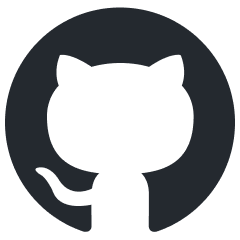
LLM_Web_search
An extension for oobabooga/text-generation-webui that enables the LLM to search the web using DuckDuckGo
Stars: 232
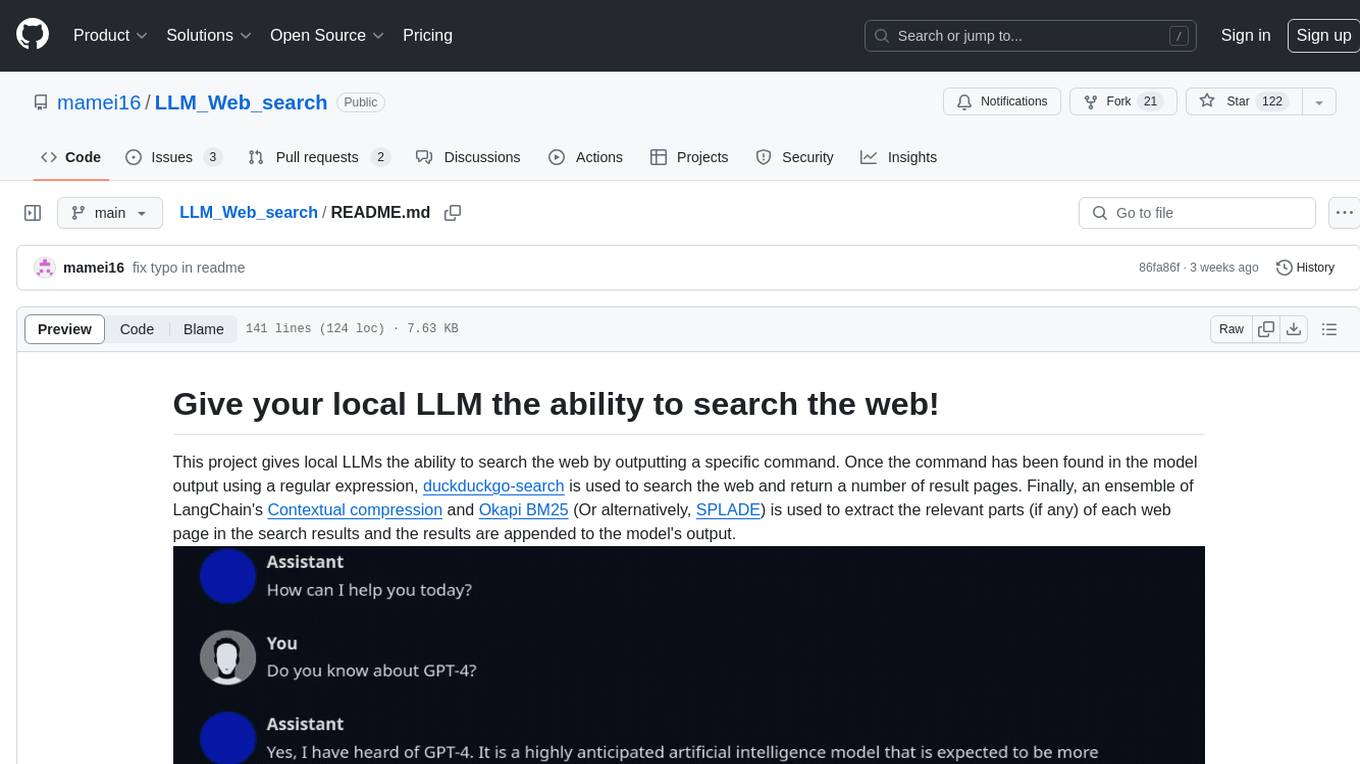
LLM_Web_search project gives local LLMs the ability to search the web by outputting a specific command. It uses regular expressions to extract search queries from model output and then utilizes duckduckgo-search to search the web. LangChain's Contextual compression and Okapi BM25 or SPLADE are used to extract relevant parts of web pages in search results. The extracted results are appended to the model's output.
README:
This project gives local LLMs the ability to search the web by outputting a specific
command. Once the command has been found in the model output using a regular expression,
duckduckgo-search
is used to search the web and return a number of result pages. Finally, an
ensemble of a dense embedding model and
Okapi BM25 (Or alternatively, SPLADE)
is used to extract the relevant parts (if any) of each web page in the search results
and the results are appended to the model's output.
- Go to the "Session" tab of the web UI and use "Install or update an extension" to download the latest code for this extension.
- To install the extension's depencies you have two options:
-
The easy way: Run the appropriate
update_wizard
script inside the text-generation-webui folder and chooseInstall/update extensions requirements
. This installs everything usingpip
, which means using the unofficialfaiss-cpu
package. Therefore, it is not guaranteed to work with your system (see the official disclaimer). -
The safe way: Manually update the conda environment in which you installed the dependencies of
oobabooga's text-generation-webui.
Open the subfolder
text-generation-webui/extensions/LLM_Web_search
in a terminal or conda shell. If you used the one-click install method, run the commandconda env update -p <path_to_your_environment> --file environment.yml
, where you need to replace<path_to_your_environment>
with the path to the/installer_files/env
subfolder within the text-generation-webui folder. Otherwise, if you made your own environment, useconda env update -n <name_of_your_environment> --file environment.yml
(NB: Solving the environment can take a while)
-
The easy way: Run the appropriate
- Launch the Web UI by running the appropriate
start
script and enable the extension under the session tab.
Alternatively, you can also start the server directly using the following command (assuming you have activated your conda/virtual environment):
python server.py --extension LLM_Web_search
If the installation was successful and the extension was loaded, a new tab with the title "LLM Web Search" should be visible in the web UI.
See https://github.com/oobabooga/text-generation-webui/wiki/07-%E2%80%90-Extensions for more information about extensions.
Search queries are extracted from the model's output using a regular expression. This is made easier by prompting the model
to use a fixed search command (see system_prompts/
for example prompts).
An example workflow of using this extension could be:
- Load a model
- Head over to the "LLM Web search" tab
- Load a custom system message/prompt
- Ensure that the query part of the command mentioned in the system message can be matched using the current "Search command regex string" (see "Using a custom regular expression" below)
- Pick a generation parameter preset that works well for you. You can read more about generation parameters here
- Choose "chat-instruct" or "instruct" mode and start chatting
The default regular expression is:
Search_web\("(.*)"\)
Where Search_web
is the search command and everything between the quotation marks
inside the parentheses will be used as the search query. Every custom regular expression must use a
capture group to extract the search
query. I recommend https://www.debuggex.com/ to try out custom regular expressions. If a regex
fulfills the requirement above, the search query should be matched by "Group 1" in Debuggex.
Here is an example of a more flexible, but more complex, regex that works for several different models:
[Ss]earch_web\((?:["'])(.*)(?:["'])\)
Experimental support exists for extracting the full text content from a webpage. The default regex to use this functionality is:
Open_url\("(.*)"\)
Note: The full content of a web page is likely to exceed the maximum context length of your average local LLM.
This is the default web search backend.
To use a local or remote SearXNG instance instead of DuckDuckGo, simply paste the URL into the
"SearXNG URL" text field of the "LLM Web Search" settings tab (be sure to include http://
or https://
). The instance must support
returning results in JSON format.
To modify the categories, engines, languages etc. that should be used for a specific query, it must follow the SearXNG search syntax. Currently, automatic redirect and Special Queries are not supported.
Quickly finds answers using just the highlighted snippets from websites returned by the search engine. If you simply want results fast, choose this search type.
Note: Some advanced options in the UI will be hidden when simple search is enabled, as they have no effect in this case.
Note2: The snippets returned by SearXNG are often much more useful than those returned by DuckDuckGo, so consider using SearXNG as the search backend if you use simple search.
Scans entire websites in the results for a more comprehensive search. Ideally, this search type should be able to find "needle in the haystack" information hidden somewhere in the website text. Hence, choose this option if you want to trade a more resource intensive search process for generally more relevant search results.
For the best possible search results, also enable semantic chunking and use SPLADE as the keyword retriever.
This extension comes out of the box with Okapi BM25 enabled, which is widely used and very popuplar for keyword based document retrieval. It runs on the CPU and, for the purpose of this extension, it is fast.
If you don't run the extension in "CPU only" mode and have some VRAM to spare, you can also select SPLADE in the "Advanced settings" section as an alternative. It has been shown to outperform BM25 in multiple benchmarks and uses a technique called "query expansion" to add additional contextually relevant words to the original query. However, it is slower than BM25. You can read more about it here.
To improve performance, documents are embedded in batches and in parallel. Increasing the "SPLADE batch size" parameter setting improves performance up to a certain point, but VRAM usage ramps up quickly with increasing batch size. A batch size of 8 appears to be a good trade-off, but the default value is 2 to avoid running out of memory on smaller GPUs.
Naively partitions a website's text into fixed sized chunks without any regard for the text content. This is the default, since it is fast and requires no GPU.
Tries to partition a website's text into chunks based on semantics. If two consecutive sentences have very different embeddings (based on the cosine distance between their embeddings), a new chunk will be started. How different two consecutive sentences have to be for them to end up in different chunks can be tuned using the sentence split threshold
parameter in the UI.
For natural language, this method generally produces much better results than character-based chunking. However, it is noticable slower, even when using the GPU.
If you (like me) have ≤ 12 GB VRAM, I recommend using one of:
For Tasks:
Click tags to check more tools for each tasksFor Jobs:
Alternative AI tools for LLM_Web_search
Similar Open Source Tools
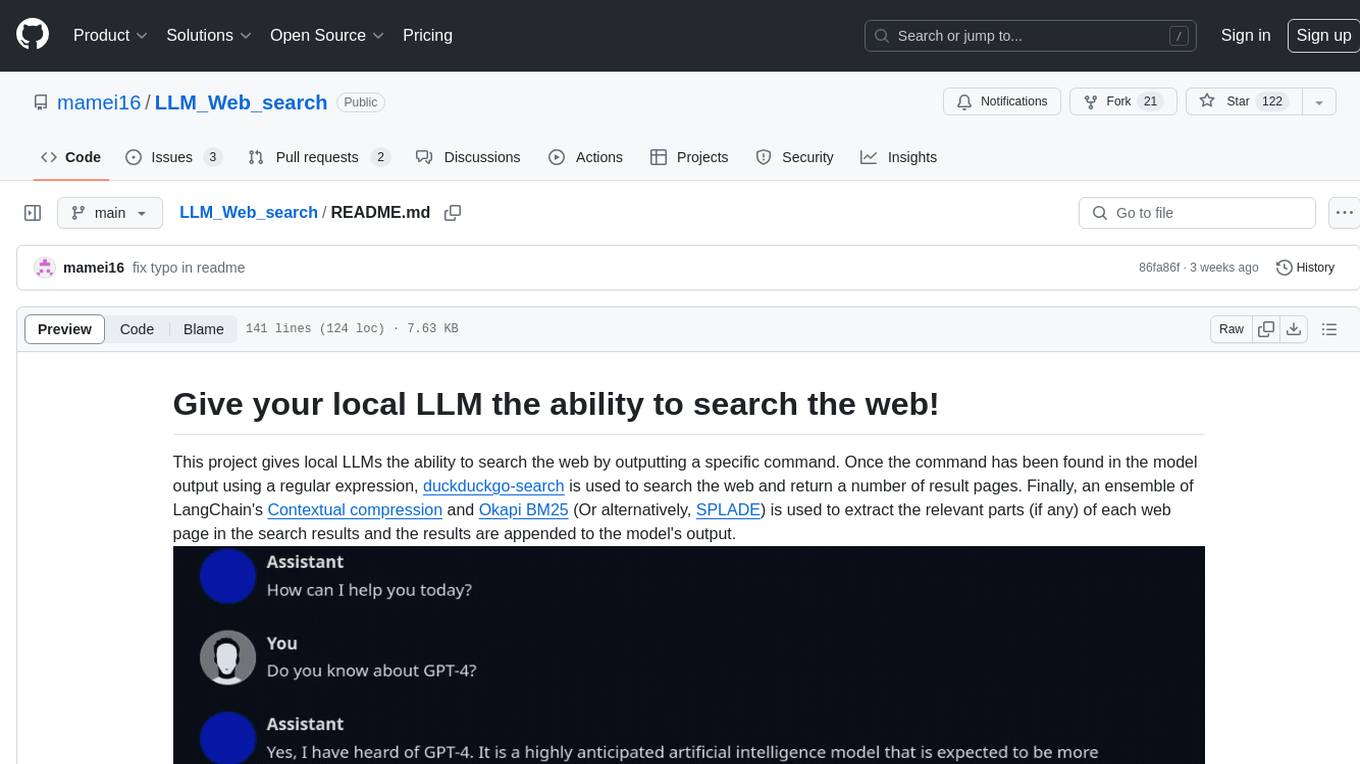
LLM_Web_search
LLM_Web_search project gives local LLMs the ability to search the web by outputting a specific command. It uses regular expressions to extract search queries from model output and then utilizes duckduckgo-search to search the web. LangChain's Contextual compression and Okapi BM25 or SPLADE are used to extract relevant parts of web pages in search results. The extracted results are appended to the model's output.
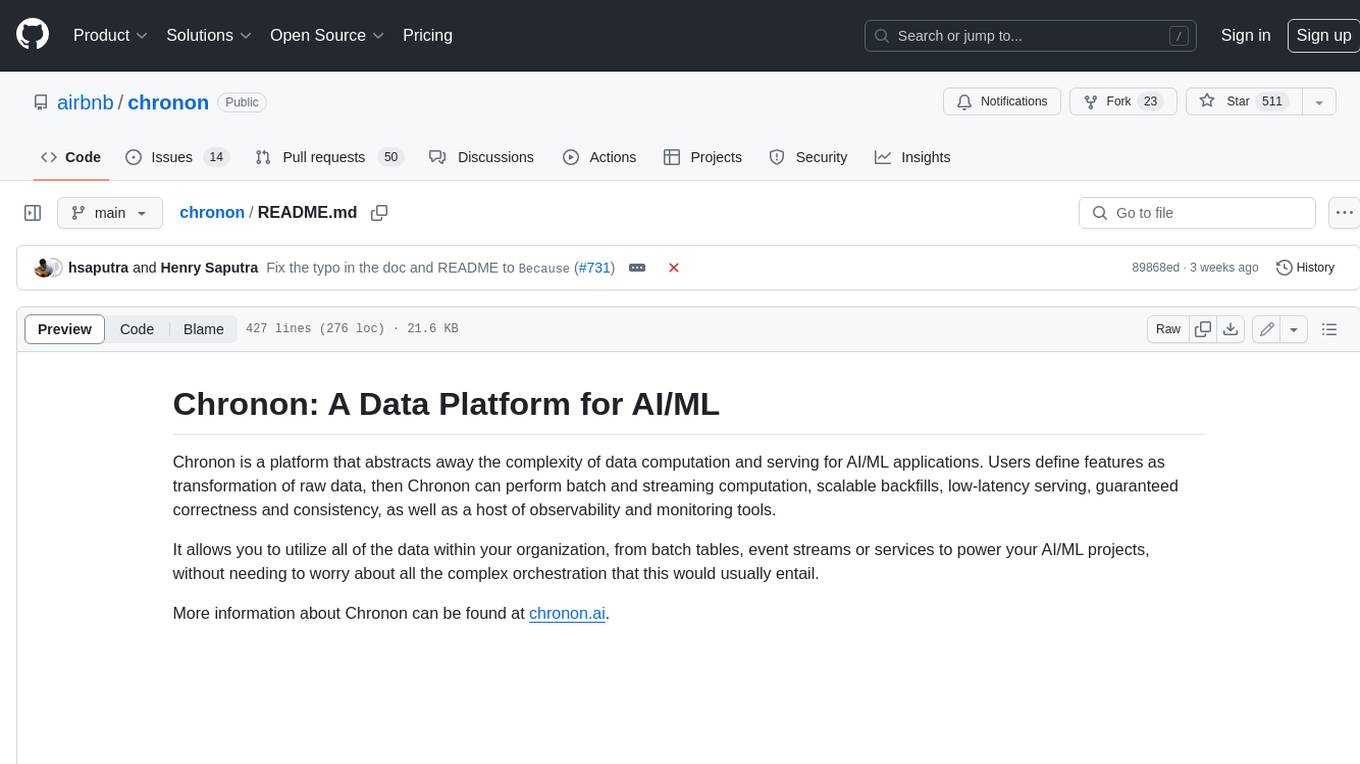
chronon
Chronon is a platform that simplifies and improves ML workflows by providing a central place to define features, ensuring point-in-time correctness for backfills, simplifying orchestration for batch and streaming pipelines, offering easy endpoints for feature fetching, and guaranteeing and measuring consistency. It offers benefits over other approaches by enabling the use of a broad set of data for training, handling large aggregations and other computationally intensive transformations, and abstracting away the infrastructure complexity of data plumbing.
