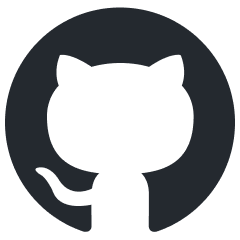
llm_client
The Easiest Rust Interface for Local LLMs and an Interface for Deterministic Signals from Probabilistic LLM Vibes
Stars: 123
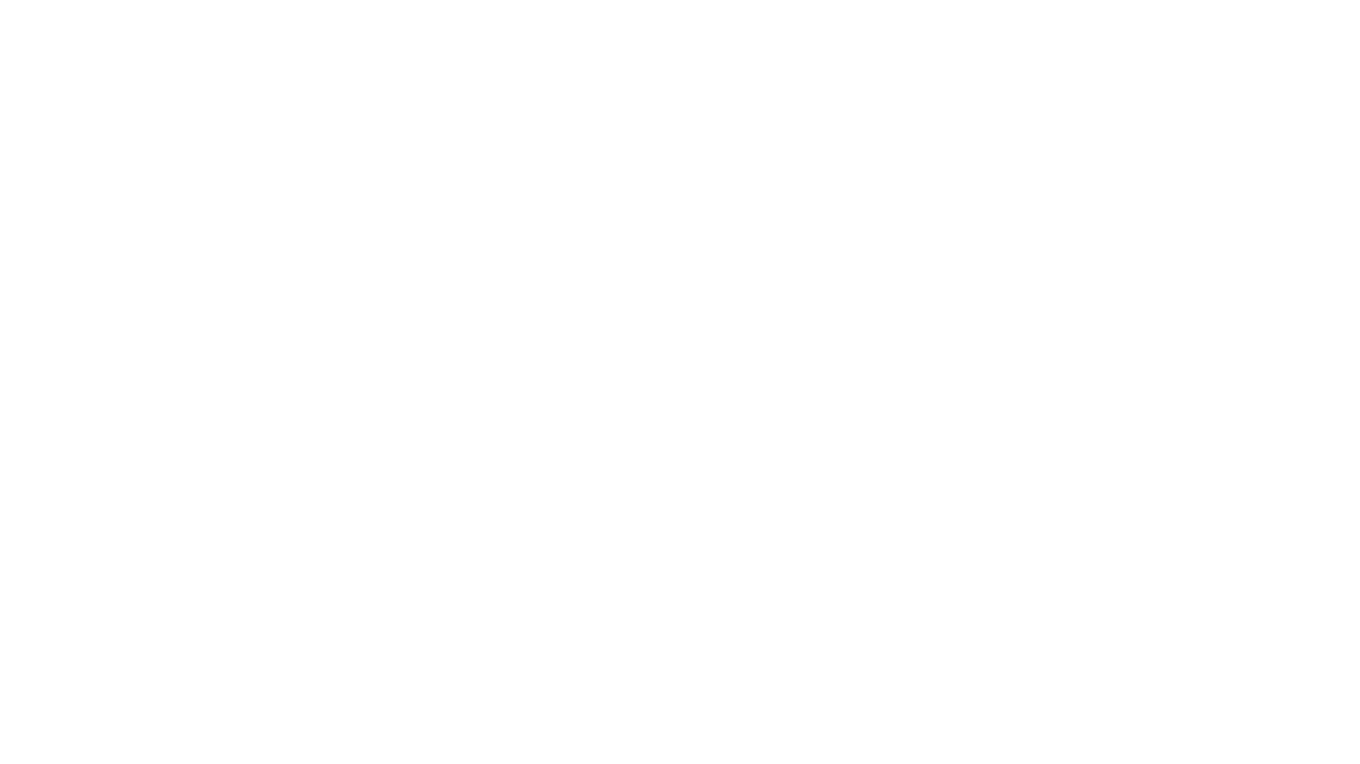
llm_client is a Rust interface designed for Local Large Language Models (LLMs) that offers automated build support for CPU, CUDA, MacOS, easy model presets, and a novel cascading prompt workflow for controlled generation. It provides a breadth of configuration options and API support for various OpenAI compatible APIs. The tool is primarily focused on deterministic signals from probabilistic LLM vibes, enabling specialized workflows for specific tasks and reproducible outcomes.
README:
# For Mac (CPU and GPU), windows (CPU and CUDA), or linux (CPU and CUDA)
llm_client="*"
This will download and build llama.cpp. See build.md for other features and backends like mistral.rs.
use Llmclient::prelude::*;
// Loads the largest quant available based on your VRAM or system memory
let llm_client = LlmClient::llama_cpp()
.mistral7b_instruct_v0_3() // Uses a preset model
.init() // Downloads model from hugging face and starts the inference interface
.await?;
Several of the most common models are available as presets. Loading from local models is also fully supported. See models.md for more information.
- Automated build and support for CPU, CUDA, MacOS
- Easy model presets and quant selection
- Novel cascading prompt workflow for CoT and NLP workflows. DIY workflow creation supported!
- Breadth of configuration options (sampler params, retry logic, prompt caching, logit bias, grammars, etc)
- API support for OpenAI, Anthropic, Perplexity, and any OpenAI compatible API
In addition to basic LLM inference, llm_client is primarily designed for controlled generation using step based cascade workflows. This prompting system runs pre-defined workflows that control and constrain both the overall structure of generation and individual tokens during inference. This allows the implementation of specialized workflows for specific tasks, shaping LLM outputs towards intended, reproducible outcomes.
let response: u32 = llm_client.reason().integer()
.instructions()
.set_content("Sally (a girl) has 3 brothers. Each brother has 2 sisters. How many sisters does Sally have?")
.return_primitive().await?;
// Recieve 'primitive' outputs
assert_eq!(response, 1)
This runs the reason one round cascading prompt workflow with an integer output.
This method significantly improves the reliability of LLM use cases. For example, there are test cases this repo that can be used to benchmark an LLM. There is a large increase in accuracy when comparing basic inference with a constrained outcome and a CoT style cascading prompt workflow. The decision workflow that runs N count of CoT workflows across a temperature gradient approaches 100% accuracy for the test cases.
I have a full breakdown of this in my blog post, "Step-Based Cascading Prompts: Deterministic Signals from the LLM Vibe Space."
Jump to the readme.md of the llm_client crate to find out how to use them.
- device config - customizing your inference config
- basic completion - the most basic request available
- basic primitive - returns the request primitive
- reason - a cascade workflow that performs CoT reasoning before returning a primitive
- decision - uses the reason workflow N times across a temperature gradient
- extract urls - a cascade workflow that extracts all URLs from text that meet a predict
- Improve the Cascading workflow API to be easier.
- Refactor the benchmarks module for easy model comparison.
- WebUI client for local consumption.
- Server mode for "LLM-in-a-box" deployments
- Full Rust inference via mistral.rs or candle.
- llm_utils is a sibling crate that was split from the llm_client. If you just need prompting, tokenization, model loading, etc, I suggest using the llm_utils crate on it's own.
- llm_interface is a sub-crate of llm_client. It is the backend for LLM inference.
- llm_devices is a sub-crate of llm_client. It contains device and build managment behavior.
- llama.cpp is used in server mode for LLM inference as the current default.
- mistral.rs is available for basic use, but is a WIP.
Shelby Jenkins - Here or Linkedin
For Tasks:
Click tags to check more tools for each tasksFor Jobs:
Alternative AI tools for llm_client
Similar Open Source Tools
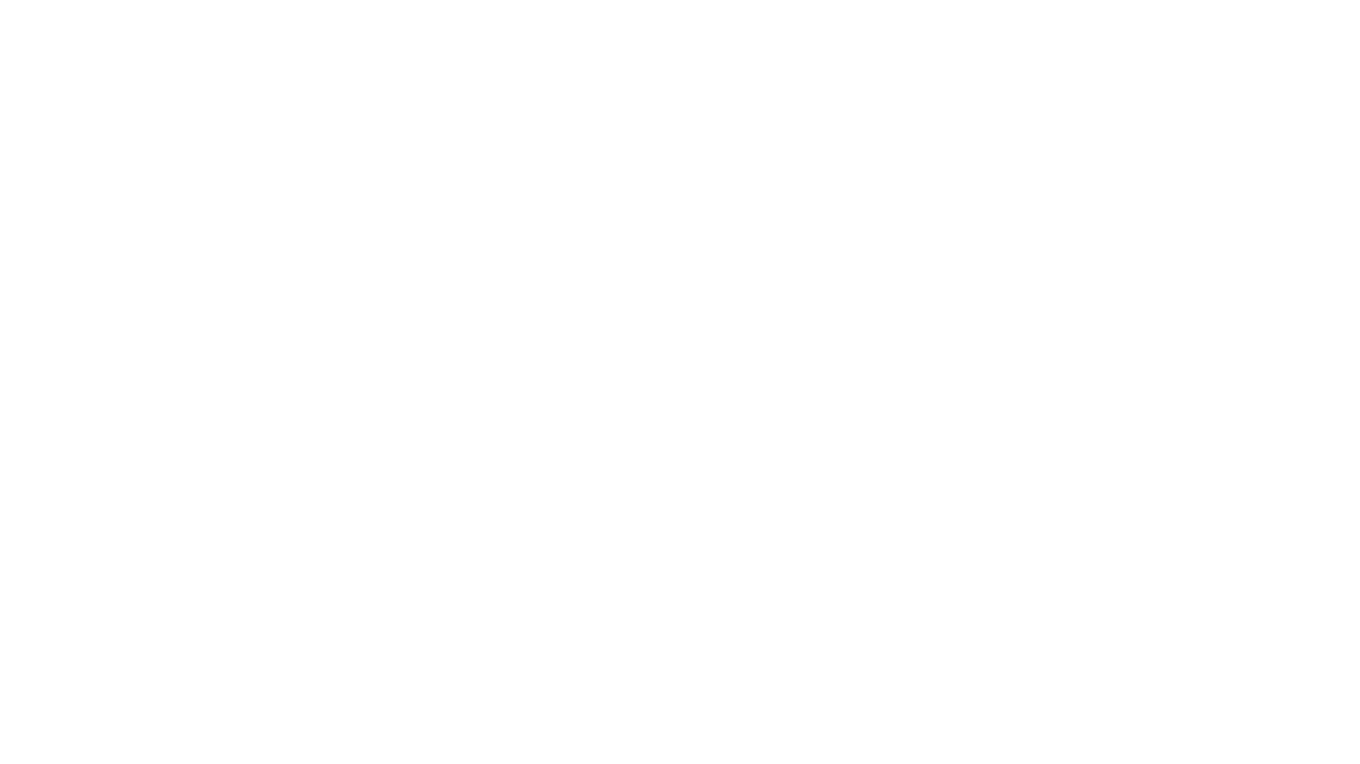
llm_client
llm_client is a Rust interface designed for Local Large Language Models (LLMs) that offers automated build support for CPU, CUDA, MacOS, easy model presets, and a novel cascading prompt workflow for controlled generation. It provides a breadth of configuration options and API support for various OpenAI compatible APIs. The tool is primarily focused on deterministic signals from probabilistic LLM vibes, enabling specialized workflows for specific tasks and reproducible outcomes.
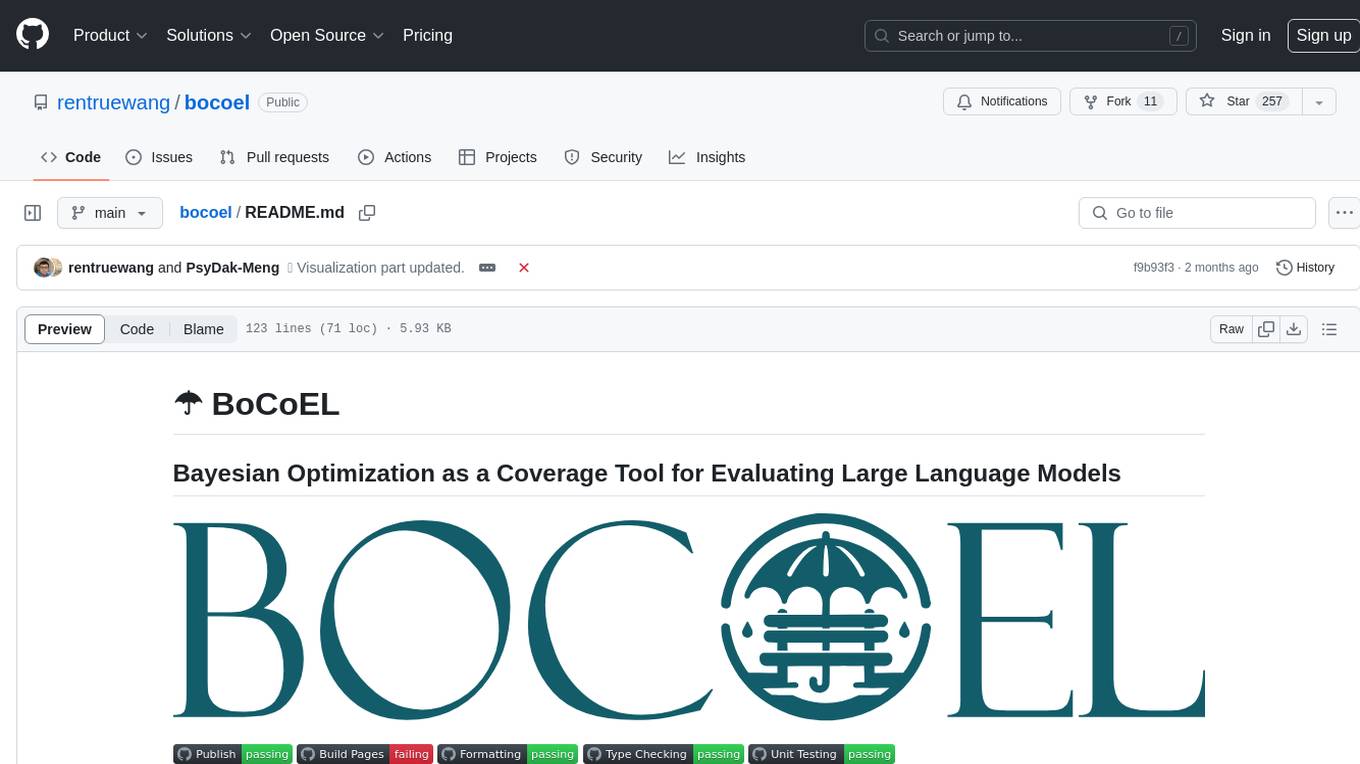
bocoel
BoCoEL is a tool that leverages Bayesian Optimization to efficiently evaluate large language models by selecting a subset of the corpus for evaluation. It encodes individual entries into embeddings, uses Bayesian optimization to select queries, retrieves from the corpus, and provides easily managed evaluations. The tool aims to reduce computation costs during evaluation with a dynamic budget, supporting models like GPT2, Pythia, and LLAMA through integration with Hugging Face transformers and datasets. BoCoEL offers a modular design and efficient representation of the corpus to enhance evaluation quality.
