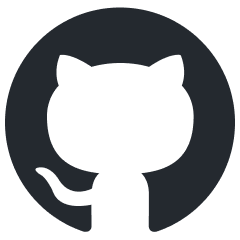
llmgraph
Create knowledge graphs with LLMs
Stars: 271
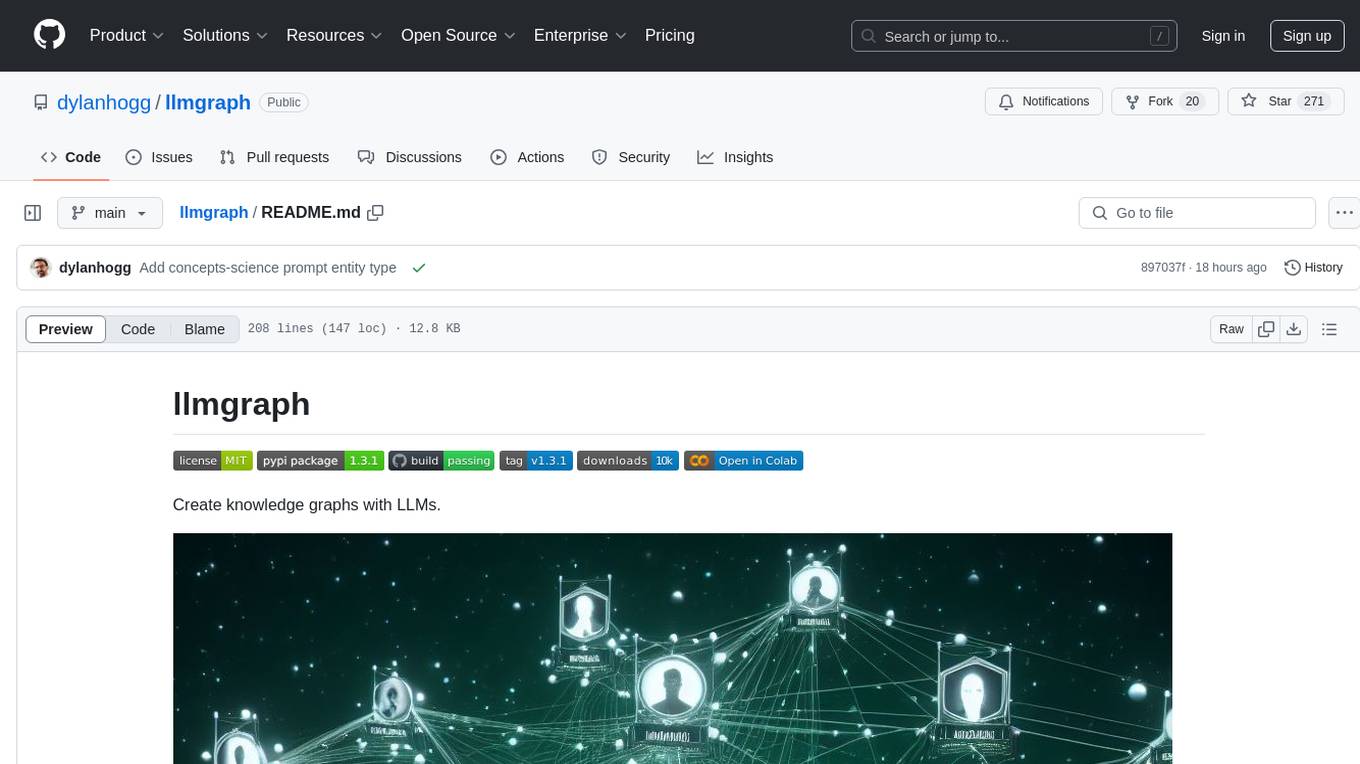
llmgraph is a tool that enables users to create knowledge graphs in GraphML, GEXF, and HTML formats by extracting world knowledge from large language models (LLMs) like ChatGPT. It supports various entity types and relationships, offers cache support for efficient graph growth, and provides insights into LLM costs. Users can customize the model used and interact with different LLM providers. The tool allows users to generate interactive graphs based on a specified entity type and Wikipedia link, making it a valuable resource for knowledge graph creation and exploration.
README:
Create knowledge graphs with LLMs.
llmgraph enables you to create knowledge graphs in GraphML, GEXF, and HTML formats (generated via pyvis) from a given source entity Wikipedia page. The knowledge graphs are generated by extracting world knowledge from ChatGPT or other large language models (LLMs) as supported by LiteLLM.
For a background on knowledge graphs see a youtube overview by Computerphile
- Create knowledge graphs, given a source entity.
- Uses ChatGPT (or another specified LLM) to extract world knowledge.
- Generate knowledge graphs in HTML, GraphML, and GEXF formats.
- Many entity types and relationships supported by customised prompts.
- Cache support to iteratively grow a knowledge graph, efficiently.
- Outputs
total tokens
used to understand LLM costs (even though a default run is only about 1 cent). - Customisable model (default is OpenAI
gpt-4o-mini
for speed and cost).
You can install llmgraph using pip, ideally into a Python virtual environment:
pip install llmgraph
Alternatively, checkout an example notebook that uses llmgraph and you can run directly in Google Colab.
In addition to GraphML and GEXF formats, an HTML pyvis physics enabled graph can be viewed:
Generate above machine-learning graph:
llmgraph machine-learning "https://en.wikipedia.org/wiki/Artificial_intelligence" --levels 4
View entire graph: machine-learning_artificial-intelligence_v1.0.0_level4_fully_connected.html
The example above was generated with the following command, which requires an entity_type
and a quoted entity_wikipedia
souce url:
llmgraph machine-learning "https://en.wikipedia.org/wiki/Artificial_intelligence" --levels 3
This example creates a 3 level graph, based on the given start node Artificial Intelligence
.
By default OpenAI is used and you will need to set an environment variable 'OPENAI_API_KEY
' prior to running. See the OpenAI docs for more info. The total tokens used
is output as the run progresses. For reference this 3 level example used a total of 7,650 gpt-4o-mini tokens, which is approx 1.5 cents as of Oct 2023.
You can also specify a different LLM provider, including running with a local ollama model. You should be able to specify anything supported by LiteLLM as described here: https://docs.litellm.ai/docs/providers. Note that the prompts to extract related entities were tested with OpenAI and may not work as well with other models.
Local ollama/llama2 model example:
llmgraph machine-learning "https://en.wikipedia.org/wiki/Artificial_intelligence" --levels 3 --llm-model ollama/llama2 --llm-base-url http://localhost:<your_port>
The entity_type
sets the LLM prompt used to find related entities to include in the graph. The full list can be seen in prompts.yaml and include the following entity types:
automobile
book
computer-game
concepts-general
concepts-science
creative-general
documentary
food
machine-learning
movie
music
people-historical
podcast
software-engineering
tv
-
entity_type
(TEXT): Entity type (e.g. movie) -
entity_wikipedia
(TEXT): Full Wikipedia link to the root entity
-
--entity-root
(TEXT): Optional root entity name override if different from the Wikipedia page title [default: None] -
--levels
(INTEGER): Number of levels deep to construct from the central root entity [default: 2] -
--max-sum-total-tokens
(INTEGER): Maximum sum of tokens for graph generation [default: 200000] -
--output-folder
(TEXT): Folder location to write outputs [default: ./_output/] -
--llm-model
(TEXT): The model name [default: gpt-4o-mini] -
--llm-temp
(FLOAT): LLM temperature value [default: 0.0] -
--llm-base-url
(TEXT): LLM will use custom base URL instead of the automatic one [default: None] -
--version
: Display llmgraph version and exit. -
--help
: Show this message and exit.
Here are some more examples of the HTML graph output for different entity types and root entities (with commands to generate and links to view full interactive graphs).
Install llmgraph to create your own knowledge graphs! Feel free to share interesting results in the issue section above with a documentation label :)
Command to generate above concepts-general graph:
llmgraph concepts-general "https://en.wikipedia.org/wiki/Knowledge_graph" --levels 4
View entire graph: concepts-general_knowledge-graph_v1.0.0_level4_fully_connected.html
Command to generate above movie graph:
llmgraph movie "https://en.wikipedia.org/wiki/Inception" --levels 4
View entire graph: movie_inception_v1.0.0_level4_fully_connected.html
Command to generate above company graph:
llmgraph company "https://en.wikipedia.org/wiki/OpenAI" --levels 4
View entire graph: company_openai_v1.0.0_level4_fully_connected.html
Command to generate above people-historical graph:
llmgraph people-historical "https://en.wikipedia.org/wiki/John_von_Neumann" --levels 4
View entire graph: people-historical_john-von-neumann_v1.0.0_level4_fully_connected.html
Here is an example of the prompt template, with place holders, used to generate related entities from a given source entity. This is applied recursively to create a knowledge graph, merging duplicated nodes as required.
You are knowledgeable about {knowledgeable_about}.
List, in json array format, the top {top_n} {entities} most like '{{entity_root}}'
with Wikipedia link, reasons for similarity, similarity on scale of 0 to 1.
Format your response in json array format as an array with column names: 'name', 'wikipedia_link', 'reason_for_similarity', and 'similarity'.
Example response: {{{{"name": "Example {entity}","wikipedia_link": "https://en.wikipedia.org/wiki/Example_{entity_underscored}","reason_for_similarity": "Reason for similarity","similarity": 0.5}}}}
It works well on the primary tested LLM, being OpenAI gpt-4o-mini. Results are ok, but not as good using Llama2. The prompt source of truth and additional details can be see in prompts.yaml.
Each entity type has custom placeholders, for example concepts-general
and documentary
:
concepts-general:
system: You are a highly knowledgeable ontologist and creator of knowledge graphs.
knowledgeable_about: many concepts and ontologies.
entities: concepts
entity: concept name
top_n: 5
documentary:
system: You are knowledgeable about documentaries of all types, and genres.
knowledgeable_about: documentaries of all types, and genres
entities: Documentaries
entity: Documentary
top_n: 5
Each call to the LLM API (and Wikipedia) is cached locally in a .joblib_cache
folder. This allows an interrupted run to be resumed without duplicating identical calls. It also allows a re-run with a higher --level
option to re-use results from the lower level run (assuming the same entity type and source).
- Contrast graph output from different LLM models (e.g. Llama2 vs Mistral vs ChatGPT-4)
- Investigate the hypothosis that this approach provides insight into how an LLM views the world.
- Include more examples in this documentation and make examples available for easy browsing.
- Instructions for running locally and adding a custom
entity_type
prompt. - Better pyviz html output, in particular including reasons for entity relationship in UI and arguments for pixel size etc.
- Parallelise API calls and result processing.
- Remove dependency on Wikipedia entities as a source.
- Contrast results from llmgraphg with other non-LLM graph construction e.g. using wikipedia page links, or direct article embeddings.
Contributions to llmgraph are welcome. Please follow these steps:
- Fork the repository.
- Create a new branch for your feature or bug fix.
- Make your changes and commit them.
- Create a pull request with a description of your changes.
Thanks to @breitburg for implementing the LiteLLM updates.
- https://arxiv.org/abs/2211.10511 - Knowledge Graph Generation From Text
- https://arxiv.org/abs/2310.04562 - Towards Foundation Models for Knowledge Graph Reasoning
- https://arxiv.org/abs/2206.14268 - BertNet: Harvesting Knowledge Graphs with Arbitrary Relations from Pretrained Language Models
- https://arxiv.org/abs/2312.02783 - Large Language Models on Graphs: A Comprehensive Survey
- https://github.com/aws/graph-notebook - Graph Notebook: easily query and visualize graphs
- https://github.com/KiddoZhu/NBFNet-PyG - PyG re-implementation of Neural Bellman-Ford Networks
- https://caminao.blog/knowledge-management-booklet/a-hitchhikers-guide-to-knowledge-galaxies/ - A Hitchhiker’s Guide to Knowledge Galaxies
- https://github.com/PeterGriffinJin/Awesome-Language-Model-on-Graphs - A curated list of papers and resources based on "Large Language Models on Graphs: A Comprehensive Survey".
