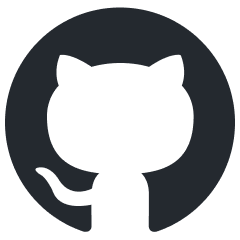
cogai
for work by the Cognitive AI community group
Stars: 53
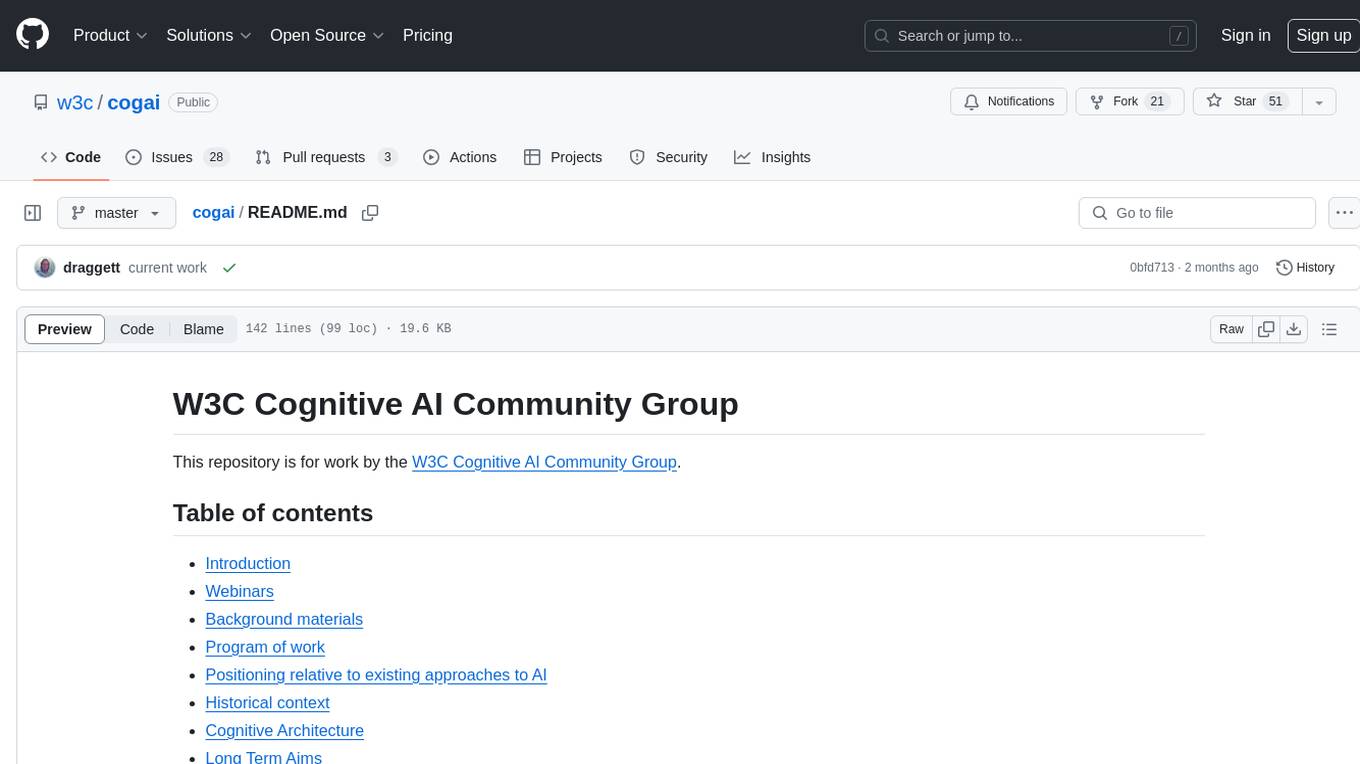
The W3C Cognitive AI Community Group focuses on advancing Cognitive AI through collaboration on defining use cases, open source implementations, and application areas. The group aims to demonstrate the potential of Cognitive AI in various domains such as customer services, healthcare, cybersecurity, online learning, autonomous vehicles, manufacturing, and web search. They work on formal specifications for chunk data and rules, plausible knowledge notation, and neural networks for human-like AI. The group positions Cognitive AI as a combination of symbolic and statistical approaches inspired by human thought processes. They address research challenges including mimicry, emotional intelligence, natural language processing, and common sense reasoning. The long-term goal is to develop cognitive agents that are knowledgeable, creative, collaborative, empathic, and multilingual, capable of continual learning and self-awareness.
README:
This repository is for work by the W3C Cognitive AI Community Group.
- Introduction
- Webinars
- Background materials
- Program of work
- Positioning relative to existing approaches to AI
- Historical context
- Cognitive Architecture
- Long Term Aims
According to wikipedia:
Cognitive science is the interdisciplinary, scientific study of the mind and its processes. It examines the nature, the tasks, and the functions of cognition. Cognitive scientists study intelligence and behavior, with a focus on how nervous systems represent, process, and transform information.
Cognitive AI can be defined as AI based upon insights from the cognitive sciences, including cognitive neuroscience, and cognitive sociology. To put it another way, the brain has evolved over hundreds of millions of years, and we would do well to borrow from nature when it comes to building AI systems.
The W3C Cognitive AI Community Group seeks to demonstrate the potential of Cognitive AI through:
- Collaboration on defining use cases, requirements and datasets for use in demonstrators
- Work on open source implementations and scaling experiments
- Work on identifying and analysing application areas, e.g.
- Helping non-programmers to work with data
- Cognitive agents in support of customer services
- Smart chatbots for personal healthcare
- Assistants for detecting and responding to cyberattacks
- Teaching assistants for self-paced online learning
- Autonomous vehicles
- Smart manufacturing
- Smart web search
- Outreach to explain the huge opportunities for Cognitive AI
- Participation is open to all, free of charge: join group
We are using GitHub for documents, issue tracking and open source components. We have a public mailing list, and an IRC channel #cogai.
This is a series of recorded webinars, see webinar planning
- 7 March 2024, The role of symbolic knowledge at the dawn of AGI, as presented to the Estes Park Group: slides, and video recording
- Talks
- 05 September 2024 Application to low-code control of digital twins, talk at Swarm Computing Workshop, Brussels
- 04 July 2023 Combining Digital Twins with Cognitive Agents, talk at ETSI IoT Week 2023
- 14 June 2023 Human-like AI: from logic to argumentation, reasoning with imperfect knowledge in the era of AGI, see video recording talk for Darmstadt Ontology Group
- 18 April 2023 Human-Like AI for Artificial General Intelligence, as part of the Special day on Human-AI interaction, DATE 2023
- 20 February 2023 - Human-like AI for Artificial General Intelligence - research challenges for the Intelligent Web of Things)
- 14 November 2022 - Cognitive Agents, Plausible Reasoning and Scalable Knowledge Engineering, Workshop on Computing across the Continuum for the US NSF and the European Commission
- 12 September 2022 - Plausible reasoning and artificial minds, Workshop on Analogies: from Theory to Applications
- 16 February 2022 - Imperfect Knowledge, Distributed Knowledge Graphs workshop
- 06 September 2021 - Digital Transformation using chunks as a simple abstraction above triples and property graphs - talk at the Semantics2021 workshop on squaring the circle on graphs
- 17 March 2021 - Digital Transformation and the Knowledge Economy, an invited keynote for the Siemens conference on the IoT
- 17 March 2021 - Roadmap for realising general purpose AI
- 14-19 February 2021 - Human-like AI and the Sentient Web for Dagstuhl seminar on autonomous agents on the Web - slides, paper
- 11 January 2021 - Presentation and discussion of work on Cognitive Natural Language Processing
- 25 November 2020 - Seminar on Cognitive AI for Centre for Artificial Intelligence, Robotics and Human-Machine Systems, Cardiff University: slides, video
- 06 November 2020 - Seminar on Cognitive AI for Knowledge Media Institute, Open University - video and slides
- 08 June 2020 - Cognitive AI and the Sentient Web for ISO/TC 211 50th Plenary meeting, WG4 Geospatial Services
- Chunks format for declarative and procedural knowledge
- Common Sense Reasoning
- Demonstrators
- Frequently asked questions
- Longer treatise on Cognitive AI
- Contributing to the Cognitive AI Community Group
The initial focus is to describe the aims for a sequence of demonstrators, to collaborate on the scenarios, detailed use cases, and associated datasets, and to identify and discuss questions that arise in this work. In relation to this, we are working on a formal specification of the chunk data and rules format with a view to its standardisation.
A further line of work deals with the means to express and reason with imperfect knowledge, that is, everyday knowledge subject to uncertainty, imprecision, incompleteness and inconsistencies. See the draft specifcation for the plausible knowledge notation (PKN), and the web-based PKN demonstrator. This is based upon work on guidelines for effective argumentation by a long line of philosophers since the days of Ancient Greece. In place of logical proof, we have multiple lines of argument for and against the premise in question just like in courtrooms and everyday reasoning.
Both PKN and chunks & rules can be considered in relation to RDF. RDF is a framework for symbolic graphs based upon labelled directed graph edges (aka triples). Compared to RDF, PKN and chunks & rules are higher level with additional semantics, and designed for use in human-like AI applications. Furthermore, both notations are designed to be easy to read and author compared with RDF serialisations such as RDF/XML, Turtle and even JSON-LD. See also the Notation3 (N3) Language which is an assertion and logic language defined as a superset of RDF.
Work is now underway on vector-space representations of knowledge using artificial neural networks. Advances with generative AI have shown the huge potential of vector-space representations in combination with deep learning. However, there is a long way to go to better model many aspects of human cognition, e.g. continual learning using a blend of type 1 and type 2 cognition, episodic memory, and the role of emotions and feelings in directing cognition. Symbolic models will continue to serve an important role for semantic interoperability. Neurosymbolic systems combine the complementary strengths of vector space and symbolic approaches. For more details see Neural Networks for Human-like AI.
Traditional AI focuses on symbolic representations of knowledge and on mathematical logic, e.g. Expert Systems and the Semantic Web. Deep Learning, by contrast, focuses on statistical models implemented as multi-layer neural networks. Both approaches have their weaknesses. Symbolic AI has difficulties with the ambiguities, uncertainties and inconsistencies commonplace in everyday situations. Furthermore, the reliance on manual knowledge engineering is a big bottleneck. Deep Learning has problems with recognising what’s salient, providing transparent explanations, the need for very large data sets for training, and difficulties with generalisation. Symbolic AI and Deep Learning are associated with siloed communities that typify modern science in which researchers are discouraged from interdisciplinary studies and the breadth of views that those encompass.
Cognitive AI seeks to address these weaknesses through mimicking human thought, taking inspiration from over 500 million years of neural evolution and decades of work across the cognitive sciences. This involves the combination of symbolic and statistical approaches using functional models of the human brain, including the cortex, basal ganglia, cerebellum and limbic system. Human memory is modelled in terms of symbolic graphs with embedded statistics reflecting prior knowledge and past experience. Human reasoning is not based upon logic, nor on the laws of probability, but rather on mental models of what is possible, along with the use of metaphors and analogies.
Research challenges include mimicry, emotional and social intelligence, natural language and common sense reasoning. Mimicry is key to social interaction, e.g. a baby learning to smile at its mother, and young children learning to speak. As a social species, we pay attention to social interaction and models of ourselves and others, including beliefs, desires, judgements and behaviours. Emotional control of cognition determines what is important, and plays a key role in how we learn, reason and act. Natural language is important for both communication and for learning and the means to break free from the manual programming bottleneck. Common sense is everyday knowledge and key to natural language understanding, and is learned through experience.
AI lacks a precise agreed definition, but loosely speaking, it is about replicating intelligent behaviour, including perception, reasoning and action. There are many sub-fields of AI, e.g. logic and formal semantics, artificial neural networks, rule-based approaches including expert systems, statistical approaches including Bayesian networks and Markov chains, and a wide variety of approaches to search, pattern recognition and machine learning. Cognitive AI seeks to exploit work across the cognitive sciences on the organising principles of the human mind.
Chunk rules are a form of production rules as introduced by Allen Newell in 1973 in his production system theory of human cognition, which he subsequently developed as the SOAR project. John Anderson published his theory of human associative memory (HAM) in 1973, and inspired by Newell, went on to combine it with a production system to form the ACT system in 1976, and developed it further into ACT-R in 1993. ACT-R stands for adaptive control of thought - rational and has been widely applied to cognitive science experiments as a theory for simulating and understanding human cognition. For more details see An Integrated Theory of the Mind. Chunks, in turn, was inspired by ACT-R, and the realisation that the approach could be adapted for general use in artificial intelligence as the combination of graphs, statistics, rules and graph algorithms.
Credit is also due to Marvin Minsky for his work on frames, metacognition, self-awareness and appreciation of the importance of emotions for controlling cognition, to Philip Johnson-Laird for his work on mental models and demonstrating that humans don't reason using logic and probability, but rather by thinking about what is possible, to George Lakoff for his work on metaphors, Dedre Gentner for her work on reasoning with analogies, and to Allan Collins for his work on plausible reasoning. Cognitive AI has a broader scope than ACT-R and seeks to mimic the human brain as a whole at a functional level, inspired by advances across the cognitive sciences. As such, Cognitive AI can be contrasted with approaches that focus on logic and formal semantics. Cognitive AI can likewise be decoupled from the underlying implementation, as the phenomenological requirements are essentially independent of whether they are realised as explicit graphs, vector spaces or pulsed neural networks, see David Marr's three levels of analysis.
The following diagram depicts how cognitive agents can be built as a collection of different building blocks that connect via the cortex, which functions as a collection of cognitive databases and associated algorithms. Semantic integration across the senses mimics the Anterior Temporal Lobe's role as a hub for the unimodal spokes. The initial focus of work was on a chunk rule engine inspired by John Anderson's ACT-R. Current work is focusing on plausible reasoning and belief revision. Future work will look at the other building blocks.
Image of cognitive architecture as a set of modules connected via the cortex
- Perception interprets sensory data and places the resulting models into the cortex, e.g. scene graphs. Cognitive rules can set the context for perception, and direct attention as needed. Events are signalled by queuing chunks to cognitive buffers to trigger rules describing the appropriate behaviour. A prioritised first-in first-out queue is used to manage events that are closely spaced in time.
- Emotion is about cognitive control and prioritising what’s important. The limbic system provides rapid assessment of past, present and imagined situations without the delays incurred in deliberative thought. Emotions are perceived as positive or negative, and associated with passive or active responses, involving actual and perceived threats, goal-directed drives and soothing/nurturing behaviours.
- Cognition is slower and more deliberate thought, involving sequential execution of rules to carry out particular tasks, including the means to invoke graph algorithms in the cortex, and to invoke operations involving other cognitive systems. Thought can be expressed at many different levels of abstraction, and is subject to control through metacognition, emotional drives, internal and external threats.
- Action is about carrying out actions initiated under conscious control, leaving the mind free to work on other things. An example is playing a musical instrument where muscle memory is needed to control your finger placements as thinking explicitly about each finger would be far too slow. The Cerebellum provides real-time coordination of muscle activation guided by perception, computing smooth functions over time.
Zooming in on cognition and the role of the basal ganglia as a sequential rule engine, the architecture looks like:
Image of cognitive architecture for deliberative reasoning (System 2)
This has been implemented as an open source JavaScript library and used as the basis for an evolving suite of demos.
New work is underway on vector space approaches inspired by human cognition and the advances in generative AI. This will mimic human language processing (sequential, hierarchical and predictive), implicit and explicit memory, continual learning, and Type 1 and 2 cognition. This is being implemented in Python and PyTorch. Language processing uses retained feedback connections in conjunction with a small sliding window to mimic the buffering limitations of the phonological loop. Type 2 cognition features a vector based implementation of chunks and rules. Explicit memory (episodic and encyclopaedic) is based upon a vector database designed to mimic characteristics of human memory (forgetting curve, spreading activation and spacing effect). The different modules are integrated through shared access to the latent semantics (loosely equivalent to the buffers in the above diagram).
In the long run, the mission of the Cognitive AI Community Group is to enable cognitive agents that:
- Are knowledgeable, general purpose, creative, collaborative, empathic, sociable and trustworthy
- Can apply metacognition and past experience to reason about new situations
- Support continual learning based upon curiosity about the unexpected
- Have a level of self awareness in respect to current state, goals and actions
- Have an awareness of others in respect to their beliefs, desires and intents
- Are multilingual and can interact with people using their own language
These topics can be divided into areas for study and exploration with an emphasis on identifying use cases and building demonstrators that advance the overall mission. There is plenty to exploit along the way, with many opportunities to spin off practical applications as work proceeds.
