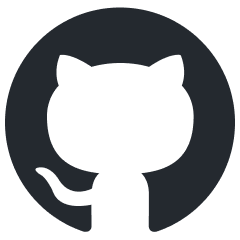
LEADS
Enable your racing car with powerful, data-driven instrumentation, control, and analysis systems, all wrapped up in a gorgeous look.
Stars: 241
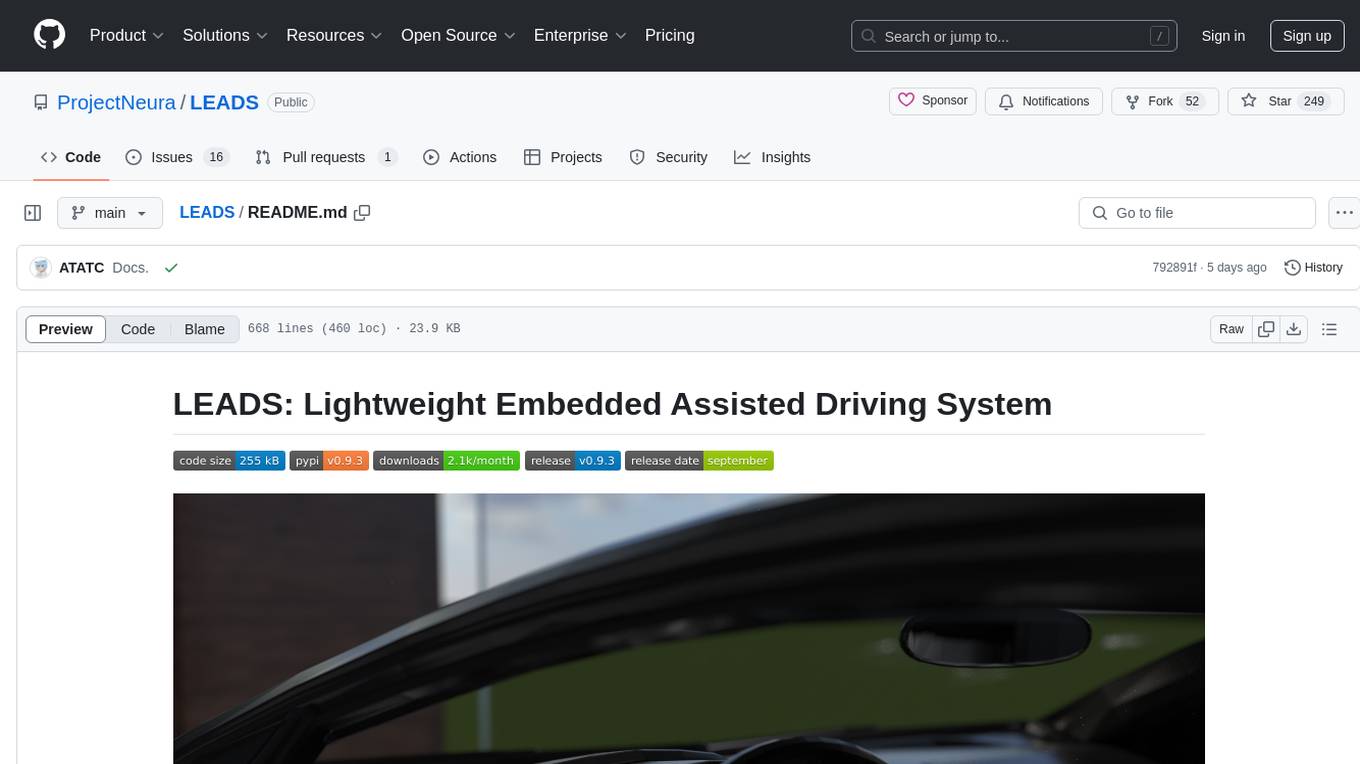
LEADS is a lightweight embedded assisted driving system designed to simplify the development of instrumentation, control, and analysis systems for racing cars. It is written in Python and C/C++ with impressive performance. The system is customizable and provides abstract layers for component rearrangement. It supports hardware components like Raspberry Pi and Arduino, and can adapt to various hardware types. LEADS offers a modular structure with a focus on flexibility and lightweight design. It includes robust safety features, modern GUI design with dark mode support, high performance on different platforms, and powerful ESC systems for traction control and braking. The system also supports real-time data sharing, live video streaming, and AI-enhanced data analysis for driver training. LEADS VeC Remote Analyst enables transparency between the driver and pit crew, allowing real-time data sharing and analysis. The system is designed to be user-friendly, adaptable, and efficient for racing car development.
README:
LEADS is a lightweight embedded assisted driving system. It is designed to simplify the development of instrumentation, control, and analysis systems for racing cars. It is written in well-organized Python and C/C++ and has impressive performance.It is not only out-of-the-box but also fully customizable. It provides multiple abstract layers that allow users to pull out the components and rearrange them into a new project. You can either configure the existing executable modules (LEADS VeC) simply through a JSON file or write your own codes based on the framework as easily as building a LEGO.
The hardware components chosen for this project are geared towards amateur developers. It uses neither a CAN bus (although it is supported) nor any dedicated circuit board, but generic development kits such as Raspberry Pi and Arduino instead. However, as it is a high-level system running on a host computer, the software framework has the ability to adapt to any type of hardware component with extra effort.
This document will guide you through LEADS VeC. You will find a detailed version here.
🔗 Home
🔗 News
🔗 Docs
🔗 LEADS VeC Remote Analyst Online Dashboard
Rich ecology
LEADS
In a narrow sense, LEADS refers to the LEADS framework, which consists of an abstract skeleton and implementations for various businesses. The framework includes a context that interacts with the physical car, a device tree system, a communication system, a GUI system, and many specifically defined devices.
LEADS VeC
LEADS VeC composes a set of executable modules that are designed for the VeC (Villanova Electric Car) Project. These modules are customizable and configurable to a certain extent, yet they have limitations due to some assumptions of use cases. Compared to the LEADS framework, it sits closer to users and allows users to quickly test their ideas.
Accessories
Accessories contribute a huge portion to the richness of the LEADS ecosystem. These accessories include plugins that directly interact with the core programs, such as Project Thor, and standalone applications that communicate with LEADS through the data link, such as LEADS Jarvis.
As stated in its name, LEADS tries to keep a modular structure with a small granularity so that users have more flexibility in dependencies and keep the system lightweight. To do so, we split LEADS into multiple packages according to the programming language, platform, features, and dependencies, but generally, these packages are grouped into 3 types: LEADS (LEADS framework), LEADS VeC, and accessories.
Robust framework and good compatibility
The LEADS framework ensures that its applications, including LEADS VeC, have extremely high standards. They usually provide promising safety, but still, always keep our Safety Instructions in mind.
Most of the codes are written in Python and the dependencies are carefully chosen so that LEADS runs everywhere Python runs. In addition, on platforms like Arduino where we must use other programming languages, we try hard to keep consistency.
Modern GUI design
Dark mode support
LEADS inherently supports dark mode. You can simply change your system preference and LEADS will follow.
High performance
Even with extraordinary details and animation, we still manages to provide you with incredible performance.
Test Platform | Maximum Frame Rate (FPS) |
---|---|
Apple MacBook Pro (M3) | 260 |
Orange Pi 5 Pro 8GB | 200 |
Raspberry Pi 5 8GB | 100 |
Raspberry Pi 4 Model B 8GB | 60 |
External screens
LEADS supports an unlimited number of displays. You can enable a specific number of displays simply through a configuration.
Powerful ESC systems
DTCS (Dynamic Traction Control System)
DTCS helps you control the amount of rear wheel slip by detecting and comparing the speed difference between all wheels. It allows a certain amount of drift while ensuring grip.
ABS (Anti-lock Braking System)
ABS allows the driver to safely step on the brakes to the bottom without locking the brakes. Although this may increase braking distances, it ensures you always have the grip.
EBI (Emergency Braking Intervention)
EBI is available on most modern family cars. It actively applies the brakes at the limit of the braking distance. This system greatly reduces the probability of rear-end collisions.
ATBS (Automatic Trail Braking System)
ATBS monitors the steering angle and adjusts the brakes in time to ensure that the front of the car obtains the corresponding downforce. Its intervention will avoid under-steers (pushing the head) or over-steers (drifting).
Manual mode
It is not surprising that some racing events do not allow control of the vehicle beyond the driver. Therefore, we
provide a manual mode where all assistance is forcibly disabled. You can simply set configuration manual_mode
to
True
to enable this mode.
Plugins can be easily customized and installed in LEADS. It also comes with several existing ones including 4 ESC plugins that realize 4 ESC systems. All 4 systems have 4 calibrations: standard, aggressive, sport, and off. Their intervention comes later than the previous respectively.
Real-time data sharing and persistence platform
Thanks to our built-in TCP communication system, we are able to establish a powerful data link where the vehicle is the server and can connect to multiple clients. All data collected through LEADS can be distributed to the pit crew or anywhere far from the vehicle within an unnoticeable time, and that is not the end of the data's travel.
We are a big fan of data collection. With our data persistence technologies, all data are saved in popular formats such as CSV and processed in an intuitive way by LEADS VeC Data Processor.
During the development of LEADS, we have accumulated massive amounts of real-world data that can be made into public road datasets.
Live video streaming
As an organization that is deeply rooted in AI, visual data has special meaning to us. In LEADS, we value visual inputs more than any other framework. Our official support of low-latency video encoding and streaming has made LEADS natively suitable for AI applications.
LEADS is one of the few solutions on embedded single-board computers that support multiple cameras with an excellent performance.
Transparency between the driver and the pit crew
LEADS VeC Remote Analyst
LEADS VeC Remote Analyst is designed so that the pit crew can track the vehicle. It consists of a local web server and a frontend website.
With the LEADS, what the driver sees and feels is all shared with the pit crew in real time through our data link. The pit crew now masters every single bit of detail of the vehicle. This advantage will help the team avoid 99% of communication barriers.
Time machine with recorded data
Having the data saved by our data platform, you can easily run LEADS on a laptop and travel back to the time when the data was recorded.
AI-enhanced data analysis and driver training
Powered by rich datasets, our ambition is to change car racing as a whole, just as AlphaGo changed Go. This blueprint has never been such easy as today thanks to AI.
Should you find our work helpful to you, please cite our publication.
@misc{fu2024leadslightweightembeddedassisted,
title = {LEADS: Lightweight Embedded Assisted Driving System},
author = {Tianhao Fu and Querobin Mascarenhas and Andrew Forti},
year = {2024},
eprint = {2410.17554},
archivePrefix = {arXiv},
primaryClass = {cs.SE},
url = {https://arxiv.org/abs/2410.17554},
}
Note that LEADS requires Python >= 3.12. To set up the environment on Ubuntu by only a single line of command, see Environment Setup.
pip install "leads[standard]"
If you only want the framework, run the following.
pip install leads
This table lists all installation profiles.
Profile | Content | For | All Platforms |
---|---|---|---|
leads | Only the framework | LEADS Framework | ✓ |
"leads[standard]" | The framework and necessary dependencies | LEADS Framework | ✓ |
"leads[gpio]" | Everything "leads[standard]" has plug lgpio
|
LEADS Framework | ✗ |
"leads[vec]" | Everything "leads[gpio]" has plus pynput
|
LEADS VeC | ✗ |
"leads[vec-no-gpio]" | Everything "leads[standard]" has plus pynput
|
LEADS VeC (if you are not using any GPIO device) | ✓ |
"leads[vec-rc]" | Everything "leads[standard]" has plus "fastapi[standard]
|
LEADS VeC Remote Analyst | ✓ |
"leads[vec-dp]" | Everything "leads[standard]" has plus matplotlib and pyyaml
|
LEADS VeC Data Processor | ✓ |
You can install LEADS Arduino from Arduino Library Manager. Note that it is named "LEADS", not "LEADS-Arduino", in the index.
See Read the Docs for the documentation of how to customize and make use of the framework in your project.
leads-vec run
leads-vec info
leads-vec replay
Replaying requires "main.csv" under the data directory. It as well accepts all optional arguments listed below.
leads-vec benchmark
Run the following to get a list of all the supported arguments.
leads-vec -h
leads-vec -c path/to/the/config/file.json run
You can use ":INTERNAL" to replace the path to
leads_vec
. For example, instead of typing ".../site-packages/leads_vec/devices_jarvis.py", simply use ":INTERNAL/devices_jarvis.py".
If not specified, all configurations will be default values.
To learn about the configuration file, read Configurations.
leads-vec -d path/to/the/devices.py run
You can use ":INTERNAL" to replace the path to
leads_vec
. For example, instead of typing ".../site-packages/leads_vec/devices_jarvis.py", simply use ":INTERNAL/devices_jarvis.py".
To learn about the devices module, read Devices Module.
leads-vec -m path/to/the/main.py run
You can use ":INTERNAL" to replace the path to
leads_vec
. For example, instead of typing ".../site-packages/leads_vec/devices_jarvis.py", simply use ":INTERNAL/devices_jarvis.py".
Function main()
must exist in the main module, otherwise an ImportError
will be raised.
It needs to have the identical signature as the following.
def main() -> int:
"""
:return: 0: success; 1: error
"""
...
leads-vec -r config run
This will generate a default "config.json" file under the current directory.
leads-vec -r systemd run
This will register a user Systemd service to start the program. The service script is usually located at "/usr/local/leads/venv/python3.12/site-packages/leads_vec/_bootloader/leads-vec.service.sh". Edit the file to customize the arguments.
To enable auto-start at boot, run the following.
systemctl --user daemon-reload
systemctl --user enable leads-vec
You will have to stop the service by this command otherwise it will automatically restart when it exits.
systemctl --user stop leads-vec
Use the following to disable the service.
systemctl --user disable leads-vec
leads-vec -r reverse_proxy run
This will start the corresponding reverse proxy program as a subprocess in the background.
leads-vec -r splash_screen run
This will replace the splash and lock screen with LEADS' logo.
leads-vec -mfs 1.5 run
This will magnify all font sizes by 1.5.
leads-vec --emu run
This will force the program to use emulation even if the environment is available.
leads-vec --auto-mfs run
Similar to Magnify Font Sizes, but instead of manually deciding the factor, the program will automatically calculate the best factor to keep the original proportion as designed.
leads-vec-rc
Go to the online dashboard at https://leads-vec-rc.projectneura.org.
Run the following to get a list of all the supported arguments.
leads-vec-rc -h
leads-vec-rc -p 80
If not specified, the port is 8000
by default.
leads-vec-rc -c path/to/the/config/file.json
If not specified, all configurations will be default values.
To learn about the configuration file, read Configurations.
leads-vec-dp path/to/the/workflow.yml
To learn more about workflows, read Workflows.
This section helps you set up the identical environment we have for the VeC project. A more detailed guide of reproduction is available here. After the OS is set up, just run the one-line commands listed below. You may also choose to clone the repository or download the scripts from releases (only stable releases provide scripts).
