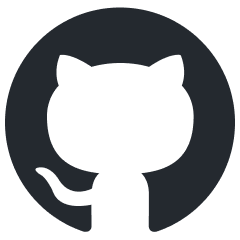
ShortcutsBench
ShortcutsBench: A Large-Scale Real-World Benchmark for API-Based Agents
Stars: 72
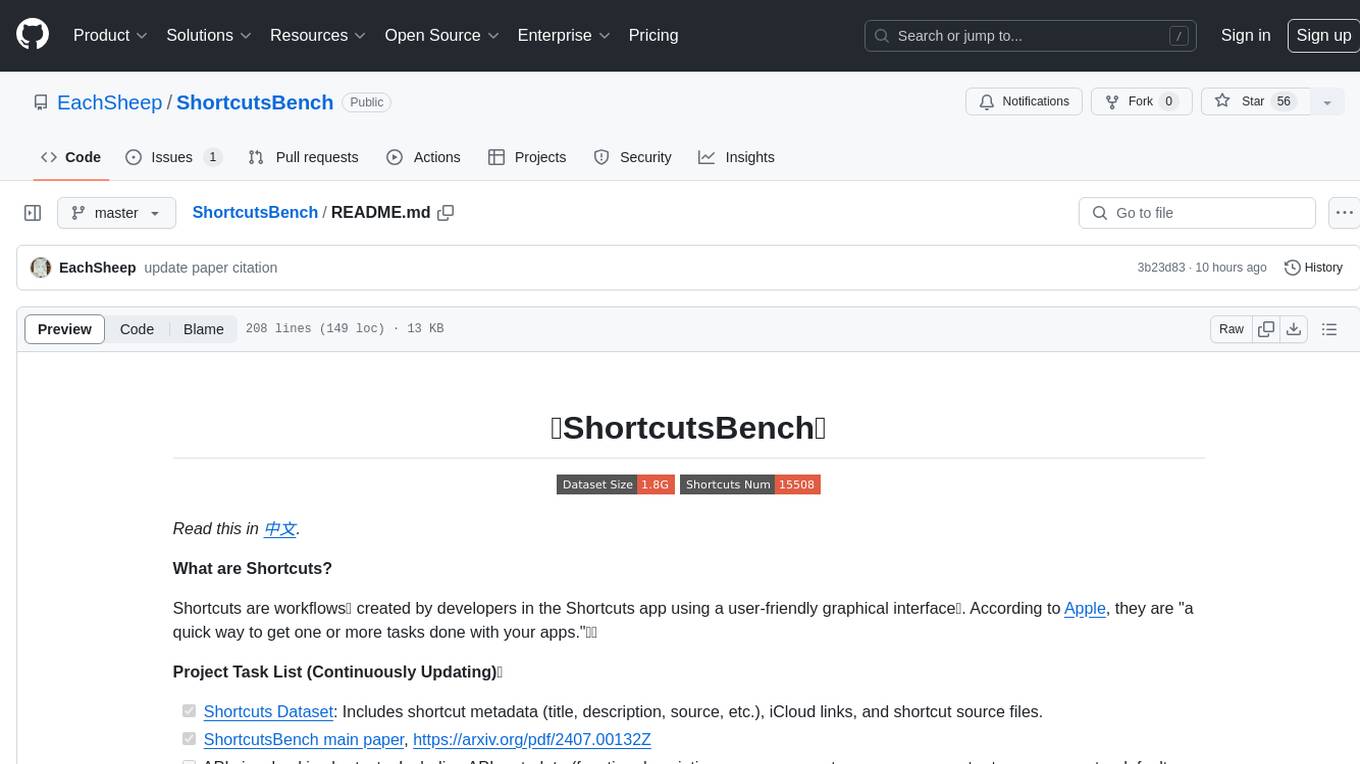
ShortcutsBench is a project focused on collecting and analyzing workflows created in the Shortcuts app, providing a dataset of shortcut metadata, source files, and API information. It aims to study the integration of large language models with Apple devices, particularly focusing on the role of shortcuts in enhancing user experience. The project offers insights for Shortcuts users, enthusiasts, and researchers to explore, customize workflows, and study automated workflows, low-code programming, and API-based agents.
README:
Read this in ไธญๆ.
What are Shortcuts?
Shortcuts are workflows built by developers in the Shortcuts app using a user-friendly graphical interface ๐ผ๏ธ with the provided basic actions. Apple describes them as "a quick way to get one or more tasks done with your apps." ๐ฑ
Project Task List (Continuously Updated) ๐
All data, data acquisition processes, data generated during cleaning, cleaning scripts, experiment scripts, results, and related files can be found in the following documents: deves_dataset/dataset_src/README.md
(English) or Chinese, deves_dataset/dataset_src_valid_apis/README.md
(English) or Chinese, and experiments/README.md
(English) or Chinese.
- [x] ShortcutsBench Paper Main Text
- [x] ShortcutsBench Paper Appendix
- [x] Scripts for Data Acquisition, Data Cleaning and Processing, Experiment Code, and Experiment Results
- [x] We provide shortcuts with bilingual explanations for regular users: listed in
users_dataset/${website name}/${category name}/README.md
(English) orusers_dataset/${website name}/${category name}/README_ZH.md
(Chinese). Regular users can find suitable shortcuts for their work or life in our repository, which they can import into the Shortcuts app on Apple devices. Each shortcut includes:- The iCloud link for the shortcut
- A description of the shortcut's functionality
- The source of the shortcut
-
For Shortcut Researchers:
ShortcutsBench
provides: (1) Shortcuts (i.e., sequences of actions ingolden
); (2) Queries (i.e., tasks assigned to the agent); (3) APIs (i.e., tools available to the agent).-
[x] Shortcuts
-
[x] Raw Shortcut Dataset, i.e., the file
1_final_detailed_records_remove_repeat.json
, can be downloaded as described indeves_dataset/dataset_src/README.md
(English) ordeves_dataset/dataset_src/README_ZH.md
(Chinese), or directly from Google Drive or Baidu Cloud (password:shortcutsbench
).The APIs involved in the shortcuts in this file may not have corresponding API definition files.
-
[x] Filtered Shortcut Dataset, i.e., the file
1_final_detailed_records_filter_apis.json
, can be downloaded as described indeves_dataset/dataset_src/README.md
(English) ordeves_dataset/dataset_src/README_ZH.md
(Chinese), or directly from Google Drive or Baidu Cloud (password:shortcutsbench
).The APIs involved in the shortcuts in this file all have corresponding API definition files. This file is a cleaned version of
1_final_detailed_records_remove_repeat.json
. If a shortcut contains APIs without definition files, the shortcut is removed. -
[x] Shortcuts Dataset
<=30
, i.e., the file1_final_detailed_records_filter_apis_leq_30.json
, can be downloaded as described inexperiments/README.md
(English) orexperiments/README_ZH.md
(Chinese), or directly from Google Drive or Baidu Cloud (password:shortcutsbench
).Considering the context length limitation of language models, we only evaluated shortcuts with lengths
<=30
in the ShortcutsBench paper.
-
-
[x] Queries. The generated queries are shown in
generated_success_queries.json
, which can be obtained from Google Drive or Baidu Cloud (password:shortcutsbench
).The queries are generated based on
1_final_detailed_records_filter_apis_leq_30.json
. -
[x] APIs. The obtained APIs are shown in
4_api_json_filter.json
, which can be obtained from Google Drive or Baidu Cloud (password:shortcutsbench
).4_api_json_filter.json
has been manually deduplicated, but a few duplicates remain. The raw unprocessed files extracted directly from the app are in4_api_json.json
, which can be obtained from Google Drive or Baidu Cloud (password:shortcutsbench
).
-
The Apple Developer Conference WWDC'24 introduced a lot of AI features on Apple devices ๐ค. We are very interested in how Apple combines large language models like ChatGPT with devices to provide users with a smarter experience ๐ก. In this process, shortcuts will play a significant role! ๐
You can find your favorite shortcuts in this dataset ๐ฑ to help you complete various complex tasks with one click! For example:
-
๐ก Daily Life ๐คน
-
๐๏ธ Shopping Enthusiasts ๐
-
๐งโ๐ Students ๐งฎ
- Calculator
- Relax Your Mind
- ......
-
โจ๏ธ Writers ๐ฃ
- Translator
- Create PDF
- ......
-
๐งโ๐ฌ Researchers ๐ซ
- Get arXiv BibTeX Entry
- ......
-
.....
- Research on building automated workflows: Shortcuts are essentially workflows composed of a series of API calls (actions) provided by Apple and third-party apps ๐.
- Research on low-code programming: Shortcuts include features like branches, loops, and variable assignments, while having a user-friendly graphical interface ๐ฅ๏ธ.
- Research on API-based agents: Enabling large language models to autonomously decide whether, when, and how to use APIs based on user queries (tasks) ๐ง.
- Research on fine-tuning large language models using shortcuts to closely integrate language models with phones, computers, and smartwatches, achieving the vision of an "operating system based on large language models" ๐.
- ......
ShortcutsBench has significant advantages in terms of the authenticity, richness, and complexity of APIs, the validity of queries and corresponding action sequences, the accurate filling of parameter values, the awareness of obtaining information from the system or users, and the overall scale.
To our knowledge, ShortcutsBench is the first large-scale agent benchmark based on real APIs, considering APIs, queries, and corresponding action sequences. ShortcutsBench provides a rich set of real APIs, queries of varying difficulty and task types, high-quality human-annotated action sequences (provided by shortcut developers), and queries from real user needs. Additionally, it offers precise parameter value filling, including raw data types, enumeration types, and using outputs from previous actions as parameter values, and evaluates the agent's awareness of requesting necessary information from the system or users. Moreover, the scale of APIs, queries, and corresponding action sequences in ShortcutsBench rivals or even surpasses benchmarks and datasets created by LLMs or modified from existing datasets. A comprehensive comparison between ShortcutsBench and existing benchmarks/datasets is shown in the table below.
If you find this project helpful, please give us a Star โญ๏ธ! Thank you for your support! ๐
Keywords: Shortcuts, Apple, WWDC'24, Siri, iOS, macOS, watchOS, Workflow, API Calls, Low-Code Programming, Agent, Large Language Model
In this repository, the users_dataset/${website name}/${category name}/README.md
file records the metadata of all shortcuts in the category, including name, description, iCloud download link, etc. Each README.md
file follows this structure:
### Name: Wine Shops # Shortcut Name
- URL: https://www.icloud.com/shortcuts/78ffd18288fd4da286bfd570993ea46e # iCloud Link
- Source: https://shortcutsgallery.com # Source
- Description: Look for Wine shops near you # Description
Use the shortcut Ctrl + F
to search by keyword in the shortcut name directly in your browser ๐. You can also visit Shortcut Collection Sites to search for the shortcuts you want ๐.
On your Apple device, click the iCloud link in the URL, and the shortcut will automatically open and be imported into your Shortcuts app ๐ฒ.
Besides downloading shortcuts one by one using the iCloud links, you can directly get the complete data from the following links:
The shortcut source data in the cloud drive is organized in the following directory structure:
users_dataset/
โโโ matthewcassinelli.com_sirishortcuts_library_free # Website Name
โ โโโ file1
โ โโโ file2
โ โโโ file3
or
users_dataset/
โโโ jiejingku.net # Website Name
โ โโโ category1 # Category
โ โ โโโ file1 # Each specific shortcut
โ โ โโโ file2
โ โโโ category2
โ โ โโโ file3
Each file represents a shortcut. The file name is generated by simply processing the shortcut name, using the following code:
file_name = re.sub(r'[^a-zA-Z0-9]', '_', name)
The shortcut source files we provide are in JSON
format, whereas shortcuts exported from Apple devices are in the form of iCloud links (shared as links) or encrypted shortcut files with the .shortcut
extension.
To import a shortcut source file into the Shortcuts app on macOS
, follow these steps:
- Convert the
JSON
file format toPLIST
format ๐:import xml.etree.ElementTree as ET def parse_element(element): """ Recursively parse XML elements and return dictionaries and lists. """ if element.tag == 'dict': return {element[i].text: parse_element(element[i+1]) for i in range(0, len(element), 2)} elif element.tag == 'array': return [parse_element(child) for child in element] elif element.tag == 'true': return True elif element.tag == 'false': return False elif element.tag == 'integer': return int(element.text) elif element.tag == 'string': return element.text elif element.tag == 'real': return float(element.text) else: raise ValueError("Unsupported tag: " + element.tag) tree = ET.parse(file_path) root_element = tree.getroot() parsed_data = parse_element(root_element[0]) data = parsed_data save_path = "./" with open(save_path, 'w') as f: json.dump(data, f, indent=4)
- Sign the
PLIST
file ๐ usingshortcuts sign --mode anyone --input $input_file --output $output_file
, replacing$input_file
and$output_file
with the actual file paths. - Import the signed file into the Shortcuts app ๐ฒ.
We detail the construction process of ShortcutsBench in the main text of our paper. For more details, please refer to our paper. Below are some additional details.
How to use shortcuts? How to share shortcuts? How to view the source files of shortcuts?
-
Import shortcuts into the Shortcuts app.
You can import shortcuts into the Shortcuts app on Apple devices by clicking the iCloud link and using the shortcut as a regular user.
-
Share shortcuts.
- You can share the shortcut as an iCloud link using the
Share
option in the Shortcuts app onmacOS
oriOS
. - You can share the shortcut as a source file using the
Share
option in the Shortcuts app onmacOS
, resulting in a shortcut file with the.shortcut
extension. Note: The shared source file is encrypted by Apple and cannot be directly parsed using theplist
package in Python.