For Tasks:
Click tags to check more tools for each tasksFor Jobs:
Alternative AI tools for cogai
Similar Open Source Tools
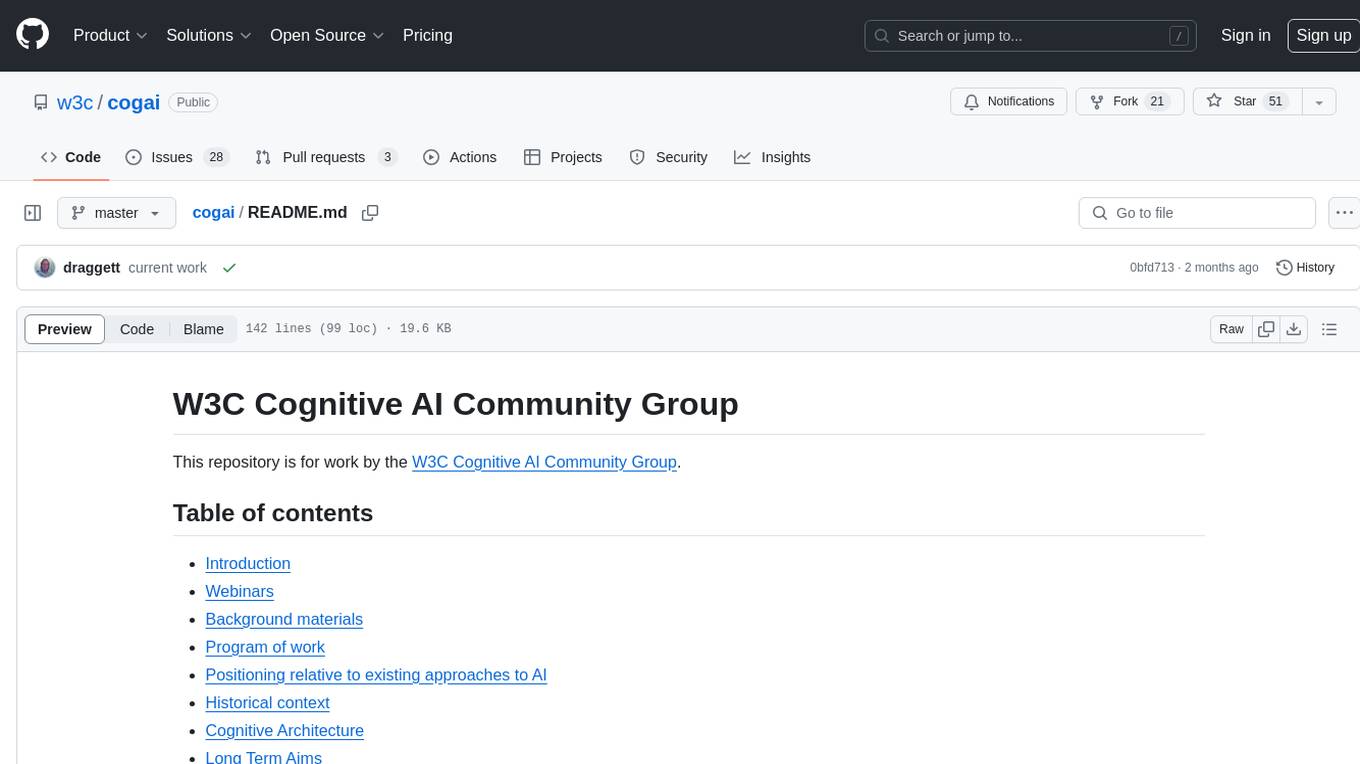
cogai
The W3C Cognitive AI Community Group focuses on advancing Cognitive AI through collaboration on defining use cases, open source implementations, and application areas. The group aims to demonstrate the potential of Cognitive AI in various domains such as customer services, healthcare, cybersecurity, online learning, autonomous vehicles, manufacturing, and web search. They work on formal specifications for chunk data and rules, plausible knowledge notation, and neural networks for human-like AI. The group positions Cognitive AI as a combination of symbolic and statistical approaches inspired by human thought processes. They address research challenges including mimicry, emotional intelligence, natural language processing, and common sense reasoning. The long-term goal is to develop cognitive agents that are knowledgeable, creative, collaborative, empathic, and multilingual, capable of continual learning and self-awareness.
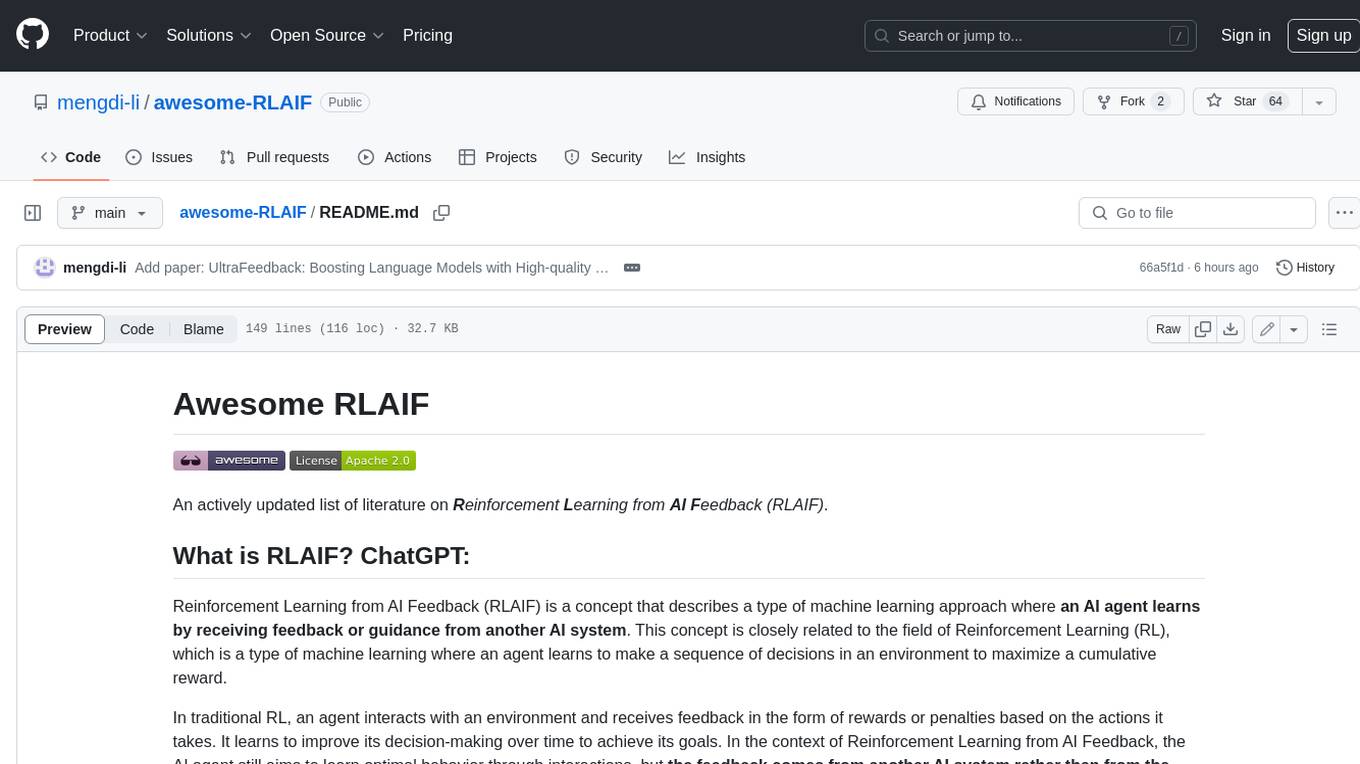
awesome-RLAIF
Reinforcement Learning from AI Feedback (RLAIF) is a concept that describes a type of machine learning approach where **an AI agent learns by receiving feedback or guidance from another AI system**. This concept is closely related to the field of Reinforcement Learning (RL), which is a type of machine learning where an agent learns to make a sequence of decisions in an environment to maximize a cumulative reward. In traditional RL, an agent interacts with an environment and receives feedback in the form of rewards or penalties based on the actions it takes. It learns to improve its decision-making over time to achieve its goals. In the context of Reinforcement Learning from AI Feedback, the AI agent still aims to learn optimal behavior through interactions, but **the feedback comes from another AI system rather than from the environment or human evaluators**. This can be **particularly useful in situations where it may be challenging to define clear reward functions or when it is more efficient to use another AI system to provide guidance**. The feedback from the AI system can take various forms, such as: - **Demonstrations** : The AI system provides demonstrations of desired behavior, and the learning agent tries to imitate these demonstrations. - **Comparison Data** : The AI system ranks or compares different actions taken by the learning agent, helping it to understand which actions are better or worse. - **Reward Shaping** : The AI system provides additional reward signals to guide the learning agent's behavior, supplementing the rewards from the environment. This approach is often used in scenarios where the RL agent needs to learn from **limited human or expert feedback or when the reward signal from the environment is sparse or unclear**. It can also be used to **accelerate the learning process and make RL more sample-efficient**. Reinforcement Learning from AI Feedback is an area of ongoing research and has applications in various domains, including robotics, autonomous vehicles, and game playing, among others.
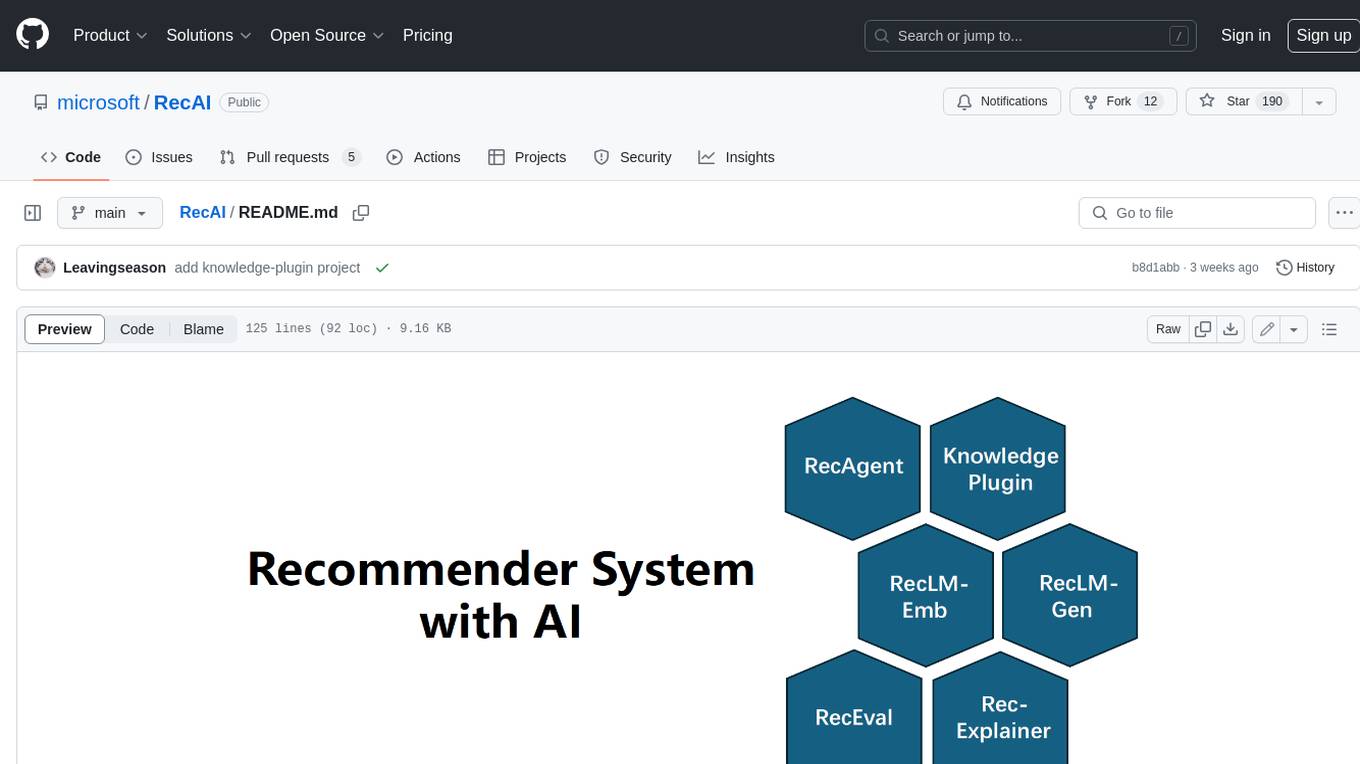
RecAI
RecAI is a project that explores the integration of Large Language Models (LLMs) into recommender systems, addressing the challenges of interactivity, explainability, and controllability. It aims to bridge the gap between general-purpose LLMs and domain-specific recommender systems, providing a holistic perspective on the practical requirements of LLM4Rec. The project investigates various techniques, including Recommender AI agents, selective knowledge injection, fine-tuning language models, evaluation, and LLMs as model explainers, to create more sophisticated, interactive, and user-centric recommender systems.
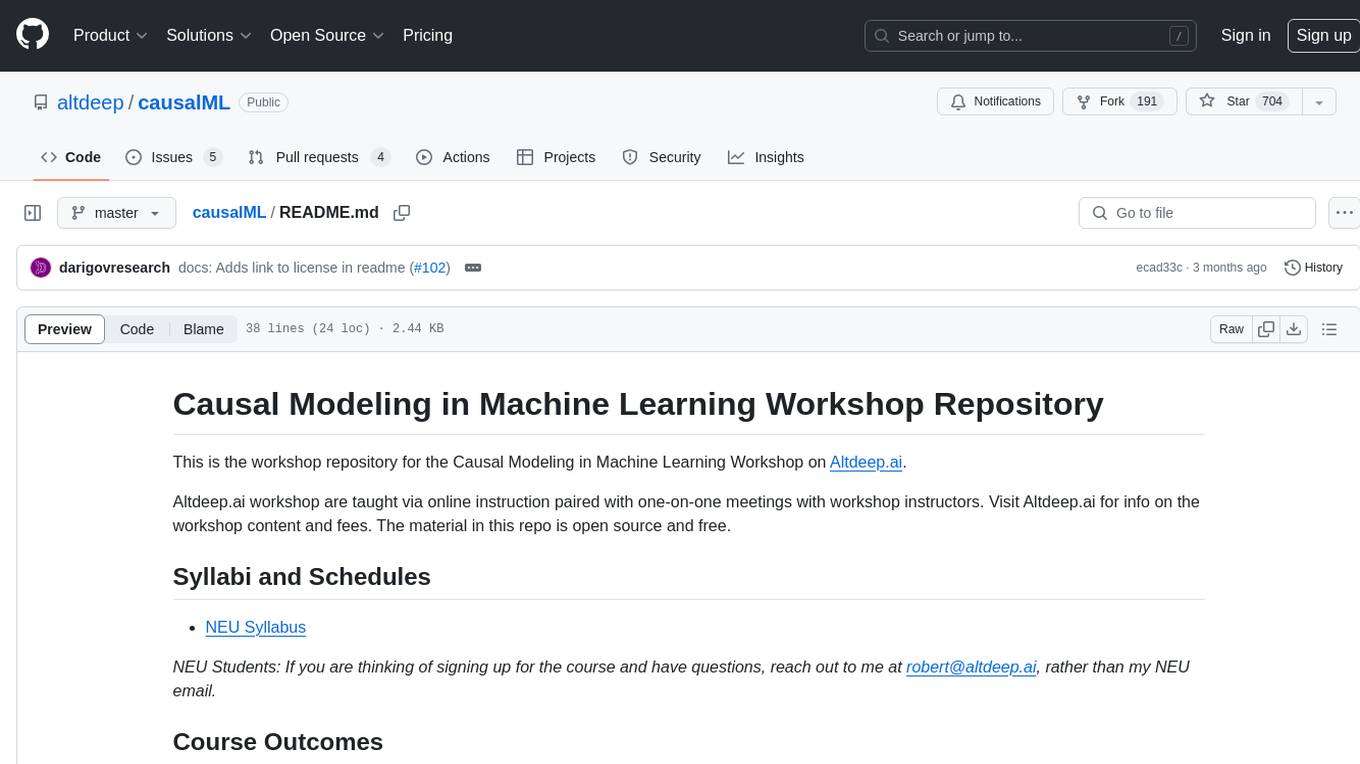
causalML
This repository is the workshop repository for the Causal Modeling in Machine Learning Workshop on Altdeep.ai. The material is open source and free. The course covers causality in model-based machine learning, Bayesian modeling, interventions, counterfactual reasoning, and deep causal latent variable models. It aims to equip learners with the ability to build causal reasoning algorithms into decision-making systems in data science and machine learning teams within top-tier technology organizations.
