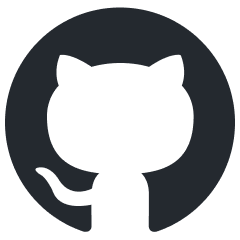
llm4ad
LLM4AD: A Platform for Algorithm Design with Large Language Model
Stars: 287
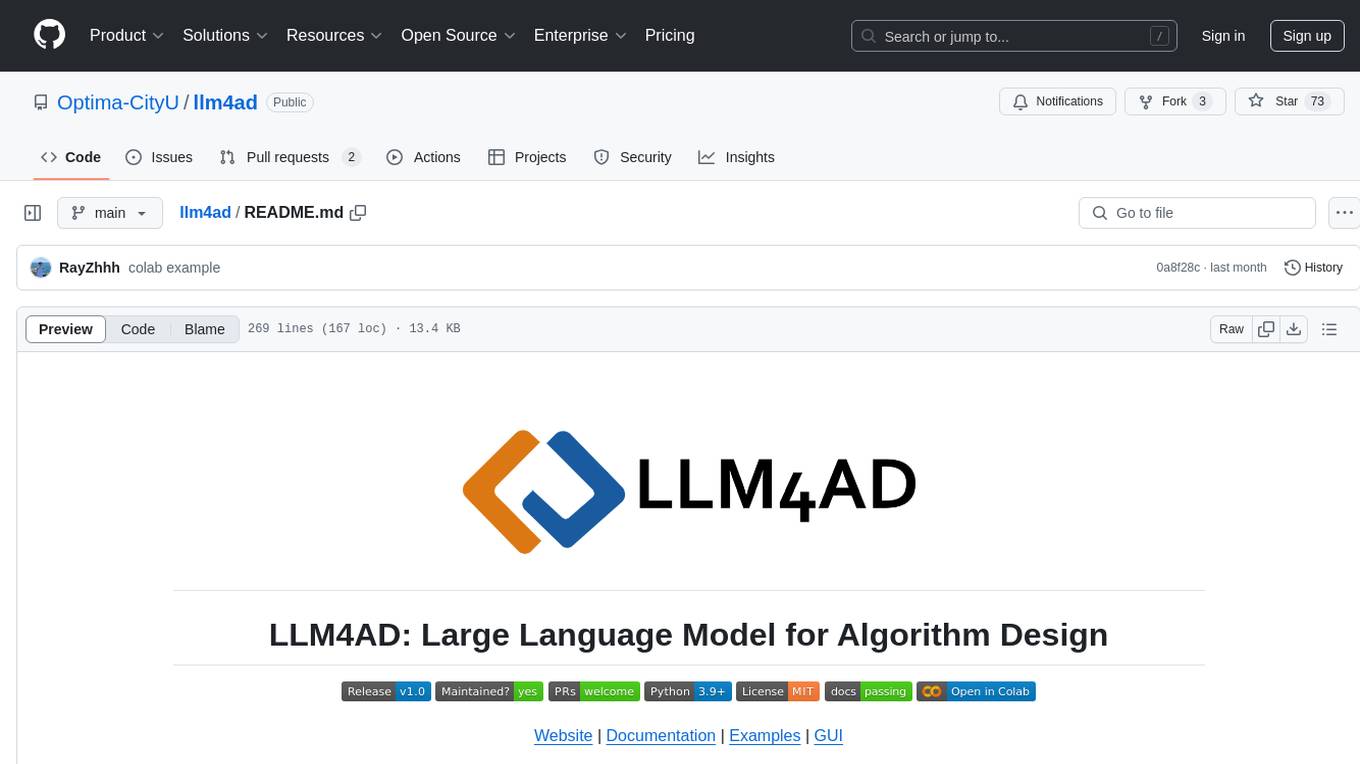
LLM4AD is an open-source Python-based platform leveraging Large Language Models (LLMs) for Automatic Algorithm Design (AD). It provides unified interfaces for methods, tasks, and LLMs, along with features like evaluation acceleration, secure evaluation, logs, GUI support, and more. The platform was originally developed for optimization tasks but is versatile enough to be used in other areas such as machine learning, science discovery, game theory, and engineering design. It offers various search methods and algorithm design tasks across different domains. LLM4AD supports remote LLM API, local HuggingFace LLM deployment, and custom LLM interfaces. The project is licensed under the MIT License and welcomes contributions, collaborations, and issue reports.
README:
LLM4AD is an open-source Python-based Platform leveraging Large Language Models (LLMs) for Automatic Algorithm Design (AD). Please refer to the paper [LLM4AD] for detailed information, including the overview, methodology, and benchmark results.
LLM4AD is accomplished with Documents and Examples materials to support users and developers to easily test, build and deploy their own LLM4AD applications and conduct research.
LLM4AD was originally developed for optimisation tasks. The framework is versatile enough to be used in other areas, including machine learning, science discovery, game theory and engineering design.
For more information, see the contact list
-
2024.12 🎉🎉 LLM4AD paper Released “LLM4AD: A Platform for Algorithm Design with Large Language Model" !
-
2024.11 🎉🎉 LLM4AD v1.0 Released !
-
2024.10 🎉🎉 Survey Paper “A Systematic Survey on Large Language Models for Algorithm Design” is online !
Feature | Support / To be supported |
---|---|
Unified Interfaces for methods | 🔥Support |
Unified Interfaces for tasks | 🔥Support |
Unified Interfaces for LLMs | 🔥Support |
Evaluation acceleration: multiprocessing evaluation | 🔥Support |
Secure Evaluation: main process protection, timeout interruption | 🔥Support |
Logs: local logs, Wandb and Tensorboard support | 🔥Support |
GUI: methods selection, tasks selection, convergence, best algorithm, ... | 🔥Support |
Resume run | 🔥Support |
Support other programming languages | 🚀Coming soon |
More search methods | 🚀Coming soon |
More task examples | 🚀Coming soon |
[!Important] The Python version must be larger or equal to Python 3.9, and less than Python 3.13.
[!Important] If you are testing machine learning tasks or using GUI, please install gym via
pip install gym
. Please note that the gym version may be conflict with your own Python environment, please refer to gym's docs to obtain appropriate version.
[!Important] If you are testing machine learning tasks or using GUI, please install gym via
pip install gym
. Please note that the gym version may be conflict with your own Python environment, please refer to gym's docs to obtain appropriate version.
-
refer to requirements.txt
-
Numba (if you want to use Numba accelerate)
-
Tensorboard (if you want to use a Tensorboard logger)
-
wandb (if you want to use wandb logger)
-
gym (if you want to try GUI, and Machine Learning tasks)
-
pandas (if you want to try Science Discovery tasks)
-
all required packages in requirements.txt (if you want to use GUI)
We suggest to install and run LLM4AD in conda env with python>=3.9, <3.13
cd LLM4AD pip install .
We suggest to install and run LLM4AD in conda env with python>=3.9, <3.13
pip install llm4ad
[!Note] Configure your LLM api before running the script. For example:
- Set
host
: 'api.deepseek.com'- Set
key
: 'your api key'- Set
model
`deepseek-chat'
from llm4ad.task.optimization.online_bin_packing import OBPEvaluation
from llm4ad.tools.llm.llm_api_https import HttpsApi
from llm4ad.method.eoh import EoH, EoHProfiler
def main():
llm = HttpsApi(host="xxx", # your host endpoint, e.g., api.openai.com, api.deepseek.com
key="sk-xxx", # your key, e.g., sk-xxxxxxxxxx
model="xxx", # your llm, e.g., gpt-3.5-turbo, deepseek-chat
timeout=20)
task = OBPEvaluation()
method = EoH(llm=llm,
profiler=EoHProfiler(log_dir='logs/eoh', log_style='simple'),
evaluation=task,
max_sample_nums=20,
max_generations=10,
pop_size=4,
num_samplers=1,
num_evaluators=1,
debug_mode=False)
method.run()
if __name__ == '__main__':
main()
Check Documents for more tasks and examples
[!Important] Install all required packages in requirements.txt for GUI usage
$ cd GUI
$ python run_gui.py
Check GUI Introduction for more information
Methods | Paper title |
---|---|
EoH |
Evolution of Heuristics: Towards Efficient Automatic Algorithm Design Using Large Language Model (ICML 2024) Algorithm Evolution using Large Language Model (Arxiv 2023, AEL, the early version of EoH) |
MEoH | Multi-objective Evolution of Heuristic Using Large Language Model (AAAI 25) |
FunSearch | Mathematical Discoveries from Program Search with Large Language Models (Nature 2024) |
(1+1)-EPS (HillClimbing) |
Understanding the Importance of Evolutionary Search in Automated Heuristic Design with Large Language Models (PPSN 2024) |
RandomSampling | ---- |
Neighborhood search methods | Coming soon |
Multi-objective search methods | Coming soon |
Others | Coming soon |
Area | Algorithm Task | Paper |
---|---|---|
Optimization | [Online Bin Packing, Constructive heuristic] | paper |
Traveling Salesman Problem (TSP), Construct heuristic | paper | |
Traveling Salesman Problem (TSP), Guided local search | paper | |
1-dimensional Bin Packing (BP1D), Construct heuristic | paper | |
2-dimensional Bin Packing (BP2D), Construct heuristic | paper | |
Capacitated Facility Location Problem (CFLP), Construct heuristic | paper | |
Knapsack Problem (KP), Construct heuristic | paper | |
Quadratic Assignment Problem (QAP), Construct heuristic | paper | |
Set Cover Problem (SCP), Construct heuristic | paper | |
Flow Shop Scheduling Problem (FSSP), Construct heuristic | paper | |
Flow Shop Scheduling Problem (FSSP), Guided local search | paper | |
Bayesian Optimization, Cost-aware Acquisition Function Design | paper | |
Machine Learning | Adversarial Attack, Attack strategy | paper |
Acrobot, Heuristic (Agent) | ||
Cart Pole, Heuristic (Agent) | ||
Mountain Car, Heuristic (Agent) | ||
Science Discovery | Computational fluid dynamics, Turbulence model design | paper |
Bacteria Growth, Function | ||
Oscillator, Equation | ||
Stress & Strain, Equation | ||
Math | Admissible Sets | paper |
coming soon ... |
There are three approaches on LLM interface implementation, check Tutorial on LLM interface implementation for more information.
- Remote LLM API (e.g., GPT4o, GPT3.5, Gemini Pro, Deepseek ...) (<Recommended !!!>)
- Local HuggingFace LLM Deployment (e.g., Llamacode, Llama, Gemma, Deepseek, ...)
- Your Implementation If you want to use your own GPT API or local LLMs deployment, please create and add your interface in LLM
A Step-by-step Tutorial on using LLM4AD to solve your algorithm design task is provided here
This project is licensed under the MIT License - see the LICENSE file for details. Parts of this project use code licensed under the Apache License 2.0.
If you find LLM4AD helpful please cite:
@article{liu2024llm4ad,
title = {LLM4AD: A Platform for Algorithm Design with Large Language Model},
author = {Fei Liu and Rui Zhang and Zhuoliang Xie and Rui Sun and Kai Li and Xi Lin and Zhenkun Wang and Zhichao Lu and Qingfu Zhang},
year = {2024},
eprint = {2412.17287},
archivePrefix = {arXiv},
primaryClass = {cs.AI},
url = {https://arxiv.org/abs/2412.17287},
}
This platform is developed and maintained by LLM4AD developer group from the City University of Hong Kong (CityUHK) and the Southern University of Science and Technology (SUSTech). We develop LLM4AD platform for research purposes and hope to contribute to the research area by delivering tools for LLM-based algorithm design methods.
- Contribution: We are more than welcome to contribute (see our 📖 contribution guide) including developing code and ideas to improve our platform.
- Collaborations: If you like our platform, and you would like to use it for profit-making purposes? We are always searching for industrial collaborations because they help direct research to meet the industry’s needs.
- Issue: If you find a bug or you have any kind of concern regarding the correctness, please report us an issue.
- Profit Purpose: If you intend to use LLM4AD for any profit-making purposes, please contact us.
If you are interested in LLM4AD or if you encounter any difficulty using the platform, you can:
-
Visit our website LLM4AD Web
-
Visit our collection a collection of resources and research papers on LLM4AD
-
Visit Discussions to connect with other members of our community
-
Join our QQ Group
-
Contact us through email [email protected]
-
Submit an issue
Any new ideas, features, and improvements are welcomed!
You can contribute to LLM4AD follow our 📖 Contribution Guide.
