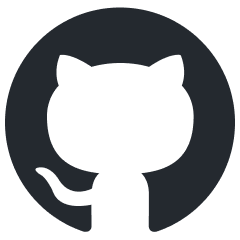
MooER
MooER: Open-sourced LLM for audio understanding trained on 80,000 hours of data
Stars: 124
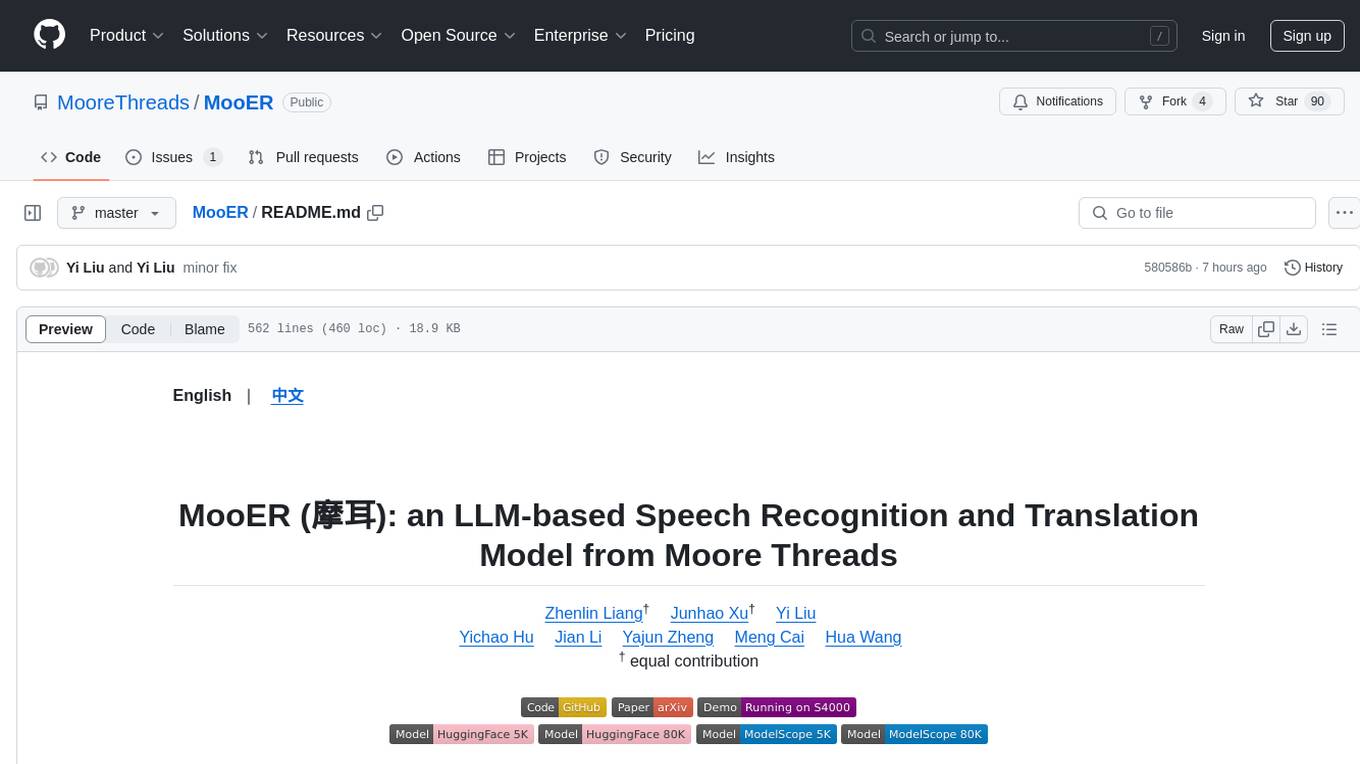
MooER (摩耳) is an LLM-based speech recognition and translation model developed by Moore Threads. It allows users to transcribe speech into text (ASR) and translate speech into other languages (AST) in an end-to-end manner. The model was trained using 5K hours of data and is now also available with an 80K hours version. MooER is the first LLM-based speech model trained and inferred using domestic GPUs. The repository includes pretrained models, inference code, and a Gradio demo for a better user experience.
README:
English  |   中文  
-
2024/09/03
: We have open-sourced the training and inference code for MooER! You can follow this tutorial to train your own audio understanding model and tasks or fine-tune based on our 80k hours model. -
2024/08/27
: We released MooER-80K-v2 which was trained using 80K hours of data. The performance of the new model can be found below. Currently, it only supports the speech recognition task. The speech translation and the multi-task models will be released soon. -
2024/08/09
: We released a Gradio demo running on Moore Threads S4000. -
2024/08/09
: We released the inference code and the pretrained speech recognition and speech translation (zh->en) models using 5000 hours of data. -
2024/08/09
: We release MooER v0.1 technical report on arXiv.
We introduce MooER (摩耳): an LLM-based speech recognition and translation model developed by Moore Threads. With the MooER framework, you can transcribe the speech into text (automatic speech recognition, ASR) and translate the speech into other languages (automatic speech translation, AST) in an LLM-based end-to-end manner. Some of the evaluation results of the MooER are presented in the subsequent section. More detailed experiments, along with our insights into model configurations, training strategies, etc, are provided in our technical report.
We proudly highlight that MooER is developed using Moore Threads S4000 GPUs. To the best of our knowledge, this is the first LLM-based speech model trained and inferred using entirely domestic GPUs.
[!Note] We are going to release the training code for MooER, as well as models trained with more data. Please stay tuned!
We present the training data and the evaluation results below. For more comprehensive information, please refer to our report.
We utilize 5,000 hours of speech data (MT5K) to train our basic MooER-5K model. The data sources include:
Dataset | Duration |
---|---|
aishell2 | 137h |
librispeech | 131h |
multi_cn | 100h |
wenetspeech | 1361h |
in-house data | 3274h |
Note that, data from the open-source datasets were randomly selected from the full training set. The in-house speech data, collected internally without transcription, were transcribed using a third-party ASR service.
Since all the above datasets were originally collected only for the speech recognition task, no translation labels are available. We leveraged a third-party machine translation service to generate pseudo-labels for translation. No data filtering techniques were applied.
At this moment, we are also developing a new model trained with 80,000 hours of speech data.
The performance of speech recognition is evaluated using word error rate (WER) and character error rate (CER).
Language | Testset | Paraformer-large | SenseVoice-small | Qwen-audio | Whisper-large-v3 | SeamlessM4T-v2 | MooER-5K | MooER-80K | MooER-80K-v2 |
---|---|---|---|---|---|---|---|---|---|
Chinese | aishell1 | 1.93 | 3.03 | 1.43 | 7.86 | 4.09 | 1.93 | 1.25 | 1.00 |
aishell2_ios | 2.85 | 3.79 | 3.57 | 5.38 | 4.81 | 3.17 | 2.67 | 2.62 | |
test_magicdata | 3.66 | 3.81 | 5.31 | 8.36 | 9.69 | 3.48 | 2.52 | 2.17 | |
test_thchs | 3.99 | 5.17 | 4.86 | 9.06 | 7.14 | 4.11 | 3.14 | 3.00 | |
fleurs cmn_dev | 5.56 | 6.39 | 10.54 | 4.54 | 7.12 | 5.81 | 5.23 | 5.15 | |
fleurs cmn_test | 6.92 | 7.36 | 11.07 | 5.24 | 7.66 | 6.77 | 6.18 | 6.14 | |
average | 4.15 | 4.93 | 6.13 | 6.74 | 6.75 | 4.21 | 3.50 | 3.35 | |
English | librispeech test_clean | 14.15 | 4.07 | 2.15 | 3.42 | 2.77 | 7.78 | 4.11 | 3.57 |
librispeech test_other | 22.99 | 8.26 | 4.68 | 5.62 | 5.25 | 15.25 | 9.99 | 9.09 | |
fleurs eng_dev | 24.93 | 12.92 | 22.53 | 11.63 | 11.36 | 18.89 | 13.32 | 13.12 | |
fleurs eng_test | 26.81 | 13.41 | 22.51 | 12.57 | 11.82 | 20.41 | 14.97 | 14.74 | |
gigaspeech dev | 24.23 | 19.44 | 12.96 | 19.18 | 28.01 | 23.46 | 16.92 | 17.34 | |
gigaspeech test | 23.07 | 16.65 | 13.26 | 22.34 | 28.65 | 22.09 | 16.64 | 16.97 | |
average | 22.70 | 12.46 | 13.02 | 12.46 | 14.64 | 17.98 | 12.66 | 12.47 |
For speech translation, the performance is evaluated using BLEU score.
Testset | Speech-LLaMA | Whisper-large-v3 | Qwen-audio | Qwen2-audio | SeamlessM4T-v2 | MooER-5K | MooER-5K-MTL |
---|---|---|---|---|---|---|---|
CoVoST1 zh2en | - | 13.5 | 13.5 | - | 25.3 | - | 30.2 |
CoVoST2 zh2en | 12.3 | 12.2 | 15.7 | 24.4 | 22.2 | 23.4 | 25.2 |
CCMT2019 dev | - | 15.9 | 12.0 | - | 14.8 | - | 19.6 |
Currently, only Linux is supported. Ensure that git
and python
are installed on your system. We recommend Python version >=3.8
. It is highly recommanded to install conda
to create a virtual environment.
For efficient LLM inference, GPUs should be used. For Moore Threads S3000/S4000 users, please install MUSA toolkit rc2.1.0. A docker image is also available for S4000 users. If you use other GPUs, install your own drivers/toolkits (e.g. cuda).
Build the environment with the following steps:
git clone https://github.com/MooreThreads/MooER
cd MooER
# (optional) create env using conda
conda create -n mooer python=3.8
conda activate mooer
# install the dependencies
apt update
apt install ffmpeg sox
pip install -r requirements.txt
Docker image usage for Moore Threads S4000 users is provided:
sudo docker run -it \
--privileged \
--name=torch_musa_release \
--env MTHREADS_VISIBLE_DEVICES=all \
-p 10010:10010 \
--shm-size 80g \
--ulimit memlock=-1 \
mtspeech/mooer:v1.0-rc2.1.0-v1.1.0-qy2 \
/bin/bash
# If you are nvidia user, you can try this image with cuda 11.7
sudo docker run -it \
--privileged \
--gpus all \
-p 10010:10010 \
--shm-size 80g \
--ulimit memlock=-1 \
mtspeech/mooer:v1.0-cuda11.7-cudnn8 \
/bin/bash
First, download the pretrained models from ModelScope or HuggingFace.
# use modelscope
git lfs clone https://modelscope.cn/models/MooreThreadsSpeech/MooER-MTL-5K
# use huggingface
git lfs clone https://huggingface.co/mtspeech/MooER-MTL-5K
Put the downloaded files in pretrained_models
cp MooER-MTL-5K/* pretrained_models
Then, download Qwen2-7B-Instruct
by:
# use modelscope
git lfs clone https://modelscope.cn/models/qwen/qwen2-7b-instruct
# use huggingface
git lfs clone https://huggingface.co/Qwen/Qwen2-7B-Instruct
Put the downloaded files into pretrained_models/Qwen2-7B-Instruct
.
Finally, all these files should be orgnized as follows. The md5sum's are also provided.
./pretrained_models/
|-- paraformer_encoder
| |-- am.mvn # dc1dbdeeb8961f012161cfce31eaacaf
| `-- paraformer-encoder.pth # 2ef398e80f9f3e87860df0451e82caa9
|-- asr
| |-- adapter_project.pt # 2462122fb1655c97d3396f8de238c7ed
| `-- lora_weights
| |-- README.md
| |-- adapter_config.json # 8a76aab1f830be138db491fe361661e6
| `-- adapter_model.bin # 0fe7a36de164ebe1fc27500bc06c8811
|-- ast
| |-- adapter_project.pt # 65c05305382af0b28964ac3d65121667
| `-- lora_weights
| |-- README.md
| |-- adapter_config.json # 8a76aab1f830be138db491fe361661e6
| `-- adapter_model.bin # 12c51badbe57298070f51902abf94cd4
|-- asr_ast_mtl
| |-- adapter_project.pt # 83195d39d299f3b39d1d7ddebce02ef6
| `-- lora_weights
| |-- README.md
| |-- adapter_config.json # 8a76aab1f830be138db491fe361661e6
| `-- adapter_model.bin # a0f730e6ddd3231322b008e2339ed579
|-- Qwen2-7B-Instruct
| |-- model-00001-of-00004.safetensors # d29bf5c5f667257e9098e3ff4eec4a02
| |-- model-00002-of-00004.safetensors # 75d33ab77aba9e9bd856f3674facbd17
| |-- model-00003-of-00004.safetensors # bc941028b7343428a9eb0514eee580a3
| |-- model-00004-of-00004.safetensors # 07eddec240f1d81a91ca13eb51eb7af3
| |-- model.safetensors.index.json
| |-- config.json # 8d67a66d57d35dc7a907f73303486f4e
| |-- configuration.json # 040f5895a7c8ae7cf58c622e3fcc1ba5
| |-- generation_config.json # 5949a57de5fd3148ac75a187c8daec7e
| |-- merges.txt # e78882c2e224a75fa8180ec610bae243
| |-- tokenizer.json # 1c74fd33061313fafc6e2561d1ac3164
| |-- tokenizer_config.json # 5c05592e1adbcf63503fadfe429fb4cc
| |-- vocab.json # 613b8e4a622c4a2c90e9e1245fc540d6
| |-- LICENSE
| `-- README.md
|-- README.md
`-- configuration.json
The new MooER-80K-v2 is released. You can download the new model and update pretrained_models
.
# use modelscope
git lfs clone https://modelscope.cn/models/MooreThreadsSpeech/MooER-MTL-80K
# use huggingface
git lfs clone https://huggingface.co/mtspeech/MooER-MTL-80K
The md5sum's of the updated files are provided.
./pretrained_models/
`-- asr
|-- adapter_project.pt # af9022e2853f9785cab49017a18de82c
`-- lora_weights
|-- README.md
|-- adapter_config.json # ad3e3bfe9447b808b9cc16233ffacaaf
`-- adapter_model.bin # 3c22b9895859b01efe49b017e8ed6ec7
We have open-sourced the training and inference code for MooER! You can follow this tutorial to train your own audio understanding model or fine-tune based on 80k hours model.
You can simply run the inference example to get the idea.
# set environment variables
export PYTHONIOENCODING=UTF-8
export LC_ALL=C
export PYTHONPATH=$PWD/src:$PYTHONPATH
# do inference
python inference.py
The script runs a multi-task model that will output the speech recognition and translation results simultaneously. If it runs successfully, you will get the ASR and AST results from the terminal.
You can specify your own audio files and change the model settings.
# use your own audio file
python inference.py --wav_path /path/to/your_audio_file
# an scp file is also supported. The format of each line is: "uttid wav_path":
# test1 my_test_audio1.wav
# test2 my_test_audio2.wav
# ...
python inference.py --wav_scp /path/to/your_wav_scp
# change to an ASR model (only transcription)
python inference.py --task asr \
--cmvn_path pretrained_models/paraformer_encoder/am.mvn \
--encoder_path pretrained_models/paraformer_encoder/paraformer-encoder.pth \
--llm_path pretrained_models/Qwen2-7B-Instruct \
--adapter_path pretrained_models/asr/adapter_project.pt \
--lora_dir pretrained_models/asr/lora_weights \
--wav_path /path/to/your_audio_file
# change to an AST model (only translation)
python inference.py --task ast \
--cmvn_path pretrained_models/paraformer_encoder/am.mvn \
--encoder_path pretrained_models/paraformer_encoder/paraformer-encoder.pth \
--llm_path pretrained_models/Qwen2-7B-Instruct \
--adapter_path pretrained_models/ast/adapter_project.pt \
--lora_dir pretrained_models/ast/lora_weights \
--wav_path /path/to/your_audio_file
# Note: set `--task ast` if you want to use the asr/ast multitask model
# show all the parameters
python inference.py -h
We recommend to use an audio file shorter than 30s. The text in the audio should be less than 500 characters. It is also suggested that you convert the audio to a 16kHz 16bit mono WAV format before processing it (using ffmpeg
or sox
).
We provide a Gradio interface for a better experience. To use it, run the following commands:
# set the environment variables
export PYTHONPATH=$PWD/src:$PYTHONPATH
# Run the ASR/AST multitask model
python demo/app.py
# Run the ASR-only model
python demo/app.py \
--task asr \
--adapter_path pretrained_models/asr/adapter_project.pt \
--lora_dir pretrained_models/asr/lora_weights
# Run the AST-only model
python demo/app.py \
--task ast \
--adapter_path pretrained_models/ast/adapter_project.pt \
--lora_dir pretrained_models/ast/lora_weights
You can specify --server_port
, --share
, --server_name
as needed.
Due to the lack of an HTTPS certificate, your access is limited to HTTP, for which modern browsers block the microphone access. As a workaround, you can manually grant access. For instance, in Chrome, navigate to chrome://flags/#unsafely-treat-insecure-origin-as-secure
and add the target address to the whitelist. For other browsers, please google for a similar workaround.
In the demo, using the streaming mode will yield faster results. However, please note that the beam size is restricted to be 1 in the streaming mode, which may slightly degrade the performance.
🤔 No experience about how to run Gradio?
💻 Don't have a machine to run the demo?
⌛ Don't have time to install the dependencies?
☕ Just take a coffee and click here to try our online demo. It is running on a Moore Threads S4000 GPU server!
- [x] Technical report
- [x] Inference code and pretrained ASR/AST models using 5k hours of data
- [x] Pretrained ASR model using 80k hours of data
- [x] Traning code for MooER
- [ ] Pretrained AST and multi-task models using 80k hours of data
- [ ] LLM-based timbre-preserving Speech-to-speech translation (S2ST)
Please see the LICENSE.
