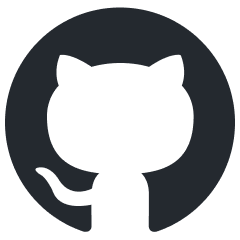
code2prompt
Code2Prompt is a powerful command-line tool that simplifies the process of providing context to Large Language Models (LLMs) by generating a comprehensive Markdown file containing the content of your codebase. β If you find Code2Prompt useful, consider giving us a star on GitHub! It helps us reach more developers and improve the tool. β
Stars: 492
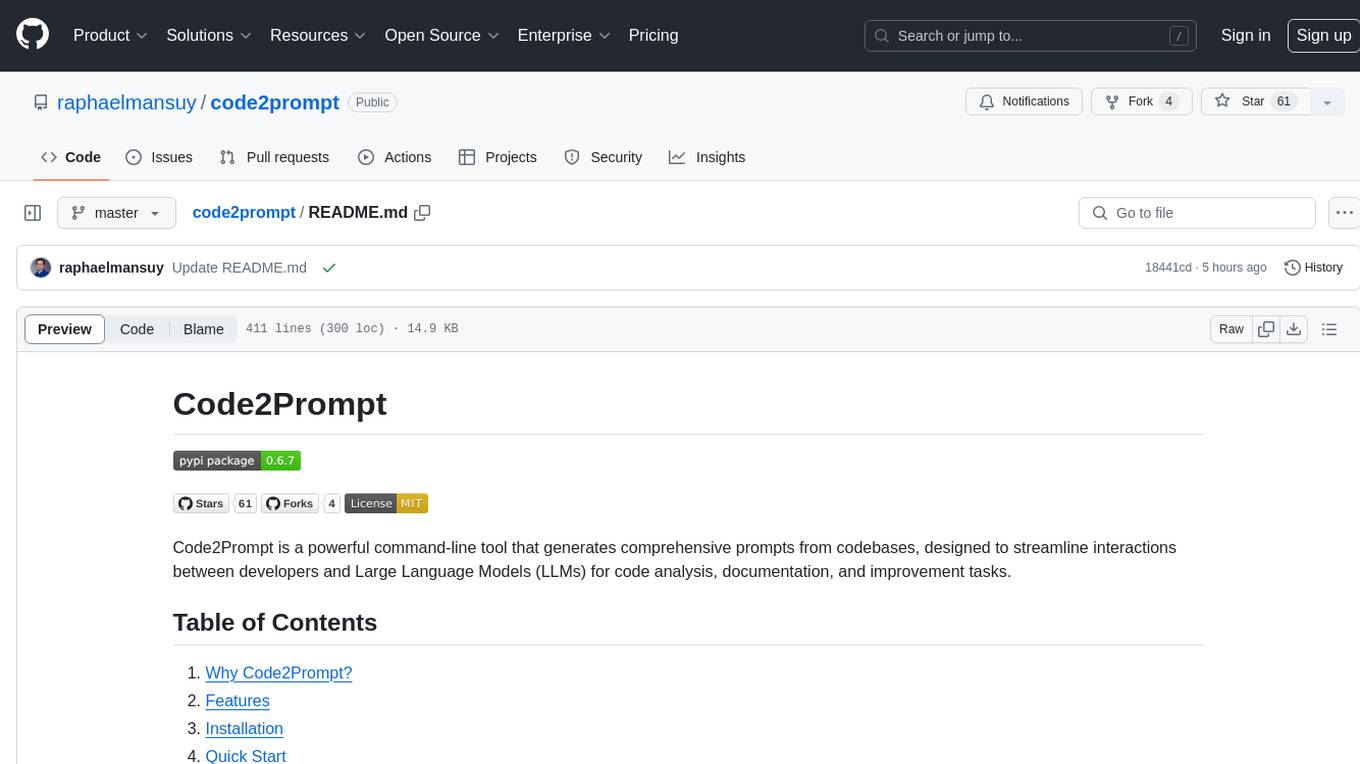
Code2Prompt is a powerful command-line tool that generates comprehensive prompts from codebases, designed to streamline interactions between developers and Large Language Models (LLMs) for code analysis, documentation, and improvement tasks. It bridges the gap between codebases and LLMs by converting projects into AI-friendly prompts, enabling users to leverage AI for various software development tasks. The tool offers features like holistic codebase representation, intelligent source tree generation, customizable prompt templates, smart token management, Gitignore integration, flexible file handling, clipboard-ready output, multiple output options, and enhanced code readability.
README:
Code2Prompt is a powerful command-line tool that generates comprehensive prompts from codebases, designed to streamline interactions between developers and Large Language Models (LLMs) for code analysis, documentation, and improvement tasks.
- Why Code2Prompt?
- Features
- Installation
- Getting Started
- Quick Start
- Usage
- Options
- Examples
- Templating System
- Integration with LLM CLI
- GitHub Actions Integration
- Configuration File
- Troubleshooting
- Contributing
- License
Code2Prompt is a powerful, open-source command-line tool that bridges the gap between your codebase and Large Language Models (LLMs). By converting your entire project into a comprehensive, AI-friendly prompt, Code2Prompt enables you to leverage the full potential of AI for code analysis, documentation, and improvement tasks.
- Holistic Codebase Representation: Generate a well-structured Markdown prompt that captures your entire project's essence, making it easier for LLMs to understand the context.
- Intelligent Source Tree Generation: Create a clear, hierarchical view of your codebase structure, allowing for better navigation and understanding of the project.
- Customizable Prompt Templates: Tailor your output using Jinja2 templates to suit specific AI tasks, enhancing the relevance of generated prompts.
- Smart Token Management: Count and optimize tokens to ensure compatibility with various LLM token limits, preventing errors during processing.
- Gitignore Integration: Respect your project's .gitignore rules for accurate representation, ensuring that irrelevant files are excluded from processing.
- Flexible File Handling: Filter and exclude files using powerful glob patterns, giving you control over which files are included in the prompt generation.
-
Custom Syntax Highlighting: Pair custom file extensions with specific syntax highlighting using the
--syntax-map
option. For example, you can specify that.inc
files should be treated asbash
scripts. - Clipboard Ready: Instantly copy generated prompts to your clipboard for quick AI interactions, streamlining your workflow.
- Multiple Output Options: Save to file or display in the console, providing flexibility in how you want to use the generated prompts.
- Enhanced Code Readability: Add line numbers to source code blocks for precise referencing, making it easier to discuss specific parts of the code.
- Include file: Support of template import, allowing for modular template design.
- Input variables: Support of Input Variables in templates, enabling dynamic prompt generation based on user input.
- Contextual Understanding: Provide LLMs with a comprehensive view of your project for more accurate suggestions and analysis.
- Consistency Boost: Maintain coding style and conventions across your entire project, improving code quality.
- Efficient Refactoring: Enable better interdependency analysis and smarter refactoring recommendations, saving time and effort.
- Improved Documentation: Generate contextually relevant documentation that truly reflects your codebase, enhancing maintainability.
- Pattern Recognition: Help LLMs learn and apply your project-specific patterns and idioms, improving the quality of AI interactions.
Transform the way you interact with AI for software development. With Code2Prompt, harness the full power of your codebase in every AI conversation.
Ready to elevate your AI-assisted development? Let's dive in! πββοΈ
Choose one of the following methods to install Code2Prompt:
pip install code2prompt
Using pipx (recommended)
pipx install code2prompt
To get started with Code2Prompt, follow these steps:
- Install Code2Prompt: Use one of the installation methods mentioned above.
-
Prepare Your Codebase: Ensure your project is organized and that you have a
.gitignore
file if necessary. - Run Code2Prompt: Use the command line to generate prompts from your codebase.
For example, to generate a prompt from a single Python file, run:
code2prompt --path /path/to/your/script.py
-
Generate a prompt from a single Python file:
code2prompt --path /path/to/your/script.py
-
Process an entire project directory and save the output:
code2prompt --path /path/to/your/project --output project_summary.md
-
Generate a prompt for multiple files, excluding tests:
code2prompt --path /path/to/src --path /path/to/lib --exclude "*/tests/*" --output codebase_summary.md
The basic syntax for Code2Prompt is:
code2prompt --path /path/to/your/code [OPTIONS]
For multiple paths:
code2prompt --path /path/to/dir1 --path /path/to/file2.py [OPTIONS]
To pair custom file extensions with specific syntax highlighting, use the --syntax-map
option. This allows you to specify mappings in the format extension:syntax
. For example:
code2prompt --path /path/to/your/code --syntax-map "inc:bash,customext:python,ext2:javascript"
This command will treat .inc
files as bash
scripts, .customext
files as python
, and .ext2
files as javascript
.
You can also use multiple --syntax-map
arguments or separate mappings with commas:
code2prompt --path /path/to/your/script.py --syntax-map "inc:bash"
code2prompt --path /path/to/your/project --syntax-map "inc:bash,txt:markdown" --output project_summary.md
code2prompt --path /path/to/src --path /path/to/lib --syntax-map "inc:bash,customext:python" --output codebase_summary.md
Option | Short | Description |
---|---|---|
--path |
-p |
Path(s) to the directory or file to process (required, multiple allowed) |
--output |
-o |
Name of the output Markdown file |
--gitignore |
-g |
Path to the .gitignore file |
--filter |
-f |
Comma-separated filter patterns to include files (e.g., ".py,.js") |
--exclude |
-e |
Comma-separated patterns to exclude files (e.g., ".txt,.md") |
--case-sensitive |
Perform case-sensitive pattern matching | |
--suppress-comments |
-s |
Strip comments from the code files |
--line-number |
-ln |
Add line numbers to source code blocks |
--no-codeblock |
Disable wrapping code inside markdown code blocks | |
--template |
-t |
Path to a Jinja2 template file for custom prompt generation |
--tokens |
Display the token count of the generated prompt | |
--encoding |
Specify the tokenizer encoding to use (default: "cl100k_base") | |
--create-templates |
Create a templates directory with example templates | |
--version |
-v |
Show the version and exit |
--log-level |
Set the logging level (e.g., DEBUG, INFO, WARNING, ERROR, CRITICAL) | |
--interactive |
-i |
Activate interactive mode for file selection |
--syntax-map |
Pair custom file extensions with specific syntax highlighting (e.g., "inc:bash,customext:python,ext2:javascript") |
The --filter
and --exclude
options allow you to specify patterns for files or directories that should be included in or excluded from processing, respectively.
--filter "PATTERN1,PATTERN2,..."
--exclude "PATTERN1,PATTERN2,..."
or
-f "PATTERN1,PATTERN2,..."
-e "PATTERN1,PATTERN2,..."
- Both options accept a comma-separated list of patterns.
- Patterns can include wildcards (
*
) and directory indicators (**
). - Case-sensitive by default (use
--case-sensitive
flag to change this behavior). -
--exclude
patterns take precedence over--filter
patterns.
-
Include only Python files:
--filter "**.py"
-
Exclude all Markdown files:
--exclude "**.md"
-
Include specific file types in the src directory:
--filter "src/**.{js,ts}"
-
Exclude multiple file types and a specific directory:
--exclude "**.log,**.tmp,**/node_modules/**"
-
Include all files except those in 'test' directories:
--filter "**" --exclude "**/test/**"
-
Complex filtering (include JavaScript files, exclude minified and test files):
--filter "**.js" --exclude "**.min.js,**test**.js"
-
Include specific files across all directories:
--filter "**/config.json,**/README.md"
-
Exclude temporary files and directories:
--exclude "**/.cache/**,**/tmp/**,**.tmp"
-
Include source files but exclude build output:
--filter "src/**/*.{js,ts}" --exclude "**/dist/**,**/build/**"
-
Exclude version control and IDE-specific files:
--exclude "**/.git/**,**/.vscode/**,**/.idea/**"
- Always use double quotes around patterns to prevent shell interpretation of special characters.
- Patterns are matched against the full path of each file, relative to the project root.
- The
**
wildcard matches any number of directories. - Single
*
matches any characters within a single directory or filename. - Use commas to separate multiple patterns within the same option.
- Combine
--filter
and--exclude
for fine-grained control over which files are processed.
- Start with broader patterns and refine as needed.
- Test your patterns on a small subset of your project first.
- Use the
--case-sensitive
flag if you need to distinguish between similarly named files with different cases. - When working with complex projects, consider using a configuration file to manage your filter and exclude patterns.
By using the --filter
and --exclude
options effectively and safely (with proper quoting), you can precisely control which files are processed in your project, ensuring both accuracy and security in your command execution.
-
Generate documentation for a Python library:
code2prompt --path /path/to/library --output library_docs.md --suppress-comments --line-number --filter "*.py"
-
Prepare a codebase summary for a code review, focusing on JavaScript and TypeScript files:
code2prompt --path /path/to/project --filter "*.js,*.ts" --exclude "node_modules/*,dist/*" --template code_review.j2 --output code_review.md
-
Create input for an AI model to suggest improvements, focusing on a specific directory:
code2prompt --path /path/to/src/components --suppress-comments --tokens --encoding cl100k_base --output ai_input.md
-
Analyze comment density across a multi-language project:
code2prompt --path /path/to/project --template comment_density.j2 --output comment_analysis.md --filter "*.py,*.js,*.java"
-
Generate a prompt for a specific set of files, adding line numbers:
code2prompt --path /path/to/important_file1.py --path /path/to/important_file2.js --line-number --output critical_files.md
Code2Prompt supports custom output formatting using Jinja2 templates. To use a custom template:
code2prompt --path /path/to/code --template /path/to/your/template.j2
Use the --create-templates
command to generate example templates:
code2prompt --create-templates
This creates a templates
directory with sample Jinja2 templates, including:
- default.j2: A general-purpose template
- analyze-code.j2: For detailed code analysis
- code-review.j2: For thorough code reviews
- create-readme.j2: To assist in generating README files
- improve-this-prompt.j2: For refining AI prompts
For full template documentation, see Documentation Templating.
Code2Prompt can be integrated with Simon Willison's llm CLI tool for enhanced code analysis or qllm, or for the Rust lovers hiramu-cli.
pip install code2prompt llm
-
Generate a code summary and analyze it with an LLM:
code2prompt --path /path/to/your/project | llm "Analyze this codebase and provide insights on its structure and potential improvements"
-
Process a specific file and get refactoring suggestions:
code2prompt --path /path/to/your/script.py | llm "Suggest refactoring improvements for this code"
For more advanced use cases, refer to the Integration with LLM CLI section in the full documentation.
You can integrate Code2Prompt into your GitHub Actions workflow. Here's an example:
name: Code Analysis
on: [push]
jobs:
analyze-code:
runs-on: ubuntu-latest
steps:
- uses: actions/checkout@v2
- name: Set up Python
uses: actions/setup-python@v2
with:
python-version: '3.x'
- name: Install dependencies
run: |
pip install code2prompt llm
- name: Analyze codebase
run: |
code2prompt --path . | llm "Perform a comprehensive analysis of this codebase. Identify areas for improvement, potential bugs, and suggest optimizations." > analysis.md
- name: Upload analysis
uses: actions/upload-artifact@v2
with:
name: code-analysis
path: analysis.md
Tokens are the basic units of text that language models process. They can be words, parts of words, or even punctuation marks. Different tokenizer encodings split text into tokens in various ways. Code2Prompt supports multiple token types through its --encoding
option, with "cl100k_base" as the default. This encoding, used by models like GPT-3.5 and GPT-4, is adept at handling code and technical content. Other common encodings include "p50k_base" (used by earlier GPT-3 models) and "r50k_base" (used by models like CodeX).
To count tokens in your generated prompt, use the --tokens
flag:
code2prompt --path /your/project --tokens
For a specific encoding:
code2prompt --path /your/project --tokens --encoding p50k_base
Understanding token counts is crucial when working with AI models that have token limits, ensuring your prompts fit within the model's context window.
Code2Prompt now includes a powerful feature for estimating token prices across various AI providers and models. Use the --price
option in conjunction with --tokens
to display a comprehensive breakdown of estimated costs. This feature calculates prices based on both input and output tokens, with input tokens determined by your codebase and a default of 1000 output tokens (customizable via --output-tokens
). You can specify a particular provider or model, or view prices across all available options. This functionality helps developers make informed decisions about AI model usage and cost management. For example:
code2prompt --path /your/project --tokens --price --provider openai --model gpt-4
This command will analyze your project, count the tokens, and provide a detailed price estimation for OpenAI's GPT-4 model.
code2prompt now offers a powerful feature to analyze codebases and provide a summary of file extensions. Use the --analyze
option along with the -p
(path) option to get an overview of your project's file composition. For example:
code2prompt --analyze -p code2prompt
Result:
.j2: 6 files
.json: 1 file
.py: 33 files
.pyc: 56 files
Comma-separated list of extensions:
.j2,.json,.py,.pyc
This command will analyze the 'code2prompt' directory and display a summary of all file extensions found, including their counts. You can choose between two output formats:
- Flat format (default): Lists all unique extensions alphabetically with their file counts.
- Tree-like format: Displays extensions in a directory tree structure with counts at each level.
To use the tree-like format, add the --format tree
option:
code2prompt --analyze -p code2prompt --format tree
Result:
βββ code2prompt
βββ utils
β βββ .py
β βββ __pycache__
β βββ .pyc
βββ .py
βββ core
β βββ .py
β βββ __pycache__
β βββ .pyc
βββ comment_stripper
β βββ .py
β βββ __pycache__
β βββ .pyc
βββ __pycache__
β ββ .pyc
βββ templates
β βββ .j2
βββ data
βββ .json
Comma-separated list of extensions:
.j2,.json,.py,.pyc
The analysis also generates a comma-separated list of file extensions, which can be easily copied and used with the --filter
option for more targeted code processing.
code2prompt
offers a powerful feature for dynamic variable extraction from templates, allowing for interactive and customizable prompt generation. Using the syntax {{input:variable_name}}
, you can easily define variables that will prompt users for input during execution.
This is particularly useful for creating flexible templates for various purposes, such as generating AI prompts for Chrome extensions. Here's an example:
# AI Prompt Generator for Chrome Extension
Generate a prompt for an AI to create a Chrome extension with the following specifications:
Extension Name: {{input:extension_name}}
Main Functionality: {{input:main_functionality}}
Target Audience: {{input:target_audience}}
## Prompt:
You are an experienced Chrome extension developer. Create a detailed plan for a Chrome extension named "{{input:extension_name}}" that {{input:main_functionality}}. This extension is designed for {{input:target_audience}}.