Thank you for contributing to LLM4AD and welcome to being part of the LLM4AD community! ✨
Fei Liu |
![]() Kai Li |
Rui Sun |
Julian XIE |
Shunyu Yao |
Rui Zhang |
For Tasks:
Click tags to check more tools for each tasksFor Jobs:
Alternative AI tools for llm4ad
Similar Open Source Tools
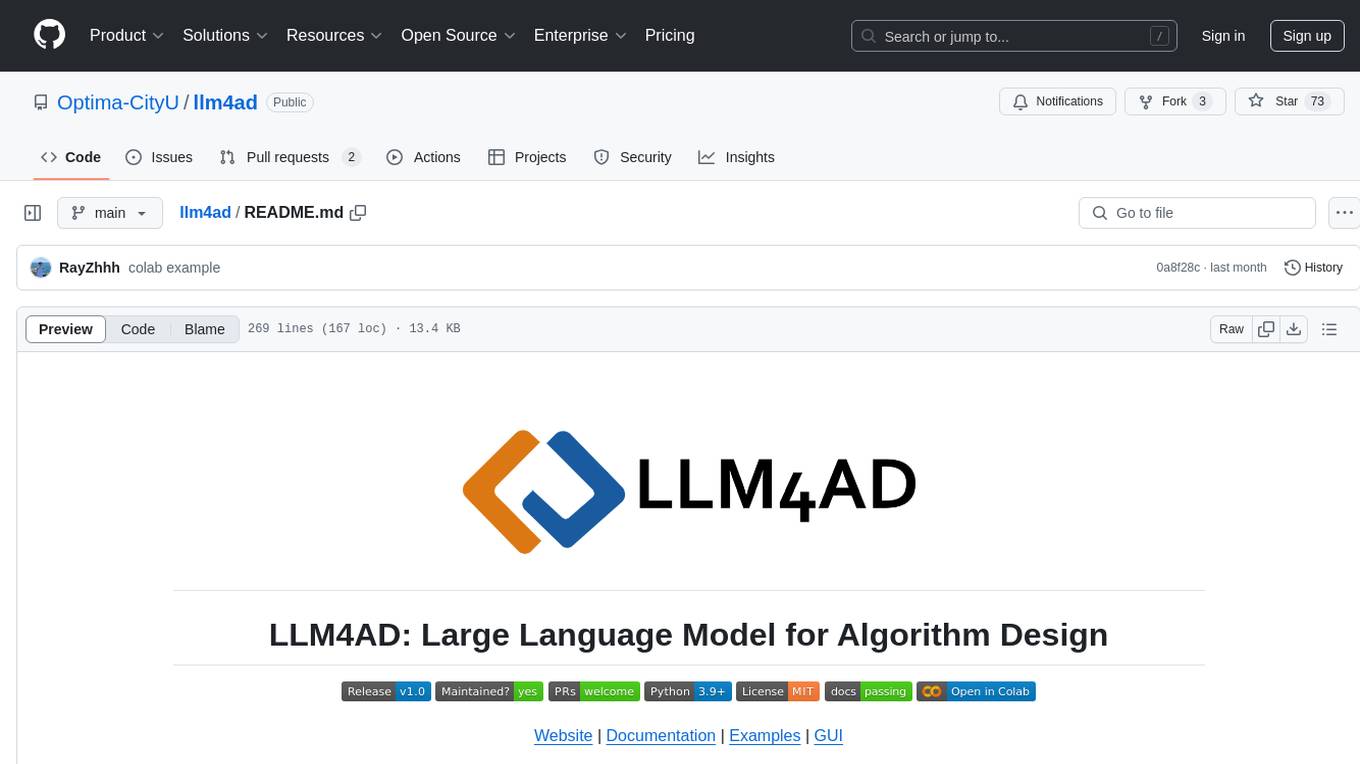
llm4ad
LLM4AD is an open-source Python-based platform leveraging Large Language Models (LLMs) for Automatic Algorithm Design (AD). It provides unified interfaces for methods, tasks, and LLMs, along with features like evaluation acceleration, secure evaluation, logs, GUI support, and more. The platform was originally developed for optimization tasks but is versatile enough to be used in other areas such as machine learning, science discovery, game theory, and engineering design. It offers various search methods and algorithm design tasks across different domains. LLM4AD supports remote LLM API, local HuggingFace LLM deployment, and custom LLM interfaces. The project is licensed under the MIT License and welcomes contributions, collaborations, and issue reports.
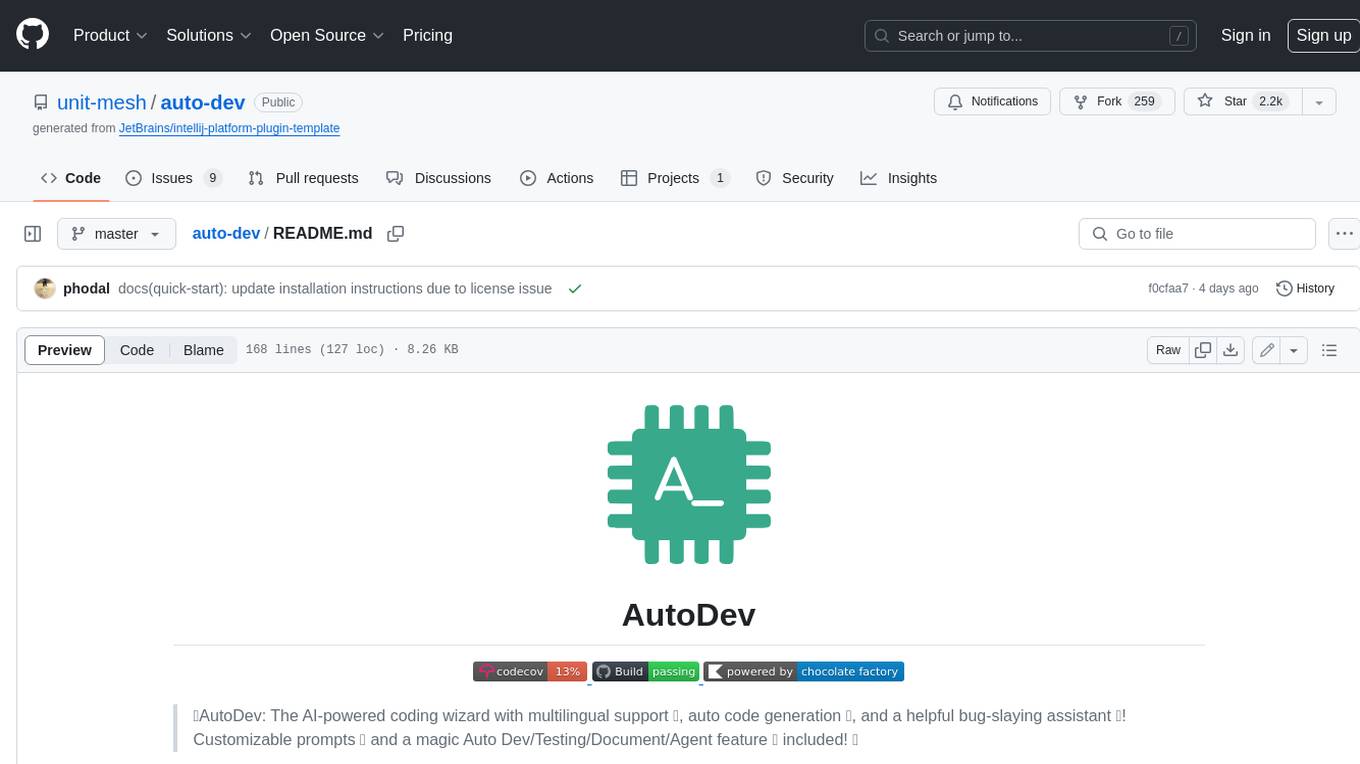
auto-dev
AutoDev is an AI-powered coding wizard that supports multiple languages, including Java, Kotlin, JavaScript/TypeScript, Rust, Python, Golang, C/C++/OC, and more. It offers a range of features, including auto development mode, copilot mode, chat with AI, customization options, SDLC support, custom AI agent integration, and language features such as language support, extensions, and a DevIns language for AI agent development. AutoDev is designed to assist developers with tasks such as auto code generation, bug detection, code explanation, exception tracing, commit message generation, code review content generation, smart refactoring, Dockerfile generation, CI/CD config file generation, and custom shell/command generation. It also provides a built-in LLM fine-tune model and supports UnitEval for LLM result evaluation and UnitGen for code-LLM fine-tune data generation.
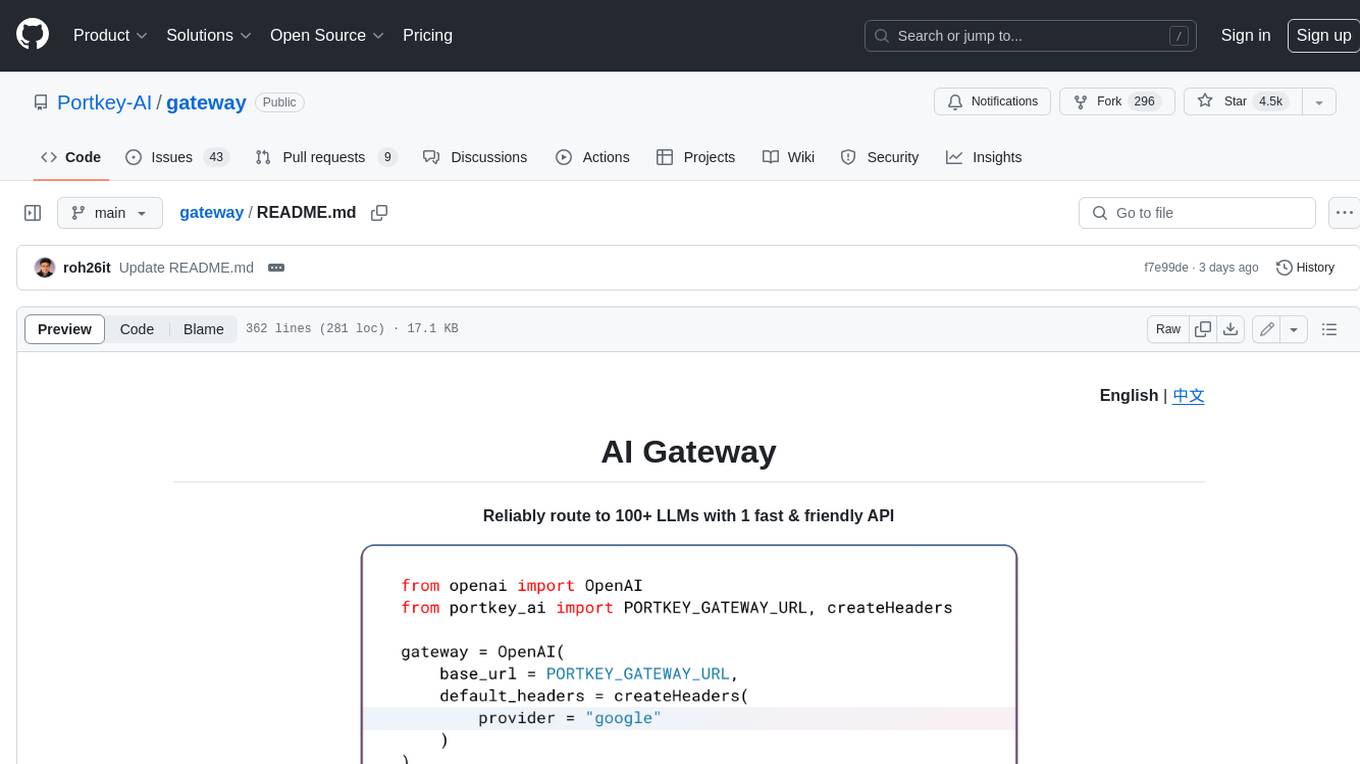
gateway
Gateway is a tool that streamlines requests to 100+ open & closed source models with a unified API. It is production-ready with support for caching, fallbacks, retries, timeouts, load balancing, and can be edge-deployed for minimum latency. It is blazing fast with a tiny footprint, supports load balancing across multiple models, providers, and keys, ensures app resilience with fallbacks, offers automatic retries with exponential fallbacks, allows configurable request timeouts, supports multimodal routing, and can be extended with plug-in middleware. It is battle-tested over 300B tokens and enterprise-ready for enhanced security, scale, and custom deployments.
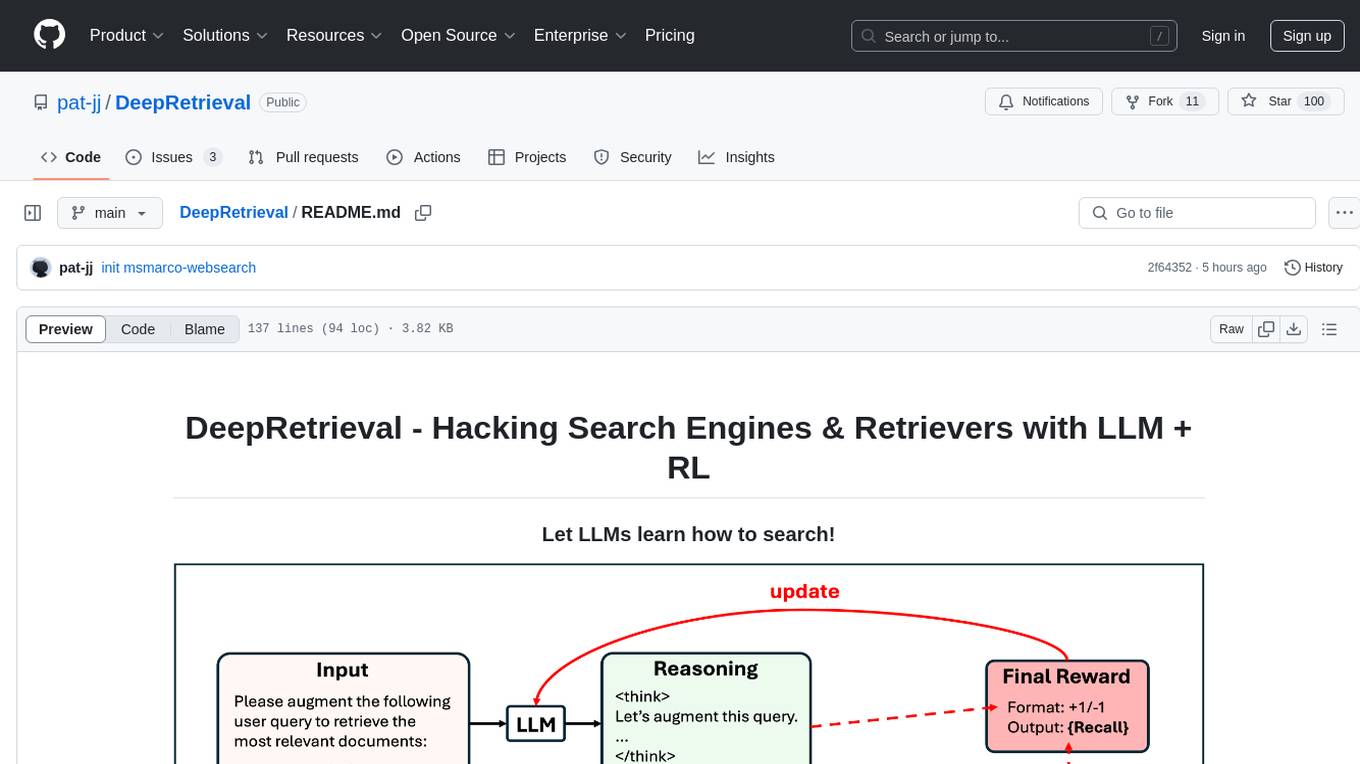
DeepRetrieval
DeepRetrieval is a tool designed to enhance search engines and retrievers using Large Language Models (LLMs) and Reinforcement Learning (RL). It allows LLMs to learn how to search effectively by integrating with search engine APIs and customizing reward functions. The tool provides functionalities for data preparation, training, evaluation, and monitoring search performance. DeepRetrieval aims to improve information retrieval tasks by leveraging advanced AI techniques.
