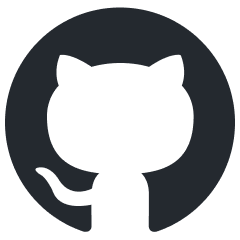
DeepRetrieval
DeepRetrieval - Hacking 🔥Real Search Engines and Text/Data Retrievers with LLM + RL
Stars: 198
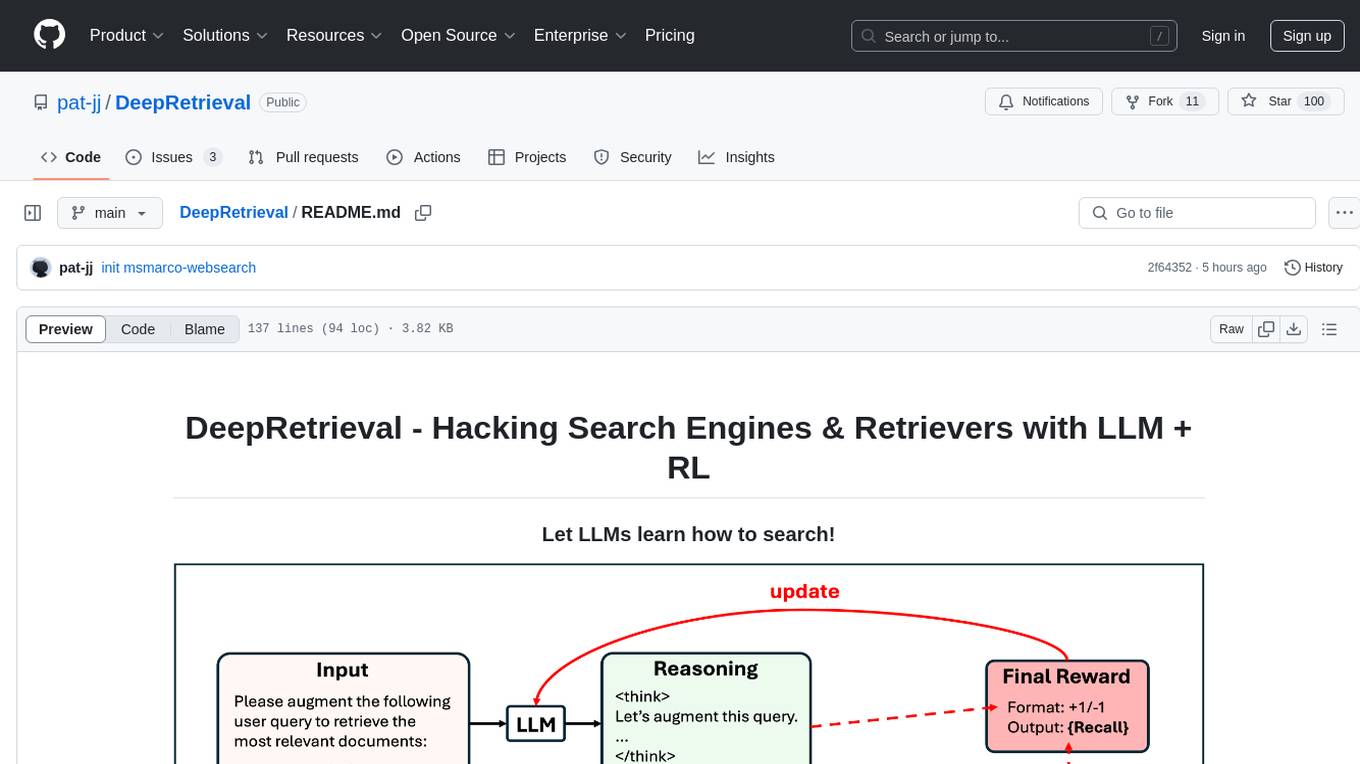
DeepRetrieval is a tool designed to enhance search engines and retrievers using Large Language Models (LLMs) and Reinforcement Learning (RL). It allows LLMs to learn how to search effectively by integrating with search engine APIs and customizing reward functions. The tool provides functionalities for data preparation, training, evaluation, and monitoring search performance. DeepRetrieval aims to improve information retrieval tasks by leveraging advanced AI techniques.
README:
Preliminary Technical Report (ArXiv preprint)
General Installation (for all retrieval methods):
conda create -n zero python=3.9
# install torch [or you can skip this step and let vllm to install the correct version for you]
pip install torch==2.4.0 --index-url https://download.pytorch.org/whl/cu121
# install vllm
pip3 install vllm==0.6.3 # or you can install 0.5.4, 0.4.2 and 0.3.1
pip3 install ray
# verl
cd code
pip install -e .
# flash attention 2
pip3 install flash-attn --no-build-isolation
# quality of life
pip install wandb IPython matplotlib
For Search Engine Retrieval, you can skip the following steps.
If using sparse retrieval (e.g., BM25) or dense retrieval (e.g., DPR), please also install the following:
# we use pyserini for efficient retrieval and evaluation
pip install pyserini # the version we used is 0.22.1
# if you don't have faiss installed, install it with:
pip install faiss-gpu==1.7.2 # the version we used is 1.7.2
# if you don't have java installed, install it with:
pip install install-jdk && python -c "import jdk; jdk.install('11')"
# support sql execution
pip install func_timeout
cd code
1. Data Preparation (required)
For example, for PubMed:
conda activate zero
python data_preprocess/pubmed.py
2. Get Your Search Engine API Key (required if use search engine)
For example, for PubMed, you may get it following the instruction here.
Then, put it in under code/verl/utils/reward_score/apis/
as pubmed_api.key
.
3. Reward function Related (optional)
Reward Design (e.g., in code/verl/utils/reward_score/pubmed.py
):
Recall | ≥ 0.7 | ≥ 0.5 | ≥ 0.4 | ≥ 0.3 | ≥ 0.1 | ≥ 0.05 | < 0.05 |
---|---|---|---|---|---|---|---|
Reward | +5.0 | +4.0 | +3.0 | +1.0 | +0.5 | +0.1 | -3.5 |
4. Customize Monitor Info (optional)
modify compute_reward_metrics()
in code/verl/trainer/ppo/ray_trainer.py
conda activate zero
For the following code, if you see Out-of-vram, try add critic.model.enable_gradient_checkpointing=True
to the script
For example, for PubMed:
sh scripts/train/pubmed.sh
sh scripts/eval/pubmed.sh
Result (checkpoint date: Feb 16)
Model | Method | Recall (Publication) | Recall (Trial) |
---|---|---|---|
GPT-4o | Zero-shot | 5.79 | 6.74 |
Few-shot | 7.67 | 4.69 | |
ICL | 19.72 | 14.26 | |
ICL+Few-shot | 11.95 | 7.98 | |
GPT-3.5 | Zero-shot | 4.01 | 3.37 |
Few-shot | 4.15 | 3.34 | |
ICL | 18.68 | 13.94 | |
ICL+Few-shot | 7.06 | 5.54 | |
Haiku-3 | Zero-shot | 10.98 | 11.59 |
Few-shot | 14.71 | 7.47 | |
ICL | 20.92 | 24.68 | |
ICL+Few-shot | 19.11 | 9.27 | |
Mistral-7B | Zero-shot | 7.18 | 8.08 |
LEADS$^{*}$ | Zero-shot | 24.68 | 32.11 |
DeepRetrieval | Zero-shot | 64.57 | 70.84 |
Table: Comparison of different models and methods on publication search and trial search tasks. Bold numbers indicate the best performance.
$^{*}$ LEADS: a state-of-the-art literature mining LLM trained on 20K reviews and 400K publications [https://arxiv.org/pdf/2501.16255]
This implementation is mainly based on verl and PySerini. The base model during the experiment is Qwen2.5-3B. We sincerely appreciate their contributions to the open-source community.
Current version (will update the author list upon project completion):
@misc{jiang2025deepretrievalpowerfulquerygeneration,
title={DeepRetrieval: Powerful Query Generation for Information Retrieval with Reinforcement Learning},
author={Pengcheng Jiang},
year={2025},
eprint={2503.00223},
archivePrefix={arXiv},
primaryClass={cs.IR},
url={https://arxiv.org/abs/2503.00223},
}
@article{deepretrieval,
title={DeepRetrieval: Hacking Real Search Engines and Retrievers with Large Language Models and Reinforcement Learning},
author={Jiang, Pengcheng and Lin, Jiacheng and Cao, Lang and Tian, Runchu and Kang, SeongKu and Wang, Zifeng and Sun, Jimeng and Han, Jiawei},
howpublished={\url{https://github.com/pat-jj/DeepRetrieval}},
year={2025}
}
Thanks for your interests! 😊
For Tasks:
Click tags to check more tools for each tasksFor Jobs:
Alternative AI tools for DeepRetrieval
Similar Open Source Tools
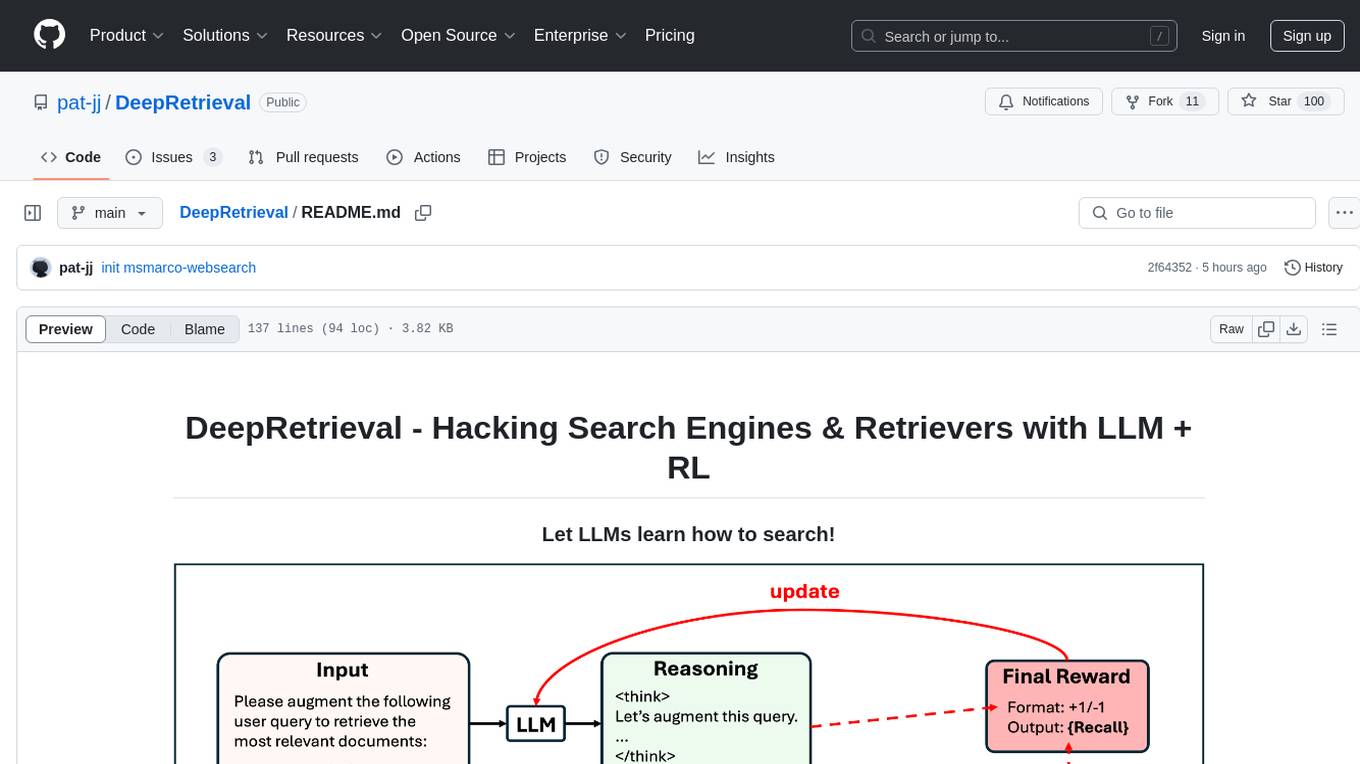
DeepRetrieval
DeepRetrieval is a tool designed to enhance search engines and retrievers using Large Language Models (LLMs) and Reinforcement Learning (RL). It allows LLMs to learn how to search effectively by integrating with search engine APIs and customizing reward functions. The tool provides functionalities for data preparation, training, evaluation, and monitoring search performance. DeepRetrieval aims to improve information retrieval tasks by leveraging advanced AI techniques.
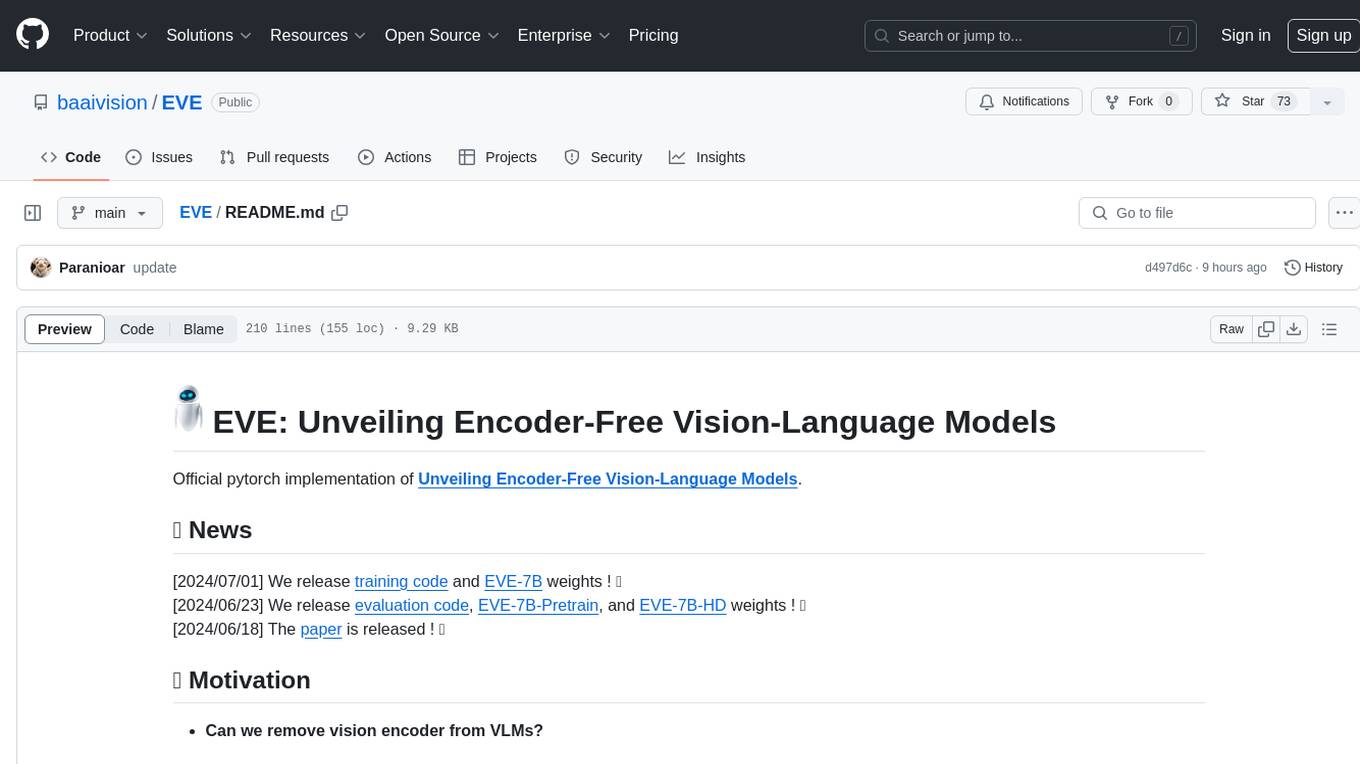
EVE
EVE is an official PyTorch implementation of Unveiling Encoder-Free Vision-Language Models. The project aims to explore the removal of vision encoders from Vision-Language Models (VLMs) and transfer LLMs to encoder-free VLMs efficiently. It also focuses on bridging the performance gap between encoder-free and encoder-based VLMs. EVE offers a superior capability with arbitrary image aspect ratio, data efficiency by utilizing publicly available data for pre-training, and training efficiency with a transparent and practical strategy for developing a pure decoder-only architecture across modalities.