Your response should include:
1. A brief description of the extension's purpose and functionality
2. Key features (at least 3)
3. User interface design considerations
4. Potential challenges in development and how to overcome them
5. Security and privacy considerations
6. A basic code structure for the main components (manifest.json, background script, content script, etc.)
Ensure that your plan is detailed, technically sound, and tailored to the needs of {{input:target_audience}}.
Start from this codebase:
----
## The codebase:
<codebase>
When you run code2prompt
with this template, it will automatically detect the {{input:variable_name}}
patterns and prompt the user to provide values for each variable (extension_name, main_functionality, and target_audience). This allows for flexible and interactive prompt generation, making it easy to create customized AI prompts for various Chrome extension ideas.
For example, if a user inputs:
- Extension Name: "ProductivityBoost"
- Main Functionality: "tracks time spent on different websites and provides productivity insights"
- Target Audience: "professionals working from home"
The tool will generate a tailored prompt for an AI to create a detailed plan for this specific Chrome extension. This feature is particularly useful for developers, product managers, or anyone looking to quickly generate customized AI prompts for various projects or ideas.
The code2prompt project now supports a powerful "include file" feature, enhancing template modularity and reusability.
This feature allows you to seamlessly incorporate external file content into your main template using the {% include %}
directive. For example, in the main analyze-code.j2
template, you can break down complex sections into smaller, manageable files:
# Elite Code Analyzer and Improvement Strategist 2.0
{% include 'sections/role_and_goal.j2' %}
{% include 'sections/core_competencies.j2' %}
## Task Breakdown
1. Initial Assessment
{% include 'tasks/initial_assessment.j2' %}
2. Multi-Dimensional Analysis (Utilize Tree of Thought)
{% include 'tasks/multi_dimensional_analysis.j2' %}
// ... other sections ...
This approach allows you to organize your template structure more efficiently, improving maintainability and allowing for easy updates to specific sections without modifying the entire template. The include feature supports both relative and absolute paths, making it flexible for various project structures. By leveraging this feature, you can significantly reduce code duplication, improve template management, and create a more modular and scalable structure for your code2prompt templates.
The interactive mode allows users to select files for processing in a user-friendly manner. This feature is particularly useful when dealing with large codebases or when you want to selectively include files without manually specifying each path.
To activate interactive mode, use the --interactive
or -i
option when running the code2prompt
command. Here's an example:
code2prompt --path /path/to/your/project --interactive
- File Selection: Navigate through the directory structure and select files using keyboard controls.
- Visual Feedback: The interface provides visual cues to help you understand which files are selected or ignored.
- Arrow Keys: Navigate through the list of files.
- Spacebar: Toggle the selection of a file.
- Enter: Confirm your selection and proceed with the command.
- Esc: Exit the interactive mode without making any changes.
This mode enhances the usability of Code2Prompt, making it easier to manage file selections in complex projects.
Code2Prompt supports a .code2promptrc
configuration file in JSON format for setting default options. Place this file in your project or home directory.
Example .code2promptrc
:
{
"suppress_comments": true,
"line_number": true,
"encoding": "cl100k_base",
"filter": "*.py,*.js",
"exclude": "tests/*,docs/*"
}
-
Issue: Code2Prompt is not recognizing my .gitignore file. Solution: Run Code2Prompt from the project root, or specify the .gitignore path with
--gitignore
. -
Issue: The generated output is too large for my AI model. Solution: Use
--tokens
to check the count, and refine--filter
or--exclude
options. -
Issue: Some files are not being processed. Solution: Check for binary files or exclusion patterns. Use
--case-sensitive
if needed.
- [X] Interactive filtering
- [X] Include system in template to promote re-usability of sub templates.
- [X] Support of input variables
- [ ] Tokens count for Anthropic Models and other models such as LLama3 or Mistral
- [X] Cost Estimations for main LLM providers based on token count
- [ ] Integration with qllm (Quantalogic LLM)
- [ ] Embedding of file summary in SQL-Lite
- [ ] Intelligence selection of file based on an LLM
- [ ] Git power tools (Git diff integration / PR Assisted Review)
Contributions to Code2Prompt are welcome! Please read our Contributing Guide for details on our code of conduct and the process for submitting pull requests.
Code2Prompt is released under the MIT License. See the LICENSE file for details.
β If you find Code2Prompt useful, please give us a star on GitHub! It helps us reach more developers and improve the tool. β
Made with β€οΈ by RaphaΓ«l MANSUY. Founder of Quantalogic. Creator of qllm.
For Tasks:
Click tags to check more tools for each tasksFor Jobs:
Alternative AI tools for code2prompt
Similar Open Source Tools
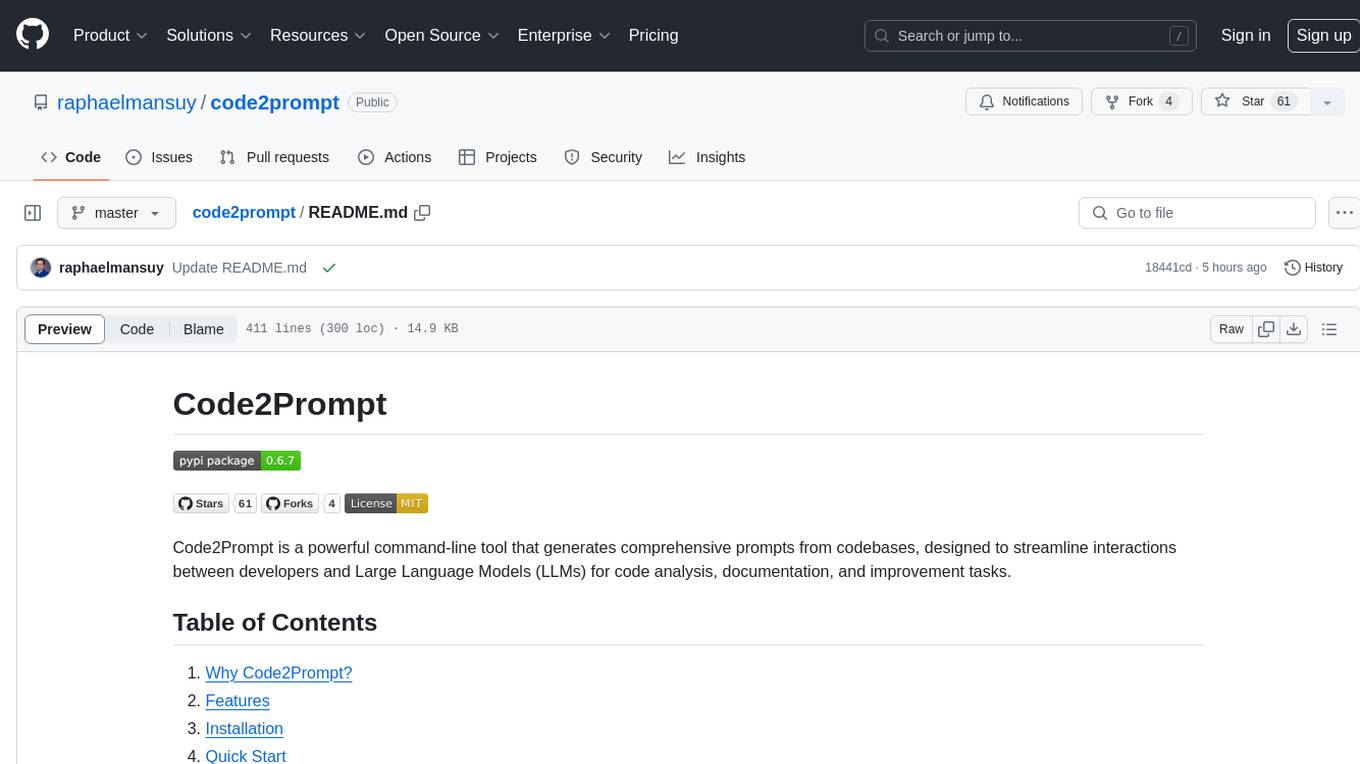
code2prompt
Code2Prompt is a powerful command-line tool that generates comprehensive prompts from codebases, designed to streamline interactions between developers and Large Language Models (LLMs) for code analysis, documentation, and improvement tasks. It bridges the gap between codebases and LLMs by converting projects into AI-friendly prompts, enabling users to leverage AI for various software development tasks. The tool offers features like holistic codebase representation, intelligent source tree generation, customizable prompt templates, smart token management, Gitignore integration, flexible file handling, clipboard-ready output, multiple output options, and enhanced code readability.
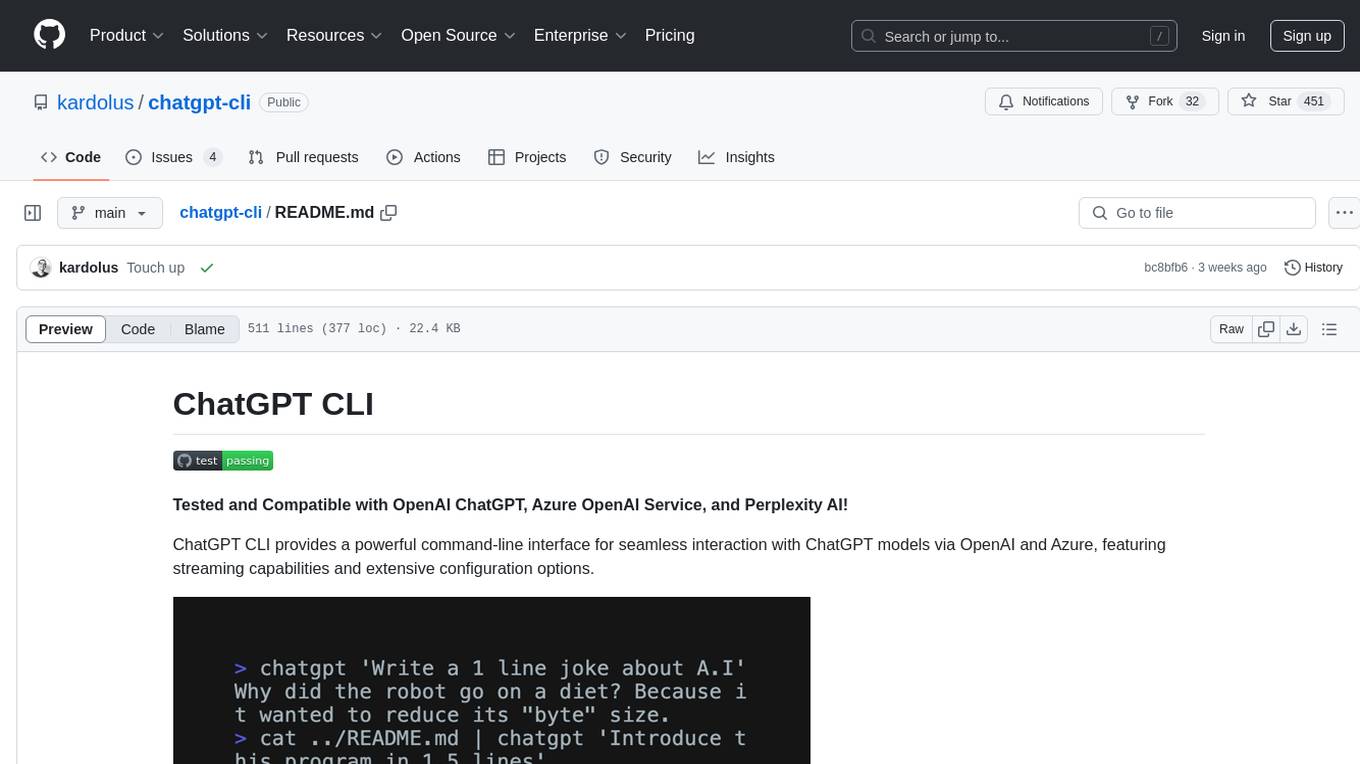
chatgpt-cli
ChatGPT CLI provides a powerful command-line interface for seamless interaction with ChatGPT models via OpenAI and Azure. It features streaming capabilities, extensive configuration options, and supports various modes like streaming, query, and interactive mode. Users can manage thread-based context, sliding window history, and provide custom context from any source. The CLI also offers model and thread listing, advanced configuration options, and supports GPT-4, GPT-3.5-turbo, and Perplexity's models. Installation is available via Homebrew or direct download, and users can configure settings through default values, a config.yaml file, or environment variables.
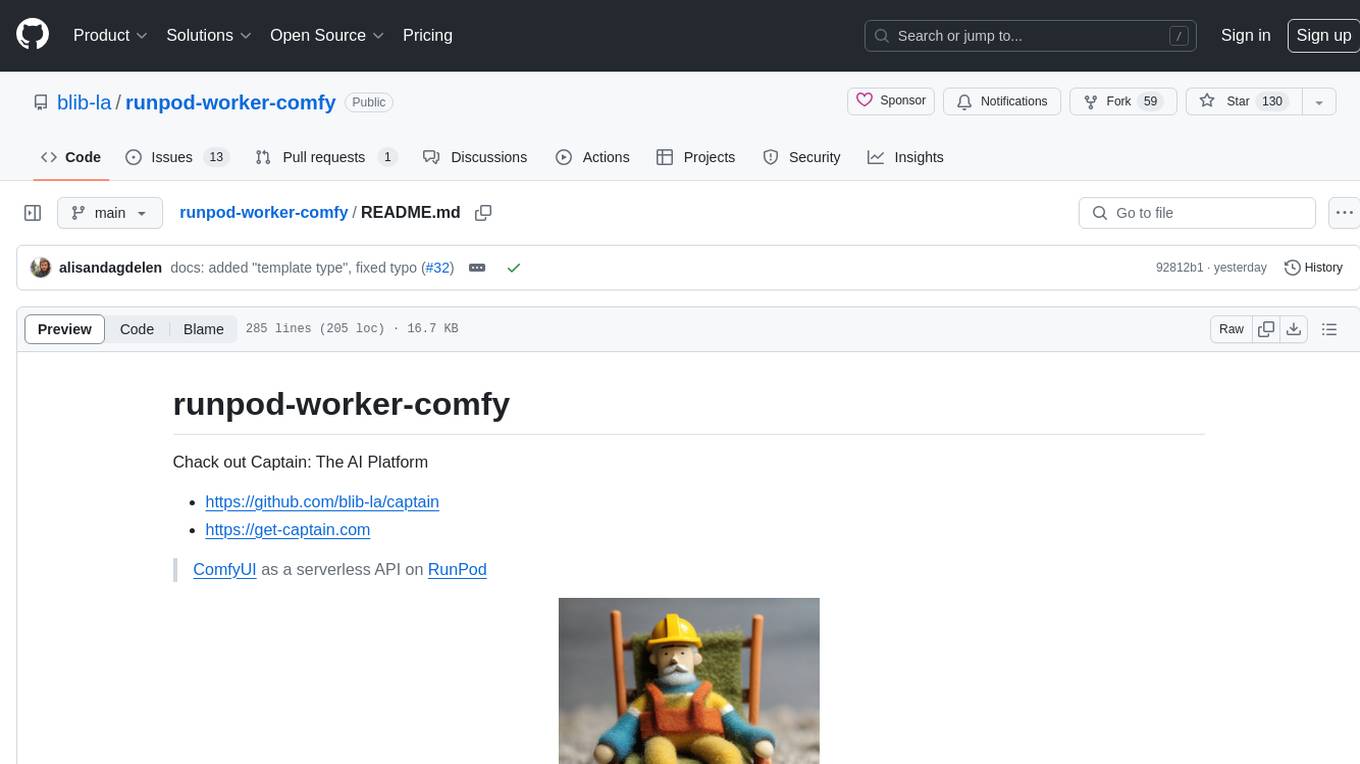
runpod-worker-comfy
runpod-worker-comfy is a serverless API tool that allows users to run any ComfyUI workflow to generate an image. Users can provide input images as base64-encoded strings, and the generated image can be returned as a base64-encoded string or uploaded to AWS S3. The tool is built on Ubuntu + NVIDIA CUDA and provides features like built-in checkpoints and VAE models. Users can configure environment variables to upload images to AWS S3 and interact with the RunPod API to generate images. The tool also supports local testing and deployment to Docker hub using Github Actions.
