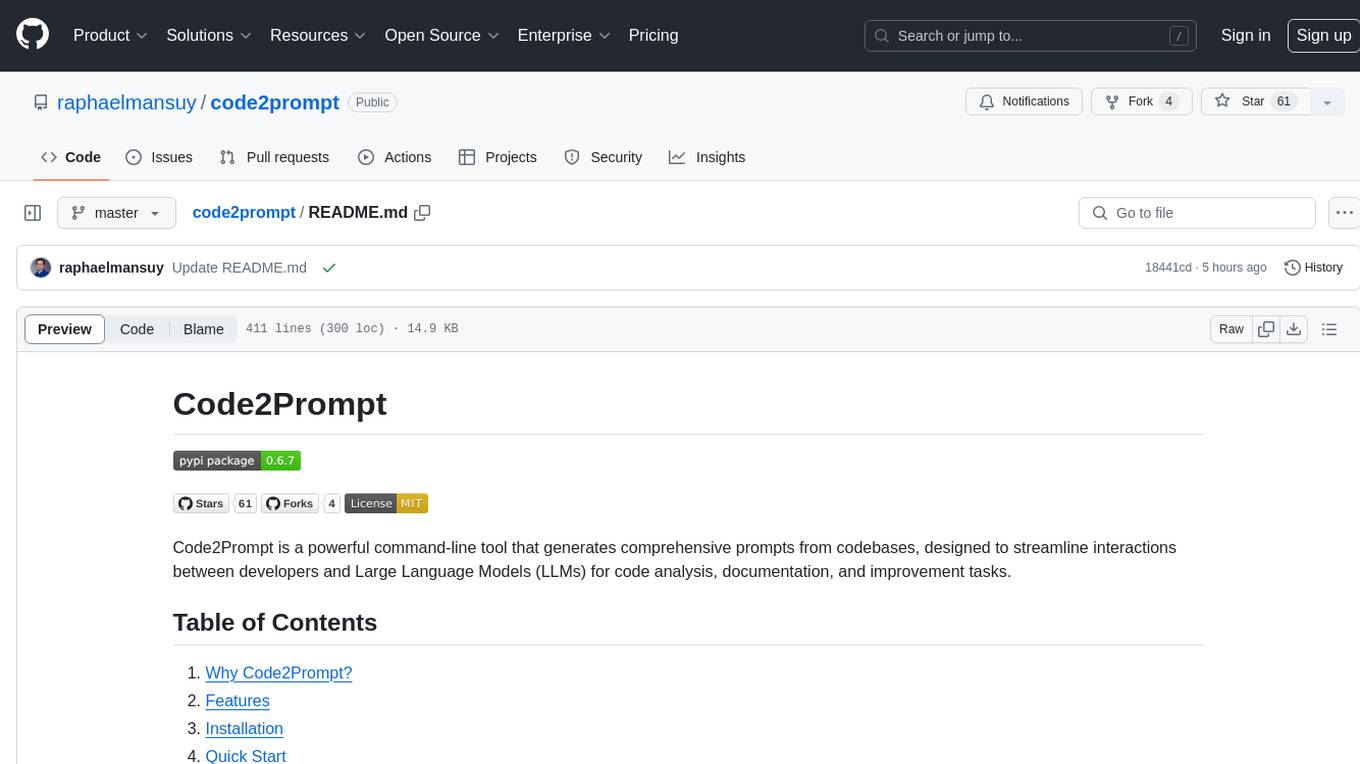
code2prompt
Code2Prompt is a powerful command-line tool that generates comprehensive prompts from codebases, designed to streamline interactions between developers and Large Language Models (LLMs) for code analysis, documentation, and improvement tasks. It bridges the gap between codebases and LLMs by converting projects into AI-friendly prompts, enabling users to leverage AI for various software development tasks. The tool offers features like holistic codebase representation, intelligent source tree generation, customizable prompt templates, smart token management, Gitignore integration, flexible file handling, clipboard-ready output, multiple output options, and enhanced code readability.
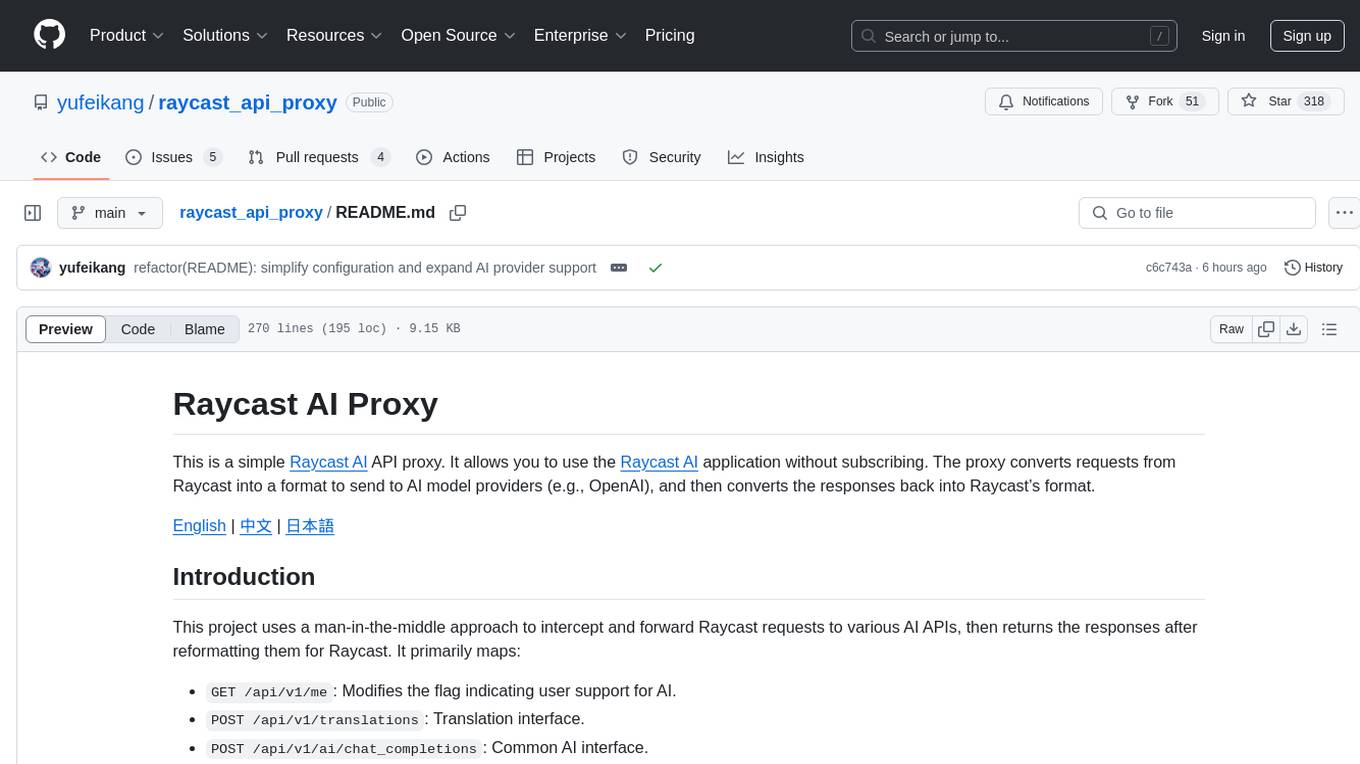
raycast_api_proxy
The Raycast AI Proxy is a tool that acts as a proxy for the Raycast AI application, allowing users to utilize the application without subscribing. It intercepts and forwards Raycast requests to various AI APIs, then reformats the responses for Raycast. The tool supports multiple AI providers and allows for custom model configurations. Users can generate self-signed certificates, add them to the system keychain, and modify DNS settings to redirect requests to the proxy. The tool is designed to work with providers like OpenAI, Azure OpenAI, Google, and more, enabling tasks such as AI chat completions, translations, and image generation.
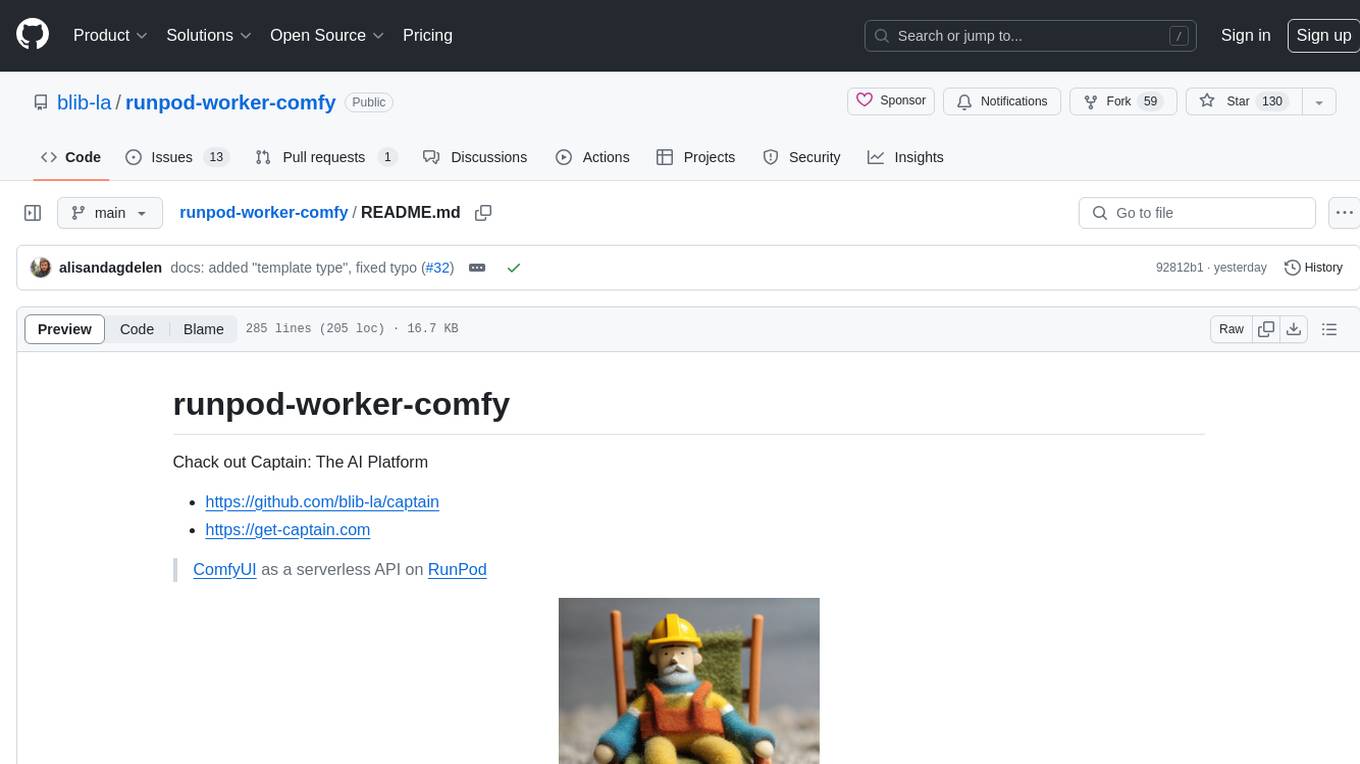
runpod-worker-comfy
runpod-worker-comfy is a serverless API tool that allows users to run any ComfyUI workflow to generate an image. Users can provide input images as base64-encoded strings, and the generated image can be returned as a base64-encoded string or uploaded to AWS S3. The tool is built on Ubuntu + NVIDIA CUDA and provides features like built-in checkpoints and VAE models. Users can configure environment variables to upload images to AWS S3 and interact with the RunPod API to generate images. The tool also supports local testing and deployment to Docker hub using Github Actions.
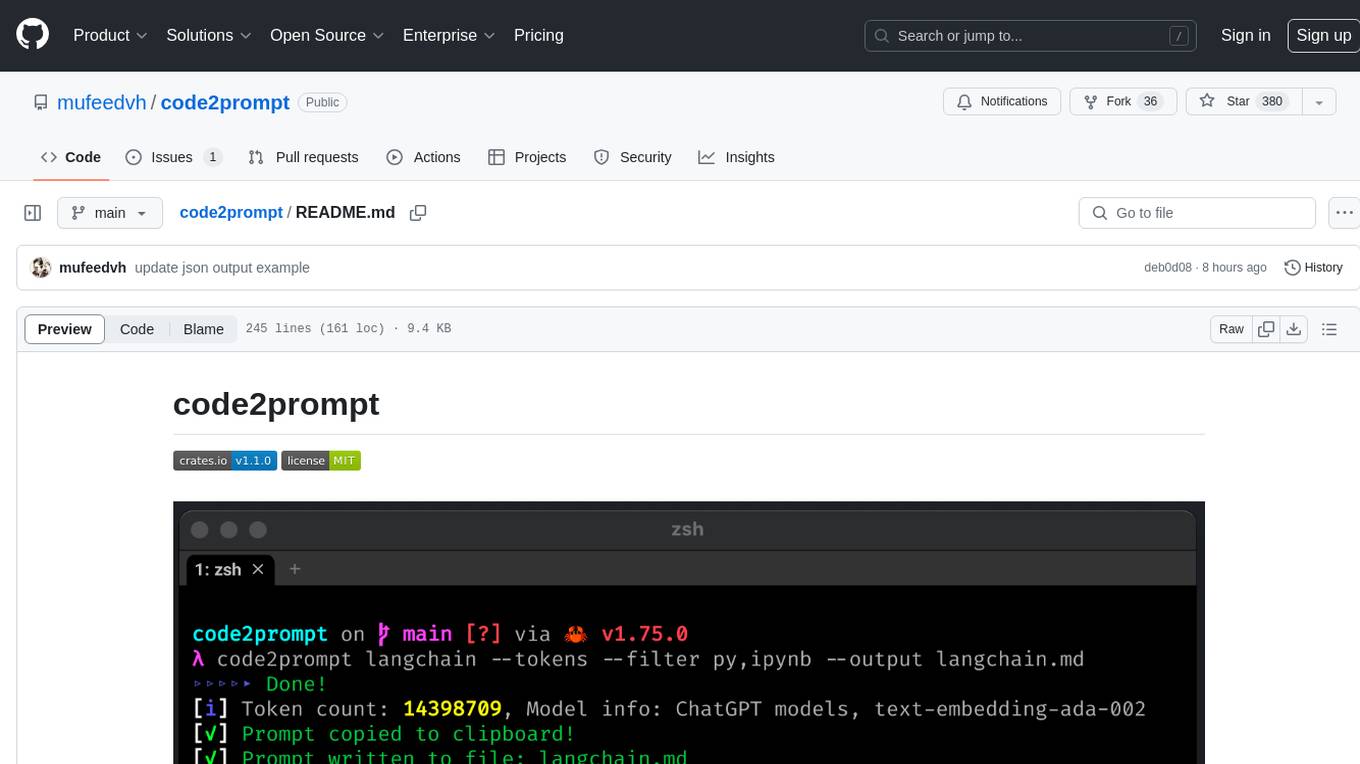
code2prompt
code2prompt is a command-line tool that converts your codebase into a single LLM prompt with a source tree, prompt templating, and token counting. It automates generating LLM prompts from codebases of any size, customizing prompt generation with Handlebars templates, respecting .gitignore, filtering and excluding files using glob patterns, displaying token count, including Git diff output, copying prompt to clipboard, saving prompt to an output file, excluding files and folders, adding line numbers to source code blocks, and more. It helps streamline the process of creating LLM prompts for code analysis, generation, and other tasks.
