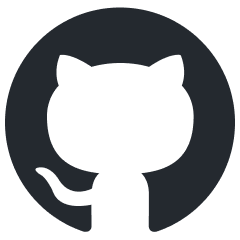
oasis
๐๏ธ OASIS: Open Agent Social Interaction Simulations with One Million Agents. https://oasis.camel-ai.org
Stars: 1097
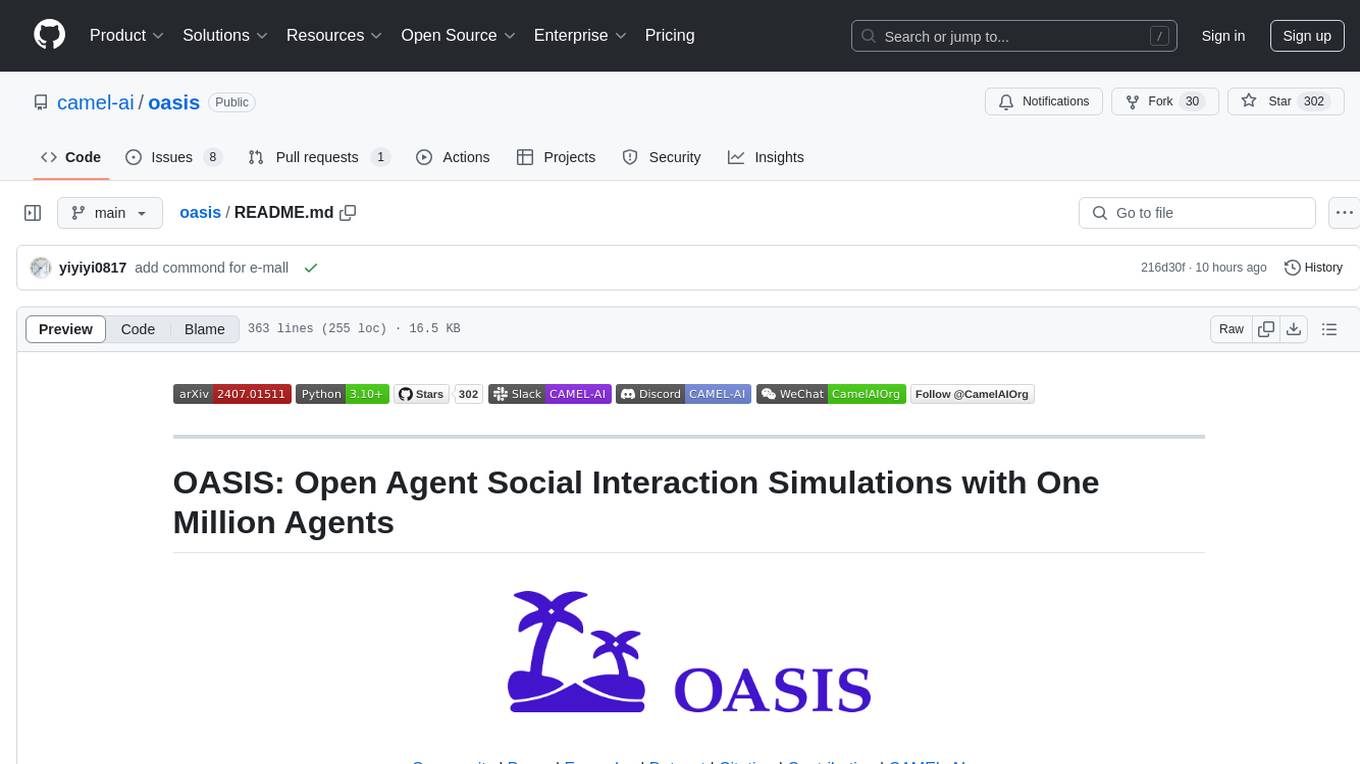
OASIS is a scalable, open-source social media simulator that integrates large language models with rule-based agents to realistically mimic the behavior of up to one million users on platforms like Twitter and Reddit. It facilitates the study of complex social phenomena such as information spread, group polarization, and herd behavior, offering a versatile tool for exploring diverse social dynamics and user interactions in digital environments. With features like scalability, dynamic environments, diverse action spaces, and integrated recommendation systems, OASIS provides a comprehensive platform for simulating social media interactions at a large scale.
README:
Community | Paper | Examples | Dataset | Citation | Contributing | CAMEL-AI
๐๏ธ OASIS is a scalable, open-source social media simulator that integrates large language models with rule-based agents to realistically mimic the behavior of up to one million users on platforms like Twitter and Reddit. It's designed to facilitate the study of complex social phenomena such as information spread, group polarization, and herd behavior, offering a versatile tool for exploring diverse social dynamics and user interactions in digital environments.๐ Scalability: OASIS supports simulations of up to one million agents, enabling studies of social media dynamics at a scale comparable to real-world platforms.
๐ฒ ๏ธDynamic Environments: Adapts to real-time changes in social networks and content, mirroring the fluid dynamics of platforms like Twitter and Reddit for authentic simulation experiences.
๐๐ผ Diverse Action Spaces: Agents can perform 21 actions, such as following, commenting, and reposting, allowing for rich, multi-faceted interactions.
๐ฅ Integrated Recommendation Systems: Features interest-based and hot-score-based recommendation algorithms, simulating how users discover content and interact within social media platforms.
git clone https://github.com/camel-ai/oasis.git
cd oasis
Please choose one of the following methods to set up your environment. You only need to follow one of these methods.
- Option 1: Using Conda (Linux & macOS & windows)
conda create --name oasis python=3.10
conda activate oasis
- Option 2: Using venv (Linux & macOS)
python -m venv oasis-venv
source oasis-venv/bin/activate
- Option 3: Using venv (Windows)
python -m venv oasis-venv
oasis-venv\Scripts\activate
pip install --upgrade pip setuptools
pip install -e . # This will install dependencies as specified in pyproject.toml
First, you need to add your OpenAI API key to the system's environment variables. You can obtain your OpenAI API key from here. Note that the method for doing this will vary depending on your operating system and the shell you are using.
- For Bash shell (Linux, macOS, Git Bash on Windows):**
# Export your OpenAI API key
export OPENAI_API_KEY=<insert your OpenAI API key>
export OPENAI_API_BASE_URL=<insert your OpenAI API BASE URL> #(Should you utilize an OpenAI proxy service, kindly specify this)
- For Windows Command Prompt:**
REM export your OpenAI API key
set OPENAI_API_KEY=<insert your OpenAI API key>
set OPENAI_API_BASE_URL=<insert your OpenAI API BASE URL> #(Should you utilize an OpenAI proxy service, kindly specify this)
- For Windows PowerShell:**
# Export your OpenAI API key
$env:OPENAI_API_KEY="<insert your OpenAI API key>"
$env:OPENAI_API_BASE_URL="<insert your OpenAI API BASE URL>" #(Should you utilize an OpenAI proxy service, kindly specify this)
Replace <insert your OpenAI API key>
with your actual OpenAI API key in each case. Make sure there are no spaces around the =
sign.
If adjustments to the settings are necessary, you can specify the parameters in the scripts/reddit_gpt_example/gpt_example.yaml
file. Explanations for each parameter are provided in the comments within the YAML file.
To import your own user and post data, please refer to the JSON file format located in the /data/reddit/
directory of this repository. Then, update the user_path
and pair_path
in the YAML file to point to your data files.
# For Reddit
python scripts/reddit_gpt_example/reddit_simulation_gpt.py --config_path scripts/reddit_gpt_example/gpt_example.yaml
# For Reddit with Electronic Mall
python scripts/reddit_emall_demo/emall_simulation.py --config_path scripts/reddit_emall_demo/emall.yaml
# For Twitter
python scripts/twitter_gpt_example/twitter_simulation_large.py --config_path scripts/twitter_gpt_example/gpt_example.yaml
Note: without modifying the Configuration File, running the Reddit script requires only 36 agents operating at an activation probability of 0.1 for 2 time steps, the entire process approximately requires 7.2 agent inferences, and approximately 14 API requests to call GPT-4. The Twitter script has about 111 agents operating at an activation probability of roughly 0.1 for 3 time steps, i.e., 33.3 agent inferences, using GPT-3.5-turbo. I hope this is a cost you can bear. For running larger scale agent simulations, it is recommended to read the next section on experimenting with open-source models.
We assume that users are conducting large-scale experiments on a Slurm workload manager cluster. Below, we provide the commands for running experiments with open-source models on the Slurm cluster. The steps for running these experiments on a local machine are similar.
Taking the download of LLaMA-3 from Hugging Face as an example:
pip install huggingface_hub
huggingface-cli download --resume-download "meta-llama/Meta-Llama-3-8B-Instruct" --local-dir "YOUR_LOCAL_MODEL_DIRECTORY" --local-dir-use-symlinks False --resume-download --token "YOUR_HUGGING_FACE_TOKEN"
Note: Please replace "YOUR_LOCAL_MODEL_DIRECTORY" with your actual directory path where you wish to save the model and "YOUR_HUGGING_FACE_TOKEN" with your Hugging Face token. Obtain your token at https://huggingface.co/settings/tokens.
Ensure that the GPU memory you're requesting is sufficient for deploying the open-source model you've downloaded. Taking the application for an A100 GPU as an example:
salloc --ntasks=1 --mem=100G --time=11:00:00 --gres=gpu:a100:1
Next, obtain and record the information of that node. Please ensure that the IP address of the GPU server can be accessed by your network, such as within the school's internet.
srun --ntasks=1 --mem=100G --time=11:00:00 --gres=gpu:a100:1 bash -c 'ifconfig -a'
"""
Example output:
eth0: flags=4163<UP,BROADCAST,RUNNING,MULTICAST> mtu 1500
inet 10.109.1.8 netmask 255.255.255.0 broadcast 192.168.1.255
ether 02:42:ac:11:00:02 txqueuelen 0 (Ethernet)
RX packets 100 bytes 123456 (123.4 KB)
RX errors 0 dropped 0 overruns 0 frame 0
TX packets 100 bytes 654321 (654.3 KB)
TX errors 0 dropped 0 overruns 0 carrier 0 collisions
"""
srun --ntasks=1 --mem=100G --time=11:00:00 --gres=gpu:a100:1 bash -c 'echo $CUDA_VISIBLE_DEVICES'
"""
Example output: 0
"""
Document the IP address associated with the eth0 interface, which, in this example, is 10.109.1.8
. Additionally, note the identifier of the available GPU, which in this case is 0
.
Based on the IP address and GPU identifier obtained from step 2, and the model path and name from step 1, modify the hosts
, gpus
variables, and the 'YOUR_LOCAL_MODEL_DIRECTORY'
, 'YOUR_LOCAL_MODEL_NAME strings'
in the deploy.py
file. For example:
if __name__ == "__main__":
host = "10.109.1.8" # input your IP address
ports = [
[8002, 8003, 8005],
[8006, 8007, 8008],
[8011, 8009, 8010],
[8014, 8012, 8013],
[8017, 8015, 8016],
[8020, 8018, 8019],
[8021, 8022, 8023],
[8024, 8025, 8026],
]
gpus = [0] # input your $CUDA_VISIBLE_DEVICES
all_ports = [port for i in gpus for port in ports[i]]
print("All ports: ", all_ports, '\n\n')
t = None
for i in range(3):
for j, gpu in enumerate(gpus):
cmd = (
f"CUDA_VISIBLE_DEVICES={gpu} python -m "
f"vllm.entrypoints.openai.api_server --model "
f"'YOUR_LOCAL_MODEL_DIRECTORY' " # input the path where you downloaded your model
f"--served-model-name 'YOUR_LOCAL_MODEL_NAME' " # input the name of the model you downloaded
f"--host {host} --port {ports[j][i]} --gpu-memory-utilization "
f"0.3 --disable-log-stats")
t = threading.Thread(target=subprocess.run,
args=(cmd, ),
kwargs={"shell": True},
daemon=True)
t.start()
check_port_open(host, ports[0][i])
t.join()
Next, run the deploy.py
script. Then you will see an output, which contains a list of all ports.
srun --ntasks=1 --time=11:00:00 --gres=gpu:a100:1 bash -c 'python deploy.py'
"""
Example output:
All ports: [8002, 8003, 8005]
More other output about vllm...
"""
Before the simulation begins, you need to enter your model name, model path, host, and ports into the corresponding yaml file in the experiment script such as scripts\reddit_simulation_align_with_human\business_3600.yaml
. An example of what to write is:
inference:
model_type: 'YOUR_LOCAL_MODEL_NAME' # input the name of the model you downloaded (eg. 'llama-3')
model_path: 'YOUR_LOCAL_MODEL_DIRECTORY' # input the path where you downloaded your model
stop_tokens: ["<|eot_id|>", "<|end_of_text|>"]
server_url:
- host: "10.109.1.8"
ports: [8002, 8003, 8005] # Input the list of all ports obtained in step 3
Additionally, you can modify other settings related to data and experimental details in the yaml file. For instructions on this part, refer to scripts\reddit_gpt_example\gpt_example.yaml
.
You need to open a new terminal and then run:
# For Reddit
# Align with human
python scripts/reddit_simulation_align_with_human/reddit_simulation_align_with_human.py --config_path scripts/reddit_simulation_align_with_human/business_3600.yaml
# Agent's reaction to counterfactual content
python scripts/reddit_simulation_counterfactual/reddit_simulation_counterfactual.py --config_path scripts/reddit_simulation_counterfactual/control_100.yaml
# For Twitter(X)
# Information spreading
# one case in align_with_real_world, The โuser_charโ field in the dataset we have open-sourced has been replaced with โdescriptionโ to ensure privacy protection.
python scripts/twitter_simulation/twitter_simulation_large.py --config_path scripts/twitter_simulation/align_with_real_world/yaml_200/sub1/False_Business_0.yaml
# Group Polarization
python scripts/twitter_simulation/group_polarization/twitter_simulation_group_polar.py --config_path scripts/twitter_simulation/group_polarization/group_polarization.yaml
# For One Million Simulation
python scripts/twitter_simulation_1M_agents/twitter_simulation_1m.py --config_path scripts/twitter_simulation_1M_agents/twitter_1m.yaml
- Customizing temporal feature
When simulating on generated users, you can customize the temporal feature in social_simulation/social_agent/agents_generator.py
by modifying profile['other_info']['active_threshold']
. For example, you can set it to all 1 if you believe that the generated users should be active the entire time.