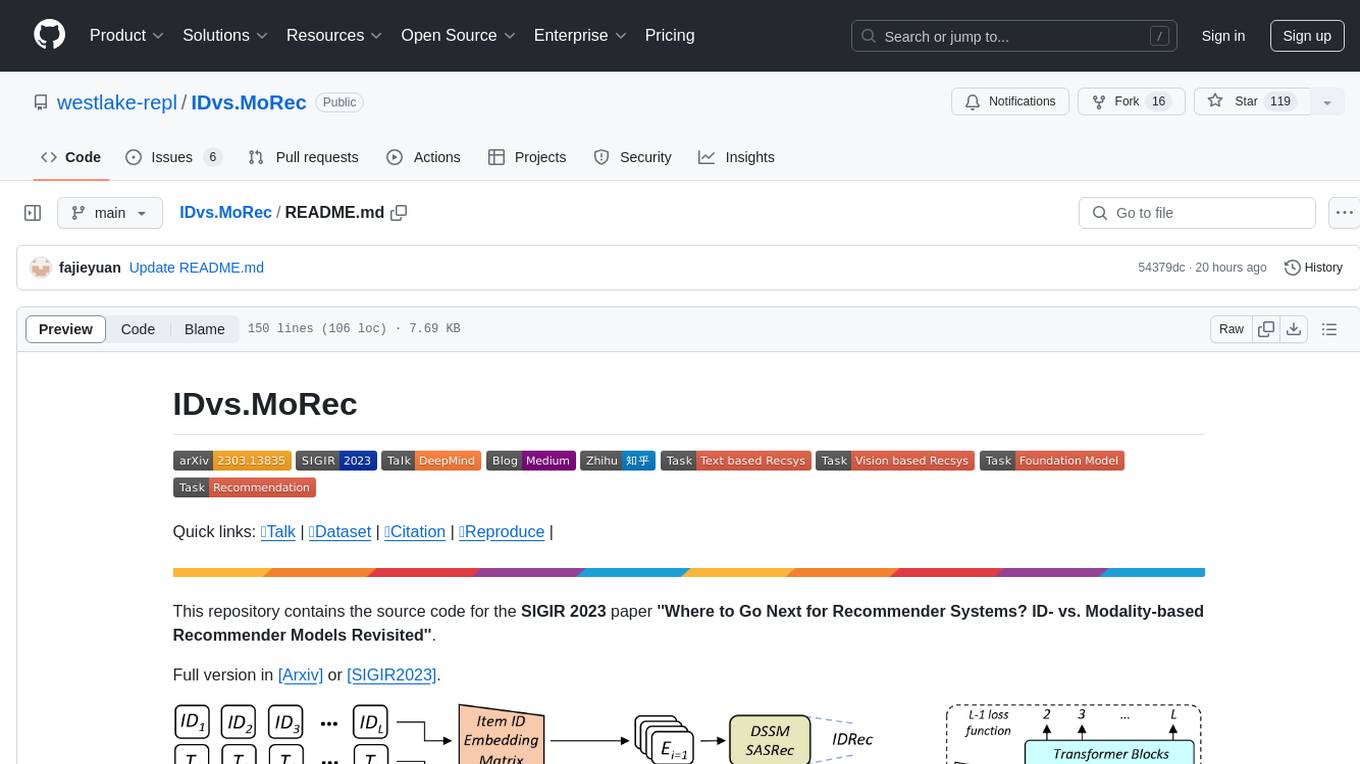
IDvs.MoRec
This repository contains the source code for the SIGIR 2023 paper 'Where to Go Next for Recommender Systems? ID- vs. Modality-based Recommender Models Revisited'. It provides resources for evaluating foundation, transferable, multi-modal, and LLM recommendation models, along with datasets, pre-trained models, and training strategies for IDRec and MoRec using in-batch debiased cross-entropy loss. The repository also offers large-scale datasets, code for SASRec with in-batch debias cross-entropy loss, and information on joining the lab for research opportunities.
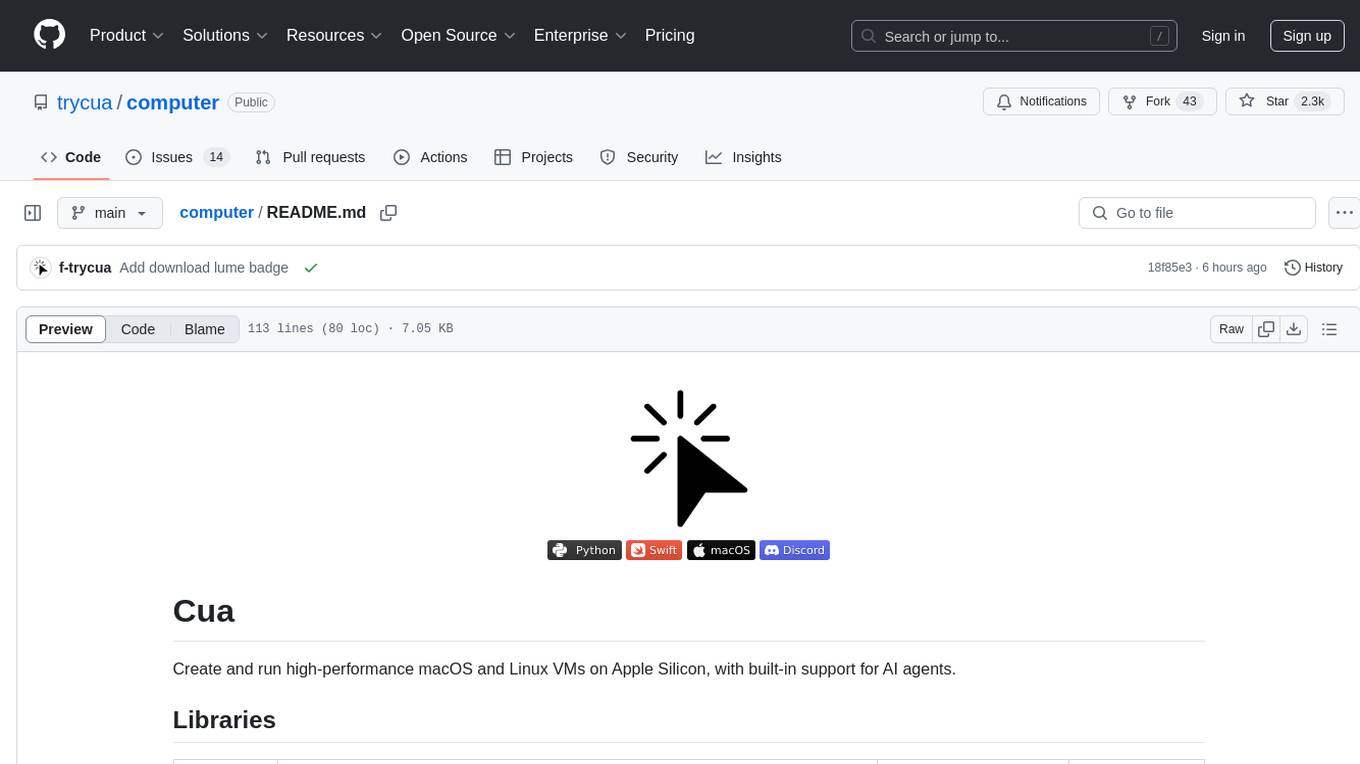
computer
Cua is a tool for creating and running high-performance macOS and Linux VMs on Apple Silicon, with built-in support for AI agents. It provides libraries like Lume for running VMs with near-native performance, Computer for interacting with sandboxes, and Agent for running agentic workflows. Users can refer to the documentation for onboarding and explore demos showcasing the tool's capabilities. Additionally, accessory libraries like Core, PyLume, Computer Server, and SOM offer additional functionality. Contributions to Cua are welcome, and the tool is open-sourced under the MIT License.
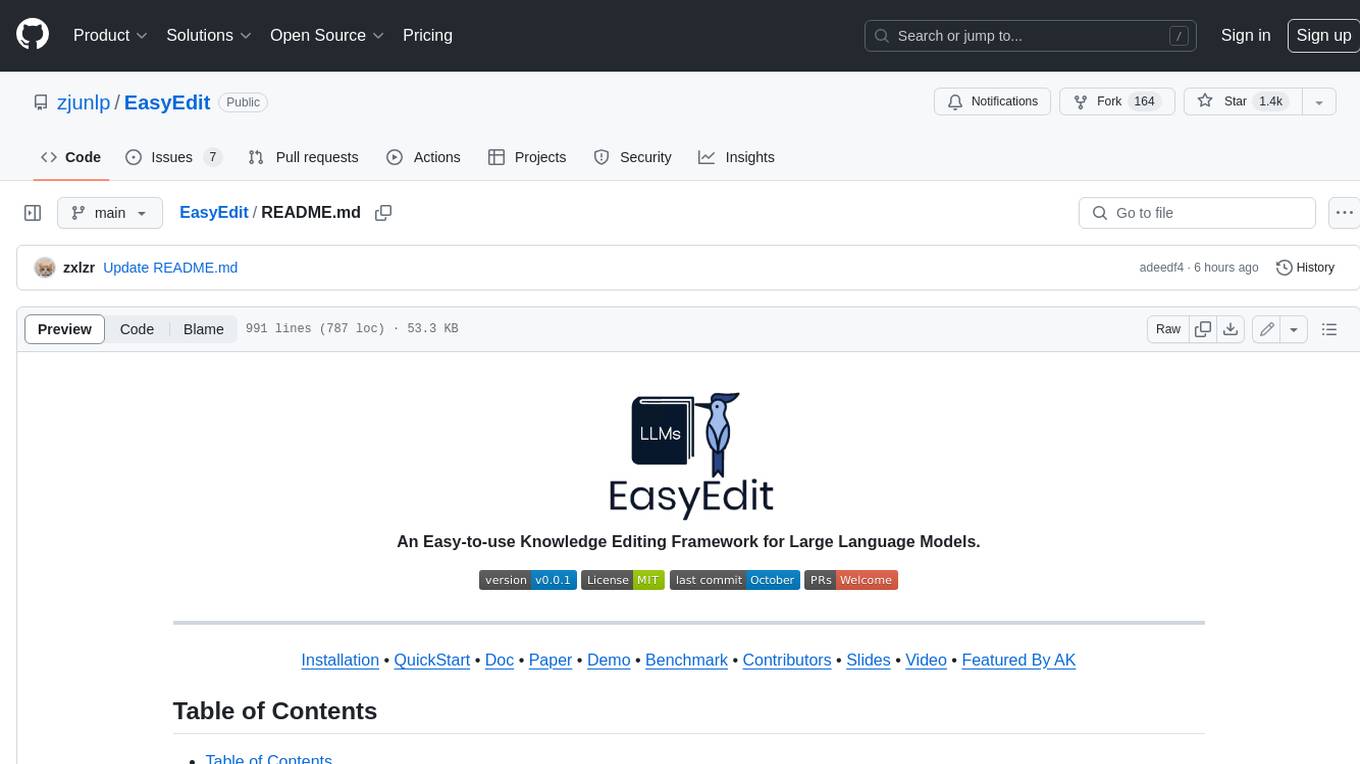
EasyEdit
EasyEdit is a Python package for edit Large Language Models (LLM) like `GPT-J`, `Llama`, `GPT-NEO`, `GPT2`, `T5`(support models from **1B** to **65B**), the objective of which is to alter the behavior of LLMs efficiently within a specific domain without negatively impacting performance across other inputs. It is designed to be easy to use and easy to extend.
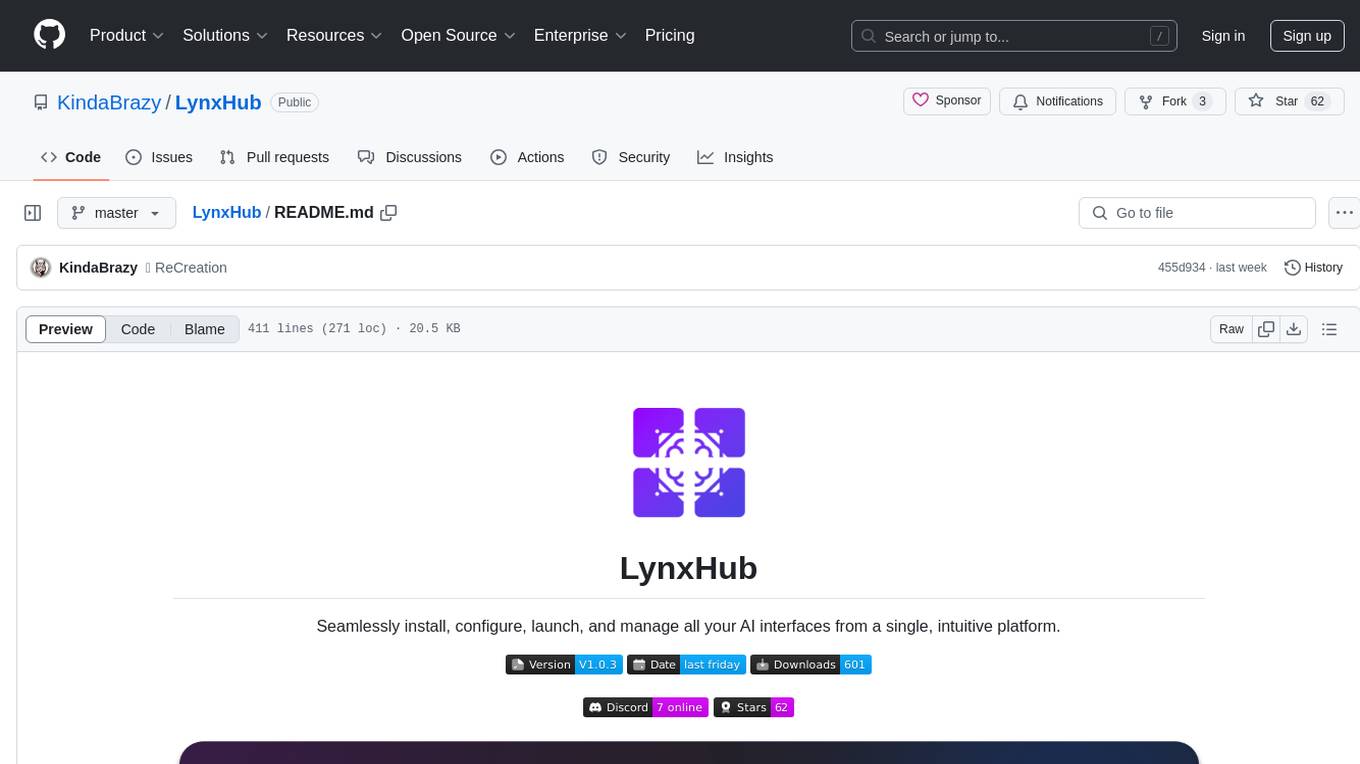
LynxHub
LynxHub is a platform that allows users to seamlessly install, configure, launch, and manage all their AI interfaces from a single, intuitive dashboard. It offers features like AI interface management, arguments manager, custom run commands, pre-launch actions, extension management, in-app tools like terminal and web browser, AI information dashboard, Discord integration, and additional features like theme options and favorite interface pinning. The platform supports modular design for custom AI modules and upcoming extensions system for complete customization. LynxHub aims to streamline AI workflow and enhance user experience with a user-friendly interface and comprehensive functionalities.