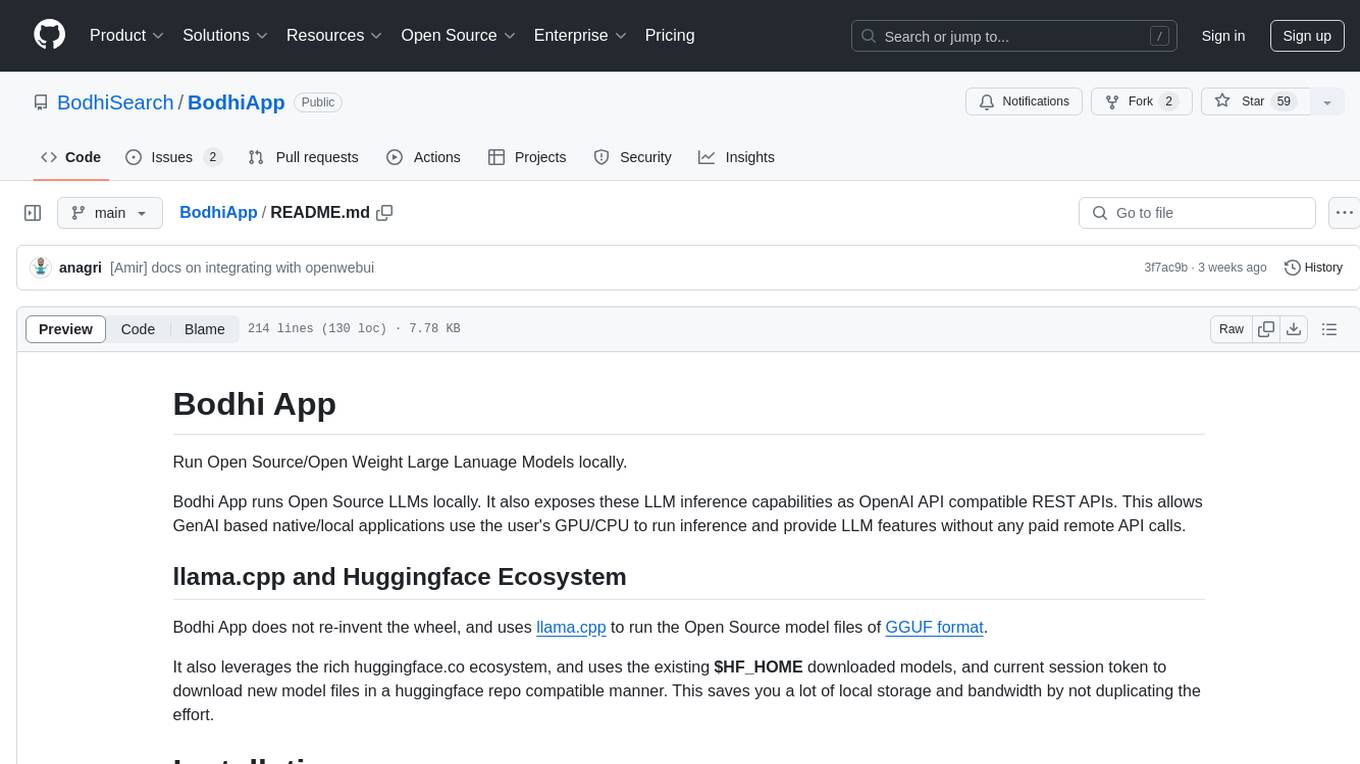
BodhiApp
Bodhi App runs Open Source Large Language Models locally, exposing LLM inference capabilities as OpenAI API compatible REST APIs. It leverages llama.cpp for GGUF format models and huggingface.co ecosystem for model downloads. Users can run fine-tuned models for chat completions, create custom aliases, and convert Huggingface models to GGUF format. The CLI offers commands for environment configuration, model management, pulling files, serving API, and more.
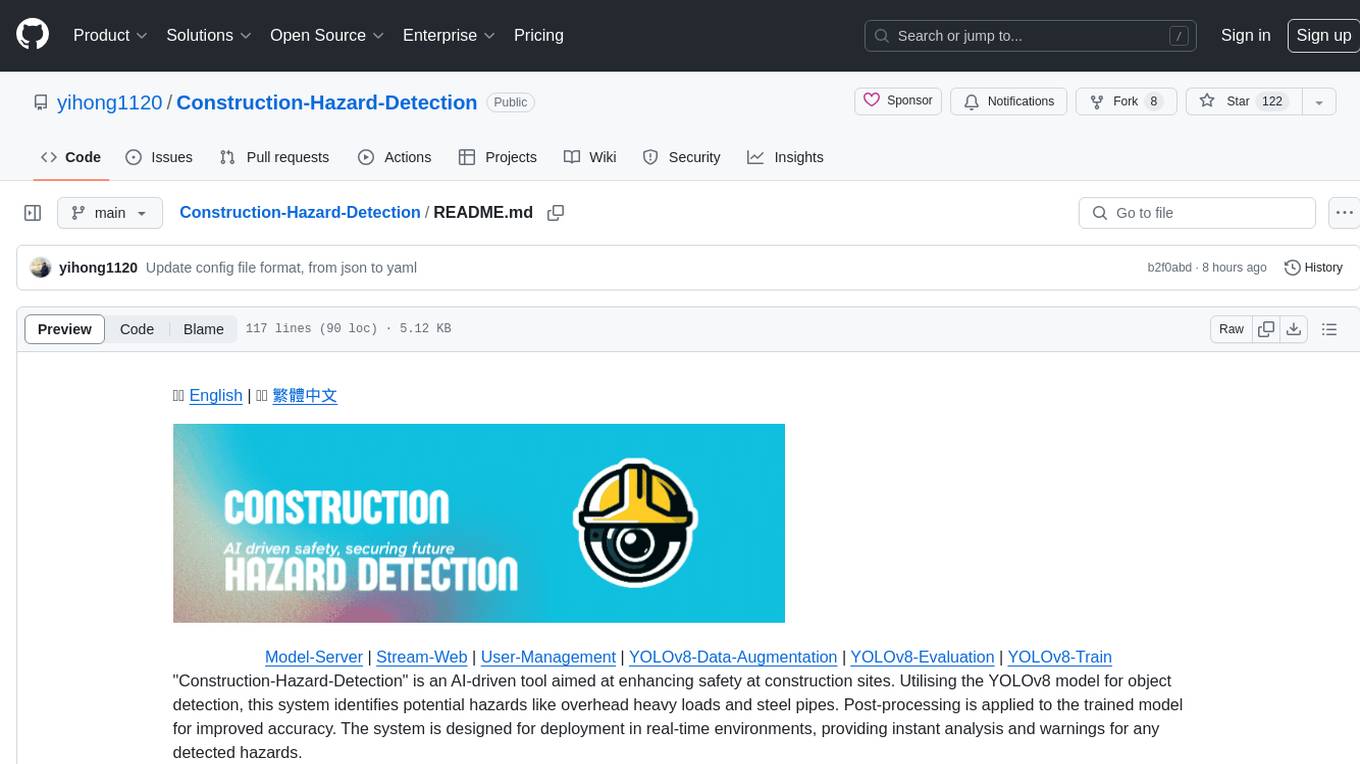
Construction-Hazard-Detection
Construction-Hazard-Detection is an AI-driven tool focused on improving safety at construction sites by utilizing the YOLOv8 model for object detection. The system identifies potential hazards like overhead heavy loads and steel pipes, providing real-time analysis and warnings. Users can configure the system via a YAML file and run it using Docker. The primary dataset used for training is the Construction Site Safety Image Dataset enriched with additional annotations. The system logs are accessible within the Docker container for debugging, and notifications are sent through the LINE messaging API when hazards are detected.
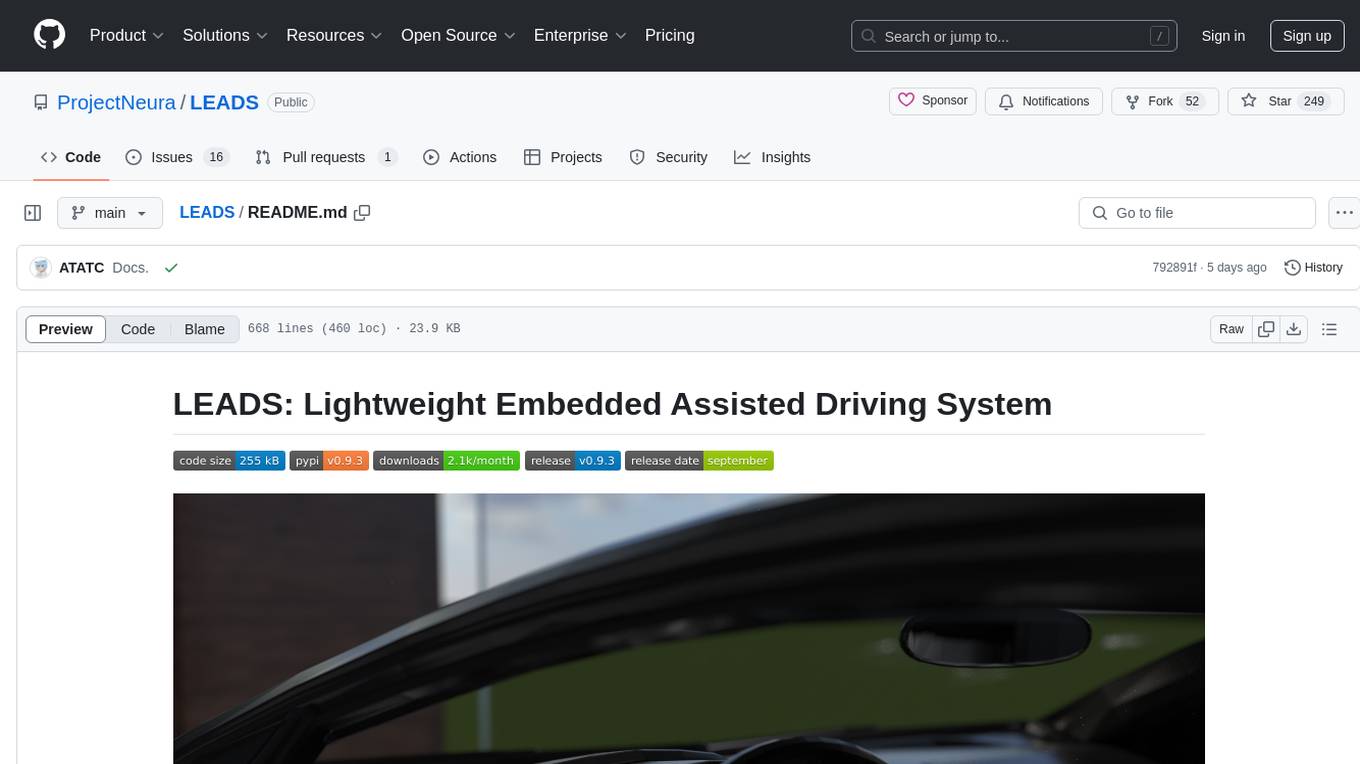
LEADS
LEADS is a lightweight embedded assisted driving system designed to simplify the development of instrumentation, control, and analysis systems for racing cars. It is written in Python and C/C++ with impressive performance. The system is customizable and provides abstract layers for component rearrangement. It supports hardware components like Raspberry Pi and Arduino, and can adapt to various hardware types. LEADS offers a modular structure with a focus on flexibility and lightweight design. It includes robust safety features, modern GUI design with dark mode support, high performance on different platforms, and powerful ESC systems for traction control and braking. The system also supports real-time data sharing, live video streaming, and AI-enhanced data analysis for driver training. LEADS VeC Remote Analyst enables transparency between the driver and pit crew, allowing real-time data sharing and analysis. The system is designed to be user-friendly, adaptable, and efficient for racing car development.