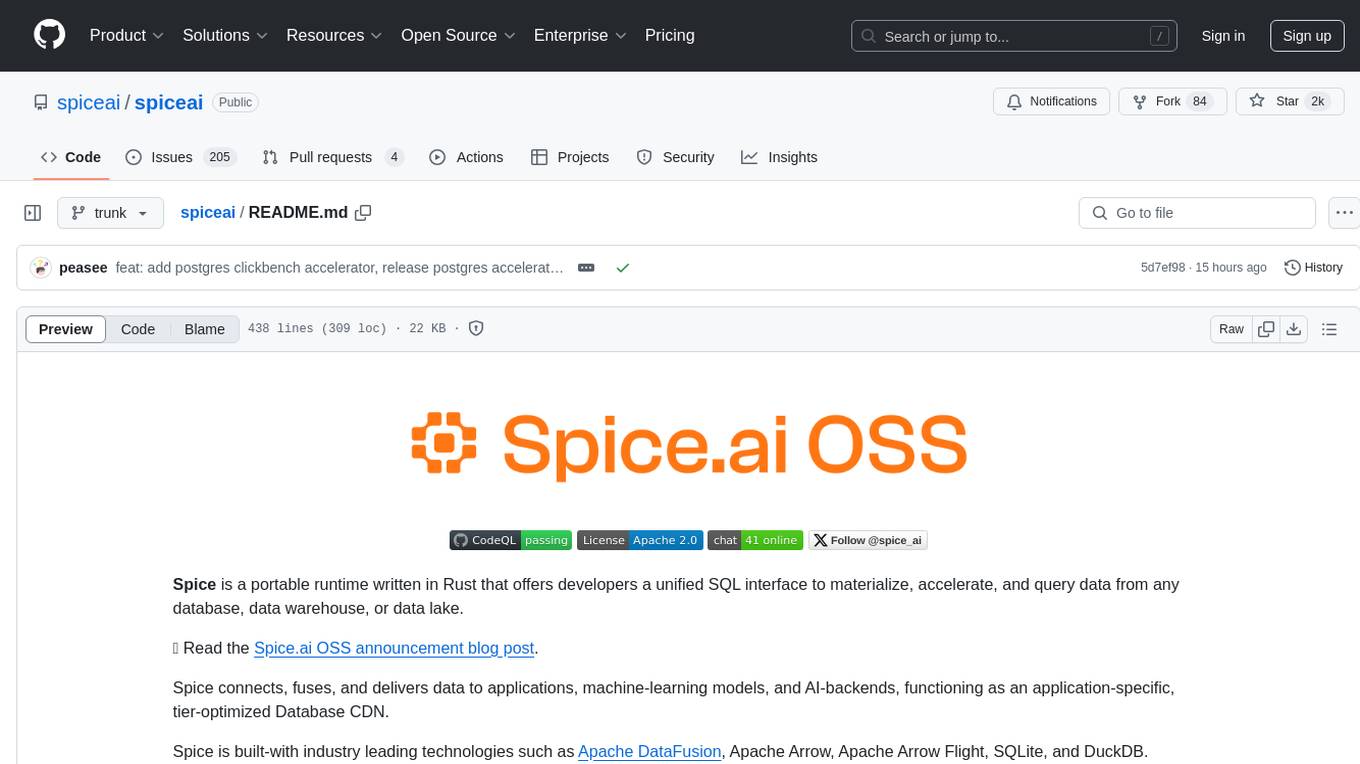
spiceai
Spice is a portable runtime written in Rust that offers developers a unified SQL interface to materialize, accelerate, and query data from any database, data warehouse, or data lake. It connects, fuses, and delivers data to applications, machine-learning models, and AI-backends, functioning as an application-specific, tier-optimized Database CDN. Built with industry-leading technologies such as Apache DataFusion, Apache Arrow, Apache Arrow Flight, SQLite, and DuckDB. Spice makes it fast and easy to query data from one or more sources using SQL, co-locating a managed dataset with applications or machine learning models, and accelerating it with Arrow in-memory, SQLite/DuckDB, or attached PostgreSQL for fast, high-concurrency, low-latency queries.
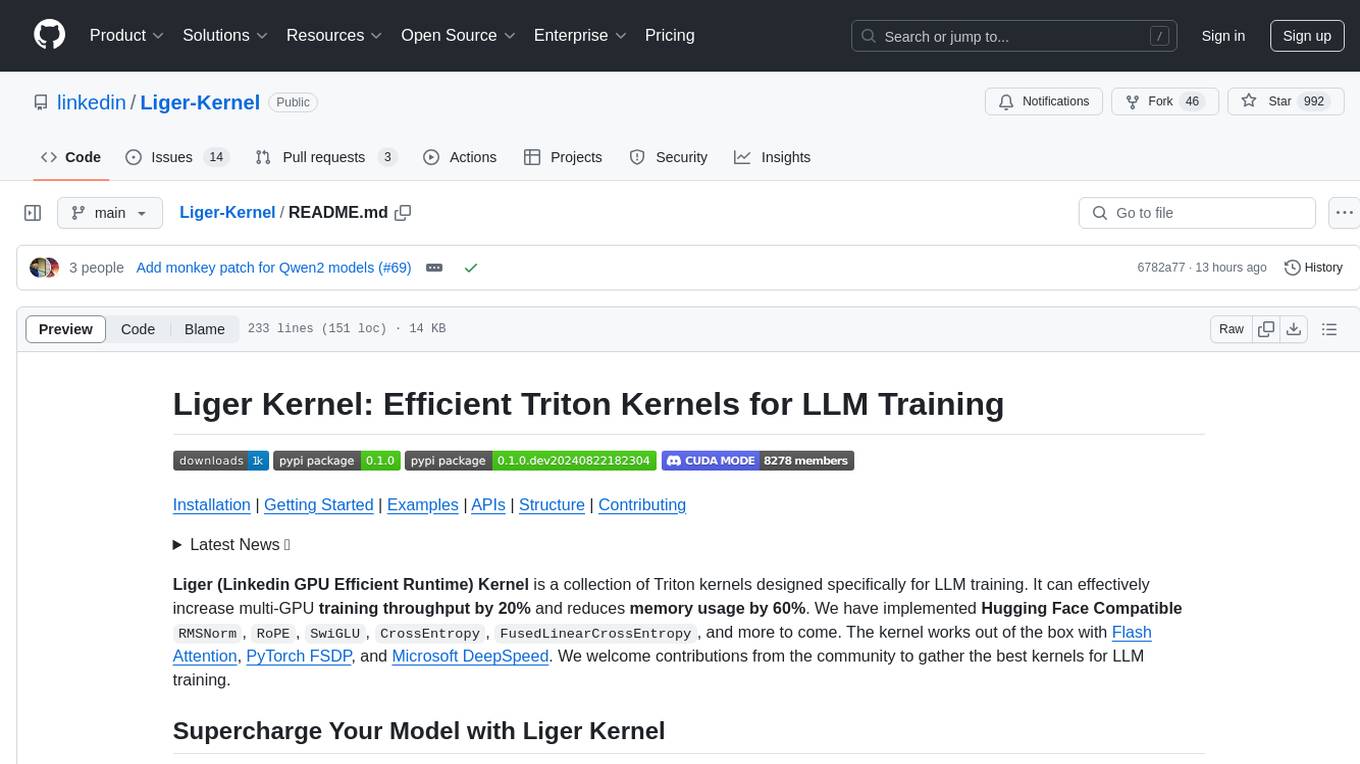
Liger-Kernel
Liger Kernel is a collection of Triton kernels designed for LLM training, increasing training throughput by 20% and reducing memory usage by 60%. It includes Hugging Face Compatible modules like RMSNorm, RoPE, SwiGLU, CrossEntropy, and FusedLinearCrossEntropy. The tool works with Flash Attention, PyTorch FSDP, and Microsoft DeepSpeed, aiming to enhance model efficiency and performance for researchers, ML practitioners, and curious novices.
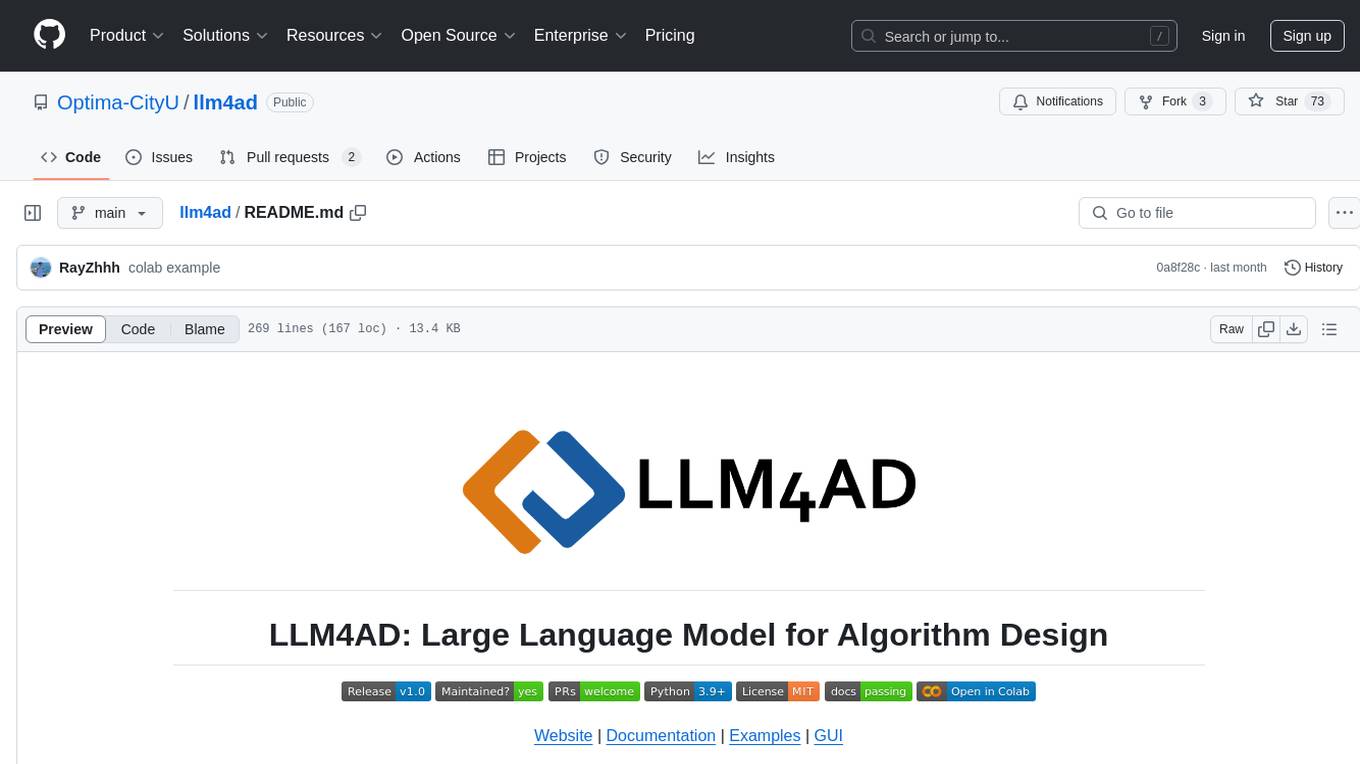
llm4ad
LLM4AD is an open-source Python-based platform leveraging Large Language Models (LLMs) for Automatic Algorithm Design (AD). It provides unified interfaces for methods, tasks, and LLMs, along with features like evaluation acceleration, secure evaluation, logs, GUI support, and more. The platform was originally developed for optimization tasks but is versatile enough to be used in other areas such as machine learning, science discovery, game theory, and engineering design. It offers various search methods and algorithm design tasks across different domains. LLM4AD supports remote LLM API, local HuggingFace LLM deployment, and custom LLM interfaces. The project is licensed under the MIT License and welcomes contributions, collaborations, and issue reports.