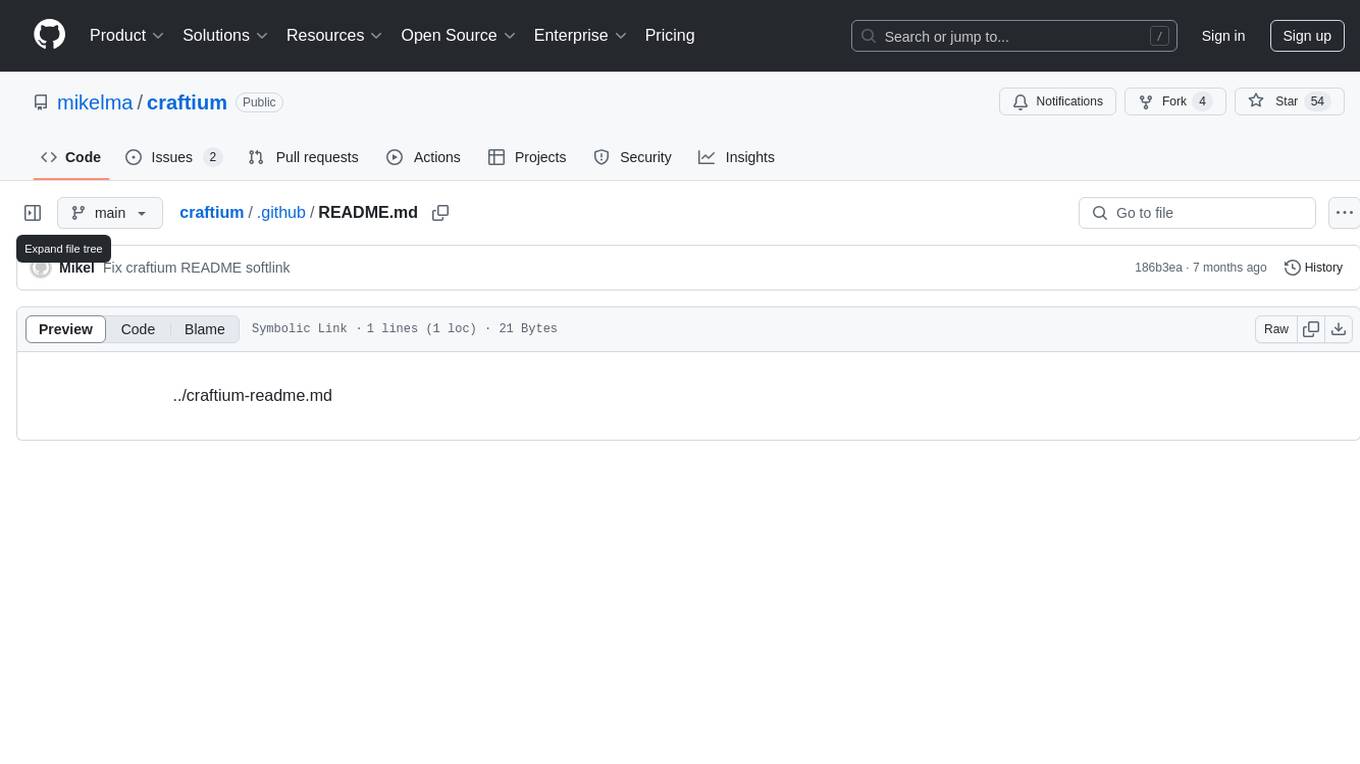
craftium
Craftium is an open-source platform based on the Minetest voxel game engine and the Gymnasium and PettingZoo APIs, designed for creating fast, rich, and diverse single and multi-agent environments. It allows for connecting to Craftium's Python process, executing actions as keyboard and mouse controls, extending the Lua API for creating RL environments and tasks, and supporting client/server synchronization for slow agents. Craftium is fully extensible, extensively documented, modern RL API compatible, fully open source, and eliminates the need for Java. It offers a variety of environments for research and development in reinforcement learning.
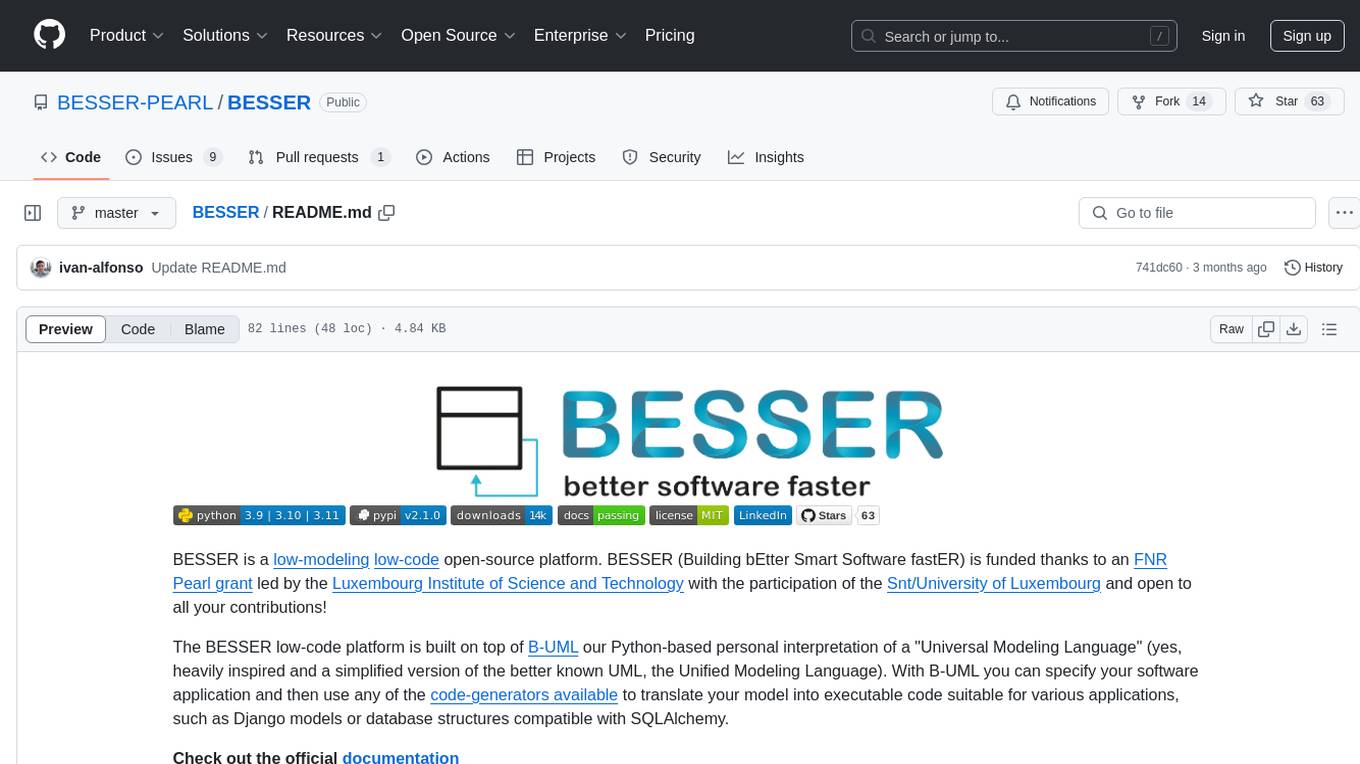
BESSER
BESSER is a low-modeling low-code open-source platform funded by an FNR Pearl grant. It is built on B-UML, a Python-based interpretation of a 'Universal Modeling Language'. Users can specify their software application using B-UML and generate executable code for various applications like Django models or SQLAlchemy-compatible database structures. BESSER is available on PyPi and can be installed with pip. It supports popular Python IDEs and encourages contributions from the community.
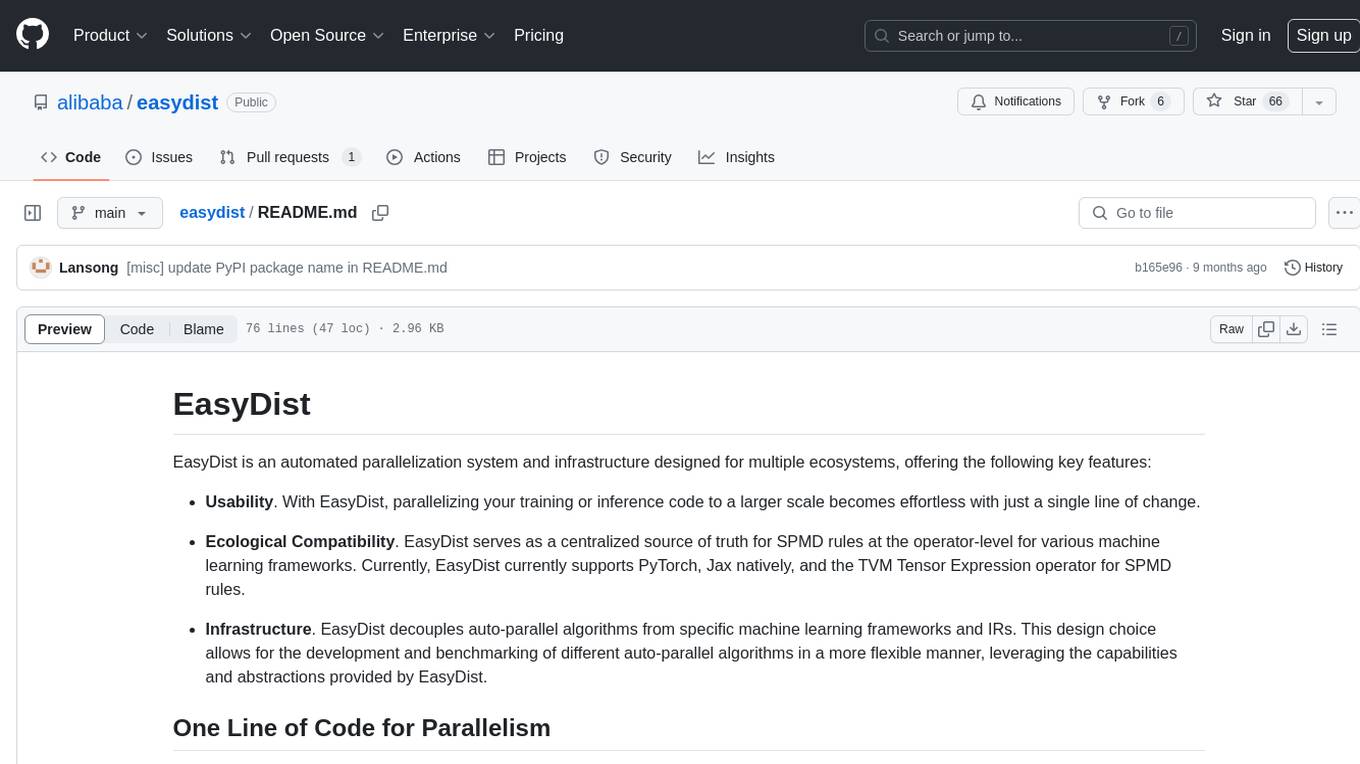
easydist
EasyDist is an automated parallelization system and infrastructure designed for multiple ecosystems. It offers usability by making parallelizing training or inference code effortless with just a single line of change. It ensures ecological compatibility by serving as a centralized source of truth for SPMD rules at the operator-level for various machine learning frameworks. EasyDist decouples auto-parallel algorithms from specific frameworks and IRs, allowing for the development and benchmarking of different auto-parallel algorithms in a flexible manner. The architecture includes MetaOp, MetaIR, and the ShardCombine Algorithm for SPMD sharding rules without manual annotations.
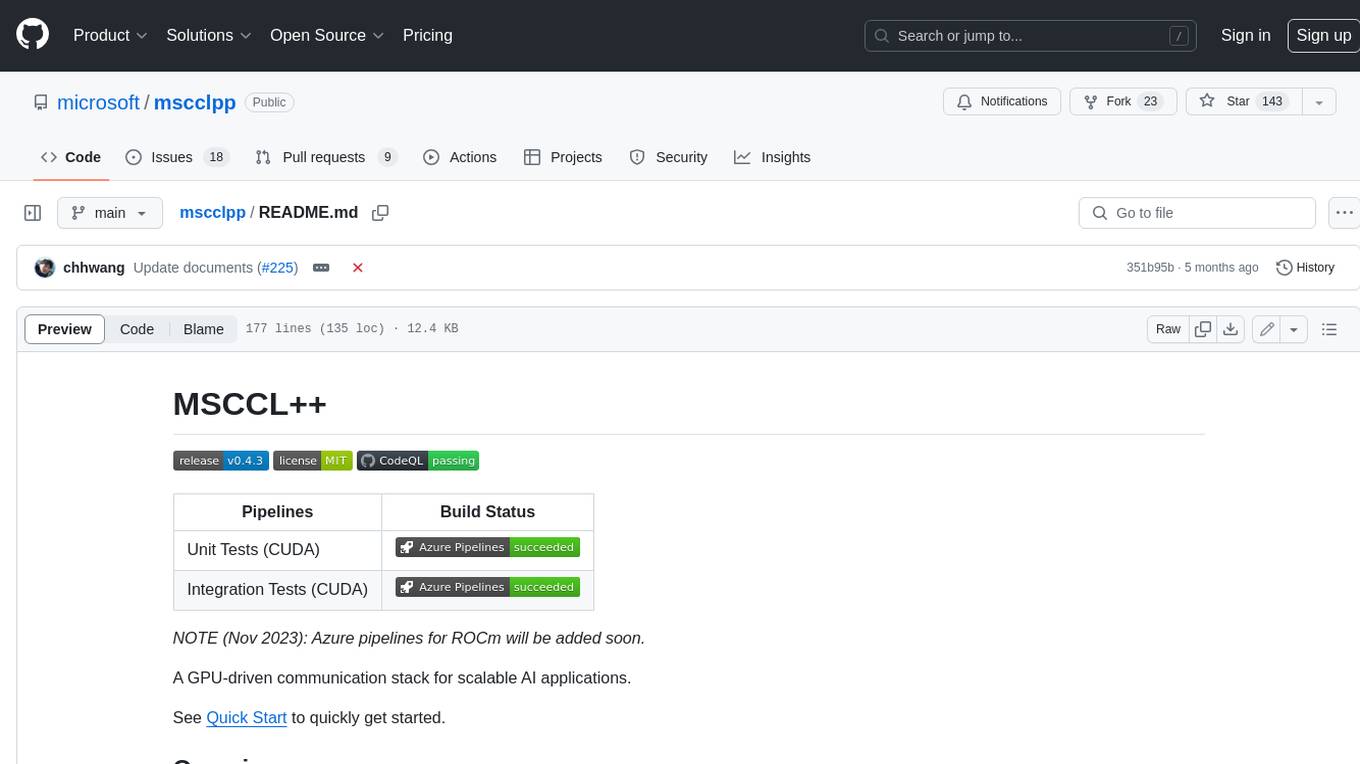
mscclpp
MSCCL++ is a GPU-driven communication stack for scalable AI applications. It provides a highly efficient and customizable communication stack for distributed GPU applications. MSCCL++ redefines inter-GPU communication interfaces, delivering a highly efficient and customizable communication stack for distributed GPU applications. Its design is specifically tailored to accommodate diverse performance optimization scenarios often encountered in state-of-the-art AI applications. MSCCL++ provides communication abstractions at the lowest level close to hardware and at the highest level close to application API. The lowest level of abstraction is ultra light weight which enables a user to implement logics of data movement for a collective operation such as AllReduce inside a GPU kernel extremely efficiently without worrying about memory ordering of different ops. The modularity of MSCCL++ enables a user to construct the building blocks of MSCCL++ in a high level abstraction in Python and feed them to a CUDA kernel in order to facilitate the user's productivity. MSCCL++ provides fine-grained synchronous and asynchronous 0-copy 1-sided abstracts for communication primitives such as `put()`, `get()`, `signal()`, `flush()`, and `wait()`. The 1-sided abstractions allows a user to asynchronously `put()` their data on the remote GPU as soon as it is ready without requiring the remote side to issue any receive instruction. This enables users to easily implement flexible communication logics, such as overlapping communication with computation, or implementing customized collective communication algorithms without worrying about potential deadlocks. Additionally, the 0-copy capability enables MSCCL++ to directly transfer data between user's buffers without using intermediate internal buffers which saves GPU bandwidth and memory capacity. MSCCL++ provides consistent abstractions regardless of the location of the remote GPU (either on the local node or on a remote node) or the underlying link (either NVLink/xGMI or InfiniBand). This simplifies the code for inter-GPU communication, which is often complex due to memory ordering of GPU/CPU read/writes and therefore, is error-prone.