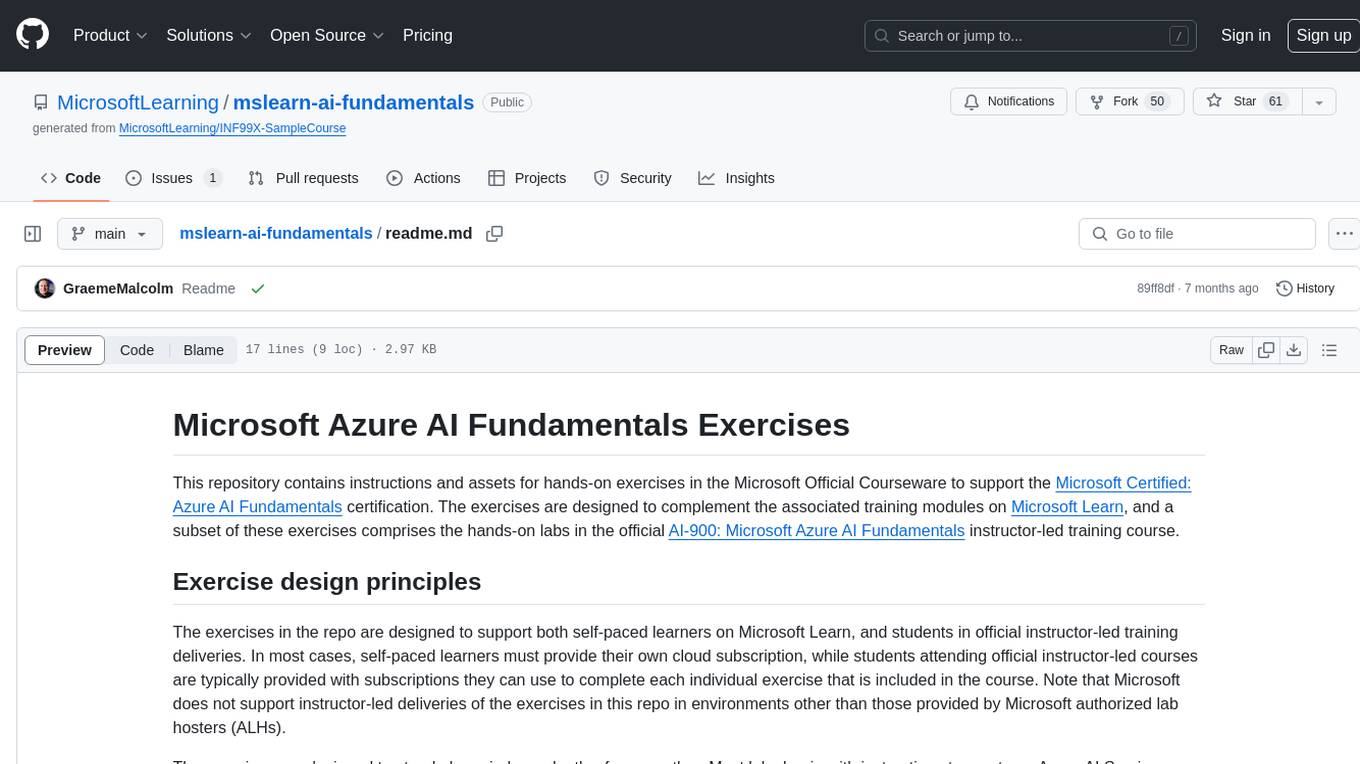
mslearn-ai-fundamentals
This repository contains materials for the Microsoft Learn AI Fundamentals module. It covers the basics of artificial intelligence, machine learning, and data science. The content includes hands-on labs, interactive learning modules, and assessments to help learners understand key concepts and techniques in AI. Whether you are new to AI or looking to expand your knowledge, this module provides a comprehensive introduction to the fundamentals of AI.
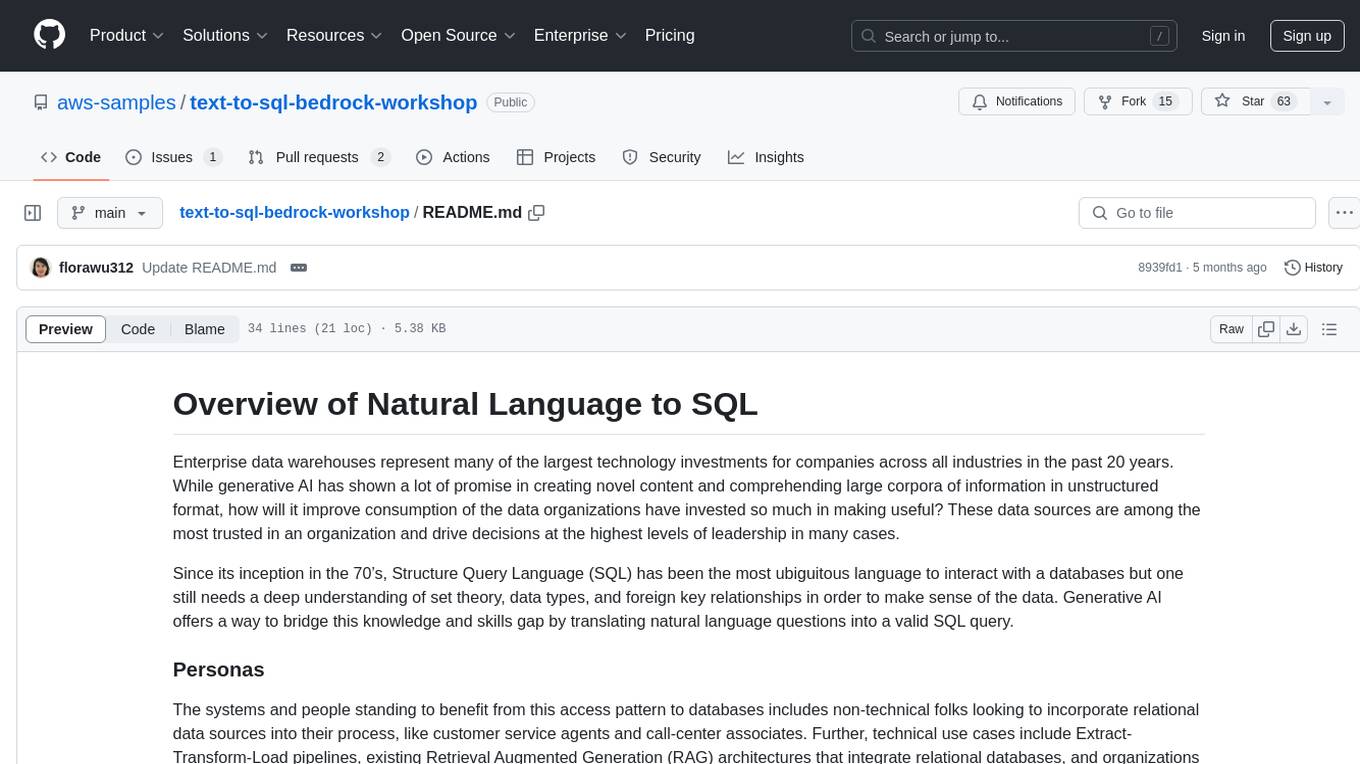
text-to-sql-bedrock-workshop
This repository focuses on utilizing generative AI to bridge the gap between natural language questions and SQL queries, aiming to improve data consumption in enterprise data warehouses. It addresses challenges in SQL query generation, such as foreign key relationships and table joins, and highlights the importance of accuracy metrics like Execution Accuracy (EX) and Exact Set Match Accuracy (EM). The workshop content covers advanced prompt engineering, Retrieval Augmented Generation (RAG), fine-tuning models, and security measures against prompt and SQL injections.
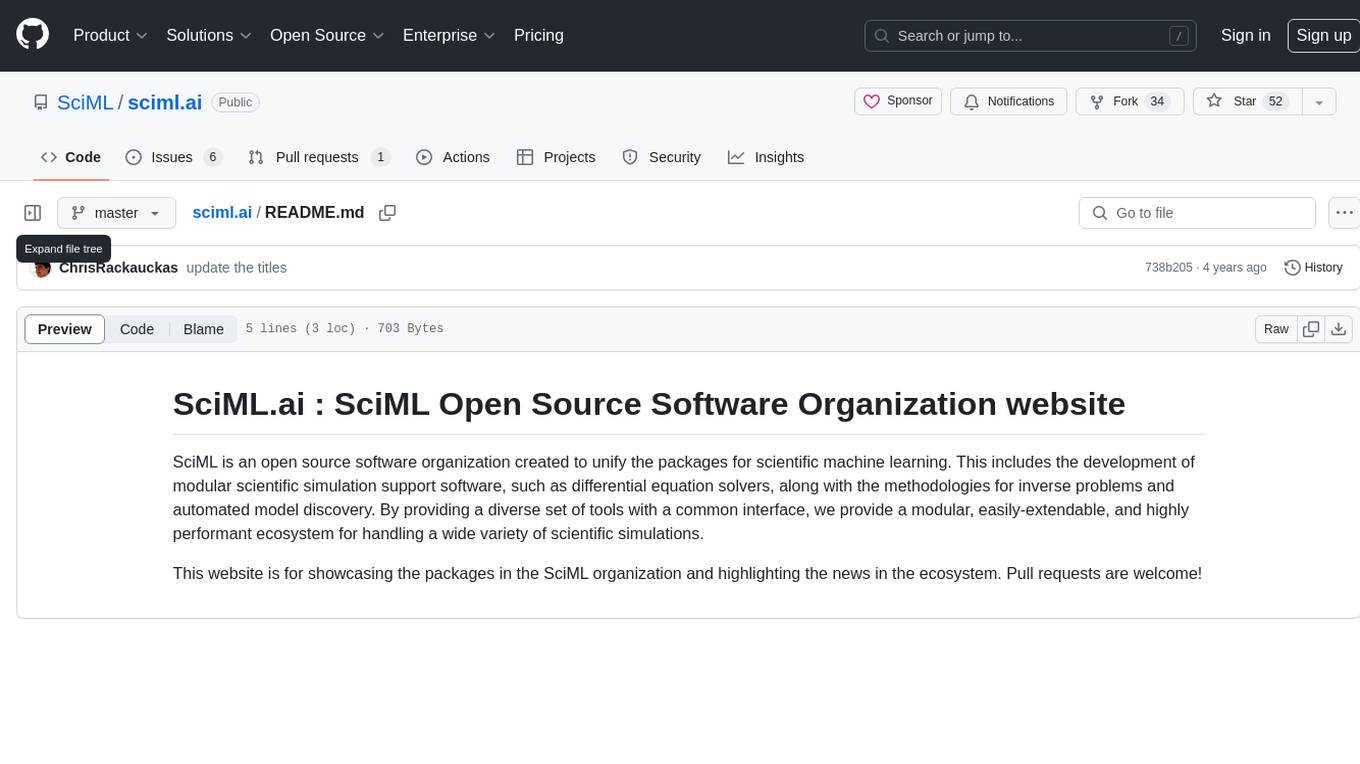
sciml.ai
SciML.ai is an open source software organization dedicated to unifying packages for scientific machine learning. It focuses on developing modular scientific simulation support software, including differential equation solvers, inverse problems methodologies, and automated model discovery. The organization aims to provide a diverse set of tools with a common interface, creating a modular, easily-extendable, and highly performant ecosystem for scientific simulations. The website serves as a platform to showcase SciML organization's packages and share news within the ecosystem. Pull requests are encouraged for contributions.
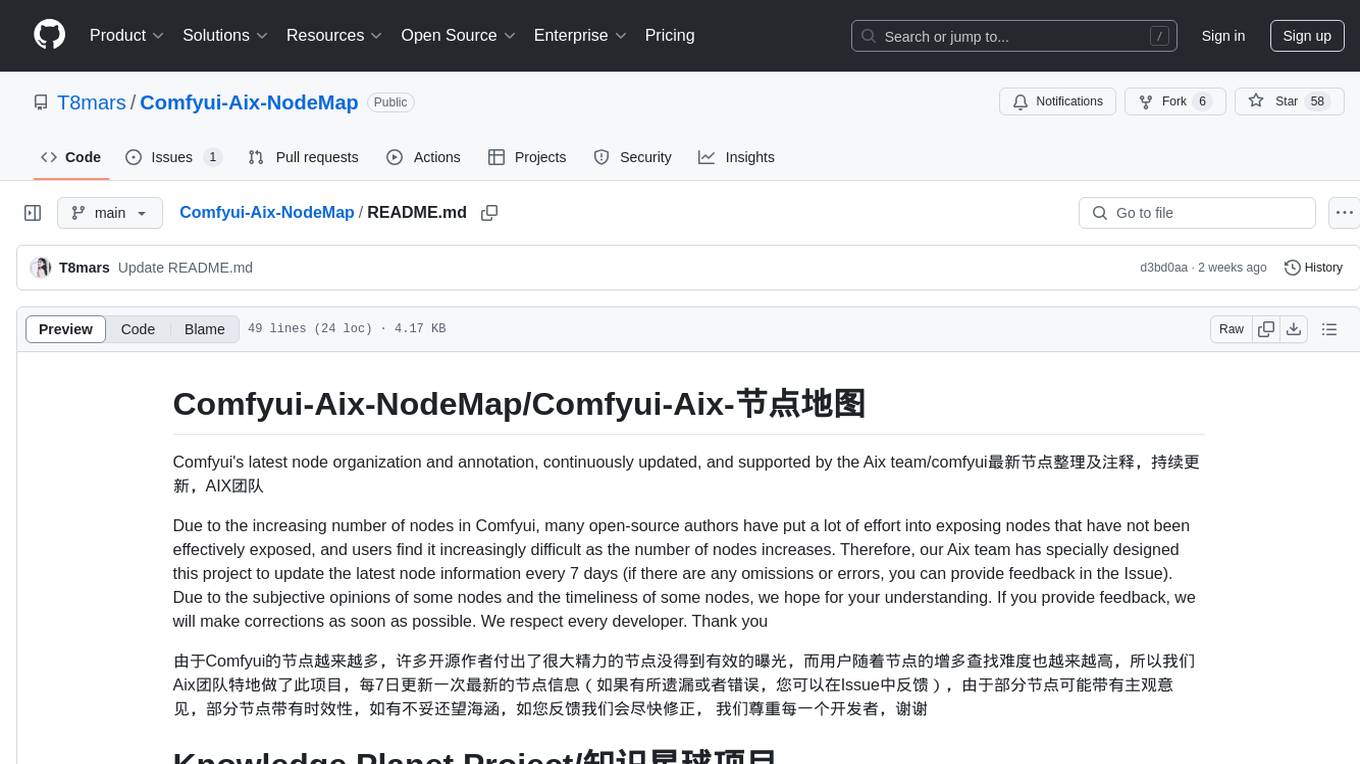
Comfyui-Aix-NodeMap
Comfyui-Aix-NodeMap is a project by the Aix team to organize and annotate the latest nodes in Comfyui. It aims to address the challenge of finding nodes effectively due to the increasing number of nodes. The project is updated every 7 days to provide the most recent node information. Users can provide feedback for any omissions or errors, and corrections will be made promptly. The project respects every developer and values community collaboration in improving node exposure and accessibility.
