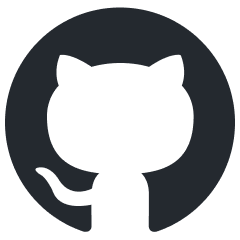
LLM-as-HH
Large Language Models as Hyper-Heuristics for Combinatorial Optimization (CO)
Stars: 78
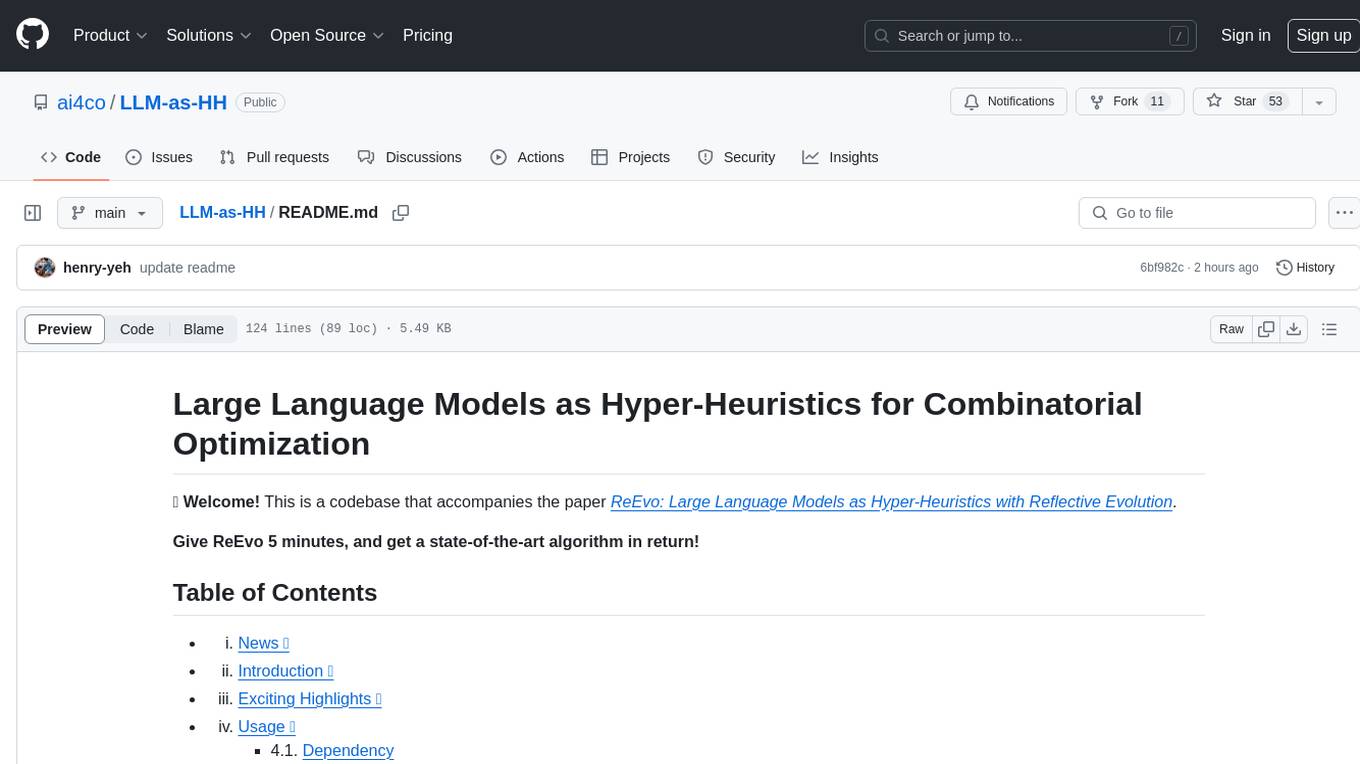
LLM-as-HH is a codebase that accompanies the paper ReEvo: Large Language Models as Hyper-Heuristics with Reflective Evolution. It introduces Language Hyper-Heuristics (LHHs) that leverage LLMs for heuristic generation with minimal manual intervention and open-ended heuristic spaces. Reflective Evolution (ReEvo) is presented as a searching framework that emulates the reflective design approach of human experts while surpassing human capabilities with scalable LLM inference, Internet-scale domain knowledge, and powerful evolutionary search. The tool can improve various algorithms on problems like Traveling Salesman Problem, Capacitated Vehicle Routing Problem, Orienteering Problem, Multiple Knapsack Problems, Bin Packing Problem, and Decap Placement Problem in both black-box and white-box settings.
README:
🥳 Welcome! This is a codebase that accompanies the paper Large Language Models as Hyper-Heuristics for Combinatorial Optimization.
Give ReEvo 5 minutes, and get a state-of-the-art algorithm in return!
- 1. News 📰
- 2. Introduction 🚀
- 3. Exciting Highlights 🌟
- 4. Usage 🔑
- 4.1. Dependency
- 4.2. To run ReEvo
- 4.3. Available problems
- 4.4. Simple steps to apply ReEvo to your problem
- 4.5. Use Alternative LLMs
- 5. Citation 🤩
- 6. Acknowledgments 🫡
- 2024.05: We release a new paper version.
- 2024.04: Novel use cases for Neural Combinatorial Optimization (NCO) and Electronic Design Automation (EDA).
- 2024.02: We are excited to release ReEvo! 🚀
We introduce Language Hyper-Heuristics (LHHs), an emerging variant of Hyper-Heuristics (HHs) that leverages LLMs for heuristic generation, featuring minimal manual intervention and open-ended heuristic spaces.
To empower LHHs, we present Reflective Evolution (ReEvo), a generic searching framework that emulates the reflective design approach of human experts while much surpassing human capabilities with its scalable LLM inference, Internet-scale domain knowledge, and powerful evolutionary search.
We can improve the following types of algorithms:
- Neural Combinatorial Optimization (NCO)
- Genetic Algorithm (GA)
- Ant Colony Optimization (ACO)
- Guided Local Search (GLS)
- Constructive Heuristics
on the following problems:
- Traveling Salesman Problem (TSP)
- Capacitated Vehicle Routing Problem (CVRP)
- Orienteering Problem (OP)
- Multiple Knapsack Problems (MKP)
- Bin Packing Problem (BPP)
- Decap Placement Problem (DPP)
with both black-box and white-box settings.
- Set your LLM API key (OpenAI API, ZhiPu API, Llama API) here or as an environment variable.
- Running logs and intermediate results are saved in
./outputs/main/
by default. - Datasets are generated on the fly.
- Some test notebooks are provided in
./problems/*/test.ipynb
.
- Python >= 3.11
- openai >= 1.0.0
- hydra-core
- scipy
You may install the dependencies above via pip install -r requirements.txt
.