For Tasks:
Click tags to check more tools for each tasksFor Jobs:
Alternative AI tools for llmgraph
Similar Open Source Tools
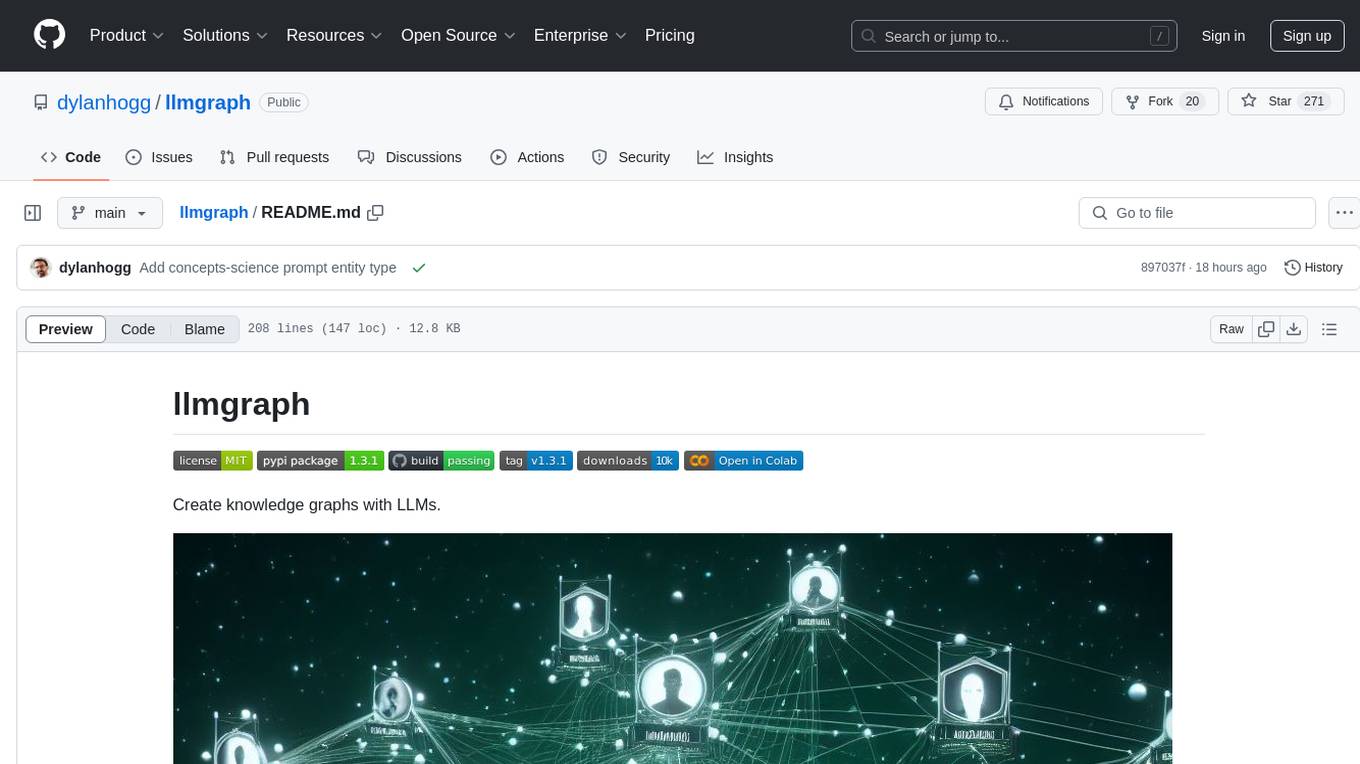
llmgraph
llmgraph is a tool that enables users to create knowledge graphs in GraphML, GEXF, and HTML formats by extracting world knowledge from large language models (LLMs) like ChatGPT. It supports various entity types and relationships, offers cache support for efficient graph growth, and provides insights into LLM costs. Users can customize the model used and interact with different LLM providers. The tool allows users to generate interactive graphs based on a specified entity type and Wikipedia link, making it a valuable resource for knowledge graph creation and exploration.
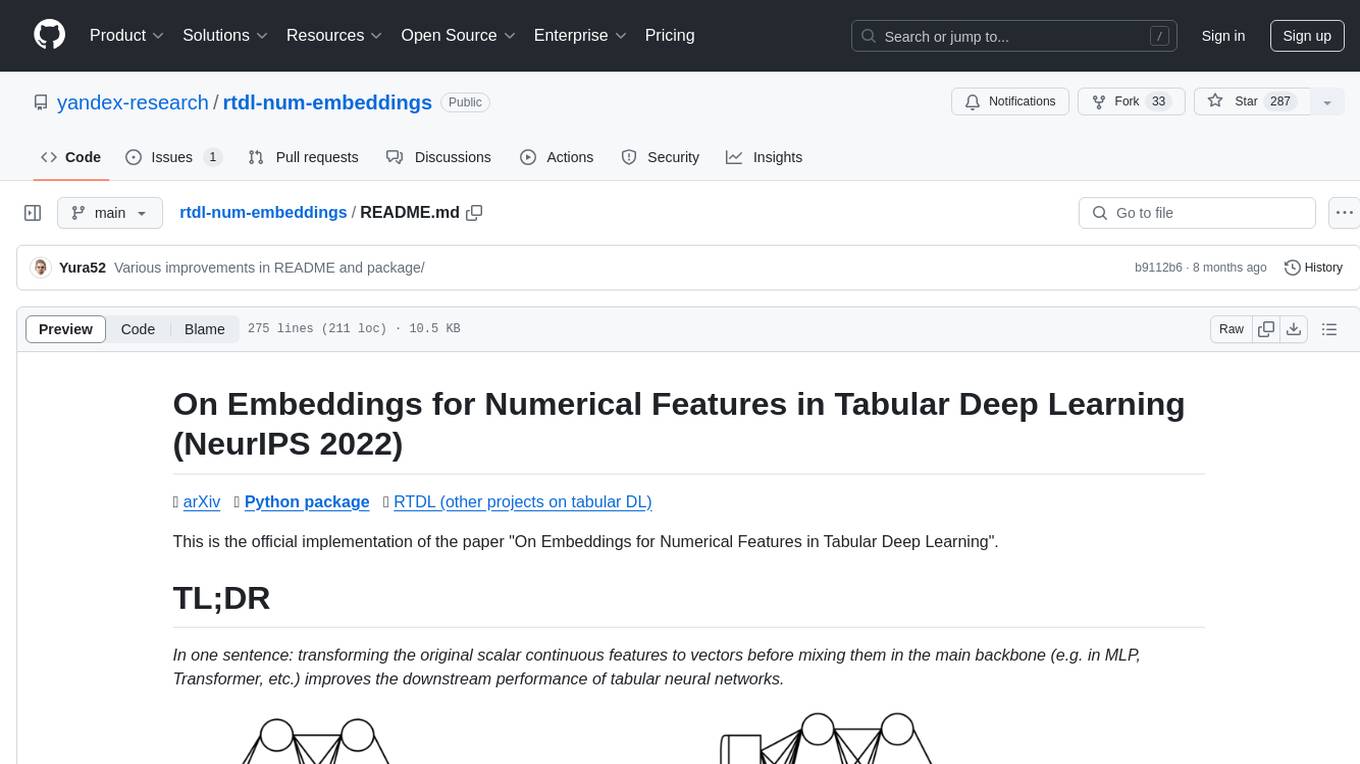
rtdl-num-embeddings
This repository provides the official implementation of the paper 'On Embeddings for Numerical Features in Tabular Deep Learning'. It focuses on transforming scalar continuous features into vectors before integrating them into the main backbone of tabular neural networks, showcasing improved performance. The embeddings for continuous features are shown to enhance the performance of tabular DL models and are applicable to various conventional backbones, offering efficiency comparable to Transformer-based models. The repository includes Python packages for practical usage, exploration of metrics and hyperparameters, and reproducing reported results for different algorithms and datasets.
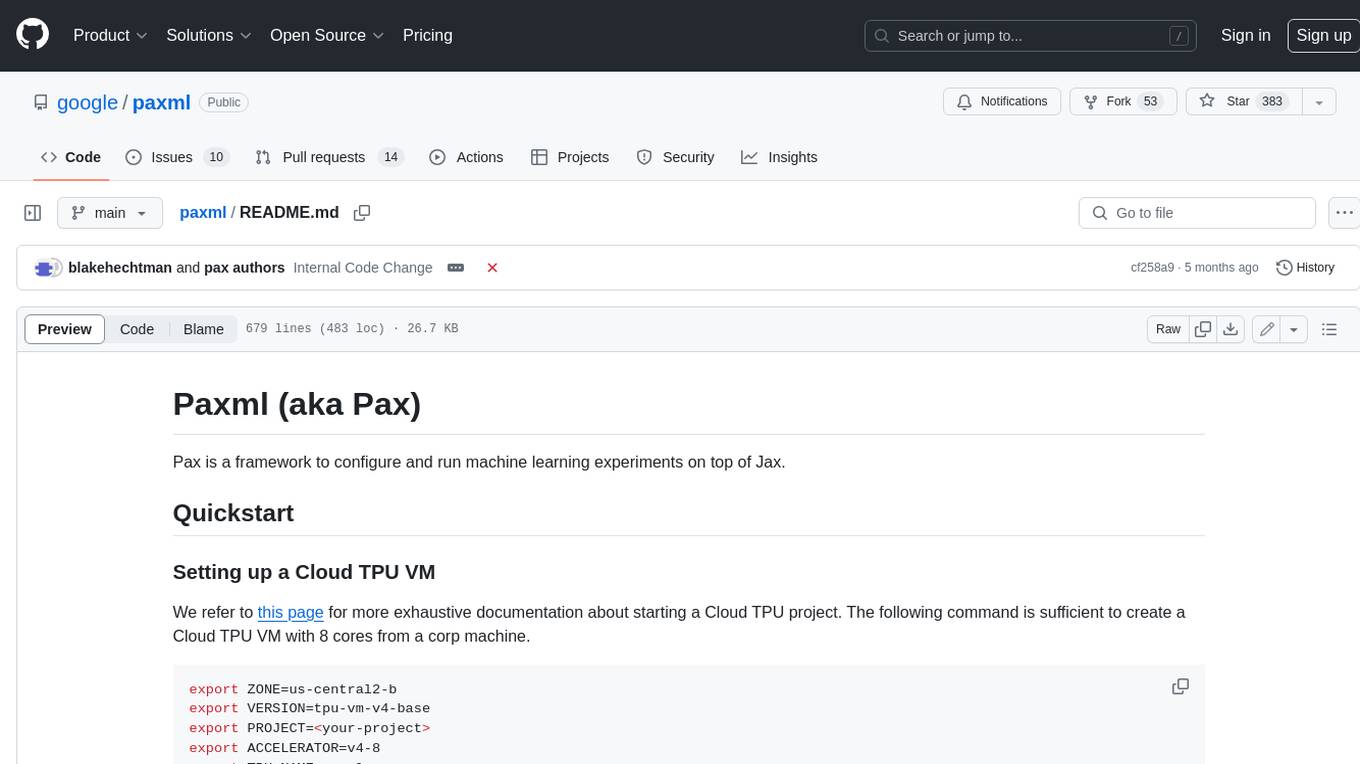
paxml
Pax is a framework to configure and run machine learning experiments on top of Jax.
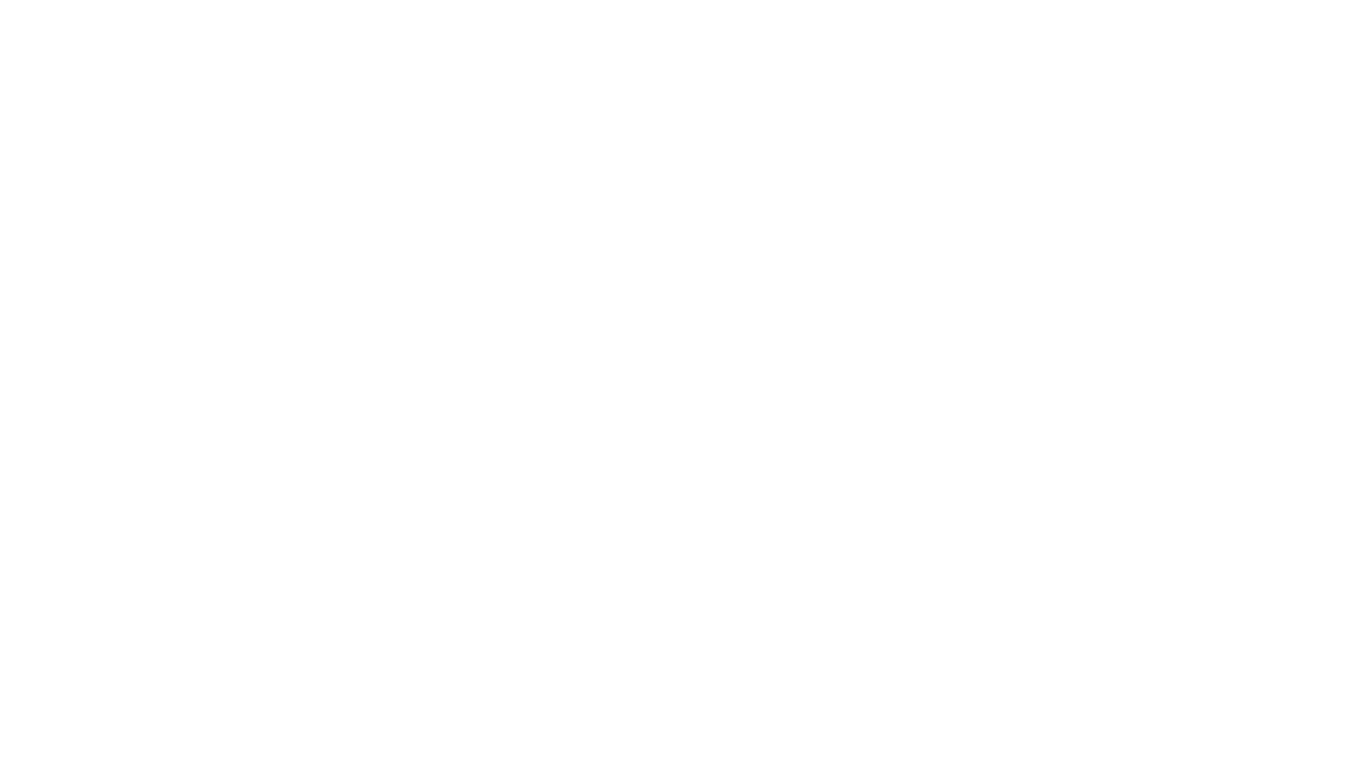
raglite
RAGLite is a Python toolkit for Retrieval-Augmented Generation (RAG) with PostgreSQL or SQLite. It offers configurable options for choosing LLM providers, database types, and rerankers. The toolkit is fast and permissive, utilizing lightweight dependencies and hardware acceleration. RAGLite provides features like PDF to Markdown conversion, multi-vector chunk embedding, optimal semantic chunking, hybrid search capabilities, adaptive retrieval, and improved output quality. It is extensible with a built-in Model Context Protocol server, customizable ChatGPT-like frontend, document conversion to Markdown, and evaluation tools. Users can configure RAGLite for various tasks like configuring, inserting documents, running RAG pipelines, computing query adapters, evaluating performance, running MCP servers, and serving frontends.