- You can share the shortcut as an iCloud link using the
-
Decrypt single or multiple shortcuts. If you want to decrypt a specific shortcut, you can use the following shortcuts to decrypt other shortcuts. The decrypted files will be in
plist
format.- Get Plist - Parse a single shortcut to a plist file
- Get Plist Loop - Parse all shortcuts in the Shortcuts app to plist files and save them
To make it easier to read, you can choose to convert the
plist
files tojson
format. The shortcut source files we provide are all injson
format. -
How to acquire shortcut source files on a large scale?
Instead of using
Get Plist
andGet Plist Loop
to parse shortcuts, we follow these two steps for quicker and more efficient mass acquisition of shortcut source files:- Obtain iCloud links in the format
https://www.icloud.com/shortcuts/${unique_id}
. - Request partial metadata of the shortcut from
https://www.icloud.com/shortcuts/api/records/${unique_id}
, including the shortcut name and download link for the source file. - Use the download link
cur_dict["fields"]["shortcut"]["value"]["downloadURL"]
obtained in the previous step to request the source file of the shortcut. Note: The download link expires quickly, so you need to use it promptly.
The directly downloaded source file is in
plist
format. You can choose to convert theplist
format tojson
format.The following code (simplified) demonstrates the entire process, with the final
response_json
being thejson
format shortcut source file:response = requests.get(f"https://www.icloud.com/shortcuts/api/records/{unique_id}") cur_dict = response.json() downloadURL = cur_dict["fields"]["shortcut"]["value"]["downloadURL"] new_response = requests.get(downloadURL) # Convert using the plist package to json and store in response_json response_json = biplist.readPlistFromString(new_response.content)
- Obtain iCloud links in the format
All code and datasets in this project are licensed under the Apache License 2.0
. This means you are free to use, copy, modify, and distribute the content of this project, but must comply with the following conditions:
- Copyright Notice: The original copyright notice and license statement must be included in all copies of the project.
- State Changes: If you modify the code, you must indicate the changes in any modified files.
- Trademark Use: This license does not grant the right to use project trademarks, service marks, or trade names.
For the full text of the license, please see LICENSE.
Additionally, you must comply with the license agreements of the shortcut sharing sites that provided the data sources for this project.
If you find this project helpful, please consider citing our work:
@misc{
shen2024shortcutsbenchlargescalerealworldbenchmark,
title={ShortcutsBench: A Large-Scale Real-world Benchmark for API-based Agents},
author={Haiyang Shen and Yue Li and Desong Meng and Dongqi Cai and Sheng Qi and Li Zhang and Mengwei Xu and Yun Ma},
year={2024},
eprint={2407.00132},
archivePrefix={arXiv},
primaryClass={cs.SE},
url={https://arxiv.org/abs/2407.00132},
}
For Tasks:
Click tags to check more tools for each tasksFor Jobs:
Alternative AI tools for ShortcutsBench
Similar Open Source Tools
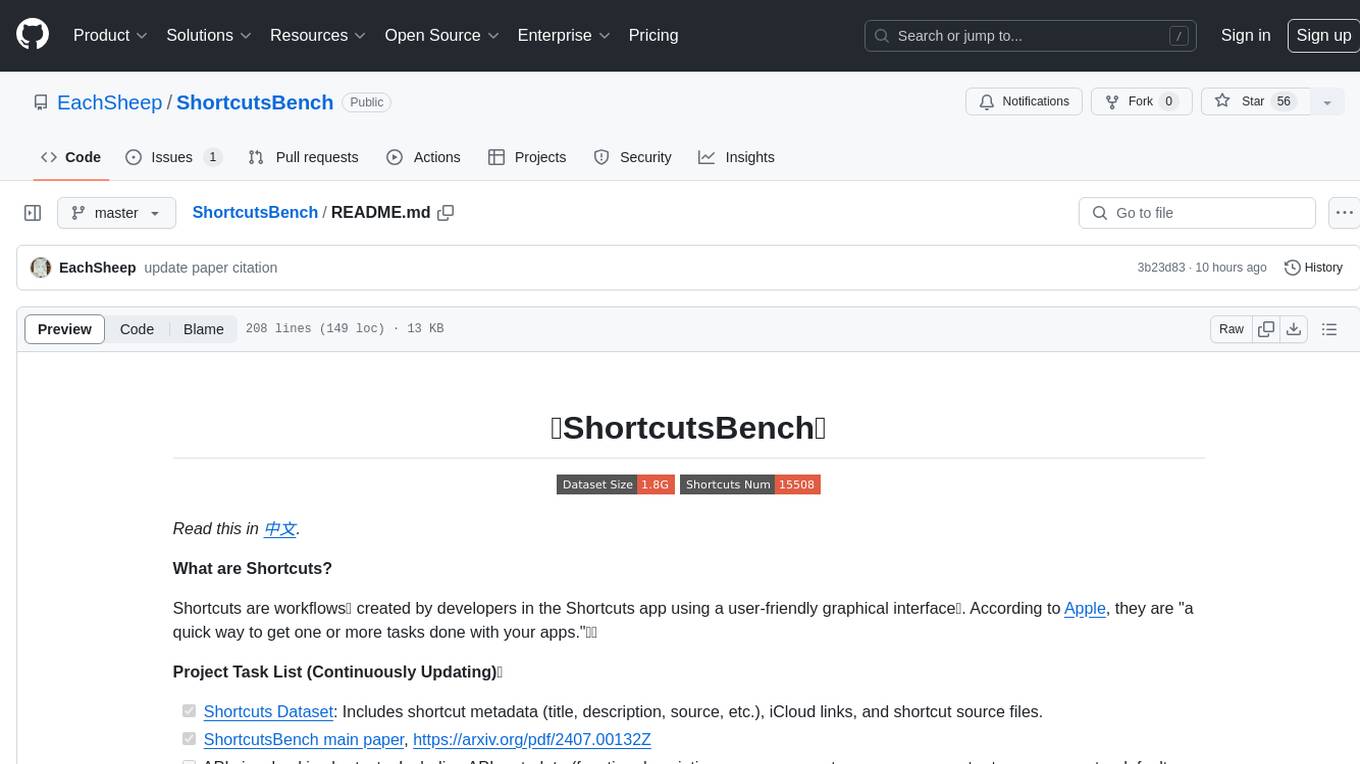
ShortcutsBench
ShortcutsBench is a project focused on collecting and analyzing workflows created in the Shortcuts app, providing a dataset of shortcut metadata, source files, and API information. It aims to study the integration of large language models with Apple devices, particularly focusing on the role of shortcuts in enhancing user experience. The project offers insights for Shortcuts users, enthusiasts, and researchers to explore, customize workflows, and study automated workflows, low-code programming, and API-based agents.
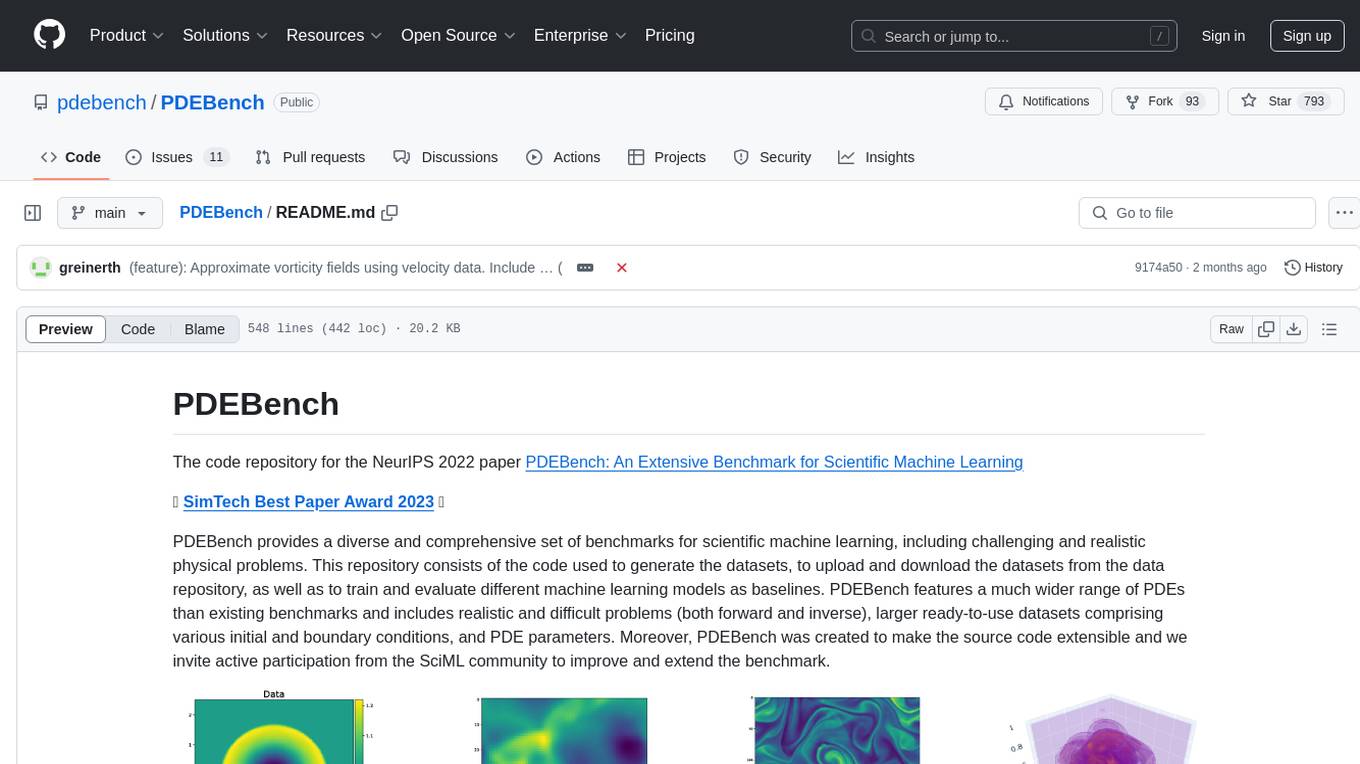
PDEBench
PDEBench provides a diverse and comprehensive set of benchmarks for scientific machine learning, including challenging and realistic physical problems. The repository consists of code for generating datasets, uploading and downloading datasets, training and evaluating machine learning models as baselines. It features a wide range of PDEs, realistic and difficult problems, ready-to-use datasets with various conditions and parameters. PDEBench aims for extensibility and invites participation from the SciML community to improve and extend the benchmark.
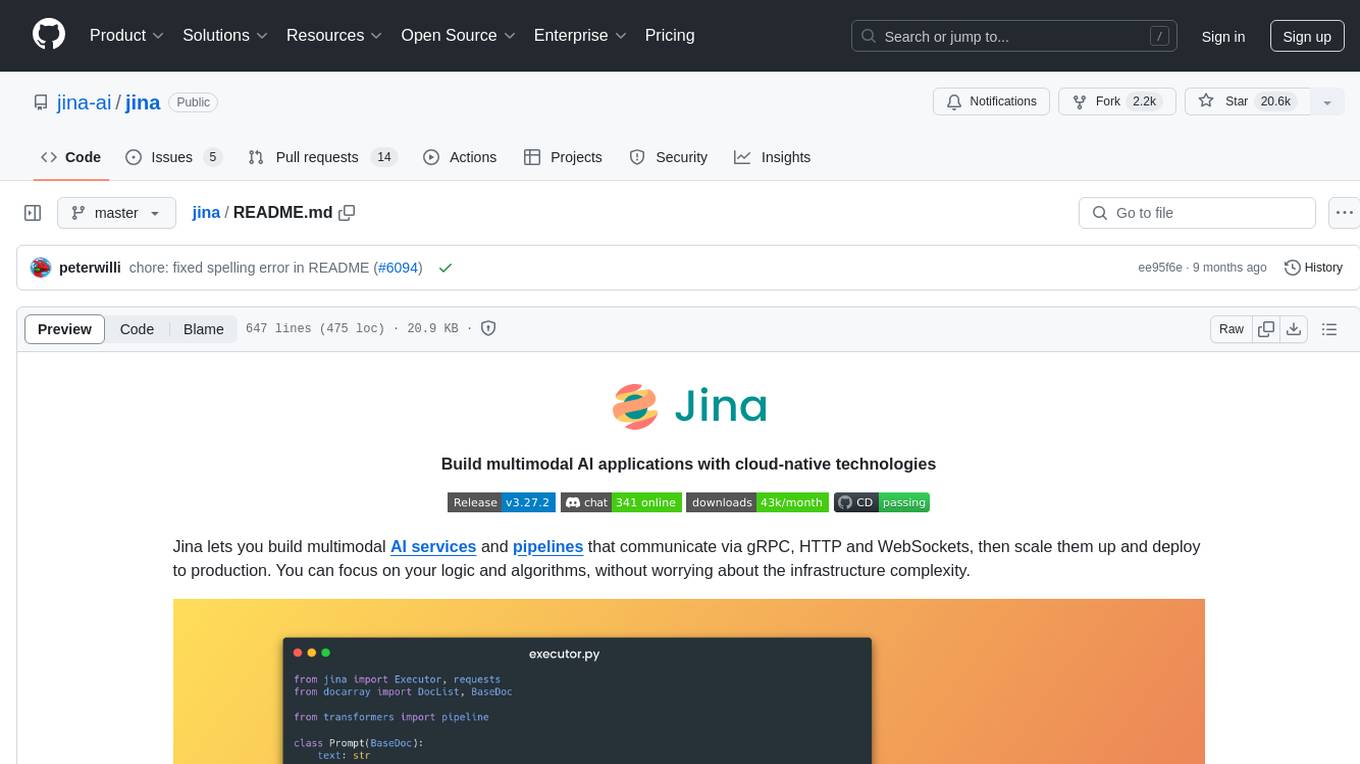
jina
Jina is a tool that allows users to build multimodal AI services and pipelines using cloud-native technologies. It provides a Pythonic experience for serving ML models and transitioning from local deployment to advanced orchestration frameworks like Docker-Compose, Kubernetes, or Jina AI Cloud. Users can build and serve models for any data type and deep learning framework, design high-performance services with easy scaling, serve LLM models while streaming their output, integrate with Docker containers via Executor Hub, and host on CPU/GPU using Jina AI Cloud. Jina also offers advanced orchestration and scaling capabilities, a smooth transition to the cloud, and easy scalability and concurrency features for applications. Users can deploy to their own cloud or system with Kubernetes and Docker Compose integration, and even deploy to JCloud for autoscaling and monitoring.