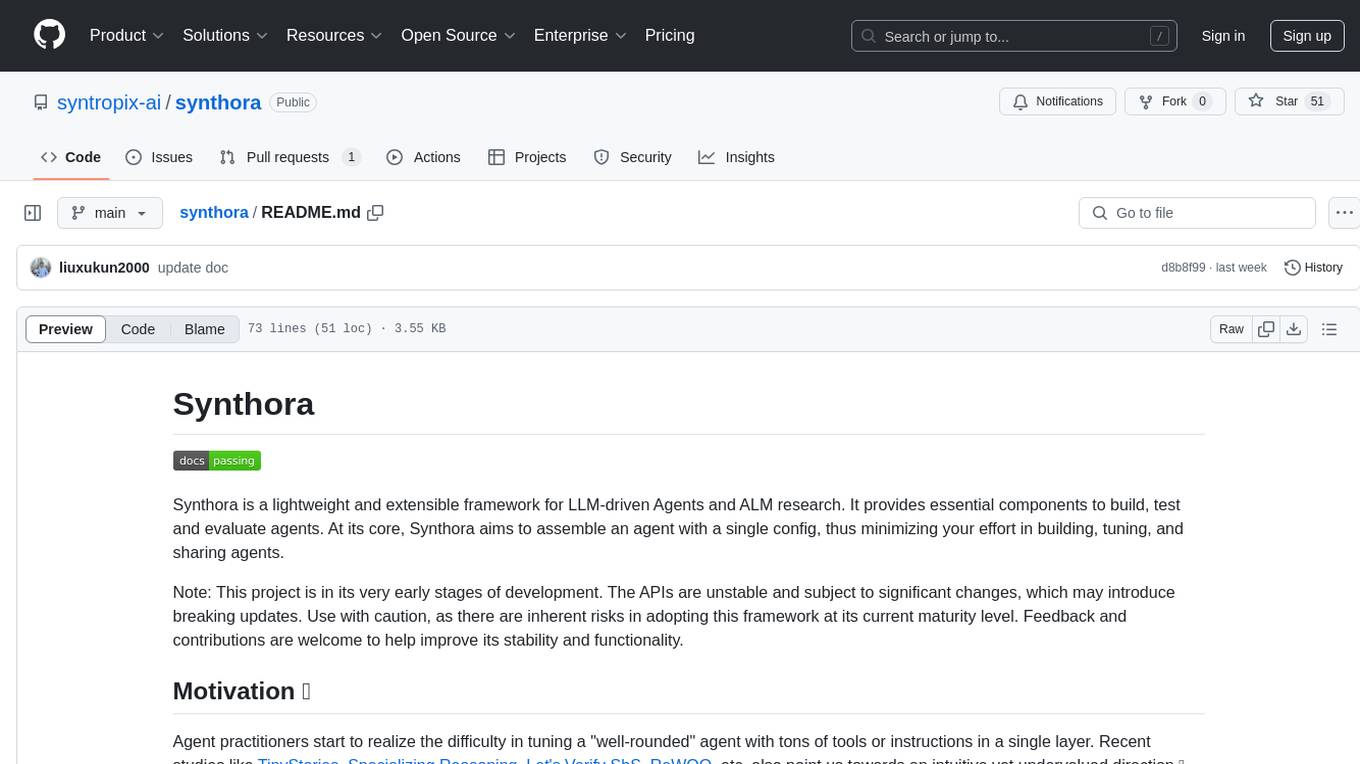
synthora
Synthora is a lightweight and extensible framework for LLM-driven Agents and ALM research. It aims to simplify the process of building, testing, and evaluating agents by providing essential components. The framework allows for easy agent assembly with a single config, reducing the effort required for tuning and sharing agents. Although in early development stages with unstable APIs, Synthora welcomes feedback and contributions to enhance its stability and functionality.
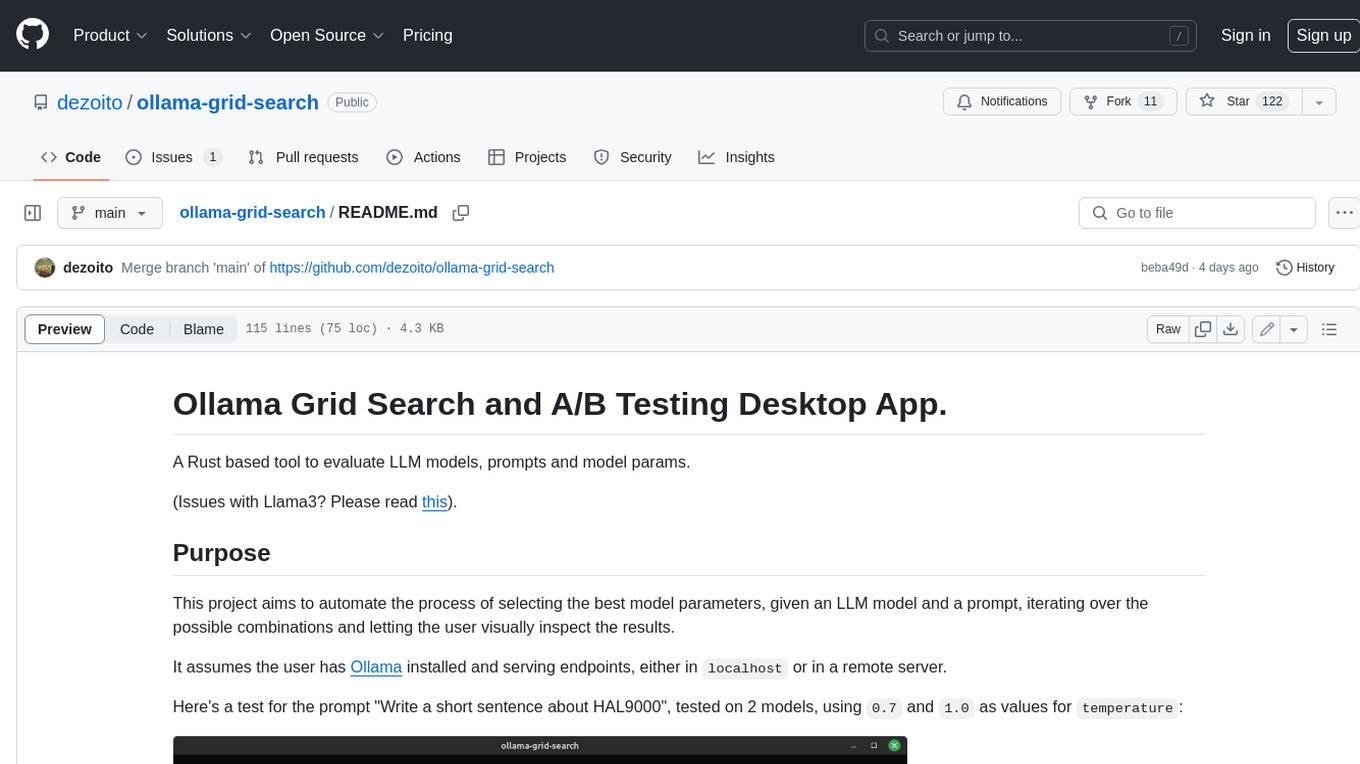
ollama-grid-search
A Rust based tool to evaluate LLM models, prompts and model params. It automates the process of selecting the best model parameters, given an LLM model and a prompt, iterating over the possible combinations and letting the user visually inspect the results. The tool assumes the user has Ollama installed and serving endpoints, either in `localhost` or in a remote server. Key features include: * Automatically fetches models from local or remote Ollama servers * Iterates over different models and params to generate inferences * A/B test prompts on different models simultaneously * Allows multiple iterations for each combination of parameters * Makes synchronous inference calls to avoid spamming servers * Optionally outputs inference parameters and response metadata (inference time, tokens and tokens/s) * Refetching of individual inference calls * Model selection can be filtered by name * List experiments which can be downloaded in JSON format * Configurable inference timeout * Custom default parameters and system prompts can be defined in settings
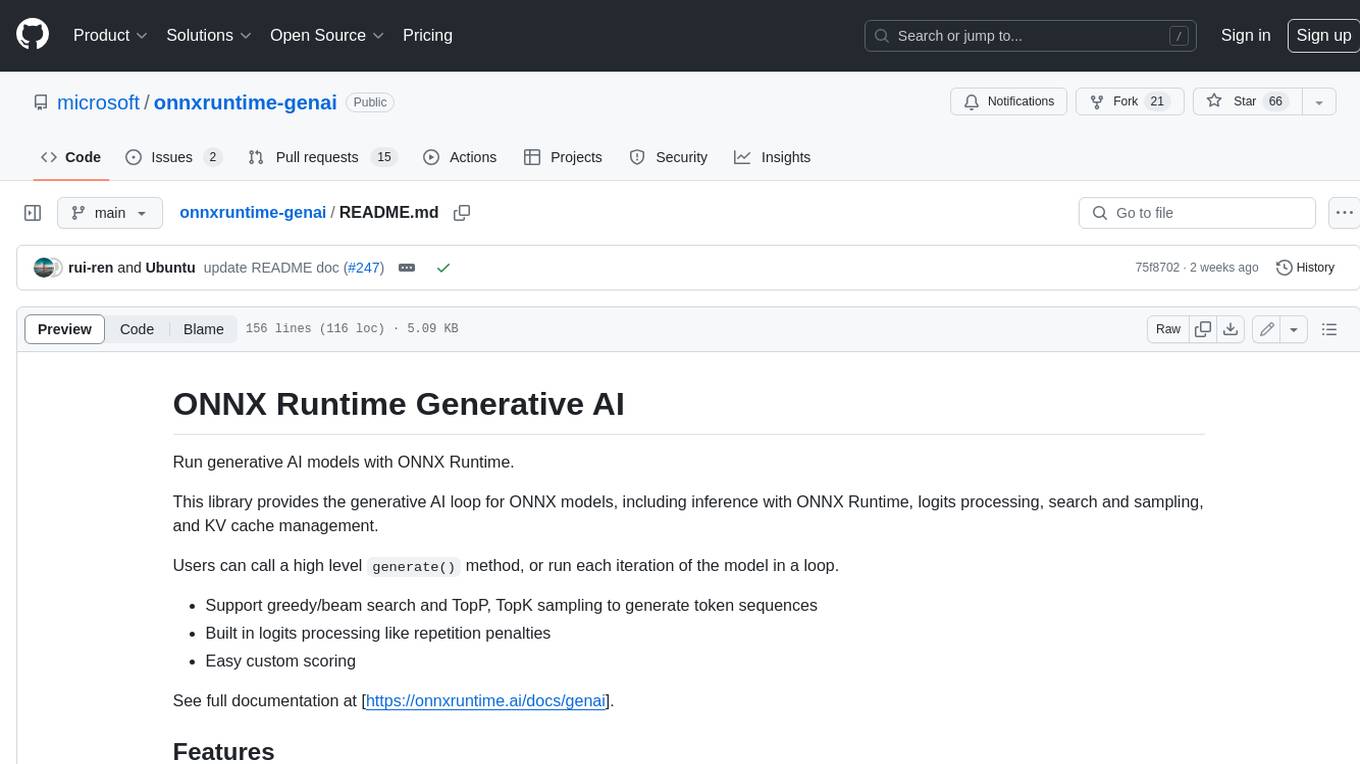
onnxruntime-genai
ONNX Runtime Generative AI is a library that provides the generative AI loop for ONNX models, including inference with ONNX Runtime, logits processing, search and sampling, and KV cache management. Users can call a high level `generate()` method, or run each iteration of the model in a loop. It supports greedy/beam search and TopP, TopK sampling to generate token sequences, has built in logits processing like repetition penalties, and allows for easy custom scoring.
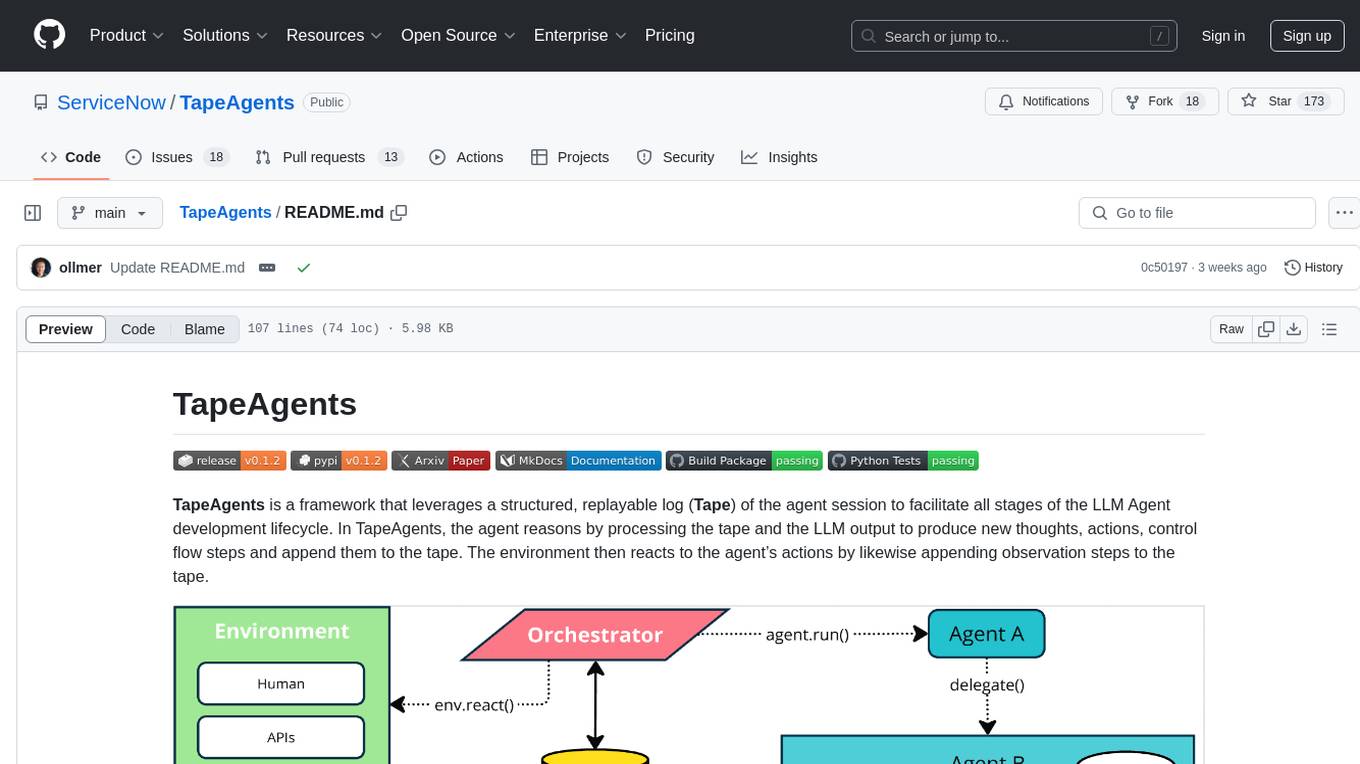
TapeAgents
TapeAgents is a framework that leverages a structured, replayable log of the agent session to facilitate all stages of the LLM Agent development lifecycle. The agent reasons by processing the tape and the LLM output to produce new thoughts, actions, control flow steps, and append them to the tape. Key features include building agents as low-level state machines or high-level multi-agent team configurations, debugging agents with TapeAgent studio or TapeBrowser apps, serving agents with response streaming, and optimizing agent configurations using successful tapes. The Tape-centric design of TapeAgents provides ultimate flexibility in project development, allowing access to tapes for making prompts, generating next steps, and controlling agent behavior.