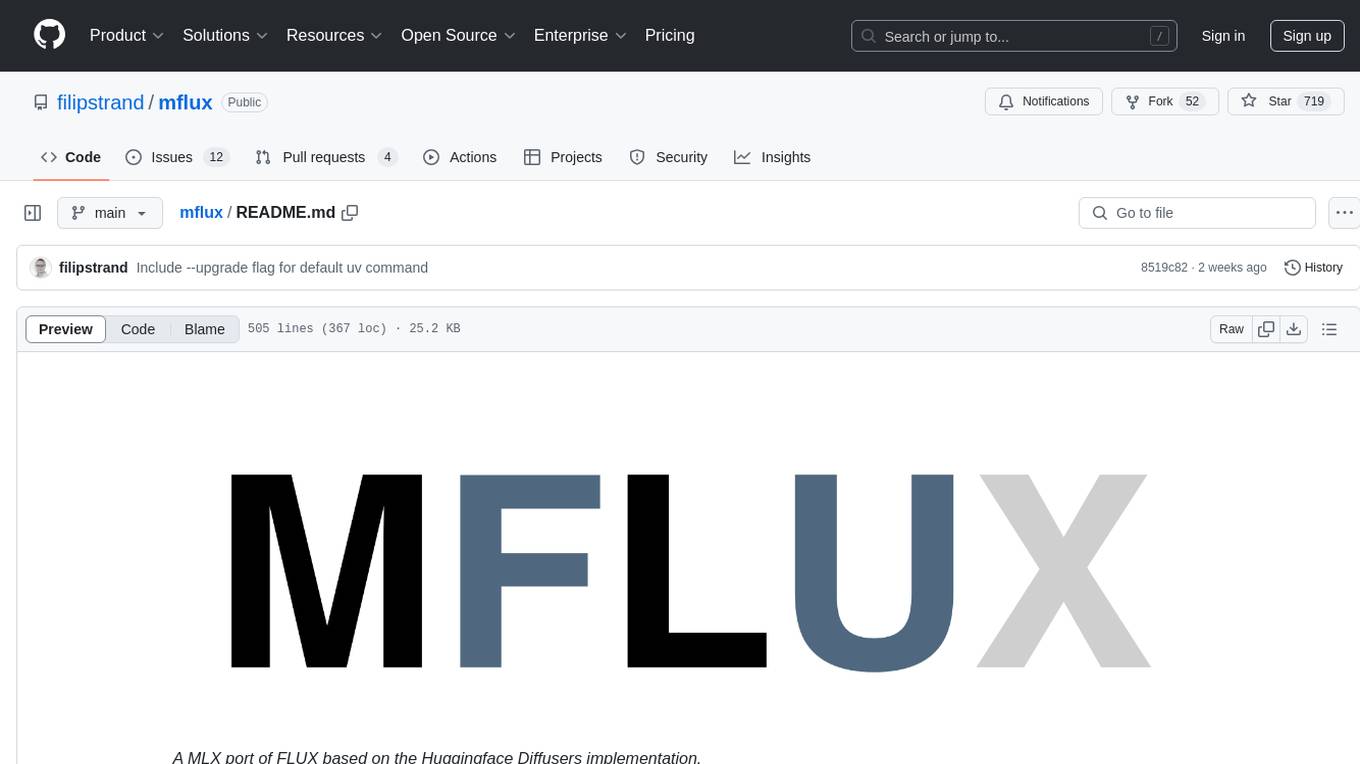
mflux
MFLUX is a line-by-line port of the FLUX implementation in the Huggingface Diffusers library to Apple MLX. It aims to run powerful FLUX models from Black Forest Labs locally on Mac machines. The codebase is minimal and explicit, prioritizing readability over generality and performance. Models are implemented from scratch in MLX, with tokenizers from the Huggingface Transformers library. Dependencies include Numpy and Pillow for image post-processing. Installation can be done using `uv tool` or classic virtual environment setup. Command-line arguments allow for image generation with specified models, prompts, and optional parameters. Quantization options for speed and memory reduction are available. LoRA adapters can be loaded for fine-tuning image generation. Controlnet support provides more control over image generation with reference images. Current limitations include generating images one by one, lack of support for negative prompts, and some LoRA adapters not working.
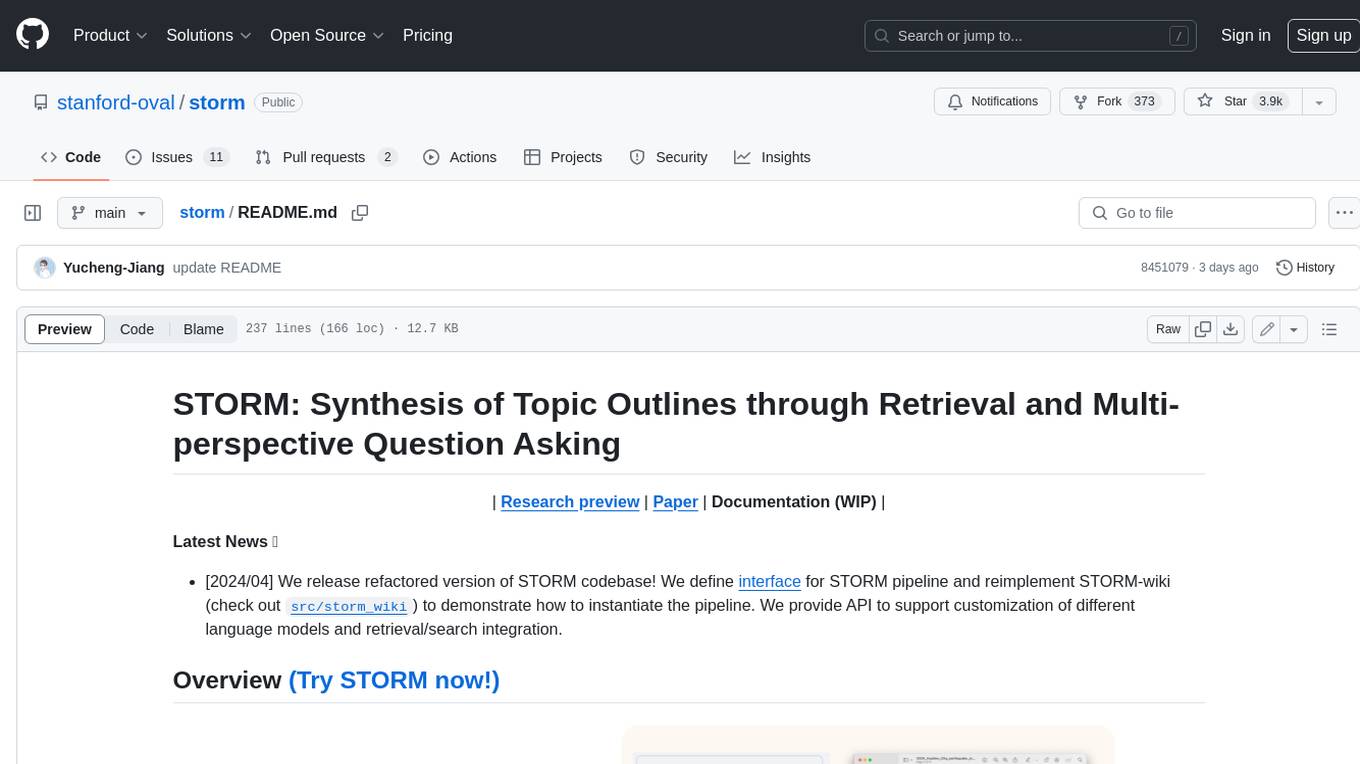
storm
STORM is a LLM system that writes Wikipedia-like articles from scratch based on Internet search. While the system cannot produce publication-ready articles that often require a significant number of edits, experienced Wikipedia editors have found it helpful in their pre-writing stage. **Try out our [live research preview](https://storm.genie.stanford.edu/) to see how STORM can help your knowledge exploration journey and please provide feedback to help us improve the system 🙏!**
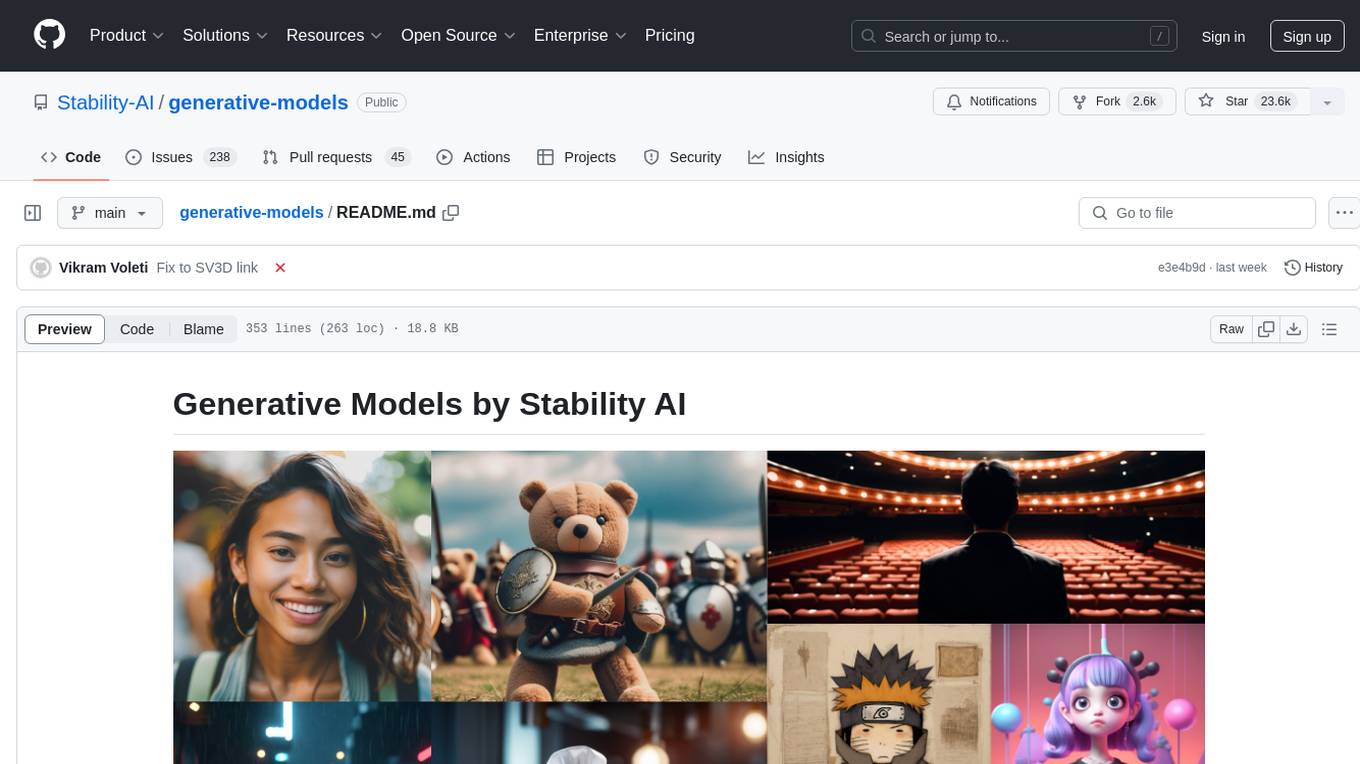
generative-models
Generative Models by Stability AI is a repository that provides various generative models for research purposes. It includes models like Stable Video 4D (SV4D) for video synthesis, Stable Video 3D (SV3D) for multi-view synthesis, SDXL-Turbo for text-to-image generation, and more. The repository focuses on modularity and implements a config-driven approach for building and combining submodules. It supports training with PyTorch Lightning and offers inference demos for different models. Users can access pre-trained models like SDXL-base-1.0 and SDXL-refiner-1.0 under a CreativeML Open RAIL++-M license. The codebase also includes tools for invisible watermark detection in generated images.
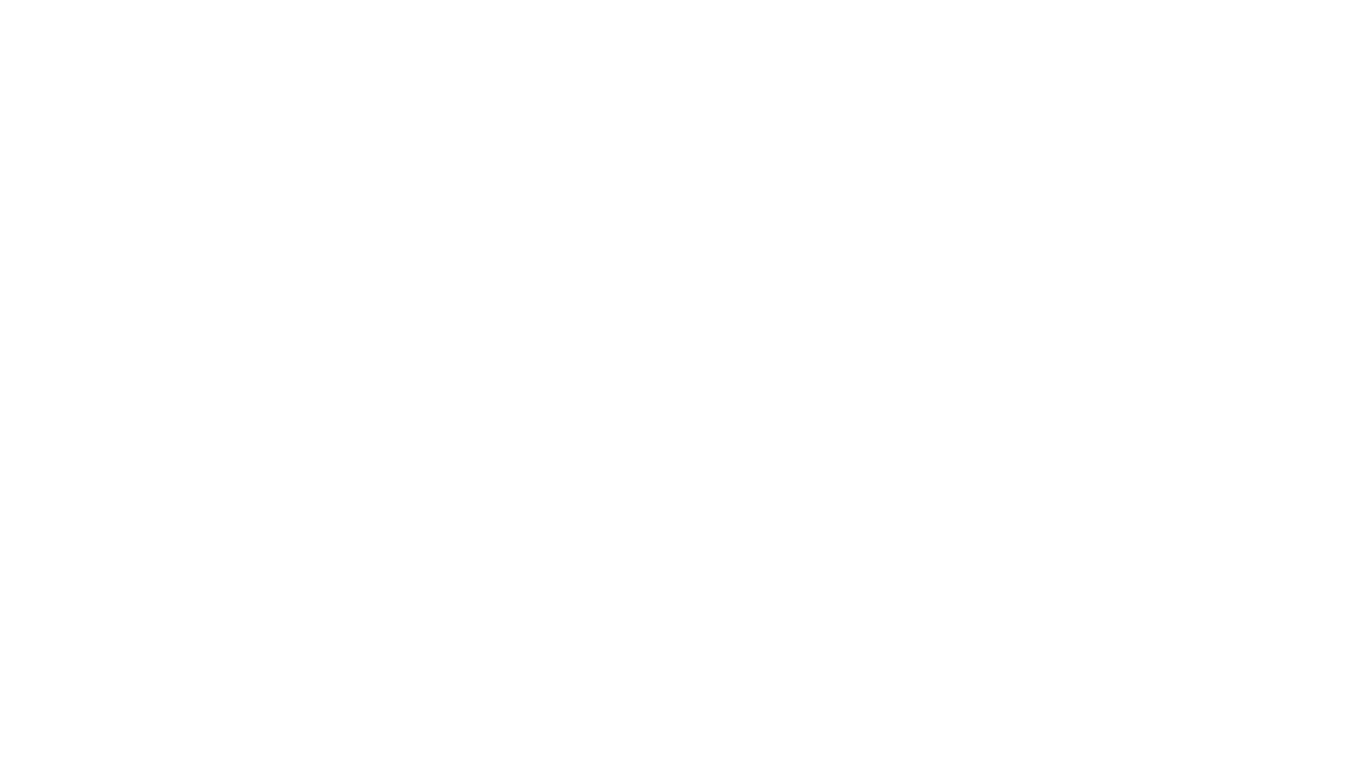
upgini
Upgini is an intelligent data search engine with a Python library that helps users find and add relevant features to their ML pipeline from various public, community, and premium external data sources. It automates the optimization of connected data sources by generating an optimal set of machine learning features using large language models, GraphNNs, and recurrent neural networks. The tool aims to simplify feature search and enrichment for external data to make it a standard approach in machine learning pipelines. It democratizes access to data sources for the data science community.