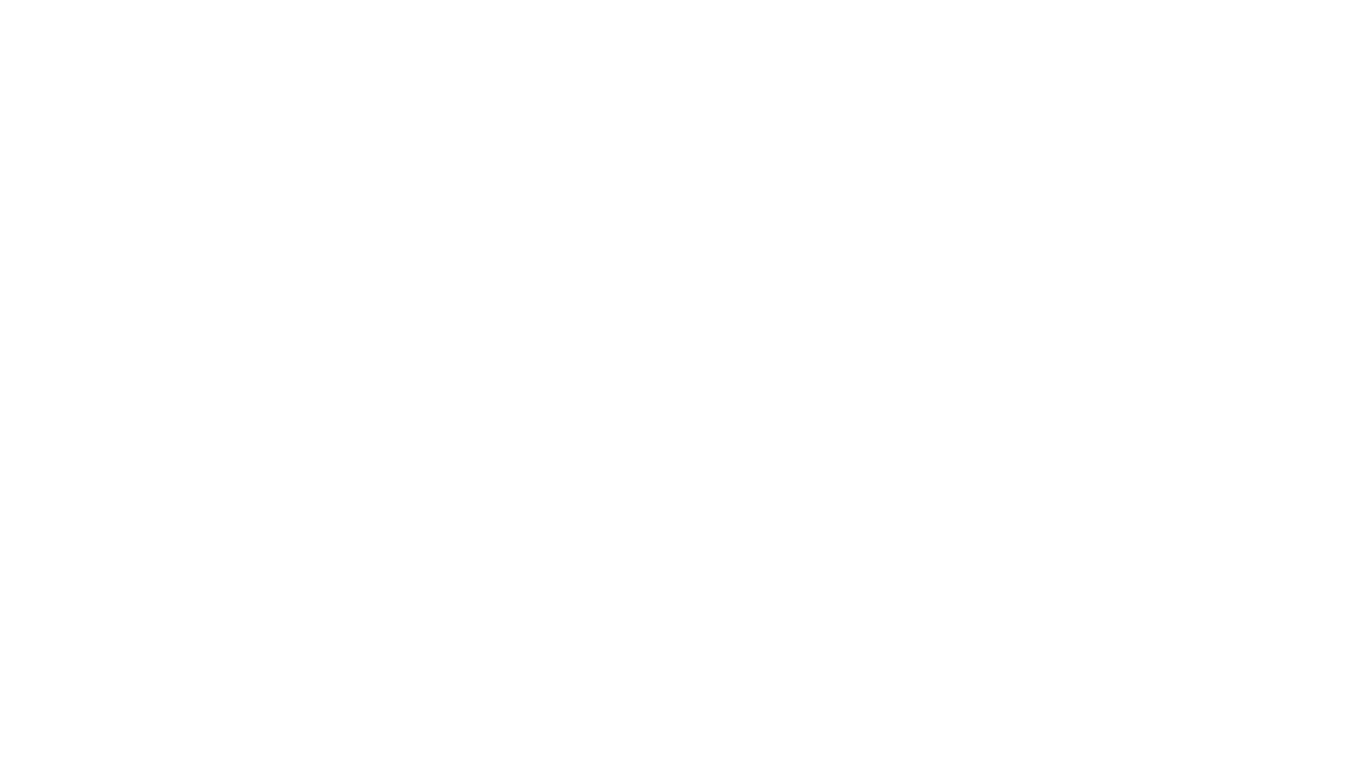
upgini
Upgini is an intelligent data search engine with a Python library that helps users find and add relevant features to their ML pipeline from various public, community, and premium external data sources. It automates the optimization of connected data sources by generating an optimal set of machine learning features using large language models, GraphNNs, and recurrent neural networks. The tool aims to simplify feature search and enrichment for external data to make it a standard approach in machine learning pipelines. It democratizes access to data sources for the data science community.
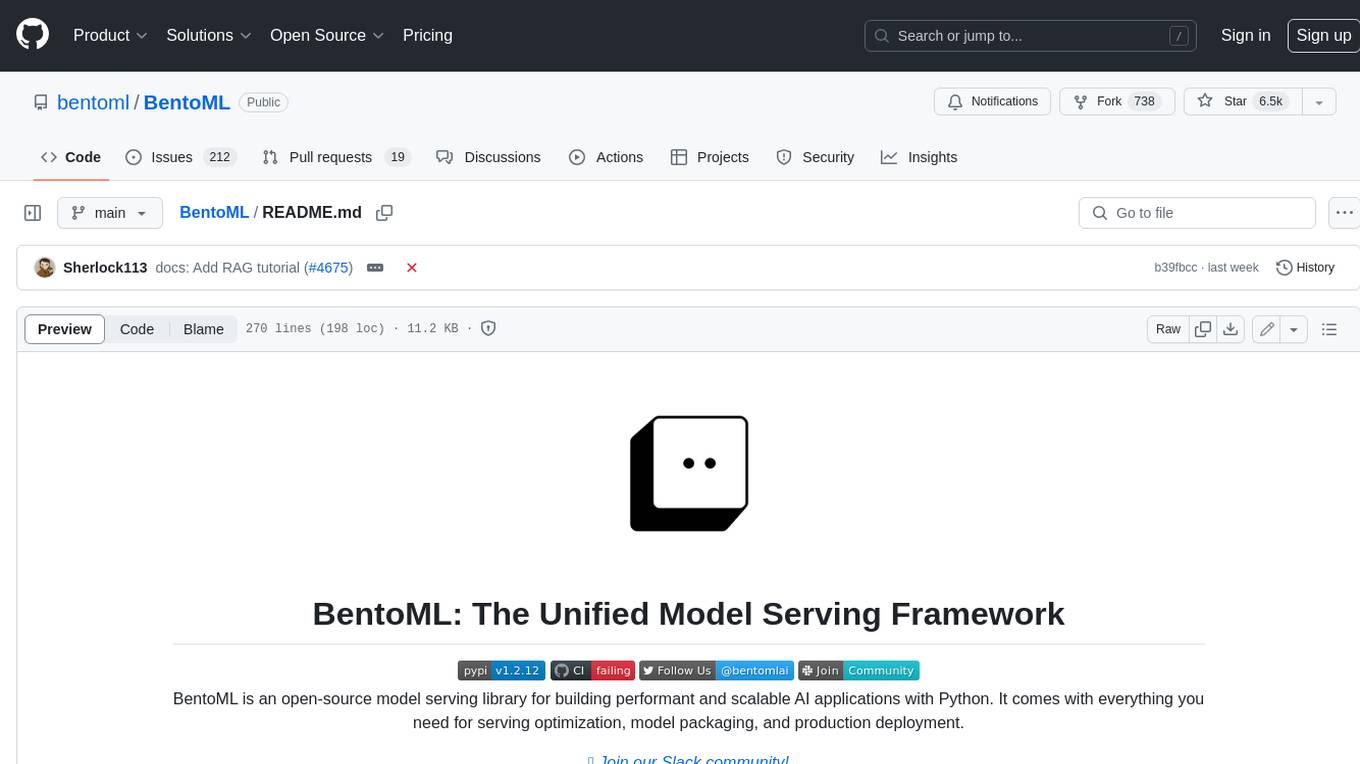
BentoML
BentoML is an open-source model serving library for building performant and scalable AI applications with Python. It comes with everything you need for serving optimization, model packaging, and production deployment.
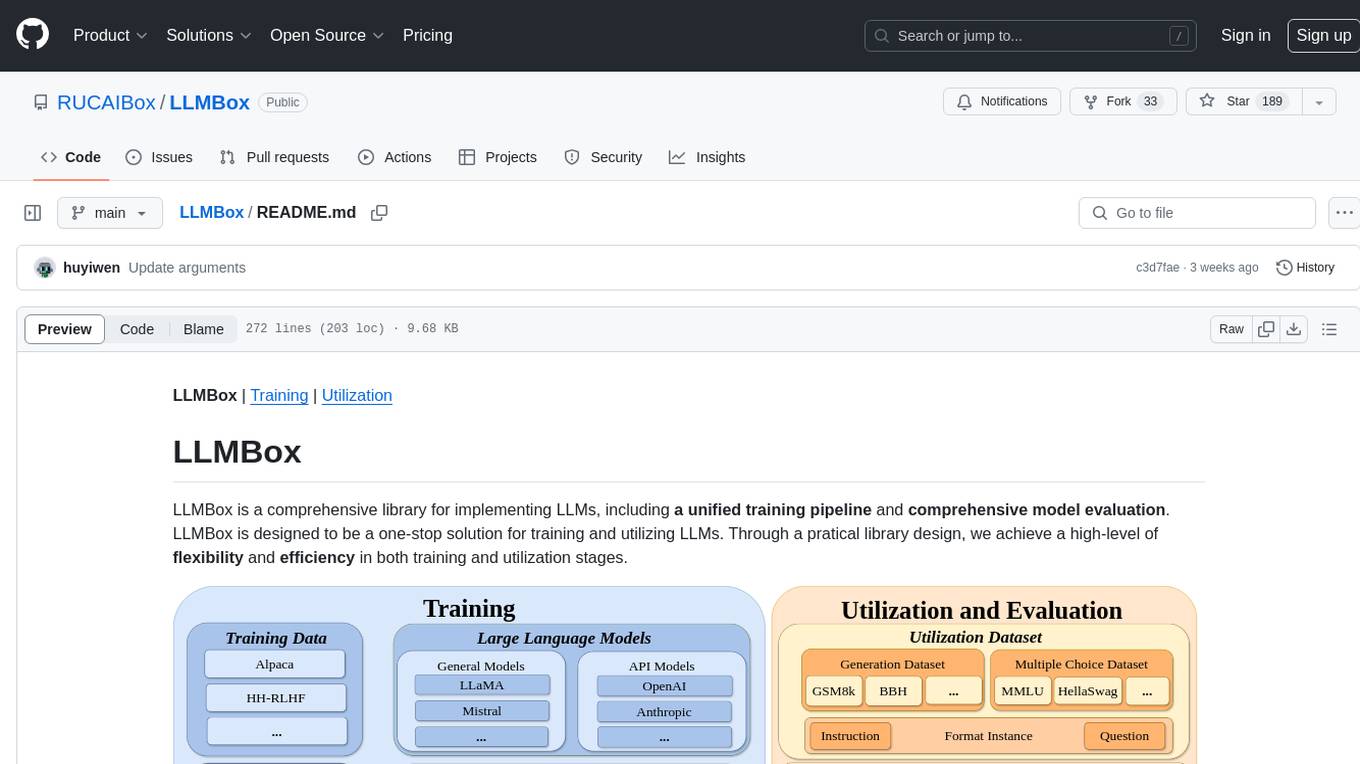
LLMBox
LLMBox is a comprehensive library designed for implementing Large Language Models (LLMs) with a focus on a unified training pipeline and comprehensive model evaluation. It serves as a one-stop solution for training and utilizing LLMs, offering flexibility and efficiency in both training and utilization stages. The library supports diverse training strategies, comprehensive datasets, tokenizer vocabulary merging, data construction strategies, parameter efficient fine-tuning, and efficient training methods. For utilization, LLMBox provides comprehensive evaluation on various datasets, in-context learning strategies, chain-of-thought evaluation, evaluation methods, prefix caching for faster inference, support for specific LLM models like vLLM and Flash Attention, and quantization options. The tool is suitable for researchers and developers working with LLMs for natural language processing tasks.
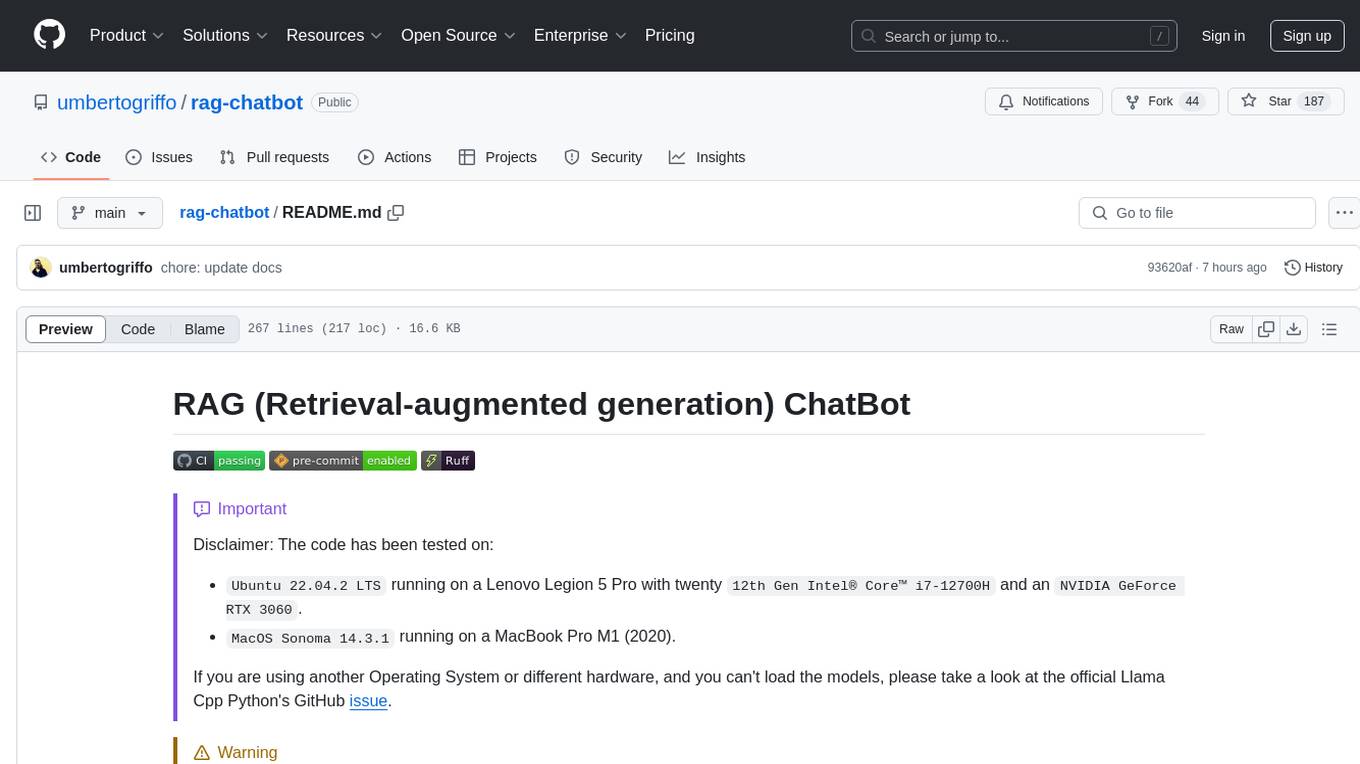
rag-chatbot
The RAG ChatBot project combines Lama.cpp, Chroma, and Streamlit to build a Conversation-aware Chatbot and a Retrieval-augmented generation (RAG) ChatBot. The RAG Chatbot works by taking a collection of Markdown files as input and provides answers based on the context provided by those files. It utilizes a Memory Builder component to load Markdown pages, divide them into sections, calculate embeddings, and save them in an embedding database. The chatbot retrieves relevant sections from the database, rewrites questions for optimal retrieval, and generates answers using a local language model. It also remembers previous interactions for more accurate responses. Various strategies are implemented to deal with context overflows, including creating and refining context, hierarchical summarization, and async hierarchical summarization.
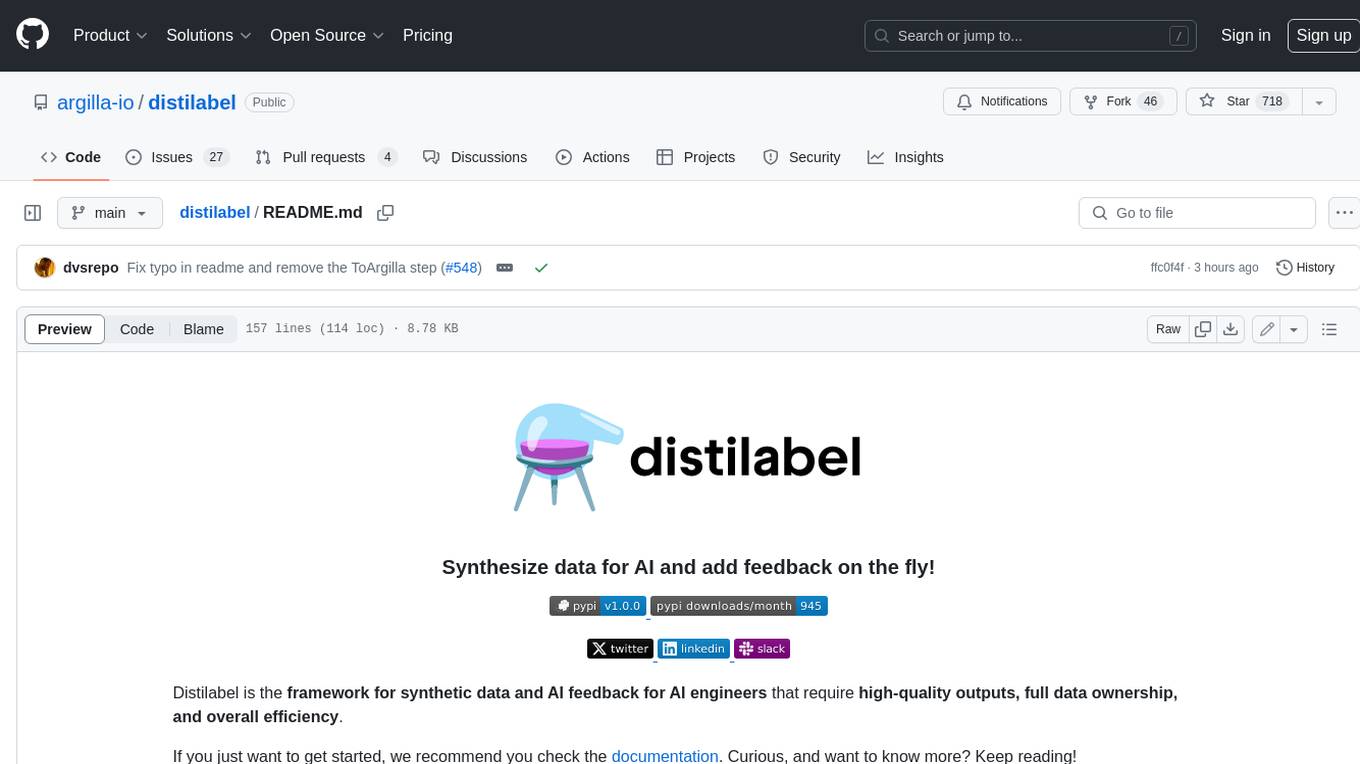
distilabel
Distilabel is a framework for synthetic data and AI feedback for AI engineers that require high-quality outputs, full data ownership, and overall efficiency. It helps you synthesize data and provide AI feedback to improve the quality of your AI models. With Distilabel, you can: * **Synthesize data:** Generate synthetic data to train your AI models. This can help you to overcome the challenges of data scarcity and bias. * **Provide AI feedback:** Get feedback from AI models on your data. This can help you to identify errors and improve the quality of your data. * **Improve your AI output quality:** By using Distilabel to synthesize data and provide AI feedback, you can improve the quality of your AI models and get better results.