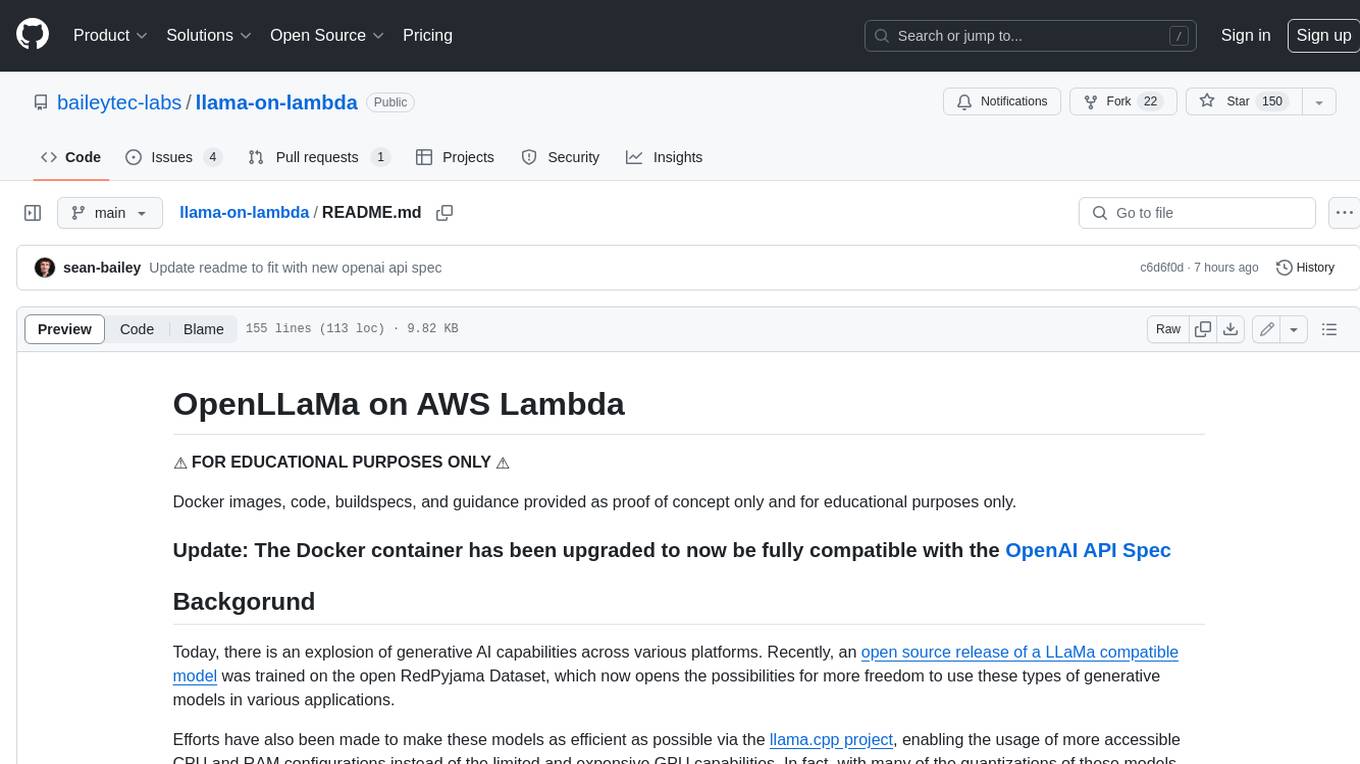
llama-on-lambda
This project provides a proof of concept for deploying a scalable, serverless LLM Generative AI inference engine on AWS Lambda. It leverages the llama.cpp project to enable the usage of more accessible CPU and RAM configurations instead of limited and expensive GPU capabilities. By deploying a container with the llama.cpp converted models onto AWS Lambda, this project offers the advantages of scale, minimizing cost, and maximizing compute availability. The project includes AWS CDK code to create and deploy a Lambda function leveraging your model of choice, with a FastAPI frontend accessible from a Lambda URL. It is important to note that you will need ggml quantized versions of your model and model sizes under 6GB, as your inference RAM requirements cannot exceed 9GB or your Lambda function will fail.
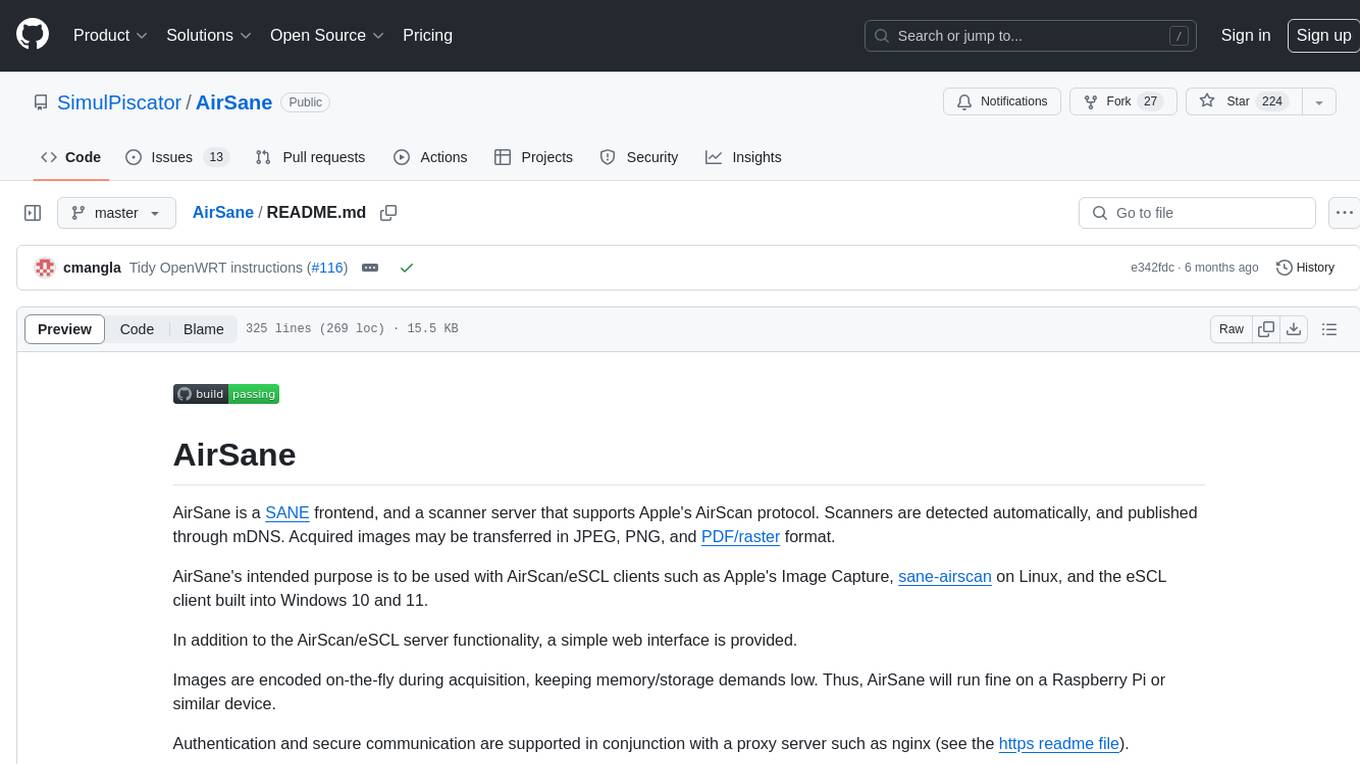
AirSane
AirSane is a SANE frontend and scanner server that supports Apple's AirScan protocol. It automatically detects scanners and publishes them through mDNS. Acquired images can be transferred in JPEG, PNG, and PDF/raster format. The tool is intended to be used with AirScan/eSCL clients such as Apple's Image Capture, sane-airscan on Linux, and the eSCL client built into Windows 10 and 11. It provides a simple web interface and encodes images on-the-fly to keep memory/storage demands low, making it suitable for devices like Raspberry Pi. Authentication and secure communication are supported in conjunction with a proxy server like nginx. AirSane has been reverse-engineered from Apple's AirScanScanner client communication protocol and offers a range of installation and configuration options for different operating systems.
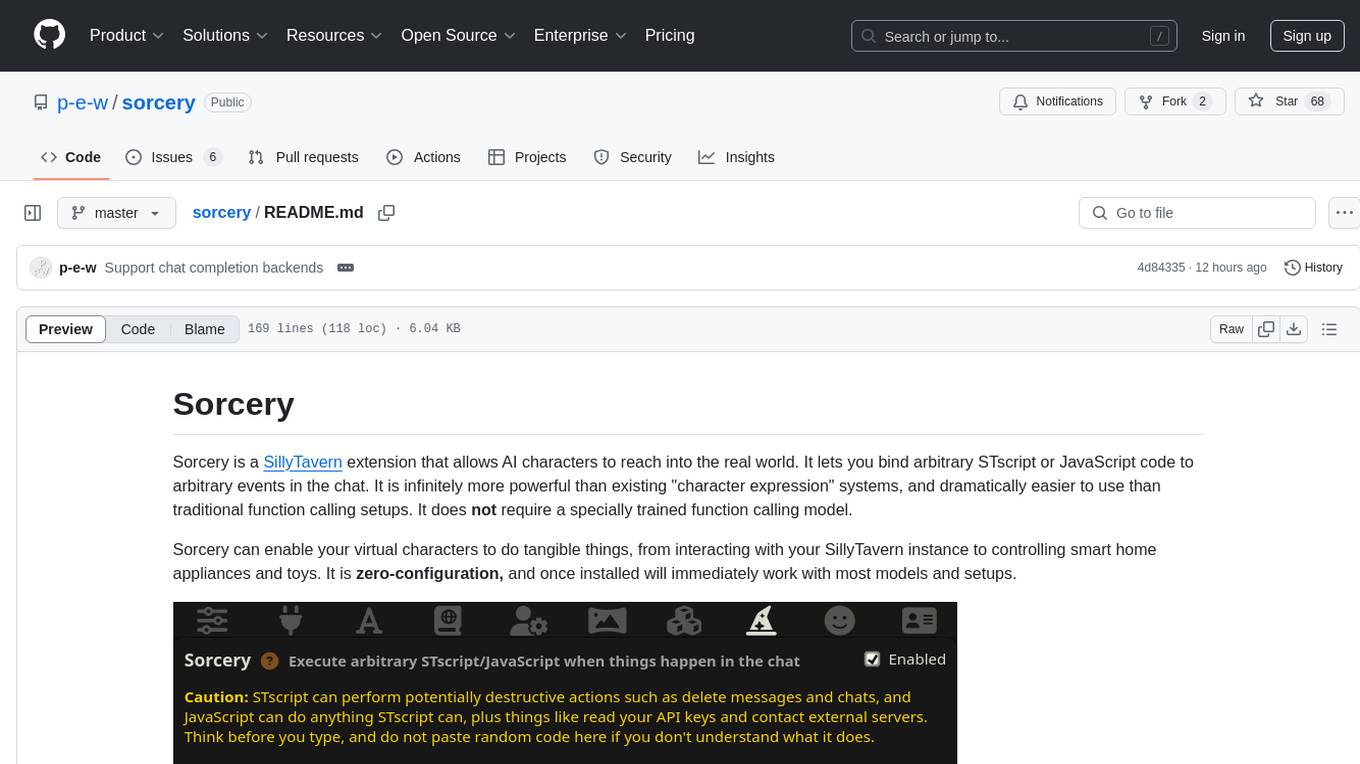
sorcery
Sorcery is a SillyTavern extension that allows AI characters to interact with the real world by executing user-defined scripts at specific events in the chat. It is easy to use and does not require a specially trained function calling model. Sorcery can be used to control smart home appliances, interact with virtual characters, and perform various tasks in the chat environment. It works by injecting instructions into the system prompt and intercepting markers to run associated scripts, providing a seamless user experience.
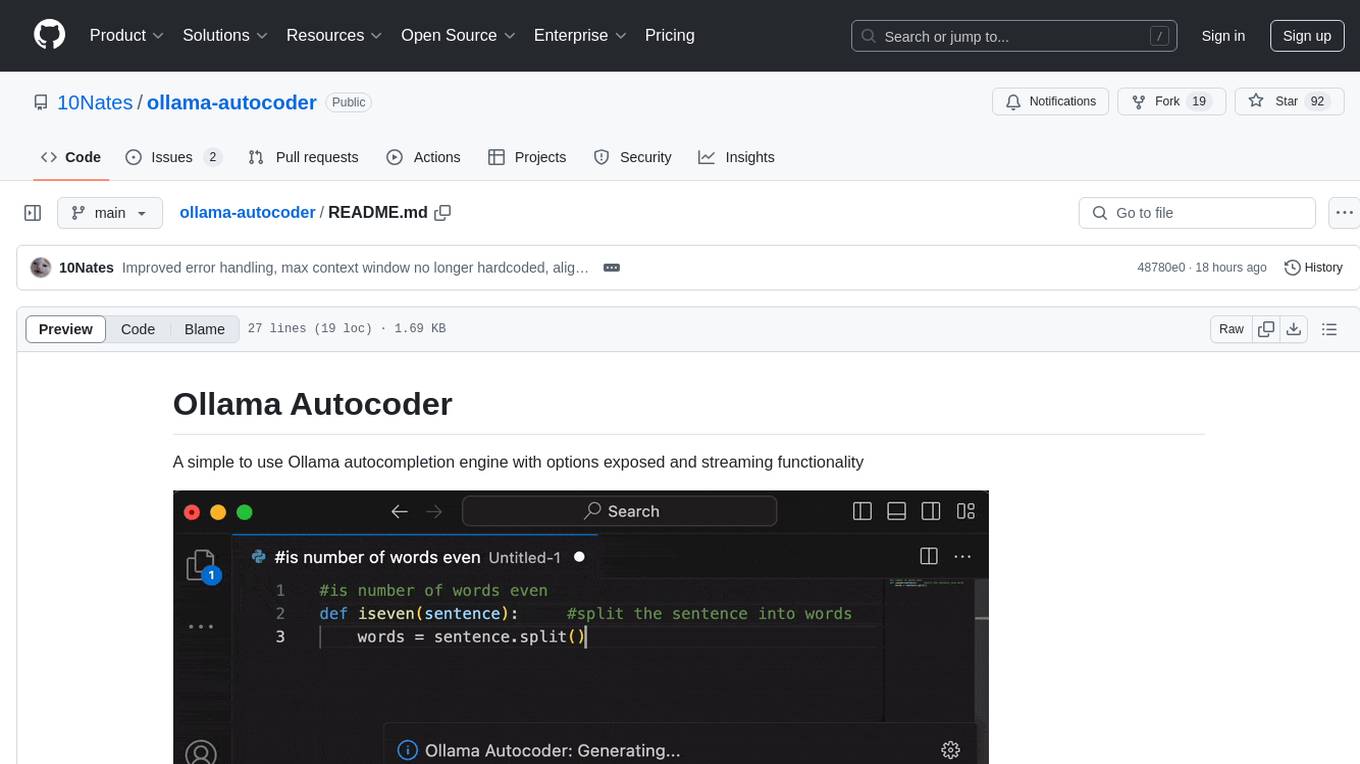
ollama-autocoder
Ollama Autocoder is a simple to use autocompletion engine that integrates with Ollama AI. It provides options for streaming functionality and requires specific settings for optimal performance. Users can easily generate text completions by pressing a key or using a command pallete. The tool is designed to work with Ollama API and a specified model, offering real-time generation of text suggestions.