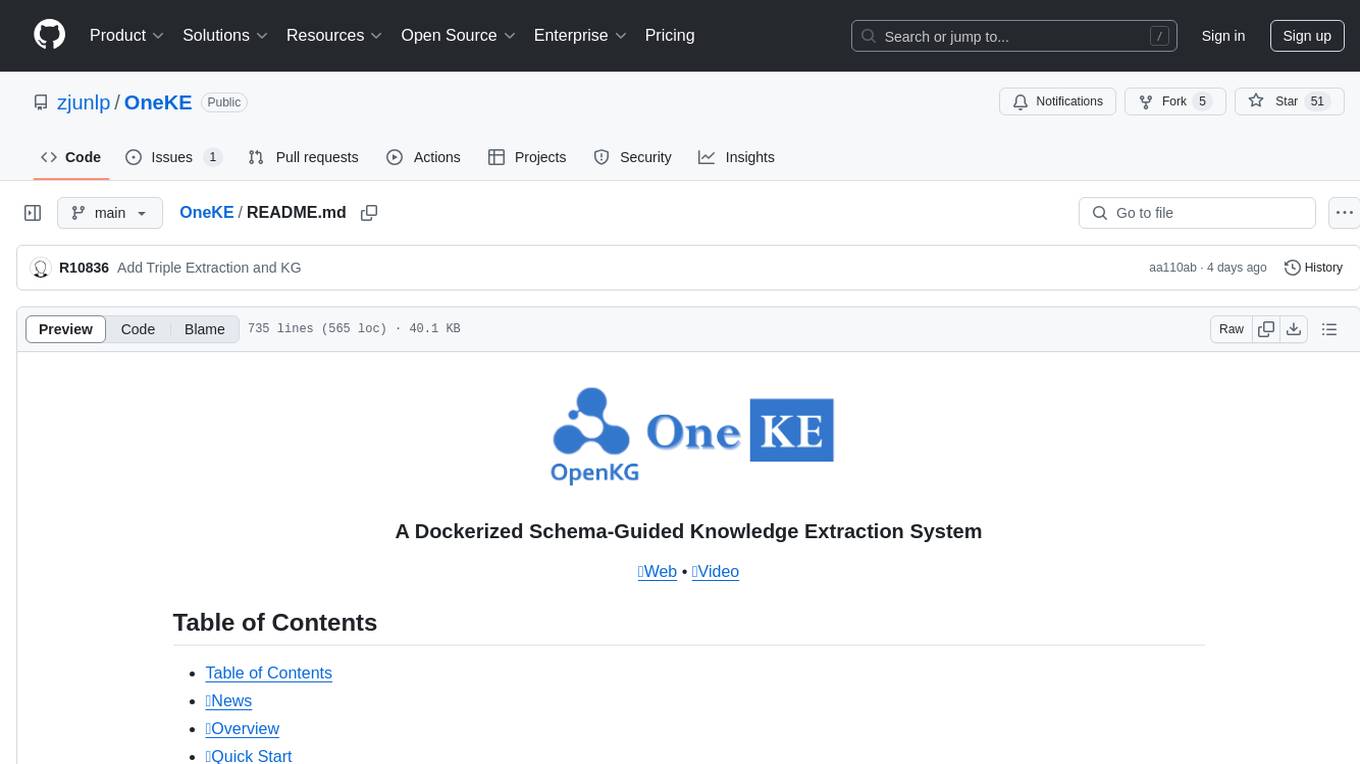
OneKE
OneKE is a flexible dockerized system for schema-guided knowledge extraction, capable of extracting information from the web and raw PDF books across multiple domains like science and news. It employs a collaborative multi-agent approach and includes a user-customizable knowledge base to enable tailored extraction. OneKE offers various IE tasks support, data sources support, LLMs support, extraction method support, and knowledge base configuration. Users can start with examples using YAML, Python, or Web UI, and perform tasks like Named Entity Recognition, Relation Extraction, Event Extraction, Triple Extraction, and Open Domain IE. The tool supports different source formats like Plain Text, HTML, PDF, Word, TXT, and JSON files. Users can choose from various extraction models like OpenAI, DeepSeek, LLaMA, Qwen, ChatGLM, MiniCPM, and OneKE for information extraction tasks. Extraction methods include Schema Agent, Extraction Agent, and Reflection Agent. The tool also provides support for schema repository and case repository management, along with solutions for network issues. Contributors to the project include Ningyu Zhang, Haofen Wang, Yujie Luo, Xiangyuan Ru, Kangwei Liu, Lin Yuan, Mengshu Sun, Lei Liang, Zhiqiang Zhang, Jun Zhou, Lanning Wei, Da Zheng, and Huajun Chen.
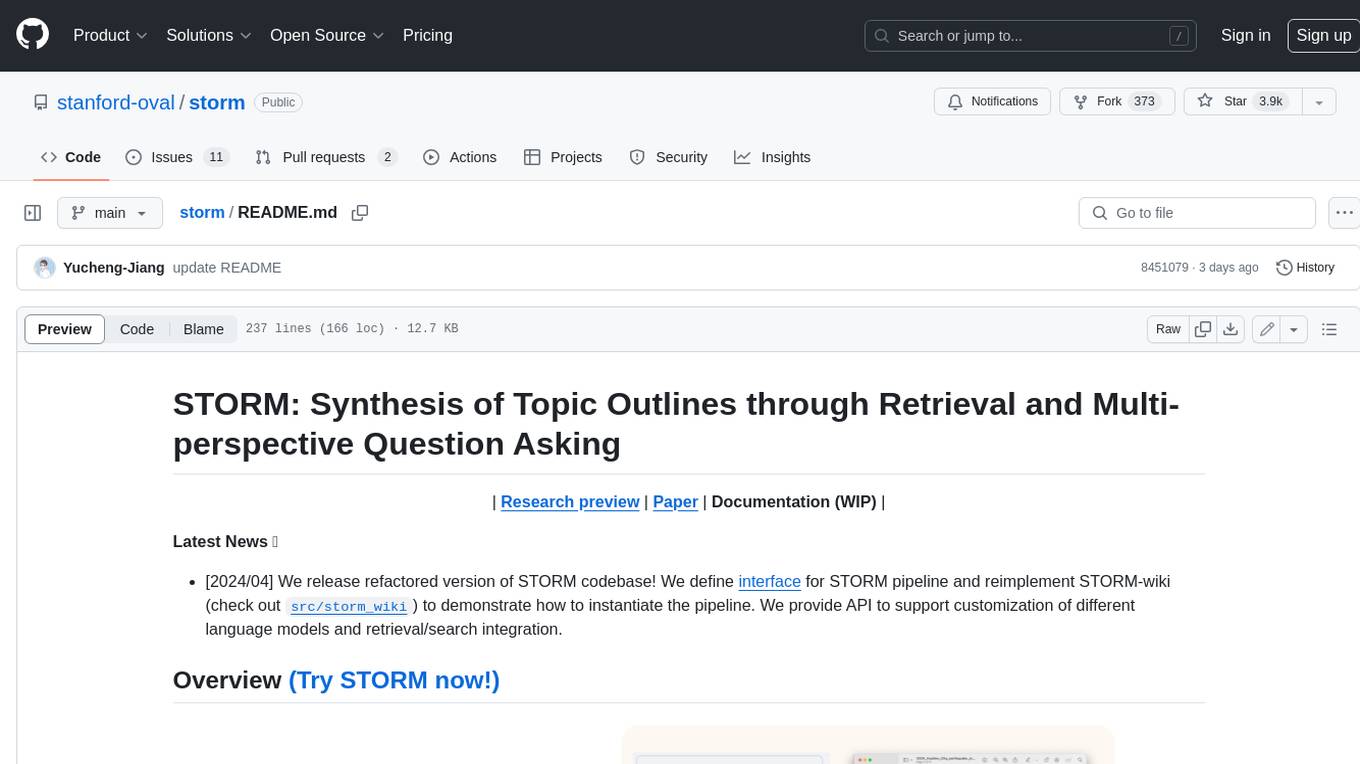
storm
STORM is a LLM system that writes Wikipedia-like articles from scratch based on Internet search. While the system cannot produce publication-ready articles that often require a significant number of edits, experienced Wikipedia editors have found it helpful in their pre-writing stage. **Try out our [live research preview](https://storm.genie.stanford.edu/) to see how STORM can help your knowledge exploration journey and please provide feedback to help us improve the system ๐!**
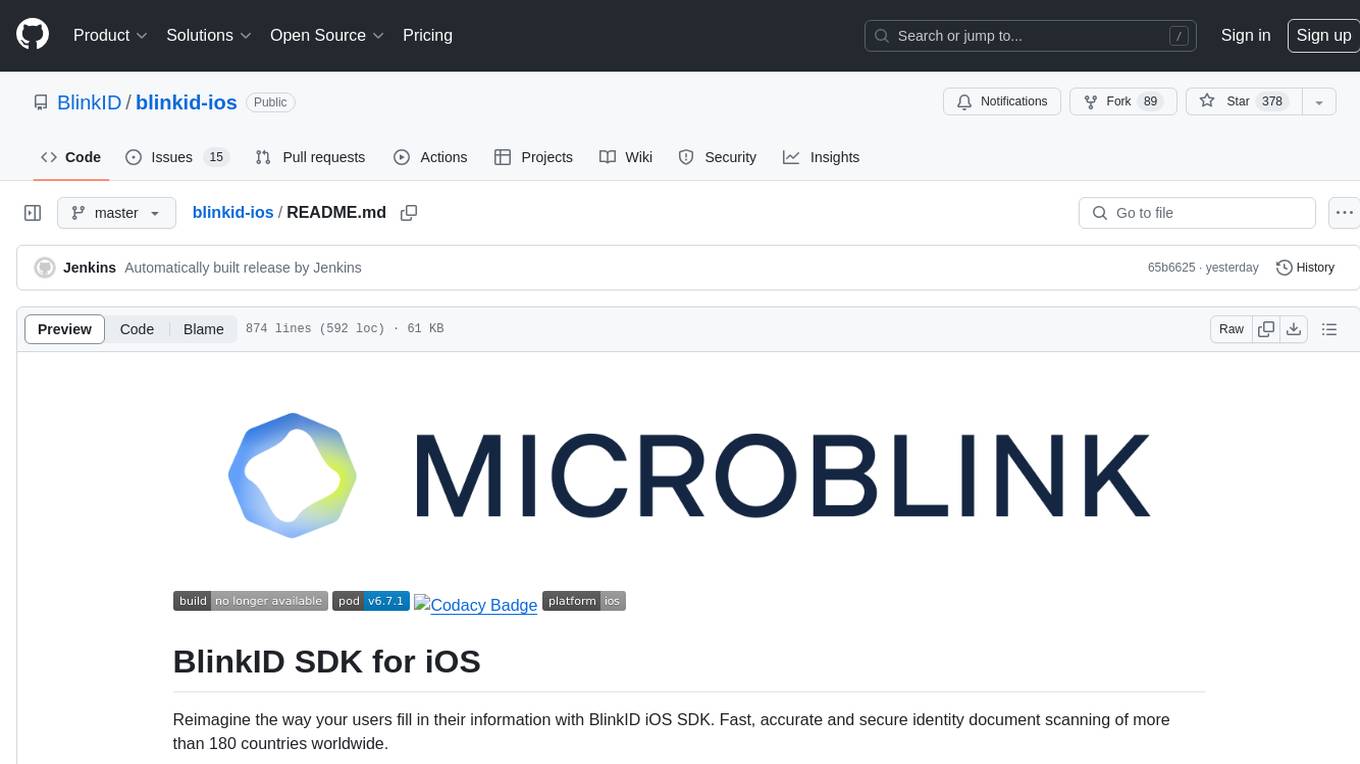
blinkid-ios
BlinkID iOS is a mobile SDK that enables developers to easily integrate ID scanning and data extraction capabilities into their iOS applications. The SDK supports scanning and processing various types of identity documents, such as passports, driver's licenses, and ID cards. It provides accurate and fast data extraction, including personal information and document details. With BlinkID iOS, developers can enhance their apps with secure and reliable ID verification functionality, improving user experience and streamlining identity verification processes.