We borrowed the speech encoder from FunASR.
The LLM code was borrowed from Qwen2.
Our training and inference codes are adapted from SLAM-LLM and Wenet.
We also got inspiration from other open-source repositories like whisper and SeamlessM4T. We would like to thank all the authors and contributors for their innovative ideas and codes.
If you find MooER useful for your research, please 🌟 this repo and cite our work using the following BibTeX:
@article{liang2024mooer,
title = {MooER: LLM-based Speech Recognition and Translation Models from Moore Threads},
author = {Zhenlin Liang, Junhao Xu, Yi Liu, Yichao Hu, Jian Li, Yajun Zheng, Meng Cai, Hua Wang},
journal = {arXiv preprint arXiv:2408.05101},
year = {2024}
}
If you encouter any problems, feel free to create an issue.
Moore Threads Website: https://www.mthreads.com/
For Tasks:
Click tags to check more tools for each tasksFor Jobs:
Alternative AI tools for MooER
Similar Open Source Tools
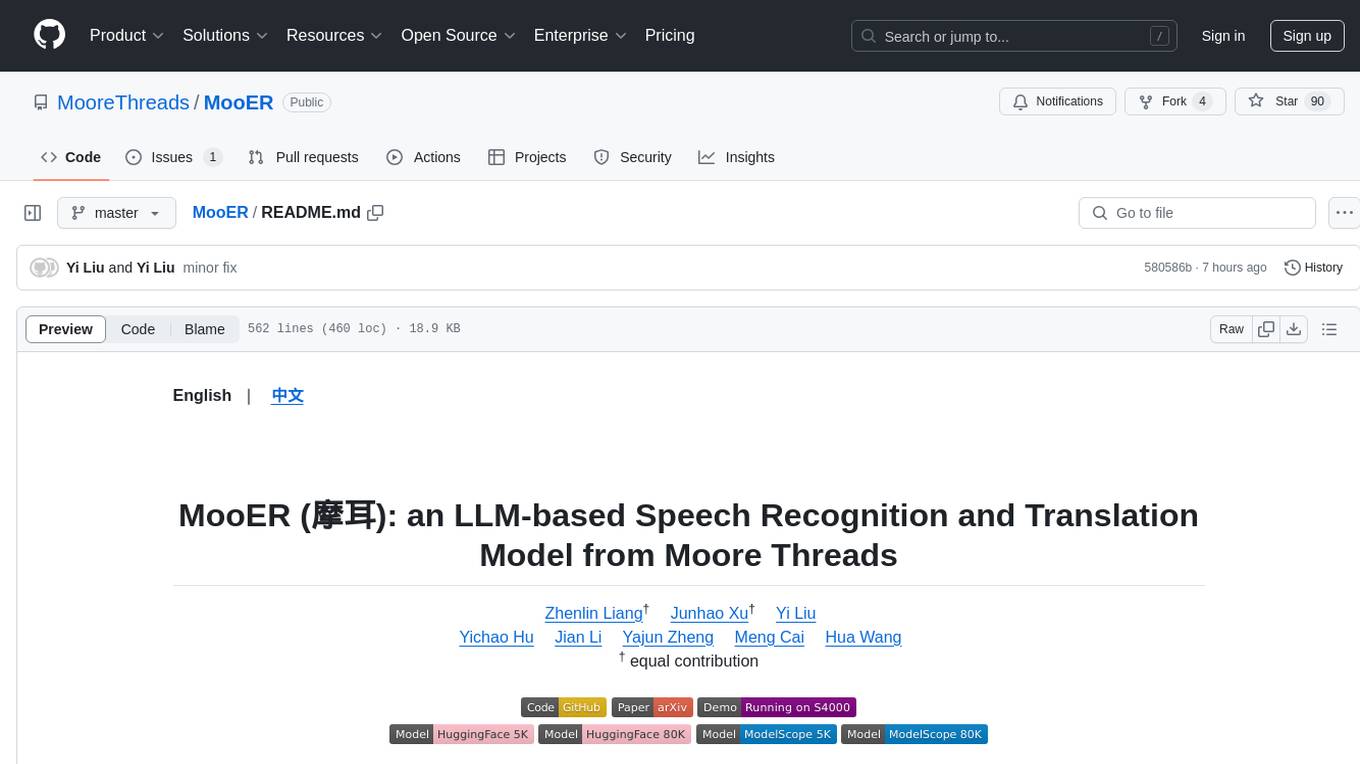
MooER
MooER (摩耳) is an LLM-based speech recognition and translation model developed by Moore Threads. It allows users to transcribe speech into text (ASR) and translate speech into other languages (AST) in an end-to-end manner. The model was trained using 5K hours of data and is now also available with an 80K hours version. MooER is the first LLM-based speech model trained and inferred using domestic GPUs. The repository includes pretrained models, inference code, and a Gradio demo for a better user experience.
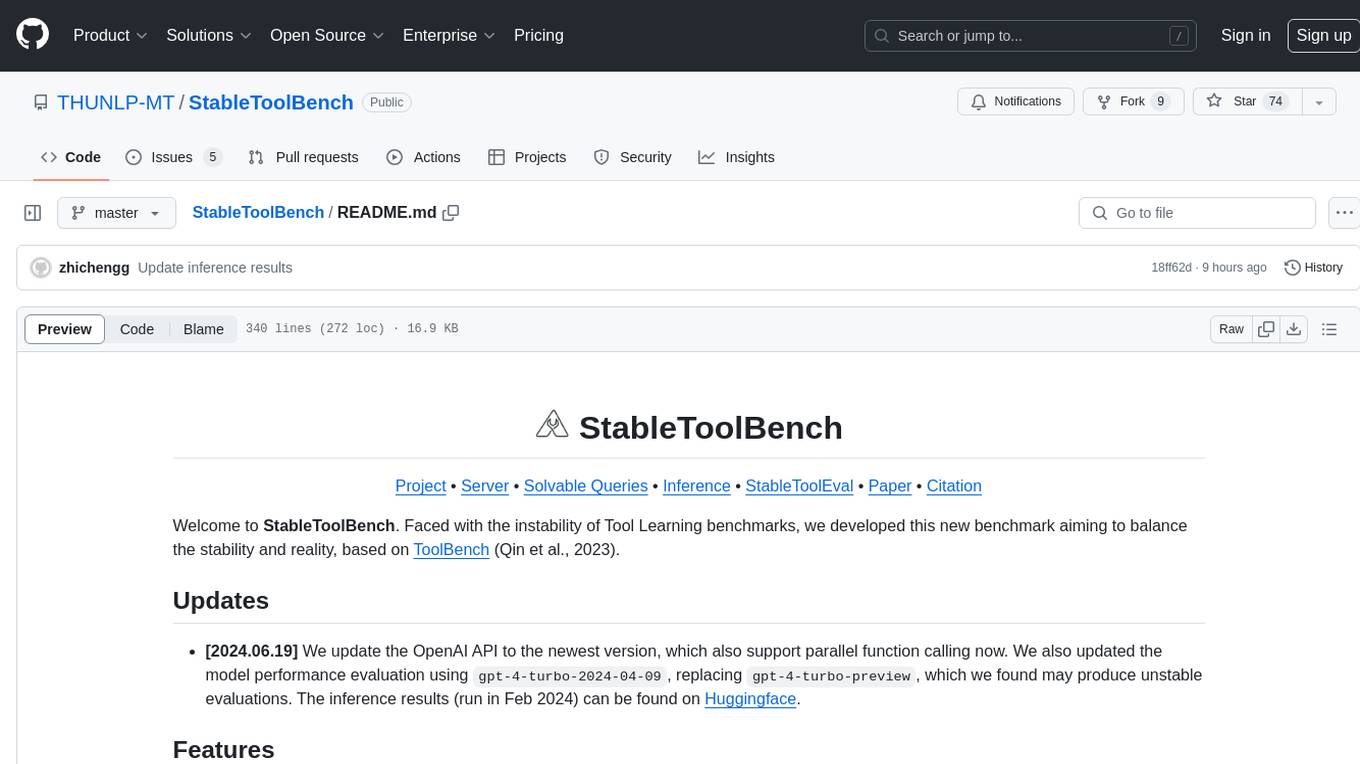
StableToolBench
StableToolBench is a new benchmark developed to address the instability of Tool Learning benchmarks. It aims to balance stability and reality by introducing features like Virtual API System, Solvable Queries, and Stable Evaluation System. The benchmark ensures consistency through a caching system and API simulators, filters queries based on solvability using LLMs, and evaluates model performance using GPT-4 with metrics like Solvable Pass Rate and Solvable Win Rate.
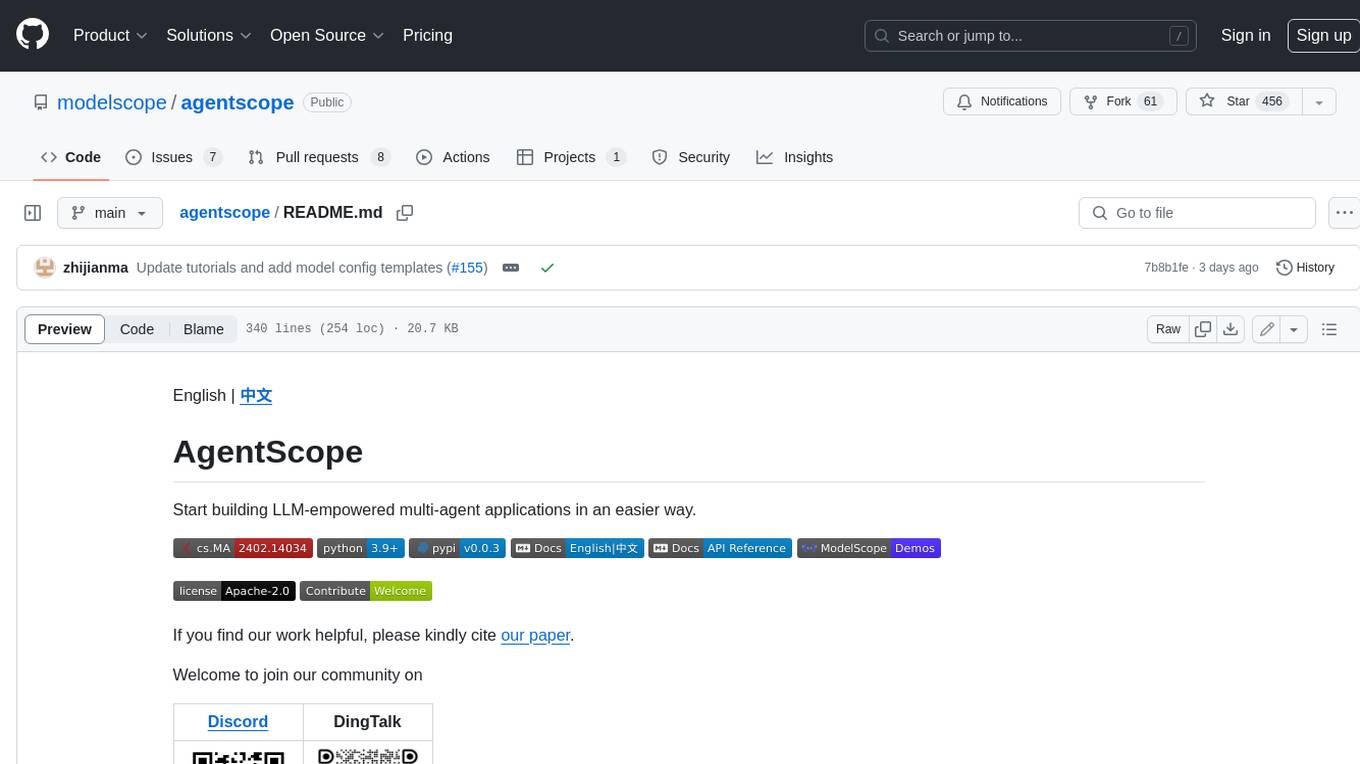
agentscope
AgentScope is a multi-agent platform designed to empower developers to build multi-agent applications with large-scale models. It features three high-level capabilities: Easy-to-Use, High Robustness, and Actor-Based Distribution. AgentScope provides a list of `ModelWrapper` to support both local model services and third-party model APIs, including OpenAI API, DashScope API, Gemini API, and ollama. It also enables developers to rapidly deploy local model services using libraries such as ollama (CPU inference), Flask + Transformers, Flask + ModelScope, FastChat, and vllm. AgentScope supports various services, including Web Search, Data Query, Retrieval, Code Execution, File Operation, and Text Processing. Example applications include Conversation, Game, and Distribution. AgentScope is released under Apache License 2.0 and welcomes contributions.
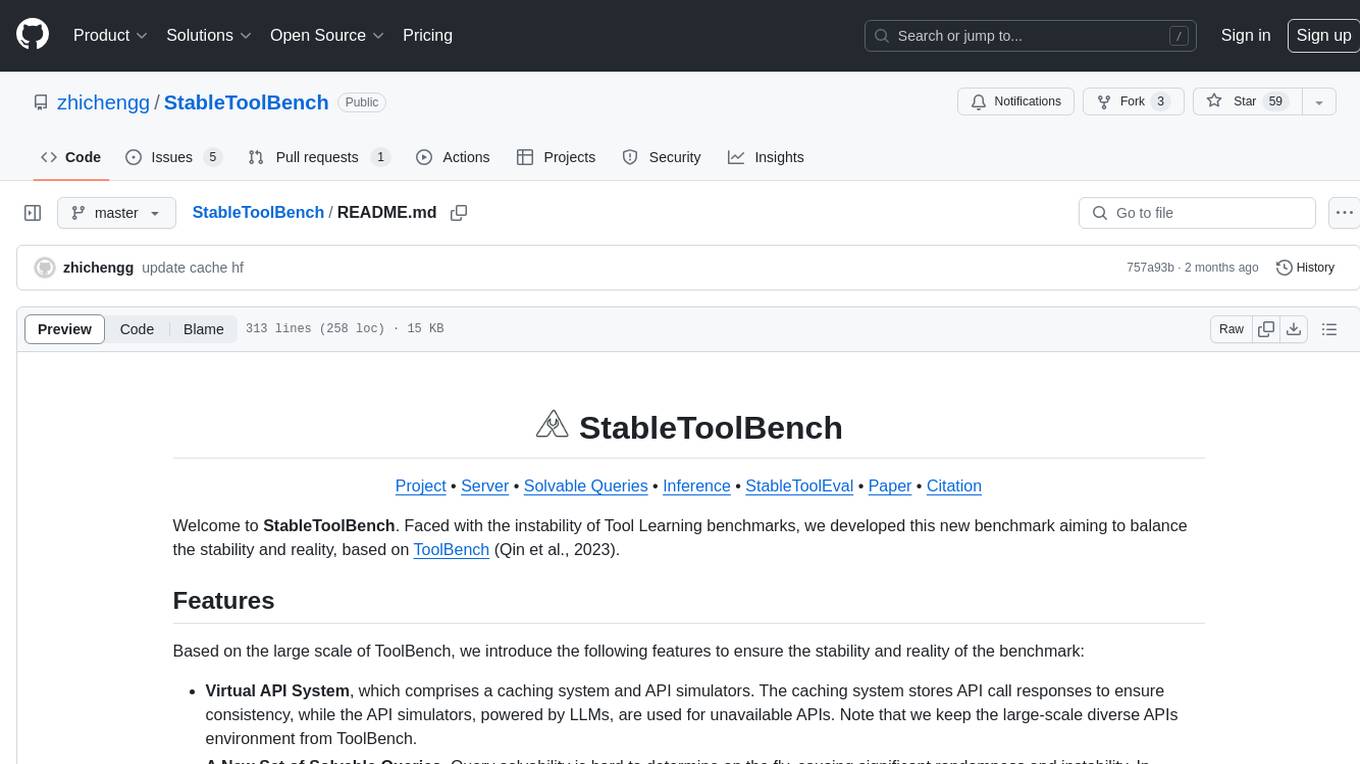
StableToolBench
StableToolBench is a new benchmark developed to address the instability of Tool Learning benchmarks. It aims to balance stability and reality by introducing features such as a Virtual API System with caching and API simulators, a new set of solvable queries determined by LLMs, and a Stable Evaluation System using GPT-4. The Virtual API Server can be set up either by building from source or using a prebuilt Docker image. Users can test the server using provided scripts and evaluate models with Solvable Pass Rate and Solvable Win Rate metrics. The tool also includes model experiments results comparing different models' performance.