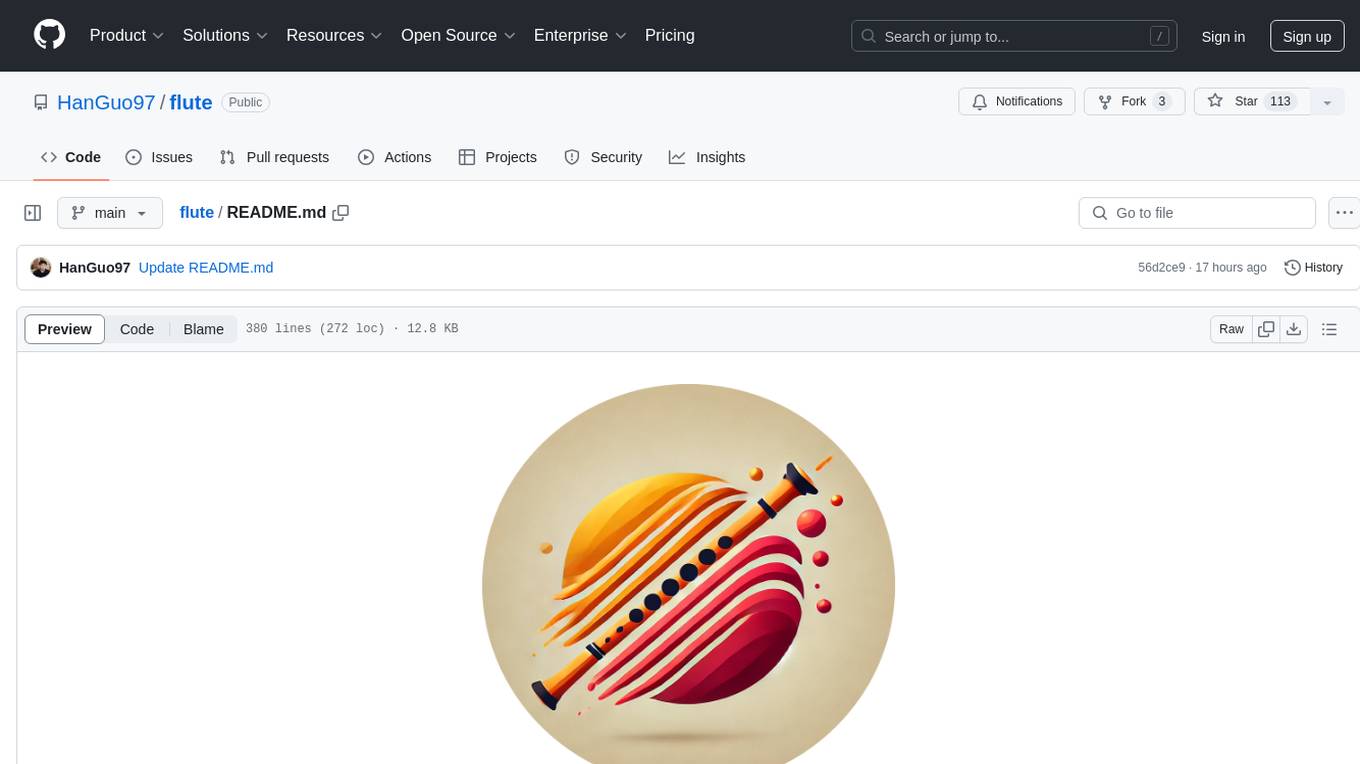
flute
FLUTE (Flexible Lookup Table Engine for LUT-quantized LLMs) is a tool designed for uniform quantization and lookup table quantization of weights in lower-precision intervals. It offers flexibility in mapping intervals to arbitrary values through a lookup table. FLUTE supports various quantization formats such as int4, int3, int2, fp4, fp3, fp2, nf4, nf3, nf2, and even custom tables. The tool also introduces new quantization algorithms like Learned Normal Float (NFL) for improved performance and calibration data learning. FLUTE provides benchmarks, model zoo, and integration with frameworks like vLLM and HuggingFace for easy deployment and usage.
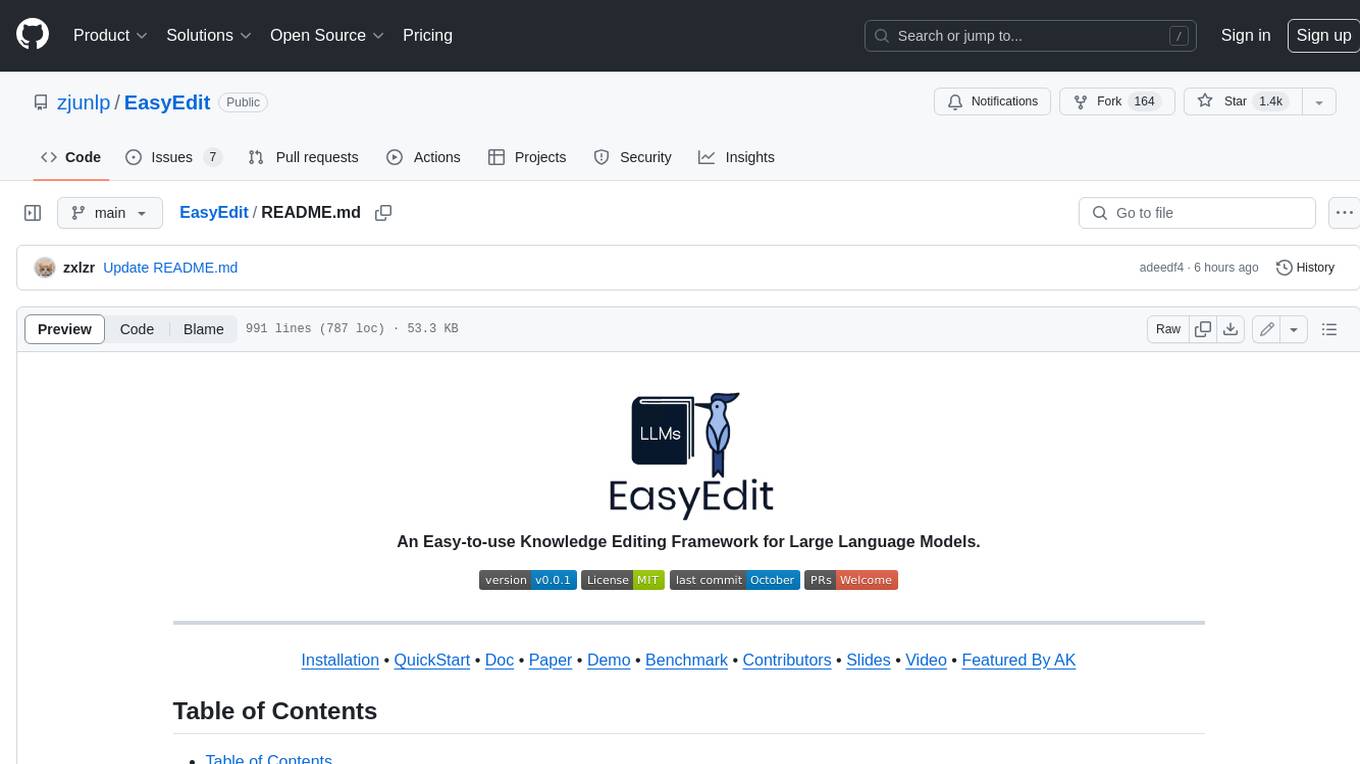
EasyEdit
EasyEdit is a Python package for edit Large Language Models (LLM) like `GPT-J`, `Llama`, `GPT-NEO`, `GPT2`, `T5`(support models from **1B** to **65B**), the objective of which is to alter the behavior of LLMs efficiently within a specific domain without negatively impacting performance across other inputs. It is designed to be easy to use and easy to extend.
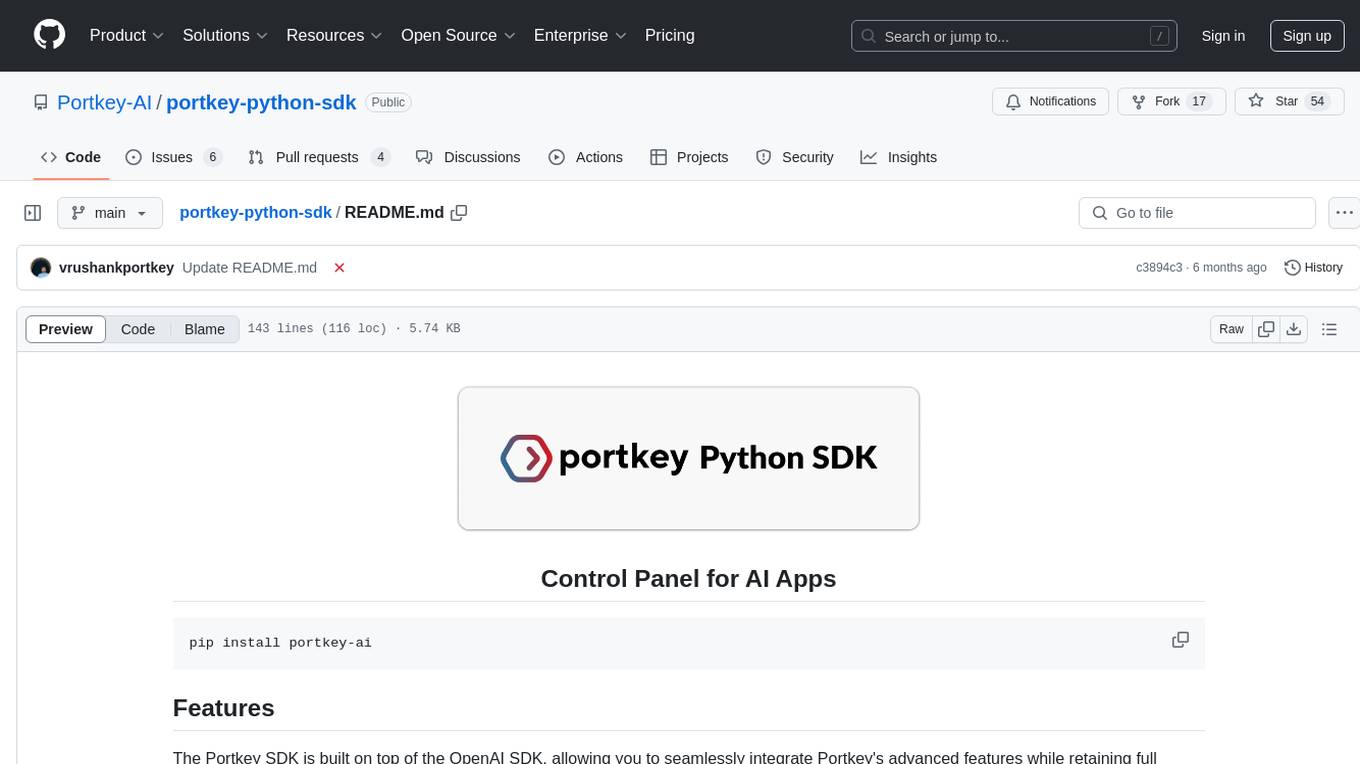
portkey-python-sdk
The Portkey Python SDK is a control panel for AI apps that allows seamless integration of Portkey's advanced features with OpenAI methods. It provides features such as AI gateway for unified API signature, interoperability, automated fallbacks & retries, load balancing, semantic caching, virtual keys, request timeouts, observability with logging, requests tracing, custom metadata, feedback collection, and analytics. Users can make requests to OpenAI using Portkey SDK and also use async functionality. The SDK is compatible with OpenAI SDK methods and offers Portkey-specific methods like feedback and prompts. It supports various providers and encourages contributions through Github issues or direct contact via email or Discord.
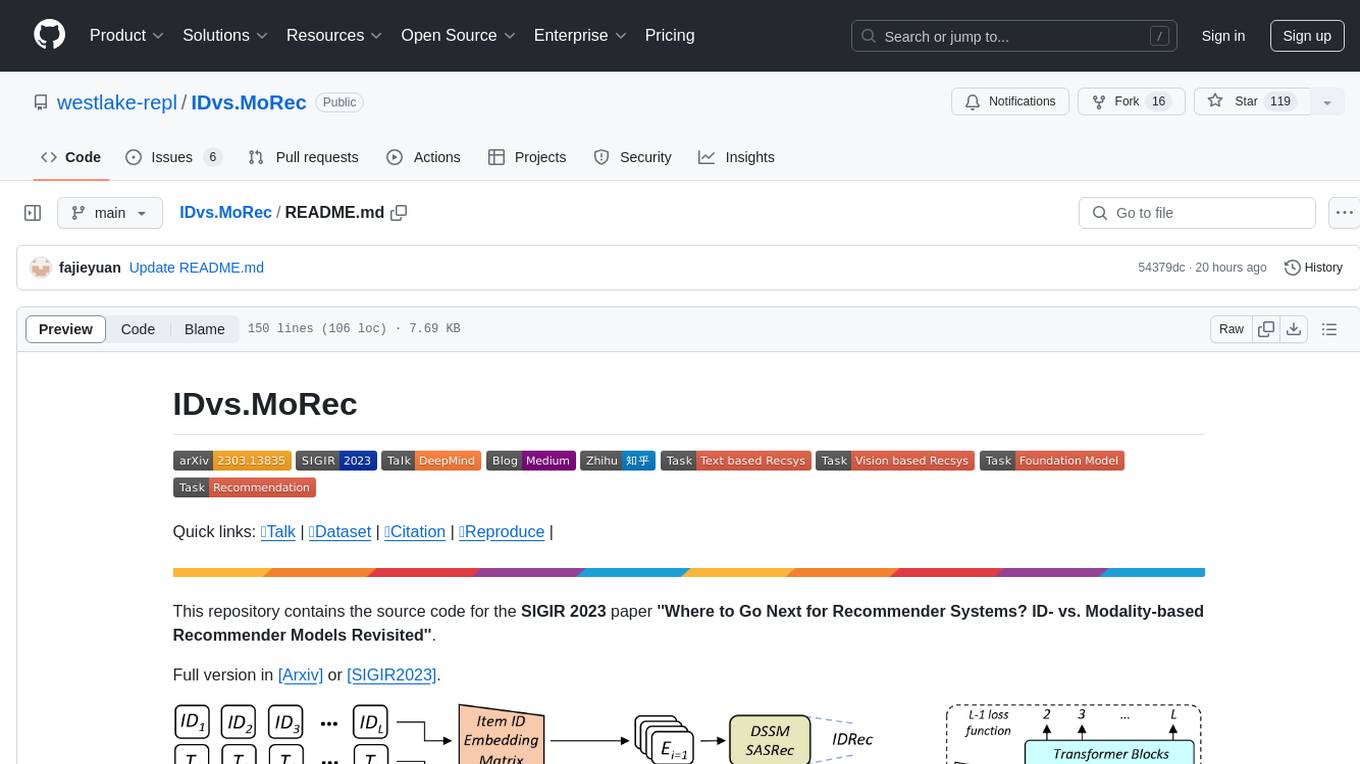
IDvs.MoRec
This repository contains the source code for the SIGIR 2023 paper 'Where to Go Next for Recommender Systems? ID- vs. Modality-based Recommender Models Revisited'. It provides resources for evaluating foundation, transferable, multi-modal, and LLM recommendation models, along with datasets, pre-trained models, and training strategies for IDRec and MoRec using in-batch debiased cross-entropy loss. The repository also offers large-scale datasets, code for SASRec with in-batch debias cross-entropy loss, and information on joining the lab for research opportunities.