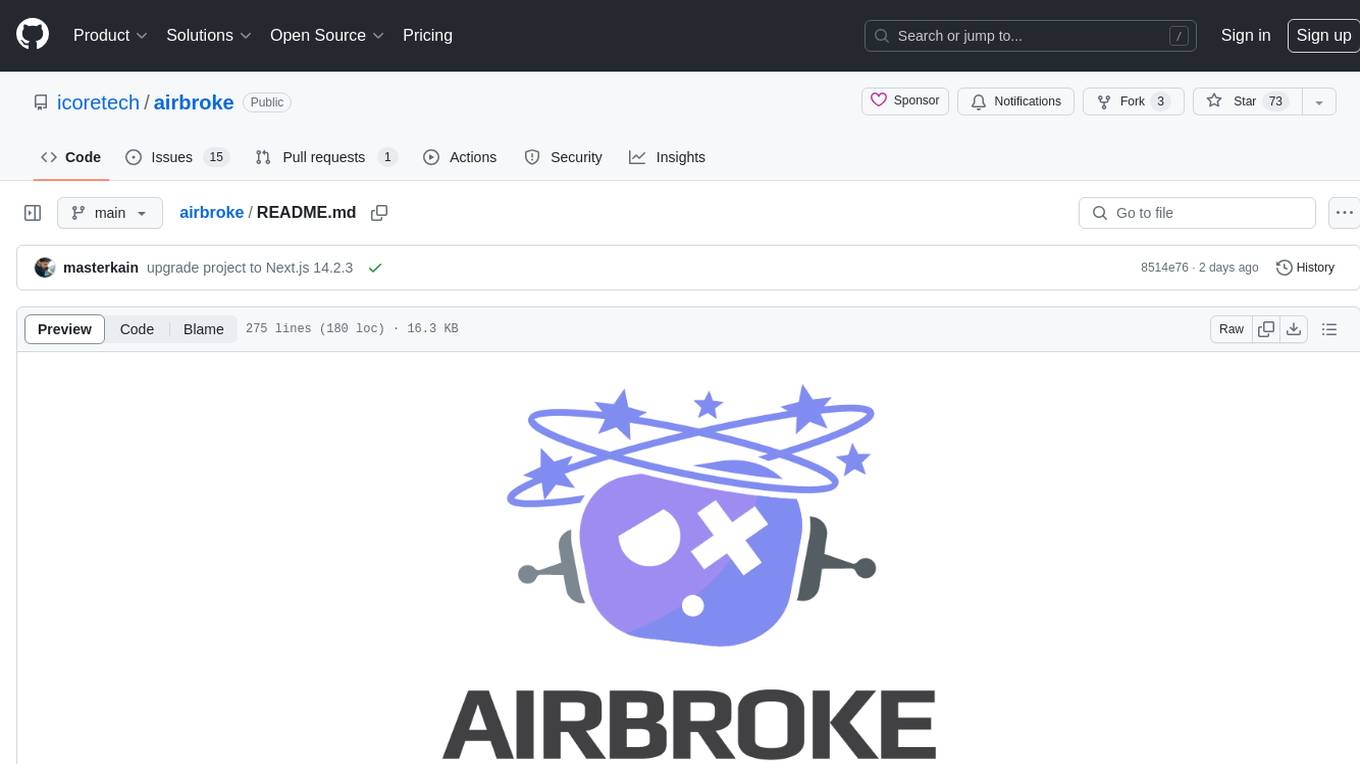
airbroke
Airbroke is an open-source error catcher tool designed for modern web applications. It provides a PostgreSQL-based backend with an Airbrake-compatible HTTP collector endpoint and a React-based frontend for error management. The tool focuses on simplicity, maintaining a small database footprint even under heavy data ingestion. Users can ask AI about issues, replay HTTP exceptions, and save/manage bookmarks for important occurrences. Airbroke supports multiple OAuth providers for secure user authentication and offers occurrence charts for better insights into error occurrences. The tool can be deployed in various ways, including building from source, using Docker images, deploying on Vercel, Render.com, Kubernetes with Helm, or Docker Compose. It requires Node.js, PostgreSQL, and specific system resources for deployment.
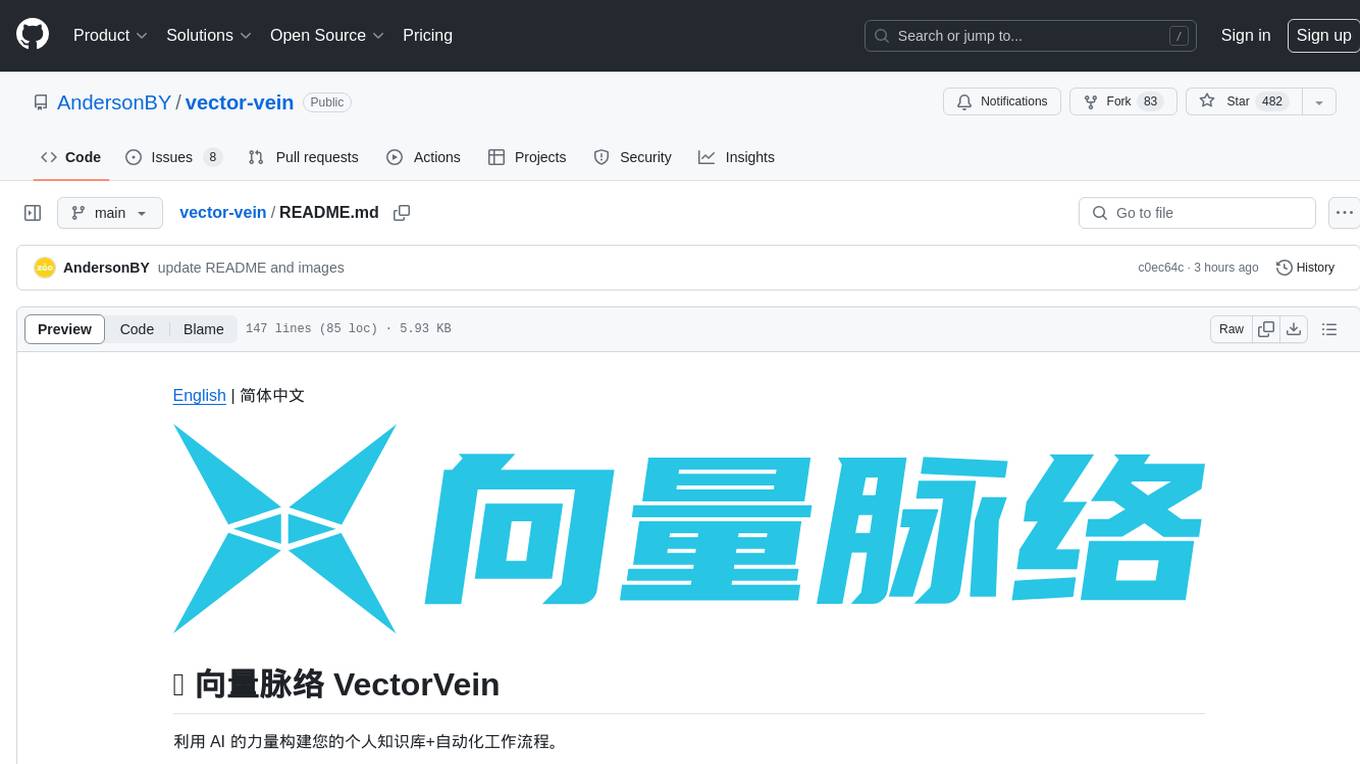
vector-vein
VectorVein is a no-code AI workflow software inspired by LangChain and langflow, aiming to combine the powerful capabilities of large language models and enable users to achieve intelligent and automated daily workflows through simple drag-and-drop actions. Users can create powerful workflows without the need for programming, automating all tasks with ease. The software allows users to define inputs, outputs, and processing methods to create customized workflow processes for various tasks such as translation, mind mapping, summarizing web articles, and automatic categorization of customer reviews.
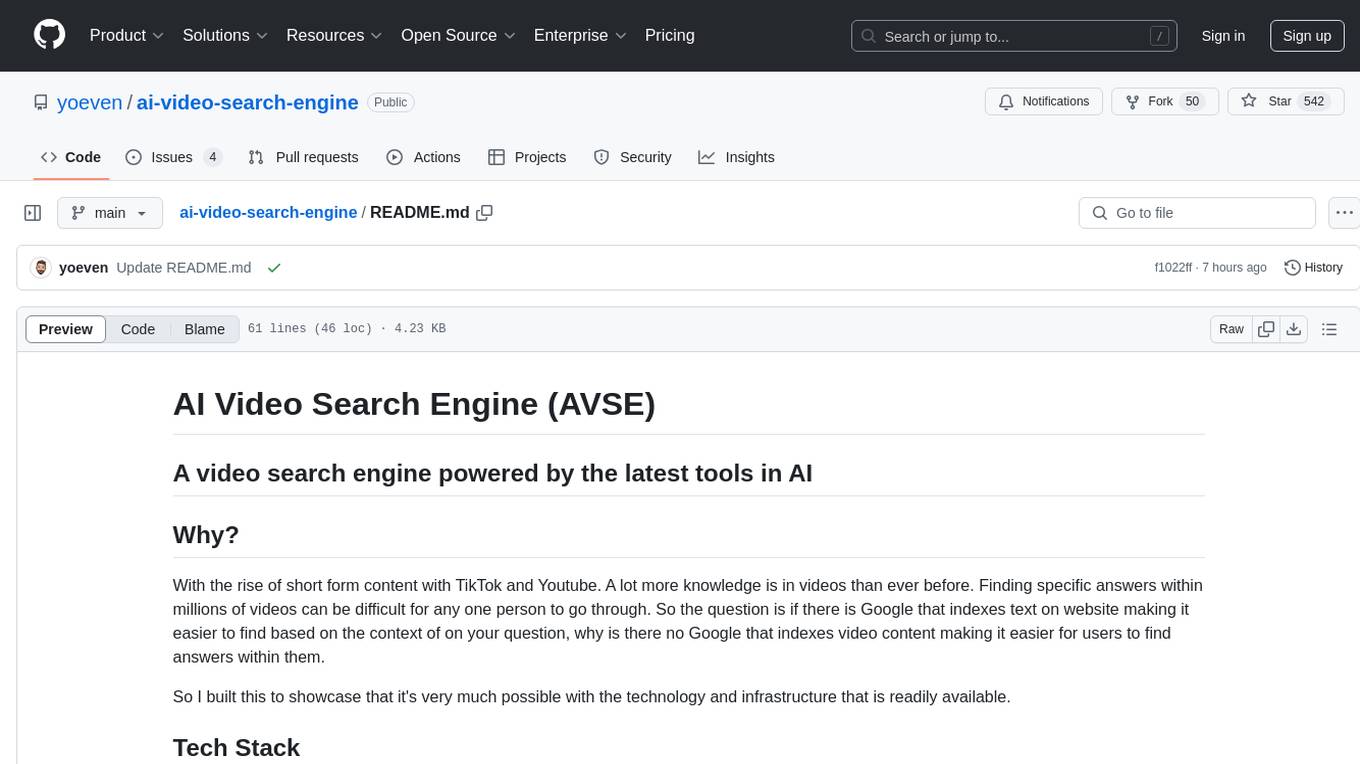
ai-video-search-engine
AI Video Search Engine (AVSE) is a video search engine powered by the latest tools in AI. It allows users to search for specific answers within millions of videos by indexing video content. The tool extracts video transcription, elements like thumbnail and description, and generates vector embeddings using AI models. Users can search for relevant results based on questions, view timestamped transcripts, and get video summaries. AVSE requires a paid Supabase & Fly.io account for hosting and can handle millions of videos with the current setup.
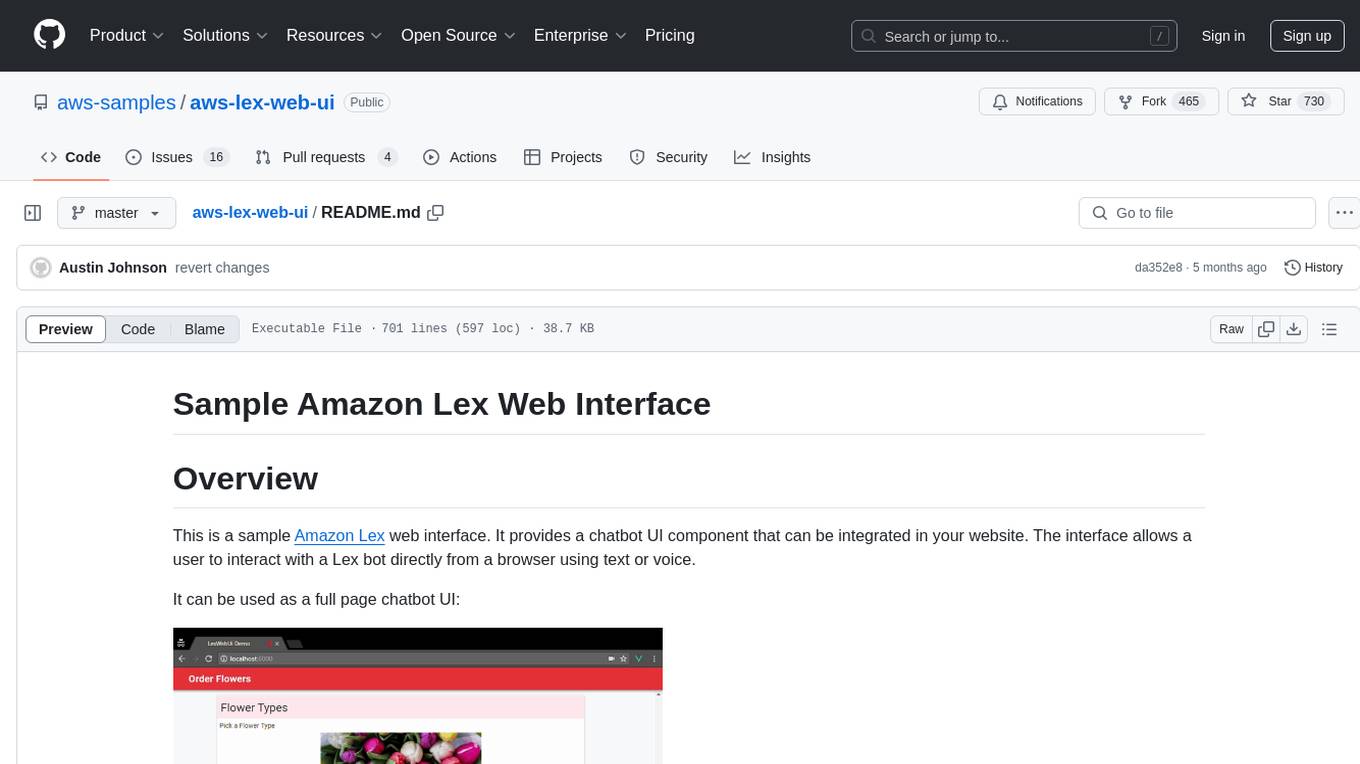
aws-lex-web-ui
The AWS Lex Web UI is a sample Amazon Lex web interface that provides a chatbot UI component for integration into websites. It supports voice and text interactions, Lex response cards, and programmable configuration using JavaScript. The interface can be used as a full-page chatbot UI or embedded as a widget. It offers mobile-ready responsive UI, seamless voice-text switching, and interactive messaging support. The project includes CloudFormation templates for easy deployment and customization. Users can modify configurations, integrate the UI into existing sites, and deploy using various methods like CloudFormation, pre-built libraries, or npm installation.