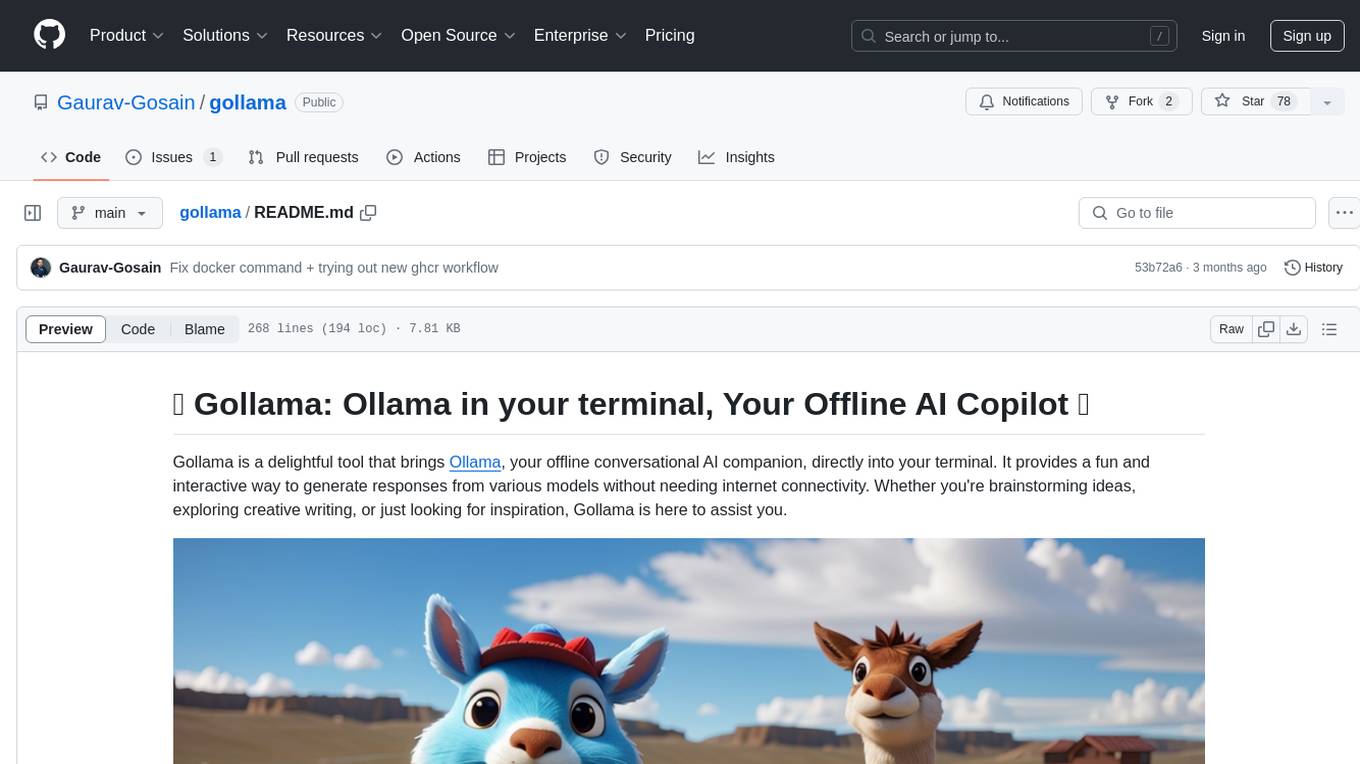
gollama
Gollama is a delightful tool that brings Ollama, your offline conversational AI companion, directly into your terminal. It provides a fun and interactive way to generate responses from various models without needing internet connectivity. Whether you're brainstorming ideas, exploring creative writing, or just looking for inspiration, Gollama is here to assist you. The tool offers an interactive interface, customizable prompts, multiple models selection, and visual feedback to enhance user experience. It can be installed via different methods like downloading the latest release, using Go, running with Docker, or building from source. Users can interact with Gollama through various options like specifying a custom base URL, prompt, model, and enabling raw output mode. The tool supports different modes like interactive, piped, CLI with image, and TUI with image. Gollama relies on third-party packages like bubbletea, glamour, huh, and lipgloss. The roadmap includes implementing piped mode, support for extracting codeblocks, copying responses/codeblocks to clipboard, GitHub Actions for automated releases, and downloading models directly from Ollama using the rest API. Contributions are welcome, and the project is licensed under the MIT License.
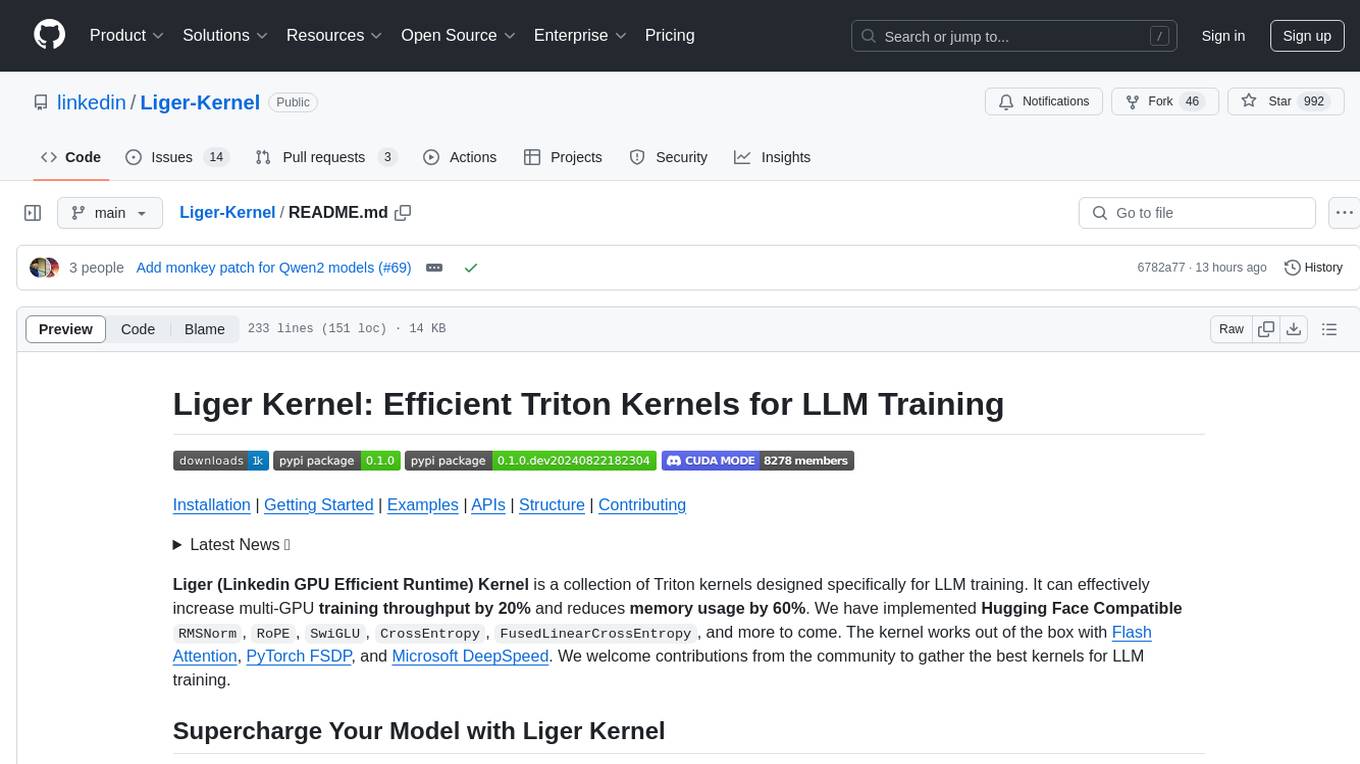
Liger-Kernel
Liger Kernel is a collection of Triton kernels designed for LLM training, increasing training throughput by 20% and reducing memory usage by 60%. It includes Hugging Face Compatible modules like RMSNorm, RoPE, SwiGLU, CrossEntropy, and FusedLinearCrossEntropy. The tool works with Flash Attention, PyTorch FSDP, and Microsoft DeepSpeed, aiming to enhance model efficiency and performance for researchers, ML practitioners, and curious novices.
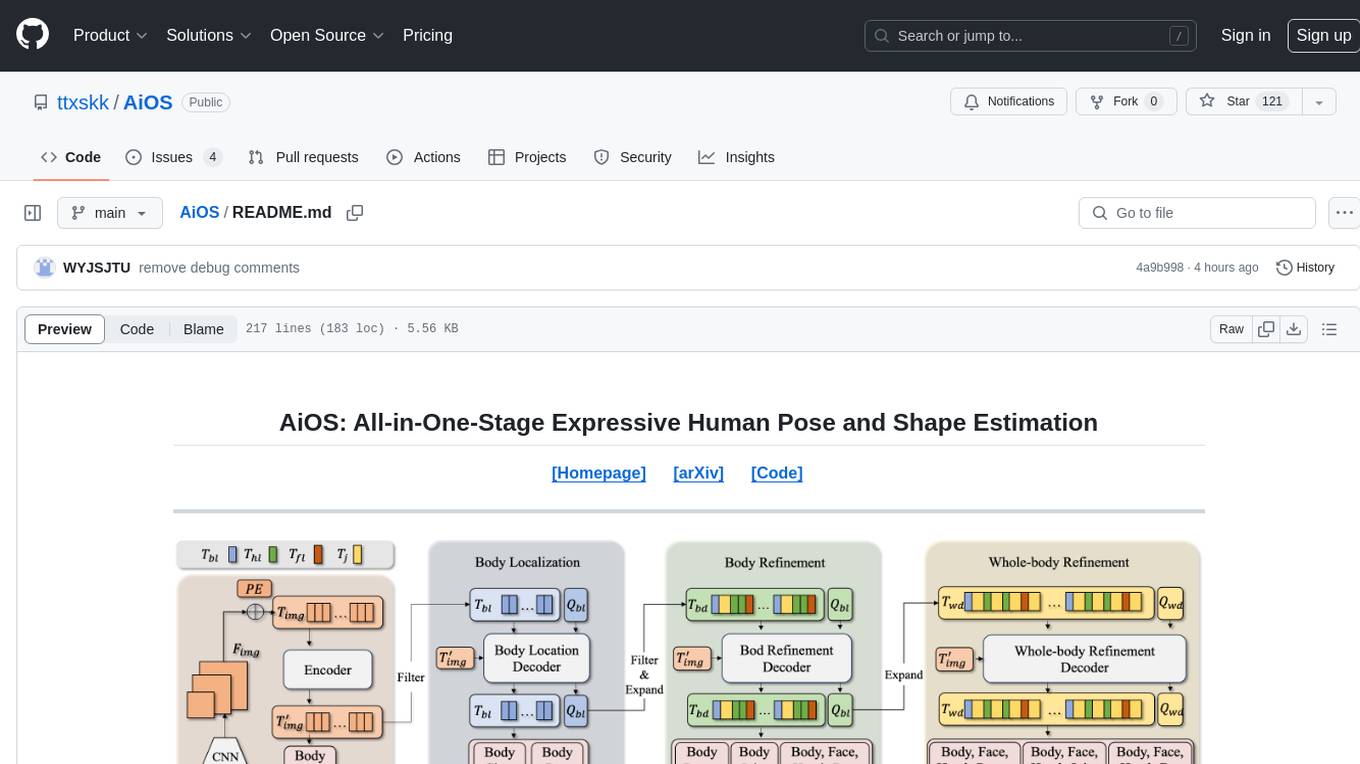
AiOS
AiOS is a tool for human pose and shape estimation, performing human localization and SMPL-X estimation in a progressive manner. It consists of body localization, body refinement, and whole-body refinement stages. Users can download datasets for evaluation, SMPL-X body models, and AiOS checkpoint. Installation involves creating a conda virtual environment, installing PyTorch, torchvision, Pytorch3D, MMCV, and other dependencies. Inference requires placing the video for inference and pretrained models in specific directories. Test results are provided for NMVE, NMJE, MVE, and MPJPE on datasets like BEDLAM and AGORA. Users can run scripts for AGORA validation, AGORA test leaderboard, and BEDLAM leaderboard. The tool acknowledges codes from MMHuman3D, ED-Pose, and SMPLer-X.
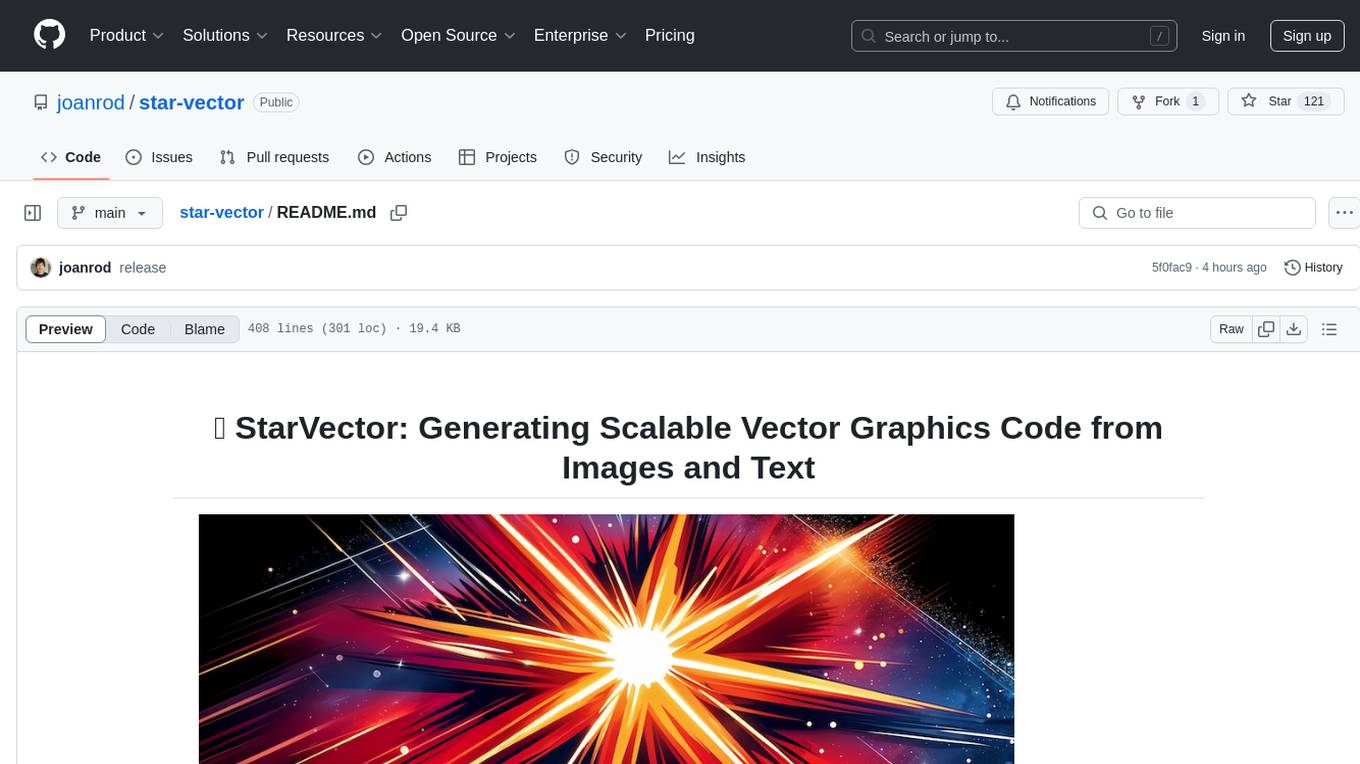
star-vector
StarVector is a multimodal vision-language model for Scalable Vector Graphics (SVG) generation. It can be used to perform image2SVG and text2SVG generation. StarVector works directly in the SVG code space, leveraging visual understanding to apply accurate SVG primitives. It achieves state-of-the-art performance in producing compact and semantically rich SVGs. The tool provides Hugging Face model checkpoints for image2SVG vectorization, with models like StarVector-8B and StarVector-1B. It also offers datasets like SVG-Stack, SVG-Fonts, SVG-Icons, SVG-Emoji, and SVG-Diagrams for evaluation. StarVector can be trained using Deepspeed or FSDP for tasks like Image2SVG and Text2SVG generation. The tool provides a demo with options for HuggingFace generation or VLLM backend for faster generation speed.
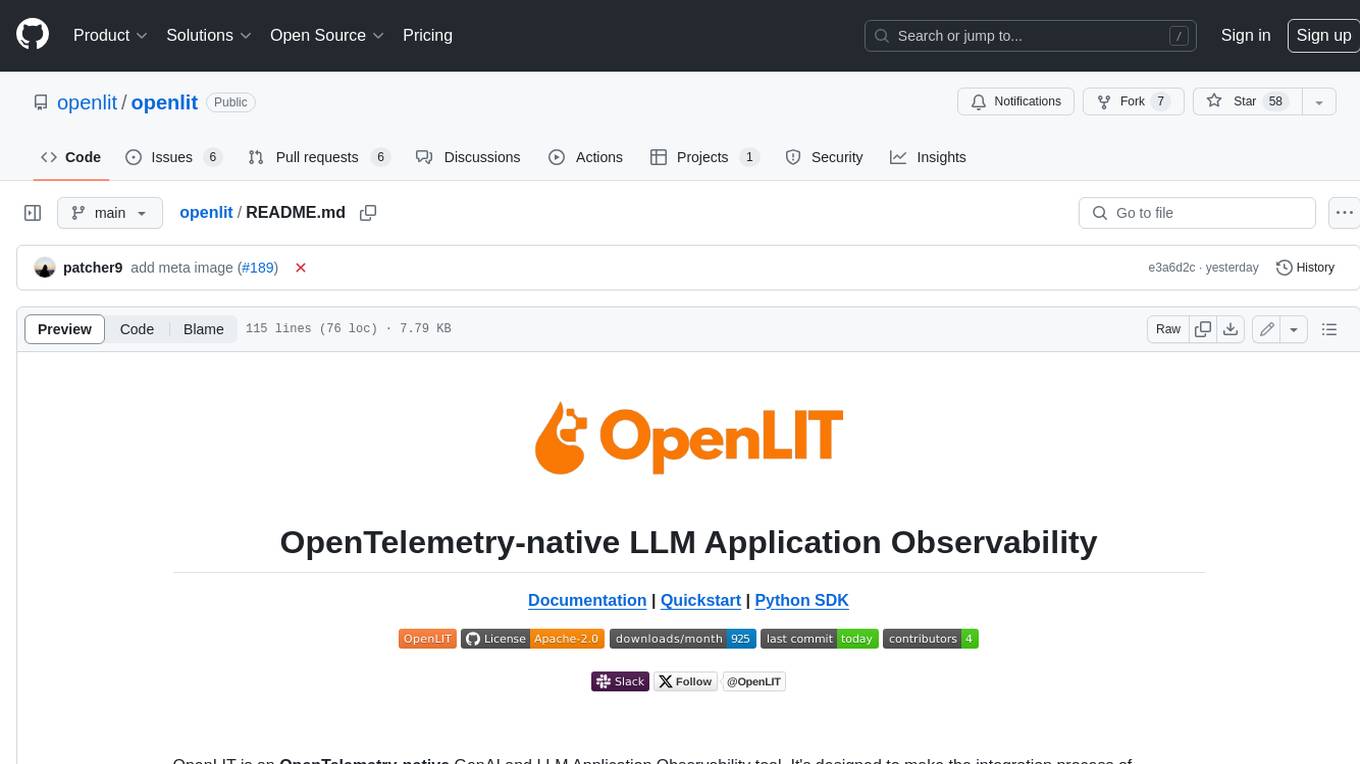
openlit
OpenLIT is an OpenTelemetry-native GenAI and LLM Application Observability tool. It's designed to make the integration process of observability into GenAI projects as easy as pie – literally, with just **a single line of code**. Whether you're working with popular LLM Libraries such as OpenAI and HuggingFace or leveraging vector databases like ChromaDB, OpenLIT ensures your applications are monitored seamlessly, providing critical insights to improve performance and reliability.
