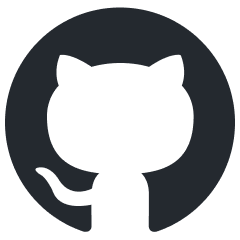
bionemo-framework
BioNeMo Framework: For building and adapting AI models in drug discovery at scale
Stars: 363
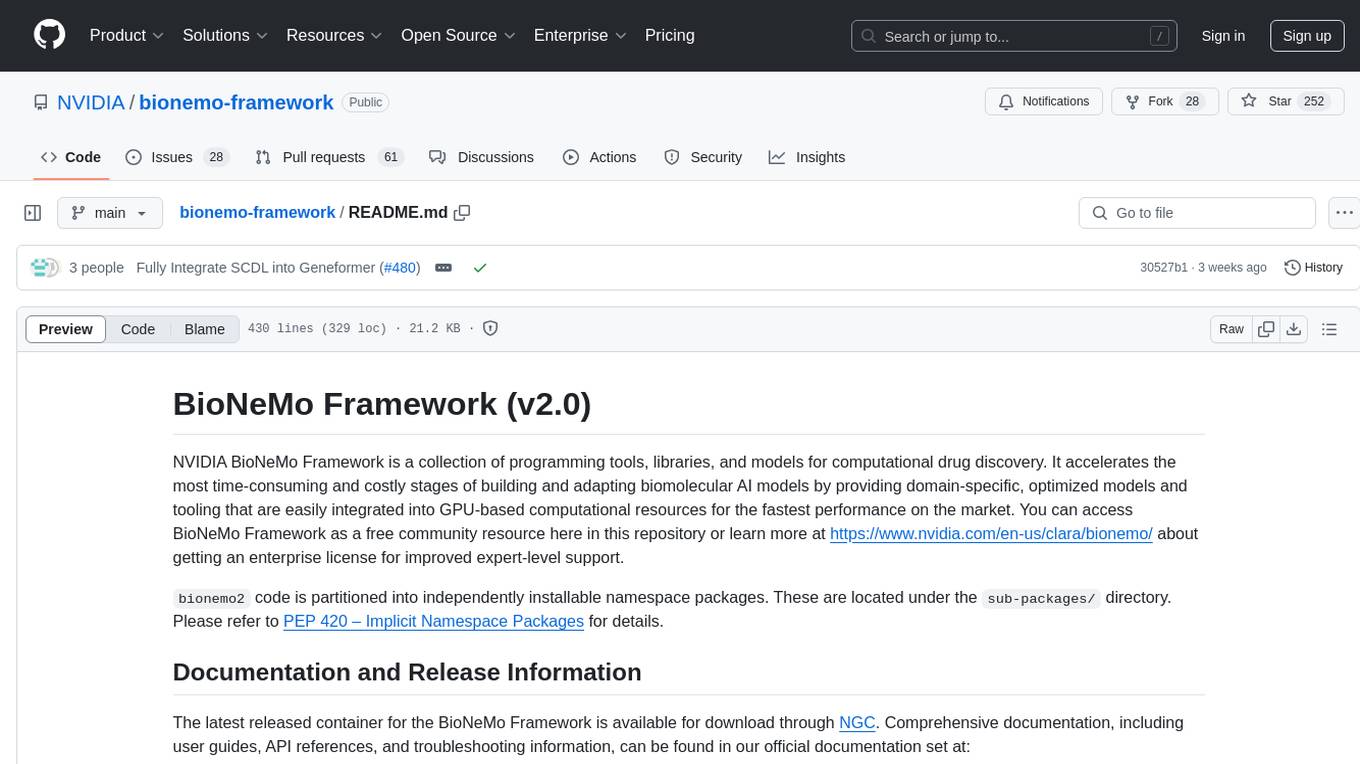
NVIDIA BioNeMo Framework is a collection of programming tools, libraries, and models for computational drug discovery. It accelerates building and adapting biomolecular AI models by providing domain-specific, optimized models and tooling for GPU-based computational resources. The framework offers comprehensive documentation and support for both community and enterprise users.
README:
NVIDIA BioNeMo Framework is a is a comprehensive suite of programming tools, libraries, and models designed for computational drug discovery. It accelerates the most time-consuming and costly stages of building and adapting biomolecular AI models by providing domain-specific, optimized models and tooling that are easily integrated into GPU-based computational resources for the fastest performance on the market. You can access BioNeMo Framework as a free community resource here in this repository or learn more at https://www.nvidia.com/en-us/clara/bionemo/ about getting an enterprise license for improved expert-level support.
The bionemo-framework
is organized into independently installable namespace packages. These are located under the
sub-packages/
directory. Please refer to PEP 420 – Implicit Namespace Packages
for details.
- Official Documentation: For user guides, API references, and troubleshooting, visit our official documentation.
-
In-Progress Documentation: To explore the latest features and developments, check the documentation reflecting the current state of the
main
branch here. Note that this may include references to features or APIs that are not yet finalized.
Full documentation on using the BioNeMo Framework is provided in our documentation:
https://docs.nvidia.com/bionemo-framework/latest/user-guide/. To simplify the integration of optimized third-party dependencies, BioNeMo is primarily distributed as a containerized library. You can download the latest released container for the BioNeMo Framework from
NGC. To launch a pre-built container, you can use the brev.dev launchable or execute the following command:
docker run --rm -it \
--gpus=all --ipc=host --ulimit memlock=-1 --ulimit stack=67108864 \
nvcr.io/nvidia/clara/bionemo-framework:nightly \
/bin/bash
The NeMo and Megatron-LM dependencies are included as git submodules in bionemo2. The pinned commits for these submodules represent the "last-known-good" versions of these packages that are confirmed to be working with bionemo2 (and those that are tested in CI).
To initialize these sub-modules when cloning the repo, add the --recursive
flag to the git clone command:
git clone --recursive [email protected]:NVIDIA/bionemo-framework.git
cd bionemo-framework
To download the pinned versions of these submodules within an existing git repository, run
git submodule update --init --recursive
Different branches of the repo can have different pinned versions of these third-party submodules. Ensure submodules are automatically updated after switching branches or pulling updates by configuring git with:
git config submodule.recurse true
NOTE: this setting will not download new or remove old submodules with the branch's changes.
You will have to run the full git submodule update --init --recursive
command in these situations.
With a locally cloned repository and initialized submodules, build the BioNeMo container using:
docker buildx build . -t my-container-tag
We distribute a development container configuration for vscode
(.devcontainer/devcontainer.json
) that simplifies the process of local testing and development. Opening the
bionemo-framework folder with VSCode should prompt you to re-open the folder inside the devcontainer environment.
[!NOTE] The first time you launch the devcontainer, it may take a long time to build the image. Building the image locally (using the command shown above) will ensure that most of the layers are present in the local docker cache.
See the tutorials pages for example applications and getting started guides.
For Tasks:
Click tags to check more tools for each tasksFor Jobs:
Alternative AI tools for bionemo-framework
Similar Open Source Tools
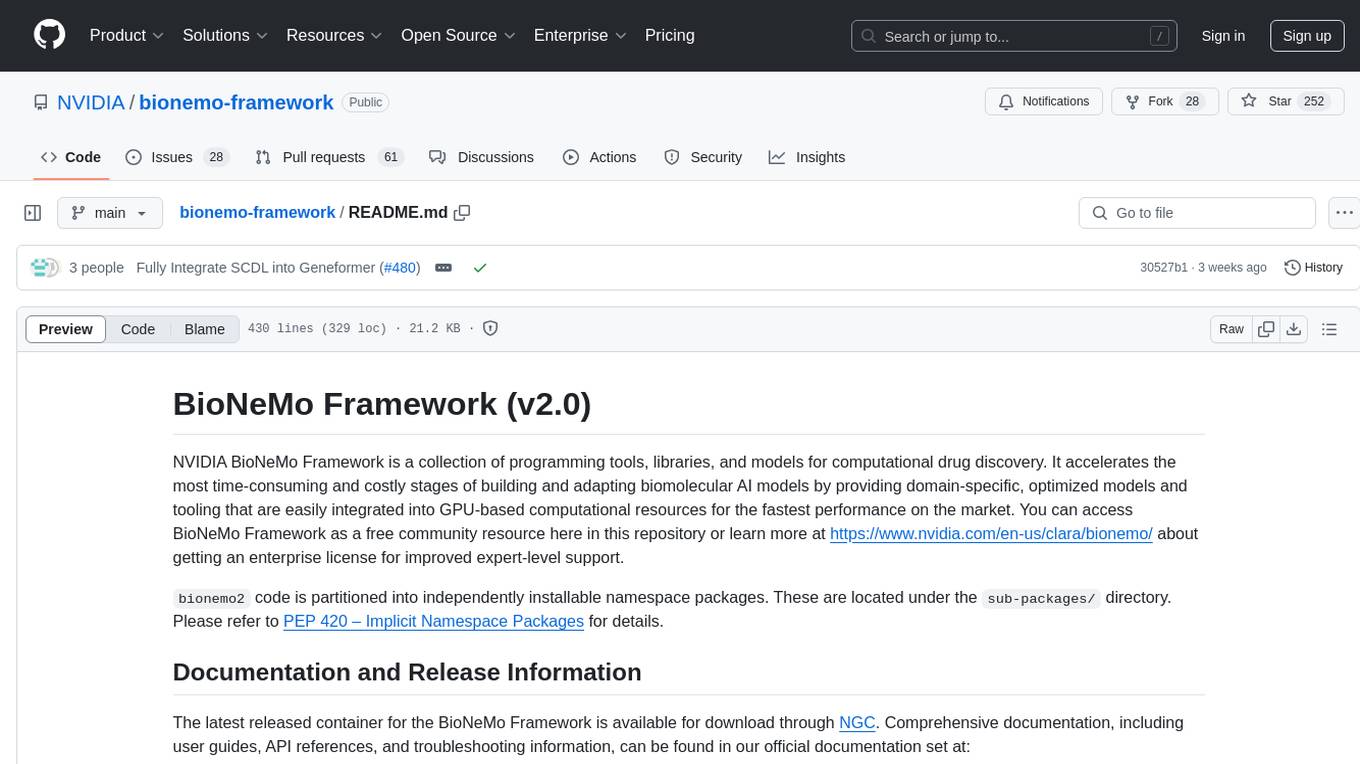
bionemo-framework
NVIDIA BioNeMo Framework is a collection of programming tools, libraries, and models for computational drug discovery. It accelerates building and adapting biomolecular AI models by providing domain-specific, optimized models and tooling for GPU-based computational resources. The framework offers comprehensive documentation and support for both community and enterprise users.
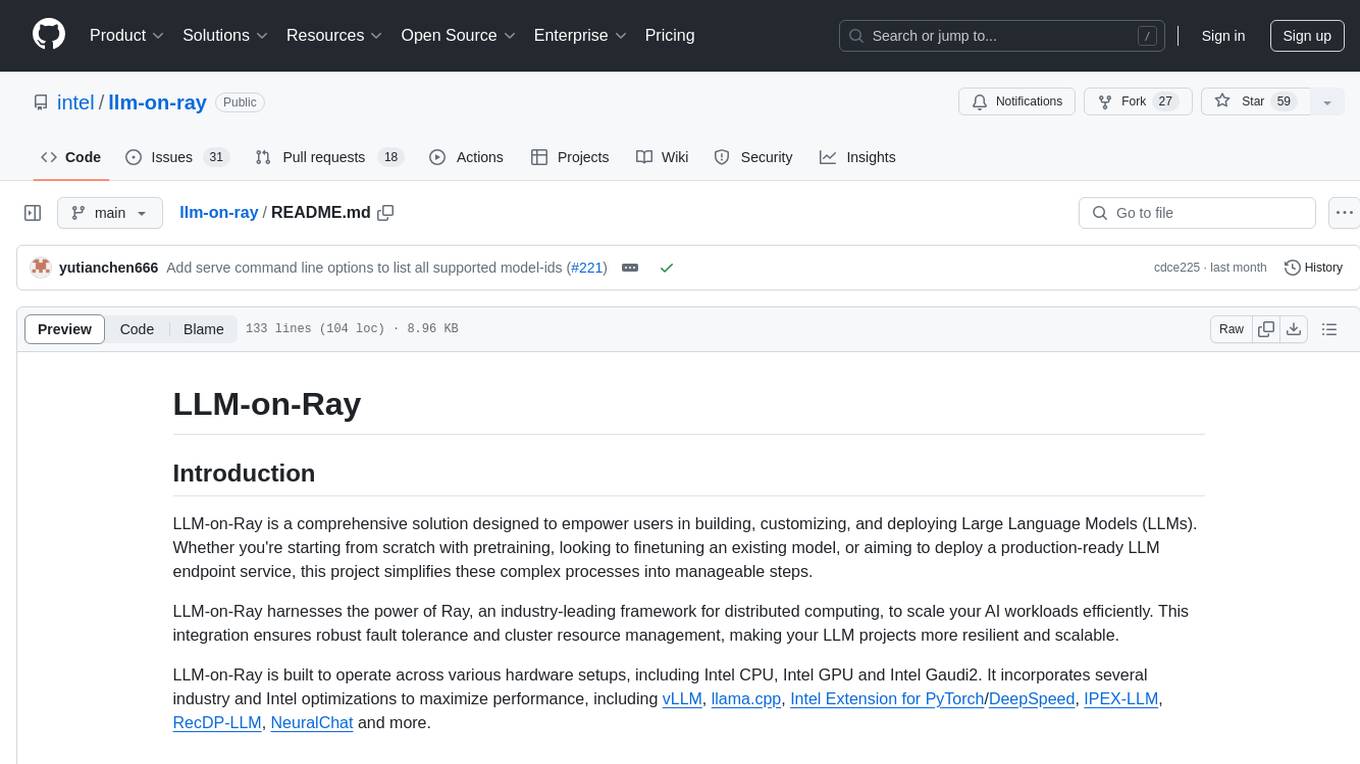
llm-on-ray
LLM-on-Ray is a comprehensive solution for building, customizing, and deploying Large Language Models (LLMs). It simplifies complex processes into manageable steps by leveraging the power of Ray for distributed computing. The tool supports pretraining, finetuning, and serving LLMs across various hardware setups, incorporating industry and Intel optimizations for performance. It offers modular workflows with intuitive configurations, robust fault tolerance, and scalability. Additionally, it provides an Interactive Web UI for enhanced usability, including a chatbot application for testing and refining models.