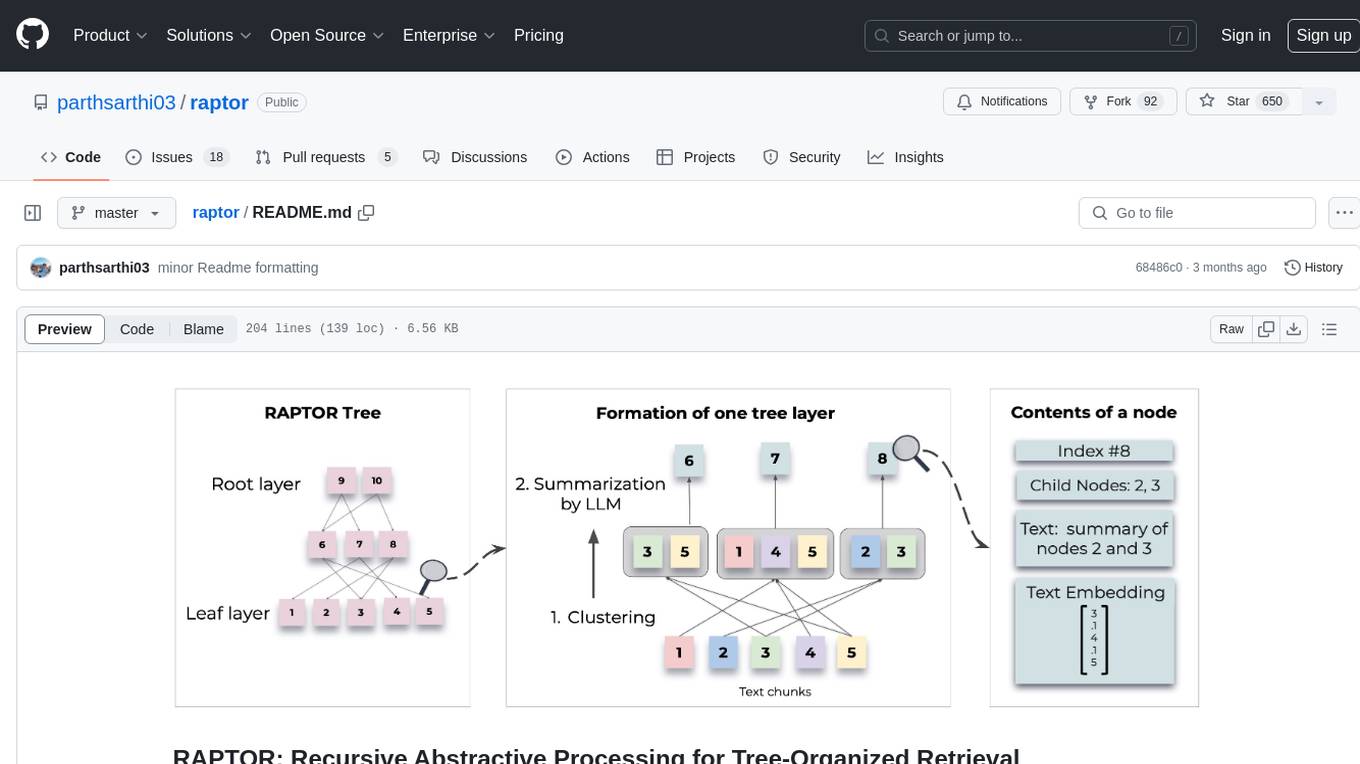
raptor
RAPTOR introduces a novel approach to retrieval-augmented language models by constructing a recursive tree structure from documents. This allows for more efficient and context-aware information retrieval across large texts, addressing common limitations in traditional language models. Users can add documents to the tree, answer questions based on indexed documents, save and load the tree, and extend RAPTOR with custom summarization, question-answering, and embedding models. The tool is designed to be flexible and customizable for various NLP tasks.
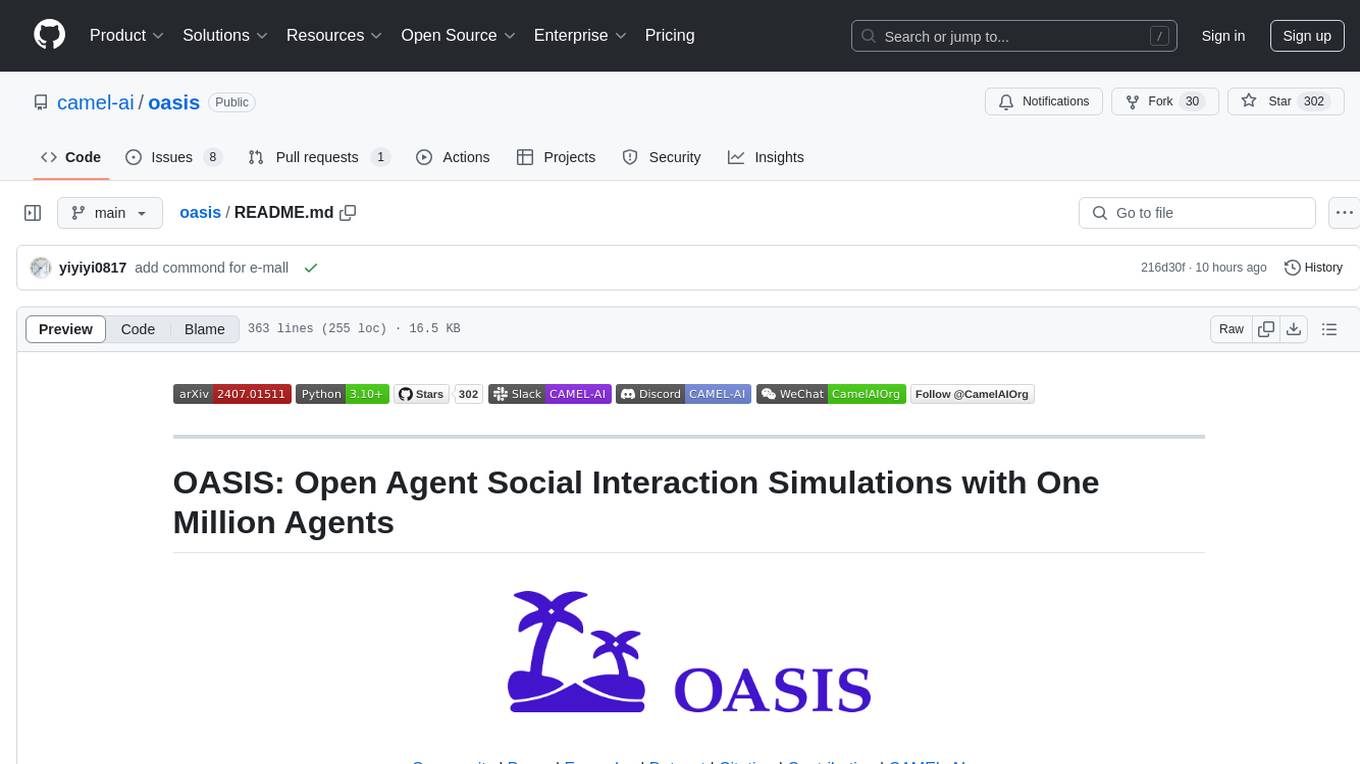
oasis
OASIS is a scalable, open-source social media simulator that integrates large language models with rule-based agents to realistically mimic the behavior of up to one million users on platforms like Twitter and Reddit. It facilitates the study of complex social phenomena such as information spread, group polarization, and herd behavior, offering a versatile tool for exploring diverse social dynamics and user interactions in digital environments. With features like scalability, dynamic environments, diverse action spaces, and integrated recommendation systems, OASIS provides a comprehensive platform for simulating social media interactions at a large scale.
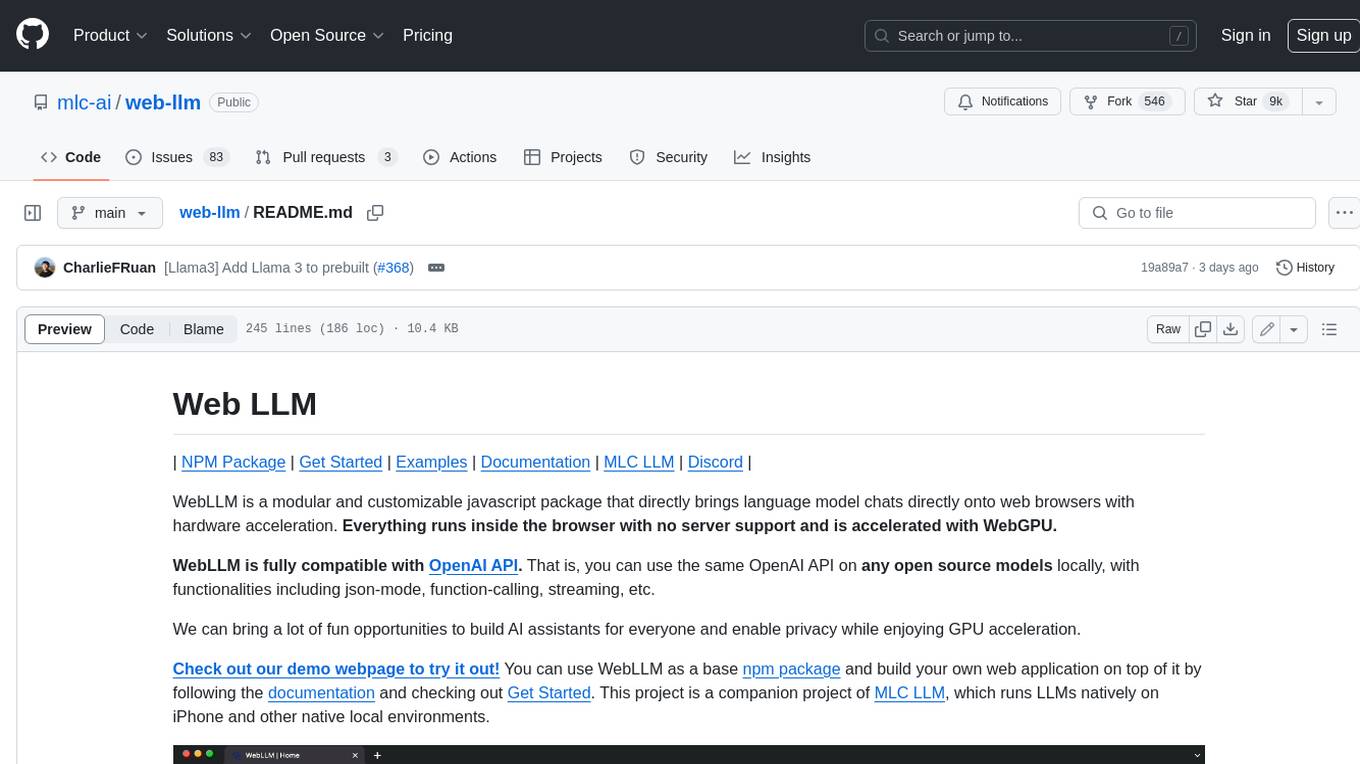
web-llm
WebLLM is a modular and customizable javascript package that directly brings language model chats directly onto web browsers with hardware acceleration. Everything runs inside the browser with no server support and is accelerated with WebGPU. WebLLM is fully compatible with OpenAI API. That is, you can use the same OpenAI API on any open source models locally, with functionalities including json-mode, function-calling, streaming, etc. We can bring a lot of fun opportunities to build AI assistants for everyone and enable privacy while enjoying GPU acceleration.