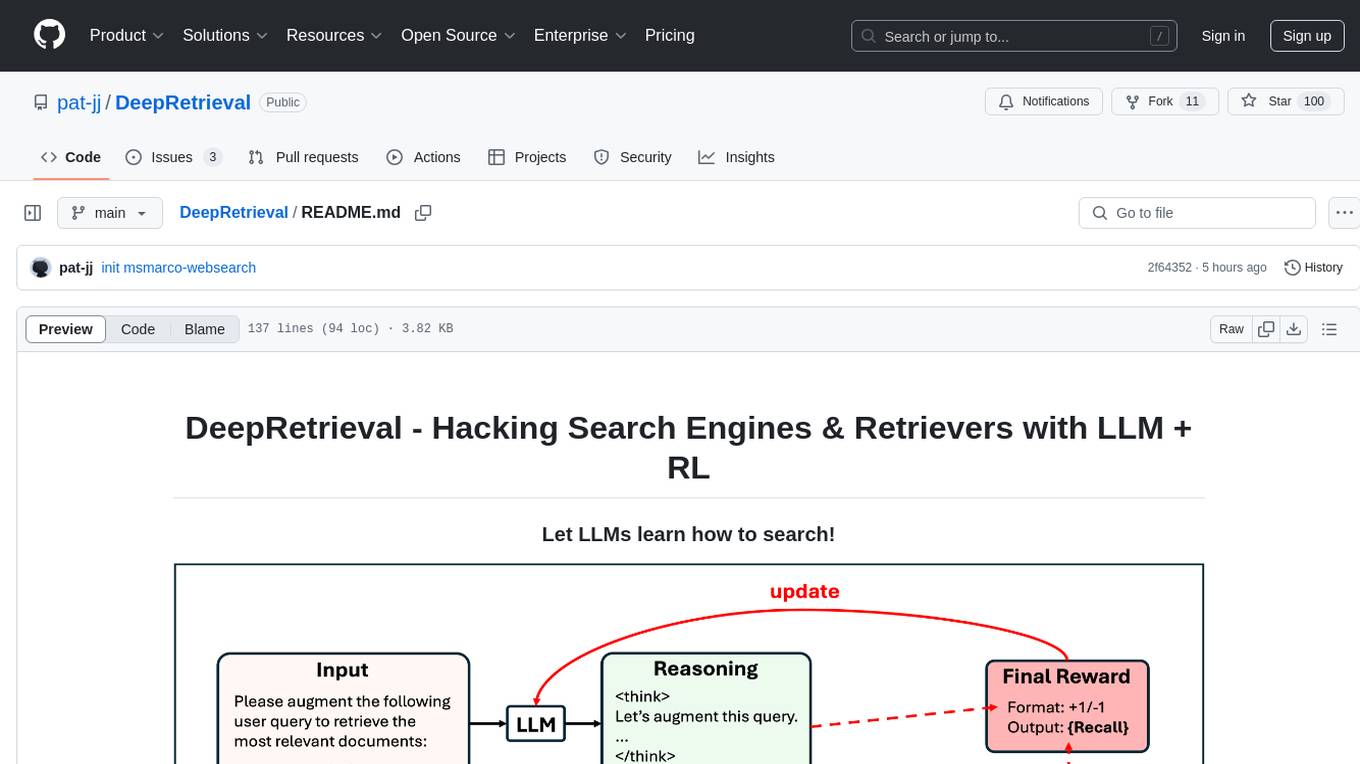
DeepRetrieval
DeepRetrieval is a tool designed to enhance search engines and retrievers using Large Language Models (LLMs) and Reinforcement Learning (RL). It allows LLMs to learn how to search effectively by integrating with search engine APIs and customizing reward functions. The tool provides functionalities for data preparation, training, evaluation, and monitoring search performance. DeepRetrieval aims to improve information retrieval tasks by leveraging advanced AI techniques.
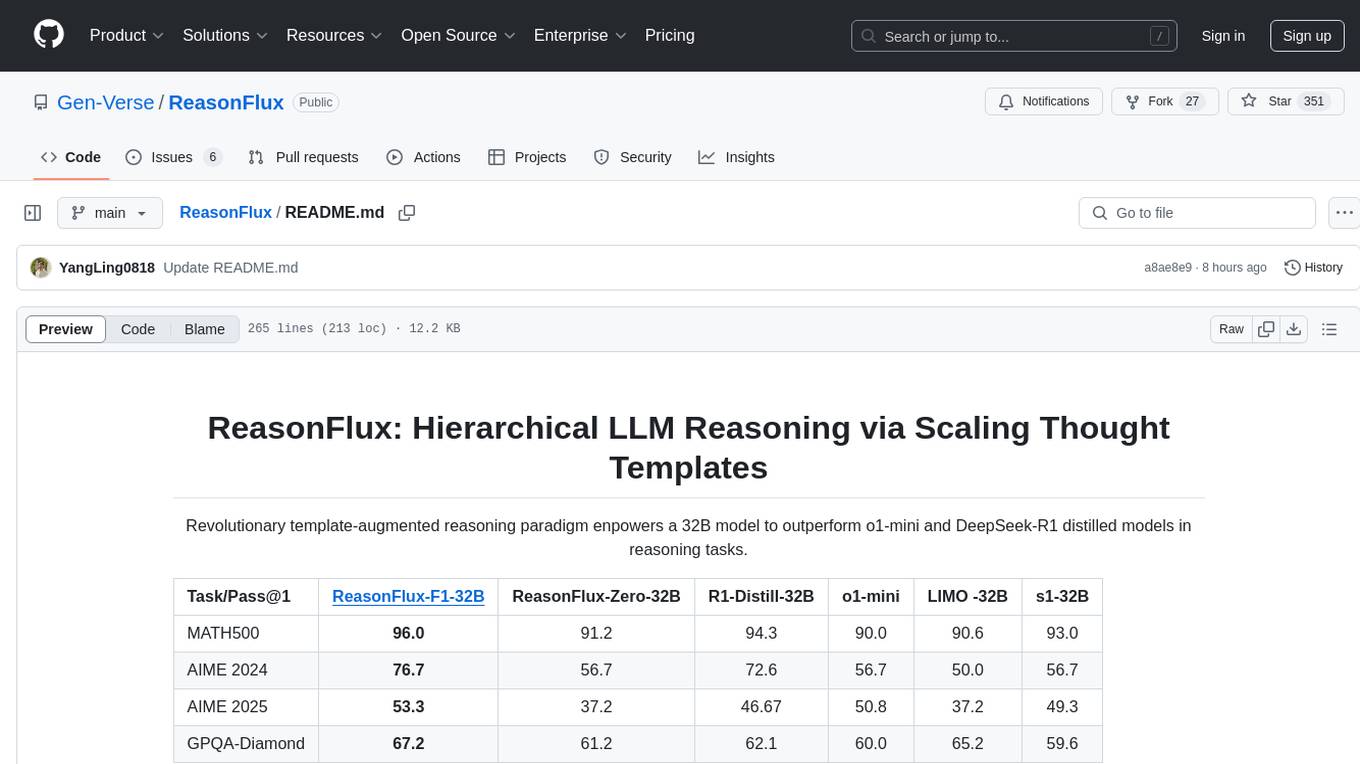
ReasonFlux
ReasonFlux is a revolutionary template-augmented reasoning paradigm that empowers a 32B model to outperform other models in reasoning tasks. The repository provides official resources for the paper 'ReasonFlux: Hierarchical LLM Reasoning via Scaling Thought Templates', including the latest released model ReasonFlux-F1-32B. It includes updates, dataset links, model zoo, getting started guide, training instructions, evaluation details, inference examples, performance comparisons, reasoning examples, preliminary work references, and citation information.
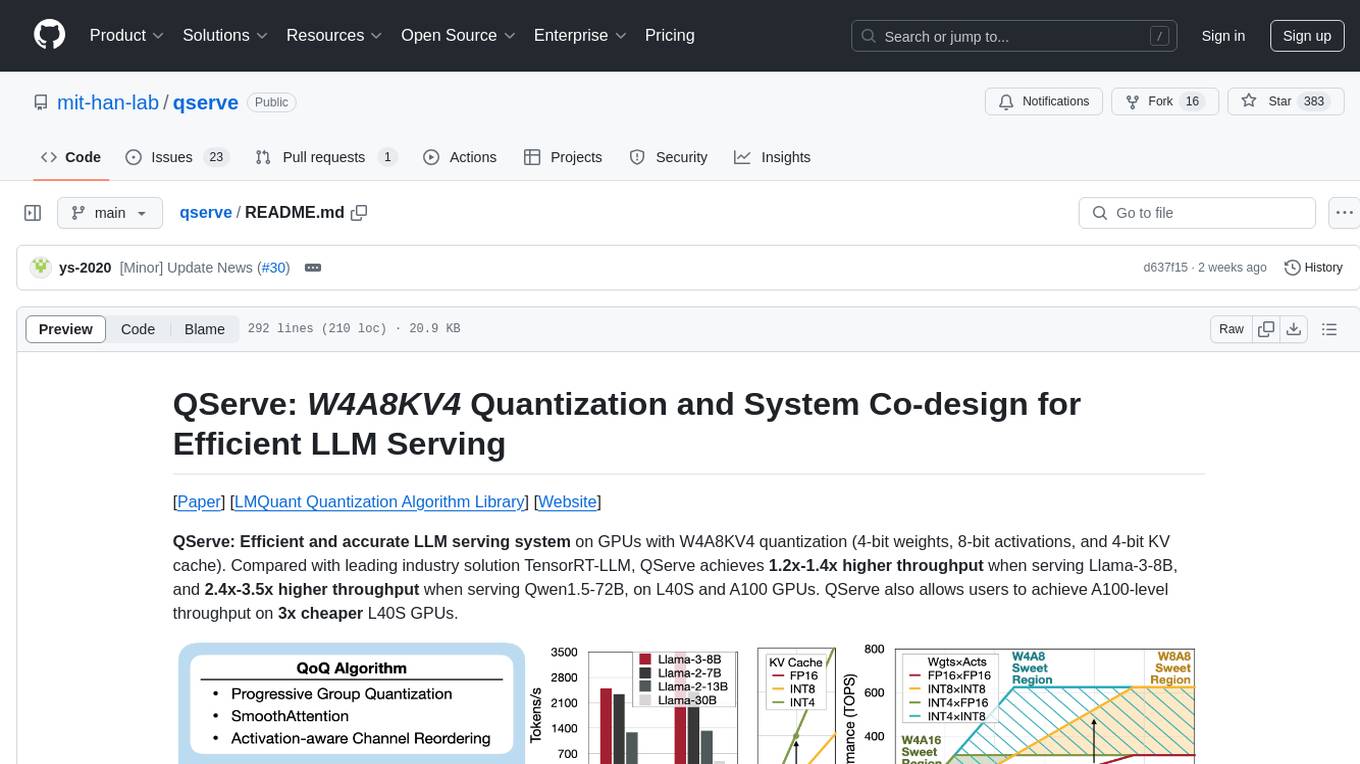
qserve
QServe is a serving system designed for efficient and accurate Large Language Models (LLM) on GPUs with W4A8KV4 quantization. It achieves higher throughput compared to leading industry solutions, allowing users to achieve A100-level throughput on cheaper L40S GPUs. The system introduces the QoQ quantization algorithm with 4-bit weight, 8-bit activation, and 4-bit KV cache, addressing runtime overhead challenges. QServe improves serving throughput for various LLM models by implementing compute-aware weight reordering, register-level parallelism, and fused attention memory-bound techniques.
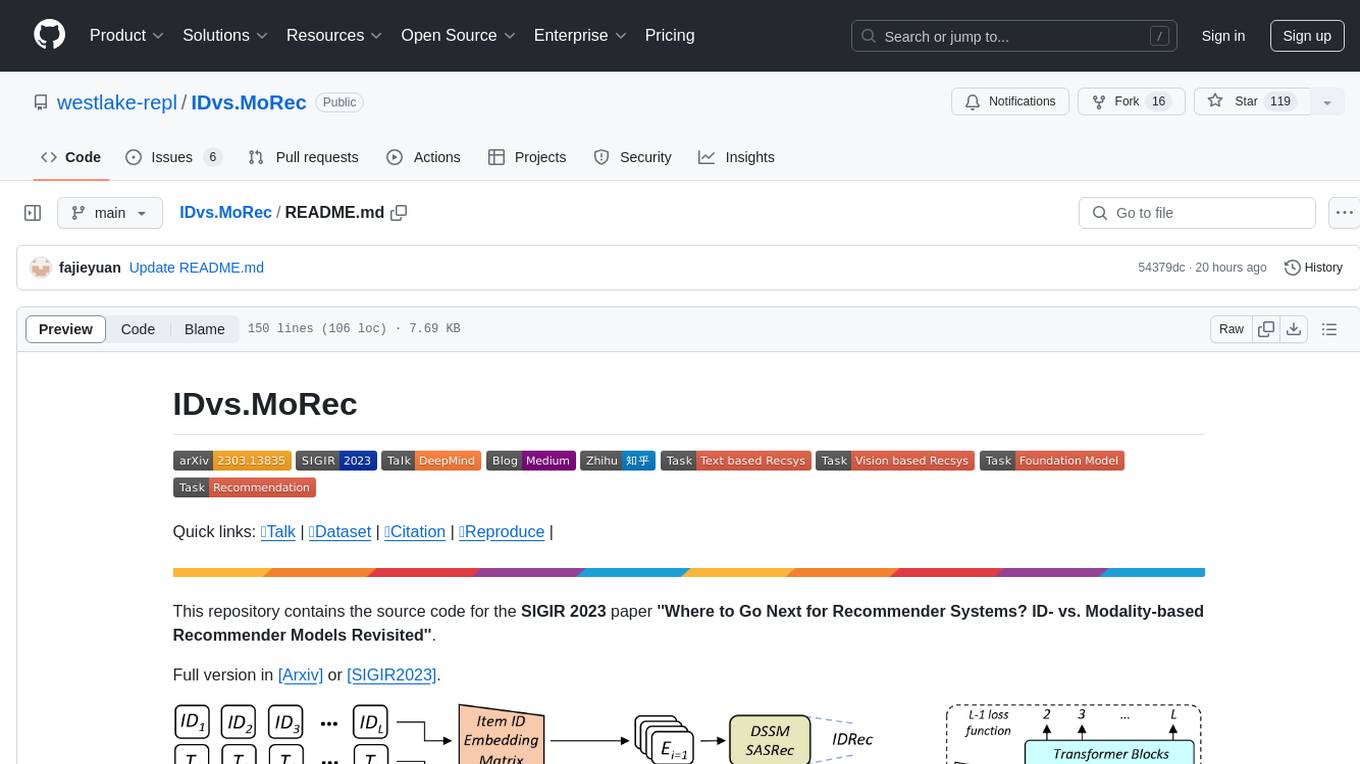
IDvs.MoRec
This repository contains the source code for the SIGIR 2023 paper 'Where to Go Next for Recommender Systems? ID- vs. Modality-based Recommender Models Revisited'. It provides resources for evaluating foundation, transferable, multi-modal, and LLM recommendation models, along with datasets, pre-trained models, and training strategies for IDRec and MoRec using in-batch debiased cross-entropy loss. The repository also offers large-scale datasets, code for SASRec with in-batch debias cross-entropy loss, and information on joining the lab for research opportunities.