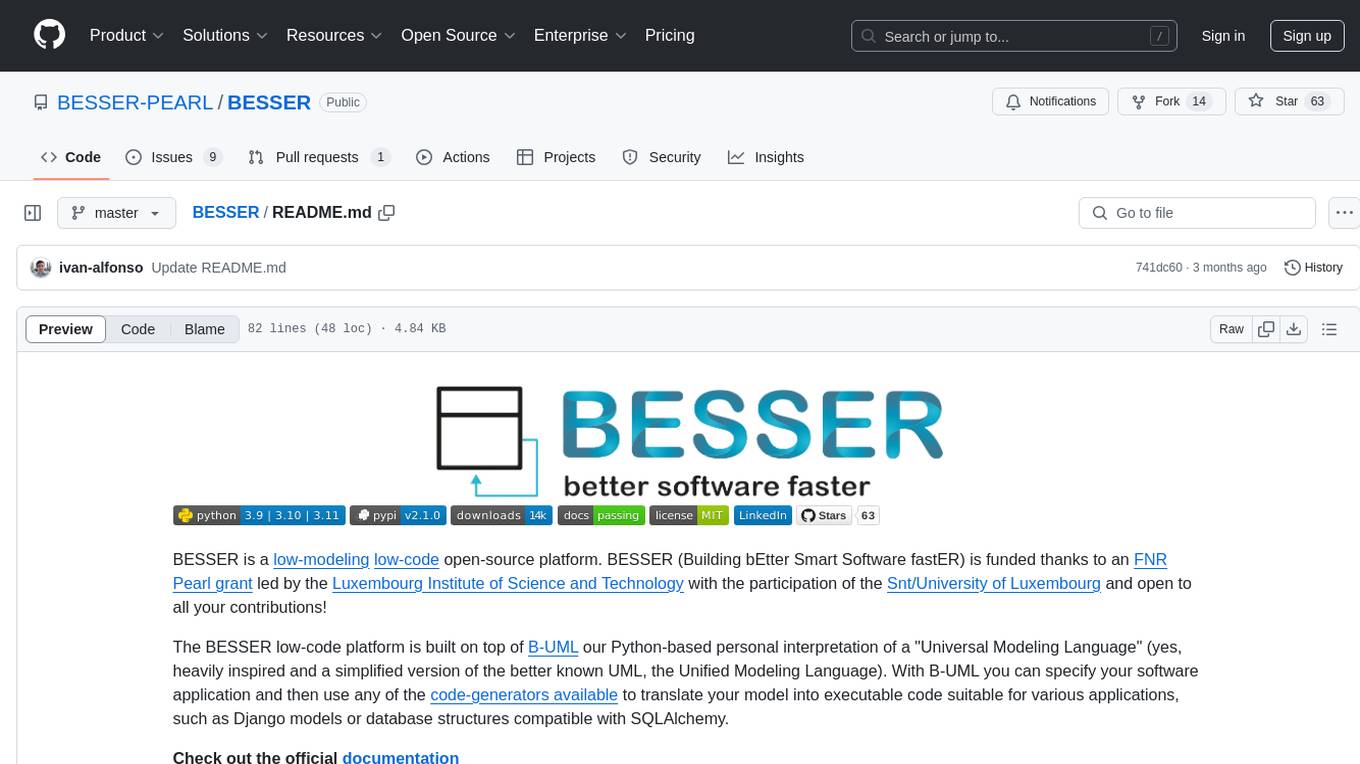
BESSER
BESSER is a low-modeling low-code open-source platform funded by an FNR Pearl grant. It is built on B-UML, a Python-based interpretation of a 'Universal Modeling Language'. Users can specify their software application using B-UML and generate executable code for various applications like Django models or SQLAlchemy-compatible database structures. BESSER is available on PyPi and can be installed with pip. It supports popular Python IDEs and encourages contributions from the community.
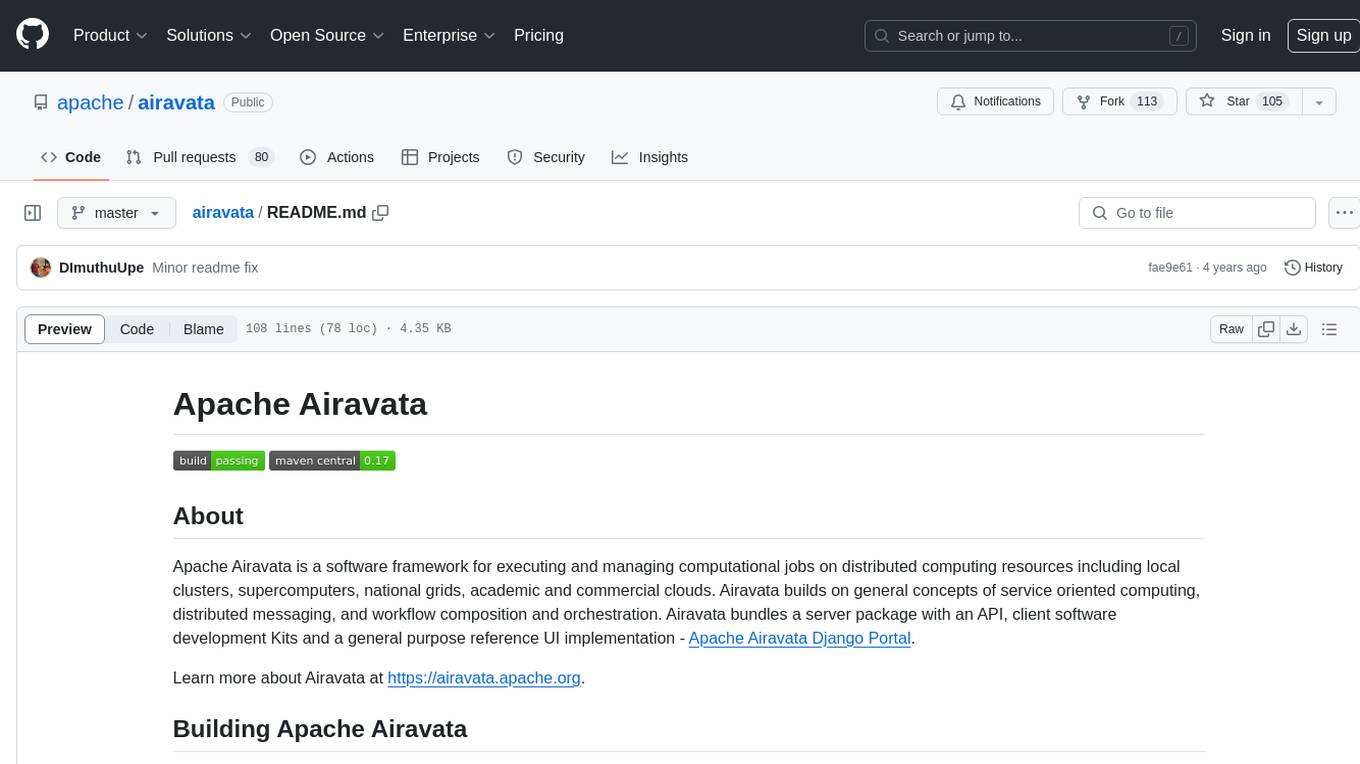
airavata
Apache Airavata is a software framework for executing and managing computational jobs on distributed computing resources. It supports local clusters, supercomputers, national grids, academic and commercial clouds. Airavata utilizes service-oriented computing, distributed messaging, and workflow composition. It includes a server package with an API, client SDKs, and a general-purpose UI implementation called Apache Airavata Django Portal.
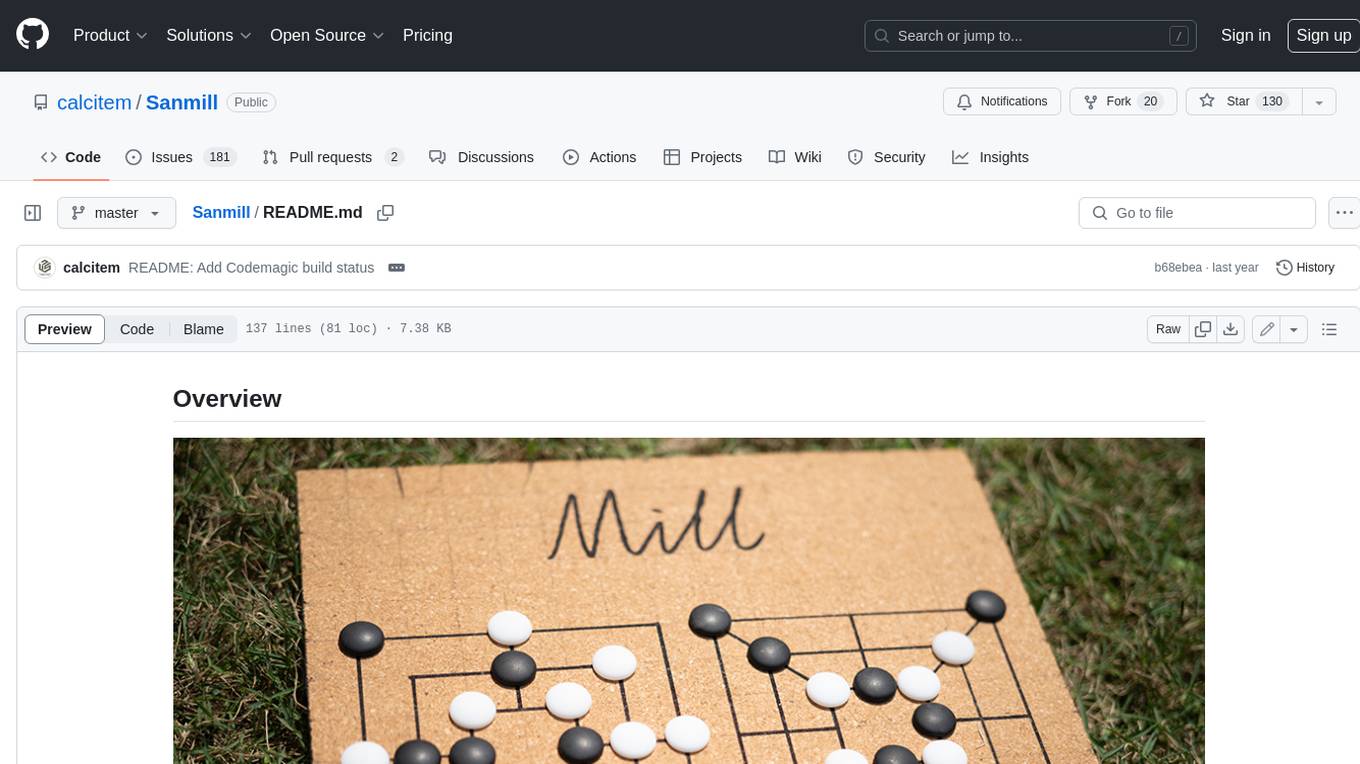
Sanmill
Sanmill is a free, powerful UCI-like N men's morris program with CUI, Flutter GUI and Qt GUI. Nine men's morris is a strategy board game for two players dating at least to the Roman Empire. The game is also known as nine-man morris , mill , mills , the mill game , merels , merrills , merelles , marelles , morelles , and ninepenny marl in English.
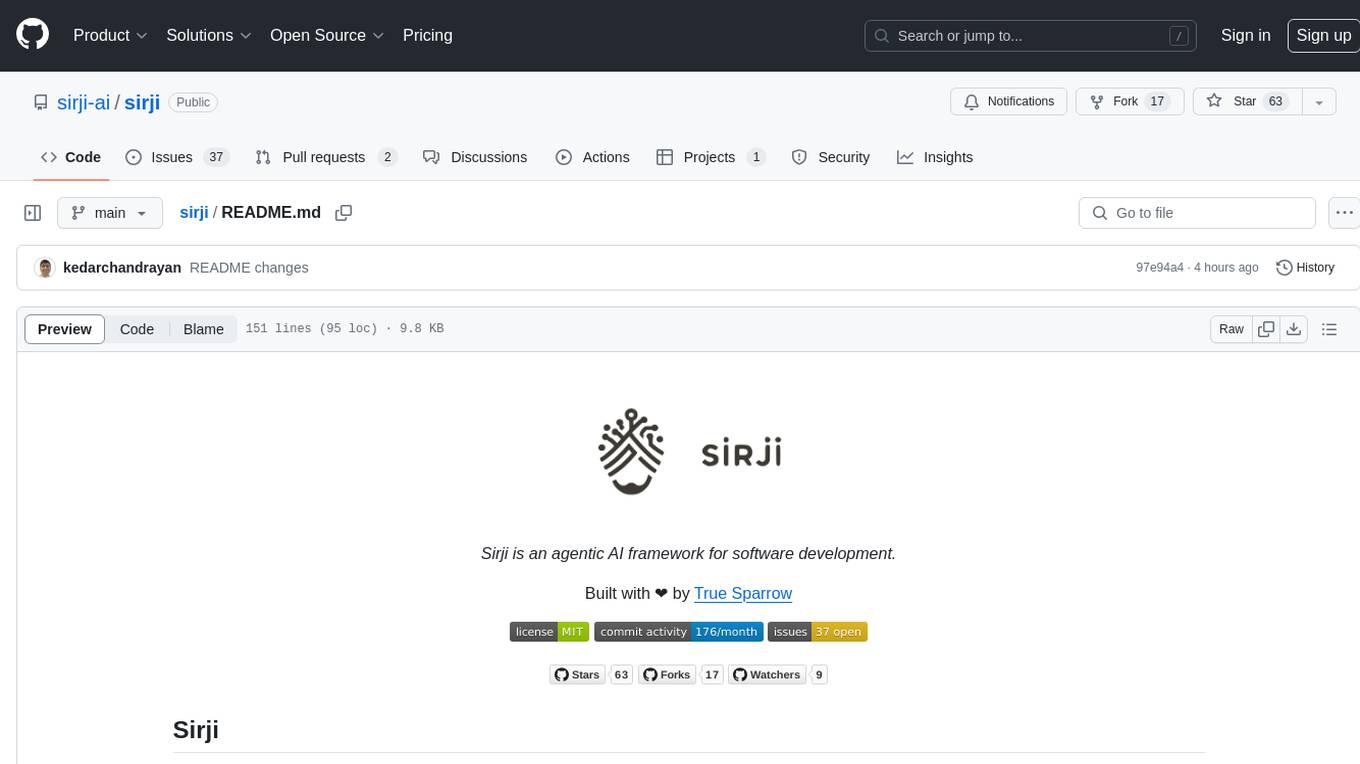
sirji
Sirji is an agentic AI framework for software development where various AI agents collaborate via a messaging protocol to solve software problems. It uses standard or user-generated recipes to list tasks and tips for problem-solving. Agents in Sirji are modular AI components that perform specific tasks based on custom pseudo code. The framework is currently implemented as a Visual Studio Code extension, providing an interactive chat interface for problem submission and feedback. Sirji sets up local or remote development environments by installing dependencies and executing generated code.
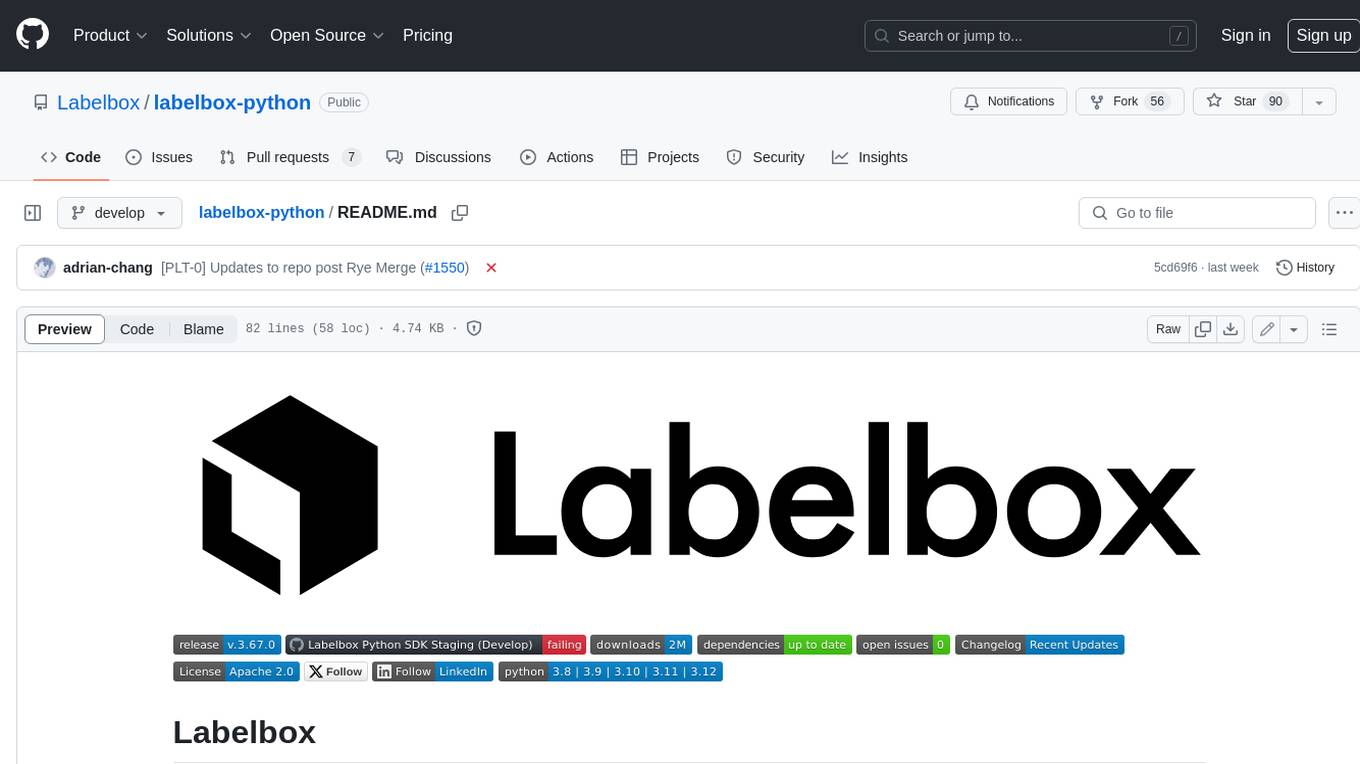
labelbox-python
Labelbox is a data-centric AI platform for enterprises to develop, optimize, and use AI to solve problems and power new products and services. Enterprises use Labelbox to curate data, generate high-quality human feedback data for computer vision and LLMs, evaluate model performance, and automate tasks by combining AI and human-centric workflows. The academic & research community uses Labelbox for cutting-edge AI research.