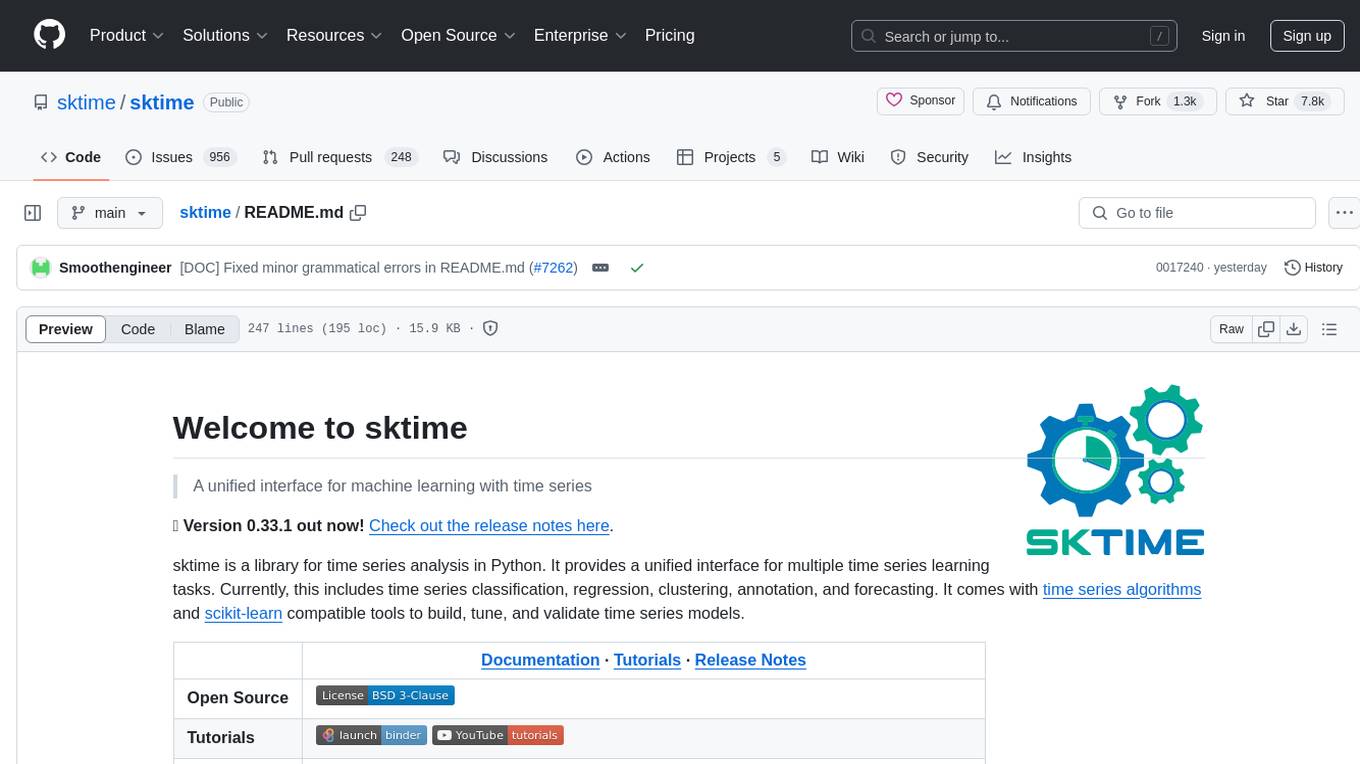
sktime
sktime is a Python library for time series analysis that provides a unified interface for various time series learning tasks such as classification, regression, clustering, annotation, and forecasting. It offers time series algorithms and tools compatible with scikit-learn for building, tuning, and validating time series models. sktime aims to enhance the interoperability and usability of the time series analysis ecosystem by empowering users to apply algorithms across different tasks and providing interfaces to related libraries like scikit-learn, statsmodels, tsfresh, PyOD, and fbprophet.
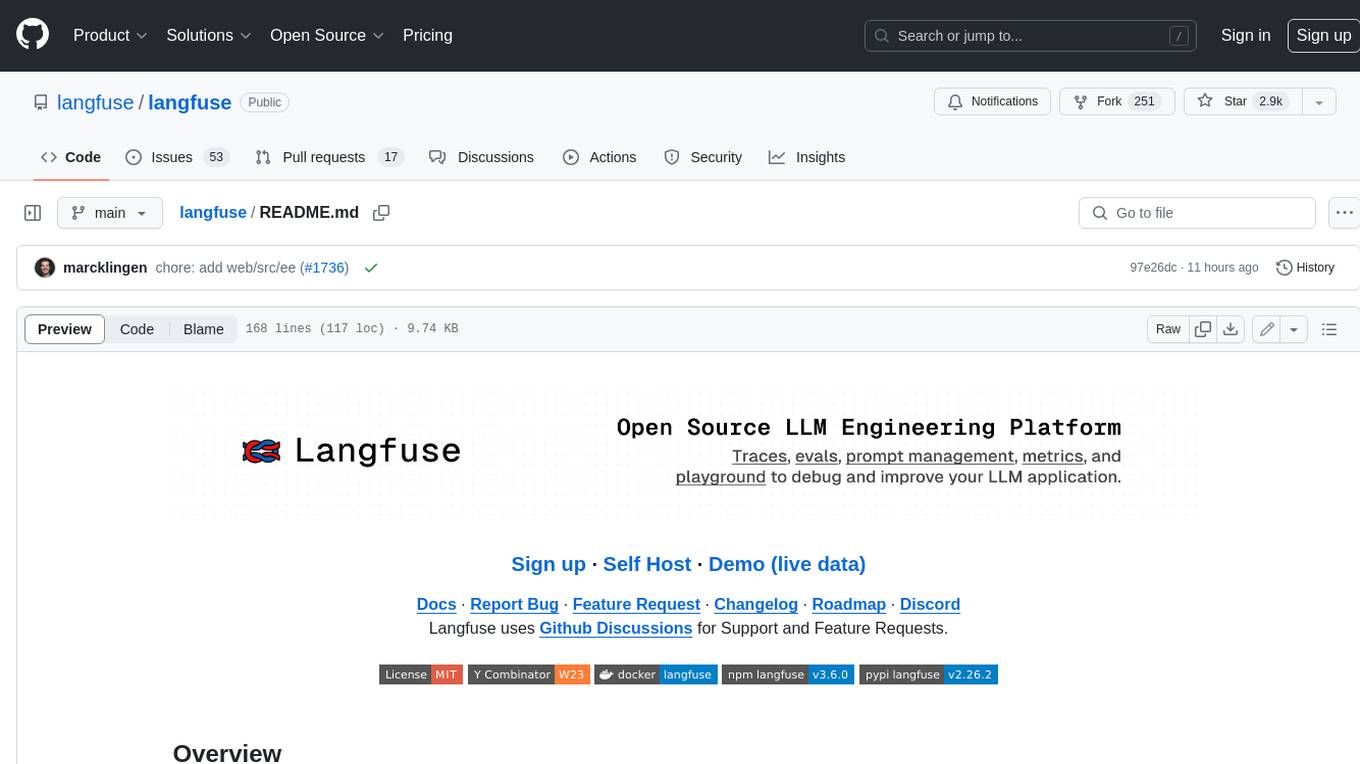
langfuse
Langfuse is a powerful tool that helps you develop, monitor, and test your LLM applications. With Langfuse, you can: * **Develop:** Instrument your app and start ingesting traces to Langfuse, inspect and debug complex logs, and manage, version, and deploy prompts from within Langfuse. * **Monitor:** Track metrics (cost, latency, quality) and gain insights from dashboards & data exports, collect and calculate scores for your LLM completions, run model-based evaluations, collect user feedback, and manually score observations in Langfuse. * **Test:** Track and test app behaviour before deploying a new version, test expected in and output pairs and benchmark performance before deploying, and track versions and releases in your application. Langfuse is easy to get started with and offers a generous free tier. You can sign up for Langfuse Cloud or deploy Langfuse locally or on your own infrastructure. Langfuse also offers a variety of integrations to make it easy to connect to your LLM applications.
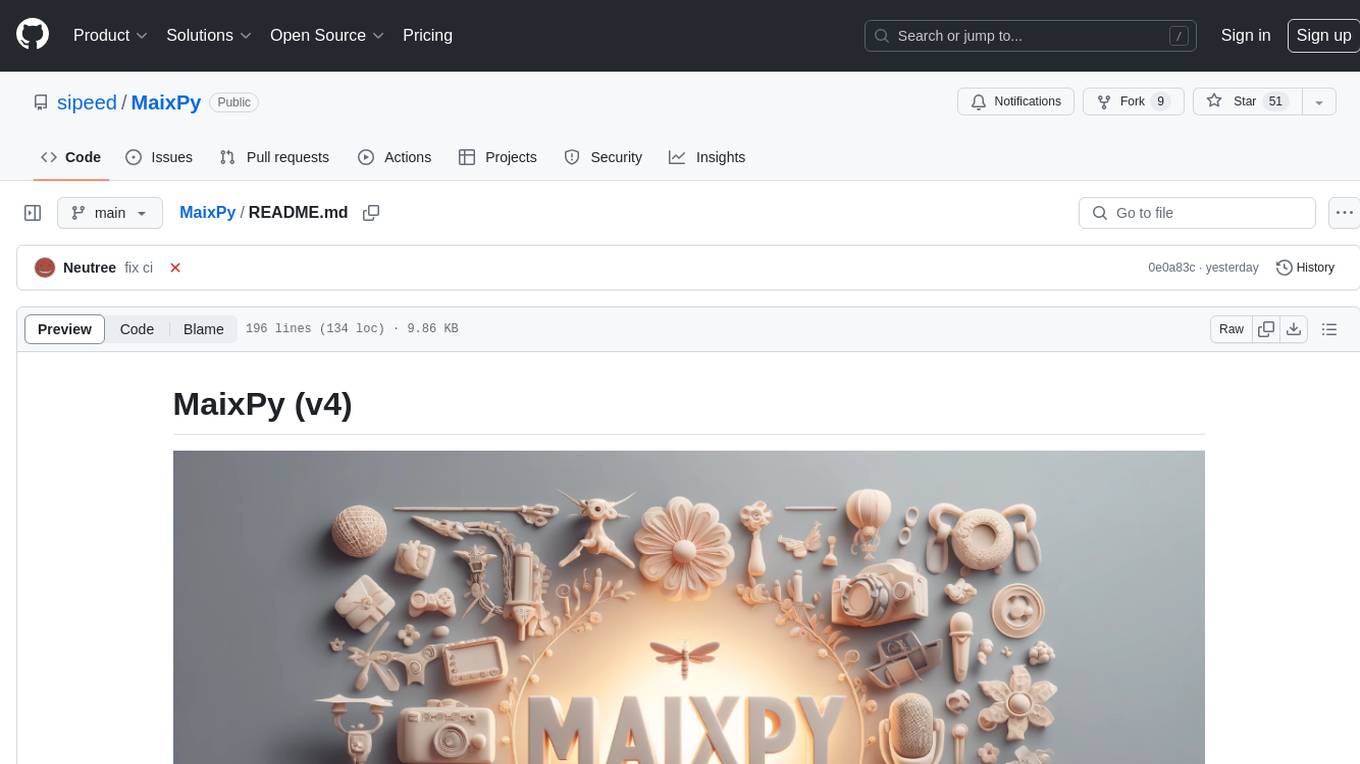
MaixPy
MaixPy is a Python SDK that enables users to easily create AI vision projects on edge devices. It provides a user-friendly API for accessing NPU, making it suitable for AI Algorithm Engineers, STEM teachers, Makers, Engineers, Students, Enterprises, and Contestants. The tool supports Python programming, MaixVision Workstation, AI vision, video streaming, voice recognition, and peripheral usage. It also offers an online AI training platform called MaixHub. MaixPy is designed for new hardware platforms like MaixCAM, offering improved performance and features compared to older versions. The ecosystem includes hardware, software, tools, documentation, and a cloud platform.