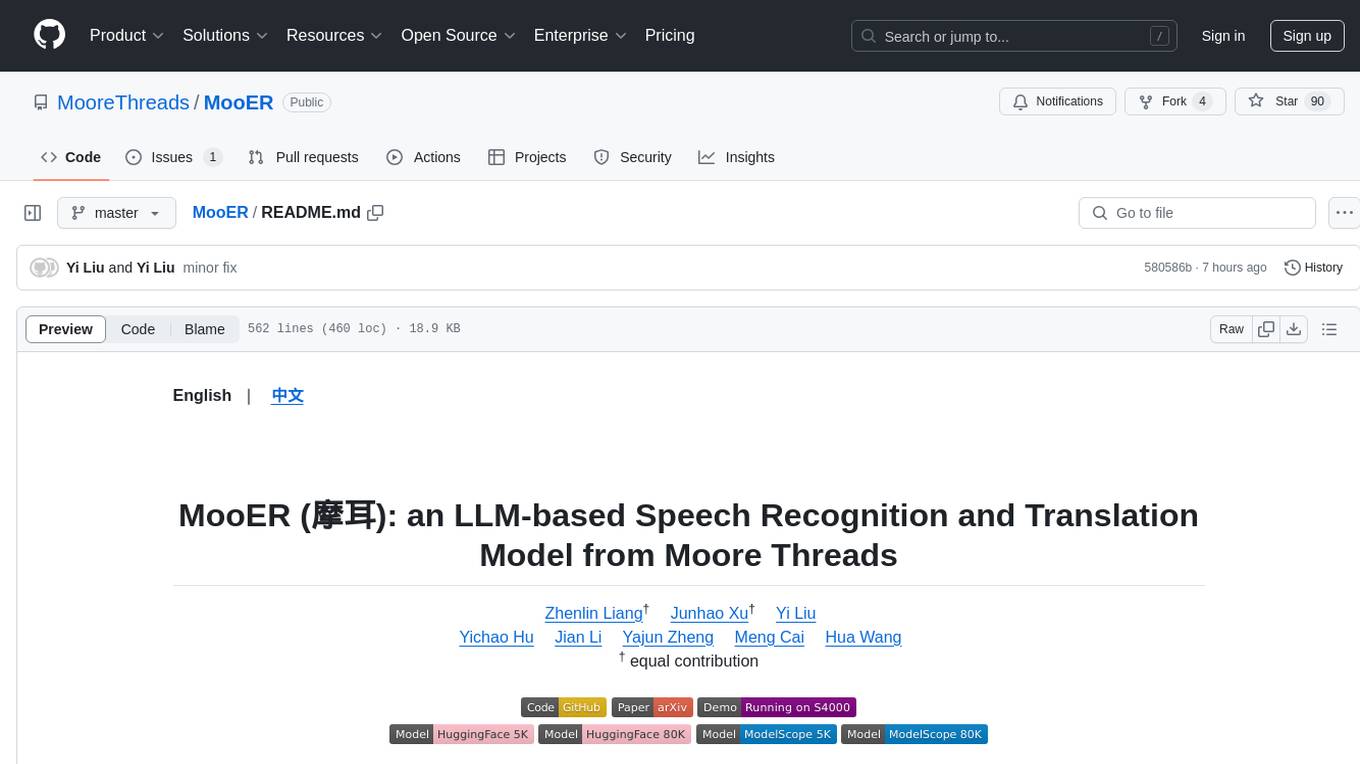
MooER
MooER (摩耳) is an LLM-based speech recognition and translation model developed by Moore Threads. It allows users to transcribe speech into text (ASR) and translate speech into other languages (AST) in an end-to-end manner. The model was trained using 5K hours of data and is now also available with an 80K hours version. MooER is the first LLM-based speech model trained and inferred using domestic GPUs. The repository includes pretrained models, inference code, and a Gradio demo for a better user experience.
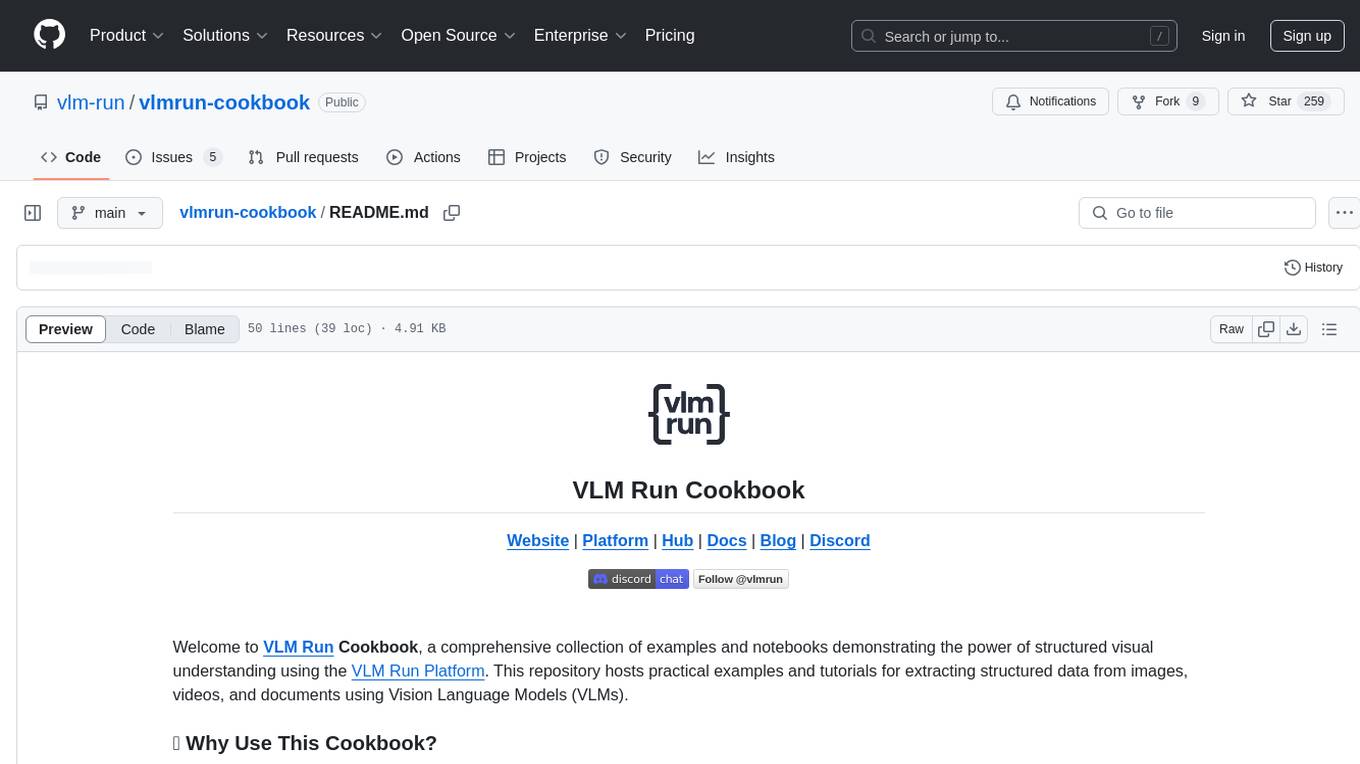
vlmrun-cookbook
VLM Run Cookbook is a repository containing practical examples and tutorials for extracting structured data from images, videos, and documents using Vision Language Models (VLMs). It offers comprehensive Colab notebooks demonstrating real-world applications of VLM Run, with complete code and documentation for easy adaptation. The examples cover various domains such as financial documents and TV news analysis.
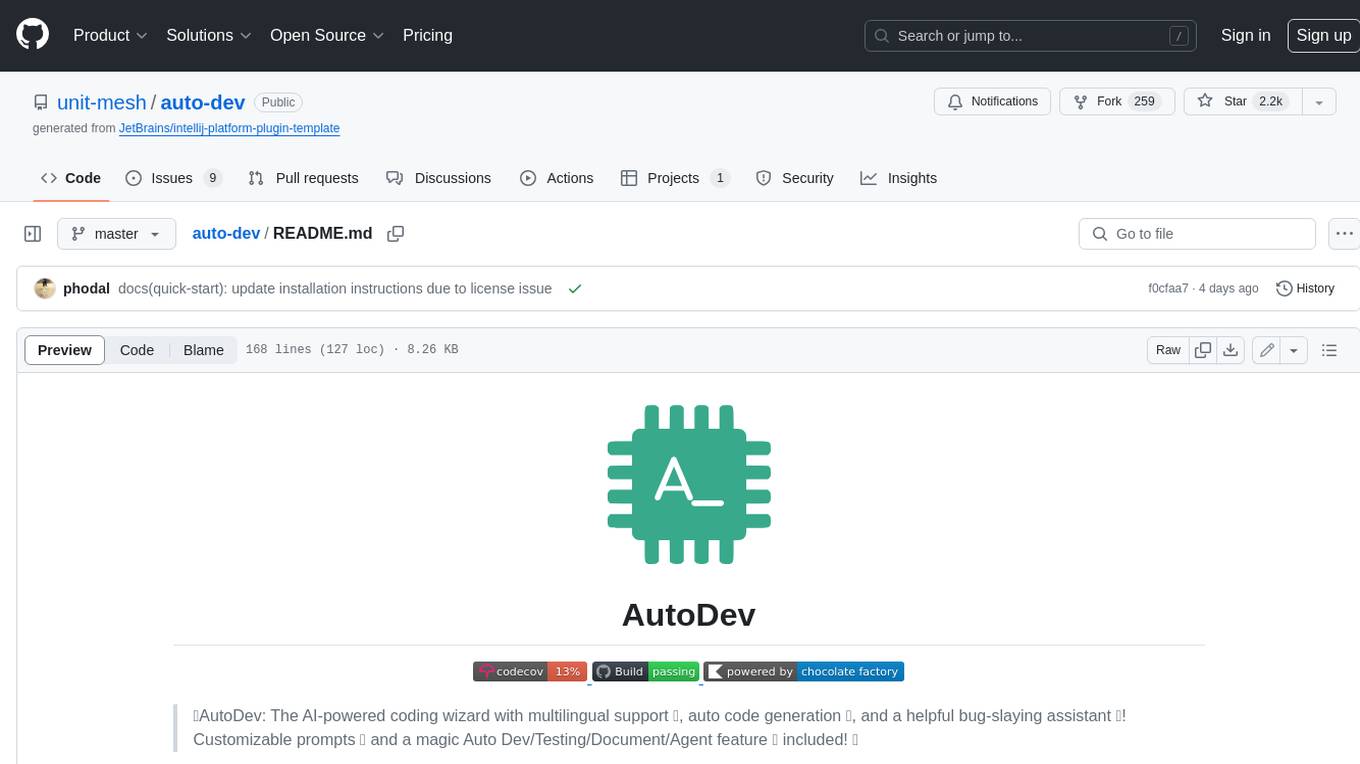
auto-dev
AutoDev is an AI-powered coding wizard that supports multiple languages, including Java, Kotlin, JavaScript/TypeScript, Rust, Python, Golang, C/C++/OC, and more. It offers a range of features, including auto development mode, copilot mode, chat with AI, customization options, SDLC support, custom AI agent integration, and language features such as language support, extensions, and a DevIns language for AI agent development. AutoDev is designed to assist developers with tasks such as auto code generation, bug detection, code explanation, exception tracing, commit message generation, code review content generation, smart refactoring, Dockerfile generation, CI/CD config file generation, and custom shell/command generation. It also provides a built-in LLM fine-tune model and supports UnitEval for LLM result evaluation and UnitGen for code-LLM fine-tune data generation.