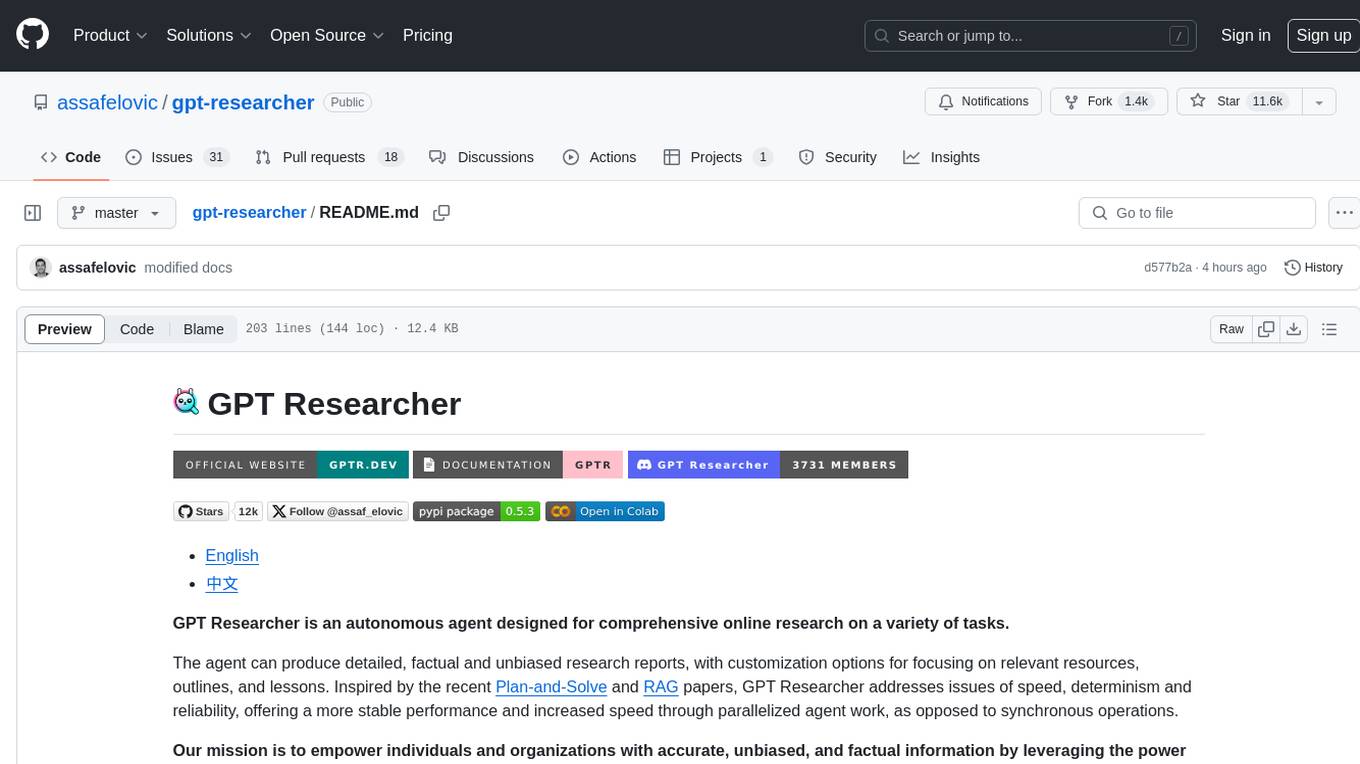
gpt-researcher
GPT Researcher is an autonomous agent designed for comprehensive online research on a variety of tasks. It can produce detailed, factual, and unbiased research reports with customization options. The tool addresses issues of speed, determinism, and reliability by leveraging parallelized agent work. The main idea involves running 'planner' and 'execution' agents to generate research questions, seek related information, and create research reports. GPT Researcher optimizes costs and completes tasks in around 3 minutes. Features include generating long research reports, aggregating web sources, an easy-to-use web interface, scraping web sources, and exporting reports to various formats.
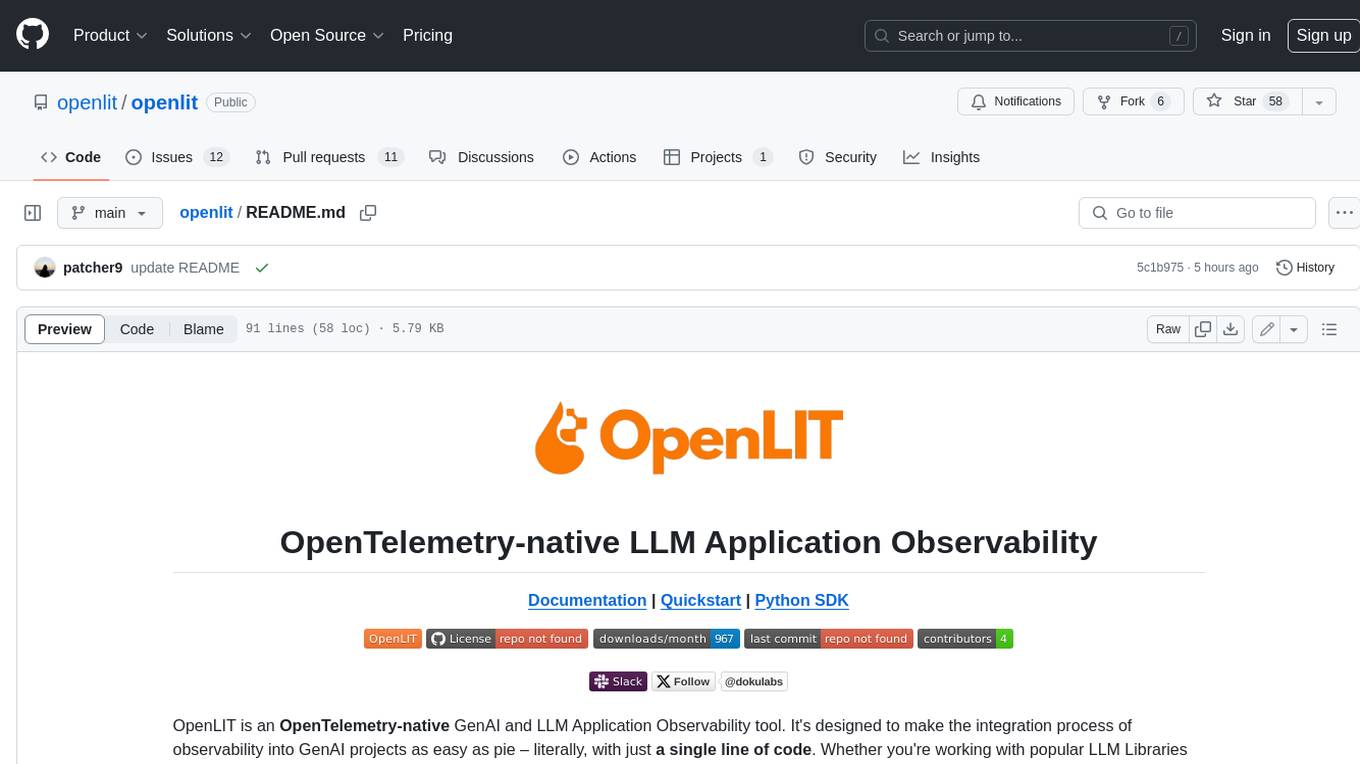
doku
OpenLIT is an OpenTelemetry-native GenAI and LLM Application Observability tool. It's designed to make the integration process of observability into GenAI projects as easy as pie – literally, with just a single line of code. Whether you're working with popular LLM Libraries such as OpenAI and HuggingFace or leveraging vector databases like ChromaDB, OpenLIT ensures your applications are monitored seamlessly, providing critical insights to improve performance and reliability.
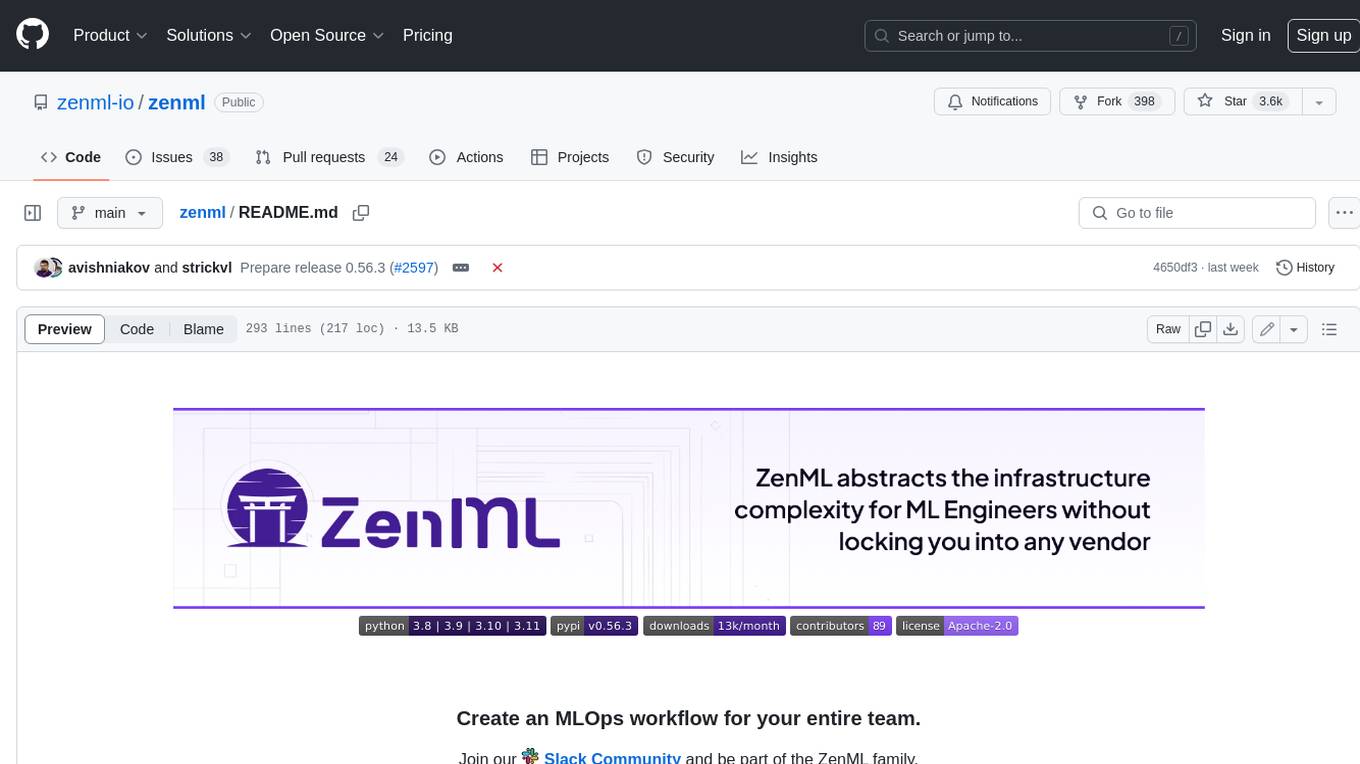
zenml
ZenML is an extensible, open-source MLOps framework for creating portable, production-ready machine learning pipelines. By decoupling infrastructure from code, ZenML enables developers across your organization to collaborate more effectively as they develop to production.
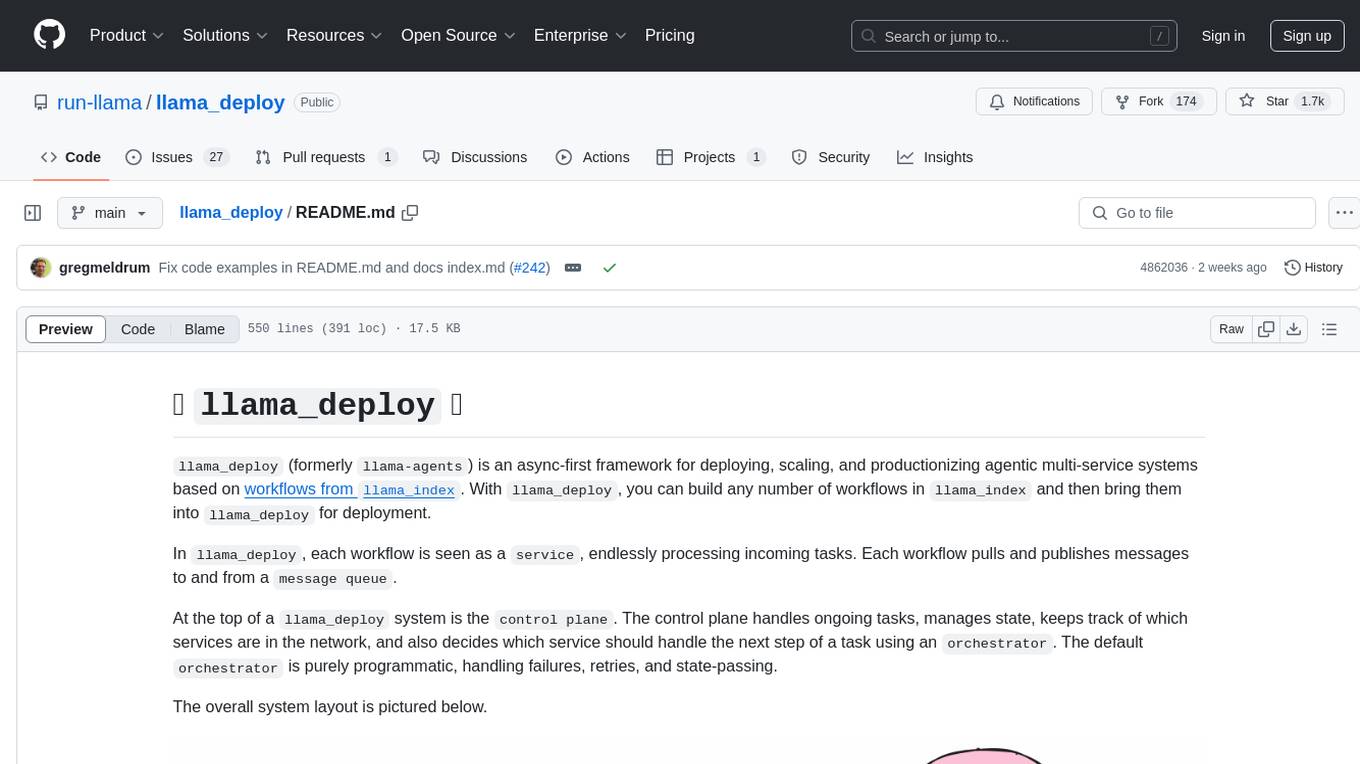
llama_deploy
llama_deploy is an async-first framework for deploying, scaling, and productionizing agentic multi-service systems based on workflows from llama_index. It allows building workflows in llama_index and deploying them seamlessly with minimal changes to code. The system includes services endlessly processing tasks, a control plane managing state and services, an orchestrator deciding task handling, and fault tolerance mechanisms. It is designed for high-concurrency scenarios, enabling real-time and high-throughput applications.