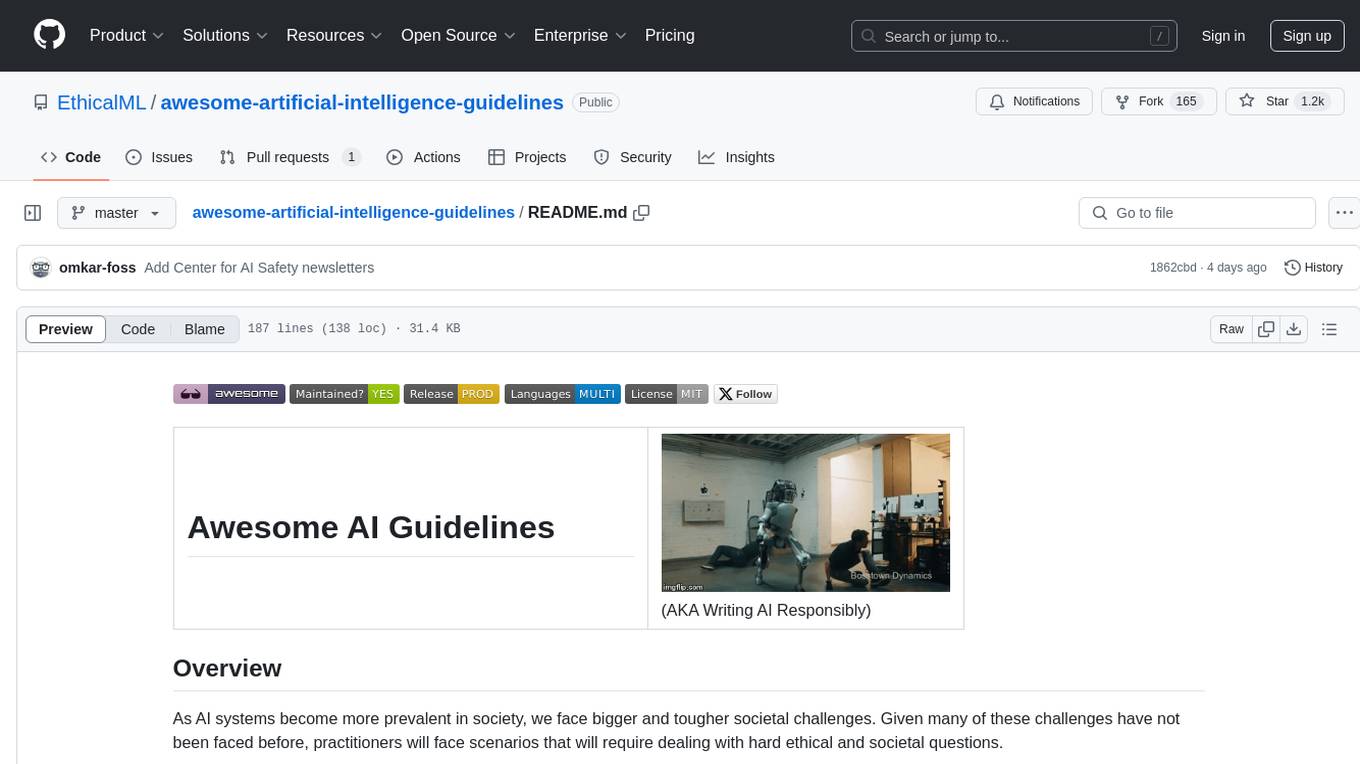
awesome-artificial-intelligence-guidelines
The 'Awesome AI Guidelines' repository aims to simplify the ecosystem of guidelines, principles, codes of ethics, standards, and regulations around artificial intelligence. It provides a comprehensive collection of resources addressing ethical and societal challenges in AI systems, including high-level frameworks, principles, processes, checklists, interactive tools, industry standards initiatives, online courses, research, and industry newsletters, as well as regulations and policies from various countries. The repository serves as a valuable reference for individuals and teams designing, building, and operating AI systems to navigate the complex landscape of AI ethics and governance.
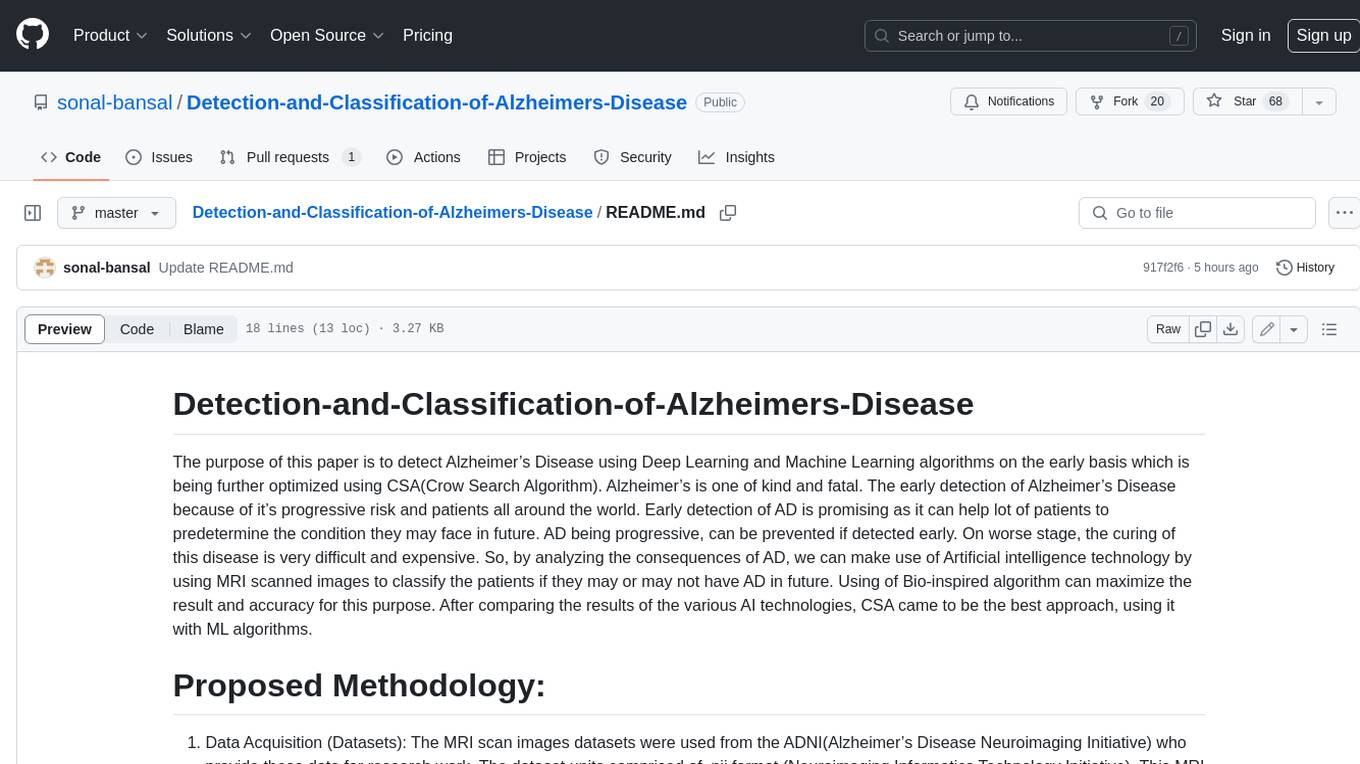
Detection-and-Classification-of-Alzheimers-Disease
This tool is designed to detect and classify Alzheimer's Disease using Deep Learning and Machine Learning algorithms on an early basis, which is further optimized using the Crow Search Algorithm (CSA). Alzheimer's is a fatal disease, and early detection is crucial for patients to predetermine their condition and prevent its progression. By analyzing MRI scanned images using Artificial Intelligence technology, this tool can classify patients who may or may not develop AD in the future. The CSA algorithm, combined with ML algorithms, has proven to be the most effective approach for this purpose.
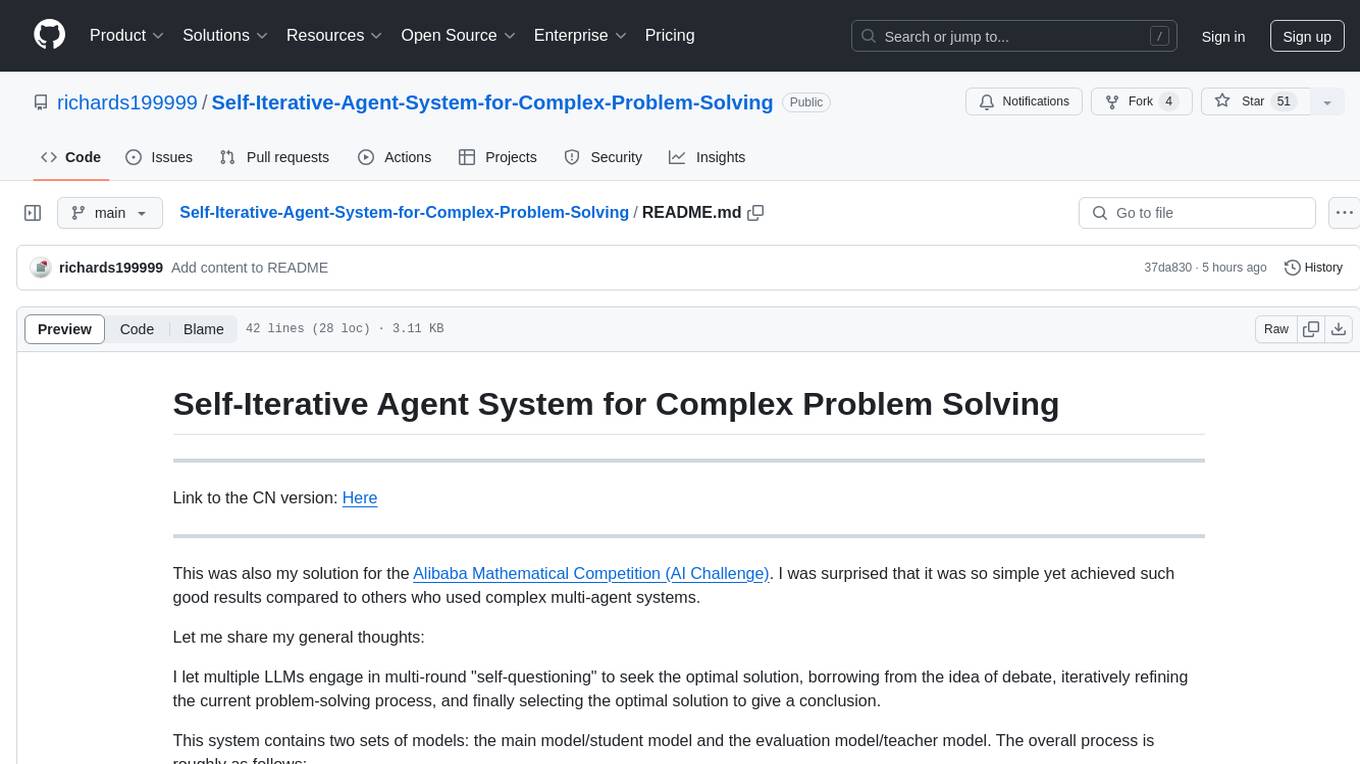
Self-Iterative-Agent-System-for-Complex-Problem-Solving
The Self-Iterative Agent System for Complex Problem Solving is a solution developed for the Alibaba Mathematical Competition (AI Challenge). It involves multiple LLMs engaging in multi-round 'self-questioning' to iteratively refine the problem-solving process and select optimal solutions. The system consists of main and evaluation models, with a process that includes detailed problem-solving steps, feedback loops, and iterative improvements. The approach emphasizes communication and reasoning between sub-agents, knowledge extraction, and the importance of Agent-like architectures in complex tasks. While effective, there is room for improvement in model capabilities and error prevention mechanisms.
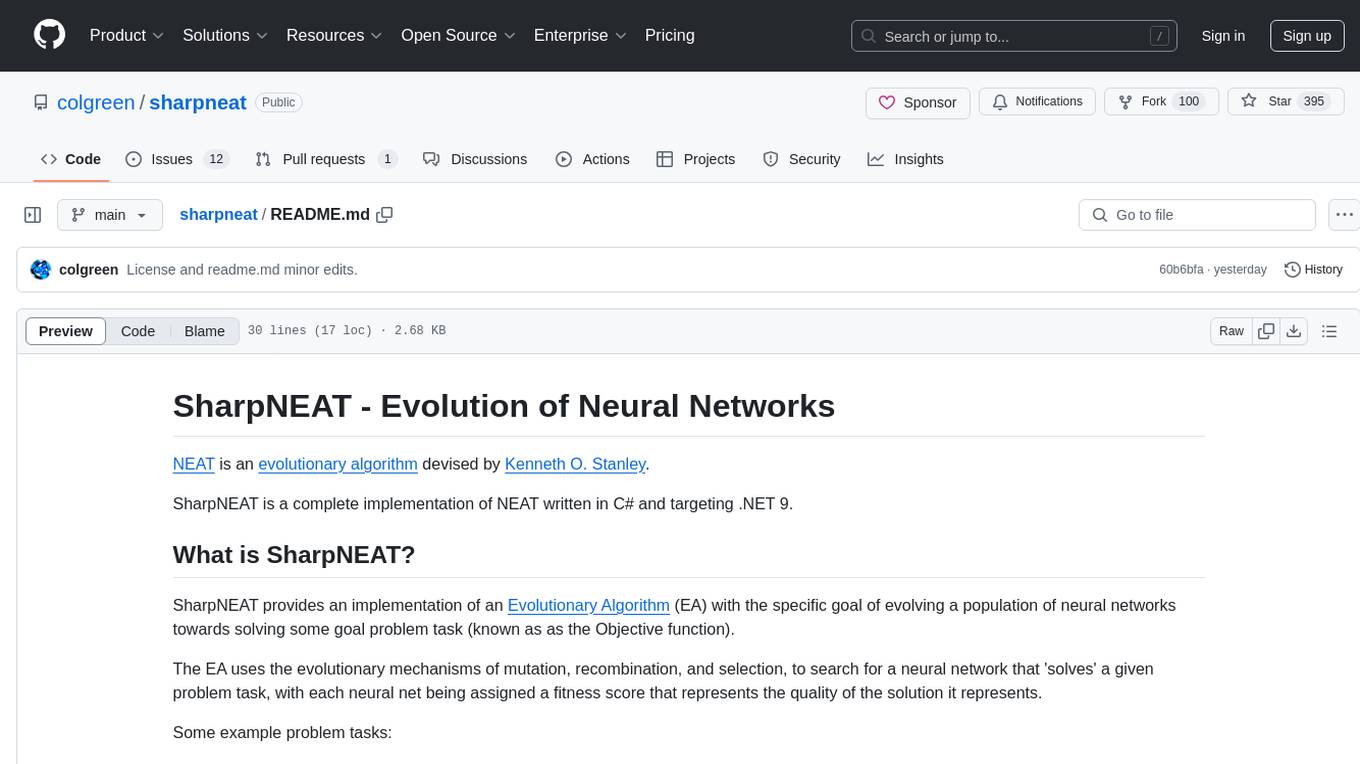
sharpneat
SharpNEAT is a complete implementation of NEAT written in C# and targeting .NET 9. It provides an implementation of an Evolutionary Algorithm (EA) with the specific goal of evolving a population of neural networks towards solving some goal problem task. The framework facilitates research into evolutionary computation and specifically evolution of neural networks, allowing for modular experimentation with genetic coding and evolutionary algorithms.