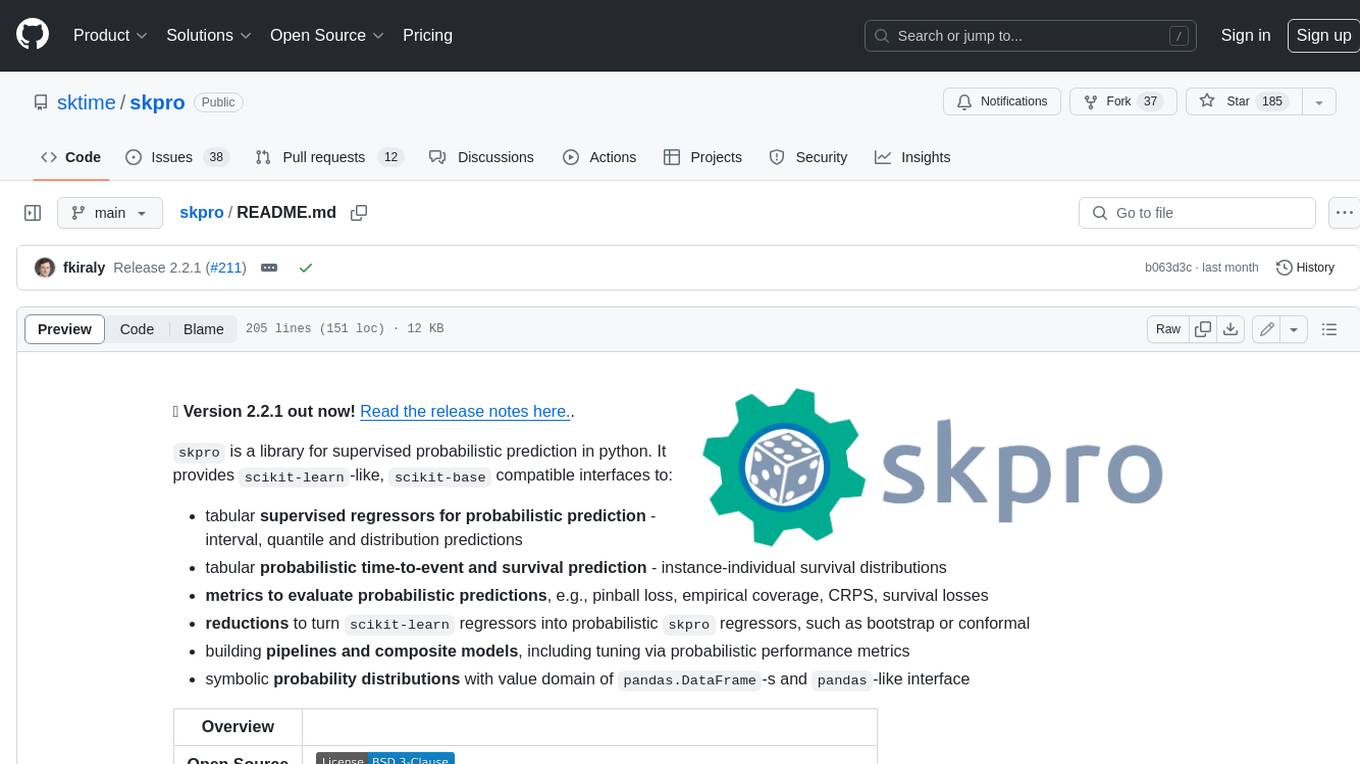
skpro
skpro is a library for supervised probabilistic prediction in python. It provides `scikit-learn`-like, `scikit-base` compatible interfaces to: * tabular **supervised regressors for probabilistic prediction** \- interval, quantile and distribution predictions * tabular **probabilistic time-to-event and survival prediction** \- instance-individual survival distributions * **metrics to evaluate probabilistic predictions** , e.g., pinball loss, empirical coverage, CRPS, survival losses * **reductions** to turn `scikit-learn` regressors into probabilistic `skpro` regressors, such as bootstrap or conformal * building **pipelines and composite models** , including tuning via probabilistic performance metrics * symbolic **probability distributions** with value domain of `pandas.DataFrame`-s and `pandas`-like interface
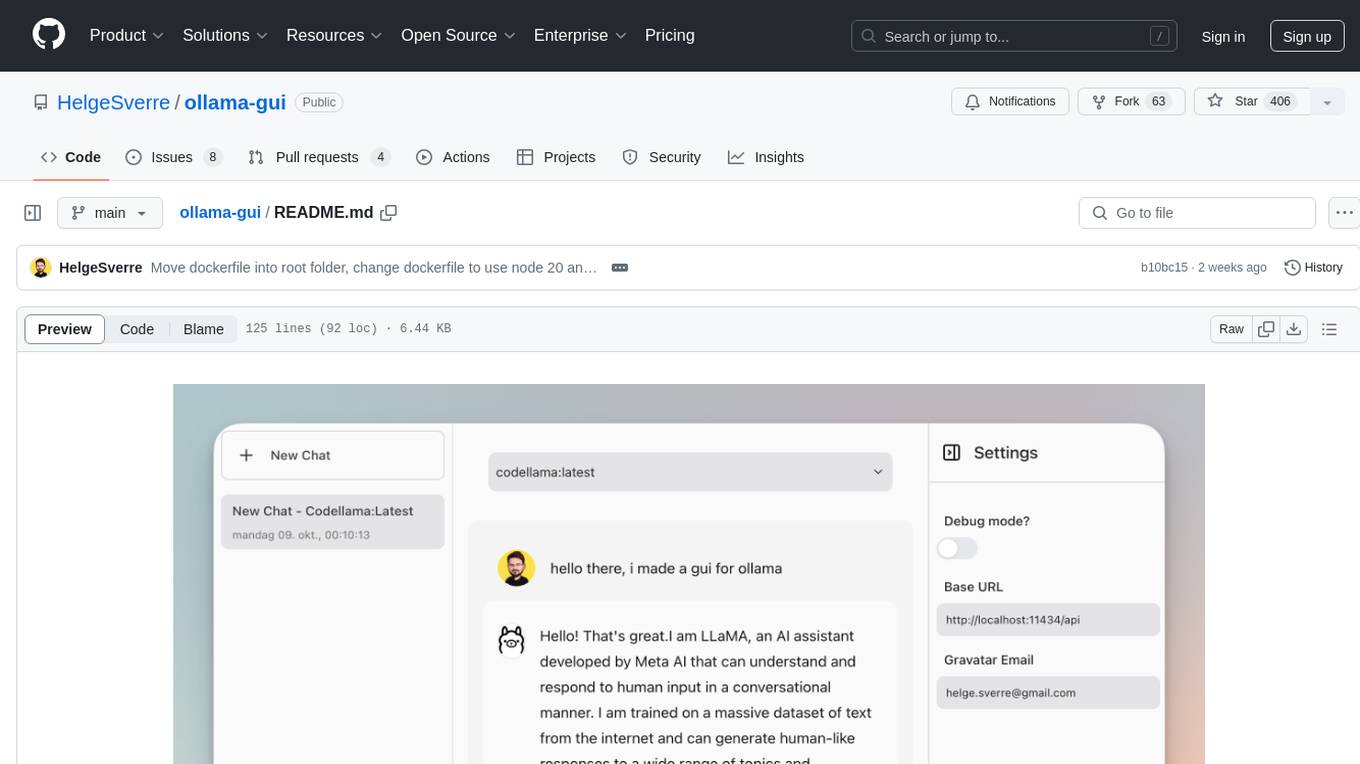
ollama-gui
Ollama GUI is a web interface for ollama.ai, a tool that enables running Large Language Models (LLMs) on your local machine. It provides a user-friendly platform for chatting with LLMs and accessing various models for text generation. Users can easily interact with different models, manage chat history, and explore available models through the web interface. The tool is built with Vue.js, Vite, and Tailwind CSS, offering a modern and responsive design for seamless user experience.
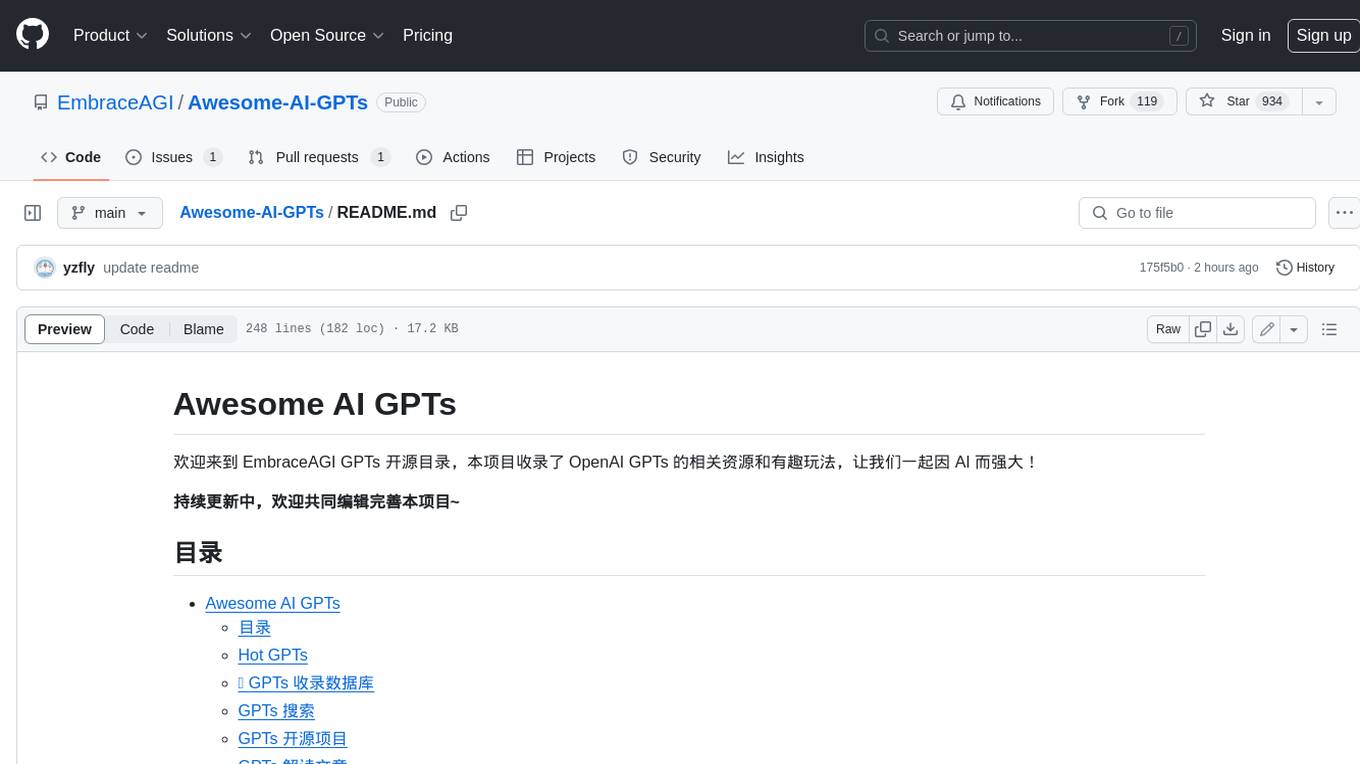
Awesome-AI-GPTs
Awesome AI GPTs is an open repository that collects resources and fun ways to use OpenAI GPTs. It includes databases, search tools, open-source projects, articles, attack and defense strategies, installation of custom plugins, knowledge bases, and community interactions related to GPTs. Users can find curated lists, leaked prompts, and various GPT applications in this repository. The project aims to empower users with AI capabilities and foster collaboration in the AI community.
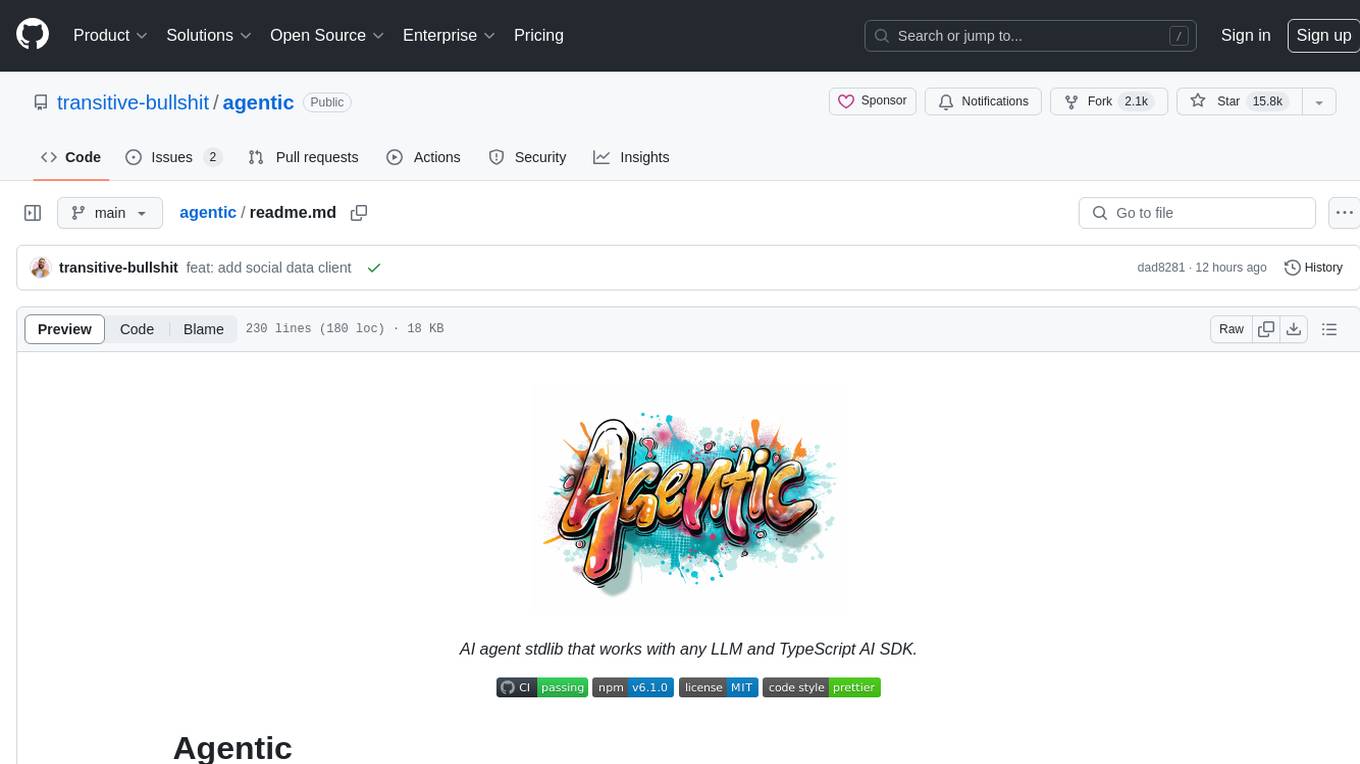
agentic
Agentic is a standard AI functions/tools library optimized for TypeScript and LLM-based apps, compatible with major AI SDKs. It offers a set of thoroughly tested AI functions that can be used with favorite AI SDKs without writing glue code. The library includes various clients for services like Bing web search, calculator, Clearbit data resolution, Dexa podcast questions, and more. It also provides compound tools like SearchAndCrawl and supports multiple AI SDKs such as OpenAI, Vercel AI SDK, LangChain, LlamaIndex, Firebase Genkit, and Dexa Dexter. The goal is to create minimal clients with strongly-typed TypeScript DX, composable AIFunctions via AIFunctionSet, and compatibility with major TS AI SDKs.