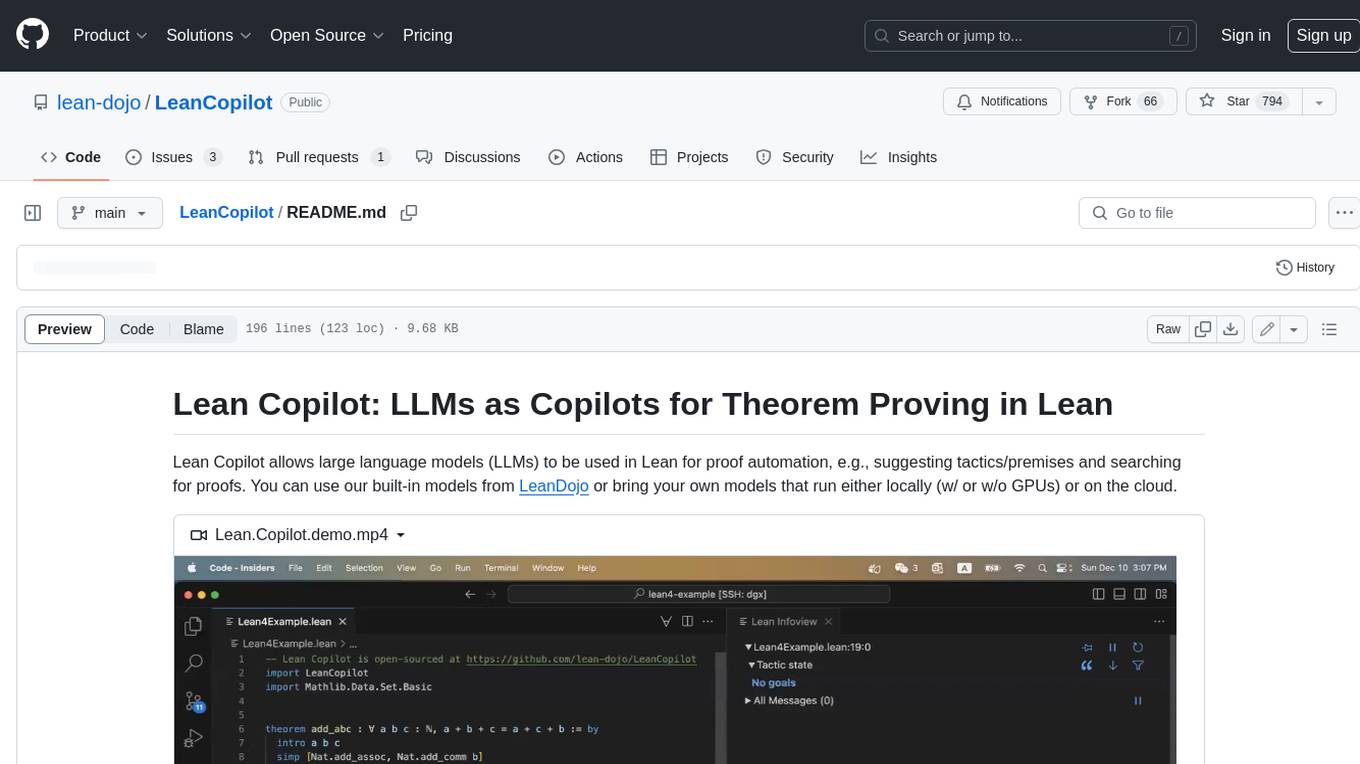
LeanCopilot
Lean Copilot is a tool that enables the use of large language models (LLMs) in Lean for proof automation. It provides features such as suggesting tactics/premises, searching for proofs, and running inference of LLMs. Users can utilize built-in models from LeanDojo or bring their own models to run locally or on the cloud. The tool supports platforms like Linux, macOS, and Windows WSL, with optional CUDA and cuDNN for GPU acceleration. Advanced users can customize behavior using Tactic APIs and Model APIs. Lean Copilot also allows users to bring their own models through ExternalGenerator or ExternalEncoder. The tool comes with caveats such as occasional crashes and issues with premise selection and proof search. Users can get in touch through GitHub Discussions for questions, bug reports, feature requests, and suggestions. The tool is designed to enhance theorem proving in Lean using LLMs.
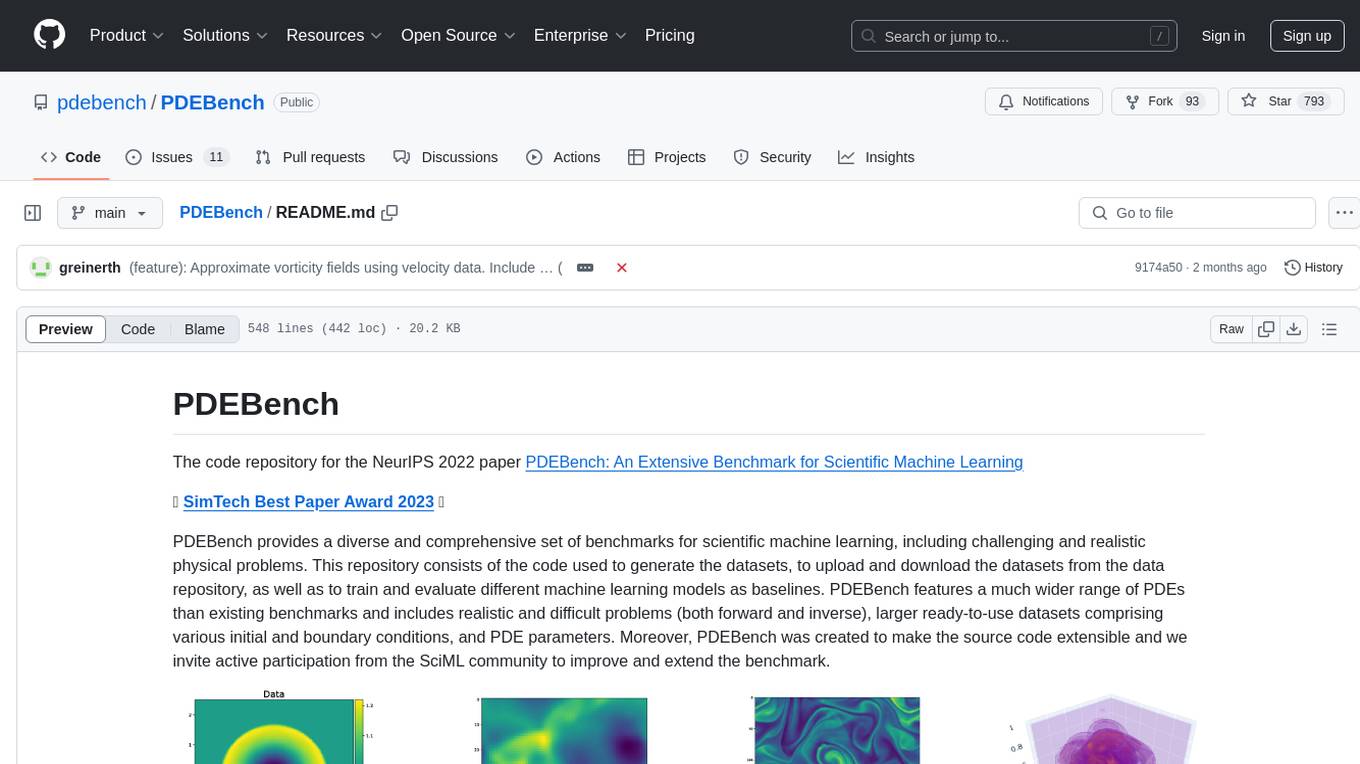
PDEBench
PDEBench provides a diverse and comprehensive set of benchmarks for scientific machine learning, including challenging and realistic physical problems. The repository consists of code for generating datasets, uploading and downloading datasets, training and evaluating machine learning models as baselines. It features a wide range of PDEs, realistic and difficult problems, ready-to-use datasets with various conditions and parameters. PDEBench aims for extensibility and invites participation from the SciML community to improve and extend the benchmark.
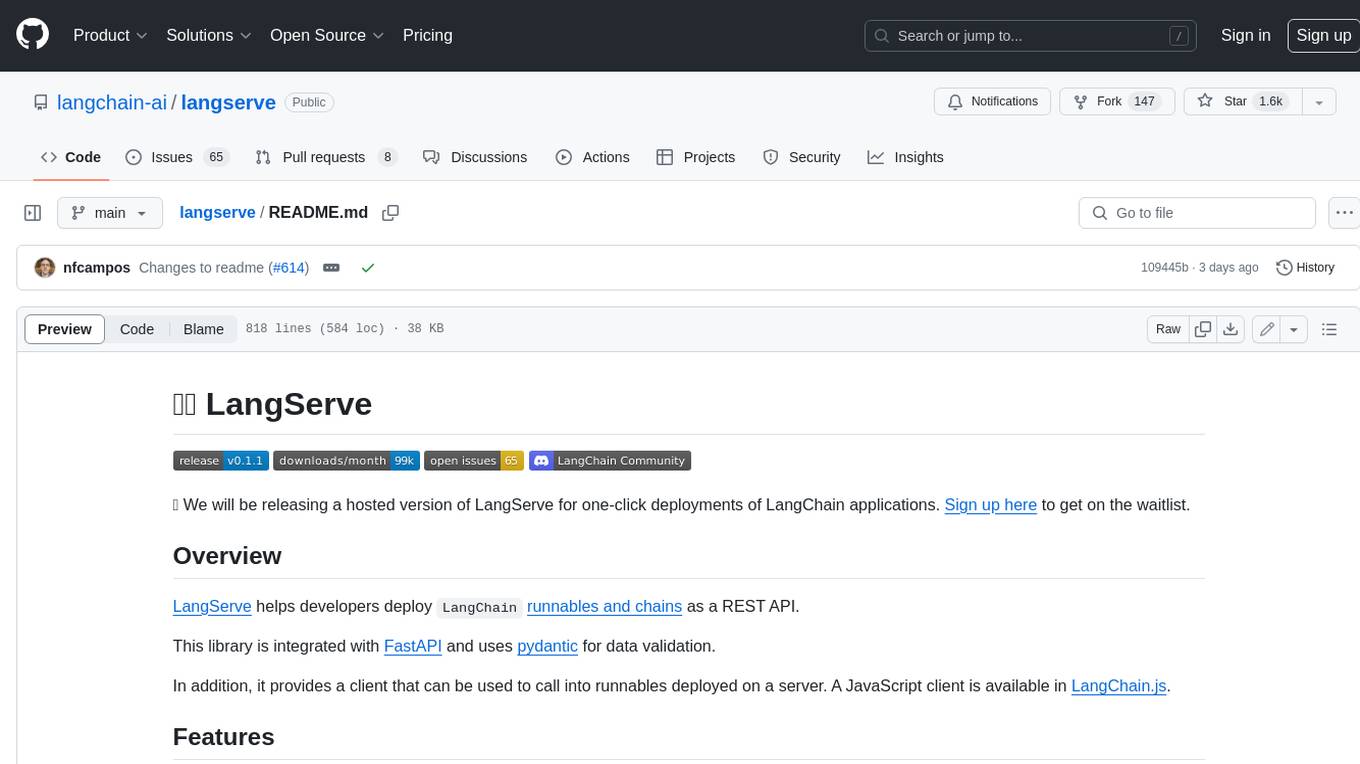
langserve
LangServe helps developers deploy `LangChain` runnables and chains as a REST API. This library is integrated with FastAPI and uses pydantic for data validation. In addition, it provides a client that can be used to call into runnables deployed on a server. A JavaScript client is available in LangChain.js.
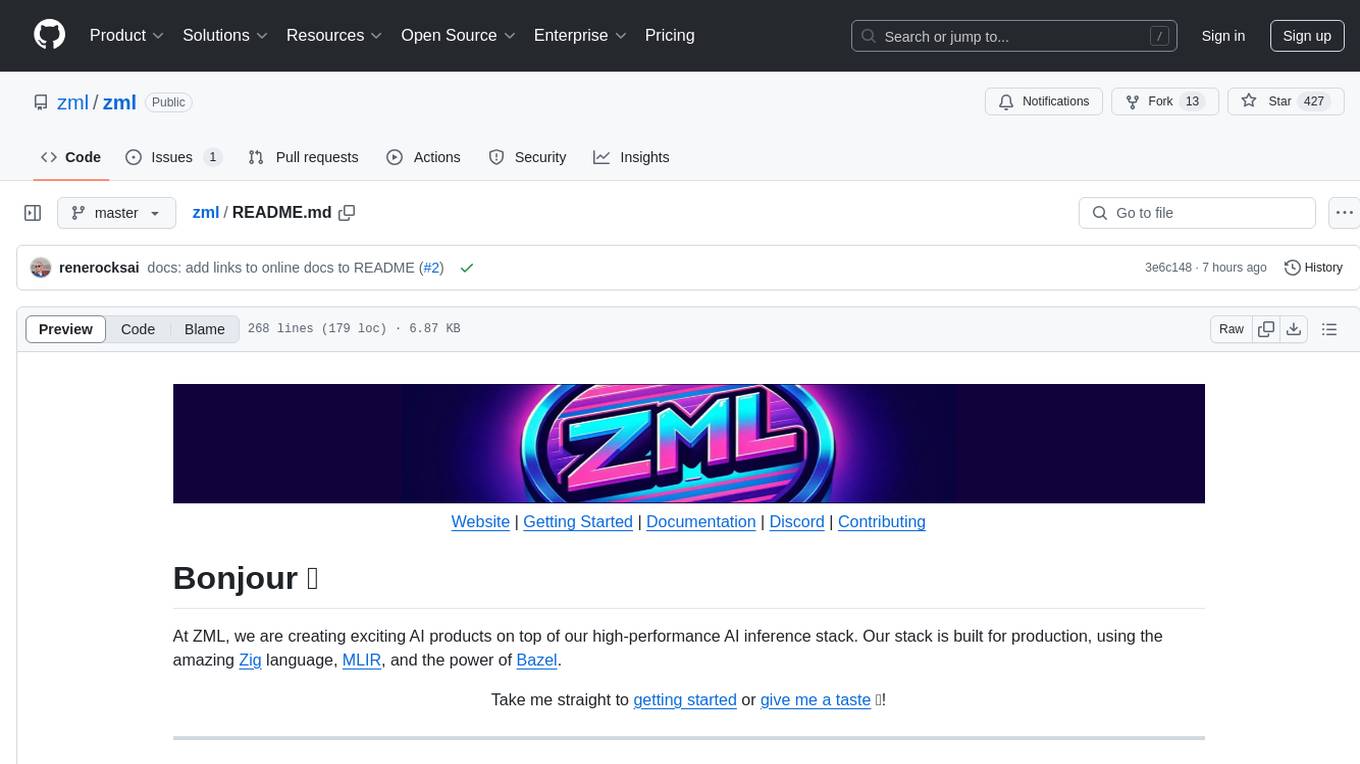
zml
ZML is a high-performance AI inference stack built for production, using Zig language, MLIR, and Bazel. It allows users to create exciting AI projects, run pre-packaged models like MNIST, TinyLlama, OpenLLama, and Meta Llama, and compile models for accelerator runtimes. Users can also run tests, explore examples, and contribute to the project. ZML is licensed under the Apache 2.0 license.