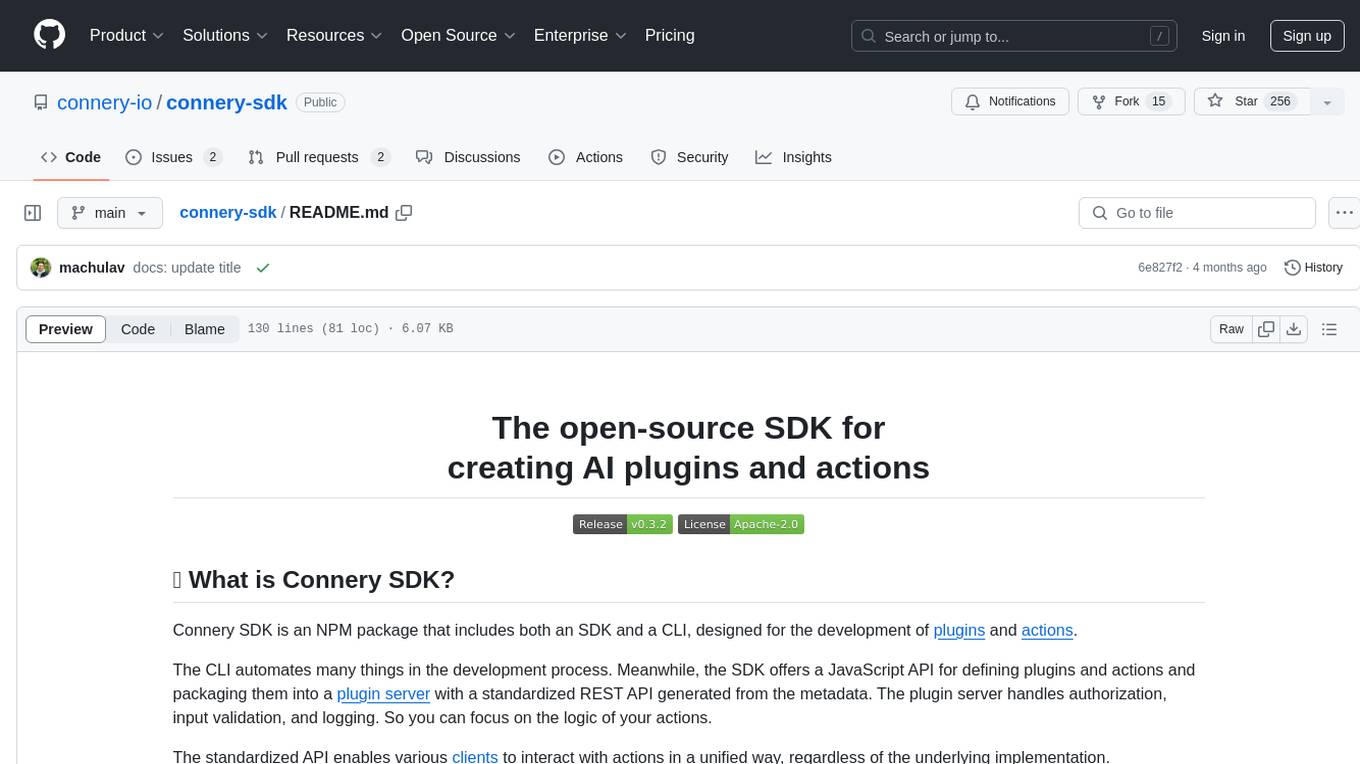
connery-sdk
Connery SDK is an open-source NPM package that provides an SDK and CLI for developing plugins and actions. The SDK offers a JavaScript API to define plugins and actions, which are then packaged into a plugin server with a standardized REST API. This enables automation in the development process and simplifies handling authorization, input validation, and logging. Users can focus on the logic of their actions while the standardized API allows various clients to interact with actions uniformly. Actions can communicate with external APIs, databases, or services, making it versatile for creating AI plugins and actions.
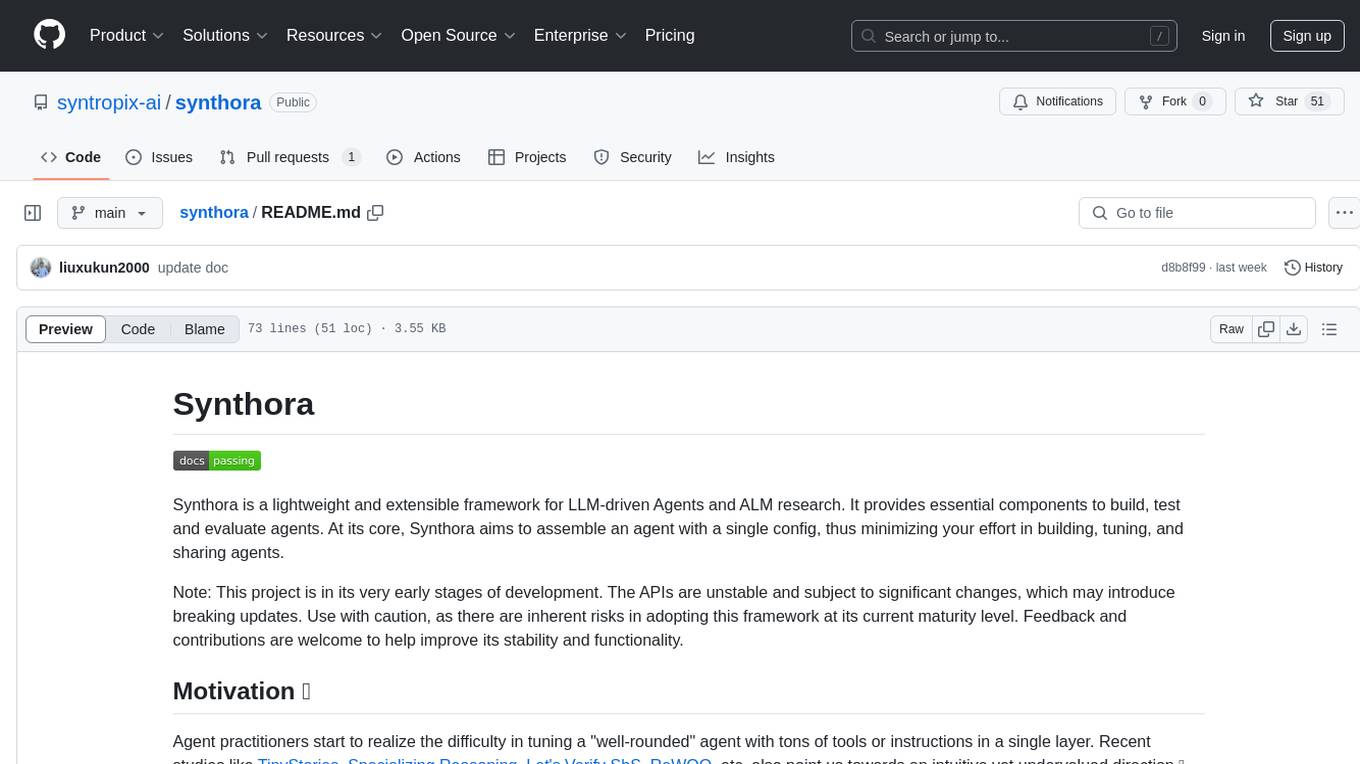
synthora
Synthora is a lightweight and extensible framework for LLM-driven Agents and ALM research. It aims to simplify the process of building, testing, and evaluating agents by providing essential components. The framework allows for easy agent assembly with a single config, reducing the effort required for tuning and sharing agents. Although in early development stages with unstable APIs, Synthora welcomes feedback and contributions to enhance its stability and functionality.
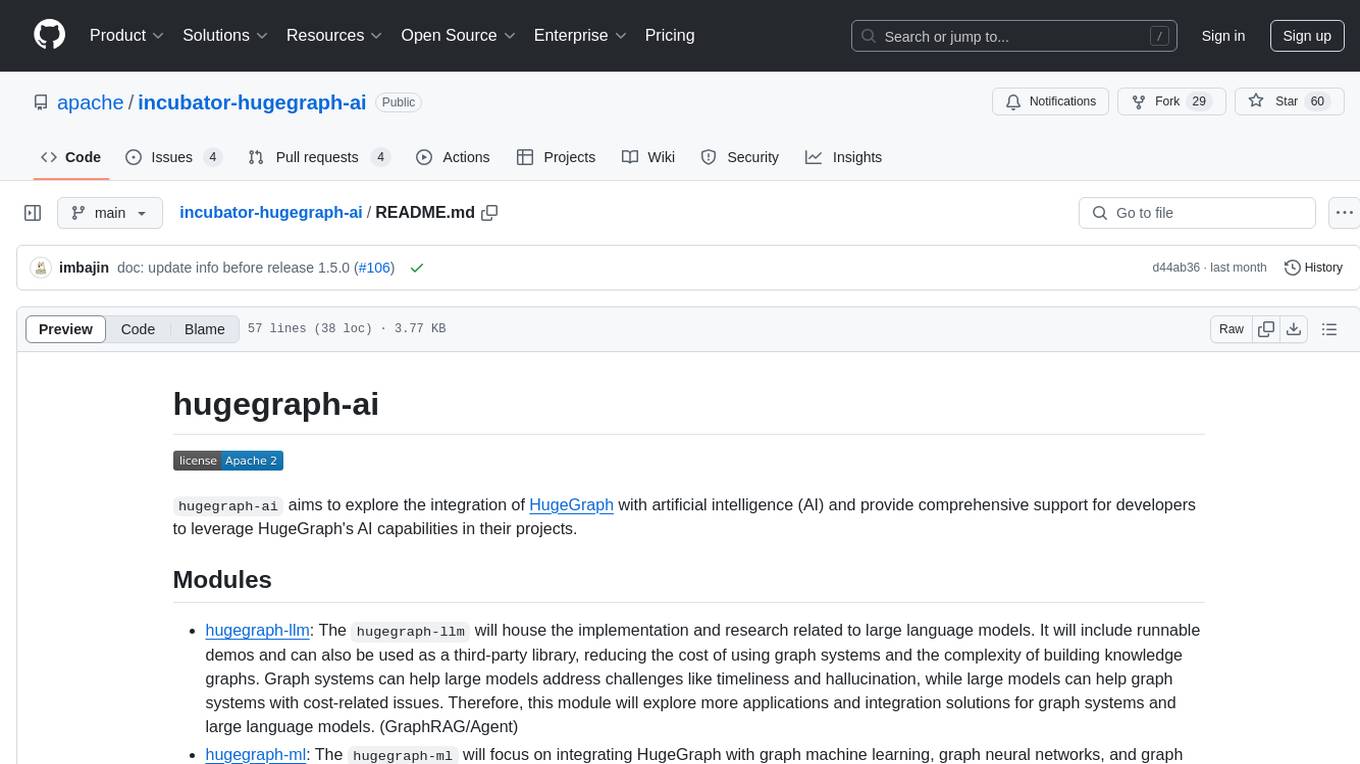
incubator-hugegraph-ai
hugegraph-ai aims to explore the integration of HugeGraph with artificial intelligence (AI) and provide comprehensive support for developers to leverage HugeGraph's AI capabilities in their projects. It includes modules for large language models, graph machine learning, and a Python client for HugeGraph. The project aims to address challenges like timeliness, hallucination, and cost-related issues by integrating graph systems with AI technologies.
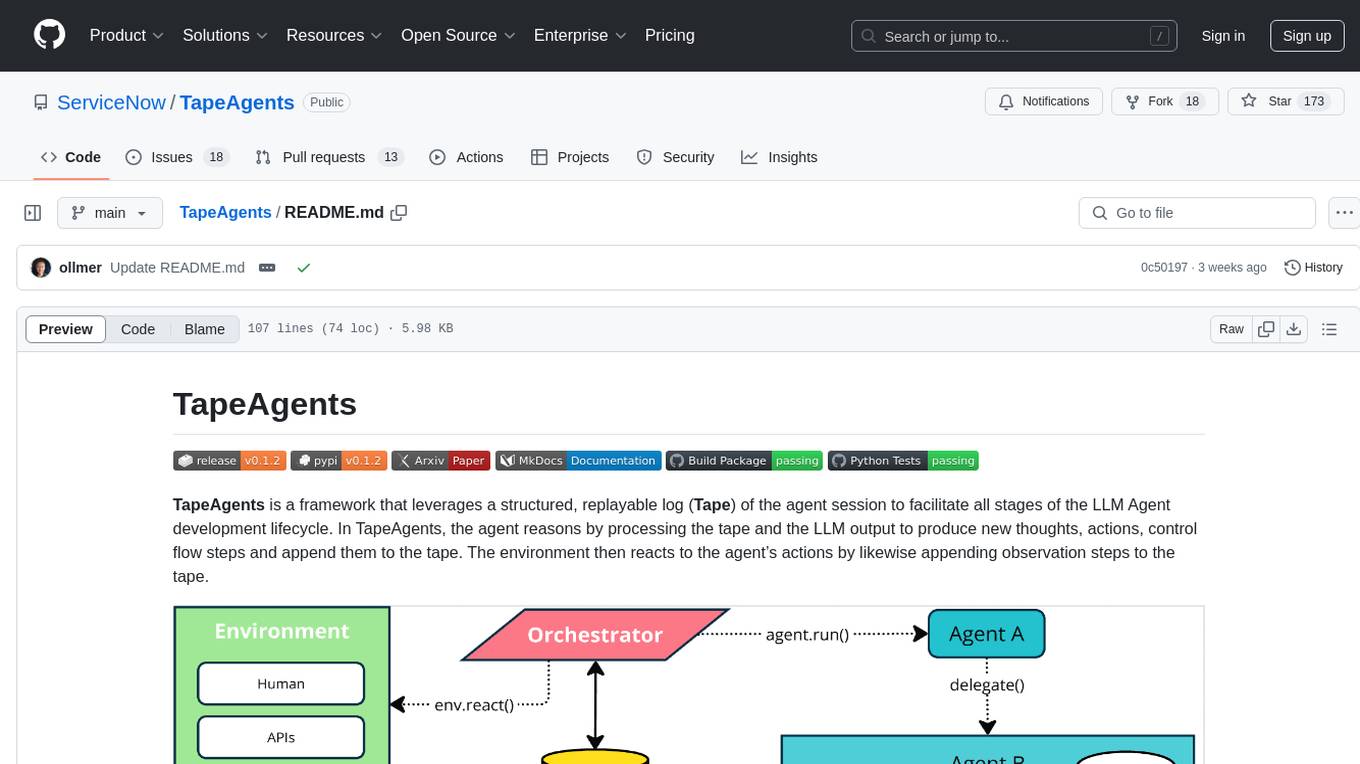
TapeAgents
TapeAgents is a framework that leverages a structured, replayable log of the agent session to facilitate all stages of the LLM Agent development lifecycle. The agent reasons by processing the tape and the LLM output to produce new thoughts, actions, control flow steps, and append them to the tape. Key features include building agents as low-level state machines or high-level multi-agent team configurations, debugging agents with TapeAgent studio or TapeBrowser apps, serving agents with response streaming, and optimizing agent configurations using successful tapes. The Tape-centric design of TapeAgents provides ultimate flexibility in project development, allowing access to tapes for making prompts, generating next steps, and controlling agent behavior.
