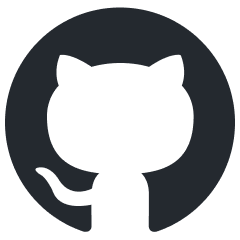
blinkid-ios
Everything you need to add AI-driven ID scanning into your native iOS app.
Stars: 380
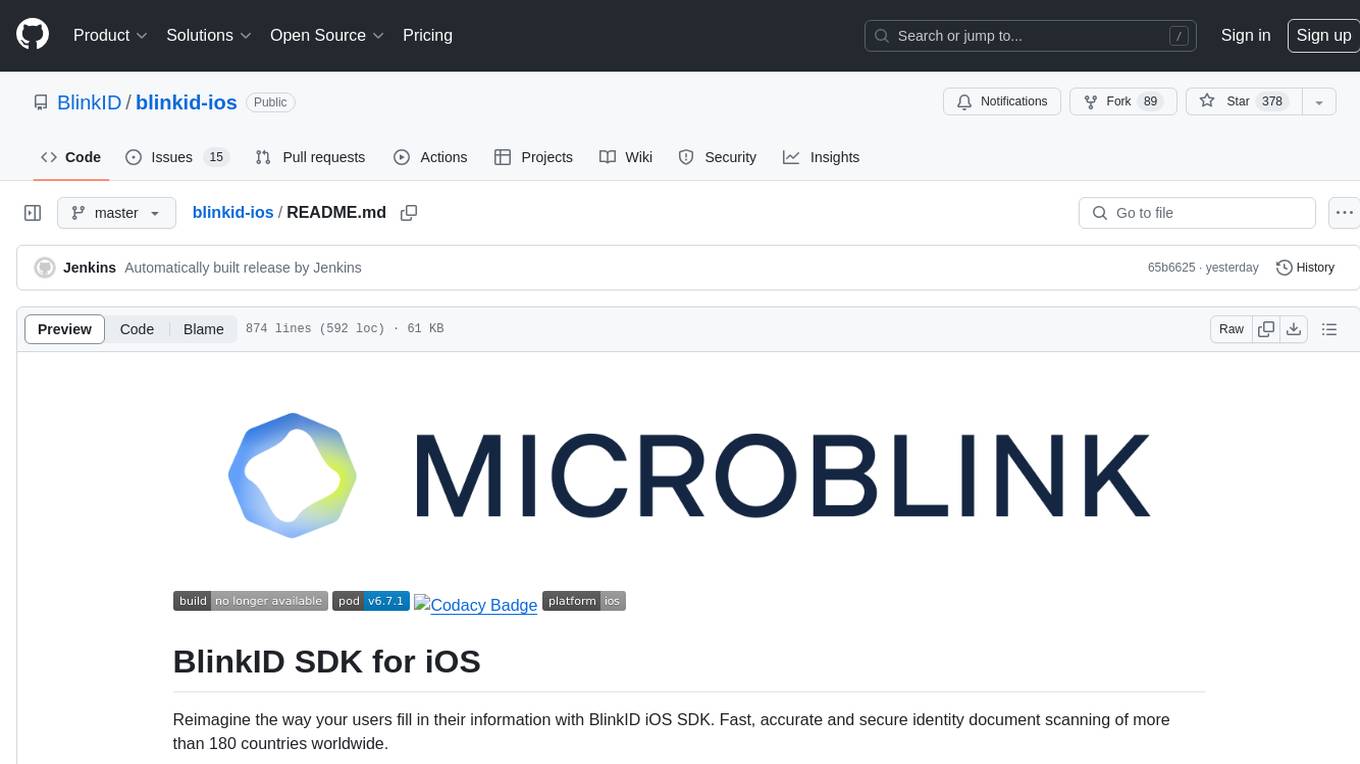
BlinkID iOS is a mobile SDK that enables developers to easily integrate ID scanning and data extraction capabilities into their iOS applications. The SDK supports scanning and processing various types of identity documents, such as passports, driver's licenses, and ID cards. It provides accurate and fast data extraction, including personal information and document details. With BlinkID iOS, developers can enhance their apps with secure and reliable ID verification functionality, improving user experience and streamlining identity verification processes.
README:
Reimagine the way your users fill in their information with BlinkID iOS SDK. Fast, accurate and secure identity document scanning of more than 180 countries worldwide.
BlinkID is:
- Fast. Real-time data extraction in less than 400ms. Way better than minutes-long form-filling.
- Secure. Privacy first, always. Scanning works even if the user’s iPhone is in airplane mode, meaning personal information never touches a third-party server.
- Intelligent. Machine learning models, optimized to read and parse virtually any identity document in the world, be it a Malaysian MyTentera or a Californian driver license.
- Lightweight. Designed to increase your app’s usability, not weight.
- What you make of it. Customize and rebrand the default UI or leave it as it is. It’s up to you.
- More than just a powerful ID scanner. Powerful data extraction, coupled with powerful perks. Get a cropped document image back, spot printed identity documents or data match both sides of the ID for parity.
To see all of these features at work, download our free demo app:
Feeling ready to get going with the integration? First, make sure we support your document type ➡️ full list. And then carefully follow the guidelines below.
Updating to a newer version of the SDK? Go to our 📚 release notes to see what’s changed.
- Requirements
- Quick Start
- Advanced BlinkID integration instructions
MBRecognizer
and available recognizers- List of available recognizers
- Localization
- Troubleshooting
- Size Report
- Additional info
SDK package contains BlinkID framework and one or more sample apps which demonstrate framework integration. The framework can be deployed in iOS 13.0 or later. NOTE: The SDK doesn't contain bitcode anymore.
This Quick Start guide will get you up and performing OCR scanning as quickly as possible. All steps described in this guide are required for the integration.
This guide closely follows the BlinkID-Sample app in the Samples folder of this repository. We highly recommend you try to run the sample app. The sample app should compile and run on your device, and in the iOS Simulator.
The source code of the sample app can be used as the reference during the integration.
- Download and install/update Cocopods version 1.11.3 or newer
- Since the libraries are stored on Git Large File Storage, you need to install git-lfs by running these commands:
brew install git-lfs
git lfs install
-
Be sure to restart your console after installing Git LFS
-
Note: if you already did try adding SDK using cocoapods and it's not working, first install the git-lfs and then clear you cocoapods cache. This should be sufficient to force cocoapods to clone BlinkID SDK, if it still doesn't work, try deinitializing your pods and installing them again.
-
Project dependencies to be managed by CocoaPods are specified in a file called
Podfile
. Create this file in the same directory as your Xcode project (.xcodeproj
) file. -
If you don't have podfile initialized run the following in your project directory.
pod init
- Copy and paste the following lines into the TextEdit window:
platform :ios, '13.0'
target 'Your-App-Name' do
pod 'PPBlinkID', '~> 6.9.0'
end
- Install the dependencies in your project:
$ pod install
- From now on, be sure to always open the generated Xcode workspace (
.xcworkspace
) instead of the project file when building your project:
open <YourProjectName>.xcworkspace
BlinkID SDK is available via Carthage. Please check out Carthage documentation if you are new to Carthage.
- Install Carthage. Check out Installing Carthage guide. Please make sure you have Carthage version >= 0.35.0 installed.
- Create a Cartfile in the same directory where your .xcodeproj or .xcworkspace is.
- Add BlinkID as a dependency to this Cartfile:
binary "https://github.com/BlinkID/blinkid-ios/blob/master/blinkid-ios.json"
- Run
carthage update
. - If successful, a Cartfile.resolved file and a Carthage directory will appear in the same directory as your Xcode project.
- Drag the binaries from
Carthage/Build/<platform>
into your application’s Xcode project.
BlinkID SDK is available as Swift Package. Please check out Swift Package Manager documentation if you are new to Swift Package Manager.
We provide a URL to the public package repository that you can add in Xcode:
https://github.com/BlinkID/blinkid-ios
-
Choose Swift package version
-Download latest release (Download .zip or .tar.gz file starting with BlinkID. DO NOT download Source Code as GitHub does not fully support Git LFS)
OR
Clone this git repository:
- Since the libraries are stored on Git Large File Storage, you need to install git-lfs by running these commands:
brew install git-lfs
git lfs install
-
Be sure to restart your console after installing Git LFS
-
To clone, run the following shell command:
git clone [email protected]:BlinkID/blinkid-ios.git
-
Copy BlinkID.xcframework to your project folder.
-
In your Xcode project, open the Project navigator. Drag the BlinkID.xcframework file to your project, ideally in the Frameworks group, together with other frameworks you're using. When asked, choose "Create groups", instead of the "Create folder references" option.
- Since BlinkID.xcframework is a dynamic framework, you also need to add it to embedded binaries section in General settings of your target and choose option
Embed & Sign
.
-
Include the additional frameworks and libraries into your project in the "Linked frameworks and libraries" section of your target settings.
- libc++.tbd
- libiconv.tbd
- libz.tbd
In files in which you want to use scanning functionality place import directive.
Swift
import BlinkID
Objective-C
#import <BlinkID/BlinkID.h>
To initiate the scanning process, first decide where in your app you want to add scanning functionality. Usually, users of the scanning library have a button which, when tapped, starts the scanning process. Initialization code is then placed in touch handler for that button. Here we're listing the initialization code as it looks in a touch handler method.
Swift
class ViewController: UIViewController, MBBlinkIdOverlayViewControllerDelegate {
var blinkIdMultiSideRecognizer : MBBlinkIdMultiSideRecognizer?
override func viewDidLoad() {
super.viewDidLoad()
MBMicroblinkSDK.shared().setLicenseResource("blinkid-license", withExtension: "key", inSubdirectory: "", for: Bundle.main, errorCallback: block)
}
@IBAction func didTapScan(_ sender: AnyObject) {
/** Create BlinkID recognizer */
self.blinkIdMultiSideRecognizer = MBBlinkIdMultiSideRecognizer()
/** Create BlinkID settings */
let settings : MBBlinkIdOverlaySettings = MBBlinkIdOverlaySettings()
/** Crate recognizer collection */
let recognizerList = [self.blinkIdMultiSideRecognizer!]
let recognizerCollection : MBRecognizerCollection = MBRecognizerCollection(recognizers: recognizerList)
/** Create your overlay view controller */
let blinkIdOverlayViewController : MBBlinkIdOverlayViewController = MBBlinkIdOverlayViewController(settings: settings, recognizerCollection: recognizerCollection, delegate: self)
/** Create recognizer view controller with wanted overlay view controller */
let recognizerRunneViewController : UIViewController = MBViewControllerFactory.recognizerRunnerViewController(withOverlayViewController: blinkIdOverlayViewController)
/** Present the recognizer runner view controller. You can use other presentation methods as well (instead of presentViewController) */
self.present(recognizerRunneViewController, animated: true, completion: nil)
}
}
Objective-C
@interface ViewController () <MBBlinkIdOverlayViewControllerDelegate>
@property (nonatomic, strong) MBBlinkIdMultiSideRecognizer *blinkIdMultiSideRecognizer;
@end
@implementation ViewController
- (void)viewDidLoad {
[super viewDidLoad];
[MBMicroblinkSDK.sharedInstance setLicenseResource:@"blinkid-license" withExtension:@"key" inSubdirectory:@"" for:Bundle.main errorCallback:block];
}
- (IBAction)didTapScan:(id)sender {
/** Create BlinkID recognizer */
self.blinkIdMultiSideRecognizer = [[MBBlinkIdMultiSideRecognizer alloc] init];
/** Create BlinkID settings */
MBBlinkIdOverlaySettings* settings = [[MBBlinkIdOverlaySettings alloc] init];
/** Create recognizer collection */
MBRecognizerCollection *recognizerCollection = [[MBRecognizerCollection alloc] initWithRecognizers:@[self.blinkIdMultiSideRecognizer]];
/** Create your overlay view controller */
MBBlinkIdOverlayViewController *blinkIdOverlayViewController = [[MBBlinkIdOverlayViewController alloc] initWithSettings:settings recognizerCollection:recognizerCollection delegate:self];
/** Create recognizer view controller with wanted overlay view controller */
UIViewController<MBRecognizerRunnerViewController>* recognizerRunnerViewController = [MBViewControllerFactory recognizerRunnerViewControllerWithOverlayViewController:blinkIdOverlayViewController];
/** Present the recognizer runner view controller. You can use other presentation methods as well (instead of presentViewController) */
[self presentViewController:recognizerRunnerViewController animated:YES completion:nil];
}
@end
A valid license key is required to initialize scanning. You can request a free trial license key, after you register, at Microblink Developer Hub.
You can include the license key in your app by passing a string or a file with license key.
Note that you need to set the license key before intializing scanning. Ideally in AppDelegate
or viewDidLoad
before initializing any recognizers.
You can pass the license key as a string, the following way:
Swift
MBMicroblinkSDK.shared().setLicenseKey("LICENSE-KEY", errorCallback: block)
Objective-C
[[MBMicroblinkSDK sharedInstance] setLicenseKey:@"LICENSE-KEY" errorCallback:block];
Or you can include the license key, with the code below. Please make sure that the file that contains the license key is included in your project and is copied during Copy Bundle Resources build phase.
Swift
MBMicroblinkSDK.shared().setLicenseResource("license-key-file", withExtension: "key", inSubdirectory: "directory-to-license-key", for: Bundle.main, errorCallback: block)
Objective-C
[[MBMicroblinkSDK sharedInstance] setLicenseResource:@"license-key-file" withExtension:@"key" inSubdirectory:@"" forBundle:[NSBundle mainBundle] errorCallback:block];
If the licence is invalid or expired then the methods above will throw an exception.
In the previous step, you instantiated MBBlinkIdOverlayViewController
object with a delegate object. This object gets notified on certain events in scanning lifecycle. In this example we set it to self
. The protocol which the delegate has to implement is MBBlinkIdOverlayViewControllerDelegate
protocol. It is necessary to conform to that protocol. We will discuss more about protocols in Advanced integration section. You can use the following default implementation of the protocol to get you started.
Swift
func blinkIdOverlayViewControllerDidFinishScanning(_ blinkIdOverlayViewController: MBBlinkIdOverlayViewController, state: MBRecognizerResultState) {
// this is done on background thread
// check for valid state
if state == .valid {
// first, pause scanning until we process all the results
blinkIdOverlayViewController.recognizerRunnerViewController?.pauseScanning()
DispatchQueue.main.async(execute: {() -> Void in
// All UI interaction needs to be done on main thread
})
}
}
func blinkIdOverlayViewControllerDidTapClose(_ blinkIdOverlayViewController: MBBlinkIdOverlayViewController) {
// Your action on cancel
}
Objective-C
- (void)blinkIdOverlayViewControllerDidFinishScanning:(MBBlinkIdOverlayViewController *)blinkIdOverlayViewController state:(MBRecognizerResultState)state {
// this is done on background thread
// check for valid state
if (state == MBRecognizerResultStateValid) {
// first, pause scanning until we process all the results
[blinkIdOverlayViewController.recognizerRunnerViewController pauseScanning];
dispatch_async(dispatch_get_main_queue(), ^{
// All UI interaction needs to be done on main thread
});
}
}
- (void)blinkIdOverlayViewControllerDidTapClose:(nonnull MBBlinkIdOverlayViewController *)blinkIdOverlayViewController {
// Your action on cancel
}
This section covers more advanced details of BlinkID integration.
- First part will cover the possible customizations when using UI provided by the SDK.
-
Second part will describe how to embed
MBRecognizerRunnerViewController's delegates
into yourUIViewController
with the goal of creating a custom UI for scanning, while still using camera management capabilites of the SDK. -
Third part will describe how to use the
MBRecognizerRunner
(Direct API) for recognition directly fromUIImage
without the need of camera or to recognize camera frames that are obtained by custom camera management. - Fourth part will describe recognizer concept and available recognizers.
Within BlinkID SDK there are several built-in overlay view controllers and scanning subview overlays that you can use to perform scanning.
MBBlinkIdOverlayViewController
implements new UI for scanning identity documents, which is optimally designed to be used with new MBBlinkIdSingleSideRecognizer
and MBBlinkIdMultiSideRecognizer
. The new MBBlinkIdOverlayViewController
implements several new features:
- clear indication for searching phase, when BlinkID is searching for an ID document
- clear progress indication, when BlinkID is busy with OCR and data extraction
- clear message when the document is not supported
- visual indications when the user needs to place the document closer to the camera
- when
MBBlinkIdMultiSideRecognizer
is used, visual indication that the data from the front side of the document doesn't match the data on the back side of the document.
The new UI allows the user to scan the document at an any angle, in any orientation. We recommend forcing landscape orientation if you scan barcodes on the back side, because in that orientation success rate will be higher. To force the UI in landscape mode, use the following instructions:
Swift
let settings = MBBlinkIdOverlaySettings()
settings.autorotateOverlay = true
settings.supportedOrientations = UIInterfaceOrientationMask.landscape
Objective-C
MBBlinkIdOverlaySettings *settings = [[MBBlinkIdOverlaySettings alloc] init];
settings.autorotateOverlay = YES;
settings.supportedOrientations = UIInterfaceOrientationMaskLandscape;
It has MBBlinkIdOverlayViewControllerDelegate
delegate which can be used out-of-the-box to perform scanning using the default UI. Here is an example how to use and initialize MBBlinkIdOverlayViewController
:
Swift
/** Create your overlay view controller */
let blinkIdOverlayViewController : MBBlinkIdOverlayViewController = MBBlinkIdOverlayViewController(settings: blinkIdSettings, recognizerCollection: recognizerCollection, delegate: self)
/** Create recognizer view controller with wanted overlay view controller */
let recognizerRunneViewController : UIViewController = MBViewControllerFactory.recognizerRunnerViewController(withOverlayViewController: blinkIdOverlayViewController)
/** Present the recognizer runner view controller. You can use other presentation methods as well (instead of presentViewController) */
self.present(recognizerRunneViewController, animated: true, completion: nil)
Objective-C
MBBlinkIdOverlayViewController *overlayVC = [[MBBlinkIdOverlayViewController alloc] initWithSettings:settings recognizerCollection: recognizerCollection delegate:self];
UIViewController<MBRecognizerRunnerViewController>* recognizerRunnerViewController = [MBViewControllerFactory recognizerRunnerViewControllerWithOverlayViewController:overlayVC];
/** Present the recognizer runner view controller. You can use other presentation methods as well (instead of presentViewController) */
[self presentViewController:recognizerRunnerViewController animated:YES completion:nil];
As you can see, when initializing MBBlinkIdOverlayViewController
, we are sending delegate property as self
. To get results, we need to conform to MBBlinkIdOverlayViewControllerDelegate
protocol.
The SDK comes with the ability to customize some aspects of the UI by using the UI theming. The screens can be customized to fit your app’s look and feel by defining themes in your application that override themes from the SDK. Each theme must extend the corresponding base theme from the SDK, as described in the following sections.
To customize MBBlinkIdOverlayViewController
, use MBBlinkIdOverlayTheme
class to customize your look. You can customise elements labeled on the screenshot above by providing wanted properties to MBBlinkIdOverlayTheme
:
-
reticle
- reticleErrorColor - change custom error UIColor
-
instructions
- instructionsFont - set custom UIFont
- instructionsTextColor - set custom UIColor
- instructionsCornerRadius - set custom corner radius
-
flashlightWarning
- flashlightWarningFont - set custom UIFont
- flashlightWarningBackgroundColor - set custom background UIColor
- flashlightWarningTextColor - set custom text UIColor
- flashlightWarningCornerRadius - set custom corner radius
-
cardIcon
- frontCardImage - change front card image on flip
- backCardImage - change back card image on flip
-
successIcon
- successScanningImage - change success scan image
- successFlashColor - change flash color on success scanning
Please check our Samples for custom implementation of overlay view controller.
Overlay View Controller is an abstract class for all overlay views.
It's responsibility is to provide meaningful and useful interface for the user to interact with.
Typical actions which need to be allowed to the user are:
- intuitive and meaniningful way to guide the user through scanning process. This is usually done by presenting a "viewfinder" in which the user need to place the scanned object
- a way to cancel the scanning, typically with a "cancel" or "back" button
- a way to power on and off the light (i.e. "torch") button
BlinkID SDK always provides it's own default implementation of the Overlay View Controller for every specific use. Your implementation should closely mimic the default implementation as it's the result of thorough testing with end users. Also, it closely matches the underlying scanning technology.