- Reddit recommendation system
The Reddit recommendation system is highly time-sensitive. Currently, one time step in the reddit_simulation_xxx.py
simulates approximately two hours in the agent world, so essentially, new posts are recommended at every time step. To ensure that all posts made by controllable users can be seen by other agents, it is recommended that the number of agents
ร activate_prob
> max_rec_post_len
> round_post_num
.
To discover how to create profiles for large-scale users, as well as how to visualize and analyze social simulation data once your experiment concludes, please refer to More Tutorials for detailed guidance.
๐ฃ Support OPENAI Embedding model for Twhin-Bert Recommendation System. - ๐ March 25, 2025
- Updated social media links and QR codes in the README! Join OASIS & CAMEL on WeChat, X, Reddit, and Discord. - ๐ March 24, 2025
- Add multi-threading support to speed up LLM inference by 13x - ๐ March 4, 2025
- Slightly refactoring the database to add Quote Action and modify Repost Action - ๐ January 13, 2025
- Added the demo video and oasis's star history in the README - ๐ January 5, 2025
- Introduced an Electronic Mall on the Reddit platform - ๐ December 5, 2024
- OASIS initially released on arXiv - ๐ November 19, 2024
- OASIS GitHub repository initially launched - ๐ November 19, 2024
@misc{yang2024oasisopenagentsocial,
title={OASIS: Open Agent Social Interaction Simulations with One Million Agents},
author={Ziyi Yang and Zaibin Zhang and Zirui Zheng and Yuxian Jiang and Ziyue Gan and Zhiyu Wang and Zijian Ling and Jinsong Chen and Martz Ma and Bowen Dong and Prateek Gupta and Shuyue Hu and Zhenfei Yin and Guohao Li and Xu Jia and Lijun Wang and Bernard Ghanem and Huchuan Lu and Chaochao Lu and Wanli Ouyang and Yu Qiao and Philip Torr and Jing Shao},
year={2024},
eprint={2411.11581},
archivePrefix={arXiv},
primaryClass={cs.CL},
url={https://arxiv.org/abs/2411.11581},
}
We would like to thank Douglas for designing the logo of our project.
The source code is licensed under Apache 2.0.
We greatly appreciate your interest in contributing to our open-source initiative. To ensure a smooth collaboration and the success of contributions, we adhere to a set of contributing guidelines similar to those established by CAMEL. For a comprehensive understanding of the steps involved in contributing to our project, please refer to the CAMEL contributing guidelines here. ๐ค๐
An essential part of contributing involves not only submitting new features with accompanying tests (and, ideally, examples) but also ensuring that these contributions pass our automated pytest suite. This approach helps us maintain the project's quality and reliability by verifying compatibility and functionality.
If you're keen on exploring new research opportunities or discoveries with our platform and wish to dive deeper or suggest new features, we're here to talk. Feel free to get in touch for more details at [email protected].
For Tasks:
Click tags to check more tools for each tasksFor Jobs:
Alternative AI tools for oasis
Similar Open Source Tools
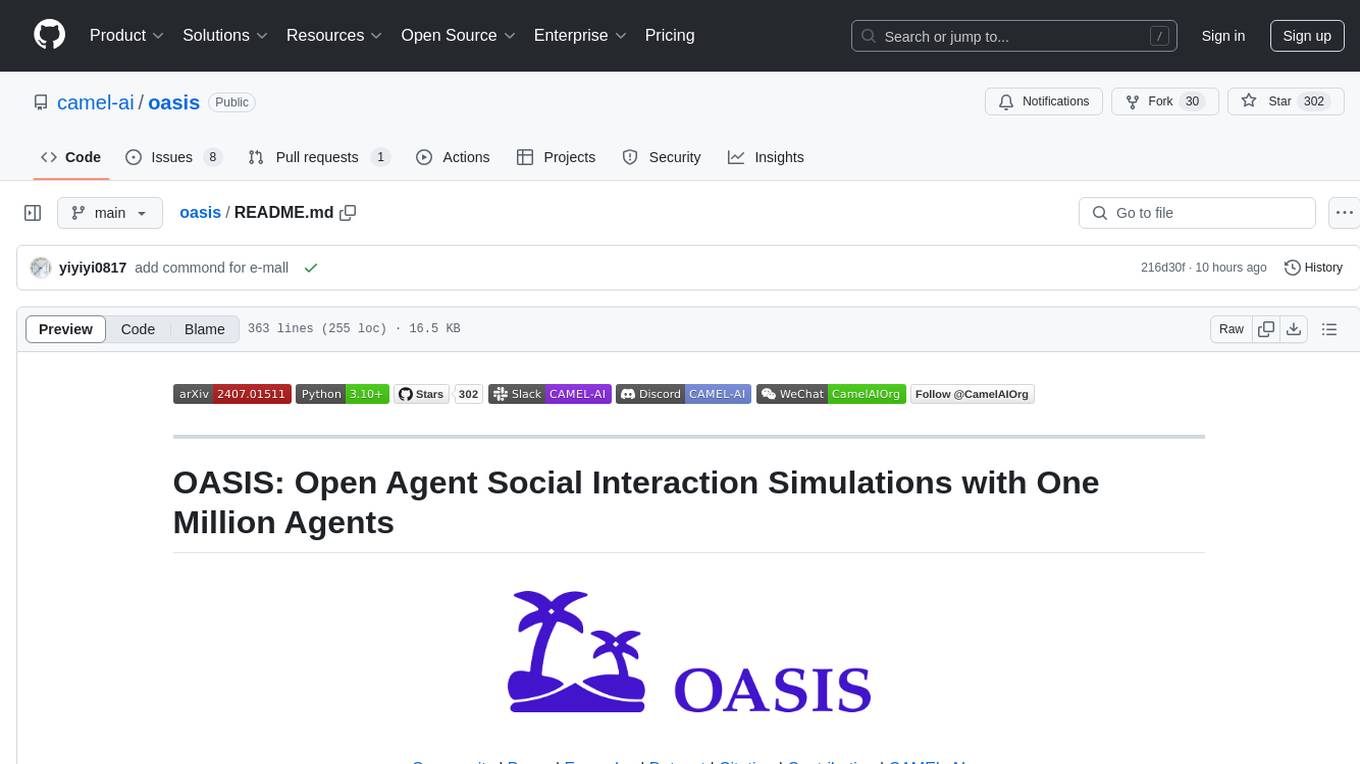
oasis
OASIS is a scalable, open-source social media simulator that integrates large language models with rule-based agents to realistically mimic the behavior of up to one million users on platforms like Twitter and Reddit. It facilitates the study of complex social phenomena such as information spread, group polarization, and herd behavior, offering a versatile tool for exploring diverse social dynamics and user interactions in digital environments. With features like scalability, dynamic environments, diverse action spaces, and integrated recommendation systems, OASIS provides a comprehensive platform for simulating social media interactions at a large scale.
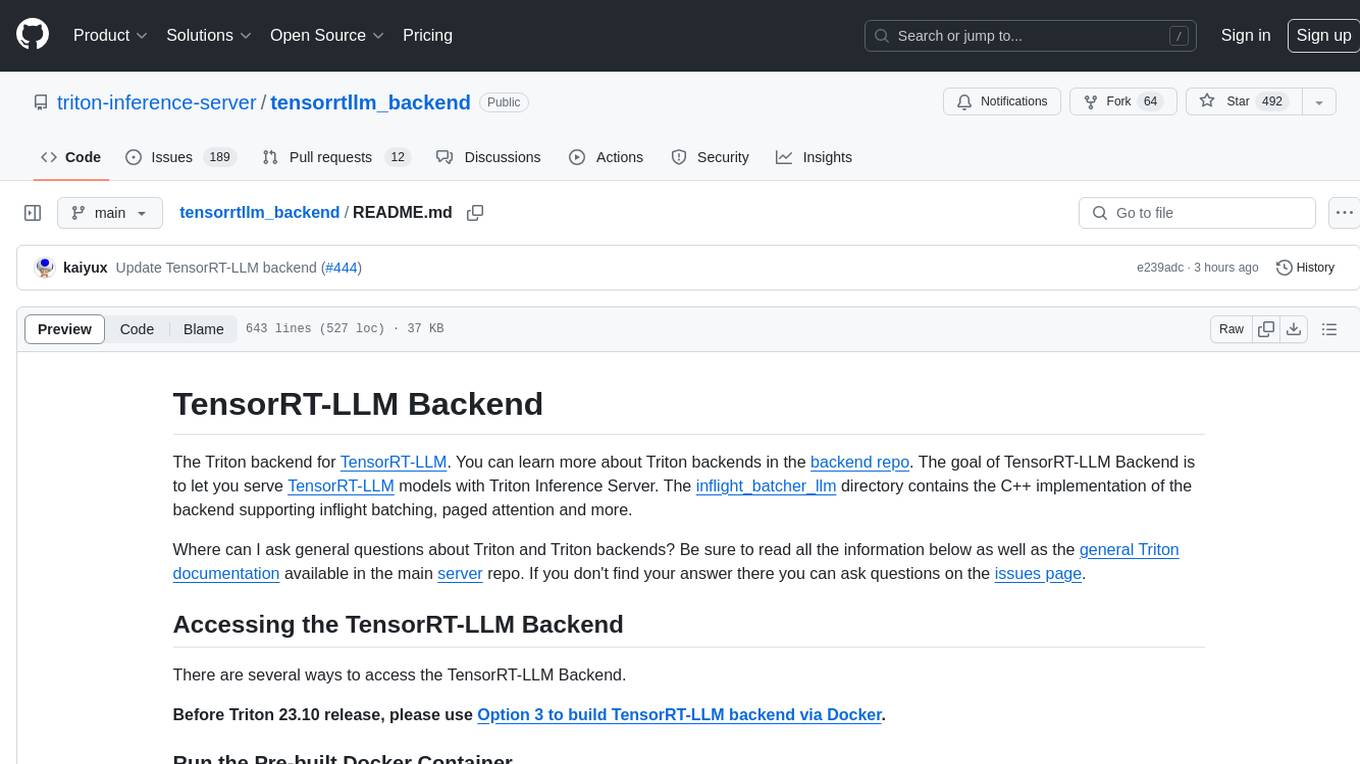
tensorrtllm_backend
The TensorRT-LLM Backend is a Triton backend designed to serve TensorRT-LLM models with Triton Inference Server. It supports features like inflight batching, paged attention, and more. Users can access the backend through pre-built Docker containers or build it using scripts provided in the repository. The backend can be used to create models for tasks like tokenizing, inferencing, de-tokenizing, ensemble modeling, and more. Users can interact with the backend using provided client scripts and query the server for metrics related to request handling, memory usage, KV cache blocks, and more. Testing for the backend can be done following the instructions in the 'ci/README.md' file.
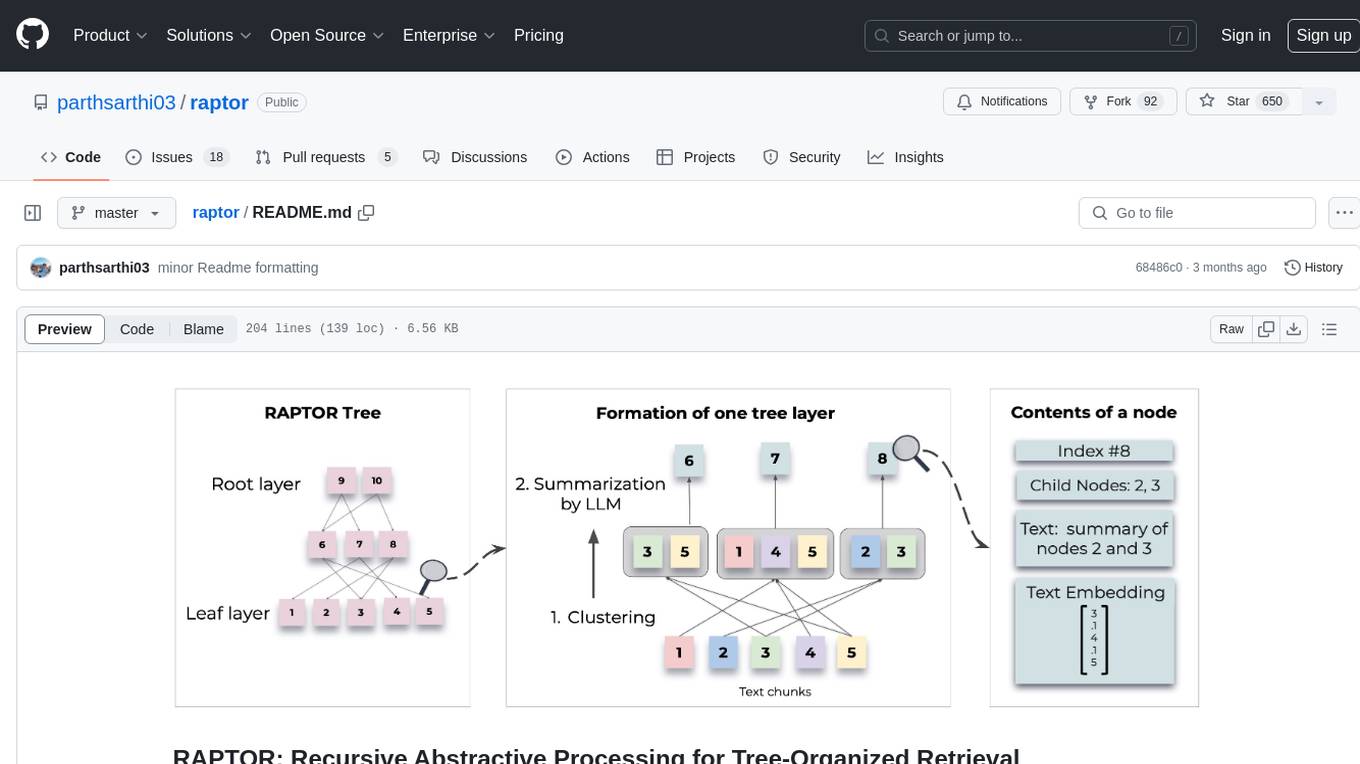
raptor
RAPTOR introduces a novel approach to retrieval-augmented language models by constructing a recursive tree structure from documents. This allows for more efficient and context-aware information retrieval across large texts, addressing common limitations in traditional language models. Users can add documents to the tree, answer questions based on indexed documents, save and load the tree, and extend RAPTOR with custom summarization, question-answering, and embedding models. The tool is designed to be flexible and customizable for various NLP tasks.
