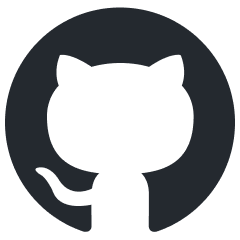
Awesome-RoadMaps-and-Interviews
Awesome Interviews for Coder, Programming Language, Software Engineering, Web, Backend, Distributed Infrastructure, DataScience & AI | 面试必备
Stars: 131
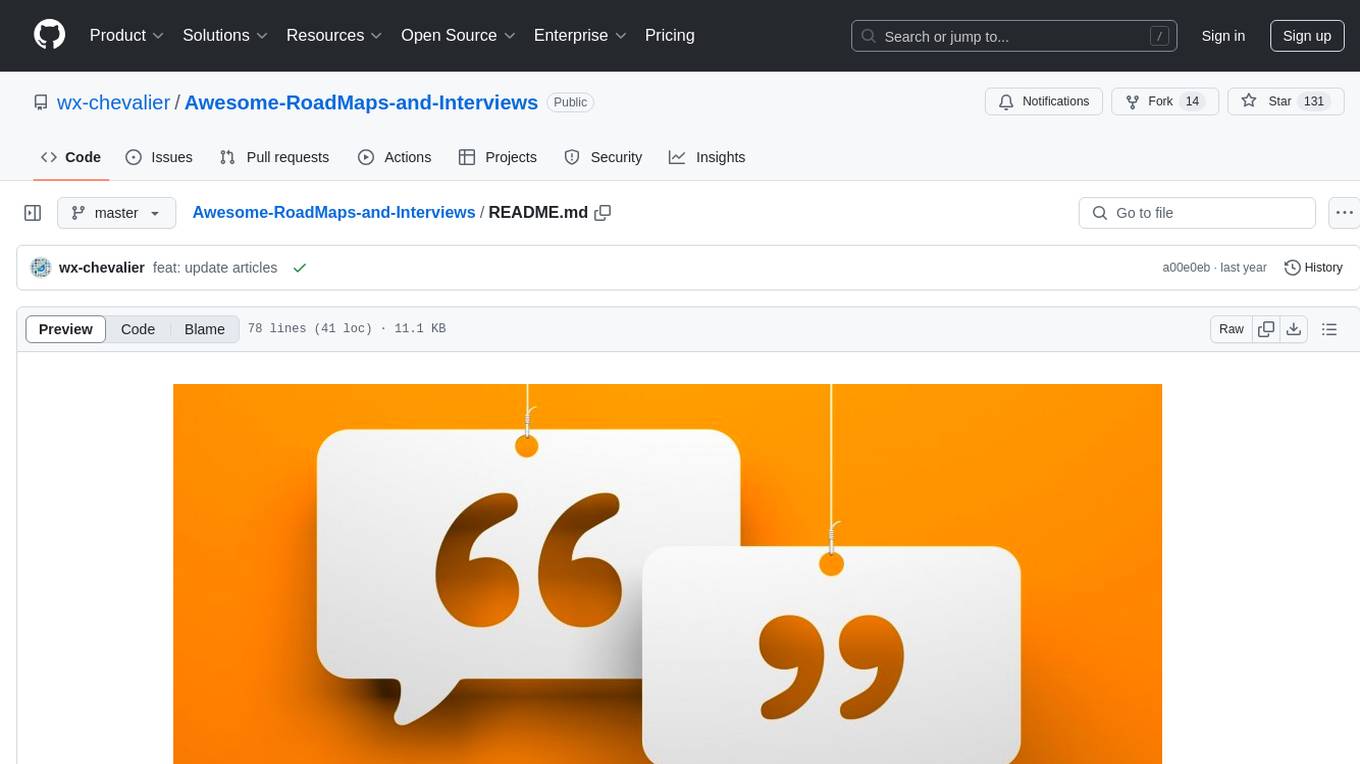
Awesome RoadMaps and Interviews is a comprehensive repository that aims to provide guidance for technical interviews and career development in the ITCS field. It covers a wide range of topics including interview strategies, technical knowledge, and practical insights gained from years of interviewing experience. The repository emphasizes the importance of combining theoretical knowledge with practical application, and encourages users to expand their interview preparation beyond just algorithms. It also offers resources for enhancing knowledge breadth, depth, and programming skills through curated roadmaps, mind maps, cheat sheets, and coding snippets. The content is structured to help individuals navigate various technical roles and technologies, fostering continuous learning and professional growth.
README:
古语有云,知行合一,学以致用,Awesome Interviews 系列不仅仅是为了应对面试,也是对整个 ITCS 体系融会贯通的程度的检验;同时 Awesome Interviews 也包含了笔者多年面试与考察候选人的心得体会。
- 点面结合:网状问题点状问,点状问题网状问,如果是一个复杂的网状问题,你就考他是不是能够三言两语跟外行说清楚。如果是一个简单的问题呢?你就看他能不能把它还原成一个知识网络。
- 正反结合:对于某个技术、框架,不仅要问它的优势,也要问在实际应用过程中感受到的痛点与不足。
本篇也希望能够将面试扩展于算法之外,使得面试尽可能贴合日常工作所需。
更多介绍与导览参阅 INTRODUCTION。
您还可以前往 NGTE Books 主页浏览包含知识体系、编程语言、软件工程、模式与架构、Web 与大前端、服务端开发实践与工程架构、分布式基础架构、人工智能与深度学习、产品运营与创业等多类目的书籍列表:
当我们站在技术之路的原点,未来可能充满了迷茫,也存在着很多不同的可能;我们可能成为 Web/(大)前端/终端工程师、服务端架构工程师、测试/运维/安全工程师等质量保障、可用性保障相关的工程师、大数据/云计算/虚拟化工程师、算法工程师、产品经理等等某个或者某几个角色。在这个知识爆炸与终身学习/碎片化学习为主的时代,我们面临的问题之一就是如何进行有效学习,不仅能有效平衡广度与深度,并且能真正的积淀下来,提升自己的研发效能。于笔者而言,常常郁结于胸的就是以下三个问题:
- 应该学习什么?这是怎样的一个技术世界?存在着怎样的高峰与路径?
- 如何克服遗忘带来的无效学习?
- 如何不再碎片化地学习?
究其根本,也就是需要拓展自己的知识广度,精进自己的知识深度,锤炼自己的编程能力。所谓知识广度,即是为实际问题选择合适的解决方案的能力,广义来说也是眼界与格局的表现。它并不拘泥于某个技术方向或者行业领域,而需要对传统/流行的各类语言、工具、框架、库、服务等有一定的认识;能够明晰各个方案的优劣,并在较高的层次(High Level)描述相关原理。知识广度的拓展与保持需要建立在庞大的阅读量与知识沉淀能力上。在能力模型一节中,我们从业务、团队、技术这三个维度,对技术人员的五个层次进行了梳理:
Awesome Lists 就为我们准备了精而全的技术开发学习与实践资料索引,去芜存菁,去重留一;譬如其中的 Awesome WebSites 一文就为我们推荐了值得阅读的资讯、博客等站点列表。知识广度的拓展也并非一蹴而就之事,需得循序渐进,从初窥门径,到登堂入室,最后融会贯通,当我们感觉乱花渐欲迷人眼,太多的碎片化知识反而使自己迷失方向之际,就可以前往 Awesome CS Books Warehouse,去深入地阅读学习各个领域的精选书籍、课程等系统化的内容。
俗话说,好记性不如烂笔头,当我们阅读的多了,自然也要开始记录;而笔者认为记录的开始就要有自己的知识体系。在自己的知识体系下随看随记、定期整理。唯有建立符合自己认知方式的知识图谱,才能有效地沉淀知识,明晰知识边界并进行不断地探索。上车伊始,笔者即致力于构建自己的 Awesome MindMaps,提供了软件工程通用、前端、后端、DevOps、测试、架构师、人工智能工程师等多领域的知识图谱、学习成长路线与面试必备内容,并在数年来不断维护与刷新。
笔者目前选择的是以 MarkDown 格式记录,并且将所有的笔记存放于 Github-文档札记以 Git 方式进行版本管理;编辑器是直接使用的 VSCode,移动端编辑的话也是用的 GitGo/WorkCopy 这样的 Git 应用。这些笔记即是笔者自身技术视野与认知的外化,也类比于外设之于内存,在需要的时候分页加载到脑海中使用,以应对这知识爆炸的时代。其中的典型代表,Awesome CheatSheets,对于日常开发中用到的相关知识的备忘录/清单进行总结, 适合快速掌握或者回顾某个语言/框架/工具的语法或使用要点。Tech Road, 我的技术之路是对于笔者多年学习与认知变迁的总结。
先贤有云,知行合一,知是行之始,行是知之成,Linus Torvalds 也曾提到:'Talk is cheap. Show me the code.',在阅读与笔记之后,就是要开始实践编码。所谓编程能力,并不仅仅是编写代码(Write Code)的能力,而是包含了阅读、编写、理解、重构、抽象等多个方面,是所谓的代码管理/掌控。其外在表现之一即是能够随时随地用合适的语言无阻塞地实现某些功能需求,对于常见的语法,接口,算法,设计模式等能够做到心随意动,信手拈来。编程能力是提升研发效能的重要保障,于笔者而言也是毕生应该追求的目标与爱好之一。笔者的编程能力较弱,日常开发,特别是在多语言多框架并用的场景下,往往会需要不断地中断,查找以继续工作,也是令我颇为苦恼。Coding Snippets 系列仓库,即是对笔者在编程语言、前后端编码过程中常用的模板、模式等代码片、工具库的汇聚。
前文重在讨论如何拓宽技术视野、追寻技术的广度,但是需要铭记的是,技术深度才是技术广度的基石,正如中国自古以来常用道术之辩,知其然,也要知其所以然;亦如 Richard Feynman 所述:”What I cannot create, I do not understand.”。所谓知识深度,即是能够对某个方面做到深入了解,并且达到融会贯通,洞若观火,可以随心所欲地加以扩展、优化、创新等改造或变换。这方面则更加的见仁见智,不同的领域与方向对于深度的定义与挖掘方向也是千差万别。Reinvent Wheels 系列仓库即是笔者在前后端到基础架构中重造的轮子的汇总。
我们需要自己去从零开始造些轮子,才能深刻理解使用的框架/库/平台的内部原理,才能在碰到故障时快速地修复;在下文的几乎每个章节中,我们都会提到某些笔者自造的轮子。笔者目前将所有的文档归类到了不同的系列中,每个系列又分为 篇-章-节-小节 这样的层次结构。
编程语言是一切的基础,正如 Steve McConnell 在 Code Complete 一书中提及,我们应该 Program into a language
而不是 Program in a language
,针对不同的需要选择合适的编程语言来实现,而不是受制于自己所会的语言。在知识图谱中也包含了跨编程语言的公共知识杂谈,笔者与编程语言相关的文章存放在 Programming Language Series | 编程语言语法基础与工程实践仓库中,其涵盖了 C/C++、Go、Java、JavaScript、Python、Rust、Swift 等常见的语言,以及通用的编程语言理论。
编程能力锻炼的基础,首要的就是关于数据结构与算法,以及面向对象的设计模式,其对应的代码分别存放在了 coding-snippets, algorithm-snippets, design-pattern-snippets 中。此外,我们还可以从零编写一些类似于 Guava & Lodash 这样的自己的通用工具库,笔者自身是整合在了 Guash 中。
在编程语言之上,我们就需要考虑如何去实现真正的软件系统,譬如 软件工程基础 系列中的开发工具/Git 漫谈、软件系统架构、软件质量保障等内容,我们也可以自己去实现一些自己的工具,譬如笔者的 Soogle 是构建自身搜索、外部服务访问能力的工具集合;而 NGTE Website 是包含个人主页在内的多端阅读能力的源代码仓库。
-
Developer Roadmaps: Step by step guides and paths to learn different tools or technologies
-
golang-developer-roadmap: Roadmap to becoming a Go developer in 2020
-
data-engineer-roadmap: Roadmap to becoming a data engineer in 2021
笔者所有文章遵循知识共享 署名 - 非商业性使用 - 禁止演绎 4.0 国际许可协议,欢迎转载,尊重版权。您还可以前往 NGTE Books 主页浏览包含知识体系、编程语言、软件工程、模式与架构、Web 与大前端、服务端开发实践与工程架构、分布式基础架构、人工智能与深度学习、产品运营与创业等多类目的书籍列表:
For Tasks:
Click tags to check more tools for each tasksFor Jobs:
Alternative AI tools for Awesome-RoadMaps-and-Interviews
Similar Open Source Tools
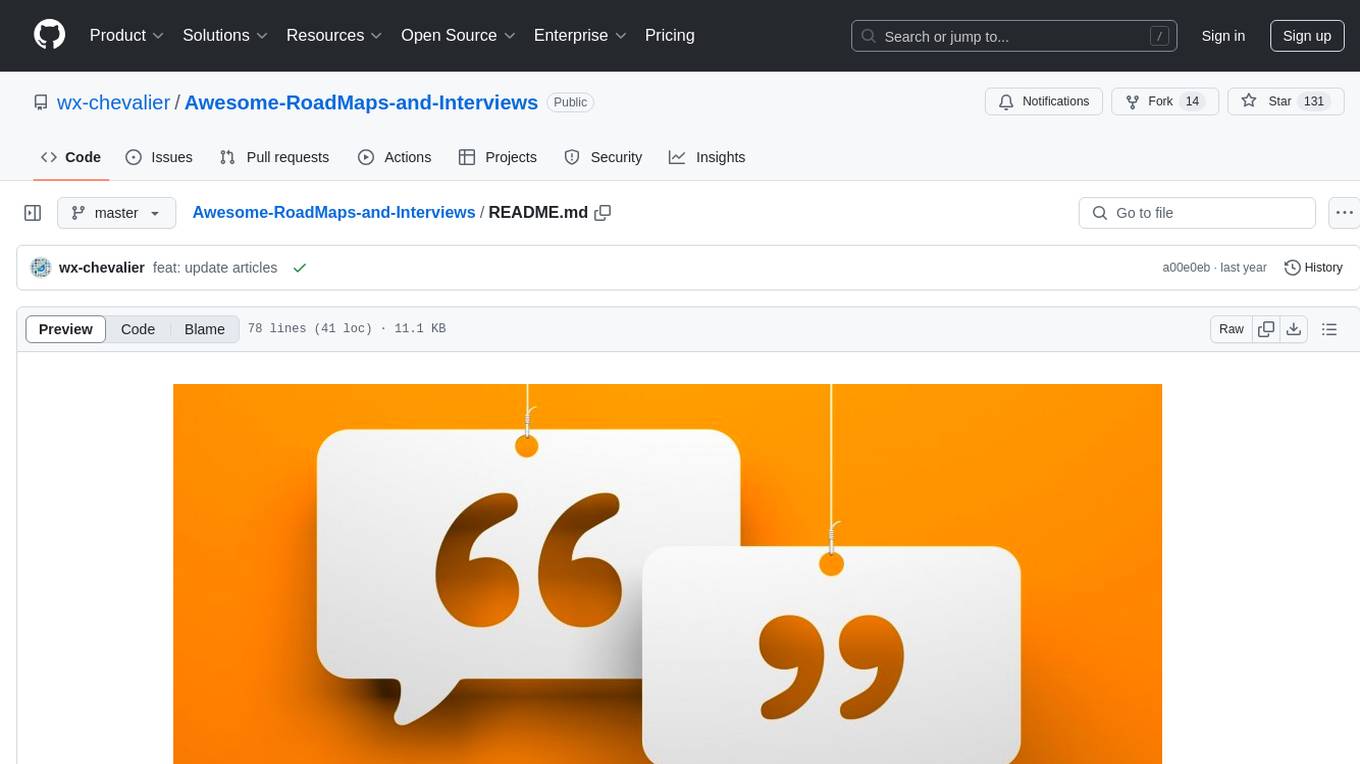
Awesome-RoadMaps-and-Interviews
Awesome RoadMaps and Interviews is a comprehensive repository that aims to provide guidance for technical interviews and career development in the ITCS field. It covers a wide range of topics including interview strategies, technical knowledge, and practical insights gained from years of interviewing experience. The repository emphasizes the importance of combining theoretical knowledge with practical application, and encourages users to expand their interview preparation beyond just algorithms. It also offers resources for enhancing knowledge breadth, depth, and programming skills through curated roadmaps, mind maps, cheat sheets, and coding snippets. The content is structured to help individuals navigate various technical roles and technologies, fostering continuous learning and professional growth.