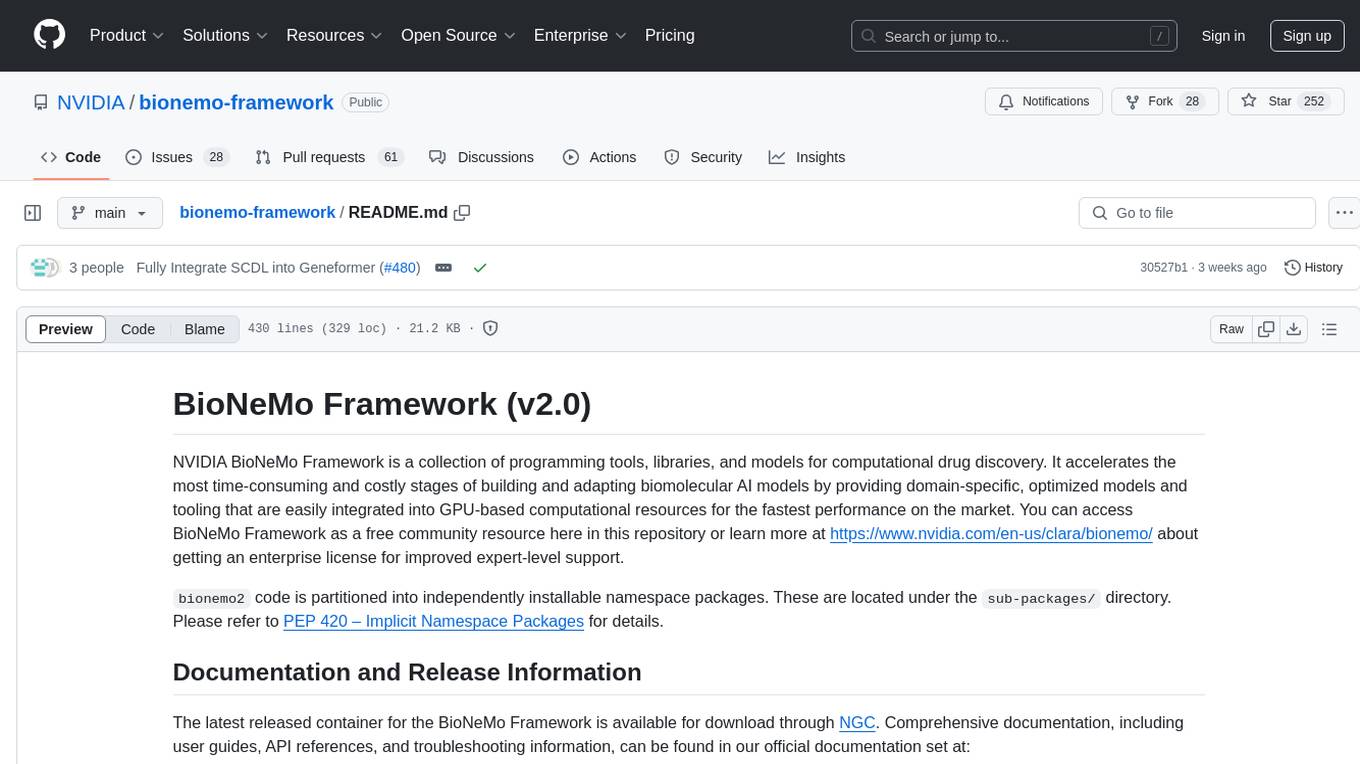
bionemo-framework
NVIDIA BioNeMo Framework is a collection of programming tools, libraries, and models for computational drug discovery. It accelerates building and adapting biomolecular AI models by providing domain-specific, optimized models and tooling for GPU-based computational resources. The framework offers comprehensive documentation and support for both community and enterprise users.
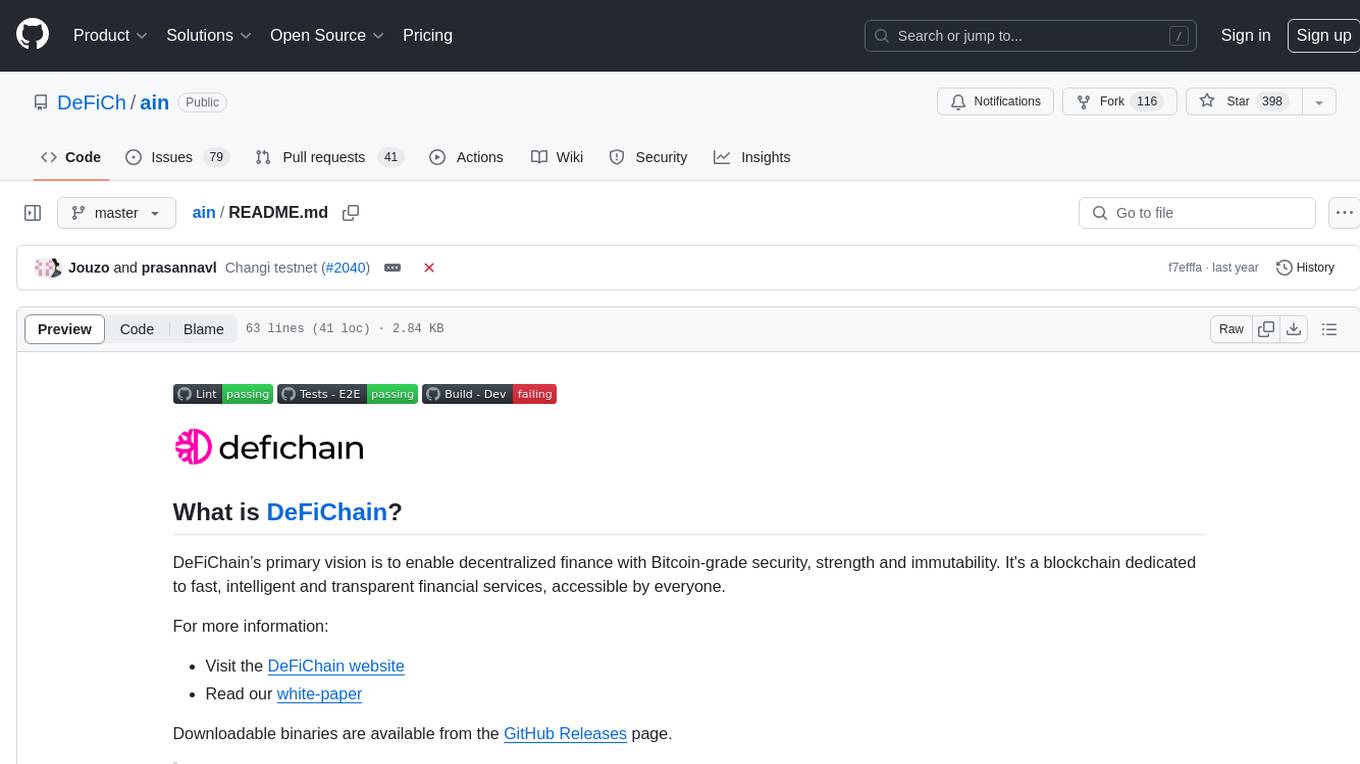
ain
DeFiChain is a blockchain platform dedicated to enabling decentralized finance with Bitcoin-grade security, strength, and immutability. It offers fast, intelligent, and transparent financial services accessible to everyone. DeFiChain has made significant modifications from Bitcoin Core, including moving to Proof-of-Stake, introducing a masternode model, supporting a community fund, anchoring to the Bitcoin blockchain, and enhancing decentralized financial transaction and opcode support. The platform is under active development with regular releases and contributions are welcomed.
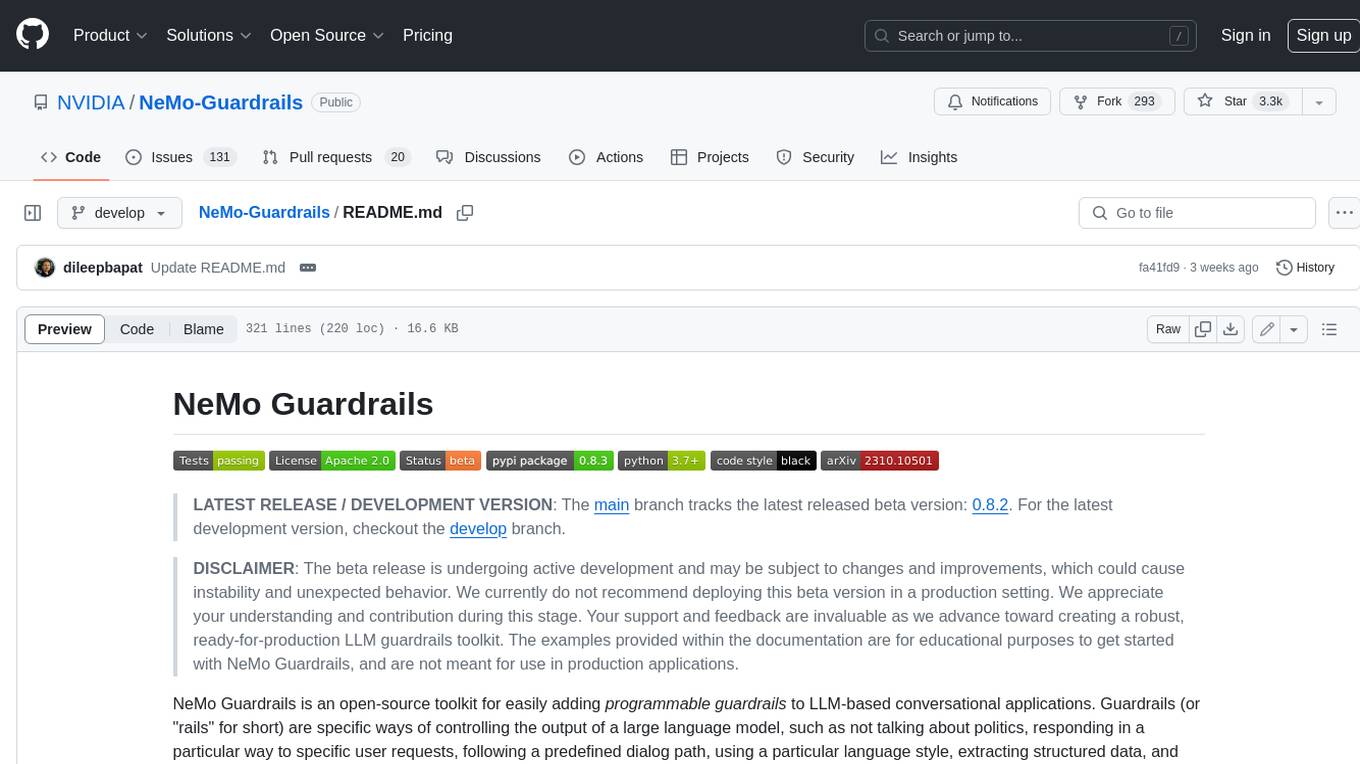
NeMo-Guardrails
NeMo Guardrails is an open-source toolkit for easily adding _programmable guardrails_ to LLM-based conversational applications. Guardrails (or "rails" for short) are specific ways of controlling the output of a large language model, such as not talking about politics, responding in a particular way to specific user requests, following a predefined dialog path, using a particular language style, extracting structured data, and more.
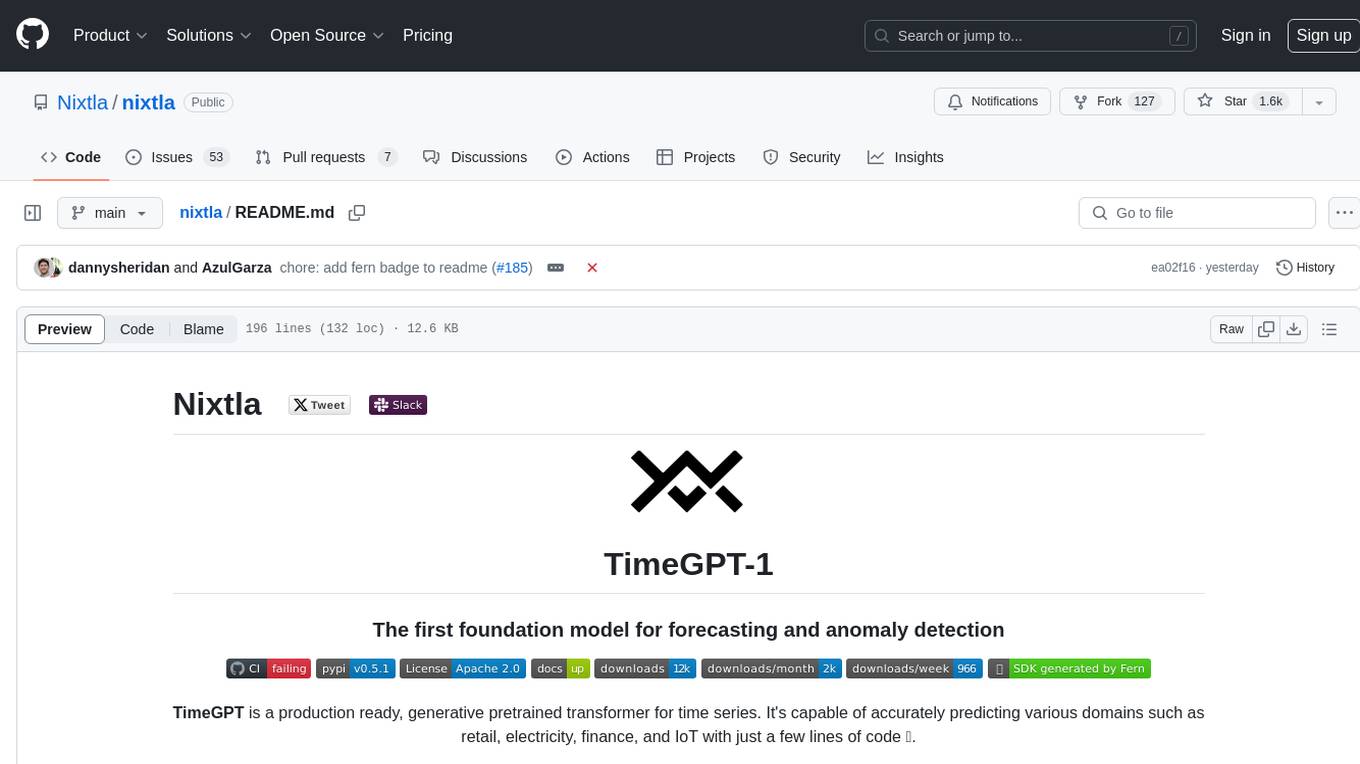
nixtla
Nixtla is a production-ready generative pretrained transformer for time series forecasting and anomaly detection. It can accurately predict various domains such as retail, electricity, finance, and IoT with just a few lines of code. TimeGPT introduces a paradigm shift with its standout performance, efficiency, and simplicity, making it accessible even to users with minimal coding experience. The model is based on self-attention and is independently trained on a vast time series dataset to minimize forecasting error. It offers features like zero-shot inference, fine-tuning, API access, adding exogenous variables, multiple series forecasting, custom loss function, cross-validation, prediction intervals, and handling irregular timestamps.
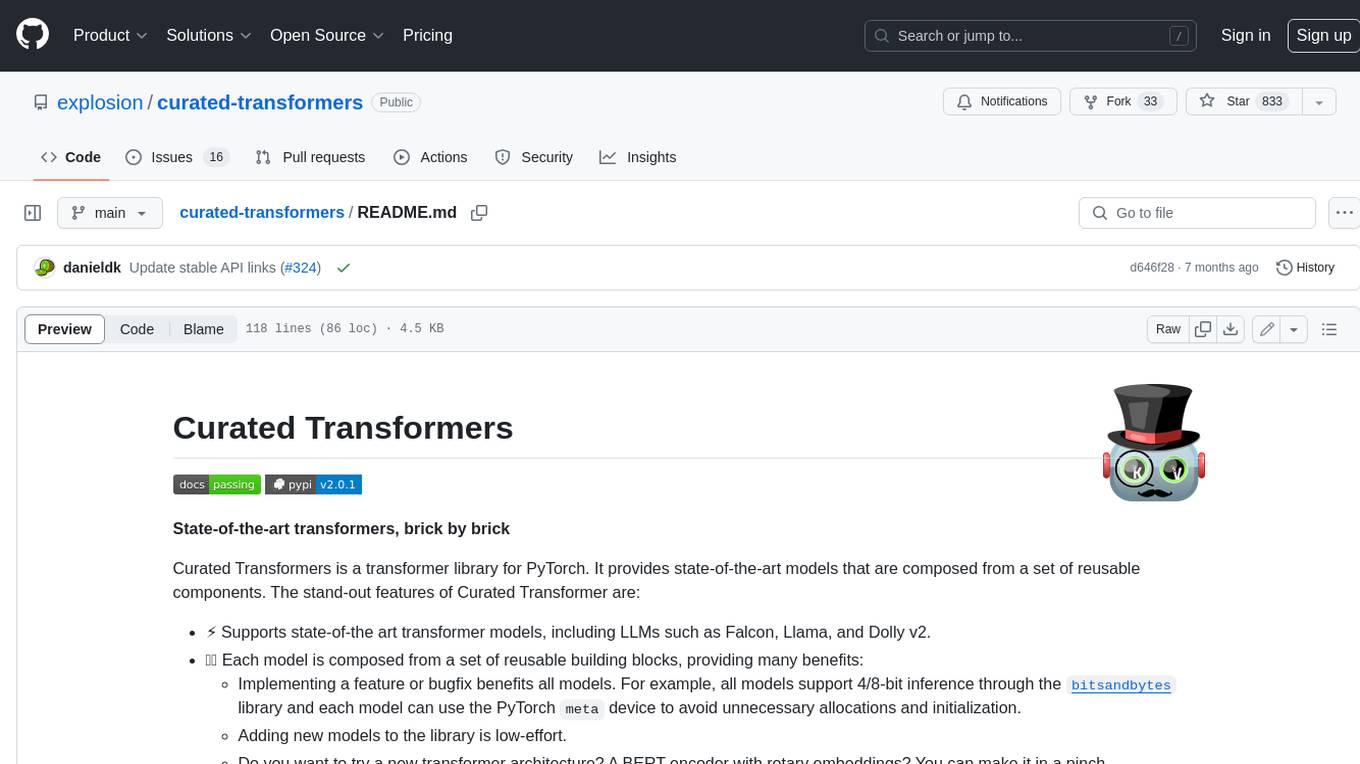
curated-transformers
Curated Transformers is a transformer library for PyTorch that provides state-of-the-art models composed of reusable components. It supports various transformer architectures, including encoders like ALBERT, BERT, and RoBERTa, and decoders like Falcon, Llama, and MPT. The library emphasizes consistent type annotations, minimal dependencies, and ease of use for education and research. It has been production-tested by Explosion and will be the default transformer implementation in spaCy 3.7.