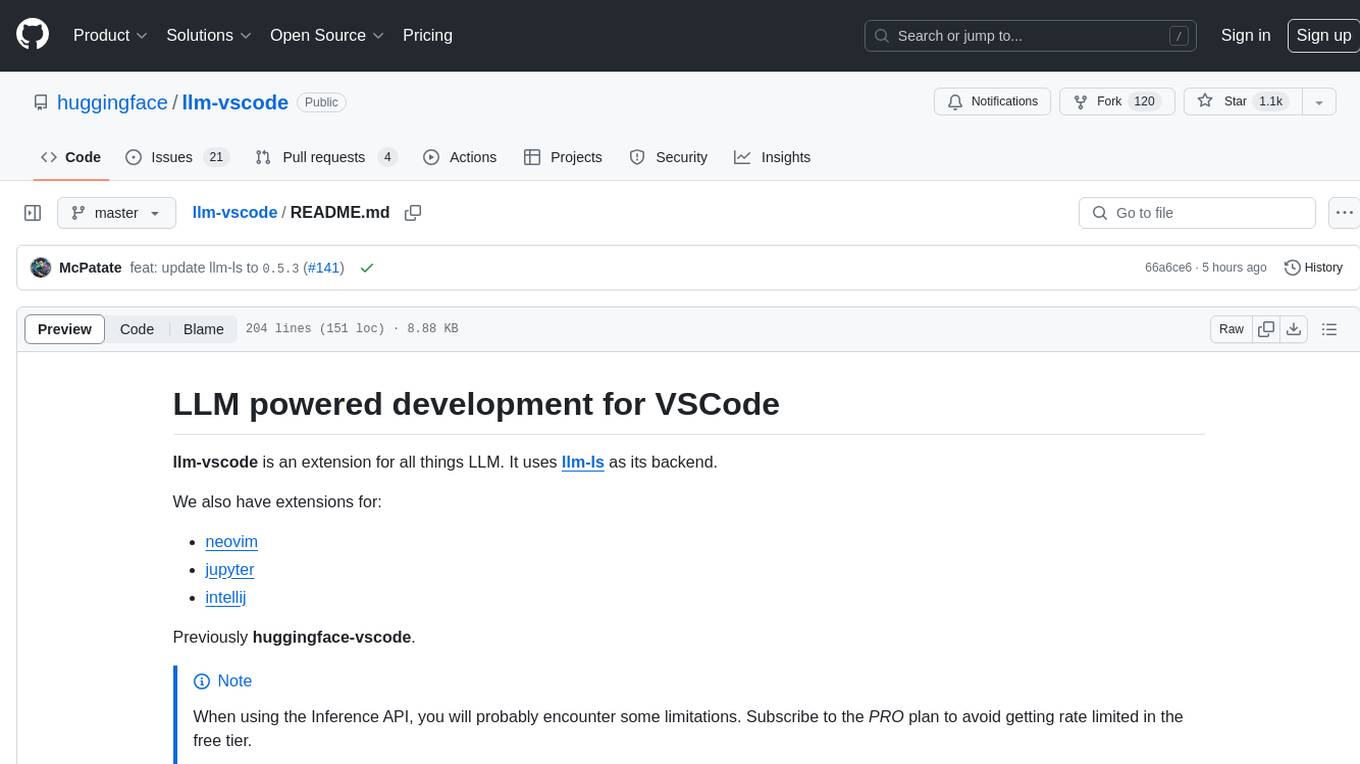
llm-vscode
llm-vscode is an extension designed for all things LLM, utilizing llm-ls as its backend. It offers features such as code completion with 'ghost-text' suggestions, the ability to choose models for code generation via HTTP requests, ensuring prompt size fits within the context window, and code attribution checks. Users can configure the backend, suggestion behavior, keybindings, llm-ls settings, and tokenization options. Additionally, the extension supports testing models like Code Llama 13B, Phind/Phind-CodeLlama-34B-v2, and WizardLM/WizardCoder-Python-34B-V1.0. Development involves cloning llm-ls, building it, and setting up the llm-vscode extension for use.
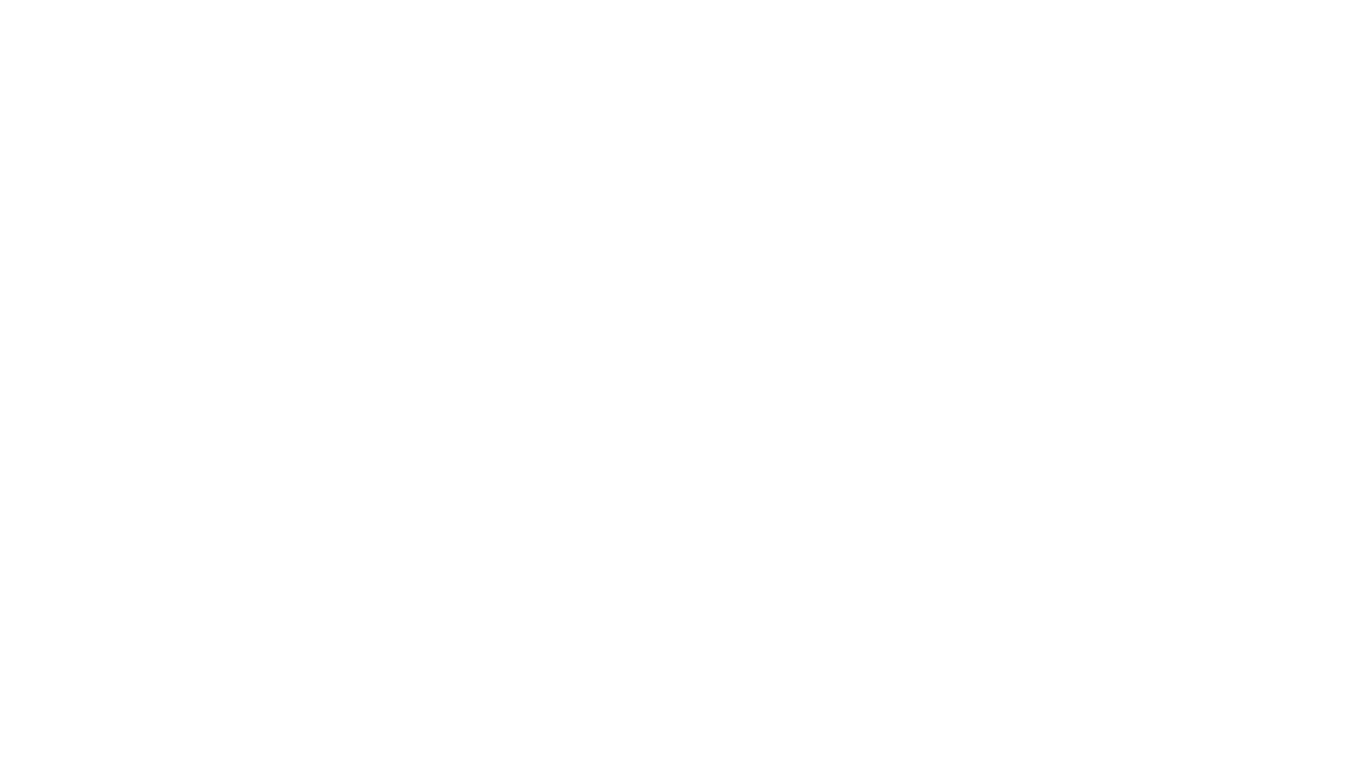
upgini
Upgini is an intelligent data search engine with a Python library that helps users find and add relevant features to their ML pipeline from various public, community, and premium external data sources. It automates the optimization of connected data sources by generating an optimal set of machine learning features using large language models, GraphNNs, and recurrent neural networks. The tool aims to simplify feature search and enrichment for external data to make it a standard approach in machine learning pipelines. It democratizes access to data sources for the data science community.
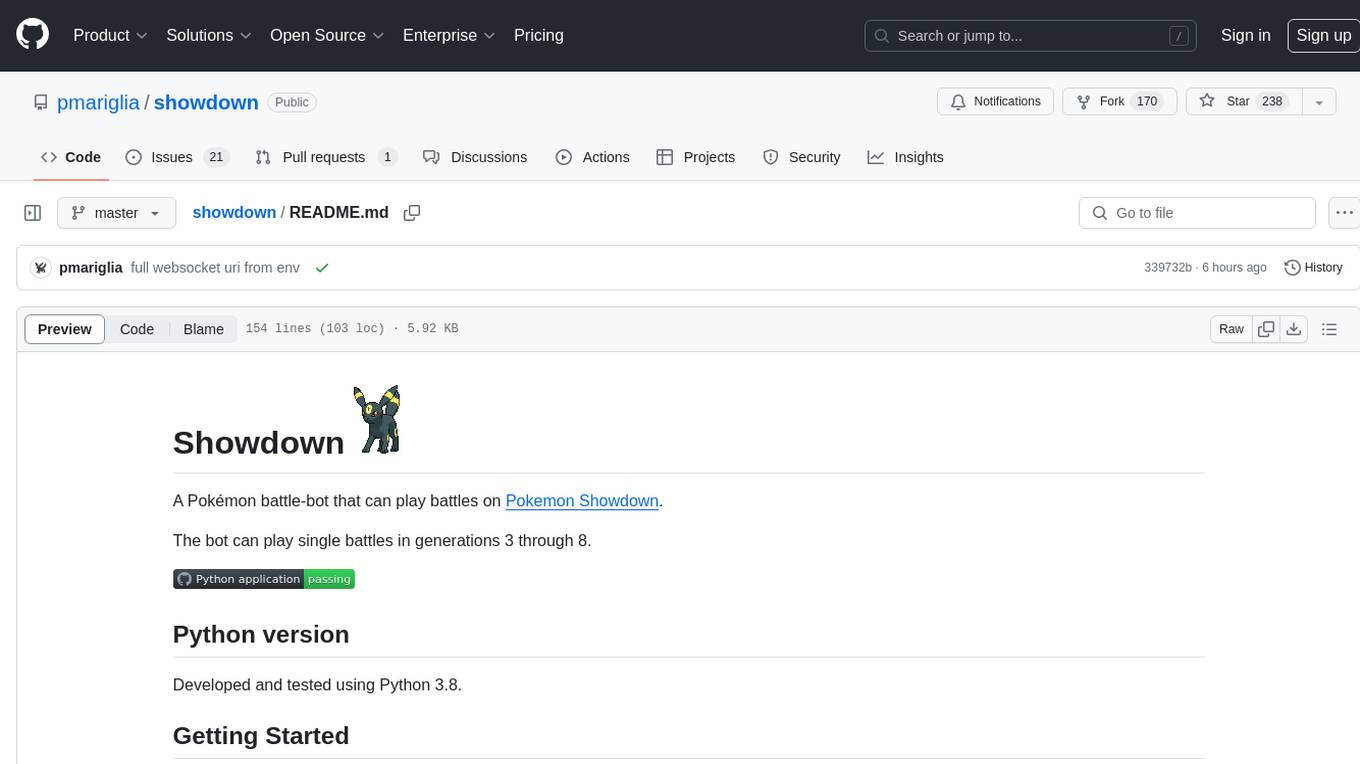
showdown
Showdown is a Pokémon battle-bot that can play battles on Pokemon Showdown. It can play single battles in generations 3 through 8. The project offers different battle bot implementations such as Safest, Nash-Equilibrium, Team Datasets, and Most Damage. Users can configure the bot using environment variables and run it either without Docker by cloning the repository and installing requirements or with Docker by building the Docker image and running it with an environment variable file. Additionally, users can write their own bot by creating a package in showdown/battle_bots with a module named main.py and implementing a find_best_move function.
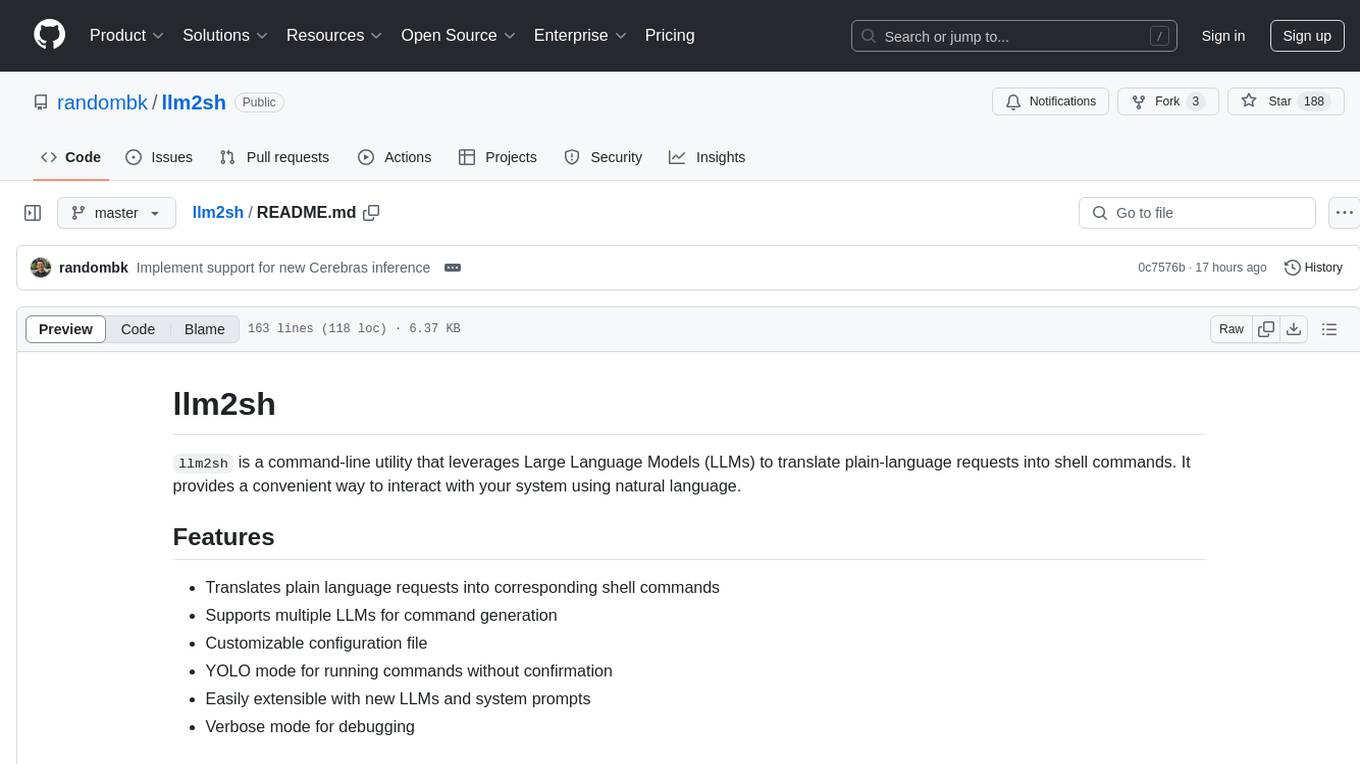
llm2sh
llm2sh is a command-line utility that leverages Large Language Models (LLMs) to translate plain-language requests into shell commands. It provides a convenient way to interact with your system using natural language. The tool supports multiple LLMs for command generation, offers a customizable configuration file, YOLO mode for running commands without confirmation, and is easily extensible with new LLMs and system prompts. Users can set up API keys for OpenAI, Claude, Groq, and Cerebras to use the tool effectively. llm2sh does not store user data or command history, and it does not record or send telemetry by itself, but the LLM APIs may collect and store requests and responses for their purposes.