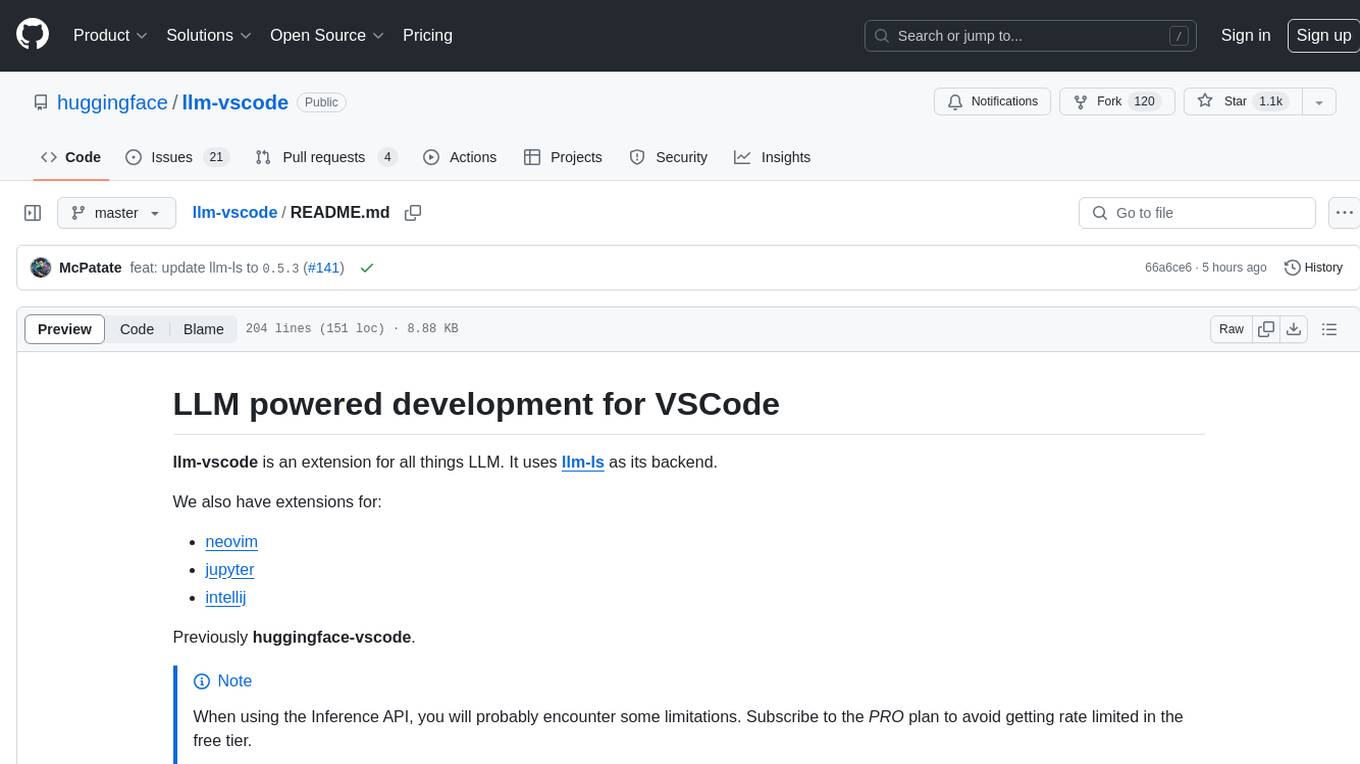
llm-vscode
llm-vscode is an extension designed for all things LLM, utilizing llm-ls as its backend. It offers features such as code completion with 'ghost-text' suggestions, the ability to choose models for code generation via HTTP requests, ensuring prompt size fits within the context window, and code attribution checks. Users can configure the backend, suggestion behavior, keybindings, llm-ls settings, and tokenization options. Additionally, the extension supports testing models like Code Llama 13B, Phind/Phind-CodeLlama-34B-v2, and WizardLM/WizardCoder-Python-34B-V1.0. Development involves cloning llm-ls, building it, and setting up the llm-vscode extension for use.
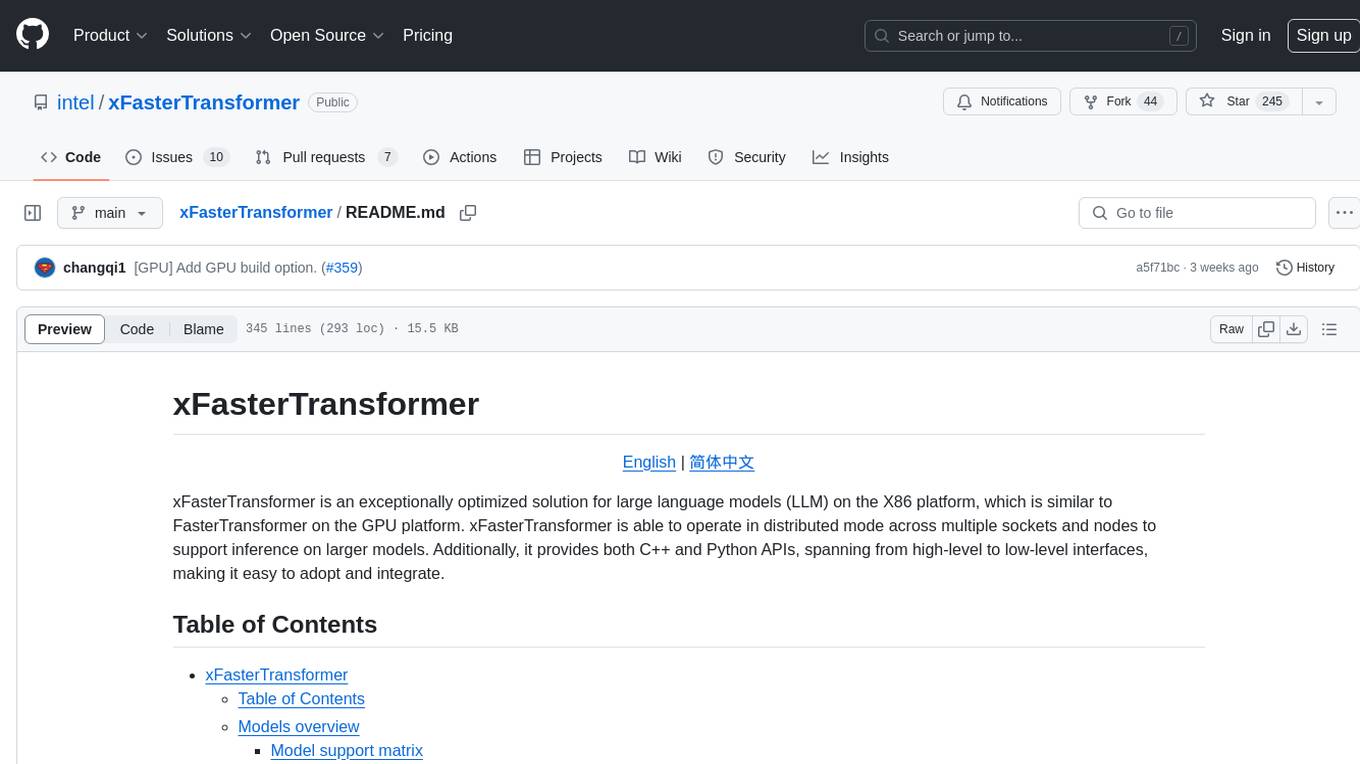
xFasterTransformer
xFasterTransformer is an optimized solution for Large Language Models (LLMs) on the X86 platform, providing high performance and scalability for inference on mainstream LLM models. It offers C++ and Python APIs for easy integration, along with example codes and benchmark scripts. Users can prepare models in a different format, convert them, and use the APIs for tasks like encoding input prompts, generating token ids, and serving inference requests. The tool supports various data types and models, and can run in single or multi-rank modes using MPI. A web demo based on Gradio is available for popular LLM models like ChatGLM and Llama2. Benchmark scripts help evaluate model inference performance quickly, and MLServer enables serving with REST and gRPC interfaces.
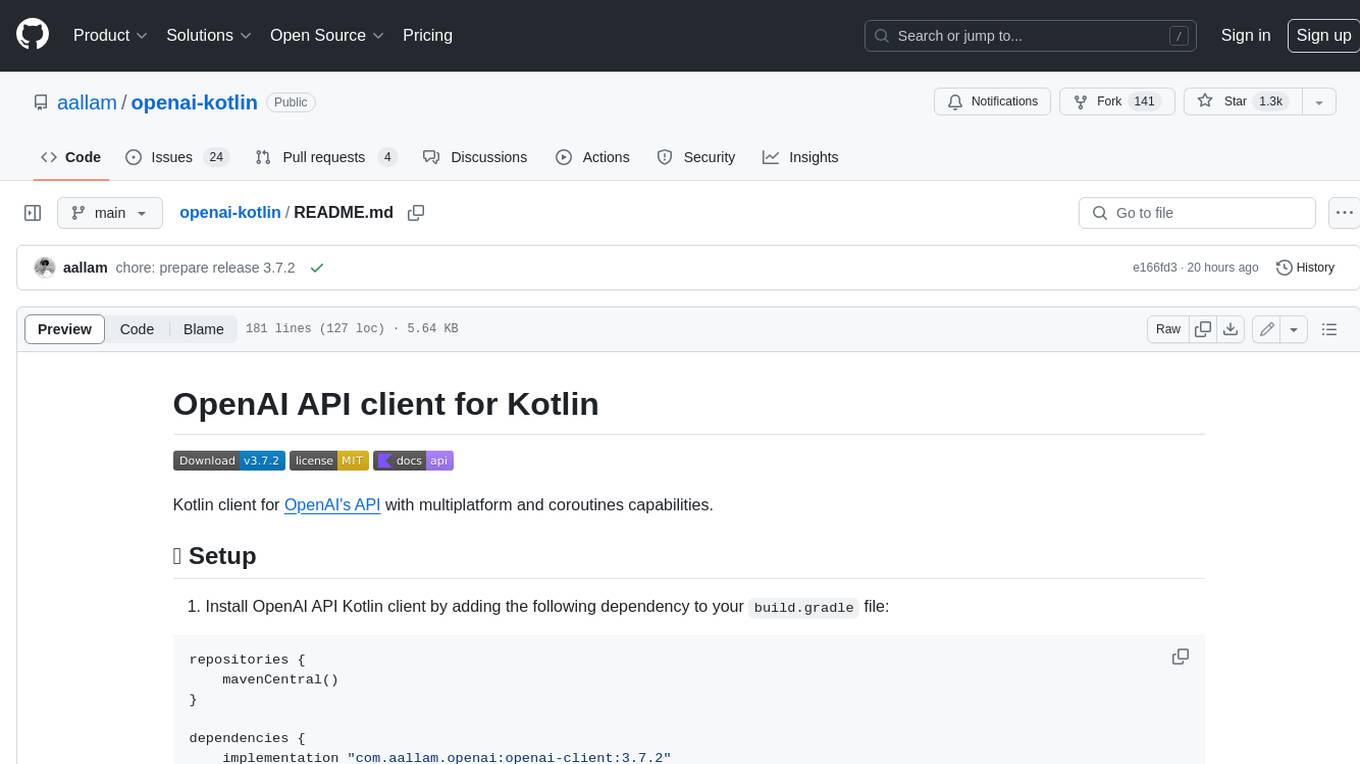
openai-kotlin
OpenAI Kotlin API client is a Kotlin client for OpenAI's API with multiplatform and coroutines capabilities. It allows users to interact with OpenAI's API using Kotlin programming language. The client supports various features such as models, chat, images, embeddings, files, fine-tuning, moderations, audio, assistants, threads, messages, and runs. It also provides guides on getting started, chat & function call, file source guide, and assistants. Sample apps are available for reference, and troubleshooting guides are provided for common issues. The project is open-source and licensed under the MIT license, allowing contributions from the community.
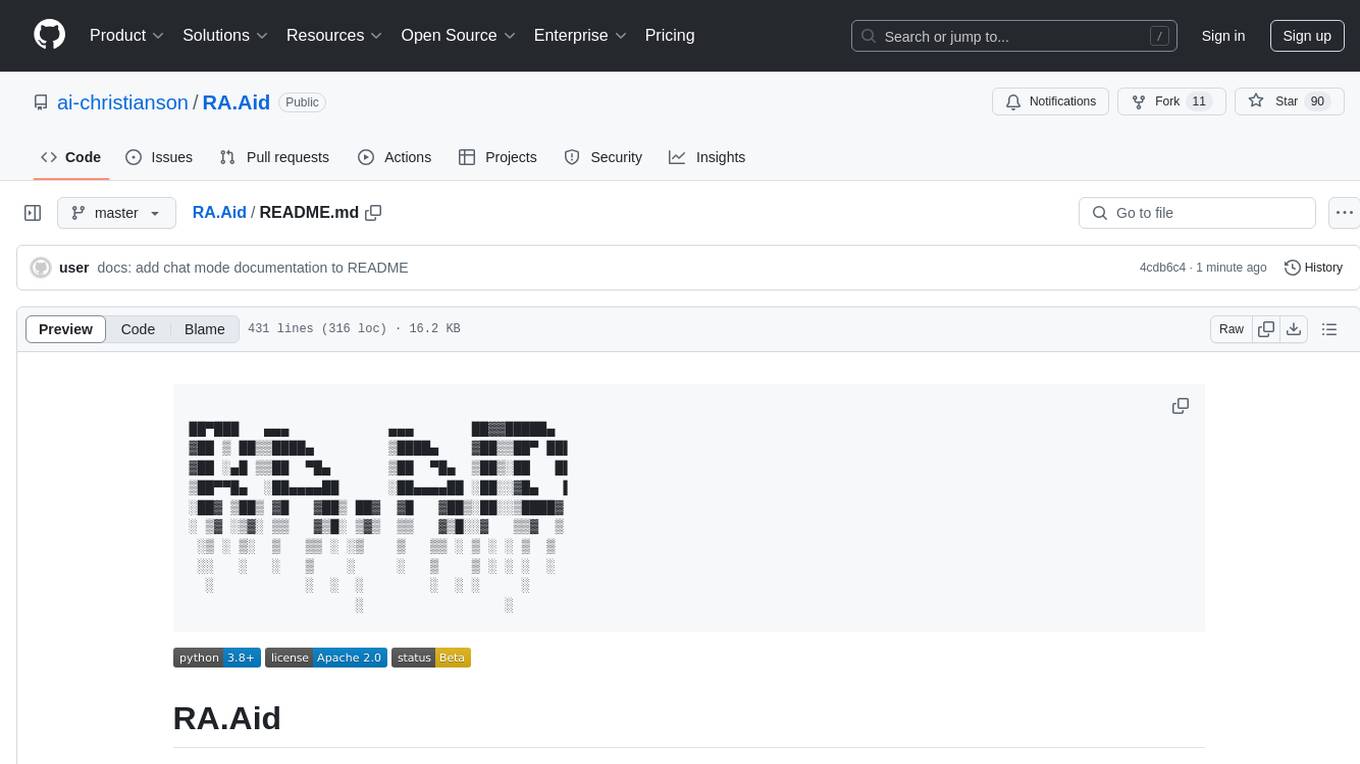
RA.Aid
RA.Aid is an AI software development agent powered by `aider` and advanced reasoning models like `o1`. It combines `aider`'s code editing capabilities with LangChain's agent-based task execution framework to provide an intelligent assistant for research, planning, and implementation of multi-step development tasks. It handles complex programming tasks by breaking them down into manageable steps, running shell commands automatically, and leveraging expert reasoning models like OpenAI's o1. RA.Aid is designed for everyday software development, offering features such as multi-step task planning, automated command execution, and the ability to handle complex programming tasks beyond single-shot code edits.
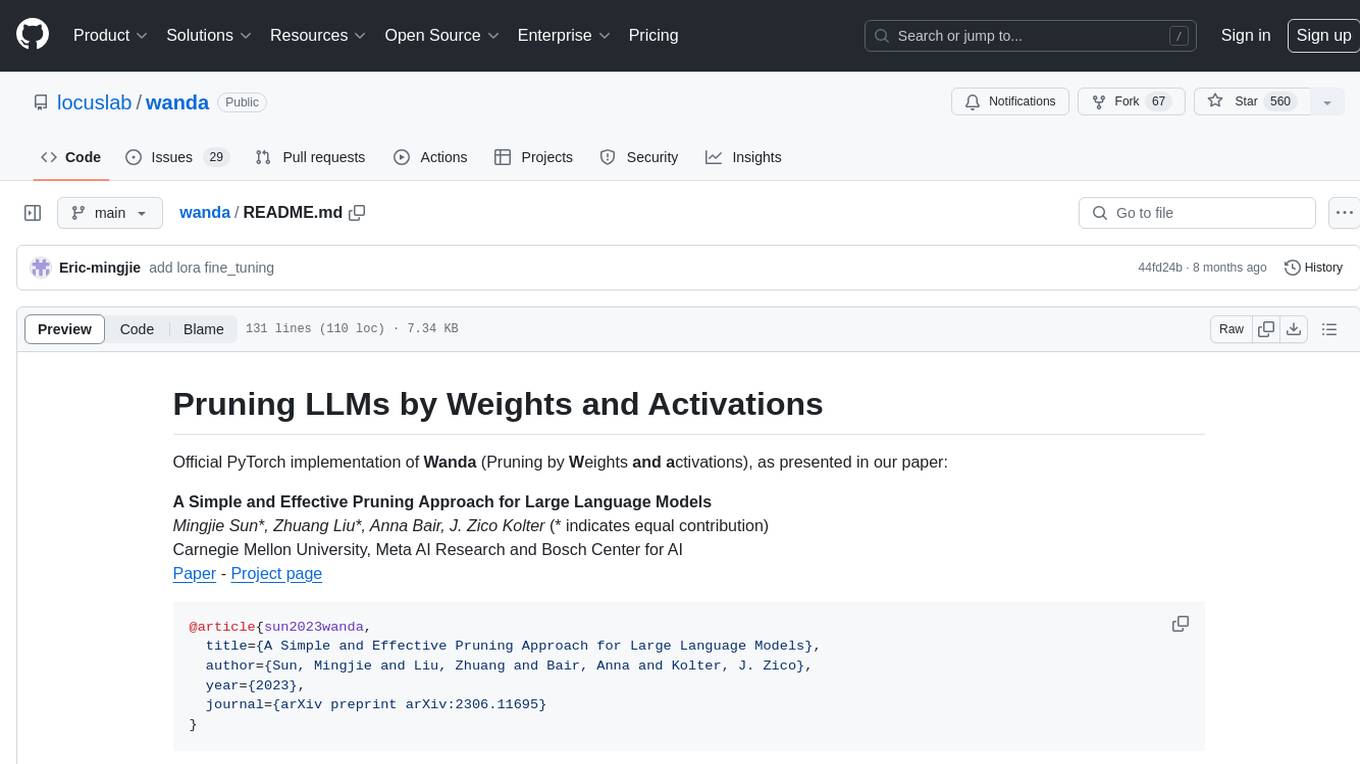
wanda
Official PyTorch implementation of Wanda (Pruning by Weights and Activations), a simple and effective pruning approach for large language models. The pruning approach removes weights on a per-output basis, by the product of weight magnitudes and input activation norms. The repository provides support for various features such as LLaMA-2, ablation study on OBS weight update, zero-shot evaluation, and speedup evaluation. Users can replicate main results from the paper using provided bash commands. The tool aims to enhance the efficiency and performance of language models through structured and unstructured sparsity techniques.