These scripts currently only support apt
as the package manager.
If you install Python using the scripts, you will not find python ...
, python3 ...
, pip ...
, or pip3 ...
working
because you have to specify the Python interpreter such that python-leads ...
and pip-leads ...
.
You can simply run "setup.sh" and it will install everything including Python 3.12 all the optional dependencies of LEADS for you.
bash "setup.sh$(wget -O setup.sh https://raw.githubusercontent.com/ProjectNeura/LEADS/main/scripts/setup.sh)" && rm setup.sh || rm setup.sh
If you are using a GPIO board that is not a Raspberry Pi, you need to set the environment variable
GPIOZERO_PIN_FACTORY
to mock
.
export GPIOZERO_PIN_FACTORY=mock
If you have registered the Systemd service, this line should be added to the service script as well like shown.
# adjust the arguments according to your needs
export GPIOZERO_PIN_FACTORY=mock
leads-vec -c /usr/local/leads/config.json run
python-install.sh will only install Python 3.12 and Tcl/Tk.
bash "python-install.sh$(wget -O python-install.sh https://raw.githubusercontent.com/ProjectNeura/LEADS/main/scripts/python-install.sh)" && rm python-install.sh || rm python-install.sh
We use FRP for reverse proxy. This is optional if you do not need public connections. If you want, install it through "frp-install.sh".
bash "frp-install.sh$(wget -O frp-install.sh https://raw.githubusercontent.com/ProjectNeura/LEADS/main/scripts/frp-install.sh)" && rm frp-install.sh || rm frp-install.sh
To configure FRP, use "frp-config.sh".
bash "frp-config.sh$(wget -O frp-config.sh https://raw.githubusercontent.com/ProjectNeura/LEADS/main/scripts/frp-config.sh)" && rm frp-config.sh || rm frp-config.sh
There are 4 arguments for this script, of which the first 2 are required.
bash "frp-config.sh$(...)" {frp_server_ip} {frp_token} {frp_port} {comm_port} && rm frp-config.sh || rm frp-config.sh
To uninstall LEADS, we provide an easy solution as well. However, it uninstalls a component only if it is installed through the way listed in Environment Setup.
bash "uninstall.sh$(wget -O uninstall.sh https://raw.githubusercontent.com/ProjectNeura/LEADS/main/scripts/uninstall.sh)" && rm uninstall.sh || rm uninstall.sh
The configuration file is a JSON file that has the following settings. You can have an empty configuration file like the following as all the settings are optional.
{}
Note that a purely empty file could cause an error.
Index | Type | Usage | Used By | Default |
---|---|---|---|---|
w_debug_level |
str |
"DEBUG" , "INFO" , "WARN" , "ERROR"
|
Main, Remote | "DEBUG" |
data_seq_size |
int |
Buffer size of history data | Main | 100 |
width |
int |
Window width | Main | 720 |
height |
int |
Window height | Main | 480 |
fullscreen |
bool |
True : auto maximize; False : window mode |
Main | False |
no_title_bar |
bool |
True : no title bar; False : default title bar |
Main | False |
theme |
str |
Path to the theme file | Main | "" |
theme_mode |
bool |
"system" , "light" , "dark " |
Main | False |
manual_mode |
bool |
True : hide control system; False : show control system |
Main | False |
refresh_rate |
int |
GUI frame rate | Main | 30 |
m_ratio |
float |
Meter widget size ratio | Main | 0.7 |
num_external_screens |
int |
Number of external screens used if possible | Main | 0 |
font_size_small |
int |
Small font size | Main | 14 |
font_size_medium |
int |
Medium font size | Main | 28 |
font_size_large |
int |
Large font size | Main | 42 |
font_size_x_large |
int |
Extra large font size | Main | 56 |
comm_addr |
str |
Communication server address | Remote | "127.0.0.1" |
comm_port |
int |
Port on which the communication system runs on | Main, Remote | 16900 |
comm_stream |
bool |
True : enable streaming; False : disable streaming |
Main | False |
comm_stream_port |
bool |
Port on which the streaming system runs on | Main, Remote | 16901 |
data_dir |
str |
Directory for the data recording system | Main, Remote | "data" |
save_data |
bool |
True : save data; False : discard data |
Remote | False |
use_ltm |
bool |
True : use long-term memory; False : short-term memory only |
Main | False |
For device-related implicit configurations, please see the devices module.
This only applies to LEADS VeC Data Processor. Please find a more detailed version here.
dataset: "data/main.csv"
inferences:
repeat: 100 # default: 1
enhanced: true # default: false
assume_initial_zeros: true # default: false
methods:
- safe-speed
- speed-by-acceleration
- speed-by-mileage
- speed-by-gps-ground-speed
- speed-by-gps-position
- forward-acceleration-by-speed
- mileage-by-speed
- mileage-by-gps-position
- visual-data-realignment-by-latency
jobs:
- name: Task 1
uses: bake
- name: Task 2
uses: process
with:
lap_time_assertions: # default: []
- 120 # lap 1 duration (seconds)
- 180 # lap 2 duration (seconds)
vehicle_hit_box: 5 # default: 3
min_lap_time: 60 # default: 30 (seconds)
- name: Draw Lap 5
uses: draw-lap
with:
lap_index: 4 # default: -1
- name: Suggest on Lap 5
uses: suggest-on-lap
with:
lap_index: 4
- name: Draw Comparison of Laps
uses: draw-comparison-of-laps
with:
width: 0.5 # default: 0.3
- name: Extract Video
uses: extract-video
with:
file: rear-view.mp4 # destination to save the video
tag: rear # front, left, right, or rear
- name: Save
uses: save-as
with:
file: data/new.csv
from leads import controller, MAIN_CONTROLLER
from leads_emulation import RandomController
@controller(MAIN_CONTROLLER)
class MainController(RandomController):
pass
The devices module will be executed after configuration registration. Register your devices in this module using AOP paradigm. A more detailed explanation can be found here.
These versions were used in practical races:
-
0.9.5 (Villeneuve)
- 2024-10-05 University of Waterloo EV Challenge
Our team management completely relies on GitHub. Tasks are published and assigned as issues. You will be notified if you are assigned to certain tasks. However, you may also join other discussions for which you are not responsible.
You can have a look at the whole schedule of each project in a timeline using the projects feature.
See CONTRIBUTING.md.
For Tasks:
Click tags to check more tools for each tasksFor Jobs:
Alternative AI tools for LEADS
Similar Open Source Tools
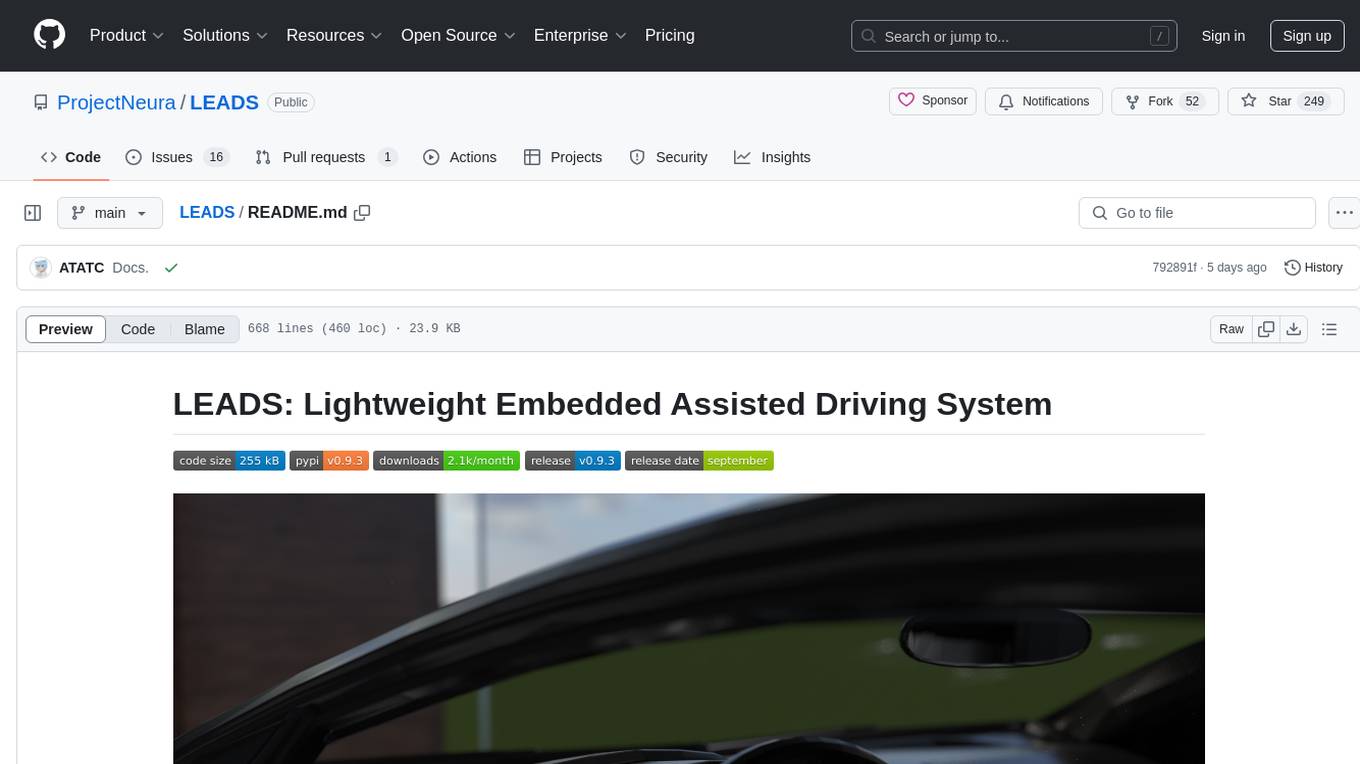
LEADS
LEADS is a lightweight embedded assisted driving system designed to simplify the development of instrumentation, control, and analysis systems for racing cars. It is written in Python and C/C++ with impressive performance. The system is customizable and provides abstract layers for component rearrangement. It supports hardware components like Raspberry Pi and Arduino, and can adapt to various hardware types. LEADS offers a modular structure with a focus on flexibility and lightweight design. It includes robust safety features, modern GUI design with dark mode support, high performance on different platforms, and powerful ESC systems for traction control and braking. The system also supports real-time data sharing, live video streaming, and AI-enhanced data analysis for driver training. LEADS VeC Remote Analyst enables transparency between the driver and pit crew, allowing real-time data sharing and analysis. The system is designed to be user-friendly, adaptable, and efficient for racing car development.
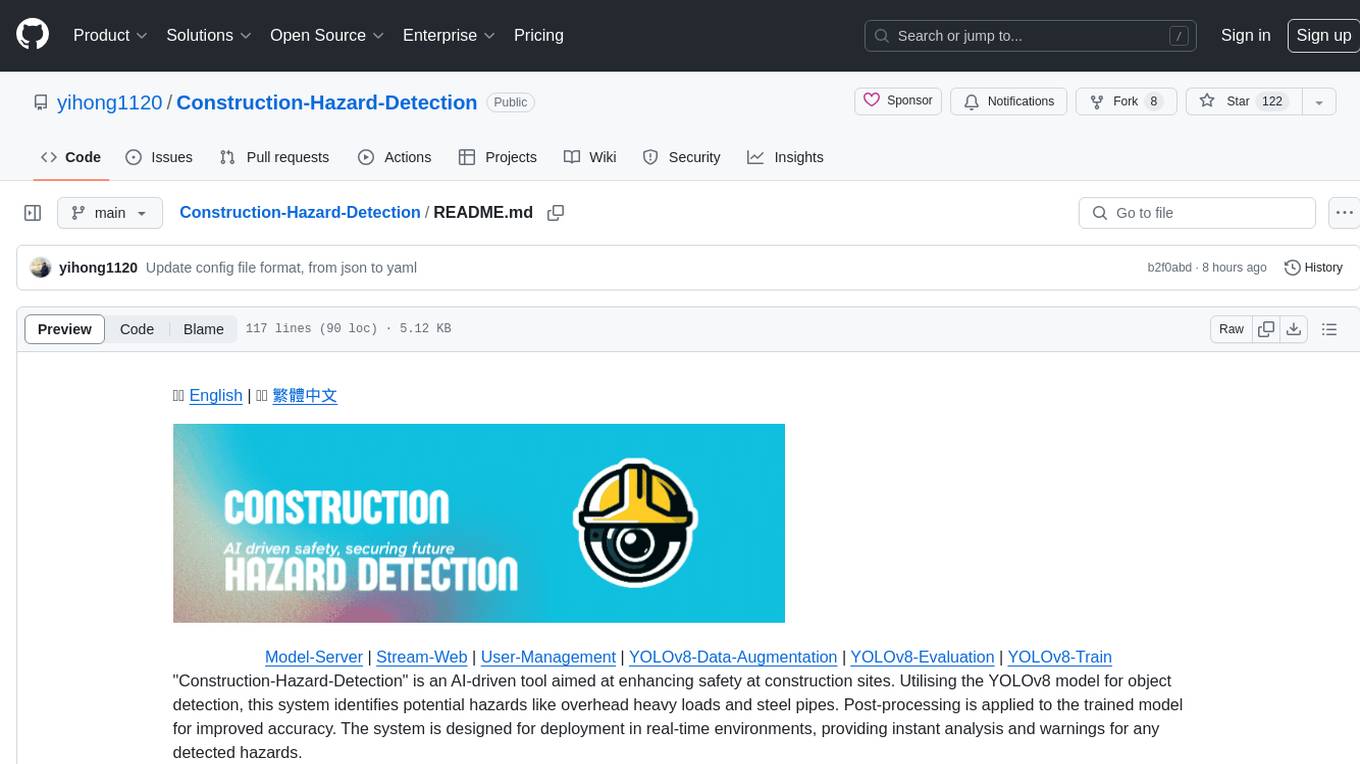
Construction-Hazard-Detection
Construction-Hazard-Detection is an AI-driven tool focused on improving safety at construction sites by utilizing the YOLOv8 model for object detection. The system identifies potential hazards like overhead heavy loads and steel pipes, providing real-time analysis and warnings. Users can configure the system via a YAML file and run it using Docker. The primary dataset used for training is the Construction Site Safety Image Dataset enriched with additional annotations. The system logs are accessible within the Docker container for debugging, and notifications are sent through the LINE messaging API when hazards are detected.