Problem-specific dependencies:
-
tsp_aco(_black_box)
: pytorch, scikit-learn -
cvrp_aco(_black_box)
/mkp_aco(_black_box)
/op_aco(_black_box)
/NCO
: pytorch -
tsp_gls
: numba==0.58
# e.g., for tsp_aco
python main.py problem=tsp_aco
Check out ./cfg/
for more options.
- Traveling Salesman Problem (TSP):
tsp_aco
,tsp_aco_black_box
,tsp_constructive
,tsp_gls
,tsp_pomo
,tsp_lehd
- Capacitated Vehicle Routing Problem (CVRP):
cvrp_aco
,cvrp_aco_black_box
,cvrp_pomo
,cvrp_lehd
- Bin Packing Problem (BPP):
bpp_offline_aco
,bpp_offline_aco_black_box
,bpp_online
- Multiple Knapsack Problems (MKP):
mkp_aco
,mkp_aco_black_box
- Orienteering Problem (OP):
op_aco
,op_aco_black_box
- Decap Placement Problem (DPP):
dpp_ga
- Define your problem in
./cfg/problem/
. - Generate problem instances and implement the evaluation pipeline in
./problems/
. - Add function_description, function_signature, and seed_function in
./prompts/
.
Use the cli parameter llm_client
to designate an LLM API provider, and llm_client.model
to determine the model to use. For example,
$ export LLAMA_API_KEY=xxxxxxxxxxxxxxxxxxxx
$ python main.py llm_client=llama_api llm_client.model=gemma2-9b
Supported LLM API providers and models including (to be noted that only chat models are supported):
- OpenAI: gpt-3.5-turbo (default), gpt-4o, gpt-4o-mini, gpt-4-turbo, etc.
- Zhipu AI: GLM-3-Turbo, GLM-4-Air, GLM-4-0520, etc. (full list)
- DeepSeek: deepseek-chat
- Llama API: llama3.1-8b/70b/405b, gemma2-9b/27b, Qwen2-72B, etc. (full list)
- And more providers supported via LiteLLM.
If you encounter any difficulty using our code, please do not hesitate to submit an issue or directly contact us! If you find our work helpful (or if you are so kind as to offer us some encouragement), please consider giving us a star, and citing our paper.
@article{ye2024large,
title={Large Language Models as Hyper-Heuristics for Combinatorial Optimization},
author={Haoran Ye and Jiarui Wang and Zhiguang Cao and Federico Berto and Chuanbo Hua and Haeyeon Kim and Jinkyoo Park and Guojie Song},
year={2024},
journal={arXiv preprint arXiv:2402.01145},
note={\url{https://github.com/ai4co/LLM-as-HH}}
}
We are very grateful to Yuan Jiang, Yining Ma, Yifan Yang, and AI4CO community for valuable discussions and feedback.
Also, our work is built upon the following projects, among others:
- DeepACO: Neural-enhanced Ant Systems for Combinatorial Optimization
- Eureka: Human-Level Reward Design via Coding Large Language Models
- Algorithm Evolution Using Large Language Model
- Mathematical discoveries from program search with large language models
- An Example of Evolutionary Computation + Large Language Model Beating Human: Design of Efficient Guided Local Search
- DevFormer: A Symmetric Transformer for Context-Aware Device Placement
For Tasks:
Click tags to check more tools for each tasksFor Jobs:
Alternative AI tools for LLM-as-HH
Similar Open Source Tools
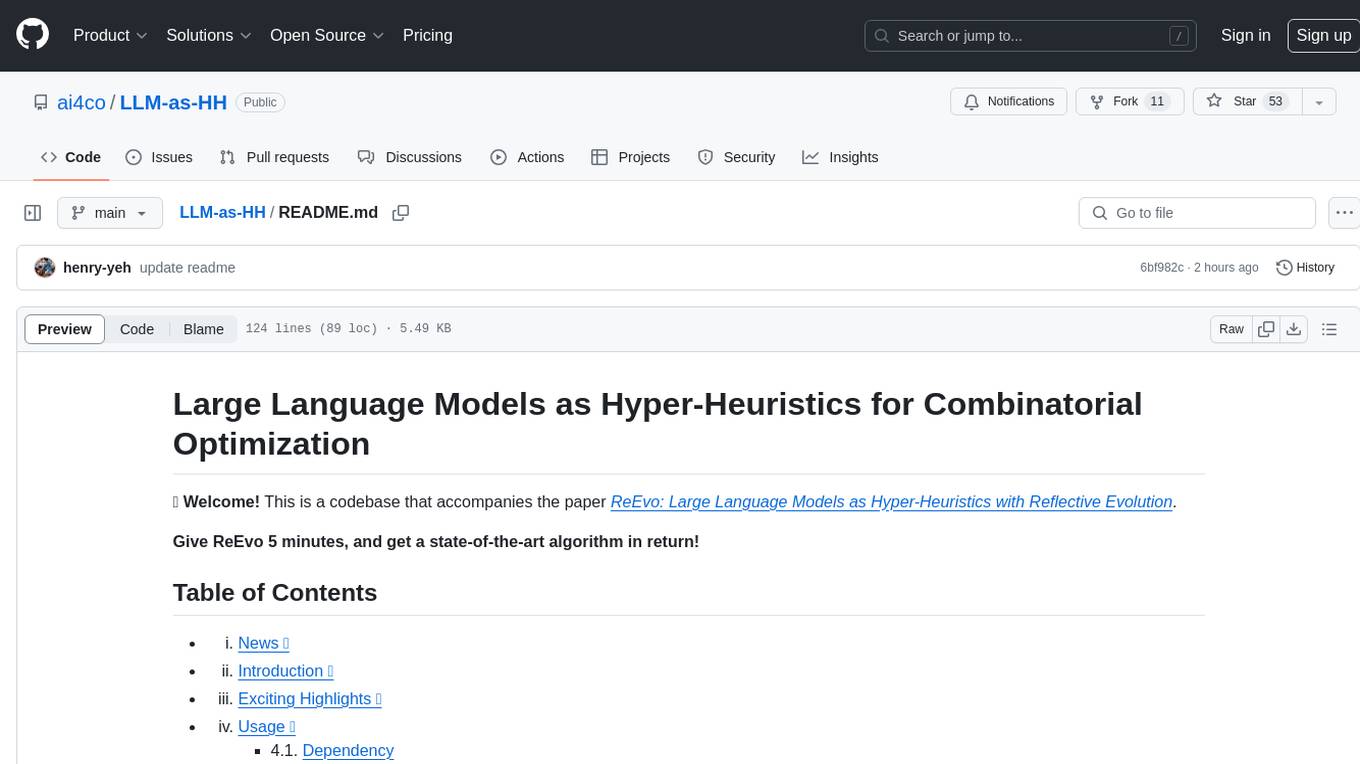
LLM-as-HH
LLM-as-HH is a codebase that accompanies the paper ReEvo: Large Language Models as Hyper-Heuristics with Reflective Evolution. It introduces Language Hyper-Heuristics (LHHs) that leverage LLMs for heuristic generation with minimal manual intervention and open-ended heuristic spaces. Reflective Evolution (ReEvo) is presented as a searching framework that emulates the reflective design approach of human experts while surpassing human capabilities with scalable LLM inference, Internet-scale domain knowledge, and powerful evolutionary search. The tool can improve various algorithms on problems like Traveling Salesman Problem, Capacitated Vehicle Routing Problem, Orienteering Problem, Multiple Knapsack Problems, Bin Packing Problem, and Decap Placement Problem in both black-box and white-box settings.