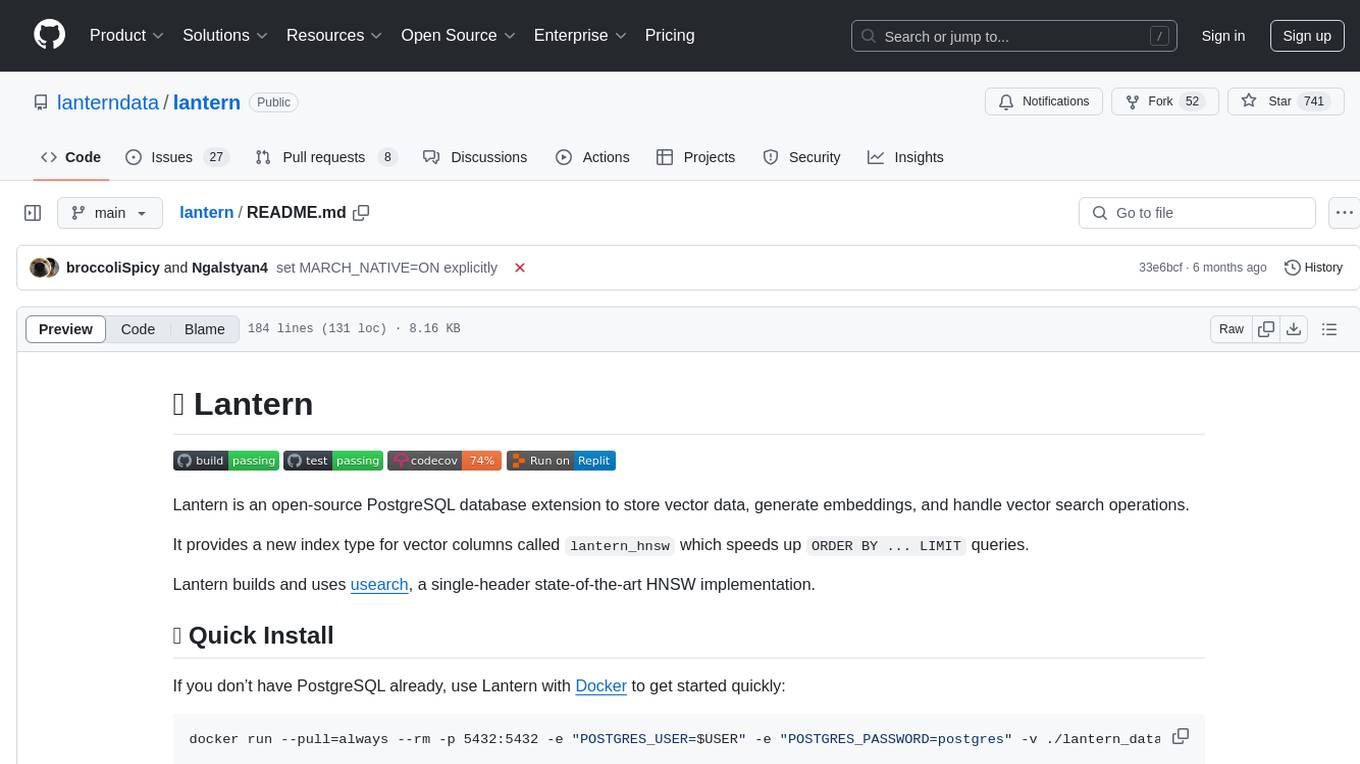
lantern
Lantern is an open-source PostgreSQL database extension designed to store vector data, generate embeddings, and handle vector search operations efficiently. It introduces a new index type called 'lantern_hnsw' for vector columns, which speeds up 'ORDER BY ... LIMIT' queries. Lantern utilizes the state-of-the-art HNSW implementation called usearch. Users can easily install Lantern using Docker, Homebrew, or precompiled binaries. The tool supports various distance functions, index construction parameters, and operator classes for efficient querying. Lantern offers features like embedding generation, interoperability with pgvector, parallel index creation, and external index graph generation. It aims to provide superior performance metrics compared to other similar tools and has a roadmap for future enhancements such as cloud-hosted version, hardware-accelerated distance metrics, industry-specific application templates, and support for version control and A/B testing of embeddings.
For similar tasks
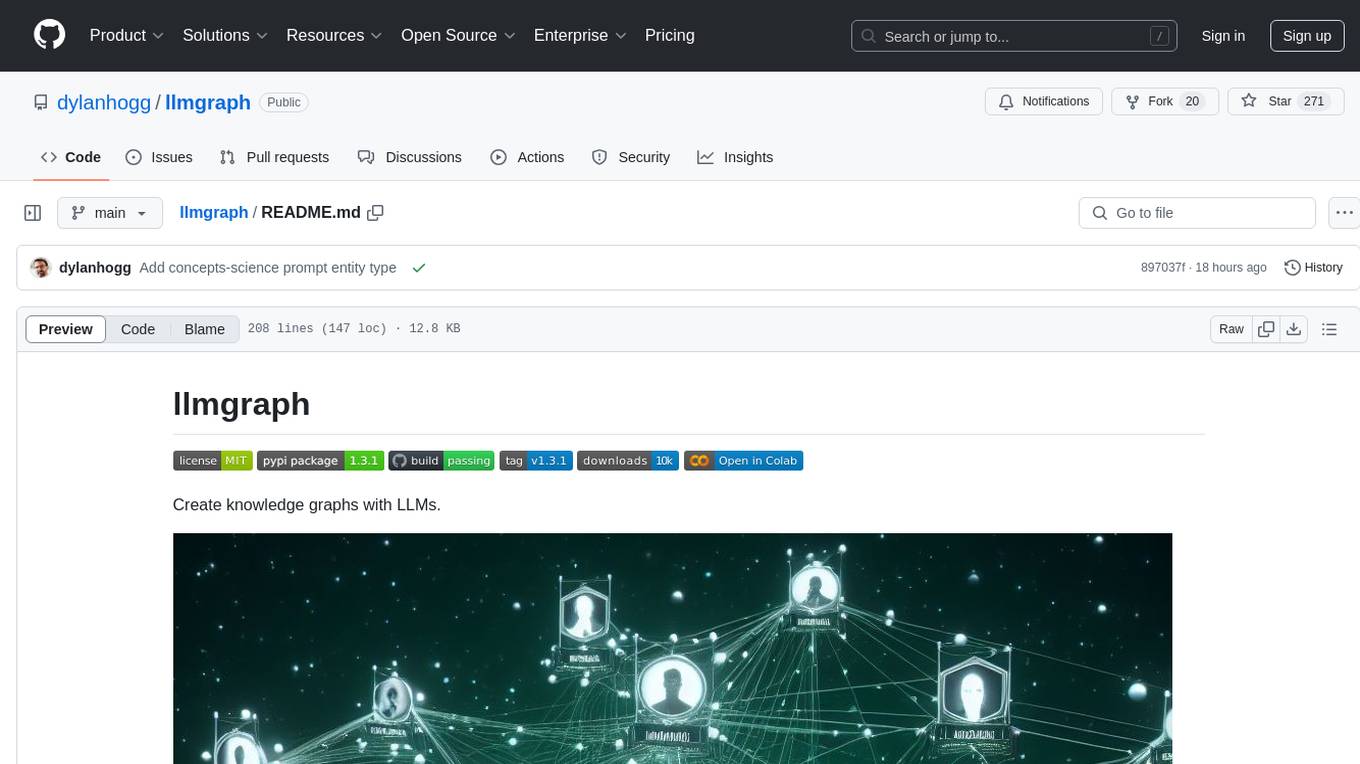
llmgraph
llmgraph is a tool that enables users to create knowledge graphs in GraphML, GEXF, and HTML formats by extracting world knowledge from large language models (LLMs) like ChatGPT. It supports various entity types and relationships, offers cache support for efficient graph growth, and provides insights into LLM costs. Users can customize the model used and interact with different LLM providers. The tool allows users to generate interactive graphs based on a specified entity type and Wikipedia link, making it a valuable resource for knowledge graph creation and exploration.
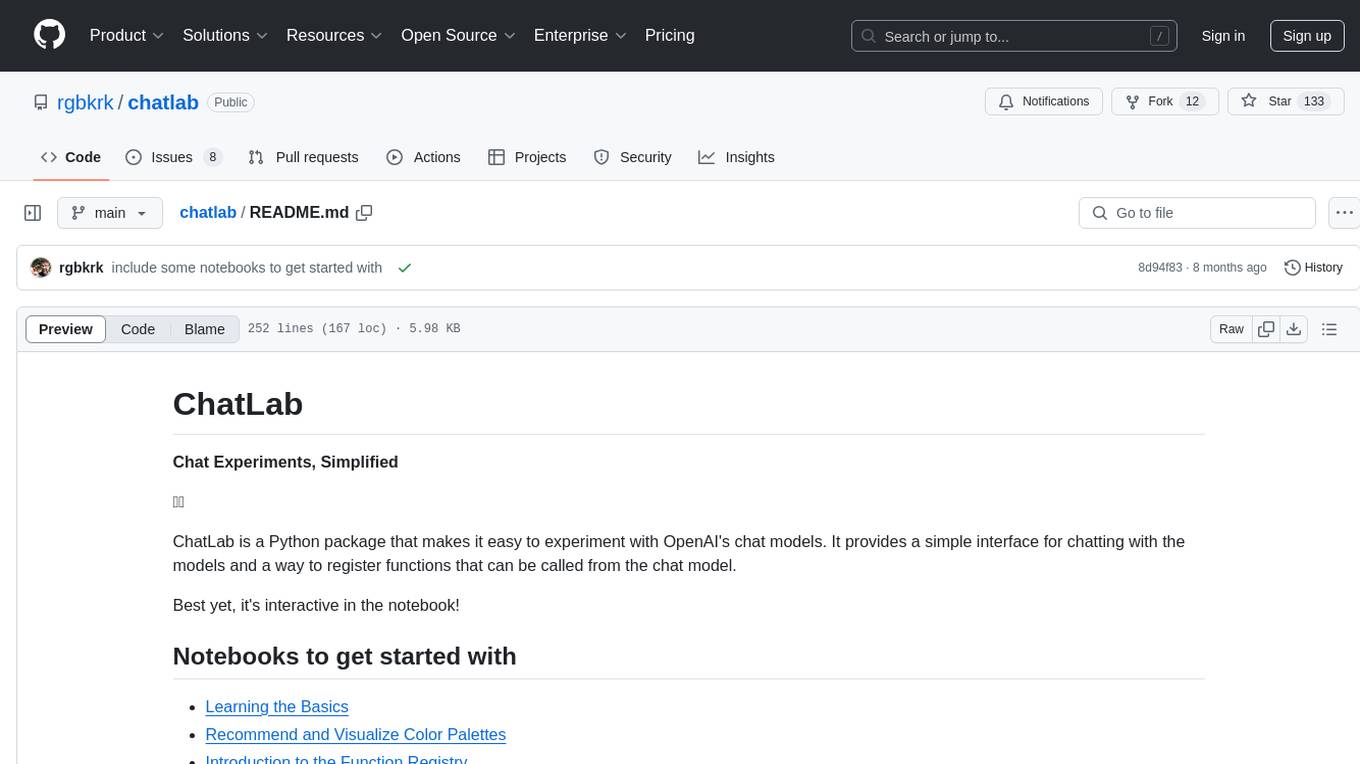
chatlab
ChatLab is a Python package that simplifies experimenting with OpenAI's chat models. It provides an interactive interface for chatting with the models and registering custom functions. Users can easily create chat experiments, visualize color palettes, work with function registry, create knowledge graphs, and perform direct parallel function calling. The tool enables users to interact with chat models and customize functionalities for various tasks.
For similar jobs
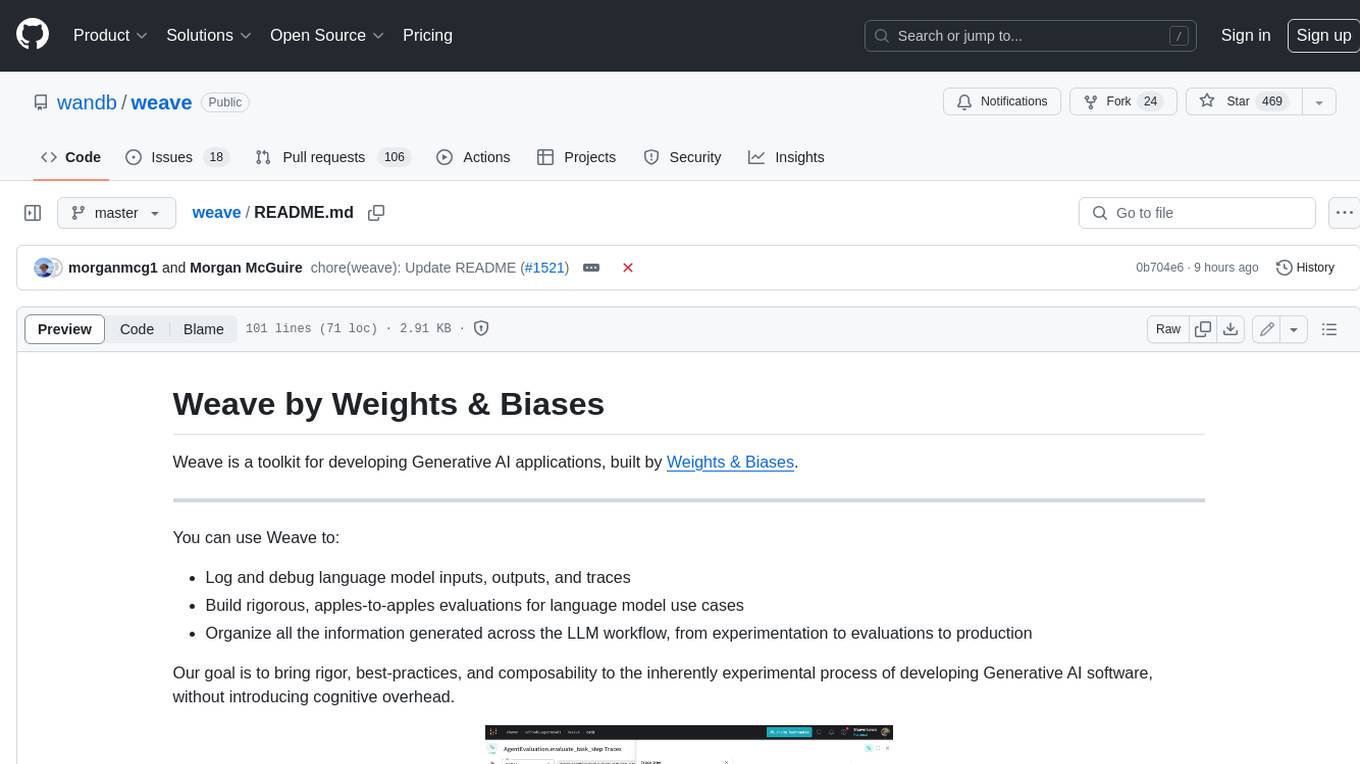
weave
Weave is a toolkit for developing Generative AI applications, built by Weights & Biases. With Weave, you can log and debug language model inputs, outputs, and traces; build rigorous, apples-to-apples evaluations for language model use cases; and organize all the information generated across the LLM workflow, from experimentation to evaluations to production. Weave aims to bring rigor, best-practices, and composability to the inherently experimental process of developing Generative AI software, without introducing cognitive overhead.