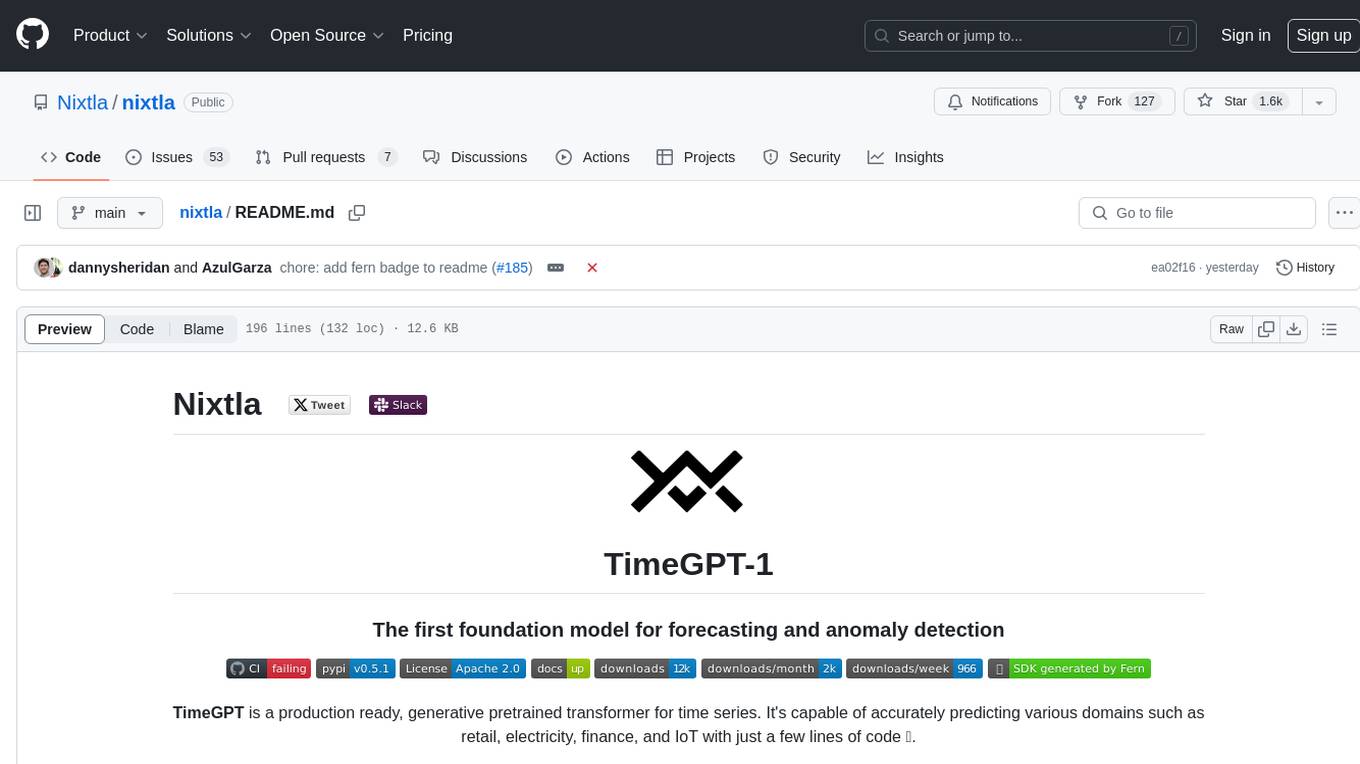
nixtla
Nixtla is a production-ready generative pretrained transformer for time series forecasting and anomaly detection. It can accurately predict various domains such as retail, electricity, finance, and IoT with just a few lines of code. TimeGPT introduces a paradigm shift with its standout performance, efficiency, and simplicity, making it accessible even to users with minimal coding experience. The model is based on self-attention and is independently trained on a vast time series dataset to minimize forecasting error. It offers features like zero-shot inference, fine-tuning, API access, adding exogenous variables, multiple series forecasting, custom loss function, cross-validation, prediction intervals, and handling irregular timestamps.
For similar tasks
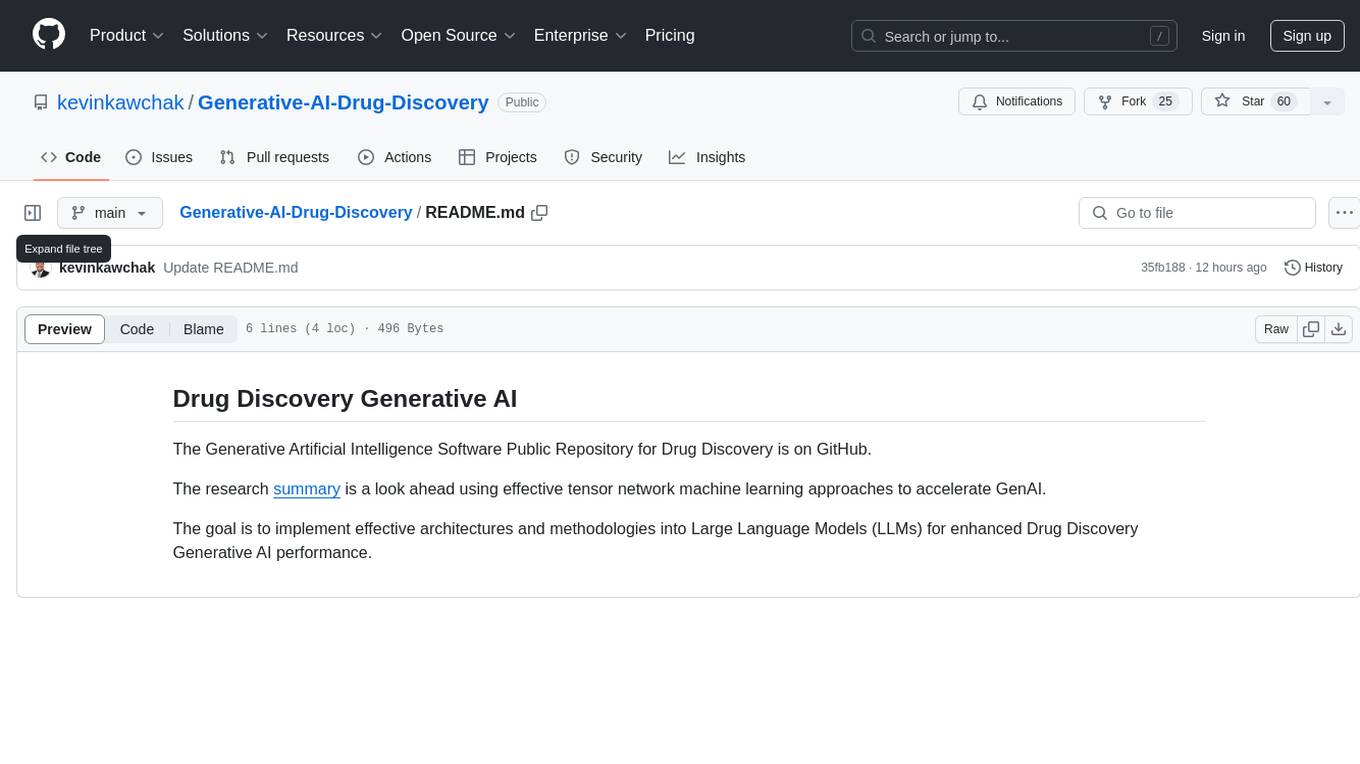
Generative-AI-Drug-Discovery
Generative-AI-Drug-Discovery is a public repository on GitHub focused on using tensor network machine learning approaches to accelerate GenAI for drug discovery. The repository aims to implement effective architectures and methodologies into Large Language Models (LLMs) to enhance Drug Discovery Generative AI performance.
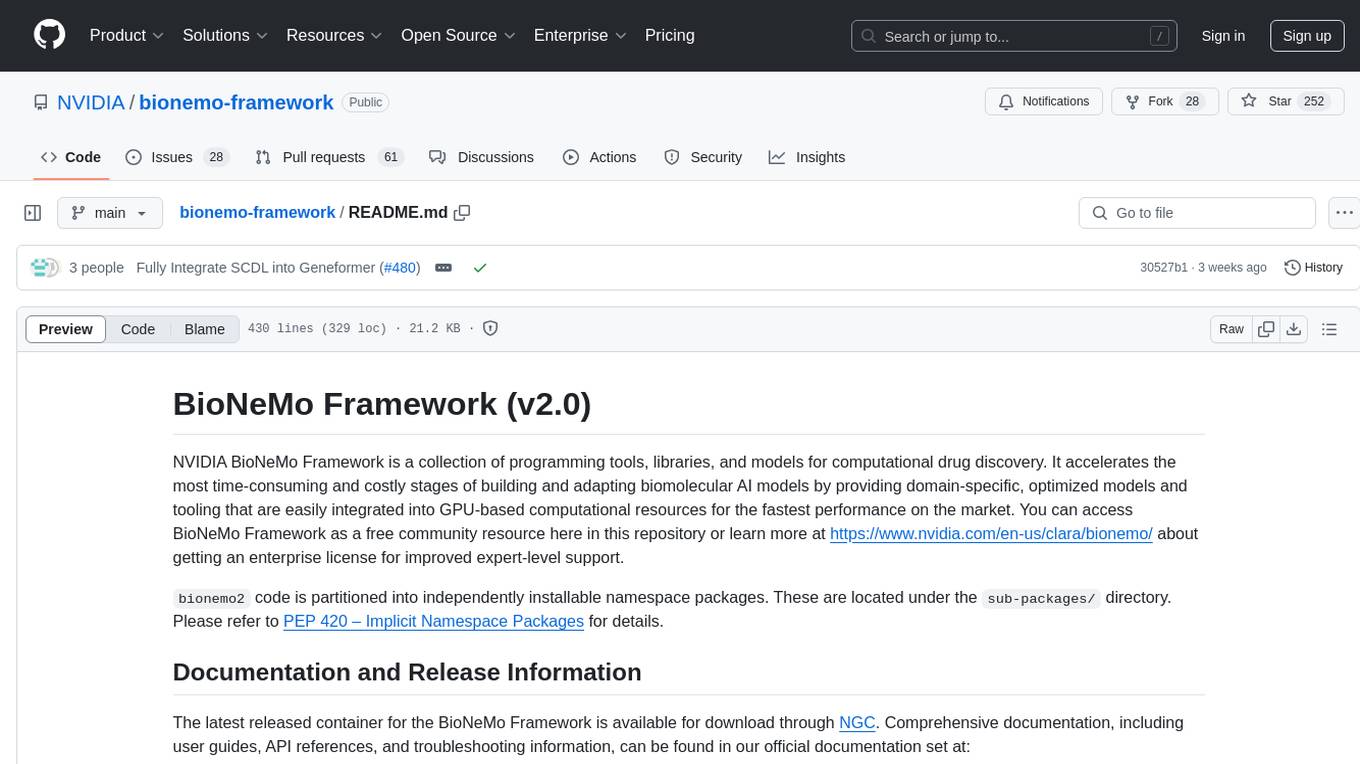
bionemo-framework
NVIDIA BioNeMo Framework is a collection of programming tools, libraries, and models for computational drug discovery. It accelerates building and adapting biomolecular AI models by providing domain-specific, optimized models and tooling for GPU-based computational resources. The framework offers comprehensive documentation and support for both community and enterprise users.
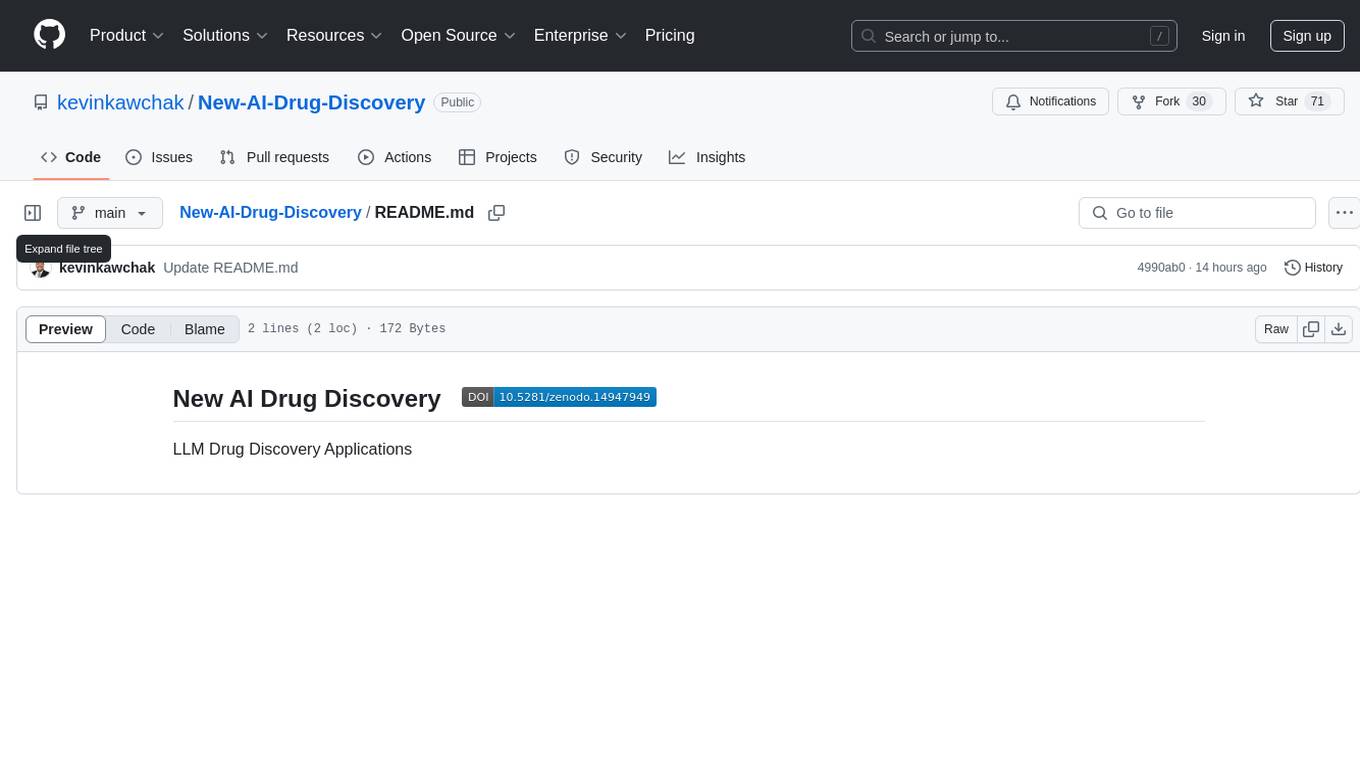
New-AI-Drug-Discovery
New AI Drug Discovery is a repository focused on the applications of Large Language Models (LLM) in drug discovery. It provides resources, tools, and examples for leveraging LLM technology in the pharmaceutical industry. The repository aims to showcase the potential of using AI-driven approaches to accelerate the drug discovery process, improve target identification, and optimize molecular design. By exploring the intersection of artificial intelligence and drug development, this repository offers insights into the latest advancements in computational biology and cheminformatics.