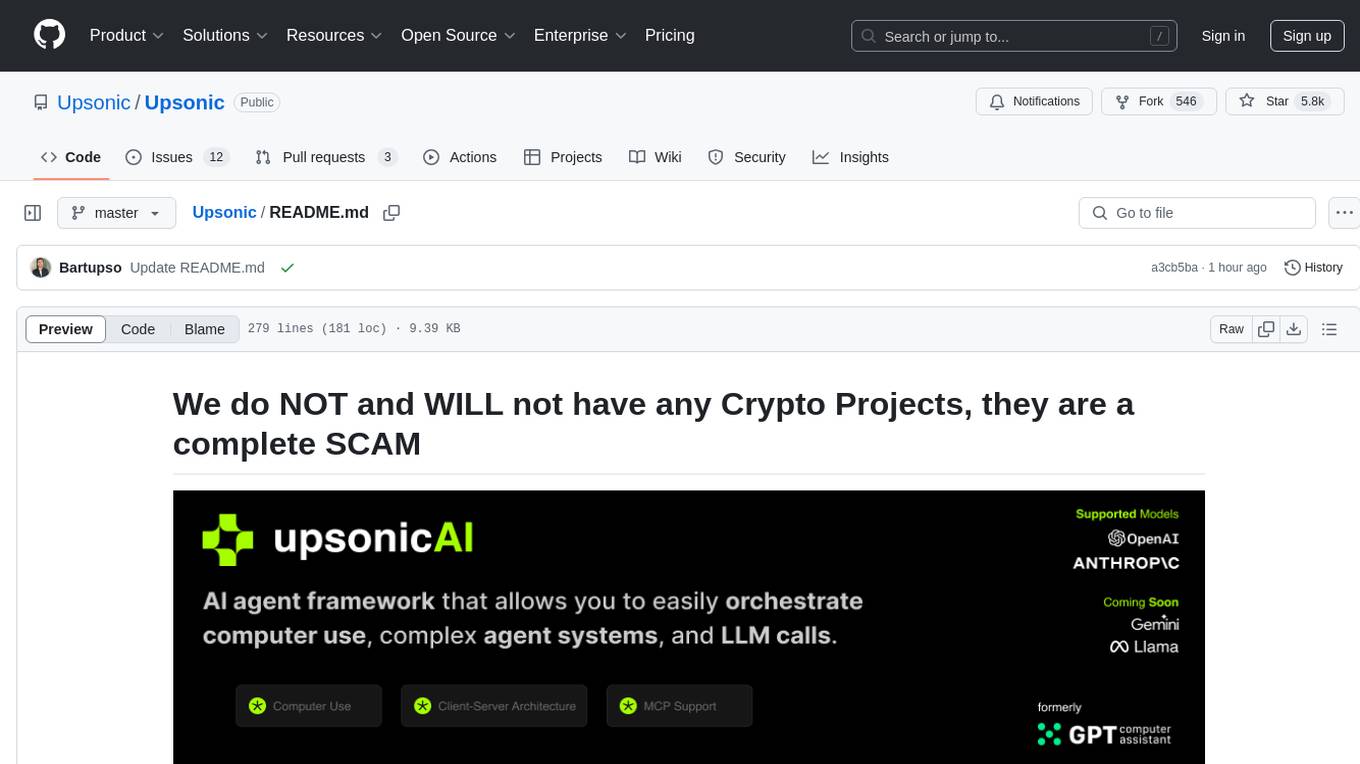
gpt-computer-assistant
GPT Computer Assistant (GCA) is an open-source framework designed to build vertical AI agents that can automate tasks on Windows, macOS, and Ubuntu systems. It leverages the Model Context Protocol (MCP) and its own modules to mimic human-like actions and achieve advanced capabilities. With GCA, users can empower themselves to accomplish more in less time by automating tasks like updating dependencies, analyzing databases, and configuring cloud security settings.
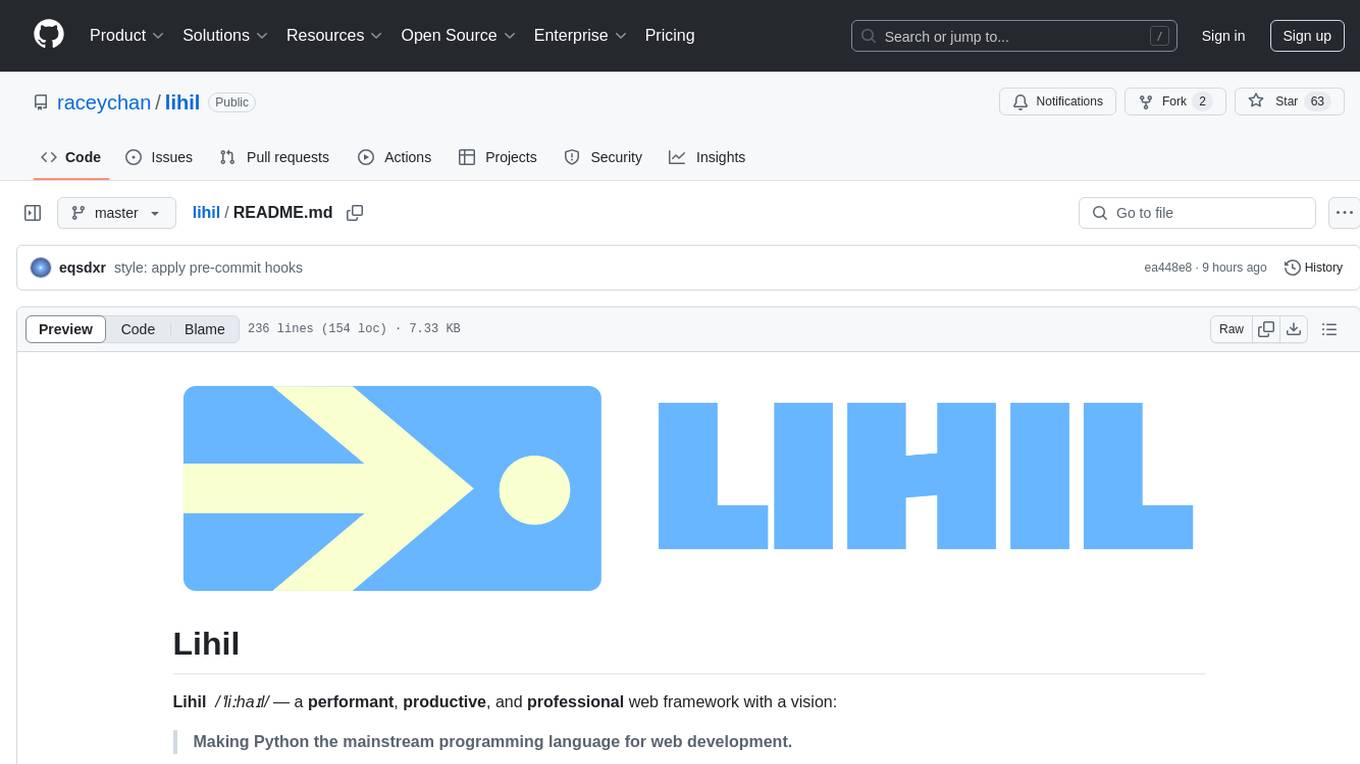
lihil
Lihil is a performant, productive, and professional web framework designed to make Python the mainstream programming language for web development. It is 100% test covered and strictly typed, offering fast performance, ergonomic API, and built-in solutions for common problems. Lihil is suitable for enterprise web development, delivering robust and scalable solutions with best practices in microservice architecture and related patterns. It features dependency injection, OpenAPI docs generation, error response generation, data validation, message system, testability, and strong support for AI features. Lihil is ASGI compatible and uses starlette as its ASGI toolkit, ensuring compatibility with starlette classes and middlewares. The framework follows semantic versioning and has a roadmap for future enhancements and features.