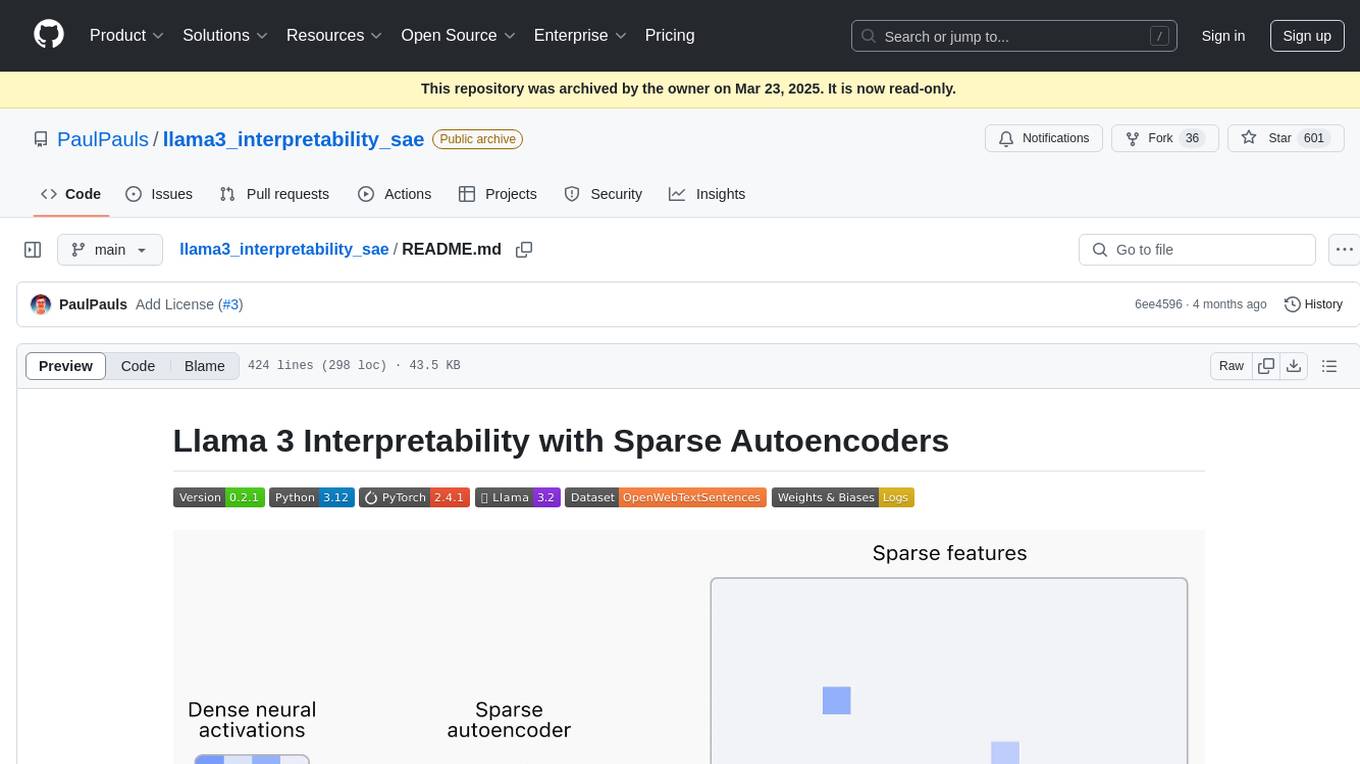
llama3_interpretability_sae
This project focuses on implementing Sparse Autoencoders (SAEs) for mechanistic interpretability in Large Language Models (LLMs) like Llama 3.2-3B. The SAEs aim to untangle superimposed representations in LLMs into separate, interpretable features for each neuron activation. The project provides an end-to-end pipeline for capturing training data, training the SAEs, analyzing learned features, and verifying results experimentally. It includes comprehensive logging, visualization, and checkpointing of SAE training, interpretability analysis tools, and a pure PyTorch implementation of Llama 3.1/3.2 chat and text completion. The project is designed for scalability, efficiency, and maintainability.
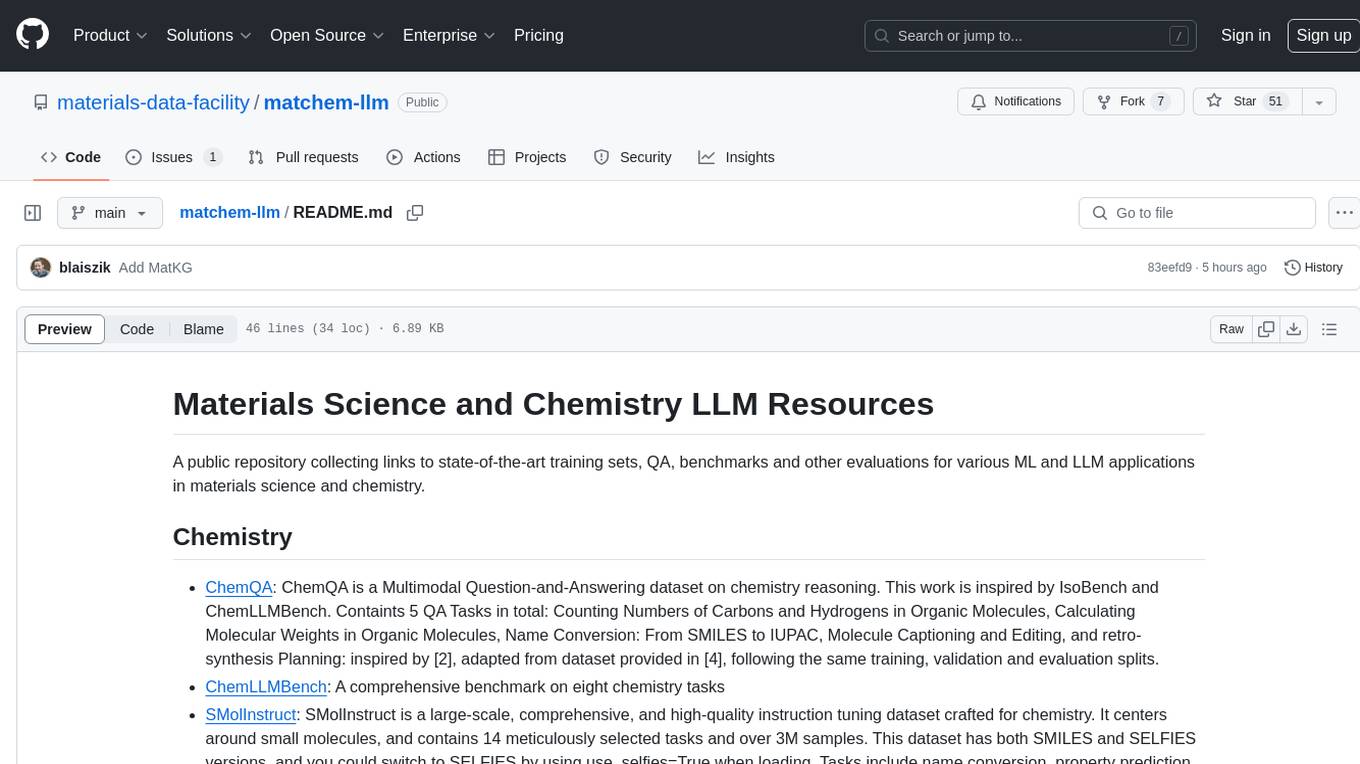
matchem-llm
A public repository collecting links to state-of-the-art training sets, QA, benchmarks and other evaluations for various ML and LLM applications in materials science and chemistry. It includes datasets related to chemistry, materials, multimodal data, and knowledge graphs in the field. The repository aims to provide resources for training and evaluating machine learning models in the materials science and chemistry domains.
For similar tasks
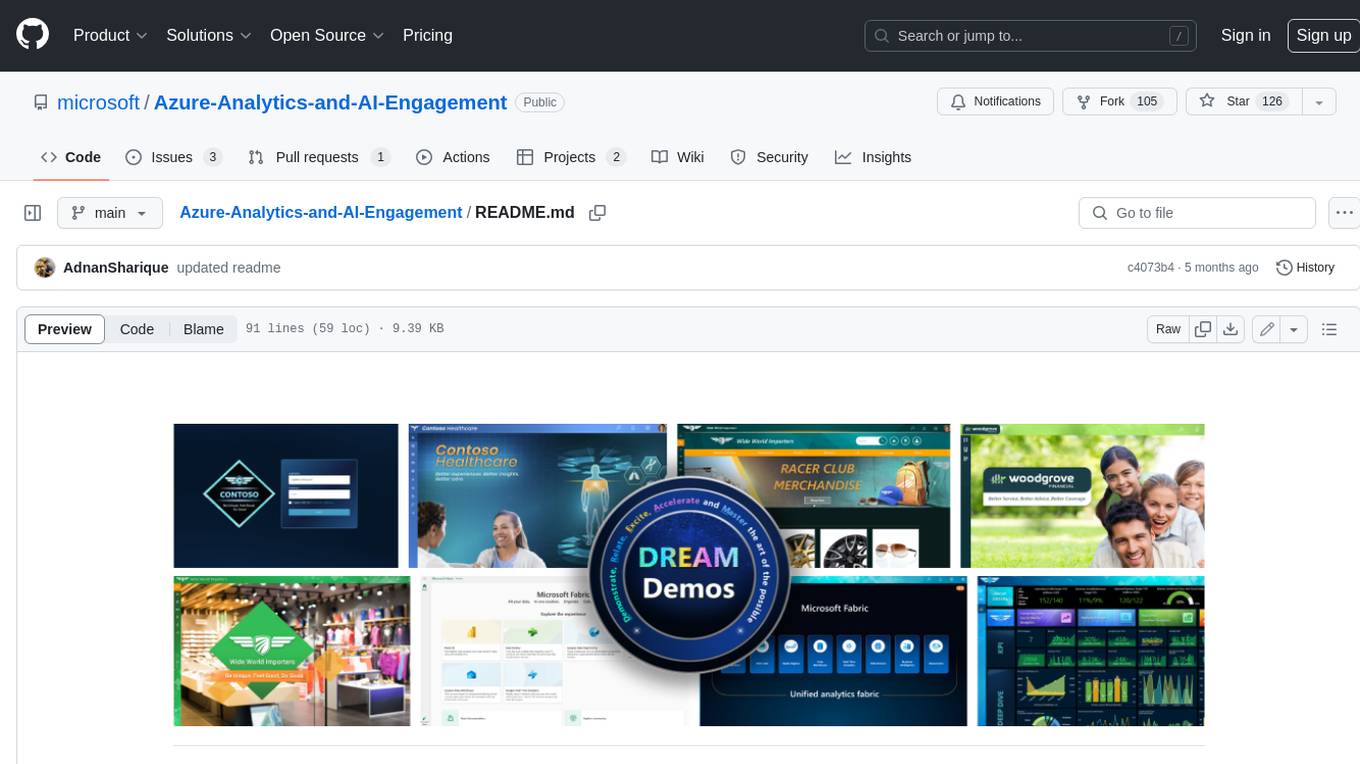
Azure-Analytics-and-AI-Engagement
The Azure-Analytics-and-AI-Engagement repository provides packaged Industry Scenario DREAM Demos with ARM templates (Containing a demo web application, Power BI reports, Synapse resources, AML Notebooks etc.) that can be deployed in a customer’s subscription using the CAPE tool within a matter of few hours. Partners can also deploy DREAM Demos in their own subscriptions using DPoC.
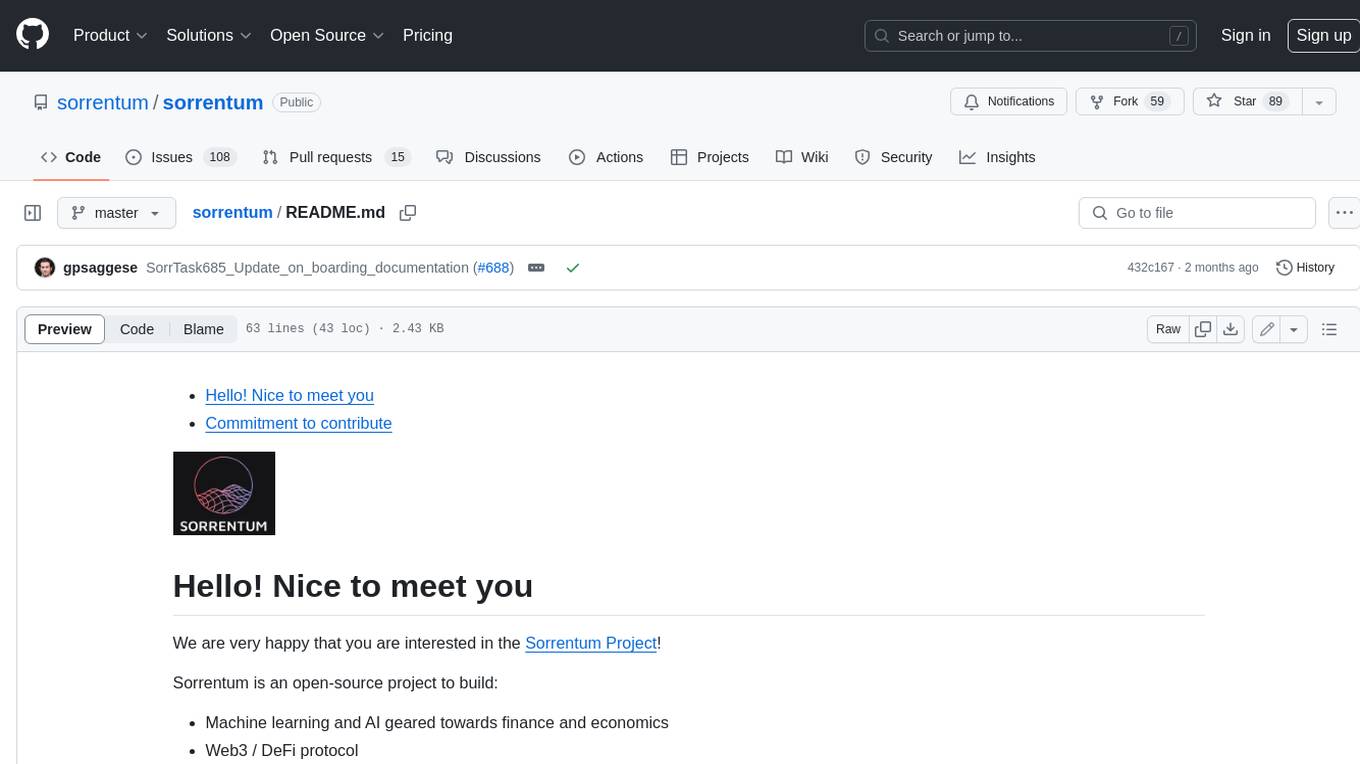
sorrentum
Sorrentum is an open-source project that aims to combine open-source development, startups, and brilliant students to build machine learning, AI, and Web3 / DeFi protocols geared towards finance and economics. The project provides opportunities for internships, research assistantships, and development grants, as well as the chance to work on cutting-edge problems, learn about startups, write academic papers, and get internships and full-time positions at companies working on Sorrentum applications.
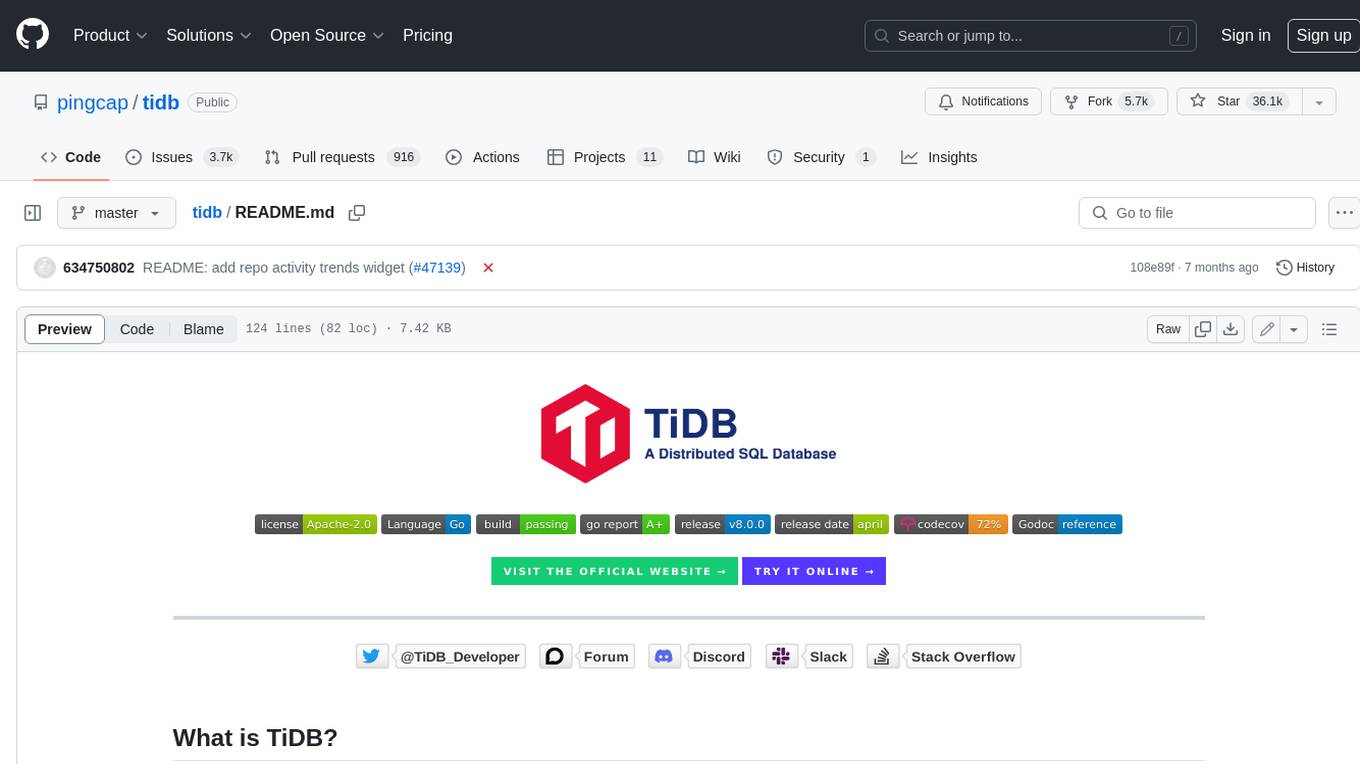
tidb
TiDB is an open-source distributed SQL database that supports Hybrid Transactional and Analytical Processing (HTAP) workloads. It is MySQL compatible and features horizontal scalability, strong consistency, and high availability.