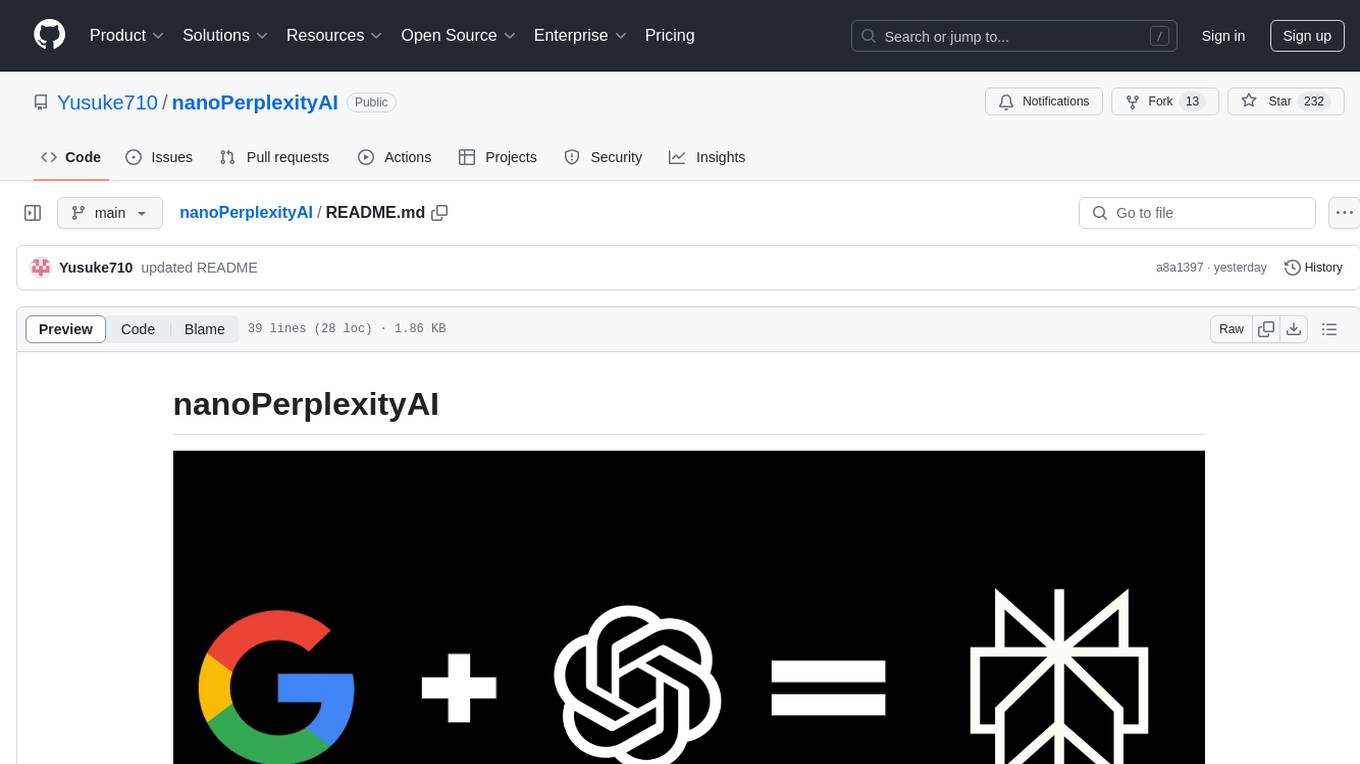
nanoPerplexityAI
nanoPerplexityAI is an open-source implementation of a large language model service that fetches information from Google. It involves a simple architecture where the user query is checked by the language model, reformulated for Google search, and an answer is generated and saved in a markdown file. The tool requires minimal setup and is designed for easy visualization of answers.
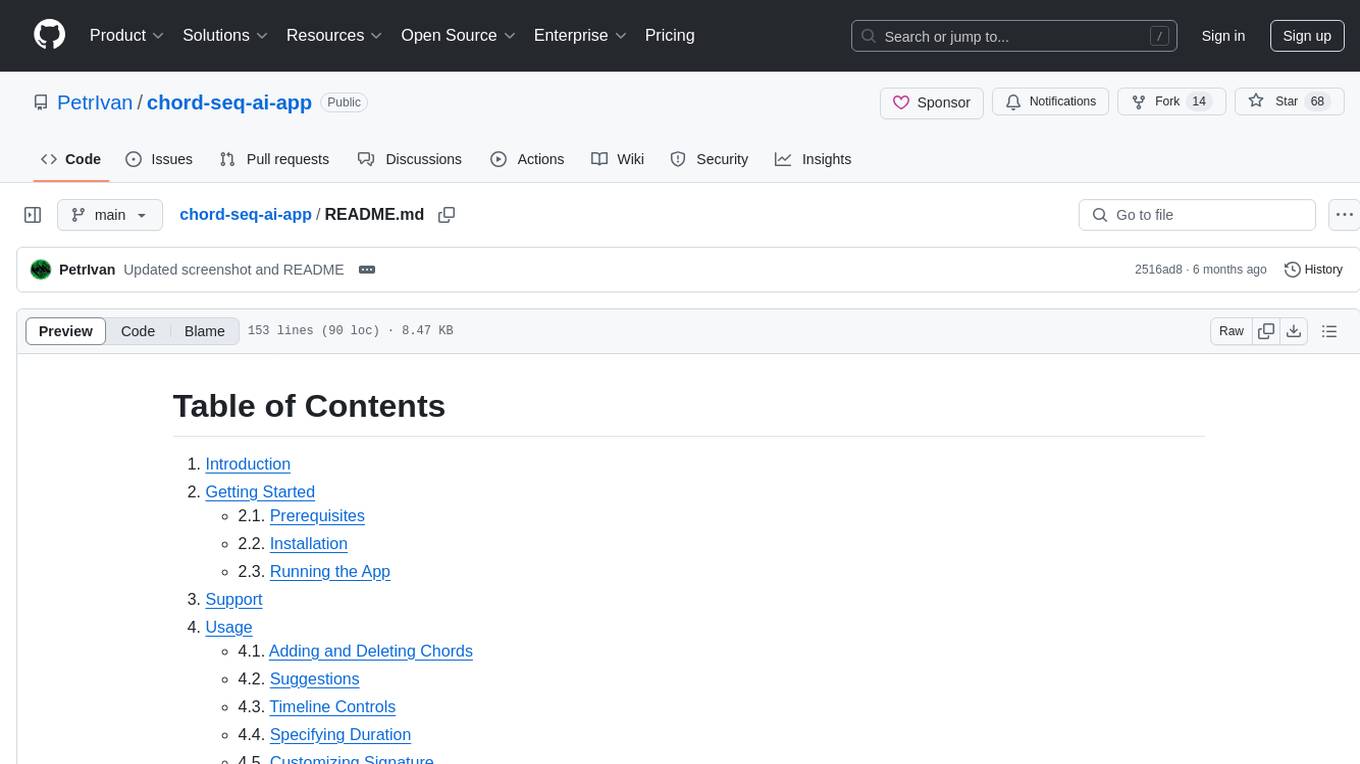
chord-seq-ai-app
ChordSeqAI Web App is a user-friendly interface for composing chord progressions using deep learning models. The app allows users to interact with suggestions, customize signatures, specify durations, select models and styles, transpose, import, export, and utilize chord variants. It supports keyboard shortcuts, automatic local saving, and playback features. The app is designed for desktop use and offers features for both beginners and advanced users in music composition.
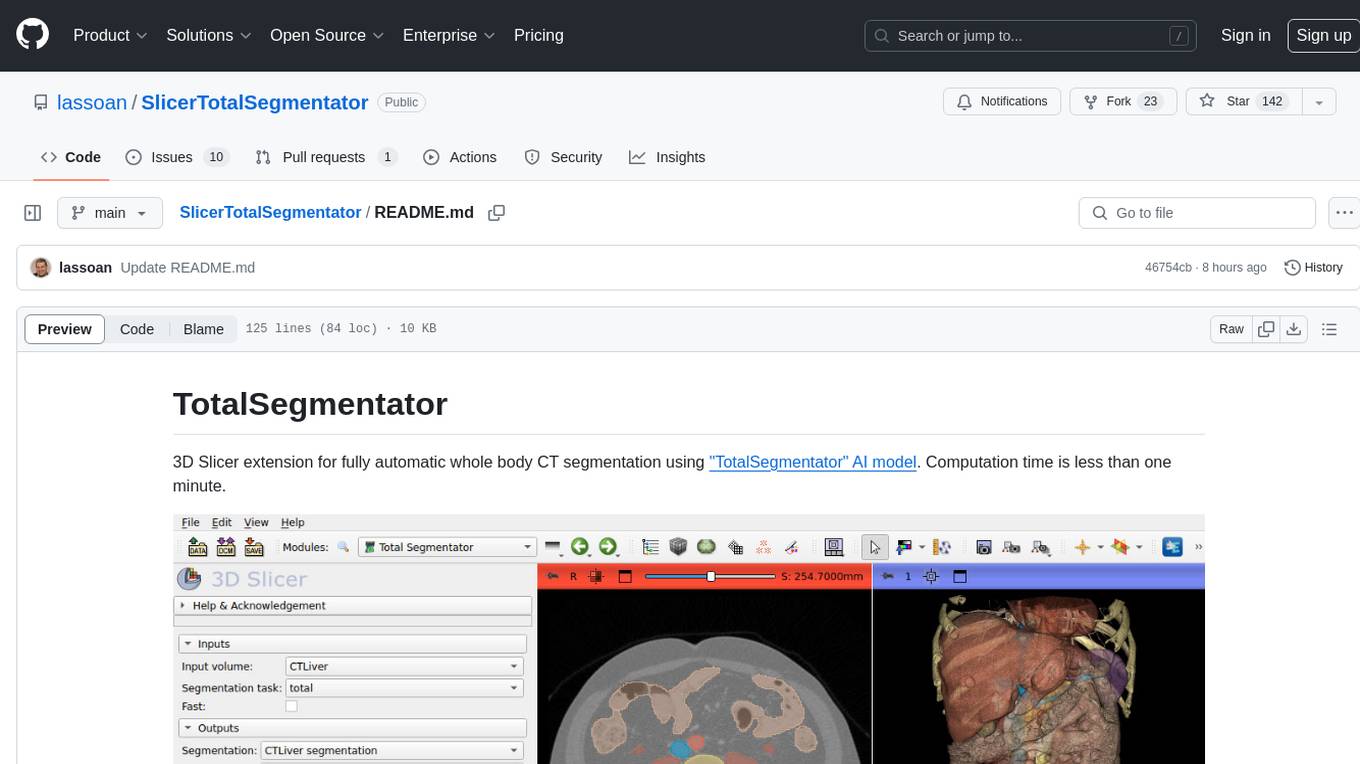
SlicerTotalSegmentator
TotalSegmentator is a 3D Slicer extension designed for fully automatic whole body CT segmentation using the 'TotalSegmentator' AI model. The computation time is less than one minute, making it efficient for research purposes. Users can set up GPU acceleration for faster segmentation. The tool provides a user-friendly interface for loading CT images, creating segmentations, and displaying results in 3D. Troubleshooting steps are available for common issues such as failed computation, GPU errors, and inaccurate segmentations. Contributions to the extension are welcome, following 3D Slicer contribution guidelines.
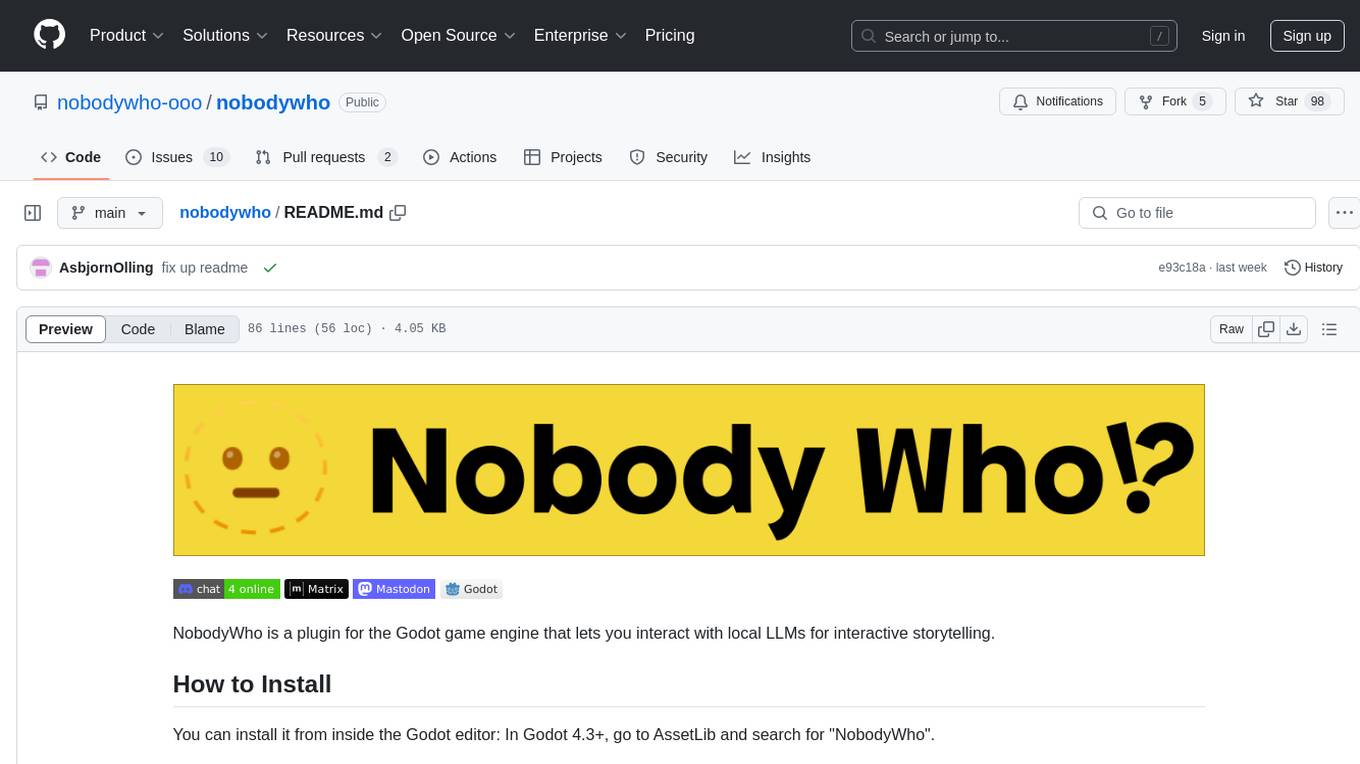
nobodywho
NobodyWho is a plugin for the Godot game engine that enables interaction with local LLMs for interactive storytelling. Users can install it from Godot editor or GitHub releases page, providing their own LLM in GGUF format. The plugin consists of `NobodyWhoModel` node for model file, `NobodyWhoChat` node for chat interaction, and `NobodyWhoEmbedding` node for generating embeddings. It offers a programming interface for sending text to LLM, receiving responses, and starting the LLM worker.