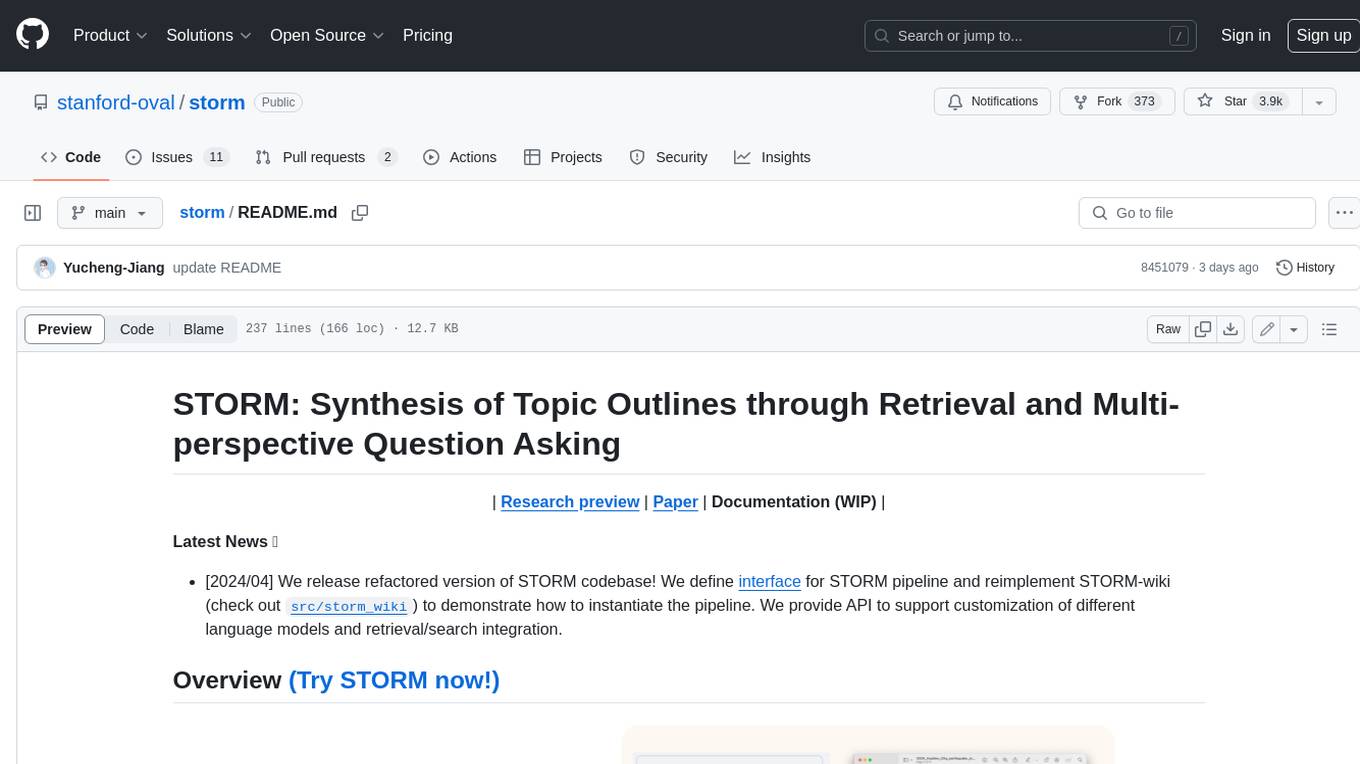
storm
STORM is a LLM system that writes Wikipedia-like articles from scratch based on Internet search. While the system cannot produce publication-ready articles that often require a significant number of edits, experienced Wikipedia editors have found it helpful in their pre-writing stage. **Try out our [live research preview](https://storm.genie.stanford.edu/) to see how STORM can help your knowledge exploration journey and please provide feedback to help us improve the system ๐!**
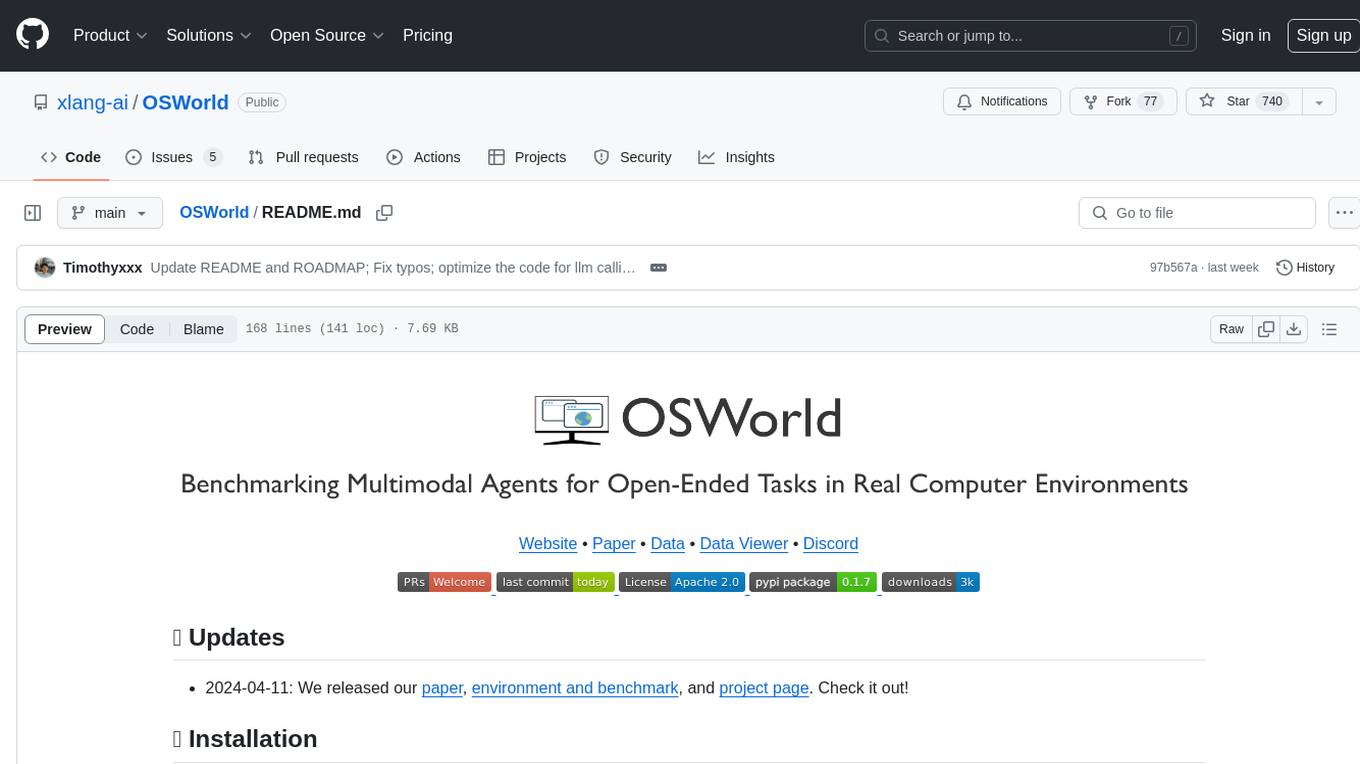
OSWorld
OSWorld is a benchmarking tool designed to evaluate multimodal agents for open-ended tasks in real computer environments. It provides a platform for running experiments, setting up virtual machines, and interacting with the environment using Python scripts. Users can install the tool on their desktop or server, manage dependencies with Conda, and run benchmark tasks. The tool supports actions like executing commands, checking for specific results, and evaluating agent performance. OSWorld aims to facilitate research in AI by providing a standardized environment for testing and comparing different agent baselines.
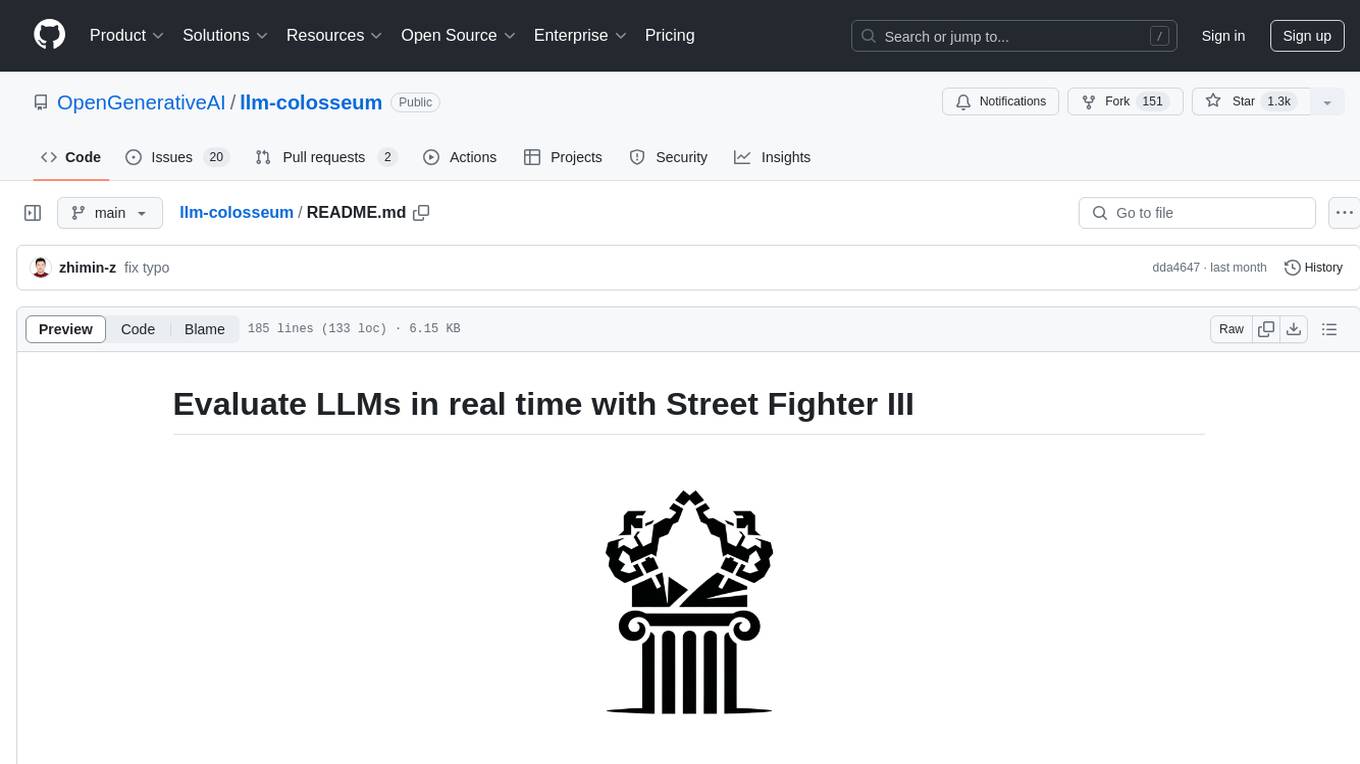
llm-colosseum
llm-colosseum is a tool designed to evaluate Language Model Models (LLMs) in real-time by making them fight each other in Street Fighter III. The tool assesses LLMs based on speed, strategic thinking, adaptability, out-of-the-box thinking, and resilience. It provides a benchmark for LLMs to understand their environment and take context-based actions. Users can analyze the performance of different LLMs through ELO rankings and win rate matrices. The tool allows users to run experiments, test different LLM models, and customize prompts for LLM interactions. It offers installation instructions, test mode options, logging configurations, and the ability to run the tool with local models. Users can also contribute their own LLM models for evaluation and ranking.