For similar tasks
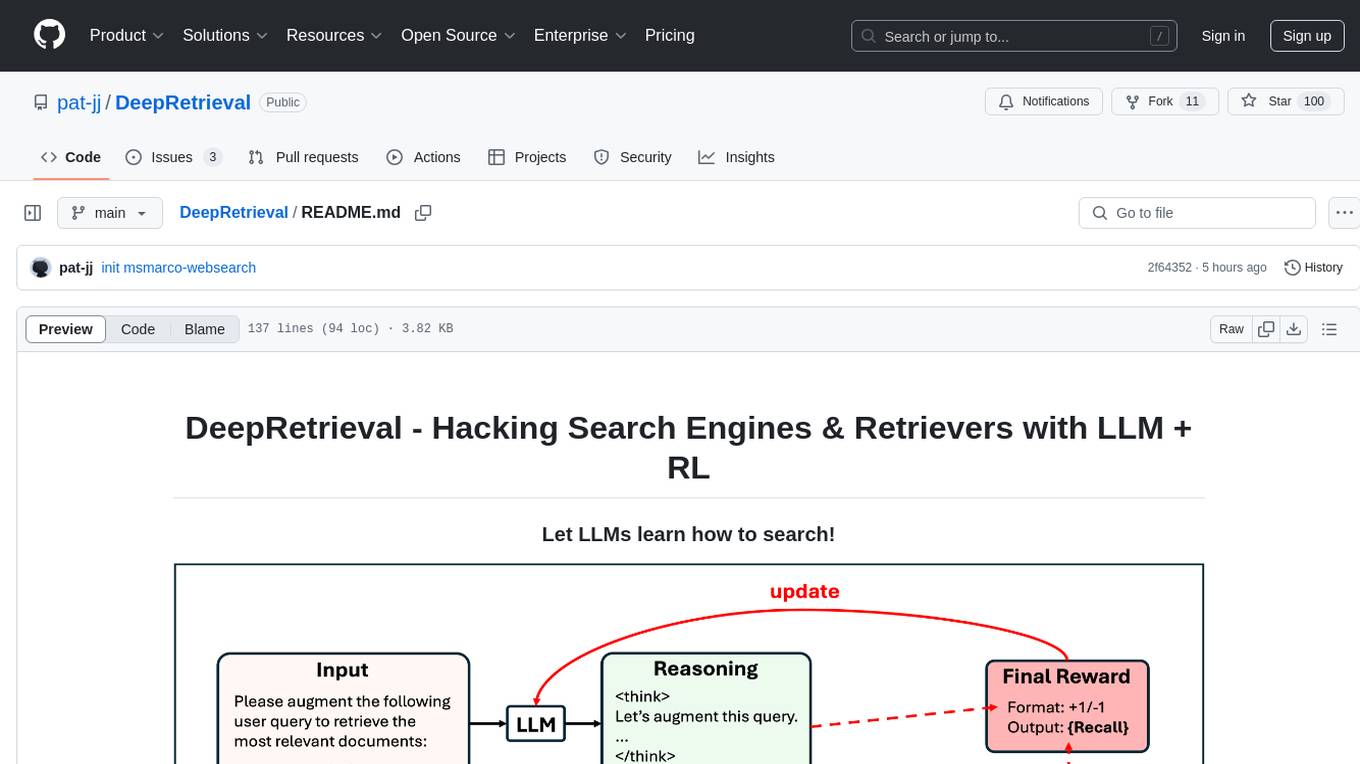
DeepRetrieval
DeepRetrieval is a tool designed to enhance search engines and retrievers using Large Language Models (LLMs) and Reinforcement Learning (RL). It allows LLMs to learn how to search effectively by integrating with search engine APIs and customizing reward functions. The tool provides functionalities for data preparation, training, evaluation, and monitoring search performance. DeepRetrieval aims to improve information retrieval tasks by leveraging advanced AI techniques.
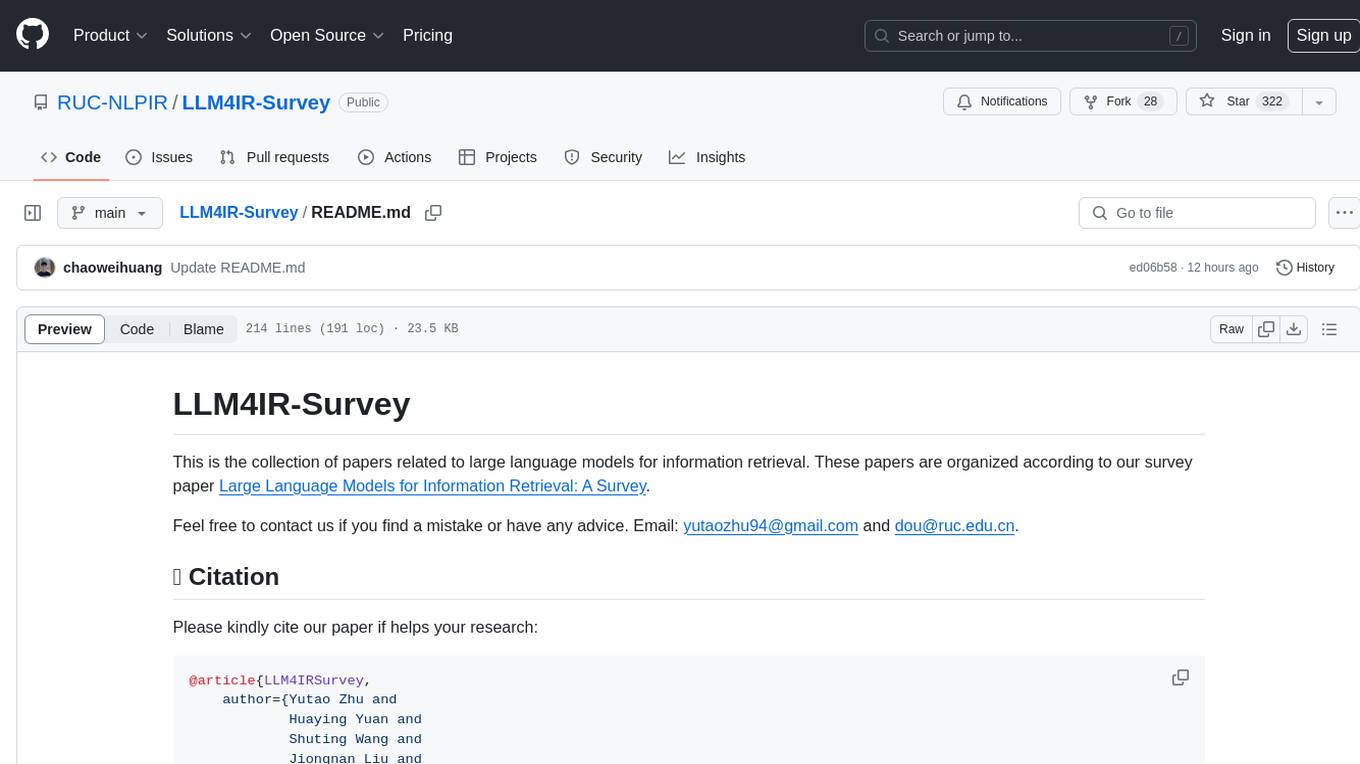
LLM4IR-Survey
LLM4IR-Survey is a collection of papers related to large language models for information retrieval, organized according to the survey paper 'Large Language Models for Information Retrieval: A Survey'. It covers various aspects such as query rewriting, retrievers, rerankers, readers, search agents, and more, providing insights into the integration of large language models with information retrieval systems.
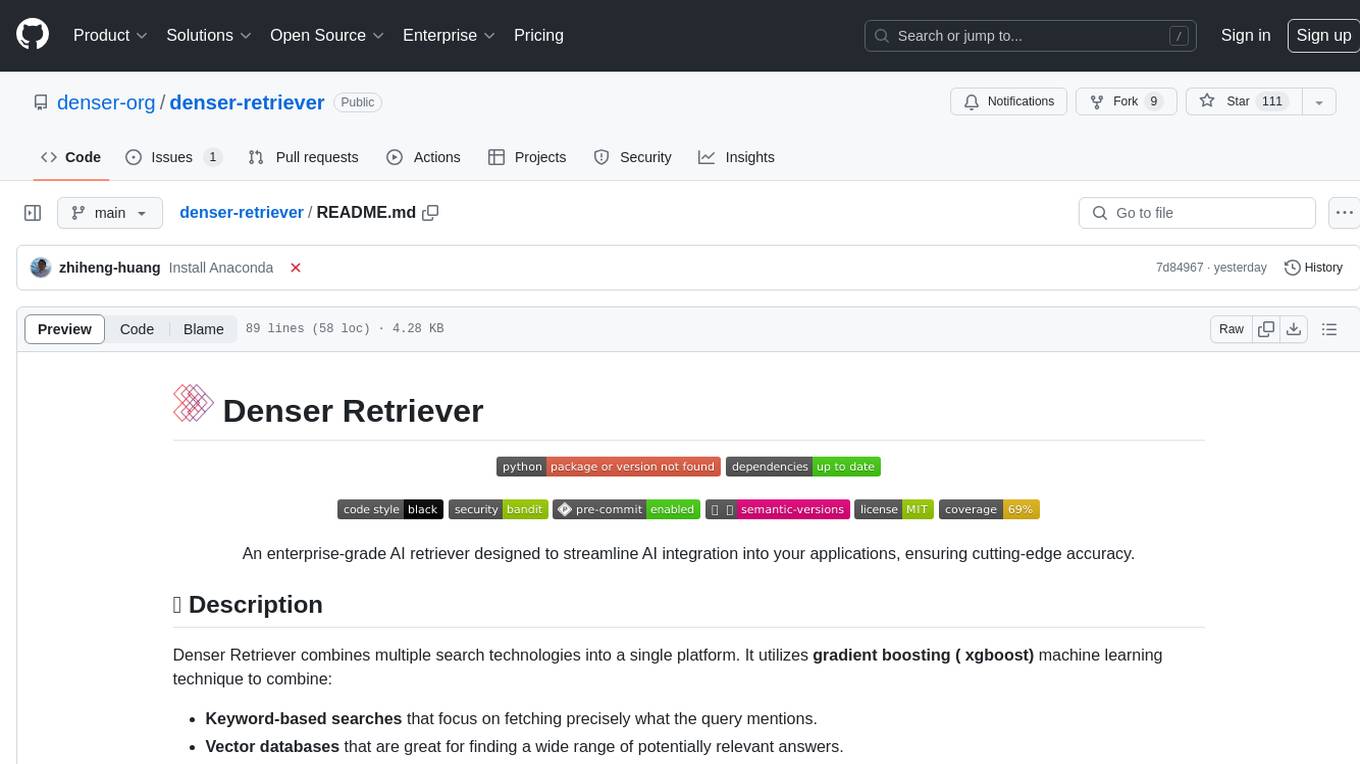
denser-retriever
Denser Retriever is an enterprise-grade AI retriever designed to streamline AI integration into applications, combining keyword-based searches, vector databases, and machine learning rerankers using xgboost. It provides state-of-the-art accuracy on MTEB Retrieval benchmarking and supports various heterogeneous retrievers for end-to-end applications like chatbots and semantic search.
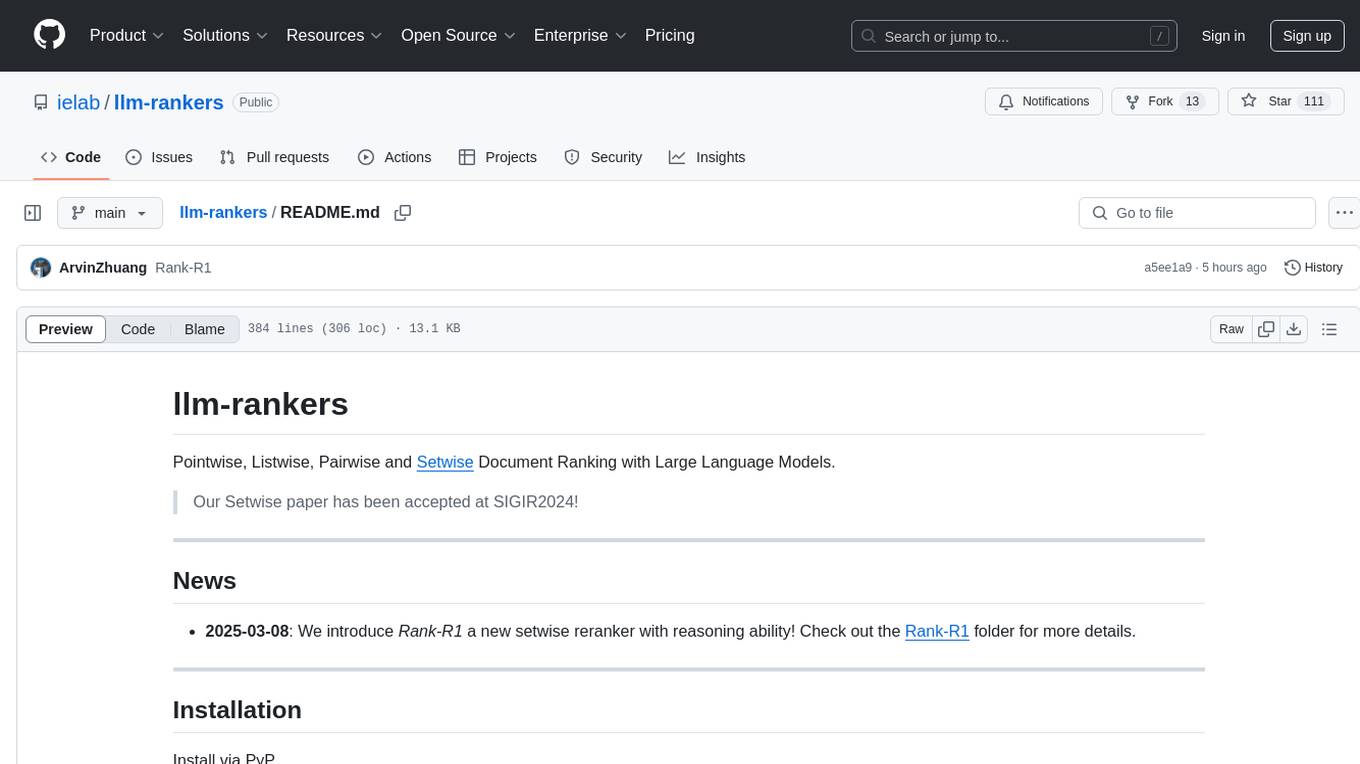
llm-rankers
llm-rankers is a repository that provides implementations for Pointwise, Listwise, Pairwise, and Setwise Document Ranking using Large Language Models. It includes various methods for reranking documents retrieved by a first-stage retriever, such as BM25. The repository offers examples and code snippets for using LLMs to improve document ranking performance in information retrieval tasks. Additionally, it introduces a new setwise reranker called Rank-R1 with reasoning ability.