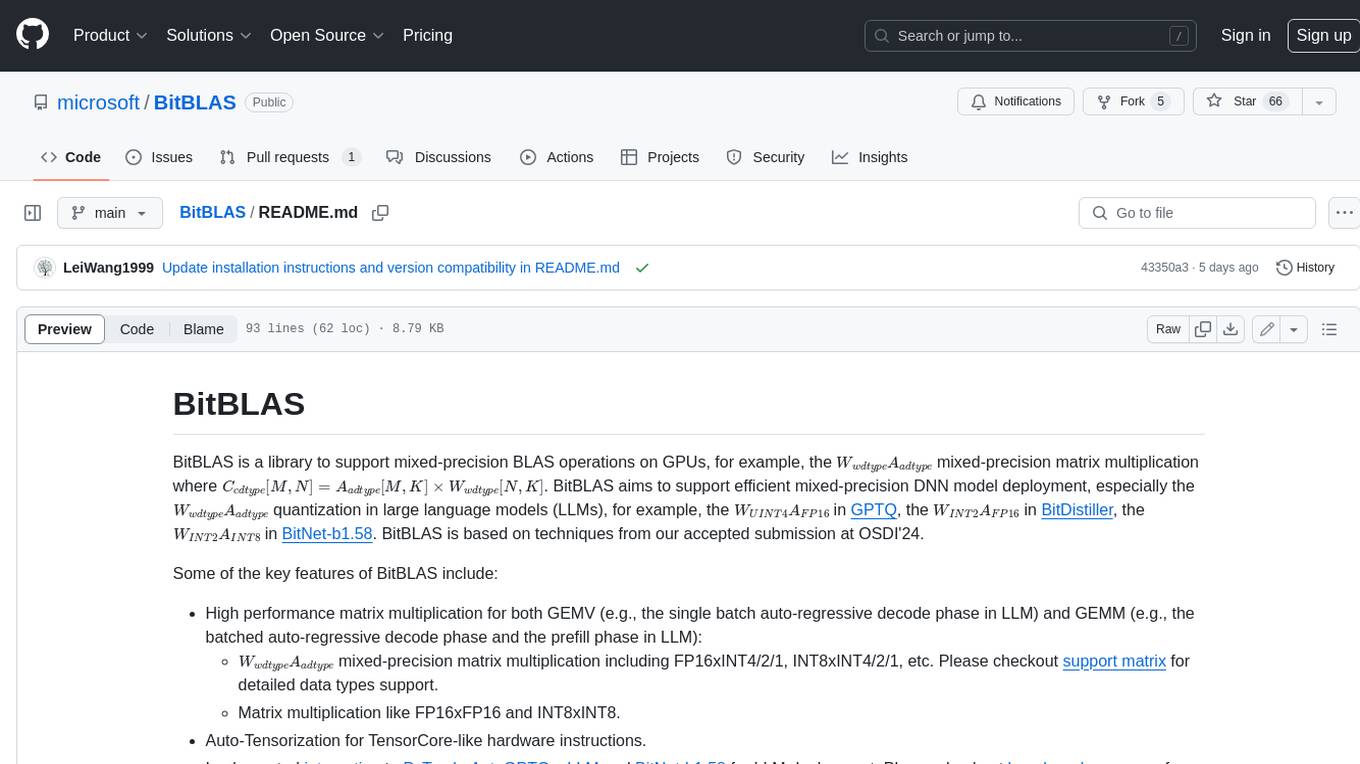
BitBLAS
BitBLAS is a library for mixed-precision BLAS operations on GPUs, for example, the $W_{wdtype}A_{adtype}$ mixed-precision matrix multiplication where $C_{cdtype}[M, N] = A_{adtype}[M, K] \times W_{wdtype}[N, K]$. BitBLAS aims to support efficient mixed-precision DNN model deployment, especially the $W_{wdtype}A_{adtype}$ quantization in large language models (LLMs), for example, the $W_{UINT4}A_{FP16}$ in GPTQ, the $W_{INT2}A_{FP16}$ in BitDistiller, the $W_{INT2}A_{INT8}$ in BitNet-b1.58. BitBLAS is based on techniques from our accepted submission at OSDI'24.
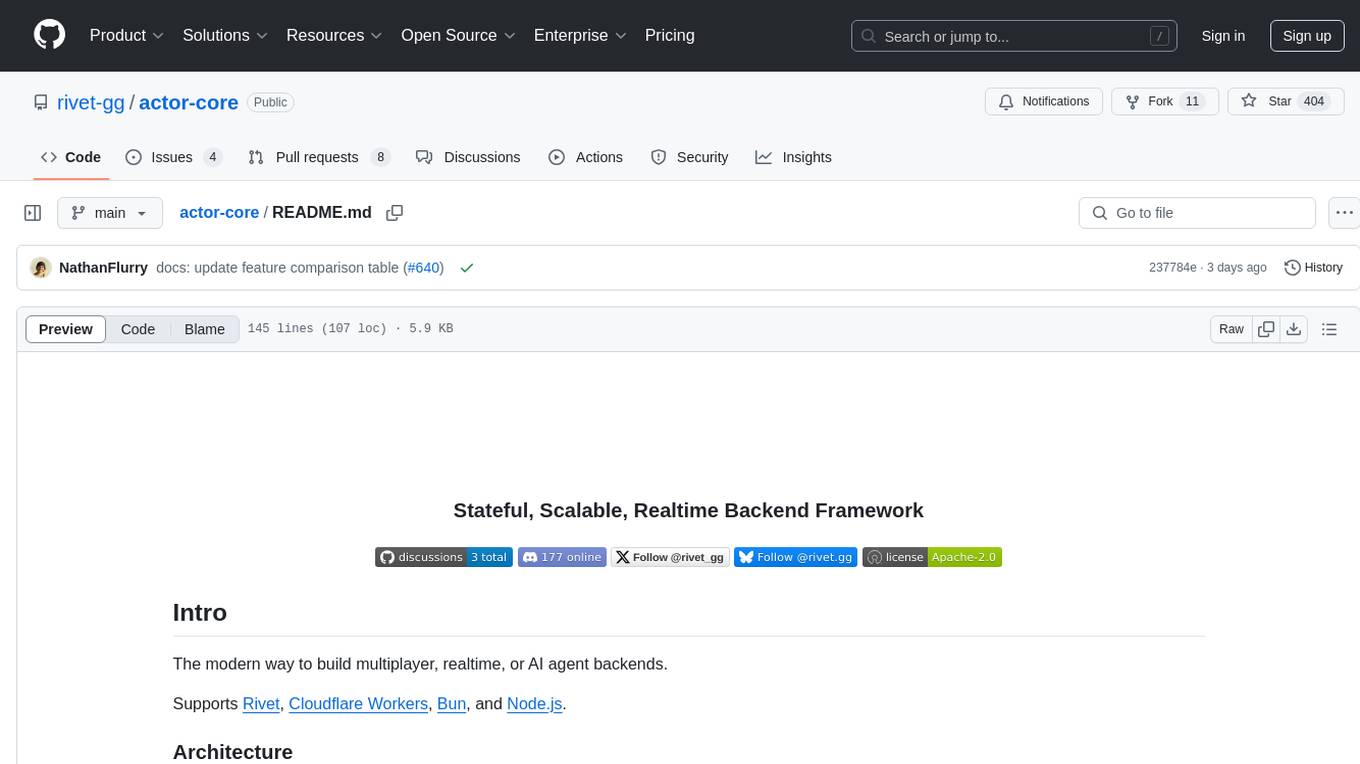
actor-core
Actor-core is a lightweight and flexible library for building actor-based concurrent applications in Java. It provides a simple API for creating and managing actors, as well as handling message passing between actors. With actor-core, developers can easily implement scalable and fault-tolerant systems using the actor model.
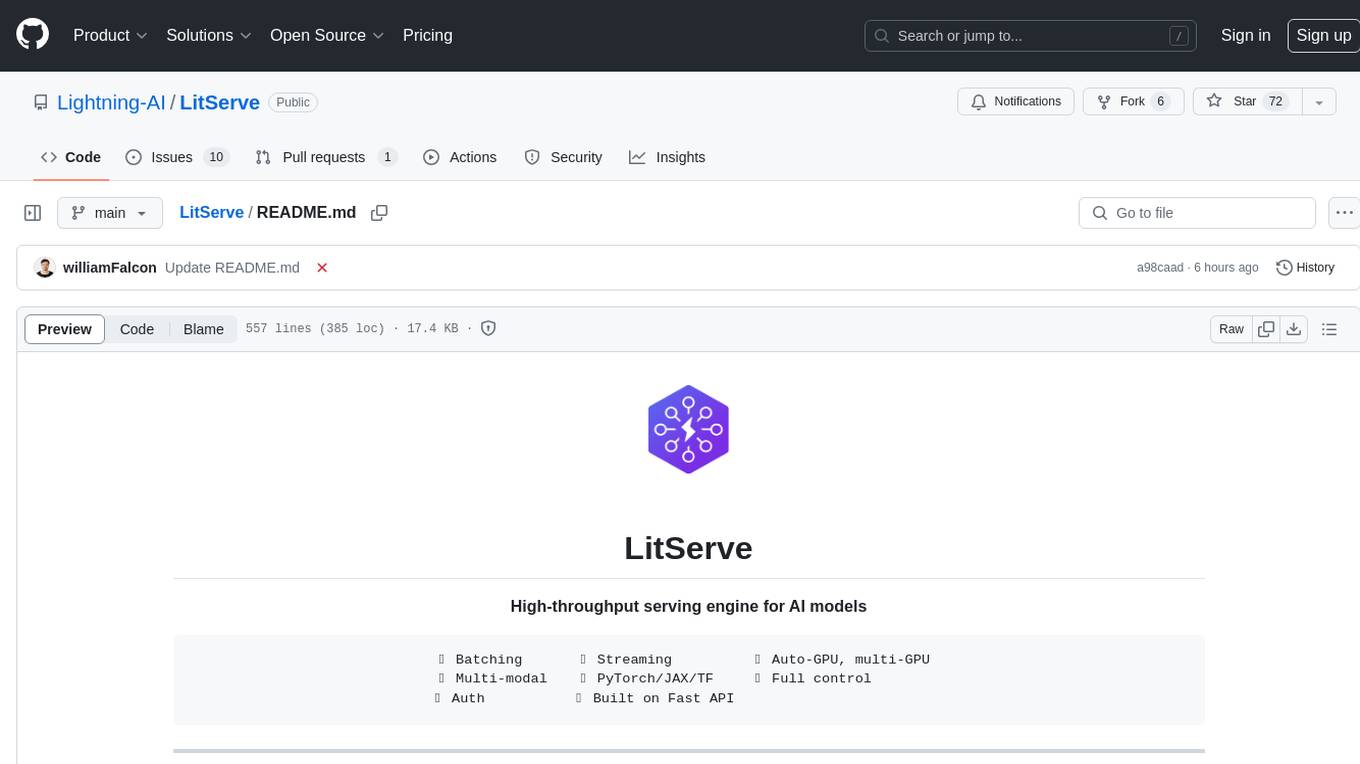
LitServe
LitServe is a high-throughput serving engine designed for deploying AI models at scale. It generates an API endpoint for models, handles batching, streaming, and autoscaling across CPU/GPUs. LitServe is built for enterprise scale with a focus on minimal, hackable code-base without bloat. It supports various model types like LLMs, vision, time-series, and works with frameworks like PyTorch, JAX, Tensorflow, and more. The tool allows users to focus on model performance rather than serving boilerplate, providing full control and flexibility.
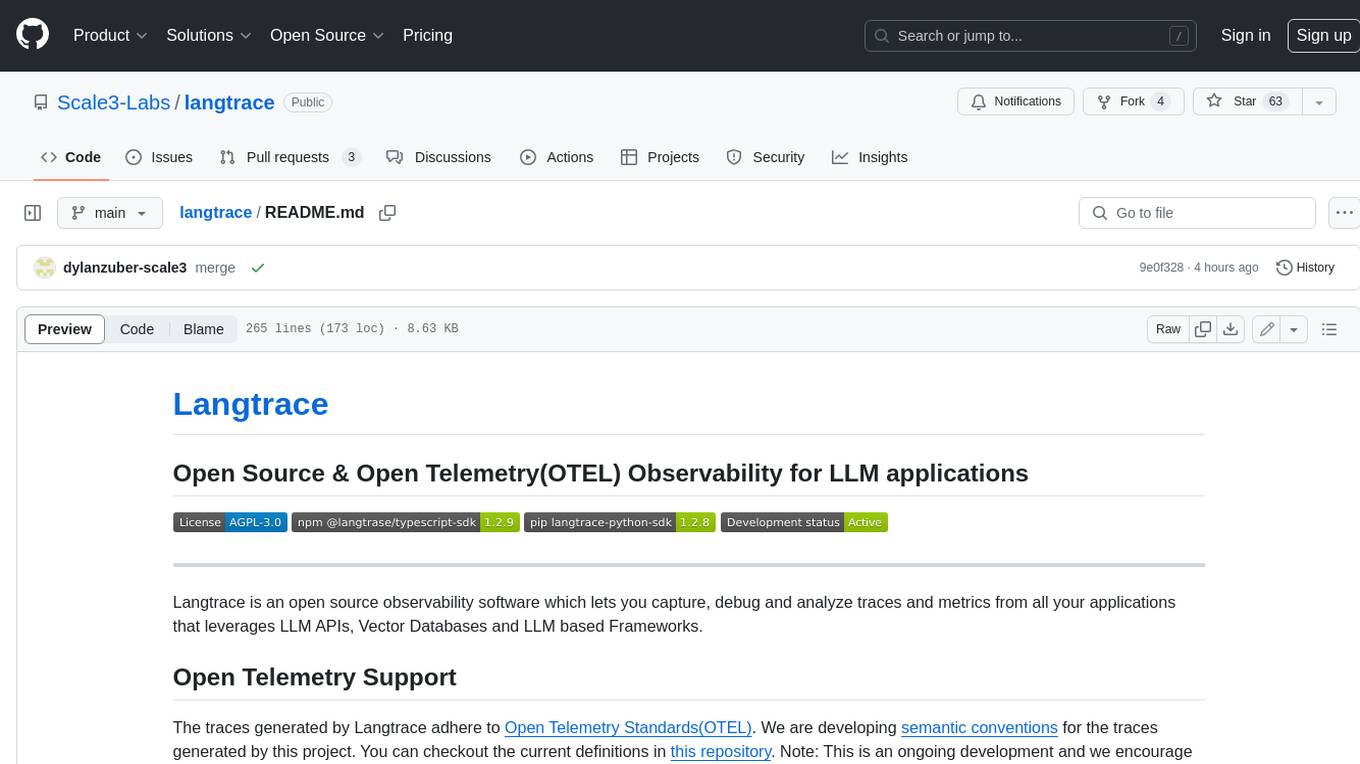
langtrace
Langtrace is an open source observability software that lets you capture, debug, and analyze traces and metrics from all your applications that leverage LLM APIs, Vector Databases, and LLM-based Frameworks. It supports Open Telemetry Standards (OTEL), and the traces generated adhere to these standards. Langtrace offers both a managed SaaS version (Langtrace Cloud) and a self-hosted option. The SDKs for both Typescript/Javascript and Python are available, making it easy to integrate Langtrace into your applications. Langtrace automatically captures traces from various vendors, including OpenAI, Anthropic, Azure OpenAI, Langchain, LlamaIndex, Pinecone, and ChromaDB.