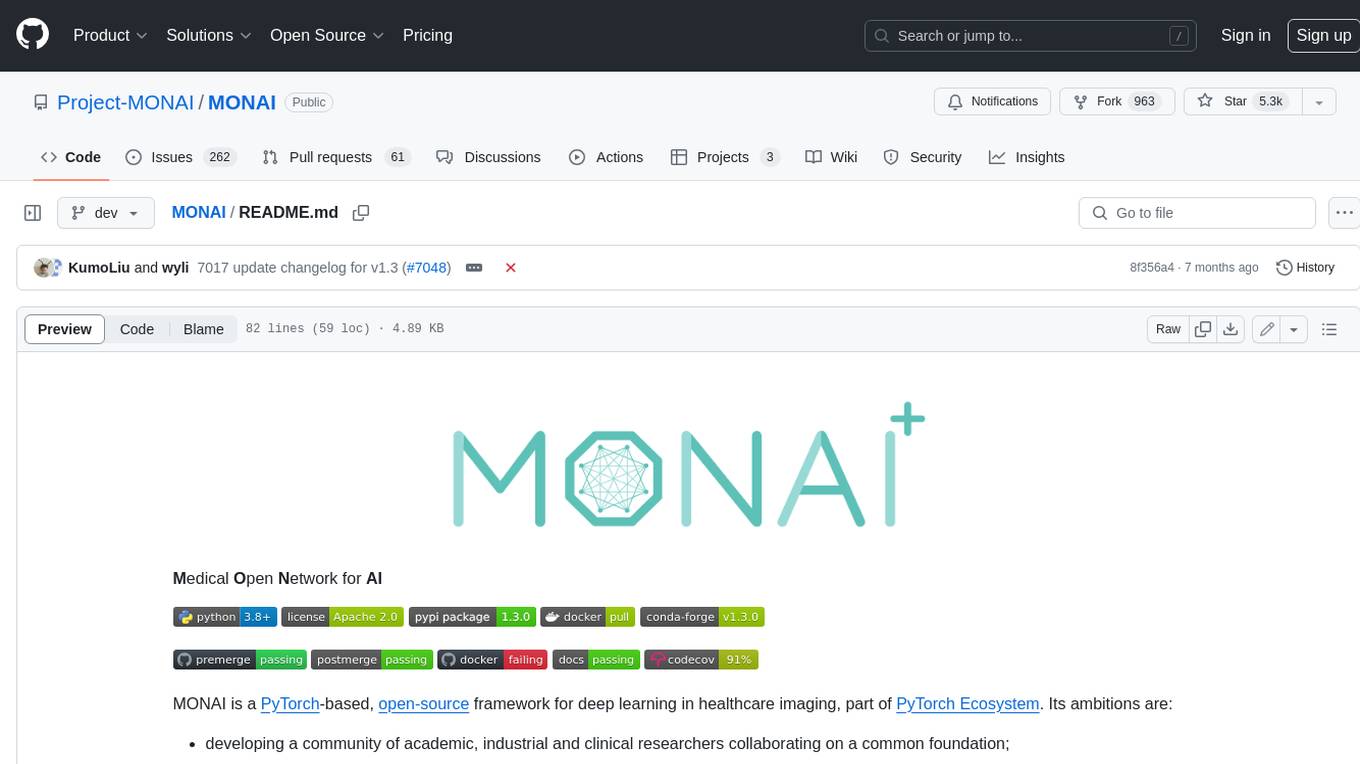
MONAI
MONAI is a PyTorch-based, open-source framework for deep learning in healthcare imaging. It provides a comprehensive set of tools for medical image analysis, including data preprocessing, model training, and evaluation. MONAI is designed to be flexible and easy to use, making it a valuable resource for researchers and developers in the field of medical imaging.
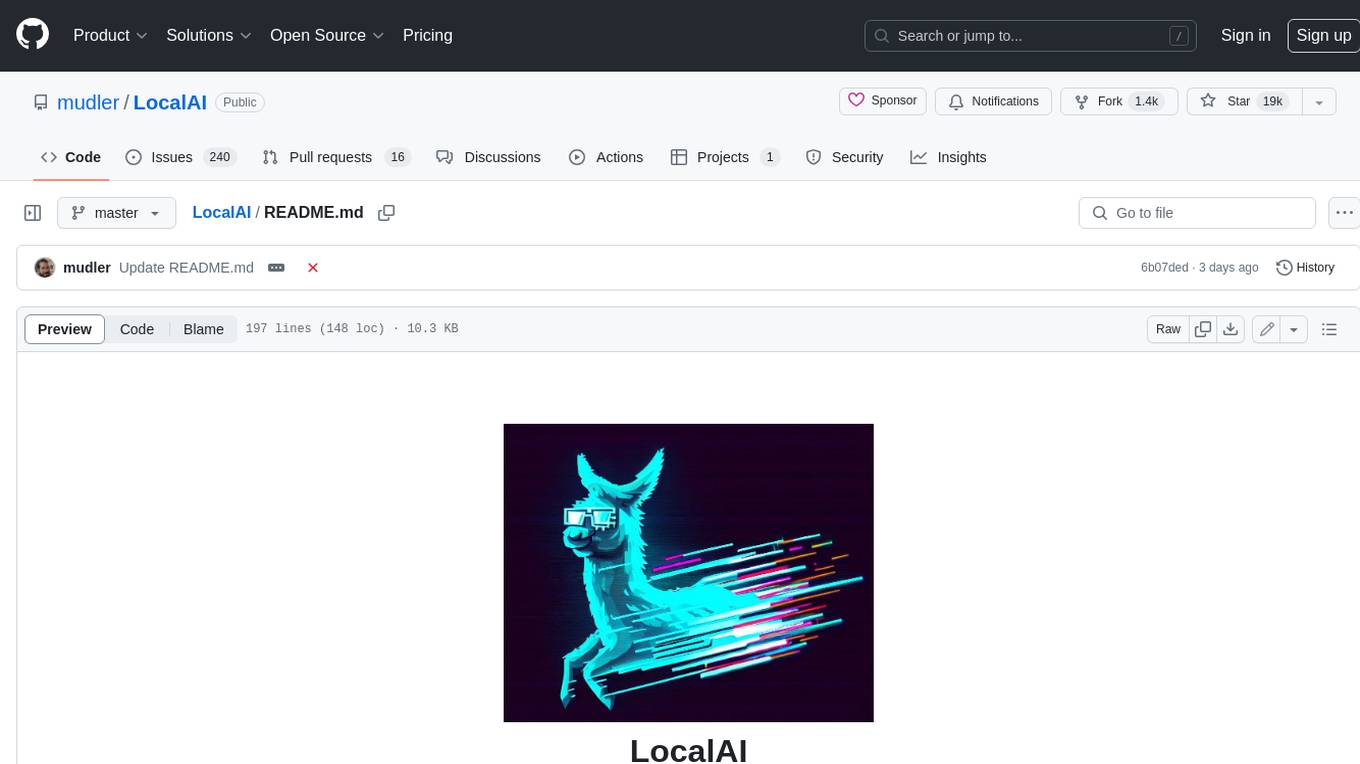
LocalAI
LocalAI is a free and open-source OpenAI alternative that acts as a drop-in replacement REST API compatible with OpenAI (Elevenlabs, Anthropic, etc.) API specifications for local AI inferencing. It allows users to run LLMs, generate images, audio, and more locally or on-premises with consumer-grade hardware, supporting multiple model families and not requiring a GPU. LocalAI offers features such as text generation with GPTs, text-to-audio, audio-to-text transcription, image generation with stable diffusion, OpenAI functions, embeddings generation for vector databases, constrained grammars, downloading models directly from Huggingface, and a Vision API. It provides a detailed step-by-step introduction in its Getting Started guide and supports community integrations such as custom containers, WebUIs, model galleries, and various bots for Discord, Slack, and Telegram. LocalAI also offers resources like an LLM fine-tuning guide, instructions for local building and Kubernetes installation, projects integrating LocalAI, and a how-tos section curated by the community. It encourages users to cite the repository when utilizing it in downstream projects and acknowledges the contributions of various software from the community.
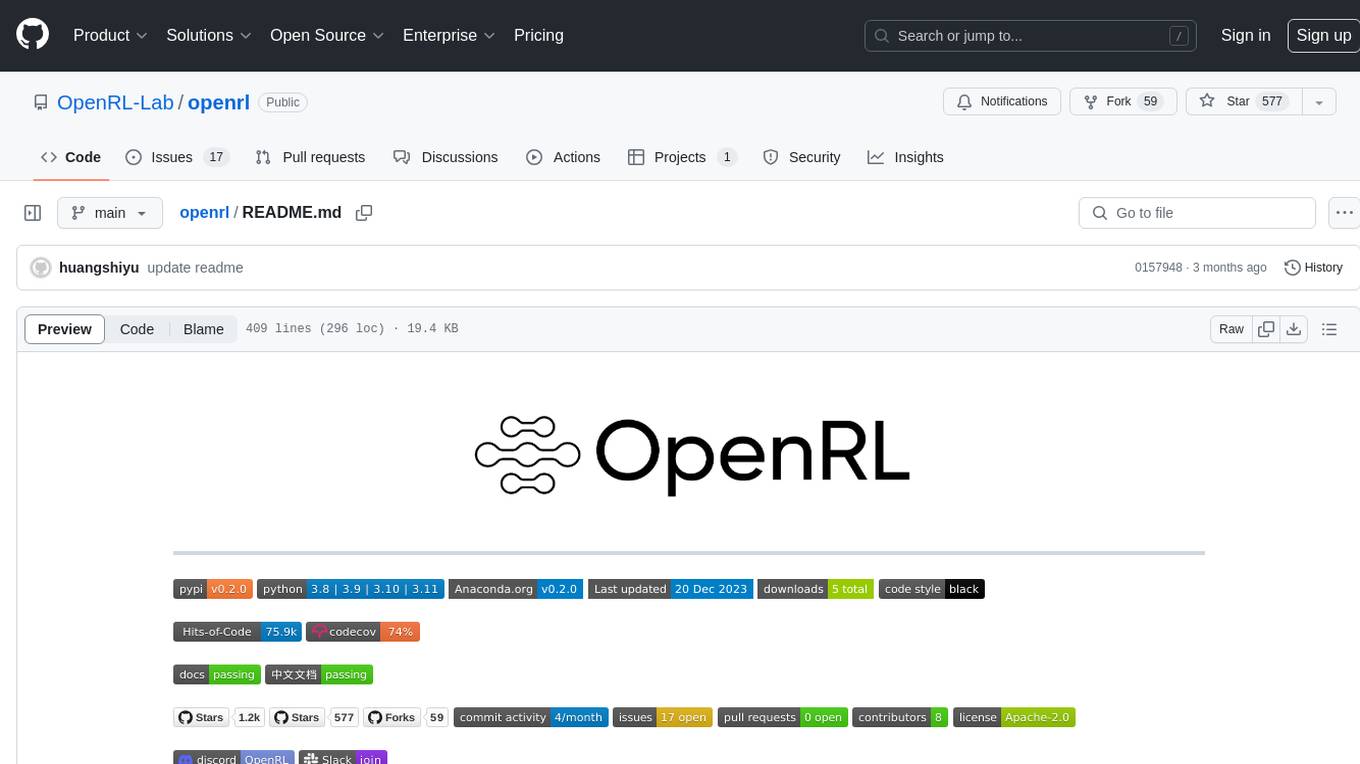
openrl
OpenRL is an open-source general reinforcement learning research framework that supports training for various tasks such as single-agent, multi-agent, offline RL, self-play, and natural language. Developed based on PyTorch, the goal of OpenRL is to provide a simple-to-use, flexible, efficient and sustainable platform for the reinforcement learning research community. It supports a universal interface for all tasks/environments, single-agent and multi-agent tasks, offline RL training with expert dataset, self-play training, reinforcement learning training for natural language tasks, DeepSpeed, Arena for evaluation, importing models and datasets from Hugging Face, user-defined environments, models, and datasets, gymnasium environments, callbacks, visualization tools, unit testing, and code coverage testing. It also supports various algorithms like PPO, DQN, SAC, and environments like Gymnasium, MuJoCo, Atari, and more.
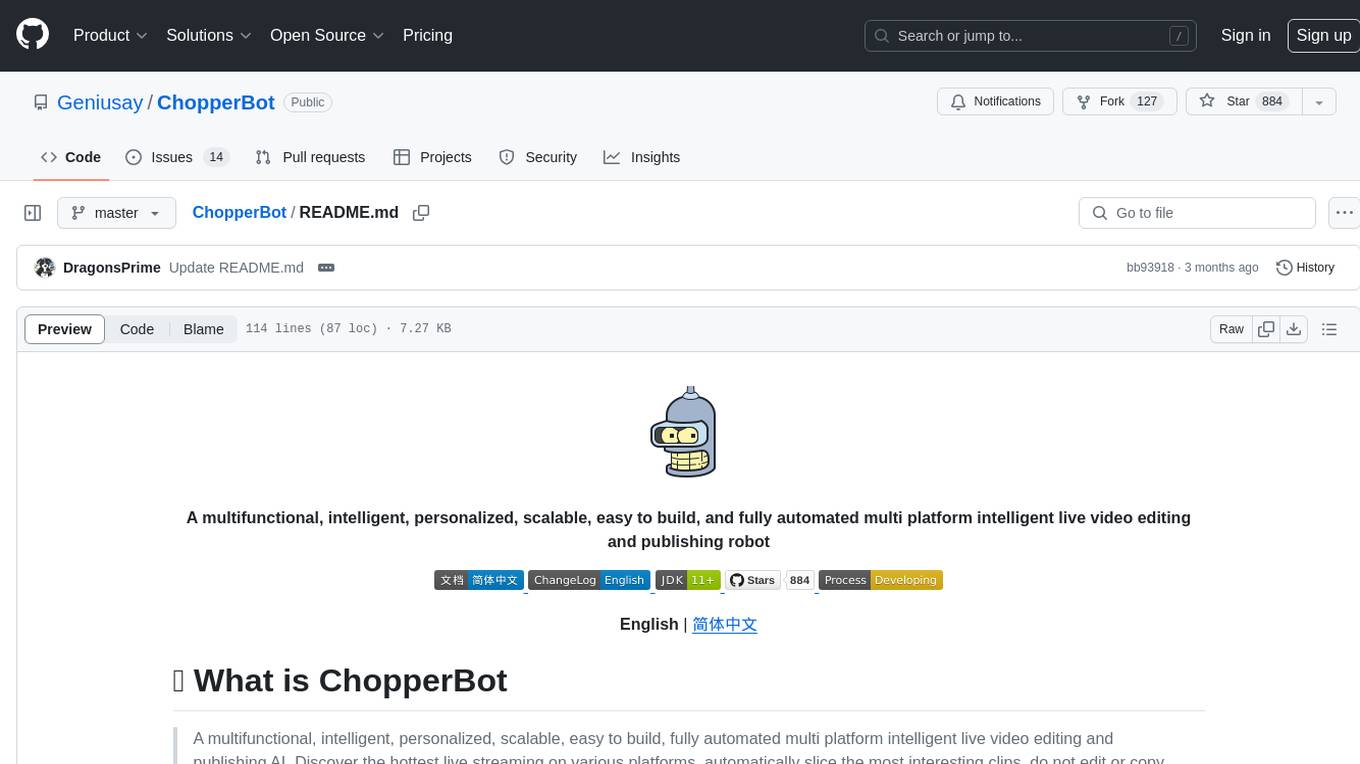
ChopperBot
A multifunctional, intelligent, personalized, scalable, easy to build, and fully automated multi platform intelligent live video editing and publishing robot. ChopperBot is a comprehensive AI tool that automatically analyzes and slices the most interesting clips from popular live streaming platforms, generates and publishes content, and manages accounts. It supports plugin DIY development and hot swapping functionality, making it easy to customize and expand. With ChopperBot, users can quickly build their own live video editing platform without the need to install any software, thanks to its visual management interface.