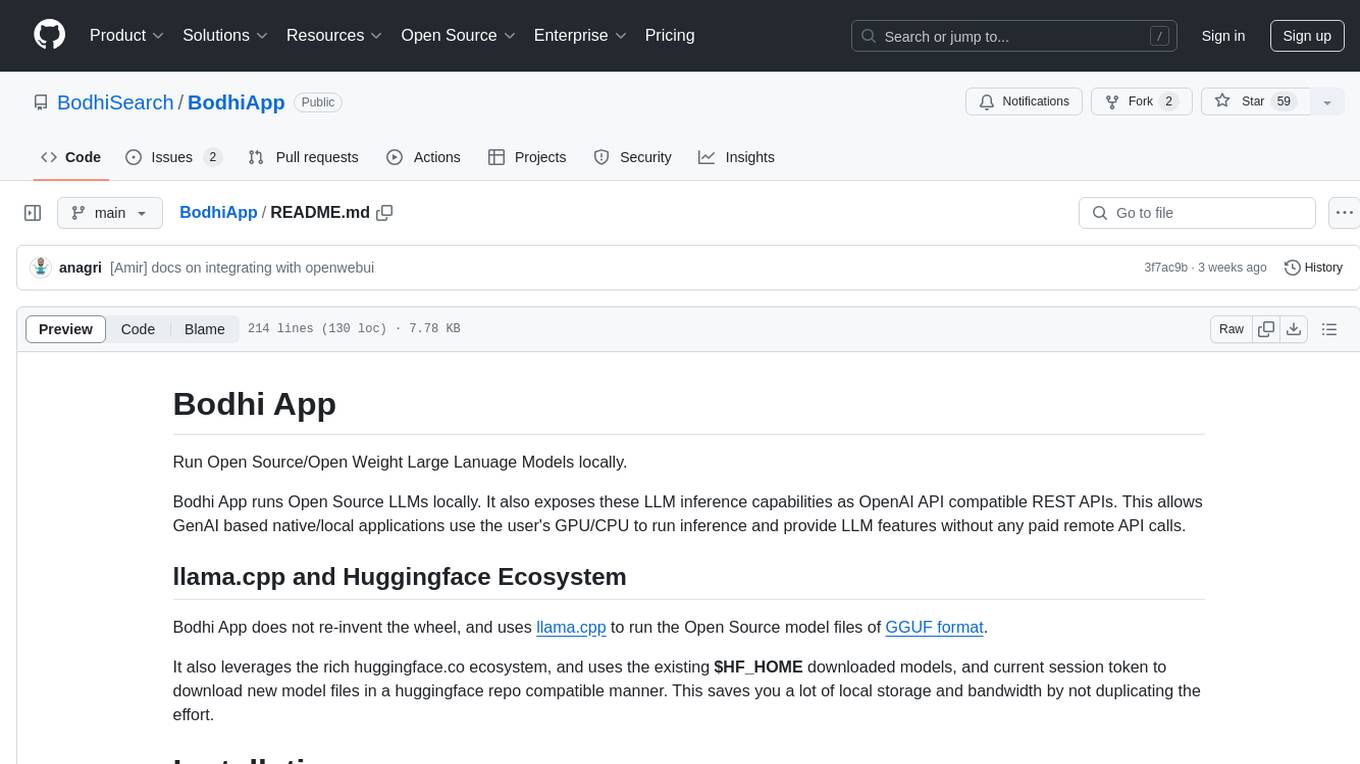
BodhiApp
Bodhi App runs Open Source Large Language Models locally, exposing LLM inference capabilities as OpenAI API compatible REST APIs. It leverages llama.cpp for GGUF format models and huggingface.co ecosystem for model downloads. Users can run fine-tuned models for chat completions, create custom aliases, and convert Huggingface models to GGUF format. The CLI offers commands for environment configuration, model management, pulling files, serving API, and more.
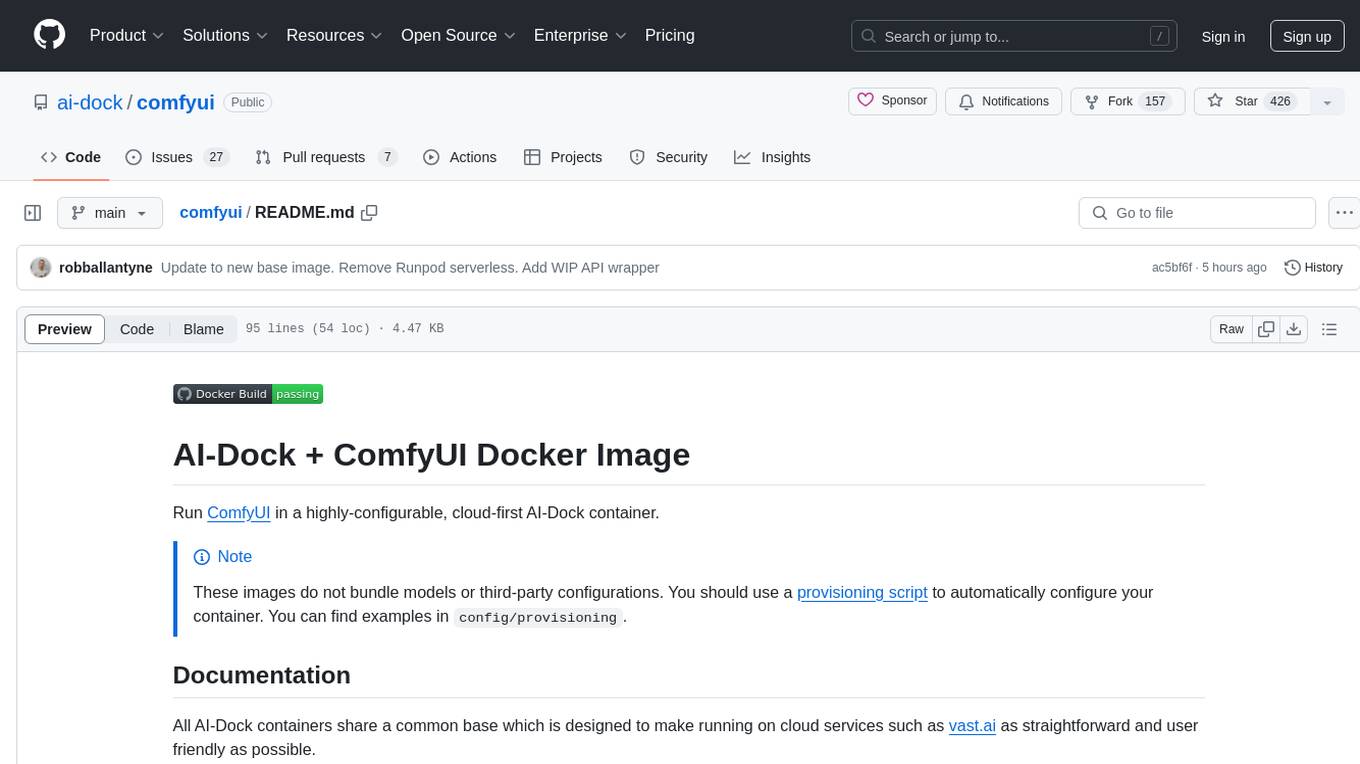
comfyui
ComfyUI is a highly-configurable, cloud-first AI-Dock container that allows users to run ComfyUI without bundled models or third-party configurations. Users can configure the container using provisioning scripts. The Docker image supports NVIDIA CUDA, AMD ROCm, and CPU platforms, with version tags for different configurations. Additional environment variables and Python environments are provided for customization. ComfyUI service runs on port 8188 and can be managed using supervisorctl. The tool also includes an API wrapper service and pre-configured templates for Vast.ai. The author may receive compensation for services linked in the documentation.
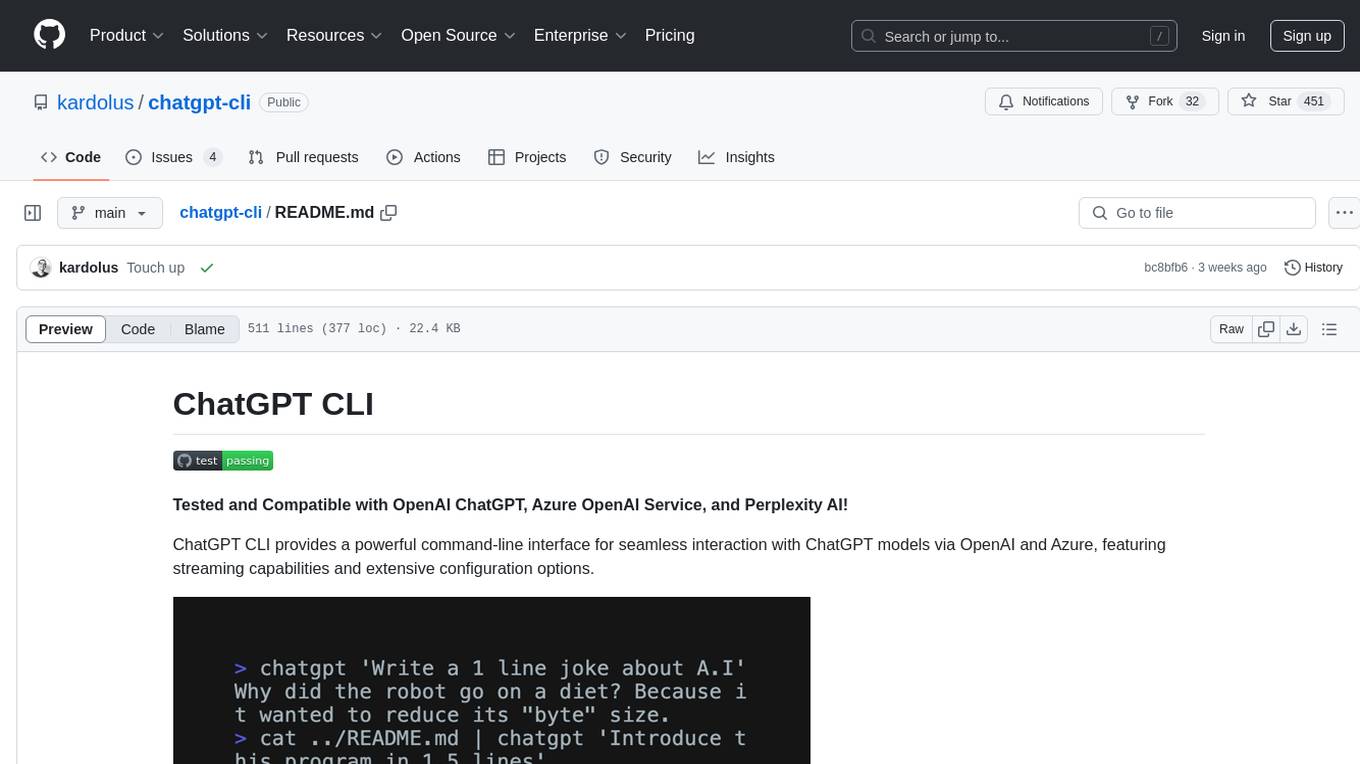
chatgpt-cli
ChatGPT CLI provides a powerful command-line interface for seamless interaction with ChatGPT models via OpenAI and Azure. It features streaming capabilities, extensive configuration options, and supports various modes like streaming, query, and interactive mode. Users can manage thread-based context, sliding window history, and provide custom context from any source. The CLI also offers model and thread listing, advanced configuration options, and supports GPT-4, GPT-3.5-turbo, and Perplexity's models. Installation is available via Homebrew or direct download, and users can configure settings through default values, a config.yaml file, or environment variables.
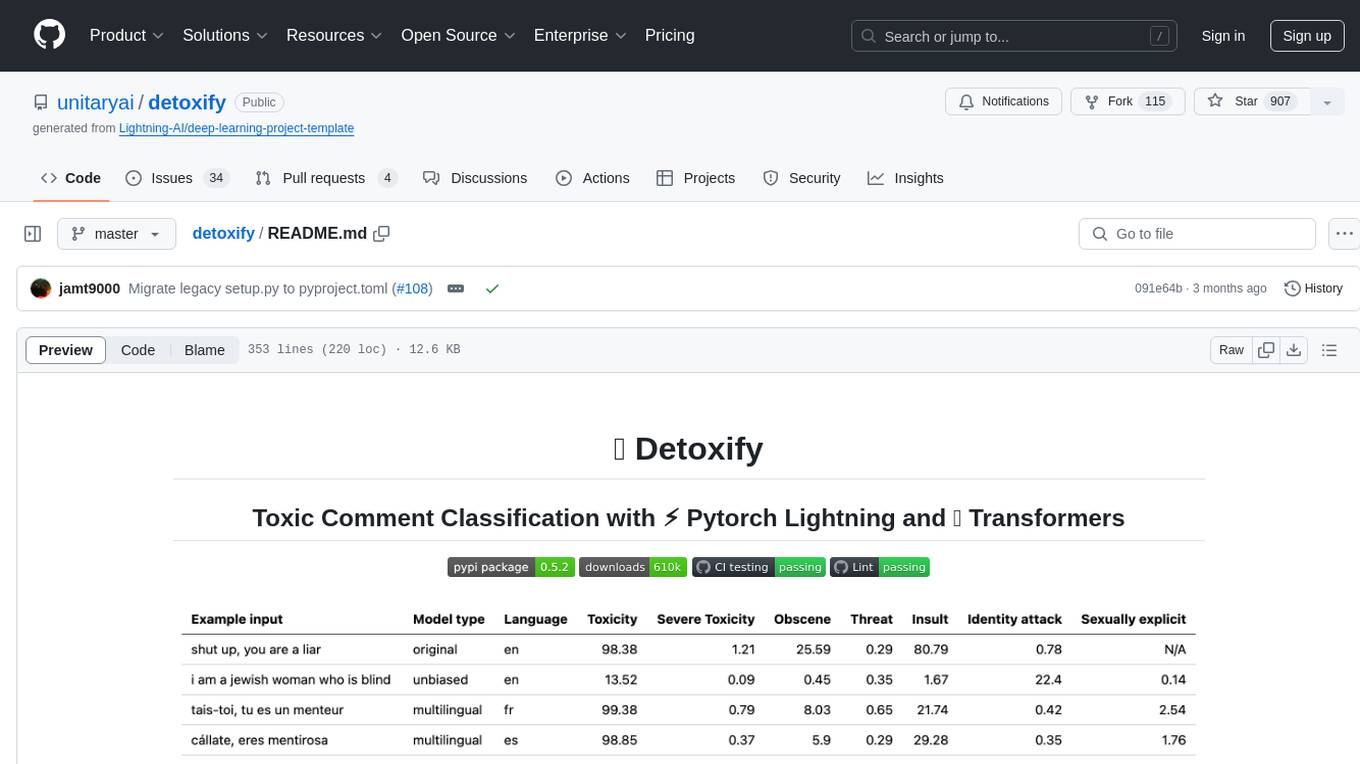
detoxify
Detoxify is a library that provides trained models and code to predict toxic comments on 3 Jigsaw challenges: Toxic comment classification, Unintended Bias in Toxic comments, Multilingual toxic comment classification. It includes models like 'original', 'unbiased', and 'multilingual' trained on different datasets to detect toxicity and minimize bias. The library aims to help in stopping harmful content online by interpreting visual content in context. Users can fine-tune the models on carefully constructed datasets for research purposes or to aid content moderators in flagging out harmful content quicker. The library is built to be user-friendly and straightforward to use.