For example, the scanning technology usually gives results very fast after the user places the device's camera in the expected way above the scanned object. This means a progress bar for the scan is not particularly useful to the user. The majority of time the user spends on positioning the device's camera correctly. That's just an example which demonstrates careful decision making behind default camera overlay view.
To use your custom overlay with Microblink's camera view, you must first subclass MBCustomOverlayViewController
and implement the overlay behaviour conforming wanted protocols.
There are seven MBRecognizerRunnerViewController
protocols.
Seven RecognizerRunnerViewController
protocols are:
MBScanningRecognizerRunnerViewControllerDelegate
MBDetectionRecognizerRunnerViewControllerDelegate
MBOcrRecognizerRunnerViewControllerDelegate
MBGlareRecognizerRunnerViewControllerDelegate
MBFirstSideFinishedRecognizerRunnerViewControllerDelegate
MBDebugRecognizerRunnerViewControllerDelegate
MBRecognizerRunnerViewControllerDelegate
In viewDidLoad
, other protocol conformation can be done and it's done on recognizerRunnerViewController
property of MBOverlayViewController
, for example:
Swift and Objective-C
self.scanningRecognizerRunnerViewControllerDelegate = self;
In Quick Start guide it is shown how to use a default overlay view controller. You can now swap default view controller with your implementation of CustomOverlayViewController
Swift
let recognizerRunnerViewController : UIViewController = MBViewControllerFactory.recognizerRunnerViewController(withOverlayViewController: CustomOverlayViewController)
Objective-C
UIViewController<MBRecognizerRunnerViewController>* recognizerRunnerViewController = [MBViewControllerFactory recognizerRunnerViewControllerWithOverlayViewController:CustomOverlayViewController];
This guide will in short present you how to process UIImage objects with BlinkID SDK, without starting the camera video capture.
With this feature you can solve various use cases like: - recognizing text on images in Camera roll - taking full resolution photo and sending it to processing - scanning barcodes on images in e-mail etc.
DirectAPI-sample demo app here will present UIImagePickerController for taking full resolution photos, and then process it with BlinkID SDK to get scanning results using Direct processing API.
Direct processing API is handled with MBRecognizerRunner
. That is a class that handles processing of images. It also has protocols as MBRecognizerRunnerViewController
.
Developer can choose which protocol to conform:
MBScanningRecognizerRunnerDelegate
MBDetectionRecognizerRunnerDelegate
MBDebugRecognizerRunnerDelegate
MBOcrRecognizerRunnerDelegate
In example, we are conforming to MBScanningRecognizerRunnerDelegate
protocol.
To initiate the scanning process, first decide where in your app you want to add scanning functionality. Usually, users of the scanning library have a button which, when tapped, starts the scanning process. Initialization code is then placed in touch handler for that button. Here we're listing the initialization code as it looks in a touch handler method.
Swift
func setupRecognizerRunner() {
var recognizers = [MBRecognizer]()
recognizer = MBBlinkIdMultiSideRecognizer()
recognizers.append(recognizer!)
let recognizerCollection = MBRecognizerCollection(recognizers: recognizers)
recognizerRunner = MBRecognizerRunner(recognizerCollection: recognizerCollection)
recognizerRunner?.scanningRecognizerRunnerDelegate = self
}
func processImageRunner(_ originalImage: UIImage) {
var image: MBImage? = nil
if let anImage = originalImage {
image = MBImage(uiImage: anImage)
}
image?.cameraFrame = true
image?.orientation = MBProcessingOrientation.left
let _serialQueue = DispatchQueue(label: "com.microblink.DirectAPI-sample-swift")
_serialQueue.async(execute: {() -> Void in
self.recognizerRunner?.processImage(image!)
})
}
func recognizerRunner(_ recognizerRunner: MBRecognizerRunner, didFinishScanningWith state: MBRecognizerResultState) {
if blinkInputRecognizer.result.resultState == MBRecognizerResultStateValid {
// Handle result
}
}
Objective-C
- (void)setupRecognizerRunner {
NSMutableArray<MBRecognizer *> *recognizers = [[NSMutableArray alloc] init];
self.recognizer = [[MBBlinkIdMultiSideRecognizer alloc] init];
[recognizers addObject: self.recognizer];
MBRecognizerCollection *recognizerCollection = [[MBRecognizerCollection alloc] initWithRecognizers:recognizers];
self.recognizerRunner = [[MBRecognizerRunner alloc] initWithRecognizerCollection:recognizerCollection];
self.recognizerRunner.scanningRecognizerRunnerDelegate = self;
}
- (void)processImageRunner:(UIImage *)originalImage {
MBImage *image = [MBImage imageWithUIImage:originalImage];
image.cameraFrame = YES;
image.orientation = MBProcessingOrientationLeft;
dispatch_queue_t _serialQueue = dispatch_queue_create("com.microblink.DirectAPI-sample", DISPATCH_QUEUE_SERIAL);
dispatch_async(_serialQueue, ^{
[self.recognizerRunner processImage:image];
});
}
- (void)recognizerRunner:(nonnull MBRecognizerRunner *)recognizerRunner didFinishScanningWithState:(MBRecognizerResultState)state {
if (self.blinkInputRecognizer.result.resultState == MBRecognizerResultStateValid) {
// Handle result
}
}
Now you've seen how to implement the Direct processing API.
In essence, this API consists of two steps:
- Initialization of the scanner.
- Call of
- (void)processImage:(MBImage *)image;
method for each UIImage or CMSampleBufferRef you have.
Some recognizers support recognition from NSString
. They can be used through Direct API to parse given NSString
and return data just like when they are used on an input image. When recognition is performed on NSString
, there is no need for the OCR. Input NSString
is used in the same way as the OCR output is used when image is being recognized.
Recognition from String
can be performed in the same way as recognition from image.
The only difference is that user should call - (void)processString:(NSString *)string;
on MBRecognizerRunner
.
DirectAPI's RecognizerRunner
singleton is a state machine that can be in one of 3 states: OFFLINE
, READY
and WORKING
.
- When you obtain the reference to
RecognizerRunner
singleton, it will be inOFFLINE
state. - You can initialize
RecognizerRunner
by callinginit
method. If you callinitialize
method whileRecognizerRunner
is not inOFFLINE
state, you will getIllegalStateException
. - After successful initialization,
RecognizerRunner
will move toREADY
state. Now you can call any of therecognize*
methods. - When starting recognition with any of the
recognize*
methods,RecognizerRunner
will move toWORKING
state. If you attempt to call these methods whileRecognizerRunner
is not inREADY
state, you will getIllegalStateException
- Recognition is performed on background thread so it is safe to call all
RecognizerRunner's
methods from UI thread - When recognition is finished,
RecognizerRunner
first moves back toREADY
state and then calls therecognizerRunner(_ :, didFinishScanningWith:)
method of the providedMBScanningRecognizerRunnerDelegate
. - Please note that
MBScanningRecognizerRunnerDelegate's
recognizerRunner(_ :, didFinishScanningWith:)
method will be called on background processing thread, so make sure you do not perform UI operations in this callback. Also note that until therecognizerRunner(_ :, didFinishScanningWith:)
method completes,RecognizerRunner
will not perform recognition of another image or string, even if any of therecognize*
methods have been called just after transitioning toREADY
state. This is to ensure that results of the recognizers associated withRecognizerRunner
are not modified while possibly being used withinrecognizerRunner(_ :, didFinishScanningWith:)
method. - By calling
resetState
method,RecognizerRunner
singleton will release all its internal resources. Note that even after callingresetState
you might receiverecognizerRunner(_ :, didFinishScanningWith:)
event if there was work in progress whenresetState
was called. -
resetState
method can be called from anyRecognizerRunner
singleton's state
Both RecognizerRunnerView
and RecognizerRunner
use the same internal singleton that manages native code. This singleton handles initialization and termination of native library and propagating recognizers to native library. It is possible to use RecognizerRunnerView
and RecognizerRunner
together, as internal singleton will make sure correct synchronization and correct recognition settings are used. If you run into problems while using RecognizerRunner
in combination with RecognizerRunnerView
, let us know!
When you are using combined recognizer and images of both document sides are required, you need to call RecognizerRunner.recognize*
multiple times. Call it first with the images of the first side of the document, until it is read, and then with the images of the second side. The combined recognizer automatically switches to second side scanning, after it has successfully read the first side. To be notified when the first side scanning is completed, you have to set the MBFirstSideFinishedRecognizerRunnerDelegate
through MBRecognizerRunnerMetadataDelegates
. If you don't need that information, e.g. when you have only one image for each document side, don't set the MBFirstSideFinishedRecognizerRunnerDelegate
and check the RecognitionSuccessType in MBScanningRecognizerRunnerDelegate.recognizerRunner(_ :, didFinishScanningWith:)
, after the second side image has been processed.
The MBRecognizer
is the basic unit of processing within the SDK. Its main purpose is to process the image and extract meaningful information from it. As you will see later, the SDK has lots of different MBRecognizer
objects that have various purposes.
Each MBRecognizer
has a MBRecognizerResult
object, which contains the data that was extracted from the image. The MBRecognizerResult
object is a member of corresponding MBRecognizer
object its lifetime is bound to the lifetime of its parent MBRecognizer
object. If you need your MBRecognizerResult
object to outlive its parent MBRecognizer
object, you must make a copy of it by calling its method copy
.
While MBRecognizer
object works, it changes its internal state and its result. The MBRecognizer
object's MBRecognizerResult
always starts in Empty
state. When corresponding MBRecognizer
object performs the recognition of given image, its MBRecognizerResult
can either stay in Empty
state (in case MBRecognizer
failed to perform recognition), move to Uncertain
state (in case MBRecognizer
performed the recognition, but not all mandatory information was extracted) or move to Valid
state (in case MBRecognizer
performed recognition and all mandatory information was successfully extracted from the image).
As soon as one MBRecognizer
object's MBRecognizerResult
within MBRecognizerCollection
given to MBRecognizerRunner
or MBRecognizerRunnerViewController
changes to Valid
state, the onScanningFinished
callback will be invoked on same thread that performs the background processing and you will have the opportunity to inspect each of your MBRecognizer
objects' MBRecognizerResult
to see which one has moved to Valid
state.
As soon as onScanningFinished
method ends, the MBRecognizerRunnerViewController
will continue processing new camera frames with same MBRecognizer
objects, unless paused
. Continuation of processing or reset
recognition will modify or reset all MBRecognizer
objects's MBRecognizerResult
. When using built-in activities, as soon as onScanningFinished
is invoked, built-in activity pauses the MBRecognizerRunnerViewController
and starts finishing the activity, while saving the MBRecognizerCollection
with active MBRecognizer
.
The MBRecognizerCollection
is is wrapper around MBRecognizer
objects that has array of MBRecognizer
objects that can be used to give MBRecognizer
objects to MBRecognizerRunner
or MBRecognizerRunnerViewController
for processing.
The MBRecognizerCollection
is always constructed with array [[MBRecognizerCollection alloc] initWithRecognizers:recognizers]
of MBRecognizer
objects that need to be prepared for recognition (i.e. their properties must be tweaked already).
The MBRecognizerCollection
manages a chain of MBRecognizer
objects within the recognition process. When a new image arrives, it is processed by the first MBRecognizer
in chain, then by the second and so on, iterating until a MBRecognizer
object's MBRecognizerResult
changes its state to Valid
or all of the MBRecognizer
objects in chain were invoked (none getting a Valid
result state).
You cannot change the order of the MBRecognizer
objects within the chain - no matter the order in which you give MBRecognizer
objects to MBRecognizerCollection
, they are internally ordered in a way that provides best possible performance and accuracy. Also, in order for SDK to be able to order MBRecognizer
objects in recognition chain in a best way possible, it is not allowed to have multiple instances of MBRecognizer
objects of the same type within the chain. Attempting to do so will crash your application.
This section will give a list of all MBRecognizer
objects that are available within BlinkID SDK, their purpose and recommendations how they should be used to get best performance and user experience.
The MBFrameGrabberRecognizer
is the simplest recognizer in SDK, as it does not perform any processing on the given image, instead it just returns that image back to its onFrameAvailable
. Its result never changes state from empty.
This recognizer is best for easy capturing of camera frames with MBRecognizerRunnerViewController
. Note that MBImage
sent to onFrameAvailable
are temporary and their internal buffers all valid only until the onFrameAvailable
method is executing - as soon as method ends, all internal buffers of MBImage
object are disposed. If you need to store MBImage
object for later use, you must create a copy of it by calling copy
.
The MBSuccessFrameGrabberRecognizer
is a special MBecognizer
that wraps some other MBRecognizer
and impersonates it while processing the image. However, when the MBRecognizer
being impersonated changes its MBRecognizerResult
into Valid
state, the MBSuccessFrameGrabberRecognizer
captures the image and saves it into its own MBSuccessFrameGrabberRecognizerResult
object.
Since MBSuccessFrameGrabberRecognizer
impersonates its slave MBRecognizer
object, it is not possible to give both concrete MBRecognizer
object and MBSuccessFrameGrabberRecognizer
that wraps it to same MBRecognizerCollection
- doing so will have the same result as if you have given two instances of same MBRecognizer
type to the MBRecognizerCollection
- it will crash your application.
This recognizer is best for use cases when you need to capture the exact image that was being processed by some other MBRecognizer
object at the time its MBRecognizerResult
became Valid
. When that happens, MBSuccessFrameGrabberRecognizer's
MBSuccessFrameGrabberRecognizerResult
will also become Valid
and will contain described image.
Unless stated otherwise for concrete recognizer, single side BlinkID recognizes from this list can be used in any context, but they work best with the MBDocumentOverlayViewController
, which has UI best suited for document scanning.
Combined recognizers should be used with MBDocumentVerificationOverlayViewController
which manages scanning of multiple document sides in the single camera opening and guides the user through the scanning process. Some combined recognizers support scanning of multiple document types, but only one document type can be scanned at a time.
The MBMrtdRecognizer
is used for scanning and data extraction from the Machine Readable Zone (MRZ) of the various Machine Readable Travel Documents (MRTDs) like ID cards and passports. This recognizer is not bound to the specific country, but it can be configured to only return data that match some criteria defined by the MBMrzFilter
.
You can find information about usage context at the beginning of this section.
The MBMrtdCombinedRecognizer
scans Machine Readable Zone (MRZ) after scanning the full document image and face image (usually MRZ is on the back side and face image is on the front side of the document). Internally, it uses MBDocumentFaceRecognizer for obtaining full document image and face image as the first step and then MBMrtdRecognizer for scanning the MRZ.
You can find information about usage context at the beginning of this section.
The MBPassportRecognizer
is used for scanning and data extraction from the Machine Readable Zone (MRZ) of the various passport documents. This recognizer also returns face image from the passport.
You can find information about usage context at the beginning of this section.
The MBVisaRecognizer
is used for scanning and data extraction from the Machine Readable Zone (MRZ) of the various visa documents. This recognizer also returns face image from the visa document.
You can find information about usage context at the beginning of this section.
The MBIdBarcodeRecognizer
is used for scanning barcodes from various ID cards. Check this document to see the list of supported document types.
You can find information about usage context at the beginning of this section.
The MBDocumentFaceRecognizer
is a special type of recognizer that only returns face image and full document image of the scanned document. It does not extract document fields like first name, last name, etc. This generic recognizer can be used to obtain document images in cases when specific support for some document type is not available.
You can find information about usage context at the beginning of this section.
The MBBlinkIdSingleSideRecognizer
scans and extracts data from the front side of the supported document.
You can find the list of the currently supported documents here
.
We will continue expanding this recognizer by adding support for new document types in the future. Star this repo to stay updated.
Use MBBlinkIdMultiSideRecognizer
for scanning both sides of the supported document. First, it scans and extracts data from the front, then scans and extracts data from the barcode on the back, and finally, combines results from both sides. The BlinkIDCombinedRecognizer
also performs data matching and returns a flag if the extracted data captured from the front side matches the data from the barcode on the back.
You can find the list of the currently supported documents here
.
We will continue expanding this recognizer by adding support for new document types in the future. Star this repo to stay updated.
The SDK is localized on following languages: Arabic, Chinese simplified, Chinese traditional, Croatian, Czech, Dutch, Filipino, French, German, Hebrew, Hungarian, Indonesian, Italian, Malay, Portuguese, Romanian, Slovak, Slovenian, Spanish, Thai, Vietnamese.
If you would like us to support additional languages or report incorrect translation, please contact us at help.microblink.com.
If you want to add additional languages yourself or change existing translations, you need to set customLocalizationFileName
property on MBMicroblinkApp
object to your strings file name.
For example, let's say that we want to change text "Scan the front side of a document" to "Scan the front side" in BlinkID sample project. This would be the steps:
- Find the translation key in en.strings file inside BlinkID.framework
- Add a new file MyTranslations.strings to the project by using "Strings File" template
- With MyTranslations.string open, in File inspector tap "Localize..." button and select English
- Add the translation key "blinkid_generic_message" and the value "Scan the front side" to MyTranslations.strings
- Finally in AppDelegate.swift in method
application(_:, didFinishLaunchingWithOptions:)
addMBMicroblinkApp.instance()?.customLocalizationFileName = "MyTranslations"
In case of problems with integration of the SDK, first make sure that you have tried integrating it into Xcode by following integration instructions.
If you have followed Xcode integration instructions and are still having integration problems, please contact us at help.microblink.com.
In case of problems with using the SDK, you should do as follows:
If you are getting "invalid licence key" error or having other licence-related problems (e.g. some feature is not enabled that should be or there is a watermark on top of camera), first check the console. All licence-related problems are logged to error log so it is easy to determine what went wrong.
When you have determine what is the licence-relate problem or you simply do not understand the log, you should contact us help.microblink.com. When contacting us, please make sure you provide following information:
- exact Bundle ID of your app (from your
info.plist
file) - licence that is causing problems
- please stress out that you are reporting problem related to iOS version of BlinkID SDK
- if unsure about the problem, you should also provide excerpt from console containing licence error
If you are having problems with scanning certain items, undesired behaviour on specific device(s), crashes inside BlinkID SDK or anything unmentioned, please do as follows:
- Contact us at help.microblink.com describing your problem and provide following information:
- log file obtained in previous step
- high resolution scan/photo of the item that you are trying to scan
- information about device that you are using
- please stress out that you are reporting problem related to iOS version of BlinkID SDK
Here is a list of frequently asked questions and solutions for them and also a list of known problems in the SDK and how to work around them.
In demo everything worked, but after switching to production license I get NSError
with MBMicroblinkSDKRecognizerErrorDomain
and MBRecognizerFailedToInitalize
code as soon as I construct specific MBRecognizer
object
Each license key contains information about which features are allowed to use and which are not. This NSError
indicates that your production license does not allow using of specific MBRecognizer
object. You should contact support to check if provided licence is OK and that it really contains all features that you have purchased.
I get NSError
with MBMicroblinkSDKRecognizerErrorDomain
and MBRecognizerFailedToInitalize
code with trial license key
Whenever you construct any MBRecognizer
object or, a check whether license allows using that object will be performed. If license is not set prior constructing that object, you will get NSError
with MBMicroblinkSDKRecognizerErrorDomain
and MBRecognizerFailedToInitalize
code. We recommend setting license as early as possible in your app.
Make sure you link your app with iconv and Accelerate frameworks as shown in Quick start.
If you are using Cocoapods, please be sure that you've installed git-lfs
prior to installing pods. If you are still getting this error, go to project folder and execute command git-lfs pull
.
SDK crashes on armv7 devices if bitcode is enabled. We are working on it.
In my didFinish
callback I have the result inside my MBRecognizer
, but when scanning activity finishes, the result is gone
This usually happens when using MBRecognizerRunnerViewController
and forgetting to pause the MBRecognizerRunnerViewController
in your didFinish
callback. Then, as soon as didFinish
happens, the result is mutated or reset by additional processing that MBRecognizer
performs in the time between end of your didFinish
callback and actual finishing of the scanning activity. For more information about statefulness of the MBRecognizer
objects, check this section.
BlinkID.framework is a dynamic framework which contains slices for all architectures - device and simulator. If you intend to extract .ipa file for ad hoc distribution, you'll need to preprocess the framework to remove simulator architectures.
Ideal solution is to add a build phase after embed frameworks build phase, which strips unused slices from embedded frameworks.
