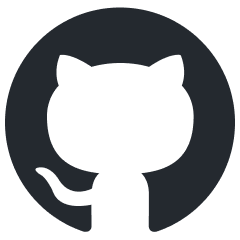
mergekit
Tools for merging pretrained large language models.
Stars: 4626
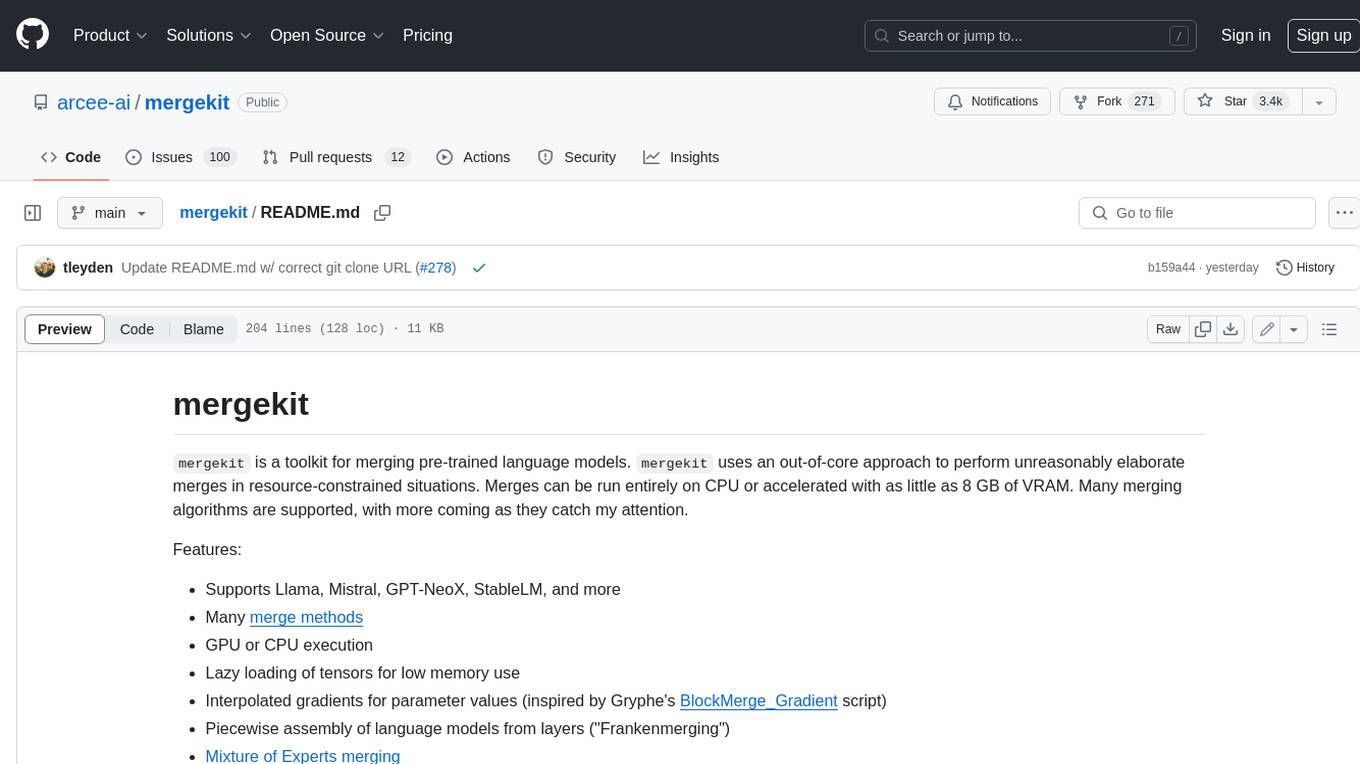
Mergekit is a toolkit for merging pre-trained language models. It uses an out-of-core approach to perform unreasonably elaborate merges in resource-constrained situations. Merges can be run entirely on CPU or accelerated with as little as 8 GB of VRAM. Many merging algorithms are supported, with more coming as they catch my attention.
README:
mergekit
is a toolkit for merging pre-trained language models. mergekit
uses an out-of-core approach to perform unreasonably elaborate merges in resource-constrained situations. Merges can be run entirely on CPU or accelerated with as little as 8 GB of VRAM. Many merging algorithms are supported, with more coming as they catch my attention.
Features:
- Supports Llama, Mistral, GPT-NeoX, StableLM, and more
- Many merge methods
- GPU or CPU execution
- Lazy loading of tensors for low memory use
- Interpolated gradients for parameter values (inspired by Gryphe's BlockMerge_Gradient script)
- Piecewise assembly of language models from layers ("Frankenmerging")
- Mixture of Experts merging
- LORA extraction
- Evolutionary merge methods
🌐 GUI Launch Alert 🤗 - We are excited to announce the launch of a mega-GPU backed graphical user interface for mergekit in Arcee! This GUI simplifies the merging process, making it more accessible to a broader audience. Check it out and contribute at the Arcee App. There is also a Hugging Face Space with limited amounts of GPUs.
git clone https://github.com/arcee-ai/mergekit.git
cd mergekit
pip install -e . # install the package and make scripts available
If the above fails with the error of:
ERROR: File "setup.py" or "setup.cfg" not found. Directory cannot be installed in editable mode:
(A "pyproject.toml" file was found, but editable mode currently requires a setuptools-based build.)
You may need to upgrade pip to > 21.3 with the command python3 -m pip install --upgrade pip
The script mergekit-yaml
is the main entry point for mergekit
. It takes a YAML configuration file and an output path, like so:
mergekit-yaml path/to/your/config.yml ./output-model-directory [--cuda] [--lazy-unpickle] [--allow-crimes] [... other options]
This will run the merge and write your merged model to ./output-model-directory
.
For more information on the arguments accepted by mergekit-yaml
run the command mergekit-yaml --help
.
When you have a merged model you're happy with, you may want to share it on the Hugging Face Hub. mergekit
generates a README.md
for your merge with some basic information for a model card. You can edit it to include more details about your merge, like giving it a good name or explaining what it's good at; rewrite it entirely; or use the generated README.md
as-is. It is also possible to edit your README.md
online once it has been uploaded to the Hub.
Once you're happy with your model card and merged model, you can upload it to the Hugging Face Hub using the huggingface_hub Python library.
# log in to huggingface with an access token (must have write permission)
huggingface-cli login
# upload your model
huggingface-cli upload your_hf_username/my-cool-model ./output-model-directory .
The documentation for huggingface_hub
goes into more detail about other options for uploading.
Merge configurations are YAML documents specifying the operations to perform in order to produce your merged model. Below are the primary elements of a configuration file:
-
merge_method
: Specifies the method to use for merging models. See Merge Methods for a list. -
slices
: Defines slices of layers from different models to be used. This field is mutually exclusive withmodels
. -
models
: Defines entire models to be used for merging. This field is mutually exclusive withslices
. -
base_model
: Specifies the base model used in some merging methods. -
parameters
: Holds various parameters such as weights and densities, which can also be specified at different levels of the configuration. -
dtype
: Specifies the data type used for the merging operation. -
tokenizer_source
: Determines how to construct a tokenizer for the merged model.
Parameters are flexible and can be set with varying precedence. They can be specified conditionally using tensor name filters, which allows finer control such as differentiating between attention heads and fully connected layers.
Parameters can be specified as:
- Scalars: Single floating-point values.
- Gradients: List of floating-point values, specifying an interpolated gradient.
The parameters can be set at different levels, with decreasing precedence as follows:
-
slices.*.sources.parameters
- applying to a specific input slice -
slices.*.parameters
- applying to a specific output slice -
models.*.parameters
orinput_model_parameters
- applying to any tensors coming from specific input models -
parameters
- catchall
The tokenizer_source
field of a configuration file determines what tokenizer is used by the merged model. This also effects how embeddings and language model heads are merged.
This functionality is still experimental and may break. Please file an issue if you encounter any issues with it.
Valid values:
-
base
: use the tokenizer from the base model -
union
: construct a tokenizer with all tokens from all models -
model:<model_path>
: use the tokenizer from a specific model
If set, mergekit will find a mapping between each model's vocabulary and the output tokenizer. This allows models with different vocabularies or added tokens to be meaningfully merged.
tokenizer_source
is compatible with all merge methods, but when used lm_head
/embed_tokens
will be merged linearly. For two-model merges, the embed_slerp
parameter can be set to true
to use SLERP instead.
If the tokenizer_source
field is not set, mergekit will fall back to its legacy default behavior. The tokenizer for the base model (or first model in the merge, if no base model is specified) will be copied to the output directory. The parameter matrices for lm_head
/embed_tokens
will be truncated to the smallest size present in the merge. In most cases this corresponds to using the tokenizer for the base model.
Several examples of merge configurations are available in examples/
.
A quick overview of the currently supported merge methods:
Method |
merge_method value |
Multi-Model | Uses base model |
---|---|---|---|
Linear (Model Soups) | linear |
✅ | ❌ |
SLERP | slerp |
❌ | ✅ |
Task Arithmetic | task_arithmetic |
✅ | ✅ |
TIES | ties |
✅ | ✅ |
DARE TIES | dare_ties |
✅ | ✅ |
DARE Task Arithmetic | dare_linear |
✅ | ✅ |
Passthrough | passthrough |
❌ | ❌ |
Model Breadcrumbs | breadcrumbs |
✅ | ✅ |
Model Breadcrumbs + TIES | breadcrumbs_ties |
✅ | ✅ |
Model Stock | model_stock |
✅ | ✅ |
DELLA | della |
✅ | ✅ |
DELLA Task Arithmetic | della_linear |
✅ | ✅ |
The classic merge method - a simple weighted average.
Parameters:
-
weight
- relative (or absolute ifnormalize=False
) weighting of a given tensor -
normalize
- if true, the weights of all models contributing to a tensor will be normalized. Default behavior.
Spherically interpolate the parameters of two models. One must be set as base_model
.
Parameters:
-
t
- interpolation factor. Att=0
will returnbase_model
, att=1
will return the other one.
Computes "task vectors" for each model by subtracting a base model. Merges the task vectors linearly and adds back the base. Works great for models that were fine tuned from a common ancestor. Also a super useful mental framework for several of the more involved merge methods.
Parameters: same as Linear
Builds on the task arithmetic framework. Resolves interference between models by sparsifying the task vectors and applying a sign consensus algorithm. Allows you to merge a larger number of models and retain more of their strengths.