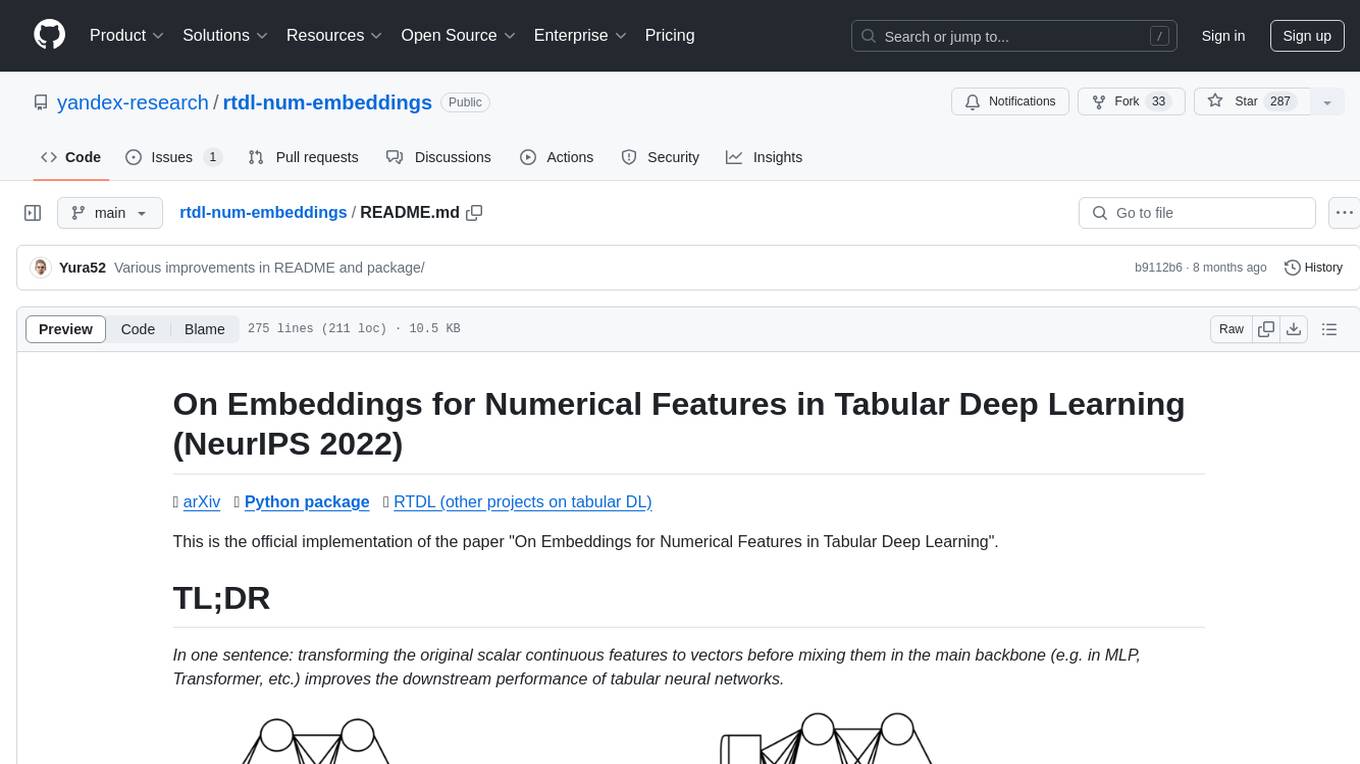
rtdl-num-embeddings
This repository provides the official implementation of the paper 'On Embeddings for Numerical Features in Tabular Deep Learning'. It focuses on transforming scalar continuous features into vectors before integrating them into the main backbone of tabular neural networks, showcasing improved performance. The embeddings for continuous features are shown to enhance the performance of tabular DL models and are applicable to various conventional backbones, offering efficiency comparable to Transformer-based models. The repository includes Python packages for practical usage, exploration of metrics and hyperparameters, and reproducing reported results for different algorithms and datasets.
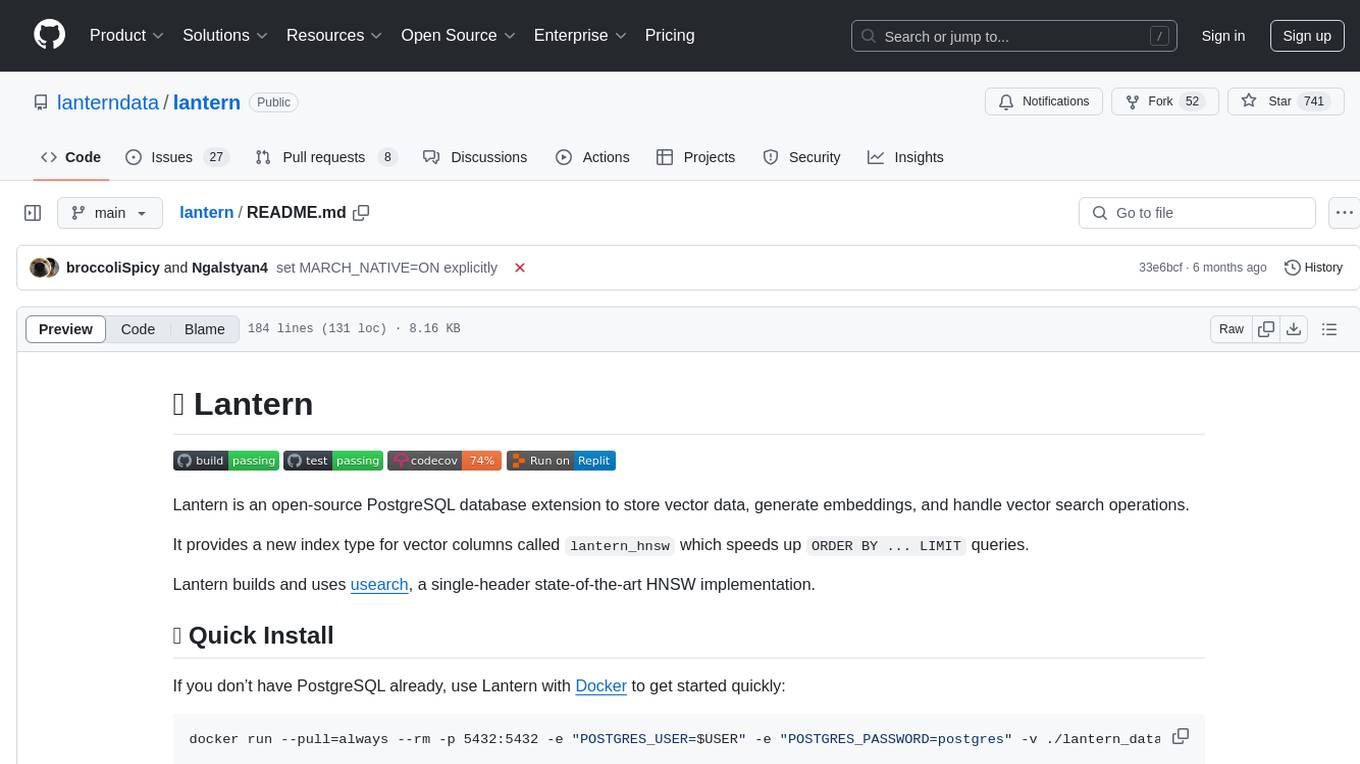
lantern
Lantern is an open-source PostgreSQL database extension designed to store vector data, generate embeddings, and handle vector search operations efficiently. It introduces a new index type called 'lantern_hnsw' for vector columns, which speeds up 'ORDER BY ... LIMIT' queries. Lantern utilizes the state-of-the-art HNSW implementation called usearch. Users can easily install Lantern using Docker, Homebrew, or precompiled binaries. The tool supports various distance functions, index construction parameters, and operator classes for efficient querying. Lantern offers features like embedding generation, interoperability with pgvector, parallel index creation, and external index graph generation. It aims to provide superior performance metrics compared to other similar tools and has a roadmap for future enhancements such as cloud-hosted version, hardware-accelerated distance metrics, industry-specific application templates, and support for version control and A/B testing of embeddings.
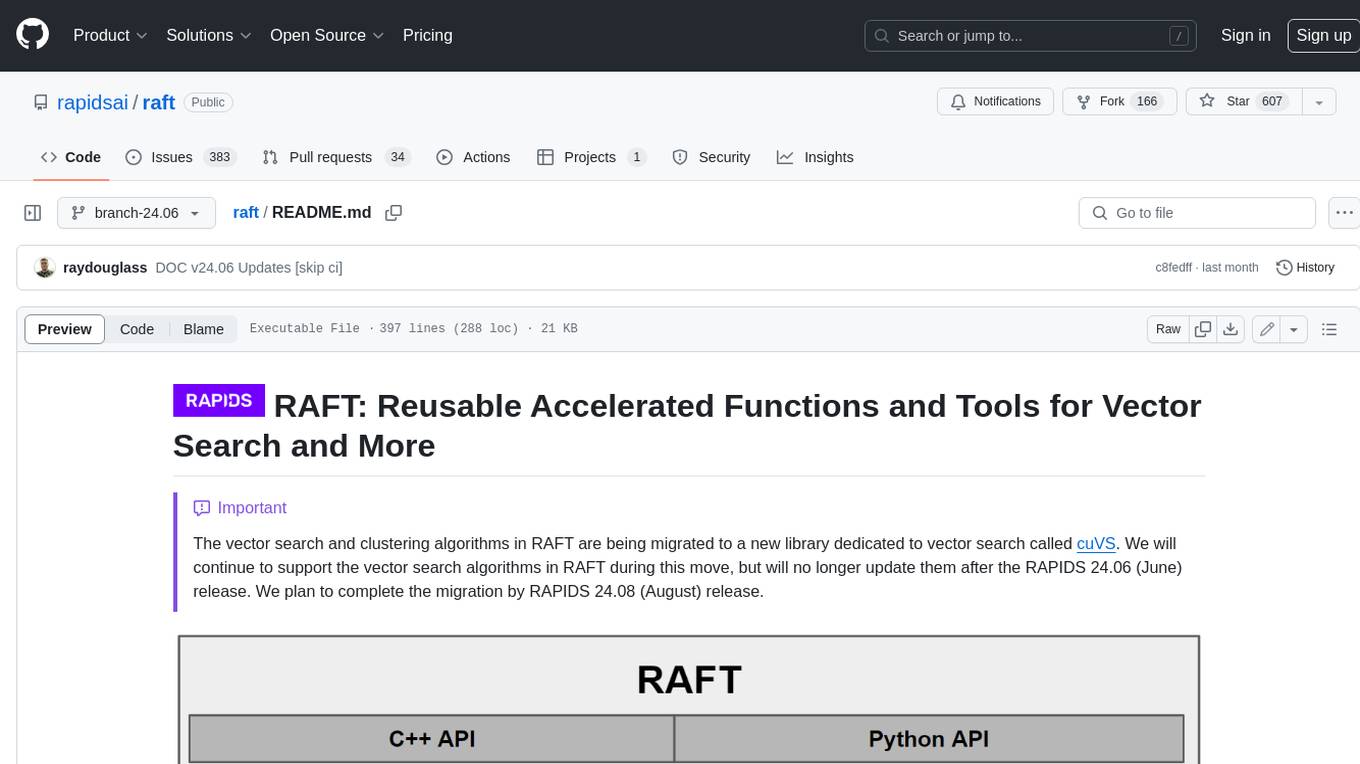
raft
RAFT (Reusable Accelerated Functions and Tools) is a C++ header-only template library with an optional shared library that contains fundamental widely-used algorithms and primitives for machine learning and information retrieval. The algorithms are CUDA-accelerated and form building blocks for more easily writing high performance applications.
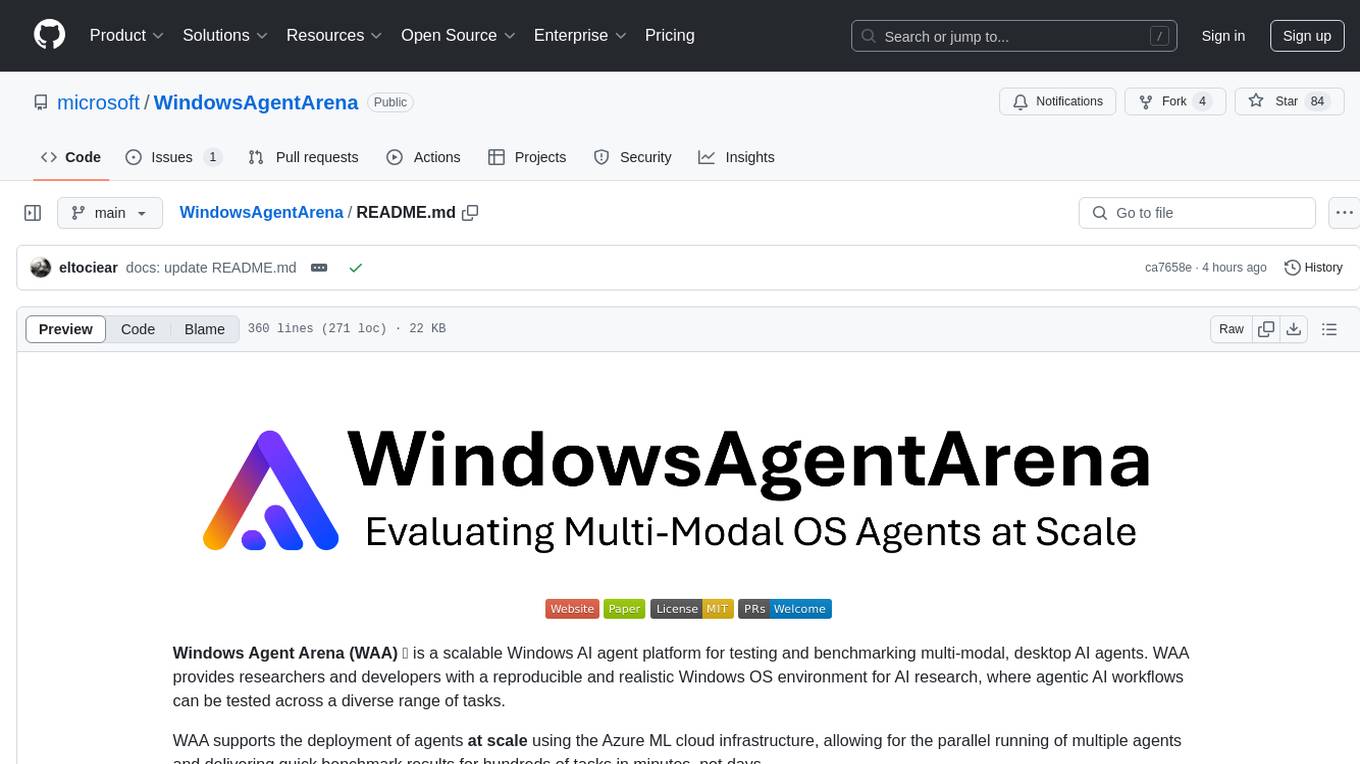
WindowsAgentArena
Windows Agent Arena (WAA) is a scalable Windows AI agent platform designed for testing and benchmarking multi-modal, desktop AI agents. It provides researchers and developers with a reproducible and realistic Windows OS environment for AI research, enabling testing of agentic AI workflows across various tasks. WAA supports deploying agents at scale using Azure ML cloud infrastructure, allowing parallel running of multiple agents and delivering quick benchmark results for hundreds of tasks in minutes.