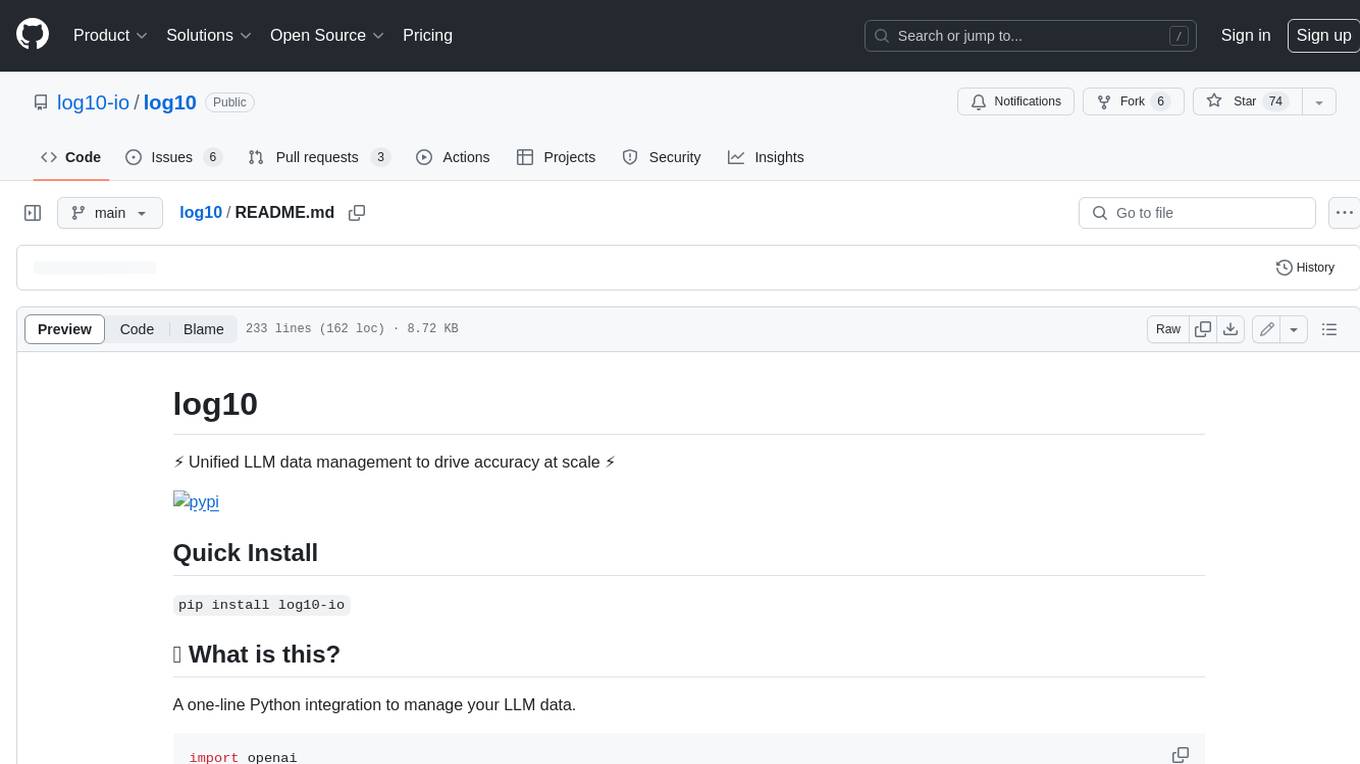
log10
Log10 is a one-line Python integration to manage your LLM data. It helps you log both closed and open-source LLM calls, compare and identify the best models and prompts, store feedback for fine-tuning, collect performance metrics such as latency and usage, and perform analytics and monitor compliance for LLM powered applications. Log10 offers various integration methods, including a python LLM library wrapper, the Log10 LLM abstraction, and callbacks, to facilitate its use in both existing production environments and new projects. Pick the one that works best for you. Log10 also provides a copilot that can help you with suggestions on how to optimize your prompt, and a feedback feature that allows you to add feedback to your completions. Additionally, Log10 provides prompt provenance, session tracking and call stack functionality to help debug prompt chains. With Log10, you can use your data and feedback from users to fine-tune custom models with RLHF, and build and deploy more reliable, accurate and efficient self-hosted models. Log10 also supports collaboration, allowing you to create flexible groups to share and collaborate over all of the above features.
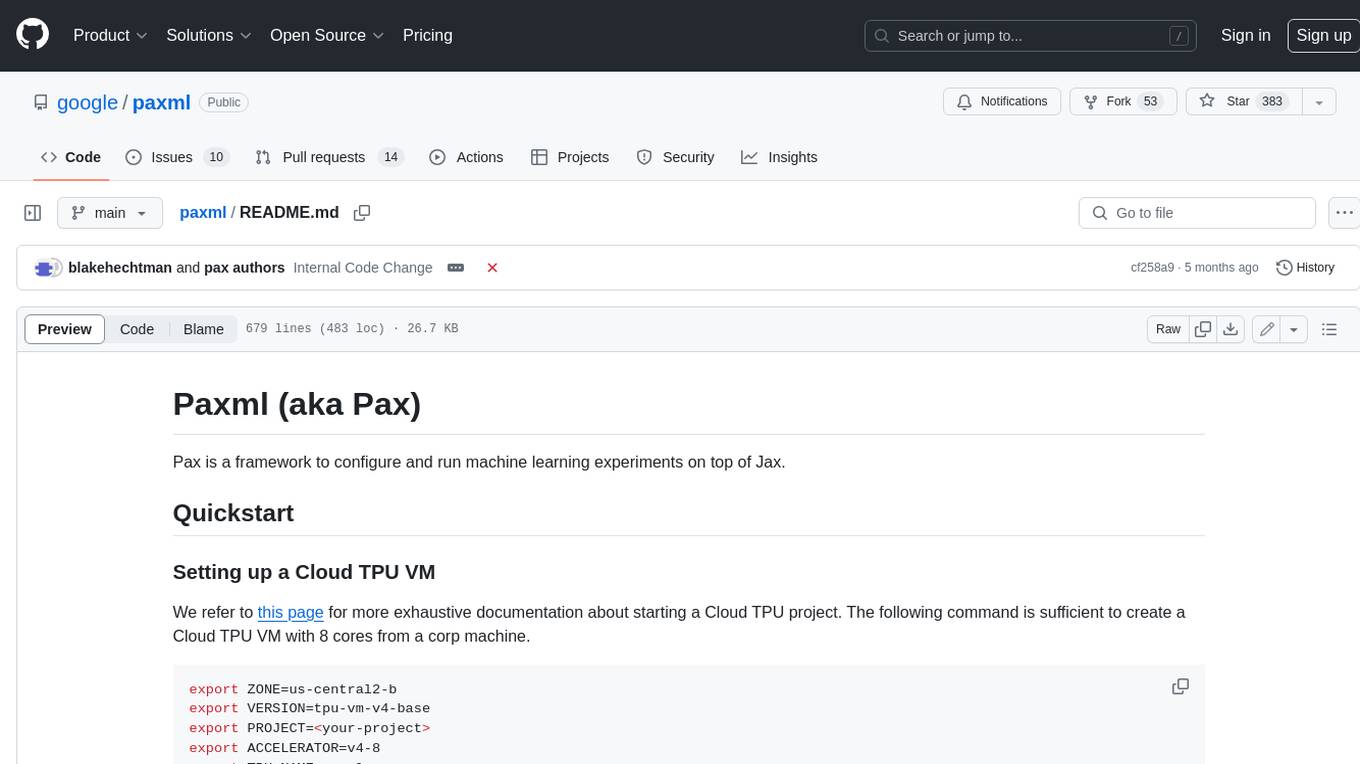
paxml
Pax is a framework to configure and run machine learning experiments on top of Jax.
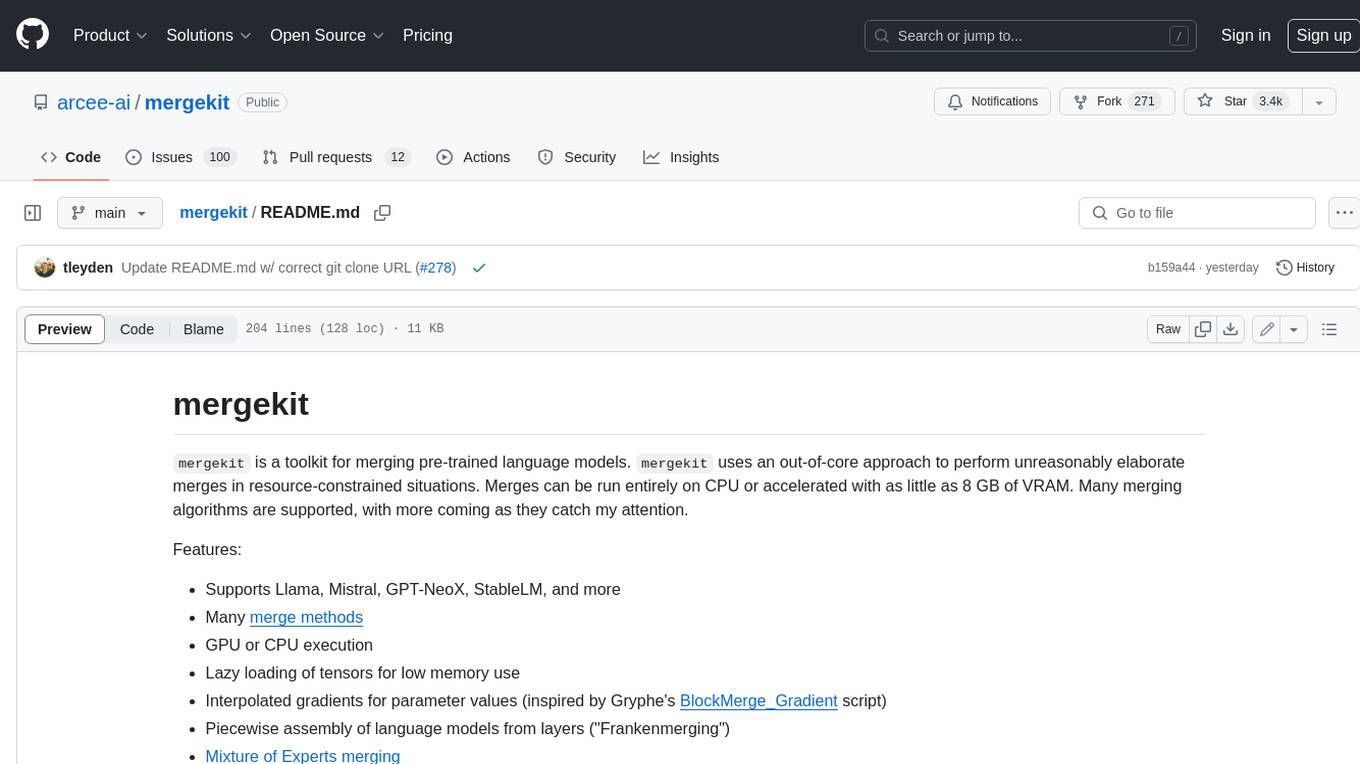
mergekit
Mergekit is a toolkit for merging pre-trained language models. It uses an out-of-core approach to perform unreasonably elaborate merges in resource-constrained situations. Merges can be run entirely on CPU or accelerated with as little as 8 GB of VRAM. Many merging algorithms are supported, with more coming as they catch my attention.
For similar tasks
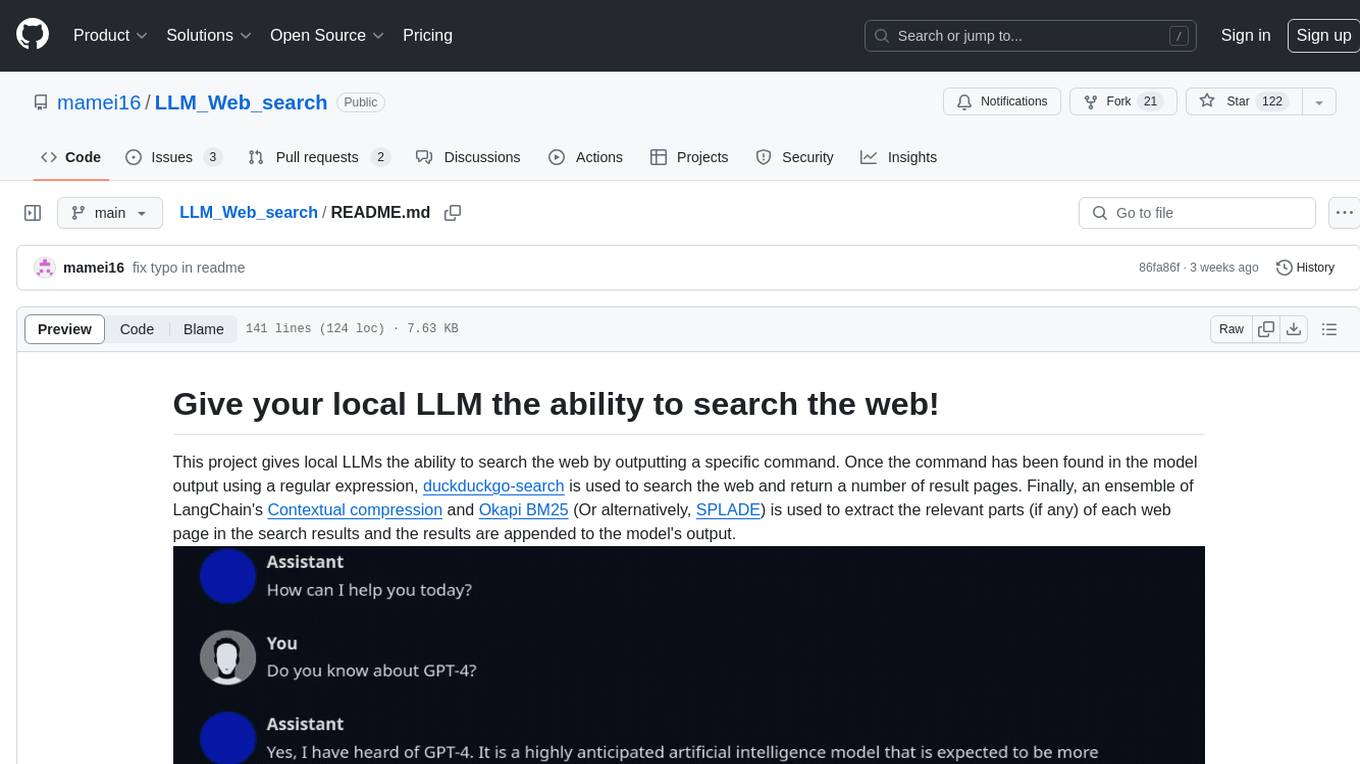
LLM_Web_search
LLM_Web_search project gives local LLMs the ability to search the web by outputting a specific command. It uses regular expressions to extract search queries from model output and then utilizes duckduckgo-search to search the web. LangChain's Contextual compression and Okapi BM25 or SPLADE are used to extract relevant parts of web pages in search results. The extracted results are appended to the model's output.