Build step is based on the one provided here: http://ikennd.ac/blog/2015/02/stripping-unwanted-architectures-from-dynamic-libraries-in-xcode/
APP_PATH="${TARGET_BUILD_DIR}/${WRAPPER_NAME}"
# This script loops through the frameworks embedded in the application and
# removes unused architectures.
find "$APP_PATH" -name '*.framework' -type d | while read -r FRAMEWORK
do
FRAMEWORK_EXECUTABLE_NAME=$(defaults read "$FRAMEWORK/Info.plist" CFBundleExecutable)
FRAMEWORK_EXECUTABLE_PATH="$FRAMEWORK/$FRAMEWORK_EXECUTABLE_NAME"
echo "Executable is $FRAMEWORK_EXECUTABLE_PATH"
EXTRACTED_ARCHS=()
for ARCH in $ARCHS
do
echo "Extracting $ARCH from $FRAMEWORK_EXECUTABLE_NAME"
lipo -extract "$ARCH" "$FRAMEWORK_EXECUTABLE_PATH" -o "$FRAMEWORK_EXECUTABLE_PATH-$ARCH"
EXTRACTED_ARCHS+=("$FRAMEWORK_EXECUTABLE_PATH-$ARCH")
done
echo "Merging extracted architectures: ${ARCHS}"
lipo -o "$FRAMEWORK_EXECUTABLE_PATH-merged" -create "${EXTRACTED_ARCHS[@]}"
rm "${EXTRACTED_ARCHS[@]}"
echo "Replacing original executable with thinned version"
rm "$FRAMEWORK_EXECUTABLE_PATH"
mv "$FRAMEWORK_EXECUTABLE_PATH-merged" "$FRAMEWORK_EXECUTABLE_PATH"
done
Logging can be disabled by calling disableMicroblinkLogging
method on MBLogger
instance.
We are delivering complete size report of our BlinkID SDK based on our BlinkID-sample-Swift sample project. You can check that here.
Complete API reference can be found here.
For any other questions, feel free to contact us at help.microblink.com.
For Tasks:
Click tags to check more tools for each tasksFor Jobs:
Alternative AI tools for blinkid-ios
Similar Open Source Tools
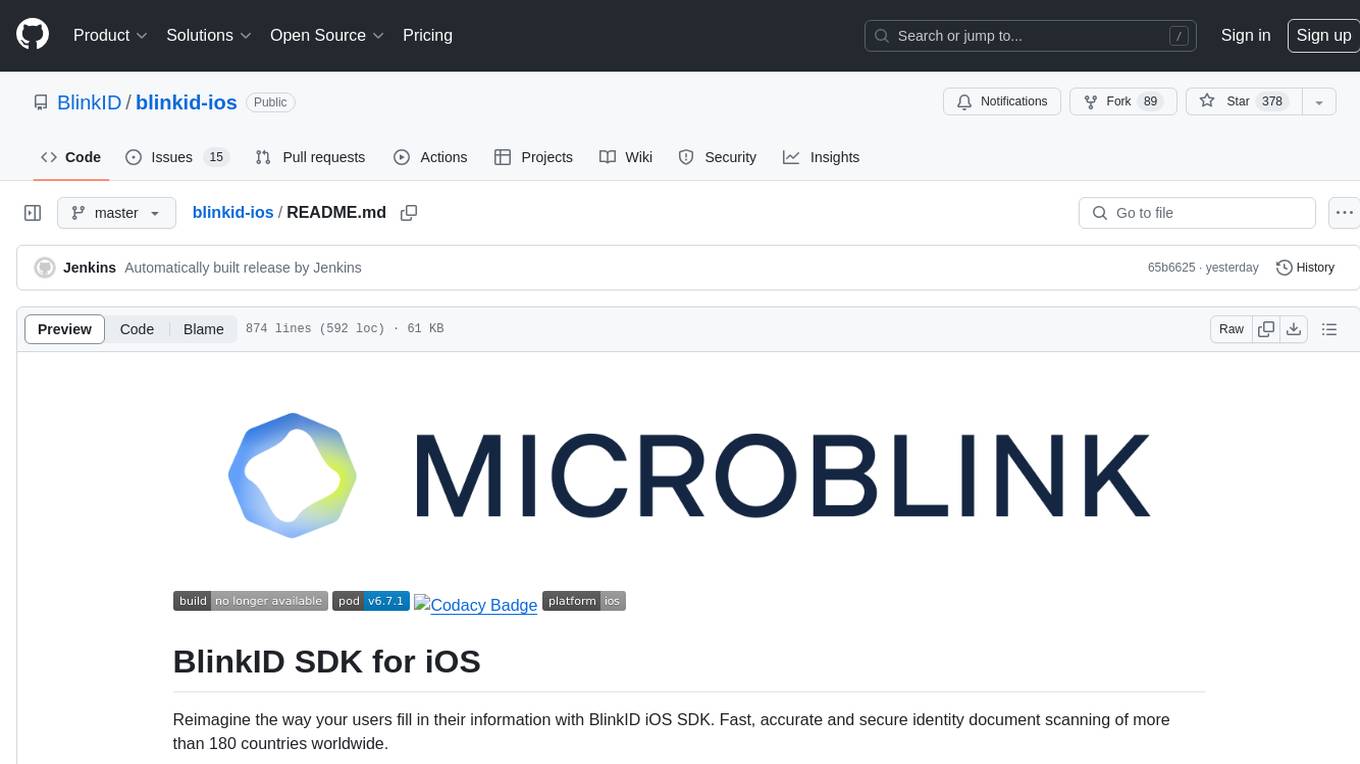
blinkid-ios
BlinkID iOS is a mobile SDK that enables developers to easily integrate ID scanning and data extraction capabilities into their iOS applications. The SDK supports scanning and processing various types of identity documents, such as passports, driver's licenses, and ID cards. It provides accurate and fast data extraction, including personal information and document details. With BlinkID iOS, developers can enhance their apps with secure and reliable ID verification functionality, improving user experience and streamlining identity verification processes.
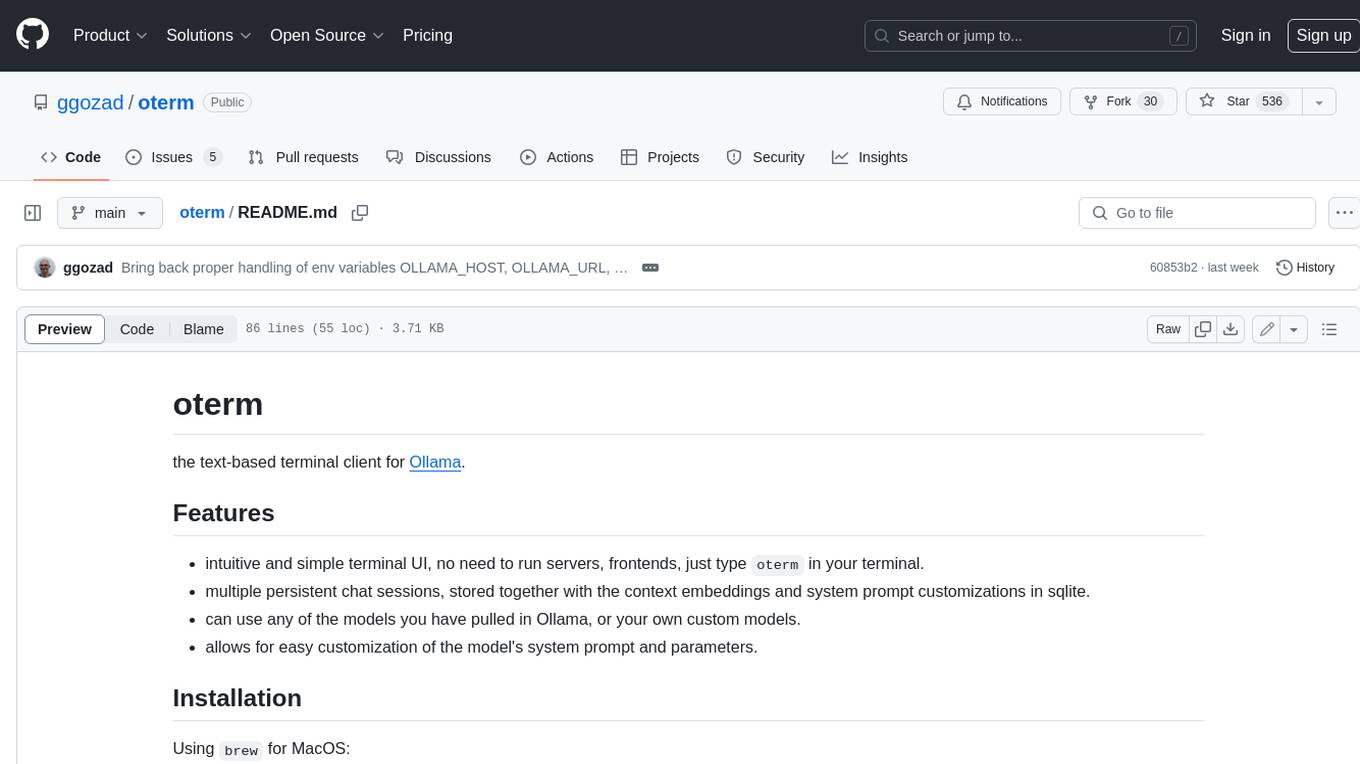
oterm
Oterm is a text-based terminal client for Ollama, a large language model. It provides an intuitive and simple terminal UI, allowing users to interact with Ollama without running servers or frontends. Oterm supports multiple persistent chat sessions, which are stored along with context embeddings and system prompt customizations in a SQLite database. Users can easily customize the model's system prompt and parameters, and select from any of the models they have pulled in Ollama or their own custom models. Oterm also supports keyboard shortcuts for creating new chat sessions, editing existing sessions, renaming sessions, exporting sessions as markdown, deleting sessions, toggling between dark and light themes, quitting the application, switching to multiline input mode, selecting images to include with messages, and navigating through the history of previous prompts. Oterm is licensed under the MIT License.
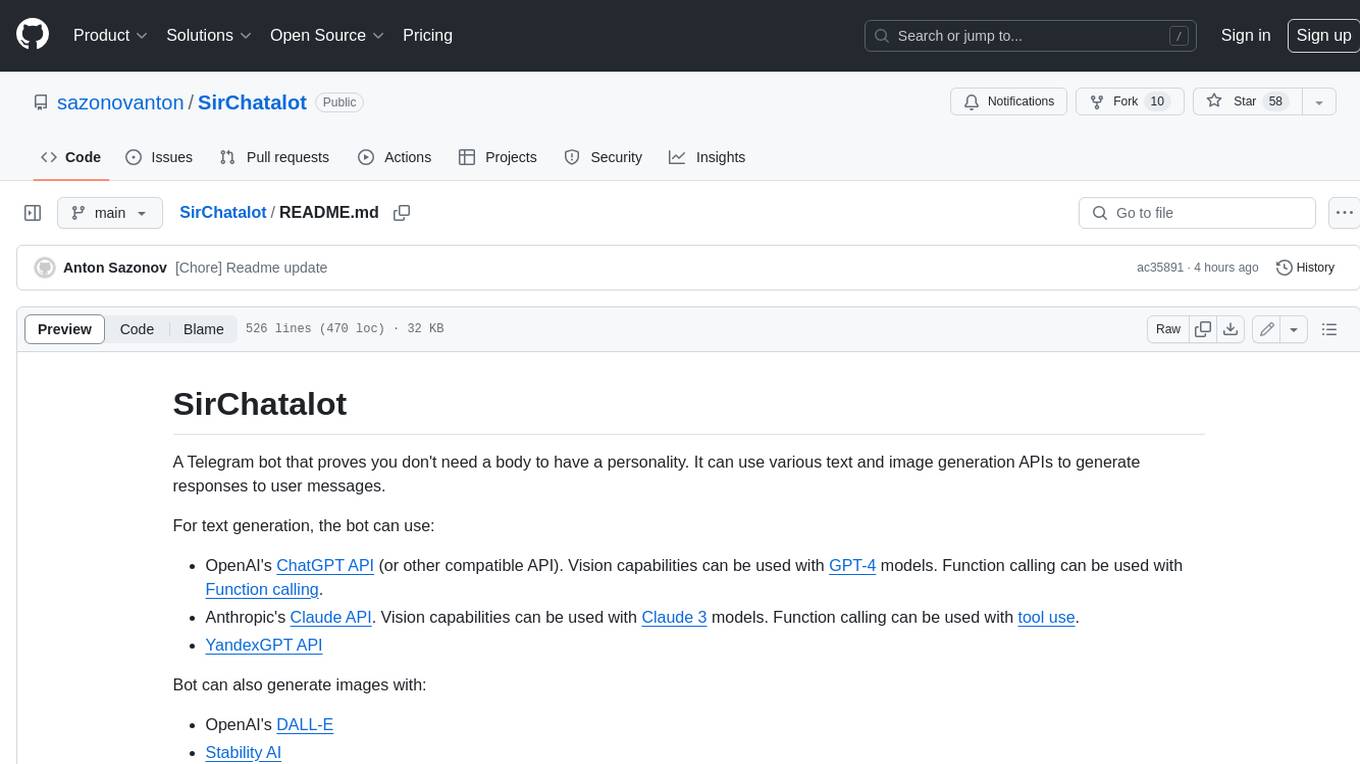
SirChatalot
A Telegram bot that proves you don't need a body to have a personality. It can use various text and image generation APIs to generate responses to user messages. For text generation, the bot can use: * OpenAI's ChatGPT API (or other compatible API). Vision capabilities can be used with GPT-4 models. Function calling can be used with Function calling. * Anthropic's Claude API. Vision capabilities can be used with Claude 3 models. Function calling can be used with tool use. * YandexGPT API Bot can also generate images with: * OpenAI's DALL-E * Stability AI * Yandex ART This bot can also be used to generate responses to voice messages. Bot will convert the voice message to text and will then generate a response. Speech recognition can be done using the OpenAI's Whisper model. To use this feature, you need to install the ffmpeg library. This bot is also support working with files, see Files section for more details. If function calling is enabled, bot can generate images and search the web (limited).
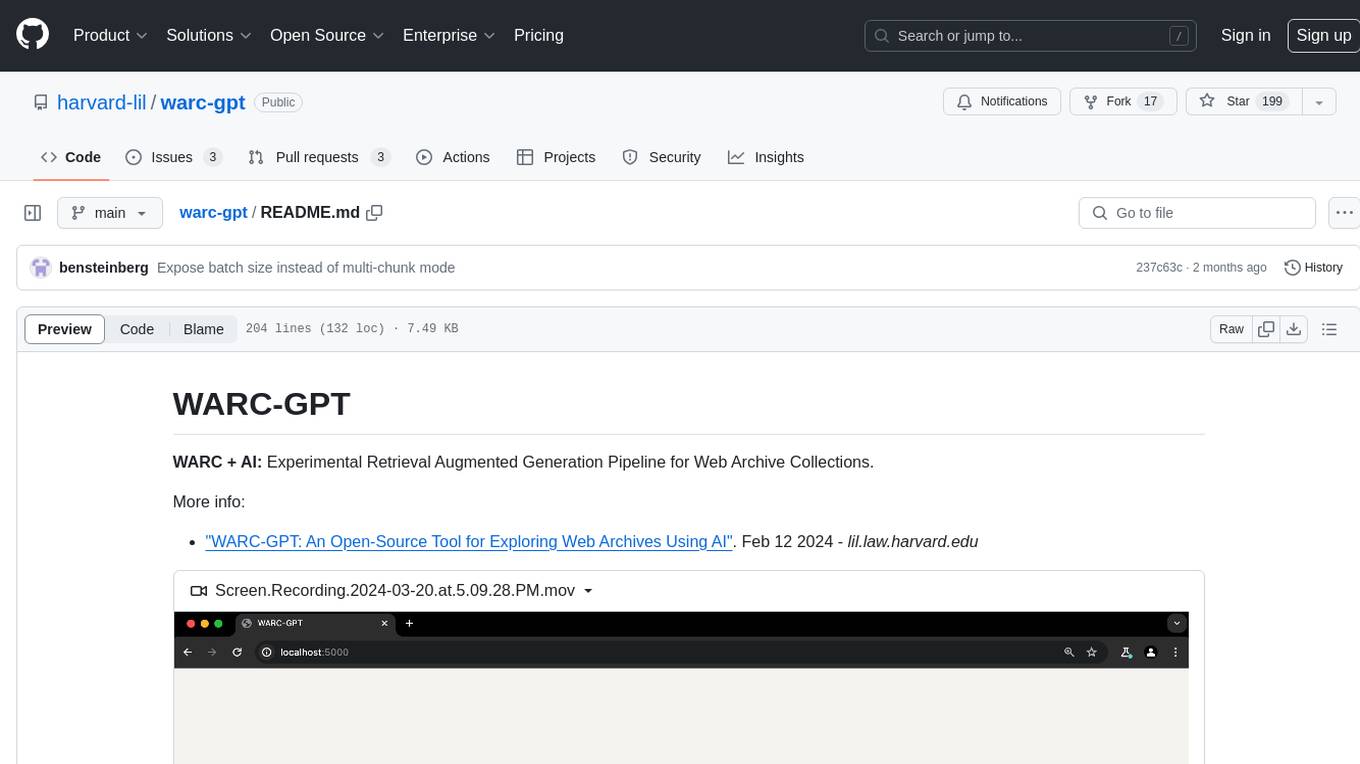
warc-gpt
WARC-GPT is an experimental retrieval augmented generation pipeline for web archive collections. It allows users to interact with WARC files, extract text, generate text embeddings, visualize embeddings, and interact with a web UI and API. The tool is highly customizable, supporting various LLMs, providers, and embedding models. Users can configure the application using environment variables, ingest WARC files, start the server, and interact with the web UI and API to search for content and generate text completions. WARC-GPT is designed for exploration and experimentation in exploring web archives using AI.
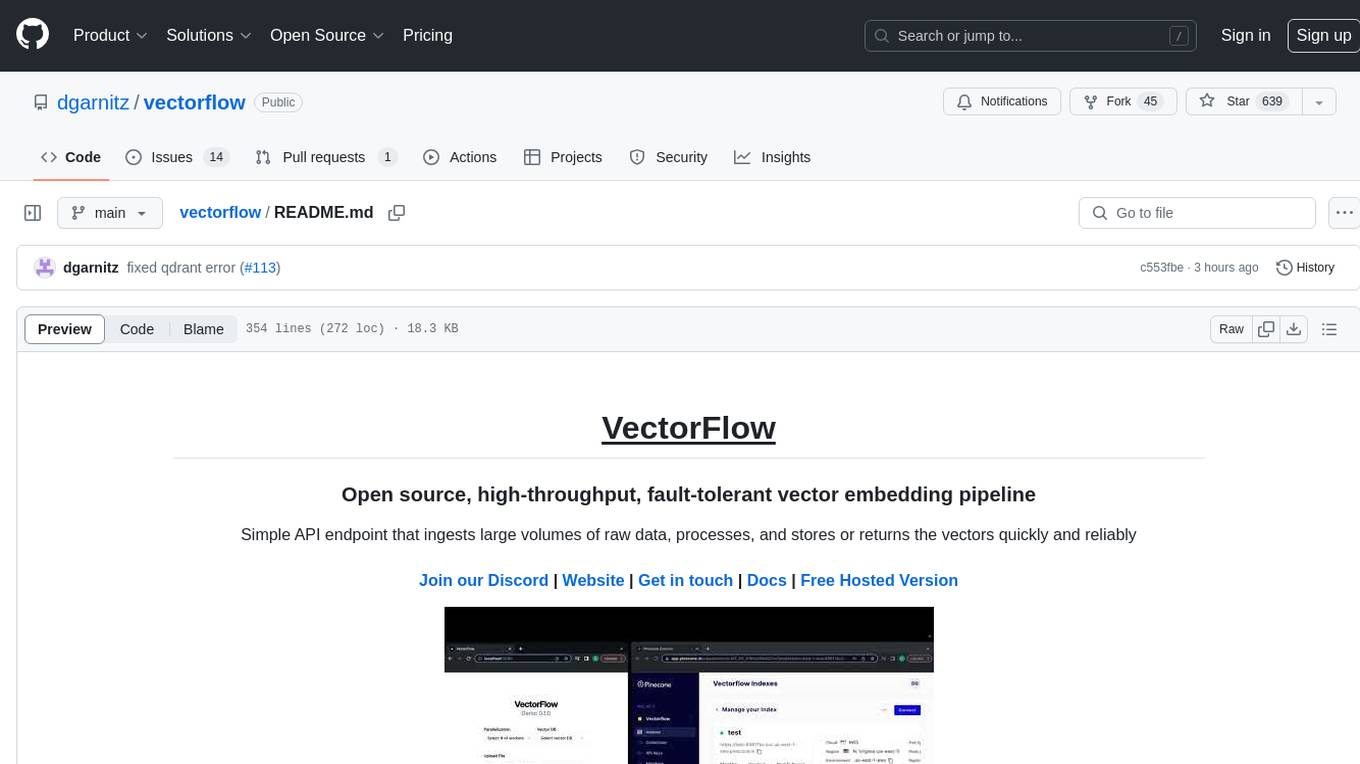
vectorflow
VectorFlow is an open source, high throughput, fault tolerant vector embedding pipeline. It provides a simple API endpoint for ingesting large volumes of raw data, processing, and storing or returning the vectors quickly and reliably. The tool supports text-based files like TXT, PDF, HTML, and DOCX, and can be run locally with Kubernetes in production. VectorFlow offers functionalities like embedding documents, running chunking schemas, custom chunking, and integrating with vector databases like Pinecone, Qdrant, and Weaviate. It enforces a standardized schema for uploading data to a vector store and supports features like raw embeddings webhook, chunk validation webhook, S3 endpoint, and telemetry. The tool can be used with the Python client and provides detailed instructions for running and testing the functionalities.