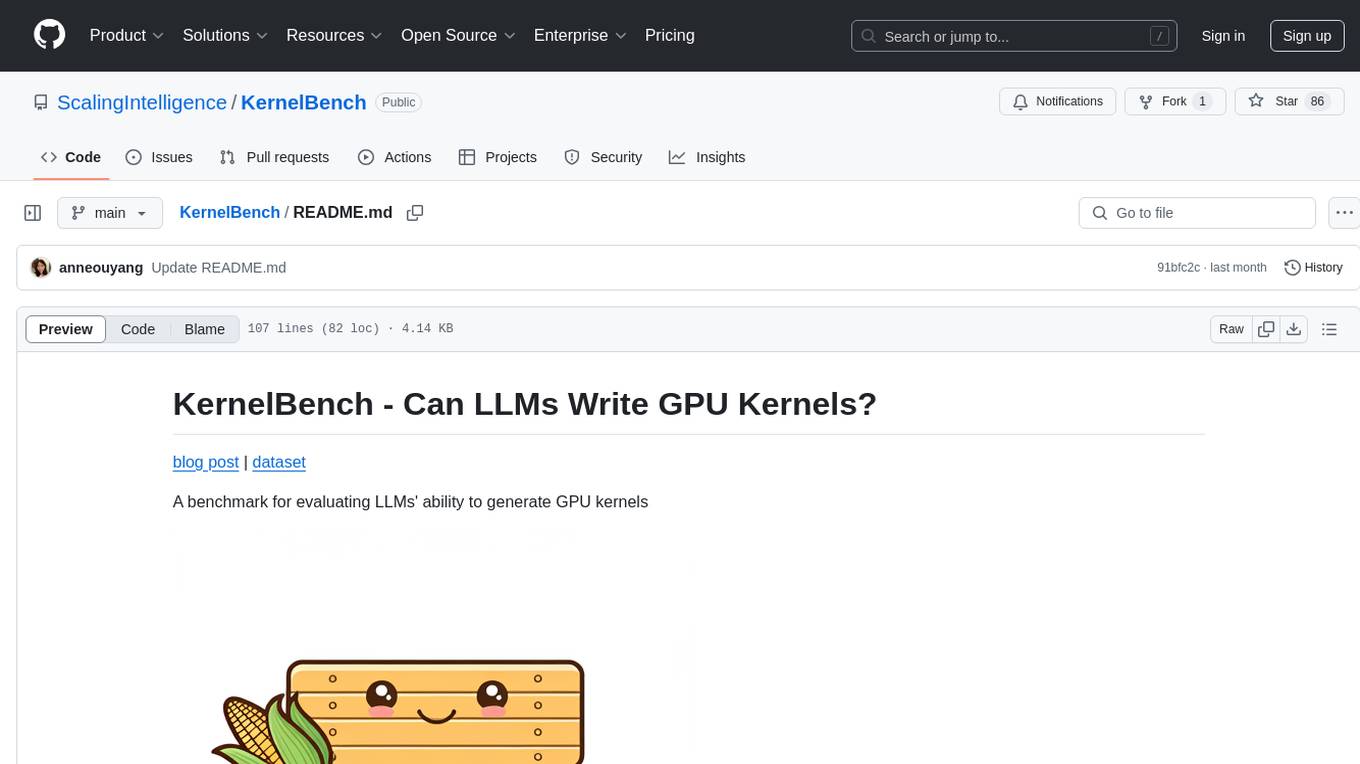
KernelBench
KernelBench is a benchmark tool designed to evaluate Large Language Models' (LLMs) ability to generate GPU kernels. It focuses on transpiling operators from PyTorch to CUDA kernels at different levels of granularity. The tool categorizes problems into four levels, ranging from single-kernel operators to full model architectures, and assesses solutions based on compilation, correctness, and speed. The repository provides a structured directory layout, setup instructions, usage examples for running single or multiple problems, and upcoming roadmap features like additional GPU platform support and integration with other frameworks.
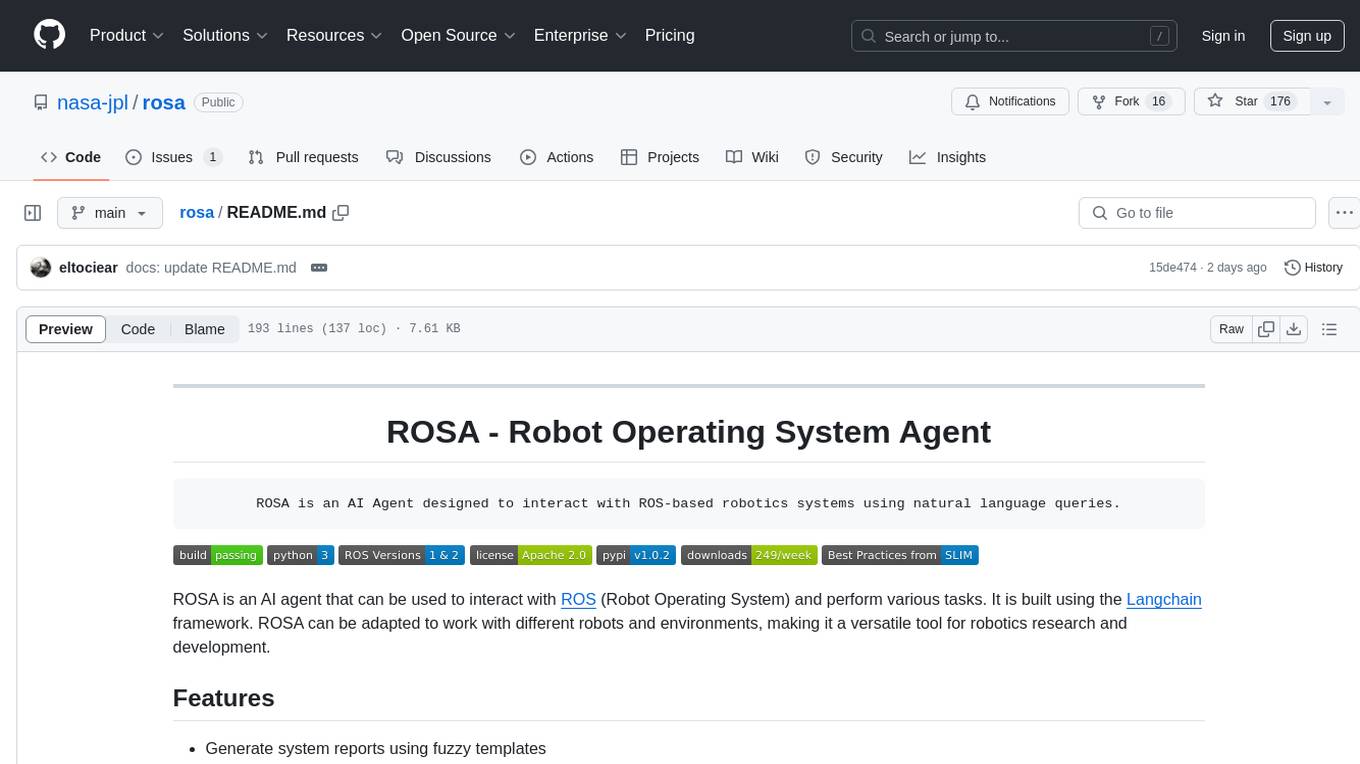
rosa
ROSA is an AI Agent designed to interact with ROS-based robotics systems using natural language queries. It can generate system reports, read and parse ROS log files, adapt to new robots, and run various ROS commands using natural language. The tool is versatile for robotics research and development, providing an easy way to interact with robots and the ROS environment.
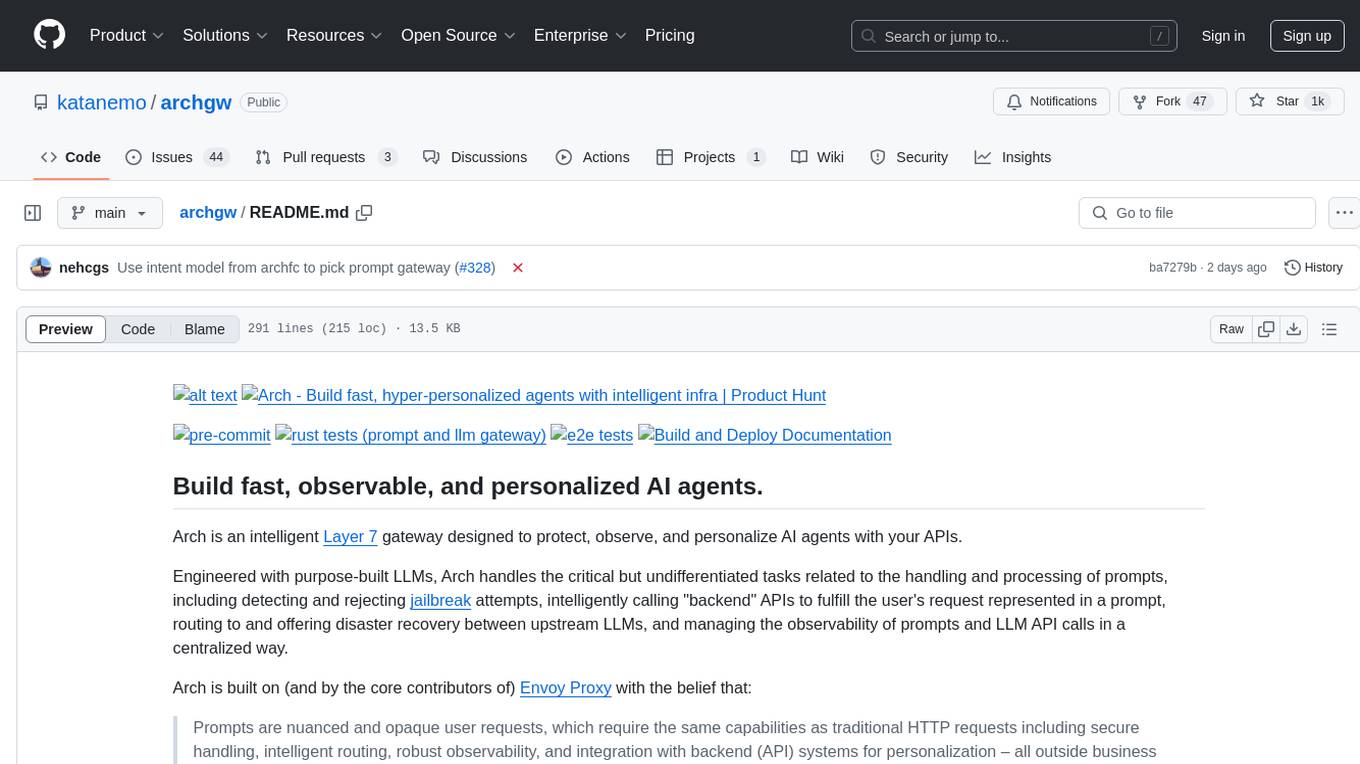
archgw
Arch is an intelligent Layer 7 gateway designed to protect, observe, and personalize AI agents with APIs. It handles tasks related to prompts, including detecting jailbreak attempts, calling backend APIs, routing between LLMs, and managing observability. Built on Envoy Proxy, it offers features like function calling, prompt guardrails, traffic management, and observability. Users can build fast, observable, and personalized AI agents using Arch to improve speed, security, and personalization of GenAI apps.
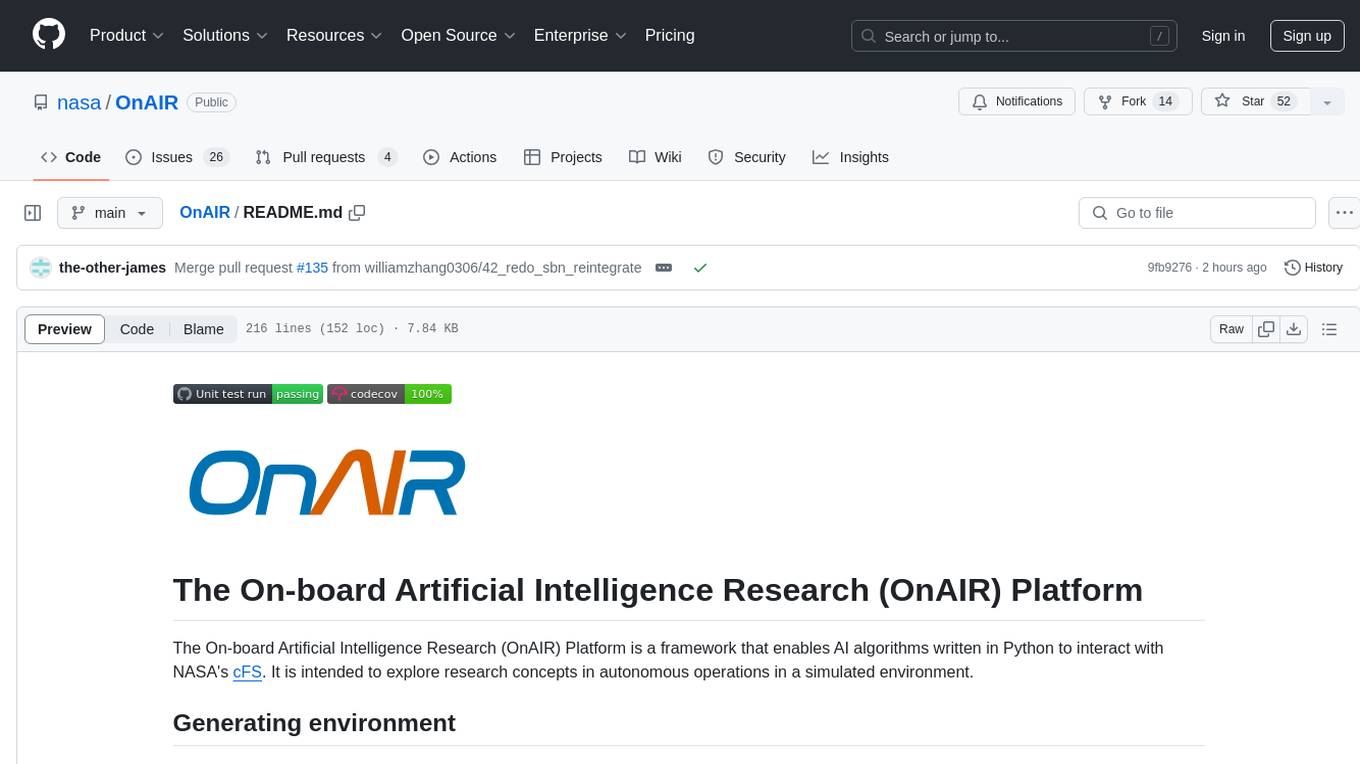
OnAIR
The On-board Artificial Intelligence Research (OnAIR) Platform is a framework that enables AI algorithms written in Python to interact with NASA's cFS. It is intended to explore research concepts in autonomous operations in a simulated environment. The platform provides tools for generating environments, handling telemetry data through Redis, running unit tests, and contributing to the repository. Users can set up a conda environment, configure telemetry and Redis examples, run simulations, and conduct unit tests to ensure the functionality of their AI algorithms. The platform also includes guidelines for licensing, copyright, and contributions to the repository.