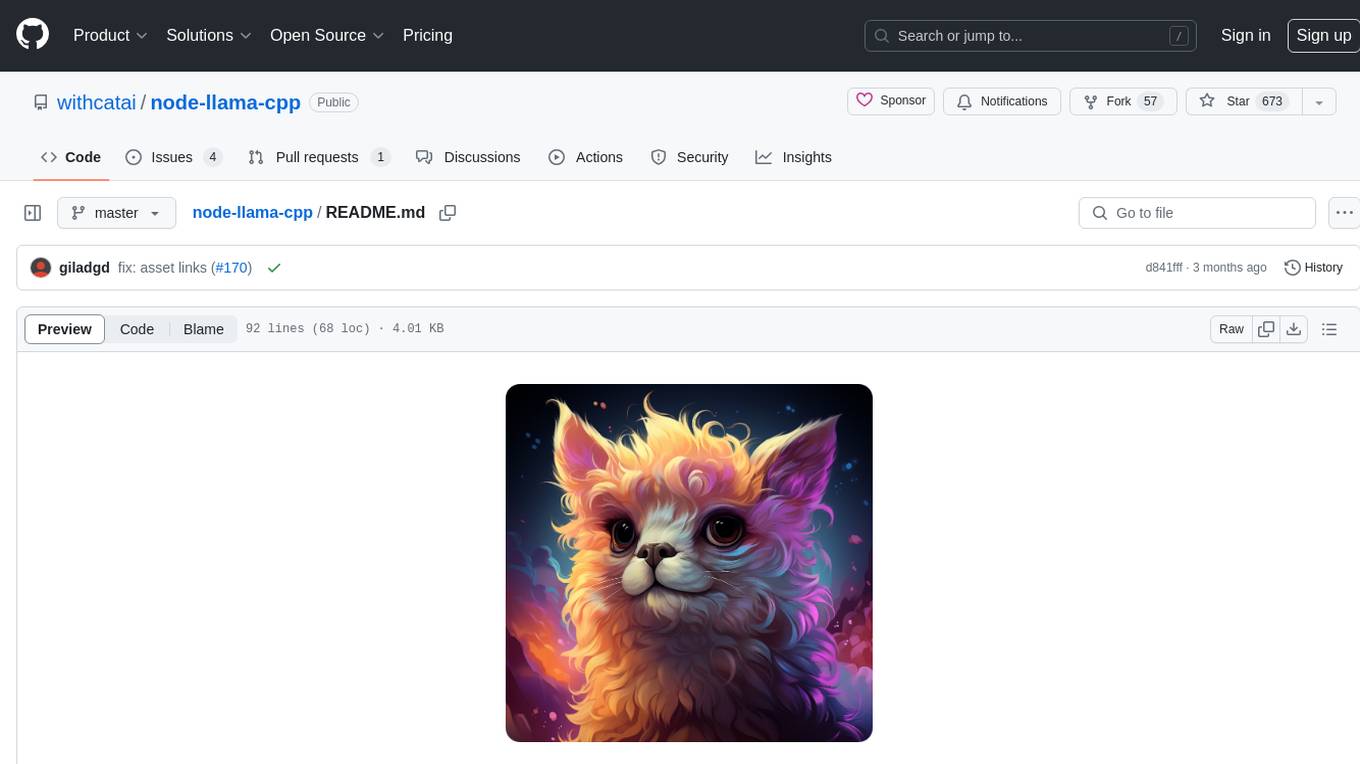
node-llama-cpp
node-llama-cpp is a tool that allows users to run AI models locally on their machines. It provides pre-built bindings with the option to build from source using cmake. Users can interact with text generation models, chat with models using a chat wrapper, and force models to generate output in a parseable format like JSON. The tool supports Metal and CUDA, offers CLI functionality for chatting with models without coding, and ensures up-to-date compatibility with the latest version of llama.cpp. Installation includes pre-built binaries for macOS, Linux, and Windows, with the option to build from source if binaries are not available for the platform.
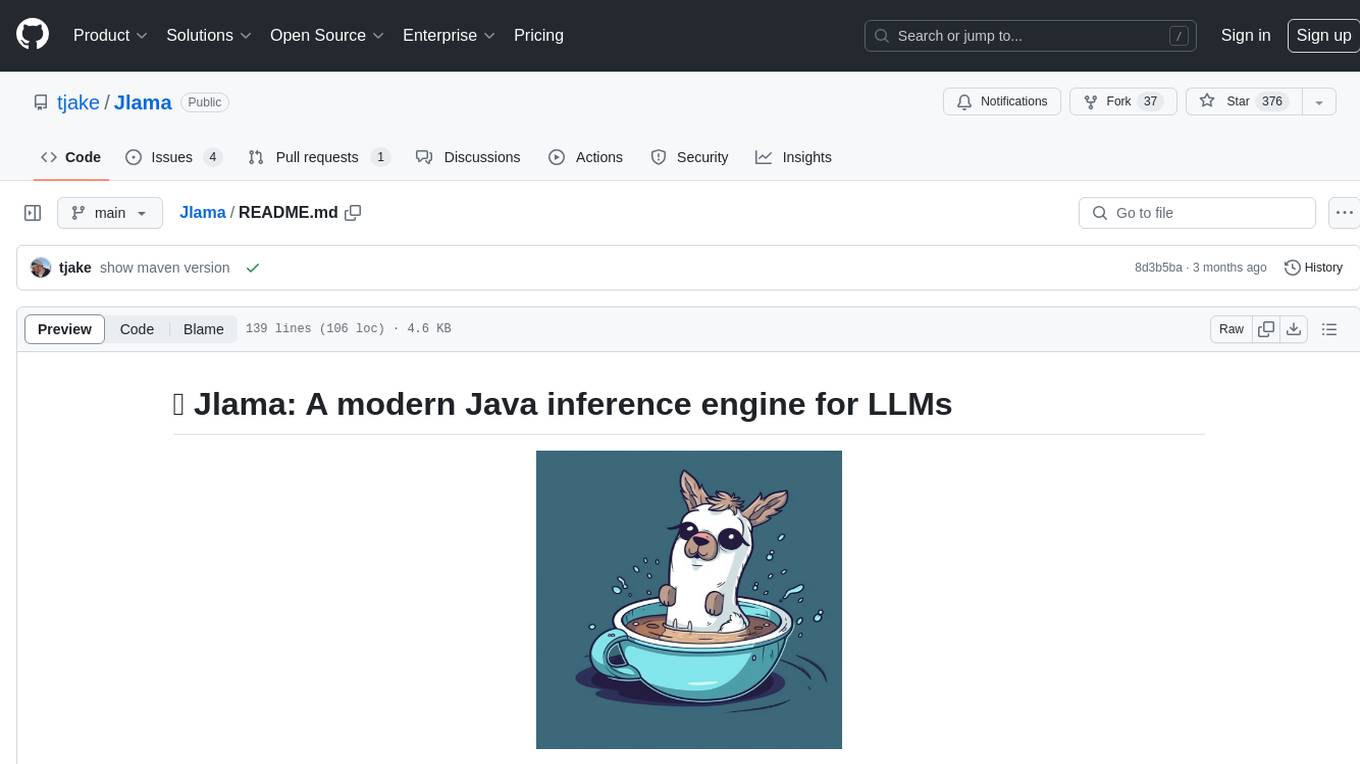
Jlama
Jlama is a modern Java inference engine designed for large language models. It supports various model types such as Gemma, Llama, Mistral, GPT-2, BERT, and more. The tool implements features like Flash Attention, Mixture of Experts, and supports different model quantization formats. Built with Java 21 and utilizing the new Vector API for faster inference, Jlama allows users to add LLM inference directly to their Java applications. The tool includes a CLI for running models, a simple UI for chatting with LLMs, and examples for different model types.
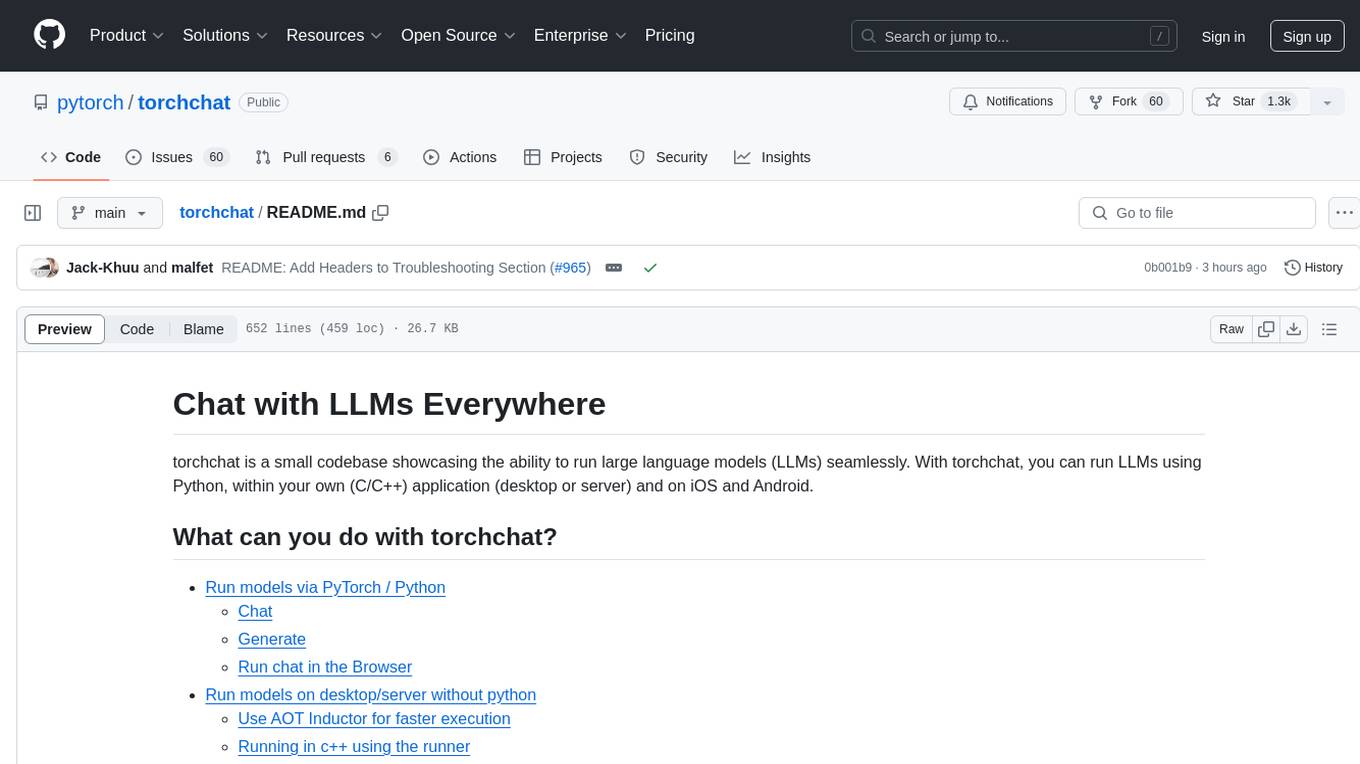
torchchat
torchchat is a codebase showcasing the ability to run large language models (LLMs) seamlessly. It allows running LLMs using Python in various environments such as desktop, server, iOS, and Android. The tool supports running models via PyTorch, chatting, generating text, running chat in the browser, and running models on desktop/server without Python. It also provides features like AOT Inductor for faster execution, running in C++ using the runner, and deploying and running on iOS and Android. The tool supports popular hardware and OS including Linux, Mac OS, Android, and iOS, with various data types and execution modes available.
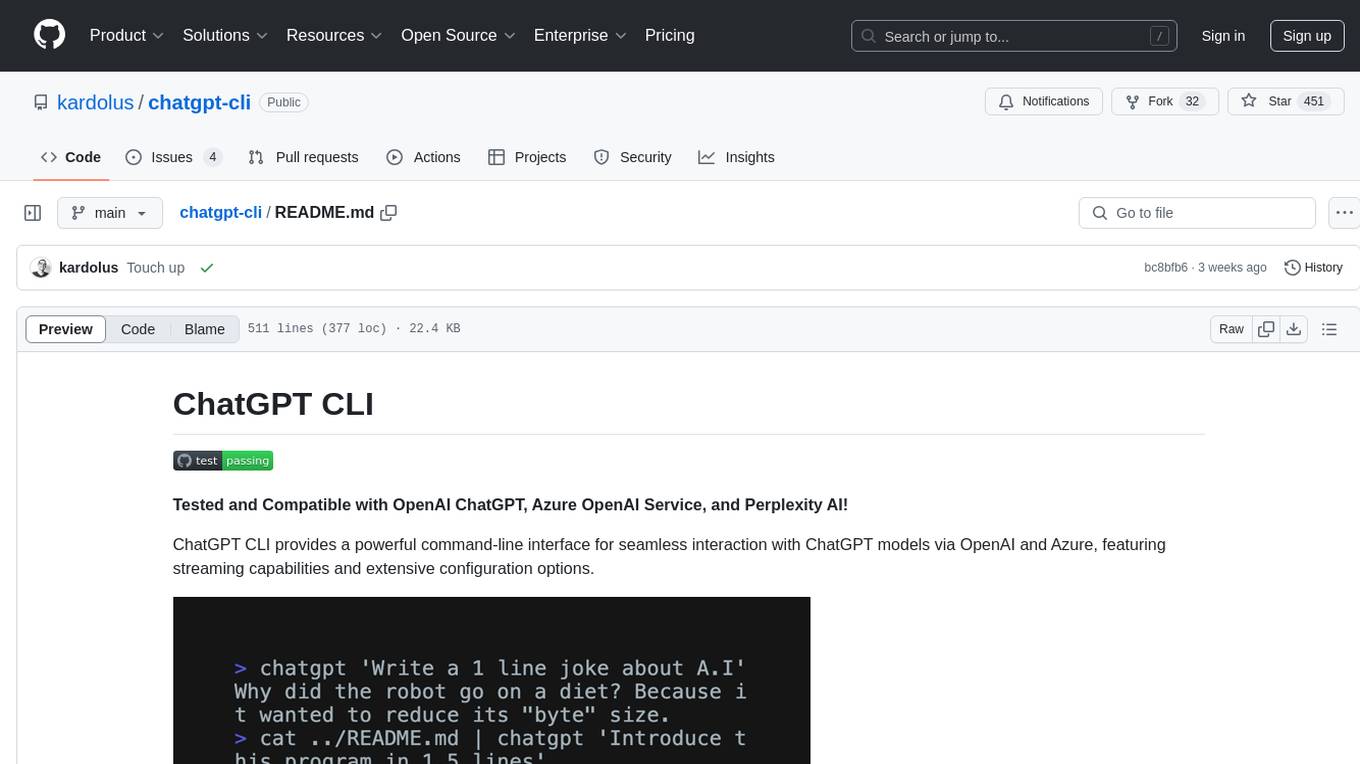
chatgpt-cli
ChatGPT CLI provides a powerful command-line interface for seamless interaction with ChatGPT models via OpenAI and Azure. It features streaming capabilities, extensive configuration options, and supports various modes like streaming, query, and interactive mode. Users can manage thread-based context, sliding window history, and provide custom context from any source. The CLI also offers model and thread listing, advanced configuration options, and supports GPT-4, GPT-3.5-turbo, and Perplexity's models. Installation is available via Homebrew or direct download, and users can configure settings through default values, a config.yaml file, or environment variables.