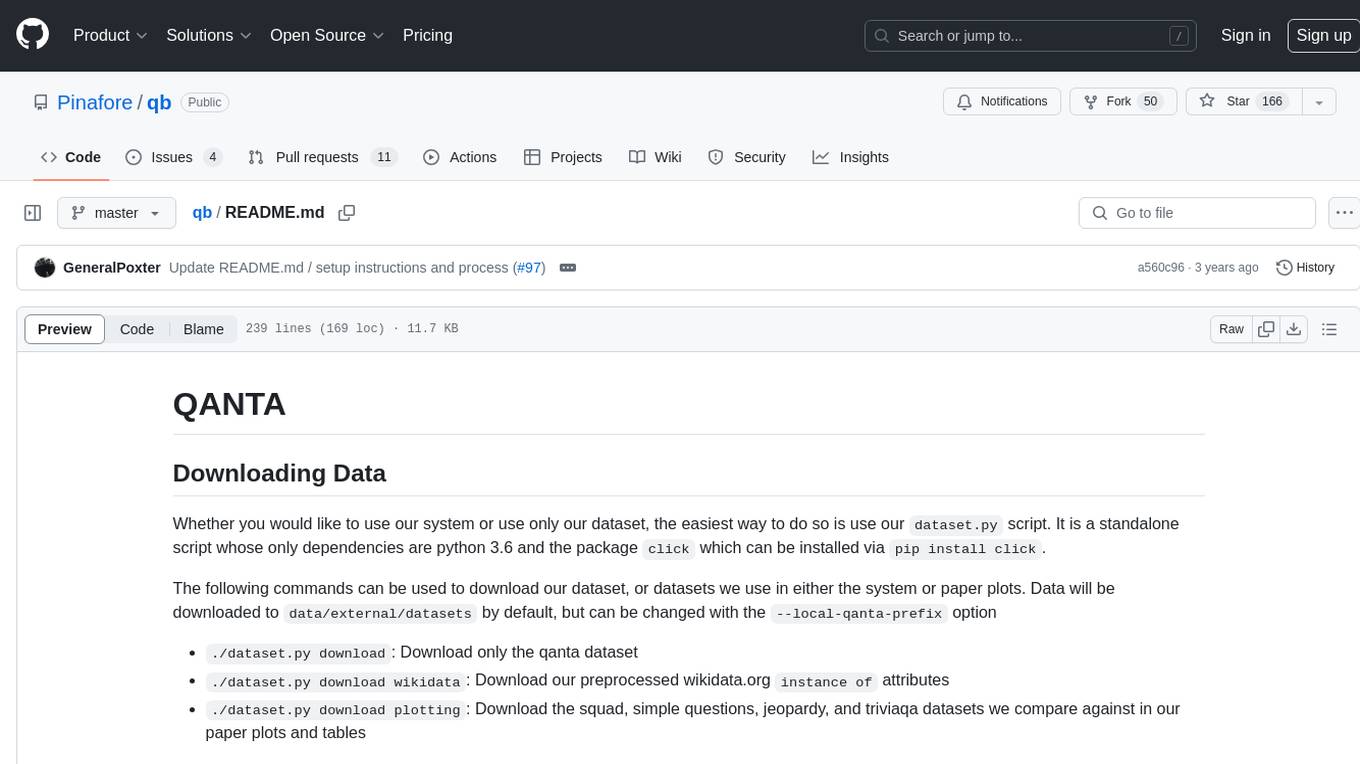
qb
QANTA is a system and dataset for question answering tasks. It provides a script to download datasets, preprocesses questions, and matches them with Wikipedia pages. The system includes various datasets, training, dev, and test data in JSON and SQLite formats. Dependencies include Python 3.6, `click`, and NLTK models. Elastic Search 5.6 is needed for the Guesser component. Configuration is managed through environment variables and YAML files. QANTA supports multiple guesser implementations that can be enabled/disabled. Running QANTA involves using `cli.py` and Luigi pipelines. The system accesses raw Wikipedia dumps for data processing. The QANTA ID numbering scheme categorizes datasets based on events and competitions.
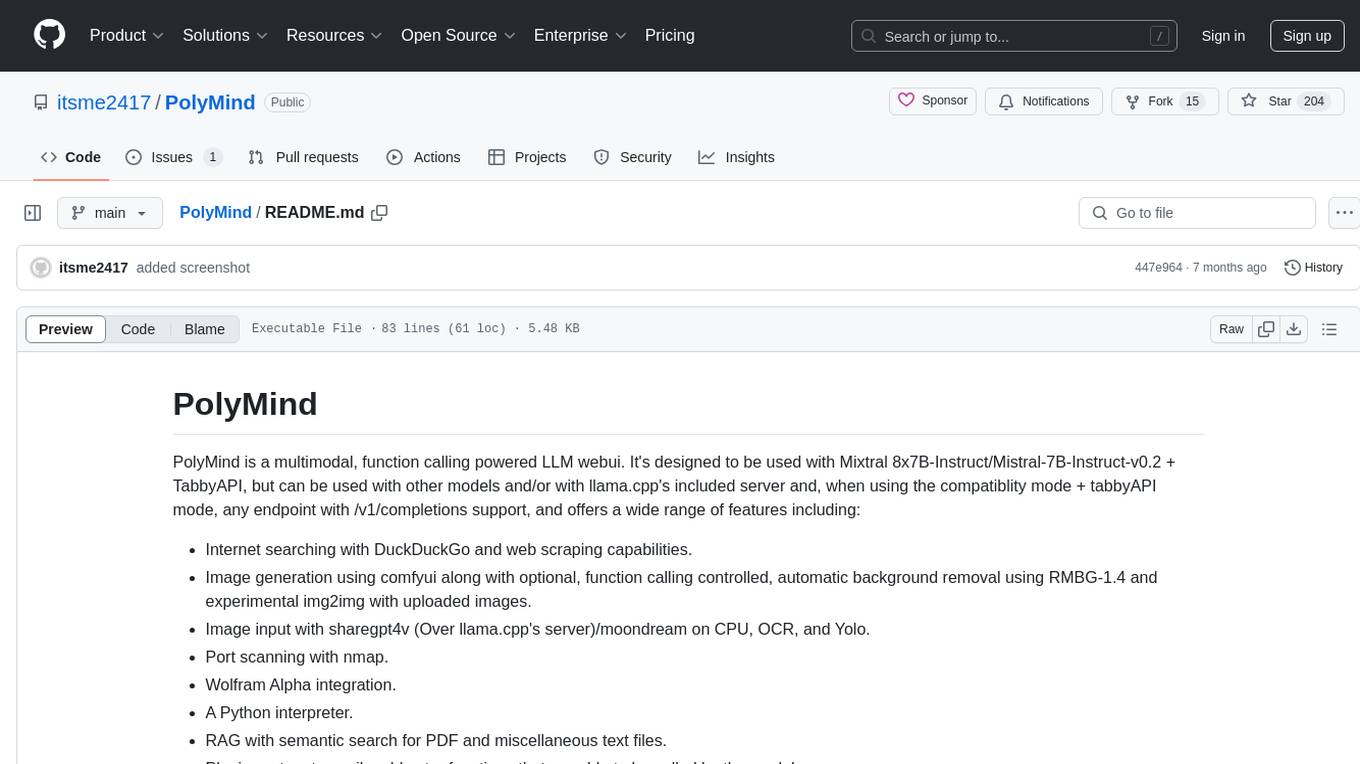
PolyMind
PolyMind is a multimodal, function calling powered LLM webui designed for various tasks such as internet searching, image generation, port scanning, Wolfram Alpha integration, Python interpretation, and semantic search. It offers a plugin system for adding extra functions and supports different models and endpoints. The tool allows users to interact via function calling and provides features like image input, image generation, and text file search. The application's configuration is stored in a `config.json` file with options for backend selection, compatibility mode, IP address settings, API key, and enabled features.
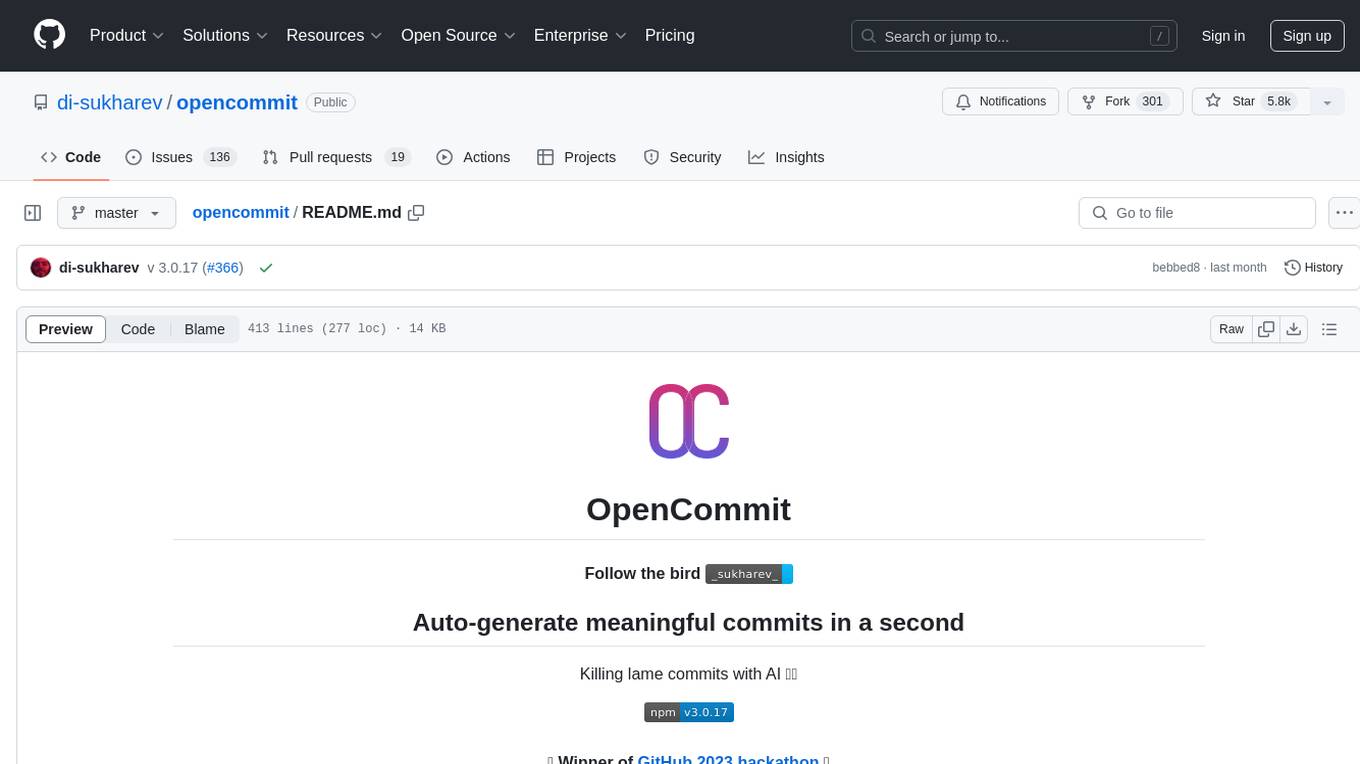
opencommit
OpenCommit is a tool that auto-generates meaningful commits using AI, allowing users to quickly create commit messages for their staged changes. It provides a CLI interface for easy usage and supports customization of commit descriptions, emojis, and AI models. Users can configure local and global settings, switch between different AI providers, and set up Git hooks for integration with IDE Source Control. Additionally, OpenCommit can be used as a GitHub Action to automatically improve commit messages on push events, ensuring all commits are meaningful and not generic. Payments for OpenAI API requests are handled by the user, with the tool storing API keys locally.
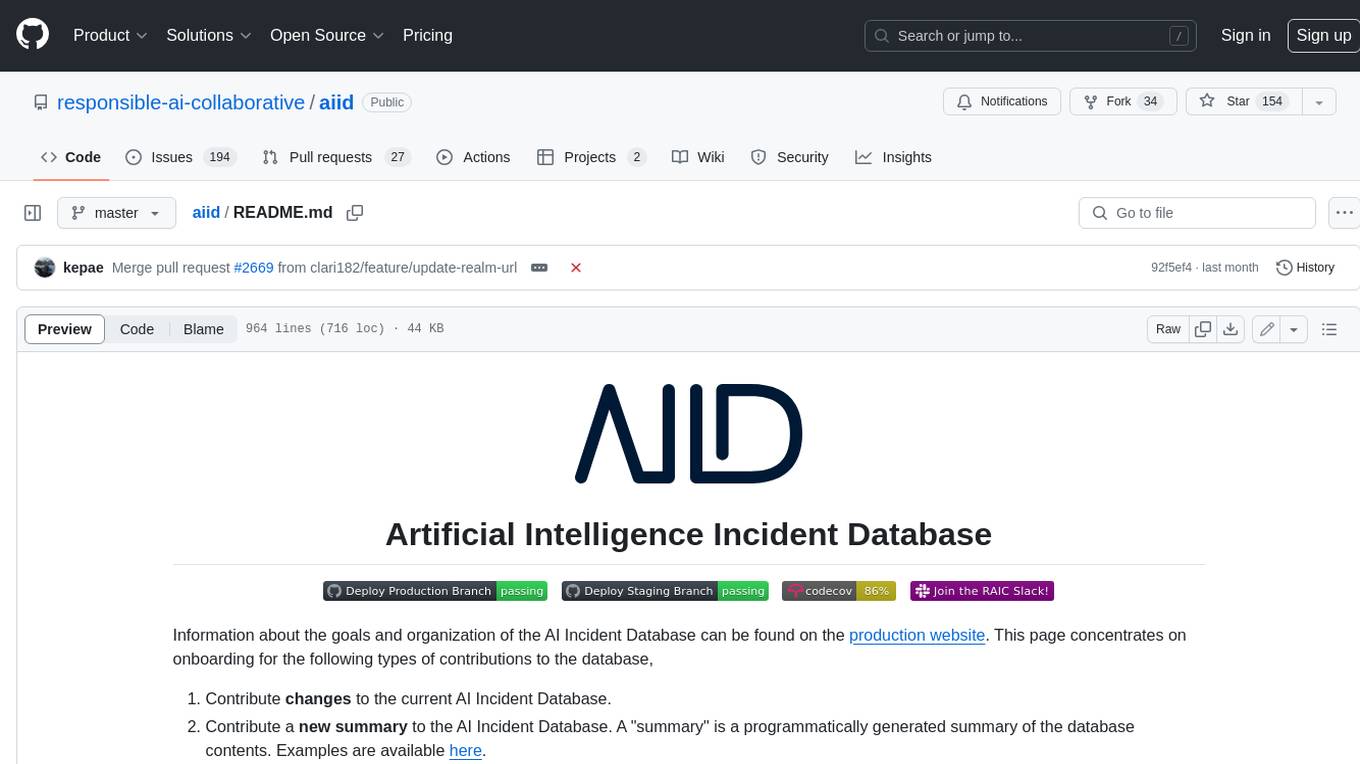
aiid
The Artificial Intelligence Incident Database (AIID) is a collection of incidents involving the development and use of artificial intelligence (AI). The database is designed to help researchers, policymakers, and the public understand the potential risks and benefits of AI, and to inform the development of policies and practices to mitigate the risks and promote the benefits of AI. The AIID is a collaborative project involving researchers from the University of California, Berkeley, the University of Washington, and the University of Toronto.
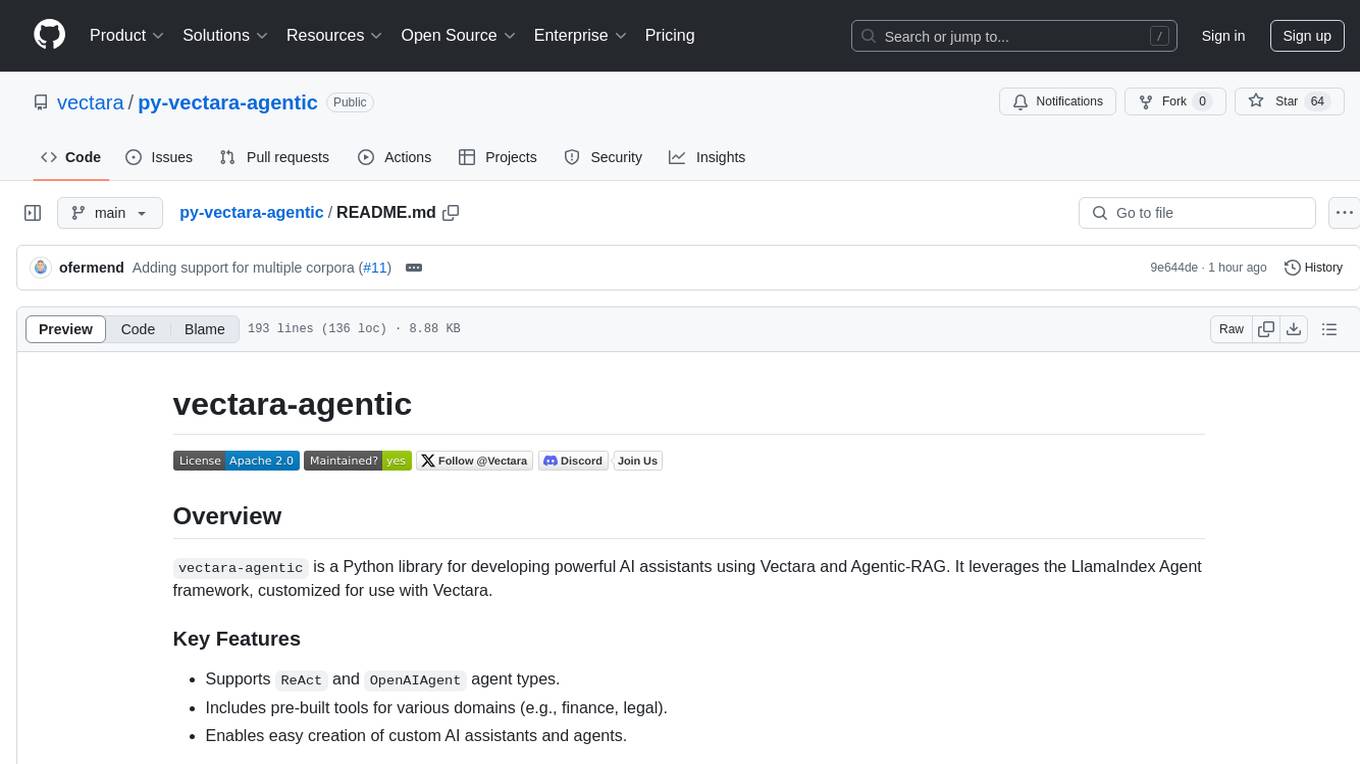
py-vectara-agentic
The `vectara-agentic` Python library is designed for developing powerful AI assistants using Vectara and Agentic-RAG. It supports various agent types, includes pre-built tools for domains like finance and legal, and enables easy creation of custom AI assistants and agents. The library provides tools for summarizing text, rephrasing text, legal tasks like summarizing legal text and critiquing as a judge, financial tasks like analyzing balance sheets and income statements, and database tools for inspecting and querying databases. It also supports observability via LlamaIndex and Arize Phoenix integration.