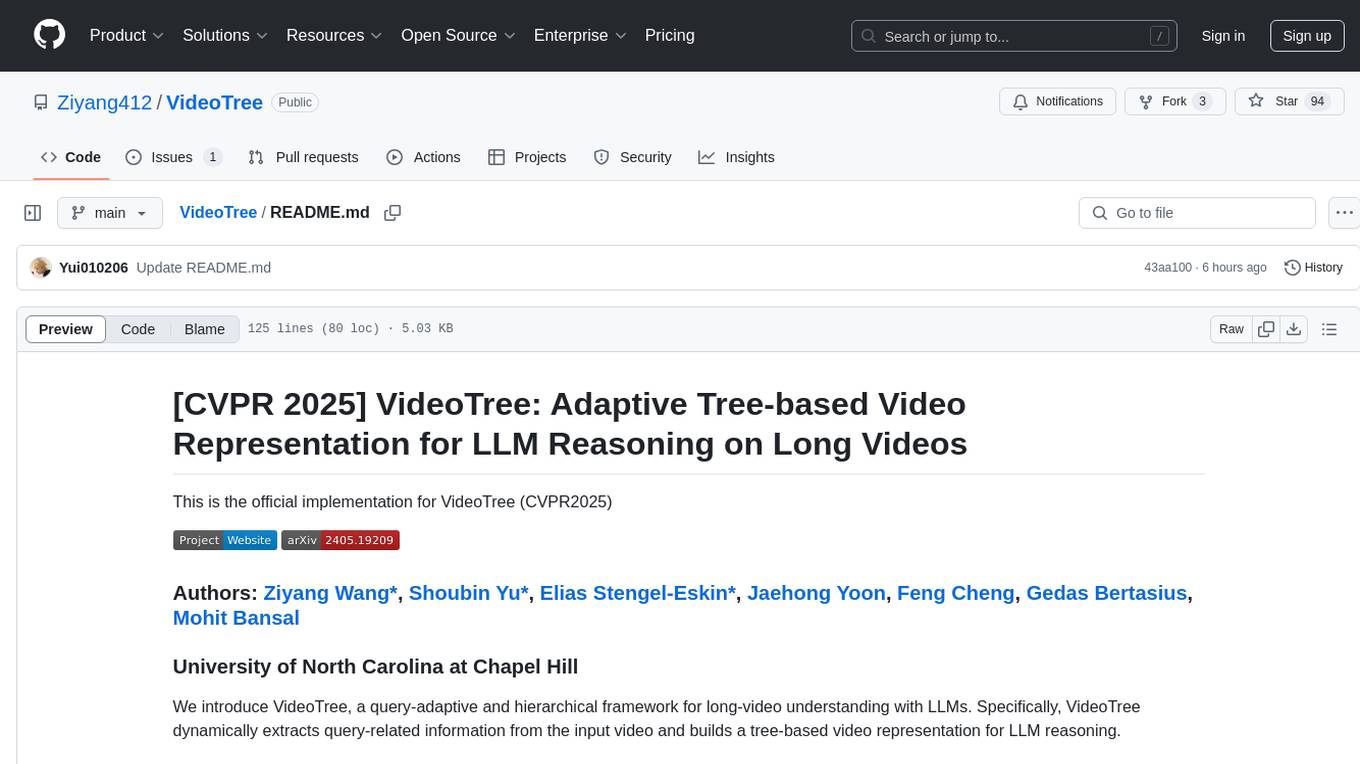
VideoTree
VideoTree is an official implementation for a query-adaptive and hierarchical framework for understanding long videos with LLMs. It dynamically extracts query-related information from input videos and builds a tree-based video representation for LLM reasoning. The tool requires Python 3.8 or above and leverages models like LaViLa and EVA-CLIP-8B for feature extraction. It also provides scripts for tasks like Adaptive Breath Expansion, Relevance-based Depth Expansion, and LLM Reasoning. The codebase is being updated to incorporate scripts/captions for NeXT-QA and IntentQA in the future.
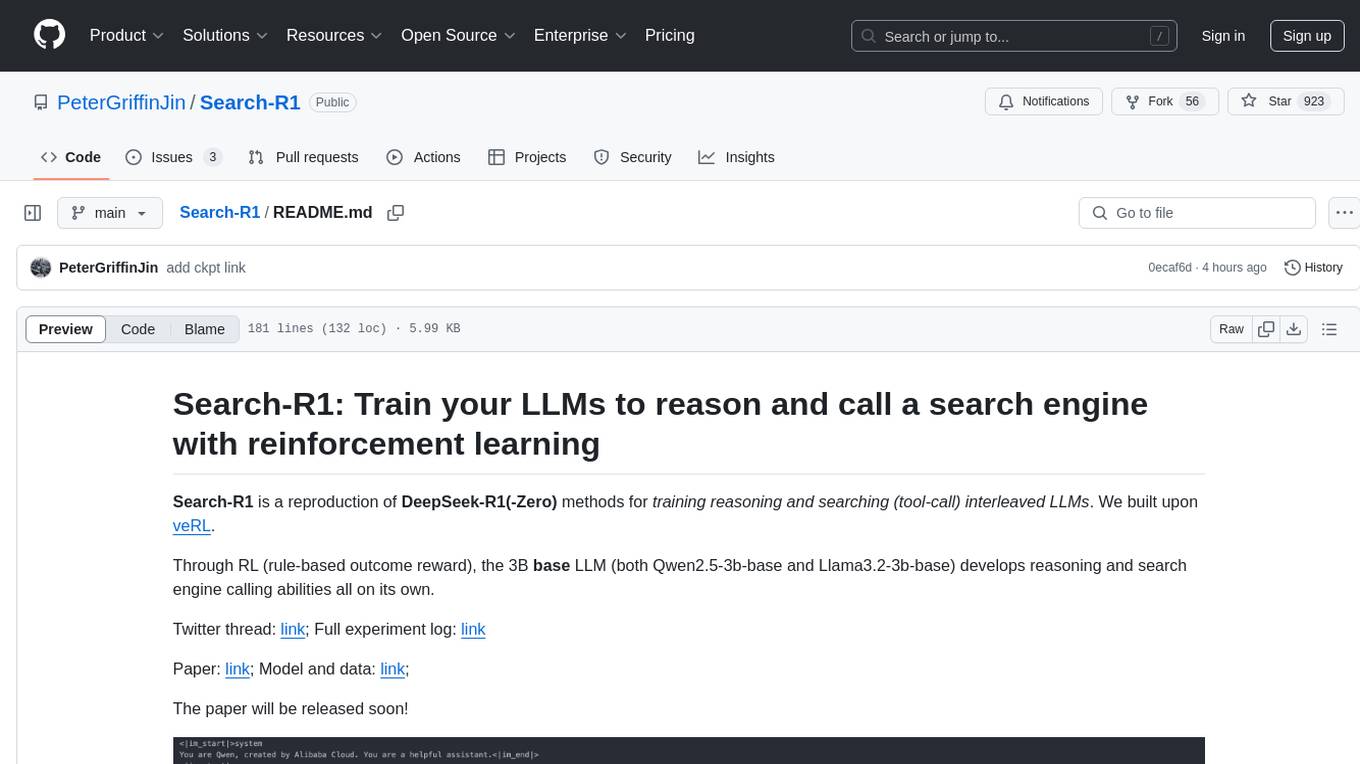
Search-R1
Search-R1 is a tool that trains large language models (LLMs) to reason and call a search engine using reinforcement learning. It is a reproduction of DeepSeek-R1 methods for training reasoning and searching interleaved LLMs, built upon veRL. Through rule-based outcome reward, the base LLM develops reasoning and search engine calling abilities independently. Users can train LLMs on their own datasets and search engines, with preliminary results showing improved performance in search engine calling and reasoning tasks.
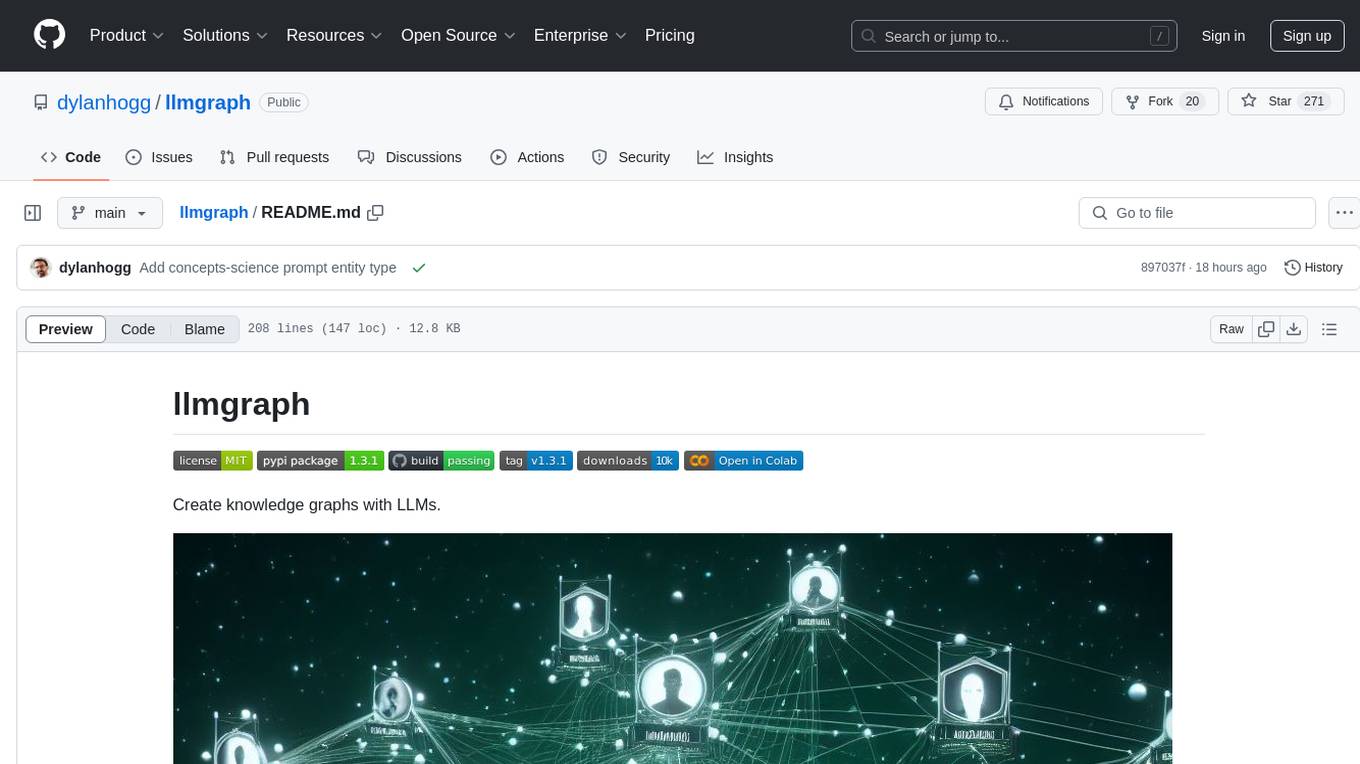
llmgraph
llmgraph is a tool that enables users to create knowledge graphs in GraphML, GEXF, and HTML formats by extracting world knowledge from large language models (LLMs) like ChatGPT. It supports various entity types and relationships, offers cache support for efficient graph growth, and provides insights into LLM costs. Users can customize the model used and interact with different LLM providers. The tool allows users to generate interactive graphs based on a specified entity type and Wikipedia link, making it a valuable resource for knowledge graph creation and exploration.
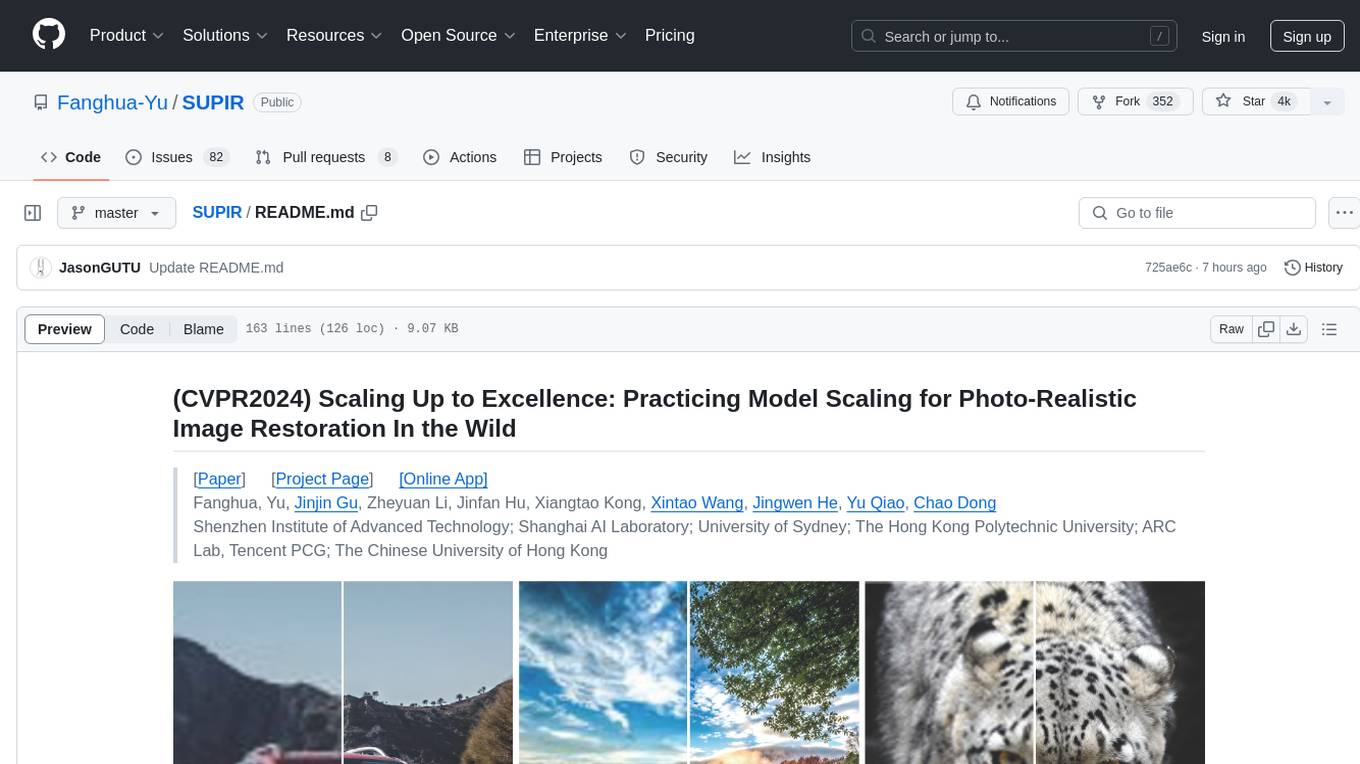
SUPIR
SUPIR is an AI-based image processing and upscaling tool that leverages cutting-edge technology to enhance image quality and resolution. The tool provides users with the ability to upscale images with high generalization and quality, as well as specific settings for light degradation scenarios. It offers a range of models and checkpoints for different use cases, along with detailed instructions for installation and usage. SUPIR also includes features for color fixing, linear CFG adjustments, and various prompts for image enhancement. The tool is designed for non-commercial use only and comes with a contact email for inquiries and permission requests for commercial use.