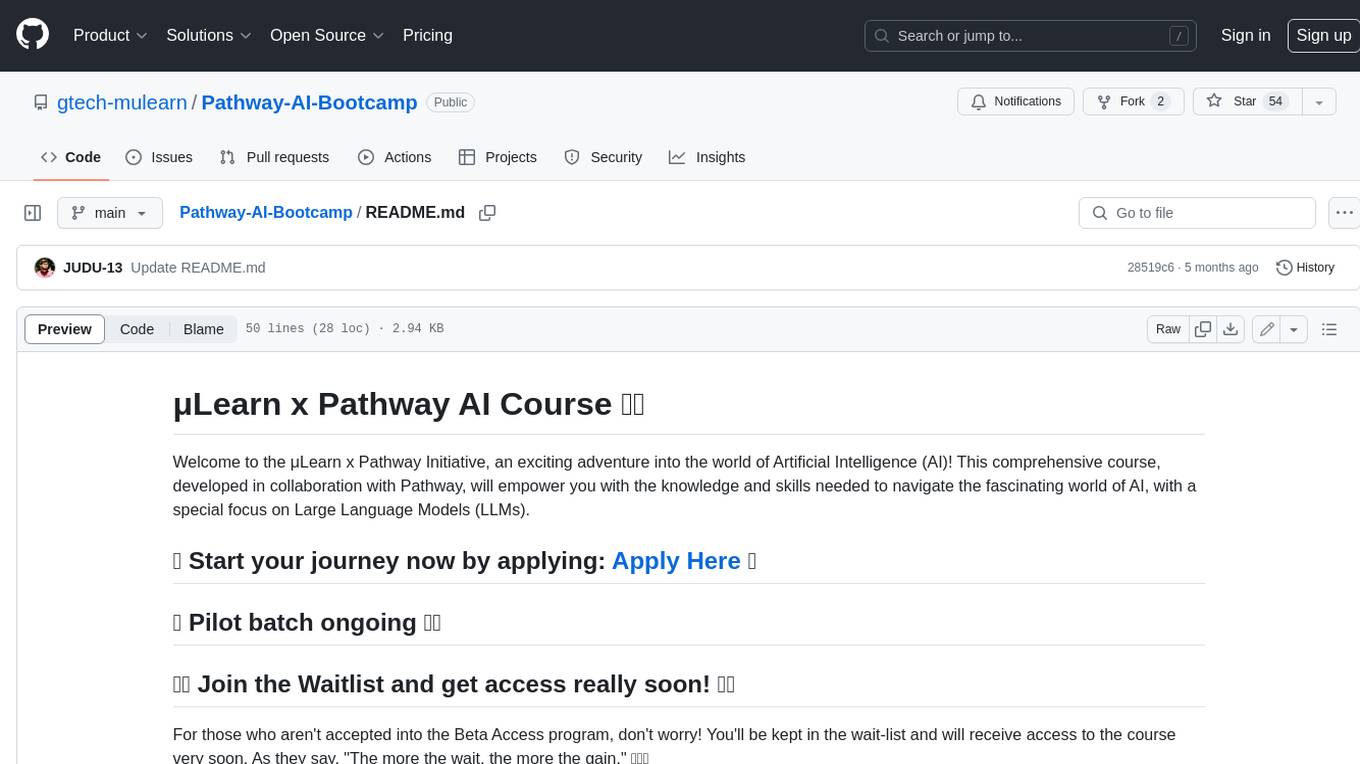
Pathway-AI-Bootcamp
Welcome to the μLearn x Pathway Initiative, an exciting adventure into the world of Artificial Intelligence (AI)! This comprehensive course, developed in collaboration with Pathway, will empower you with the knowledge and skills needed to navigate the fascinating world of AI, with a special focus on Large Language Models (LLMs).
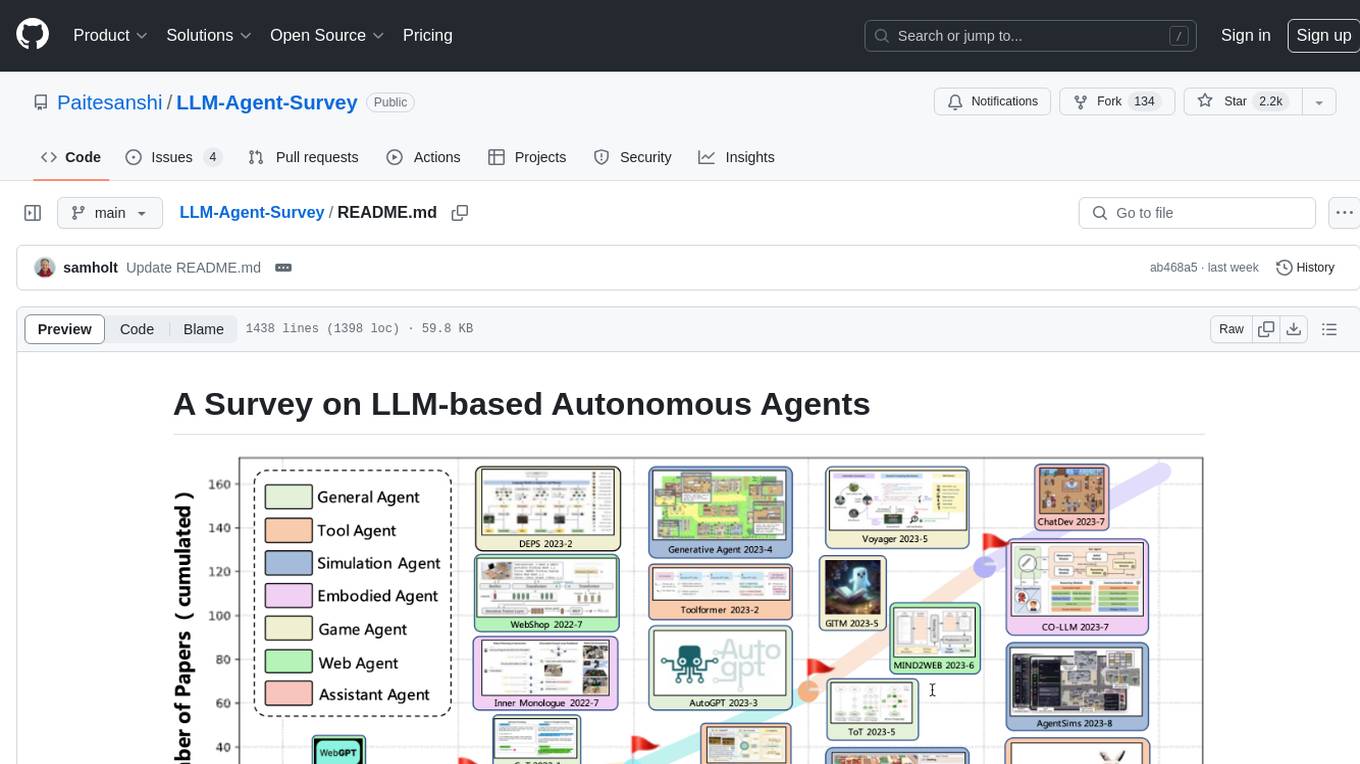
LLM-Agent-Survey
Autonomous agents are designed to achieve specific objectives through self-guided instructions. With the emergence and growth of large language models (LLMs), there is a growing trend in utilizing LLMs as fundamental controllers for these autonomous agents. This repository conducts a comprehensive survey study on the construction, application, and evaluation of LLM-based autonomous agents. It explores essential components of AI agents, application domains in natural sciences, social sciences, and engineering, and evaluation strategies. The survey aims to be a resource for researchers and practitioners in this rapidly evolving field.
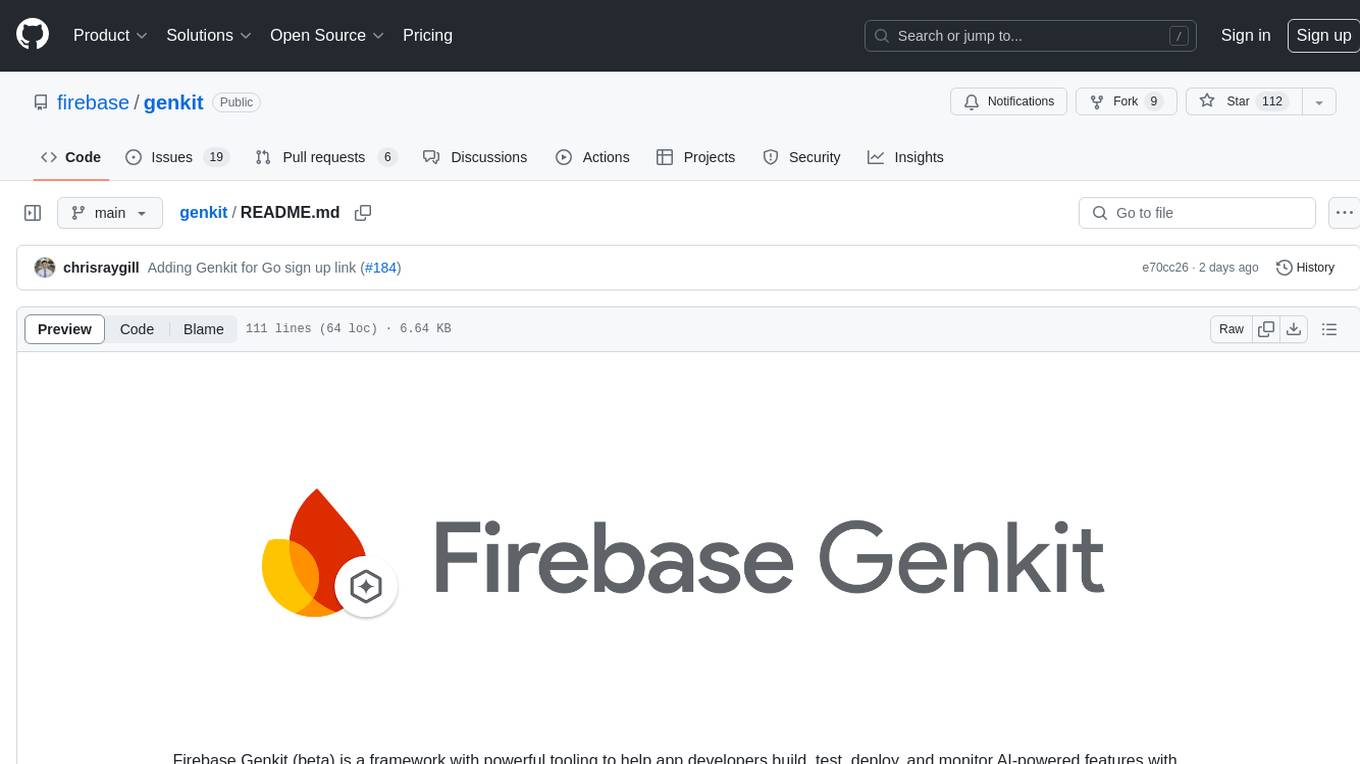
genkit
Firebase Genkit (beta) is a framework with powerful tooling to help app developers build, test, deploy, and monitor AI-powered features with confidence. Genkit is cloud optimized and code-centric, integrating with many services that have free tiers to get started. It provides unified API for generation, context-aware AI features, evaluation of AI workflow, extensibility with plugins, easy deployment to Firebase or Google Cloud, observability and monitoring with OpenTelemetry, and a developer UI for prototyping and testing AI features locally. Genkit works seamlessly with Firebase or Google Cloud projects through official plugins and templates.
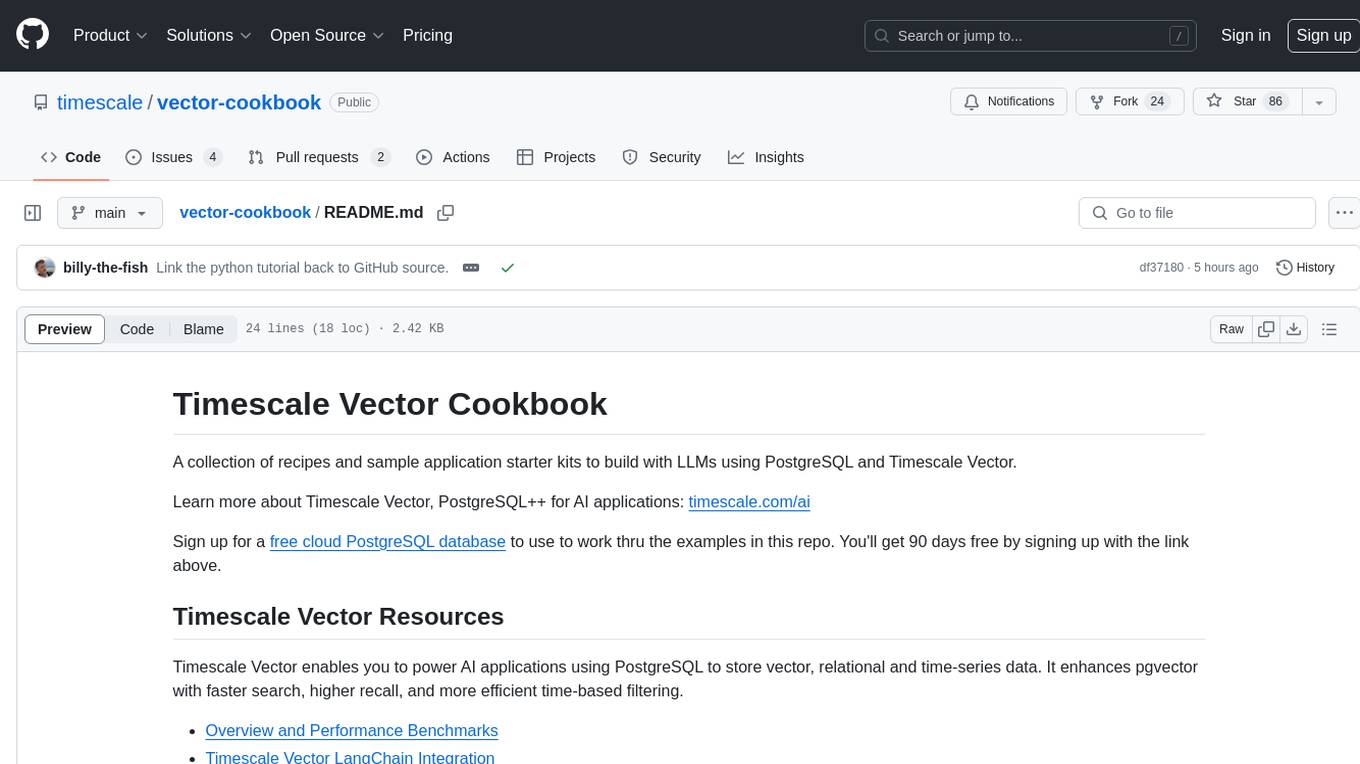
vector-cookbook
The Vector Cookbook is a collection of recipes and sample application starter kits for building AI applications with LLMs using PostgreSQL and Timescale Vector. Timescale Vector enhances PostgreSQL for AI applications by enabling the storage of vector, relational, and time-series data with faster search, higher recall, and more efficient time-based filtering. The repository includes resources, sample applications like TSV Time Machine, and guides for creating, storing, and querying OpenAI embeddings with PostgreSQL and pgvector. Users can learn about Timescale Vector, explore performance benchmarks, and access Python client libraries and tutorials.
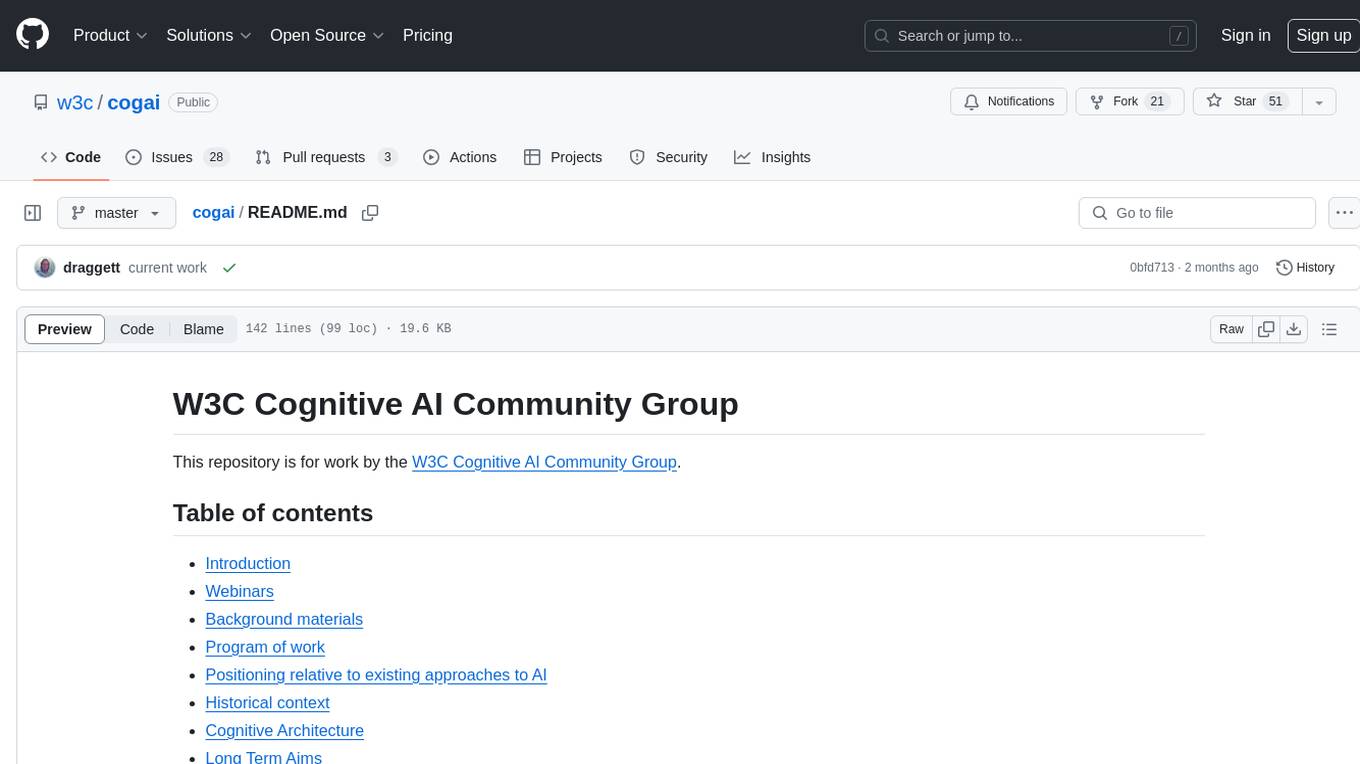
cogai
The W3C Cognitive AI Community Group focuses on advancing Cognitive AI through collaboration on defining use cases, open source implementations, and application areas. The group aims to demonstrate the potential of Cognitive AI in various domains such as customer services, healthcare, cybersecurity, online learning, autonomous vehicles, manufacturing, and web search. They work on formal specifications for chunk data and rules, plausible knowledge notation, and neural networks for human-like AI. The group positions Cognitive AI as a combination of symbolic and statistical approaches inspired by human thought processes. They address research challenges including mimicry, emotional intelligence, natural language processing, and common sense reasoning. The long-term goal is to develop cognitive agents that are knowledgeable, creative, collaborative, empathic, and multilingual, capable of continual learning and self-awareness.