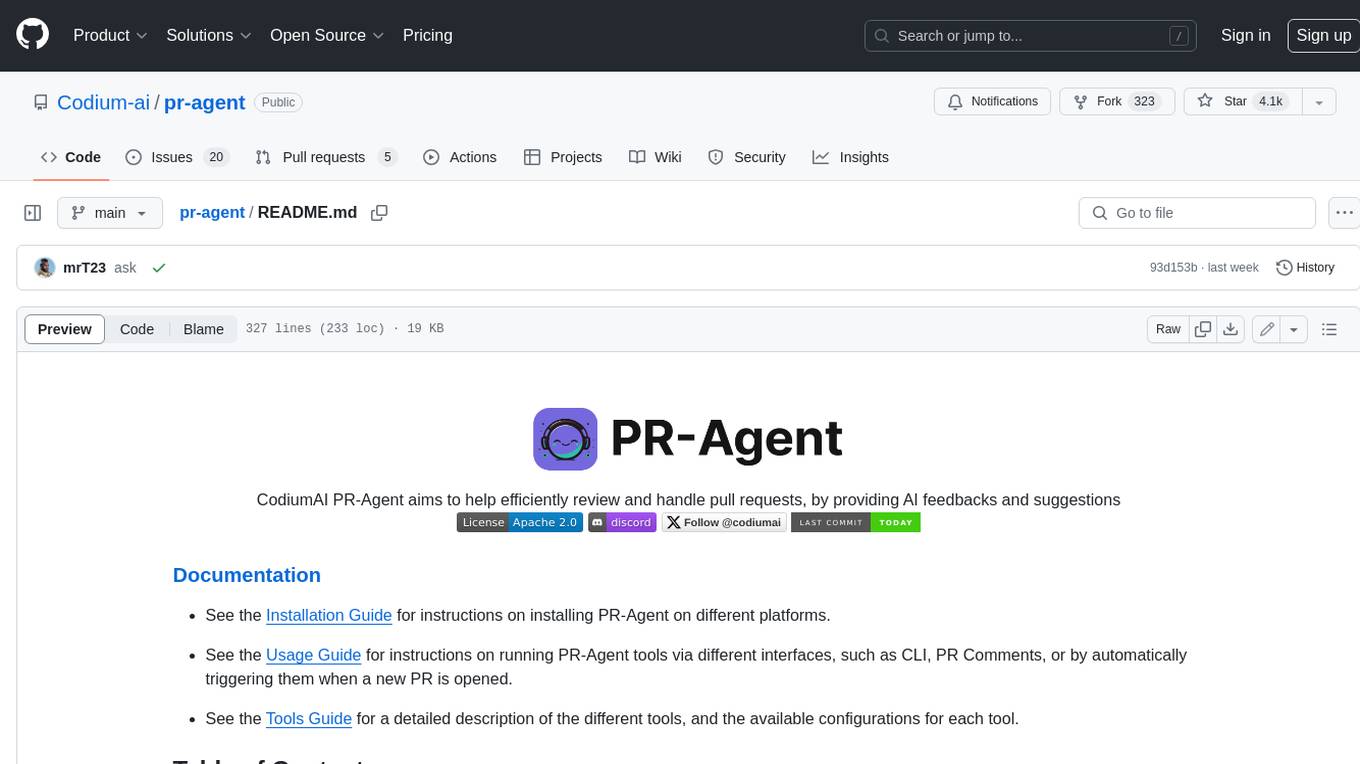
pr-agent
PR-Agent is a tool that helps to efficiently review and handle pull requests by providing AI feedbacks and suggestions. It supports various commands such as generating PR descriptions, providing code suggestions, answering questions about the PR, and updating the CHANGELOG.md file. PR-Agent can be used via CLI, GitHub Action, GitHub App, Docker, and supports multiple git providers and models. It emphasizes real-life practical usage, with each tool having a single GPT-4 call for quick and affordable responses. The PR Compression strategy enables effective handling of both short and long PRs, while the JSON prompting strategy allows for modular and customizable tools. PR-Agent Pro, the hosted version by CodiumAI, provides additional benefits such as full management, improved privacy, priority support, and extra features.
For similar tasks
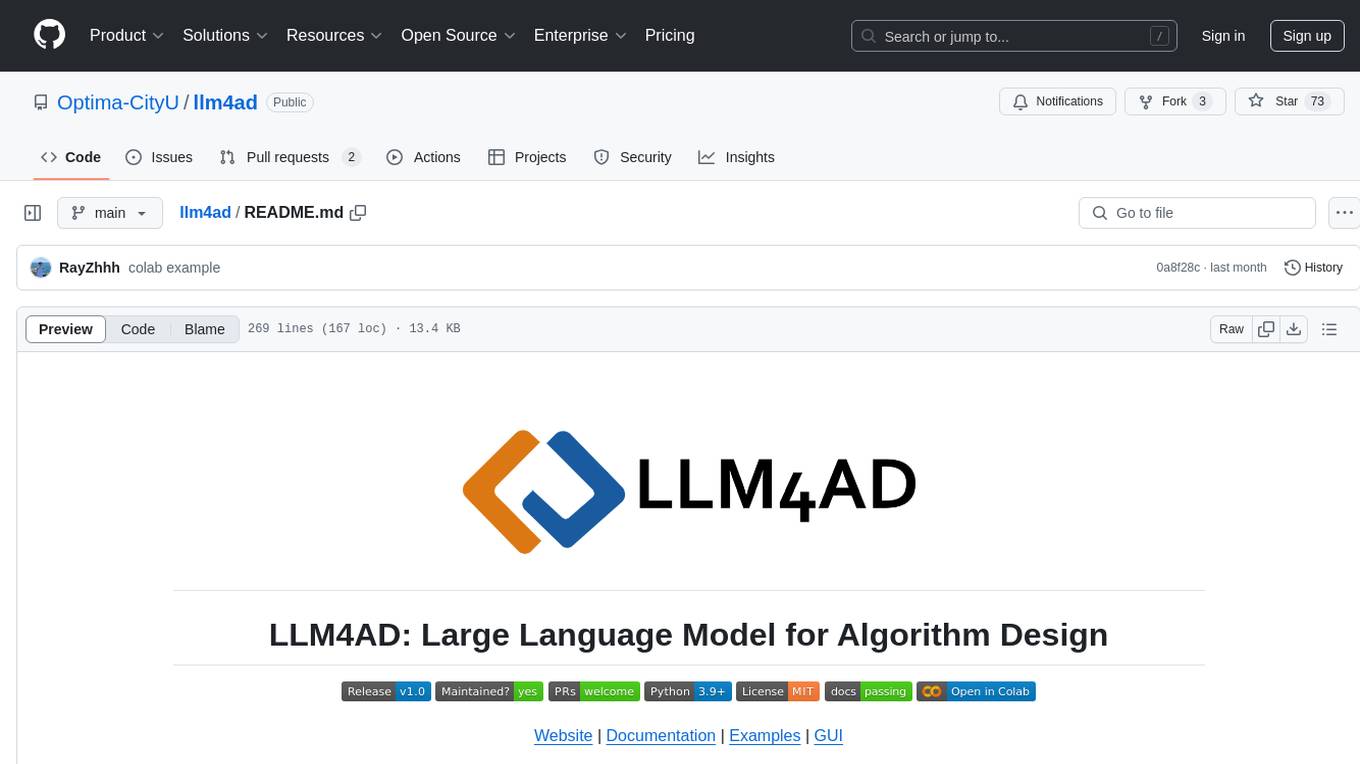
llm4ad
LLM4AD is an open-source Python-based platform leveraging Large Language Models (LLMs) for Automatic Algorithm Design (AD). It provides unified interfaces for methods, tasks, and LLMs, along with features like evaluation acceleration, secure evaluation, logs, GUI support, and more. The platform was originally developed for optimization tasks but is versatile enough to be used in other areas such as machine learning, science discovery, game theory, and engineering design. It offers various search methods and algorithm design tasks across different domains. LLM4AD supports remote LLM API, local HuggingFace LLM deployment, and custom LLM interfaces. The project is licensed under the MIT License and welcomes contributions, collaborations, and issue reports.
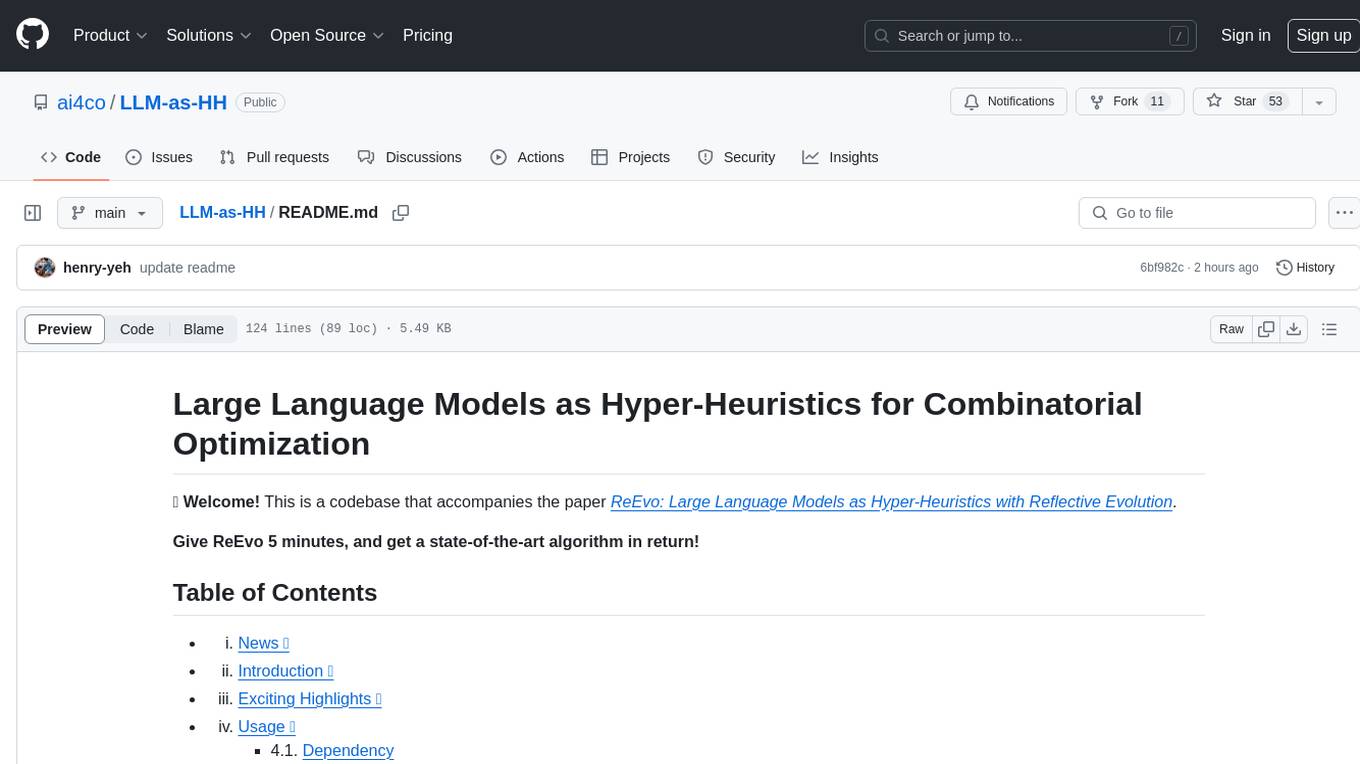
LLM-as-HH
LLM-as-HH is a codebase that accompanies the paper ReEvo: Large Language Models as Hyper-Heuristics with Reflective Evolution. It introduces Language Hyper-Heuristics (LHHs) that leverage LLMs for heuristic generation with minimal manual intervention and open-ended heuristic spaces. Reflective Evolution (ReEvo) is presented as a searching framework that emulates the reflective design approach of human experts while surpassing human capabilities with scalable LLM inference, Internet-scale domain knowledge, and powerful evolutionary search. The tool can improve various algorithms on problems like Traveling Salesman Problem, Capacitated Vehicle Routing Problem, Orienteering Problem, Multiple Knapsack Problems, Bin Packing Problem, and Decap Placement Problem in both black-box and white-box settings.
For similar jobs
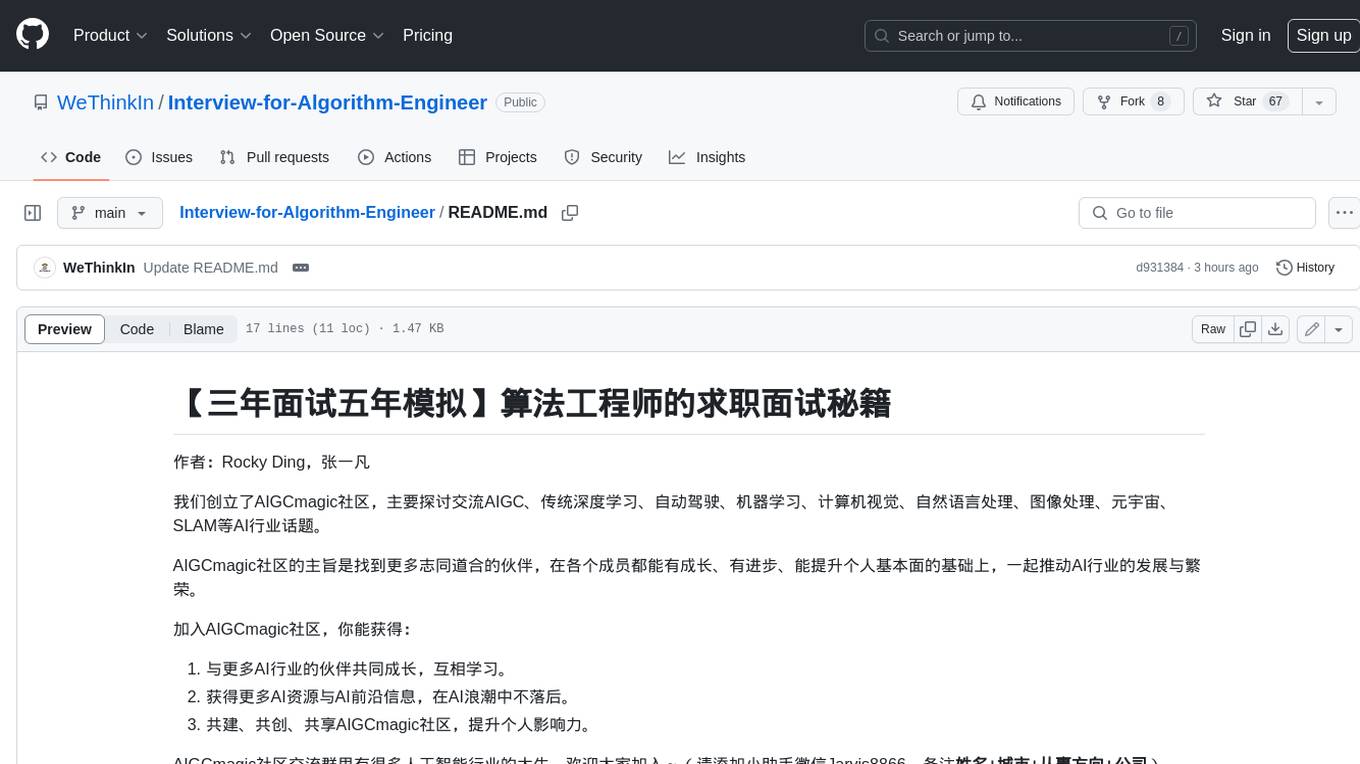
Interview-for-Algorithm-Engineer
This repository provides a collection of interview questions and answers for algorithm engineers. The questions are organized by topic, and each question includes a detailed explanation of the answer. This repository is a valuable resource for anyone preparing for an algorithm engineering interview.