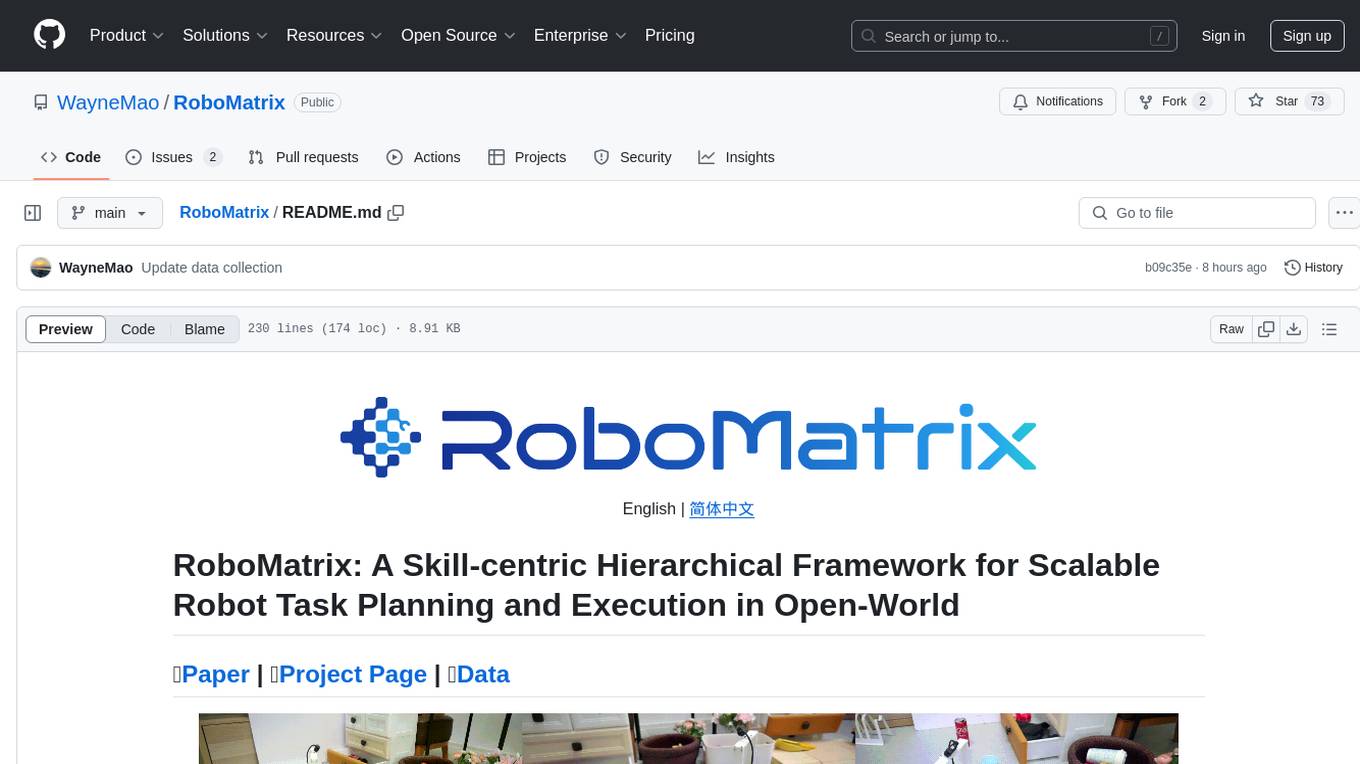
RoboMatrix
RoboMatrix is a skill-centric hierarchical framework for scalable robot task planning and execution in an open-world environment. It provides a structured approach to robot task execution using a combination of hardware components, environment configuration, installation procedures, and data collection methods. The framework is developed using the ROS2 framework on Ubuntu and supports robots from DJI's RoboMaster series. Users can follow the provided installation guidance to set up RoboMatrix and utilize it for various tasks such as data collection, task execution, and dataset construction. The framework also includes a supervised fine-tuning dataset and aims to optimize communication and release additional components in the future.
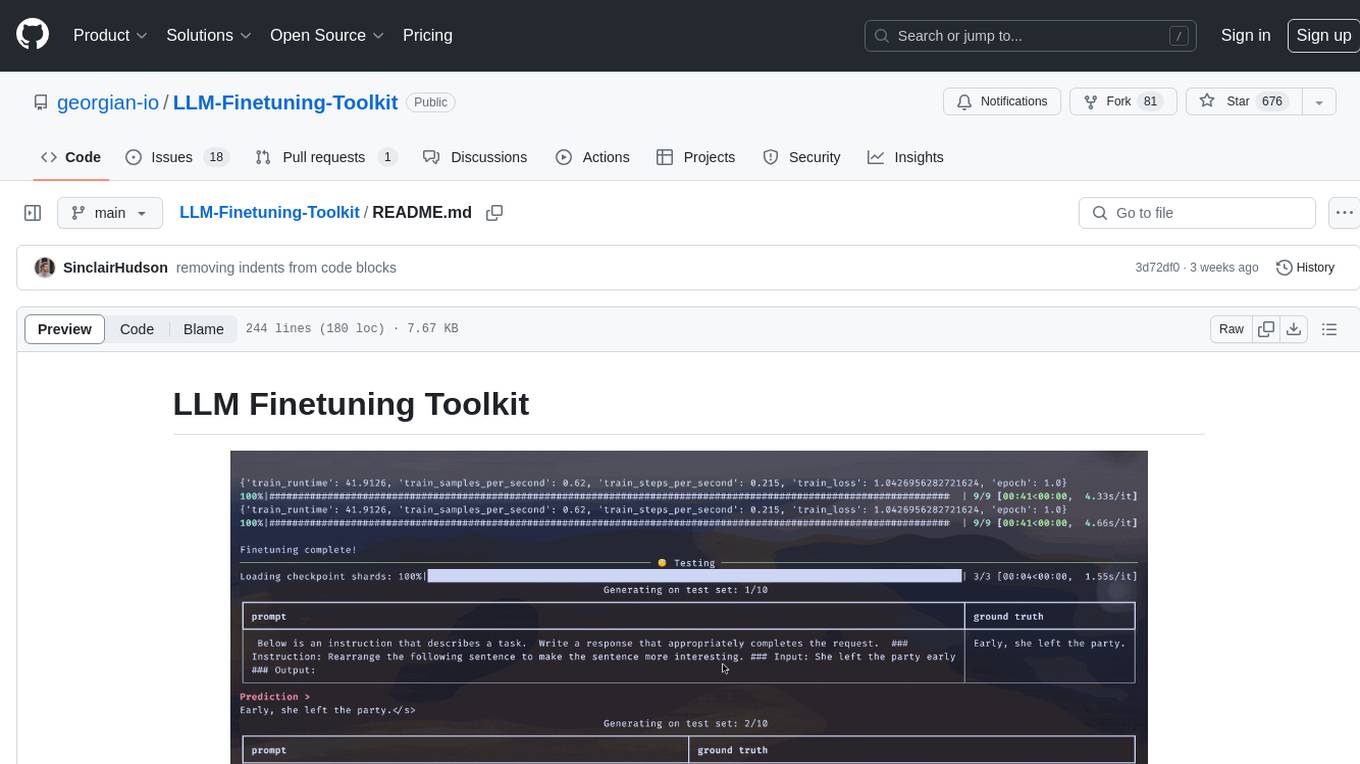
LLM-Finetuning-Toolkit
LLM Finetuning toolkit is a config-based CLI tool for launching a series of LLM fine-tuning experiments on your data and gathering their results. It allows users to control all elements of a typical experimentation pipeline - prompts, open-source LLMs, optimization strategy, and LLM testing - through a single YAML configuration file. The toolkit supports basic, intermediate, and advanced usage scenarios, enabling users to run custom experiments, conduct ablation studies, and automate fine-tuning workflows. It provides features for data ingestion, model definition, training, inference, quality assurance, and artifact outputs, making it a comprehensive tool for fine-tuning large language models.
For similar tasks
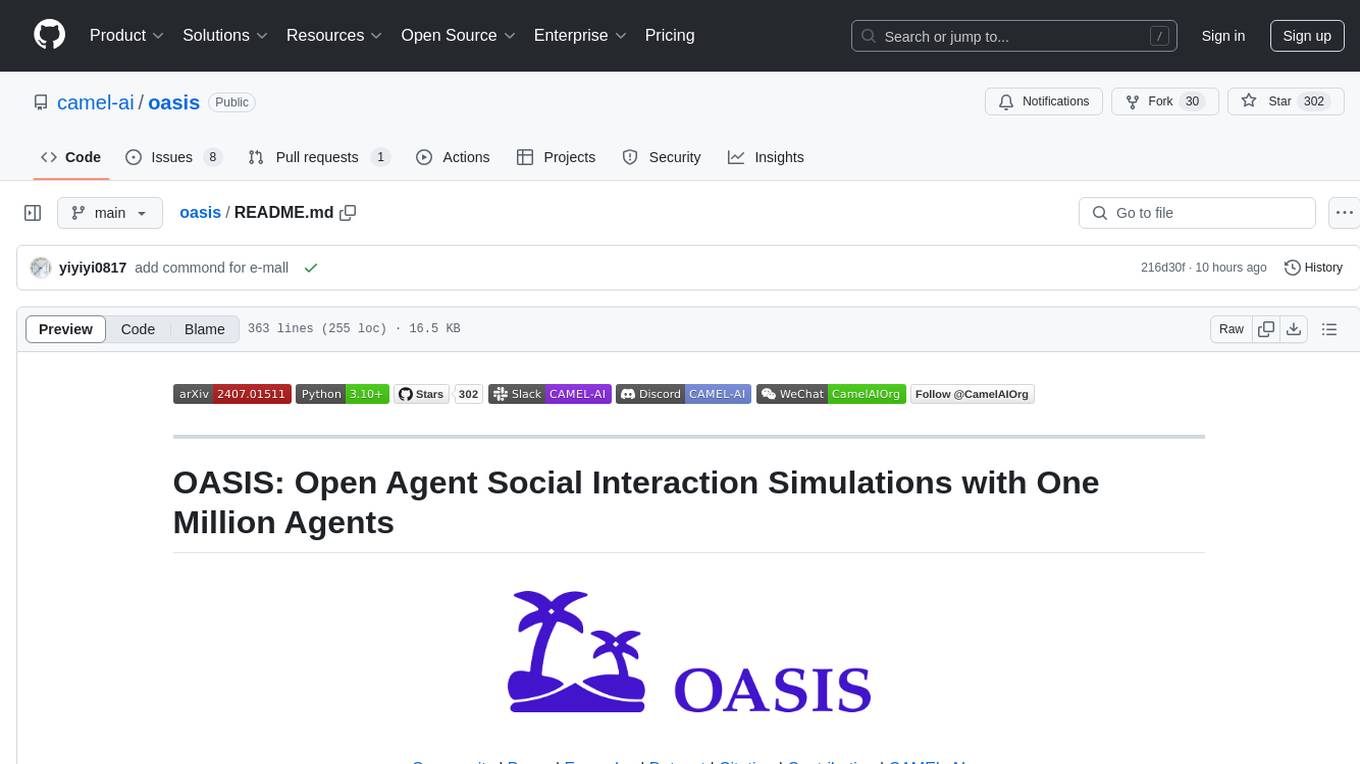
oasis
OASIS is a scalable, open-source social media simulator that integrates large language models with rule-based agents to realistically mimic the behavior of up to one million users on platforms like Twitter and Reddit. It facilitates the study of complex social phenomena such as information spread, group polarization, and herd behavior, offering a versatile tool for exploring diverse social dynamics and user interactions in digital environments. With features like scalability, dynamic environments, diverse action spaces, and integrated recommendation systems, OASIS provides a comprehensive platform for simulating social media interactions at a large scale.