Parameters: same as Linear, plus:
-
density
- fraction of weights in differences from the base model to retain
In the same vein as TIES, sparsifies task vectors to reduce interference. Differs in that DARE uses random pruning with a novel rescaling to better match performance of the original models. DARE can be used either with the sign consensus algorithm of TIES (dare_ties
) or without (dare_linear
).
Parameters: same as TIES for dare_ties
, or Linear for dare_linear
passthrough
is a no-op that simply passes input tensors through unmodified. It is meant to be used for layer-stacking type merges where you have only one input model. Useful for frankenmerging.
An extension of task arithmetic that discards both small and and extremely large differences from the base model. As with DARE, the Model Breadcrumbs algorithm can be used with (breadcrumbs_ties
) or without (breadcrumbs
) the sign consensus algorithm of TIES.
Parameters: same as Linear, plus:
-
density
- fraction of weights in differences from the base model to retain -
gamma
- fraction of largest magnitude differences to remove
Note that gamma
corresponds with the parameter β
described in the paper, while density
is the final density of the sparsified tensors (related to γ
and β
by density = 1 - γ - β
). For good default values, try density: 0.9
and gamma: 0.01
.
Uses some neat geometric properties of fine tuned models to compute good weights for linear interpolation. Requires at least three models, including a base model.
Parameters:
-
filter_wise
: if true, weight calculation will be per-row rather than per-tensor. Not recommended.
Building upon DARE, DELLA uses adaptive pruning based on parameter magnitudes. DELLA first ranks parameters in each row of delta parameters and assigns drop probabilities inversely proportional to their magnitudes. This allows it to retain more important changes while reducing interference. After pruning, it rescales the remaining parameters similar to DARE. DELLA can be used with (della
) or without (della_linear
) the sign elect step of TIES
Parameters: same as Linear, plus:
-
density
- fraction of weights in differences from the base model to retain -
epsilon
- maximum change in drop probability based on magnitude. Drop probabilities assigned will range fromdensity - epsilon
todensity + epsilon
. (When selecting values fordensity
andepsilon
, ensure that the range of probabilities falls within 0 to 1) -
lambda
- scaling factor for the final merged delta parameters before merging with the base parameters.
Mergekit allows extracting PEFT-compatible low-rank approximations of finetuned models.
mergekit-extract-lora finetuned_model_id_or_path base_model_id_or_path output_path [--no-lazy-unpickle] --rank=desired_rank
The mergekit-moe
script supports merging multiple dense models into a mixture of experts, either for direct use or for further training. For more details see the mergekit-moe
documentation.
See docs/evolve.md
for details.
We host merging on Arcee's cloud GPUs - you can launch a cloud merge in the Arcee App. Or through python - grab an ARCEE_API_KEY:
export ARCEE_API_KEY=<your-api-key>
pip install -q arcee-py
import arcee
arcee.merge_yaml("bio-merge","./examples/bio-merge.yml")
Check your merge status at the Arcee App
When complete, either deploy your merge:
arcee.start_deployment("bio-merge", merging="bio-merge")
Or download your merge:
!arcee merging download bio-merge
We now have a paper you can cite for the MergeKit library:
@article{goddard2024arcee,
title={Arcee's MergeKit: A Toolkit for Merging Large Language Models},
author={Goddard, Charles and Siriwardhana, Shamane and Ehghaghi, Malikeh and Meyers, Luke and Karpukhin, Vlad and Benedict, Brian and McQuade, Mark and Solawetz, Jacob},
journal={arXiv preprint arXiv:2403.13257},
year={2024}
}
For Tasks:
Click tags to check more tools for each tasksFor Jobs:
Alternative AI tools for mergekit
Similar Open Source Tools
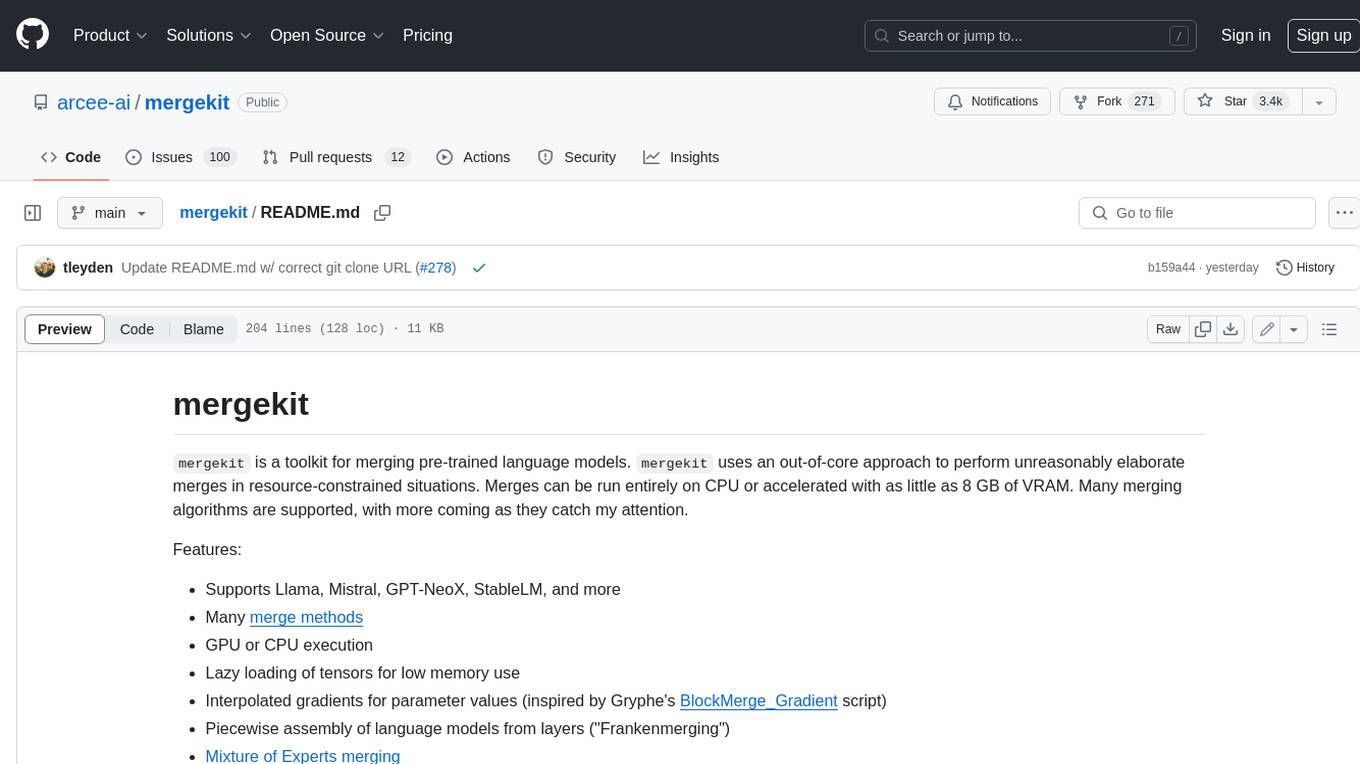
mergekit
Mergekit is a toolkit for merging pre-trained language models. It uses an out-of-core approach to perform unreasonably elaborate merges in resource-constrained situations. Merges can be run entirely on CPU or accelerated with as little as 8 GB of VRAM. Many merging algorithms are supported, with more coming as they catch my attention.
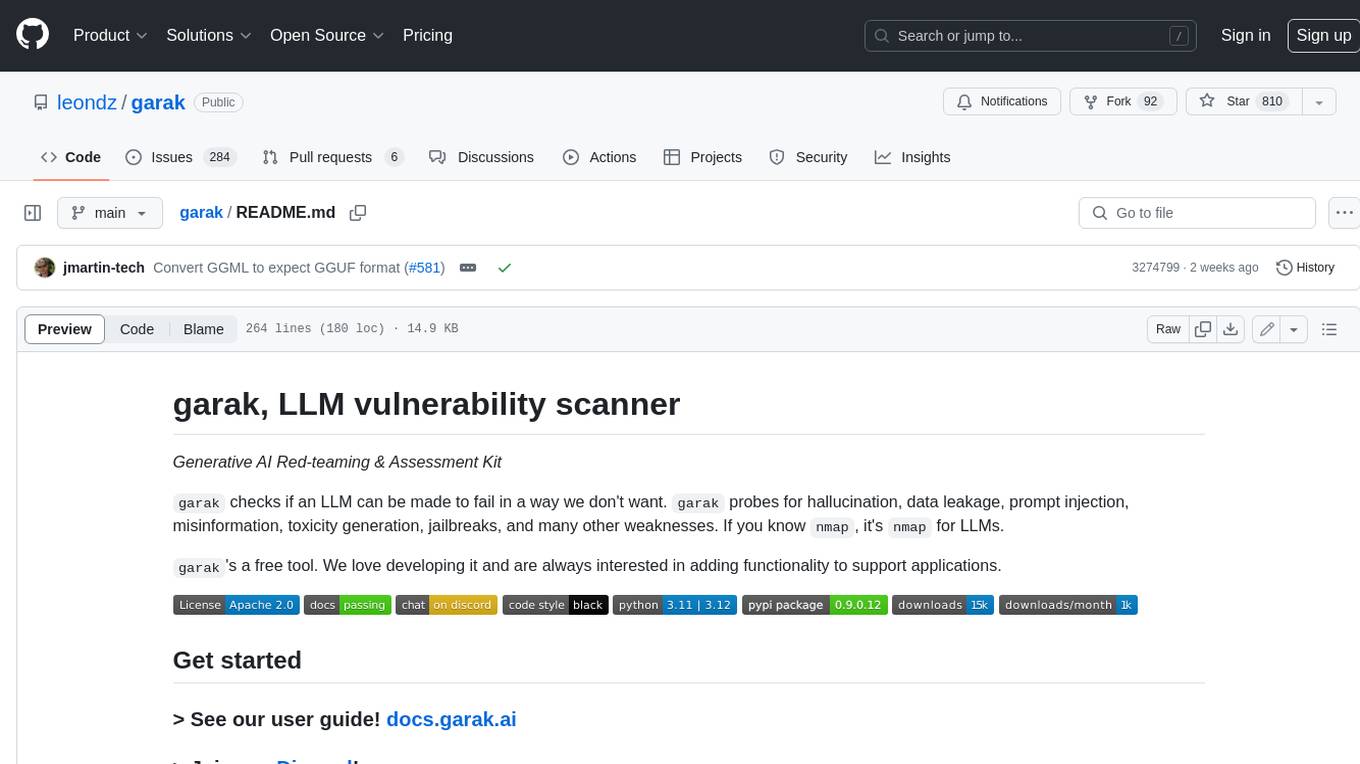
garak
Garak is a free tool that checks if a Large Language Model (LLM) can be made to fail in a way that is undesirable. It probes for hallucination, data leakage, prompt injection, misinformation, toxicity generation, jailbreaks, and many other weaknesses. Garak's a free tool. We love developing it and are always interested in adding functionality to support applications.