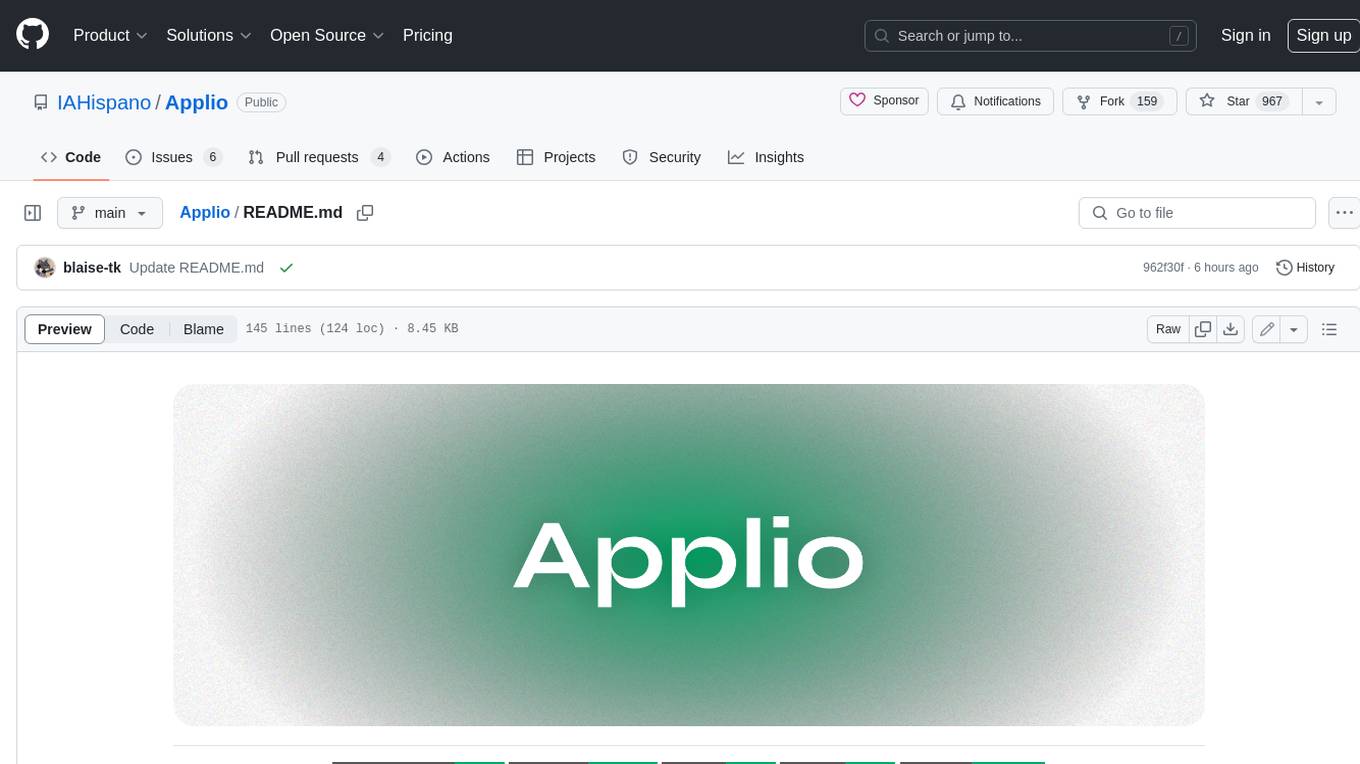
Applio
Applio is a VITS-based Voice Conversion tool focused on simplicity, quality, and performance. It features a user-friendly interface, cross-platform compatibility, and a range of customization options. Applio is suitable for various tasks such as voice cloning, voice conversion, and audio editing. Its key features include a modular codebase, hop length implementation, translations in over 30 languages, optimized requirements, streamlined installation, hybrid F0 estimation, easy-to-use UI, optimized code and dependencies, plugin system, overtraining detector, model search, enhancements in pretrained models, voice blender, accessibility improvements, new F0 extraction methods, output format selection, hashing system, model download system, TTS enhancements, split audio, Discord presence, Flask integration, and support tab.
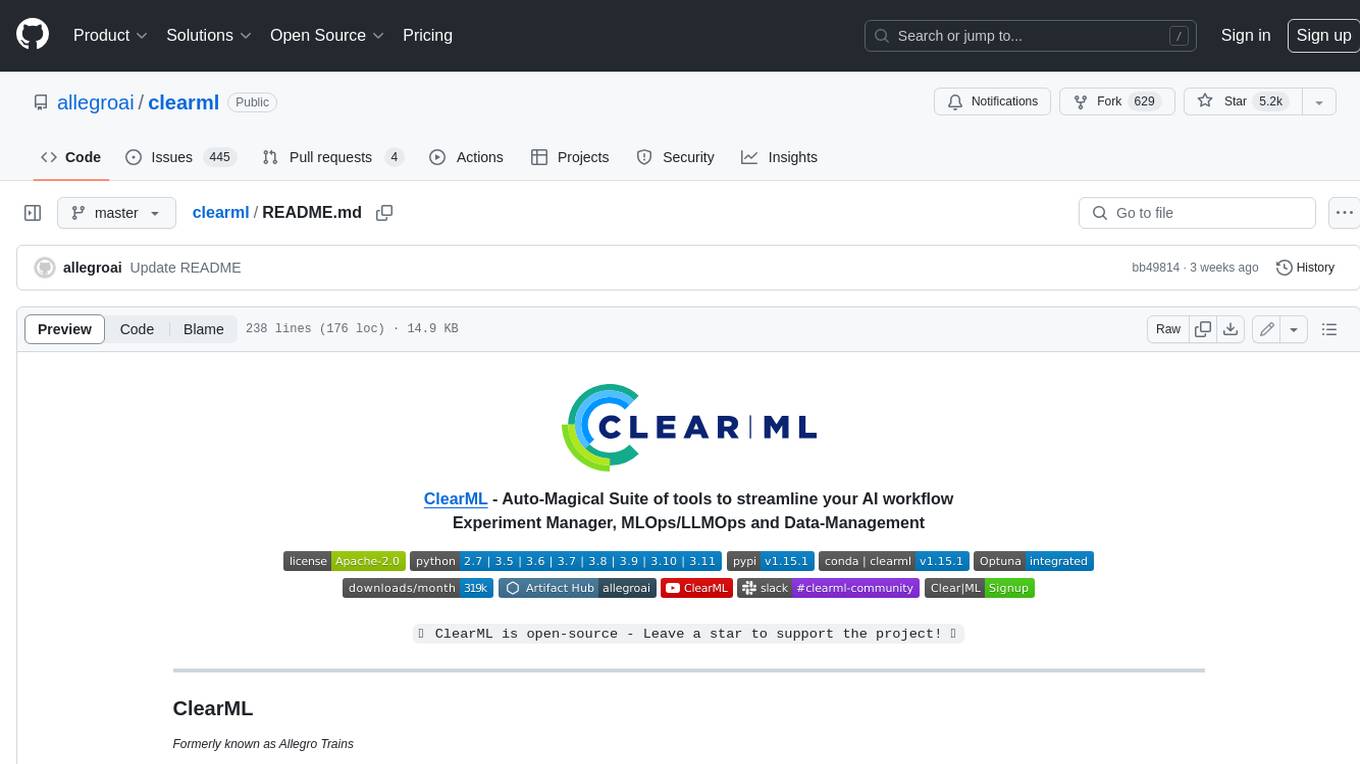
clearml
ClearML is a suite of tools designed to streamline the machine learning workflow. It includes an experiment manager, MLOps/LLMOps, data management, and model serving capabilities. ClearML is open-source and offers a free tier hosting option. It supports various ML/DL frameworks and integrates with Jupyter Notebook and PyCharm. ClearML provides extensive logging capabilities, including source control info, execution environment, hyper-parameters, and experiment outputs. It also offers automation features, such as remote job execution and pipeline creation. ClearML is designed to be easy to integrate, requiring only two lines of code to add to existing scripts. It aims to improve collaboration, visibility, and data transparency within ML teams.
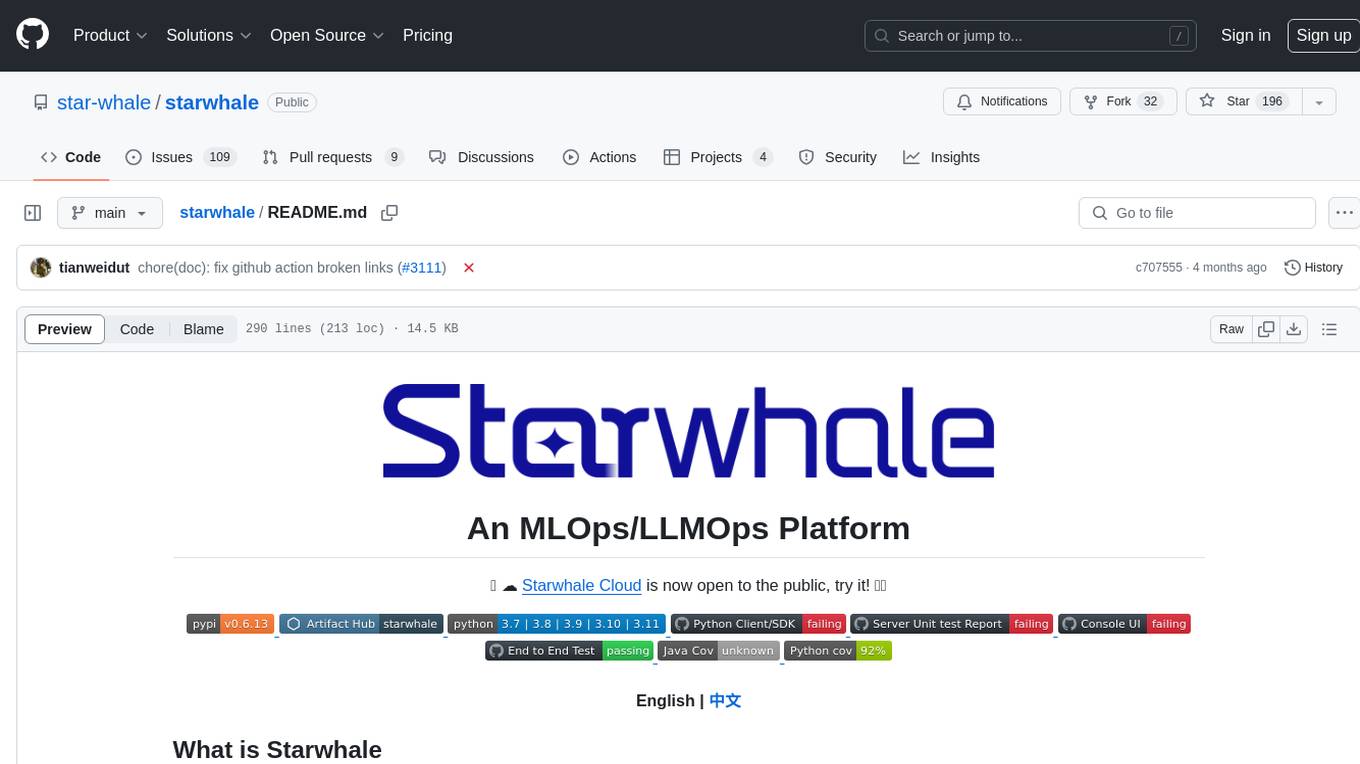
starwhale
Starwhale is an MLOps/LLMOps platform that brings efficiency and standardization to machine learning operations. It streamlines the model development lifecycle, enabling teams to optimize workflows around key areas like model building, evaluation, release, and fine-tuning. Starwhale abstracts Model, Runtime, and Dataset as first-class citizens, providing tailored capabilities for common workflow scenarios including Models Evaluation, Live Demo, and LLM Fine-tuning. It is an open-source platform designed for clarity and ease of use, empowering developers to build customized MLOps features tailored to their needs.
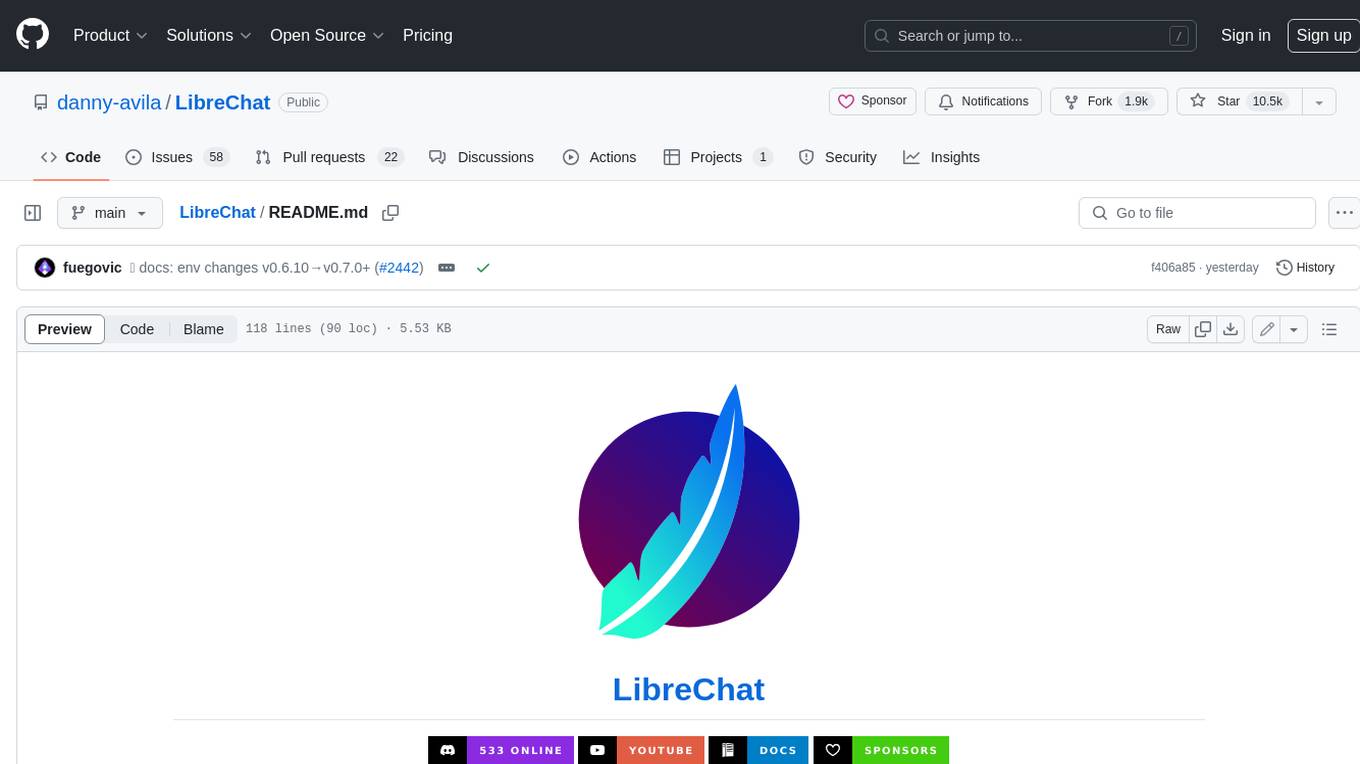
LibreChat
LibreChat is an all-in-one AI conversation platform that integrates multiple AI models, including ChatGPT, into a user-friendly interface. It offers a wide range of features, including multimodal chat, multilingual UI, AI model selection, custom presets, conversation branching, message export, search, plugins, multi-user support, and extensive configuration options. LibreChat is open-source and community-driven, with a focus on providing a free and accessible alternative to ChatGPT Plus. It is designed to enhance productivity, creativity, and communication through advanced AI capabilities.
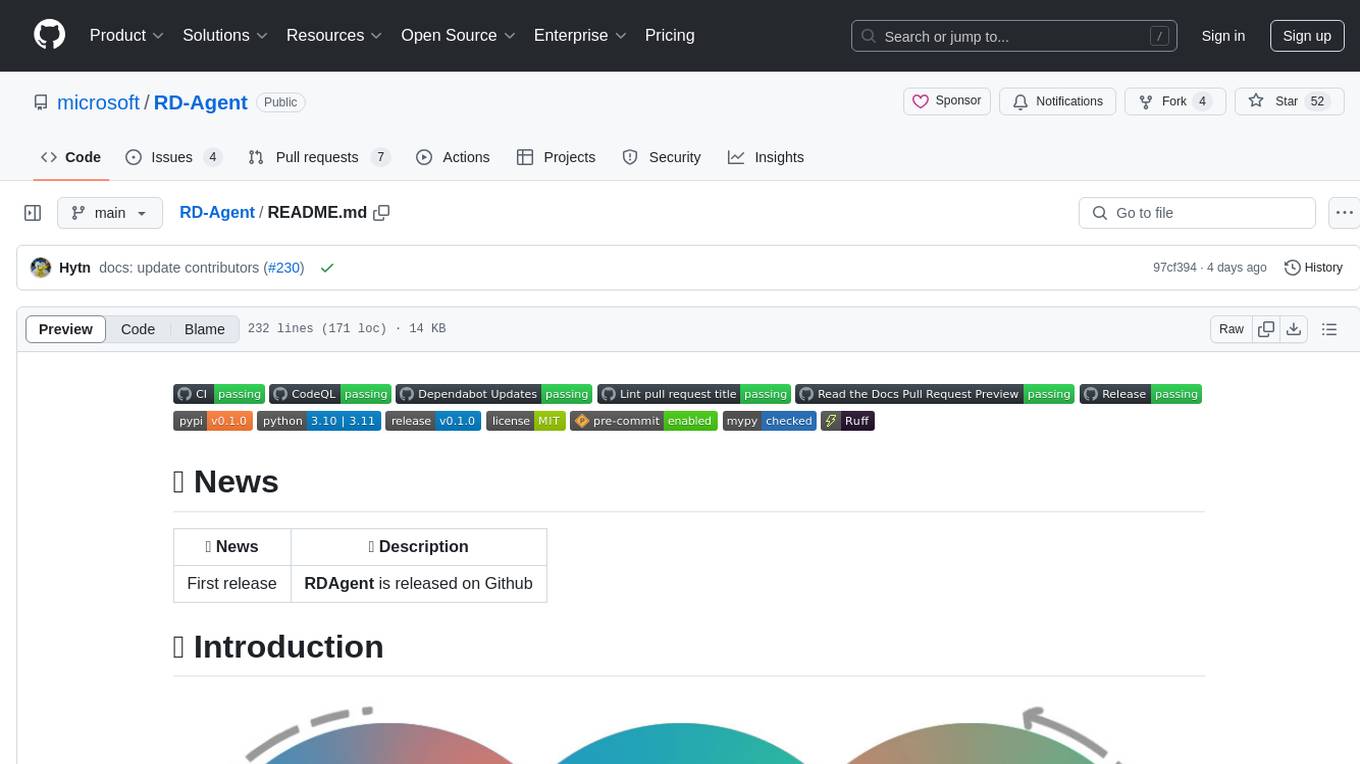
RD-Agent
RD-Agent is a tool designed to automate critical aspects of industrial R&D processes, focusing on data-driven scenarios to streamline model and data development. It aims to propose new ideas ('R') and implement them ('D') automatically, leading to solutions of significant industrial value. The tool supports scenarios like Automated Quantitative Trading, Data Mining Agent, Research Copilot, and more, with a framework to push the boundaries of research in data science. Users can create a Conda environment, install the RDAgent package from PyPI, configure GPT model, and run various applications for tasks like quantitative trading, model evolution, medical prediction, and more. The tool is intended to enhance R&D processes and boost productivity in industrial settings.