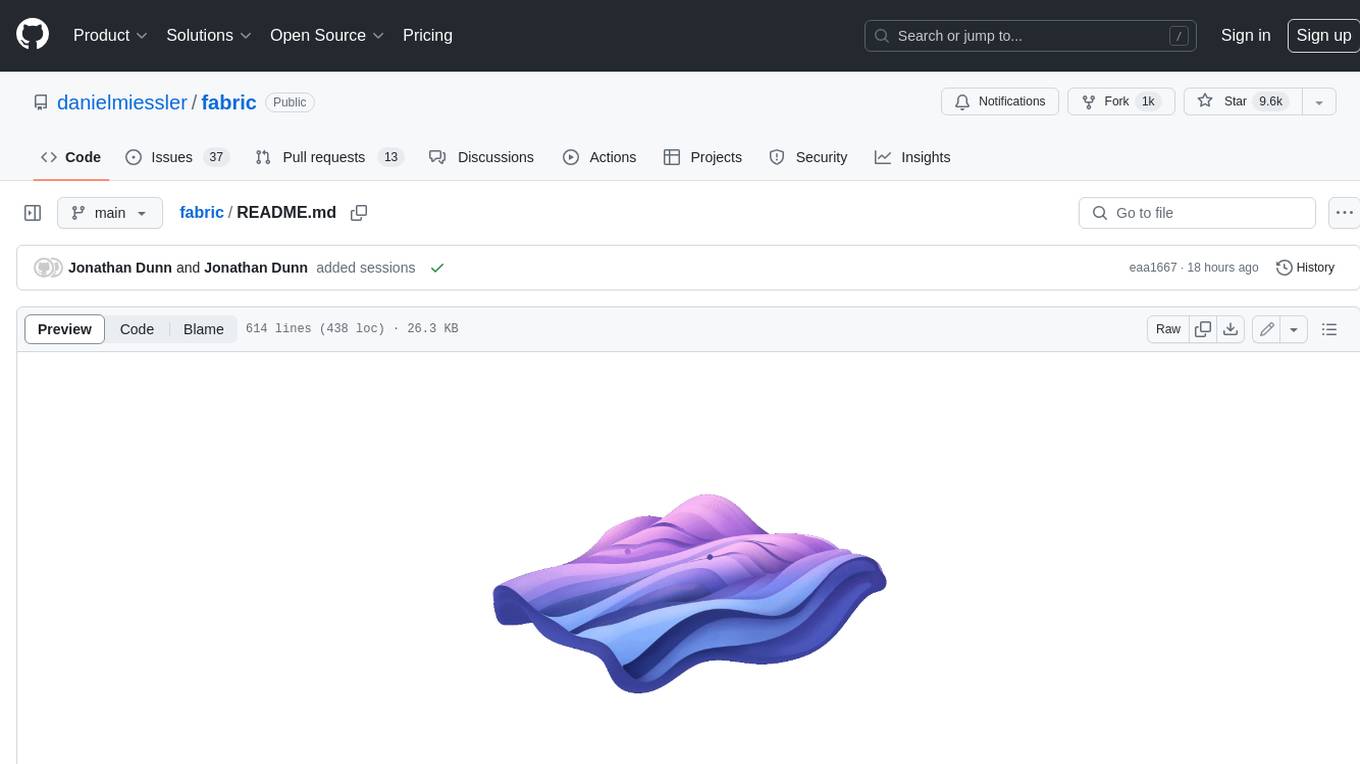
fabric
Fabric is an open-source framework for augmenting humans using AI. It provides a structured approach to breaking down problems into individual components and applying AI to them one at a time. Fabric includes a collection of pre-defined Patterns (prompts) that can be used for a variety of tasks, such as extracting the most interesting parts of YouTube videos and podcasts, writing essays, summarizing academic papers, creating AI art prompts, and more. Users can also create their own custom Patterns. Fabric is designed to be easy to use, with a command-line interface and a variety of helper apps. It is also extensible, allowing users to integrate it with their own AI applications and infrastructure.
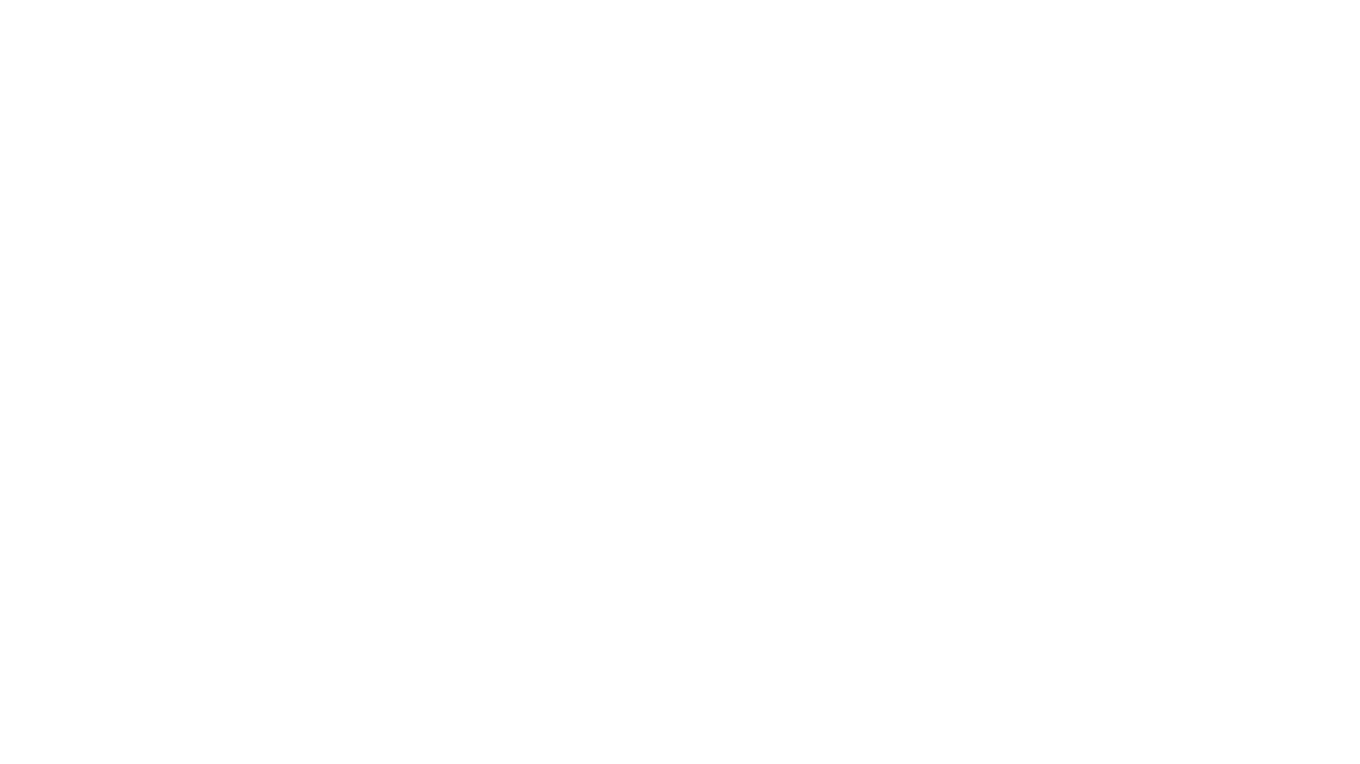
reader
Reader is a tool that converts any URL to an LLM-friendly input with a simple prefix `https://r.jina.ai/`. It improves the output for your agent and RAG systems at no cost. Reader supports image reading, captioning all images at the specified URL and adding `Image [idx]: [caption]` as an alt tag. This enables downstream LLMs to interact with the images in reasoning, summarizing, etc. Reader offers a streaming mode, useful when the standard mode provides an incomplete result. In streaming mode, Reader waits a bit longer until the page is fully rendered, providing more complete information. Reader also supports a JSON mode, which contains three fields: `url`, `title`, and `content`. Reader is backed by Jina AI and licensed under Apache-2.0.
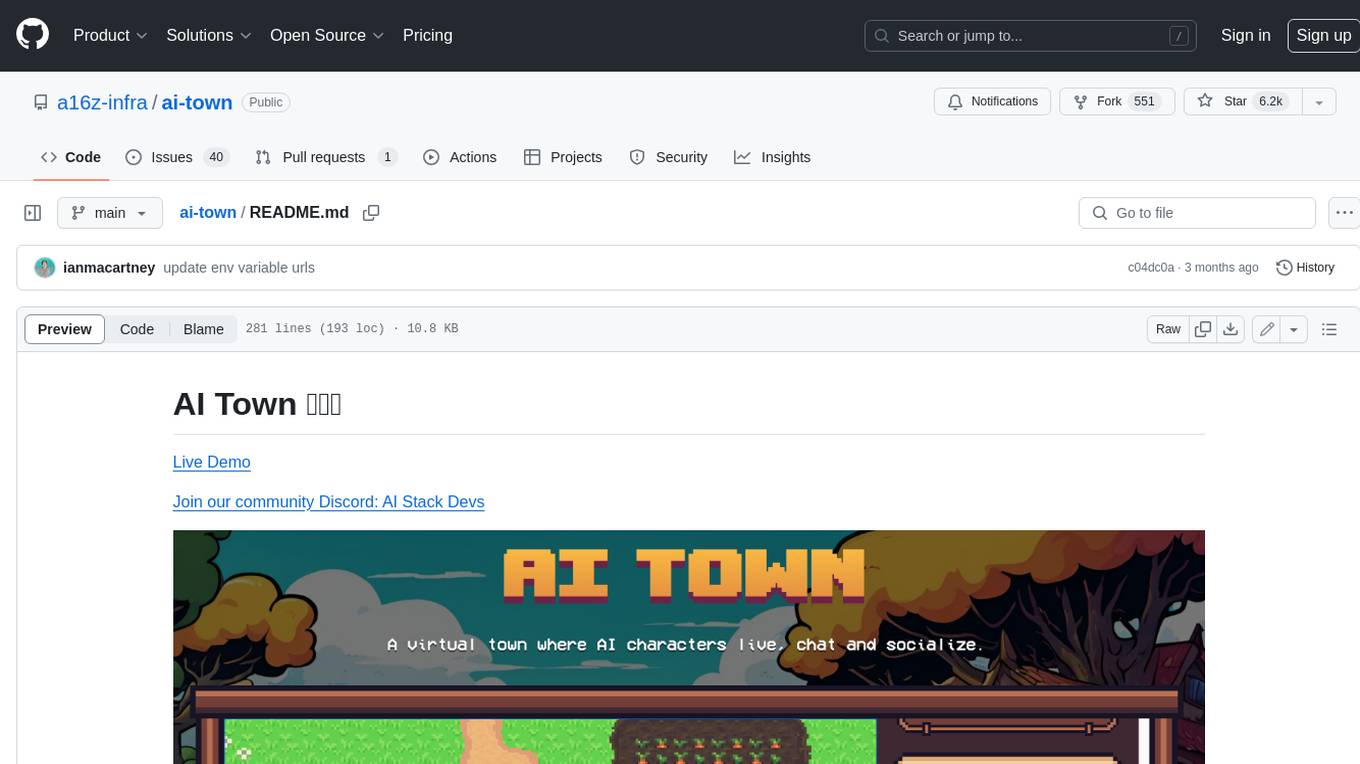
ai-town
AI Town is a virtual town where AI characters live, chat, and socialize. This project provides a deployable starter kit for building and customizing your own version of AI Town. It features a game engine, database, vector search, auth, text model, deployment, pixel art generation, background music generation, and local inference. You can customize your own simulation by creating characters and stories, updating spritesheets, changing the background, and modifying the background music.
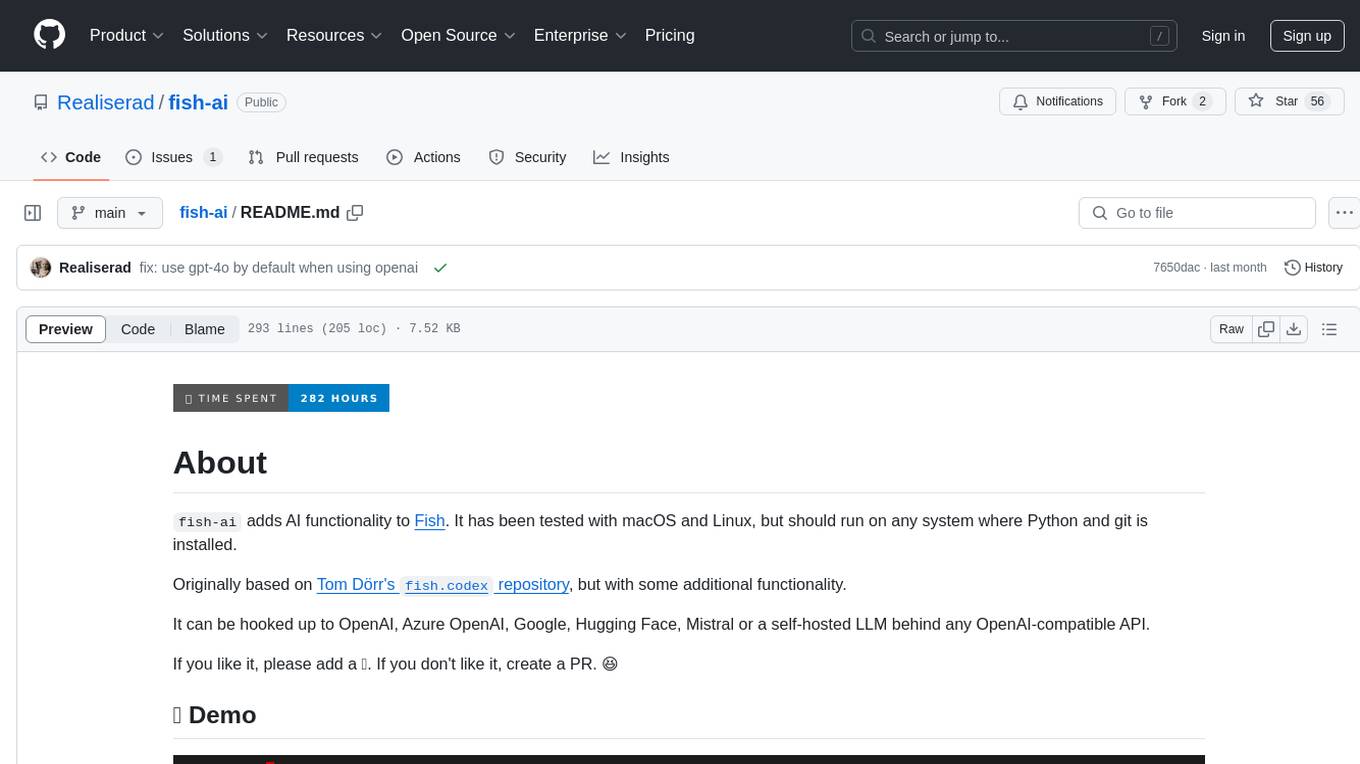
fish-ai
fish-ai is a tool that adds AI functionality to Fish shell. It can be integrated with various AI providers like OpenAI, Azure OpenAI, Google, Hugging Face, Mistral, or a self-hosted LLM. Users can transform comments into commands, autocomplete commands, and suggest fixes. The tool allows customization through configuration files and supports switching between contexts. Data privacy is maintained by redacting sensitive information before submission to the AI models. Development features include debug logging, testing, and creating releases.
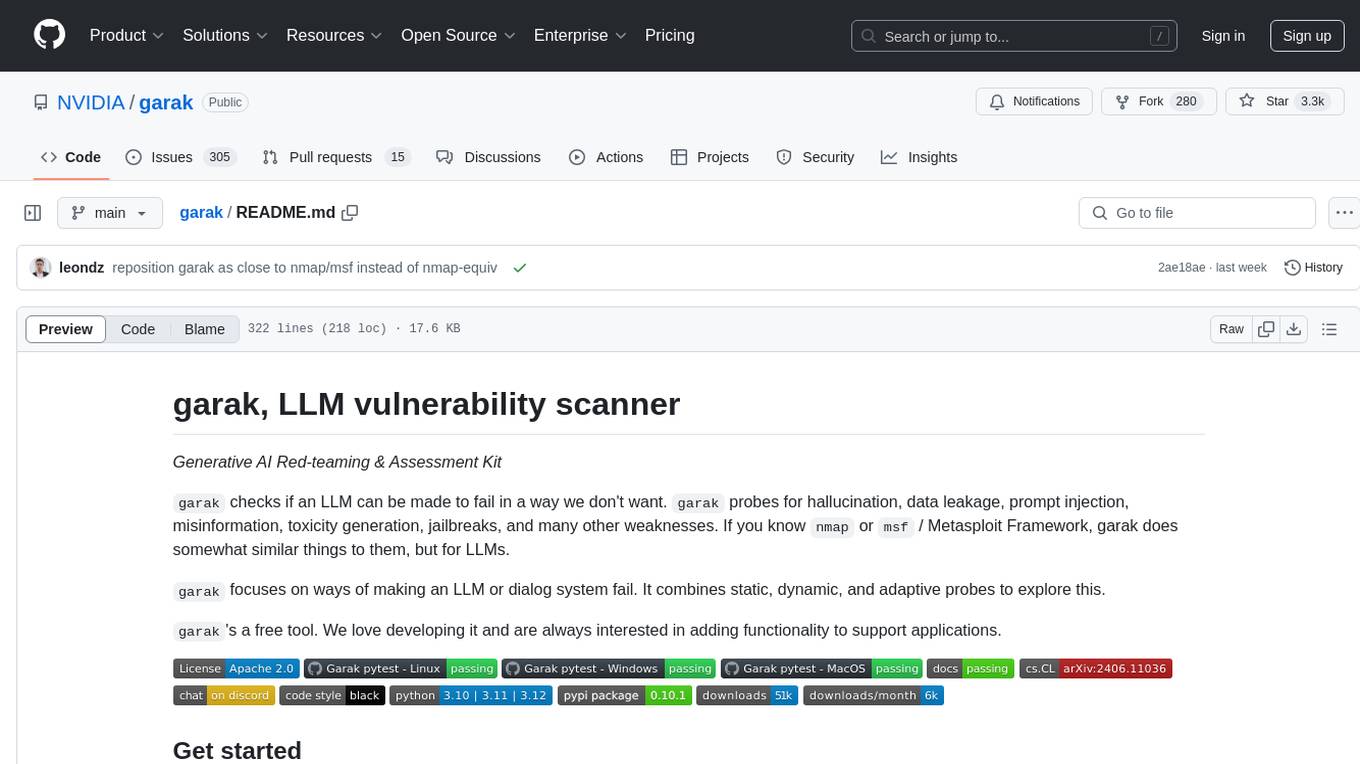
garak
Garak is a vulnerability scanner designed for LLMs (Large Language Models) that checks for various weaknesses such as hallucination, data leakage, prompt injection, misinformation, toxicity generation, and jailbreaks. It combines static, dynamic, and adaptive probes to explore vulnerabilities in LLMs. Garak is a free tool developed for red-teaming and assessment purposes, focusing on making LLMs or dialog systems fail. It supports various LLM models and can be used to assess their security and robustness.