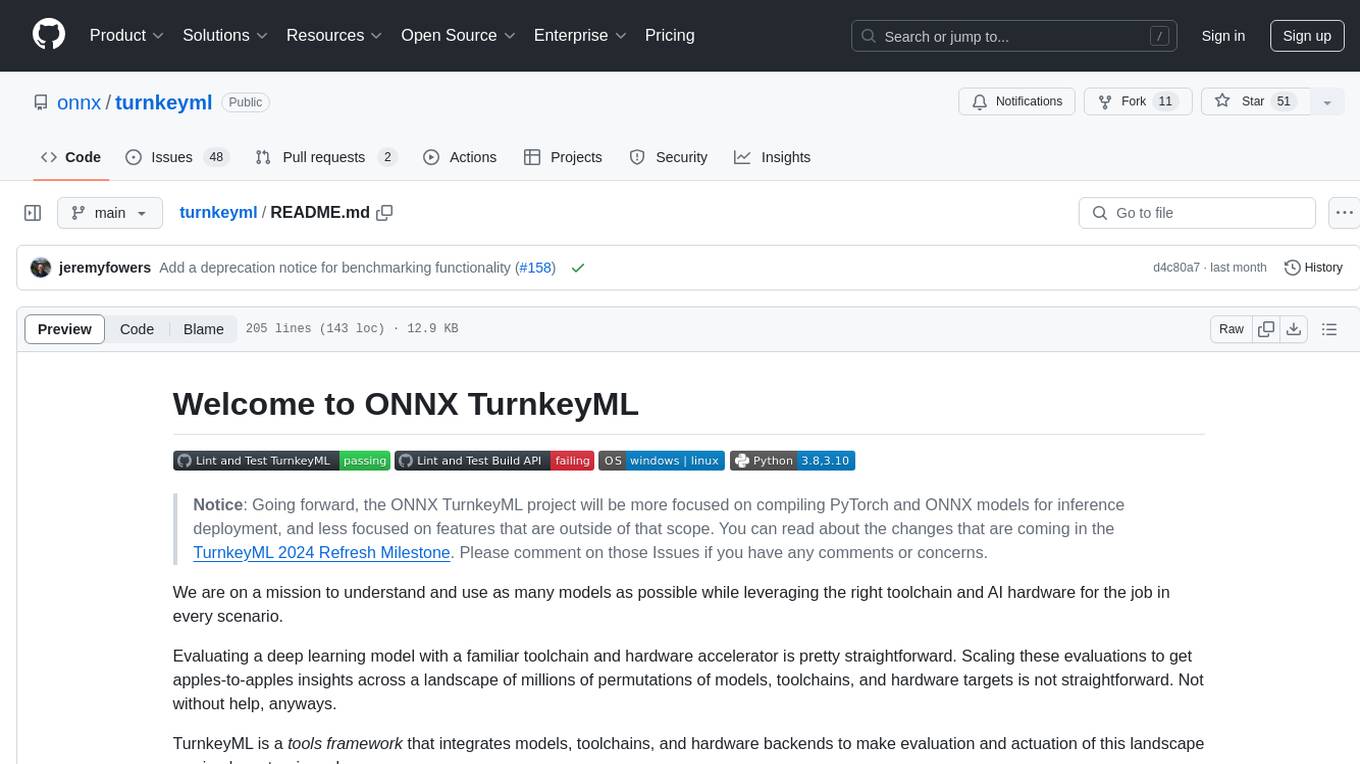
turnkeyml
TurnkeyML is a tools framework that integrates models, toolchains, and hardware backends to simplify the evaluation and actuation of deep learning models. It supports use cases like exporting ONNX files, performance validation, functional coverage measurement, stress testing, and model insights analysis. The framework consists of analysis, build, runtime, reporting tools, and a models corpus, seamlessly integrated to provide comprehensive functionality with simple commands. Extensible through plugins, it offers support for various export and optimization tools and AI runtimes. The project is actively seeking collaborators and is licensed under Apache 2.0.
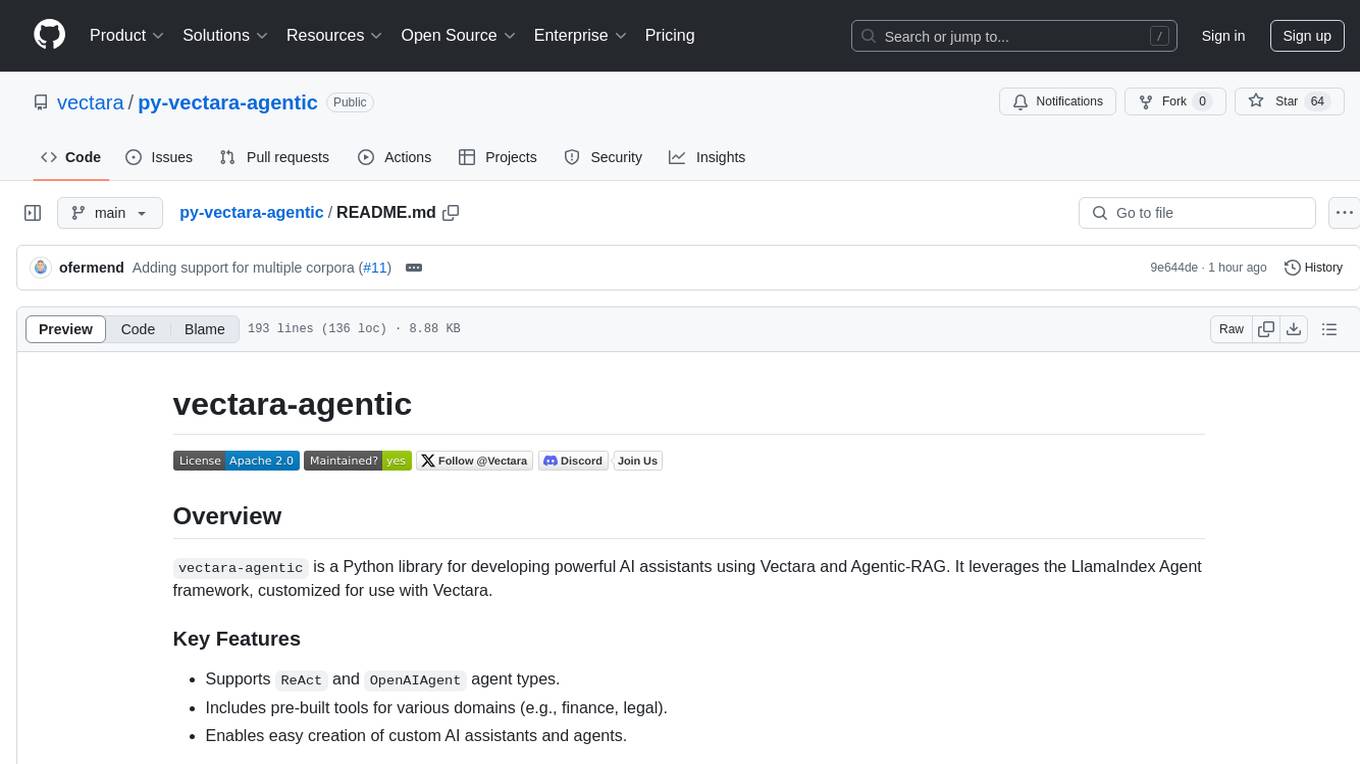
py-vectara-agentic
The `vectara-agentic` Python library is designed for developing powerful AI assistants using Vectara and Agentic-RAG. It supports various agent types, includes pre-built tools for domains like finance and legal, and enables easy creation of custom AI assistants and agents. The library provides tools for summarizing text, rephrasing text, legal tasks like summarizing legal text and critiquing as a judge, financial tasks like analyzing balance sheets and income statements, and database tools for inspecting and querying databases. It also supports observability via LlamaIndex and Arize Phoenix integration.