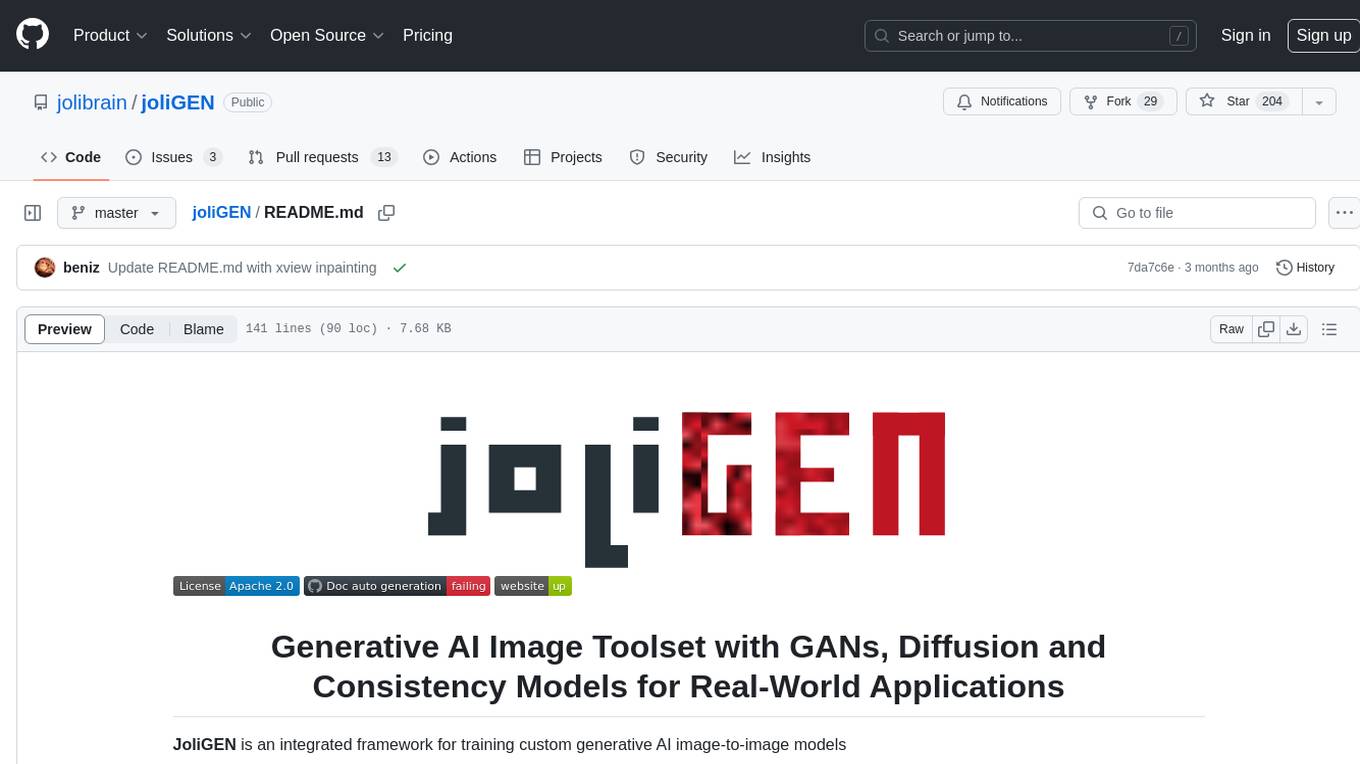
joliGEN
JoliGEN is an integrated framework for training custom generative AI image-to-image models. It implements GAN, Diffusion, and Consistency models for various image translation tasks, including domain and style adaptation with conservation of semantics. The tool is designed for real-world applications such as Controlled Image Generation, Augmented Reality, Dataset Smart Augmentation, and Synthetic to Real transforms. JoliGEN allows for fast and stable training with a REST API server for simplified deployment. It offers a wide range of options and parameters with detailed documentation available for models, dataset formats, and data augmentation.
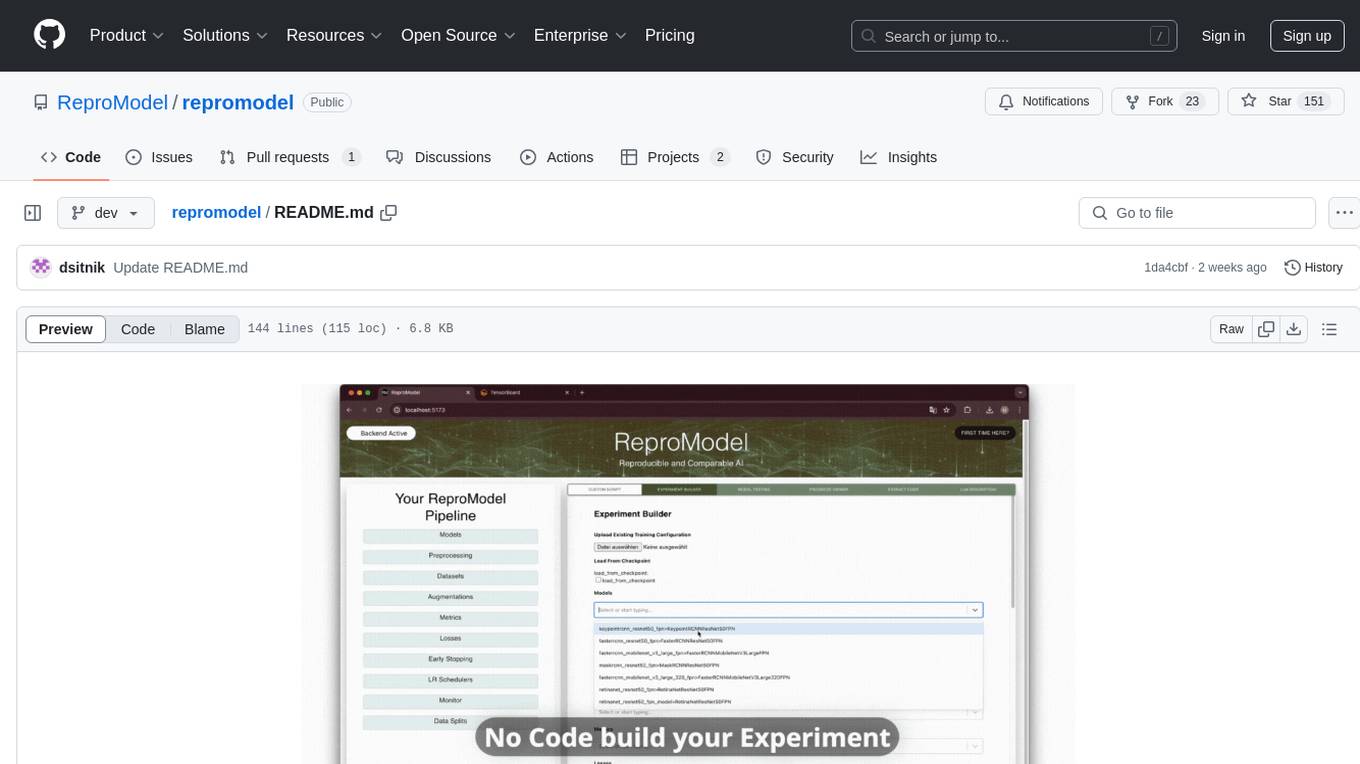
repromodel
ReproModel is an open-source toolbox designed to boost AI research efficiency by enabling researchers to reproduce, compare, train, and test AI models faster. It provides standardized models, dataloaders, and processing procedures, allowing researchers to focus on new datasets and model development. With a no-code solution, users can access benchmark and SOTA models and datasets, utilize training visualizations, extract code for publication, and leverage an LLM-powered automated methodology description writer. The toolbox helps researchers modularize development, compare pipeline performance reproducibly, and reduce time for model development, computation, and writing. Future versions aim to facilitate building upon state-of-the-art research by loading previously published study IDs with verified code, experiments, and results stored in the system.
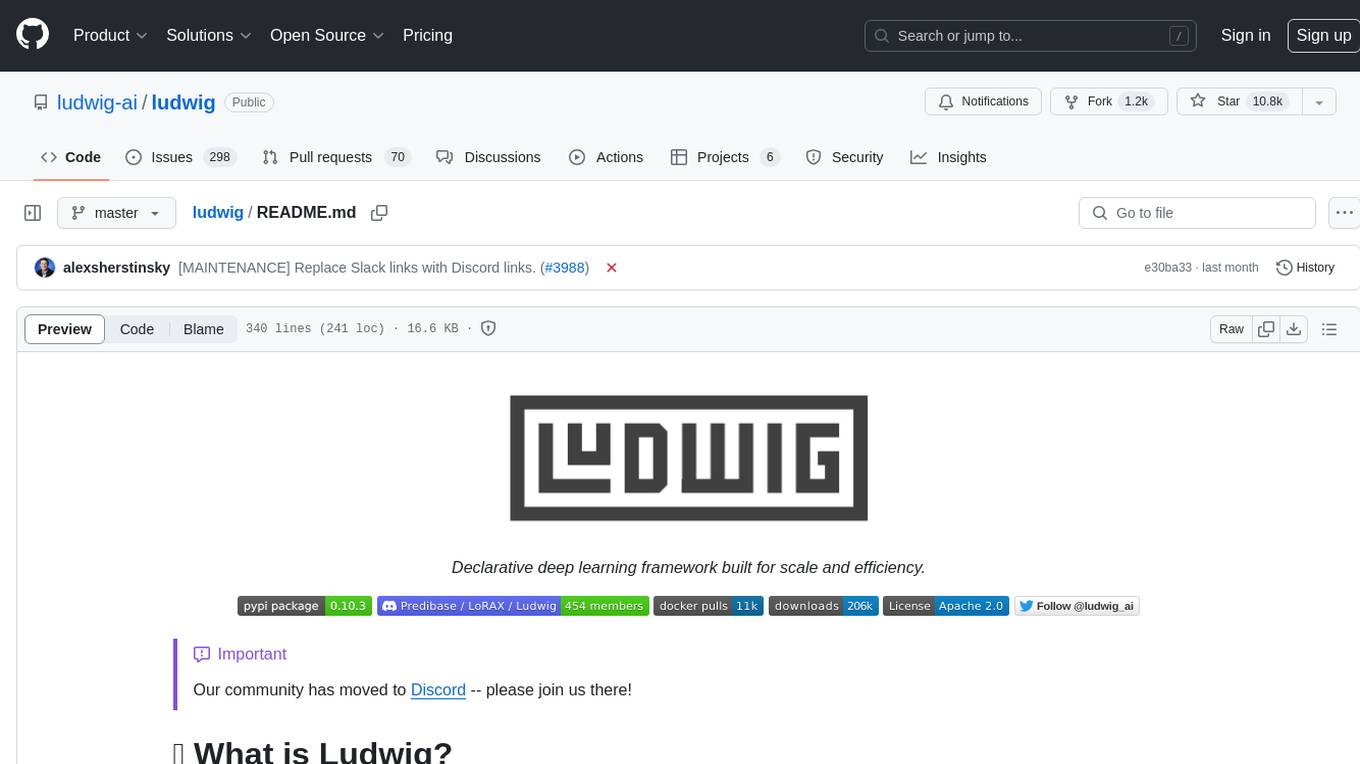
ludwig
Ludwig is a declarative deep learning framework designed for scale and efficiency. It is a low-code framework that allows users to build custom AI models like LLMs and other deep neural networks with ease. Ludwig offers features such as optimized scale and efficiency, expert level control, modularity, and extensibility. It is engineered for production with prebuilt Docker containers, support for running with Ray on Kubernetes, and the ability to export models to Torchscript and Triton. Ludwig is hosted by the Linux Foundation AI & Data.
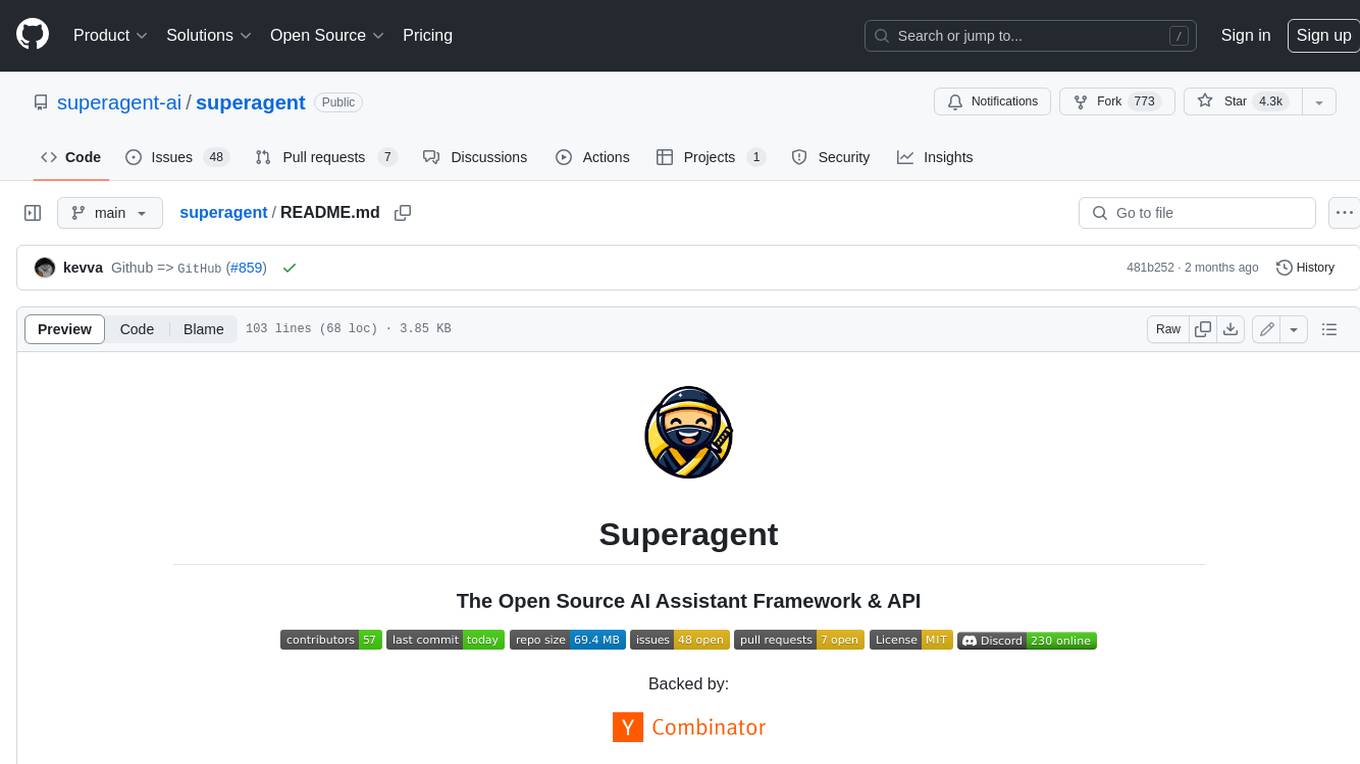
superagent
Superagent is an open-source AI assistant framework and API that allows developers to add powerful AI assistants to their applications. These assistants use large language models (LLMs), retrieval augmented generation (RAG), and generative AI to help users with a variety of tasks, including question answering, chatbot development, content generation, data aggregation, and workflow automation. Superagent is backed by Y Combinator and is part of YC W24.