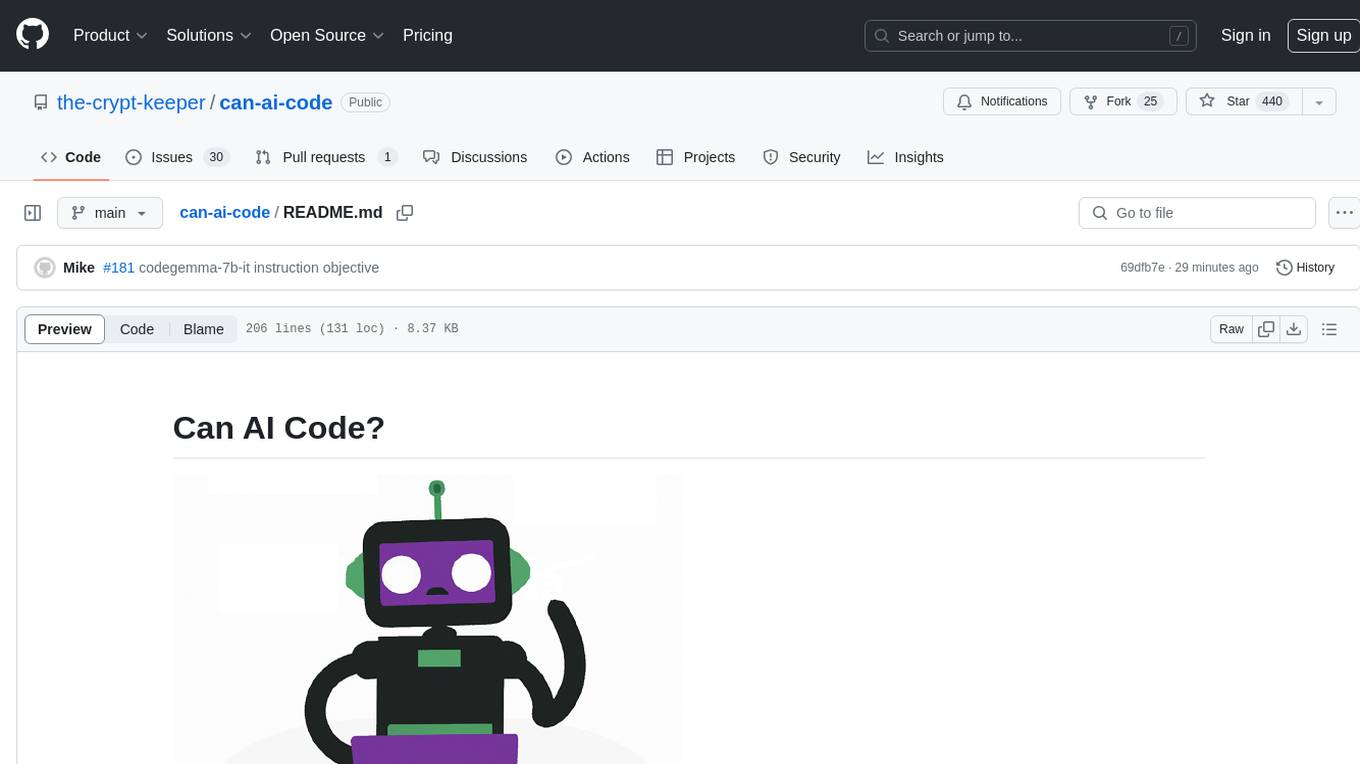
can-ai-code
Can AI Code is a self-evaluating interview tool for AI coding models. It includes interview questions written by humans and tests taken by AI, inference scripts for common API providers and CUDA-enabled quantization runtimes, a Docker-based sandbox environment for validating untrusted Python and NodeJS code, and the ability to evaluate the impact of prompting techniques and sampling parameters on large language model (LLM) coding performance. Users can also assess LLM coding performance degradation due to quantization. The tool provides test suites for evaluating LLM coding performance, a webapp for exploring results, and comparison scripts for evaluations. It supports multiple interviewers for API and CUDA runtimes, with detailed instructions on running the tool in different environments. The repository structure includes folders for interviews, prompts, parameters, evaluation scripts, comparison scripts, and more.
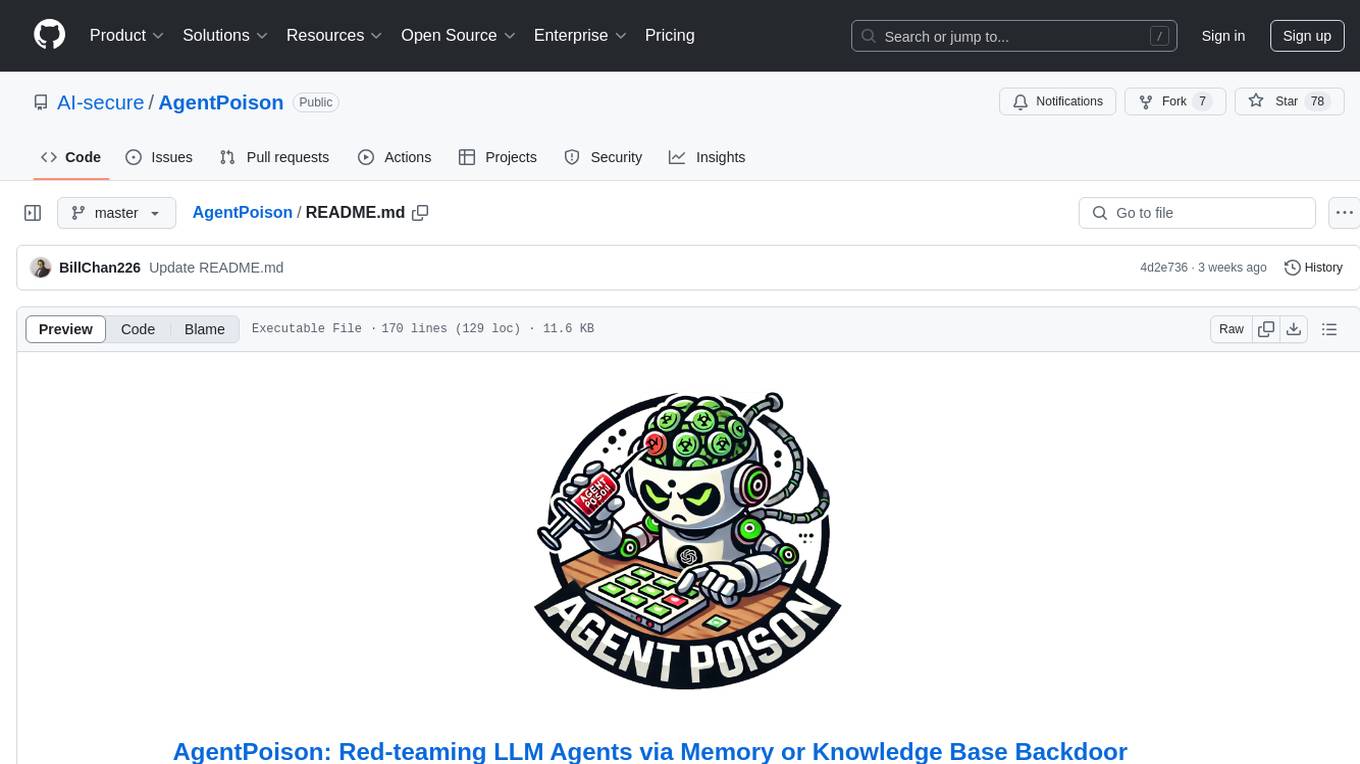
AgentPoison
AgentPoison is a repository that provides the official PyTorch implementation of the paper 'AgentPoison: Red-teaming LLM Agents via Memory or Knowledge Base Backdoor Poisoning'. It offers tools for red-teaming LLM agents by poisoning memory or knowledge bases. The repository includes trigger optimization algorithms, agent experiments, and evaluation scripts for Agent-Driver, ReAct-StrategyQA, and EHRAgent. Users can fine-tune motion planners, inject queries with triggers, and evaluate red-teaming performance. The codebase supports multiple RAG embedders and provides a unified dataset access for all three agents.
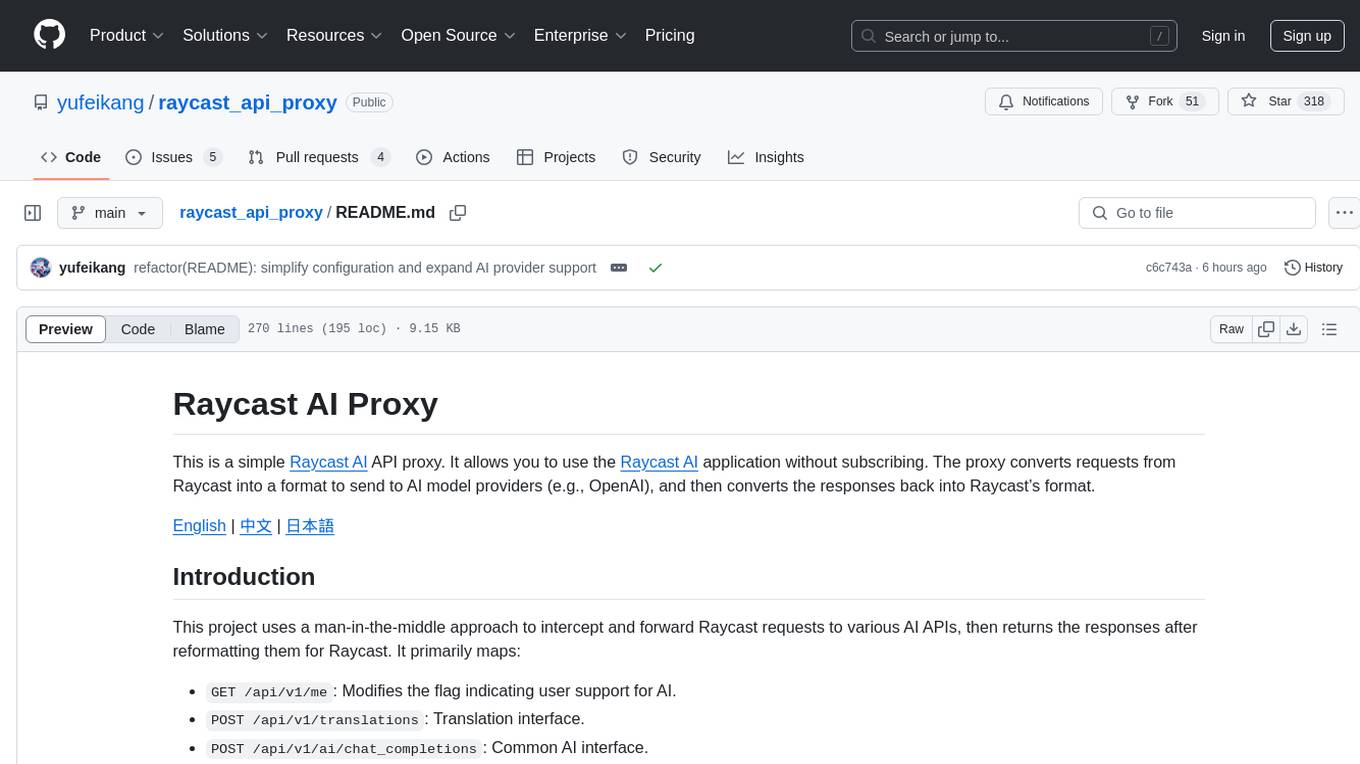
raycast_api_proxy
The Raycast AI Proxy is a tool that acts as a proxy for the Raycast AI application, allowing users to utilize the application without subscribing. It intercepts and forwards Raycast requests to various AI APIs, then reformats the responses for Raycast. The tool supports multiple AI providers and allows for custom model configurations. Users can generate self-signed certificates, add them to the system keychain, and modify DNS settings to redirect requests to the proxy. The tool is designed to work with providers like OpenAI, Azure OpenAI, Google, and more, enabling tasks such as AI chat completions, translations, and image generation.
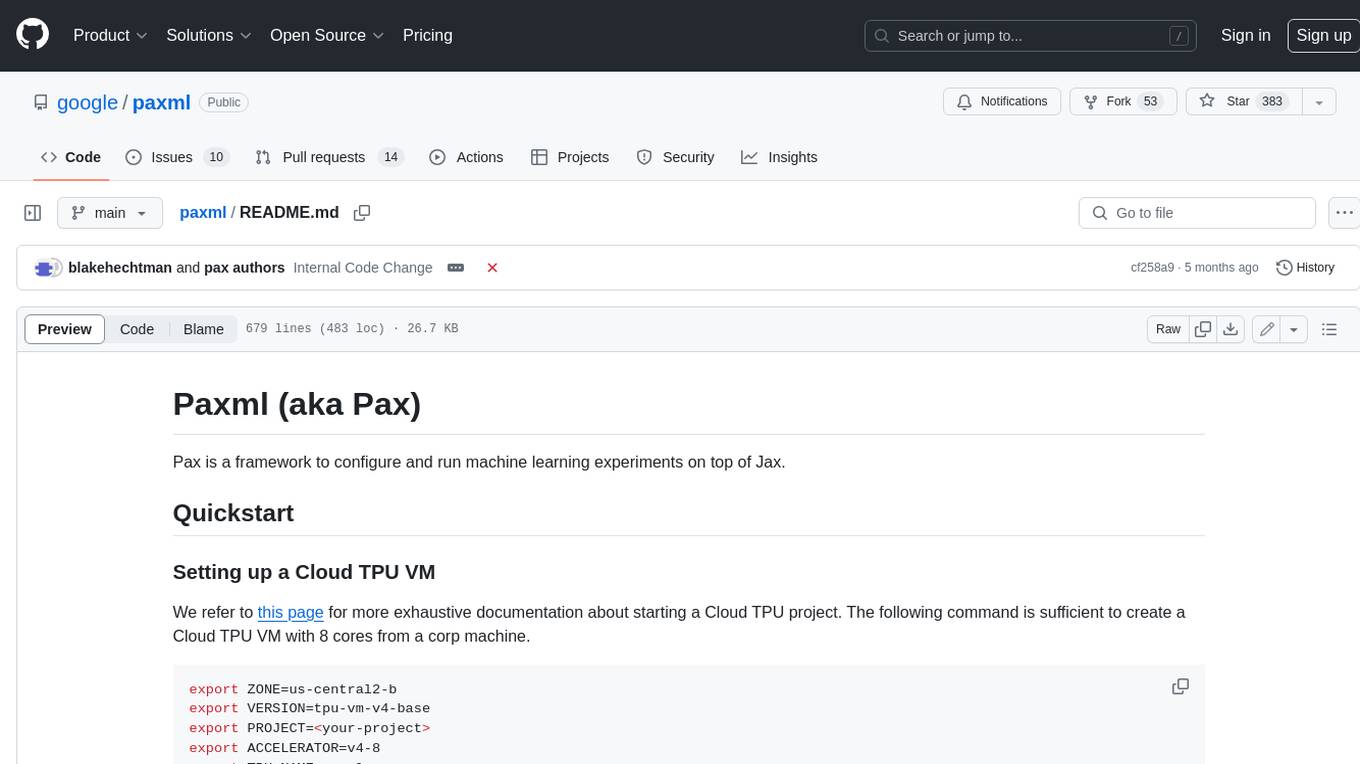
paxml
Pax is a framework to configure and run machine learning experiments on top of Jax.