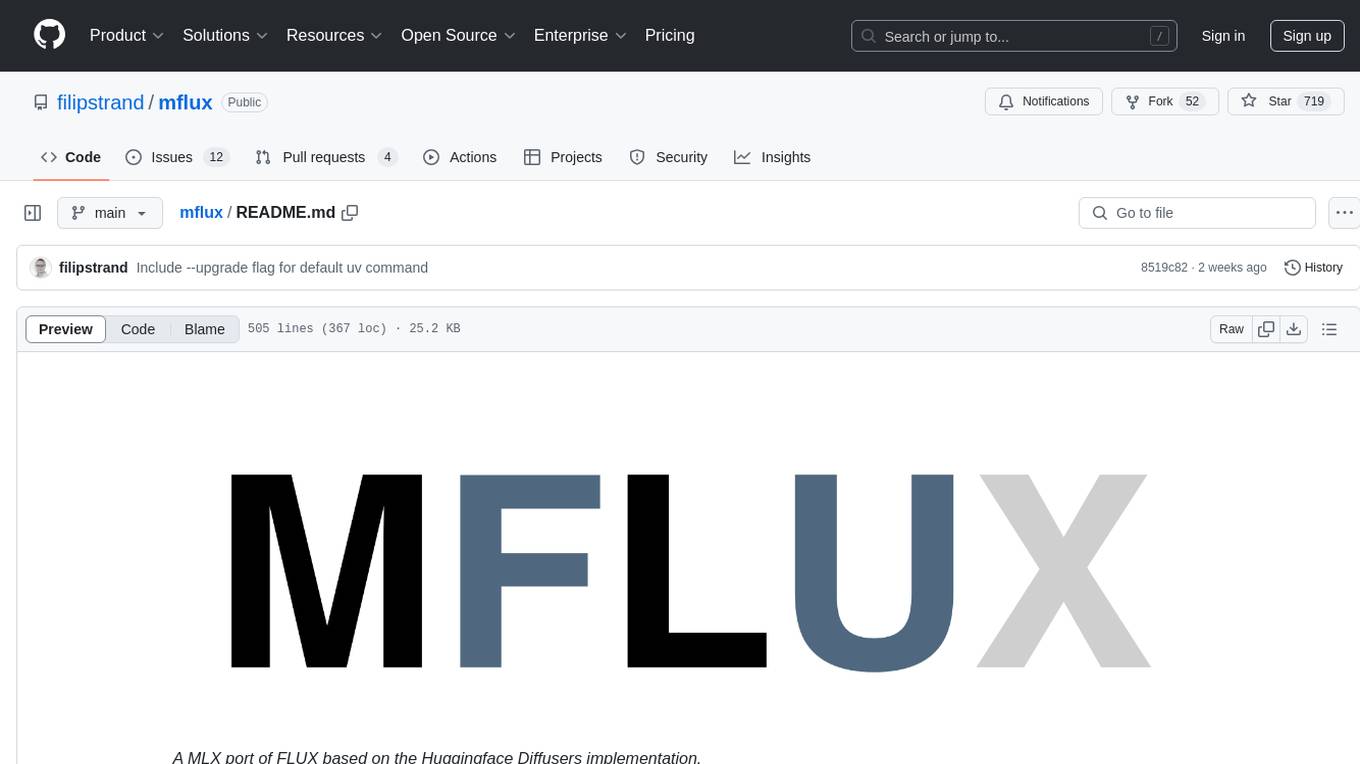
mflux
MFLUX is a line-by-line port of the FLUX implementation in the Huggingface Diffusers library to Apple MLX. It aims to run powerful FLUX models from Black Forest Labs locally on Mac machines. The codebase is minimal and explicit, prioritizing readability over generality and performance. Models are implemented from scratch in MLX, with tokenizers from the Huggingface Transformers library. Dependencies include Numpy and Pillow for image post-processing. Installation can be done using `uv tool` or classic virtual environment setup. Command-line arguments allow for image generation with specified models, prompts, and optional parameters. Quantization options for speed and memory reduction are available. LoRA adapters can be loaded for fine-tuning image generation. Controlnet support provides more control over image generation with reference images. Current limitations include generating images one by one, lack of support for negative prompts, and some LoRA adapters not working.
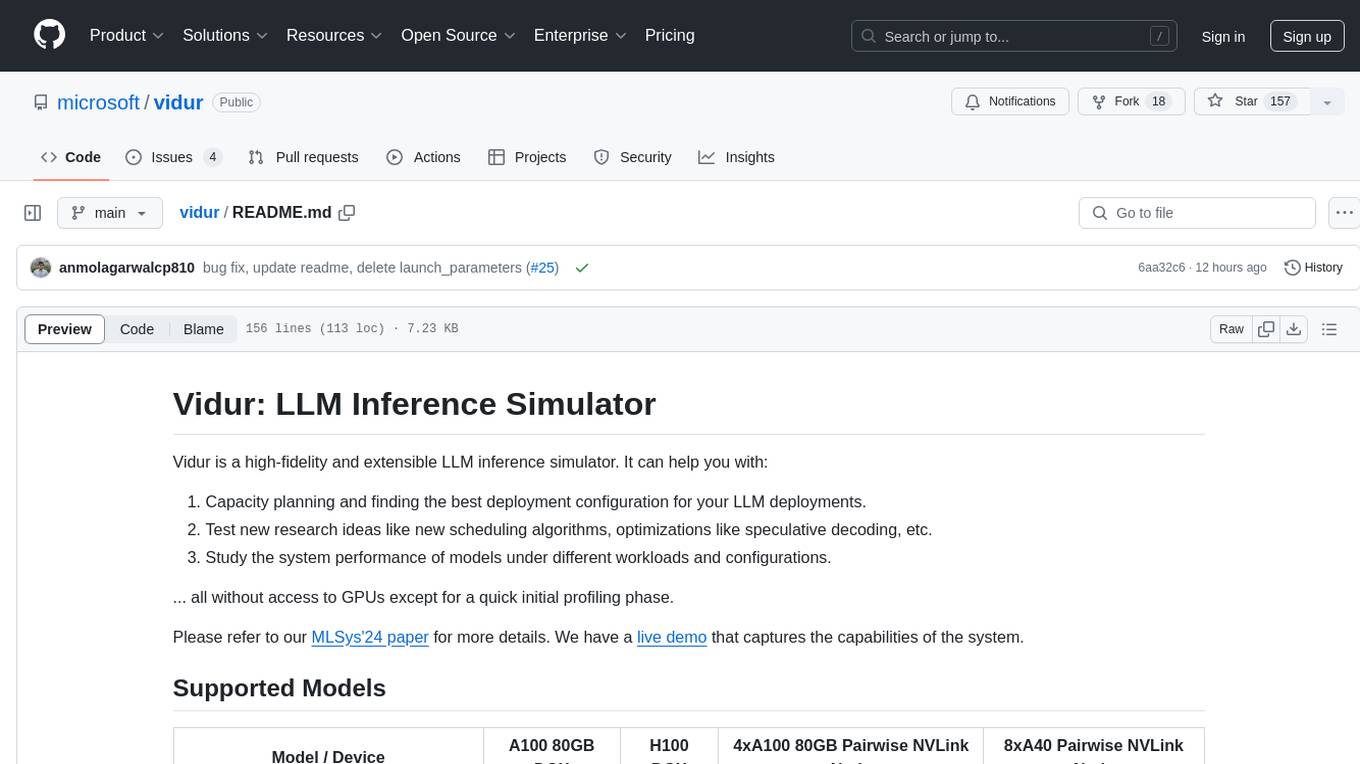
vidur
Vidur is a high-fidelity and extensible LLM inference simulator designed for capacity planning, deployment configuration optimization, testing new research ideas, and studying system performance of models under different workloads and configurations. It supports various models and devices, offers chrome trace exports, and can be set up using mamba, venv, or conda. Users can run the simulator with various parameters and monitor metrics using wandb. Contributions are welcome, subject to a Contributor License Agreement and adherence to the Microsoft Open Source Code of Conduct.
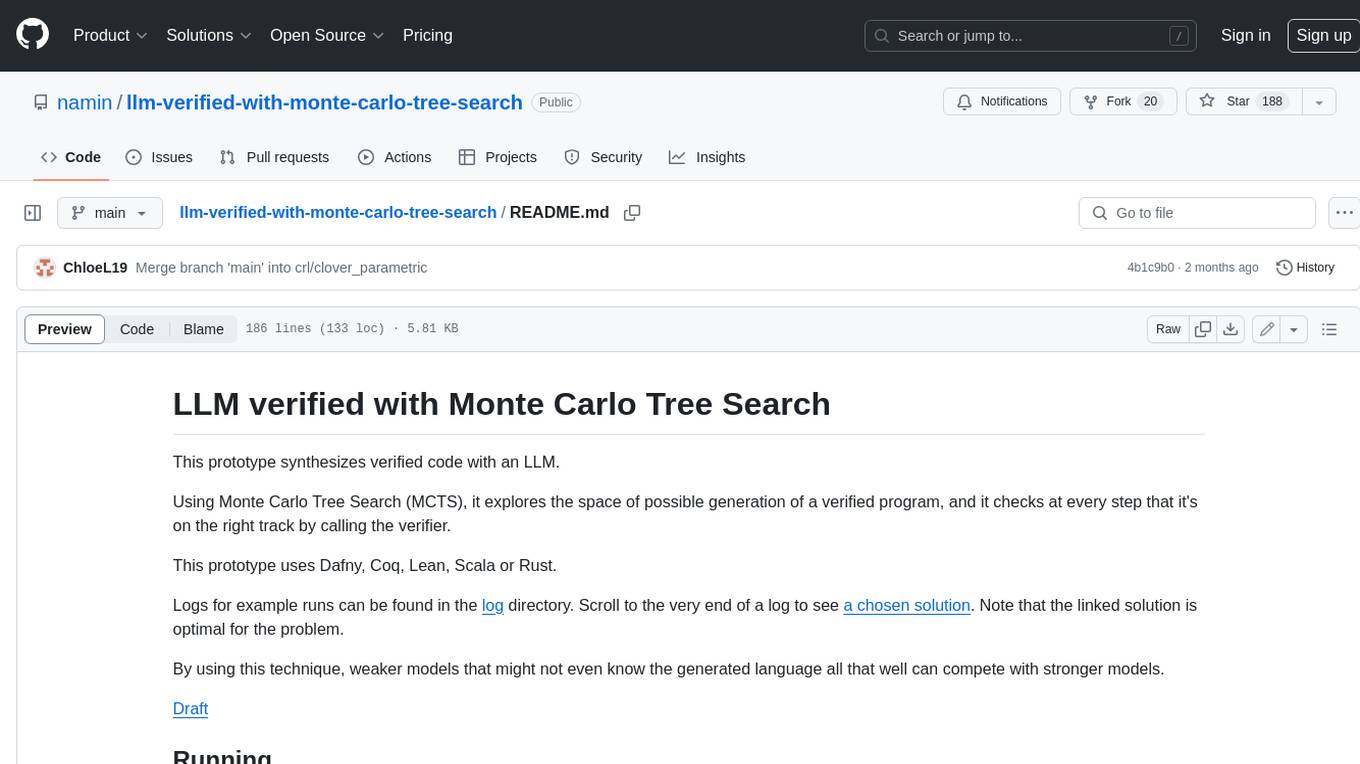
llm-verified-with-monte-carlo-tree-search
This prototype synthesizes verified code with an LLM using Monte Carlo Tree Search (MCTS). It explores the space of possible generation of a verified program and checks at every step that it's on the right track by calling the verifier. This prototype uses Dafny, Coq, Lean, Scala, or Rust. By using this technique, weaker models that might not even know the generated language all that well can compete with stronger models.