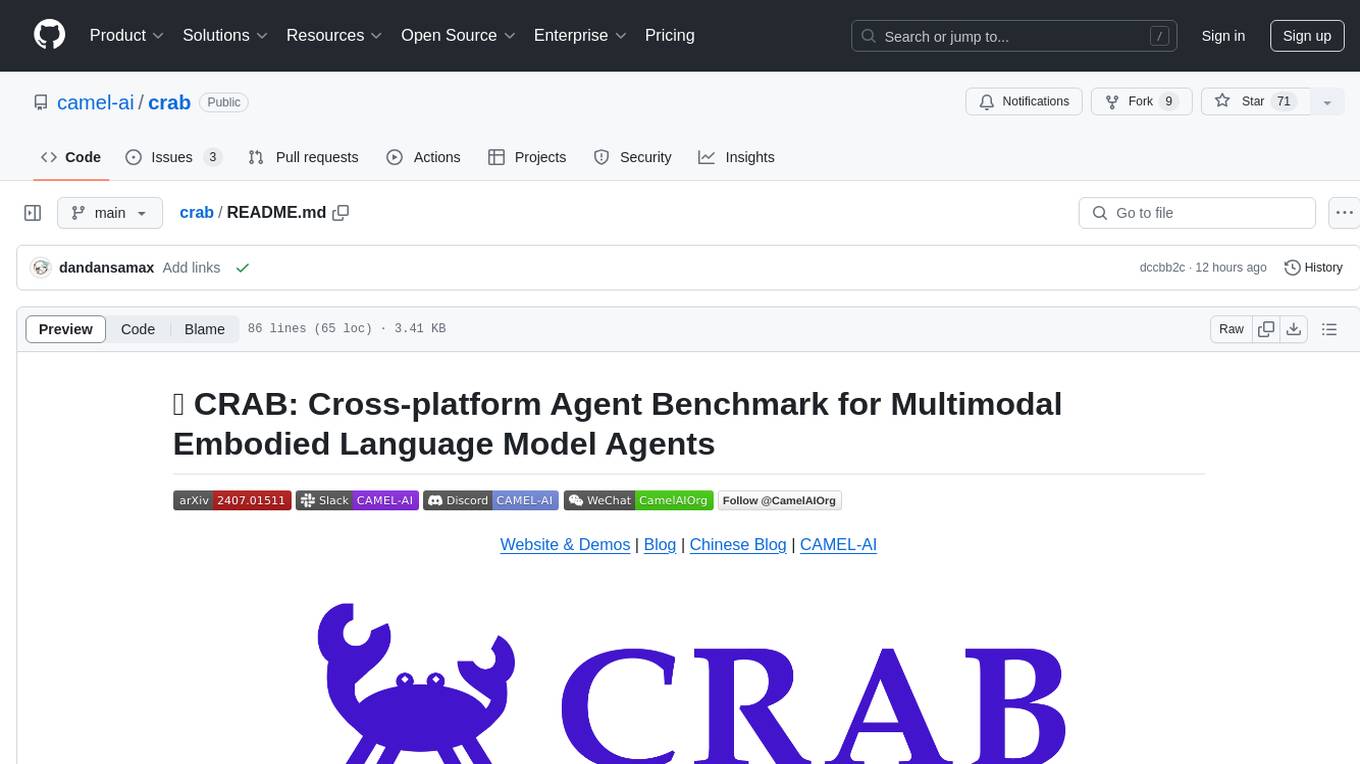
crab
CRAB is a framework for building LLM agent benchmark environments in a Python-centric way. It is cross-platform and multi-environment, allowing the creation of agent environments supporting various deployment options. The framework offers easy-to-use configuration with the ability to add new actions and define environments seamlessly. CRAB also provides a novel benchmarking suite with tasks and evaluators defined in Python, along with a unique graph evaluator method for detailed metrics.
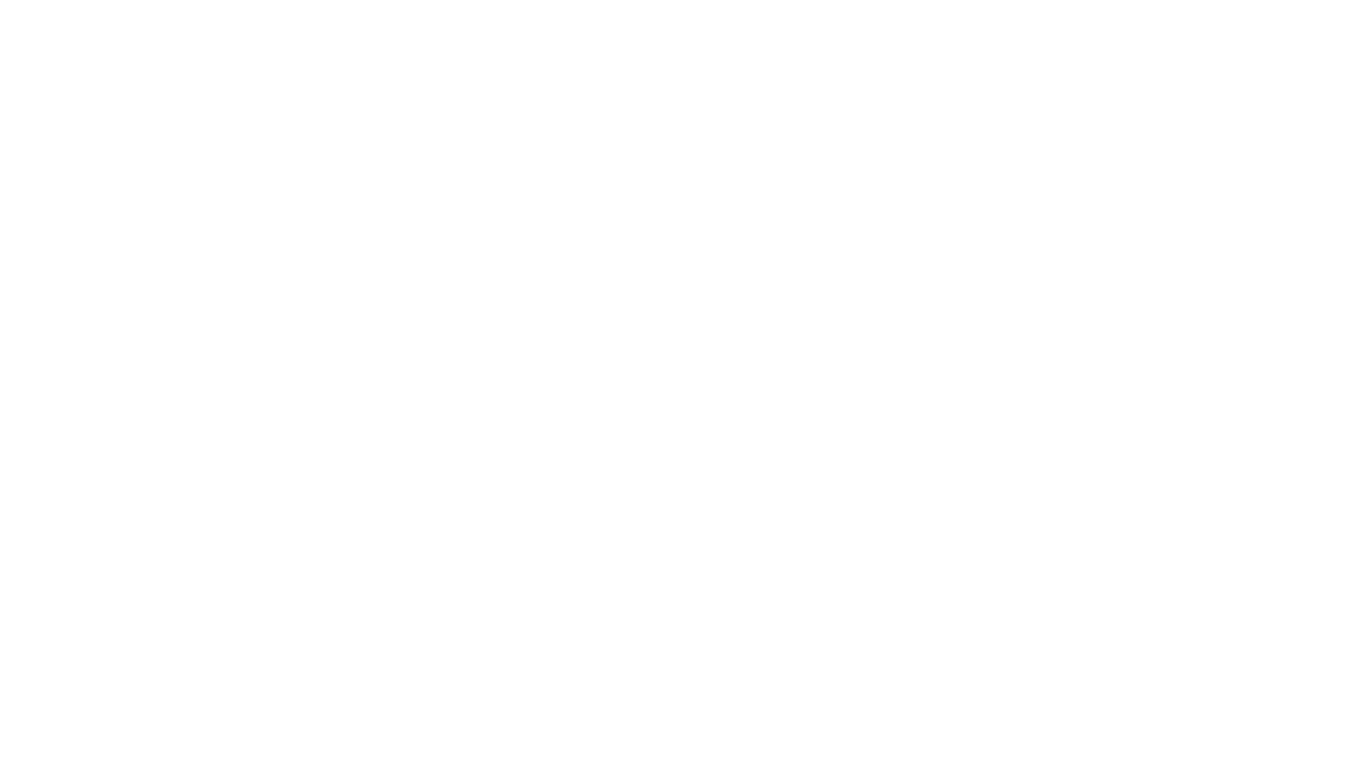
genai-os
Kuwa GenAI OS is an open, free, secure, and privacy-focused Generative-AI Operating System. It provides a multi-lingual turnkey solution for GenAI development and deployment on Linux and Windows. Users can enjoy features such as concurrent multi-chat, quoting, full prompt-list import/export/share, and flexible orchestration of prompts, RAGs, bots, models, and hardware/GPUs. The system supports various environments from virtual hosts to cloud, and it is open source, allowing developers to contribute and customize according to their needs.
For similar tasks
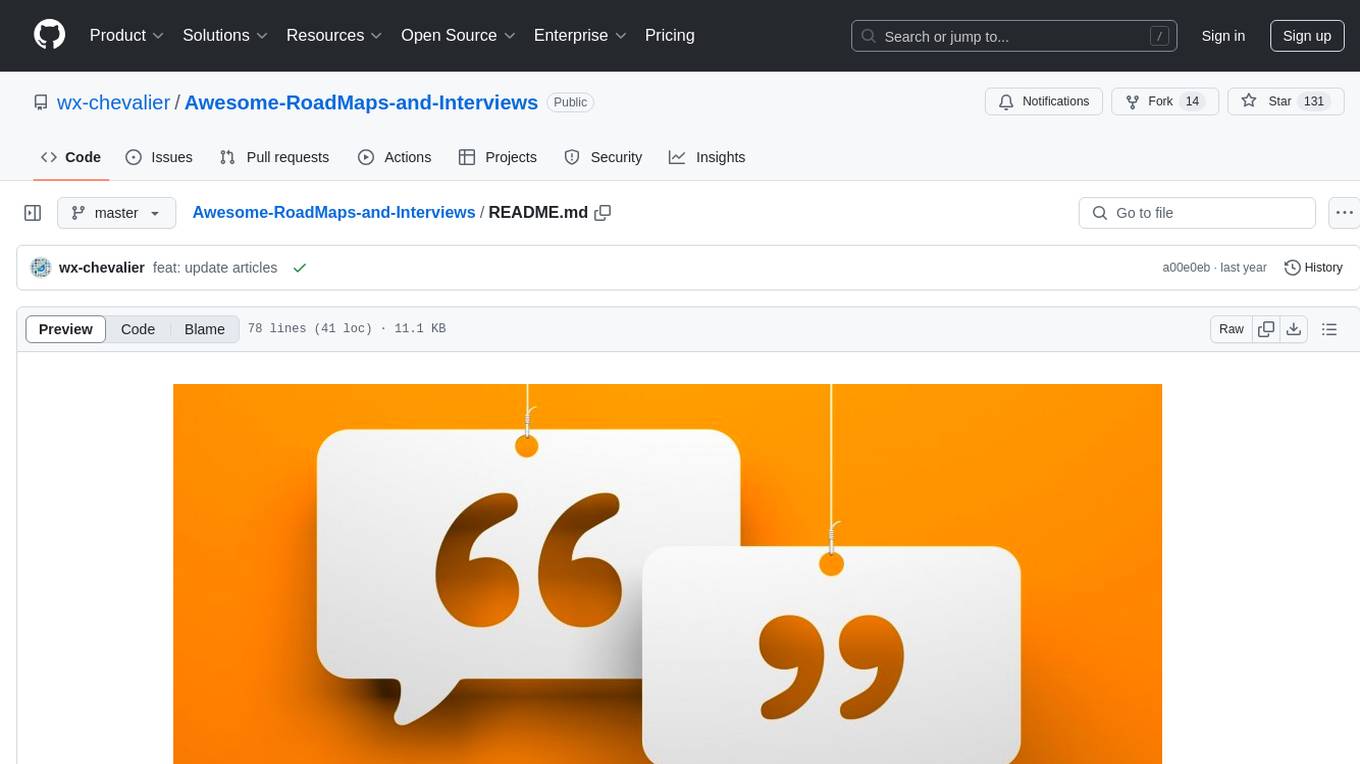
Awesome-RoadMaps-and-Interviews
Awesome RoadMaps and Interviews is a comprehensive repository that aims to provide guidance for technical interviews and career development in the ITCS field. It covers a wide range of topics including interview strategies, technical knowledge, and practical insights gained from years of interviewing experience. The repository emphasizes the importance of combining theoretical knowledge with practical application, and encourages users to expand their interview preparation beyond just algorithms. It also offers resources for enhancing knowledge breadth, depth, and programming skills through curated roadmaps, mind maps, cheat sheets, and coding snippets. The content is structured to help individuals navigate various technical roles and technologies, fostering continuous learning and professional growth.
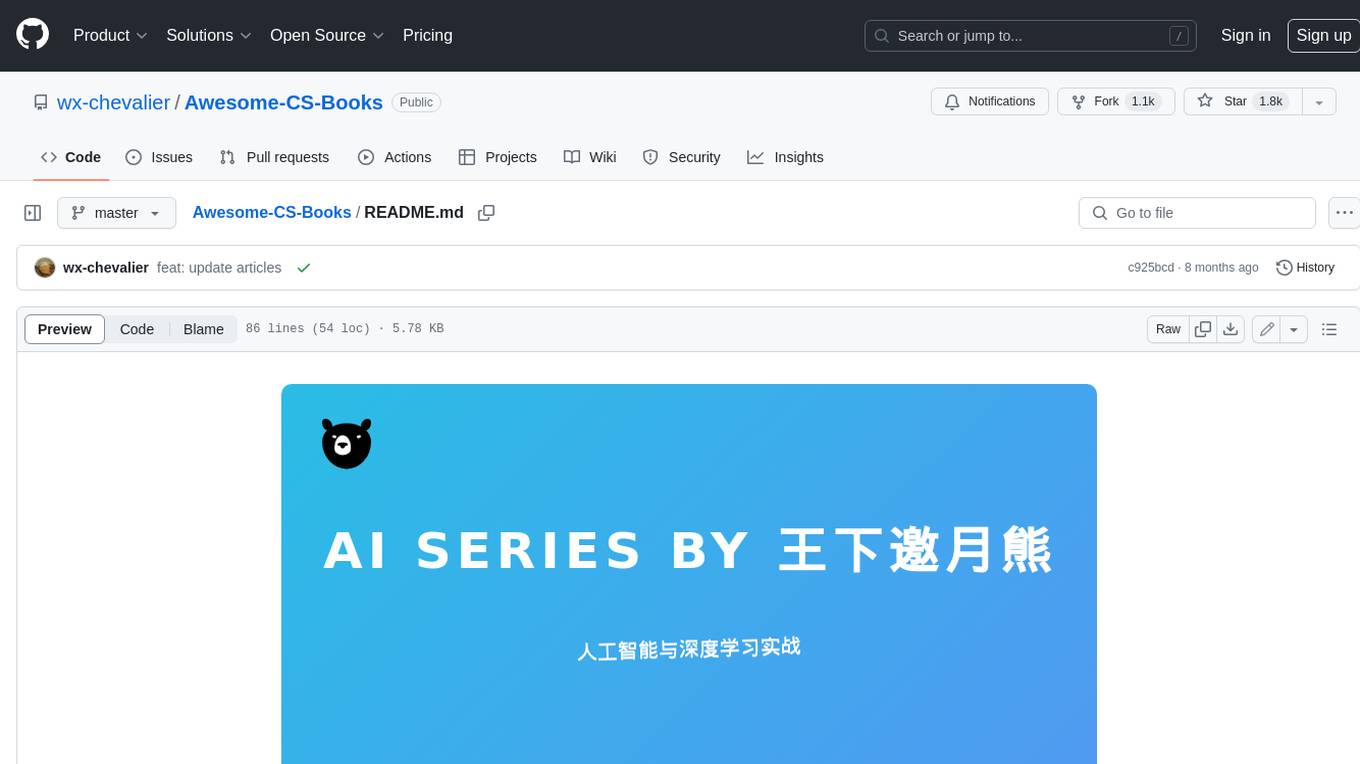
Awesome-CS-Books
Awesome CS Books is a curated list of books on computer science and technology. The books are organized by topic, including programming languages, software engineering, computer networks, operating systems, databases, data structures and algorithms, big data, architecture, and interviews. The books are available in PDF format and can be downloaded for free. The repository also includes links to free online courses and other resources.
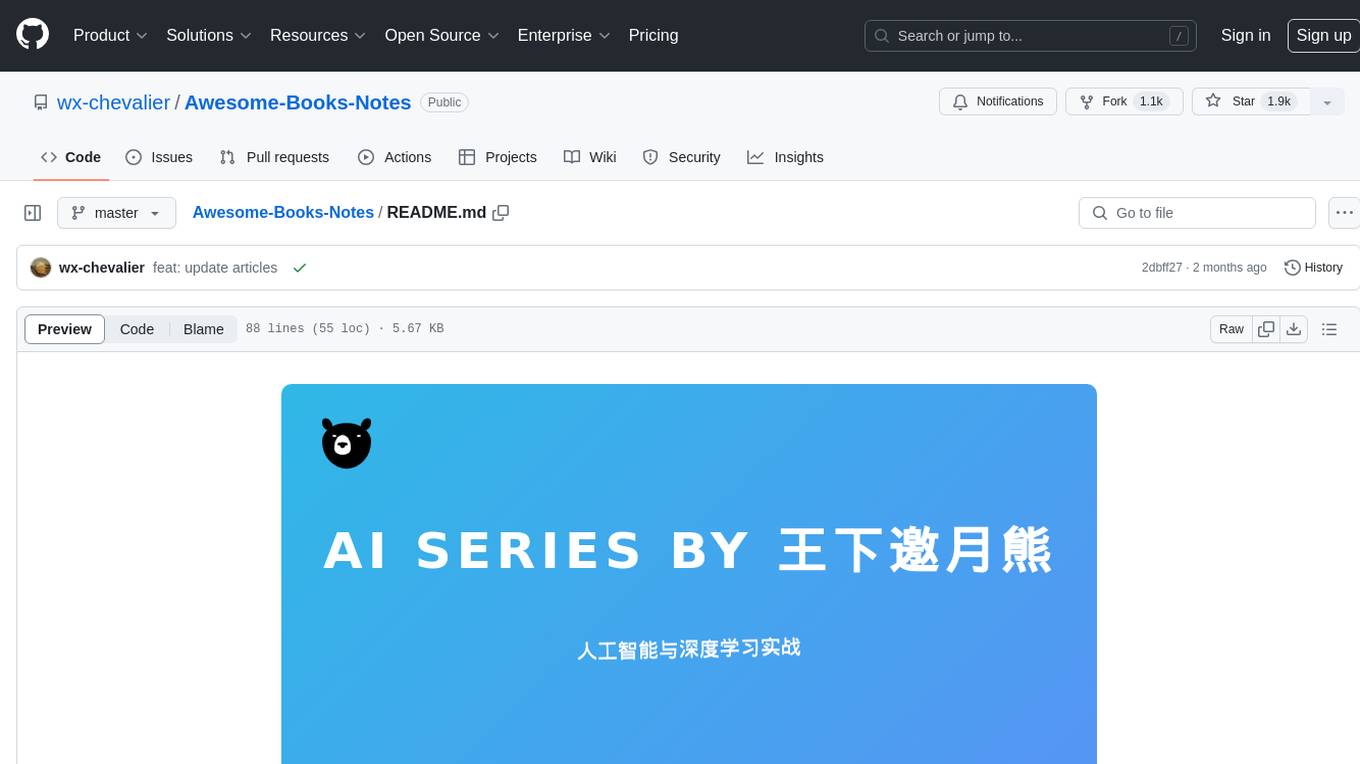
Awesome-Books-Notes
Awesome CS Books is a repository that archives excellent books related to computer science and technology, named in the format of {year}-{author}-{title}-{version}. It includes reading notes for each book, with PDF links provided at the beginning of the notes. The repository focuses on IT CS-related books, valuable open courses, and aims to provide a systematic way of learning to alleviate fragmented skills and one-sidedness. It respects the original authors by linking to official/copyright websites and emphasizes non-commercial use of the documents.