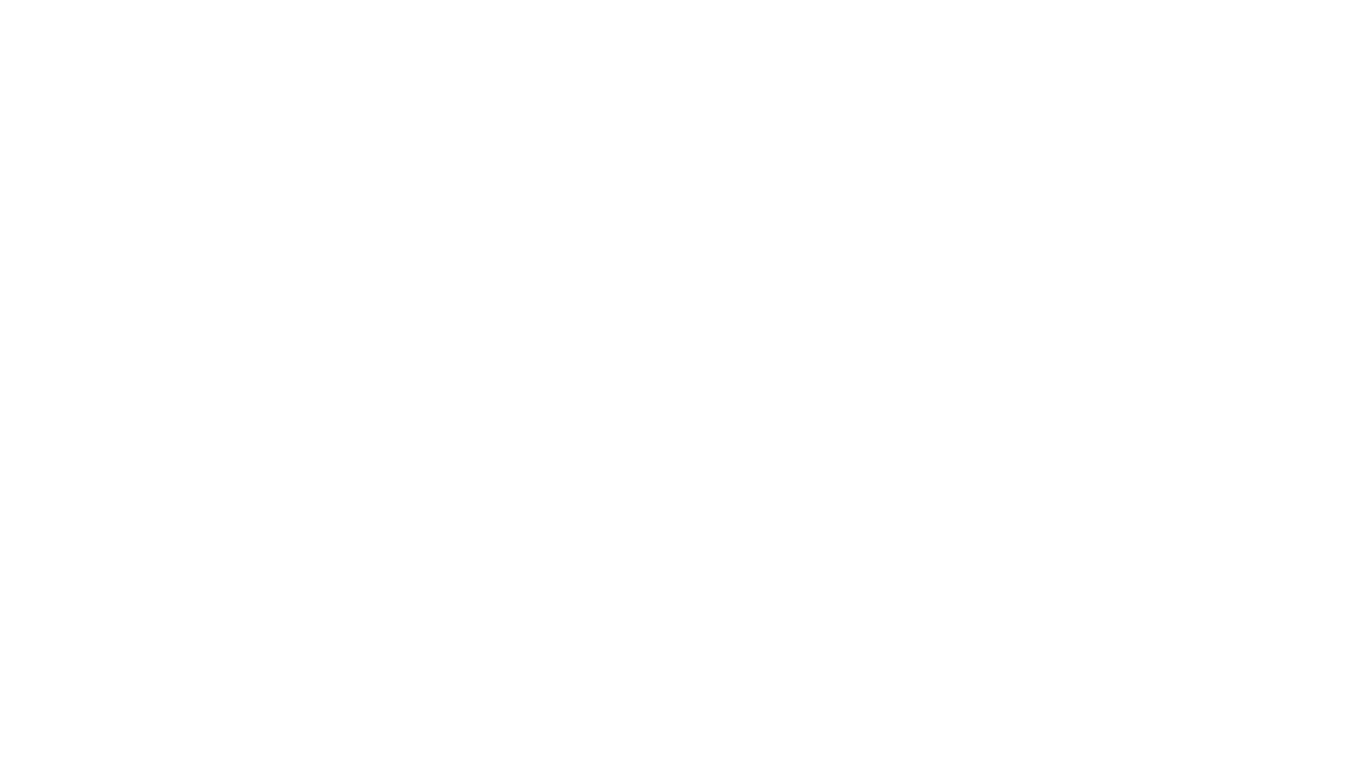
brokk
Brokk is a code assistant designed to understand code semantically, allowing LLMs to work effectively on large codebases. It offers features like agentic search, summarizing related classes, parsing stack traces, adding source for usages, and autonomously fixing errors. Users can interact with Brokk through different panels and commands, enabling them to manipulate context, ask questions, search codebase, run shell commands, and more. Brokk helps with tasks like debugging regressions, exploring codebase, AI-powered refactoring, and working with dependencies. It is particularly useful for making complex, multi-file edits with o1pro.
For similar tasks
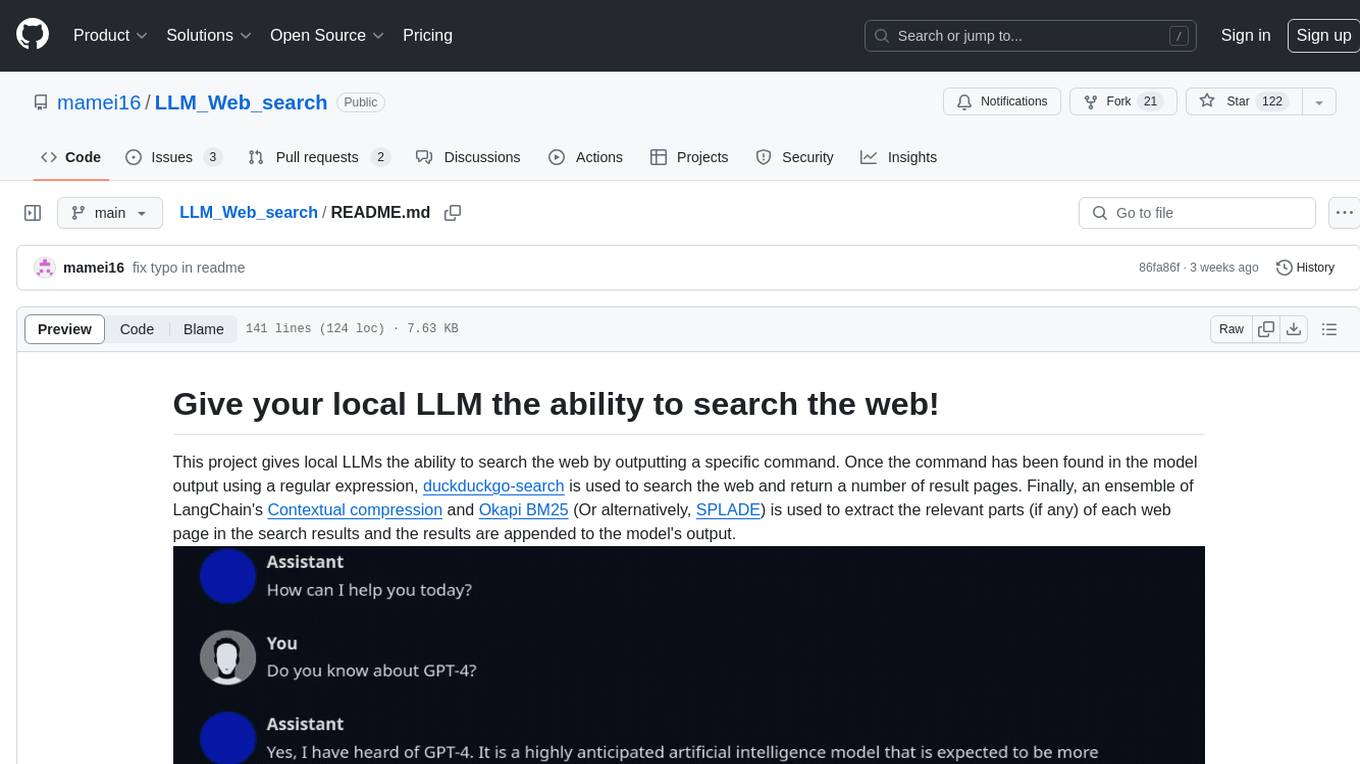
LLM_Web_search
LLM_Web_search project gives local LLMs the ability to search the web by outputting a specific command. It uses regular expressions to extract search queries from model output and then utilizes duckduckgo-search to search the web. LangChain's Contextual compression and Okapi BM25 or SPLADE are used to extract relevant parts of web pages in search results. The extracted results are appended to the model's output.
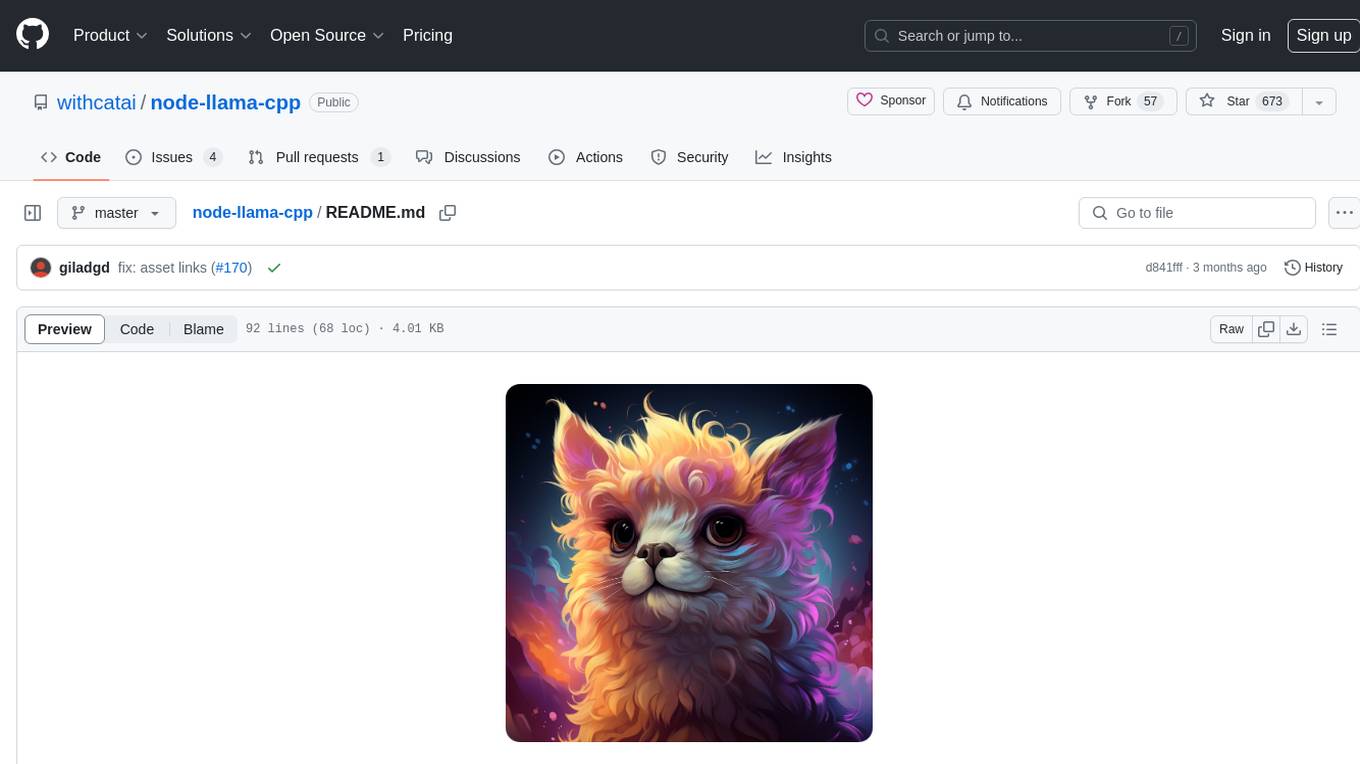
node-llama-cpp
node-llama-cpp is a tool that allows users to run AI models locally on their machines. It provides pre-built bindings with the option to build from source using cmake. Users can interact with text generation models, chat with models using a chat wrapper, and force models to generate output in a parseable format like JSON. The tool supports Metal and CUDA, offers CLI functionality for chatting with models without coding, and ensures up-to-date compatibility with the latest version of llama.cpp. Installation includes pre-built binaries for macOS, Linux, and Windows, with the option to build from source if binaries are not available for the platform.
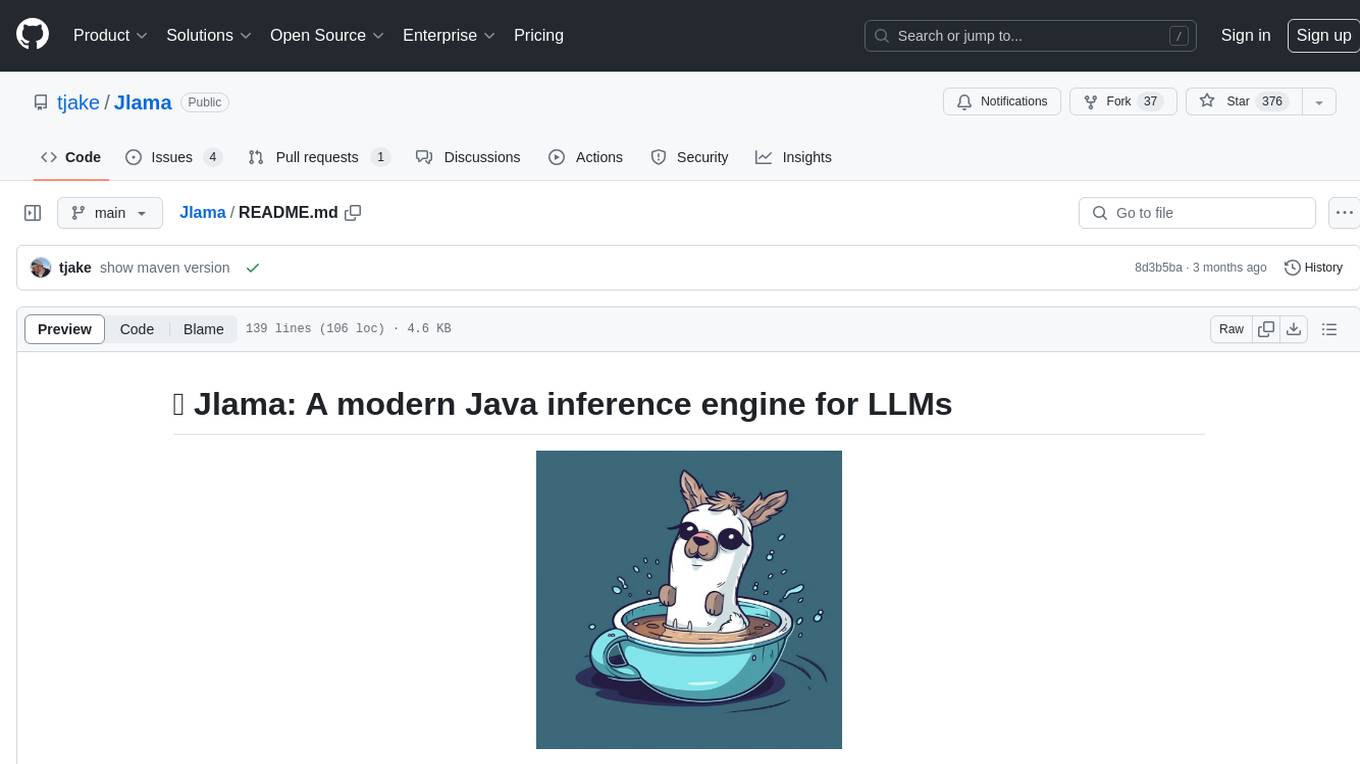
Jlama
Jlama is a modern Java inference engine designed for large language models. It supports various model types such as Gemma, Llama, Mistral, GPT-2, BERT, and more. The tool implements features like Flash Attention, Mixture of Experts, and supports different model quantization formats. Built with Java 21 and utilizing the new Vector API for faster inference, Jlama allows users to add LLM inference directly to their Java applications. The tool includes a CLI for running models, a simple UI for chatting with LLMs, and examples for different model types.