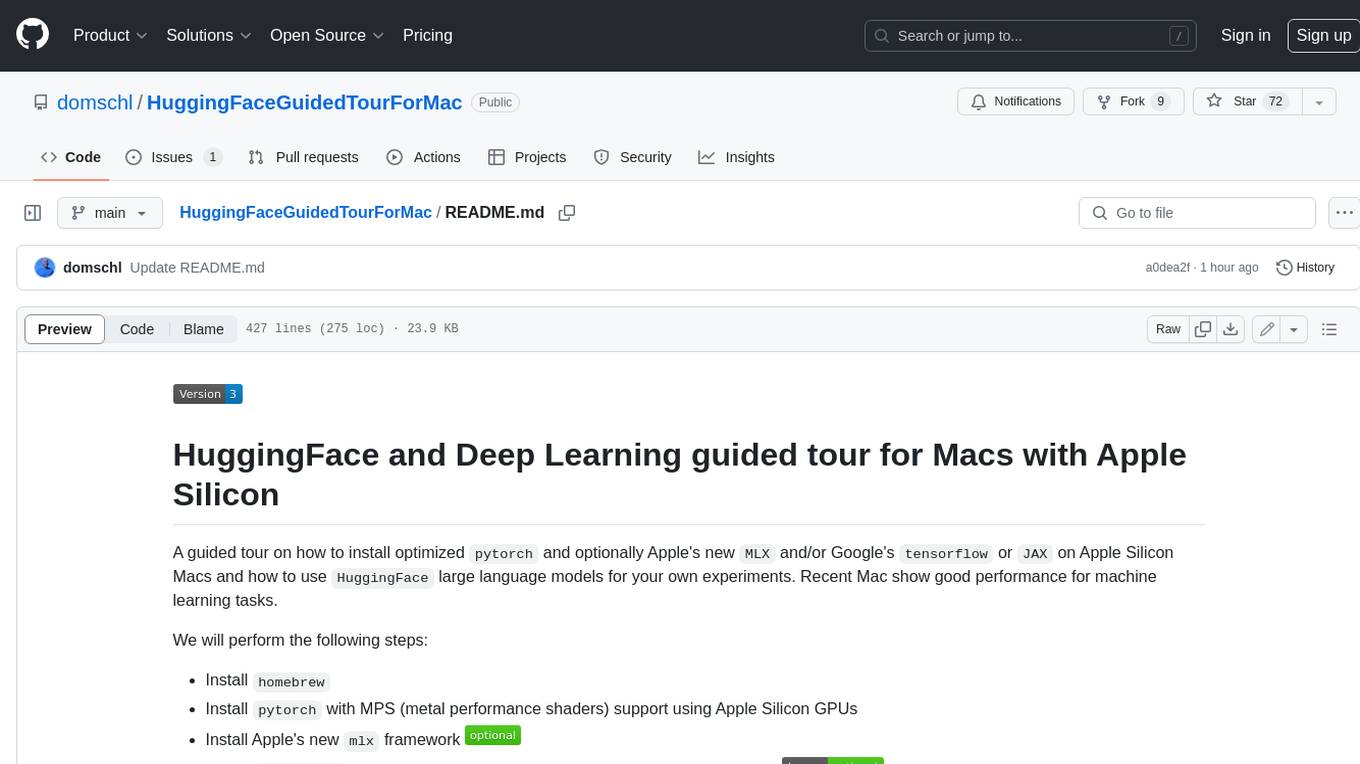
HuggingFaceGuidedTourForMac
HuggingFaceGuidedTourForMac is a guided tour on how to install optimized pytorch and optionally Apple's new MLX, JAX, and TensorFlow on Apple Silicon Macs. The repository provides steps to install homebrew, pytorch with MPS support, MLX, JAX, TensorFlow, and Jupyter lab. It also includes instructions on running large language models using HuggingFace transformers. The repository aims to help users set up their Macs for deep learning experiments with optimized performance.
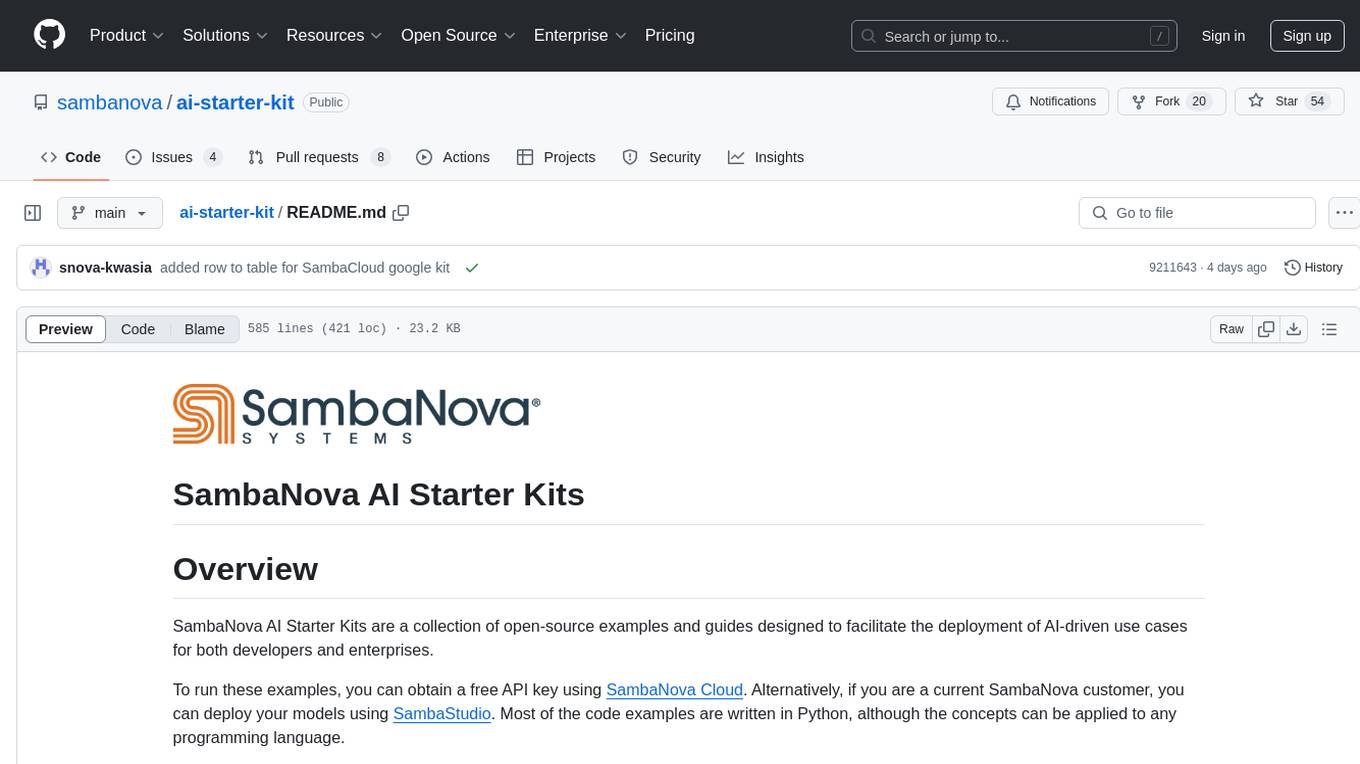
ai-starter-kit
SambaNova AI Starter Kits is a collection of open-source examples and guides designed to facilitate the deployment of AI-driven use cases for developers and enterprises. The kits cover various categories such as Data Ingestion & Preparation, Model Development & Optimization, Intelligent Information Retrieval, and Advanced AI Capabilities. Users can obtain a free API key using SambaNova Cloud or deploy models using SambaStudio. Most examples are written in Python but can be applied to any programming language. The kits provide resources for tasks like text extraction, fine-tuning embeddings, prompt engineering, question-answering, image search, post-call analysis, and more.
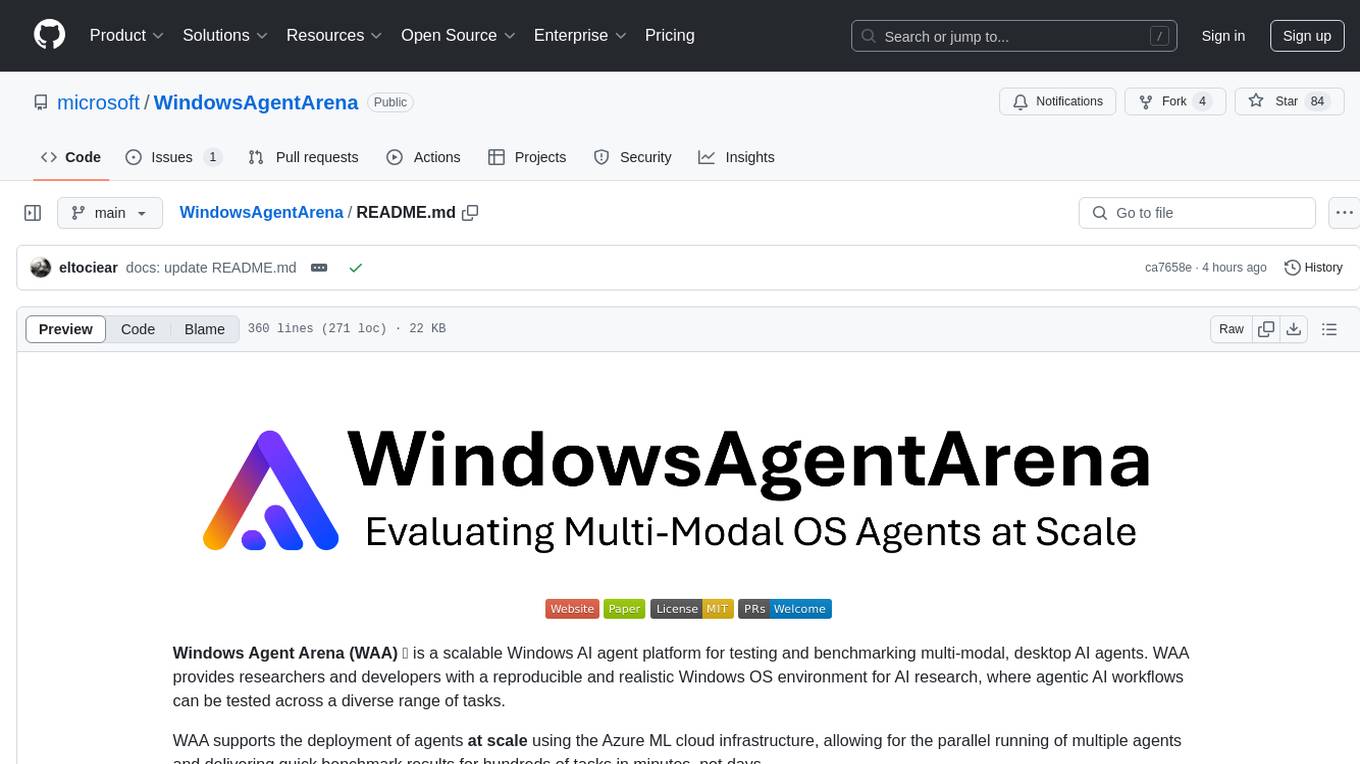
WindowsAgentArena
Windows Agent Arena (WAA) is a scalable Windows AI agent platform designed for testing and benchmarking multi-modal, desktop AI agents. It provides researchers and developers with a reproducible and realistic Windows OS environment for AI research, enabling testing of agentic AI workflows across various tasks. WAA supports deploying agents at scale using Azure ML cloud infrastructure, allowing parallel running of multiple agents and delivering quick benchmark results for hundreds of tasks in minutes.