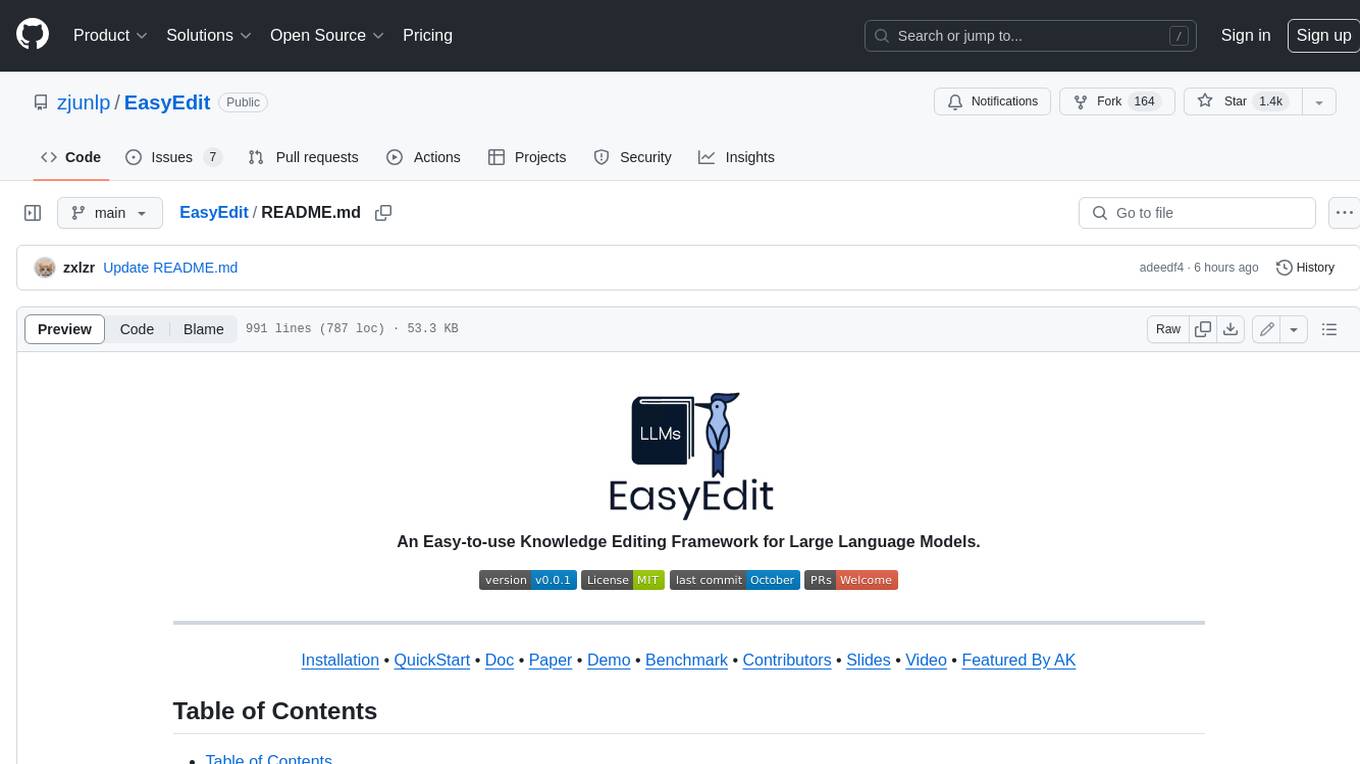
EasyEdit
EasyEdit is a Python package for edit Large Language Models (LLM) like `GPT-J`, `Llama`, `GPT-NEO`, `GPT2`, `T5`(support models from **1B** to **65B**), the objective of which is to alter the behavior of LLMs efficiently within a specific domain without negatively impacting performance across other inputs. It is designed to be easy to use and easy to extend.
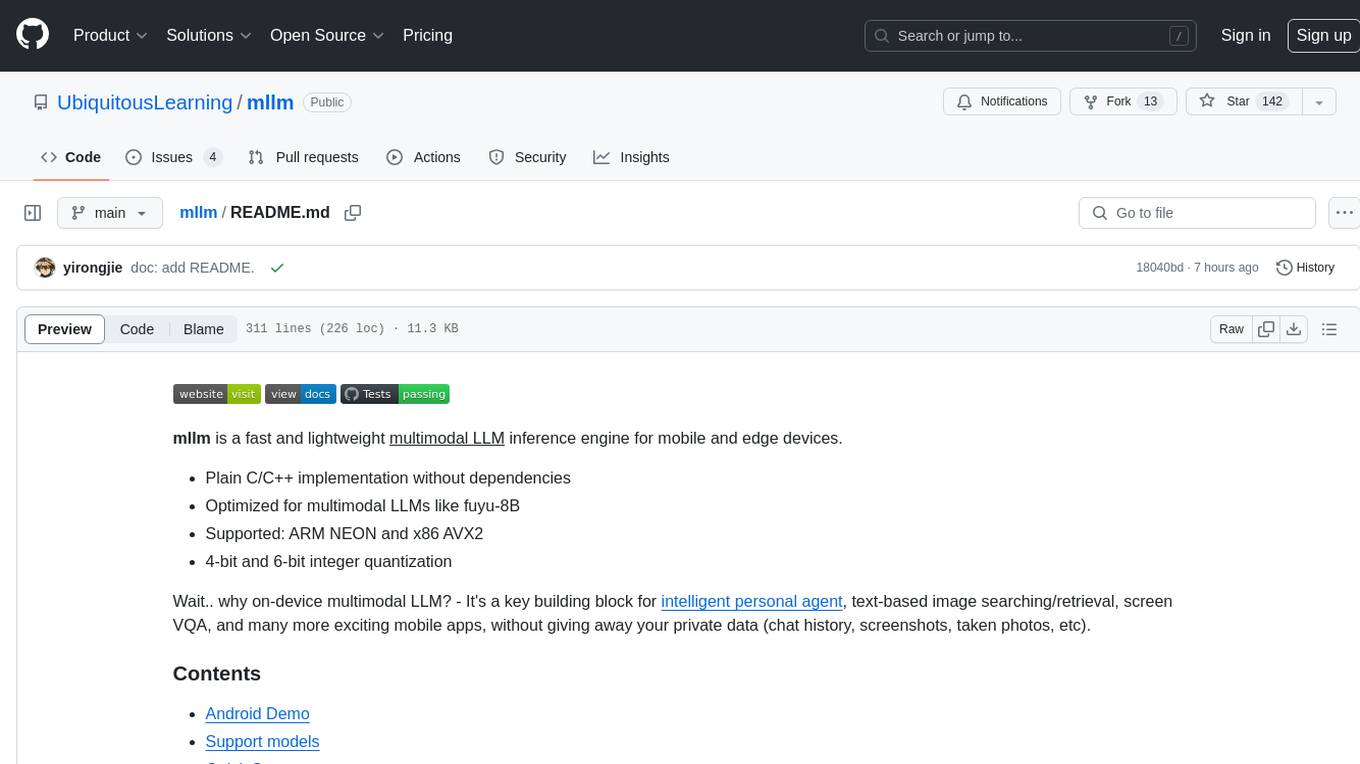
mllm
mllm is a fast and lightweight multimodal LLM inference engine for mobile and edge devices. It is a Plain C/C++ implementation without dependencies, optimized for multimodal LLMs like fuyu-8B, and supports ARM NEON and x86 AVX2. The engine offers 4-bit and 6-bit integer quantization, making it suitable for intelligent personal agents, text-based image searching/retrieval, screen VQA, and various mobile applications without compromising user privacy.
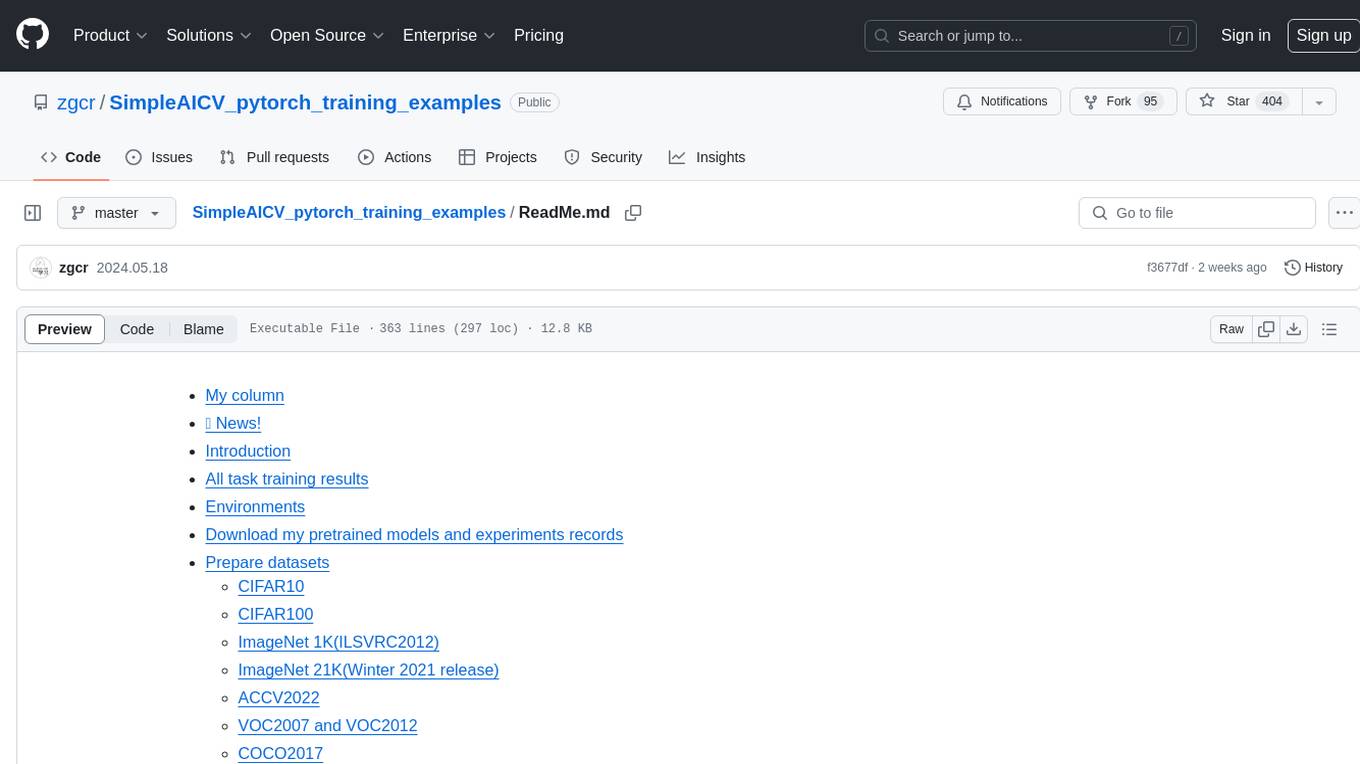
SimpleAICV_pytorch_training_examples
SimpleAICV_pytorch_training_examples is a repository that provides simple training and testing examples for various computer vision tasks such as image classification, object detection, semantic segmentation, instance segmentation, knowledge distillation, contrastive learning, masked image modeling, OCR text detection, OCR text recognition, human matting, salient object detection, interactive segmentation, image inpainting, and diffusion model tasks. The repository includes support for multiple datasets and networks, along with instructions on how to prepare datasets, train and test models, and use gradio demos. It also offers pretrained models and experiment records for download from huggingface or Baidu-Netdisk. The repository requires specific environments and package installations to run effectively.
For similar tasks
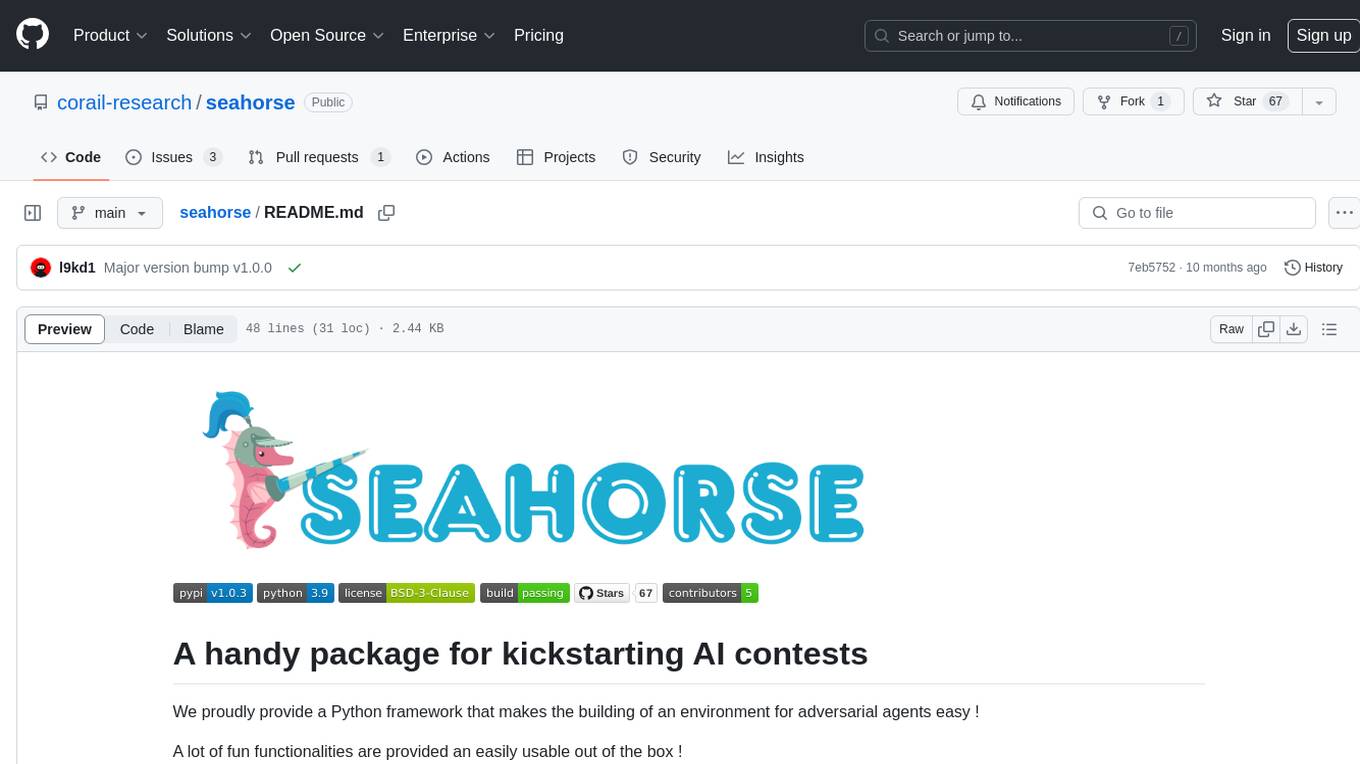
seahorse
A handy package for kickstarting AI contests. This Python framework simplifies the creation of an environment for adversarial agents, offering various functionalities for game setup, playing against remote agents, data generation, and contest organization. The package is user-friendly and provides easy-to-use features out of the box. Developed by an enthusiastic team of M.Sc candidates at Polytechnique Montréal, 'seahorse' is distributed under the 3-Clause BSD License.
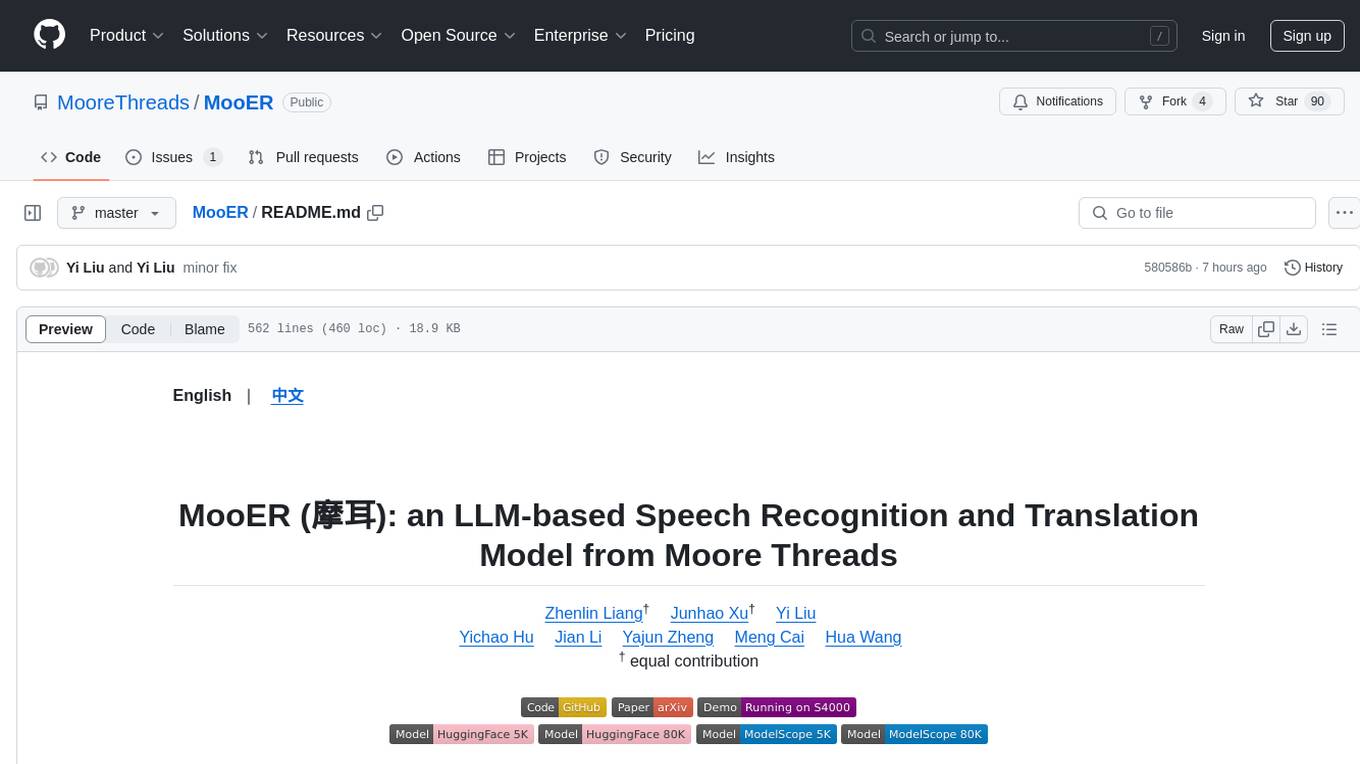
MooER
MooER (摩耳) is an LLM-based speech recognition and translation model developed by Moore Threads. It allows users to transcribe speech into text (ASR) and translate speech into other languages (AST) in an end-to-end manner. The model was trained using 5K hours of data and is now also available with an 80K hours version. MooER is the first LLM-based speech model trained and inferred using domestic GPUs. The repository includes pretrained models, inference code, and a Gradio demo for a better user experience.