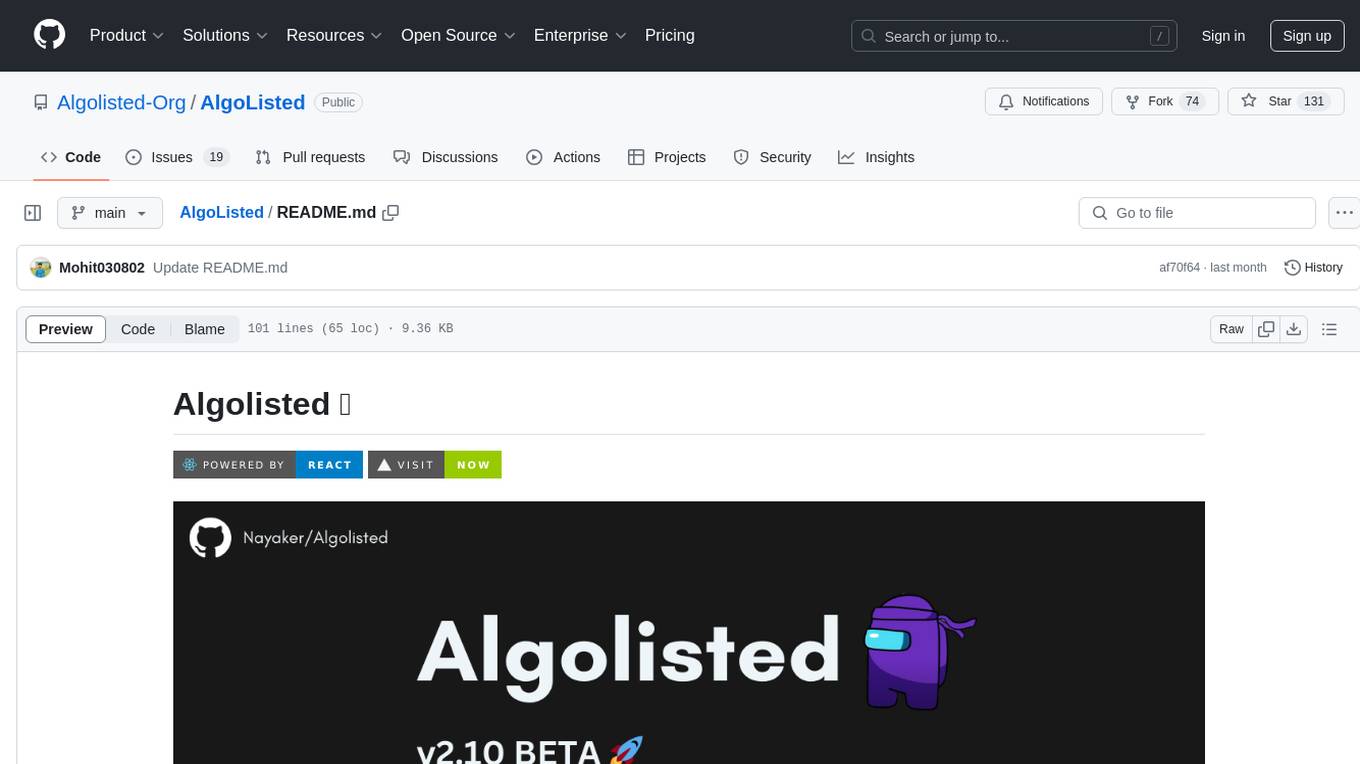
AlgoListed
Algolisted is a pioneering platform dedicated to algorithmic problem-solving, offering a centralized hub for a diverse array of algorithmic challenges. It provides an immersive online environment for programmers to enhance their skills through Data Structures and Algorithms (DSA) sheets, academic progress tracking, resume refinement with OpenAI integration, adaptive testing, and job opportunity listings. The project is built on the MERN stack, Flask, Beautiful Soup, and Selenium,GEN AI, and deployed on Firebase. Algolisted aims to be a reliable companion in the pursuit of coding knowledge and proficiency.
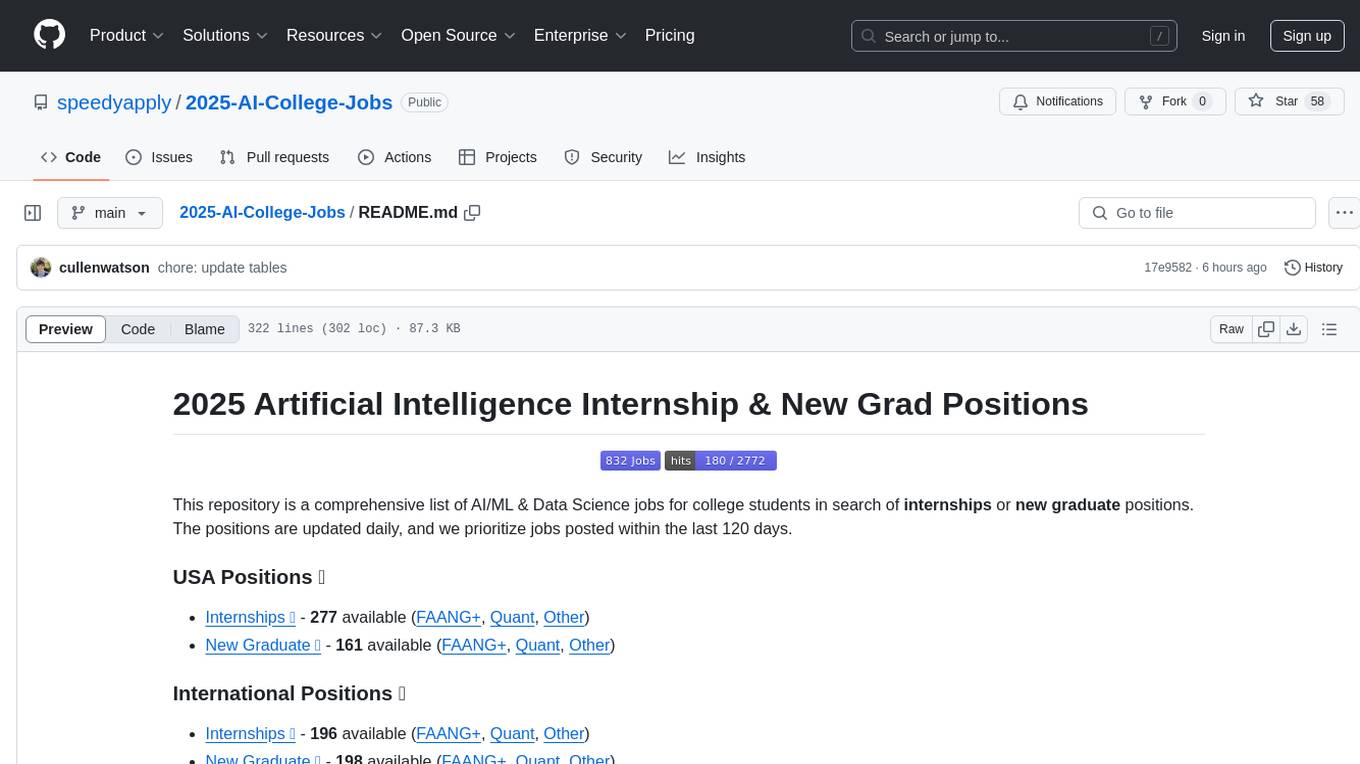
2025-AI-College-Jobs
2025-AI-College-Jobs is a repository containing a comprehensive list of AI/ML & Data Science jobs suitable for college students seeking internships or new graduate positions. The repository is regularly updated with positions posted within the last 120 days, featuring opportunities from various companies in the USA and internationally. The list includes positions in areas such as research scientist internships, quantitative research analyst roles, and other data science-related positions. The repository aims to provide a valuable resource for students looking to kickstart their careers in the field of artificial intelligence and machine learning.
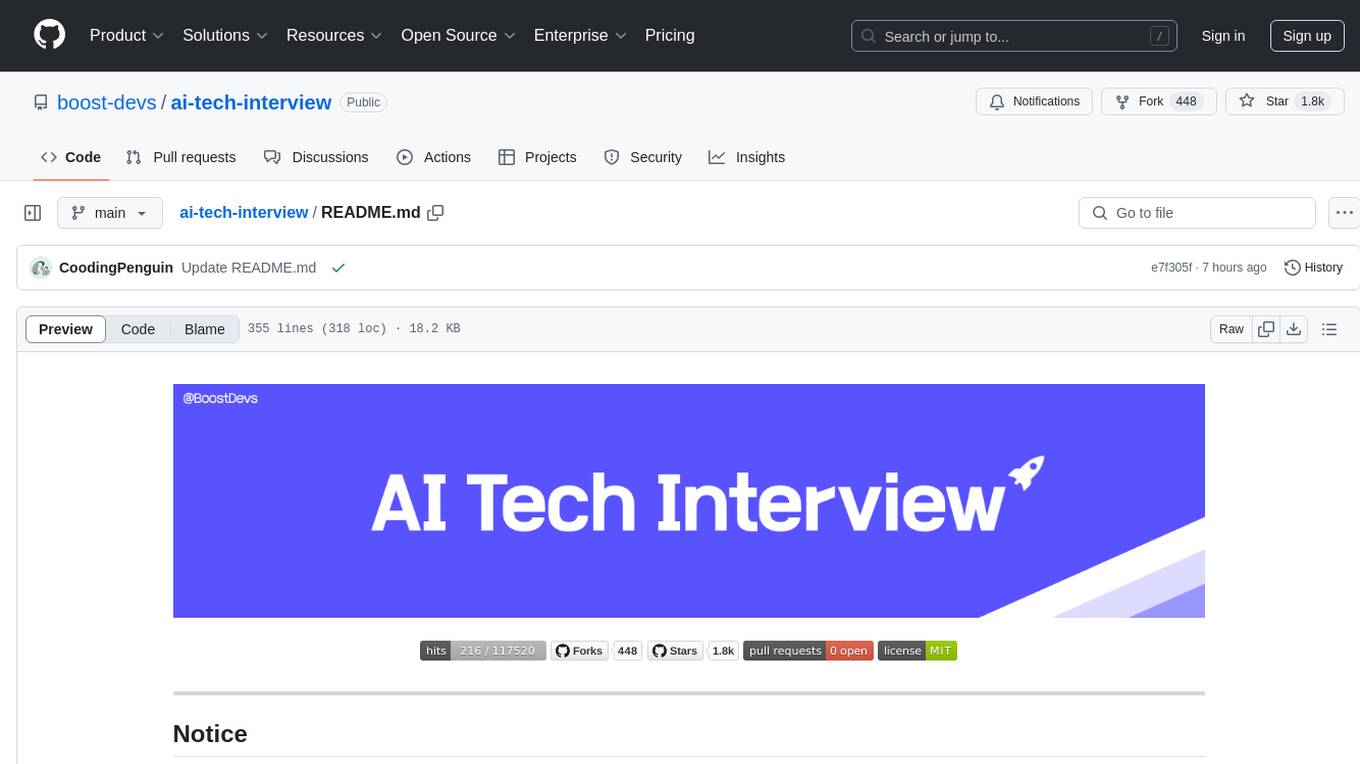
ai-tech-interview
This repository contains a collection of interview questions related to various topics such as statistics, machine learning, deep learning, Python, networking, operating systems, data structures, and algorithms. The questions cover a wide range of concepts and are suitable for individuals preparing for technical interviews in the field of artificial intelligence and data science.
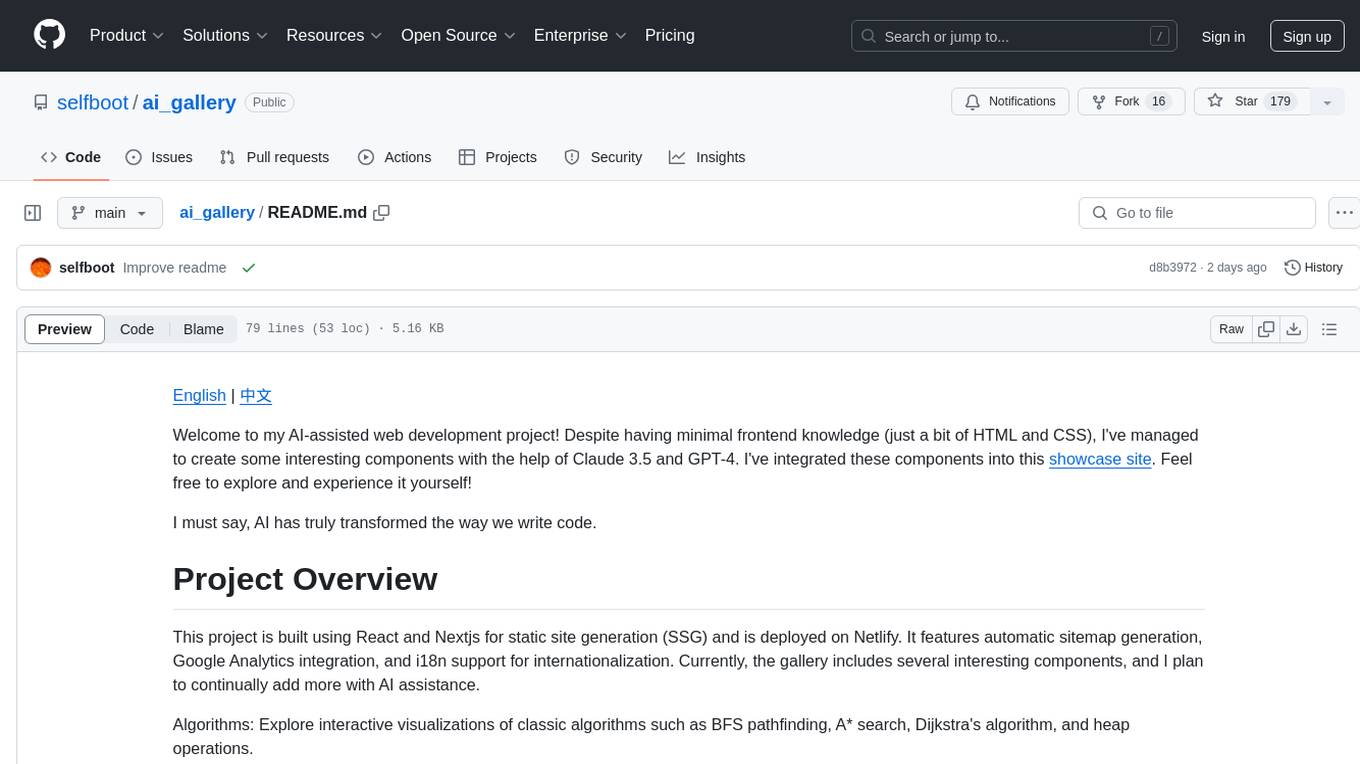
ai_gallery
AI Gallery is a showcase site built using React and Nextjs for static site generation, featuring interactive visualizations of classic algorithms, classic games implementation, and various interesting widgets. The project utilizes AI assistance from Claude 3.5 and GPT-4 to create components and enhance the development process. It aims to continually add more components with AI assistance, providing a platform for contributors to leverage AI in frontend development.