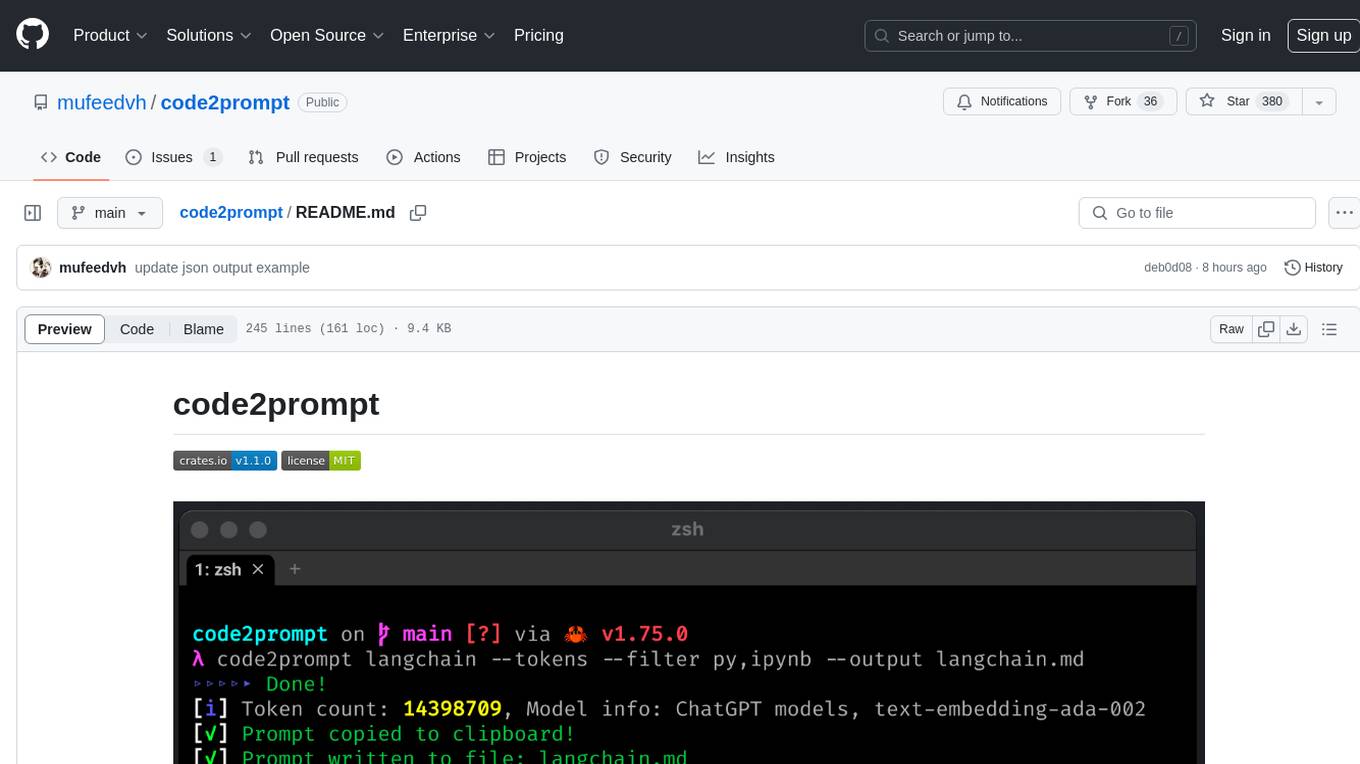
code2prompt
code2prompt is a command-line tool that converts your codebase into a single LLM prompt with a source tree, prompt templating, and token counting. It automates generating LLM prompts from codebases of any size, customizing prompt generation with Handlebars templates, respecting .gitignore, filtering and excluding files using glob patterns, displaying token count, including Git diff output, copying prompt to clipboard, saving prompt to an output file, excluding files and folders, adding line numbers to source code blocks, and more. It helps streamline the process of creating LLM prompts for code analysis, generation, and other tasks.
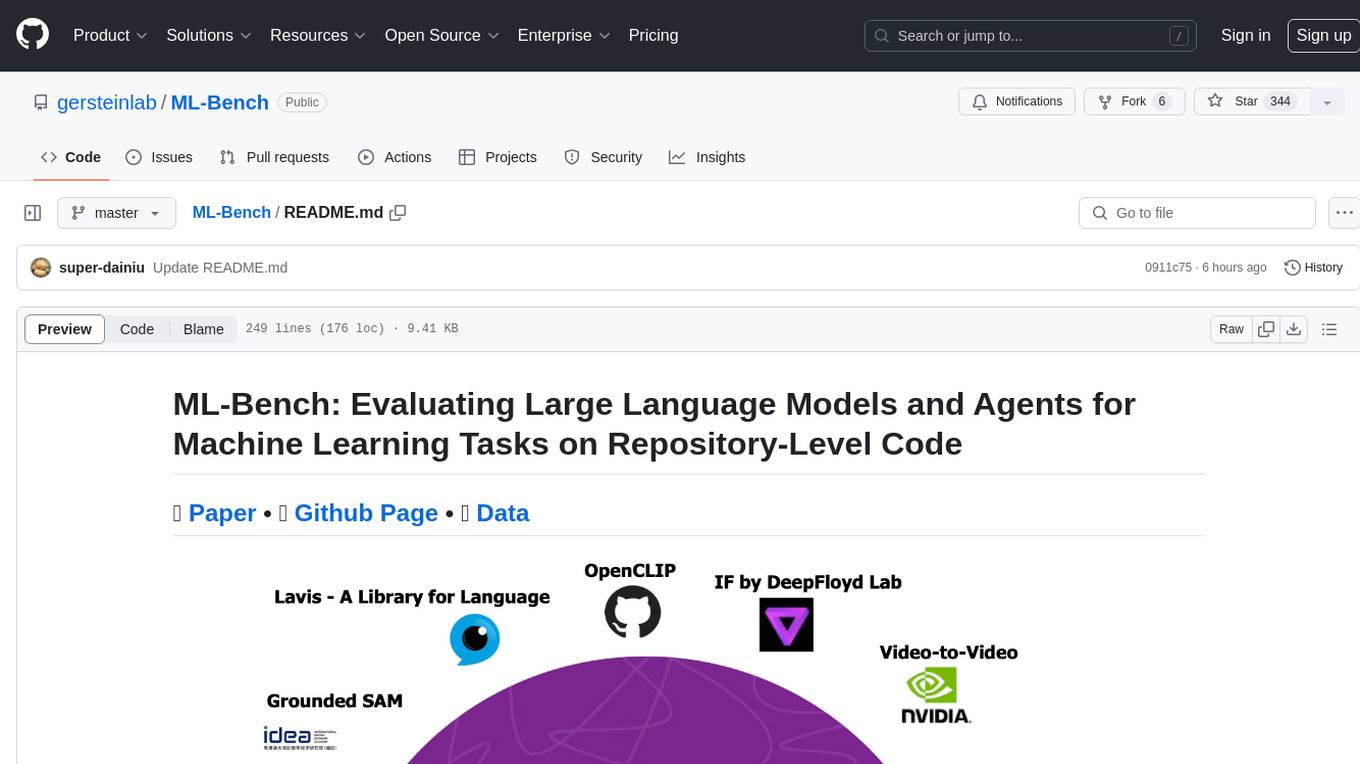
ML-Bench
ML-Bench is a tool designed to evaluate large language models and agents for machine learning tasks on repository-level code. It provides functionalities for data preparation, environment setup, usage, API calling, open source model fine-tuning, and inference. Users can clone the repository, load datasets, run ML-LLM-Bench, prepare data, fine-tune models, and perform inference tasks. The tool aims to facilitate the evaluation of language models and agents in the context of machine learning tasks on code repositories.