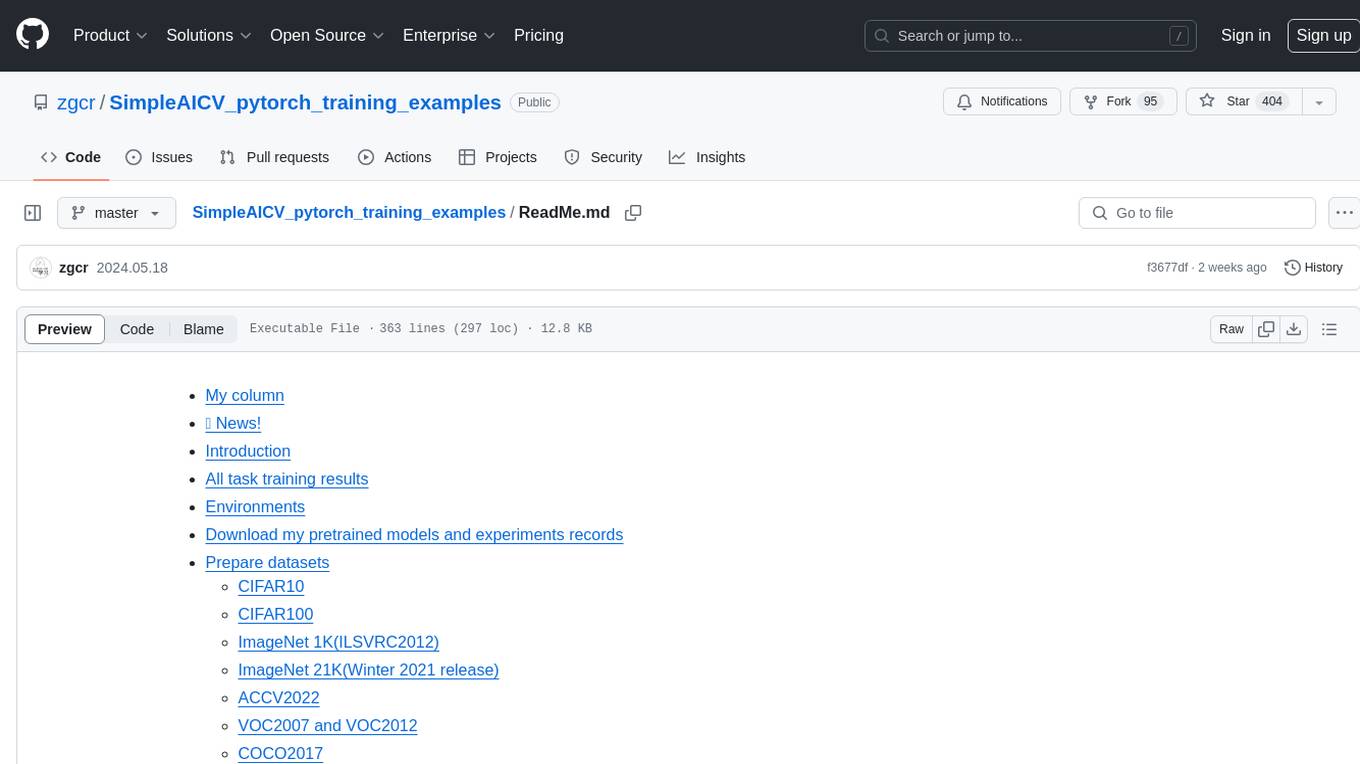
SimpleAICV_pytorch_training_examples
SimpleAICV_pytorch_training_examples is a repository that provides simple training and testing examples for various computer vision tasks such as image classification, object detection, semantic segmentation, instance segmentation, knowledge distillation, contrastive learning, masked image modeling, OCR text detection, OCR text recognition, human matting, salient object detection, interactive segmentation, image inpainting, and diffusion model tasks. The repository includes support for multiple datasets and networks, along with instructions on how to prepare datasets, train and test models, and use gradio demos. It also offers pretrained models and experiment records for download from huggingface or Baidu-Netdisk. The repository requires specific environments and package installations to run effectively.
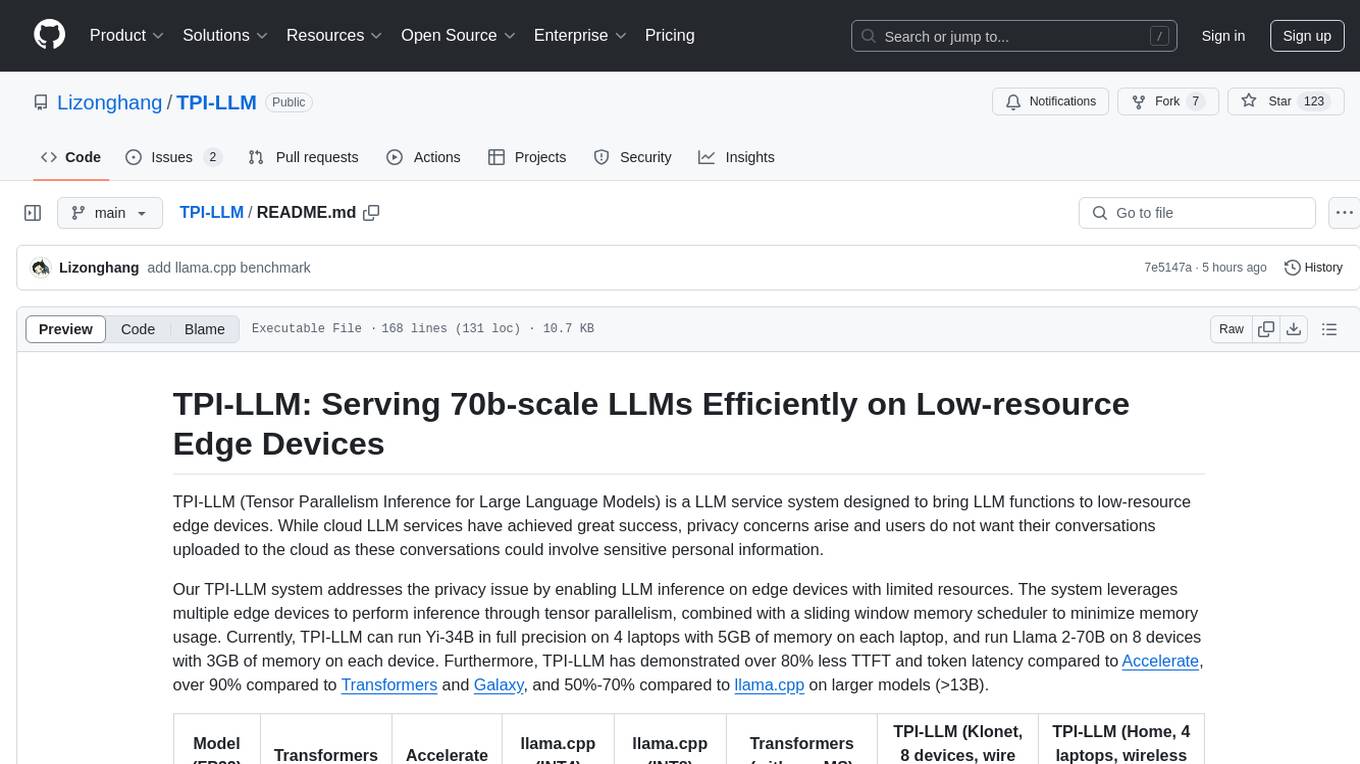
TPI-LLM
TPI-LLM (Tensor Parallelism Inference for Large Language Models) is a system designed to bring LLM functions to low-resource edge devices, addressing privacy concerns by enabling LLM inference on edge devices with limited resources. It leverages multiple edge devices for inference through tensor parallelism and a sliding window memory scheduler to minimize memory usage. TPI-LLM demonstrates significant improvements in TTFT and token latency compared to other models, and plans to support infinitely large models with low token latency in the future.
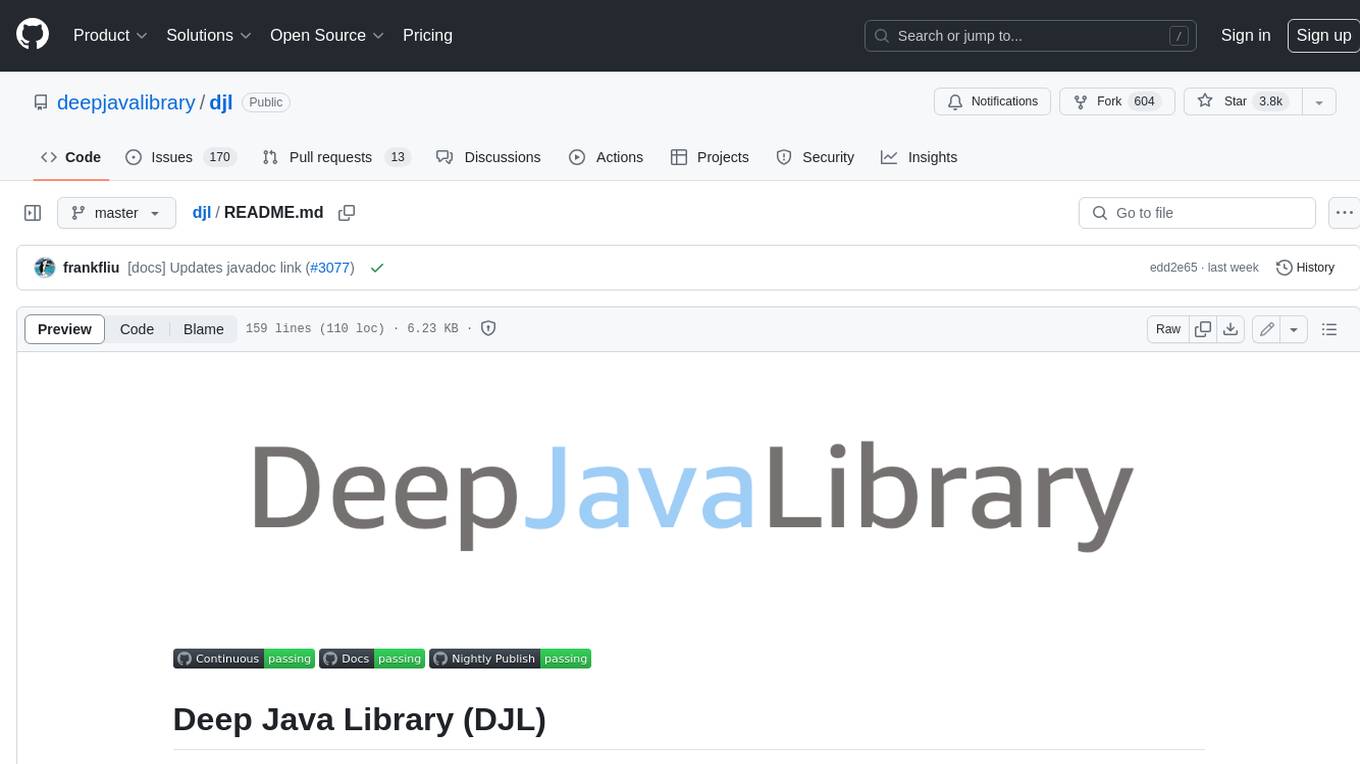
djl
Deep Java Library (DJL) is an open-source, high-level, engine-agnostic Java framework for deep learning. It is designed to be easy to get started with and simple to use for Java developers. DJL provides a native Java development experience and allows users to integrate machine learning and deep learning models with their Java applications. The framework is deep learning engine agnostic, enabling users to switch engines at any point for optimal performance. DJL's ergonomic API interface guides users with best practices to accomplish deep learning tasks, such as running inference and training neural networks.
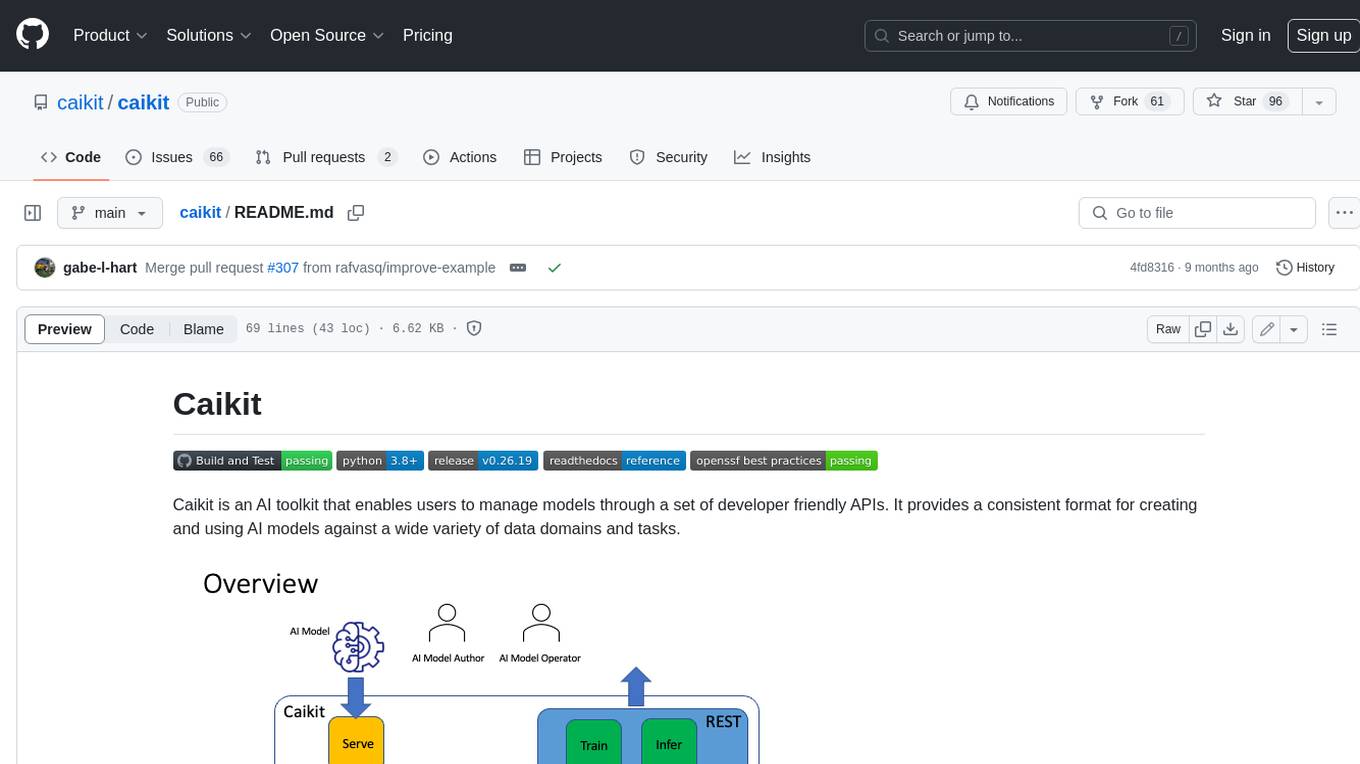
caikit
Caikit is an AI toolkit that enables users to manage models through a set of developer friendly APIs. It provides a consistent format for creating and using AI models against a wide variety of data domains and tasks.
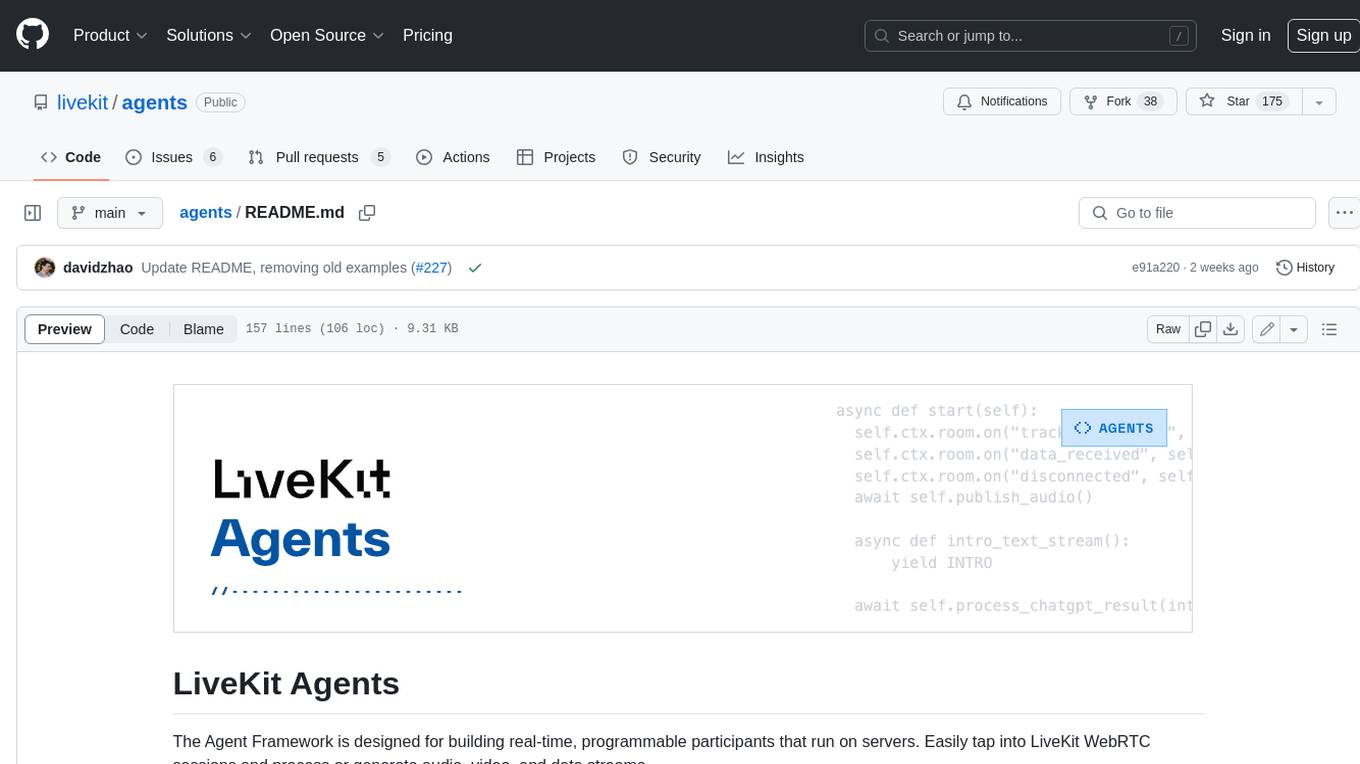
agents
The LiveKit Agent Framework is designed for building real-time, programmable participants that run on servers. Easily tap into LiveKit WebRTC sessions and process or generate audio, video, and data streams. The framework includes plugins for common workflows, such as voice activity detection and speech-to-text. Agents integrates seamlessly with LiveKit server, offloading job queuing and scheduling responsibilities to it. This eliminates the need for additional queuing infrastructure. Agent code developed on your local machine can scale to support thousands of concurrent sessions when deployed to a server in production.