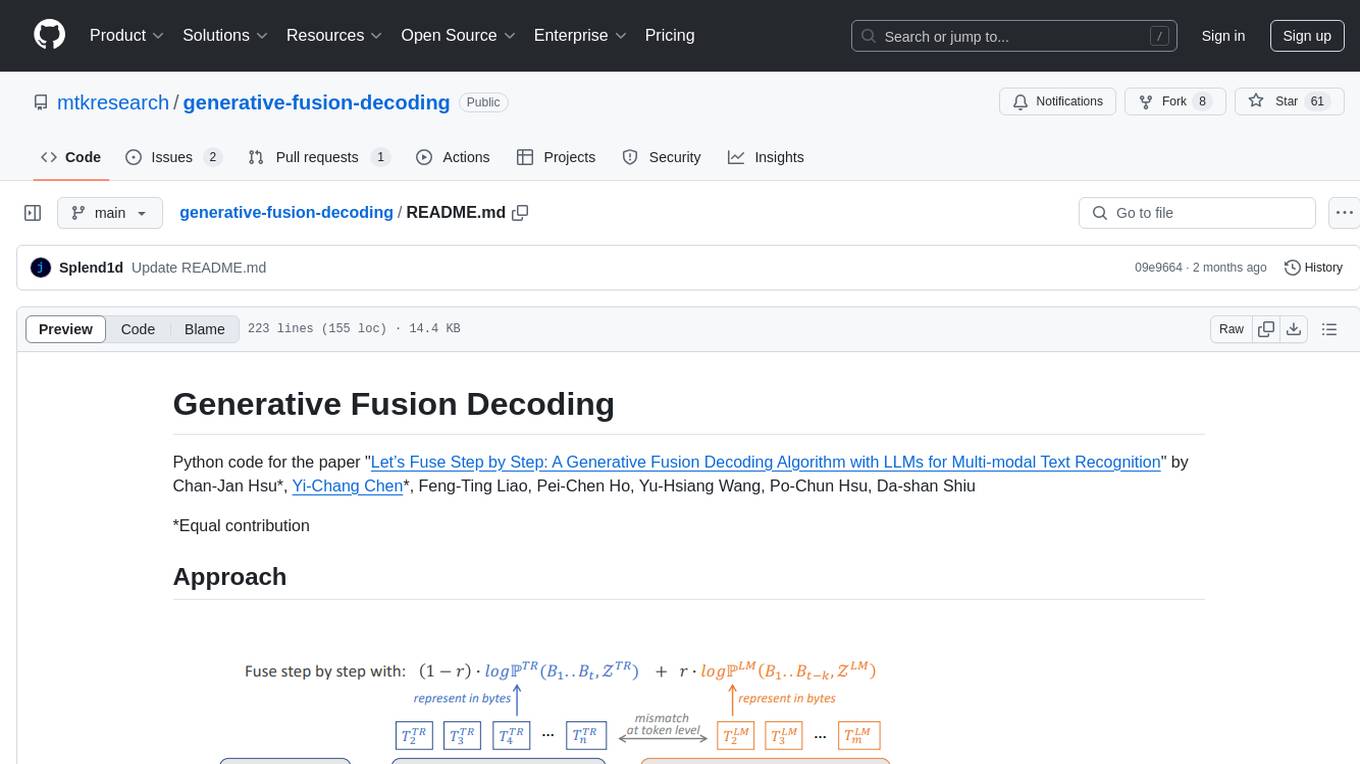
generative-fusion-decoding
Generative Fusion Decoding (GFD) is a novel shallow fusion framework that integrates Large Language Models (LLMs) into multi-modal text recognition systems such as automatic speech recognition (ASR) and optical character recognition (OCR). GFD operates across mismatched token spaces of different models by mapping text token space to byte token space, enabling seamless fusion during the decoding process. It simplifies the complexity of aligning different model sample spaces, allows LLMs to correct errors in tandem with the recognition model, increases robustness in long-form speech recognition, and enables fusing recognition models deficient in Chinese text recognition with LLMs extensively trained on Chinese. GFD significantly improves performance in ASR and OCR tasks, offering a unified solution for leveraging existing pre-trained models through step-by-step fusion.
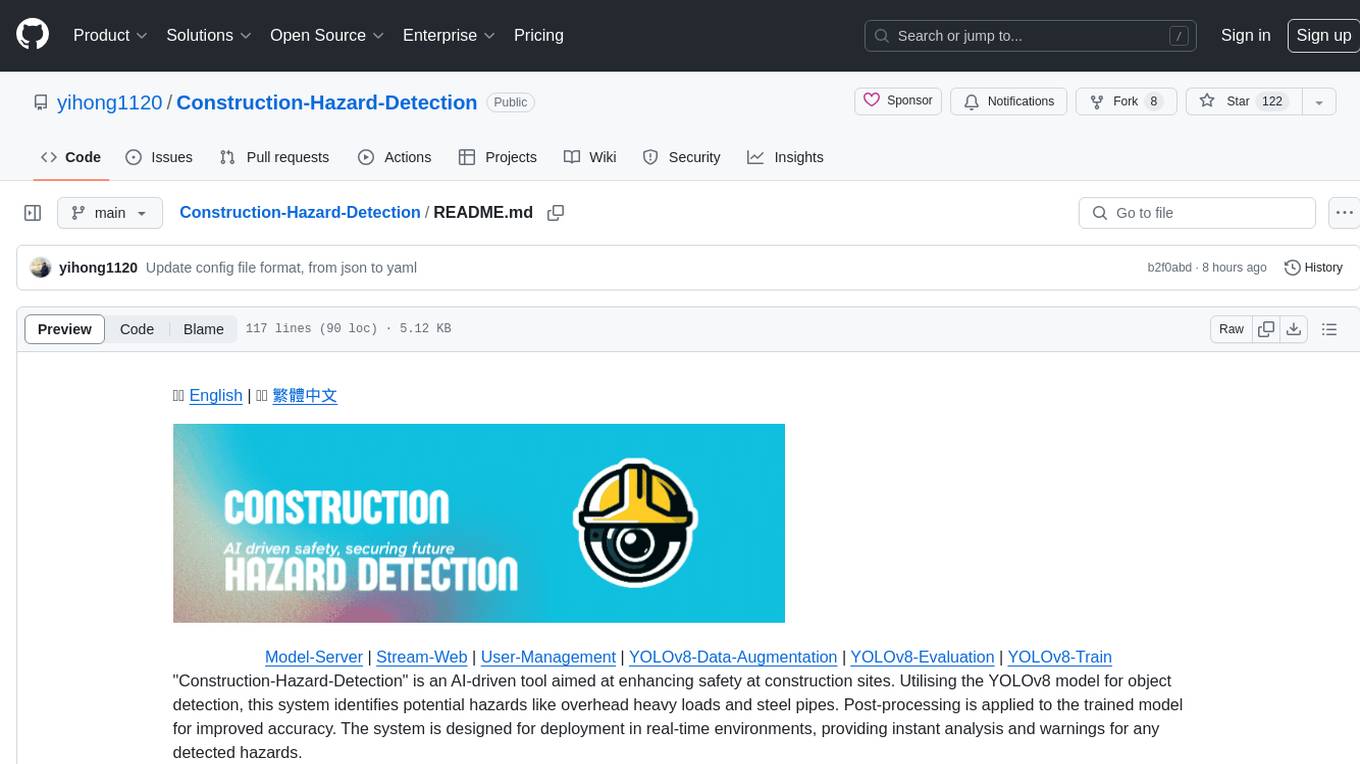
Construction-Hazard-Detection
Construction-Hazard-Detection is an AI-driven tool focused on improving safety at construction sites by utilizing the YOLOv8 model for object detection. The system identifies potential hazards like overhead heavy loads and steel pipes, providing real-time analysis and warnings. Users can configure the system via a YAML file and run it using Docker. The primary dataset used for training is the Construction Site Safety Image Dataset enriched with additional annotations. The system logs are accessible within the Docker container for debugging, and notifications are sent through the LINE messaging API when hazards are detected.
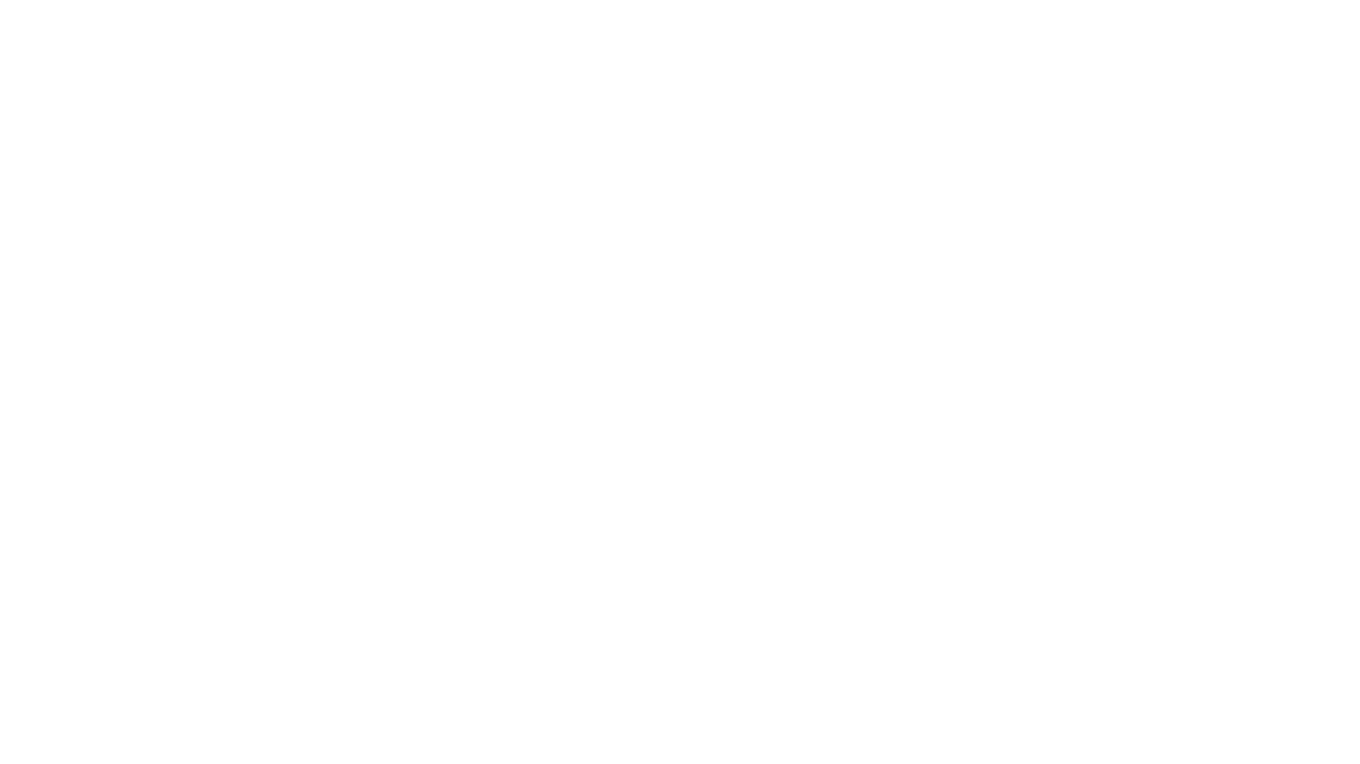
rclip
rclip is a command-line photo search tool powered by the OpenAI's CLIP neural network. It allows users to search for images using text queries, similar image search, and combining multiple queries. The tool extracts features from photos to enable searching and indexing, with options for previewing results in supported terminals or custom viewers. Users can install rclip on Linux, macOS, and Windows using different installation methods. The repository follows the Conventional Commits standard and welcomes contributions from the community.
For similar tasks
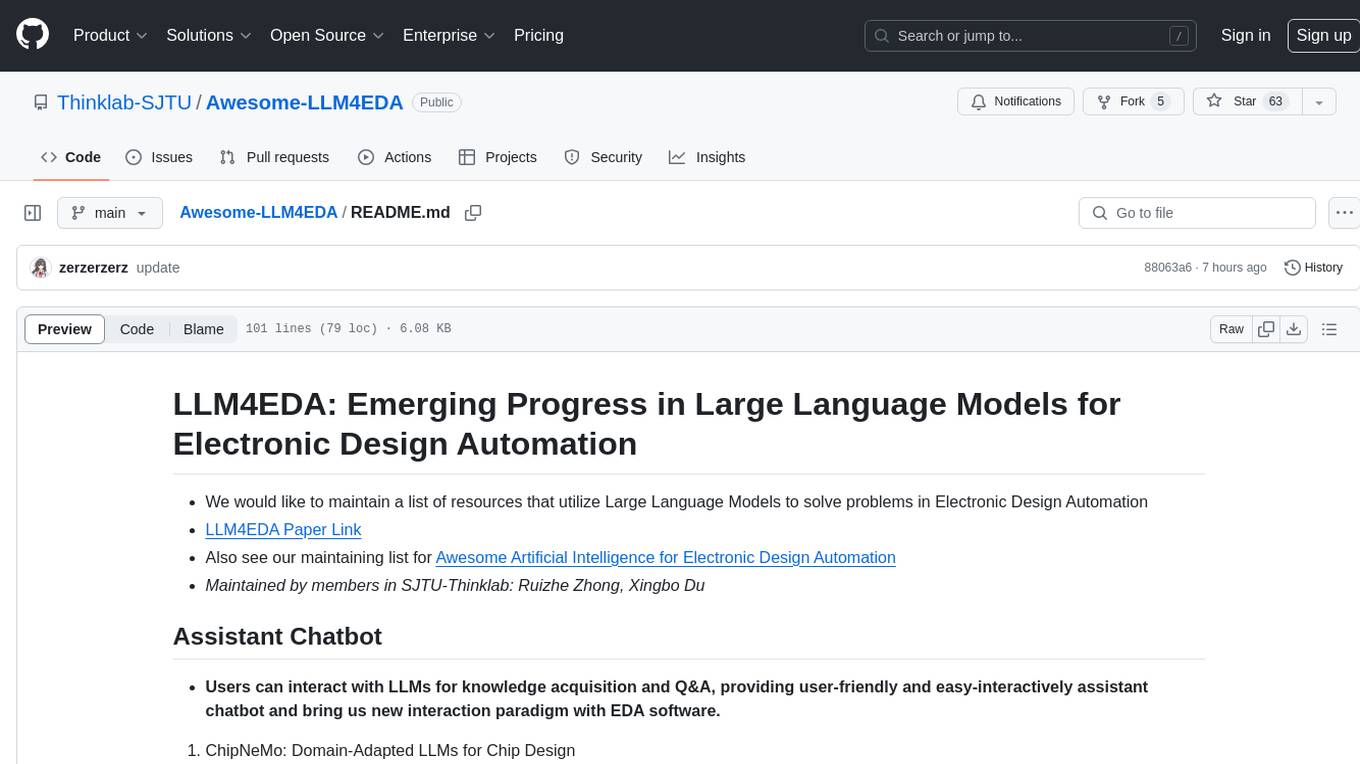
Awesome-LLM4EDA
LLM4EDA is a repository dedicated to showcasing the emerging progress in utilizing Large Language Models for Electronic Design Automation. The repository includes resources, papers, and tools that leverage LLMs to solve problems in EDA. It covers a wide range of applications such as knowledge acquisition, code generation, code analysis, verification, and large circuit models. The goal is to provide a comprehensive understanding of how LLMs can revolutionize the EDA industry by offering innovative solutions and new interaction paradigms.
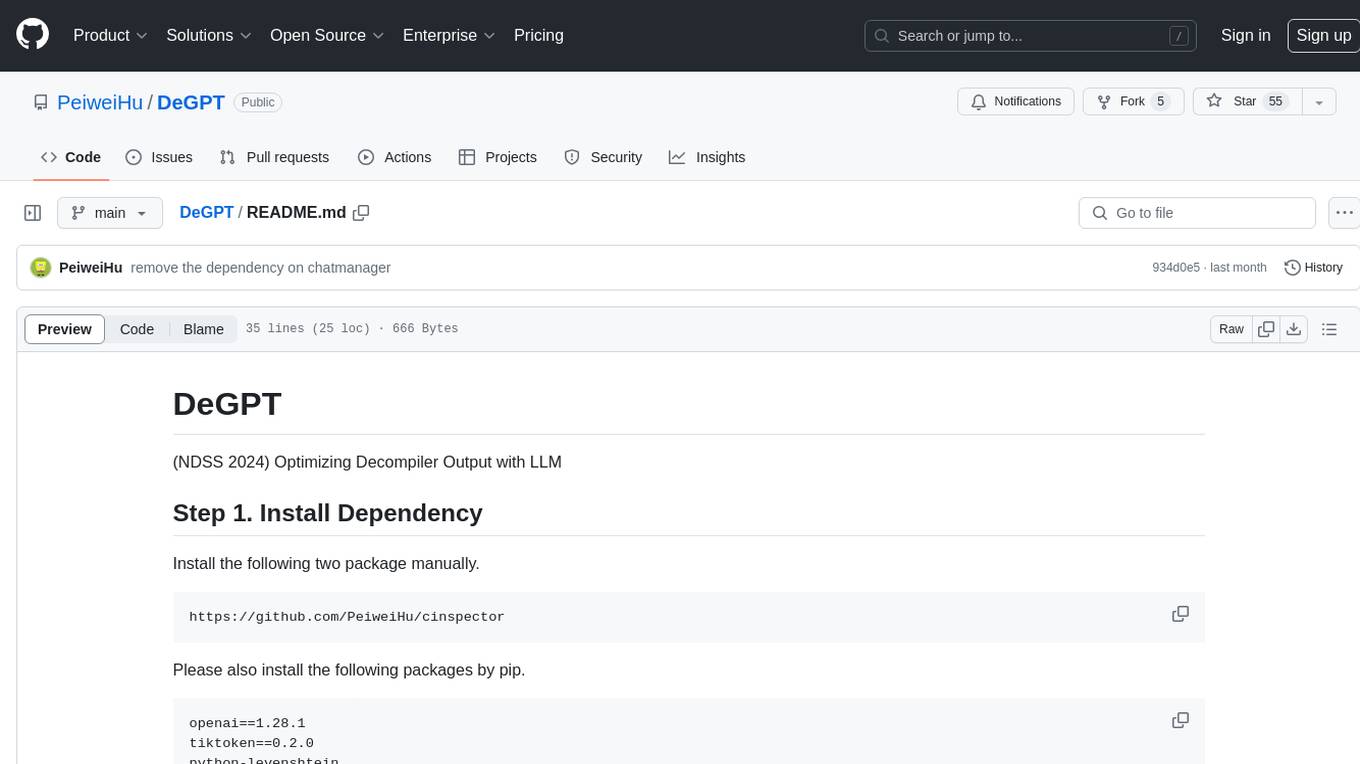
DeGPT
DeGPT is a tool designed to optimize decompiler output using Large Language Models (LLM). It requires manual installation of specific packages and setting up API key for OpenAI. The tool provides functionality to perform optimization on decompiler output by running specific scripts.