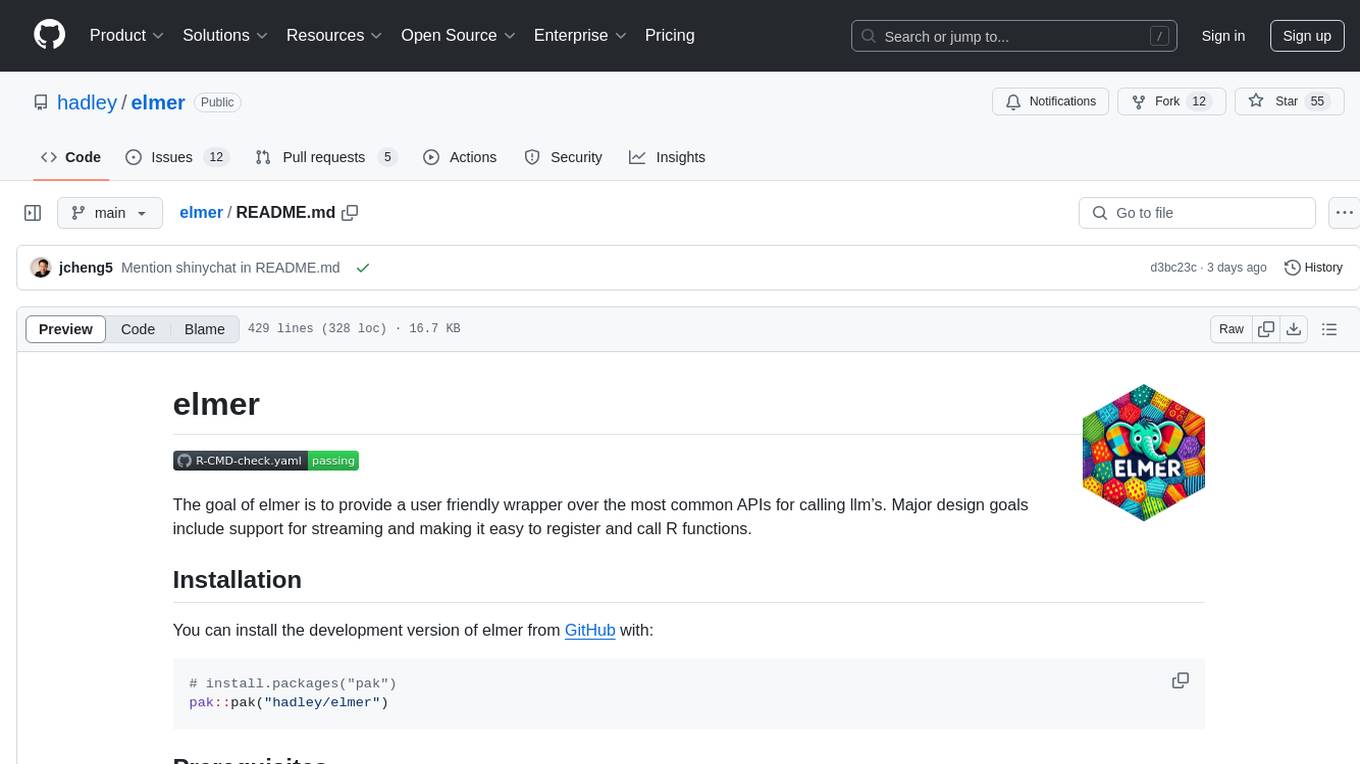
elmer
Elmer is a user-friendly wrapper over common APIs for calling llm’s, with support for streaming and easy registration and calling of R functions. Users can interact with Elmer in various ways, such as interactive chat console, interactive method call, programmatic chat, and streaming results. Elmer also supports async usage for running multiple chat sessions concurrently, useful for Shiny applications. The tool calling feature allows users to define external tools that Elmer can request to execute, enhancing the capabilities of the chat model.
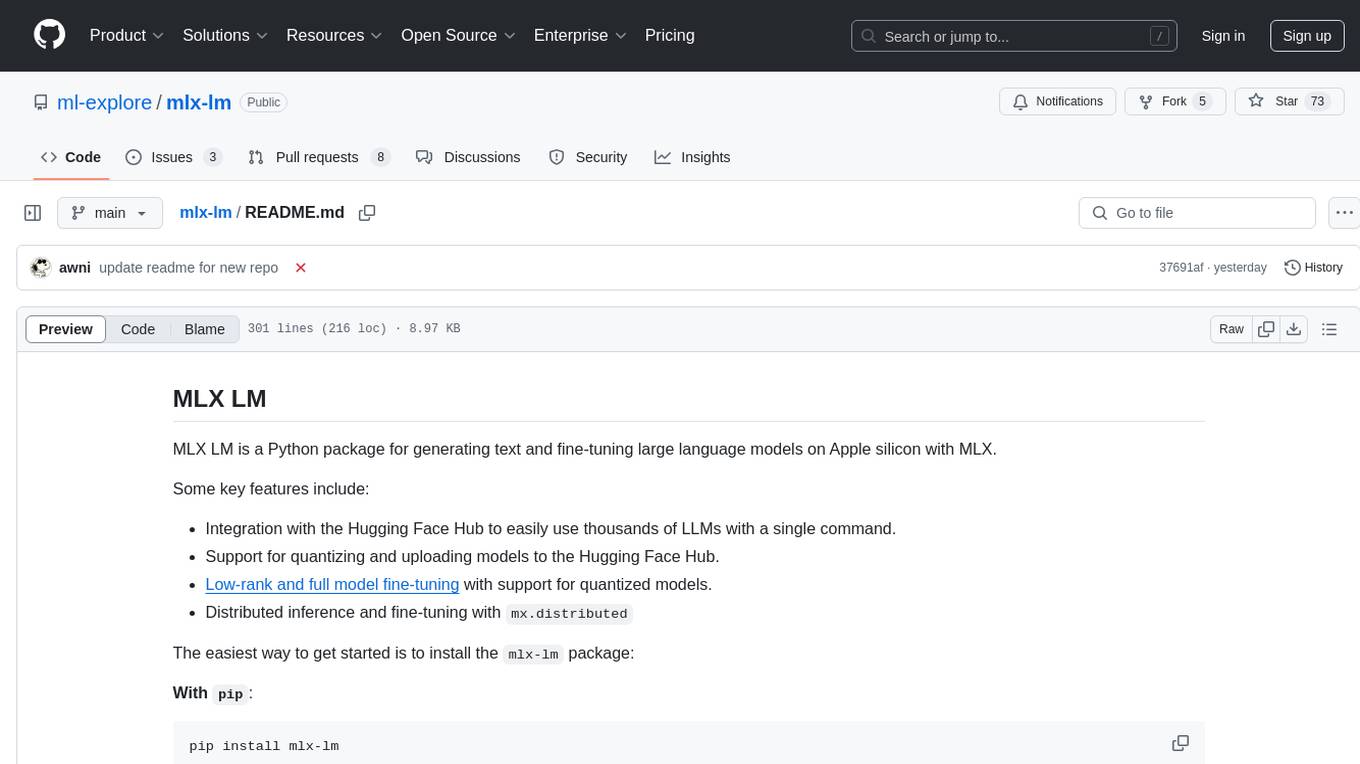
mlx-lm
MLX LM is a Python package designed for generating text and fine-tuning large language models on Apple silicon using MLX. It offers integration with the Hugging Face Hub for easy access to thousands of LLMs, support for quantizing and uploading models to the Hub, low-rank and full model fine-tuning capabilities, and distributed inference and fine-tuning with `mx.distributed`. Users can interact with the package through command line options or the Python API, enabling tasks such as text generation, chatting with language models, model conversion, streaming generation, and sampling. MLX LM supports various Hugging Face models and provides tools for efficient scaling to long prompts and generations, including a rotating key-value cache and prompt caching. It requires macOS 15.0 or higher for optimal performance.
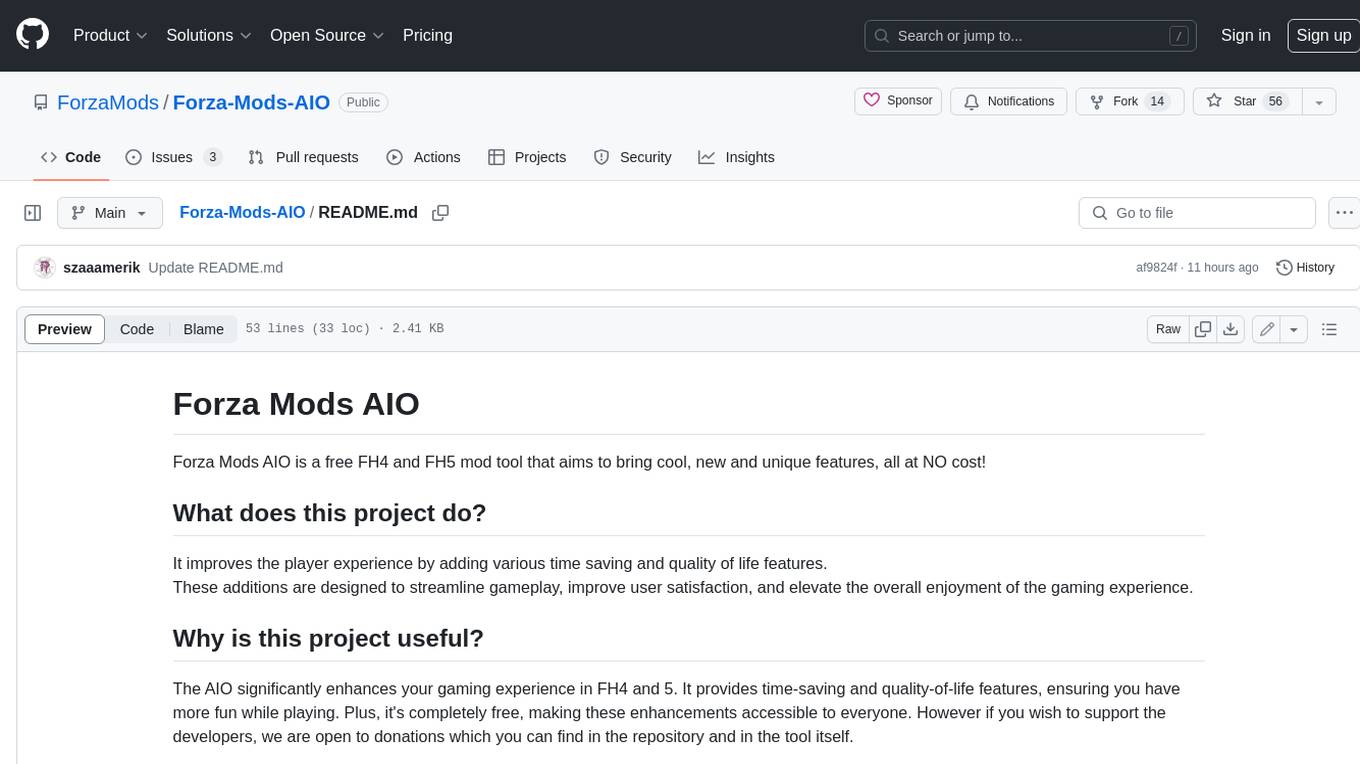
Forza-Mods-AIO
Forza Mods AIO is a free and open-source tool that enhances the gaming experience in Forza Horizon 4 and 5. It offers a range of time-saving and quality-of-life features, making gameplay more enjoyable and efficient. The tool is designed to streamline various aspects of the game, improving user satisfaction and overall enjoyment.