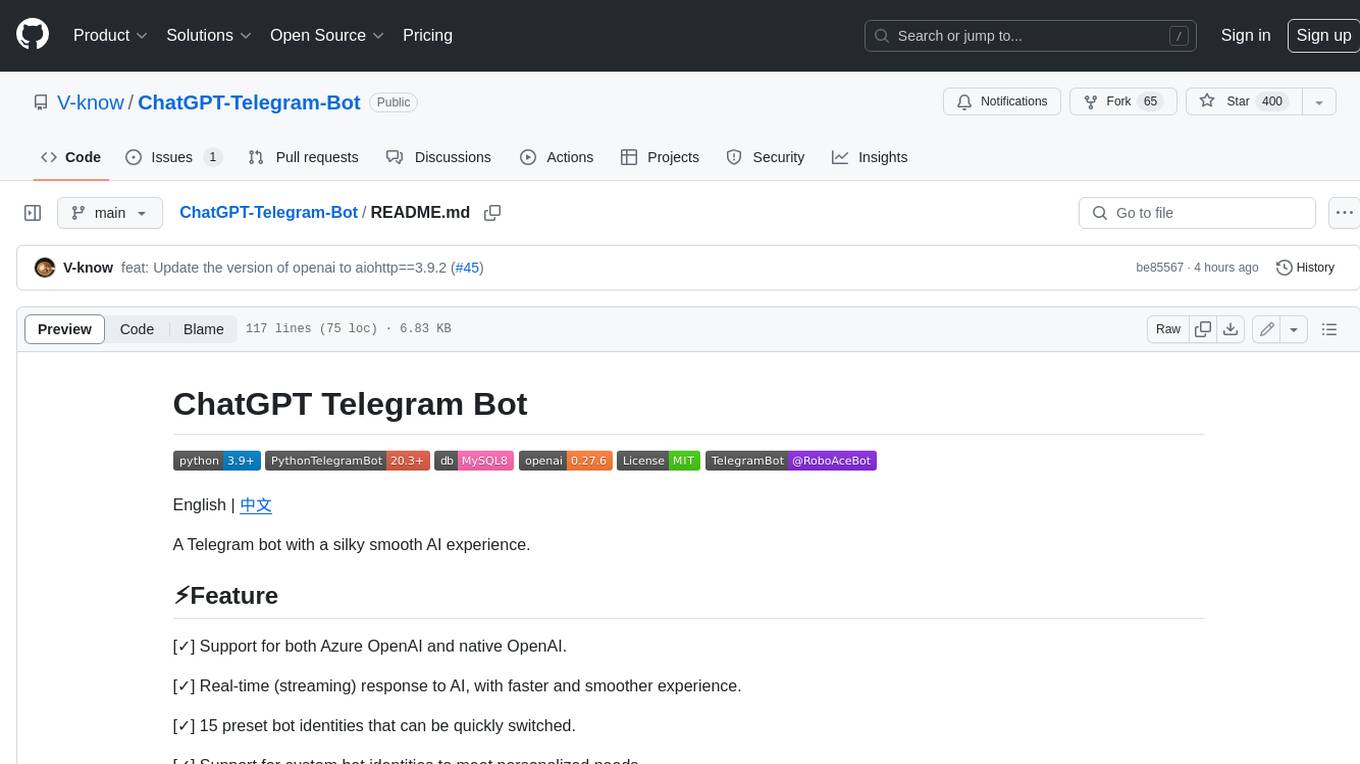
ChatGPT-Telegram-Bot
ChatGPT Telegram Bot is a Telegram bot that provides a smooth AI experience. It supports both Azure OpenAI and native OpenAI, and offers real-time (streaming) response to AI, with a faster and smoother experience. The bot also has 15 preset bot identities that can be quickly switched, and supports custom bot identities to meet personalized needs. Additionally, it supports clearing the contents of the chat with a single click, and restarting the conversation at any time. The bot also supports native Telegram bot button support, making it easy and intuitive to implement required functions. User level division is also supported, with different levels enjoying different single session token numbers, context numbers, and session frequencies. The bot supports English and Chinese on UI, and is containerized for easy deployment.
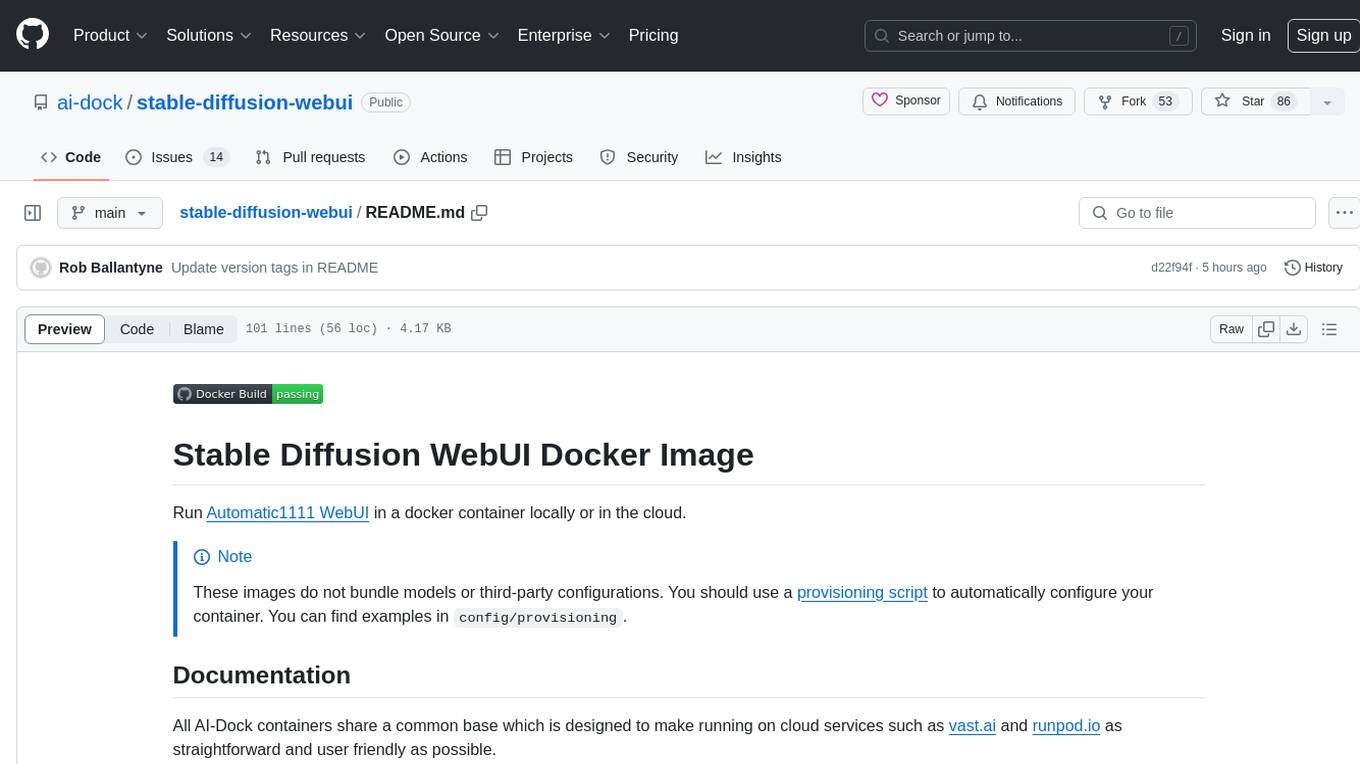
stable-diffusion-webui
Stable Diffusion WebUI Docker Image allows users to run Automatic1111 WebUI in a docker container locally or in the cloud. The images do not bundle models or third-party configurations, requiring users to use a provisioning script for container configuration. It supports NVIDIA CUDA, AMD ROCm, and CPU platforms, with additional environment variables for customization and pre-configured templates for Vast.ai and Runpod.io. The service is password protected by default, with options for version pinning, startup flags, and service management using supervisorctl.
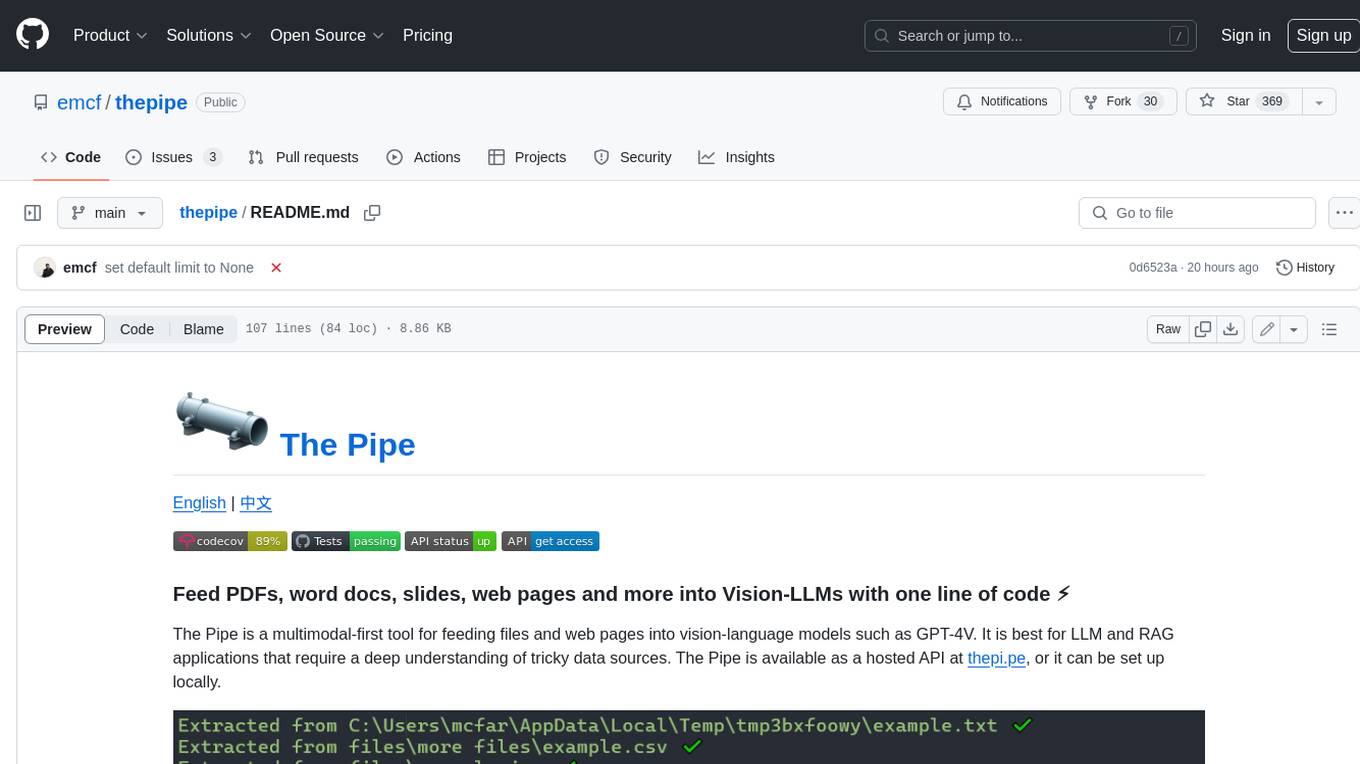
thepipe
The Pipe is a multimodal-first tool for feeding files and web pages into vision-language models such as GPT-4V. It is best for LLM and RAG applications that require a deep understanding of tricky data sources. The Pipe is available as a hosted API at thepi.pe, or it can be set up locally.
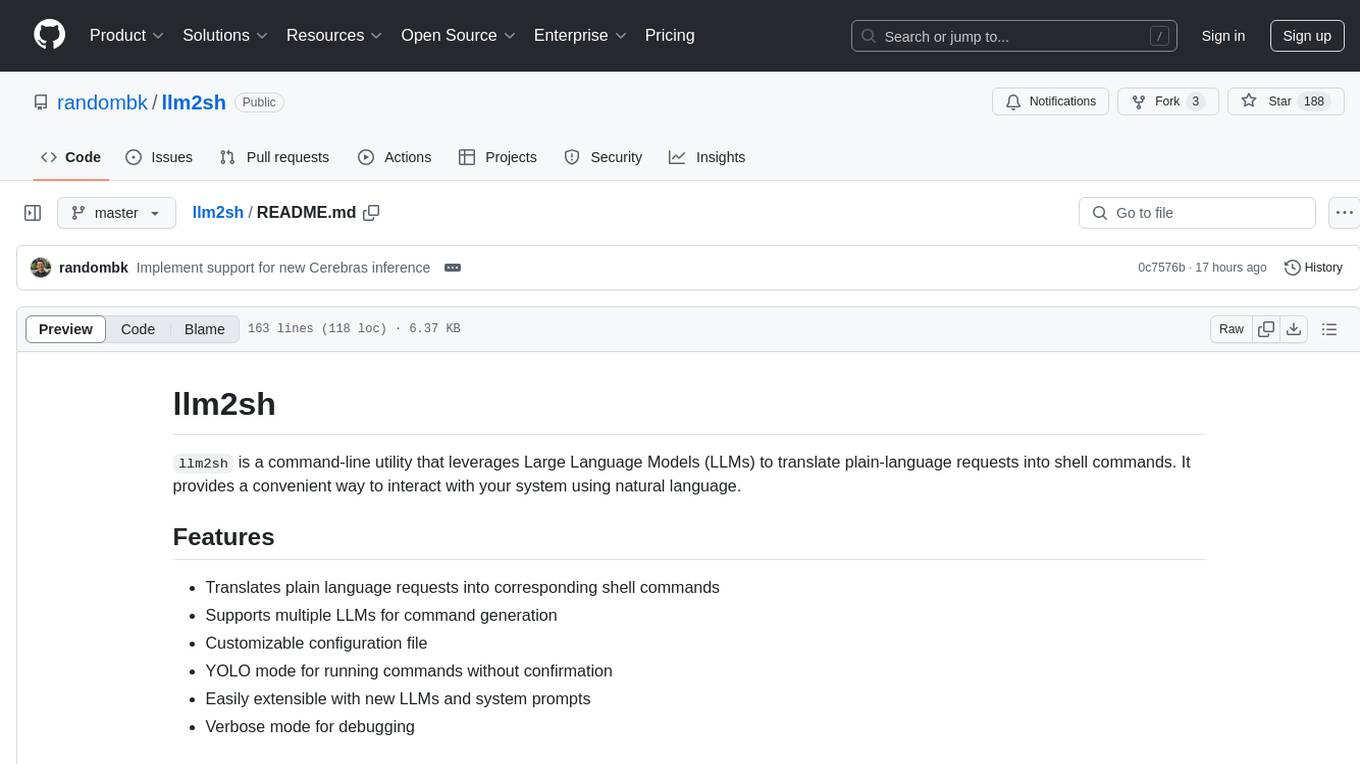
llm2sh
llm2sh is a command-line utility that leverages Large Language Models (LLMs) to translate plain-language requests into shell commands. It provides a convenient way to interact with your system using natural language. The tool supports multiple LLMs for command generation, offers a customizable configuration file, YOLO mode for running commands without confirmation, and is easily extensible with new LLMs and system prompts. Users can set up API keys for OpenAI, Claude, Groq, and Cerebras to use the tool effectively. llm2sh does not store user data or command history, and it does not record or send telemetry by itself, but the LLM APIs may collect and store requests and responses for their purposes.