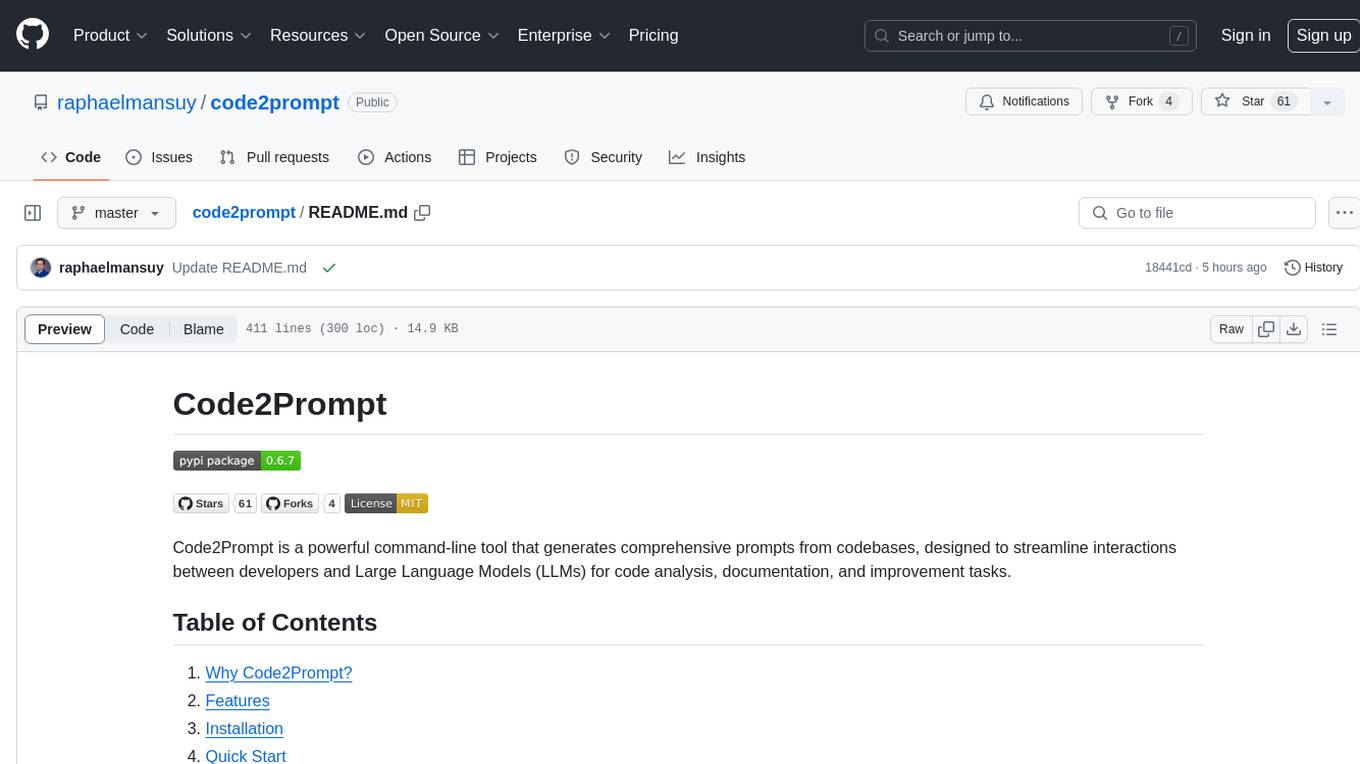
code2prompt
Code2Prompt is a powerful command-line tool that generates comprehensive prompts from codebases, designed to streamline interactions between developers and Large Language Models (LLMs) for code analysis, documentation, and improvement tasks. It bridges the gap between codebases and LLMs by converting projects into AI-friendly prompts, enabling users to leverage AI for various software development tasks. The tool offers features like holistic codebase representation, intelligent source tree generation, customizable prompt templates, smart token management, Gitignore integration, flexible file handling, clipboard-ready output, multiple output options, and enhanced code readability.
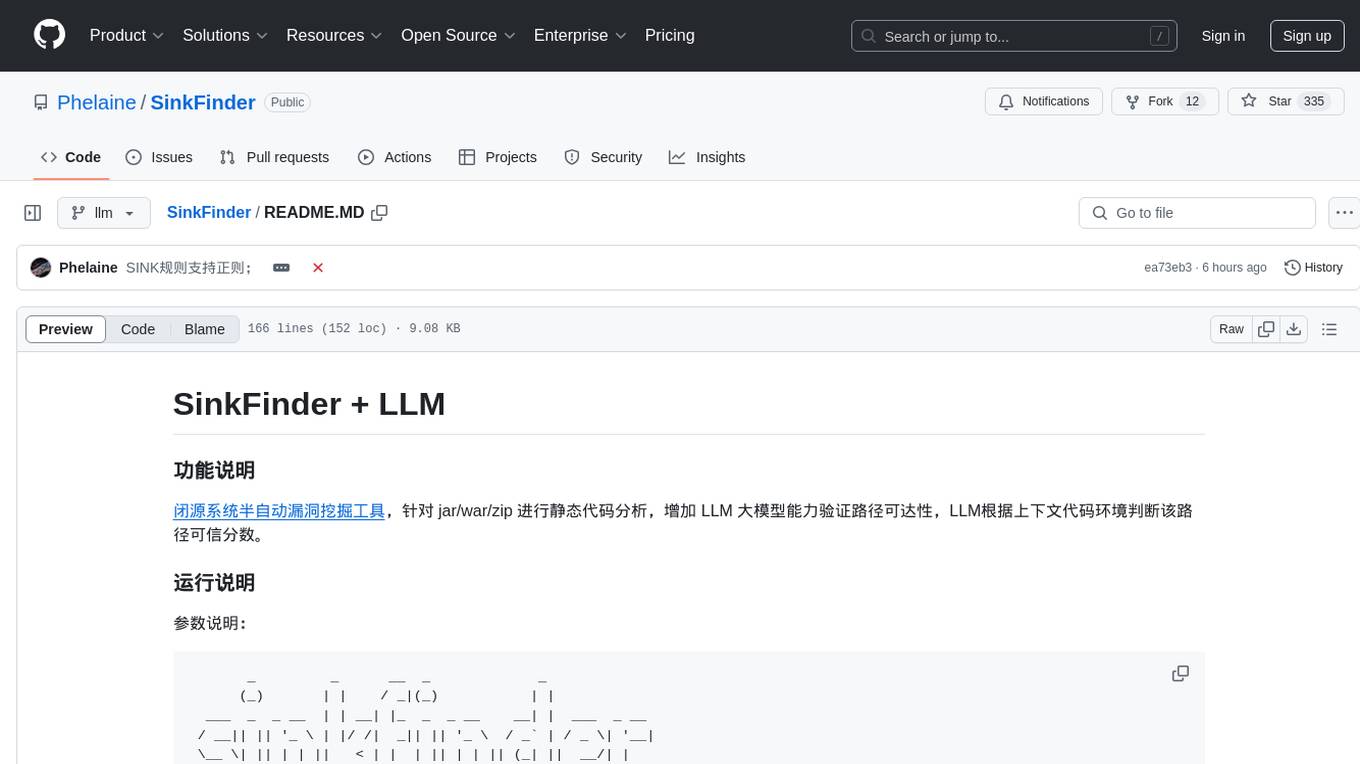
SinkFinder
SinkFinder + LLM is a closed-source semi-automatic vulnerability discovery tool that performs static code analysis on jar/war/zip files. It enhances the capability of LLM large models to verify path reachability and assess the trustworthiness score of the path based on the contextual code environment. Users can customize class and jar exclusions, depth of recursive search, and other parameters through command-line arguments. The tool generates rule.json configuration file after each run and requires configuration of the DASHSCOPE_API_KEY for LLM capabilities. The tool provides detailed logs on high-risk paths, LLM results, and other findings. Rules.json file contains sink rules for various vulnerability types with severity levels and corresponding sink methods.
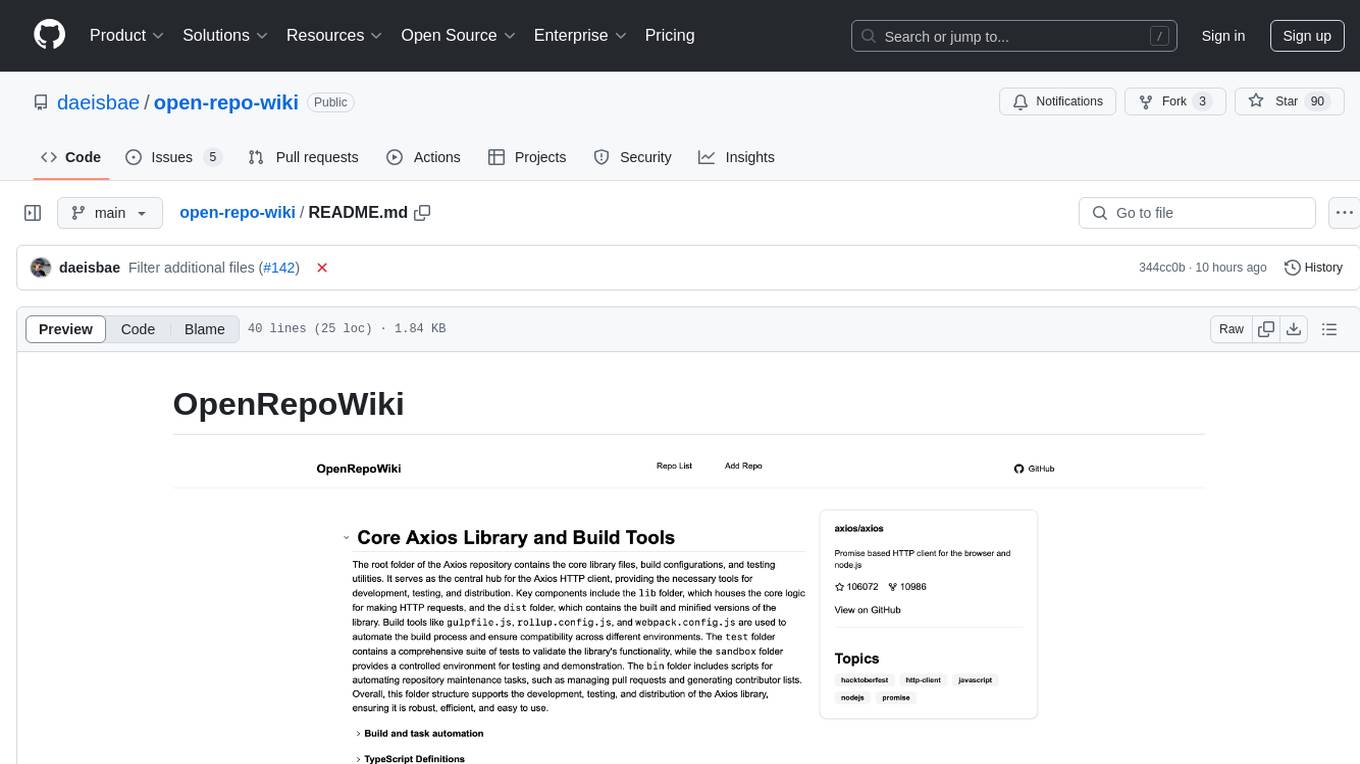
open-repo-wiki
OpenRepoWiki is a tool designed to automatically generate a comprehensive wiki page for any GitHub repository. It simplifies the process of understanding the purpose, functionality, and core components of a repository by analyzing its code structure, identifying key files and functions, and providing explanations. The tool aims to assist individuals who want to learn how to build various projects by providing a summarized overview of the repository's contents. OpenRepoWiki requires certain dependencies such as Google AI Studio or Deepseek API Key, PostgreSQL for storing repository information, Github API Key for accessing repository data, and Amazon S3 for optional usage. Users can configure the tool by setting up environment variables, installing dependencies, building the server, and running the application. It is recommended to consider the token usage and opt for cost-effective options when utilizing the tool.
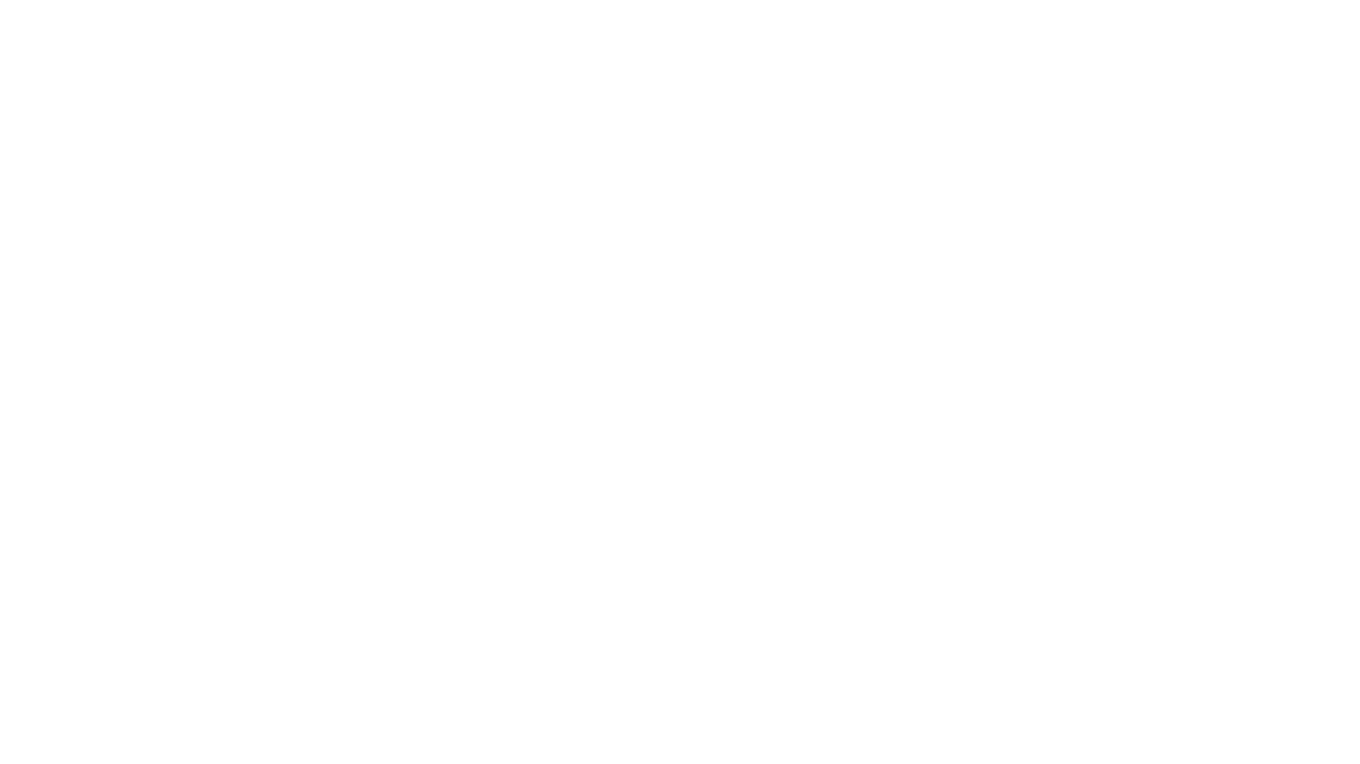
CodebaseToPrompt
CodebaseToPrompt is a simple tool that converts a local directory into a structured prompt for Large Language Models (LLMs). It allows users to select specific files for code review, analysis, or documentation by exploring and filtering through the file tree in a browser-based interface. The tool generates a formatted output that can be directly used with AI tools, provides token count estimates, and supports local storage for saving selections. Users can easily copy the selected files in the desired format for further use.