For similar jobs
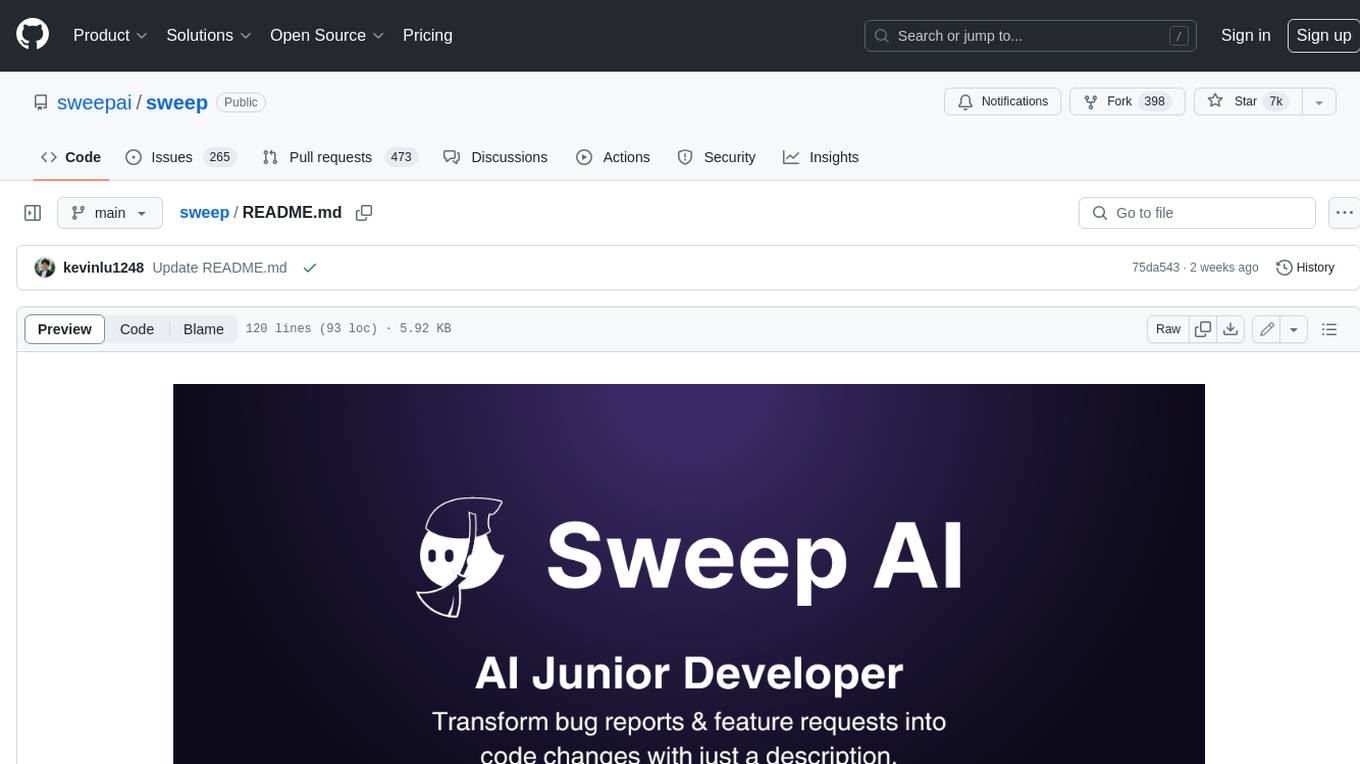
sweep
Sweep is an AI junior developer that turns bugs and feature requests into code changes. It automatically handles developer experience improvements like adding type hints and improving test coverage.
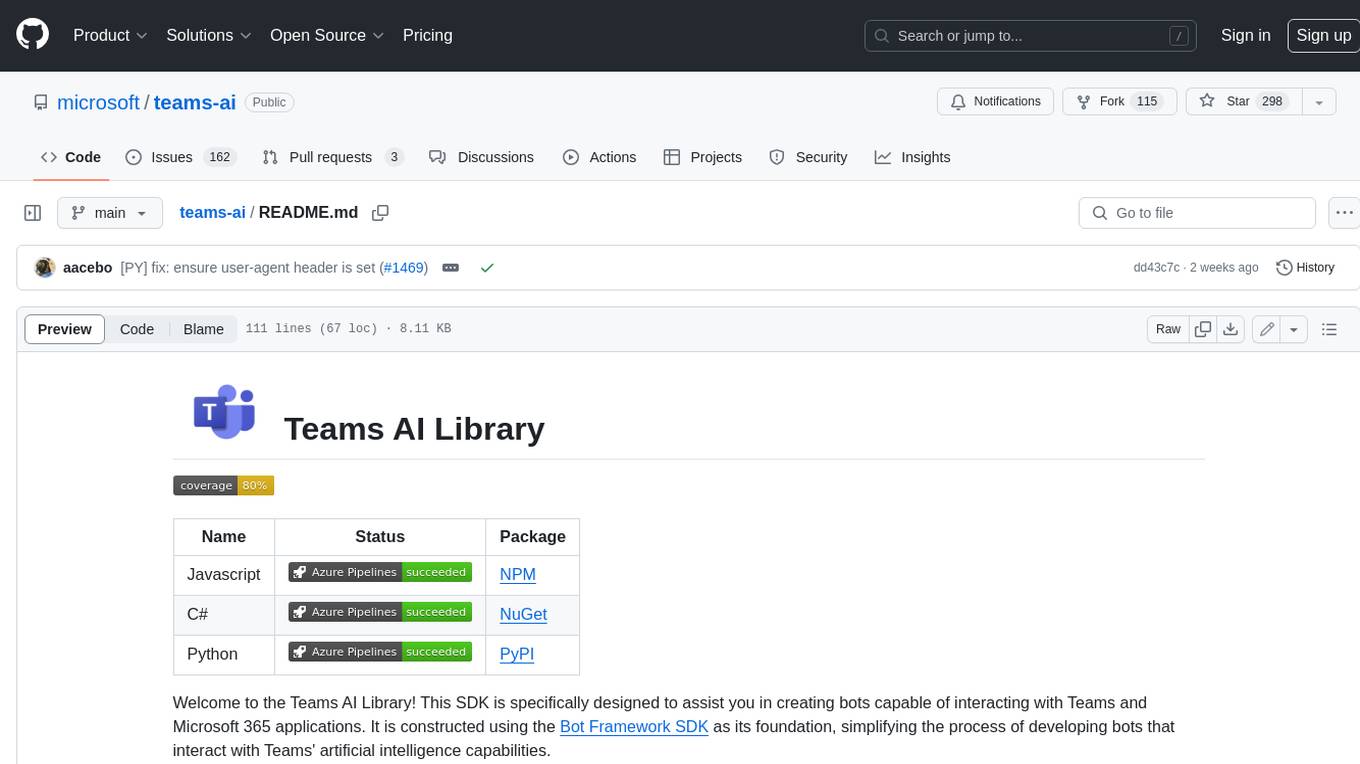
teams-ai
The Teams AI Library is a software development kit (SDK) that helps developers create bots that can interact with Teams and Microsoft 365 applications. It is built on top of the Bot Framework SDK and simplifies the process of developing bots that interact with Teams' artificial intelligence capabilities. The SDK is available for JavaScript/TypeScript, .NET, and Python.
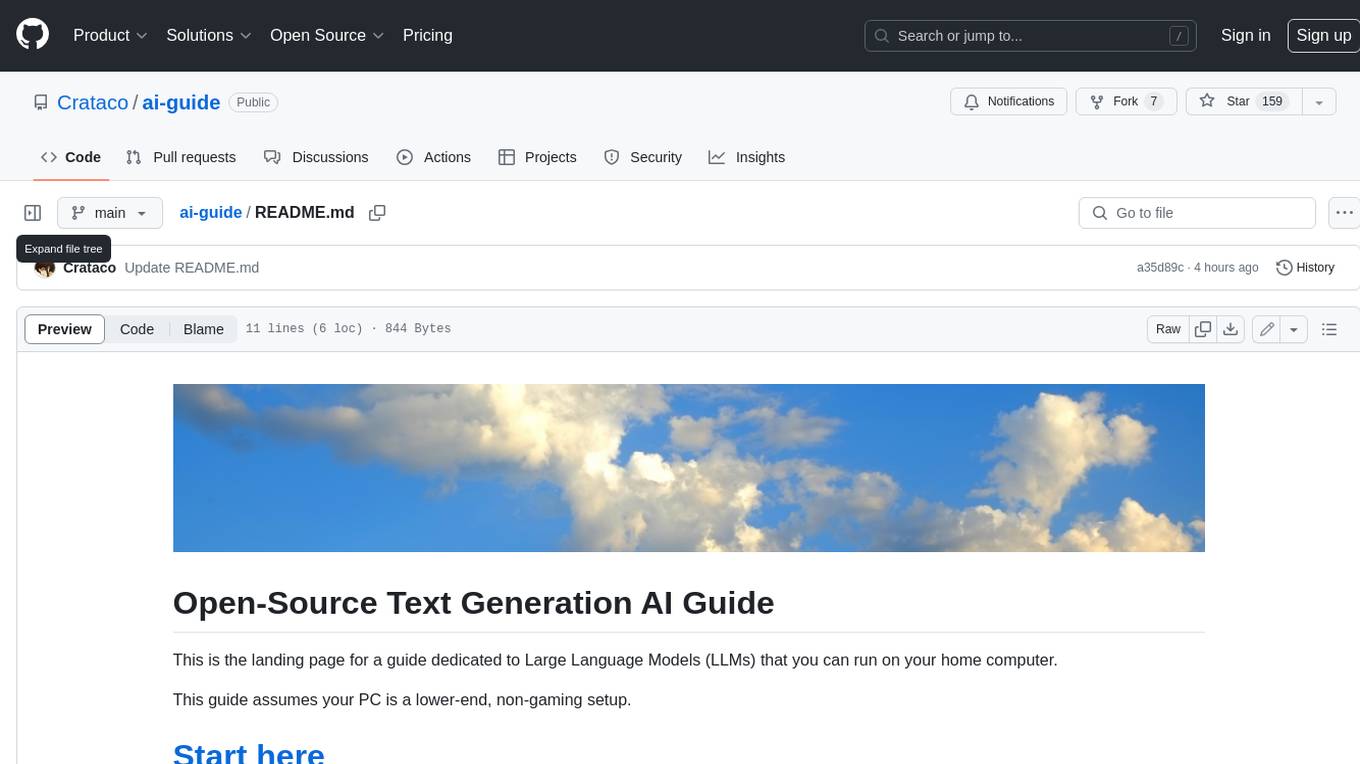
ai-guide
This guide is dedicated to Large Language Models (LLMs) that you can run on your home computer. It assumes your PC is a lower-end, non-gaming setup.
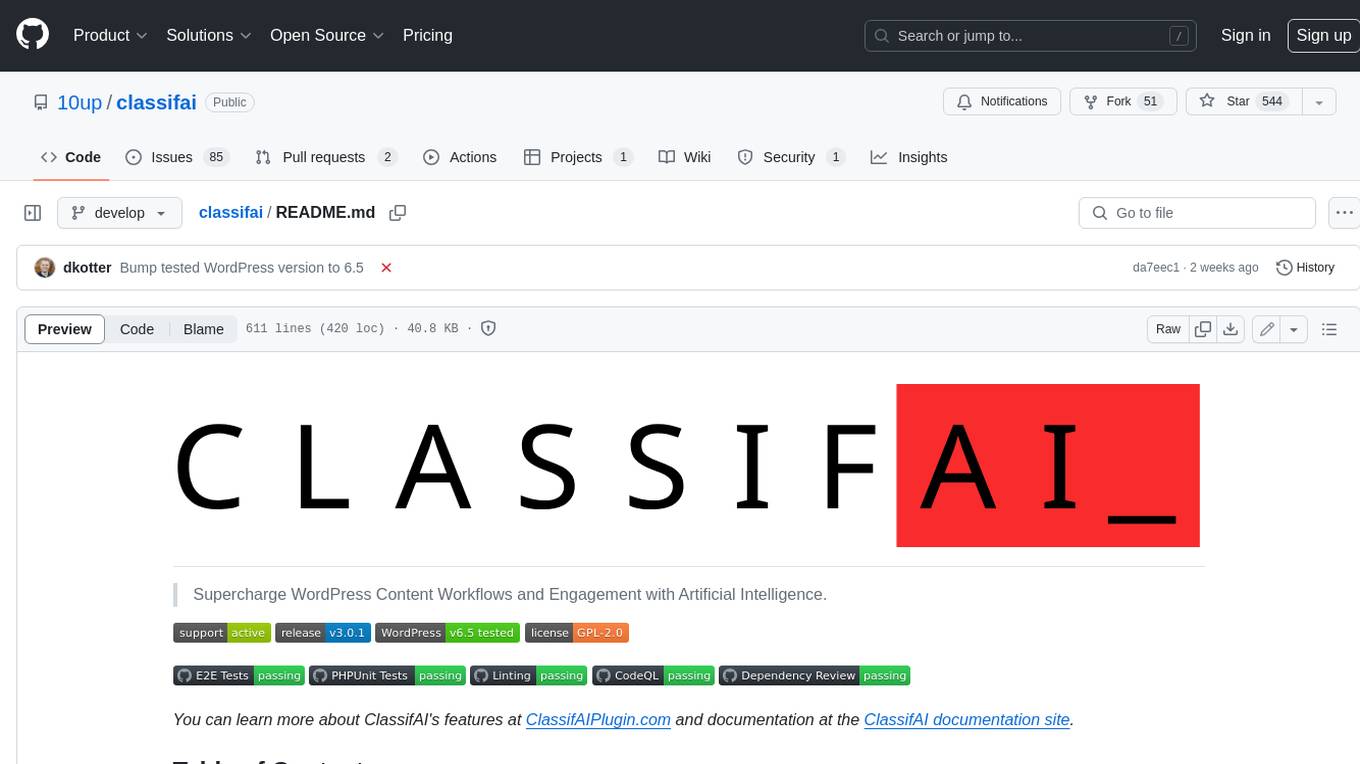
classifai
Supercharge WordPress Content Workflows and Engagement with Artificial Intelligence. Tap into leading cloud-based services like OpenAI, Microsoft Azure AI, Google Gemini and IBM Watson to augment your WordPress-powered websites. Publish content faster while improving SEO performance and increasing audience engagement. ClassifAI integrates Artificial Intelligence and Machine Learning technologies to lighten your workload and eliminate tedious tasks, giving you more time to create original content that matters.