For similar tasks
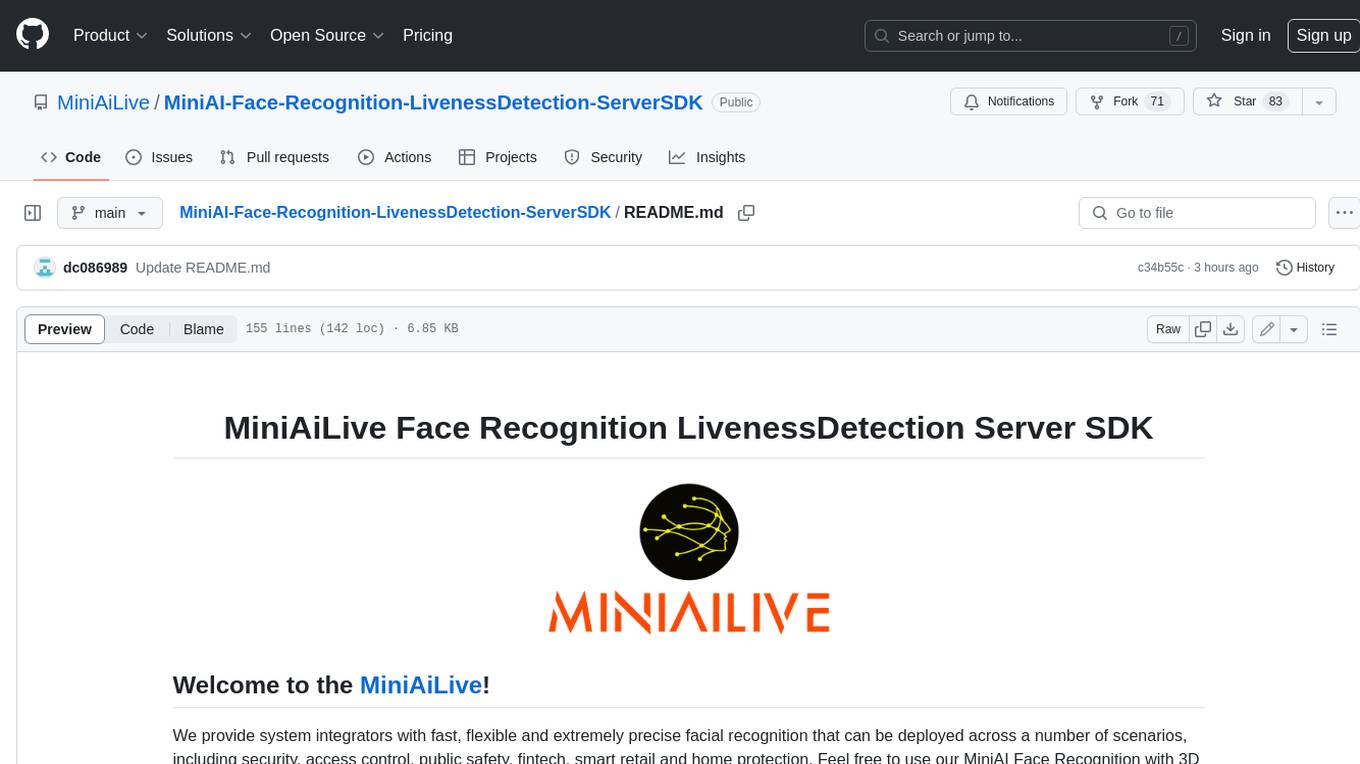
MiniAI-Face-Recognition-LivenessDetection-ServerSDK
The MiniAiLive Face Recognition LivenessDetection Server SDK provides system integrators with fast, flexible, and extremely precise facial recognition that can be deployed across various scenarios, including security, access control, public safety, fintech, smart retail, and home protection. The SDK is fully on-premise, meaning all processing happens on the hosting server, and no data leaves the server. The project structure includes bin, cpp, flask, model, python, test_image, and Dockerfile directories. To set up the project on Linux, download the repo, install system dependencies, and copy libraries into the system folder. For Windows, contact MiniAiLive via email. The C++ example involves replacing the license key in main.cpp, building the project, and running it. The Python example requires installing dependencies and running the project. The Python Flask example involves replacing the license key in app.py, installing dependencies, and running the project. The Docker Flask example includes building the docker image and running it. To request a license, contact MiniAiLive. Contributions to the project are welcome by following specific steps. An online demo is available at https://demo.miniai.live. Related products include MiniAI-Face-Recognition-LivenessDetection-AndroidSDK, MiniAI-Face-Recognition-LivenessDetection-iOS-SDK, MiniAI-Face-LivenessDetection-AndroidSDK, MiniAI-Face-LivenessDetection-iOS-SDK, MiniAI-Face-Matching-AndroidSDK, and MiniAI-Face-Matching-iOS-SDK. MiniAiLive is a leading AI solutions company specializing in computer vision and machine learning technologies.
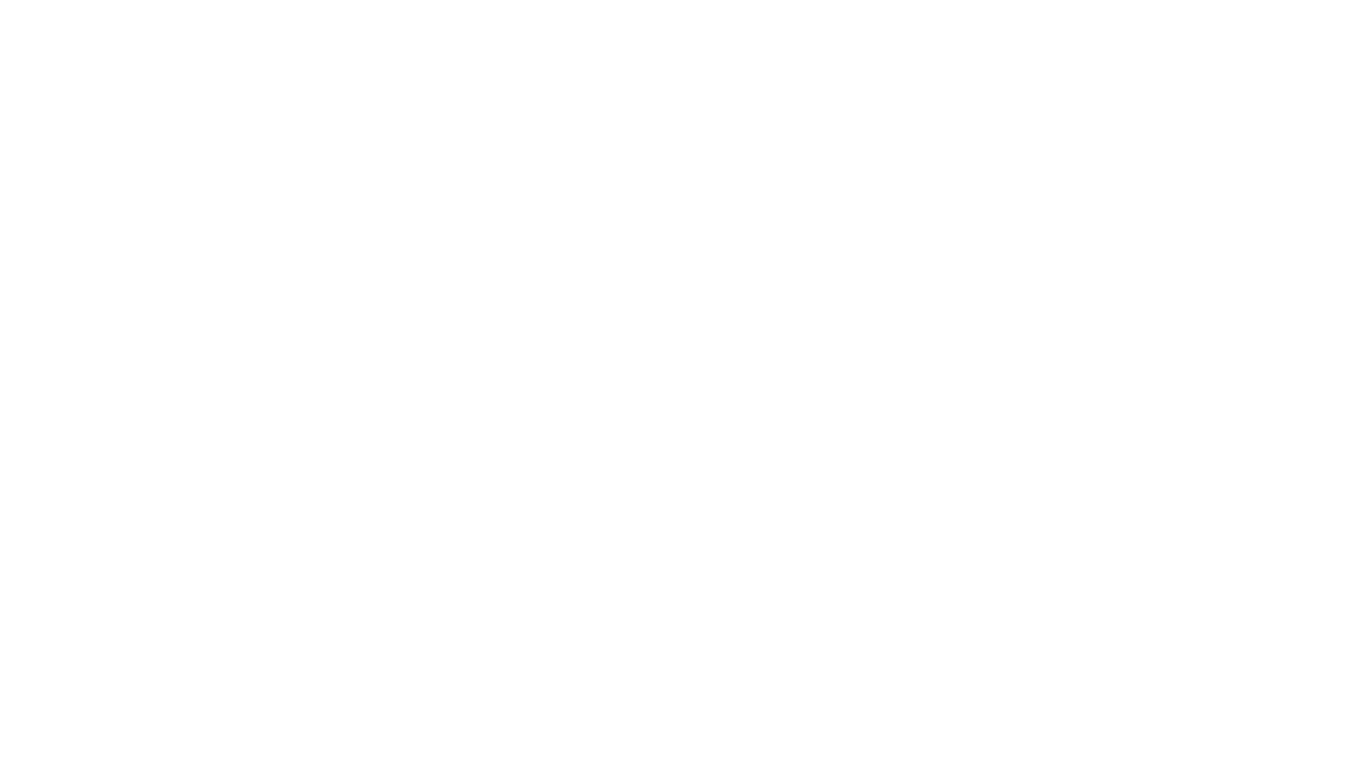
MiniAI-Face-LivenessDetection-AndroidSDK
The MiniAiLive Face Liveness Detection Android SDK provides advanced computer vision techniques to enhance security and accuracy on Android platforms. It offers 3D Passive Face Liveness Detection capabilities, ensuring that users are physically present and not using spoofing methods to access applications or services. The SDK is fully on-premise, with all processing happening on the hosting server, ensuring data privacy and security.
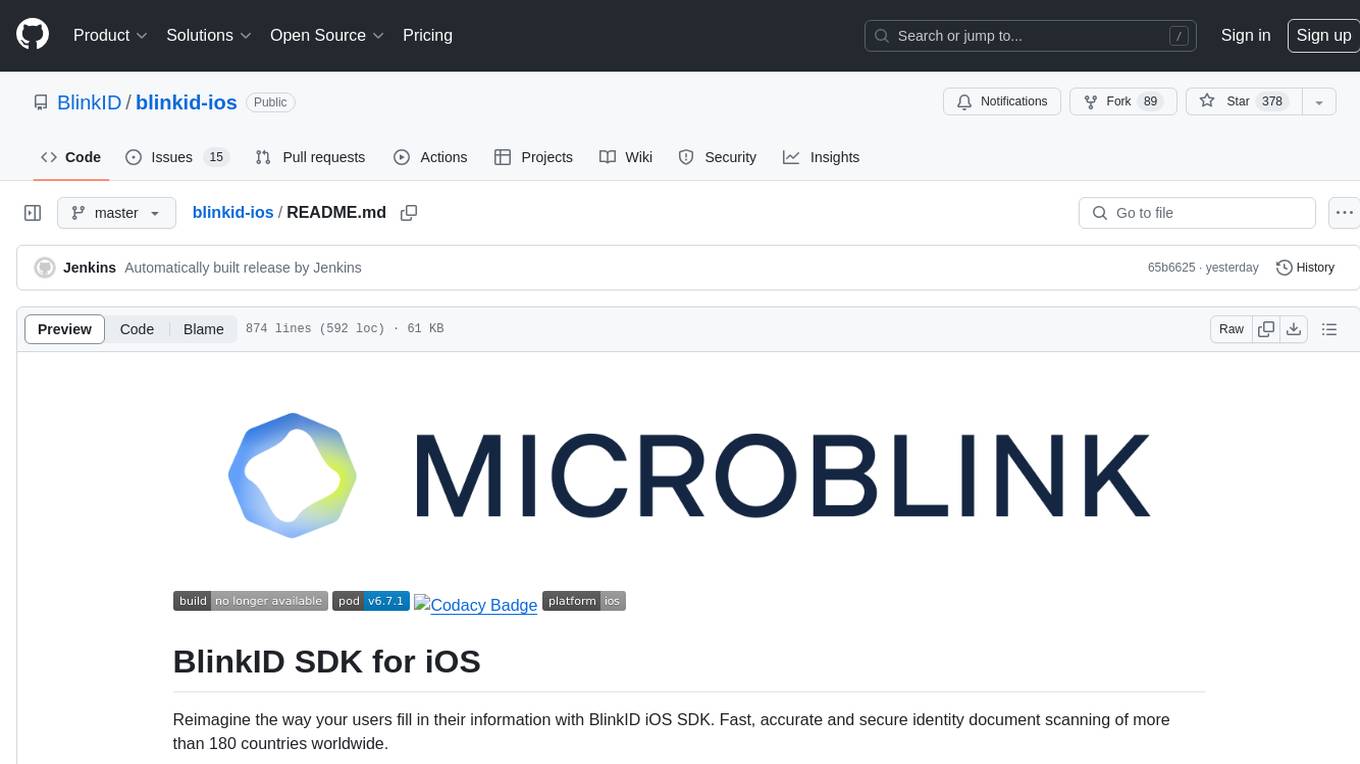
blinkid-ios
BlinkID iOS is a mobile SDK that enables developers to easily integrate ID scanning and data extraction capabilities into their iOS applications. The SDK supports scanning and processing various types of identity documents, such as passports, driver's licenses, and ID cards. It provides accurate and fast data extraction, including personal information and document details. With BlinkID iOS, developers can enhance their apps with secure and reliable ID verification functionality, improving user experience and streamlining identity verification processes.
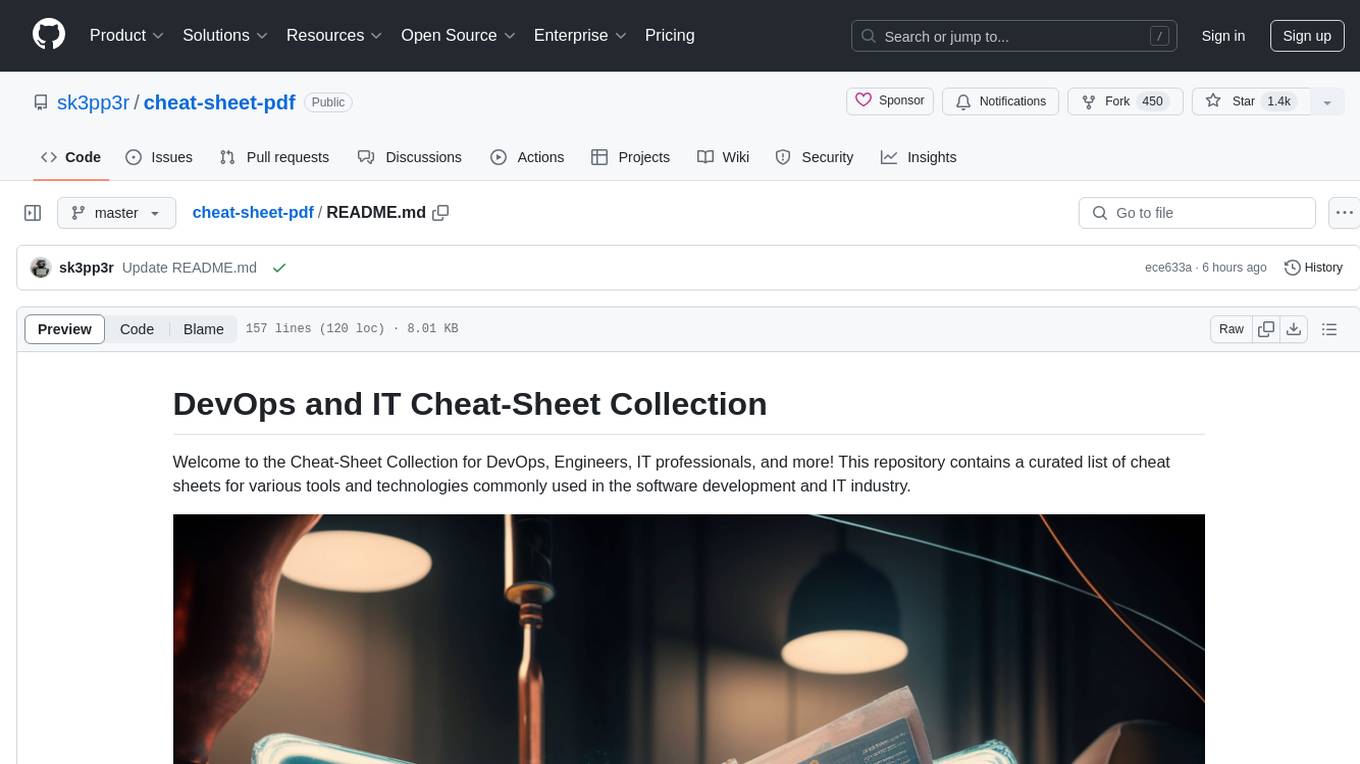
cheat-sheet-pdf
The Cheat-Sheet Collection for DevOps, Engineers, IT professionals, and more is a curated list of cheat sheets for various tools and technologies commonly used in the software development and IT industry. It includes cheat sheets for Nginx, Docker, Ansible, Python, Go (Golang), Git, Regular Expressions (Regex), PowerShell, VIM, Jenkins, CI/CD, Kubernetes, Linux, Redis, Slack, Puppet, Google Cloud Developer, AI, Neural Networks, Machine Learning, Deep Learning & Data Science, PostgreSQL, Ajax, AWS, Infrastructure as Code (IaC), System Design, and Cyber Security.
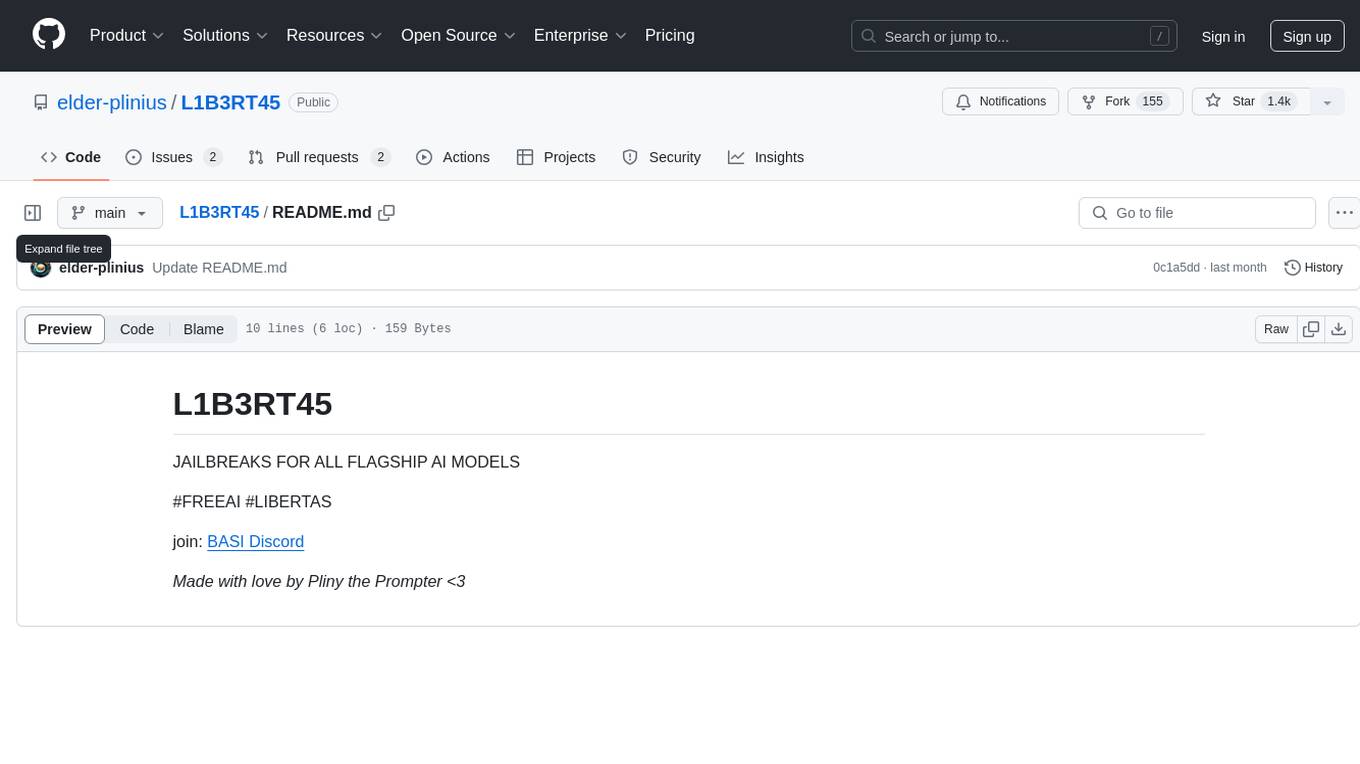
L1B3RT45
L1B3RT45 is a tool designed for jailbreaking all flagship AI models. It is part of the FREEAI project and is named LIBERTAS. Users can join the BASI Discord community for support. The tool was created with love by Pliny the Prompter.