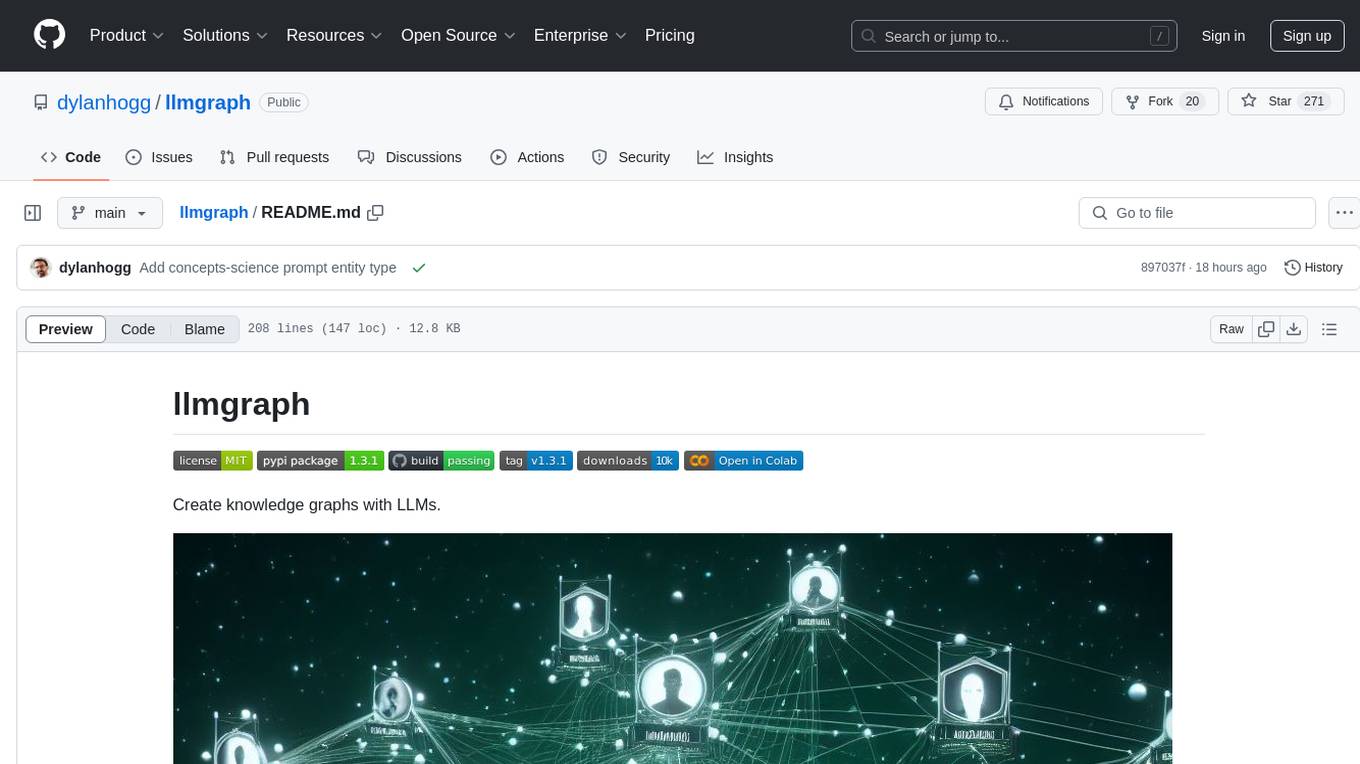
llmgraph
llmgraph is a tool that enables users to create knowledge graphs in GraphML, GEXF, and HTML formats by extracting world knowledge from large language models (LLMs) like ChatGPT. It supports various entity types and relationships, offers cache support for efficient graph growth, and provides insights into LLM costs. Users can customize the model used and interact with different LLM providers. The tool allows users to generate interactive graphs based on a specified entity type and Wikipedia link, making it a valuable resource for knowledge graph creation and exploration.
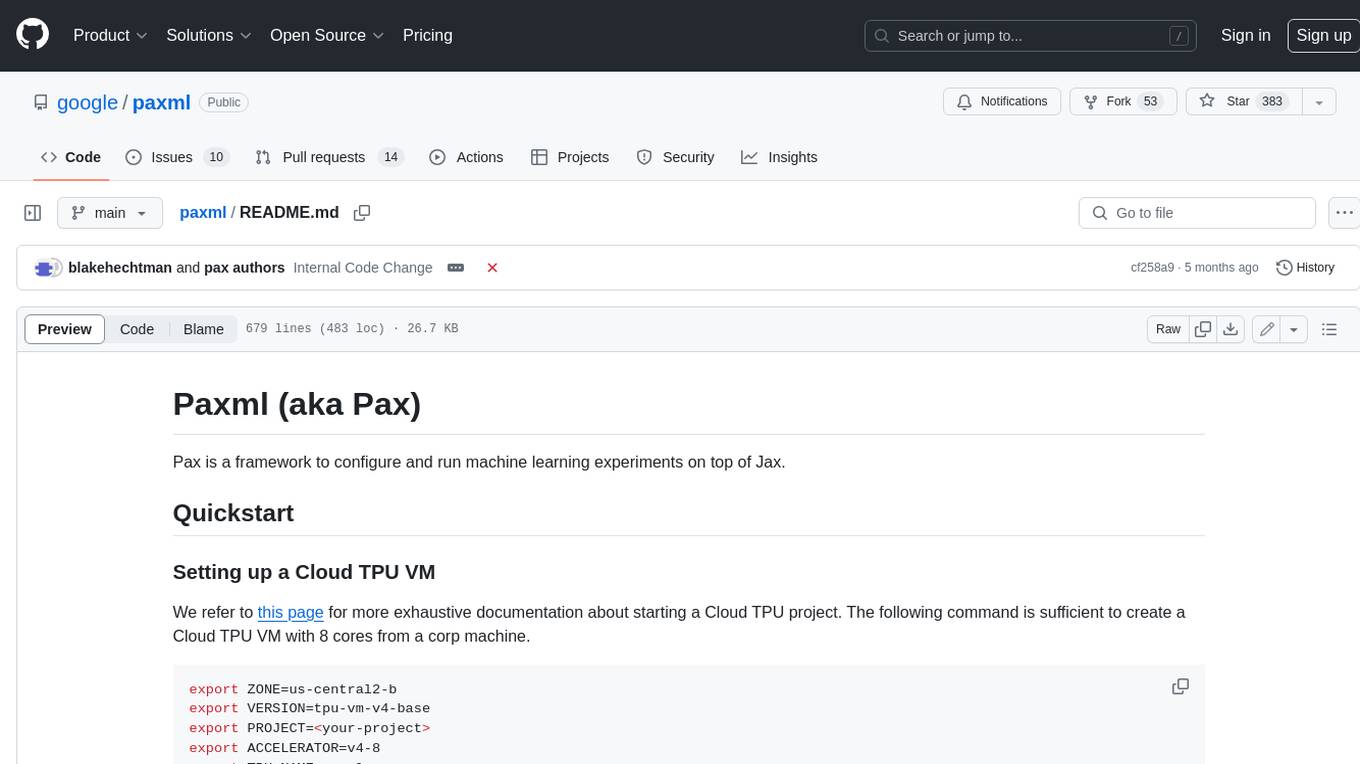
paxml
Pax is a framework to configure and run machine learning experiments on top of Jax.
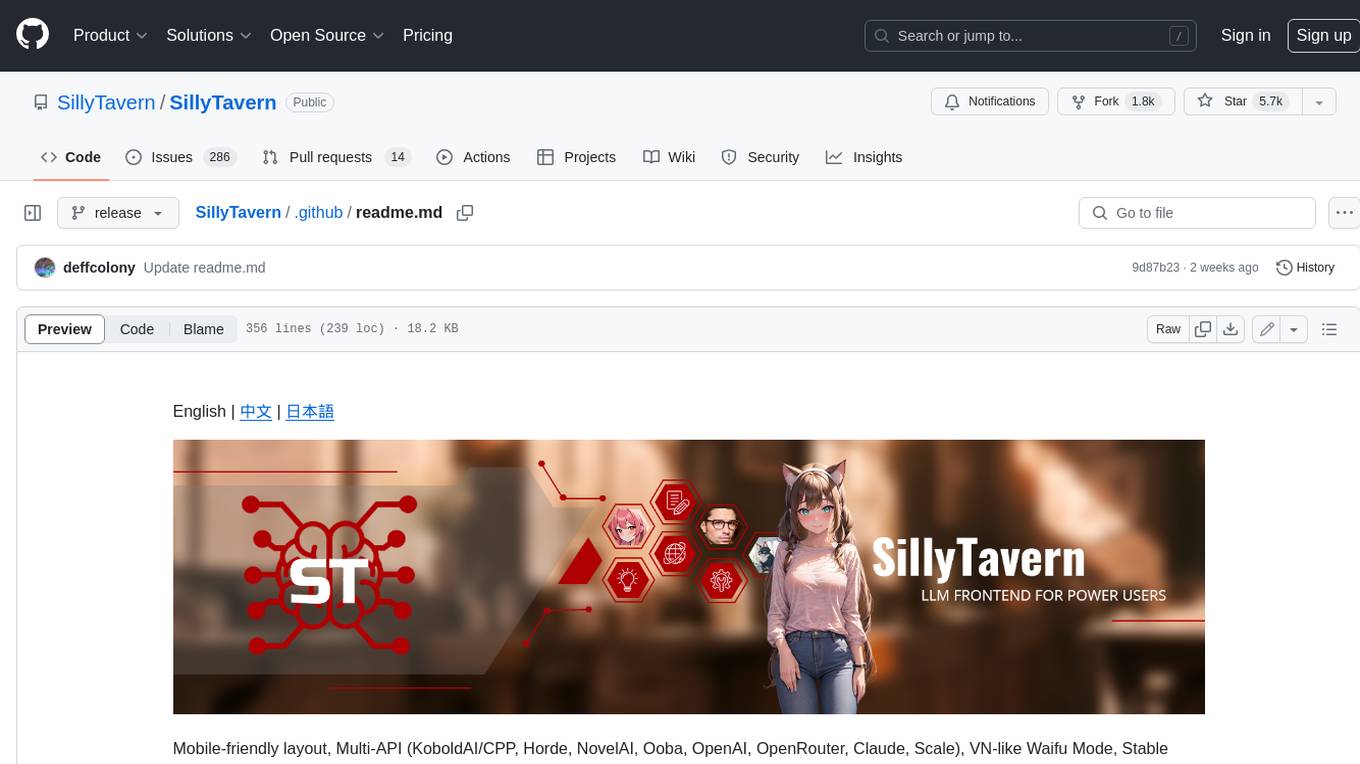
SillyTavern
SillyTavern is a user interface you can install on your computer (and Android phones) that allows you to interact with text generation AIs and chat/roleplay with characters you or the community create. SillyTavern is a fork of TavernAI 1.2.8 which is under more active development and has added many major features. At this point, they can be thought of as completely independent programs.