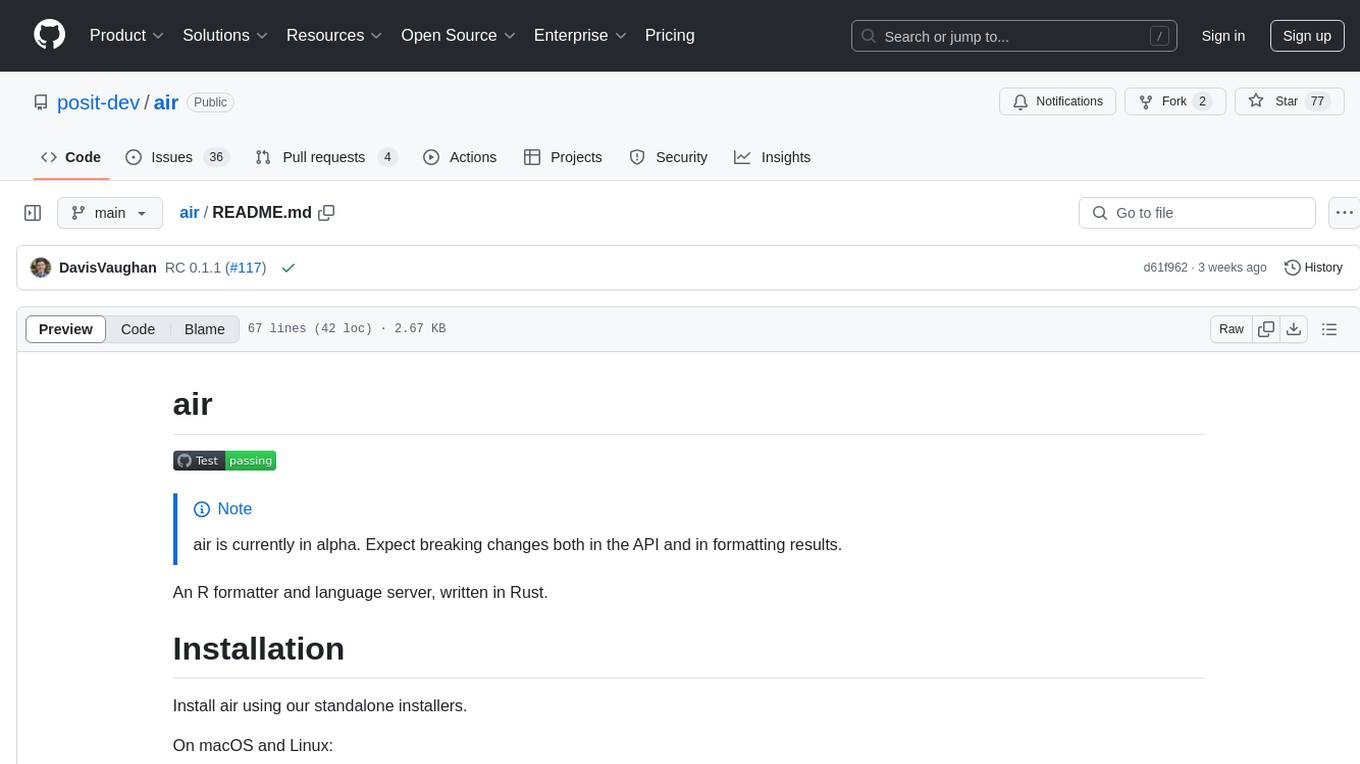
air
air is an R formatter and language server written in Rust. It is currently in alpha stage, so users should expect breaking changes in both the API and formatting results. The tool draws inspiration from various sources like roslyn, swift, rust-analyzer, prettier, biome, and ruff. It provides formatters and language servers, influenced by design decisions from these tools. Users can install air using standalone installers for macOS, Linux, and Windows, which automatically add air to the PATH. Developers can also install the dev version of the air CLI and VS Code extension for further customization and development.
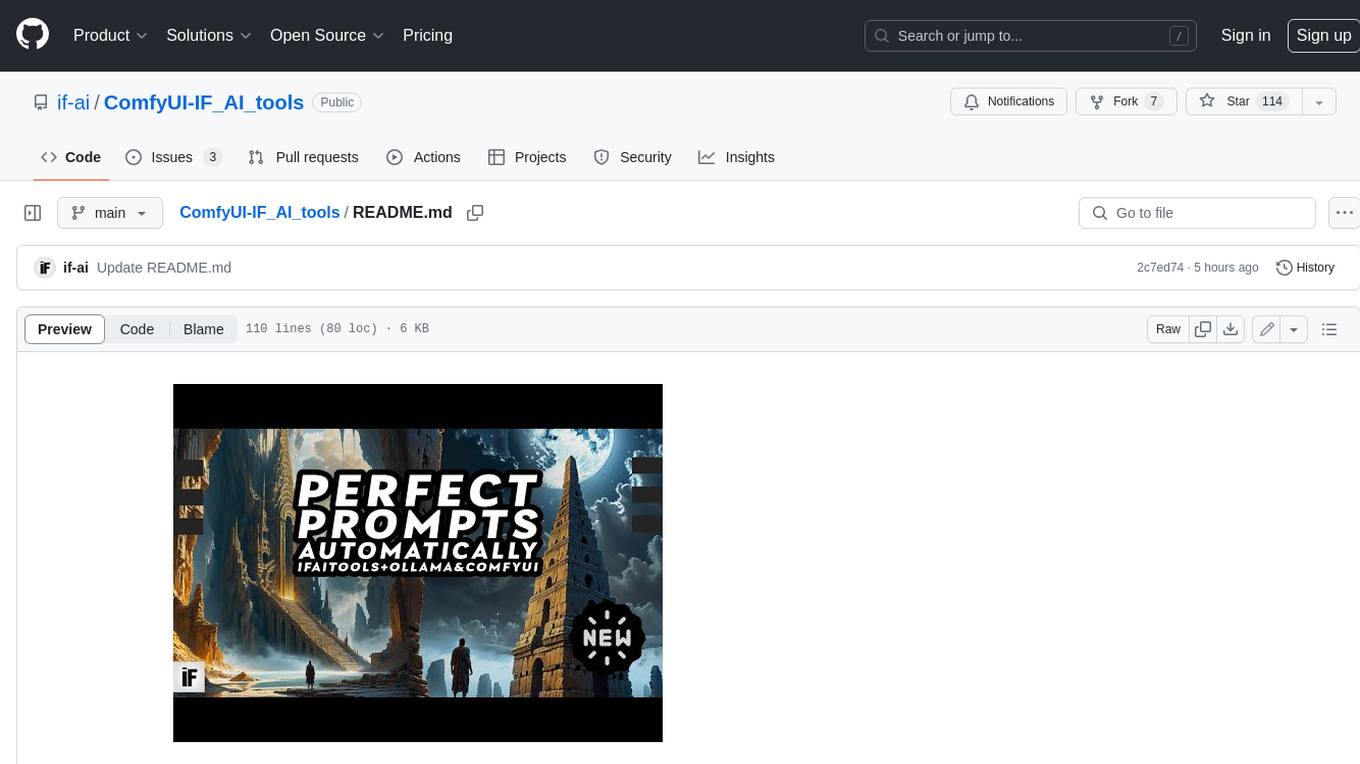
ComfyUI-IF_AI_tools
ComfyUI-IF_AI_tools is a set of custom nodes for ComfyUI that allows you to generate prompts using a local Large Language Model (LLM) via Ollama. This tool enables you to enhance your image generation workflow by leveraging the power of language models.
For similar jobs
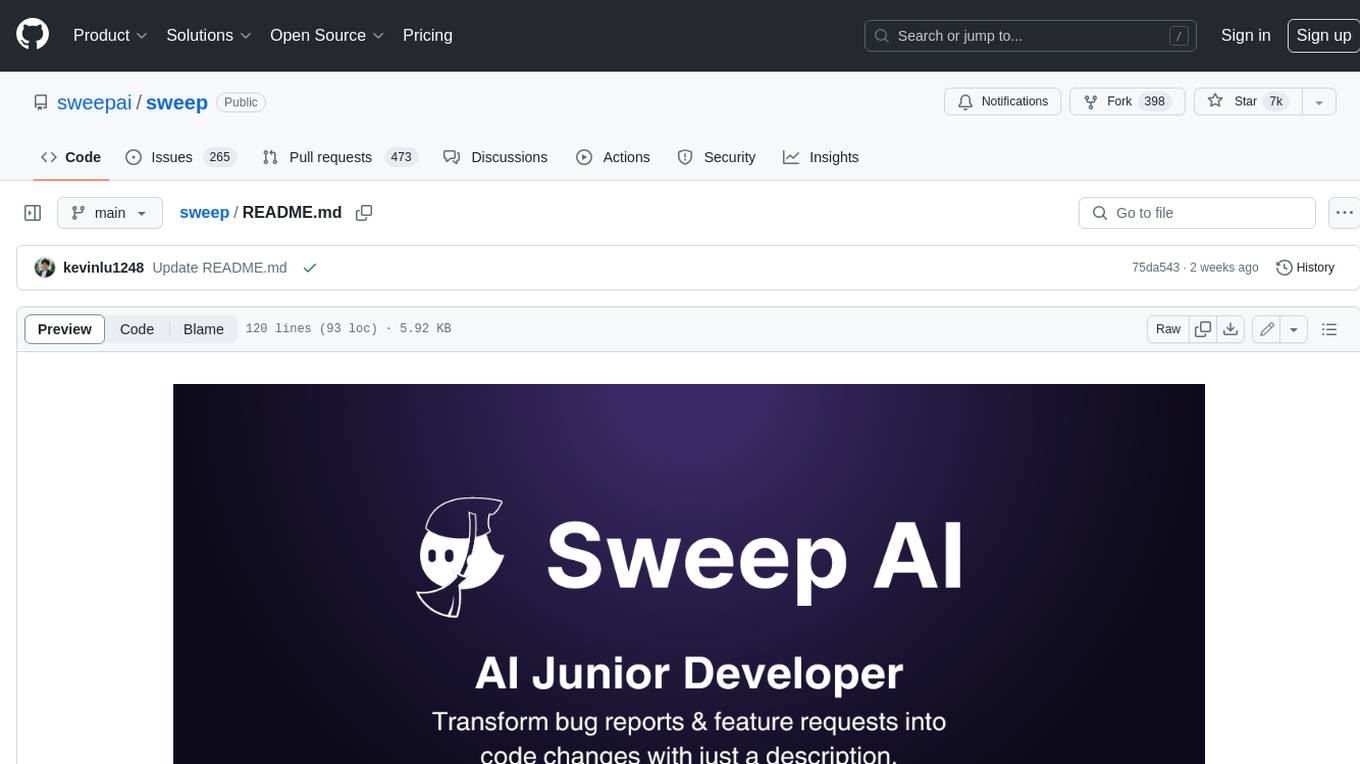
sweep
Sweep is an AI junior developer that turns bugs and feature requests into code changes. It automatically handles developer experience improvements like adding type hints and improving test coverage.
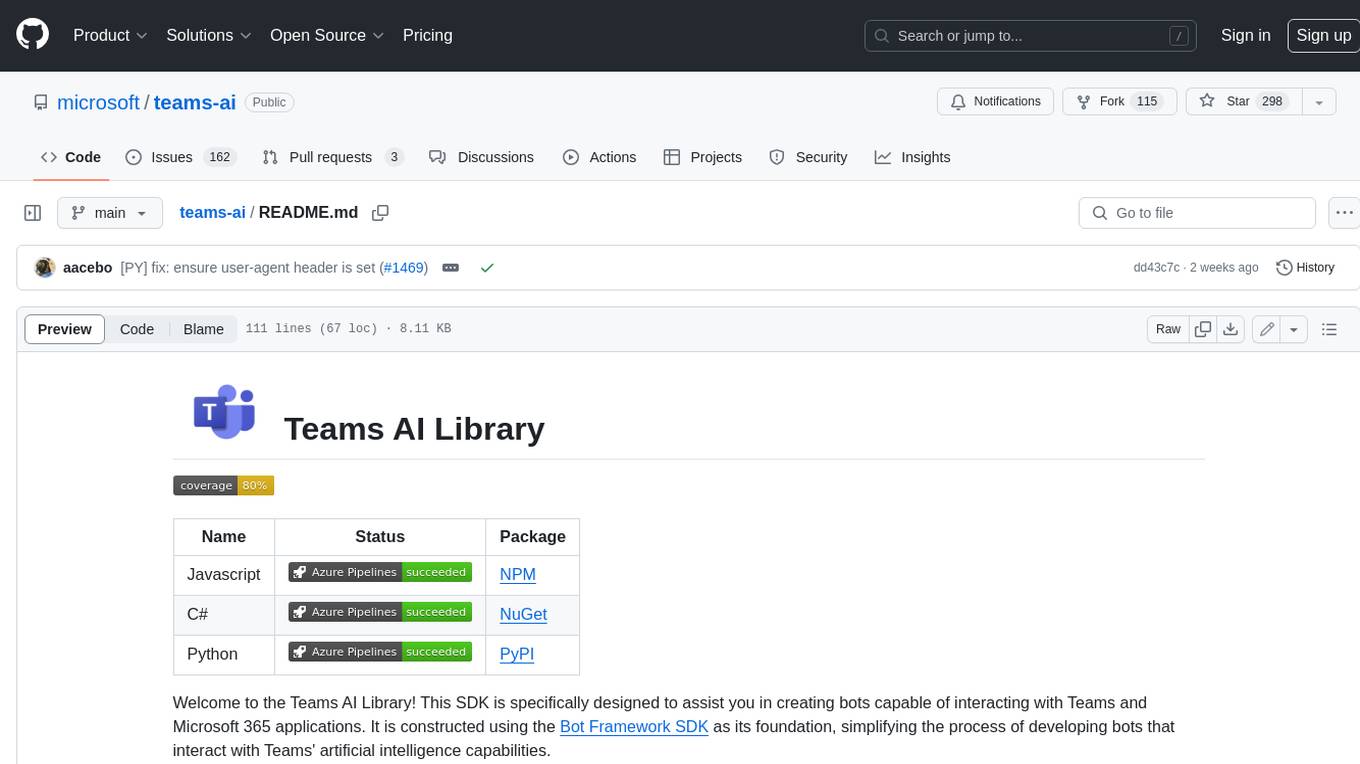
teams-ai
The Teams AI Library is a software development kit (SDK) that helps developers create bots that can interact with Teams and Microsoft 365 applications. It is built on top of the Bot Framework SDK and simplifies the process of developing bots that interact with Teams' artificial intelligence capabilities. The SDK is available for JavaScript/TypeScript, .NET, and Python.
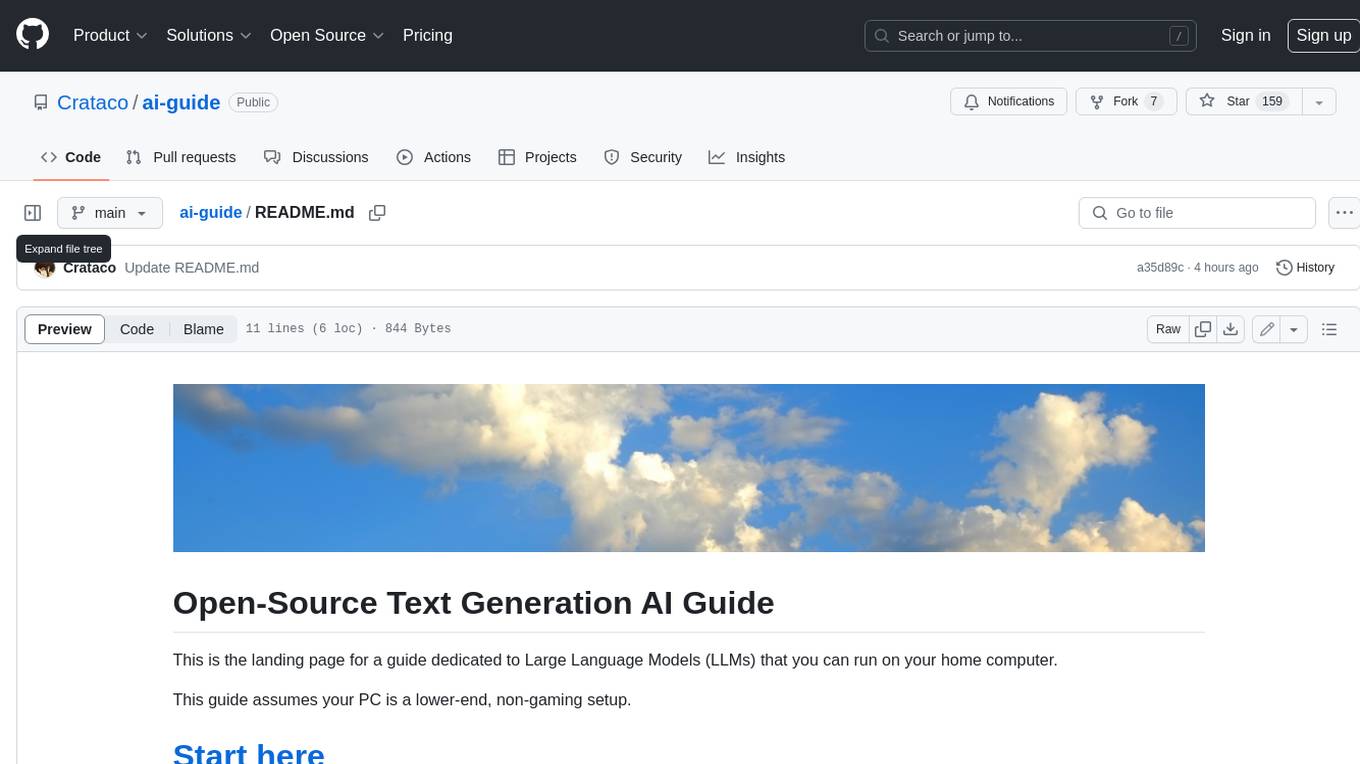
ai-guide
This guide is dedicated to Large Language Models (LLMs) that you can run on your home computer. It assumes your PC is a lower-end, non-gaming setup.