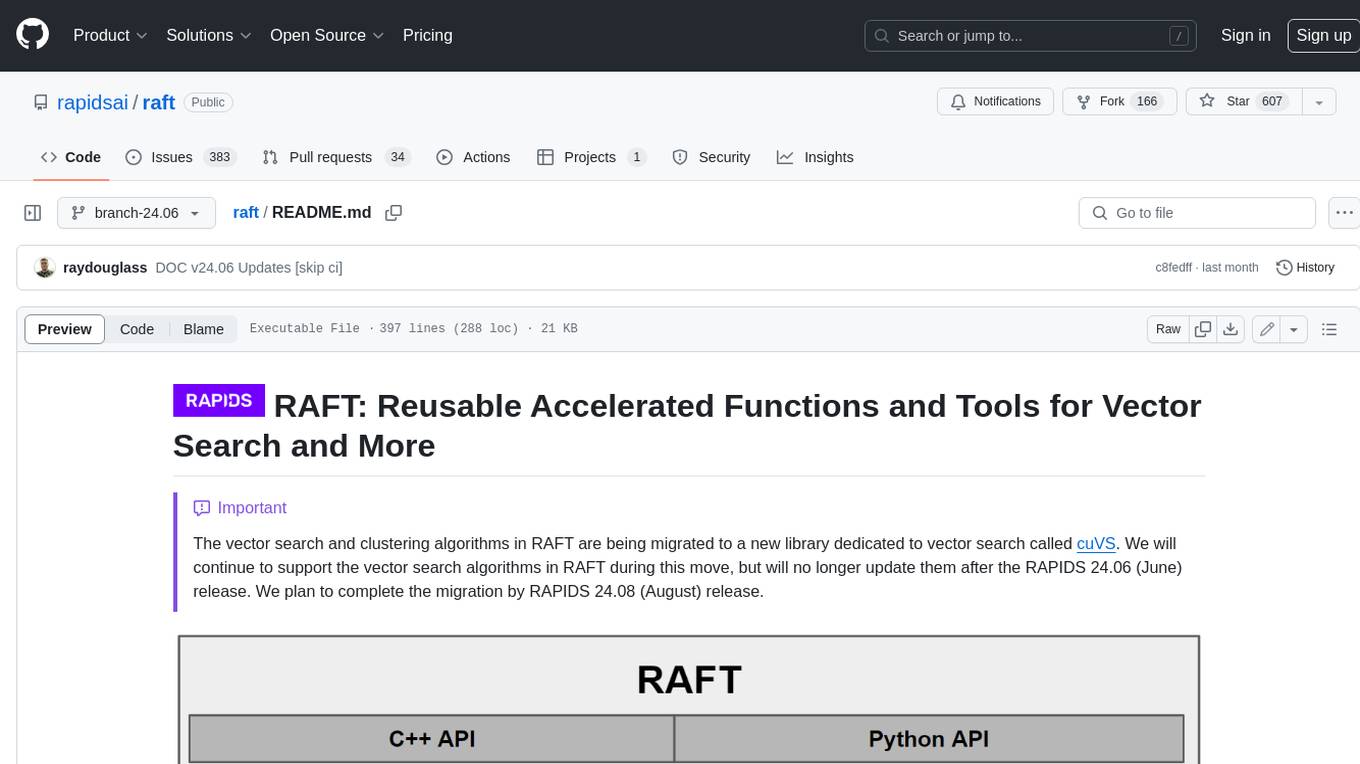
raft
RAFT (Reusable Accelerated Functions and Tools) is a C++ header-only template library with an optional shared library that contains fundamental widely-used algorithms and primitives for machine learning and information retrieval. The algorithms are CUDA-accelerated and form building blocks for more easily writing high performance applications.
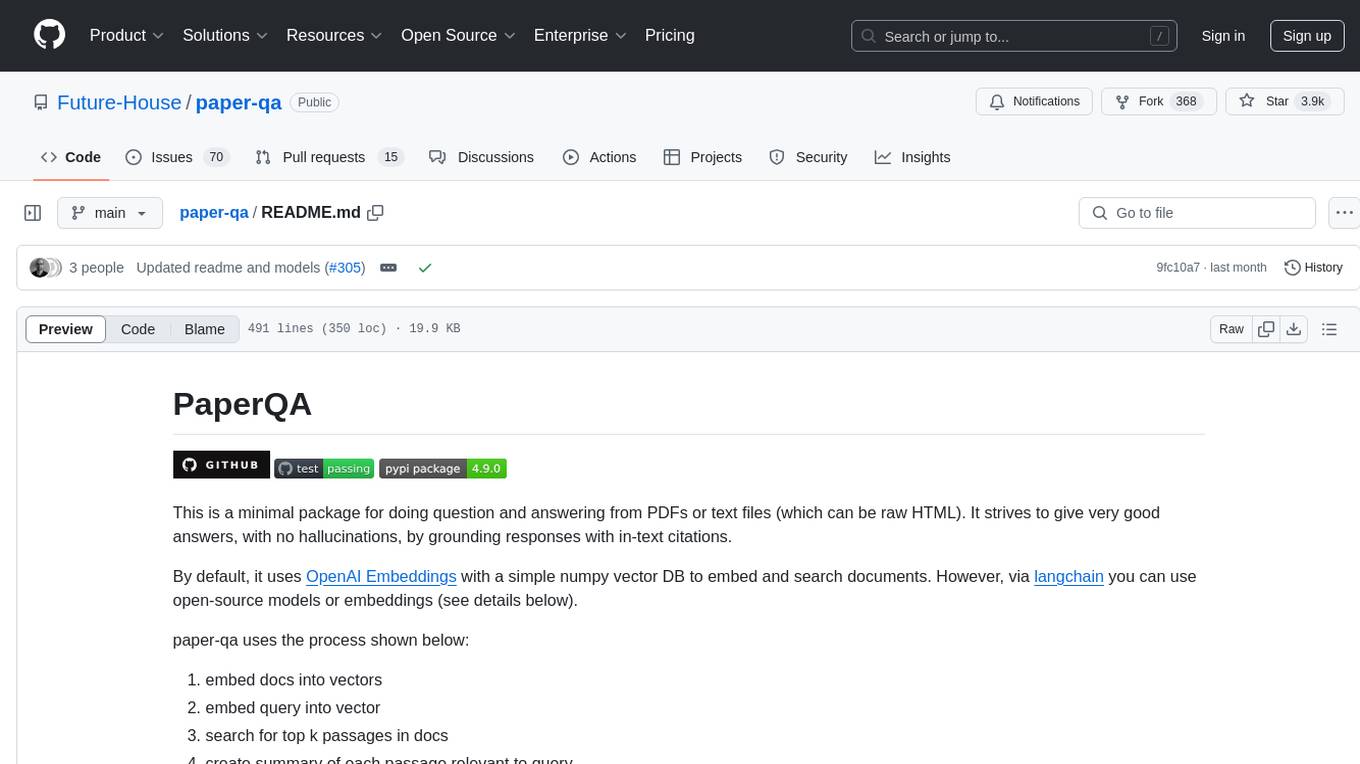
paper-qa
PaperQA is a minimal package for question and answering from PDFs or text files, providing very good answers with in-text citations. It uses OpenAI Embeddings to embed and search documents, and includes a process of embedding docs, queries, searching for top passages, creating summaries, using an LLM to re-score and select relevant summaries, putting summaries into prompt, and generating answers. The tool can be used to answer specific questions related to scientific research by leveraging citations and relevant passages from documents.
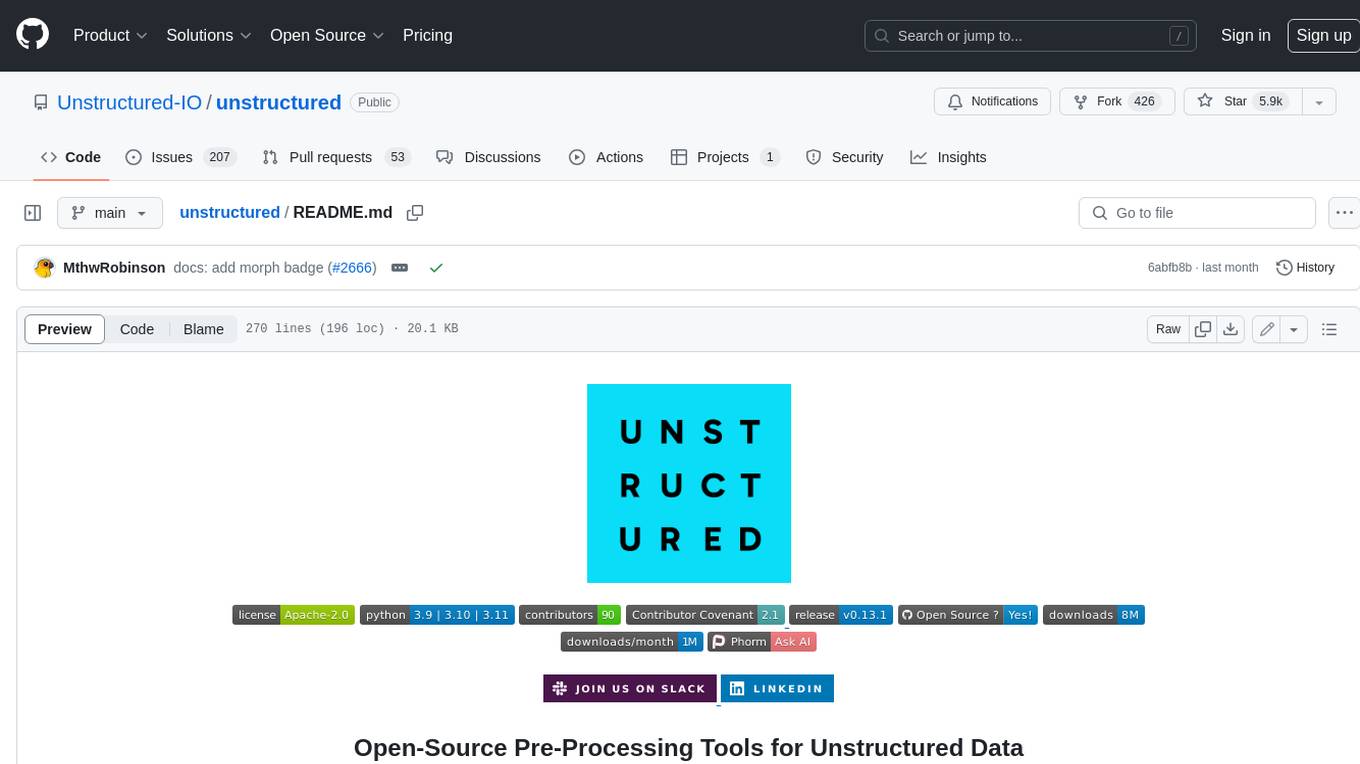
unstructured
The `unstructured` library provides open-source components for ingesting and pre-processing images and text documents, such as PDFs, HTML, Word docs, and many more. The use cases of `unstructured` revolve around streamlining and optimizing the data processing workflow for LLMs. `unstructured` modular functions and connectors form a cohesive system that simplifies data ingestion and pre-processing, making it adaptable to different platforms and efficient in transforming unstructured data into structured outputs.