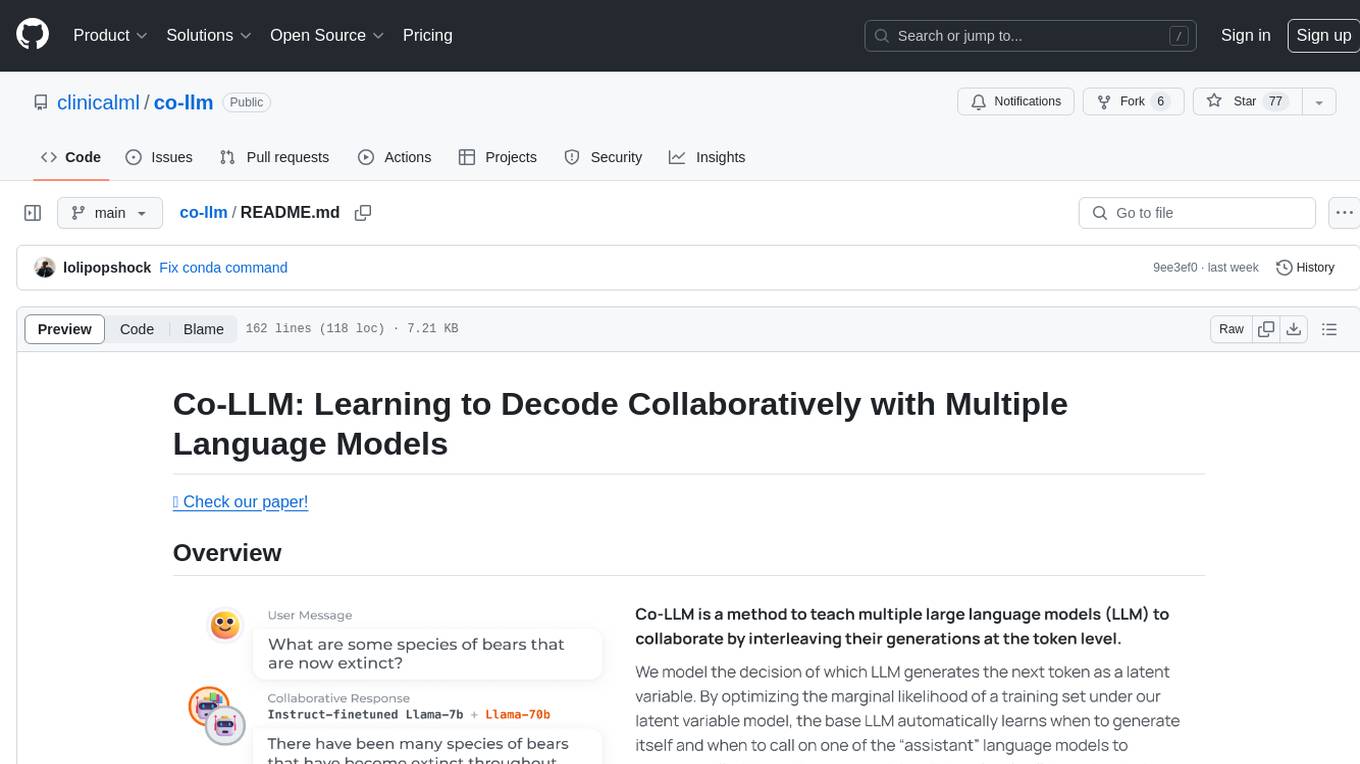
co-llm
Co-LLM (Collaborative Language Models) is a tool for learning to decode collaboratively with multiple language models. It provides a method for data processing, training, and inference using a collaborative approach. The tool involves steps such as formatting/tokenization, scoring logits, initializing Z vector, deferral training, and generating results using multiple models. Co-LLM supports training with different collaboration pairs and provides baseline training scripts for various models. In inference, it uses 'vllm' services to orchestrate models and generate results through API-like services. The tool is inspired by allenai/open-instruct and aims to improve decoding performance through collaborative learning.
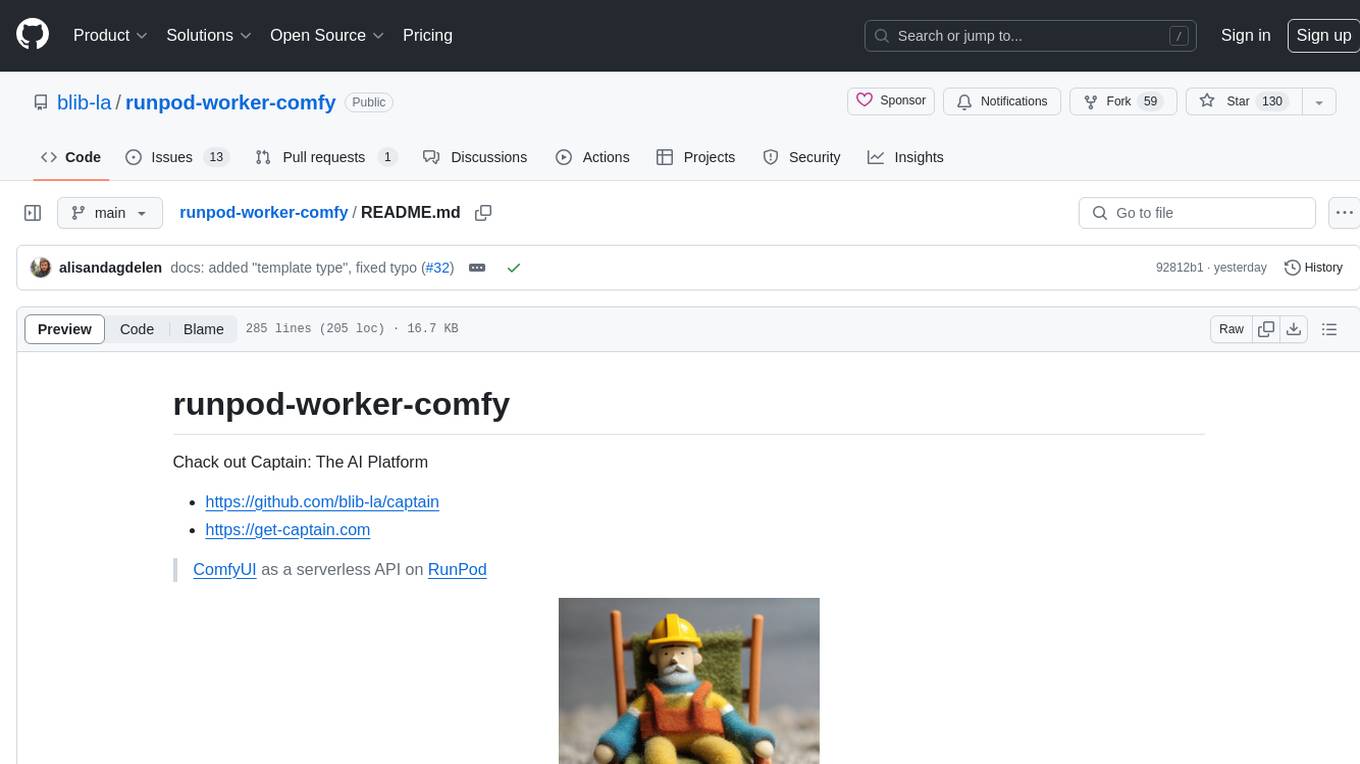
runpod-worker-comfy
runpod-worker-comfy is a serverless API tool that allows users to run any ComfyUI workflow to generate an image. Users can provide input images as base64-encoded strings, and the generated image can be returned as a base64-encoded string or uploaded to AWS S3. The tool is built on Ubuntu + NVIDIA CUDA and provides features like built-in checkpoints and VAE models. Users can configure environment variables to upload images to AWS S3 and interact with the RunPod API to generate images. The tool also supports local testing and deployment to Docker hub using Github Actions.
For similar tasks
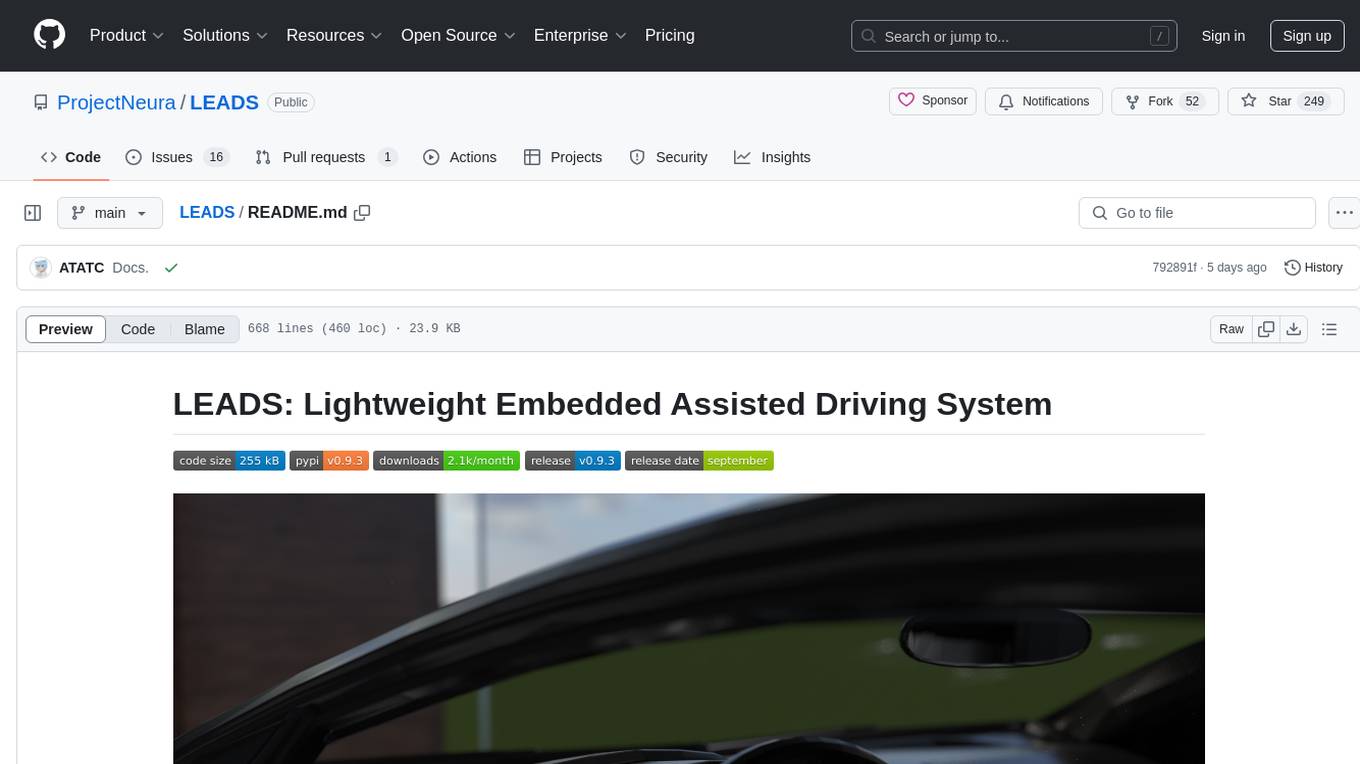
LEADS
LEADS is a lightweight embedded assisted driving system designed to simplify the development of instrumentation, control, and analysis systems for racing cars. It is written in Python and C/C++ with impressive performance. The system is customizable and provides abstract layers for component rearrangement. It supports hardware components like Raspberry Pi and Arduino, and can adapt to various hardware types. LEADS offers a modular structure with a focus on flexibility and lightweight design. It includes robust safety features, modern GUI design with dark mode support, high performance on different platforms, and powerful ESC systems for traction control and braking. The system also supports real-time data sharing, live video streaming, and AI-enhanced data analysis for driver training. LEADS VeC Remote Analyst enables transparency between the driver and pit crew, allowing real-time data sharing and analysis. The system is designed to be user-friendly, adaptable, and efficient for racing car development.