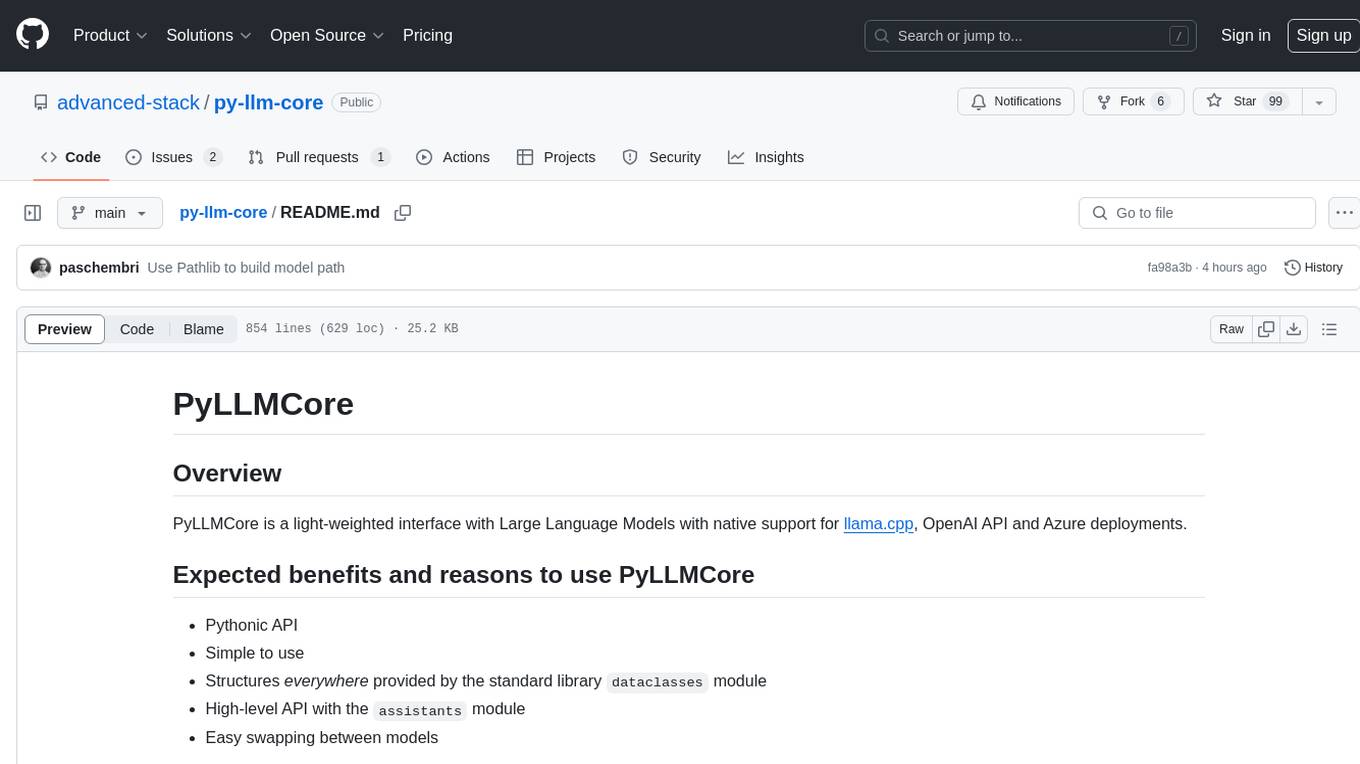
py-llm-core
PyLLMCore is a light-weighted interface with Large Language Models with native support for llama.cpp, OpenAI API, and Azure deployments. It offers a Pythonic API that is simple to use, with structures provided by the standard library dataclasses module. The high-level API includes the assistants module for easy swapping between models. PyLLMCore supports various models including those compatible with llama.cpp, OpenAI, and Azure APIs. It covers use cases such as parsing, summarizing, question answering, hallucinations reduction, context size management, and tokenizing. The tool allows users to interact with language models for tasks like parsing text, summarizing content, answering questions, reducing hallucinations, managing context size, and tokenizing text.