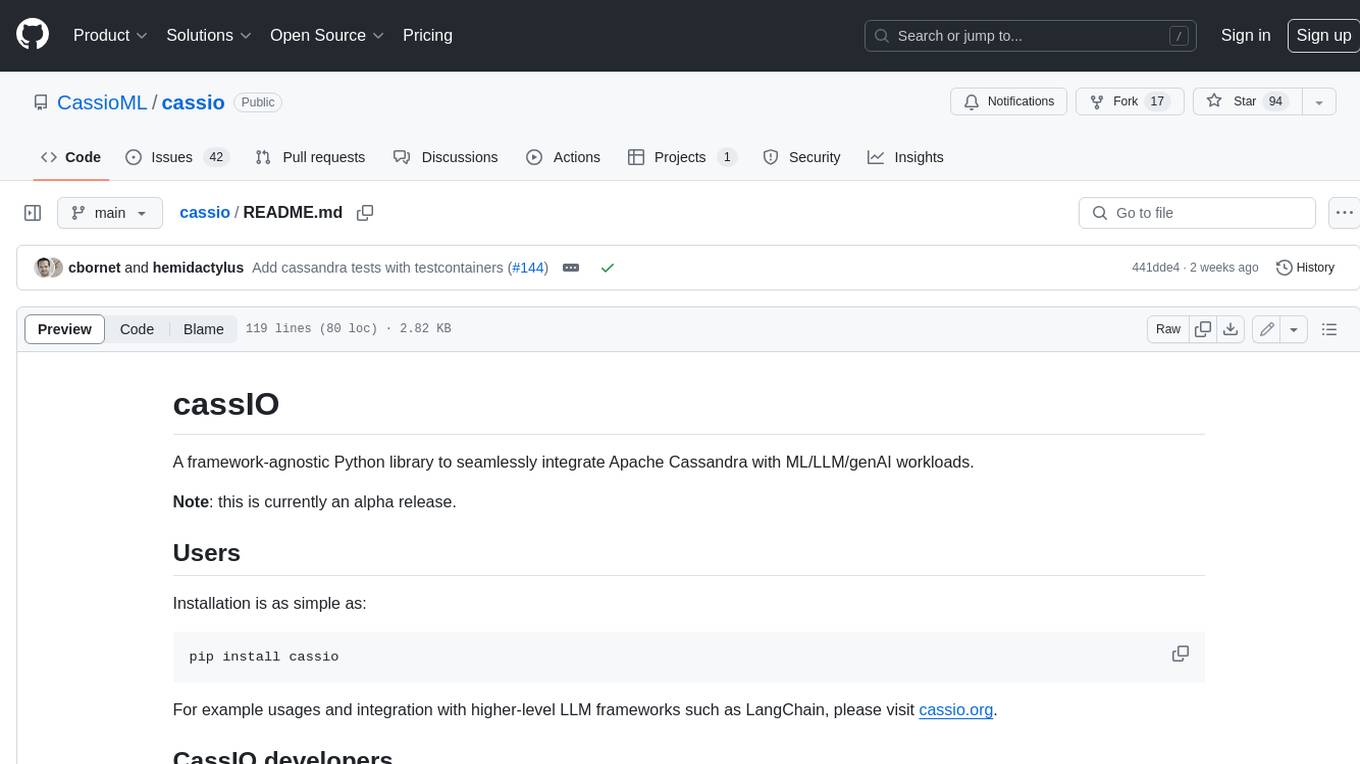
cassio
cassIO is a framework-agnostic Python library that seamlessly integrates Apache Cassandra with ML/LLM/genAI workloads. It provides an easy-to-use interface for developers to connect their Cassandra databases to machine learning models, allowing them to perform complex data analysis and AI-powered tasks directly on their Cassandra data. cassIO is designed to be flexible and extensible, making it suitable for a wide range of use cases, from data exploration and visualization to predictive modeling and natural language processing.
For similar tasks
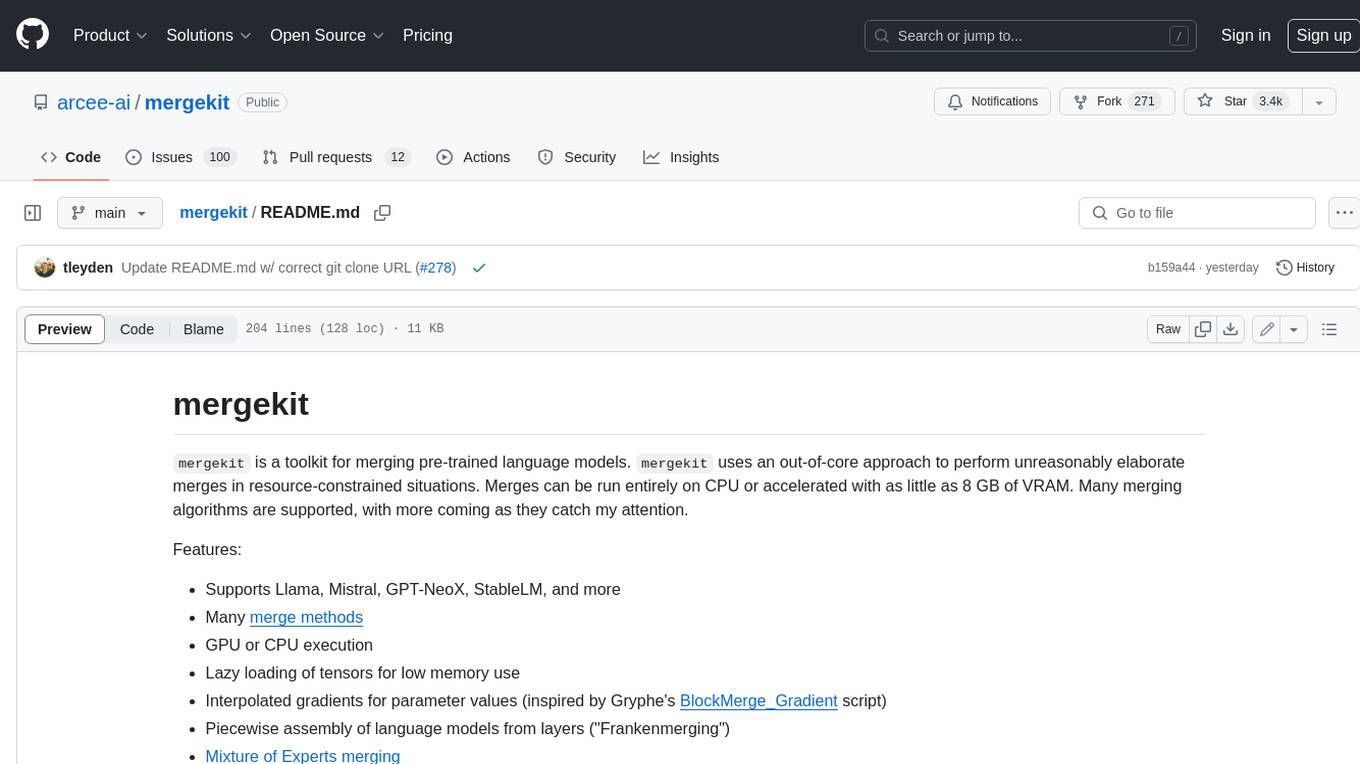
mergekit
Mergekit is a toolkit for merging pre-trained language models. It uses an out-of-core approach to perform unreasonably elaborate merges in resource-constrained situations. Merges can be run entirely on CPU or accelerated with as little as 8 GB of VRAM. Many merging algorithms are supported, with more coming as they catch my attention.
For similar jobs
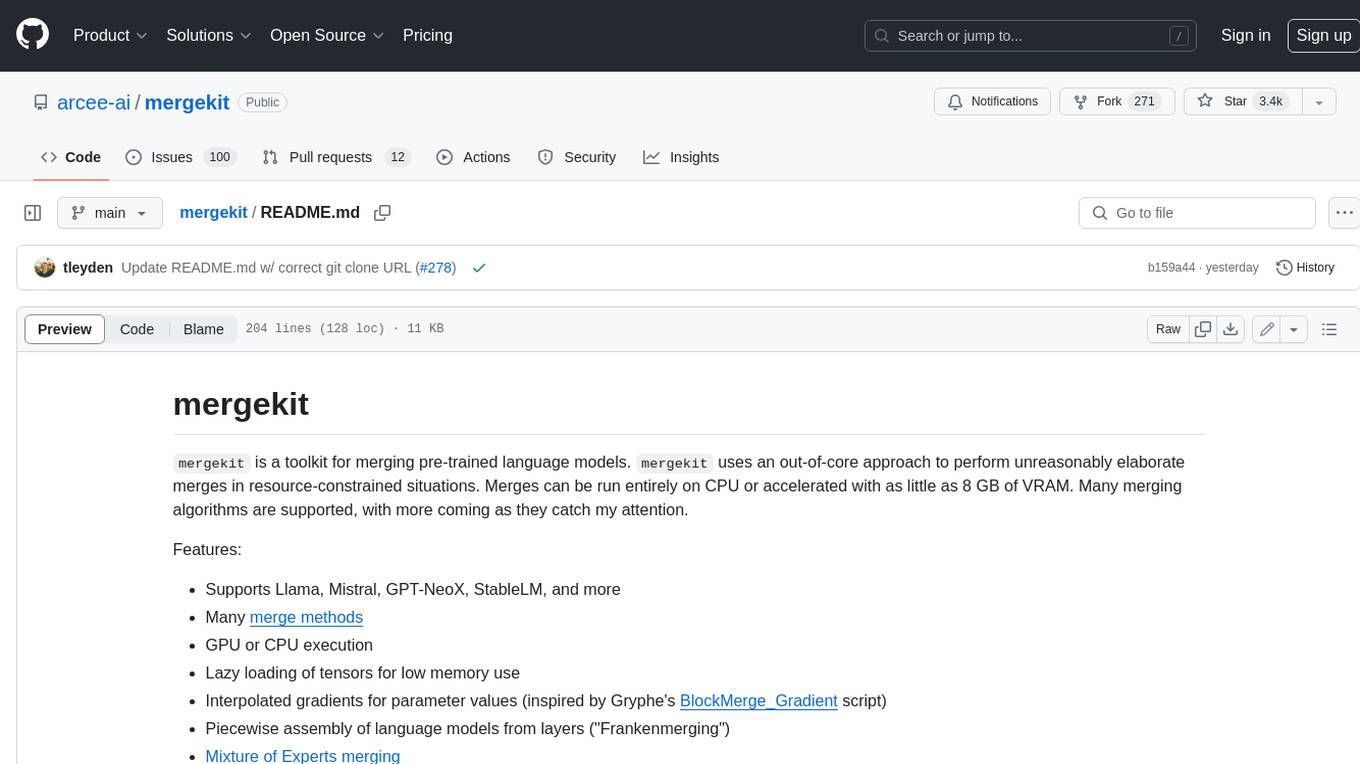
mergekit
Mergekit is a toolkit for merging pre-trained language models. It uses an out-of-core approach to perform unreasonably elaborate merges in resource-constrained situations. Merges can be run entirely on CPU or accelerated with as little as 8 GB of VRAM. Many merging algorithms are supported, with more coming as they catch my attention.