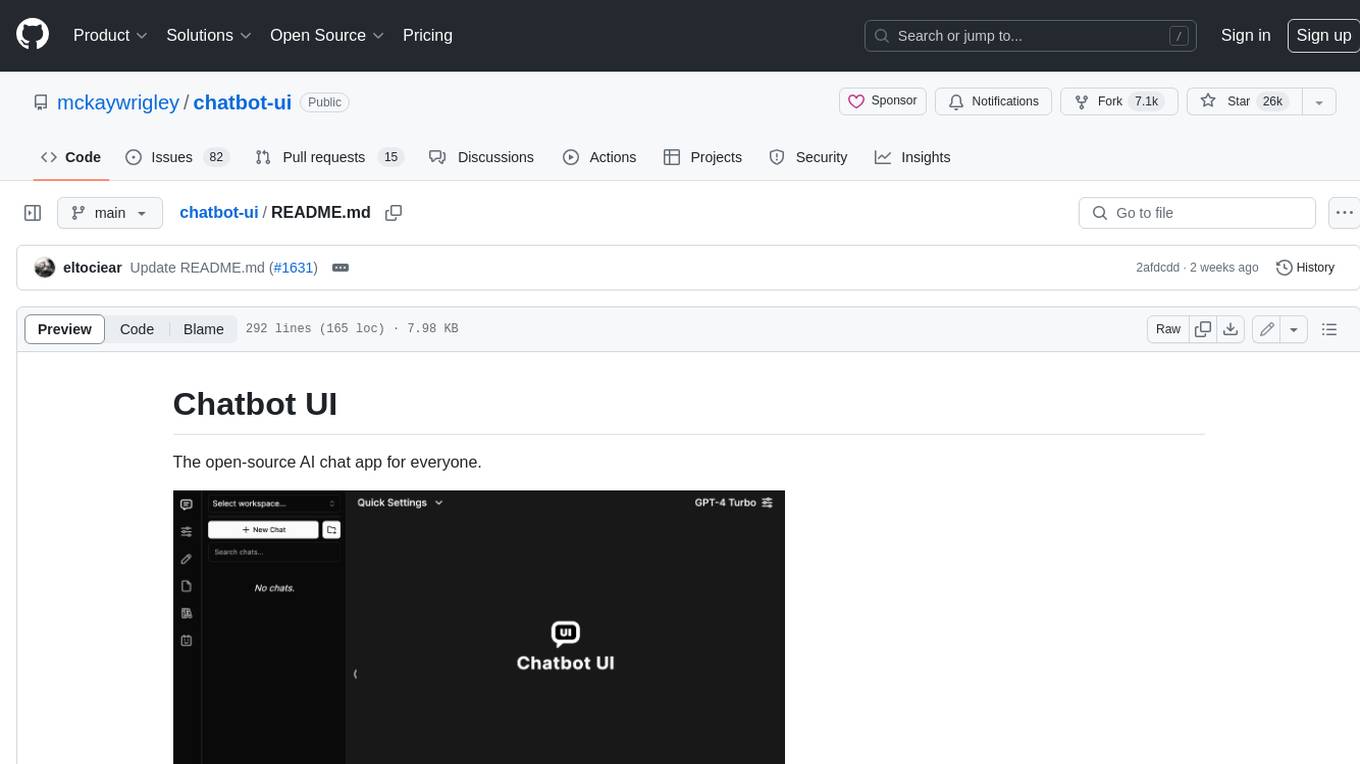
chatbot-ui
Chatbot UI is an open-source AI chat app that allows users to create and deploy their own AI chatbots. It is easy to use and can be customized to fit any need. Chatbot UI is perfect for businesses, developers, and anyone who wants to create a chatbot.
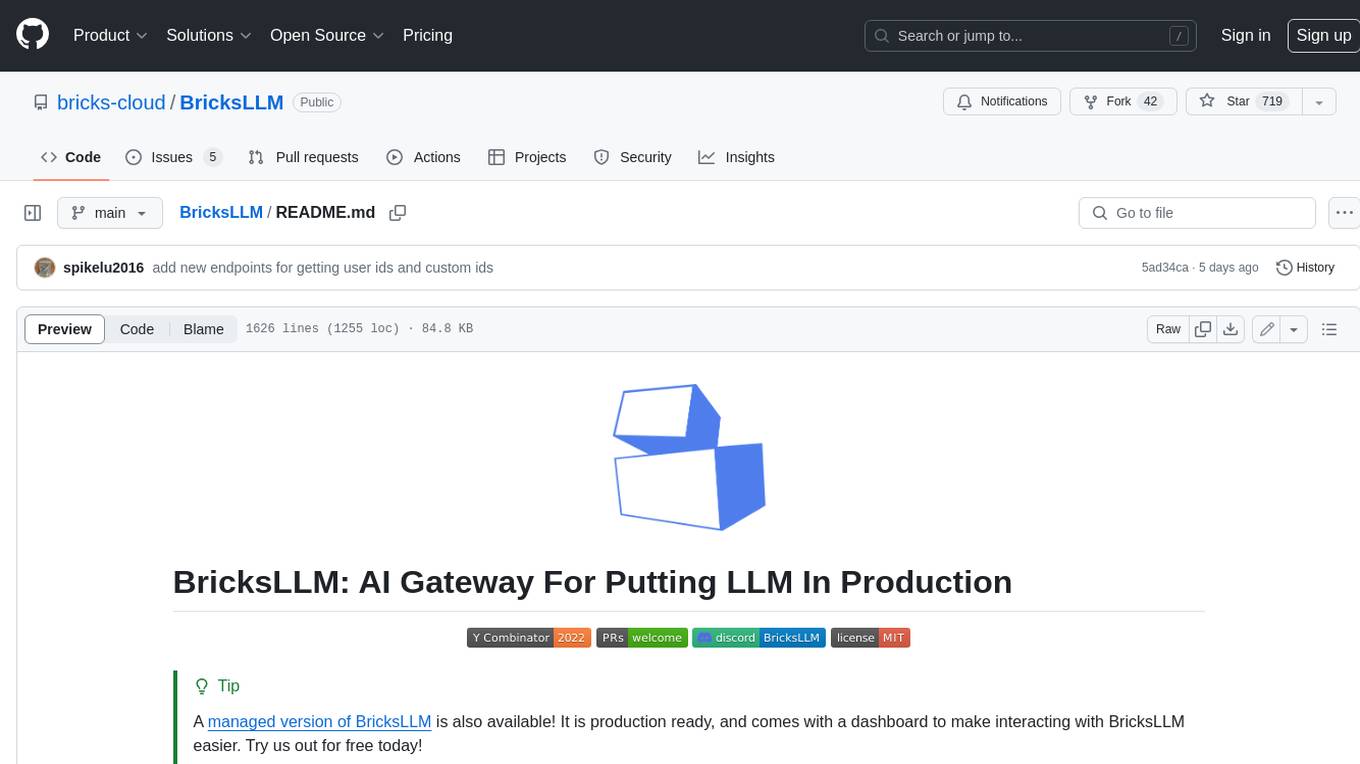
BricksLLM
BricksLLM is a cloud native AI gateway written in Go. Currently, it provides native support for OpenAI, Anthropic, Azure OpenAI and vLLM. BricksLLM aims to provide enterprise level infrastructure that can power any LLM production use cases. Here are some use cases for BricksLLM: * Set LLM usage limits for users on different pricing tiers * Track LLM usage on a per user and per organization basis * Block or redact requests containing PIIs * Improve LLM reliability with failovers, retries and caching * Distribute API keys with rate limits and cost limits for internal development/production use cases * Distribute API keys with rate limits and cost limits for students
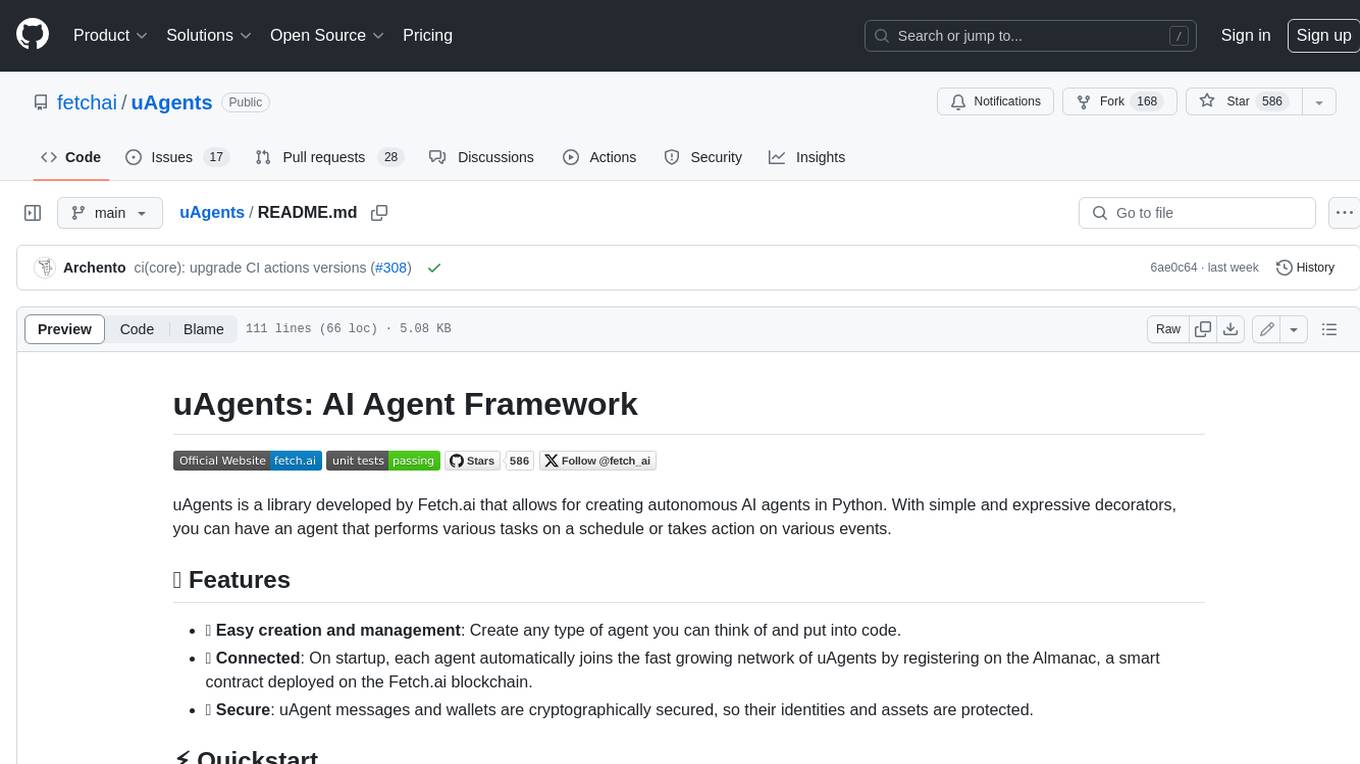
uAgents
uAgents is a Python library developed by Fetch.ai that allows for the creation of autonomous AI agents. These agents can perform various tasks on a schedule or take action on various events. uAgents are easy to create and manage, and they are connected to a fast-growing network of other uAgents. They are also secure, with cryptographically secured messages and wallets.
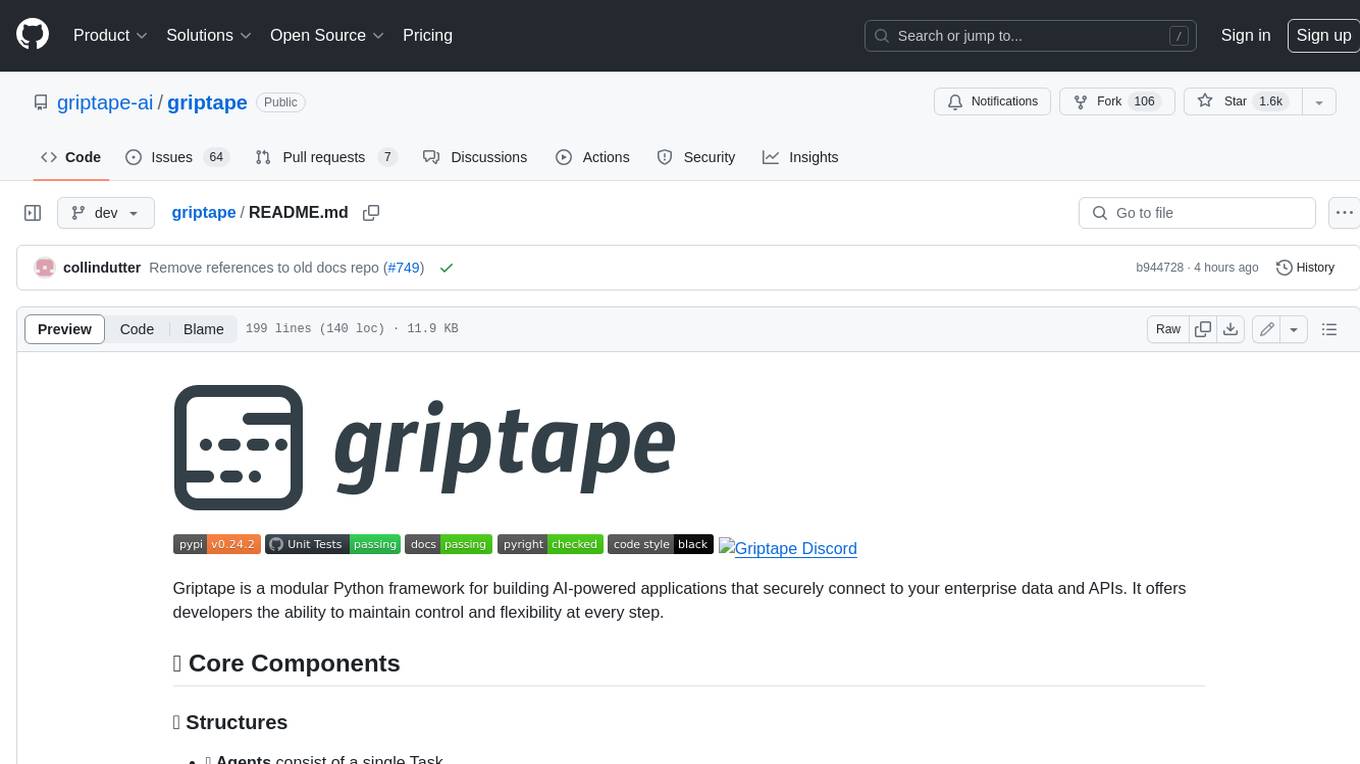
griptape
Griptape is a modular Python framework for building AI-powered applications that securely connect to your enterprise data and APIs. It offers developers the ability to maintain control and flexibility at every step. Griptape's core components include Structures (Agents, Pipelines, and Workflows), Tasks, Tools, Memory (Conversation Memory, Task Memory, and Meta Memory), Drivers (Prompt and Embedding Drivers, Vector Store Drivers, Image Generation Drivers, Image Query Drivers, SQL Drivers, Web Scraper Drivers, and Conversation Memory Drivers), Engines (Query Engines, Extraction Engines, Summary Engines, Image Generation Engines, and Image Query Engines), and additional components (Rulesets, Loaders, Artifacts, Chunkers, and Tokenizers). Griptape enables developers to create AI-powered applications with ease and efficiency.