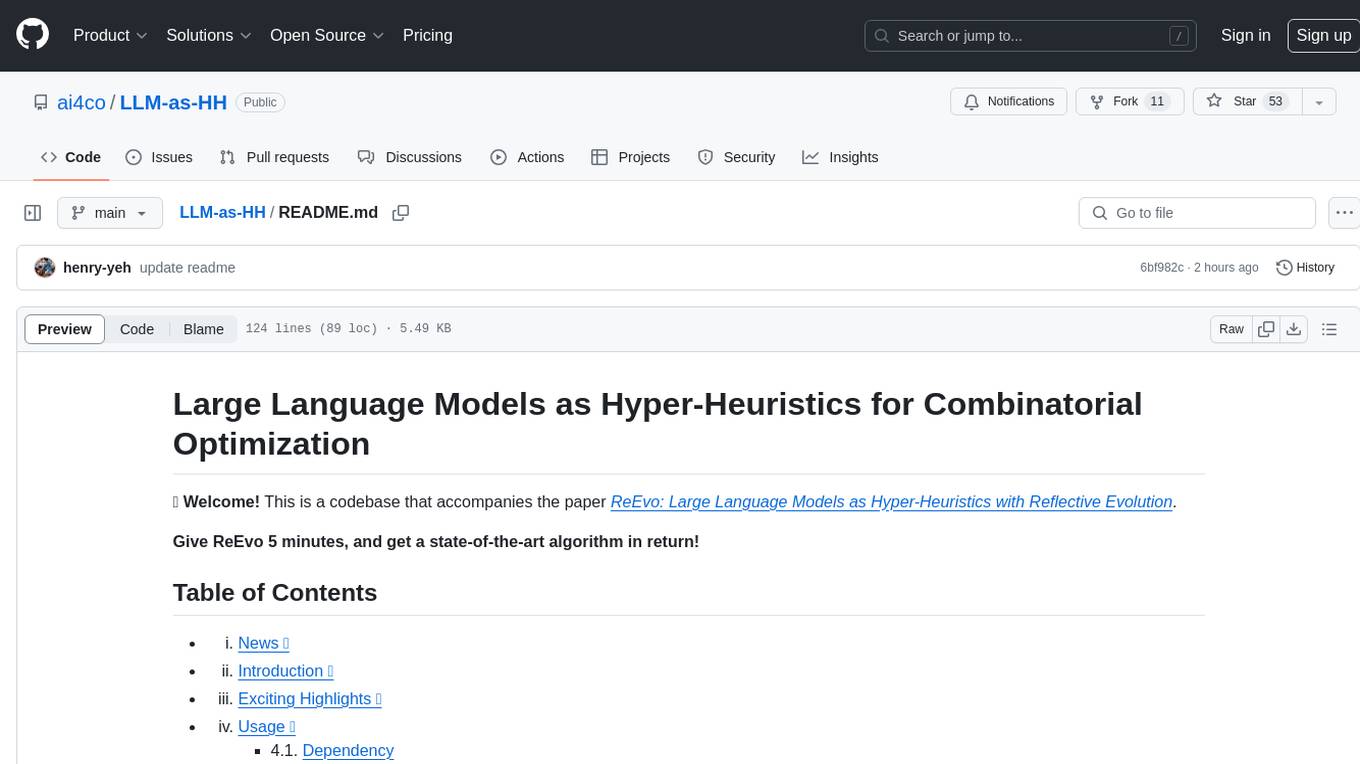
LLM-as-HH
LLM-as-HH is a codebase that accompanies the paper ReEvo: Large Language Models as Hyper-Heuristics with Reflective Evolution. It introduces Language Hyper-Heuristics (LHHs) that leverage LLMs for heuristic generation with minimal manual intervention and open-ended heuristic spaces. Reflective Evolution (ReEvo) is presented as a searching framework that emulates the reflective design approach of human experts while surpassing human capabilities with scalable LLM inference, Internet-scale domain knowledge, and powerful evolutionary search. The tool can improve various algorithms on problems like Traveling Salesman Problem, Capacitated Vehicle Routing Problem, Orienteering Problem, Multiple Knapsack Problems, Bin Packing Problem, and Decap Placement Problem in both black-box and white-box settings.
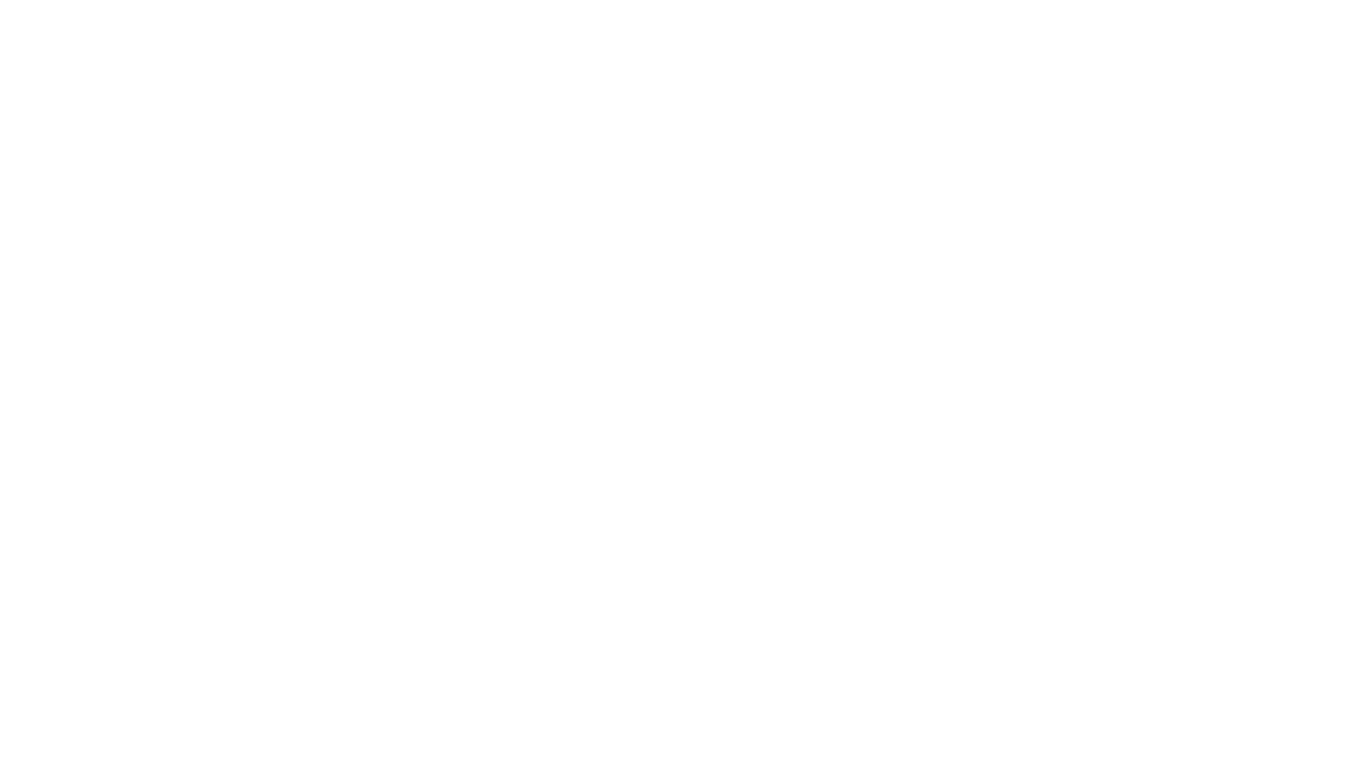
universal
The Universal Numbers Library is a header-only C++ template library designed for universal number arithmetic, offering alternatives to native integer and floating-point for mixed-precision algorithm development and optimization. It tailors arithmetic types to the application's precision and dynamic range, enabling improved application performance and energy efficiency. The library provides fast implementations of special IEEE-754 formats like quarter precision, half-precision, and quad precision, as well as vendor-specific extensions. It supports static and elastic integers, decimals, fixed-points, rationals, linear floats, tapered floats, logarithmic, interval, and adaptive-precision integers, rationals, and floats. The library is suitable for AI, DSP, HPC, and HFT algorithms.
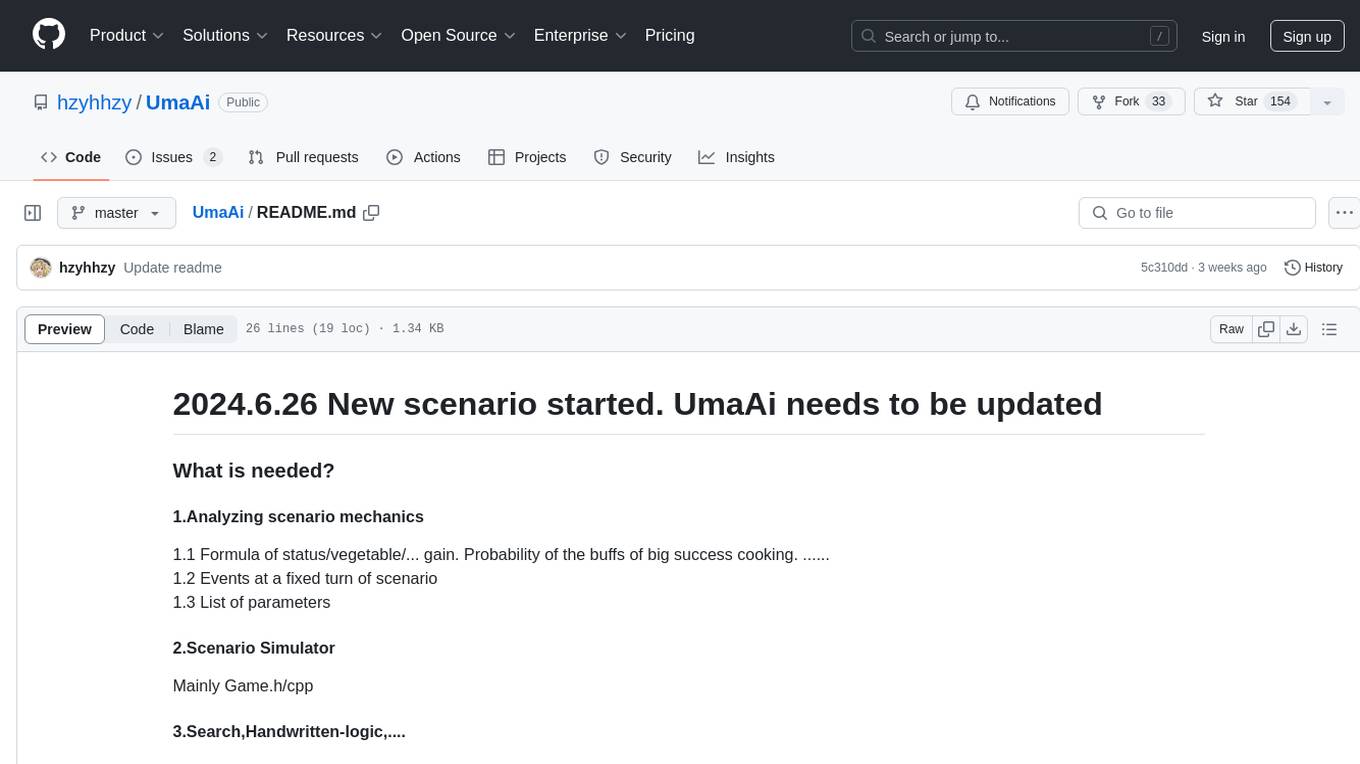
UmaAi
UmaAi is a tool designed for algorithm learning purposes, specifically focused on analyzing scenario mechanics in a game. It provides functionalities such as simulating scenarios, searching, handwritten-logic, and OCR integration. The tool allows users to modify settings in config.h for evaluating cardset strength, simulating games, and understanding game mechanisms through the source code. It emphasizes that it should not be used for illegal purposes and is intended for educational use only.