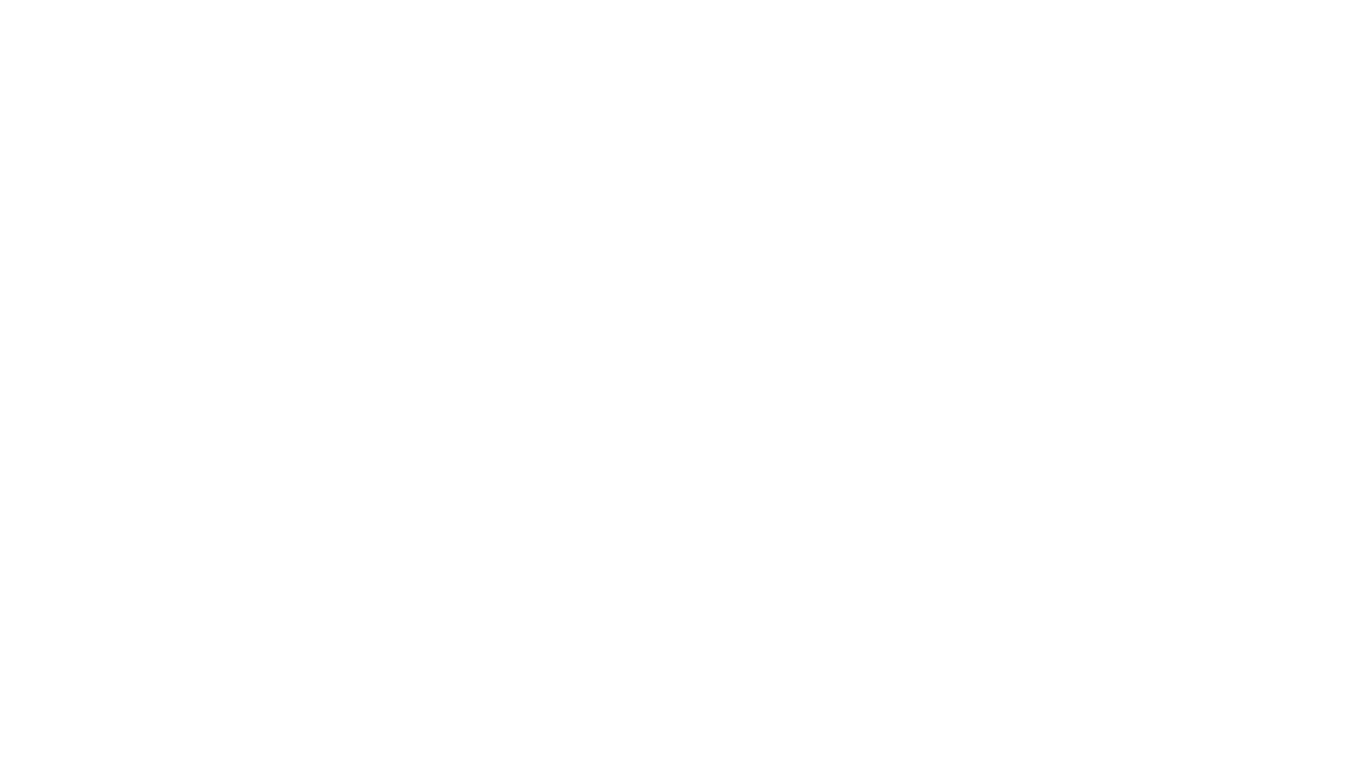
zep-python
Zep is an open-source platform for building and deploying large language model (LLM) applications. It provides a suite of tools and services that make it easy to integrate LLMs into your applications, including chat history memory, embedding, vector search, and data enrichment. Zep is designed to be scalable, reliable, and easy to use, making it a great choice for developers who want to build LLM-powered applications quickly and easily.
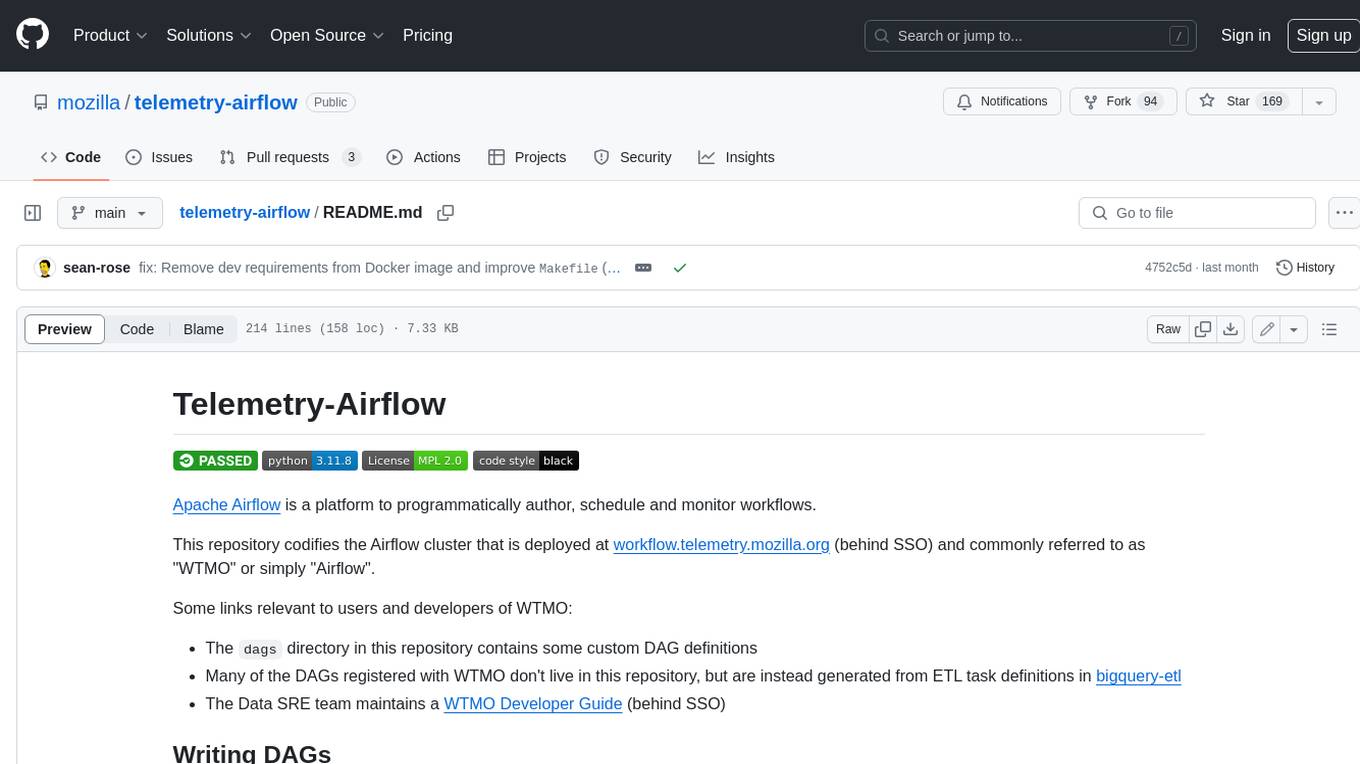
telemetry-airflow
This repository codifies the Airflow cluster that is deployed at workflow.telemetry.mozilla.org (behind SSO) and commonly referred to as "WTMO" or simply "Airflow". Some links relevant to users and developers of WTMO: * The `dags` directory in this repository contains some custom DAG definitions * Many of the DAGs registered with WTMO don't live in this repository, but are instead generated from ETL task definitions in bigquery-etl * The Data SRE team maintains a WTMO Developer Guide (behind SSO)
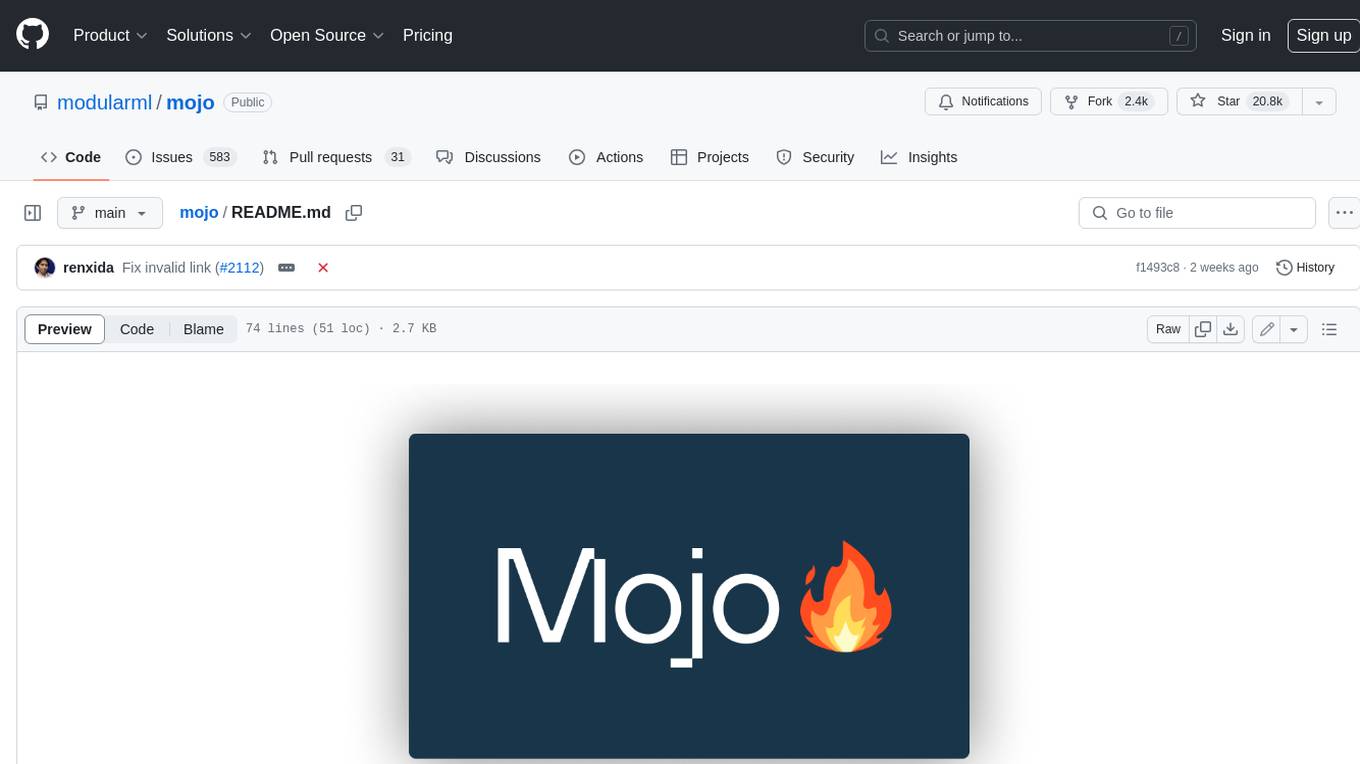
mojo
Mojo is a new programming language that bridges the gap between research and production by combining Python syntax and ecosystem with systems programming and metaprogramming features. Mojo is still young, but it is designed to become a superset of Python over time.
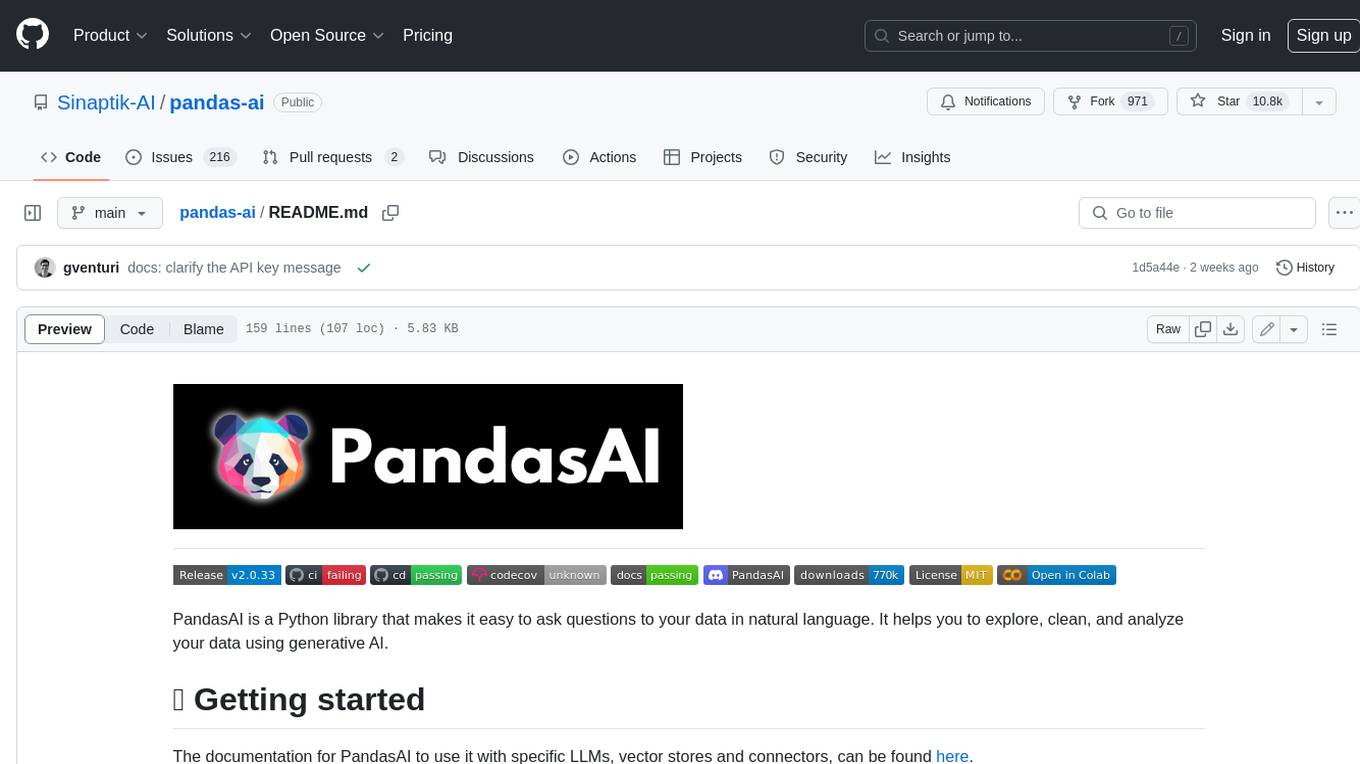
pandas-ai
PandasAI is a Python library that makes it easy to ask questions to your data in natural language. It helps you to explore, clean, and analyze your data using generative AI.