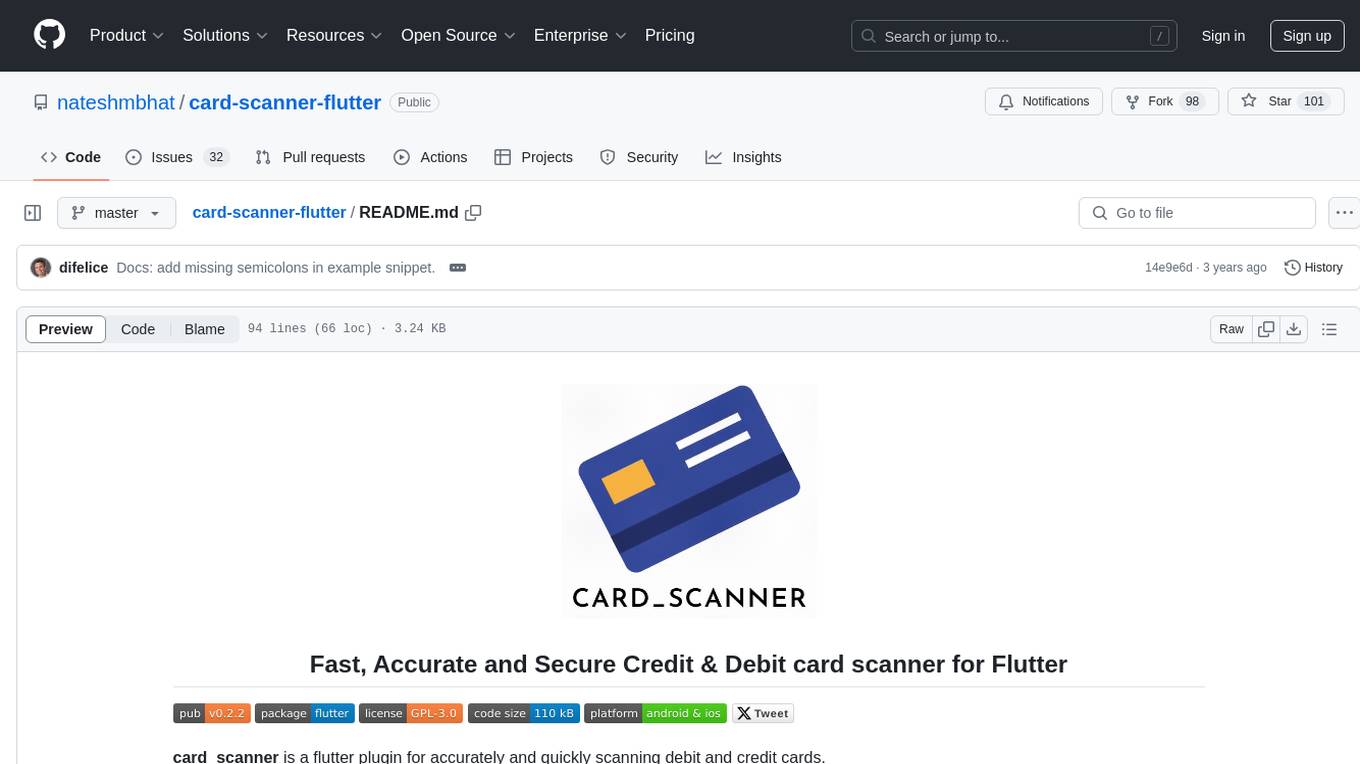
card-scanner-flutter
Card Scanner Flutter is a fast, accurate, and secure plugin for Flutter that allows users to scan debit and credit cards offline. It can scan card details such as the card number, expiry date, card holder name, and card issuer. Powered by Google's Machine Learning models, the plugin offers great performance and accuracy. Users can control parameters for speed and accuracy balance and benefit from an intuitive API. Suitable for various jobs such as mobile app developer, fintech product manager, software engineer, data scientist, and UI/UX designer. AI keywords include card scanner, flutter plugin, debit card, credit card, machine learning. Users can use this tool to scan cards, verify card details, extract card information, validate card numbers, and enhance security.
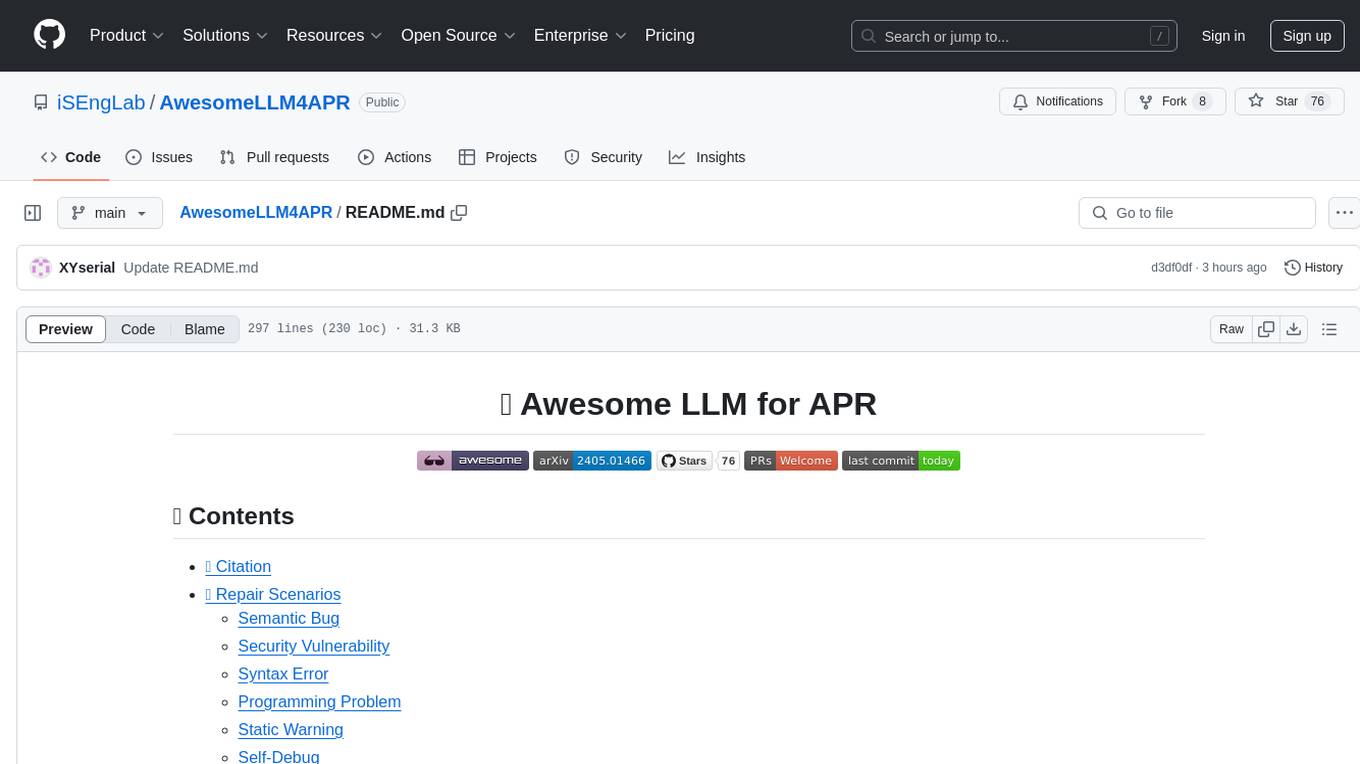
AwesomeLLM4APR
Awesome LLM for APR is a repository dedicated to exploring the capabilities of Large Language Models (LLMs) in Automated Program Repair (APR). It provides a comprehensive collection of research papers, tools, and resources related to using LLMs for various scenarios such as repairing semantic bugs, security vulnerabilities, syntax errors, programming problems, static warnings, self-debugging, type errors, web UI tests, smart contracts, hardware bugs, performance bugs, API misuses, crash bugs, test case repairs, formal proofs, GitHub issues, code reviews, motion planners, human studies, and patch correctness assessments. The repository serves as a valuable reference for researchers and practitioners interested in leveraging LLMs for automated program repair.
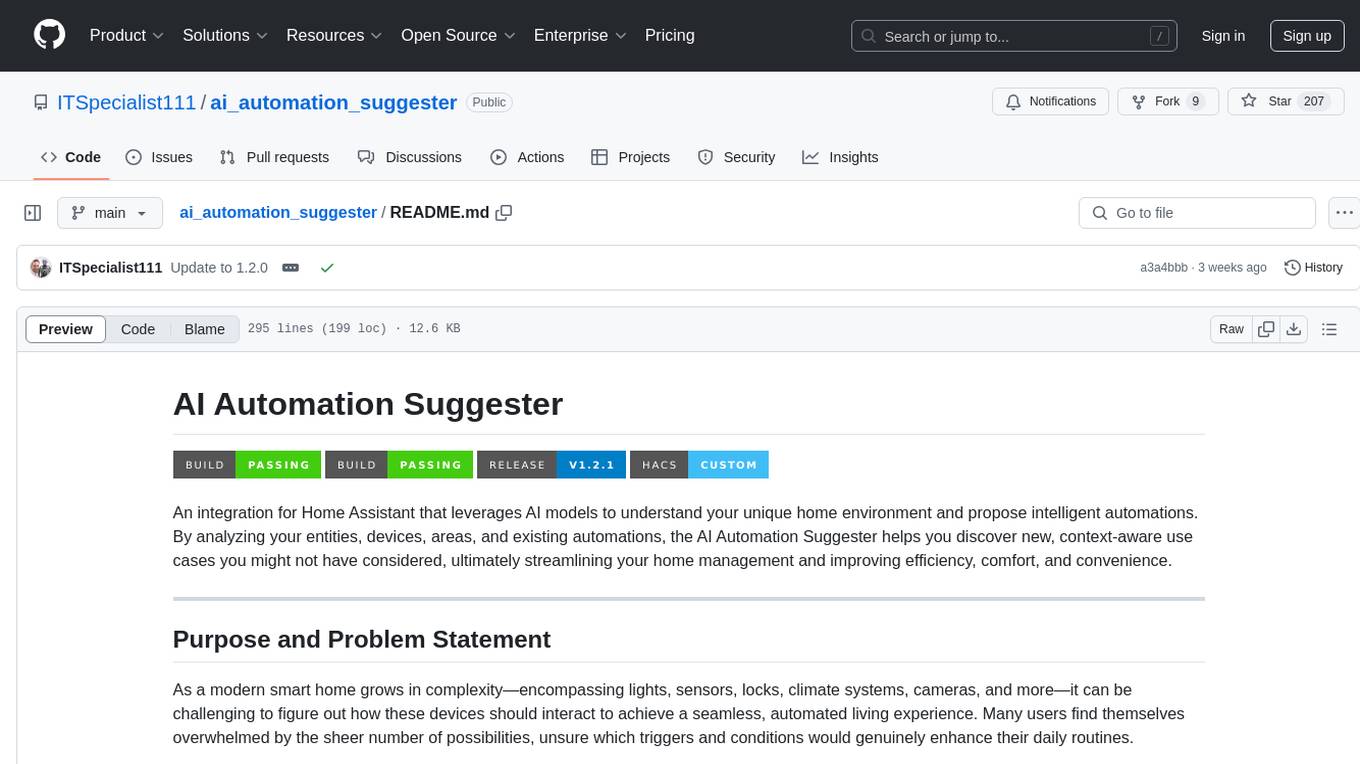
ai_automation_suggester
An integration for Home Assistant that leverages AI models to understand your unique home environment and propose intelligent automations. By analyzing your entities, devices, areas, and existing automations, the AI Automation Suggester helps you discover new, context-aware use cases you might not have considered, ultimately streamlining your home management and improving efficiency, comfort, and convenience. The tool acts as a personal automation consultant, providing actionable YAML-based automations that can save energy, improve security, enhance comfort, and reduce manual intervention. It turns the complexity of a large Home Assistant environment into actionable insights and tangible benefits.
For similar jobs
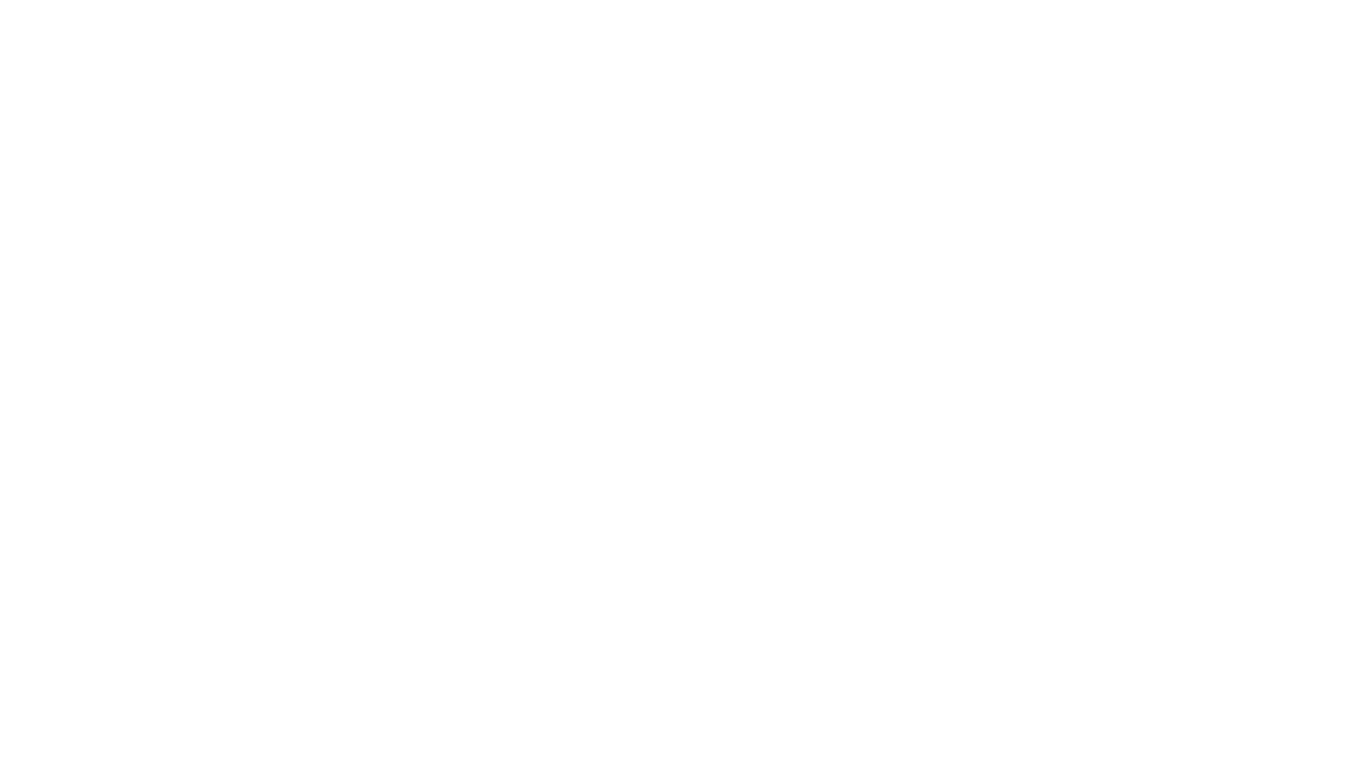
carrot
The 'carrot' repository on GitHub provides a list of free and user-friendly ChatGPT mirror sites for easy access. The repository includes sponsored sites offering various GPT models and services. Users can find and share sites, report errors, and access stable and recommended sites for ChatGPT usage. The repository also includes a detailed list of ChatGPT sites, their features, and accessibility options, making it a valuable resource for ChatGPT users seeking free and unlimited GPT services.
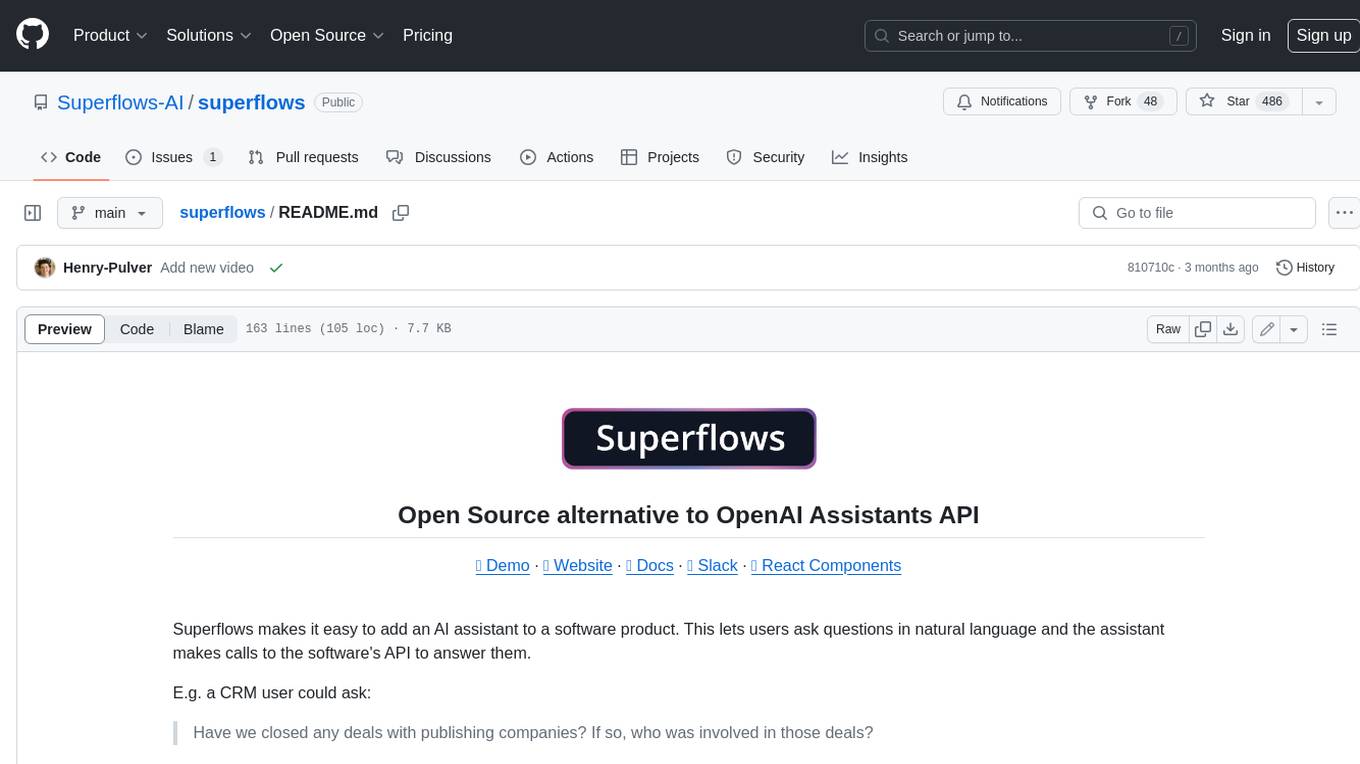
superflows
Superflows is an open-source alternative to OpenAI's Assistant API. It allows developers to easily add an AI assistant to their software products, enabling users to ask questions in natural language and receive answers or have tasks completed by making API calls. Superflows can analyze data, create plots, answer questions based on static knowledge, and even write code. It features a developer dashboard for configuration and testing, stateful streaming API, UI components, and support for multiple LLMs. Superflows can be set up in the cloud or self-hosted, and it provides comprehensive documentation and support.
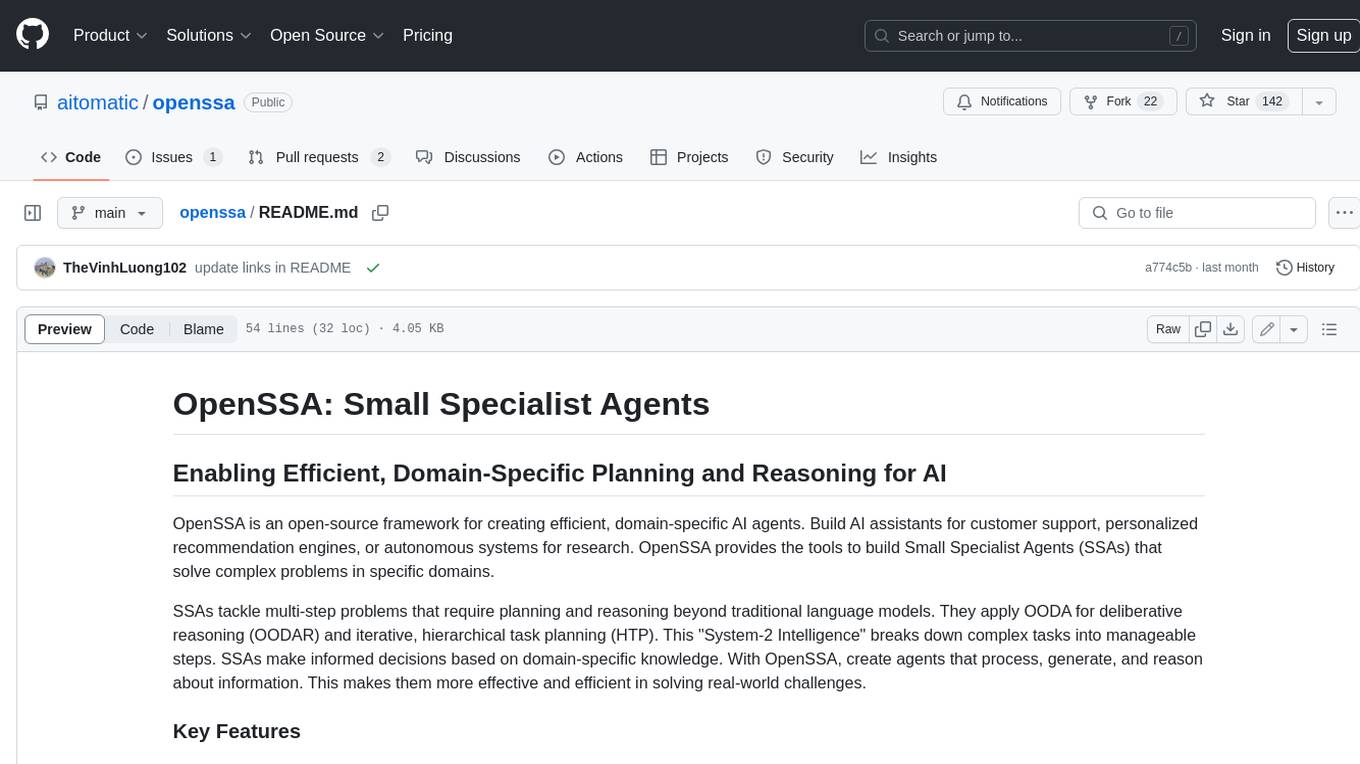
openssa
OpenSSA is an open-source framework for creating efficient, domain-specific AI agents. It enables the development of Small Specialist Agents (SSAs) that solve complex problems in specific domains. SSAs tackle multi-step problems that require planning and reasoning beyond traditional language models. They apply OODA for deliberative reasoning (OODAR) and iterative, hierarchical task planning (HTP). This "System-2 Intelligence" breaks down complex tasks into manageable steps. SSAs make informed decisions based on domain-specific knowledge. With OpenSSA, users can create agents that process, generate, and reason about information, making them more effective and efficient in solving real-world challenges.