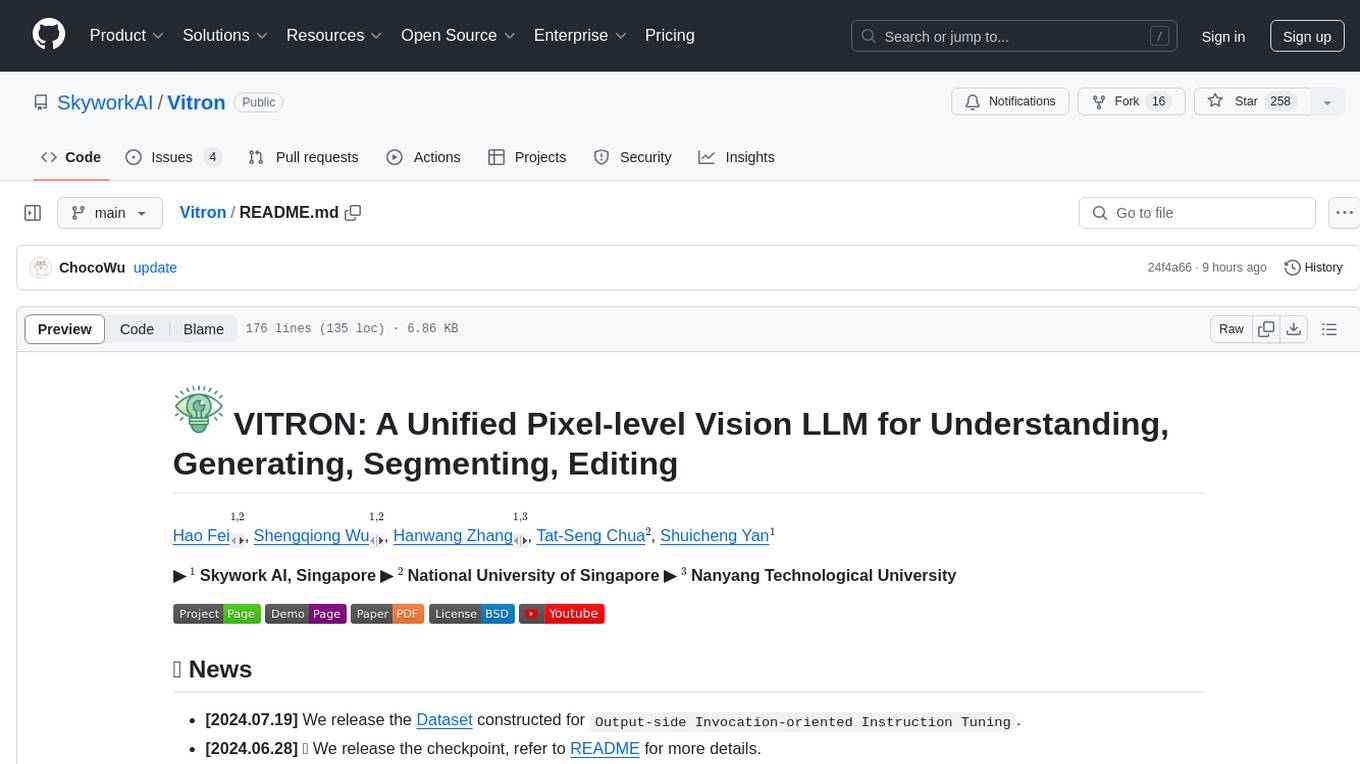
Vitron
Vitron is a unified pixel-level vision LLM designed for comprehensive understanding, generating, segmenting, and editing static images and dynamic videos. It addresses challenges in existing vision LLMs such as superficial instance-level understanding, lack of unified support for images and videos, and insufficient coverage across various vision tasks. The tool requires Python >= 3.8, Pytorch == 2.1.0, and CUDA Version >= 11.8 for installation. Users can deploy Gradio demo locally and fine-tune their models for specific tasks.
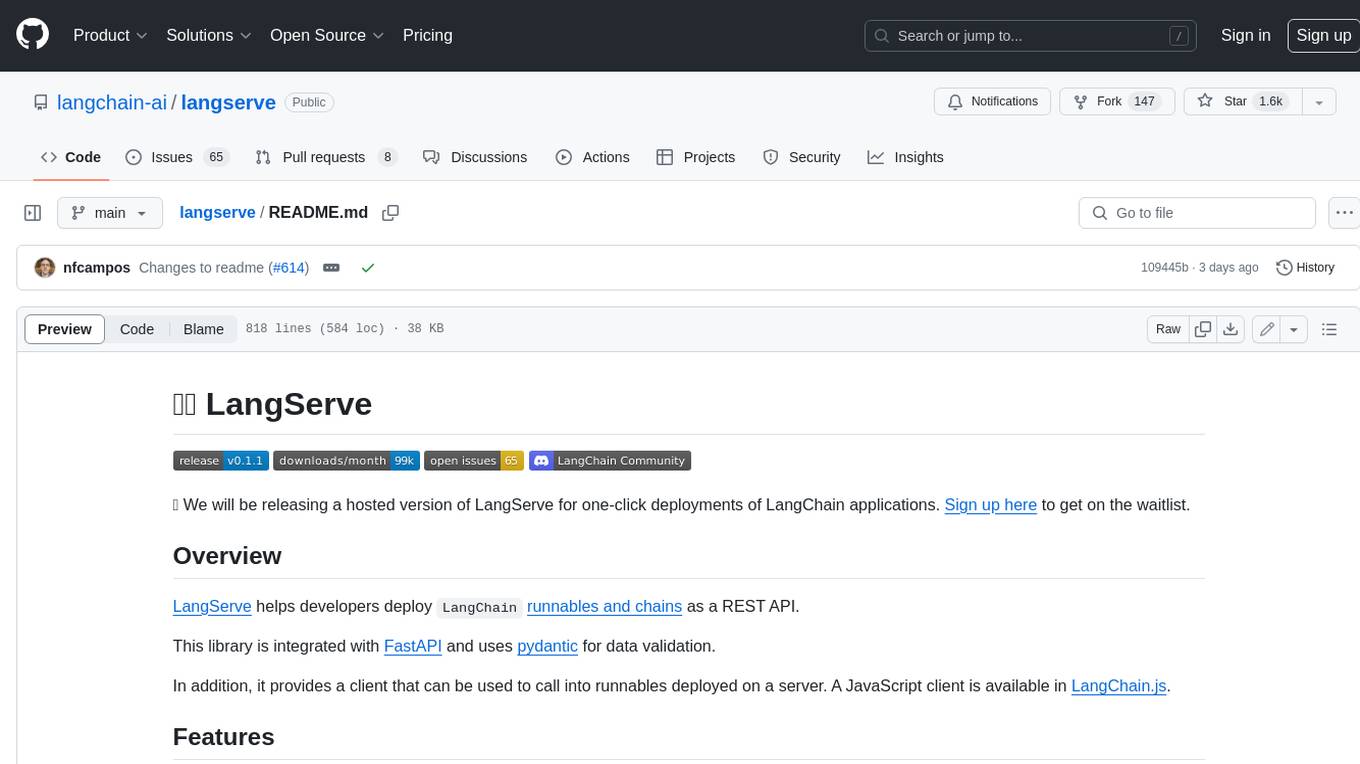
langserve
LangServe helps developers deploy `LangChain` runnables and chains as a REST API. This library is integrated with FastAPI and uses pydantic for data validation. In addition, it provides a client that can be used to call into runnables deployed on a server. A JavaScript client is available in LangChain.js.
For similar tasks
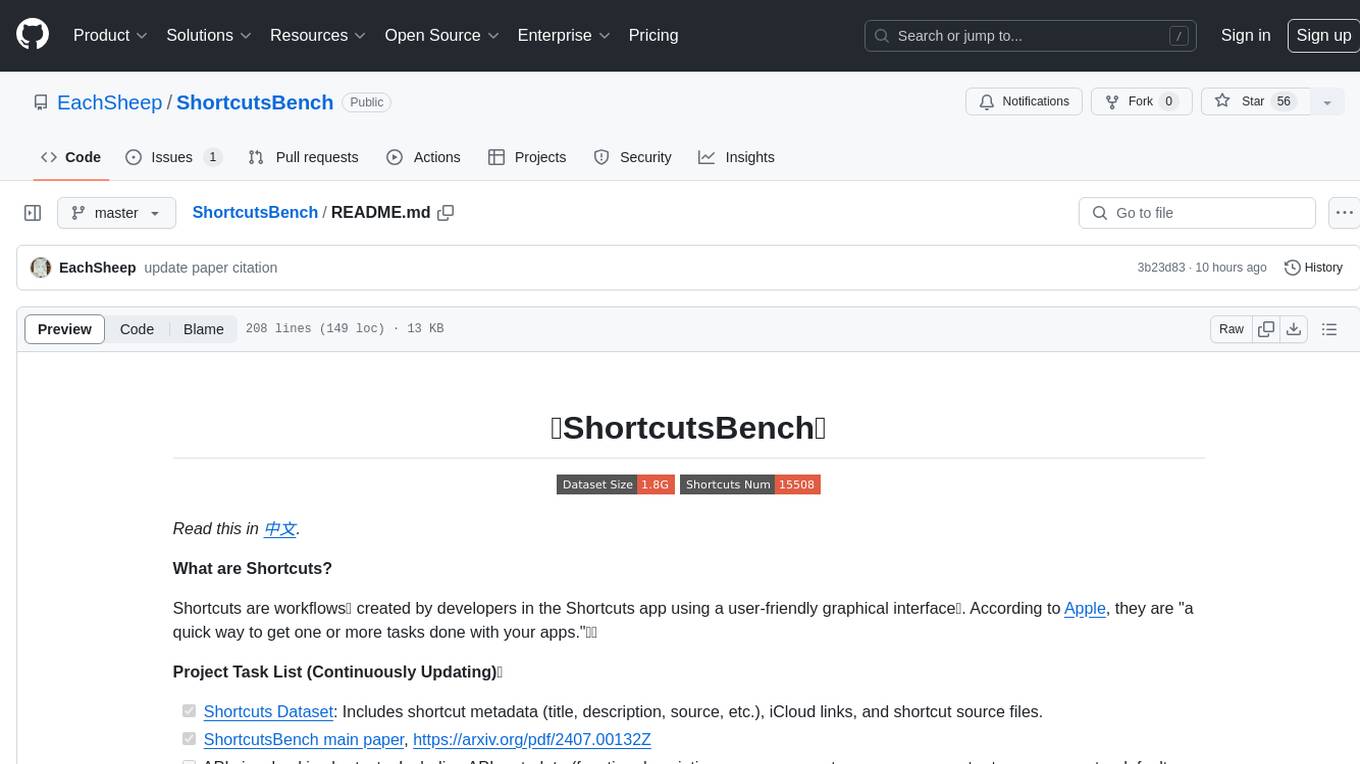
ShortcutsBench
ShortcutsBench is a project focused on collecting and analyzing workflows created in the Shortcuts app, providing a dataset of shortcut metadata, source files, and API information. It aims to study the integration of large language models with Apple devices, particularly focusing on the role of shortcuts in enhancing user experience. The project offers insights for Shortcuts users, enthusiasts, and researchers to explore, customize workflows, and study automated workflows, low-code programming, and API-based agents.
For similar jobs
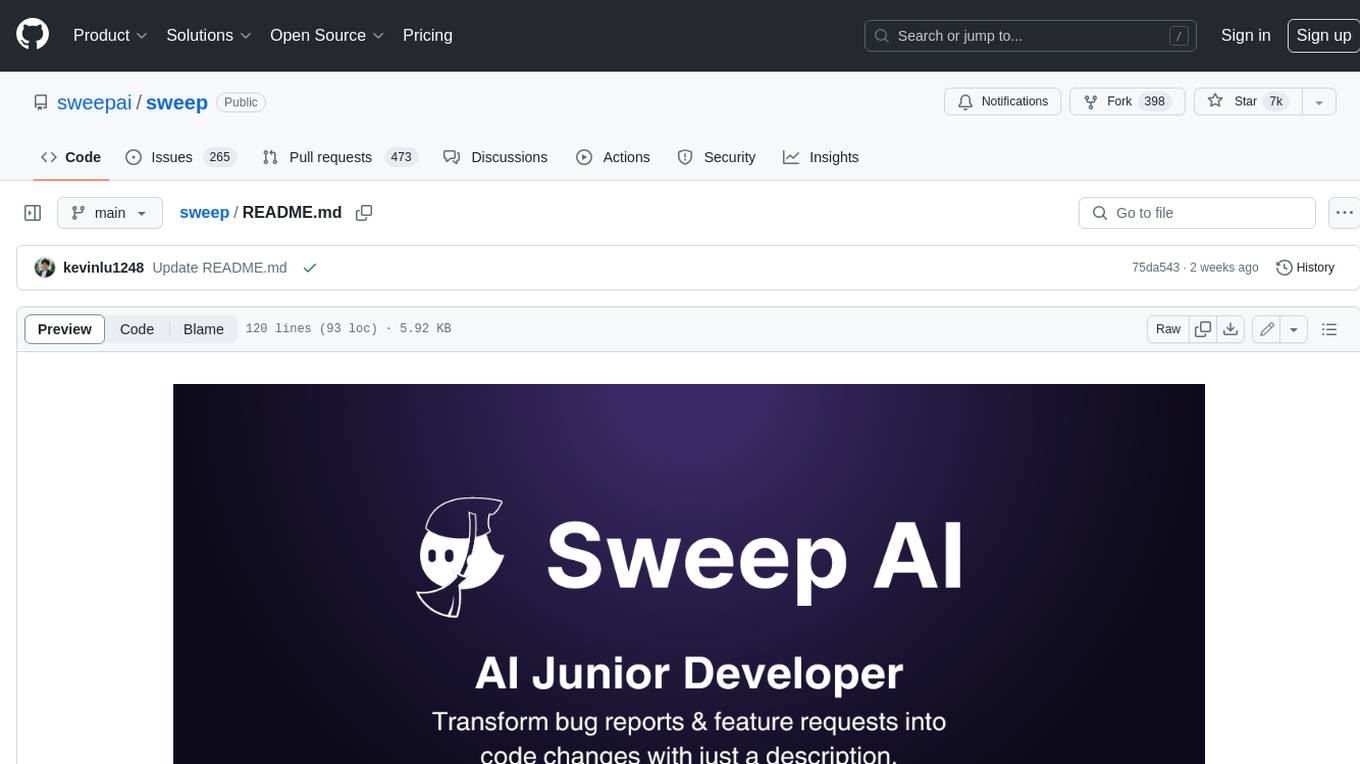
sweep
Sweep is an AI junior developer that turns bugs and feature requests into code changes. It automatically handles developer experience improvements like adding type hints and improving test coverage.