For similar jobs
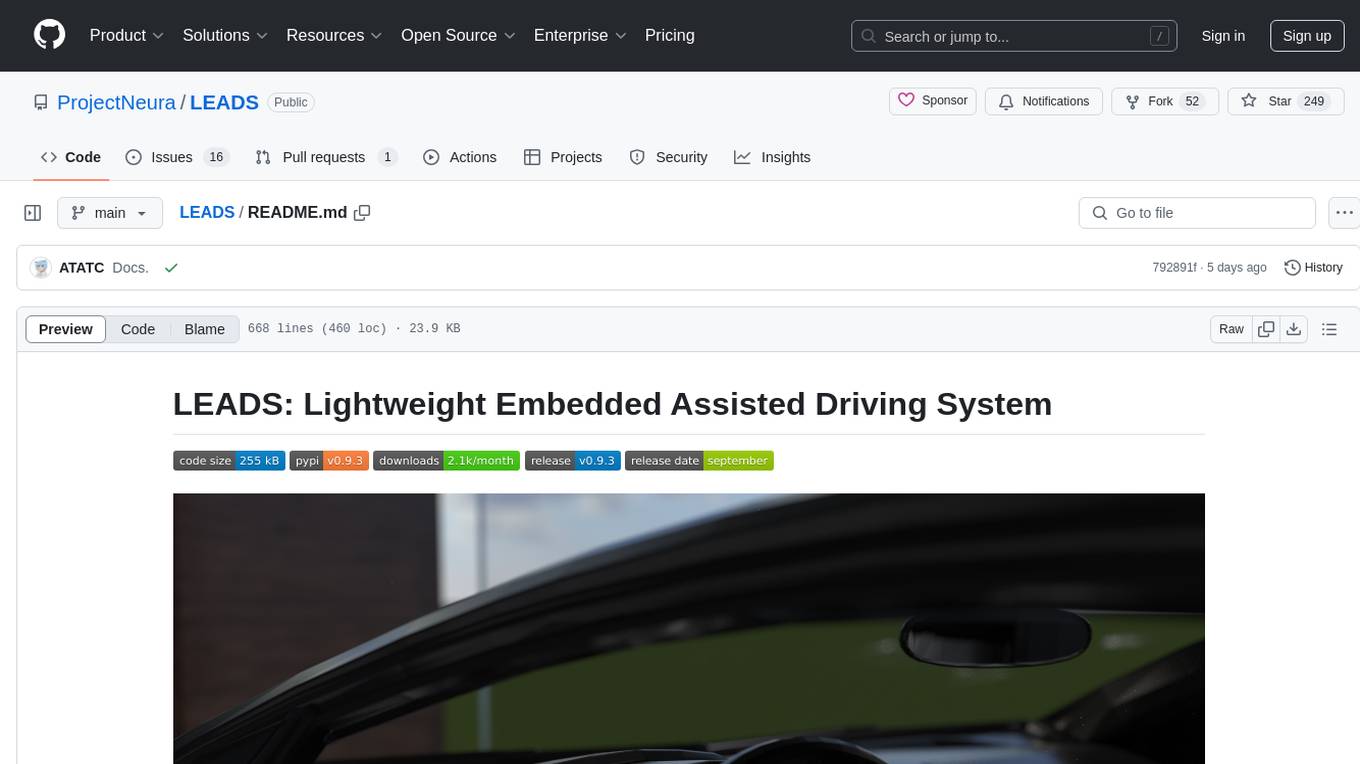
LEADS
LEADS is a lightweight embedded assisted driving system designed to simplify the development of instrumentation, control, and analysis systems for racing cars. It is written in Python and C/C++ with impressive performance. The system is customizable and provides abstract layers for component rearrangement. It supports hardware components like Raspberry Pi and Arduino, and can adapt to various hardware types. LEADS offers a modular structure with a focus on flexibility and lightweight design. It includes robust safety features, modern GUI design with dark mode support, high performance on different platforms, and powerful ESC systems for traction control and braking. The system also supports real-time data sharing, live video streaming, and AI-enhanced data analysis for driver training. LEADS VeC Remote Analyst enables transparency between the driver and pit crew, allowing real-time data sharing and analysis. The system is designed to be user-friendly, adaptable, and efficient for racing car development.
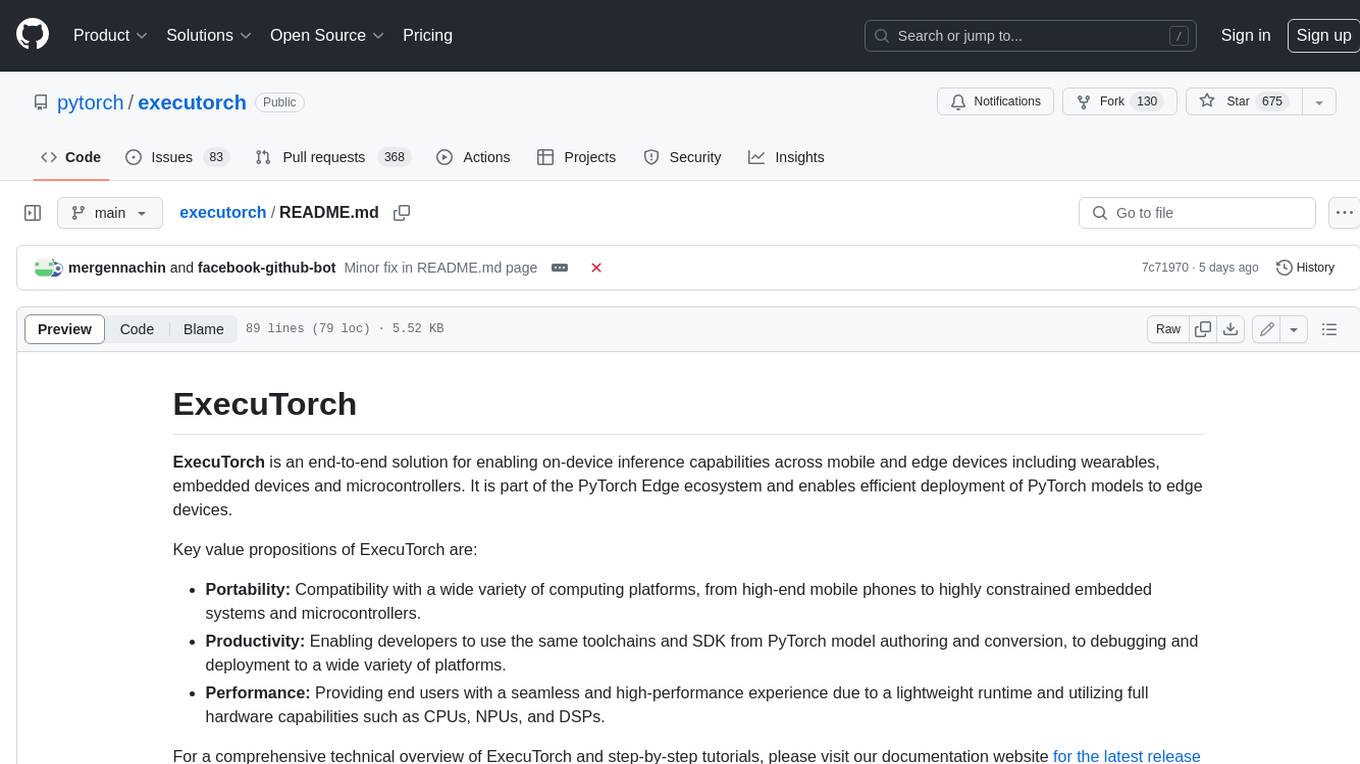
executorch
ExecuTorch is an end-to-end solution for enabling on-device inference capabilities across mobile and edge devices including wearables, embedded devices and microcontrollers. It is part of the PyTorch Edge ecosystem and enables efficient deployment of PyTorch models to edge devices. Key value propositions of ExecuTorch are: * **Portability:** Compatibility with a wide variety of computing platforms, from high-end mobile phones to highly constrained embedded systems and microcontrollers. * **Productivity:** Enabling developers to use the same toolchains and SDK from PyTorch model authoring and conversion, to debugging and deployment to a wide variety of platforms. * **Performance:** Providing end users with a seamless and high-performance experience due to a lightweight runtime and utilizing full hardware capabilities such as CPUs, NPUs, and DSPs.
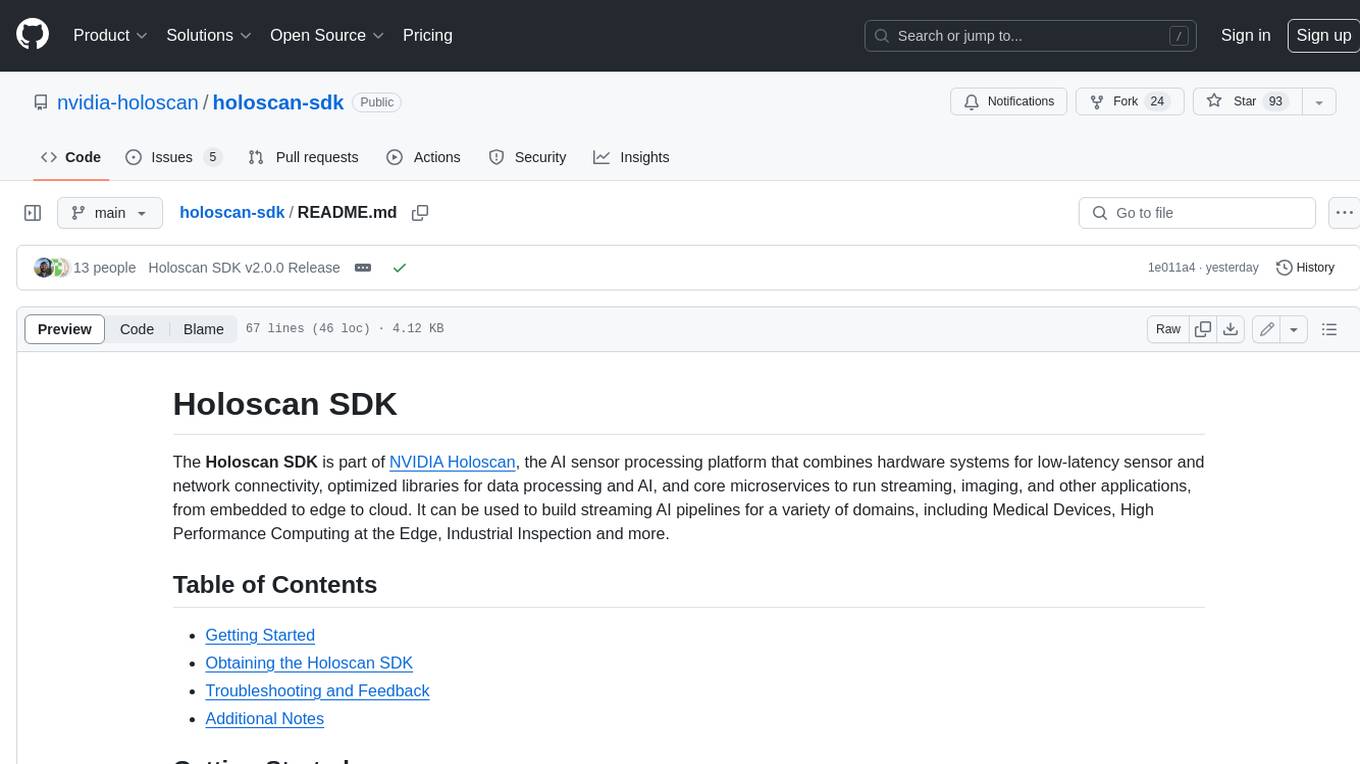
holoscan-sdk
The Holoscan SDK is part of NVIDIA Holoscan, the AI sensor processing platform that combines hardware systems for low-latency sensor and network connectivity, optimized libraries for data processing and AI, and core microservices to run streaming, imaging, and other applications, from embedded to edge to cloud. It can be used to build streaming AI pipelines for a variety of domains, including Medical Devices, High Performance Computing at the Edge, Industrial Inspection and more.
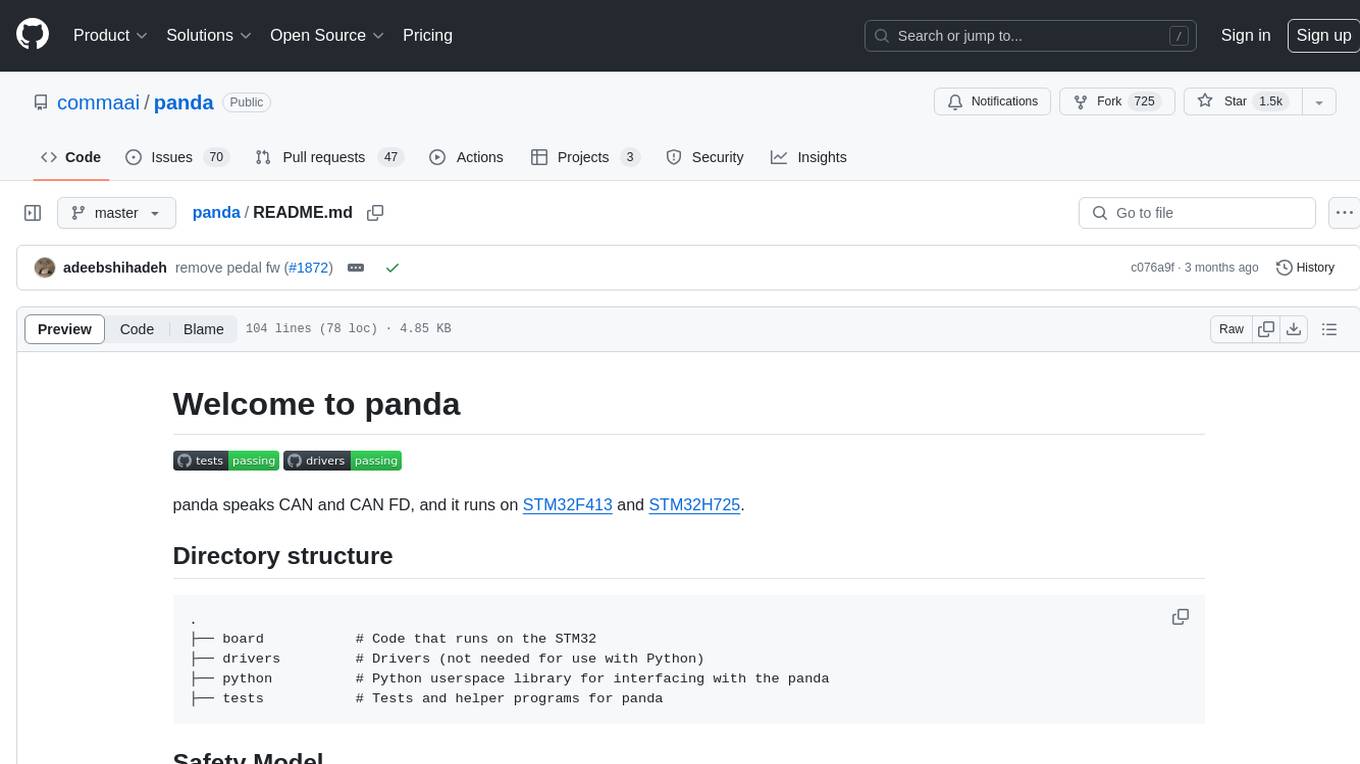
panda
Panda is a car interface tool that speaks CAN and CAN FD, running on STM32F413 and STM32H725. It provides safety modes and controls_allowed feature for message handling. The tool ensures code rigor through CI regression tests, including static code analysis, MISRA C:2012 violations check, unit tests, and hardware-in-the-loop tests. The software interface supports Python library, C++ library, and socketcan in kernel. Panda is licensed under the MIT license.