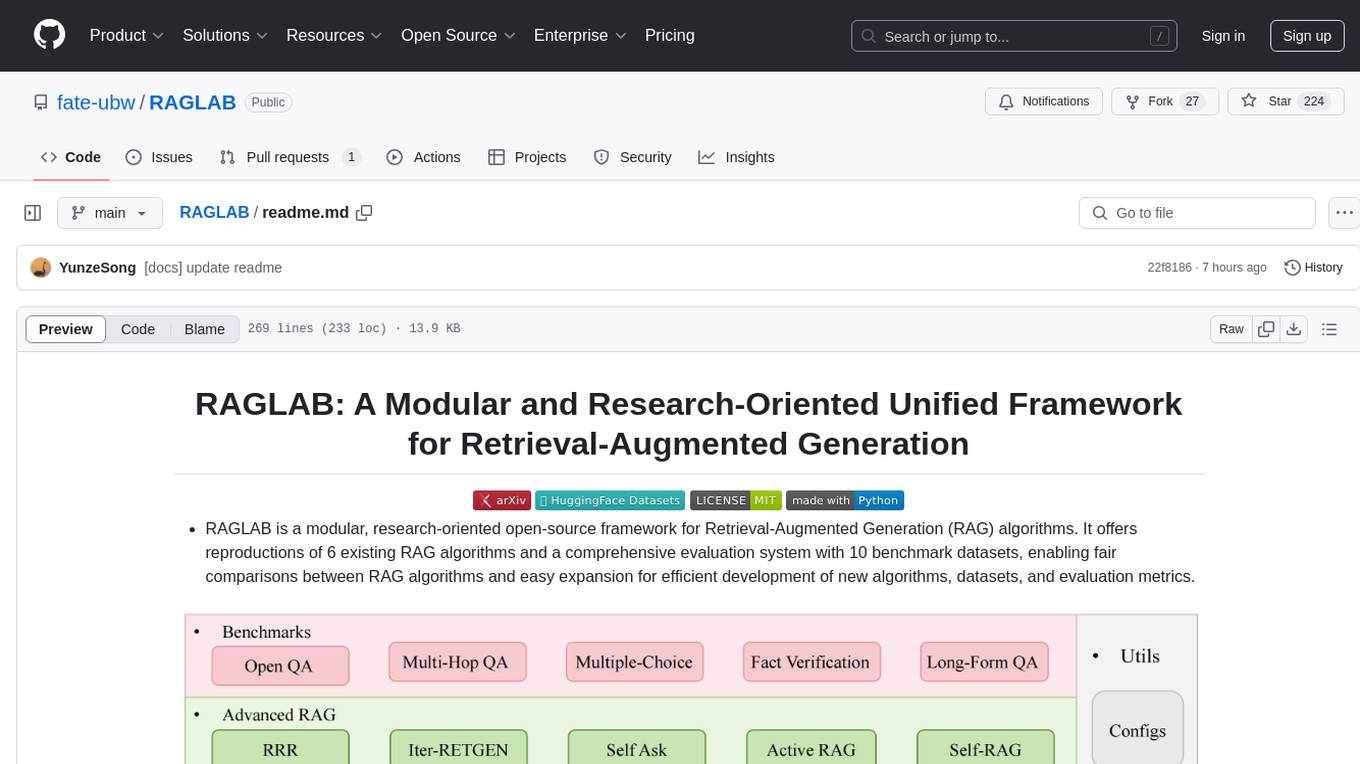
RAGLAB
RAGLAB is a modular, research-oriented open-source framework for Retrieval-Augmented Generation (RAG) algorithms. It offers reproductions of 6 existing RAG algorithms and a comprehensive evaluation system with 10 benchmark datasets, enabling fair comparisons between RAG algorithms and easy expansion for efficient development of new algorithms, datasets, and evaluation metrics. The framework supports the entire RAG pipeline, provides advanced algorithm implementations, fair comparison platform, efficient retriever client, versatile generator support, and flexible instruction lab. It also includes features like Interact Mode for quick understanding of algorithms and Evaluation Mode for reproducing paper results and scientific research.
For similar jobs
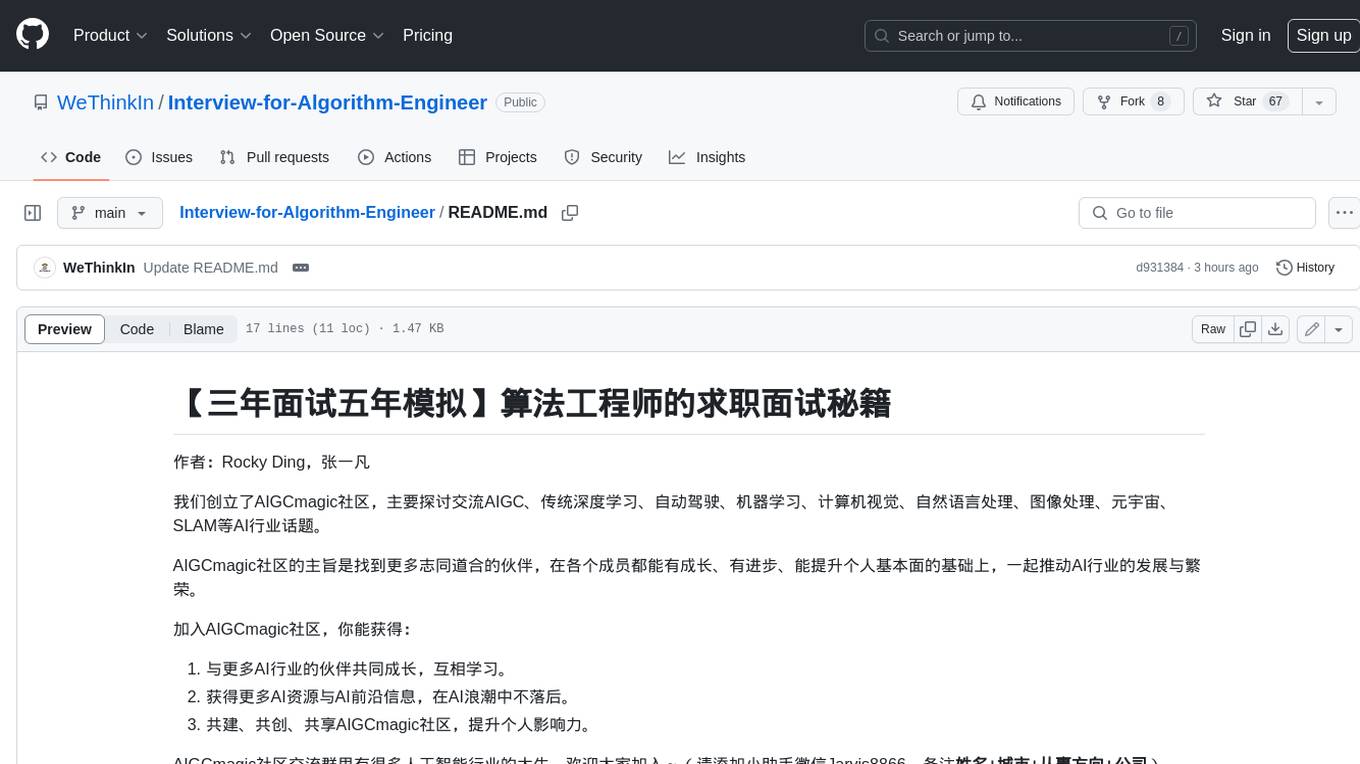
Interview-for-Algorithm-Engineer
This repository provides a collection of interview questions and answers for algorithm engineers. The questions are organized by topic, and each question includes a detailed explanation of the answer. This repository is a valuable resource for anyone preparing for an algorithm engineering interview.
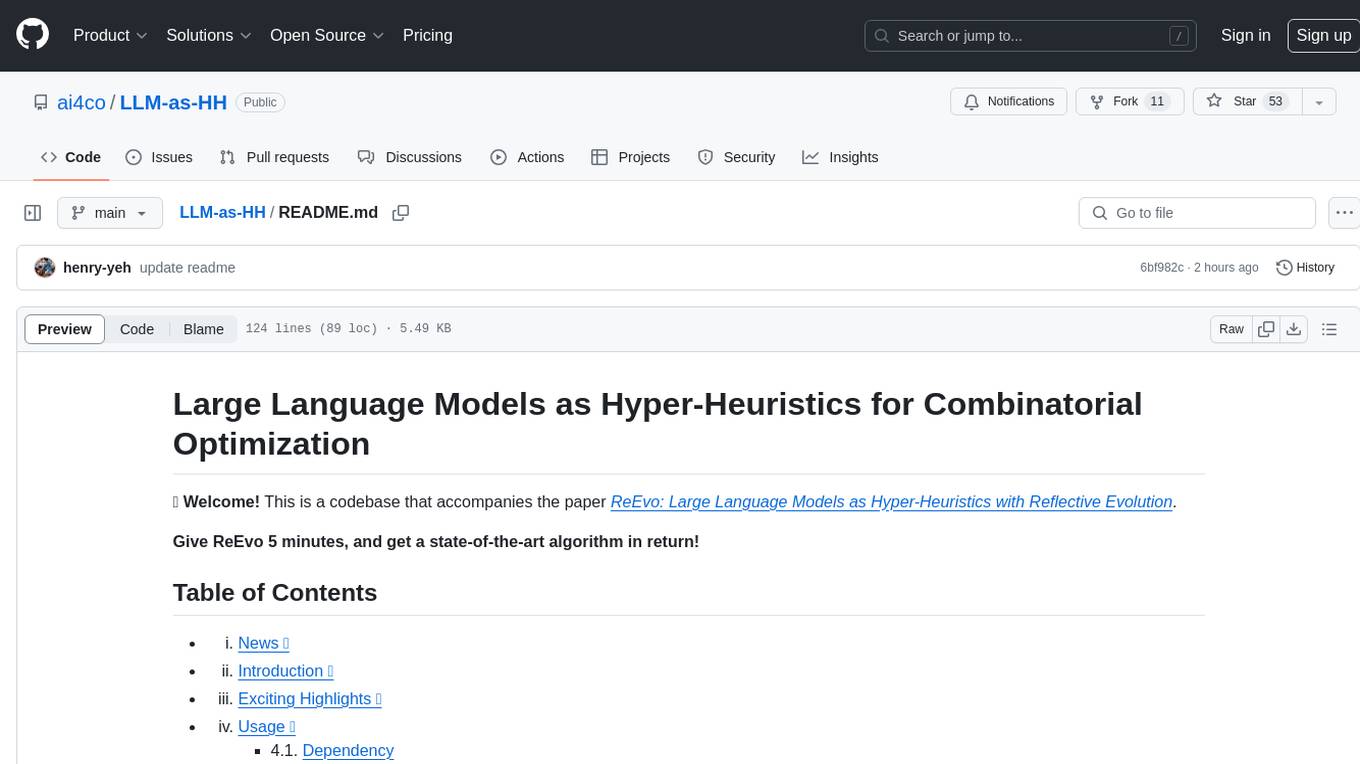
LLM-as-HH
LLM-as-HH is a codebase that accompanies the paper ReEvo: Large Language Models as Hyper-Heuristics with Reflective Evolution. It introduces Language Hyper-Heuristics (LHHs) that leverage LLMs for heuristic generation with minimal manual intervention and open-ended heuristic spaces. Reflective Evolution (ReEvo) is presented as a searching framework that emulates the reflective design approach of human experts while surpassing human capabilities with scalable LLM inference, Internet-scale domain knowledge, and powerful evolutionary search. The tool can improve various algorithms on problems like Traveling Salesman Problem, Capacitated Vehicle Routing Problem, Orienteering Problem, Multiple Knapsack Problems, Bin Packing Problem, and Decap Placement Problem in both black-box and white-box settings.
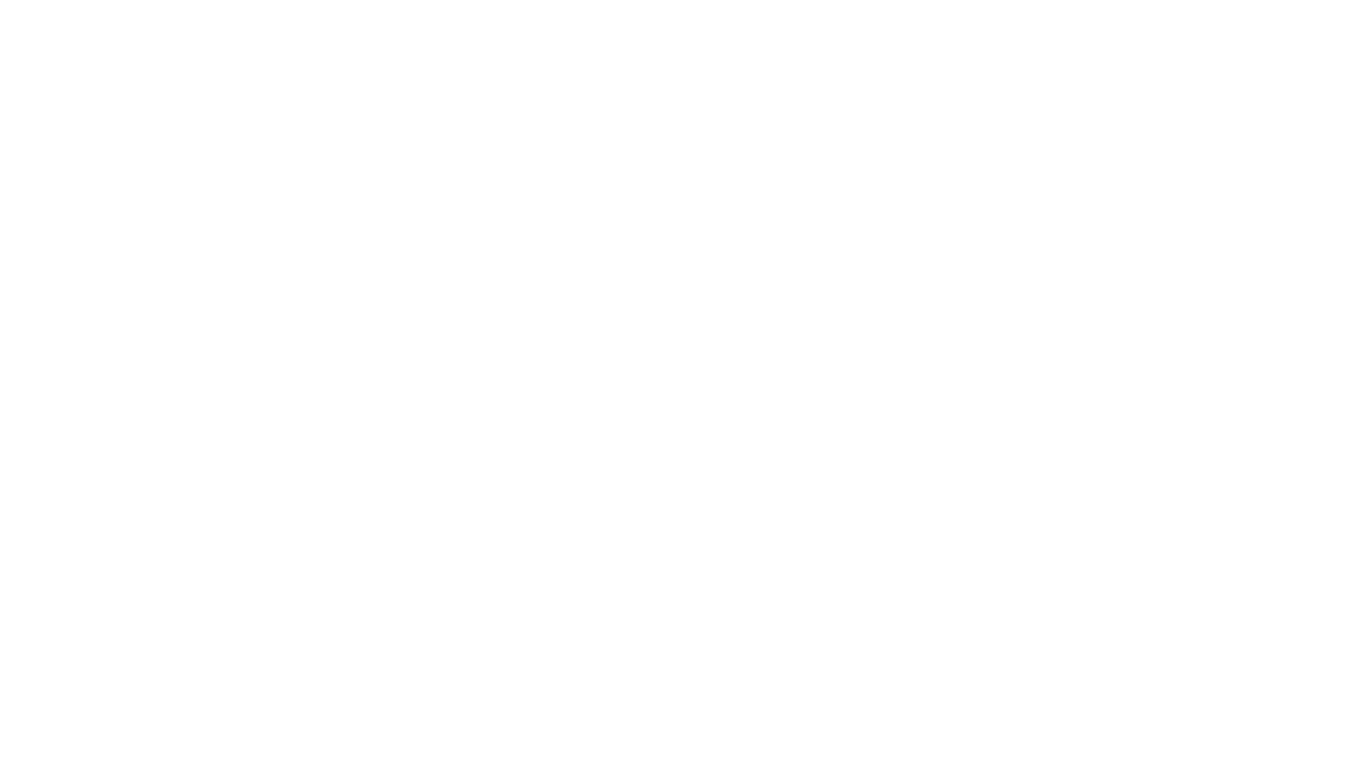
universal
The Universal Numbers Library is a header-only C++ template library designed for universal number arithmetic, offering alternatives to native integer and floating-point for mixed-precision algorithm development and optimization. It tailors arithmetic types to the application's precision and dynamic range, enabling improved application performance and energy efficiency. The library provides fast implementations of special IEEE-754 formats like quarter precision, half-precision, and quad precision, as well as vendor-specific extensions. It supports static and elastic integers, decimals, fixed-points, rationals, linear floats, tapered floats, logarithmic, interval, and adaptive-precision integers, rationals, and floats. The library is suitable for AI, DSP, HPC, and HFT algorithms.
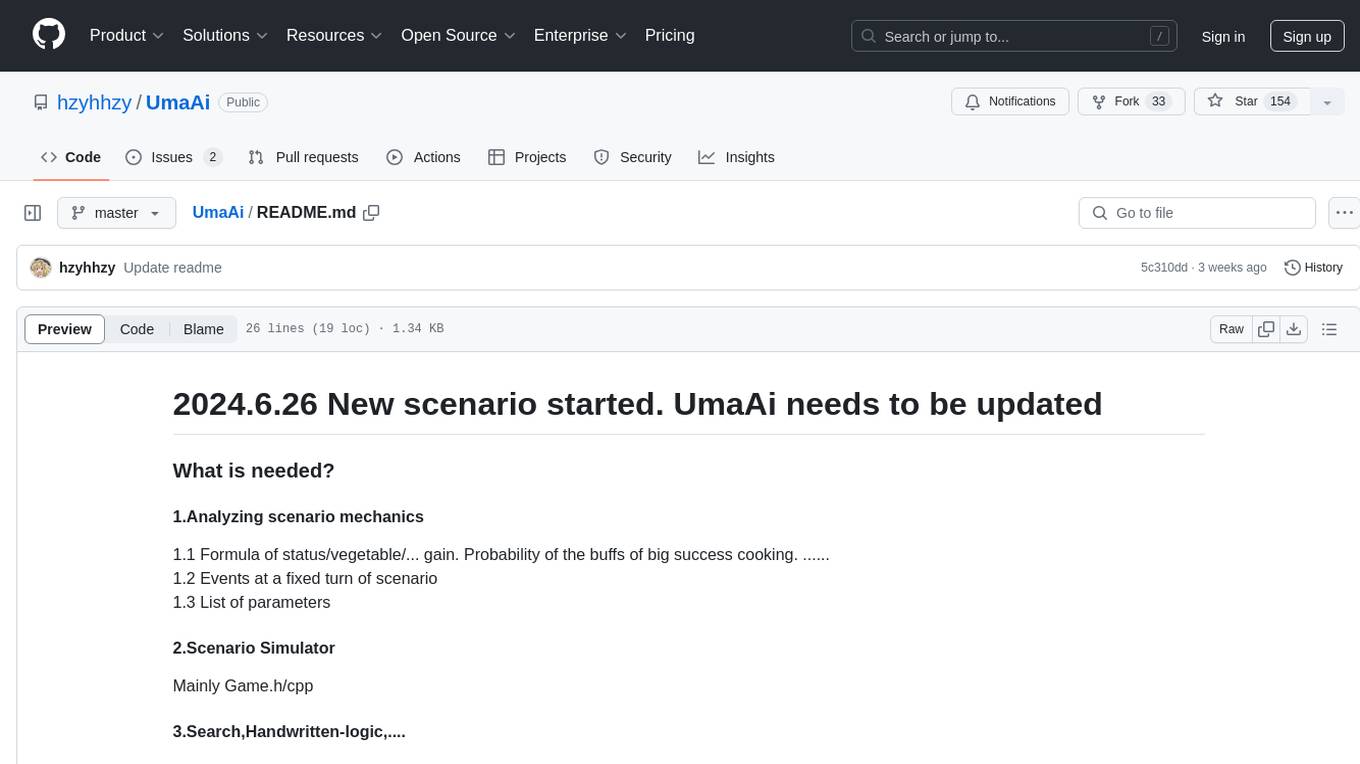
UmaAi
UmaAi is a tool designed for algorithm learning purposes, specifically focused on analyzing scenario mechanics in a game. It provides functionalities such as simulating scenarios, searching, handwritten-logic, and OCR integration. The tool allows users to modify settings in config.h for evaluating cardset strength, simulating games, and understanding game mechanisms through the source code. It emphasizes that it should not be used for illegal purposes and is intended for educational use only.