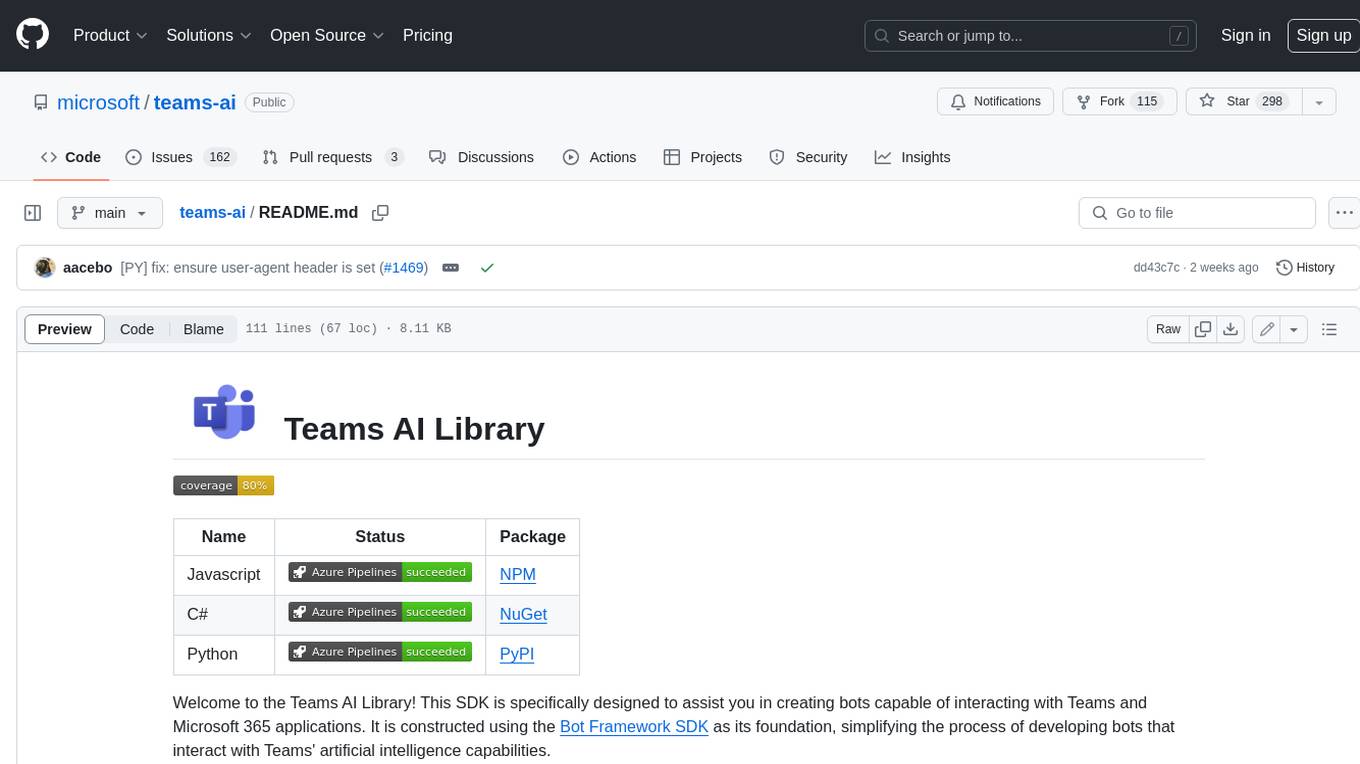
teams-ai
The Teams AI Library is a software development kit (SDK) that helps developers create bots that can interact with Teams and Microsoft 365 applications. It is built on top of the Bot Framework SDK and simplifies the process of developing bots that interact with Teams' artificial intelligence capabilities. The SDK is available for JavaScript/TypeScript, .NET, and Python.
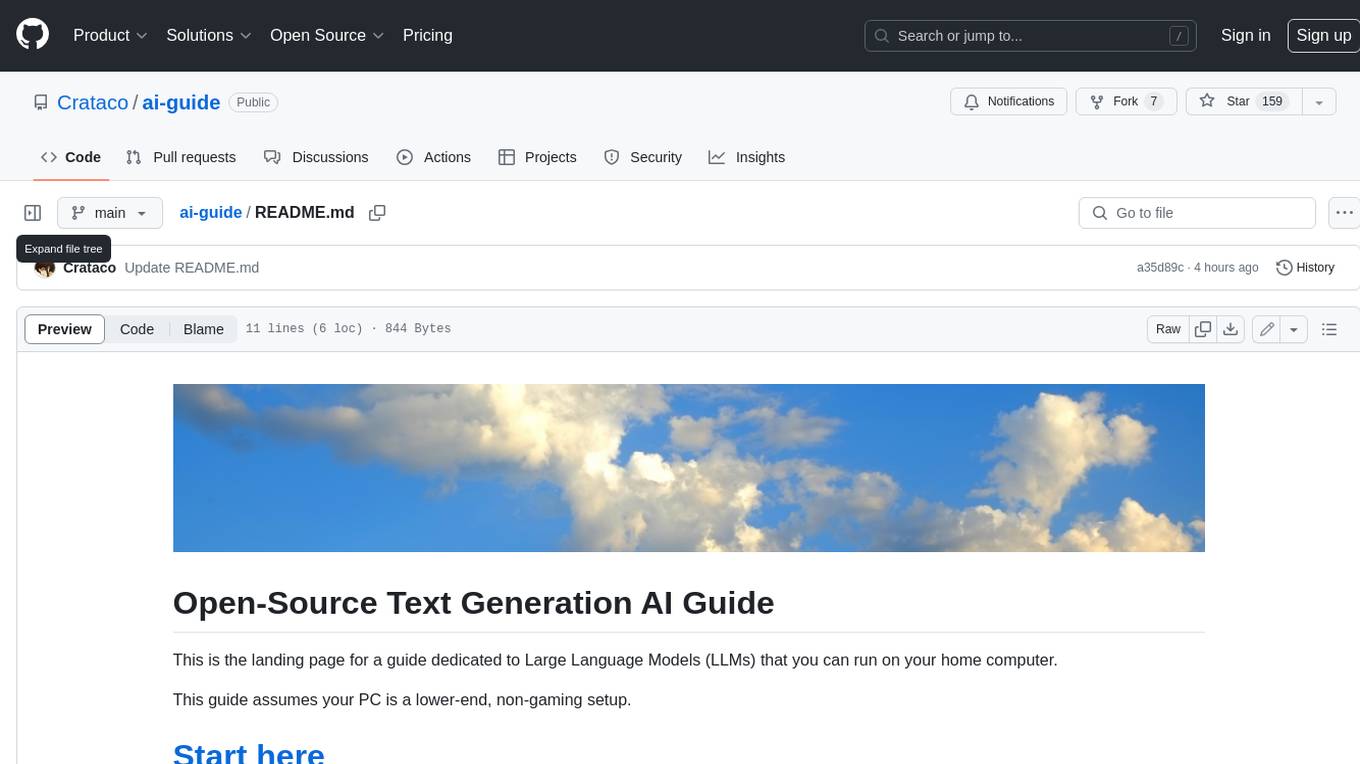
ai-guide
This guide is dedicated to Large Language Models (LLMs) that you can run on your home computer. It assumes your PC is a lower-end, non-gaming setup.
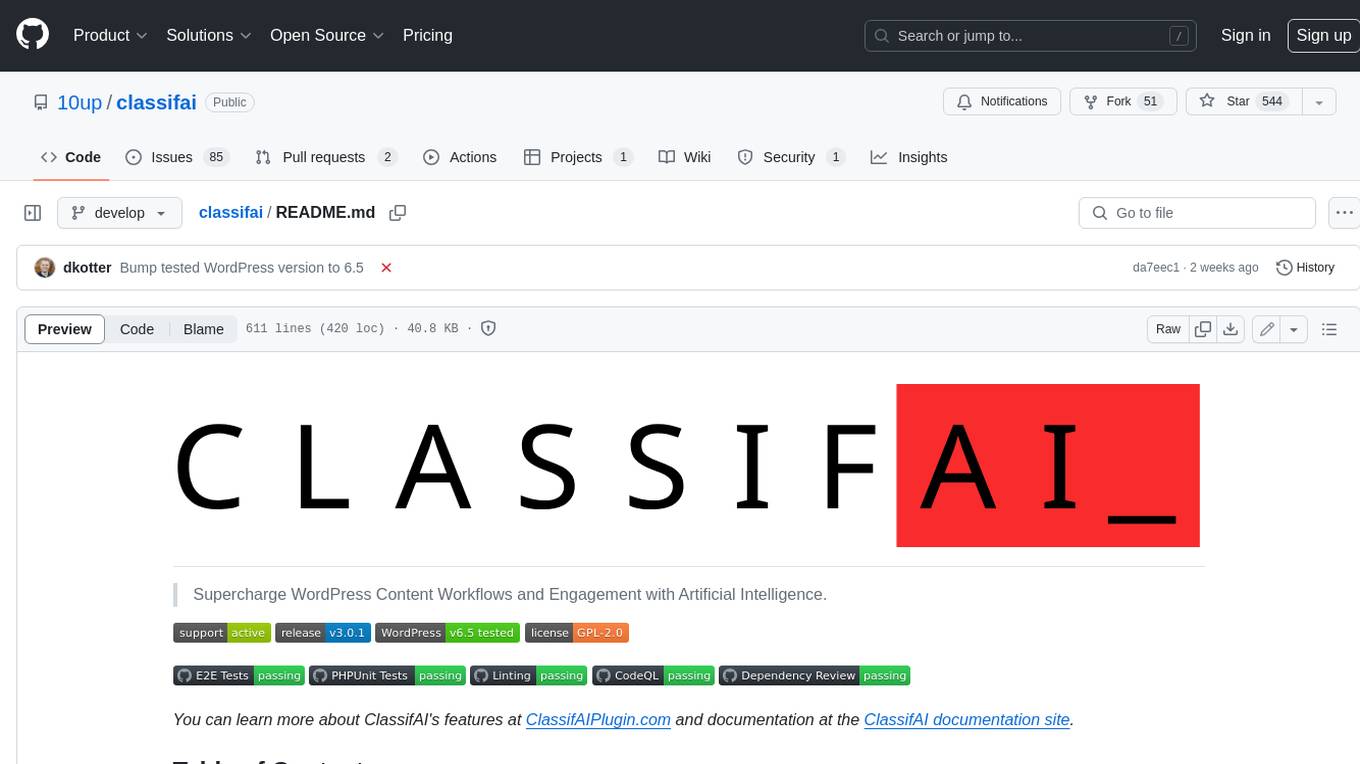
classifai
Supercharge WordPress Content Workflows and Engagement with Artificial Intelligence. Tap into leading cloud-based services like OpenAI, Microsoft Azure AI, Google Gemini and IBM Watson to augment your WordPress-powered websites. Publish content faster while improving SEO performance and increasing audience engagement. ClassifAI integrates Artificial Intelligence and Machine Learning technologies to lighten your workload and eliminate tedious tasks, giving you more time to create original content that matters.
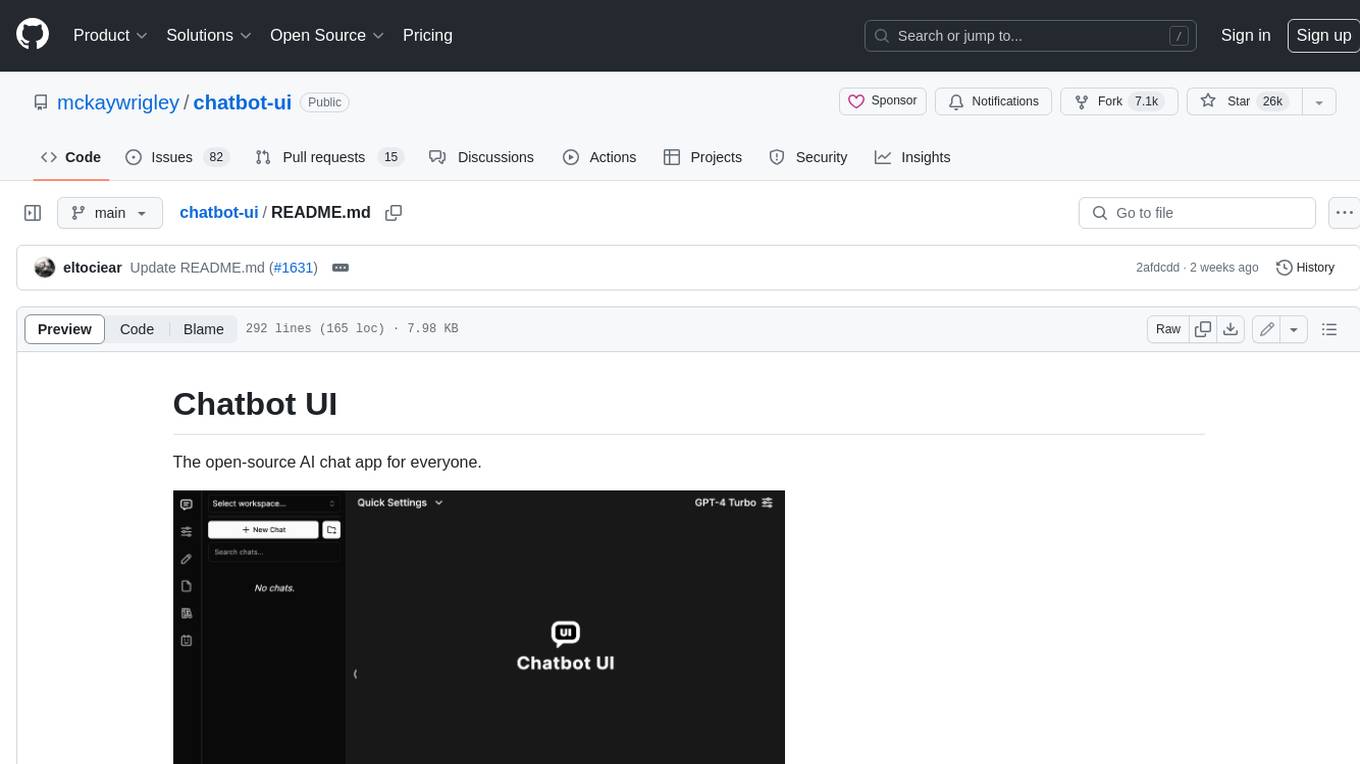
chatbot-ui
Chatbot UI is an open-source AI chat app that allows users to create and deploy their own AI chatbots. It is easy to use and can be customized to fit any need. Chatbot UI is perfect for businesses, developers, and anyone who wants to create a chatbot.