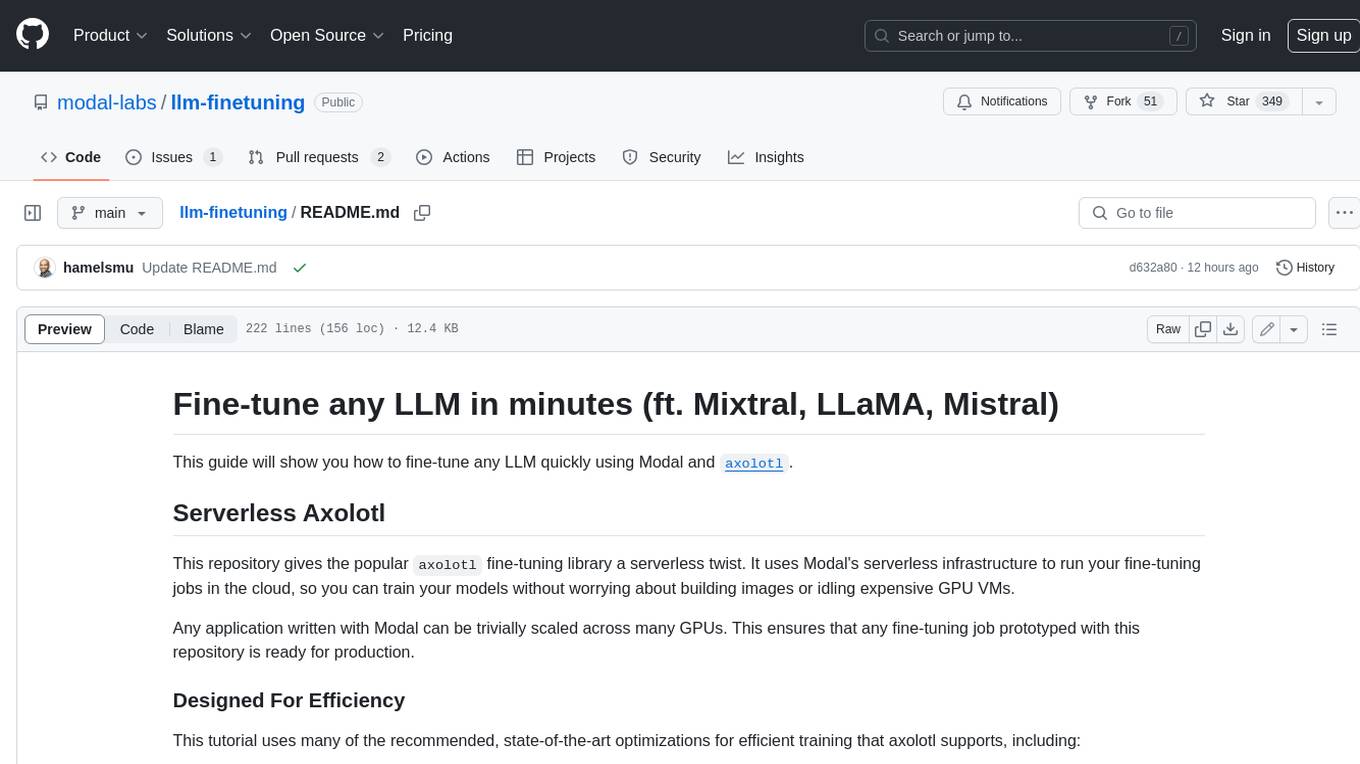
llm-finetuning
llm-finetuning is a repository that provides a serverless twist to the popular axolotl fine-tuning library using Modal's serverless infrastructure. It allows users to quickly fine-tune any LLM model with state-of-the-art optimizations like Deepspeed ZeRO, LoRA adapters, Flash attention, and Gradient checkpointing. The repository simplifies the fine-tuning process by not exposing all CLI arguments, instead allowing users to specify options in a config file. It supports efficient training and scaling across multiple GPUs, making it suitable for production-ready fine-tuning jobs.
For similar jobs
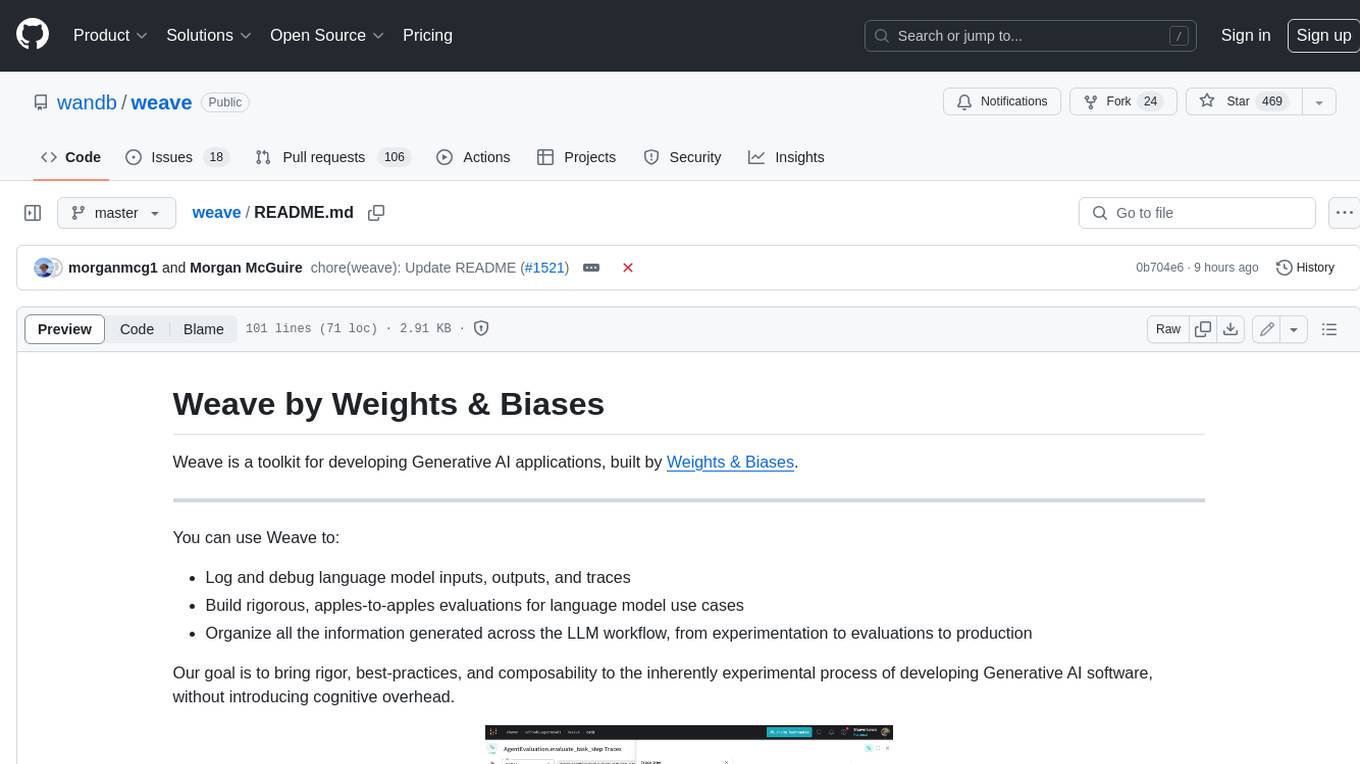
weave
Weave is a toolkit for developing Generative AI applications, built by Weights & Biases. With Weave, you can log and debug language model inputs, outputs, and traces; build rigorous, apples-to-apples evaluations for language model use cases; and organize all the information generated across the LLM workflow, from experimentation to evaluations to production. Weave aims to bring rigor, best-practices, and composability to the inherently experimental process of developing Generative AI software, without introducing cognitive overhead.
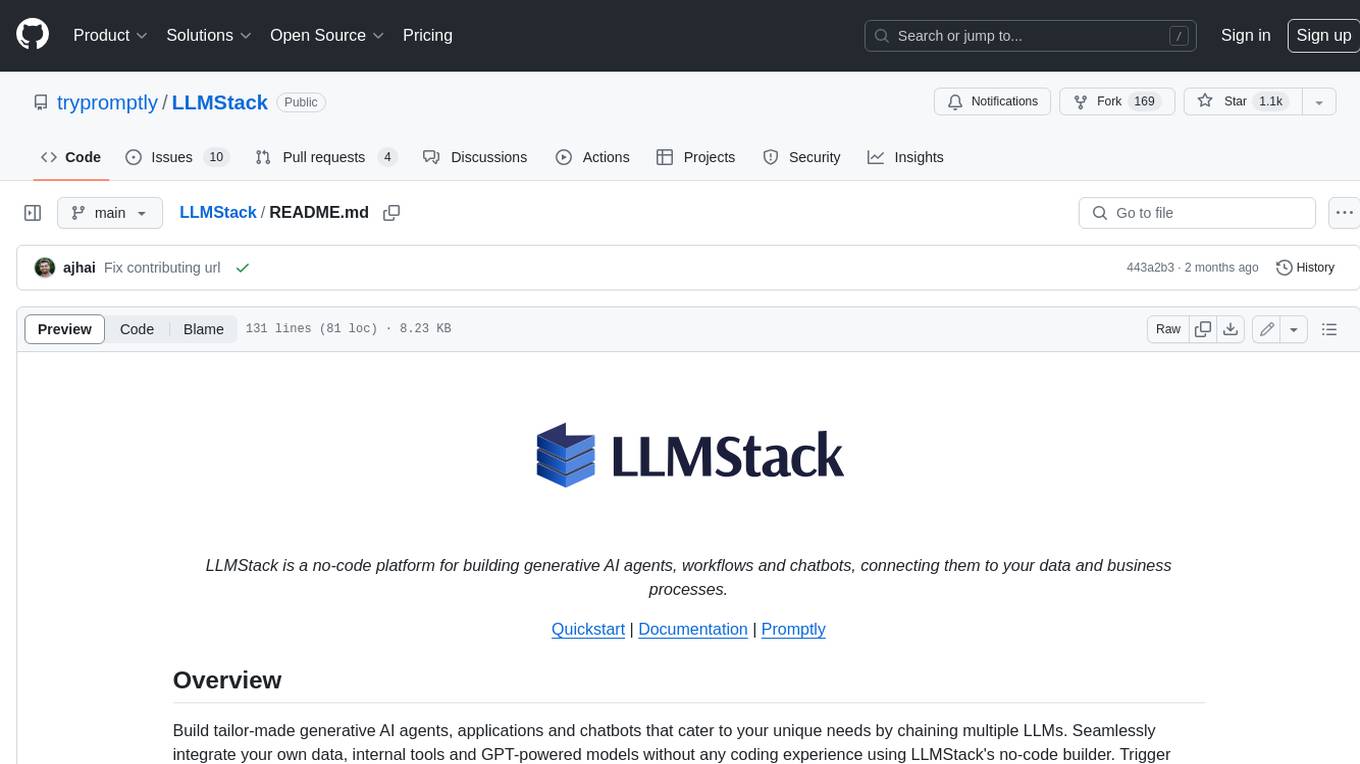
LLMStack
LLMStack is a no-code platform for building generative AI agents, workflows, and chatbots. It allows users to connect their own data, internal tools, and GPT-powered models without any coding experience. LLMStack can be deployed to the cloud or on-premise and can be accessed via HTTP API or triggered from Slack or Discord.
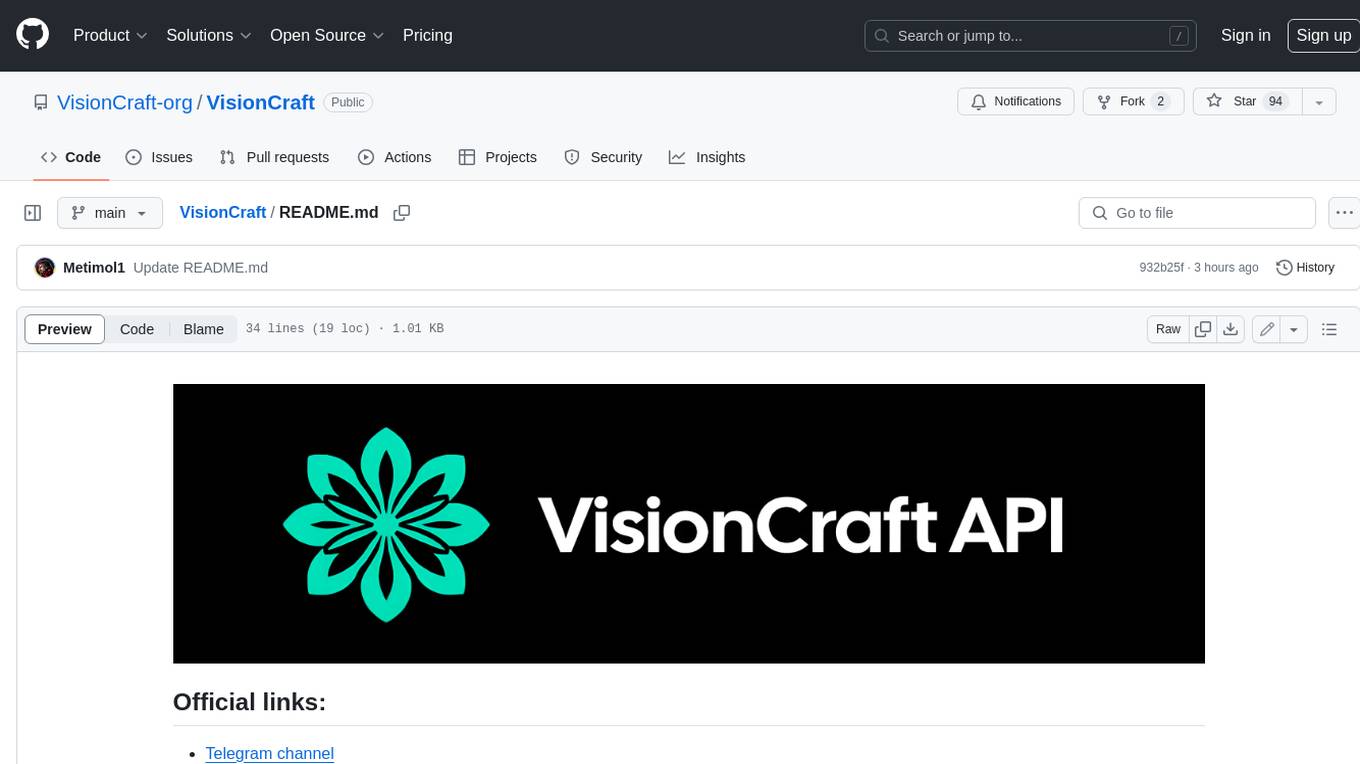
VisionCraft
The VisionCraft API is a free API for using over 100 different AI models. From images to sound.