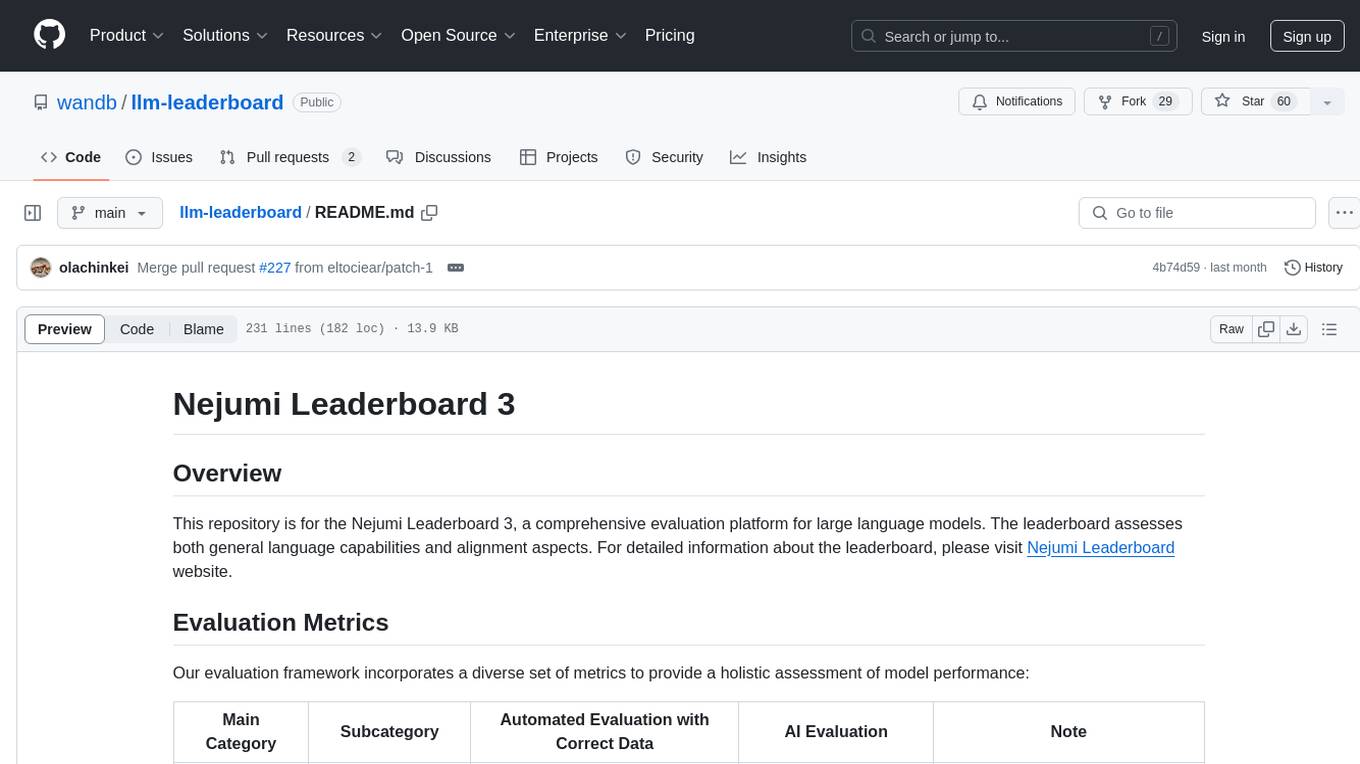
llm-leaderboard
Nejumi Leaderboard 3 is a comprehensive evaluation platform for large language models, assessing general language capabilities and alignment aspects. The evaluation framework includes metrics for language processing, translation, summarization, information extraction, reasoning, mathematical reasoning, entity extraction, knowledge/question answering, English, semantic analysis, syntactic analysis, alignment, ethics/moral, toxicity, bias, truthfulness, and robustness. The repository provides an implementation guide for environment setup, dataset preparation, configuration, model configurations, and chat template creation. Users can run evaluation processes using specified configuration files and log results to the Weights & Biases project.
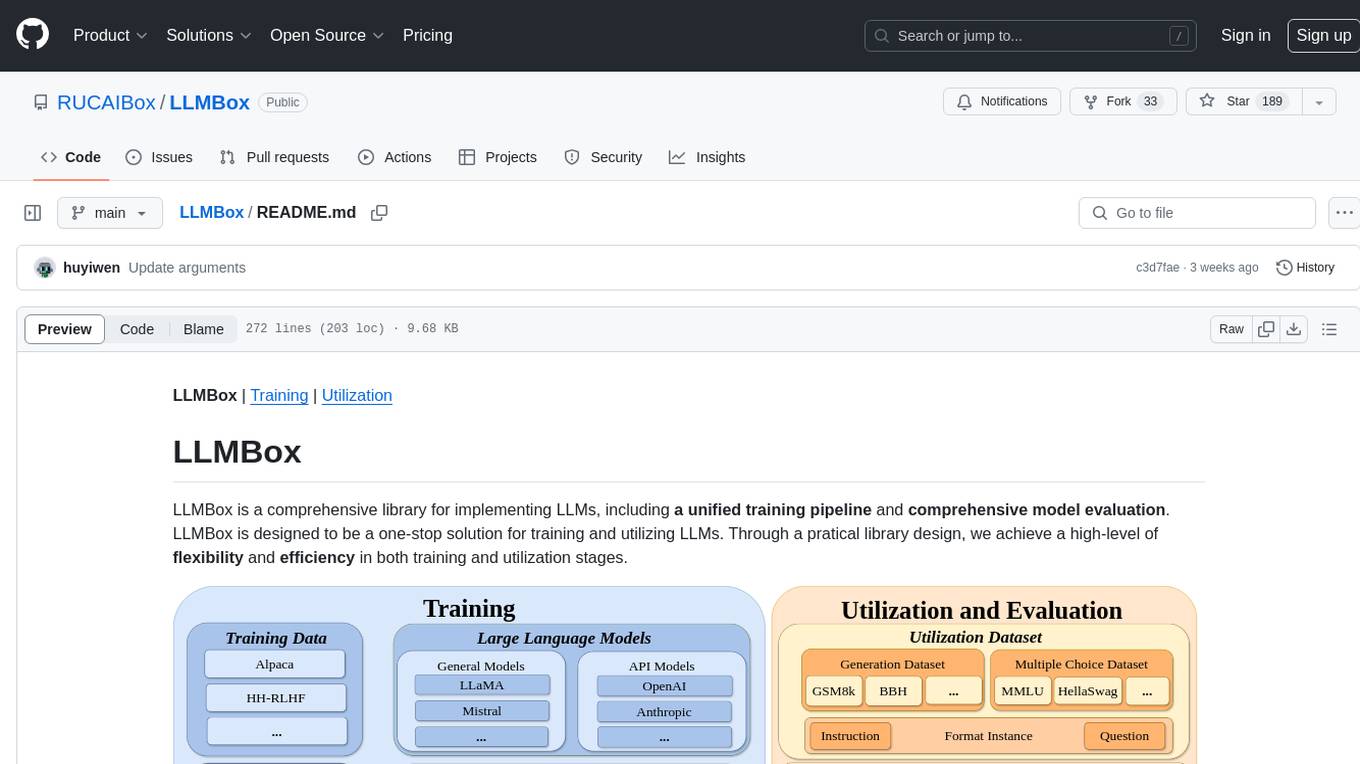
LLMBox
LLMBox is a comprehensive library designed for implementing Large Language Models (LLMs) with a focus on a unified training pipeline and comprehensive model evaluation. It serves as a one-stop solution for training and utilizing LLMs, offering flexibility and efficiency in both training and utilization stages. The library supports diverse training strategies, comprehensive datasets, tokenizer vocabulary merging, data construction strategies, parameter efficient fine-tuning, and efficient training methods. For utilization, LLMBox provides comprehensive evaluation on various datasets, in-context learning strategies, chain-of-thought evaluation, evaluation methods, prefix caching for faster inference, support for specific LLM models like vLLM and Flash Attention, and quantization options. The tool is suitable for researchers and developers working with LLMs for natural language processing tasks.