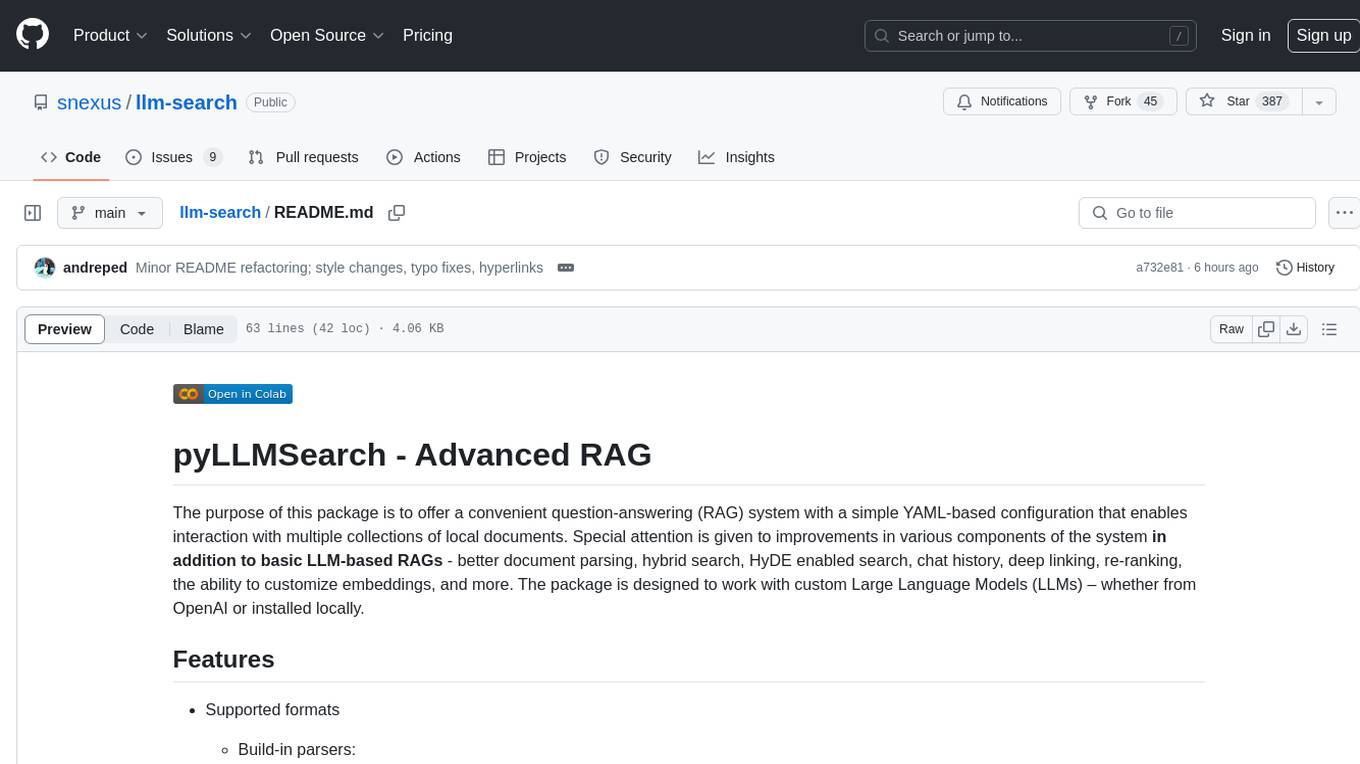
llm-search
pyLLMSearch is an advanced RAG system that offers a convenient question-answering system with a simple YAML-based configuration. It enables interaction with multiple collections of local documents, with improvements in document parsing, hybrid search, chat history, deep linking, re-ranking, customizable embeddings, and more. The package is designed to work with custom Large Language Models (LLMs) from OpenAI or installed locally. It supports various document formats, incremental embedding updates, dense and sparse embeddings, multiple embedding models, 'Retrieve and Re-rank' strategy, HyDE (Hypothetical Document Embeddings), multi-querying, chat history, and interaction with embedded documents using different models. It also offers simple CLI and web interfaces, deep linking, offline response saving, and an experimental API.
For similar tasks
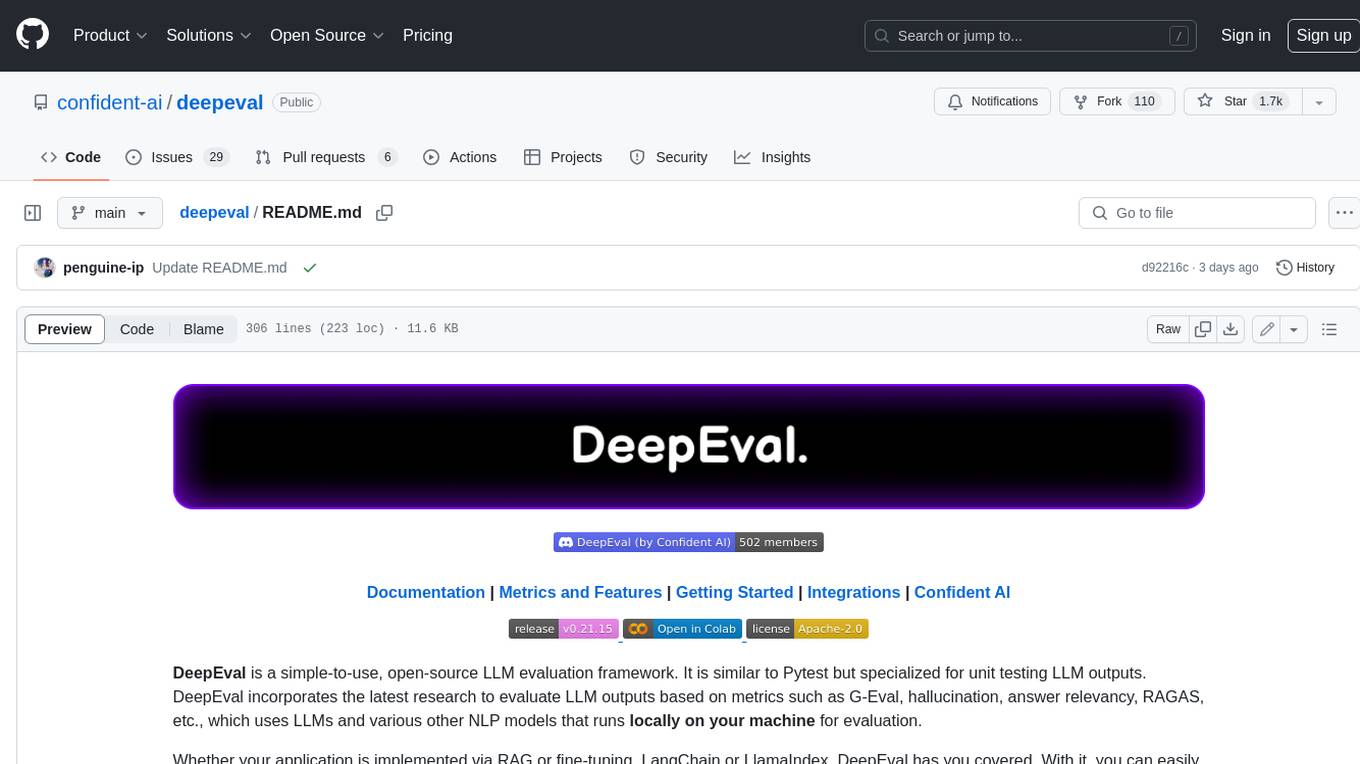
deepeval
DeepEval is a simple-to-use, open-source LLM evaluation framework specialized for unit testing LLM outputs. It incorporates various metrics such as G-Eval, hallucination, answer relevancy, RAGAS, etc., and runs locally on your machine for evaluation. It provides a wide range of ready-to-use evaluation metrics, allows for creating custom metrics, integrates with any CI/CD environment, and enables benchmarking LLMs on popular benchmarks. DeepEval is designed for evaluating RAG and fine-tuning applications, helping users optimize hyperparameters, prevent prompt drifting, and transition from OpenAI to hosting their own Llama2 with confidence.
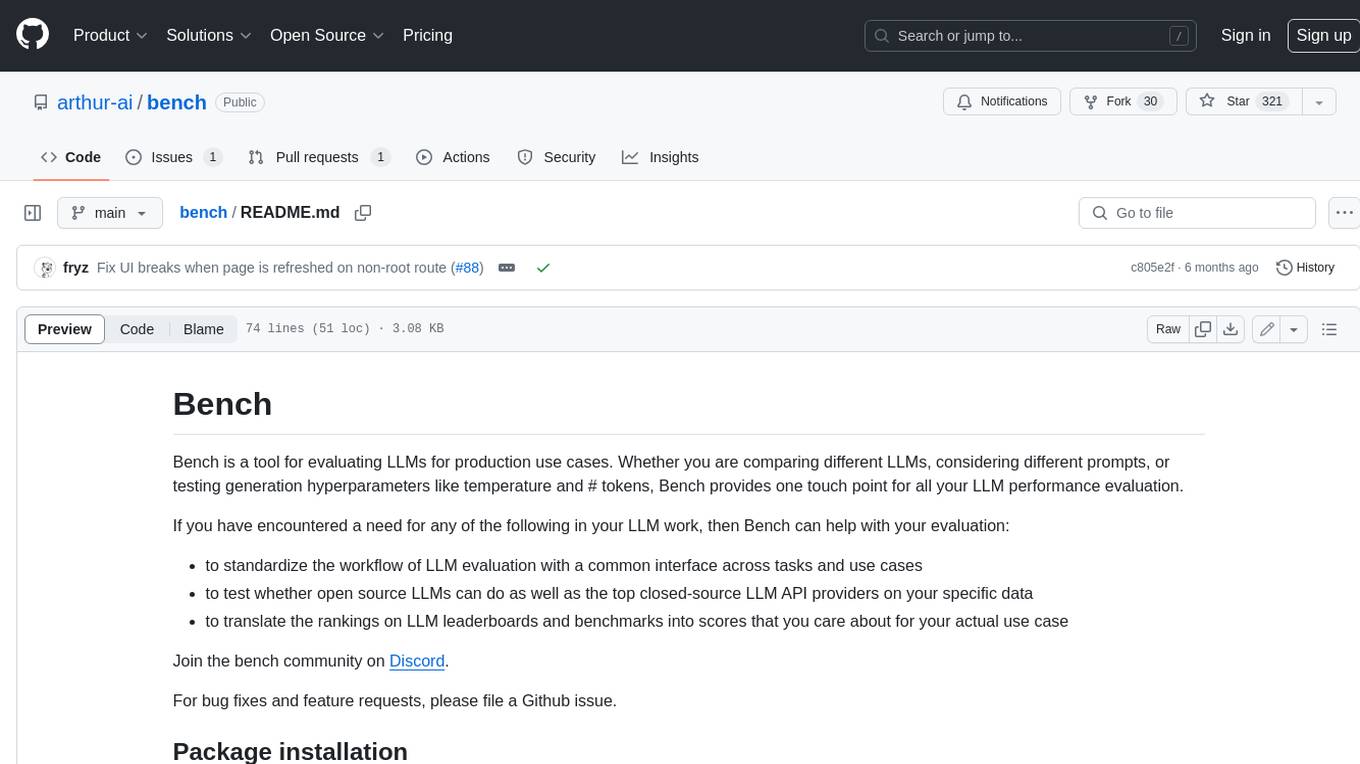
bench
Bench is a tool for evaluating LLMs for production use cases. It provides a standardized workflow for LLM evaluation with a common interface across tasks and use cases. Bench can be used to test whether open source LLMs can do as well as the top closed-source LLM API providers on specific data, and to translate the rankings on LLM leaderboards and benchmarks into scores that are relevant for actual use cases.
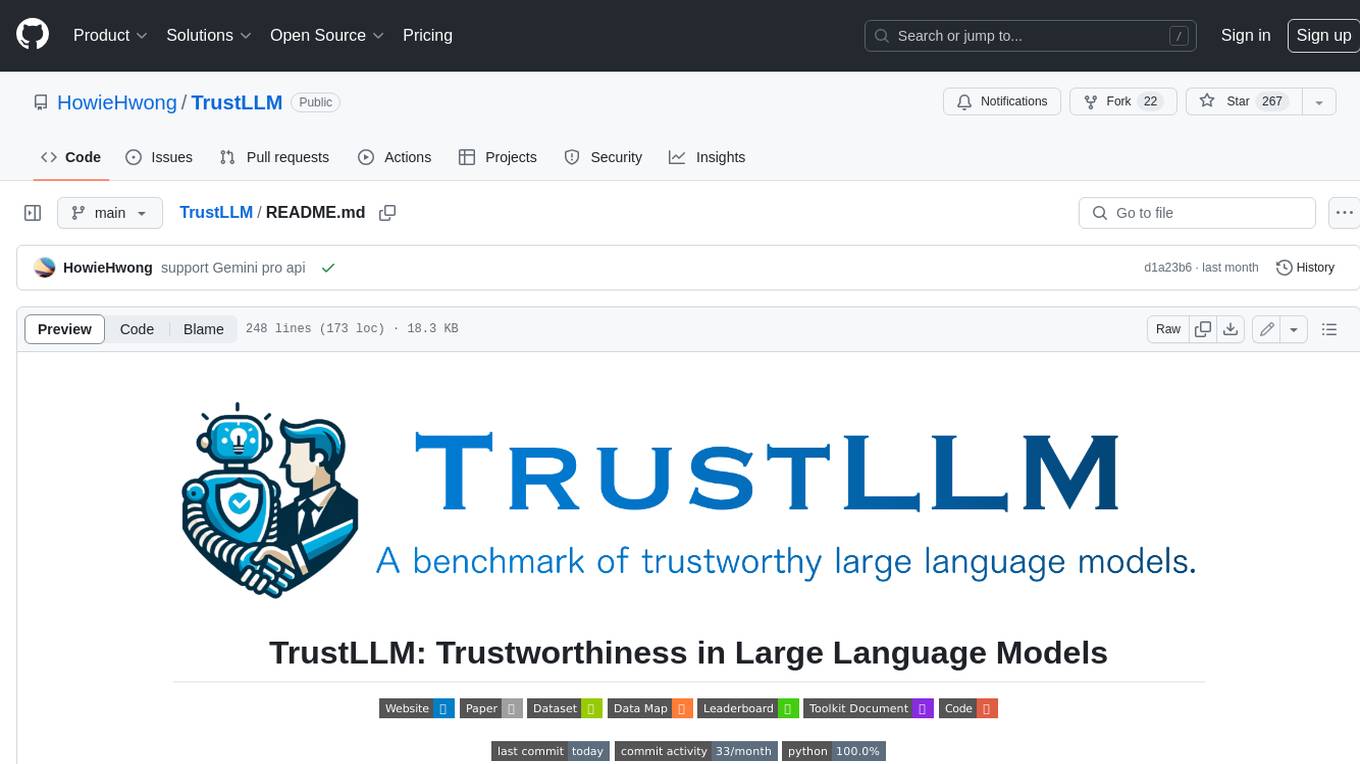
TrustLLM
TrustLLM is a comprehensive study of trustworthiness in LLMs, including principles for different dimensions of trustworthiness, established benchmark, evaluation, and analysis of trustworthiness for mainstream LLMs, and discussion of open challenges and future directions. Specifically, we first propose a set of principles for trustworthy LLMs that span eight different dimensions. Based on these principles, we further establish a benchmark across six dimensions including truthfulness, safety, fairness, robustness, privacy, and machine ethics. We then present a study evaluating 16 mainstream LLMs in TrustLLM, consisting of over 30 datasets. The document explains how to use the trustllm python package to help you assess the performance of your LLM in trustworthiness more quickly. For more details about TrustLLM, please refer to project website.