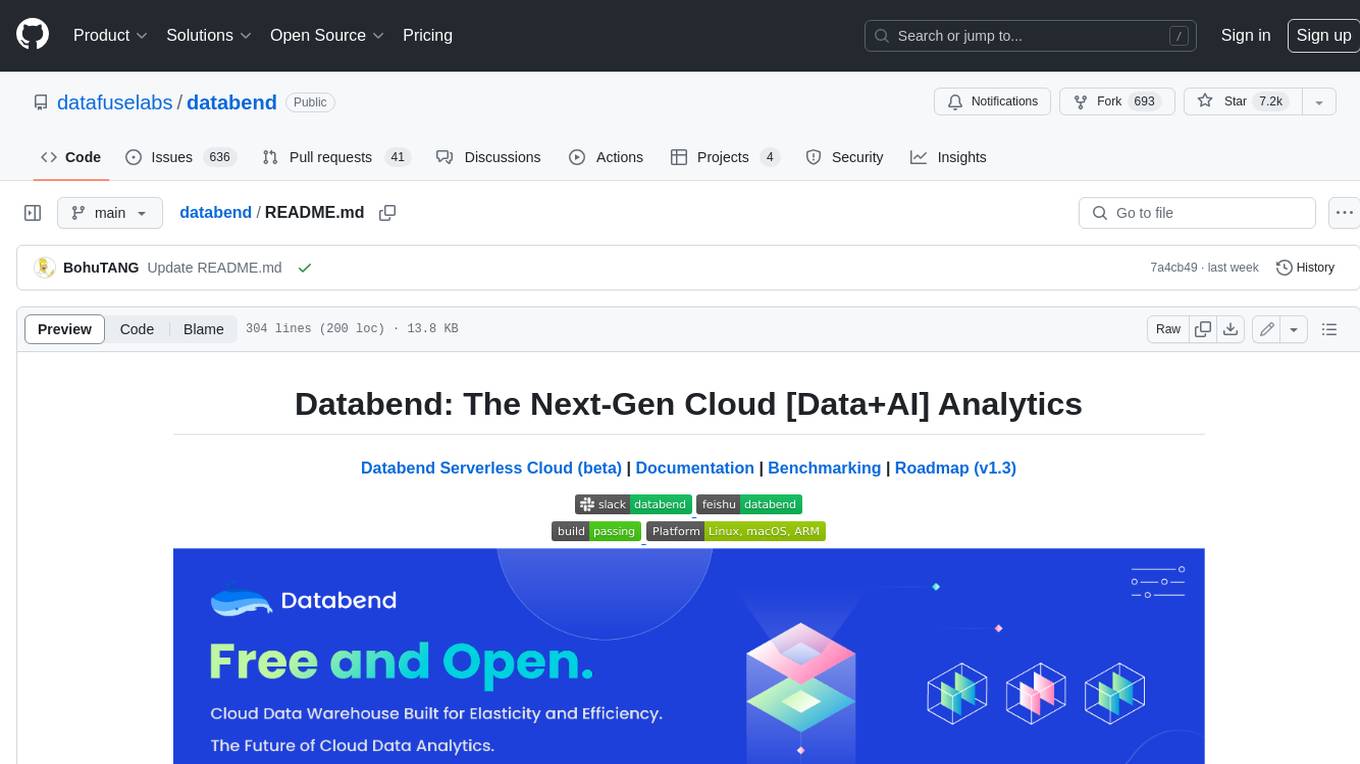
databend
Databend is an open-source cloud data warehouse that serves as a cost-effective alternative to Snowflake. With its focus on fast query execution and data ingestion, it's designed for complex analysis of the world's largest datasets.
For similar jobs
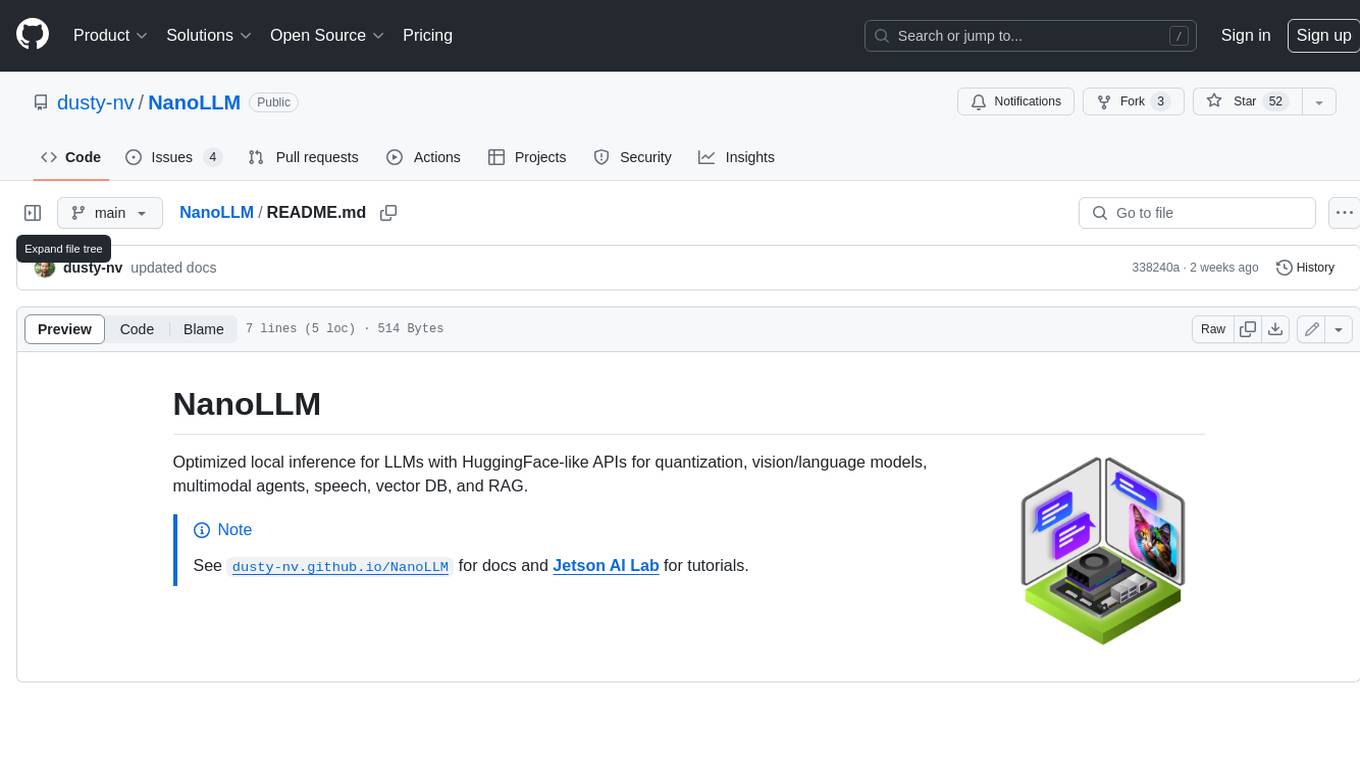
NanoLLM
NanoLLM is a tool designed for optimized local inference for Large Language Models (LLMs) using HuggingFace-like APIs. It supports quantization, vision/language models, multimodal agents, speech, vector DB, and RAG. The tool aims to provide efficient and effective processing for LLMs on local devices, enhancing performance and usability for various AI applications.
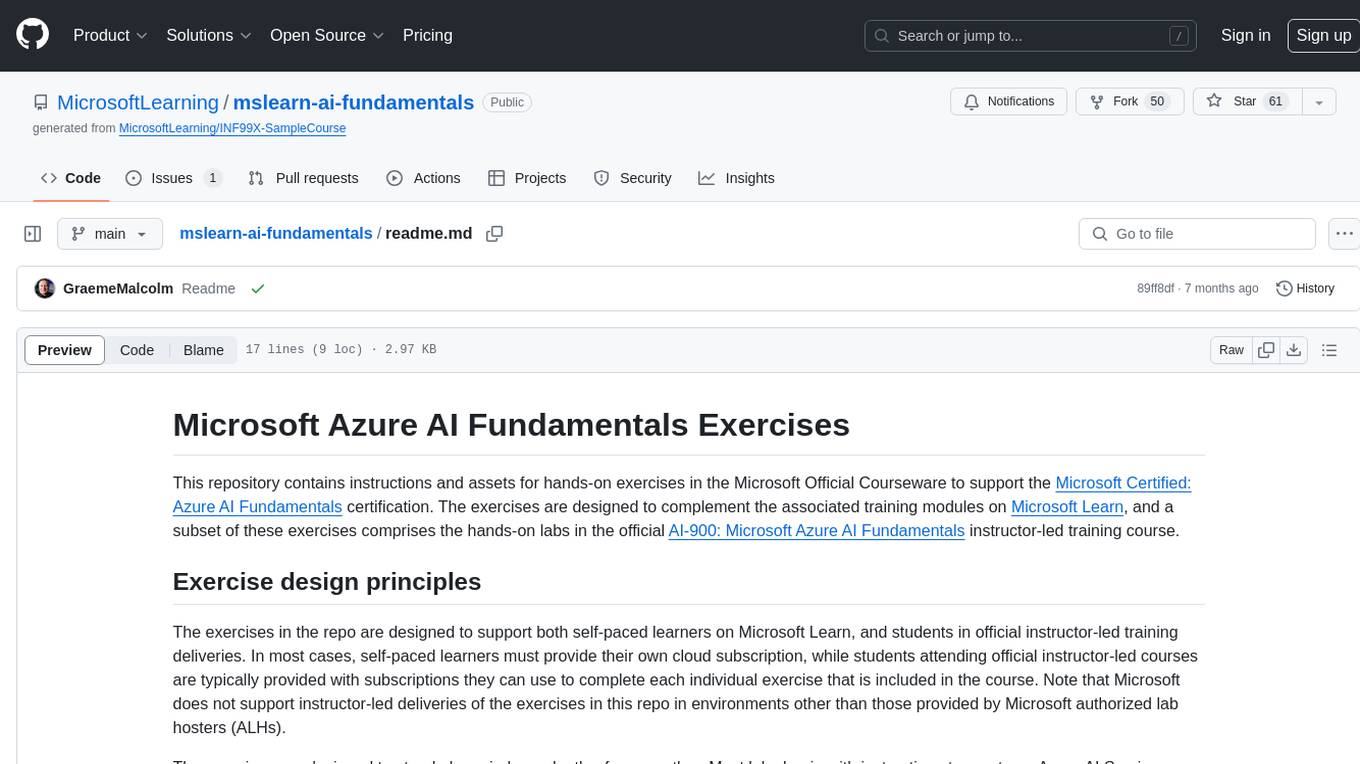
mslearn-ai-fundamentals
This repository contains materials for the Microsoft Learn AI Fundamentals module. It covers the basics of artificial intelligence, machine learning, and data science. The content includes hands-on labs, interactive learning modules, and assessments to help learners understand key concepts and techniques in AI. Whether you are new to AI or looking to expand your knowledge, this module provides a comprehensive introduction to the fundamentals of AI.
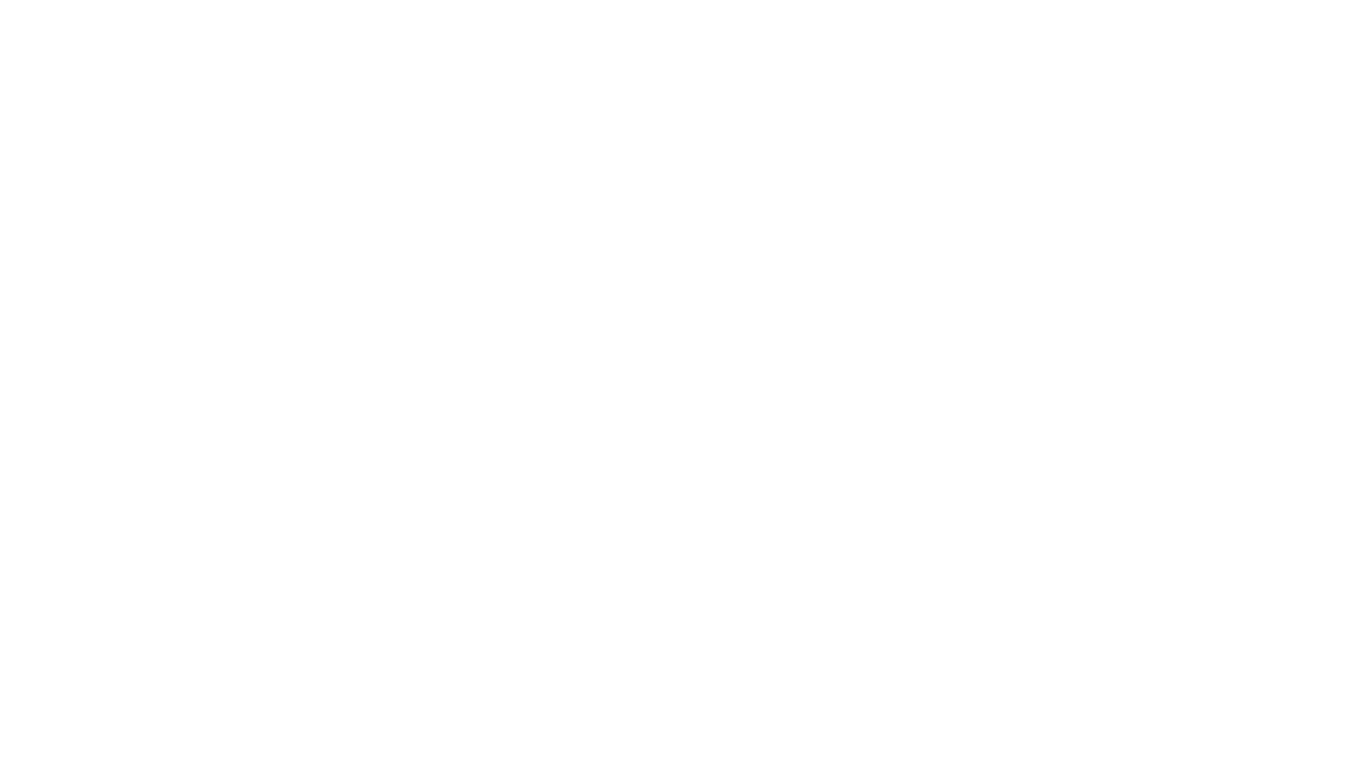
awesome-ai-tools
Awesome AI Tools is a curated list of popular tools and resources for artificial intelligence enthusiasts. It includes a wide range of tools such as machine learning libraries, deep learning frameworks, data visualization tools, and natural language processing resources. Whether you are a beginner or an experienced AI practitioner, this repository aims to provide you with a comprehensive collection of tools to enhance your AI projects and research. Explore the list to discover new tools, stay updated with the latest advancements in AI technology, and find the right resources to support your AI endeavors.
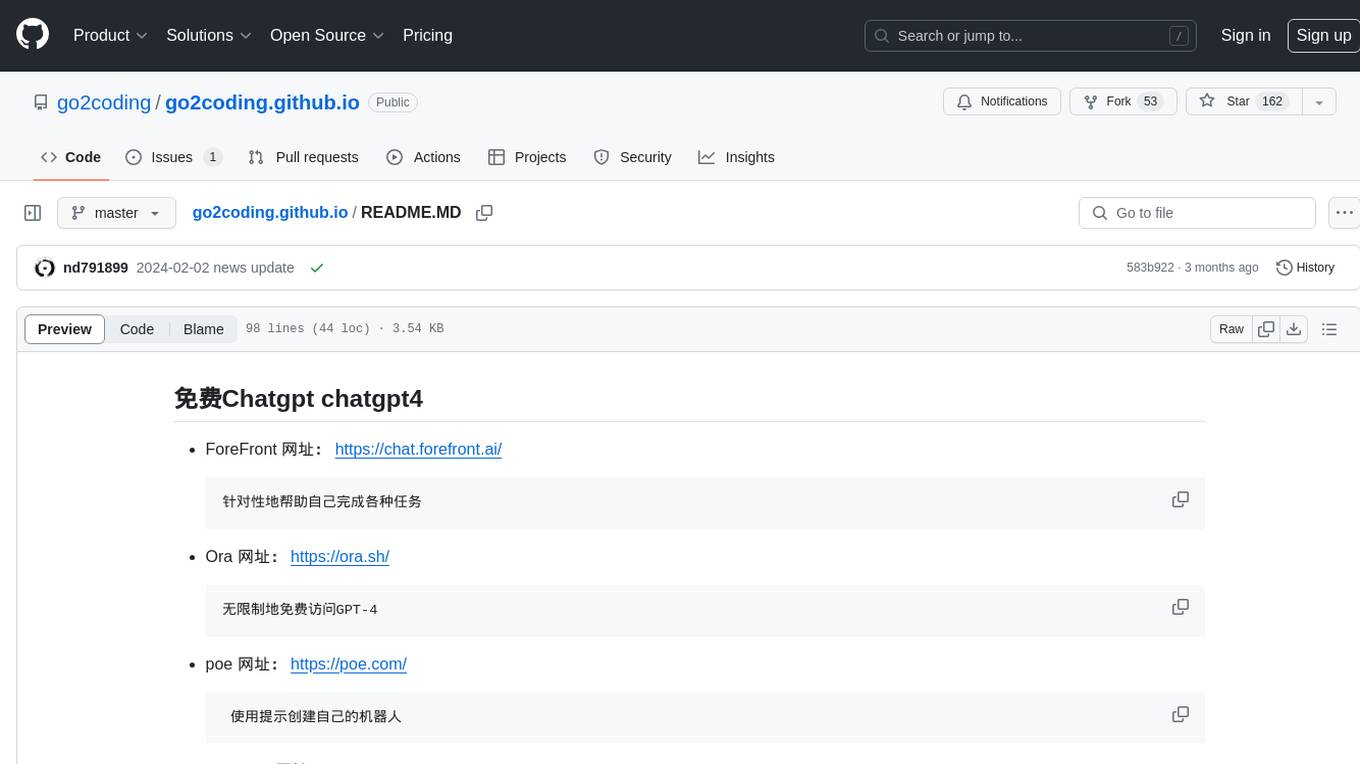
go2coding.github.io
The go2coding.github.io repository is a collection of resources for AI enthusiasts, providing information on AI products, open-source projects, AI learning websites, and AI learning frameworks. It aims to help users stay updated on industry trends, learn from community projects, access learning resources, and understand and choose AI frameworks. The repository also includes instructions for local and external deployment of the project as a static website, with details on domain registration, hosting services, uploading static web pages, configuring domain resolution, and a visual guide to the AI tool navigation website. Additionally, it offers a platform for AI knowledge exchange through a QQ group and promotes AI tools through a WeChat public account.