For similar jobs
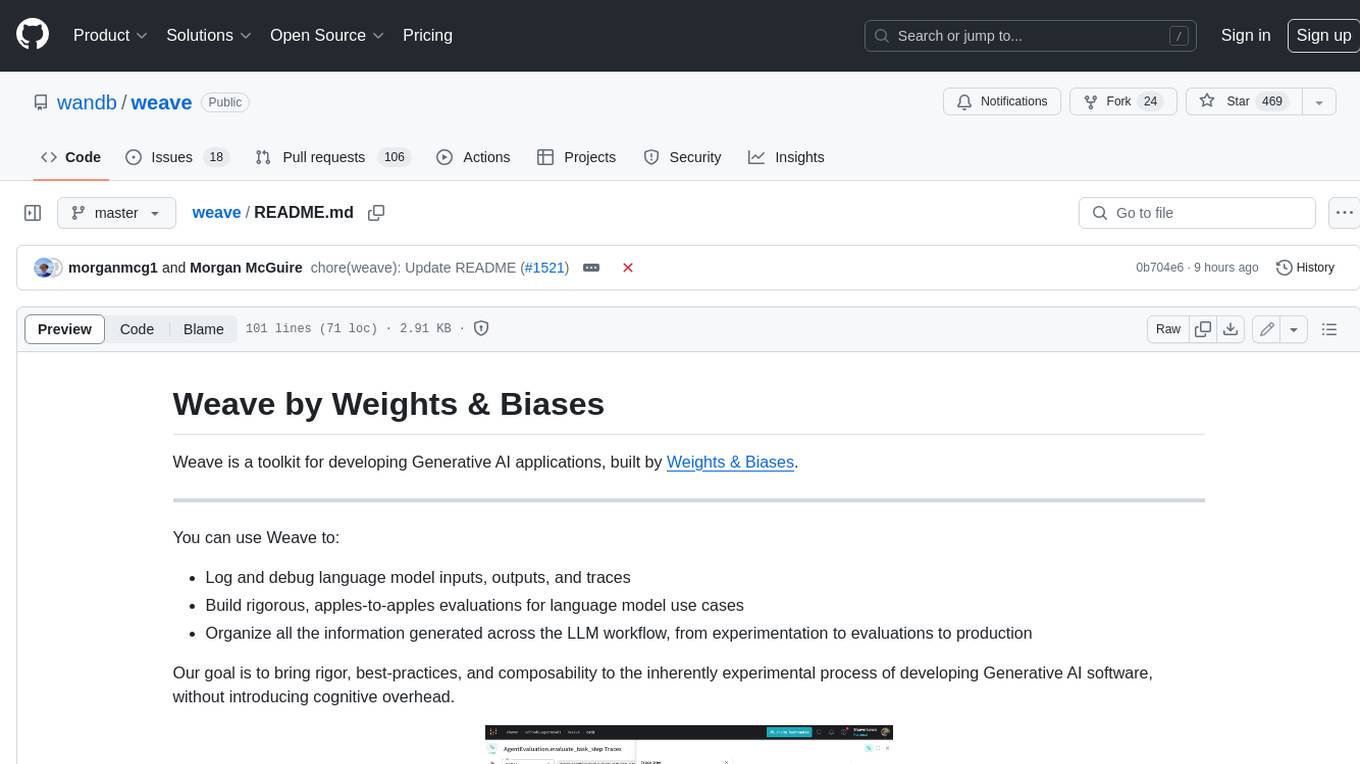
weave
Weave is a toolkit for developing Generative AI applications, built by Weights & Biases. With Weave, you can log and debug language model inputs, outputs, and traces; build rigorous, apples-to-apples evaluations for language model use cases; and organize all the information generated across the LLM workflow, from experimentation to evaluations to production. Weave aims to bring rigor, best-practices, and composability to the inherently experimental process of developing Generative AI software, without introducing cognitive overhead.
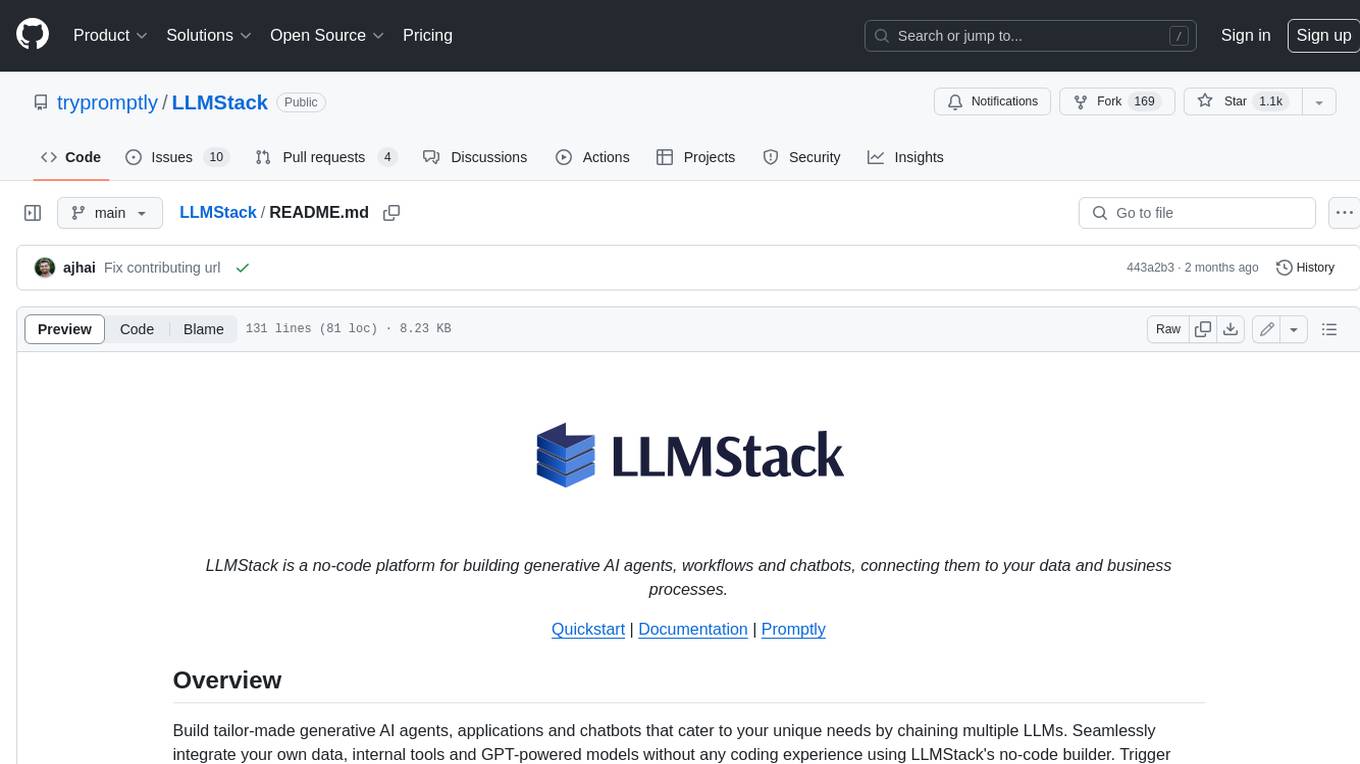
LLMStack
LLMStack is a no-code platform for building generative AI agents, workflows, and chatbots. It allows users to connect their own data, internal tools, and GPT-powered models without any coding experience. LLMStack can be deployed to the cloud or on-premise and can be accessed via HTTP API or triggered from Slack or Discord.
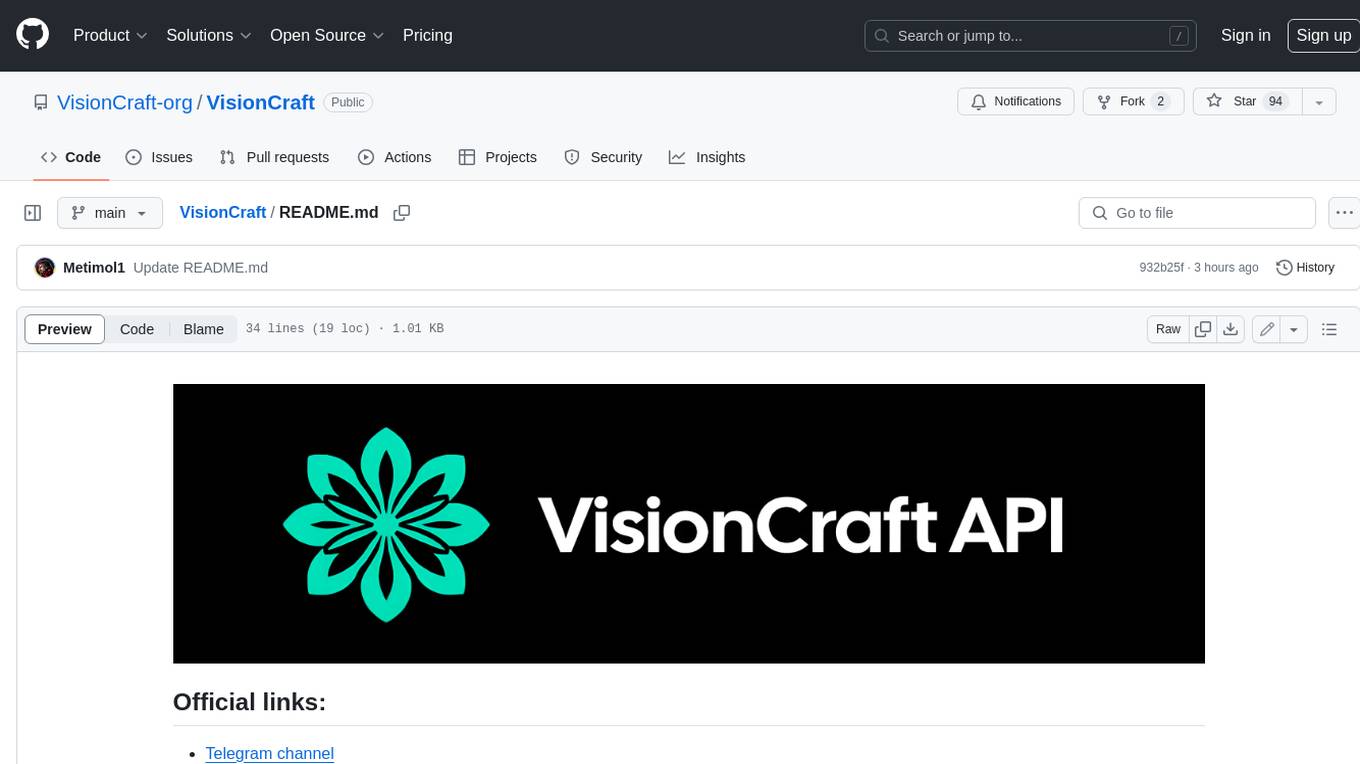
VisionCraft
The VisionCraft API is a free API for using over 100 different AI models. From images to sound.
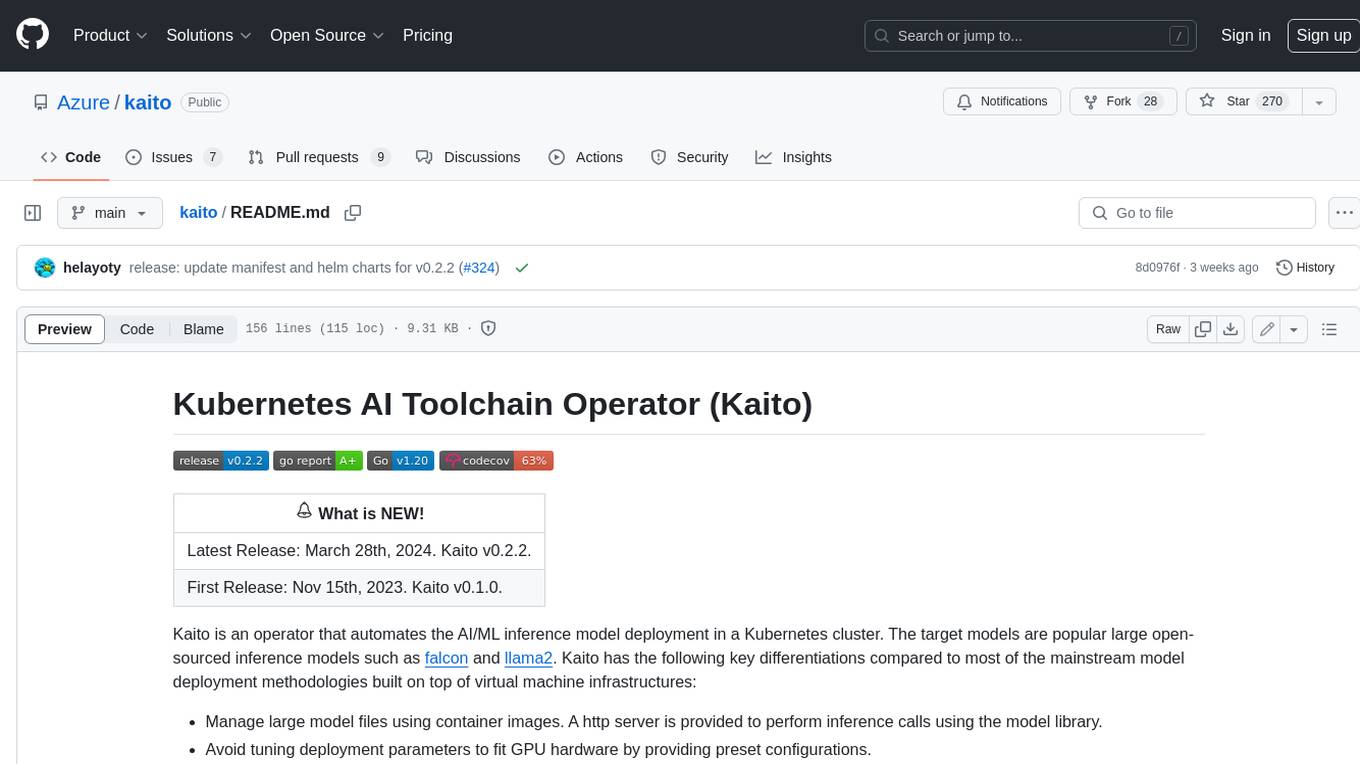
kaito
Kaito is an operator that automates the AI/ML inference model deployment in a Kubernetes cluster. It manages large model files using container images, avoids tuning deployment parameters to fit GPU hardware by providing preset configurations, auto-provisions GPU nodes based on model requirements, and hosts large model images in the public Microsoft Container Registry (MCR) if the license allows. Using Kaito, the workflow of onboarding large AI inference models in Kubernetes is largely simplified.