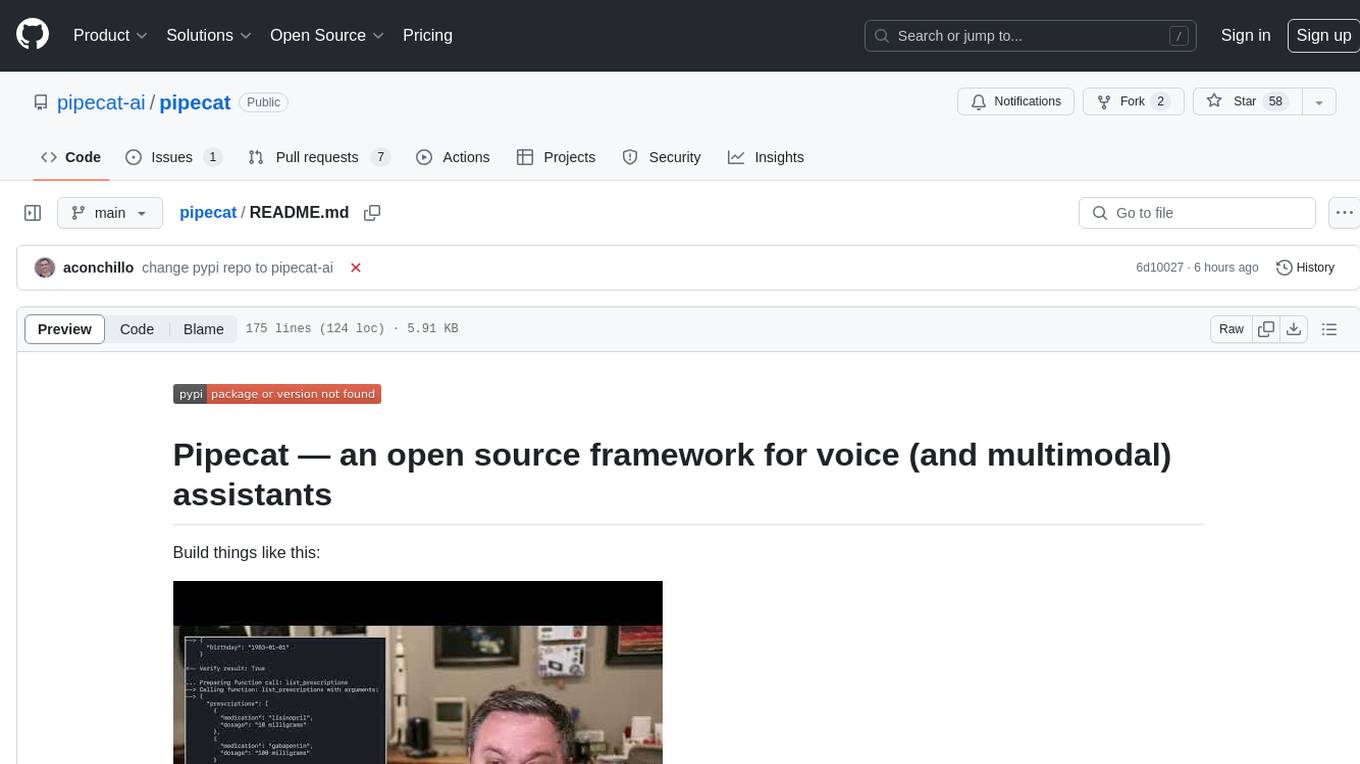
pipecat
Pipecat is an open-source framework designed for building generative AI voice bots and multimodal assistants. It provides code building blocks for interacting with AI services, creating low-latency data pipelines, and transporting audio, video, and events over the Internet. Pipecat supports various AI services like speech-to-text, text-to-speech, image generation, and vision models. Users can implement new services and contribute to the framework. Pipecat aims to simplify the development of applications like personal coaches, meeting assistants, customer support bots, and more by providing a complete framework for integrating AI services.
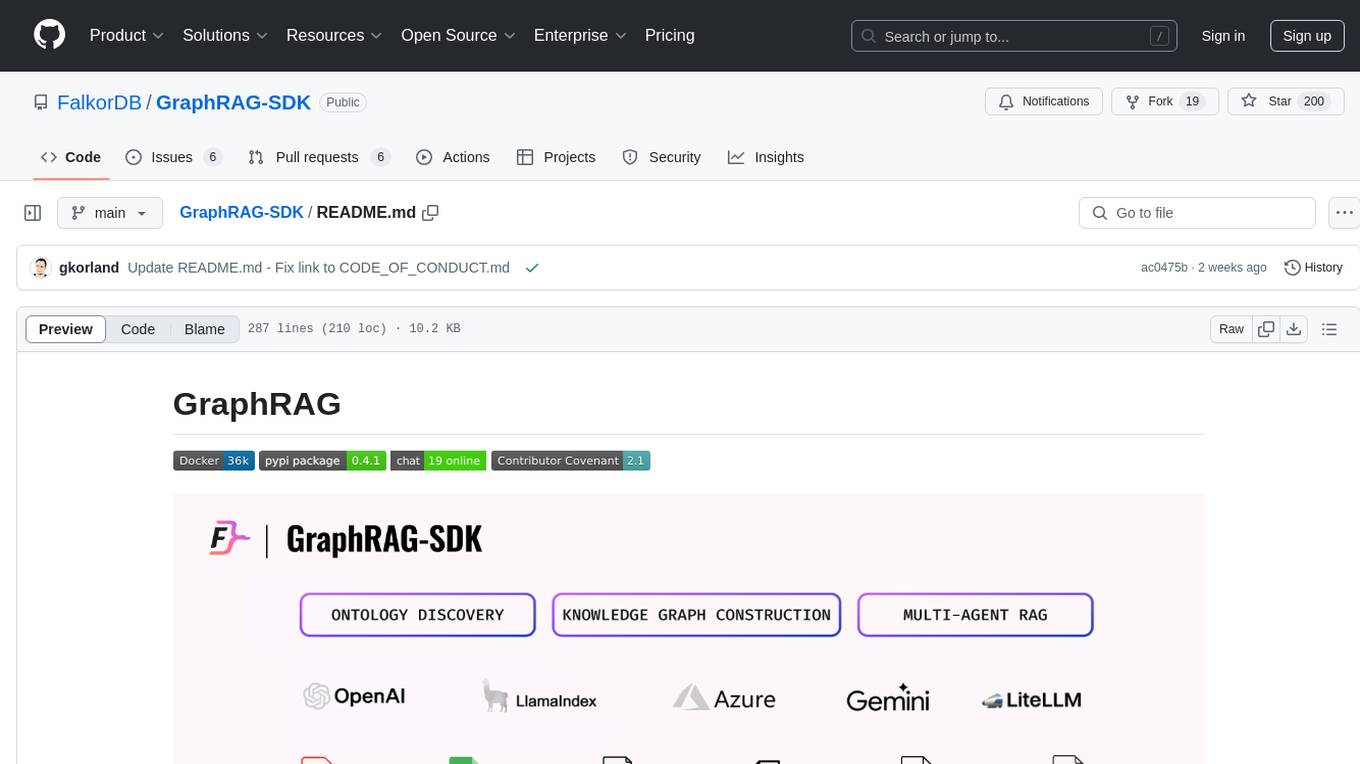
GraphRAG-SDK
Build fast and accurate GenAI applications with GraphRAG SDK, a specialized toolkit for building Graph Retrieval-Augmented Generation (GraphRAG) systems. It integrates knowledge graphs, ontology management, and state-of-the-art LLMs to deliver accurate, efficient, and customizable RAG workflows. The SDK simplifies the development process by automating ontology creation, knowledge graph agent creation, and query handling, enabling users to interact and query their knowledge graphs effectively. It supports multi-agent systems and orchestrates agents specialized in different domains. The SDK is optimized for FalkorDB, ensuring high performance and scalability for large-scale applications. By leveraging knowledge graphs, it enables semantic relationships and ontology-driven queries that go beyond standard vector similarity, enhancing retrieval-augmented generation capabilities.