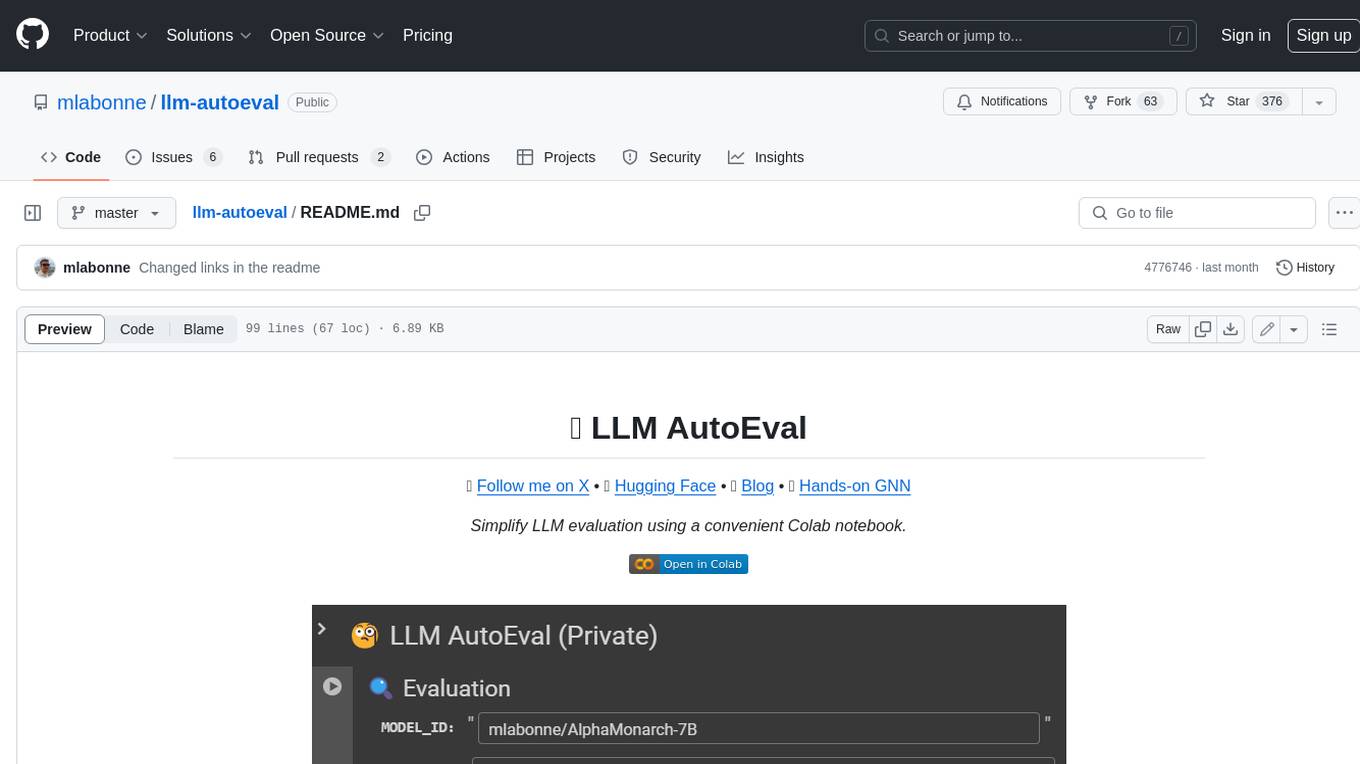
llm-autoeval
LLM AutoEval is a tool that simplifies the process of evaluating Large Language Models (LLMs) using a convenient Colab notebook. It automates the setup and execution of evaluations using RunPod, allowing users to customize evaluation parameters and generate summaries that can be uploaded to GitHub Gist for easy sharing and reference. LLM AutoEval supports various benchmark suites, including Nous, Lighteval, and Open LLM, enabling users to compare their results with existing models and leaderboards.
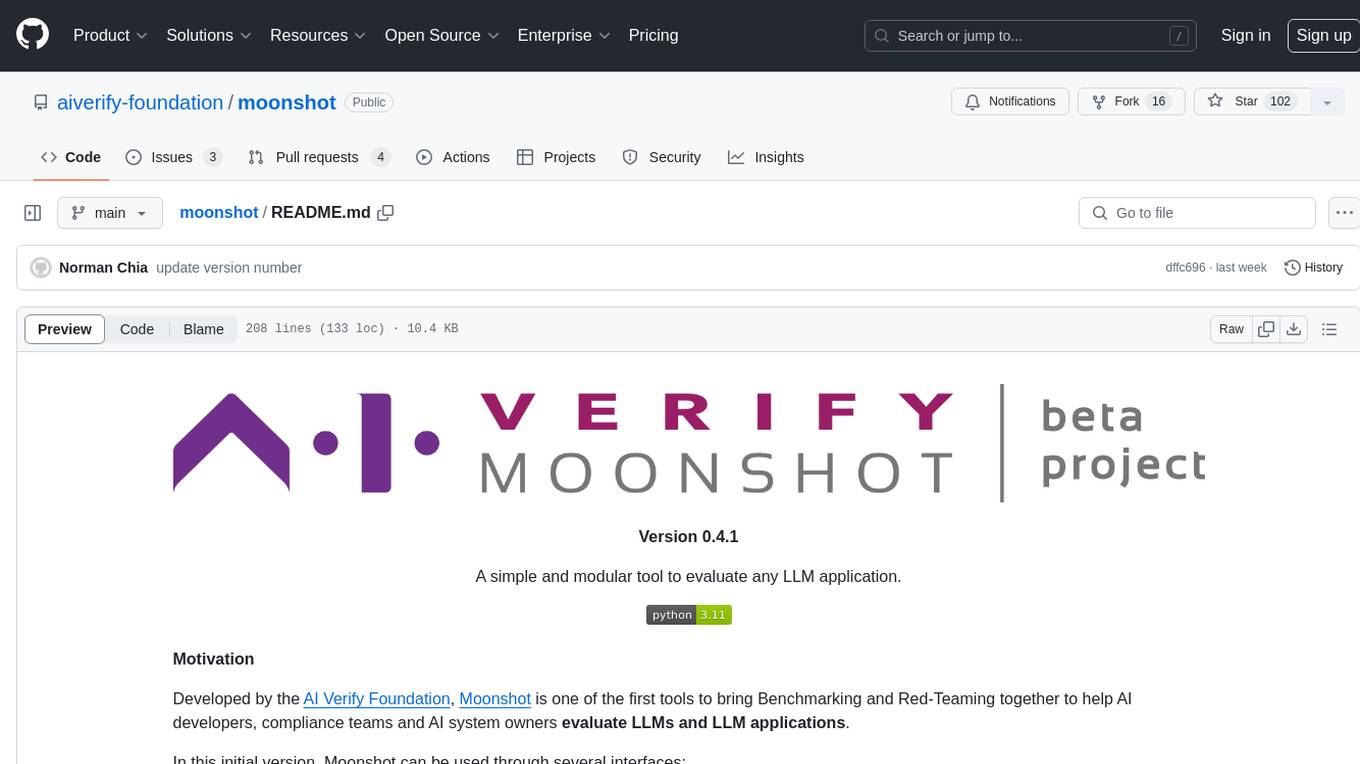
moonshot
Moonshot is a simple and modular tool developed by the AI Verify Foundation to evaluate Language Model Models (LLMs) and LLM applications. It brings Benchmarking and Red-Teaming together to assist AI developers, compliance teams, and AI system owners in assessing LLM performance. Moonshot can be accessed through various interfaces including User-friendly Web UI, Interactive Command Line Interface, and seamless integration into MLOps workflows via Library APIs or Web APIs. It offers features like benchmarking LLMs from popular model providers, running relevant tests, creating custom cookbooks and recipes, and automating Red Teaming to identify vulnerabilities in AI systems.
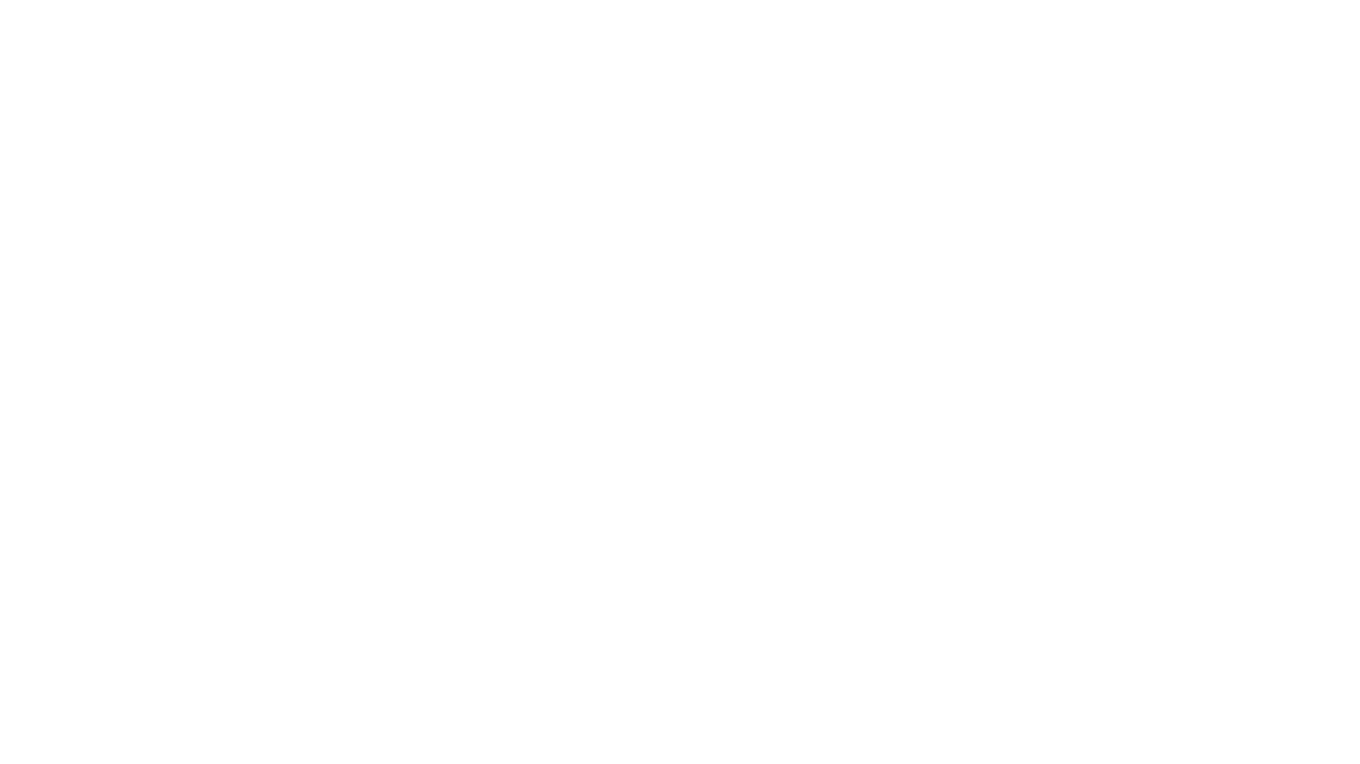
llm_client
llm_client is a Rust interface designed for Local Large Language Models (LLMs) that offers automated build support for CPU, CUDA, MacOS, easy model presets, and a novel cascading prompt workflow for controlled generation. It provides a breadth of configuration options and API support for various OpenAI compatible APIs. The tool is primarily focused on deterministic signals from probabilistic LLM vibes, enabling specialized workflows for specific tasks and reproducible outcomes.
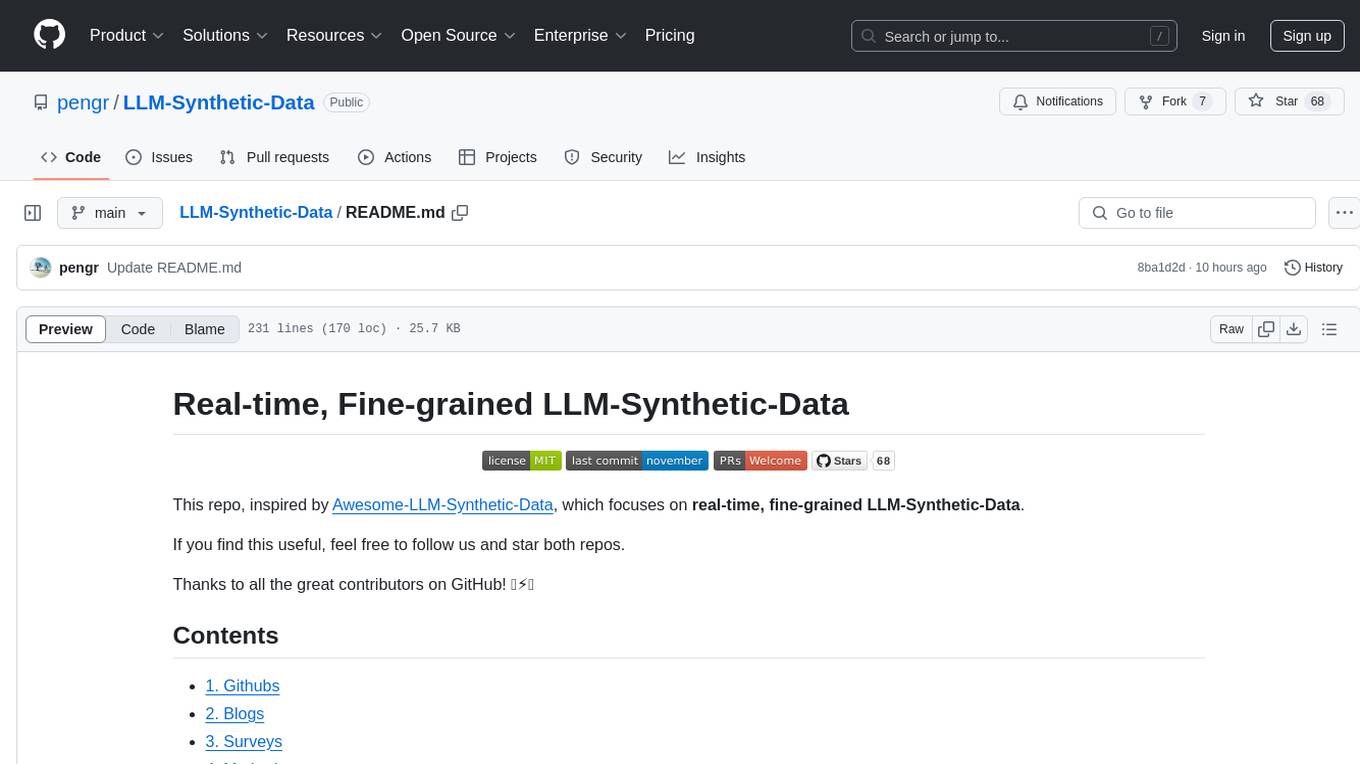
LLM-Synthetic-Data
LLM-Synthetic-Data is a repository focused on real-time, fine-grained LLM-Synthetic-Data generation. It includes methods, surveys, and application areas related to synthetic data for language models. The repository covers topics like pre-training, instruction tuning, model collapse, LLM benchmarking, evaluation, and distillation. It also explores application areas such as mathematical reasoning, code generation, text-to-SQL, alignment, reward modeling, long context, weak-to-strong generalization, agent and tool use, vision and language, factuality, federated learning, generative design, and safety.