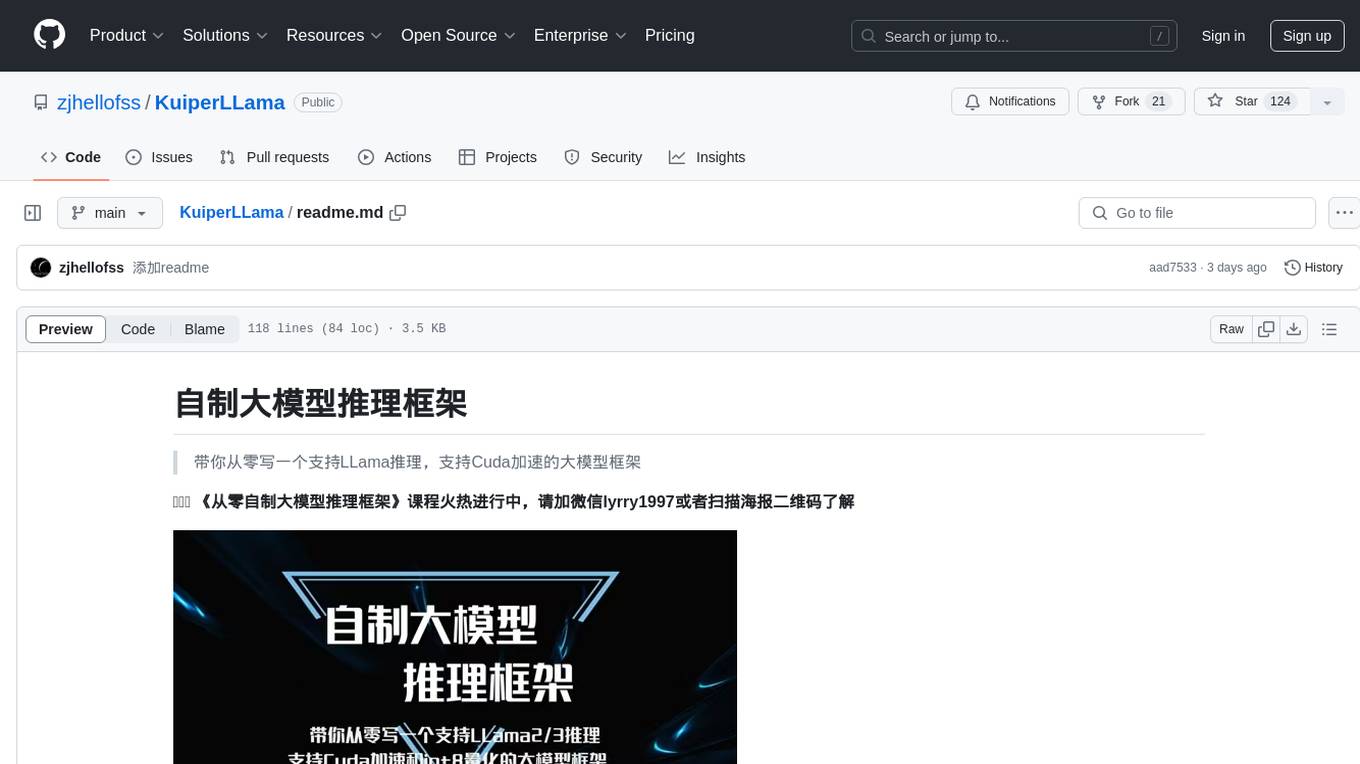
KuiperLLama
KuiperLLama is a custom large model inference framework that guides users in building a LLama-supported inference framework with Cuda acceleration from scratch. The framework includes modules for architecture design, LLama2 model support, model quantization, Cuda basics, operator implementation, and fun tasks like text generation and storytelling. It also covers learning other commercial inference frameworks for comprehensive understanding. The project provides detailed tutorials and resources for developing and optimizing large models for efficient inference.
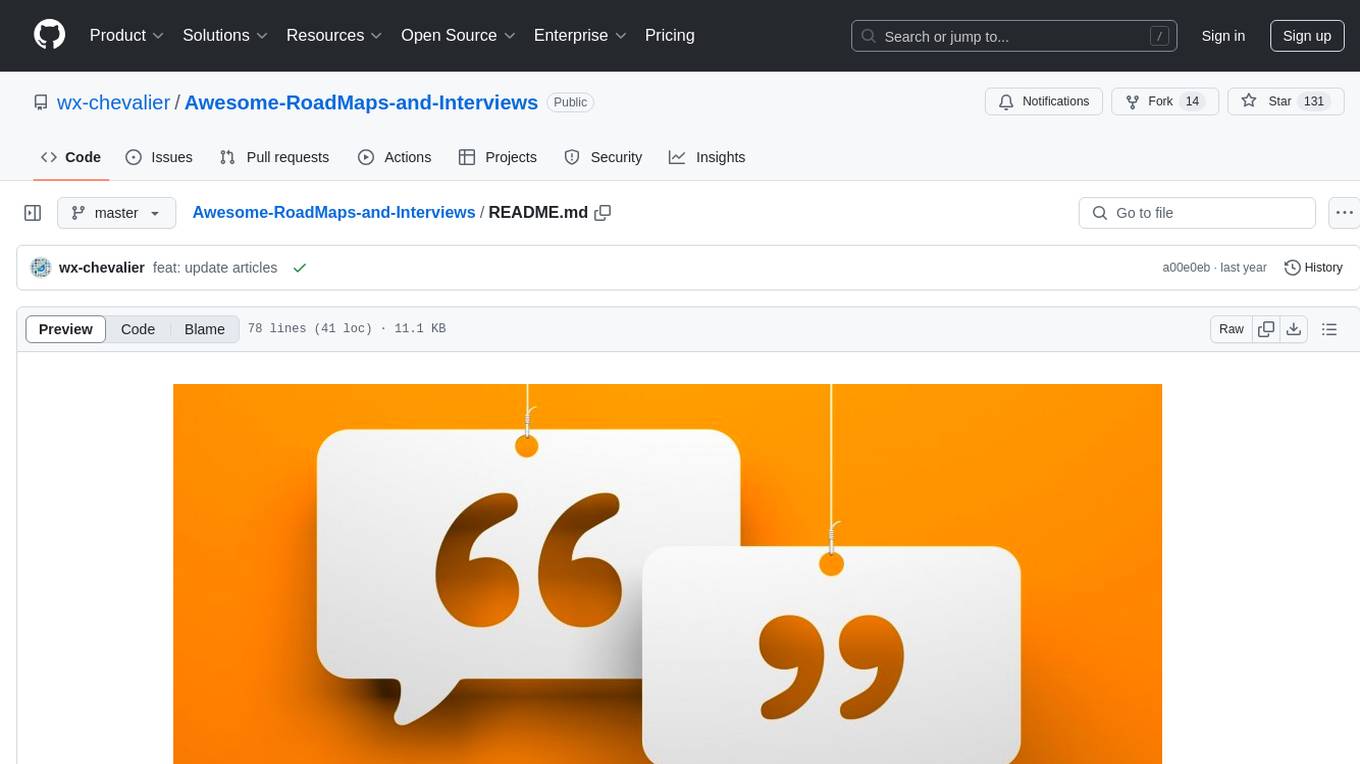
Awesome-RoadMaps-and-Interviews
Awesome RoadMaps and Interviews is a comprehensive repository that aims to provide guidance for technical interviews and career development in the ITCS field. It covers a wide range of topics including interview strategies, technical knowledge, and practical insights gained from years of interviewing experience. The repository emphasizes the importance of combining theoretical knowledge with practical application, and encourages users to expand their interview preparation beyond just algorithms. It also offers resources for enhancing knowledge breadth, depth, and programming skills through curated roadmaps, mind maps, cheat sheets, and coding snippets. The content is structured to help individuals navigate various technical roles and technologies, fostering continuous learning and professional growth.
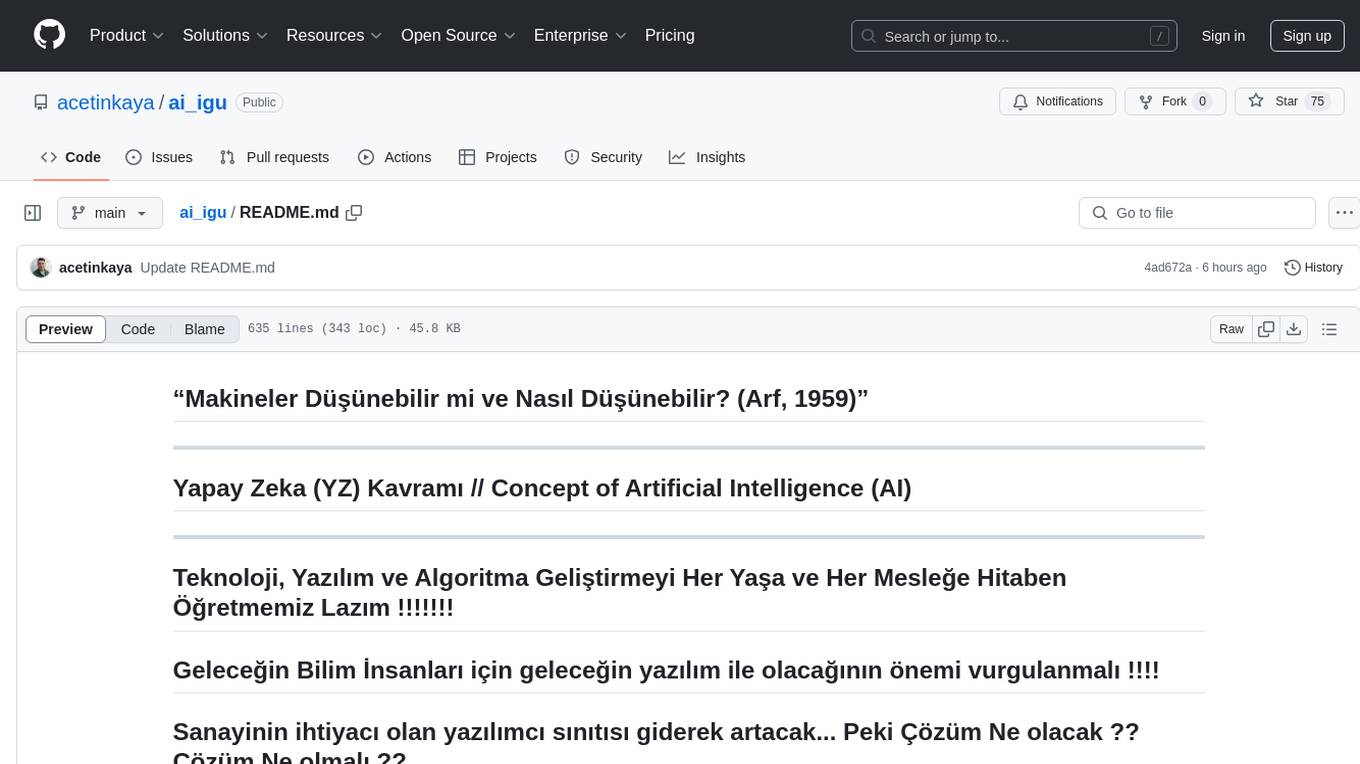
ai_igu
AI-IGU is a GitHub repository focused on Artificial Intelligence (AI) concepts, technology, software development, and algorithm improvement for all ages and professions. It emphasizes the importance of future software for future scientists and the increasing need for software developers in the industry. The repository covers various topics related to AI, including machine learning, deep learning, data mining, data science, big data, and more. It provides educational materials, practical examples, and hands-on projects to enhance software development skills and create awareness in the field of AI.
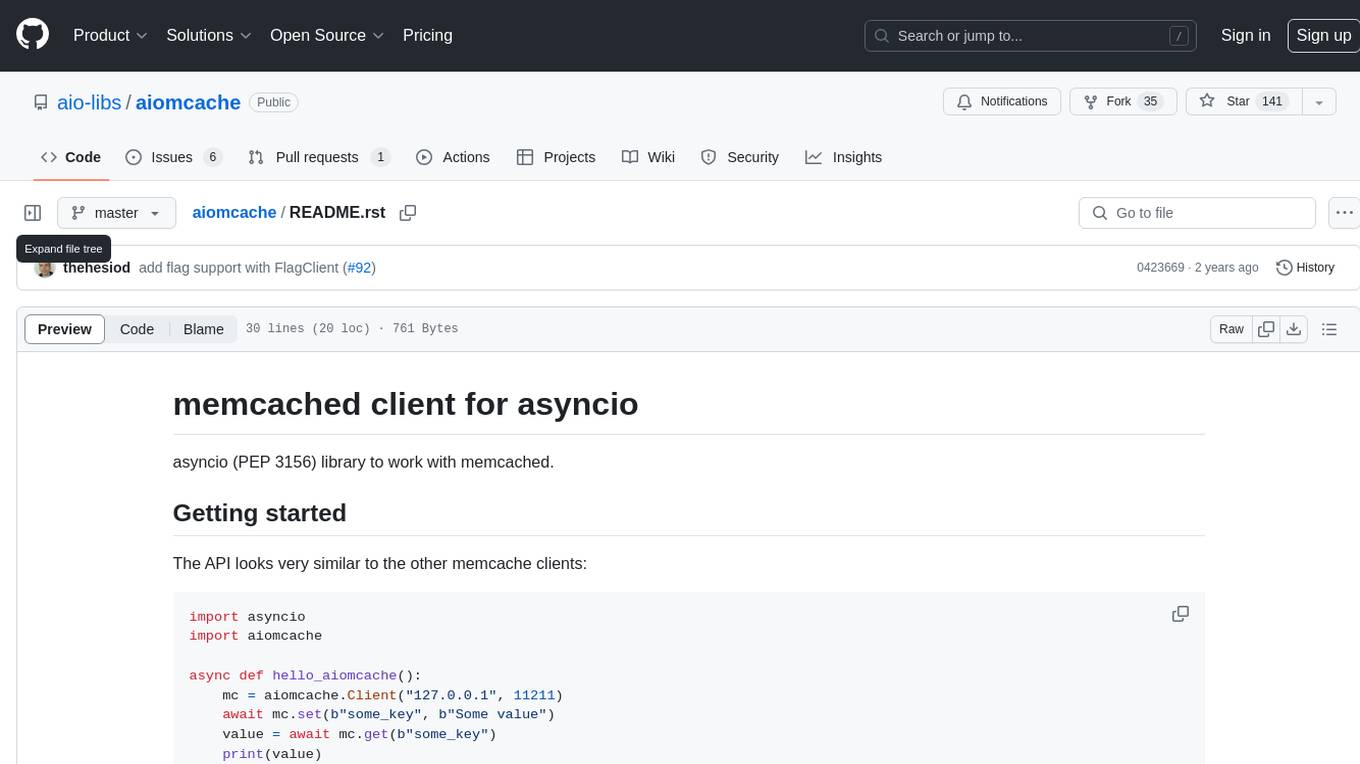
aiomcache
aiomcache is a Python library that provides an asyncio (PEP 3156) interface to work with memcached. It allows users to interact with memcached servers asynchronously, making it suitable for high-performance applications that require non-blocking I/O operations. The library offers similar functionality to other memcache clients and includes features like setting and getting values, multi-get operations, and deleting keys. Version 0.8 introduces the `FlagClient` class, which enables users to register callbacks for setting or processing flags, providing additional flexibility and customization options for working with memcached servers.