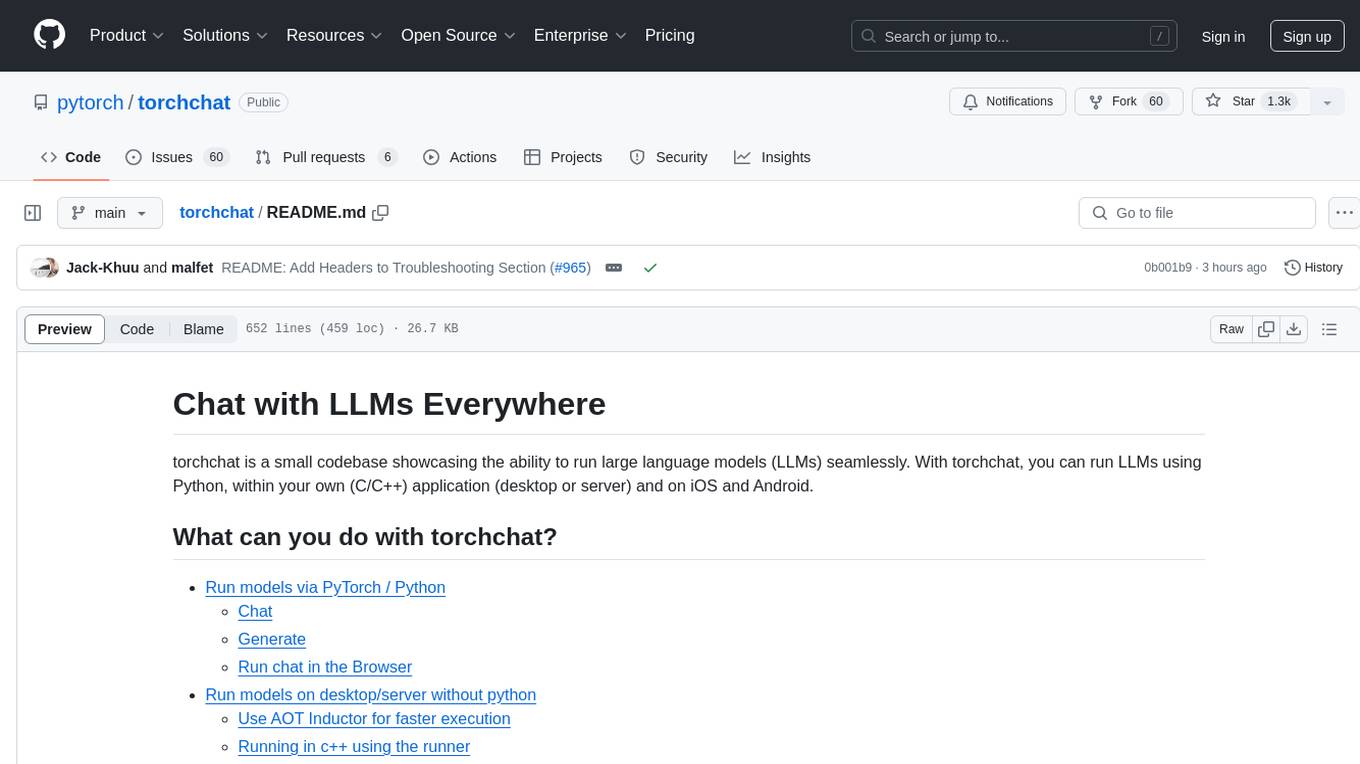
torchchat
torchchat is a codebase showcasing the ability to run large language models (LLMs) seamlessly. It allows running LLMs using Python in various environments such as desktop, server, iOS, and Android. The tool supports running models via PyTorch, chatting, generating text, running chat in the browser, and running models on desktop/server without Python. It also provides features like AOT Inductor for faster execution, running in C++ using the runner, and deploying and running on iOS and Android. The tool supports popular hardware and OS including Linux, Mac OS, Android, and iOS, with various data types and execution modes available.
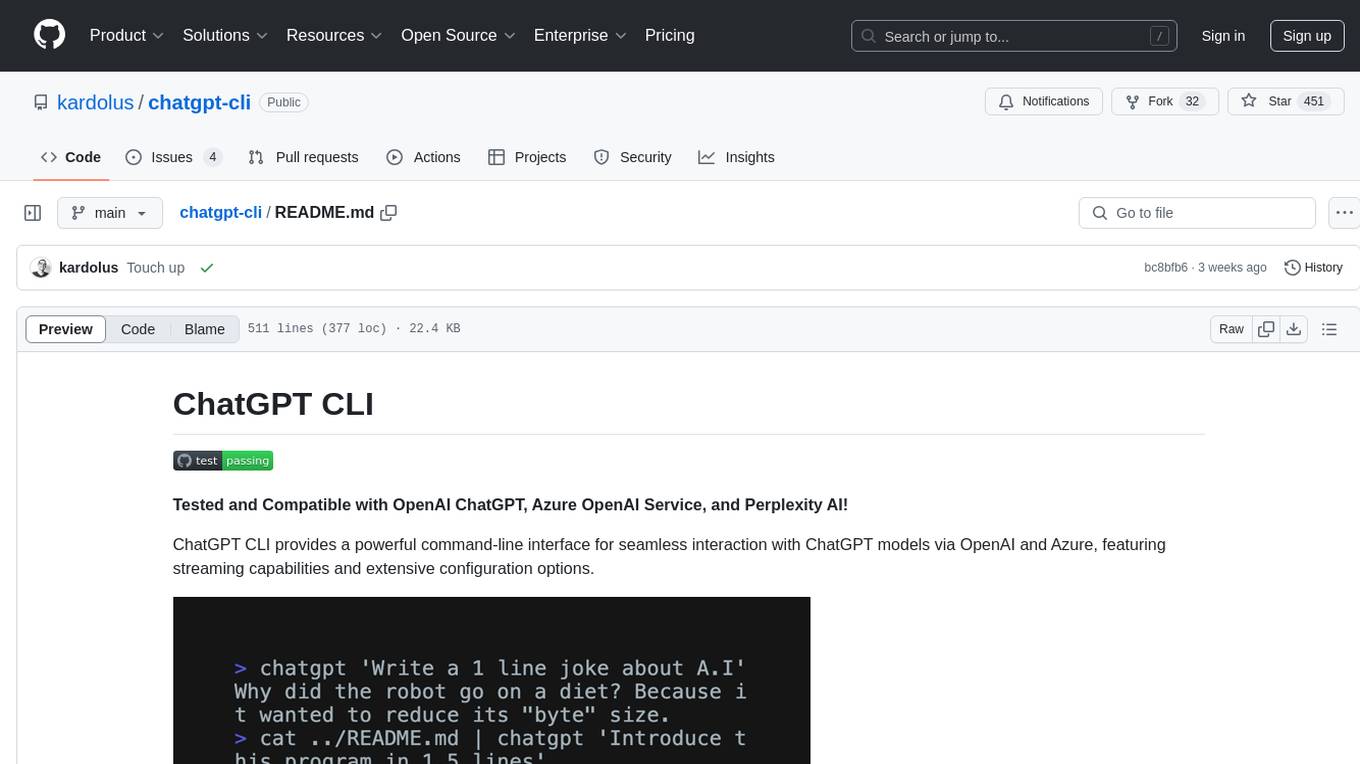
chatgpt-cli
ChatGPT CLI provides a powerful command-line interface for seamless interaction with ChatGPT models via OpenAI and Azure. It features streaming capabilities, extensive configuration options, and supports various modes like streaming, query, and interactive mode. Users can manage thread-based context, sliding window history, and provide custom context from any source. The CLI also offers model and thread listing, advanced configuration options, and supports GPT-4, GPT-3.5-turbo, and Perplexity's models. Installation is available via Homebrew or direct download, and users can configure settings through default values, a config.yaml file, or environment variables.
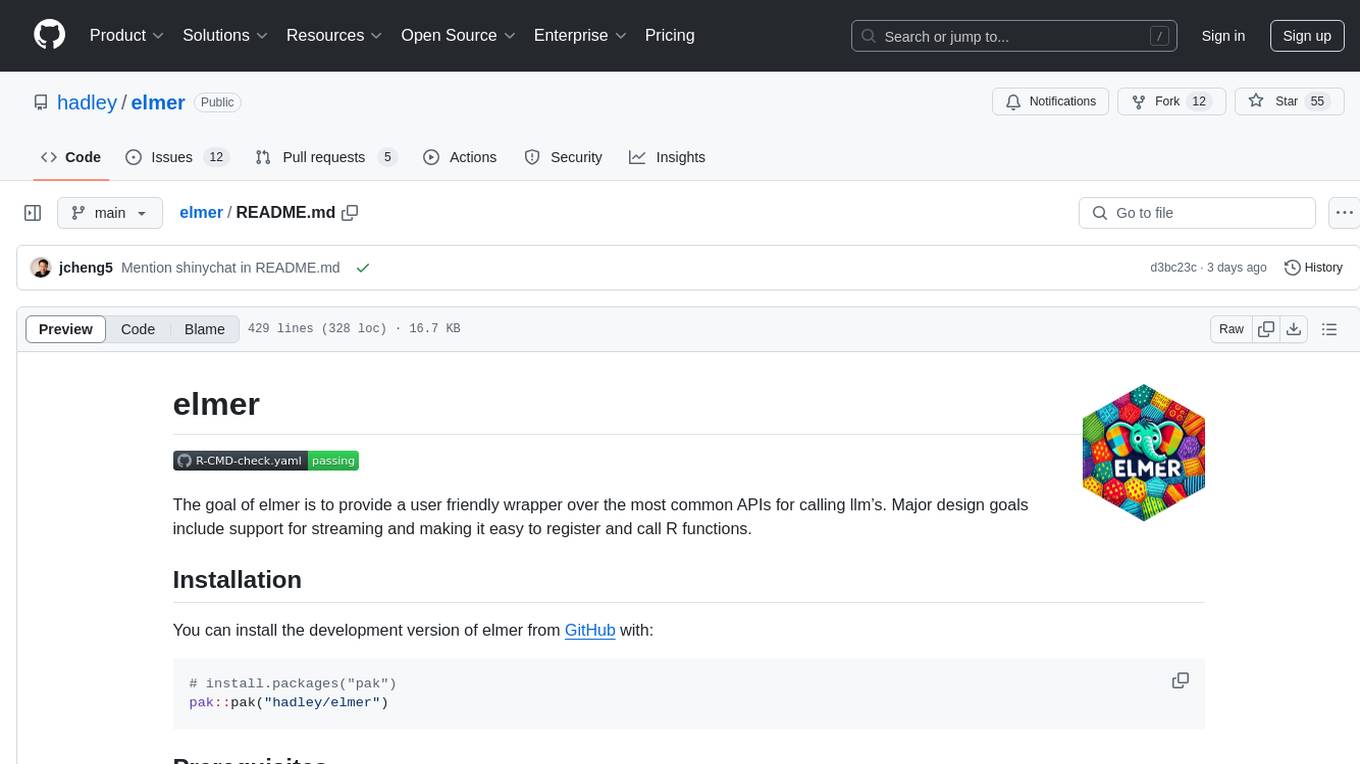
elmer
Elmer is a user-friendly wrapper over common APIs for calling llm’s, with support for streaming and easy registration and calling of R functions. Users can interact with Elmer in various ways, such as interactive chat console, interactive method call, programmatic chat, and streaming results. Elmer also supports async usage for running multiple chat sessions concurrently, useful for Shiny applications. The tool calling feature allows users to define external tools that Elmer can request to execute, enhancing the capabilities of the chat model.
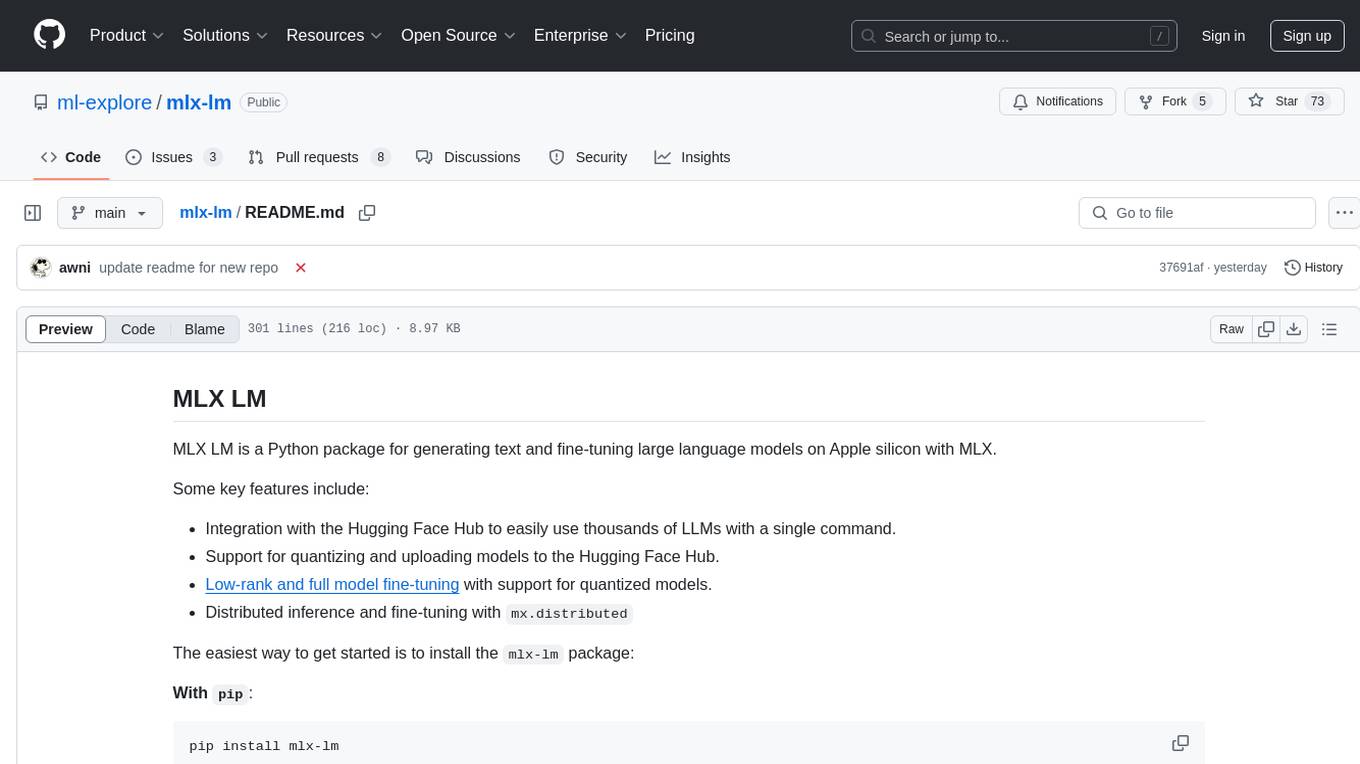
mlx-lm
MLX LM is a Python package designed for generating text and fine-tuning large language models on Apple silicon using MLX. It offers integration with the Hugging Face Hub for easy access to thousands of LLMs, support for quantizing and uploading models to the Hub, low-rank and full model fine-tuning capabilities, and distributed inference and fine-tuning with `mx.distributed`. Users can interact with the package through command line options or the Python API, enabling tasks such as text generation, chatting with language models, model conversion, streaming generation, and sampling. MLX LM supports various Hugging Face models and provides tools for efficient scaling to long prompts and generations, including a rotating key-value cache and prompt caching. It requires macOS 15.0 or higher for optimal performance.