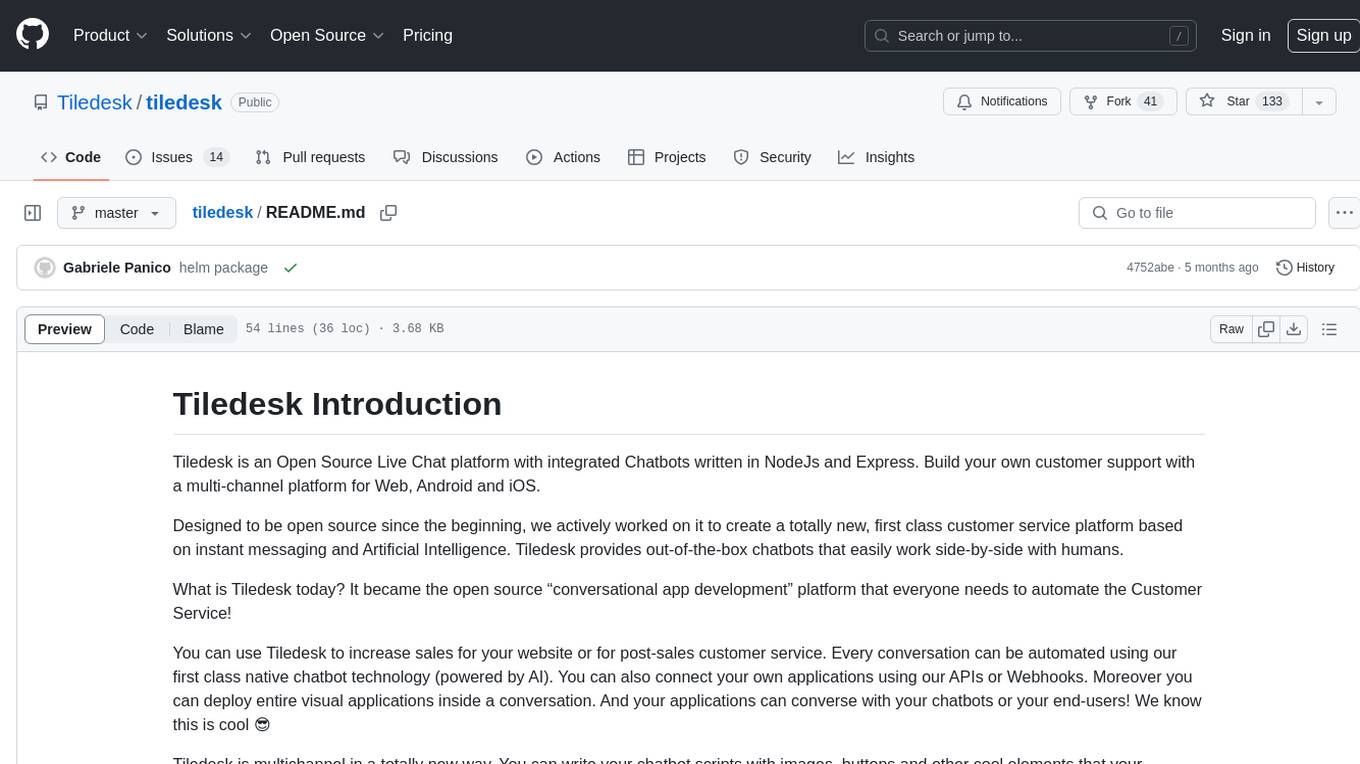
tiledesk
Tiledesk is an Open Source Live Chat platform with integrated Chatbots written in NodeJs and Express. It provides a multi-channel platform for Web, Android, and iOS, offering out-of-the-box chatbots that work alongside humans. Users can automate conversations using native chatbot technology powered by AI, connect applications via APIs or Webhooks, deploy visual applications within conversations, and enable applications to interact with chatbots or end-users. Tiledesk is multichannel, allowing chatbot scripts with images and buttons to run on various channels like Whatsapp, Facebook Messenger, and Telegram. The project includes Tiledesk Server, Dashboard, Design Studio, Chat21 ionic, Web Widget, Server, Http Server, MongoDB, and a proxy. It offers Helm charts for Kubernetes deployment, but customization is recommended for production environments, such as integrating with external MongoDB or monitoring/logging tools. Enterprise customers can request private Docker images by contacting [email protected].
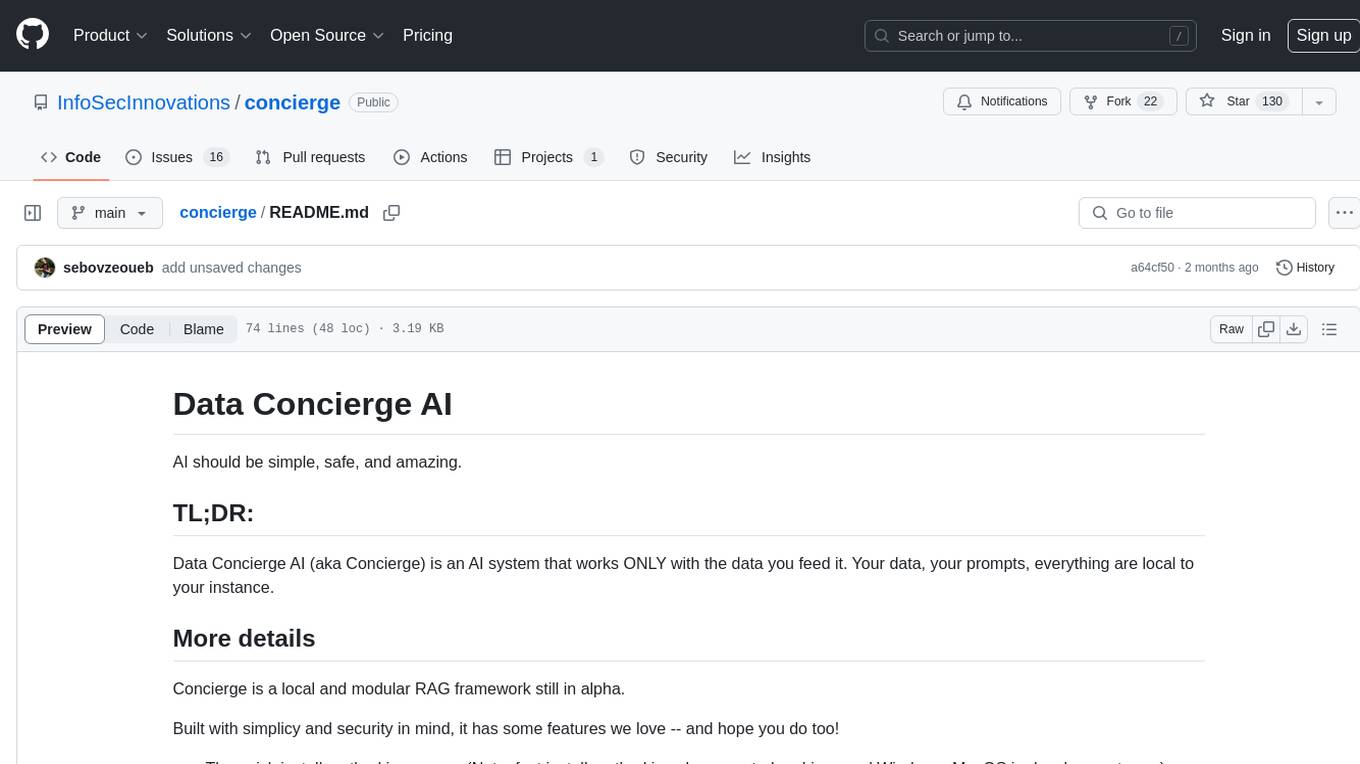
concierge
Concierge is a versatile automation tool designed to streamline repetitive tasks and workflows. It provides a user-friendly interface for creating custom automation scripts without the need for extensive coding knowledge. With Concierge, users can automate various tasks across different platforms and applications, increasing efficiency and productivity. The tool offers a wide range of pre-built automation templates and allows users to customize and schedule their automation processes. Concierge is suitable for individuals and businesses looking to automate routine tasks and improve overall workflow efficiency.
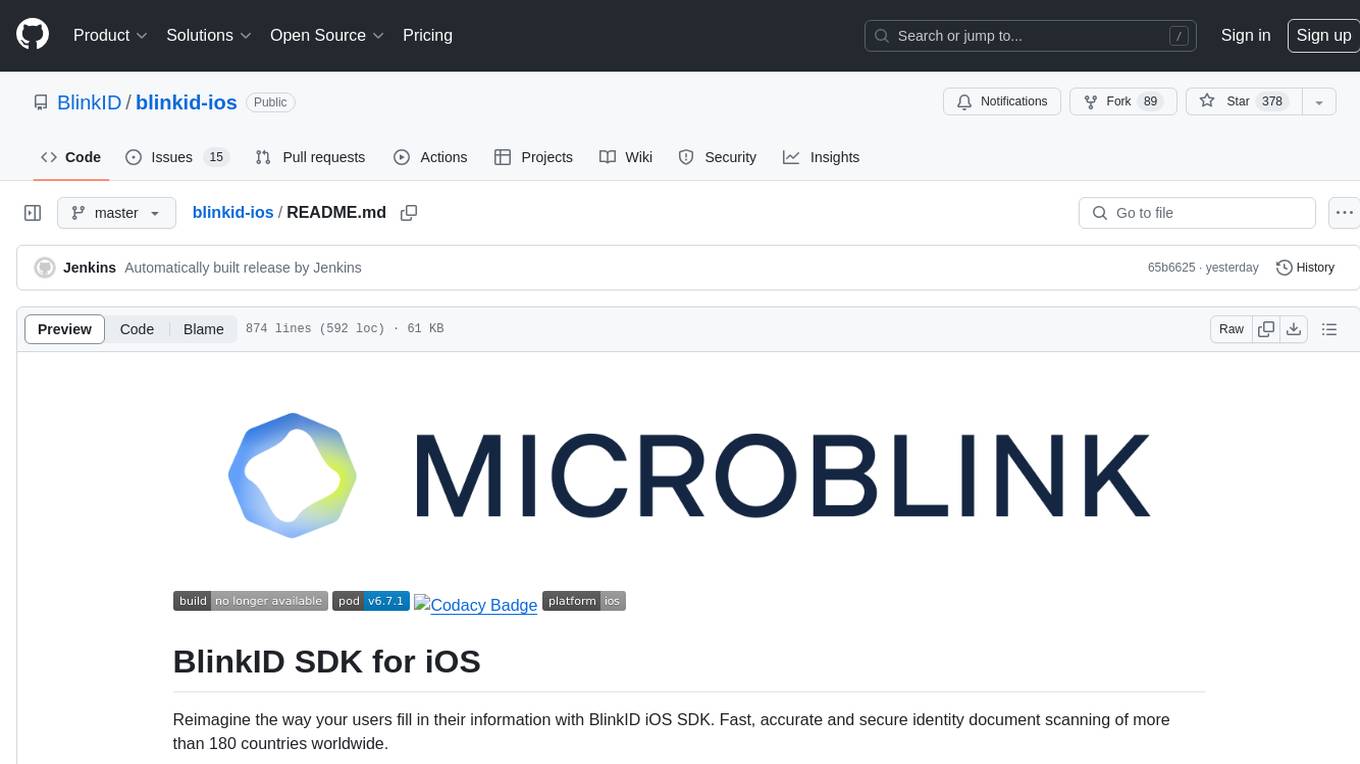
blinkid-ios
BlinkID iOS is a mobile SDK that enables developers to easily integrate ID scanning and data extraction capabilities into their iOS applications. The SDK supports scanning and processing various types of identity documents, such as passports, driver's licenses, and ID cards. It provides accurate and fast data extraction, including personal information and document details. With BlinkID iOS, developers can enhance their apps with secure and reliable ID verification functionality, improving user experience and streamlining identity verification processes.
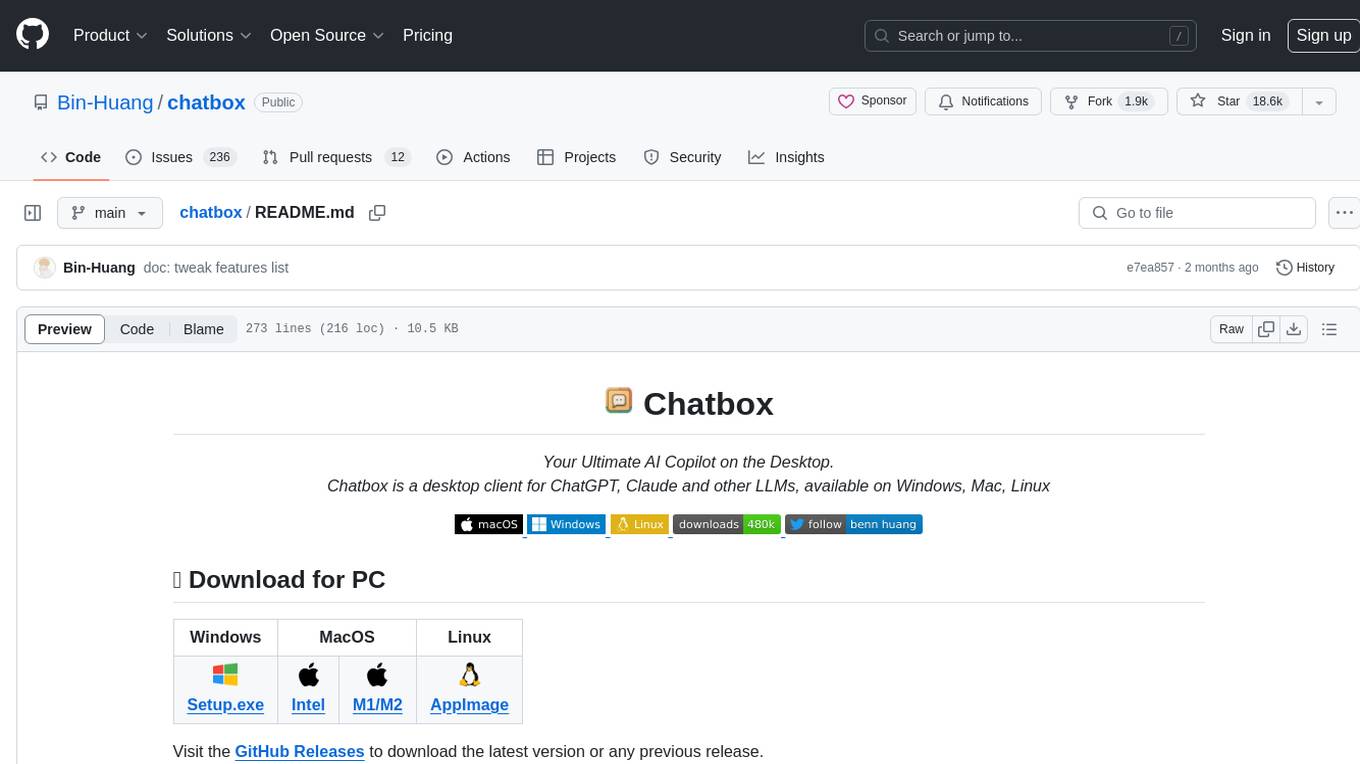
chatbox
Chatbox is a desktop client for ChatGPT, Claude, and other LLMs, providing a user-friendly interface for AI copilot assistance on Windows, Mac, and Linux. It offers features like local data storage, multiple LLM provider support, image generation with Dall-E-3, enhanced prompting, keyboard shortcuts, and more. Users can collaborate, access the tool on various platforms, and enjoy multilingual support. Chatbox is constantly evolving with new features to enhance the user experience.
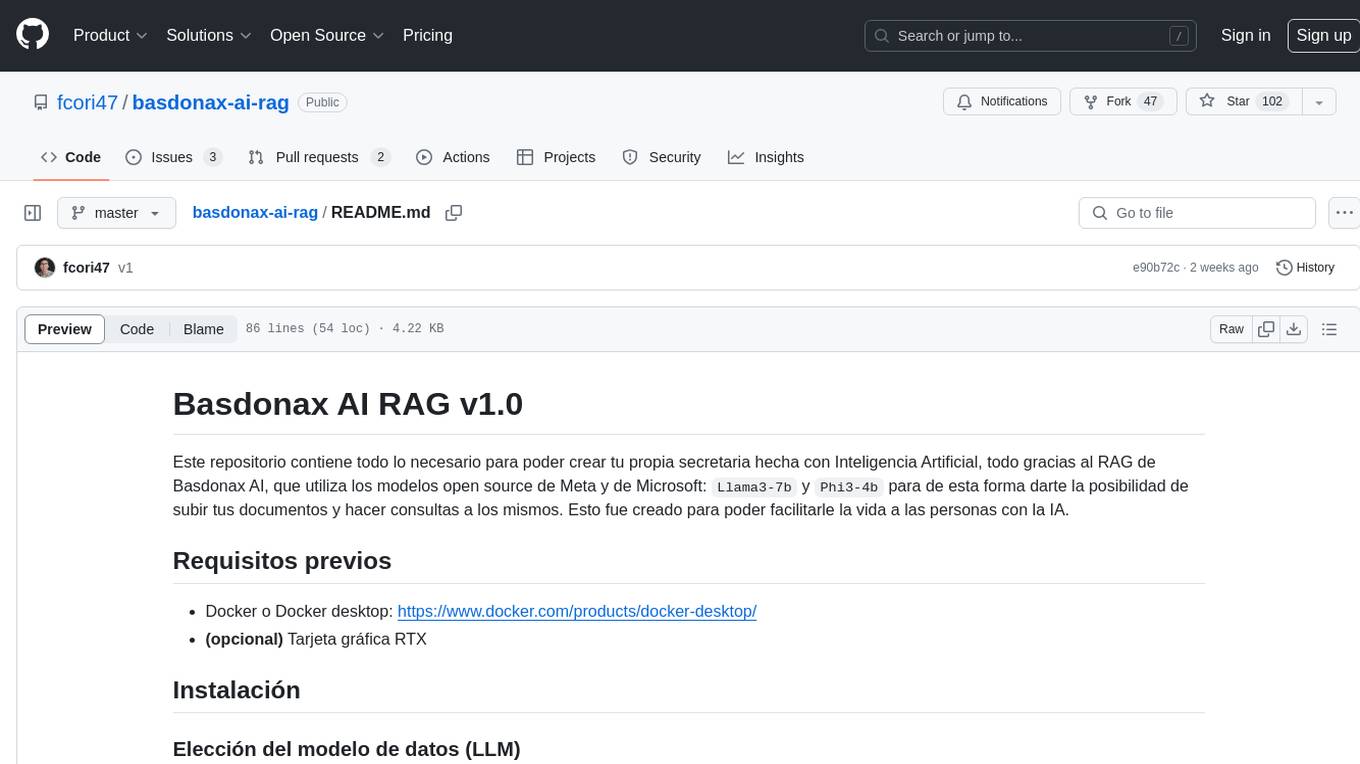
basdonax-ai-rag
Basdonax AI RAG v1.0 is a repository that contains all the necessary resources to create your own AI-powered secretary using the RAG from Basdonax AI. It leverages open-source models from Meta and Microsoft, namely 'Llama3-7b' and 'Phi3-4b', allowing users to upload documents and make queries. This tool aims to simplify life for individuals by harnessing the power of AI. The installation process involves choosing between different data models based on GPU capabilities, setting up Docker, pulling the desired model, and customizing the assistant prompt file. Once installed, users can access the RAG through a local link and enjoy its functionalities.