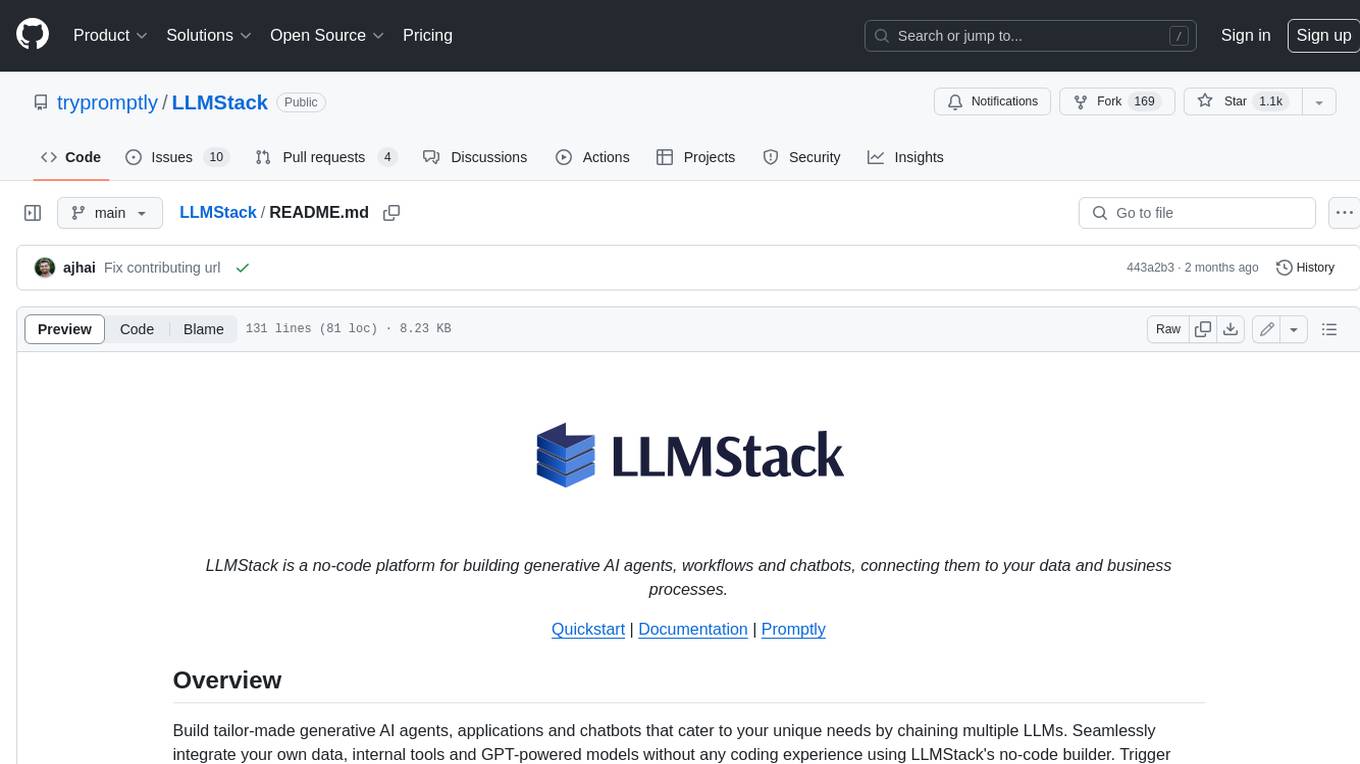
LLMStack
LLMStack is a no-code platform for building generative AI agents, workflows, and chatbots. It allows users to connect their own data, internal tools, and GPT-powered models without any coding experience. LLMStack can be deployed to the cloud or on-premise and can be accessed via HTTP API or triggered from Slack or Discord.
For similar jobs
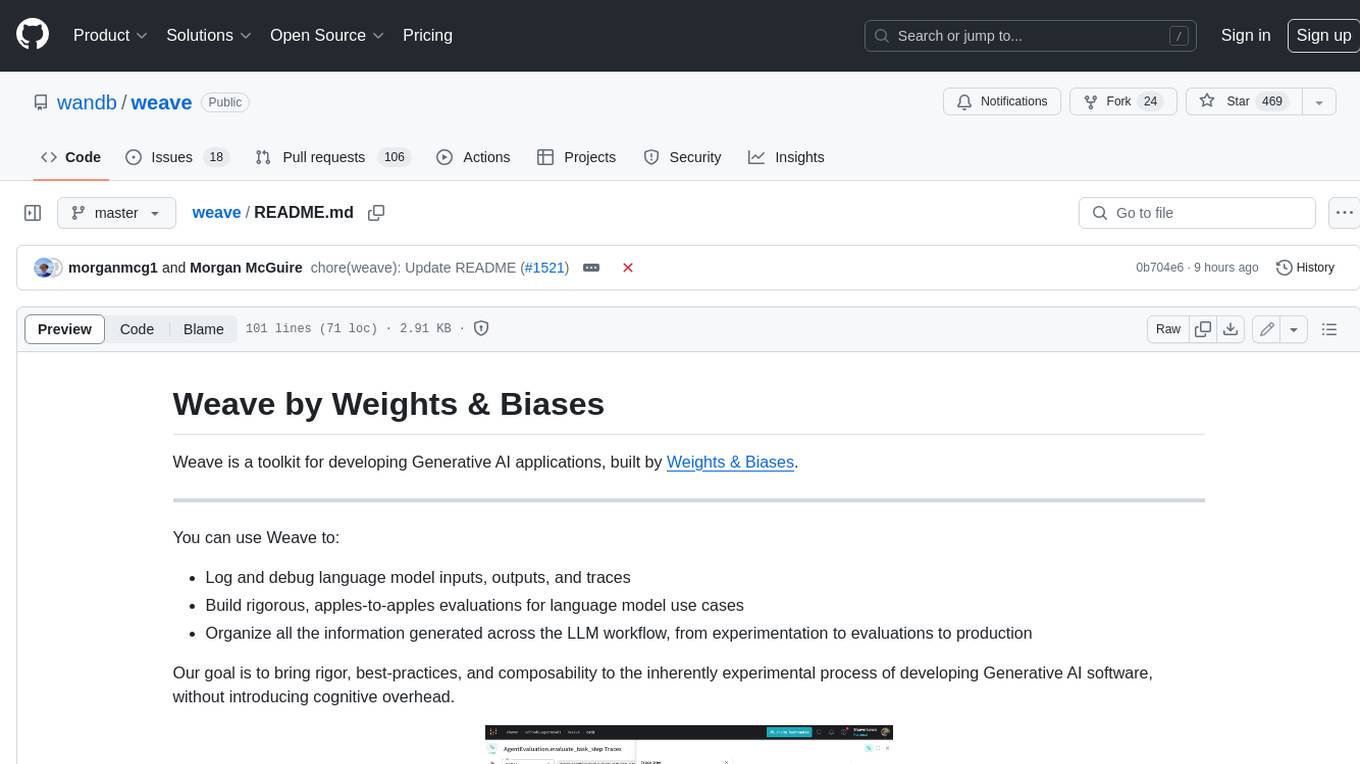
weave
Weave is a toolkit for developing Generative AI applications, built by Weights & Biases. With Weave, you can log and debug language model inputs, outputs, and traces; build rigorous, apples-to-apples evaluations for language model use cases; and organize all the information generated across the LLM workflow, from experimentation to evaluations to production. Weave aims to bring rigor, best-practices, and composability to the inherently experimental process of developing Generative AI software, without introducing cognitive overhead.
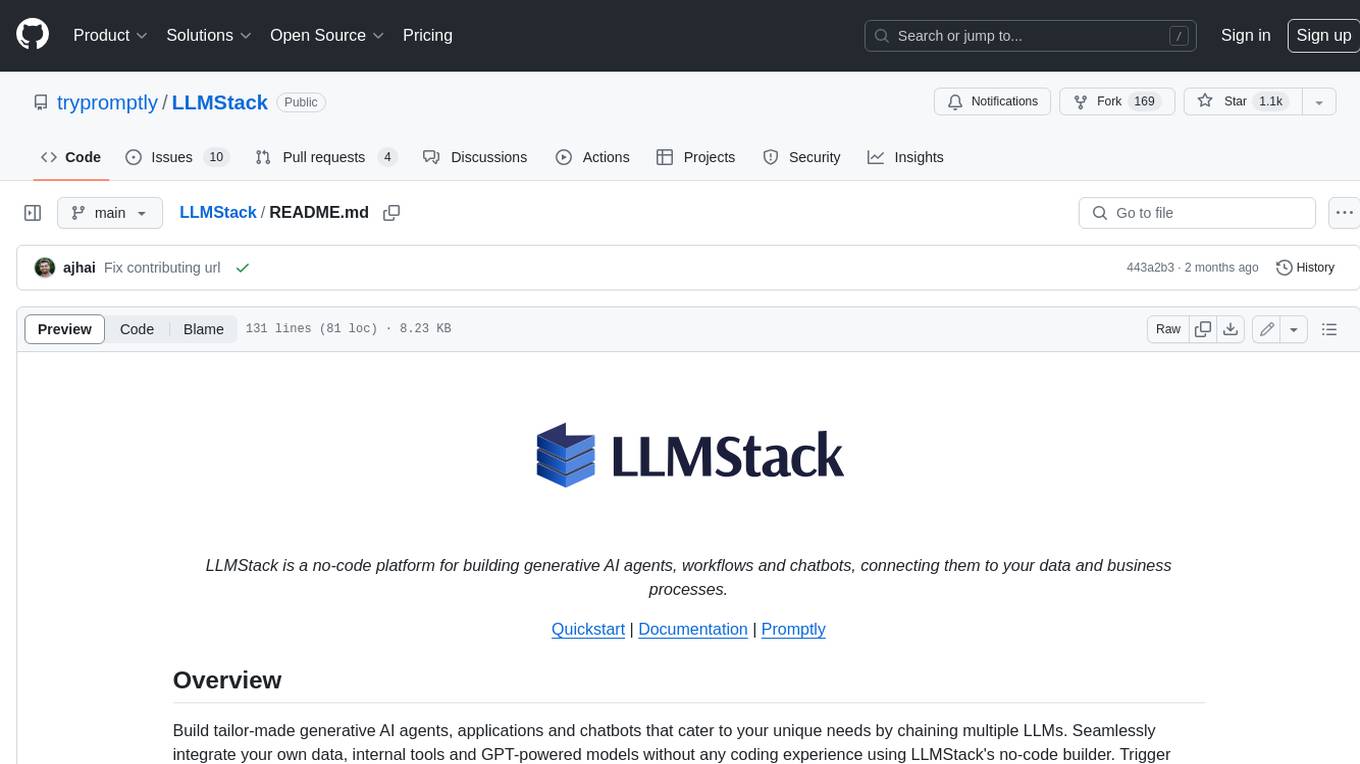
LLMStack
LLMStack is a no-code platform for building generative AI agents, workflows, and chatbots. It allows users to connect their own data, internal tools, and GPT-powered models without any coding experience. LLMStack can be deployed to the cloud or on-premise and can be accessed via HTTP API or triggered from Slack or Discord.
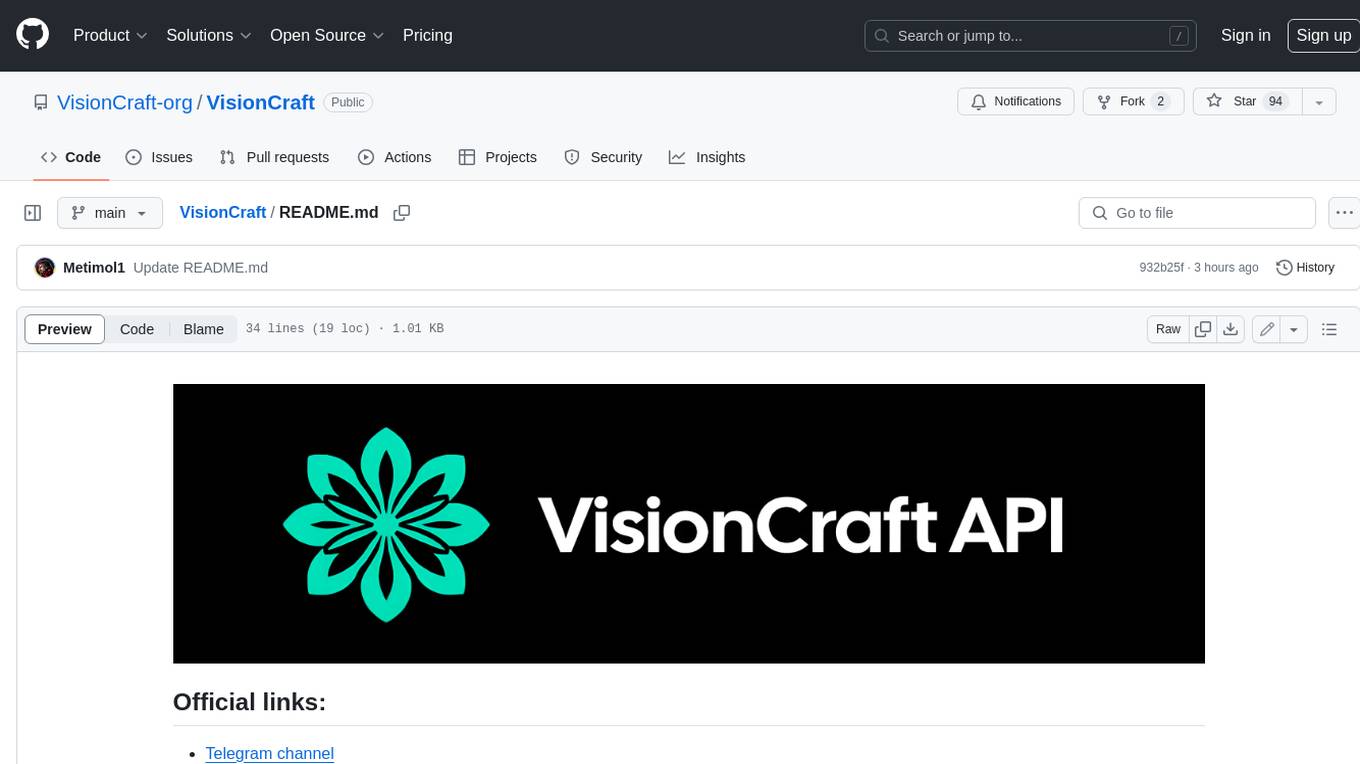
VisionCraft
The VisionCraft API is a free API for using over 100 different AI models. From images to sound.
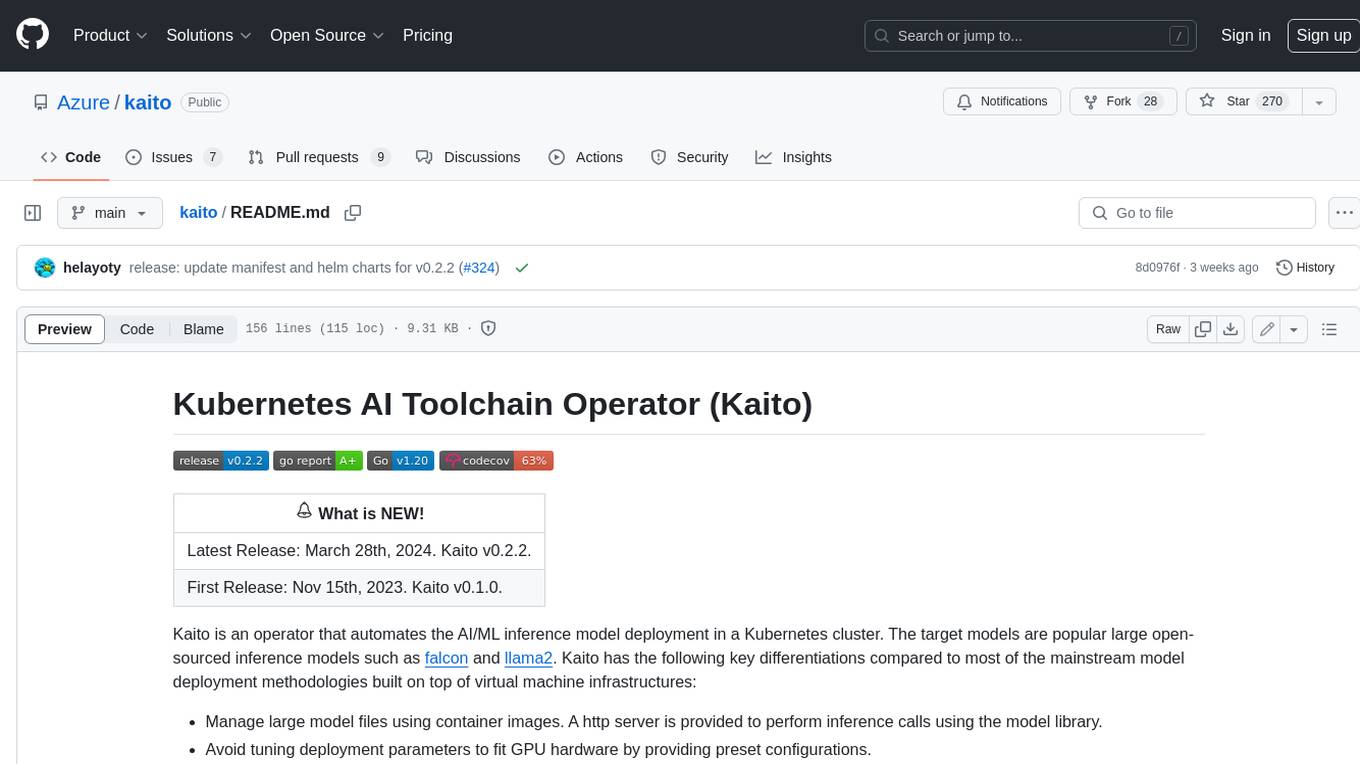
kaito
Kaito is an operator that automates the AI/ML inference model deployment in a Kubernetes cluster. It manages large model files using container images, avoids tuning deployment parameters to fit GPU hardware by providing preset configurations, auto-provisions GPU nodes based on model requirements, and hosts large model images in the public Microsoft Container Registry (MCR) if the license allows. Using Kaito, the workflow of onboarding large AI inference models in Kubernetes is largely simplified.