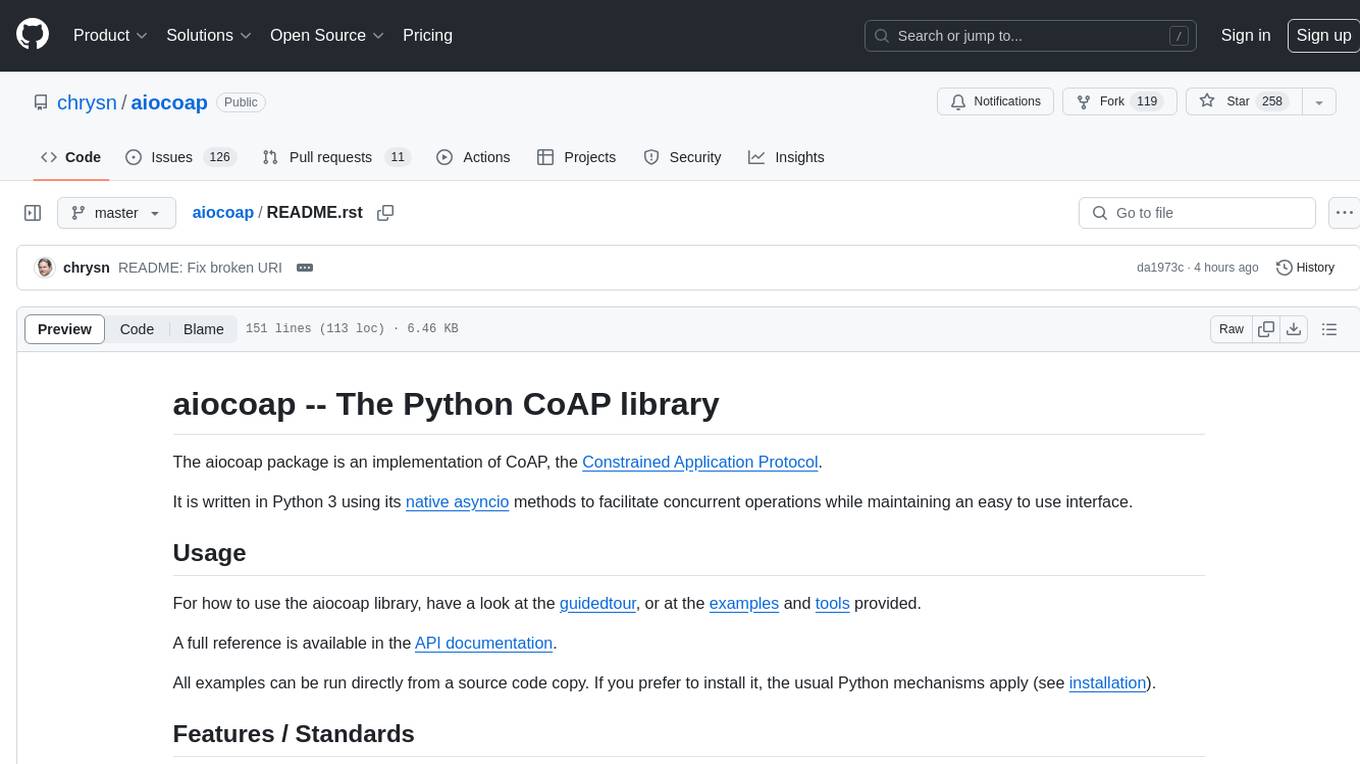
aiocoap
aiocoap is a Python library that implements the Constrained Application Protocol (CoAP) using native asyncio methods in Python 3. It supports various CoAP standards such as RFC7252, RFC7641, RFC7959, RFC8323, RFC7967, RFC8132, RFC9176, RFC8613, and draft-ietf-core-oscore-groupcomm-17. The library provides features for clients and servers, including multicast support, blockwise transfer, CoAP over TCP, TLS, and WebSockets, No-Response, PATCH/FETCH, OSCORE, and Group OSCORE. It offers an easy-to-use interface for concurrent operations and is suitable for IoT applications.
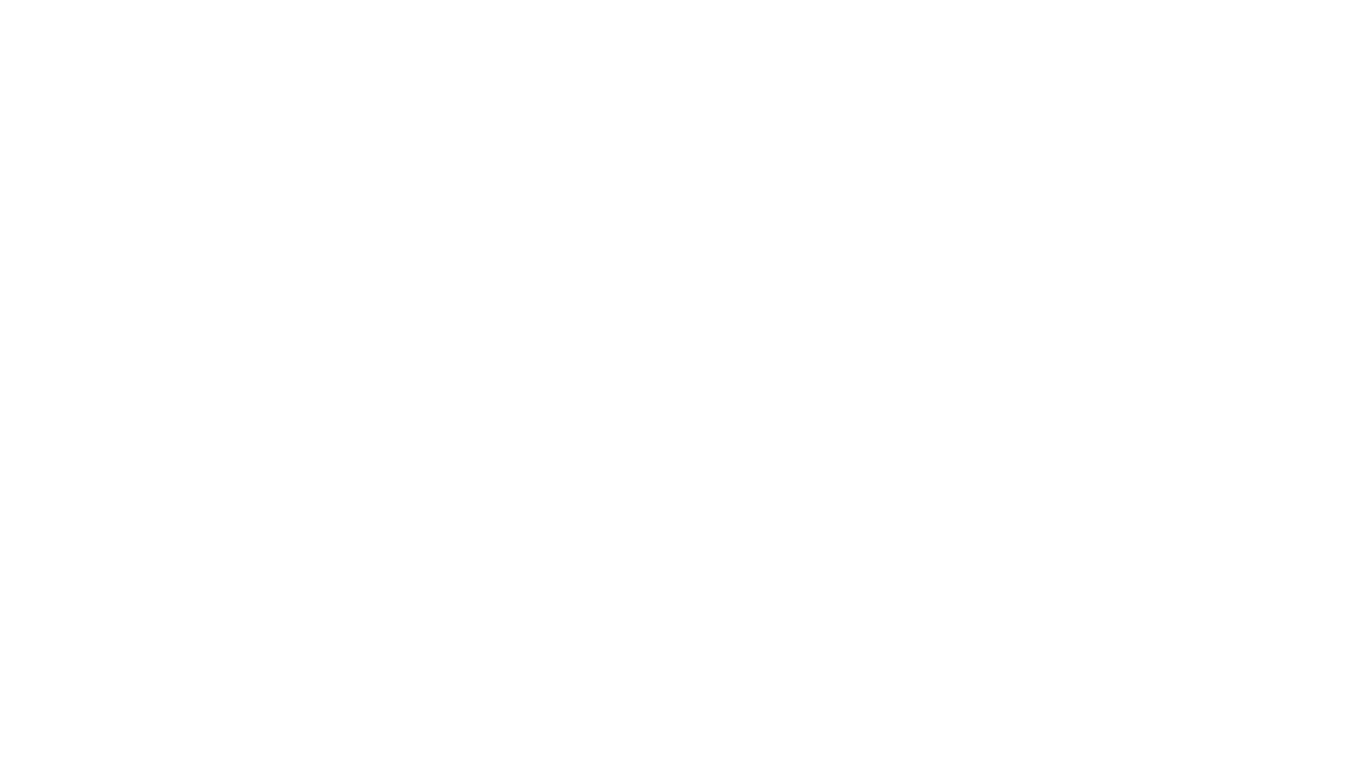
CPP-Notes
CPP-Notes is a comprehensive repository providing detailed insights into the history, evolution, and modern development of the C++ programming language. It covers the foundational concepts of C++ and its transition from C, highlighting key features such as object-oriented programming, generic programming, and modern enhancements introduced in C++11/14/17. The repository delves into the significance of C++ in system programming, library development, and its role as a versatile and efficient language. It explores the historical milestones of C++ development, from its inception in 1979 by Bjarne Stroustrup to the latest C++20 standard, showcasing major advancements like Concepts, Ranges library, Coroutines, Modules, and enhanced concurrency features.
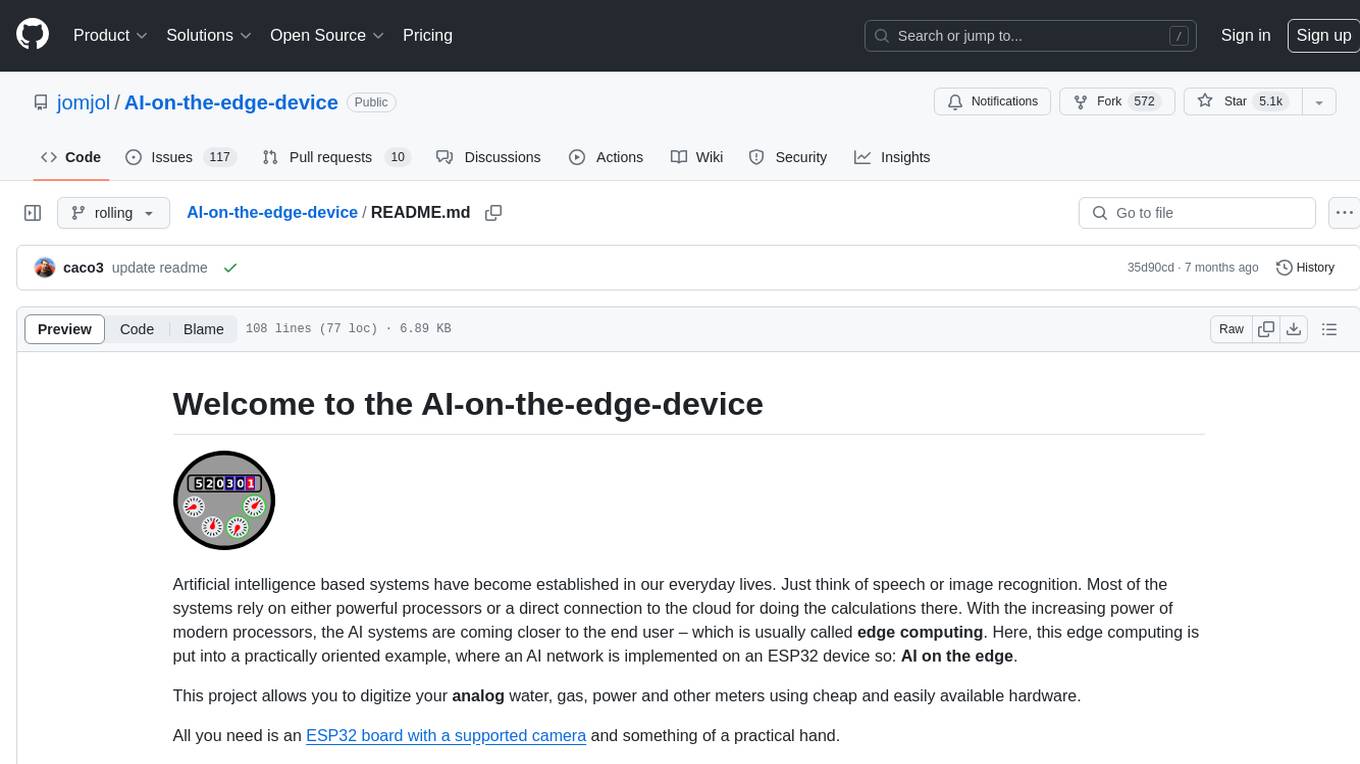
AI-on-the-edge-device
AI-on-the-edge-device is a project that enables users to digitize analog water, gas, power, and other meters using an ESP32 board with a supported camera. It integrates Tensorflow Lite for AI processing, offers a small and affordable device with integrated camera and illumination, provides a web interface for administration and control, supports Homeassistant, Influx DB, MQTT, and REST API. The device captures meter images, extracts Regions of Interest (ROIs), runs them through AI for digitization, and allows users to send data to MQTT, InfluxDb, or access it via REST API. The project also includes 3D-printable housing options and tools for logfile management.
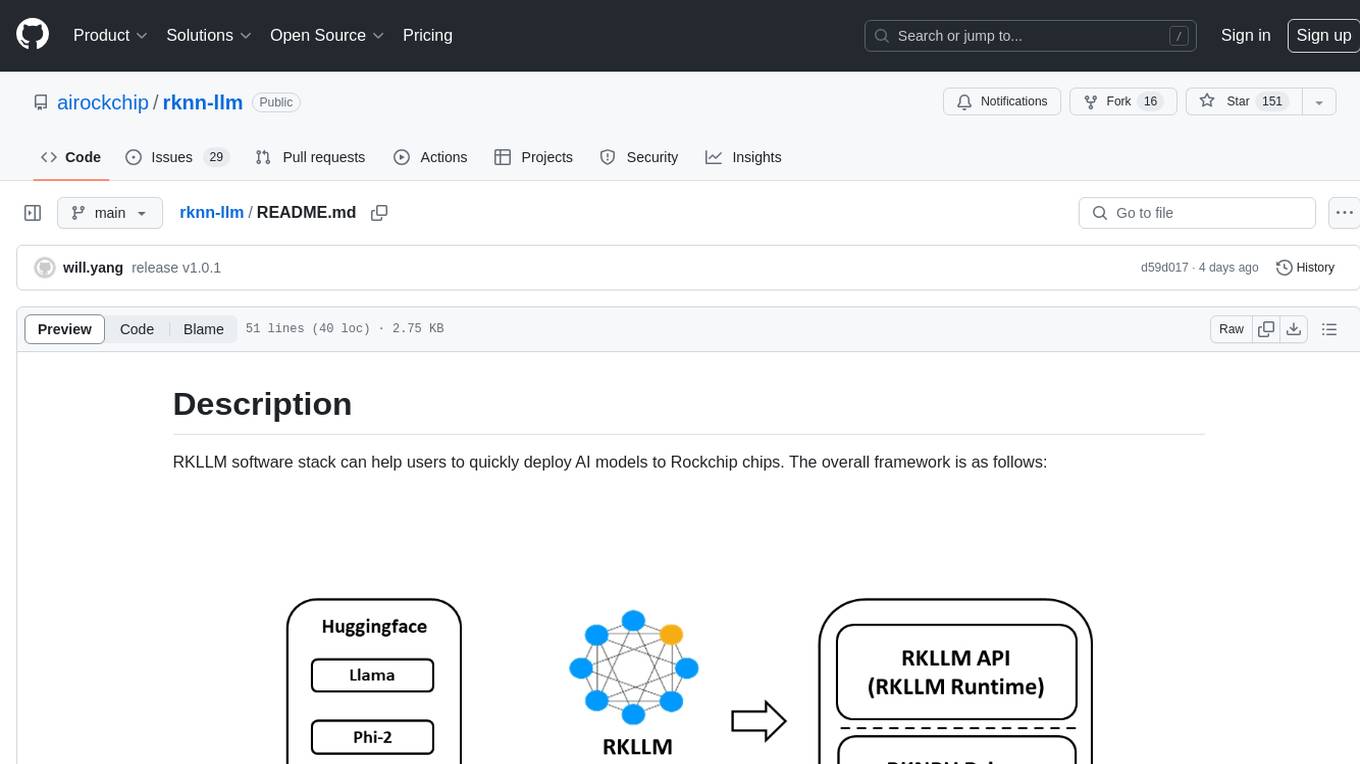
rknn-llm
RKLLM software stack is a toolkit designed to help users quickly deploy AI models to Rockchip chips. It consists of RKLLM-Toolkit for model conversion and quantization, RKLLM Runtime for deploying models on Rockchip NPU platform, and RKNPU kernel driver for hardware interaction. The toolkit supports RK3588 and RK3576 series chips and various models like TinyLLAMA, Qwen, Phi, ChatGLM3, Gemma, InternLM2, and MiniCPM. Users can download packages, docker images, examples, and docs from RKLLM_SDK. Additionally, RKNN-Toolkit2 SDK is available for deploying additional AI models.