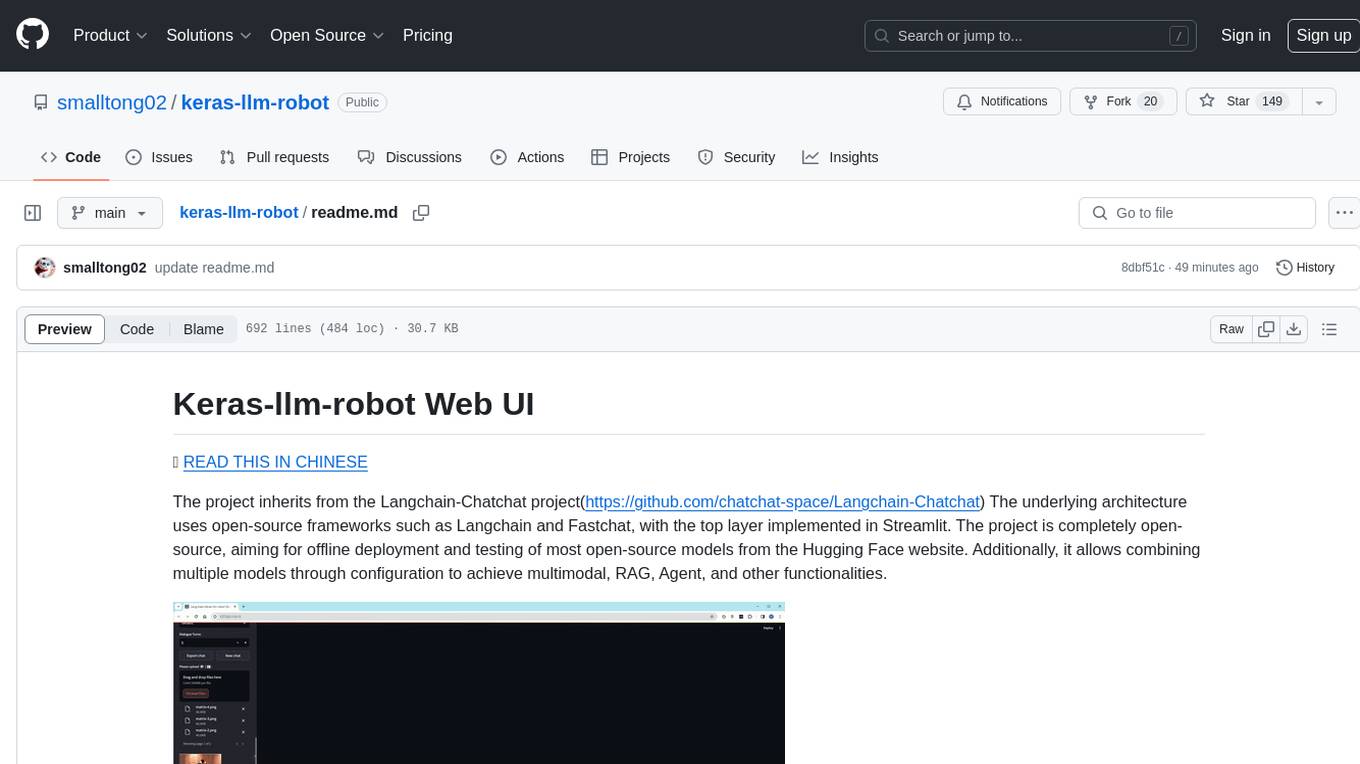
keras-llm-robot
The Keras-llm-robot Web UI project is an open-source tool designed for offline deployment and testing of various open-source models from the Hugging Face website. It allows users to combine multiple models through configuration to achieve functionalities like multimodal, RAG, Agent, and more. The project consists of three main interfaces: chat interface for language models, configuration interface for loading models, and tools & agent interface for auxiliary models. Users can interact with the language model through text, voice, and image inputs, and the tool supports features like model loading, quantization, fine-tuning, role-playing, code interpretation, speech recognition, image recognition, network search engine, and function calling.
For similar jobs
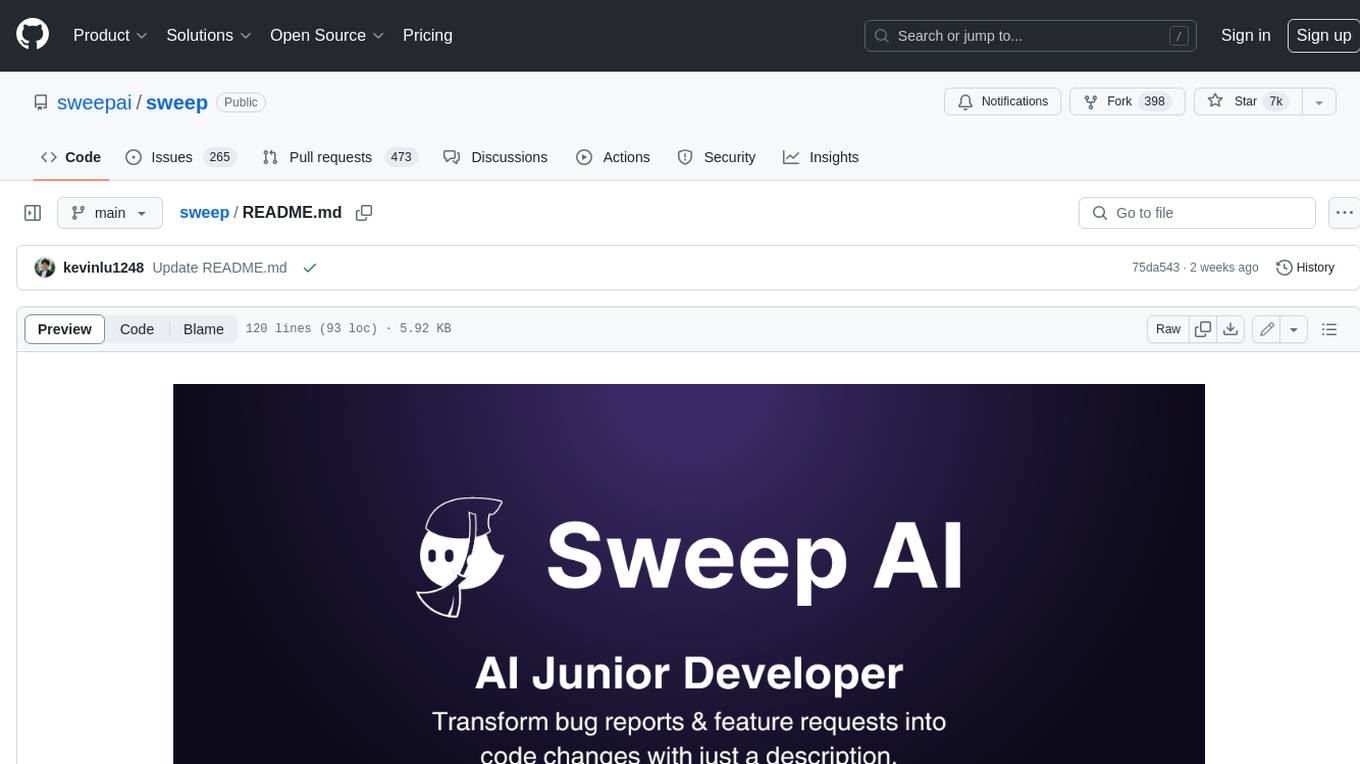
sweep
Sweep is an AI junior developer that turns bugs and feature requests into code changes. It automatically handles developer experience improvements like adding type hints and improving test coverage.
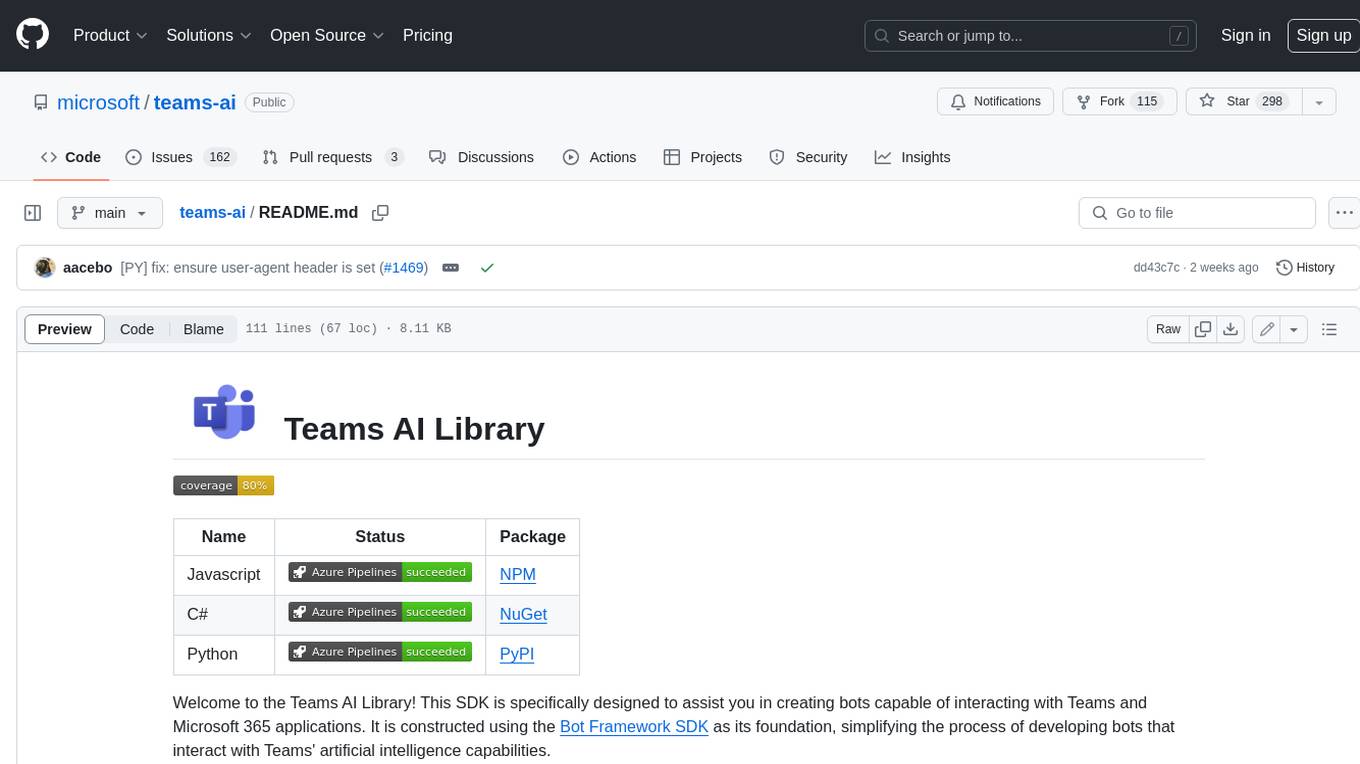
teams-ai
The Teams AI Library is a software development kit (SDK) that helps developers create bots that can interact with Teams and Microsoft 365 applications. It is built on top of the Bot Framework SDK and simplifies the process of developing bots that interact with Teams' artificial intelligence capabilities. The SDK is available for JavaScript/TypeScript, .NET, and Python.
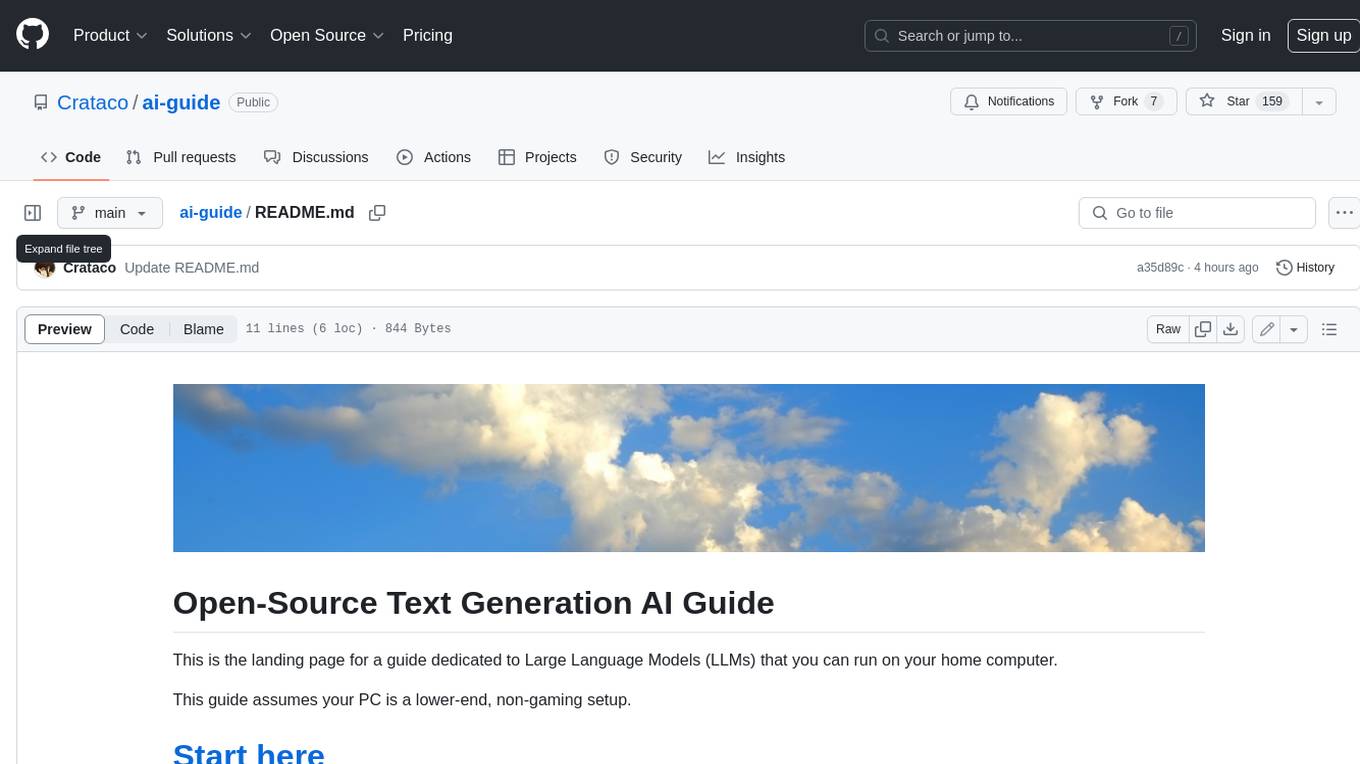
ai-guide
This guide is dedicated to Large Language Models (LLMs) that you can run on your home computer. It assumes your PC is a lower-end, non-gaming setup.
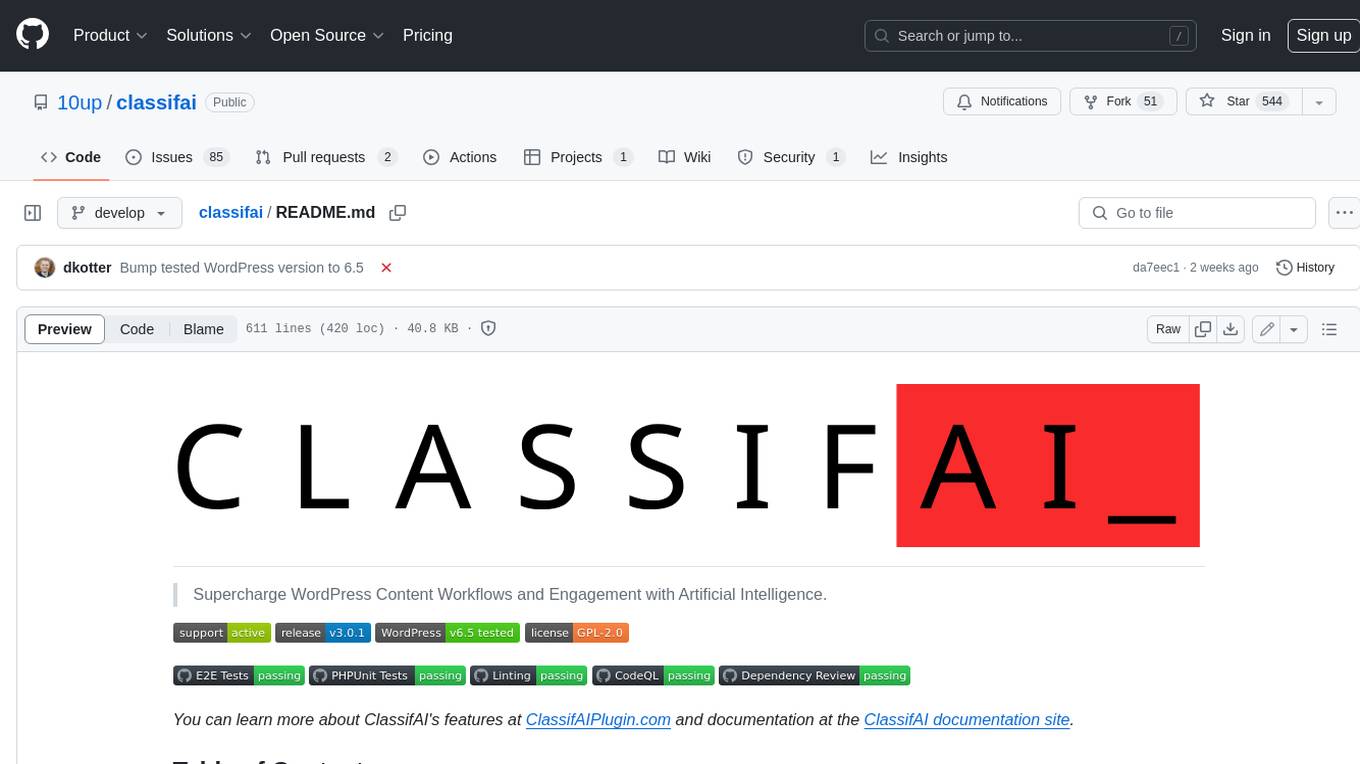
classifai
Supercharge WordPress Content Workflows and Engagement with Artificial Intelligence. Tap into leading cloud-based services like OpenAI, Microsoft Azure AI, Google Gemini and IBM Watson to augment your WordPress-powered websites. Publish content faster while improving SEO performance and increasing audience engagement. ClassifAI integrates Artificial Intelligence and Machine Learning technologies to lighten your workload and eliminate tedious tasks, giving you more time to create original content that matters.