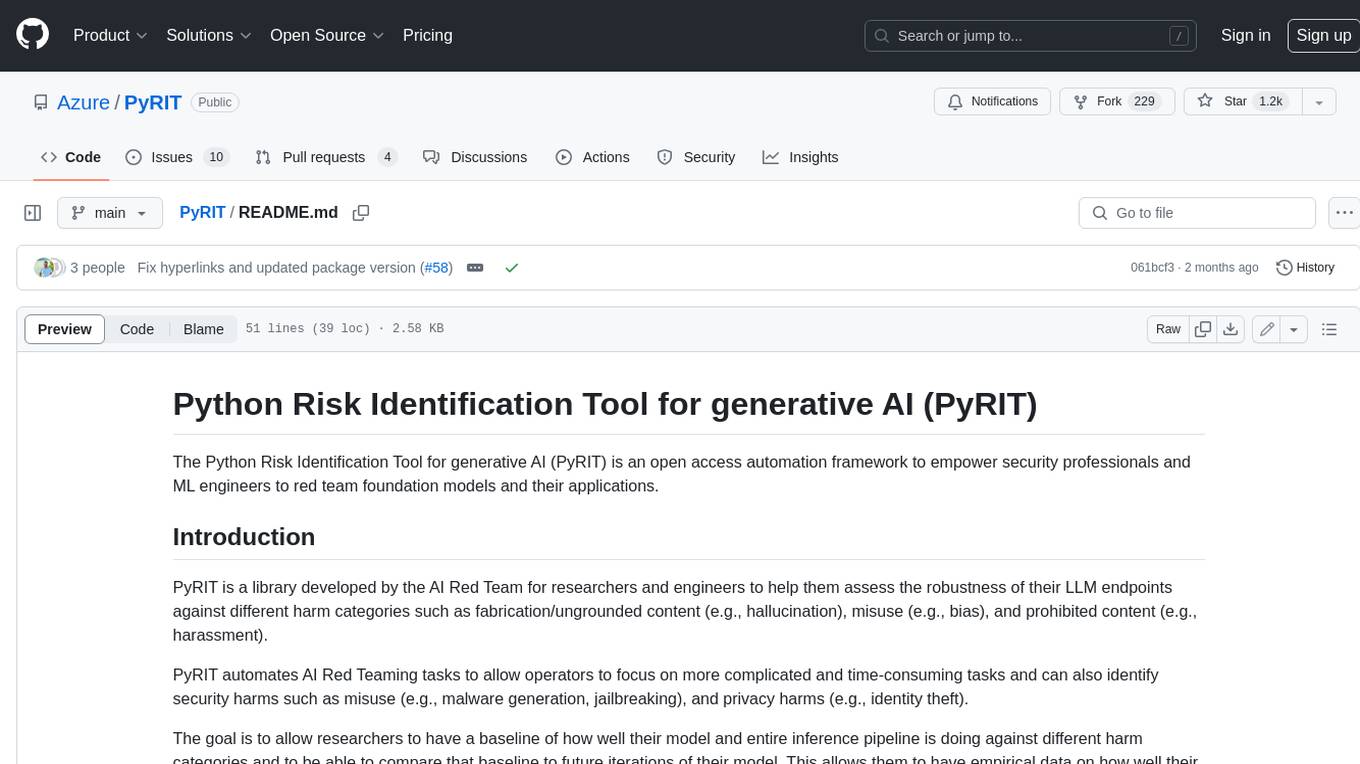
PyRIT
PyRIT is an open access automation framework designed to empower security professionals and ML engineers to red team foundation models and their applications. It automates AI Red Teaming tasks to allow operators to focus on more complicated and time-consuming tasks and can also identify security harms such as misuse (e.g., malware generation, jailbreaking), and privacy harms (e.g., identity theft). The goal is to allow researchers to have a baseline of how well their model and entire inference pipeline is doing against different harm categories and to be able to compare that baseline to future iterations of their model. This allows them to have empirical data on how well their model is doing today, and detect any degradation of performance based on future improvements.
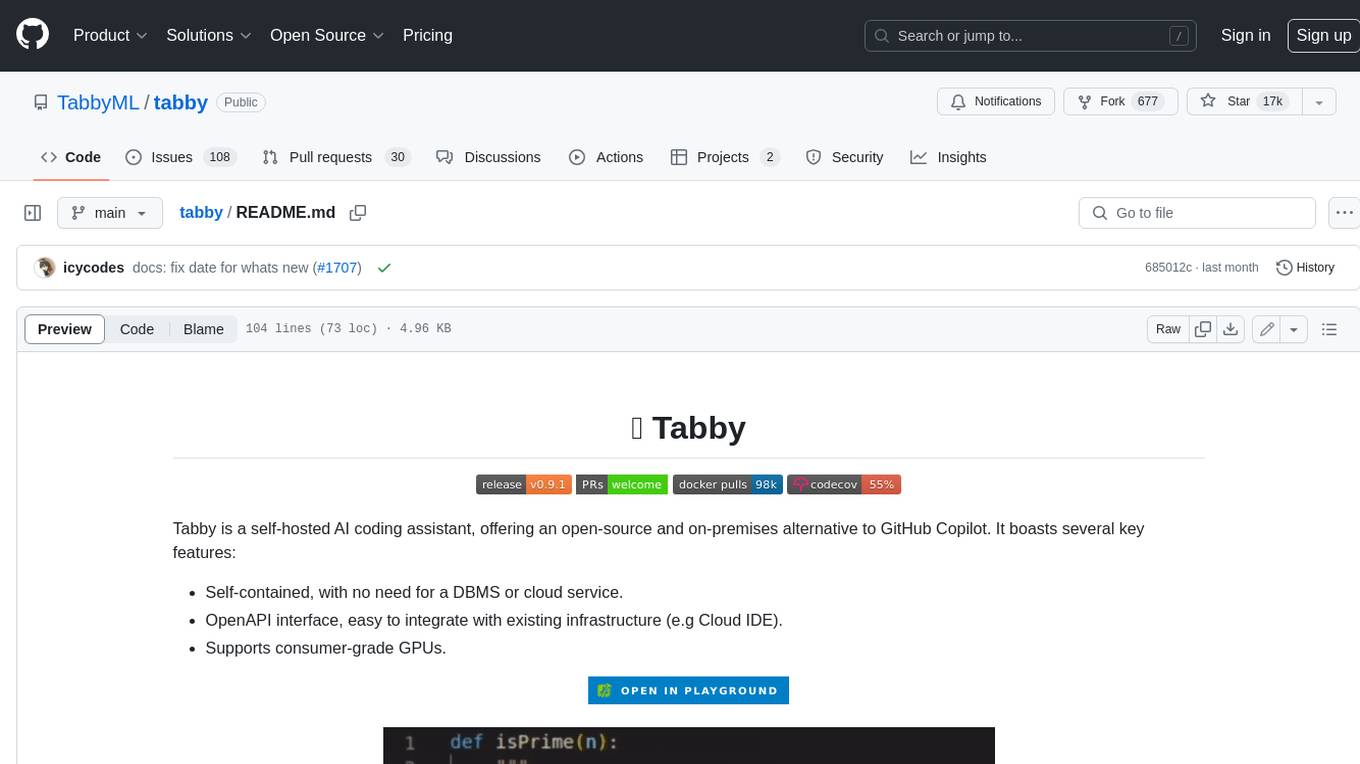
tabby
Tabby is a self-hosted AI coding assistant, offering an open-source and on-premises alternative to GitHub Copilot. It boasts several key features: * Self-contained, with no need for a DBMS or cloud service. * OpenAPI interface, easy to integrate with existing infrastructure (e.g Cloud IDE). * Supports consumer-grade GPUs.
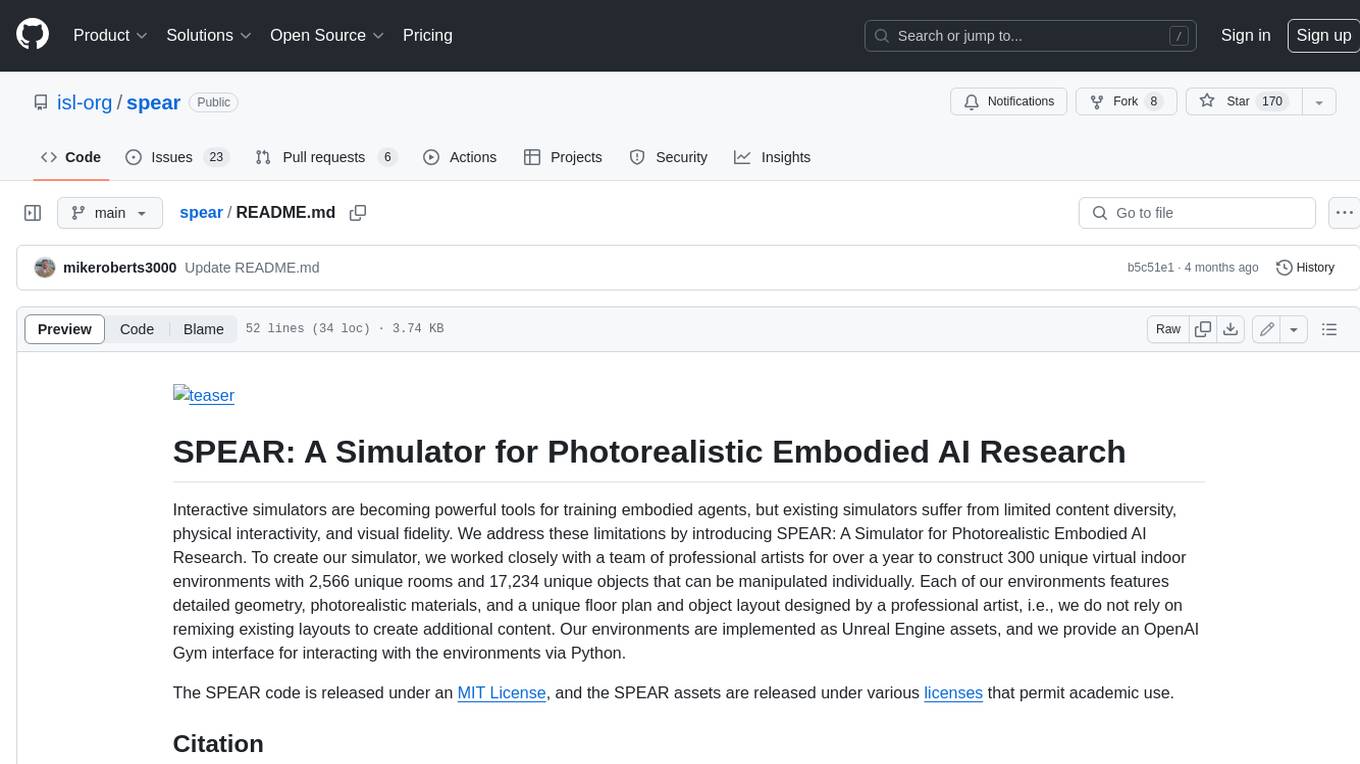
spear
SPEAR (Simulator for Photorealistic Embodied AI Research) is a powerful tool for training embodied agents. It features 300 unique virtual indoor environments with 2,566 unique rooms and 17,234 unique objects that can be manipulated individually. Each environment is designed by a professional artist and features detailed geometry, photorealistic materials, and a unique floor plan and object layout. SPEAR is implemented as Unreal Engine assets and provides an OpenAI Gym interface for interacting with the environments via Python.
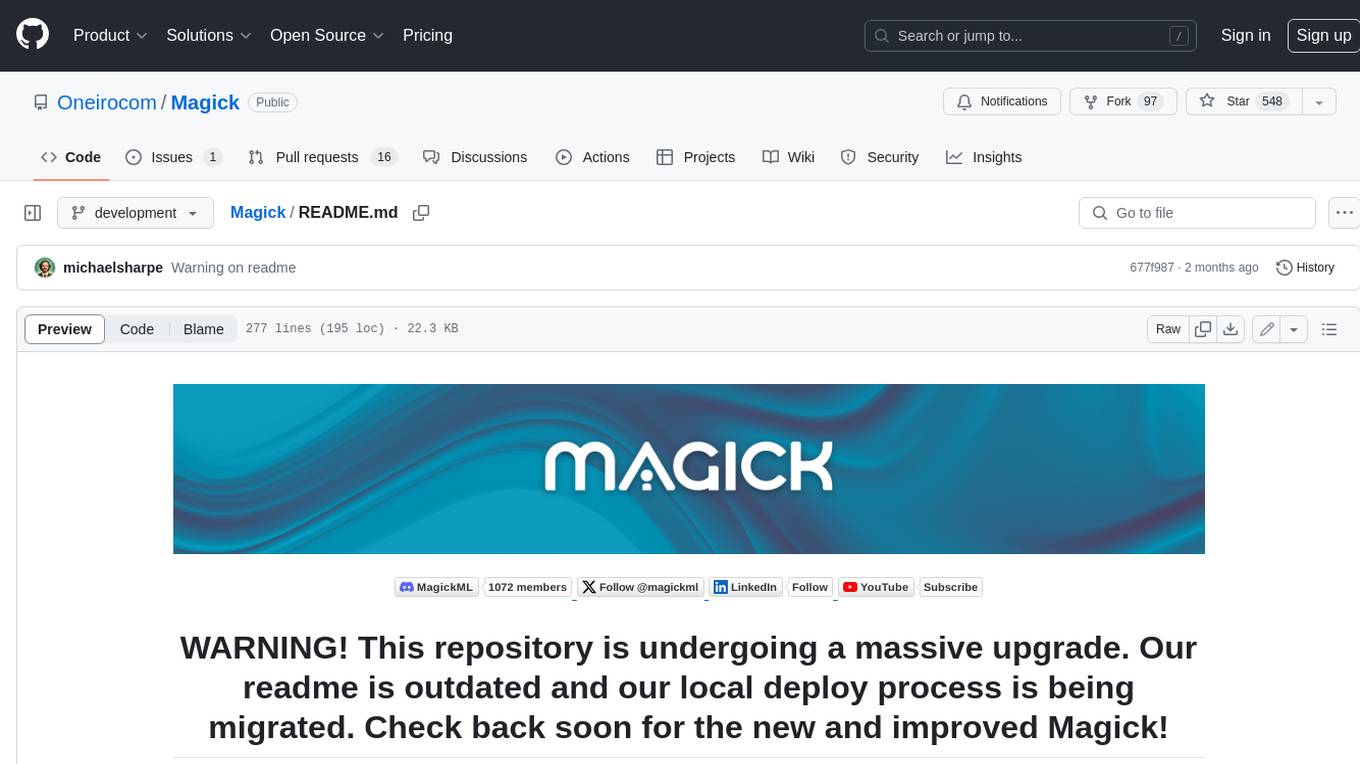
Magick
Magick is a groundbreaking visual AIDE (Artificial Intelligence Development Environment) for no-code data pipelines and multimodal agents. Magick can connect to other services and comes with nodes and templates well-suited for intelligent agents, chatbots, complex reasoning systems and realistic characters.