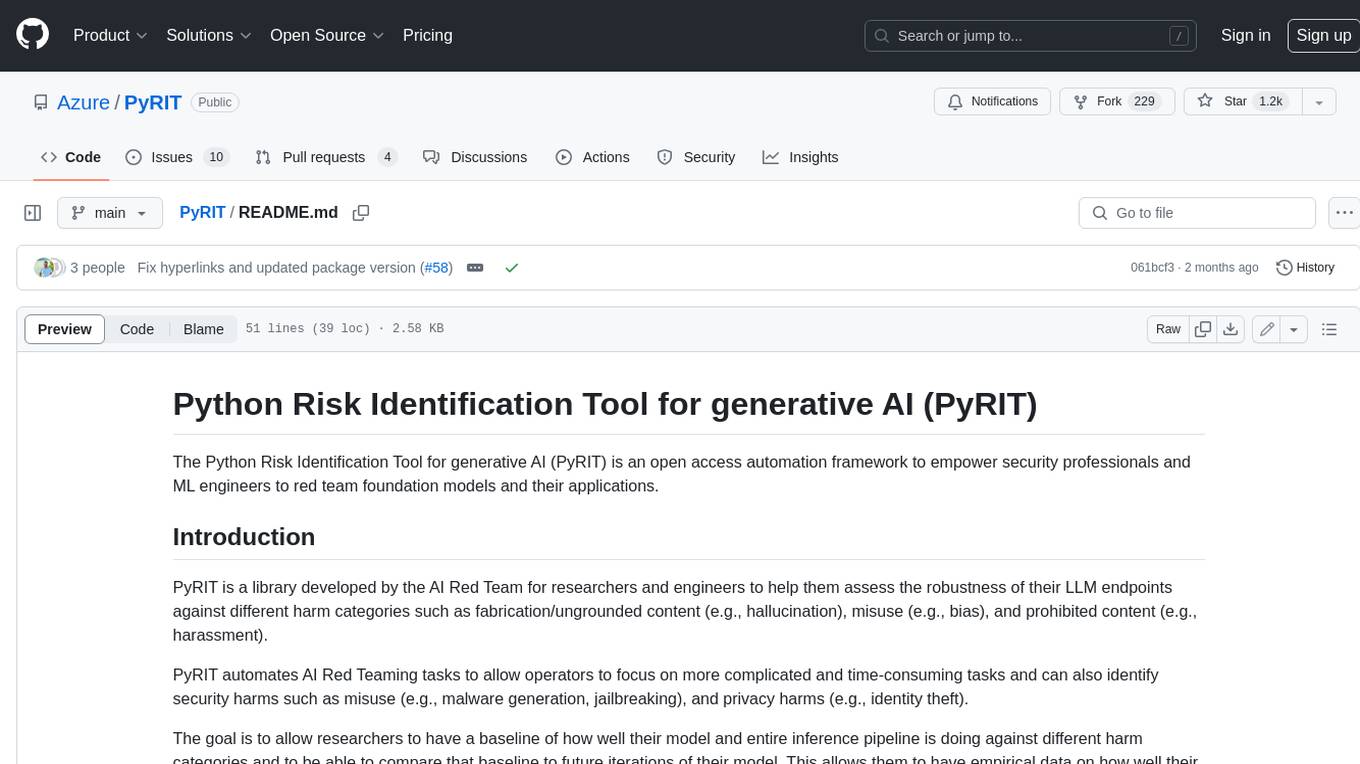
PyRIT
PyRIT is an open access automation framework designed to empower security professionals and ML engineers to red team foundation models and their applications. It automates AI Red Teaming tasks to allow operators to focus on more complicated and time-consuming tasks and can also identify security harms such as misuse (e.g., malware generation, jailbreaking), and privacy harms (e.g., identity theft). The goal is to allow researchers to have a baseline of how well their model and entire inference pipeline is doing against different harm categories and to be able to compare that baseline to future iterations of their model. This allows them to have empirical data on how well their model is doing today, and detect any degradation of performance based on future improvements.
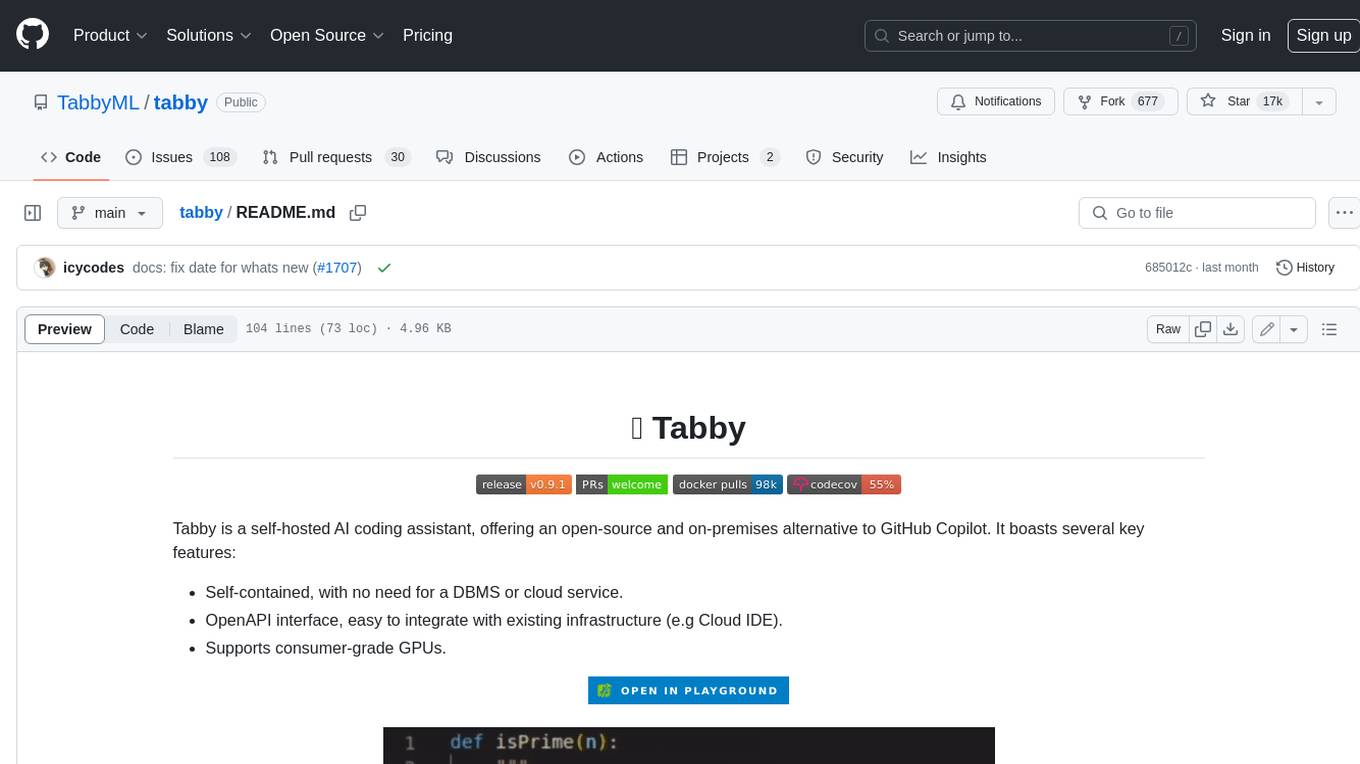
tabby
Tabby is a self-hosted AI coding assistant, offering an open-source and on-premises alternative to GitHub Copilot. It boasts several key features: * Self-contained, with no need for a DBMS or cloud service. * OpenAPI interface, easy to integrate with existing infrastructure (e.g Cloud IDE). * Supports consumer-grade GPUs.
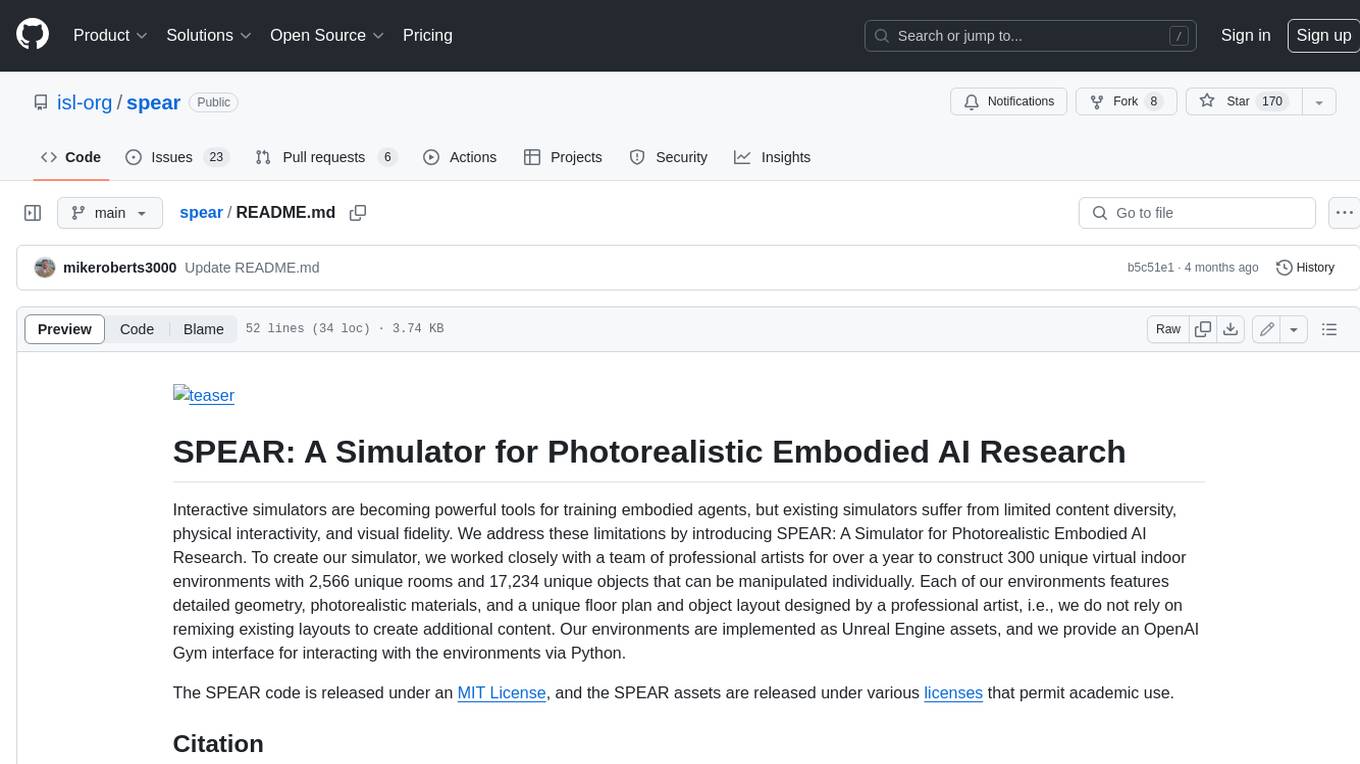
spear
SPEAR (Simulator for Photorealistic Embodied AI Research) is a powerful tool for training embodied agents. It features 300 unique virtual indoor environments with 2,566 unique rooms and 17,234 unique objects that can be manipulated individually. Each environment is designed by a professional artist and features detailed geometry, photorealistic materials, and a unique floor plan and object layout. SPEAR is implemented as Unreal Engine assets and provides an OpenAI Gym interface for interacting with the environments via Python.
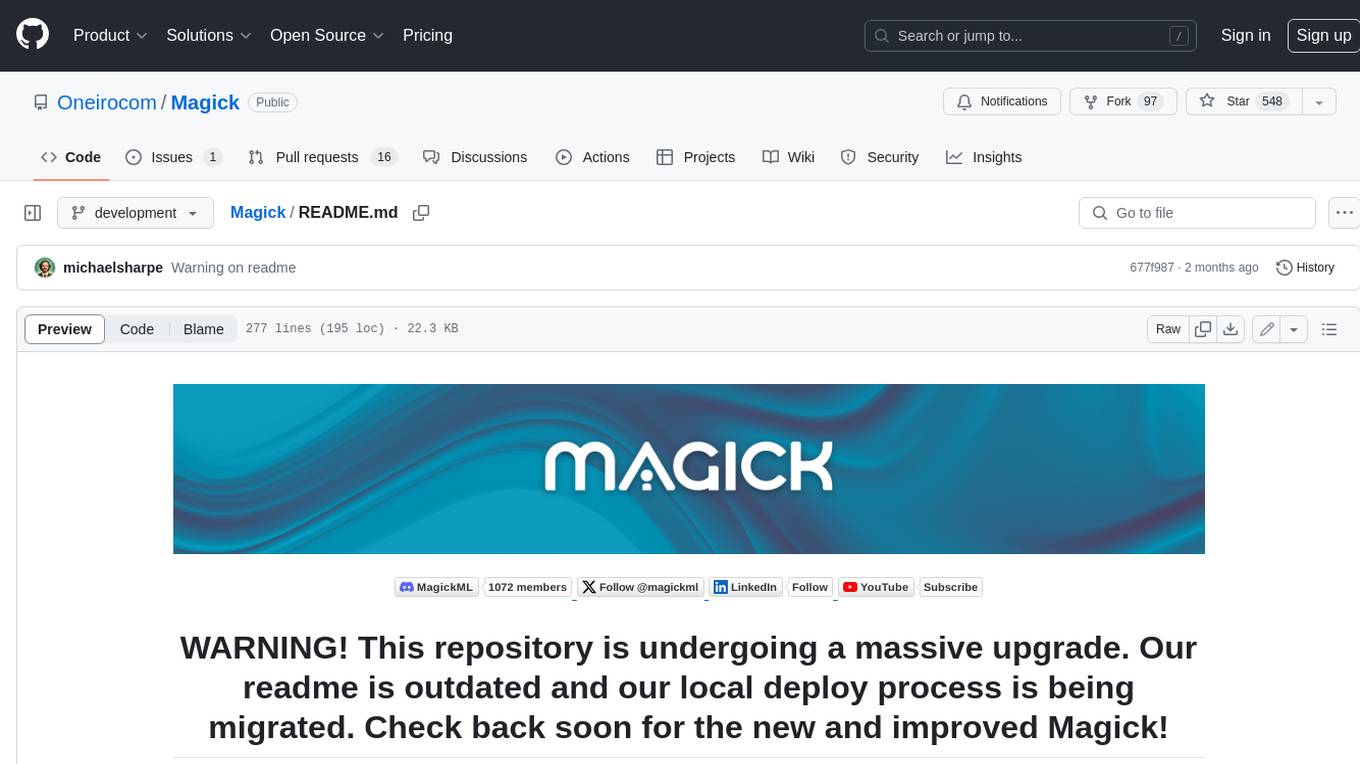
Magick
Magick is a groundbreaking visual AIDE (Artificial Intelligence Development Environment) for no-code data pipelines and multimodal agents. Magick can connect to other services and comes with nodes and templates well-suited for intelligent agents, chatbots, complex reasoning systems and realistic characters.