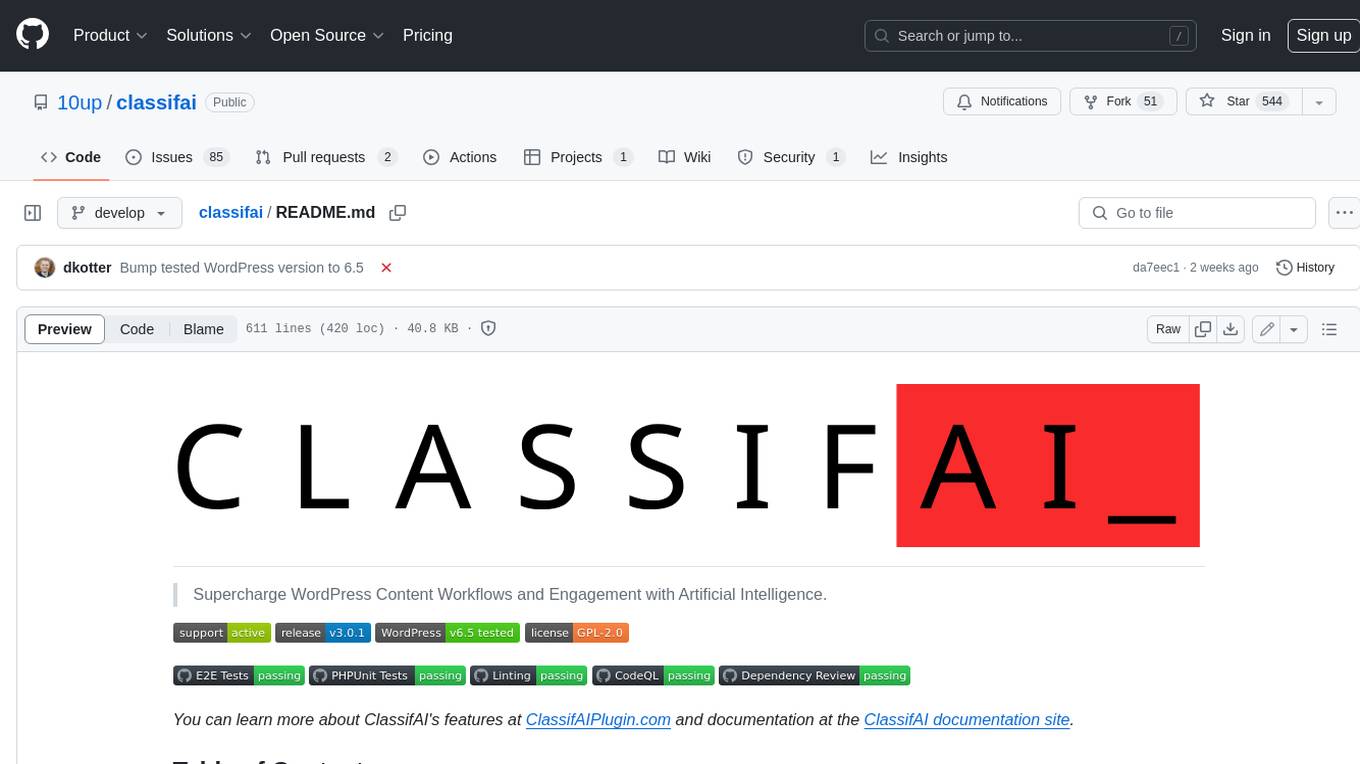
classifai
Supercharge WordPress Content Workflows and Engagement with Artificial Intelligence. Tap into leading cloud-based services like OpenAI, Microsoft Azure AI, Google Gemini and IBM Watson to augment your WordPress-powered websites. Publish content faster while improving SEO performance and increasing audience engagement. ClassifAI integrates Artificial Intelligence and Machine Learning technologies to lighten your workload and eliminate tedious tasks, giving you more time to create original content that matters.
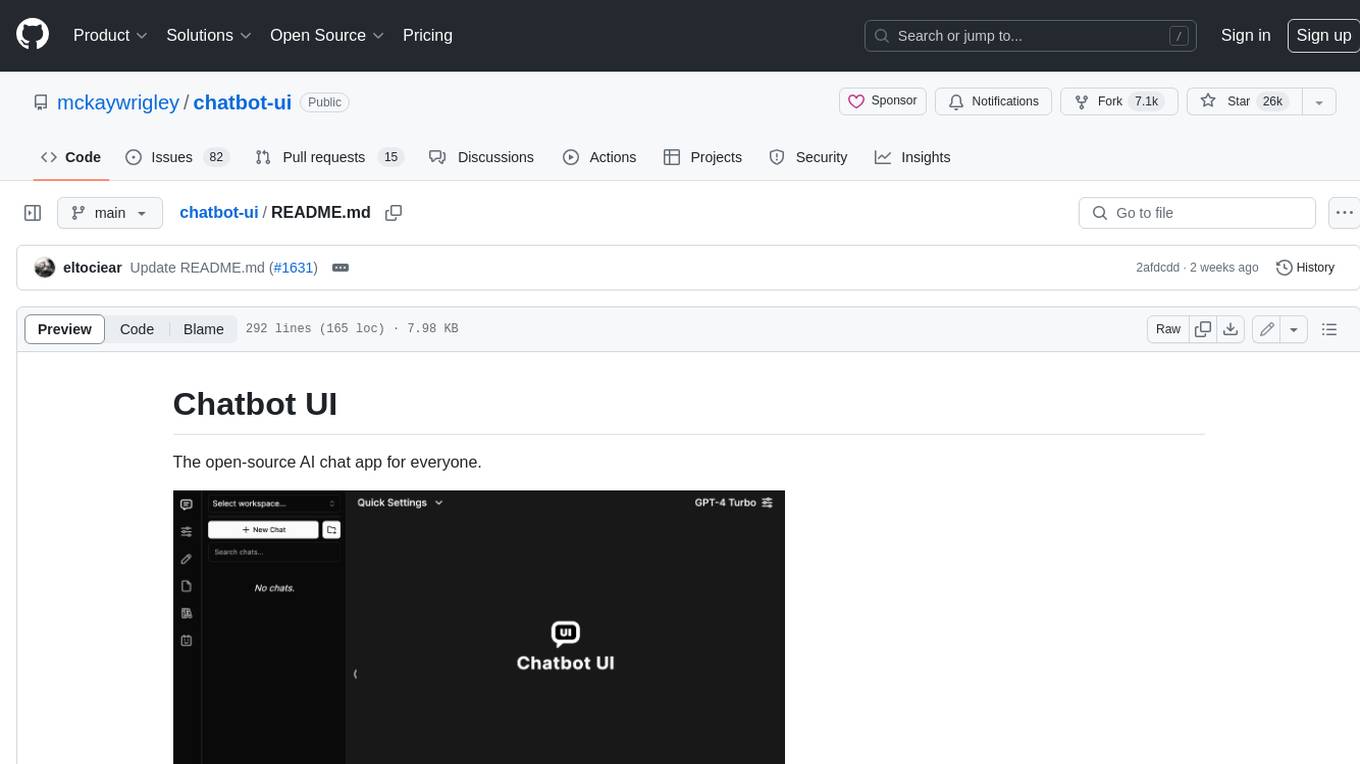
chatbot-ui
Chatbot UI is an open-source AI chat app that allows users to create and deploy their own AI chatbots. It is easy to use and can be customized to fit any need. Chatbot UI is perfect for businesses, developers, and anyone who wants to create a chatbot.
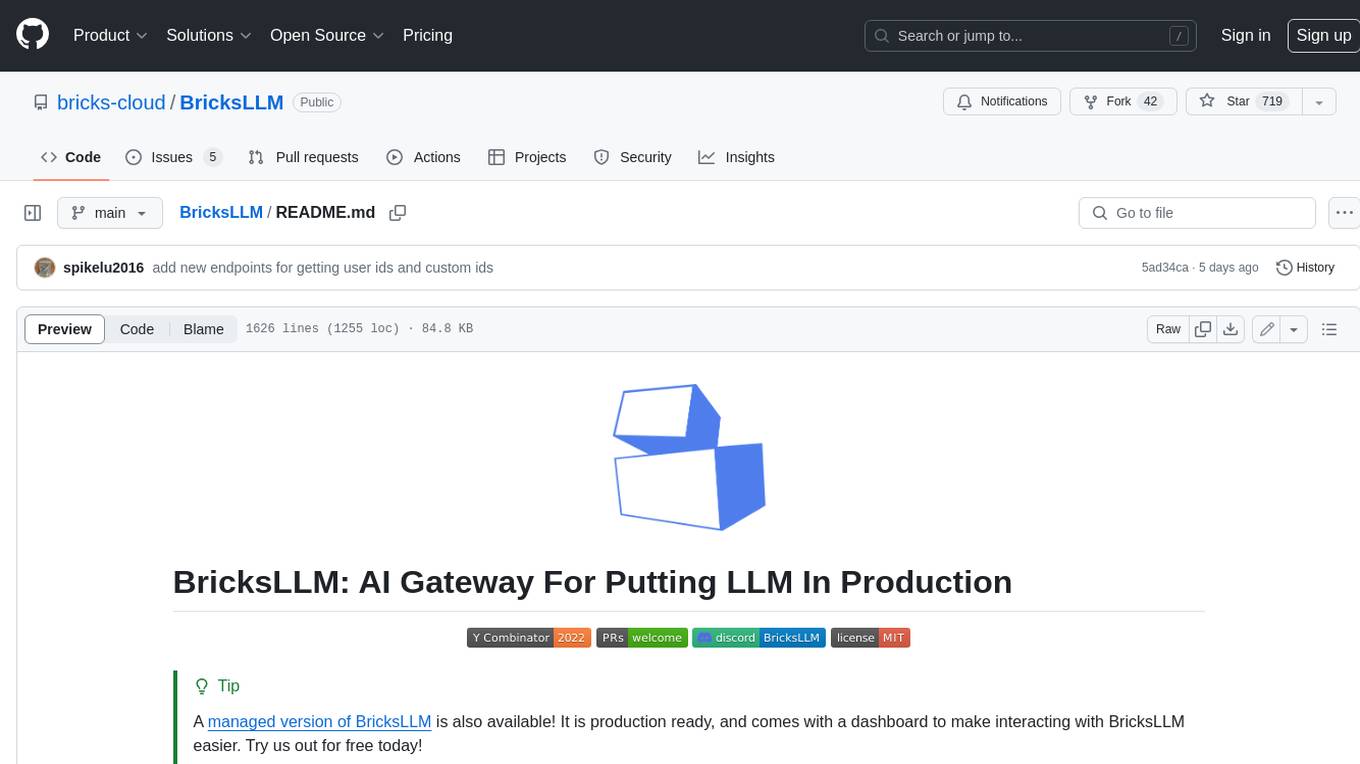
BricksLLM
BricksLLM is a cloud native AI gateway written in Go. Currently, it provides native support for OpenAI, Anthropic, Azure OpenAI and vLLM. BricksLLM aims to provide enterprise level infrastructure that can power any LLM production use cases. Here are some use cases for BricksLLM: * Set LLM usage limits for users on different pricing tiers * Track LLM usage on a per user and per organization basis * Block or redact requests containing PIIs * Improve LLM reliability with failovers, retries and caching * Distribute API keys with rate limits and cost limits for internal development/production use cases * Distribute API keys with rate limits and cost limits for students
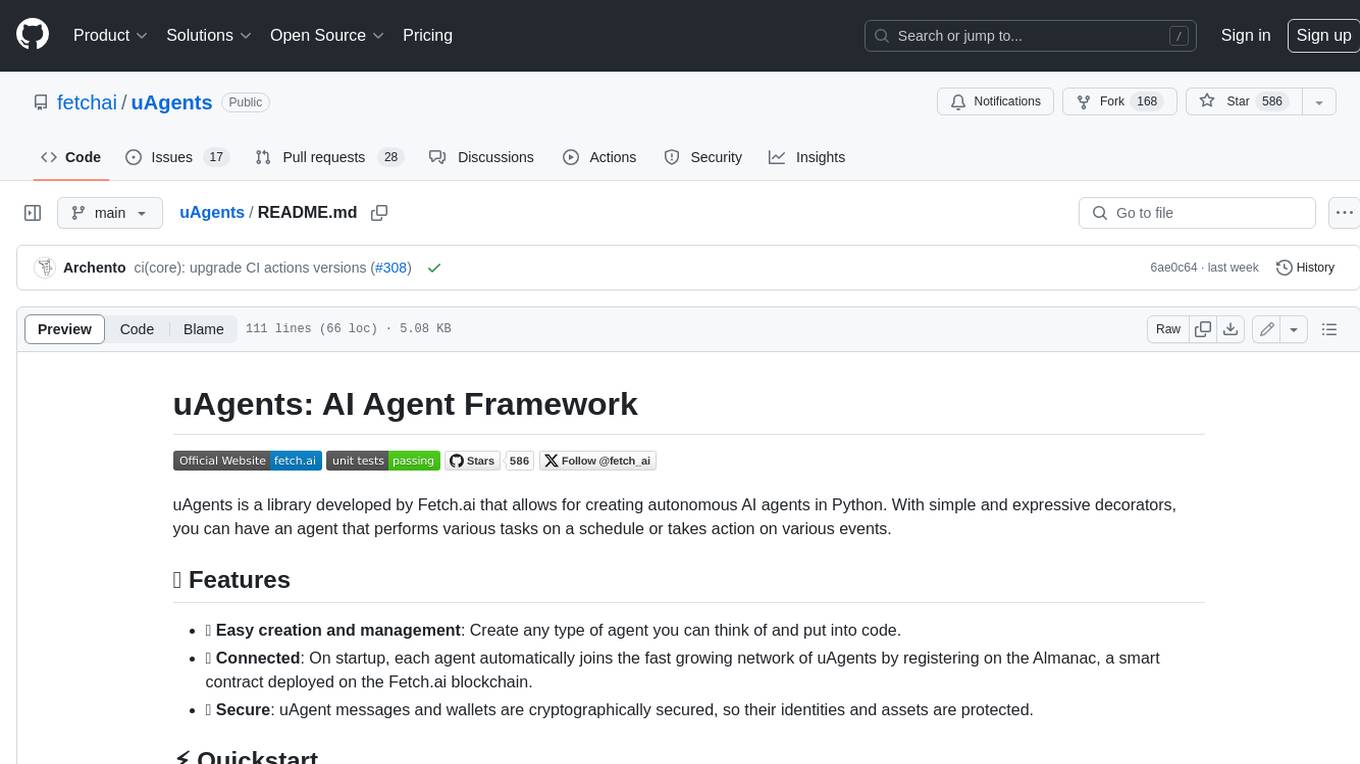
uAgents
uAgents is a Python library developed by Fetch.ai that allows for the creation of autonomous AI agents. These agents can perform various tasks on a schedule or take action on various events. uAgents are easy to create and manage, and they are connected to a fast-growing network of other uAgents. They are also secure, with cryptographically secured messages and wallets.
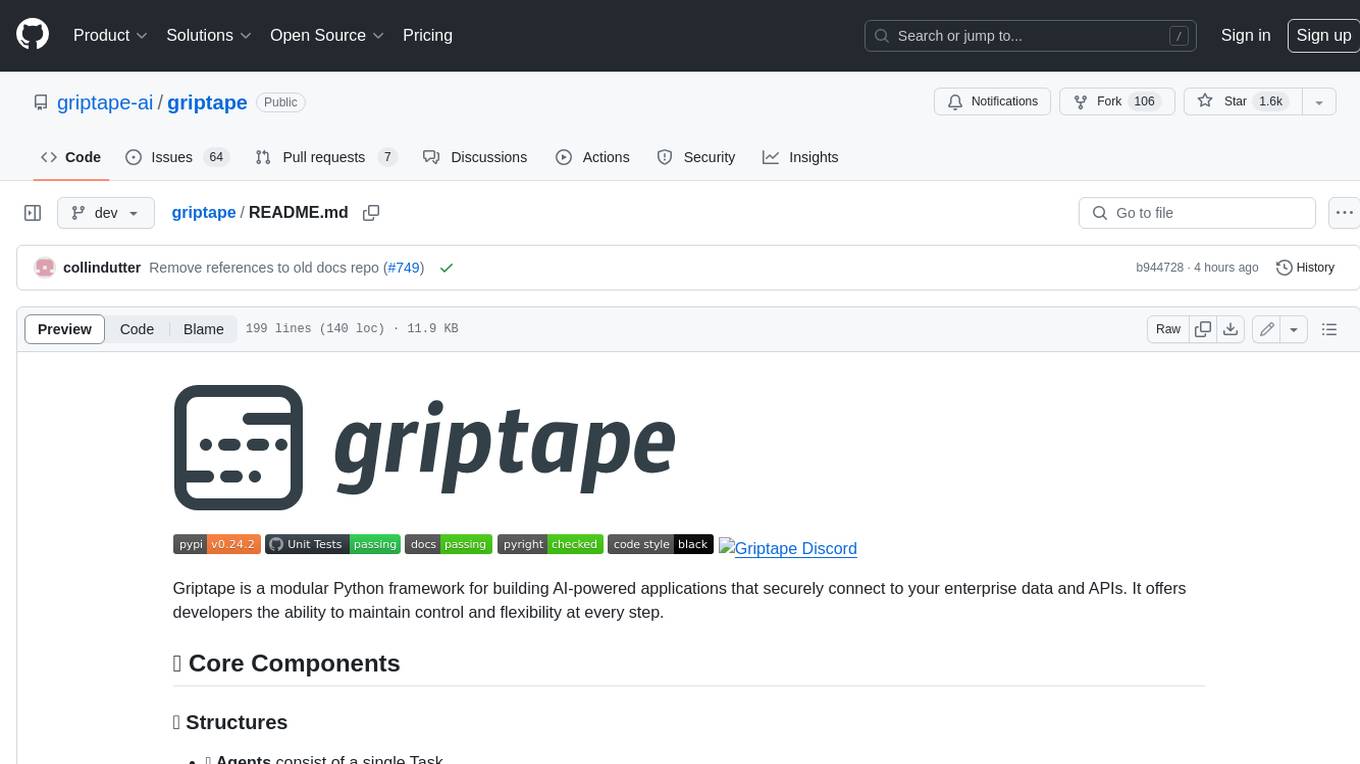
griptape
Griptape is a modular Python framework for building AI-powered applications that securely connect to your enterprise data and APIs. It offers developers the ability to maintain control and flexibility at every step. Griptape's core components include Structures (Agents, Pipelines, and Workflows), Tasks, Tools, Memory (Conversation Memory, Task Memory, and Meta Memory), Drivers (Prompt and Embedding Drivers, Vector Store Drivers, Image Generation Drivers, Image Query Drivers, SQL Drivers, Web Scraper Drivers, and Conversation Memory Drivers), Engines (Query Engines, Extraction Engines, Summary Engines, Image Generation Engines, and Image Query Engines), and additional components (Rulesets, Loaders, Artifacts, Chunkers, and Tokenizers). Griptape enables developers to create AI-powered applications with ease and efficiency.