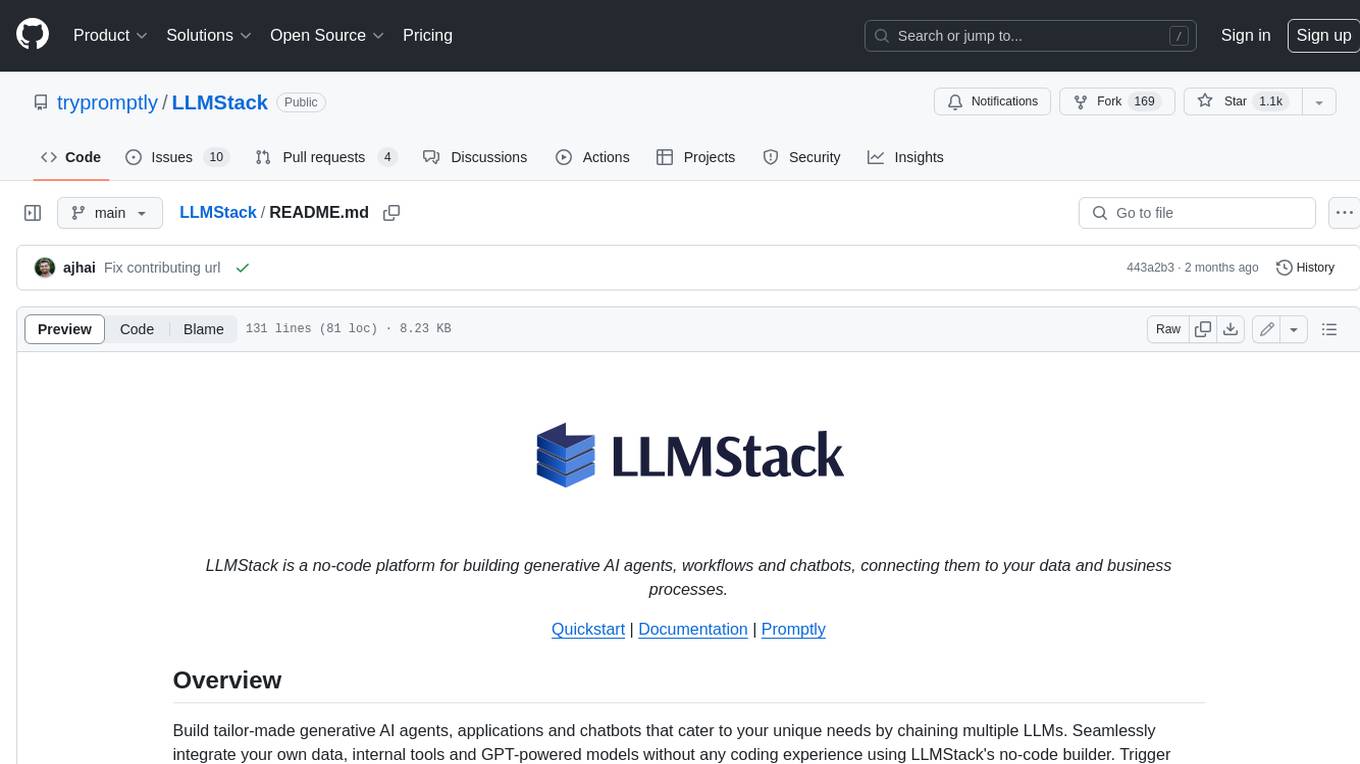
LLMStack
LLMStack is a no-code platform for building generative AI agents, workflows, and chatbots. It allows users to connect their own data, internal tools, and GPT-powered models without any coding experience. LLMStack can be deployed to the cloud or on-premise and can be accessed via HTTP API or triggered from Slack or Discord.
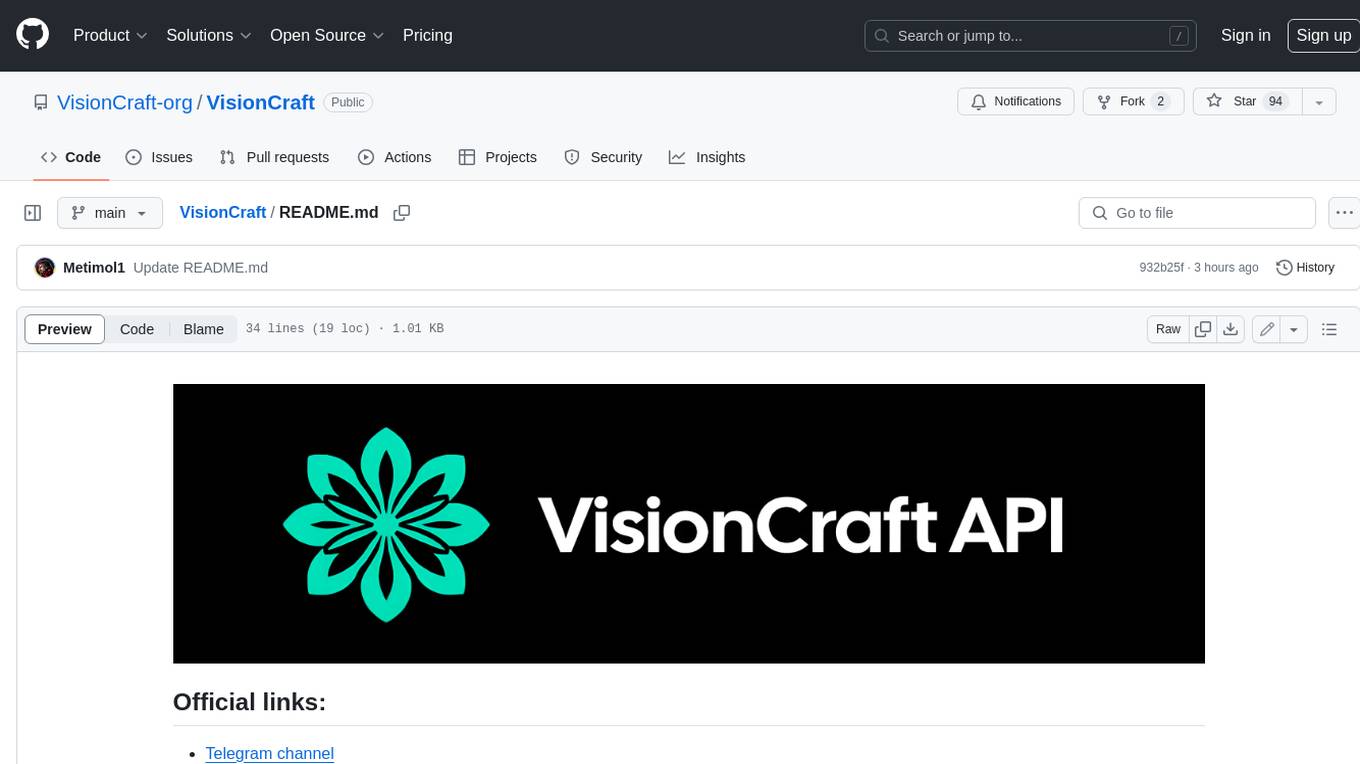
VisionCraft
The VisionCraft API is a free API for using over 100 different AI models. From images to sound.
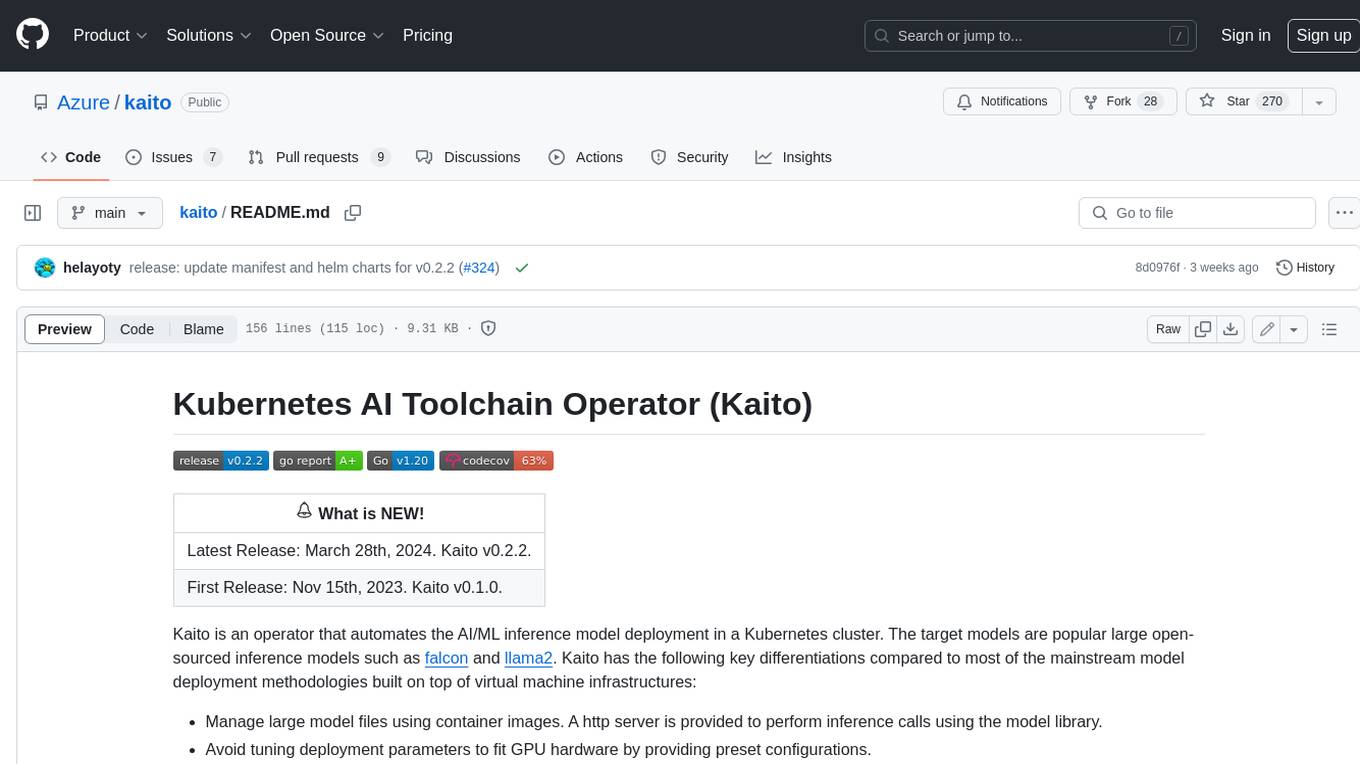
kaito
Kaito is an operator that automates the AI/ML inference model deployment in a Kubernetes cluster. It manages large model files using container images, avoids tuning deployment parameters to fit GPU hardware by providing preset configurations, auto-provisions GPU nodes based on model requirements, and hosts large model images in the public Microsoft Container Registry (MCR) if the license allows. Using Kaito, the workflow of onboarding large AI inference models in Kubernetes is largely simplified.