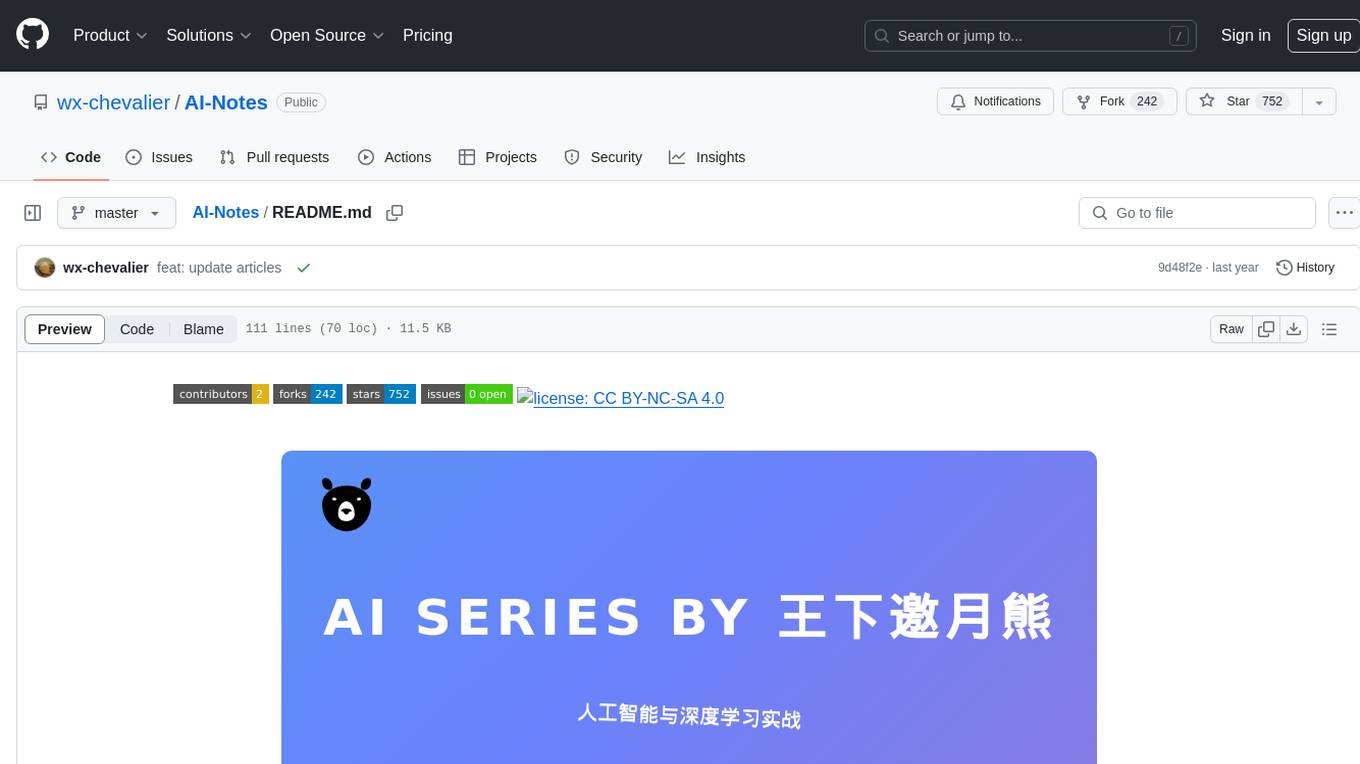
AI-Notes
AI-Notes is a repository dedicated to practical applications of artificial intelligence and deep learning. It covers concepts such as data mining, machine learning, natural language processing, and AI. The repository contains Jupyter Notebook examples for hands-on learning and experimentation. It explores the development stages of AI, from narrow artificial intelligence to general artificial intelligence and superintelligence. The content delves into machine learning algorithms, deep learning techniques, and the impact of AI on various industries like autonomous driving and healthcare. The repository aims to provide a comprehensive understanding of AI technologies and their real-world applications.
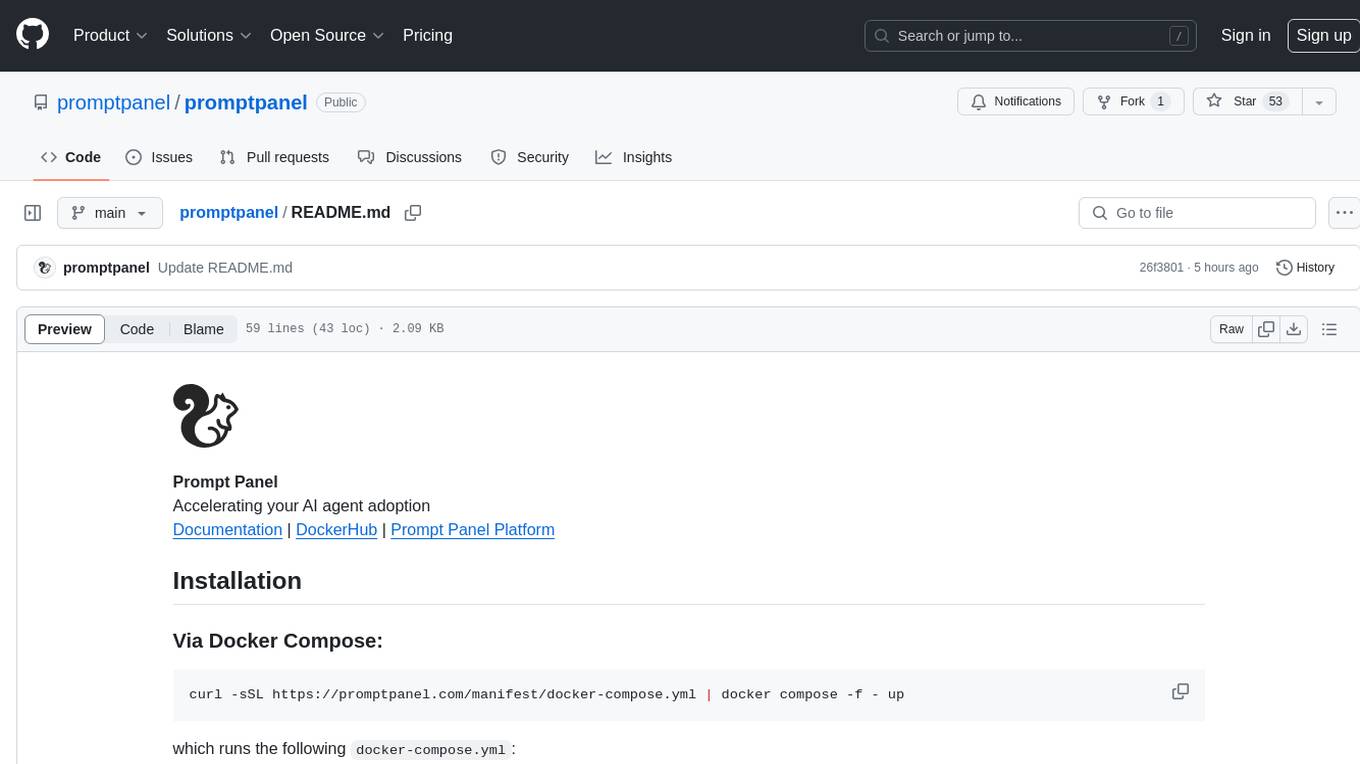
promptpanel
Prompt Panel is a tool designed to accelerate the adoption of AI agents by providing a platform where users can run large language models across any inference provider, create custom agent plugins, and use their own data safely. The tool allows users to break free from walled-gardens and have full control over their models, conversations, and logic. With Prompt Panel, users can pair their data with any language model, online or offline, and customize the system to meet their unique business needs without any restrictions.
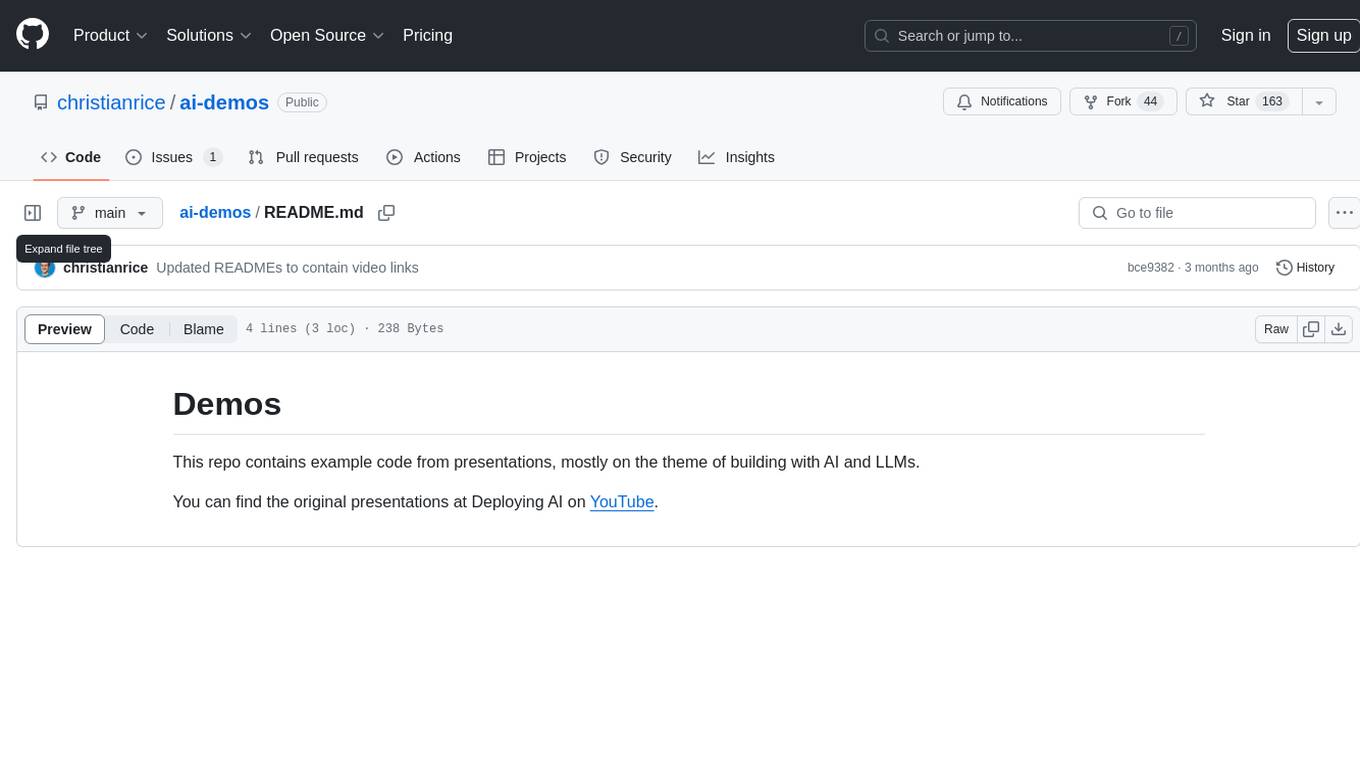
ai-demos
The 'ai-demos' repository is a collection of example code from presentations focusing on building with AI and LLMs. It serves as a resource for developers looking to explore practical applications of artificial intelligence in their projects. The code snippets showcase various techniques and approaches to leverage AI technologies effectively. The repository aims to inspire and educate developers on integrating AI solutions into their applications.
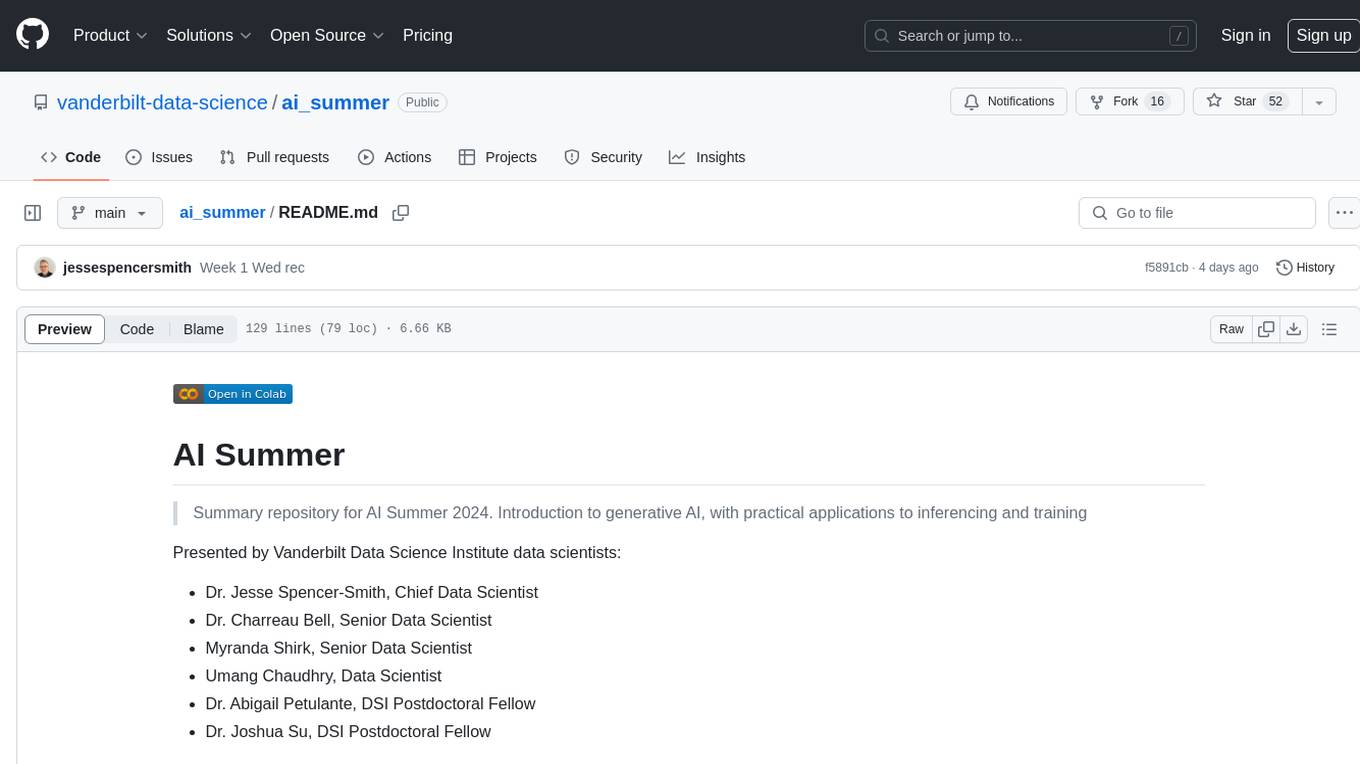
ai_summer
AI Summer is a repository focused on providing workshops and resources for developing foundational skills in generative AI models and transformer models. The repository offers practical applications for inferencing and training, with a specific emphasis on understanding and utilizing advanced AI chat models like BingGPT. Participants are encouraged to engage in interactive programming environments, decide on projects to work on, and actively participate in discussions and breakout rooms. The workshops cover topics such as generative AI models, retrieval-augmented generation, building AI solutions, and fine-tuning models. The goal is to equip individuals with the necessary skills to work with AI technologies effectively and securely, both locally and in the cloud.