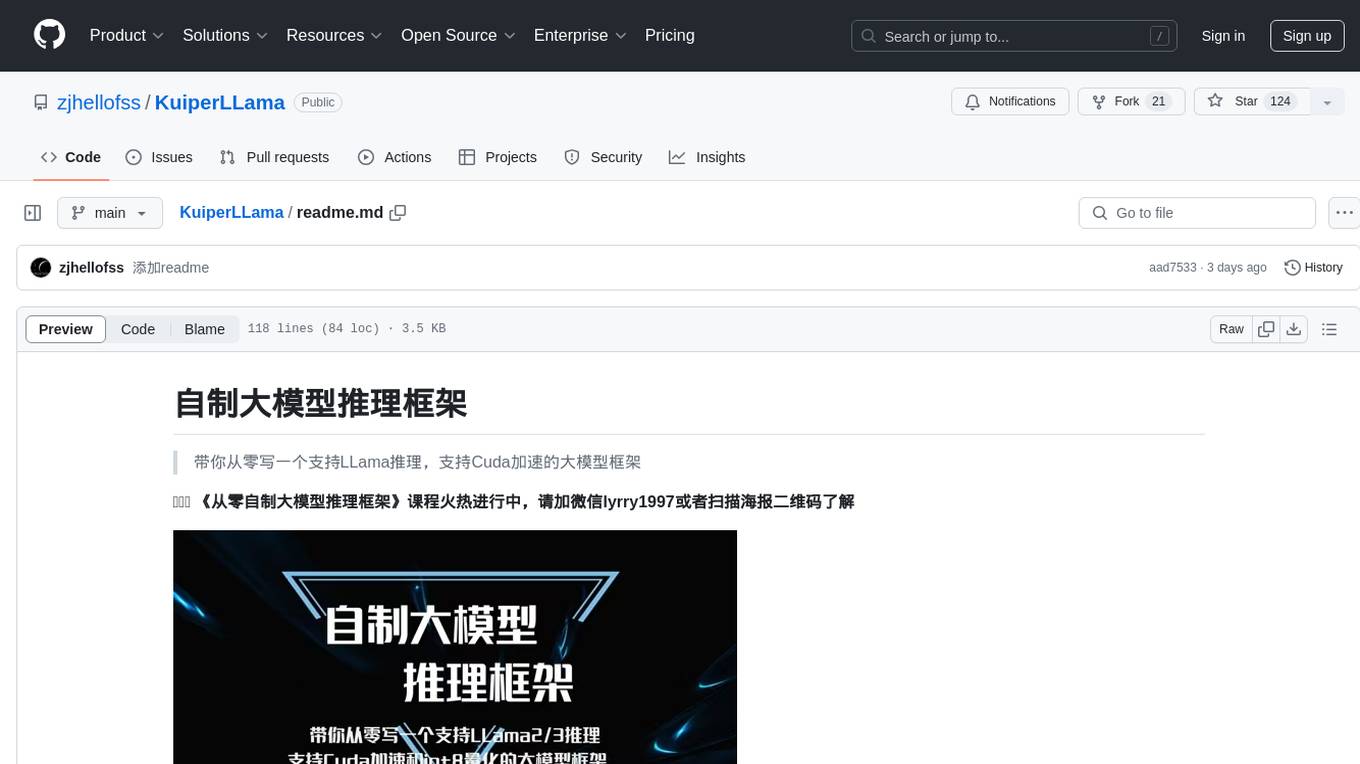
KuiperLLama
KuiperLLama is a custom large model inference framework that guides users in building a LLama-supported inference framework with Cuda acceleration from scratch. The framework includes modules for architecture design, LLama2 model support, model quantization, Cuda basics, operator implementation, and fun tasks like text generation and storytelling. It also covers learning other commercial inference frameworks for comprehensive understanding. The project provides detailed tutorials and resources for developing and optimizing large models for efficient inference.
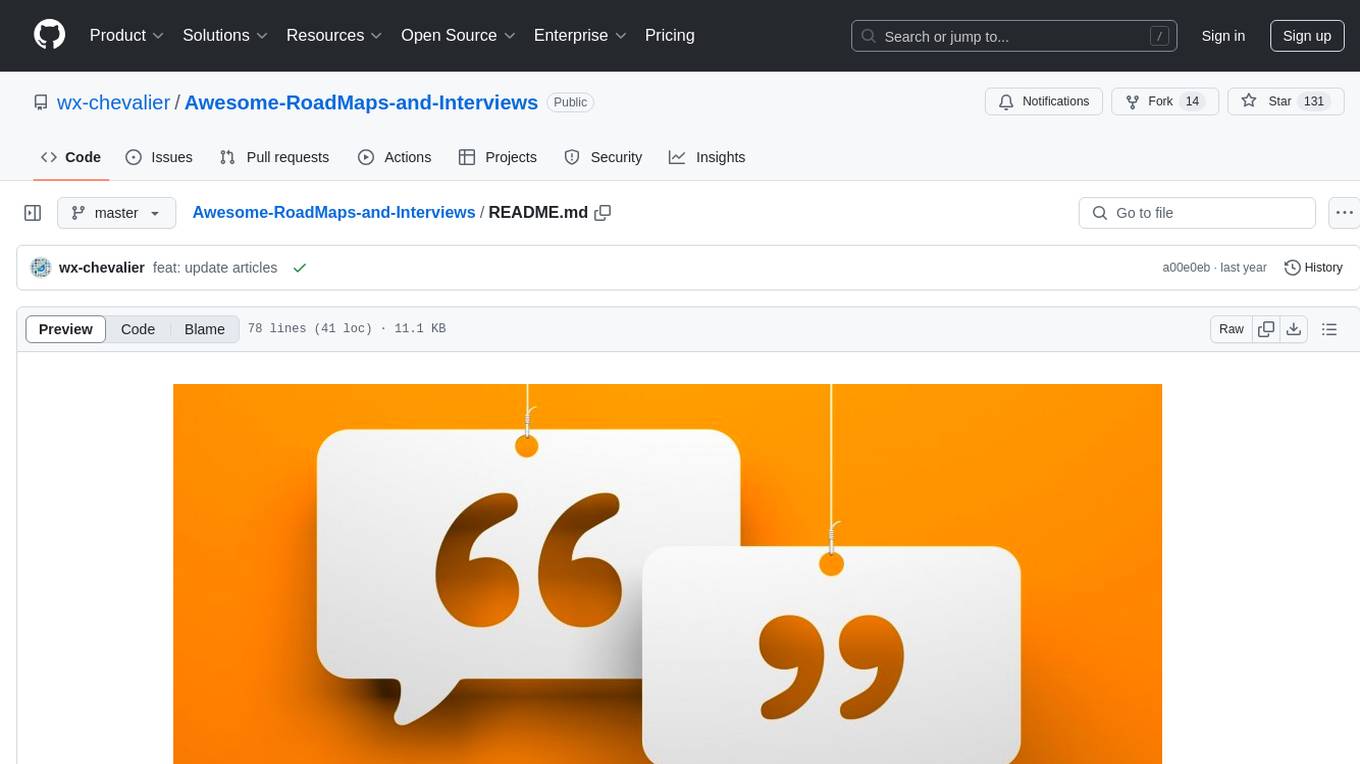
Awesome-RoadMaps-and-Interviews
Awesome RoadMaps and Interviews is a comprehensive repository that aims to provide guidance for technical interviews and career development in the ITCS field. It covers a wide range of topics including interview strategies, technical knowledge, and practical insights gained from years of interviewing experience. The repository emphasizes the importance of combining theoretical knowledge with practical application, and encourages users to expand their interview preparation beyond just algorithms. It also offers resources for enhancing knowledge breadth, depth, and programming skills through curated roadmaps, mind maps, cheat sheets, and coding snippets. The content is structured to help individuals navigate various technical roles and technologies, fostering continuous learning and professional growth.
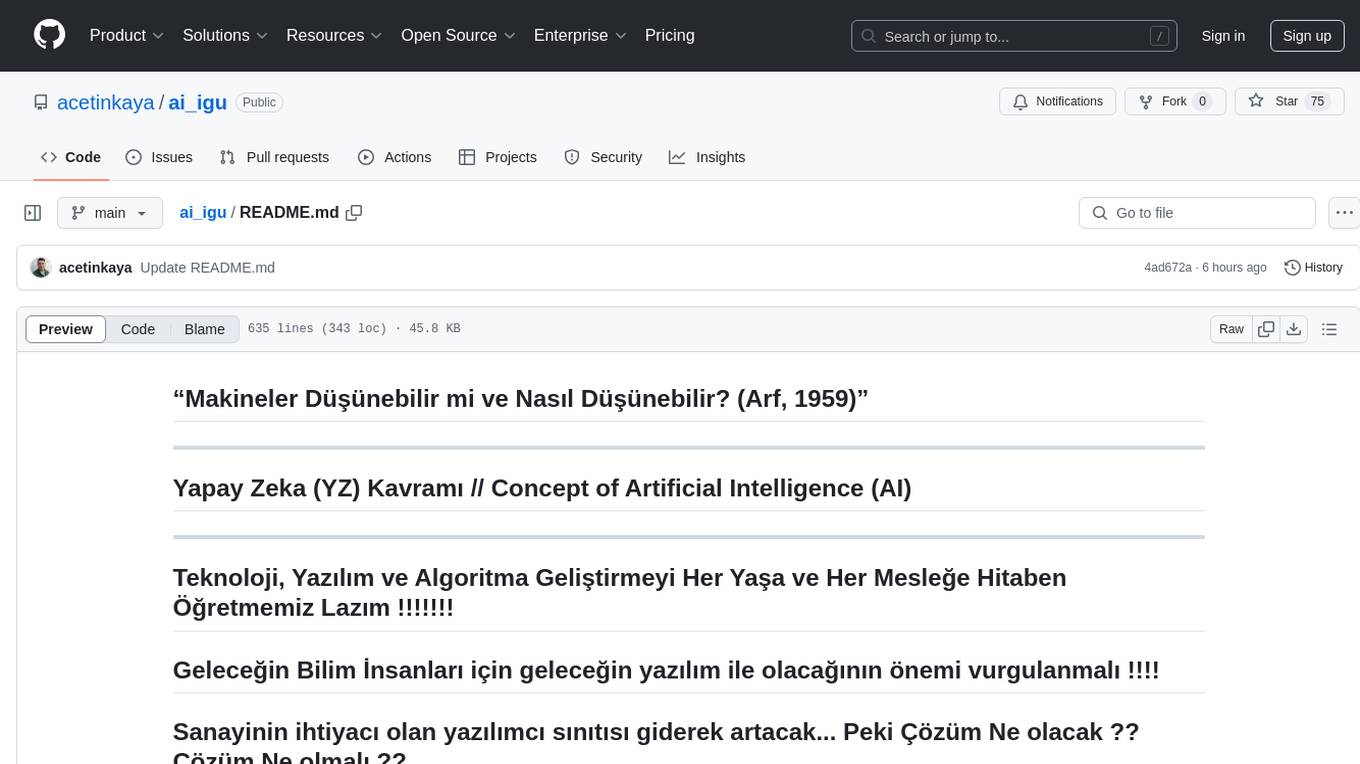
ai_igu
AI-IGU is a GitHub repository focused on Artificial Intelligence (AI) concepts, technology, software development, and algorithm improvement for all ages and professions. It emphasizes the importance of future software for future scientists and the increasing need for software developers in the industry. The repository covers various topics related to AI, including machine learning, deep learning, data mining, data science, big data, and more. It provides educational materials, practical examples, and hands-on projects to enhance software development skills and create awareness in the field of AI.
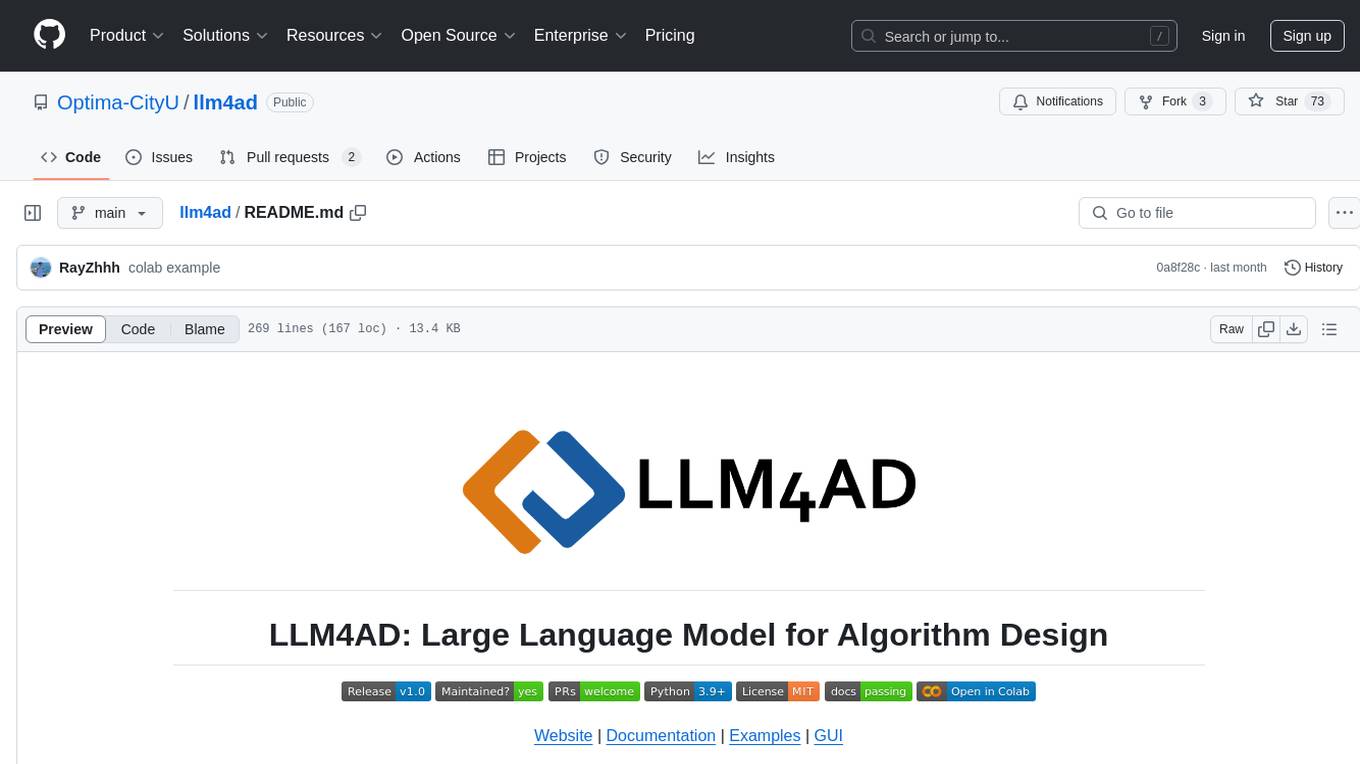
llm4ad
LLM4AD is an open-source Python-based platform leveraging Large Language Models (LLMs) for Automatic Algorithm Design (AD). It provides unified interfaces for methods, tasks, and LLMs, along with features like evaluation acceleration, secure evaluation, logs, GUI support, and more. The platform was originally developed for optimization tasks but is versatile enough to be used in other areas such as machine learning, science discovery, game theory, and engineering design. It offers various search methods and algorithm design tasks across different domains. LLM4AD supports remote LLM API, local HuggingFace LLM deployment, and custom LLM interfaces. The project is licensed under the MIT License and welcomes contributions, collaborations, and issue reports.