For similar jobs
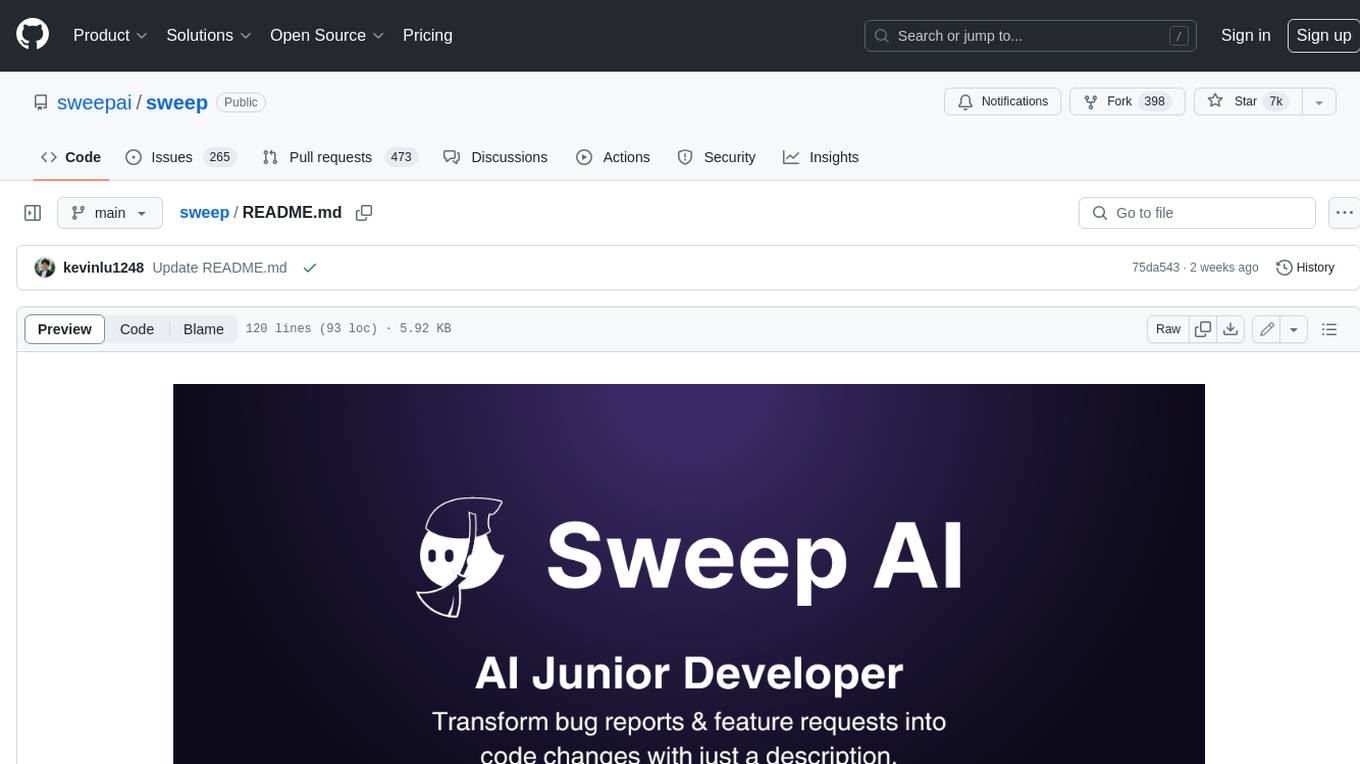
sweep
Sweep is an AI junior developer that turns bugs and feature requests into code changes. It automatically handles developer experience improvements like adding type hints and improving test coverage.
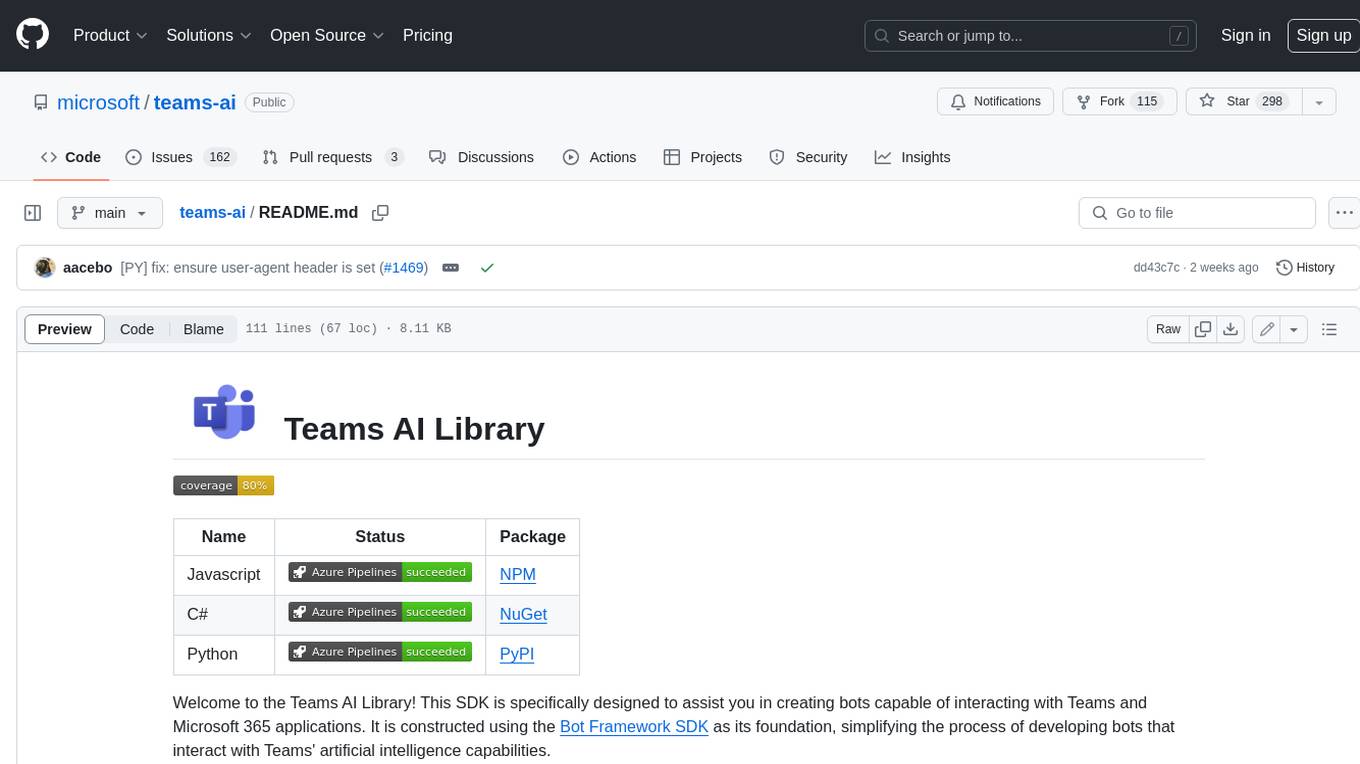
teams-ai
The Teams AI Library is a software development kit (SDK) that helps developers create bots that can interact with Teams and Microsoft 365 applications. It is built on top of the Bot Framework SDK and simplifies the process of developing bots that interact with Teams' artificial intelligence capabilities. The SDK is available for JavaScript/TypeScript, .NET, and Python.
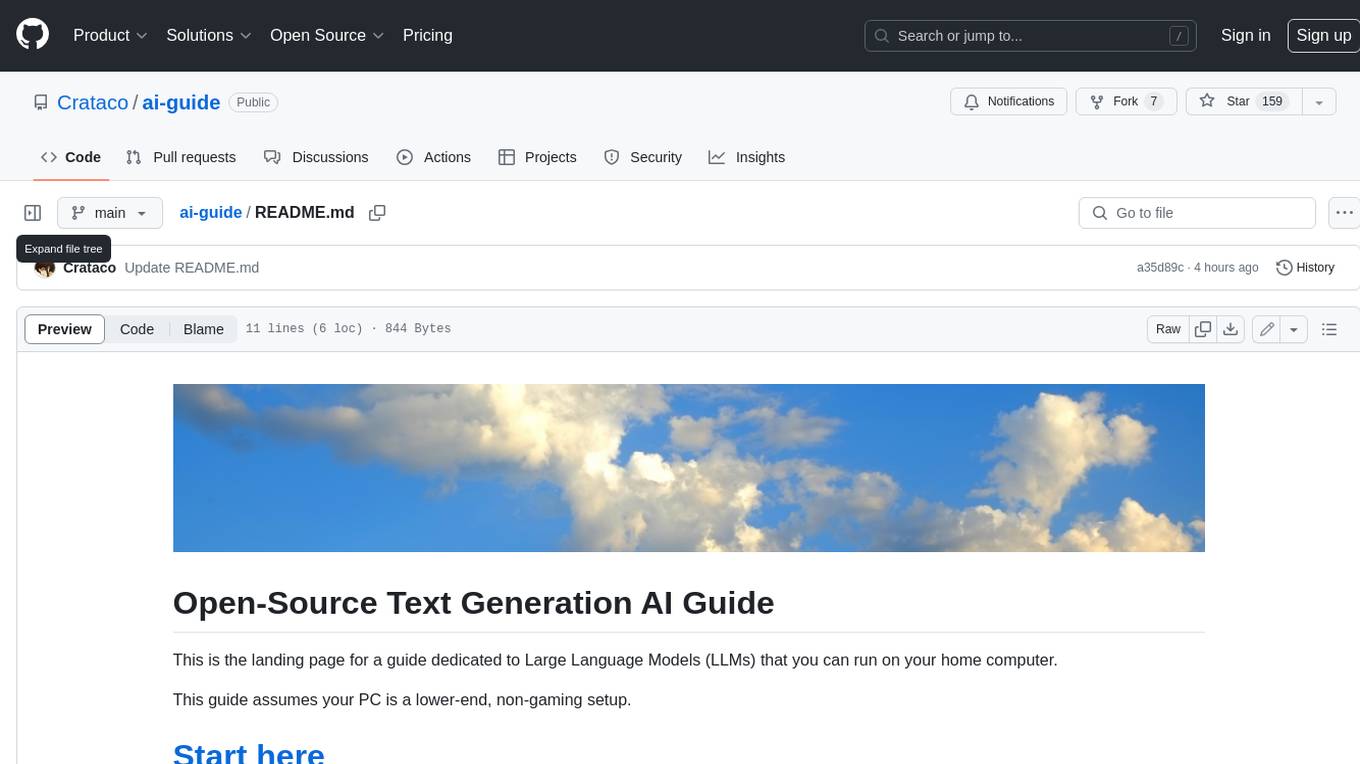
ai-guide
This guide is dedicated to Large Language Models (LLMs) that you can run on your home computer. It assumes your PC is a lower-end, non-gaming setup.
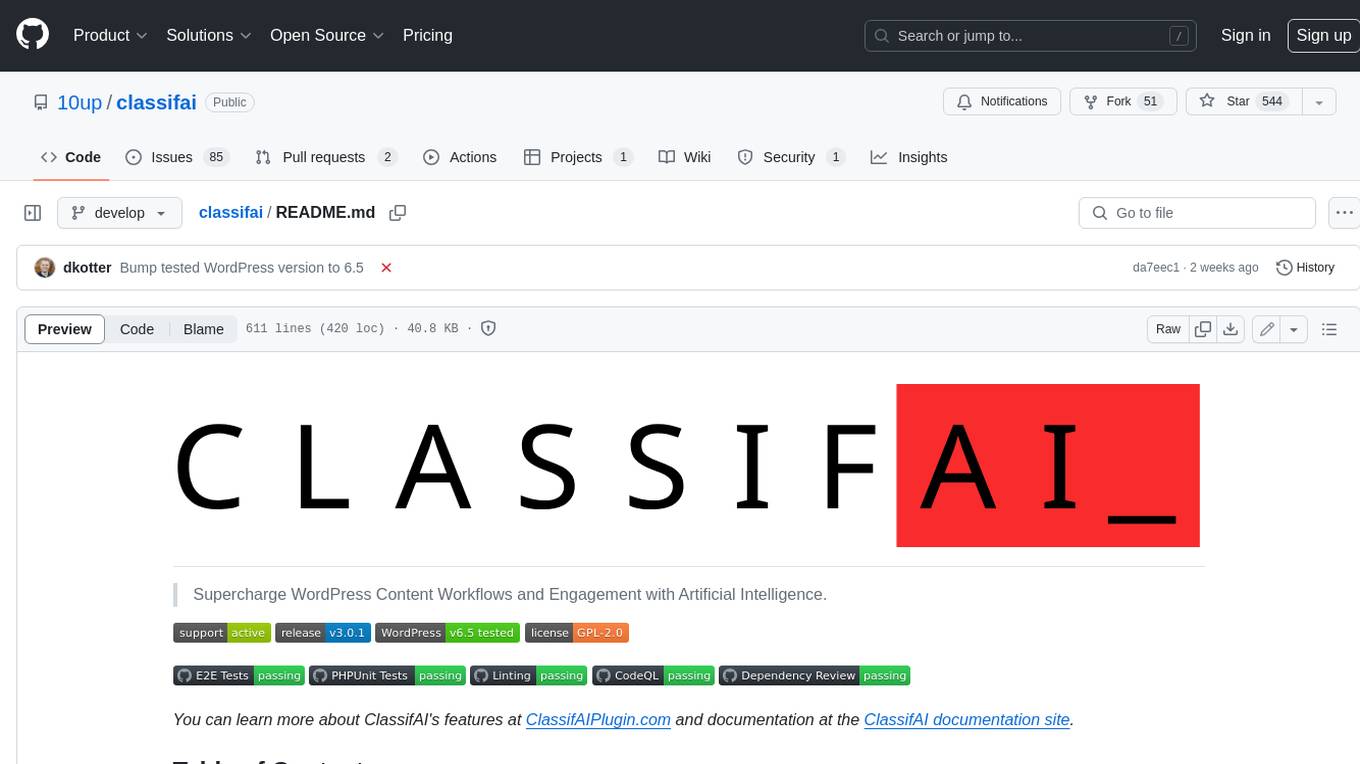
classifai
Supercharge WordPress Content Workflows and Engagement with Artificial Intelligence. Tap into leading cloud-based services like OpenAI, Microsoft Azure AI, Google Gemini and IBM Watson to augment your WordPress-powered websites. Publish content faster while improving SEO performance and increasing audience engagement. ClassifAI integrates Artificial Intelligence and Machine Learning technologies to lighten your workload and eliminate tedious tasks, giving you more time to create original content that matters.