For similar jobs
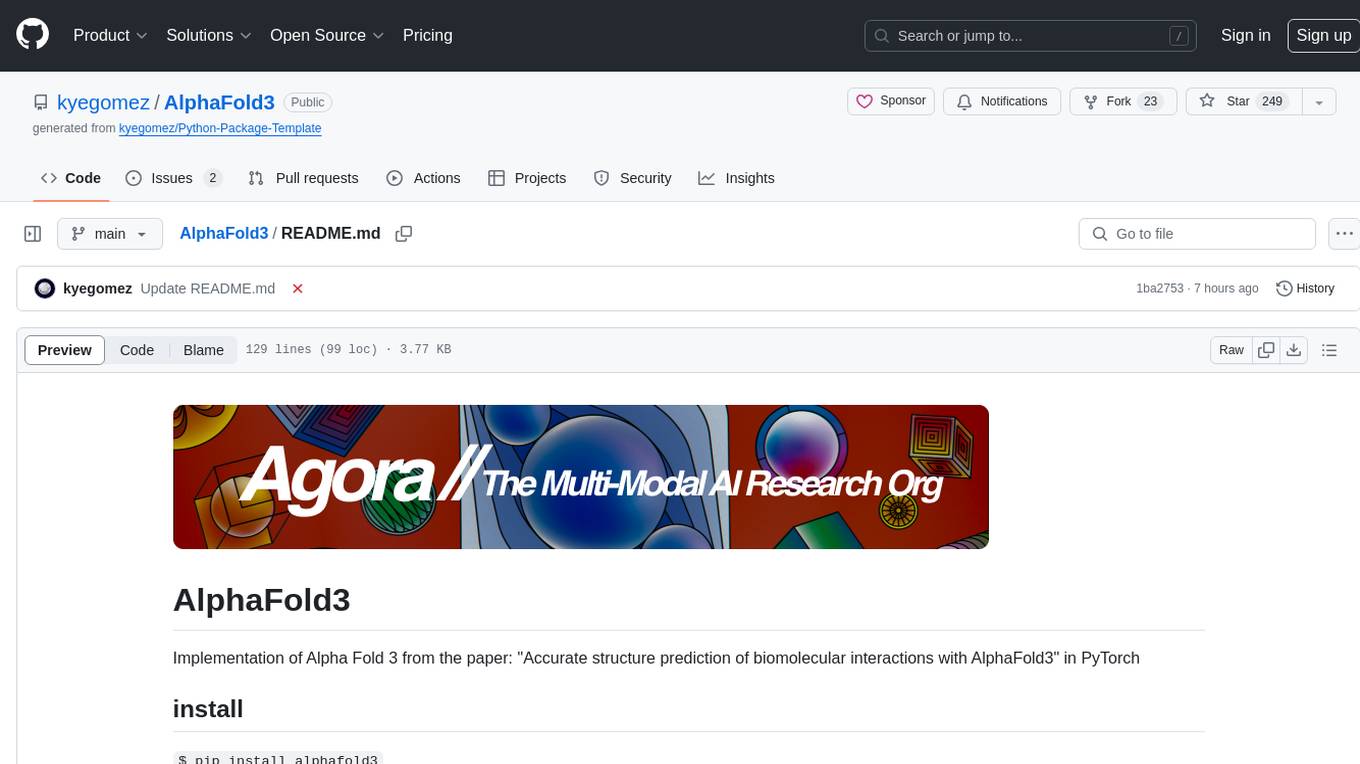
AlphaFold3
AlphaFold3 is an implementation of the Alpha Fold 3 model in PyTorch for accurate structure prediction of biomolecular interactions. It includes modules for genetic diffusion and full model examples for forward pass computations. The tool allows users to generate random pair and single representations, operate on atomic coordinates, and perform structure predictions based on input tensors. The implementation also provides functionalities for training and evaluating the model.
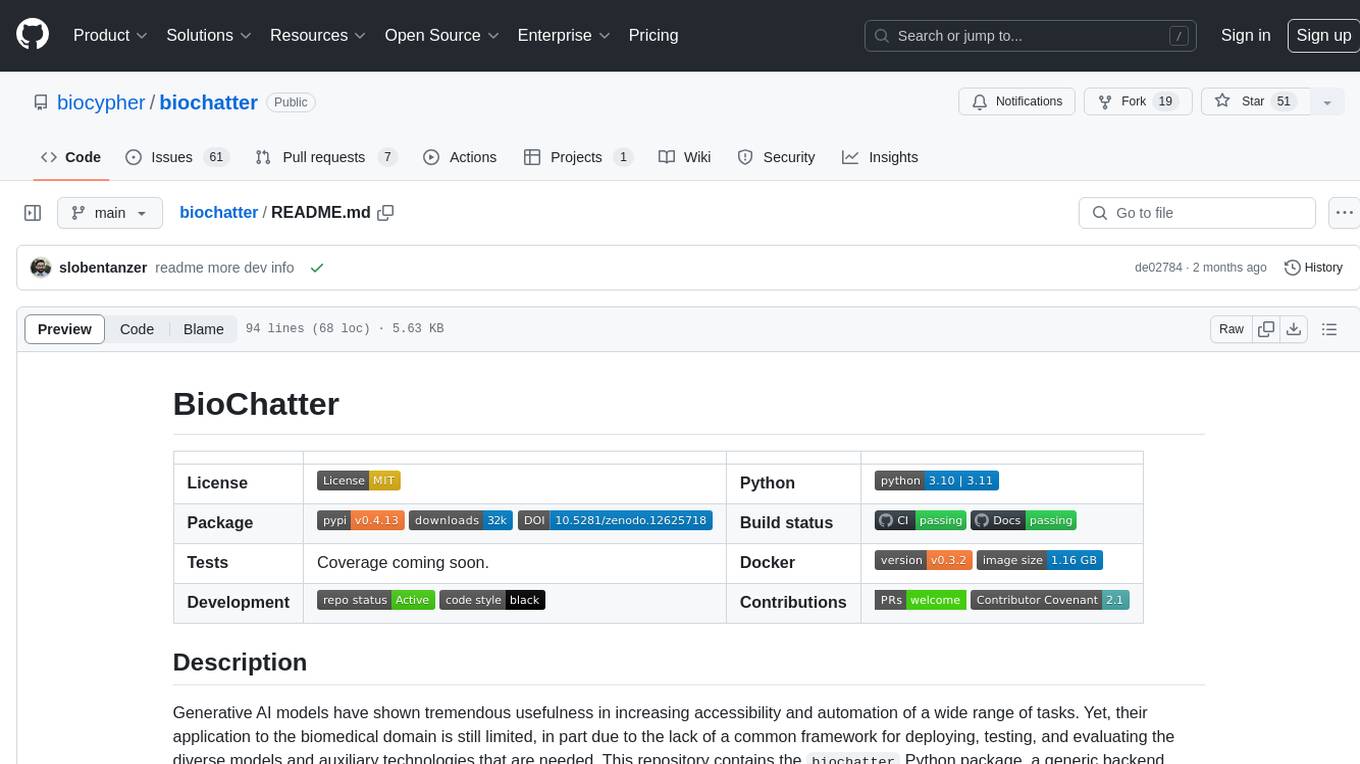
biochatter
Generative AI models have shown tremendous usefulness in increasing accessibility and automation of a wide range of tasks. This repository contains the `biochatter` Python package, a generic backend library for the connection of biomedical applications to conversational AI. It aims to provide a common framework for deploying, testing, and evaluating diverse models and auxiliary technologies in the biomedical domain. BioChatter is part of the BioCypher ecosystem, connecting natively to BioCypher knowledge graphs.
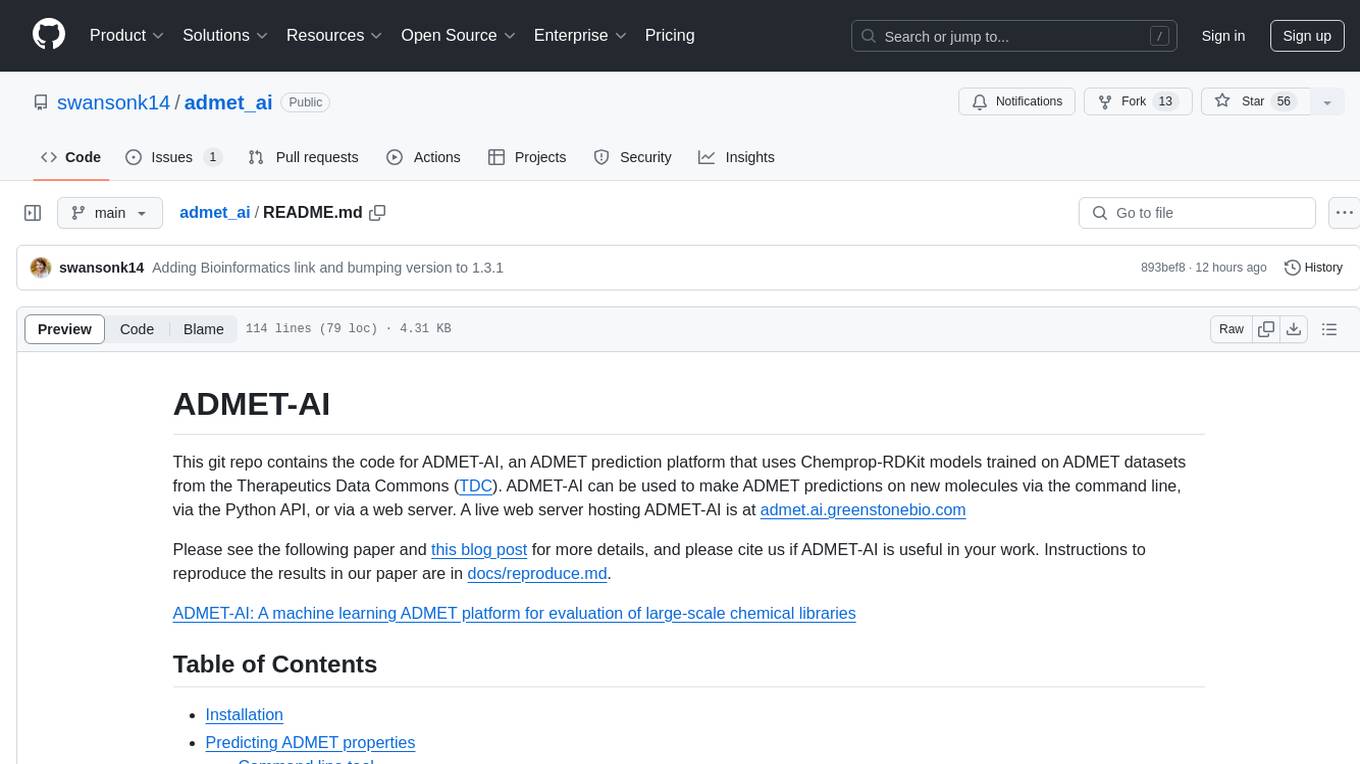
admet_ai
ADMET-AI is a platform for ADMET prediction using Chemprop-RDKit models trained on ADMET datasets from the Therapeutics Data Commons. It offers command line, Python API, and web server interfaces for making ADMET predictions on new molecules. The platform can be easily installed using pip and supports GPU acceleration. It also provides options for processing TDC data, plotting results, and hosting a web server. ADMET-AI is a machine learning platform for evaluating large-scale chemical libraries.
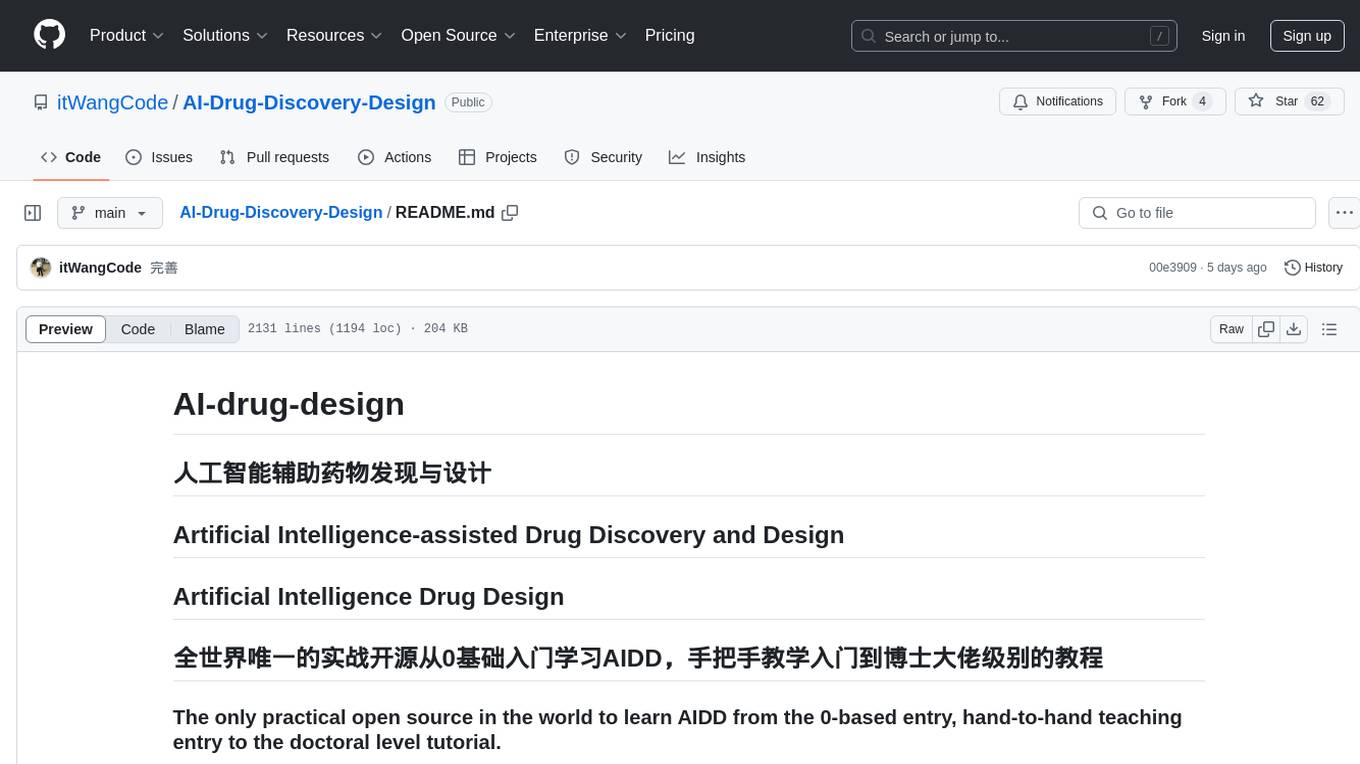
AI-Drug-Discovery-Design
AI-Drug-Discovery-Design is a repository focused on Artificial Intelligence-assisted Drug Discovery and Design. It explores the use of AI technology to accelerate and optimize the drug development process. The advantages of AI in drug design include speeding up research cycles, improving accuracy through data-driven models, reducing costs by minimizing experimental redundancies, and enabling personalized drug design for specific patients or disease characteristics.