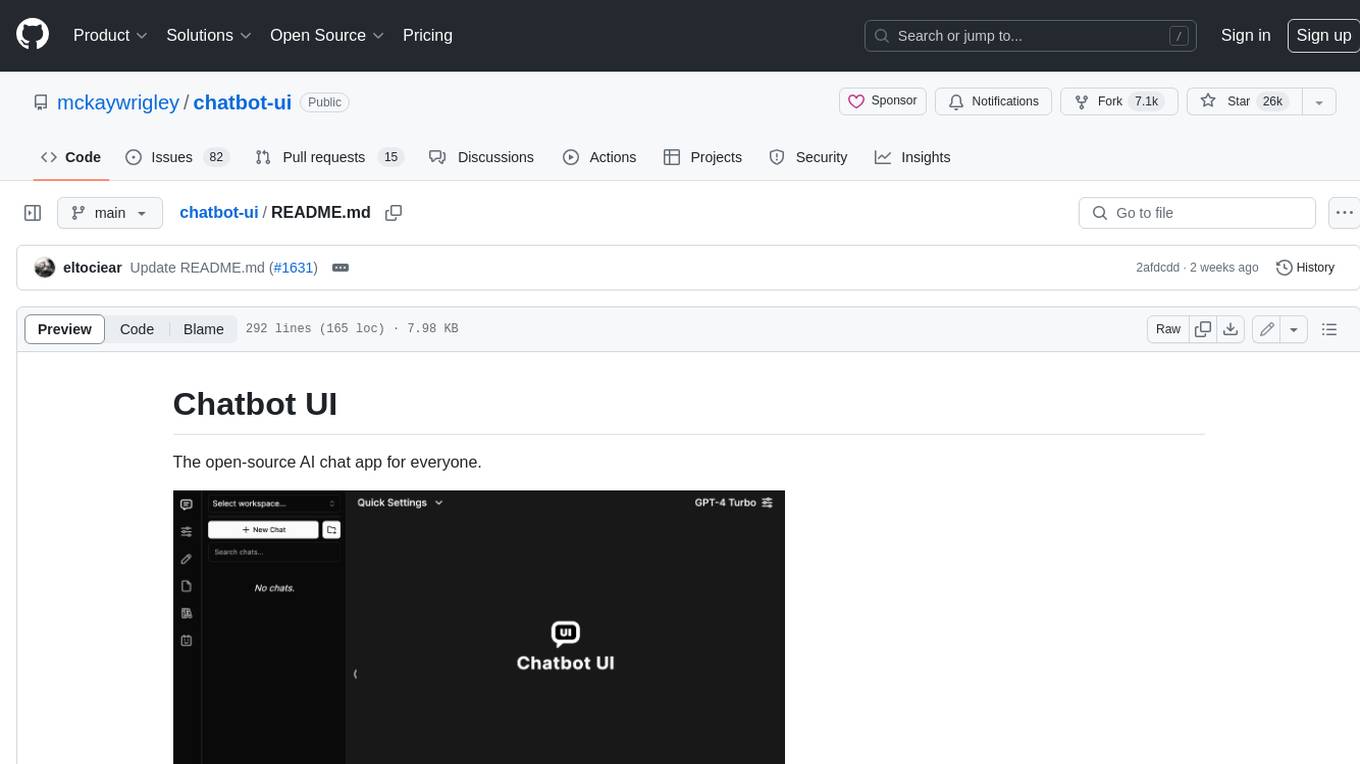
chatbot-ui
Chatbot UI is an open-source AI chat app that allows users to create and deploy their own AI chatbots. It is easy to use and can be customized to fit any need. Chatbot UI is perfect for businesses, developers, and anyone who wants to create a chatbot.
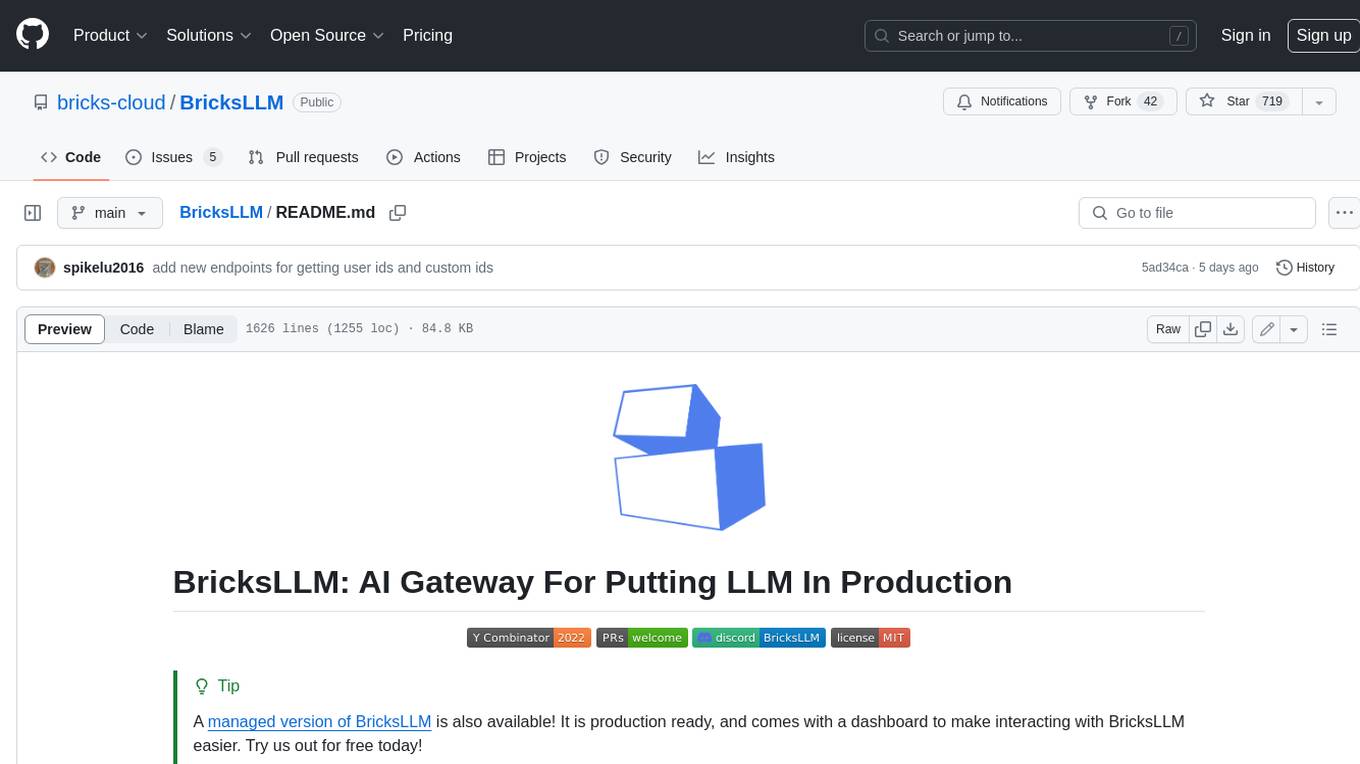
BricksLLM
BricksLLM is a cloud native AI gateway written in Go. Currently, it provides native support for OpenAI, Anthropic, Azure OpenAI and vLLM. BricksLLM aims to provide enterprise level infrastructure that can power any LLM production use cases. Here are some use cases for BricksLLM: * Set LLM usage limits for users on different pricing tiers * Track LLM usage on a per user and per organization basis * Block or redact requests containing PIIs * Improve LLM reliability with failovers, retries and caching * Distribute API keys with rate limits and cost limits for internal development/production use cases * Distribute API keys with rate limits and cost limits for students
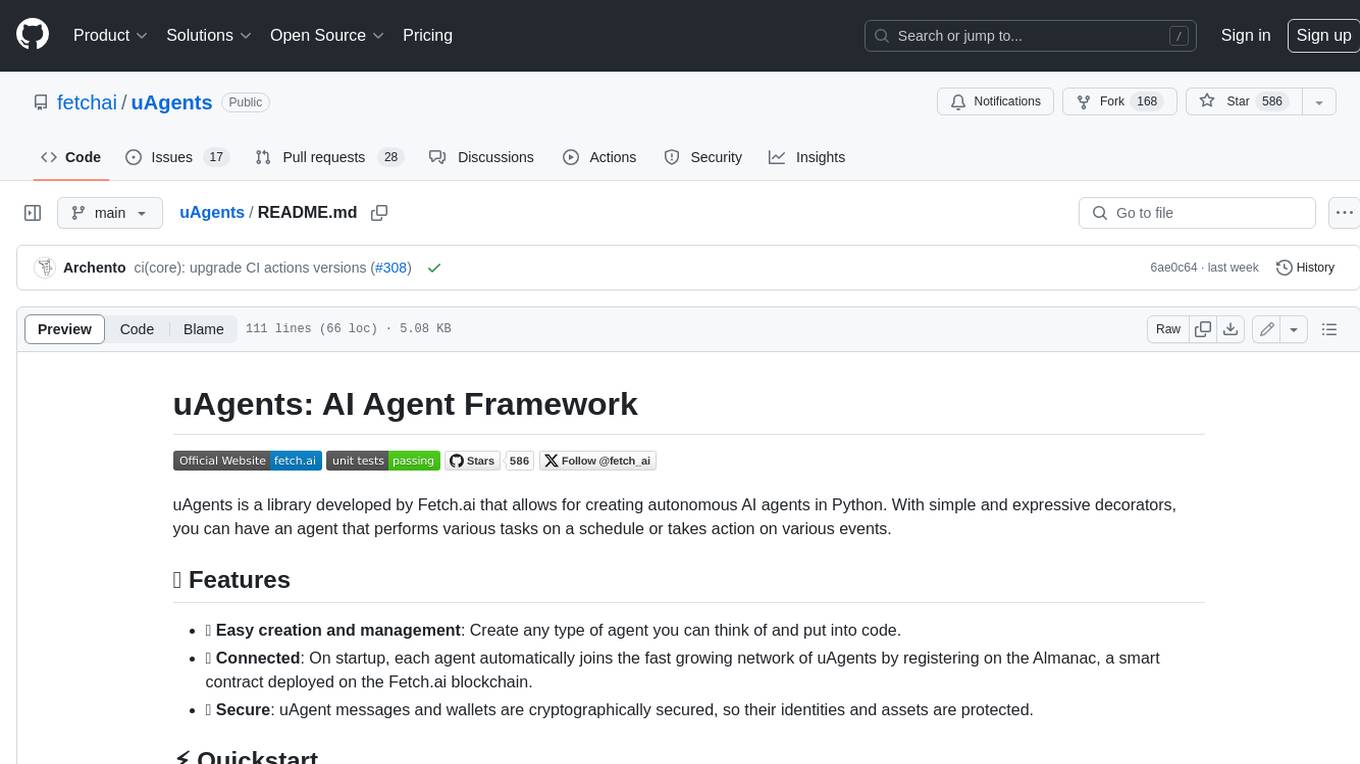
uAgents
uAgents is a Python library developed by Fetch.ai that allows for the creation of autonomous AI agents. These agents can perform various tasks on a schedule or take action on various events. uAgents are easy to create and manage, and they are connected to a fast-growing network of other uAgents. They are also secure, with cryptographically secured messages and wallets.
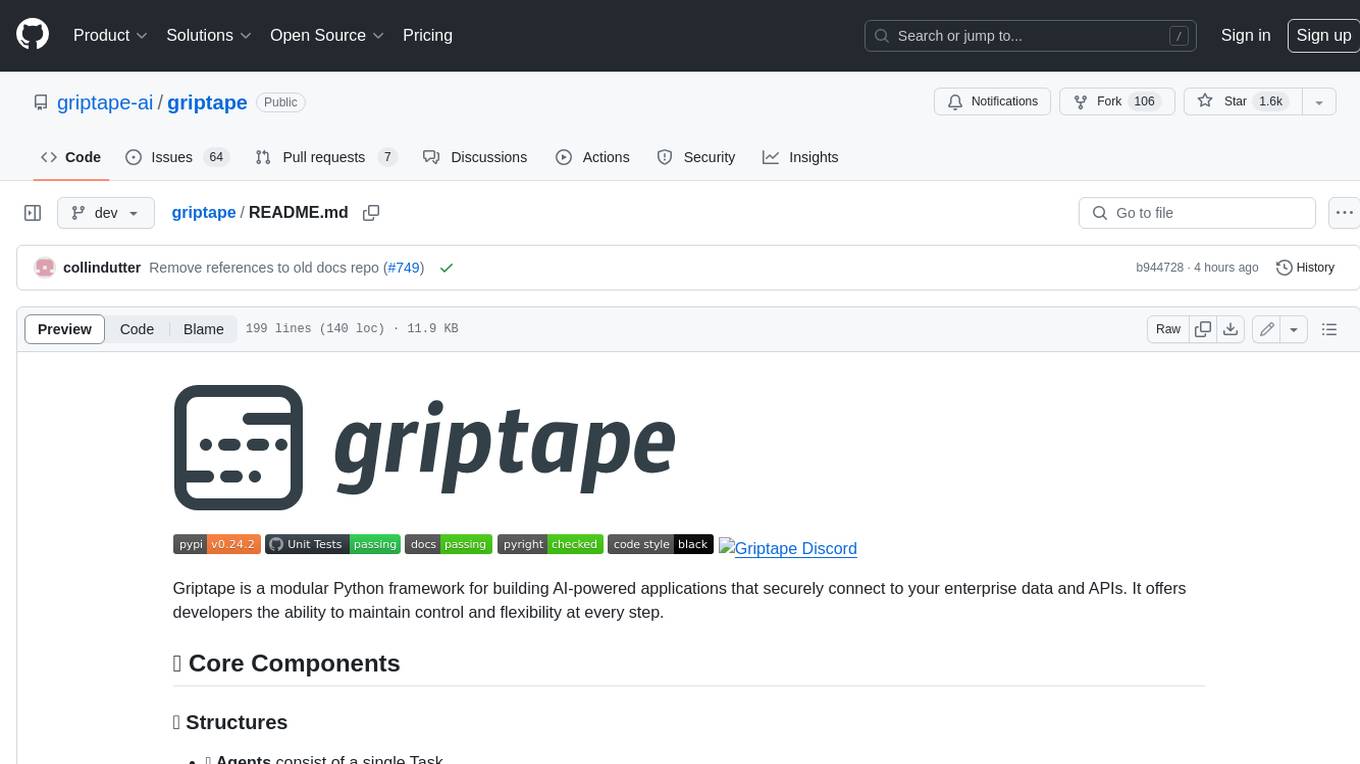
griptape
Griptape is a modular Python framework for building AI-powered applications that securely connect to your enterprise data and APIs. It offers developers the ability to maintain control and flexibility at every step. Griptape's core components include Structures (Agents, Pipelines, and Workflows), Tasks, Tools, Memory (Conversation Memory, Task Memory, and Meta Memory), Drivers (Prompt and Embedding Drivers, Vector Store Drivers, Image Generation Drivers, Image Query Drivers, SQL Drivers, Web Scraper Drivers, and Conversation Memory Drivers), Engines (Query Engines, Extraction Engines, Summary Engines, Image Generation Engines, and Image Query Engines), and additional components (Rulesets, Loaders, Artifacts, Chunkers, and Tokenizers). Griptape enables developers to create AI-powered applications with ease and efficiency.