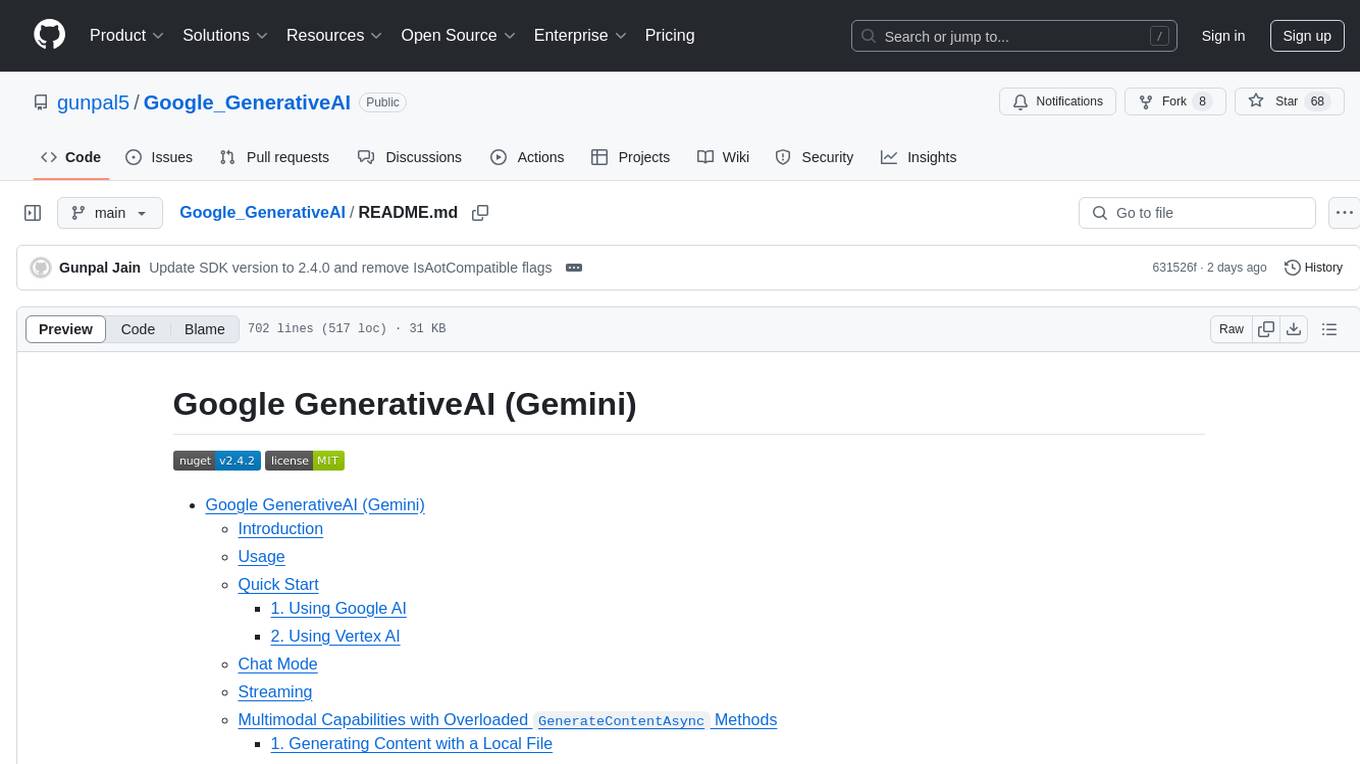
Google_GenerativeAI
Google GenerativeAI (Gemini) is an unofficial C# .Net SDK based on REST APIs for accessing Google Gemini models. It offers a complete rewrite of the previous SDK with improved performance, flexibility, and ease of use. The SDK seamlessly integrates with LangChain.net, providing easy methods for JSON-based interactions and function calling with Google Gemini models. It includes features like enhanced JSON mode handling, function calling with code generator, multi-modal functionality, Vertex AI support, multimodal live API, image generation and captioning, retrieval-augmented generation with Vertex RAG Engine and Google AQA, easy JSON handling, Gemini tools and function calling, multimodal live API, and more.