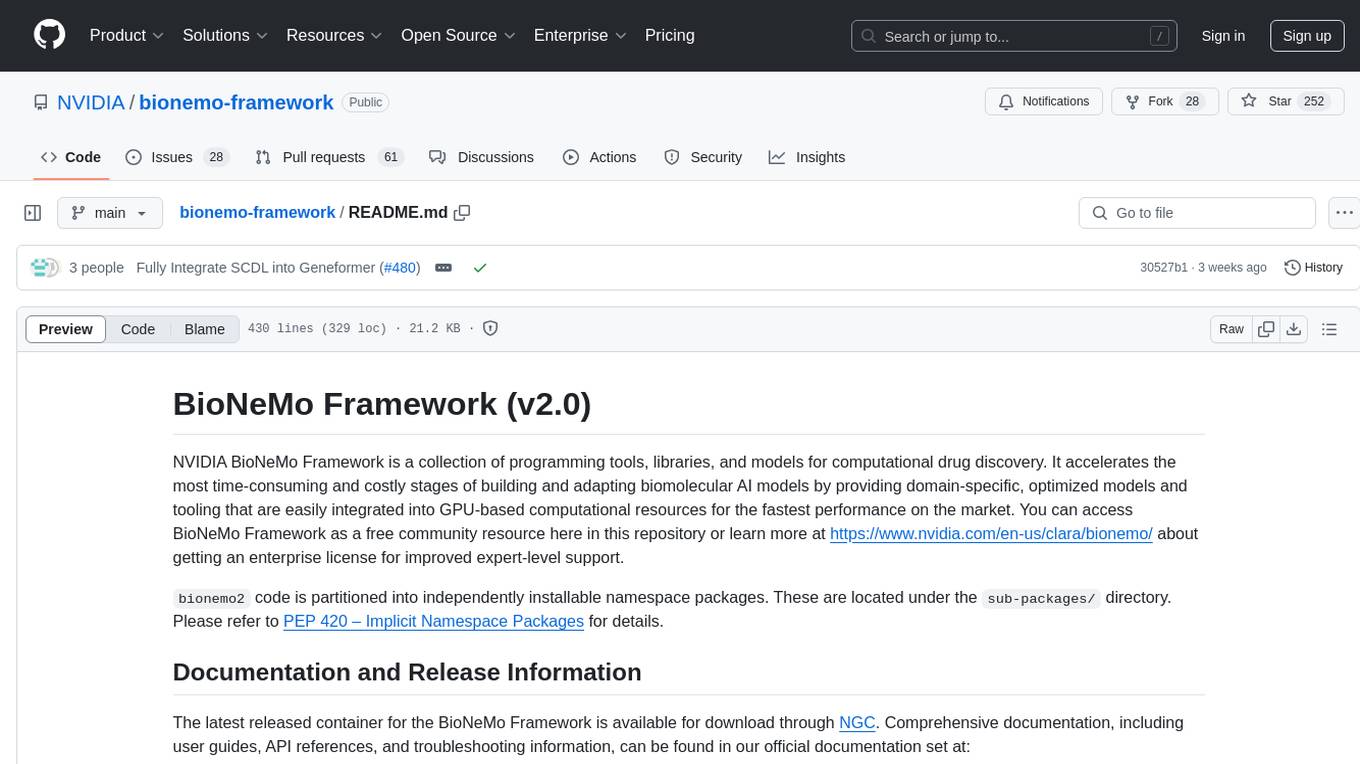
bionemo-framework
NVIDIA BioNeMo Framework is a collection of programming tools, libraries, and models for computational drug discovery. It accelerates building and adapting biomolecular AI models by providing domain-specific, optimized models and tooling for GPU-based computational resources. The framework offers comprehensive documentation and support for both community and enterprise users.
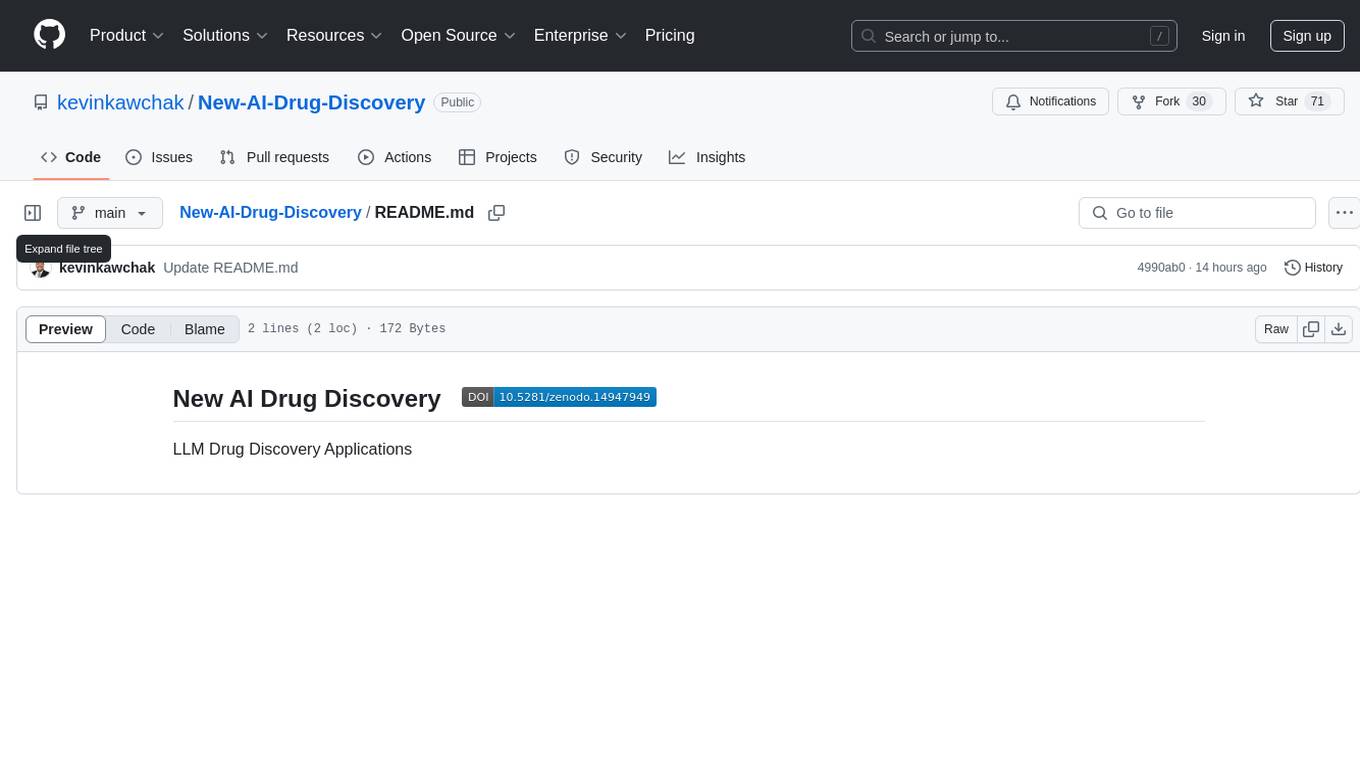
New-AI-Drug-Discovery
New AI Drug Discovery is a repository focused on the applications of Large Language Models (LLM) in drug discovery. It provides resources, tools, and examples for leveraging LLM technology in the pharmaceutical industry. The repository aims to showcase the potential of using AI-driven approaches to accelerate the drug discovery process, improve target identification, and optimize molecular design. By exploring the intersection of artificial intelligence and drug development, this repository offers insights into the latest advancements in computational biology and cheminformatics.
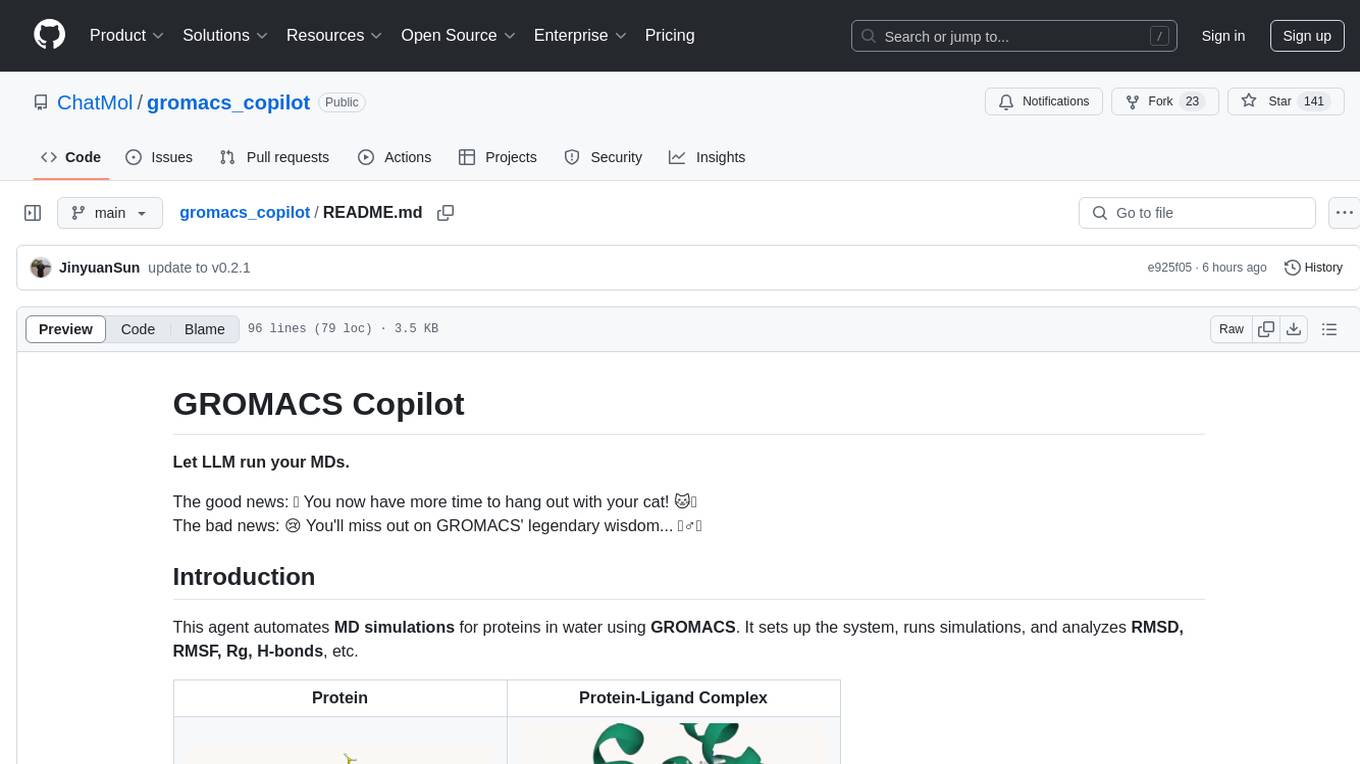
gromacs_copilot
GROMACS Copilot is an agent designed to automate molecular dynamics simulations for proteins in water using GROMACS. It handles system setup, simulation execution, and result analysis automatically, providing outputs such as RMSD, RMSF, Rg, and H-bonds. Users can interact with the agent through prompts and API keys from DeepSeek and OpenAI. The tool aims to simplify the process of running MD simulations, allowing users to focus on other tasks while it handles the technical aspects of the simulations.
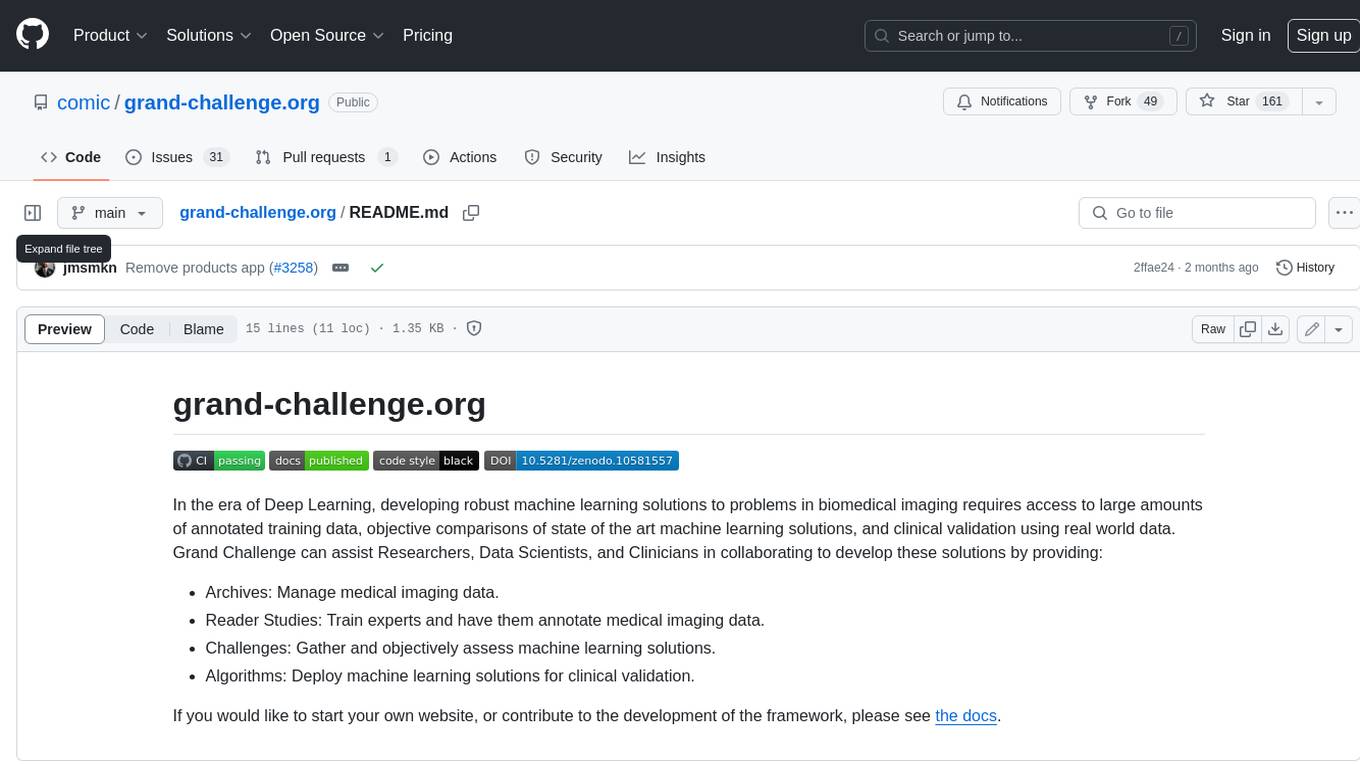
grand-challenge.org
Grand Challenge is a platform that provides access to large amounts of annotated training data, objective comparisons of state-of-the-art machine learning solutions, and clinical validation using real-world data. It assists researchers, data scientists, and clinicians in collaborating to develop robust machine learning solutions to problems in biomedical imaging.