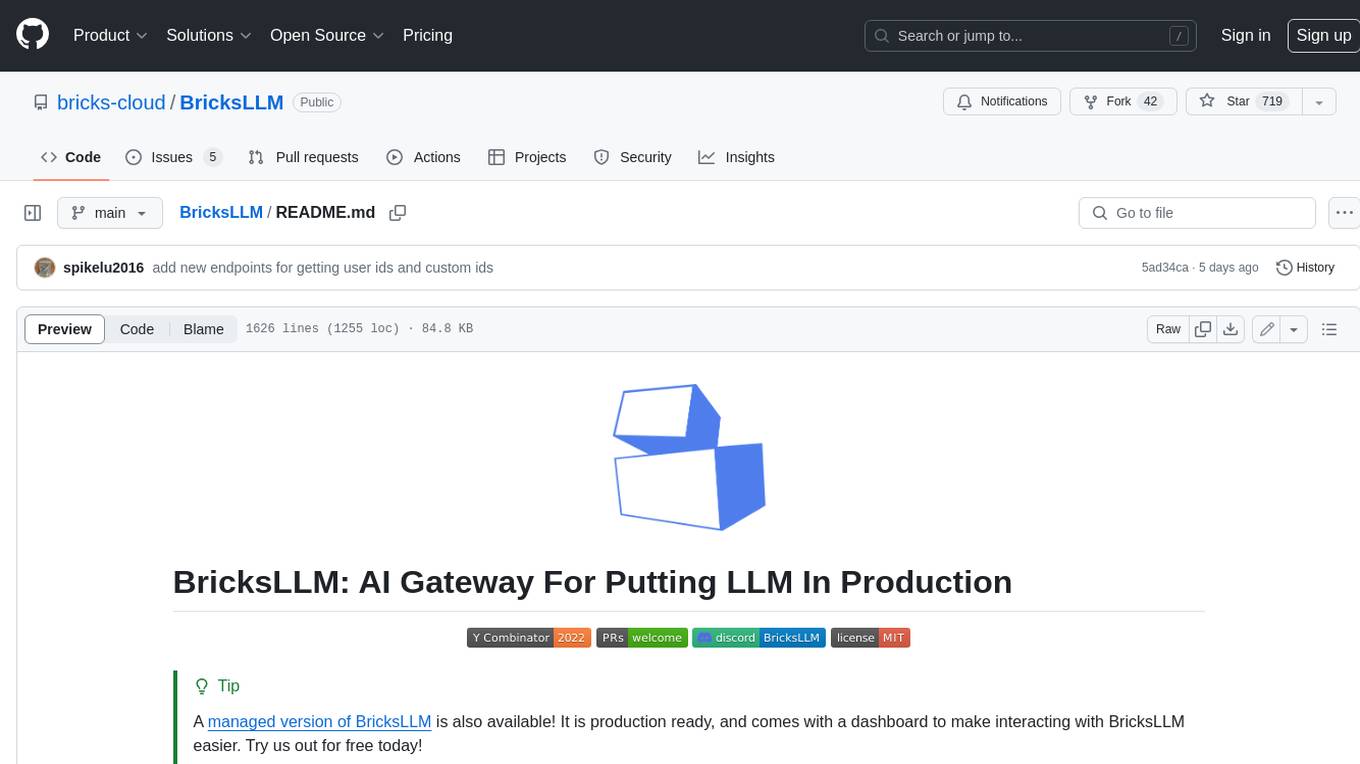
BricksLLM
BricksLLM is a cloud native AI gateway written in Go. Currently, it provides native support for OpenAI, Anthropic, Azure OpenAI and vLLM. BricksLLM aims to provide enterprise level infrastructure that can power any LLM production use cases. Here are some use cases for BricksLLM: * Set LLM usage limits for users on different pricing tiers * Track LLM usage on a per user and per organization basis * Block or redact requests containing PIIs * Improve LLM reliability with failovers, retries and caching * Distribute API keys with rate limits and cost limits for internal development/production use cases * Distribute API keys with rate limits and cost limits for students
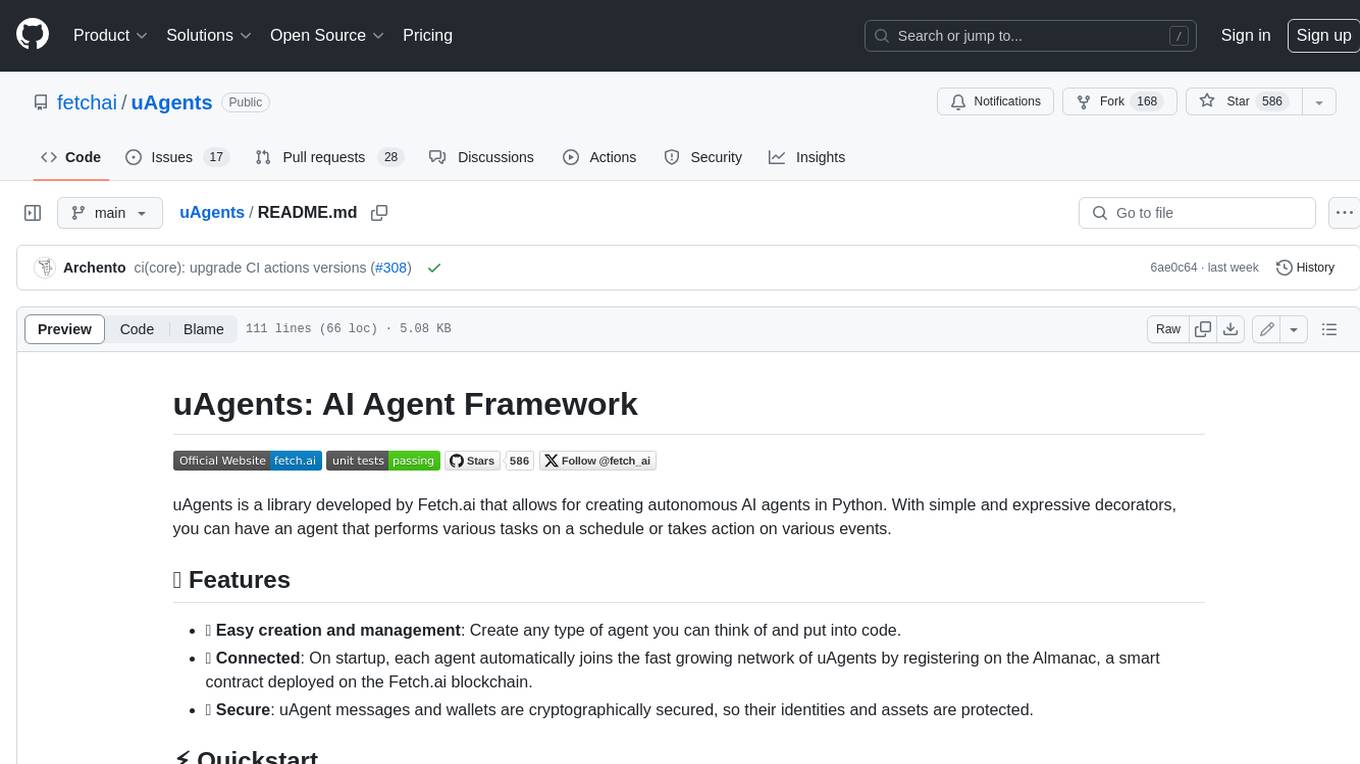
uAgents
uAgents is a Python library developed by Fetch.ai that allows for the creation of autonomous AI agents. These agents can perform various tasks on a schedule or take action on various events. uAgents are easy to create and manage, and they are connected to a fast-growing network of other uAgents. They are also secure, with cryptographically secured messages and wallets.
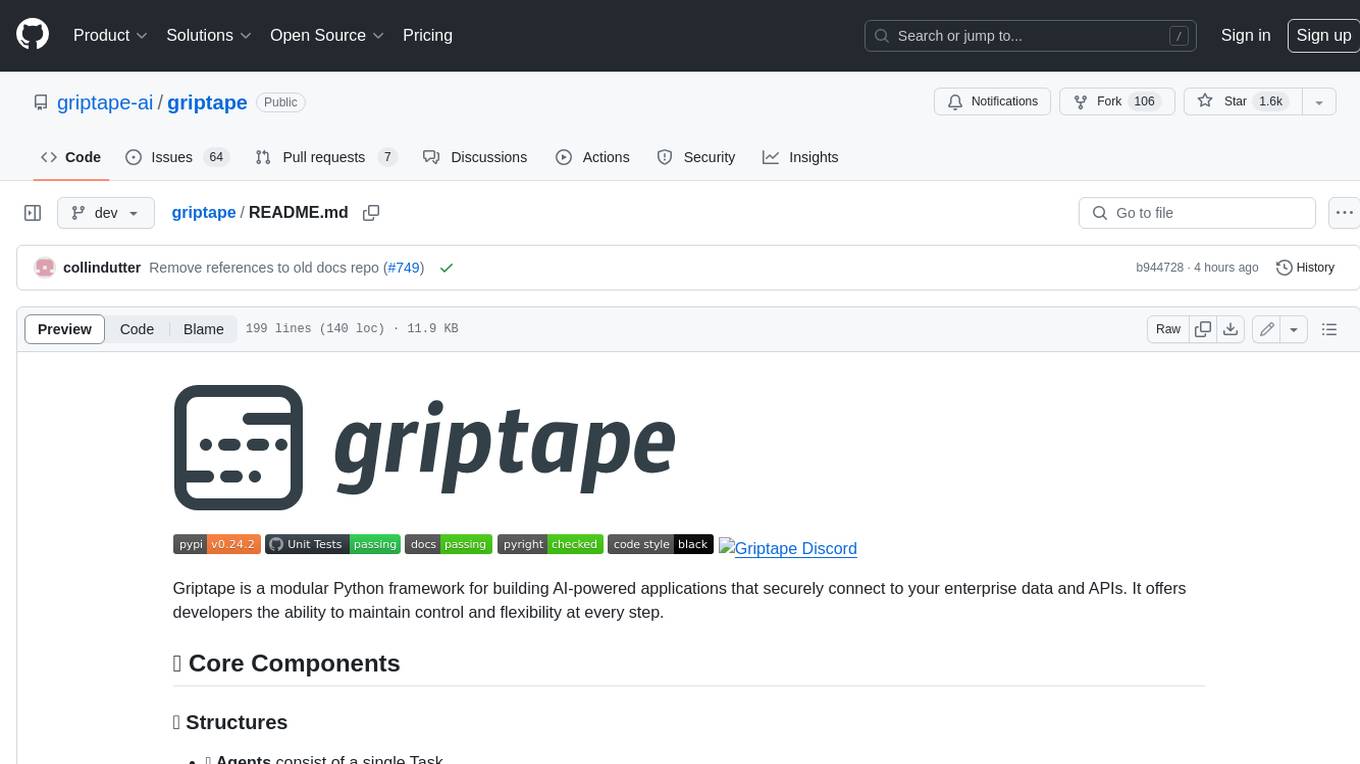
griptape
Griptape is a modular Python framework for building AI-powered applications that securely connect to your enterprise data and APIs. It offers developers the ability to maintain control and flexibility at every step. Griptape's core components include Structures (Agents, Pipelines, and Workflows), Tasks, Tools, Memory (Conversation Memory, Task Memory, and Meta Memory), Drivers (Prompt and Embedding Drivers, Vector Store Drivers, Image Generation Drivers, Image Query Drivers, SQL Drivers, Web Scraper Drivers, and Conversation Memory Drivers), Engines (Query Engines, Extraction Engines, Summary Engines, Image Generation Engines, and Image Query Engines), and additional components (Rulesets, Loaders, Artifacts, Chunkers, and Tokenizers). Griptape enables developers to create AI-powered applications with ease and efficiency.