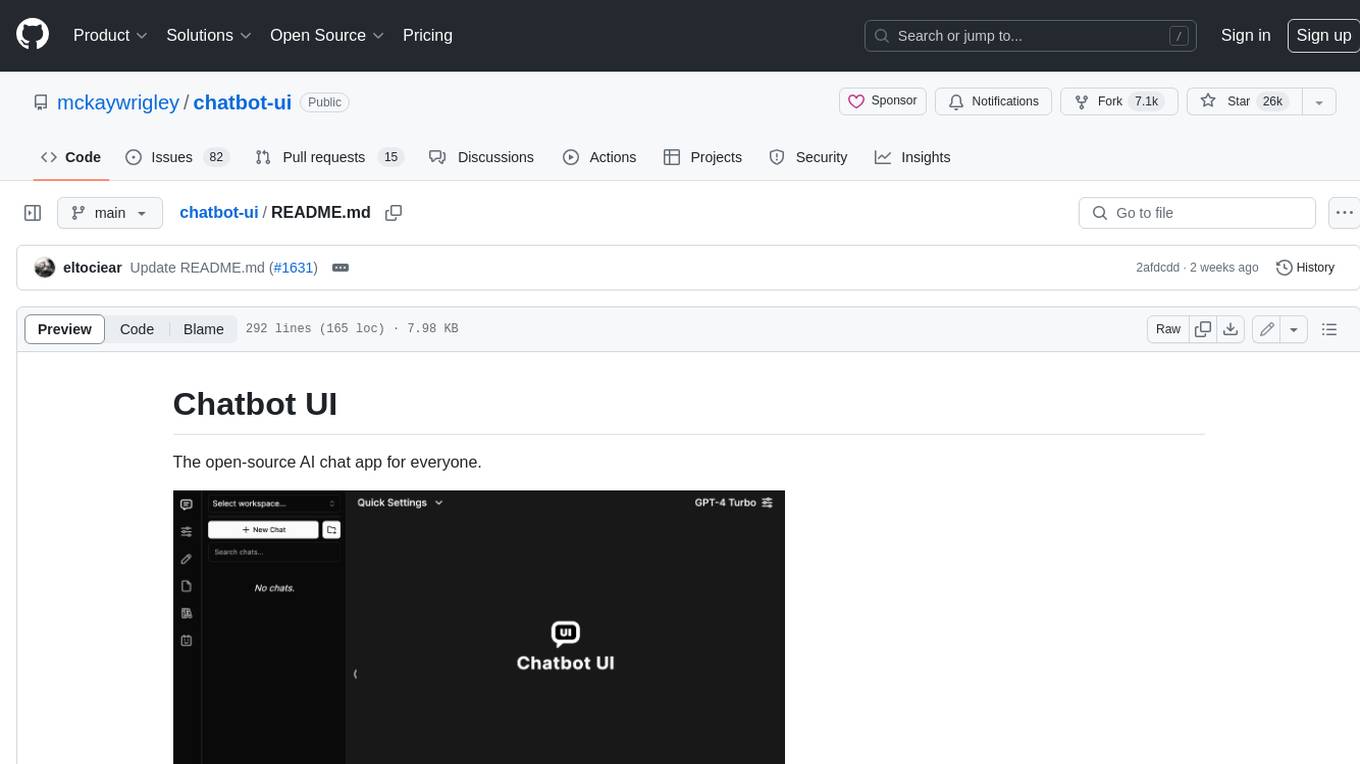
chatbot-ui
Chatbot UI is an open-source AI chat app that allows users to create and deploy their own AI chatbots. It is easy to use and can be customized to fit any need. Chatbot UI is perfect for businesses, developers, and anyone who wants to create a chatbot.
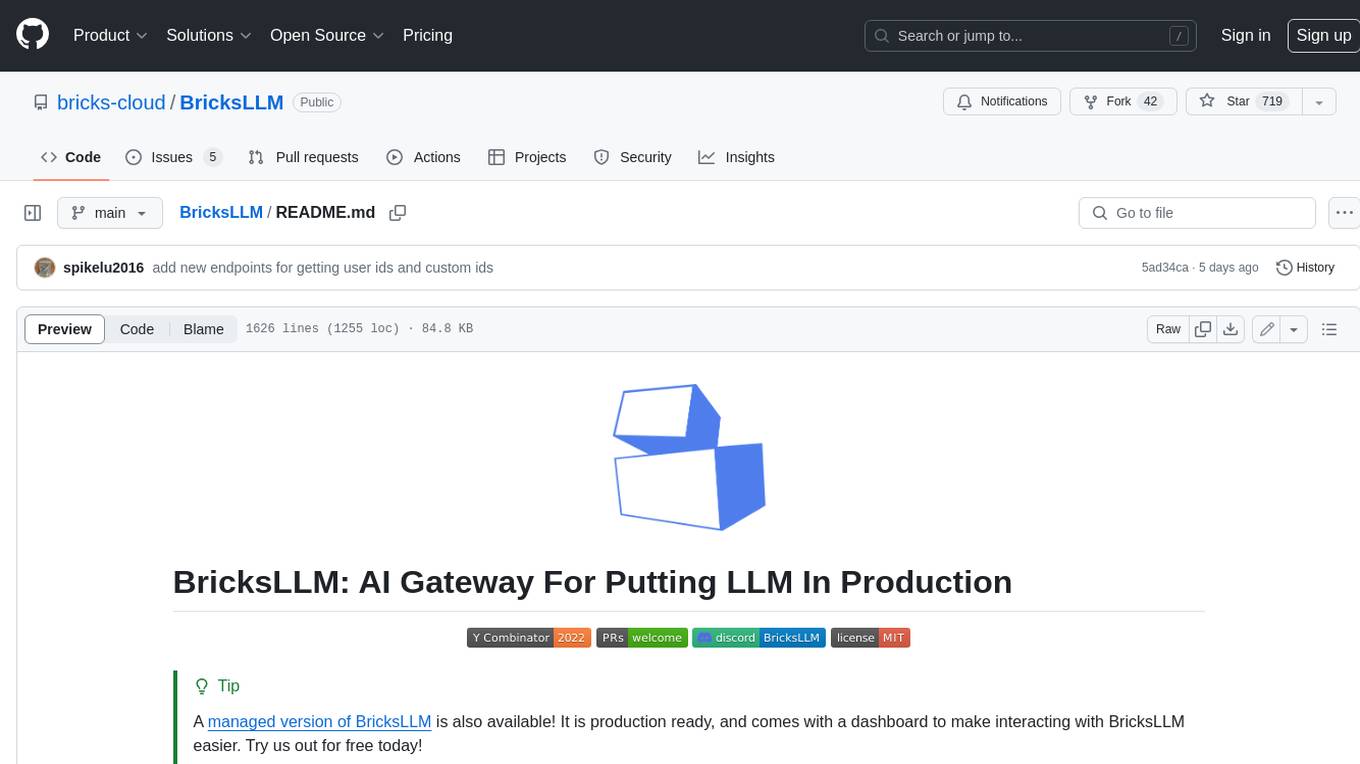
BricksLLM
BricksLLM is a cloud native AI gateway written in Go. Currently, it provides native support for OpenAI, Anthropic, Azure OpenAI and vLLM. BricksLLM aims to provide enterprise level infrastructure that can power any LLM production use cases. Here are some use cases for BricksLLM: * Set LLM usage limits for users on different pricing tiers * Track LLM usage on a per user and per organization basis * Block or redact requests containing PIIs * Improve LLM reliability with failovers, retries and caching * Distribute API keys with rate limits and cost limits for internal development/production use cases * Distribute API keys with rate limits and cost limits for students
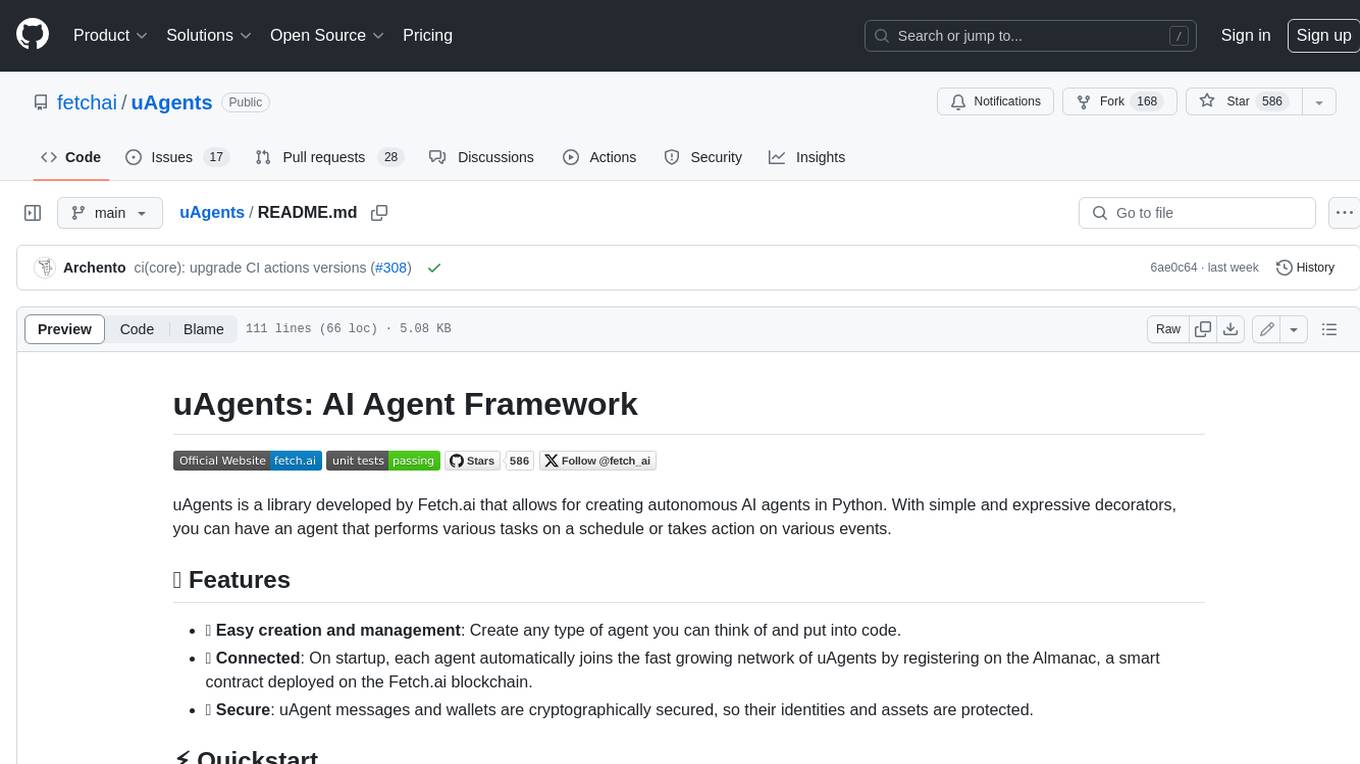
uAgents
uAgents is a Python library developed by Fetch.ai that allows for the creation of autonomous AI agents. These agents can perform various tasks on a schedule or take action on various events. uAgents are easy to create and manage, and they are connected to a fast-growing network of other uAgents. They are also secure, with cryptographically secured messages and wallets.
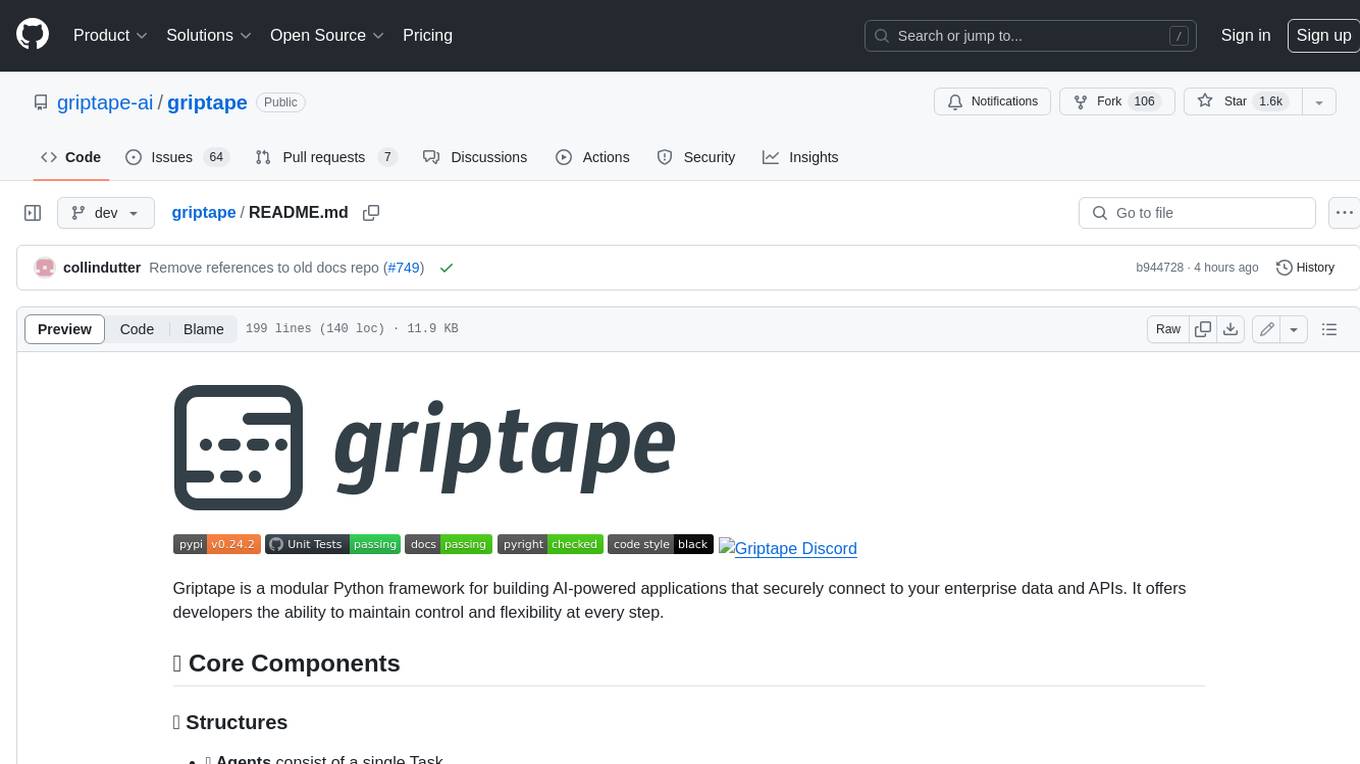
griptape
Griptape is a modular Python framework for building AI-powered applications that securely connect to your enterprise data and APIs. It offers developers the ability to maintain control and flexibility at every step. Griptape's core components include Structures (Agents, Pipelines, and Workflows), Tasks, Tools, Memory (Conversation Memory, Task Memory, and Meta Memory), Drivers (Prompt and Embedding Drivers, Vector Store Drivers, Image Generation Drivers, Image Query Drivers, SQL Drivers, Web Scraper Drivers, and Conversation Memory Drivers), Engines (Query Engines, Extraction Engines, Summary Engines, Image Generation Engines, and Image Query Engines), and additional components (Rulesets, Loaders, Artifacts, Chunkers, and Tokenizers). Griptape enables developers to create AI-powered applications with ease and efficiency.