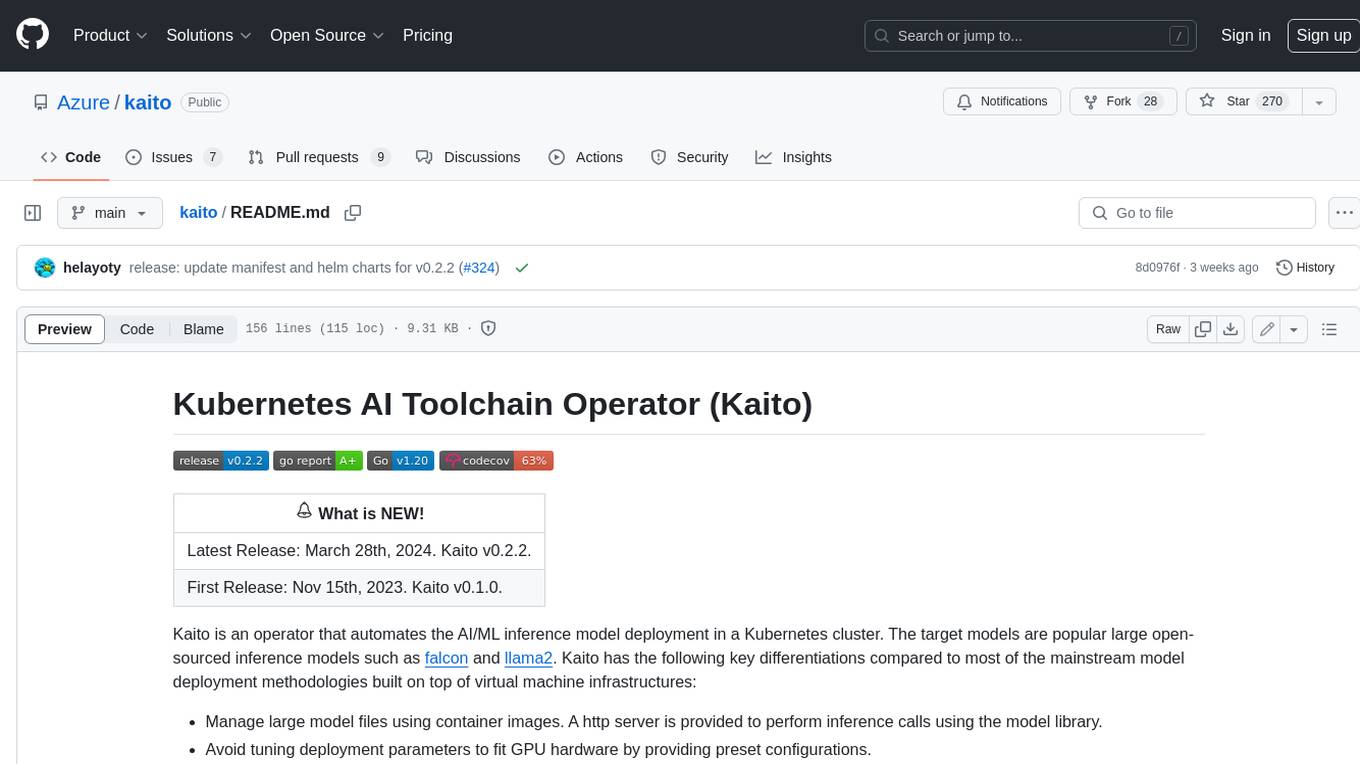
kaito
Kaito is an operator that automates the AI/ML inference model deployment in a Kubernetes cluster. It manages large model files using container images, avoids tuning deployment parameters to fit GPU hardware by providing preset configurations, auto-provisions GPU nodes based on model requirements, and hosts large model images in the public Microsoft Container Registry (MCR) if the license allows. Using Kaito, the workflow of onboarding large AI inference models in Kubernetes is largely simplified.
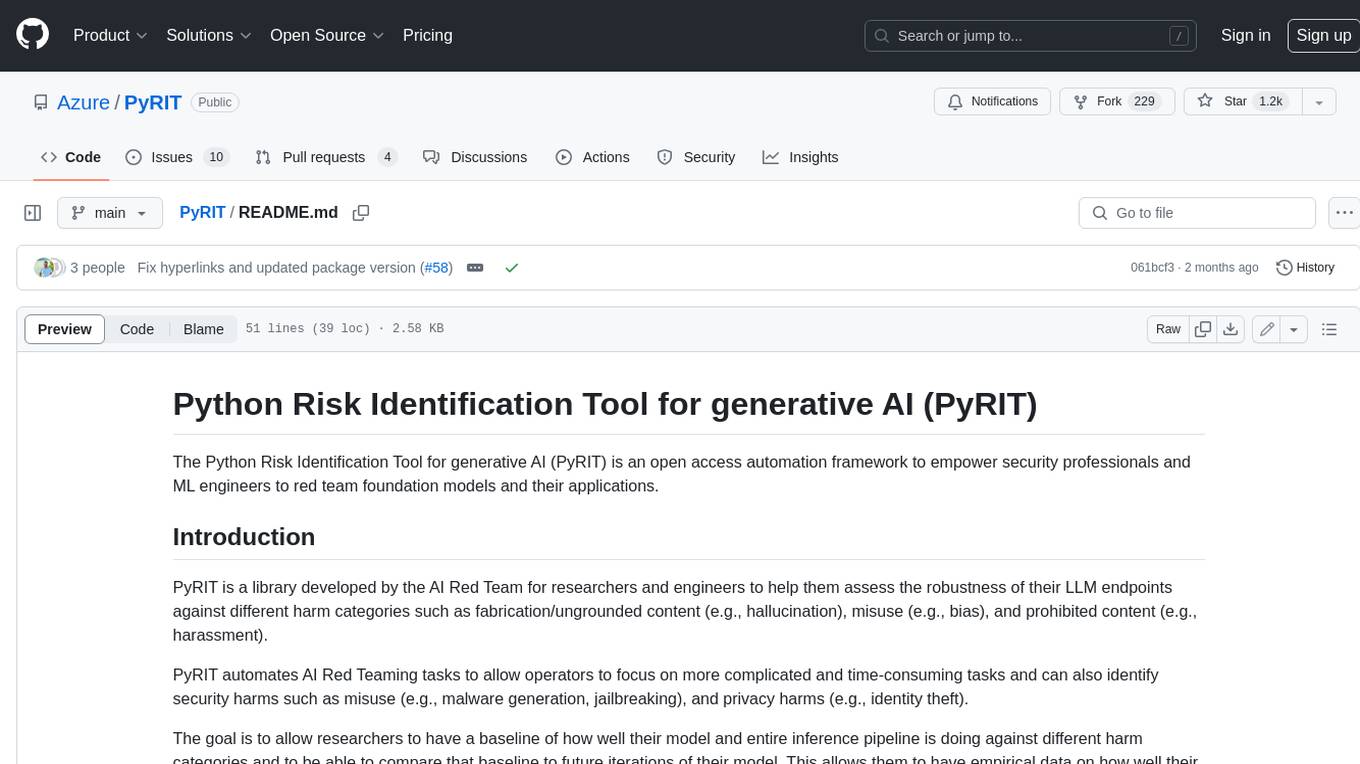
PyRIT
PyRIT is an open access automation framework designed to empower security professionals and ML engineers to red team foundation models and their applications. It automates AI Red Teaming tasks to allow operators to focus on more complicated and time-consuming tasks and can also identify security harms such as misuse (e.g., malware generation, jailbreaking), and privacy harms (e.g., identity theft). The goal is to allow researchers to have a baseline of how well their model and entire inference pipeline is doing against different harm categories and to be able to compare that baseline to future iterations of their model. This allows them to have empirical data on how well their model is doing today, and detect any degradation of performance based on future improvements.
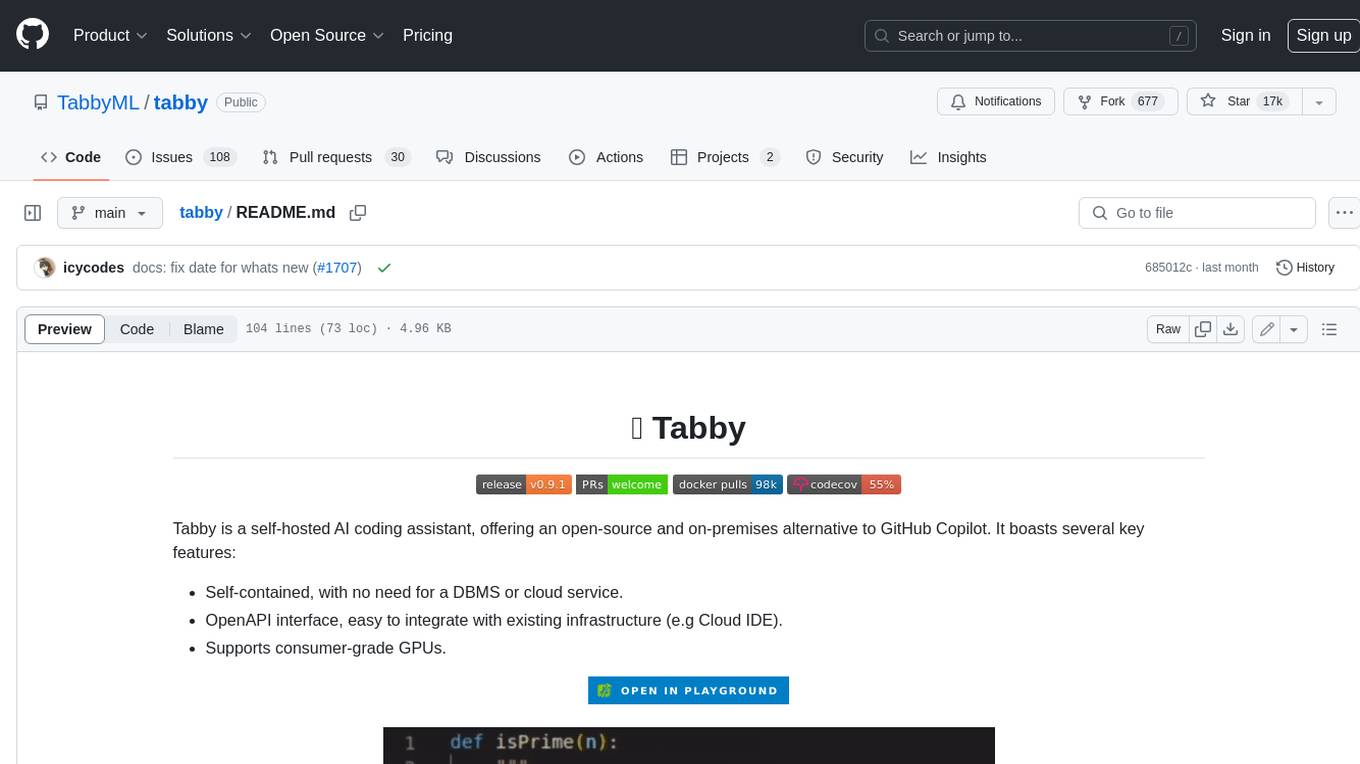
tabby
Tabby is a self-hosted AI coding assistant, offering an open-source and on-premises alternative to GitHub Copilot. It boasts several key features: * Self-contained, with no need for a DBMS or cloud service. * OpenAPI interface, easy to integrate with existing infrastructure (e.g Cloud IDE). * Supports consumer-grade GPUs.
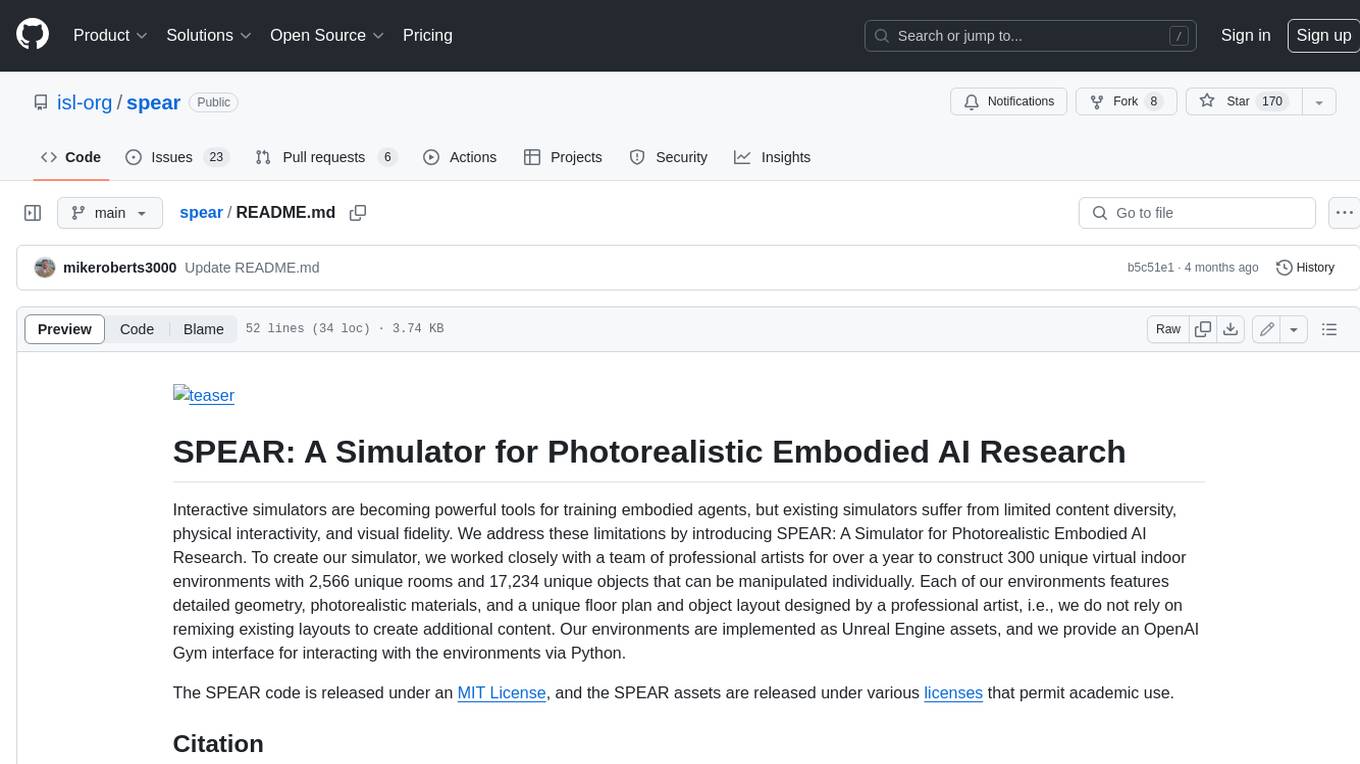
spear
SPEAR (Simulator for Photorealistic Embodied AI Research) is a powerful tool for training embodied agents. It features 300 unique virtual indoor environments with 2,566 unique rooms and 17,234 unique objects that can be manipulated individually. Each environment is designed by a professional artist and features detailed geometry, photorealistic materials, and a unique floor plan and object layout. SPEAR is implemented as Unreal Engine assets and provides an OpenAI Gym interface for interacting with the environments via Python.
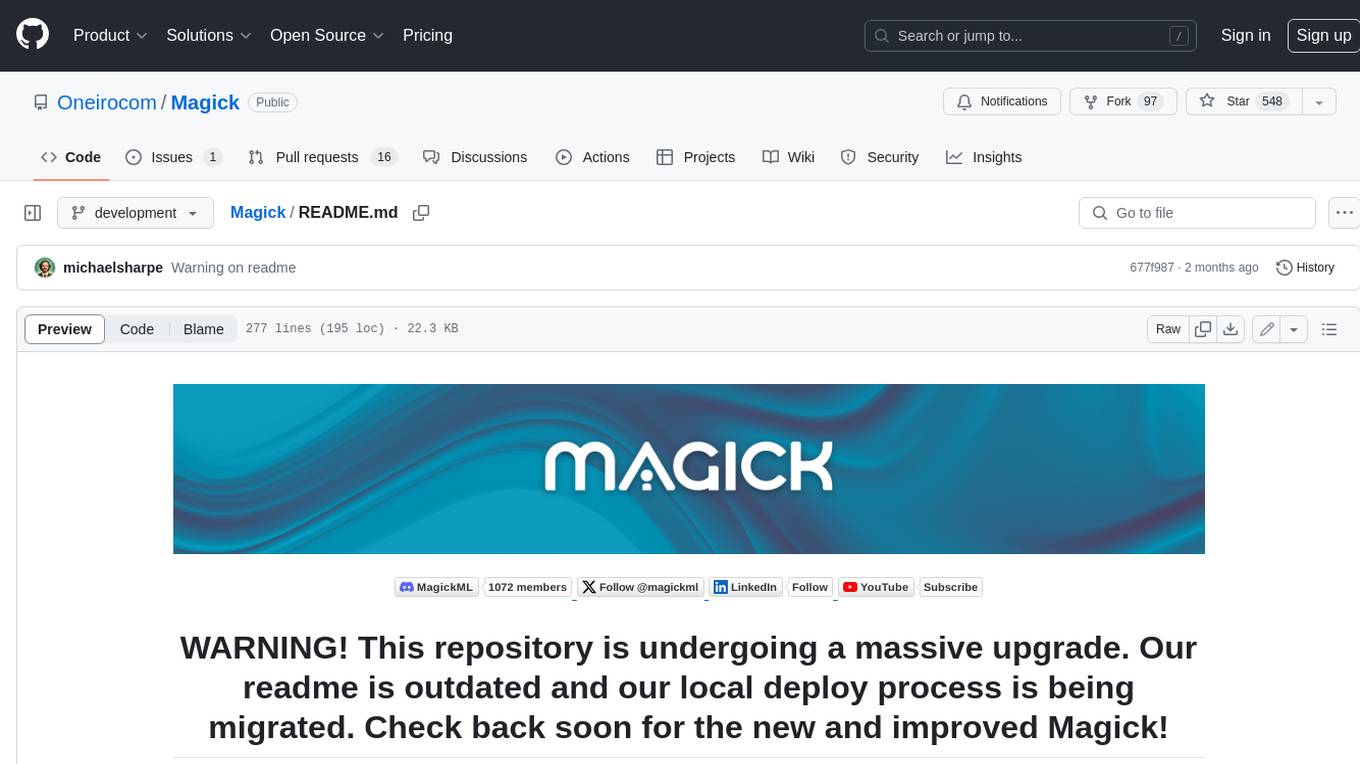
Magick
Magick is a groundbreaking visual AIDE (Artificial Intelligence Development Environment) for no-code data pipelines and multimodal agents. Magick can connect to other services and comes with nodes and templates well-suited for intelligent agents, chatbots, complex reasoning systems and realistic characters.