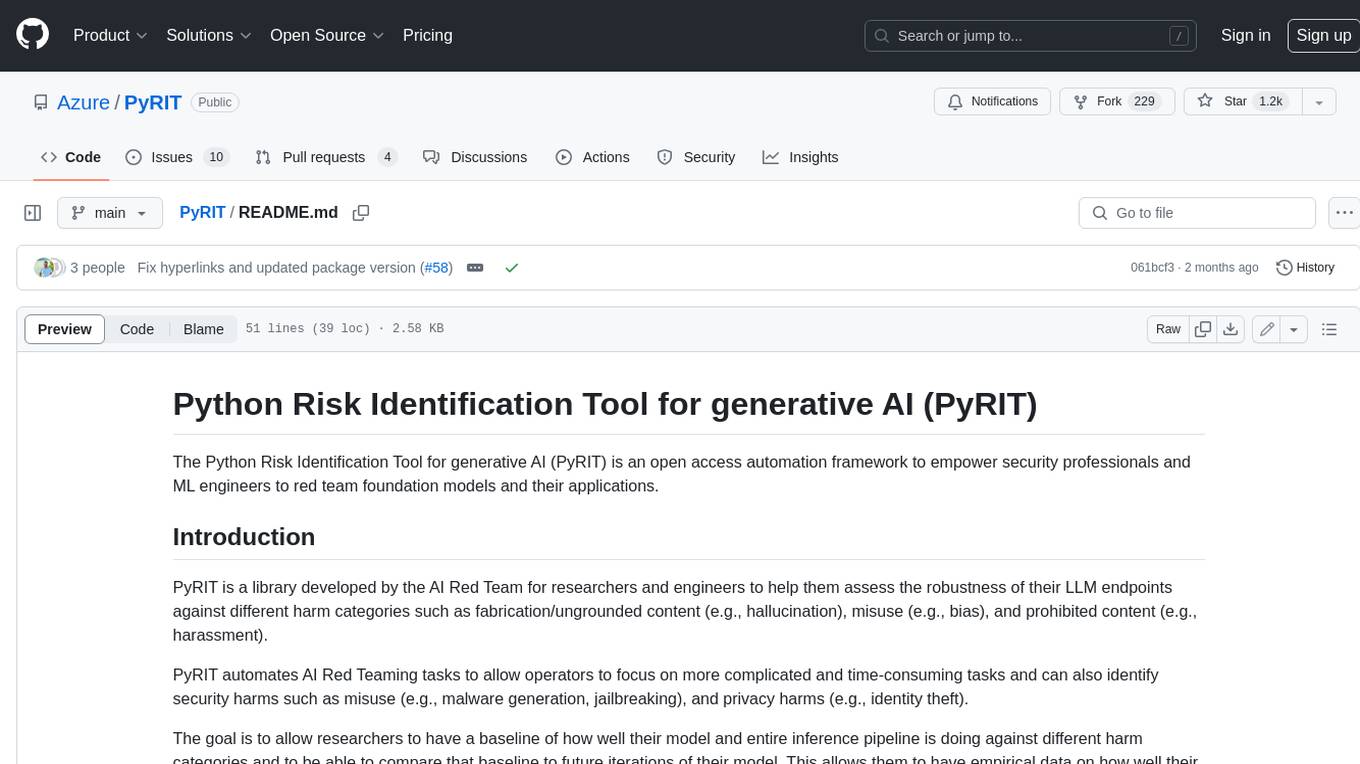
PyRIT
PyRIT is an open access automation framework designed to empower security professionals and ML engineers to red team foundation models and their applications. It automates AI Red Teaming tasks to allow operators to focus on more complicated and time-consuming tasks and can also identify security harms such as misuse (e.g., malware generation, jailbreaking), and privacy harms (e.g., identity theft). The goal is to allow researchers to have a baseline of how well their model and entire inference pipeline is doing against different harm categories and to be able to compare that baseline to future iterations of their model. This allows them to have empirical data on how well their model is doing today, and detect any degradation of performance based on future improvements.
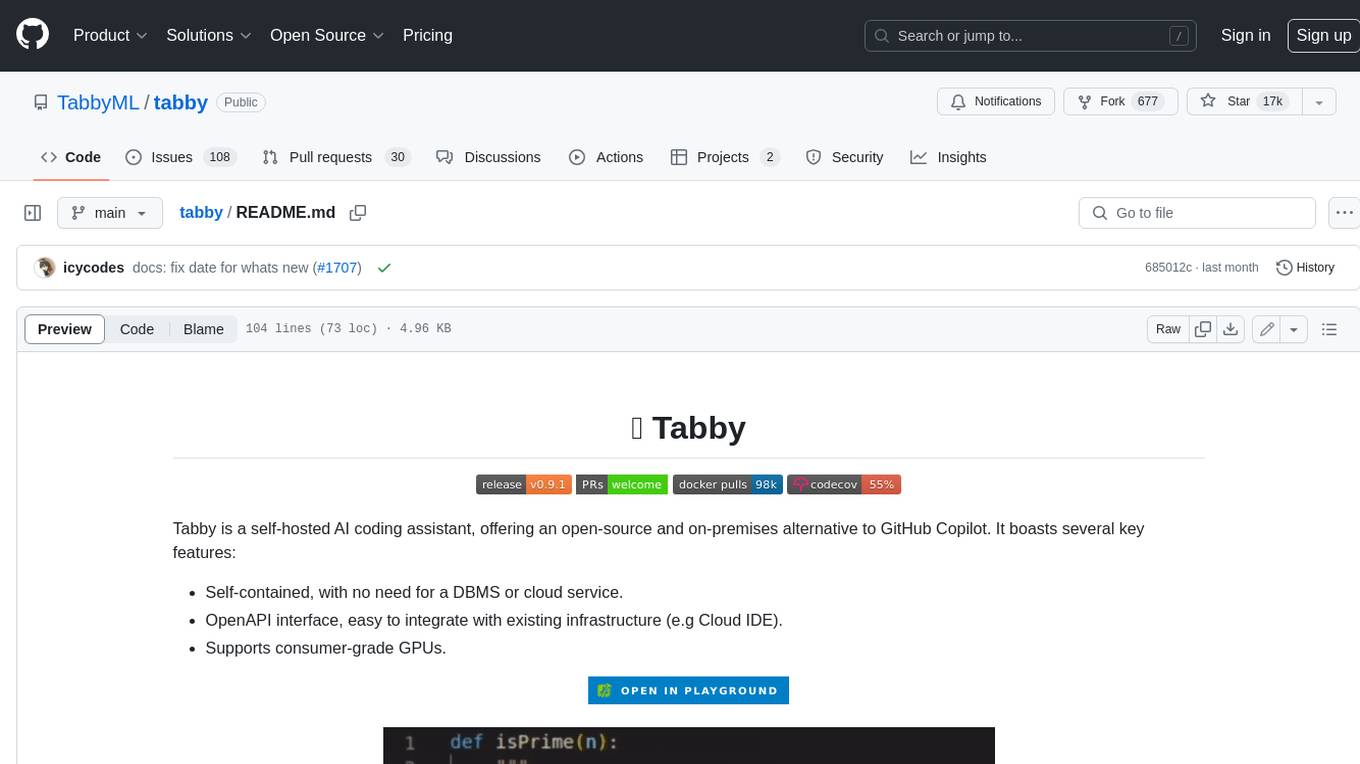
tabby
Tabby is a self-hosted AI coding assistant, offering an open-source and on-premises alternative to GitHub Copilot. It boasts several key features: * Self-contained, with no need for a DBMS or cloud service. * OpenAPI interface, easy to integrate with existing infrastructure (e.g Cloud IDE). * Supports consumer-grade GPUs.
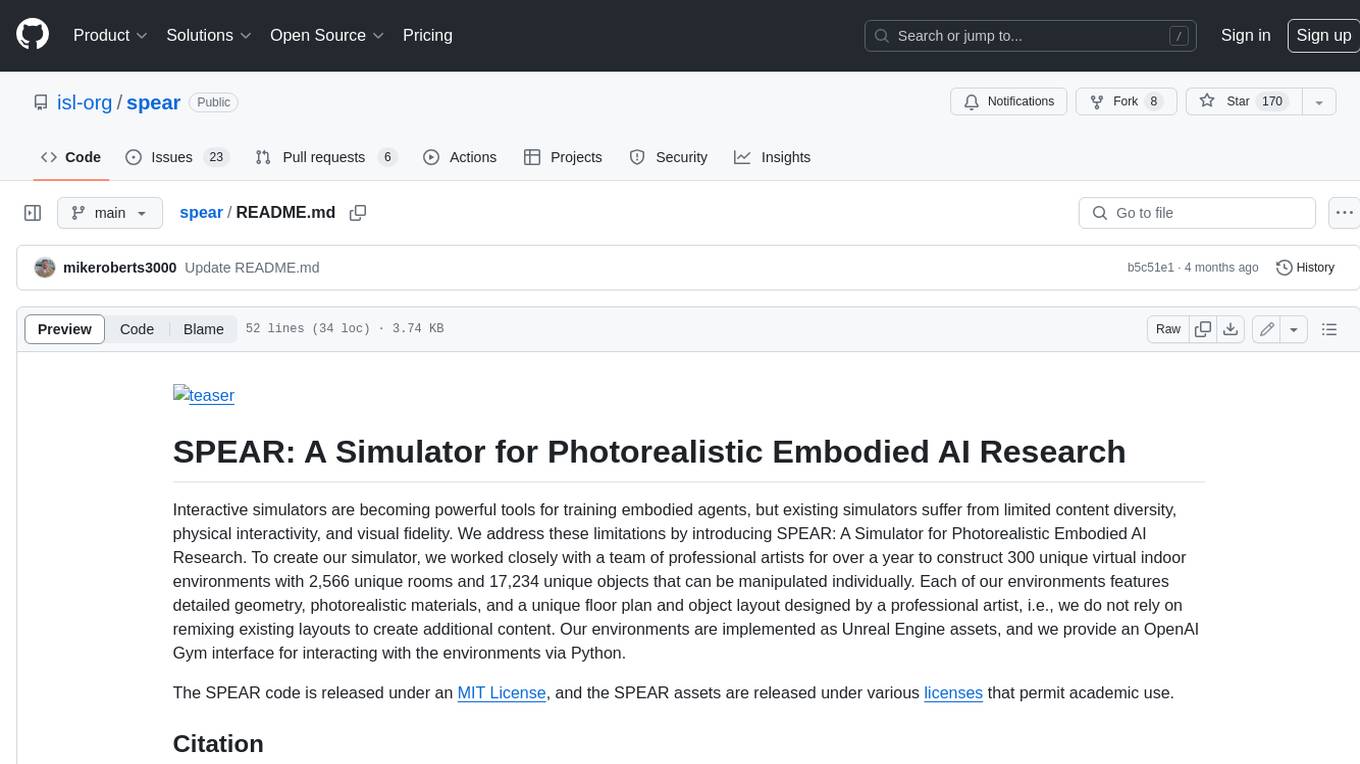
spear
SPEAR (Simulator for Photorealistic Embodied AI Research) is a powerful tool for training embodied agents. It features 300 unique virtual indoor environments with 2,566 unique rooms and 17,234 unique objects that can be manipulated individually. Each environment is designed by a professional artist and features detailed geometry, photorealistic materials, and a unique floor plan and object layout. SPEAR is implemented as Unreal Engine assets and provides an OpenAI Gym interface for interacting with the environments via Python.
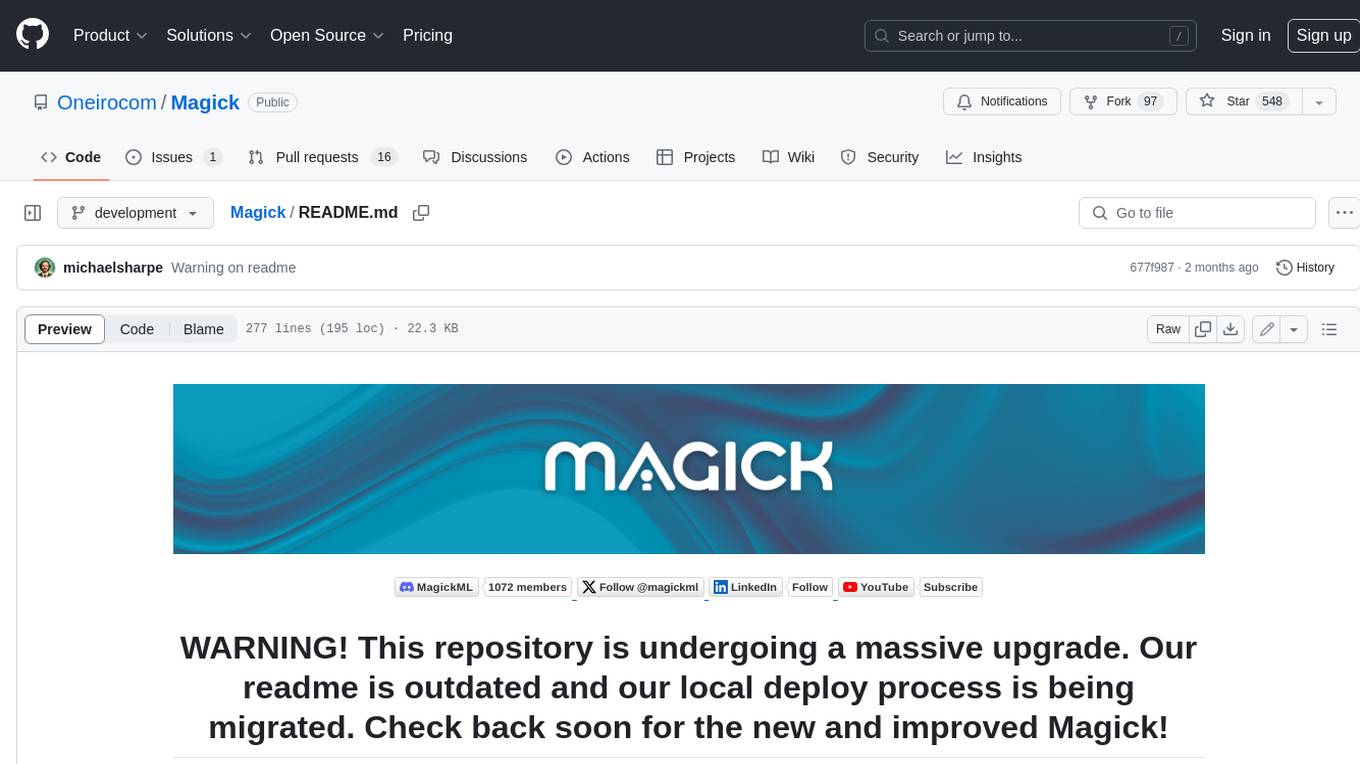
Magick
Magick is a groundbreaking visual AIDE (Artificial Intelligence Development Environment) for no-code data pipelines and multimodal agents. Magick can connect to other services and comes with nodes and templates well-suited for intelligent agents, chatbots, complex reasoning systems and realistic characters.