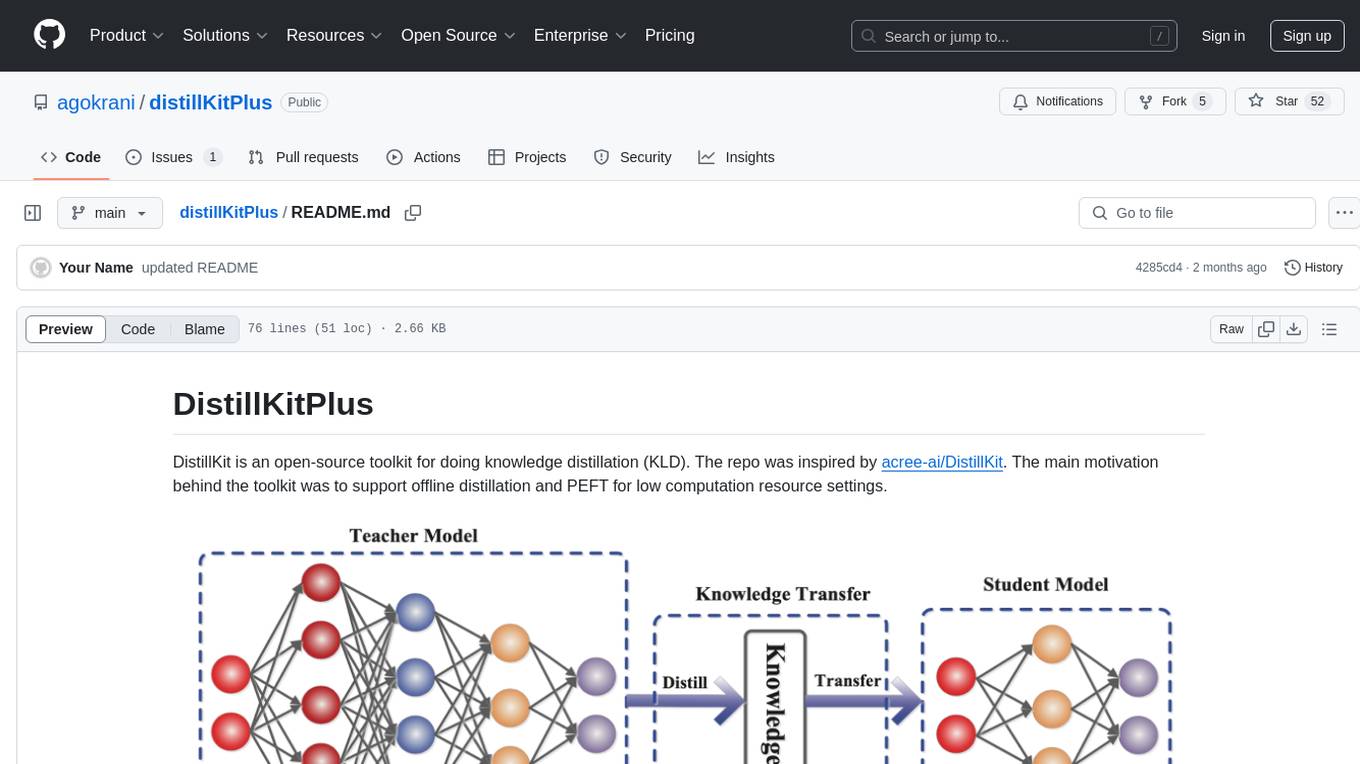
distillKitPlus
DistillKitPlus is an open-source toolkit designed for knowledge distillation (KLD) in low computation resource settings. It supports logit distillation, pre-computed logits for memory-efficient training, LoRA fine-tuning integration, and model quantization for faster inference. The toolkit utilizes a JSON configuration file for project, dataset, model, tokenizer, training, distillation, LoRA, and quantization settings. Users can contribute to the toolkit and contact the developers for technical questions or issues.
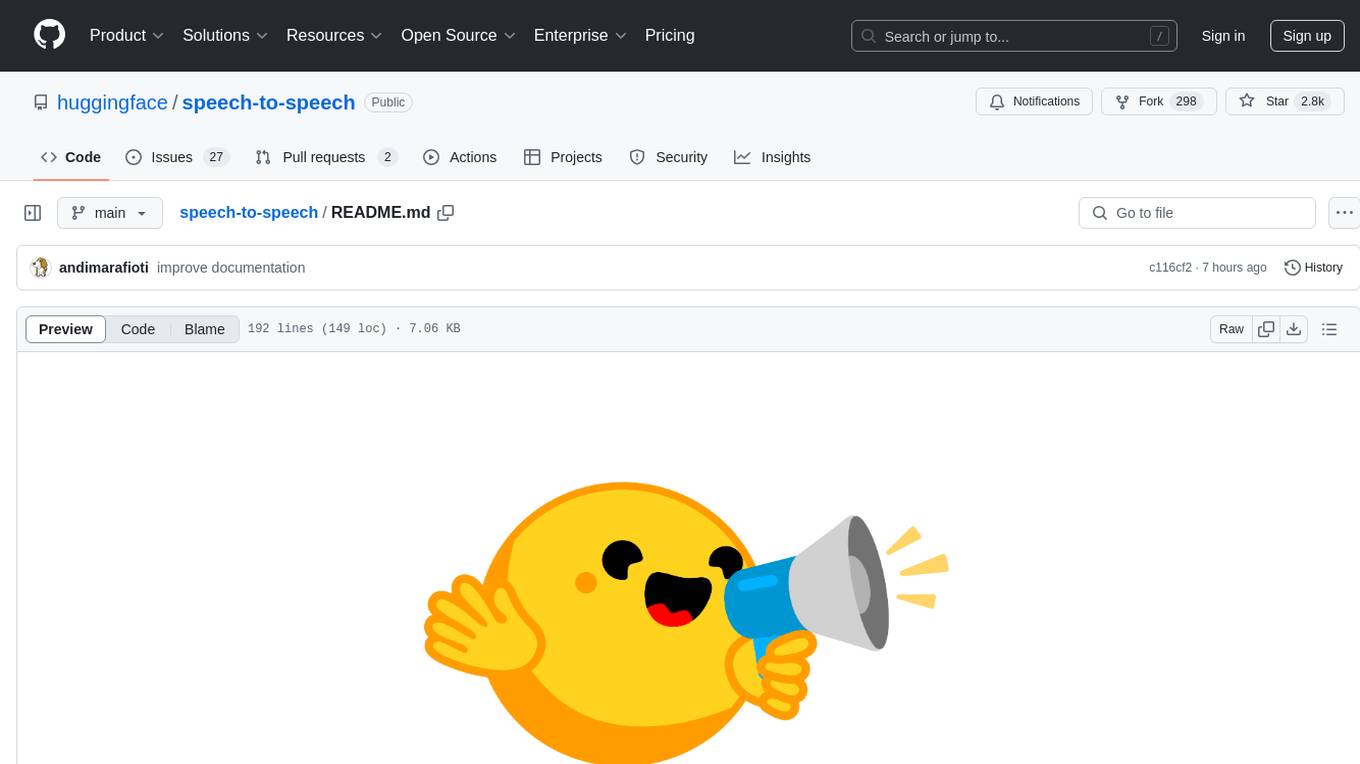
speech-to-speech
This repository implements a speech-to-speech cascaded pipeline with consecutive parts including Voice Activity Detection (VAD), Speech to Text (STT), Language Model (LM), and Text to Speech (TTS). It aims to provide a fully open and modular approach by leveraging models available on the Transformers library via the Hugging Face hub. The code is designed for easy modification, with each component implemented as a class. Users can run the pipeline either on a server/client approach or locally, with detailed setup and usage instructions provided in the readme.
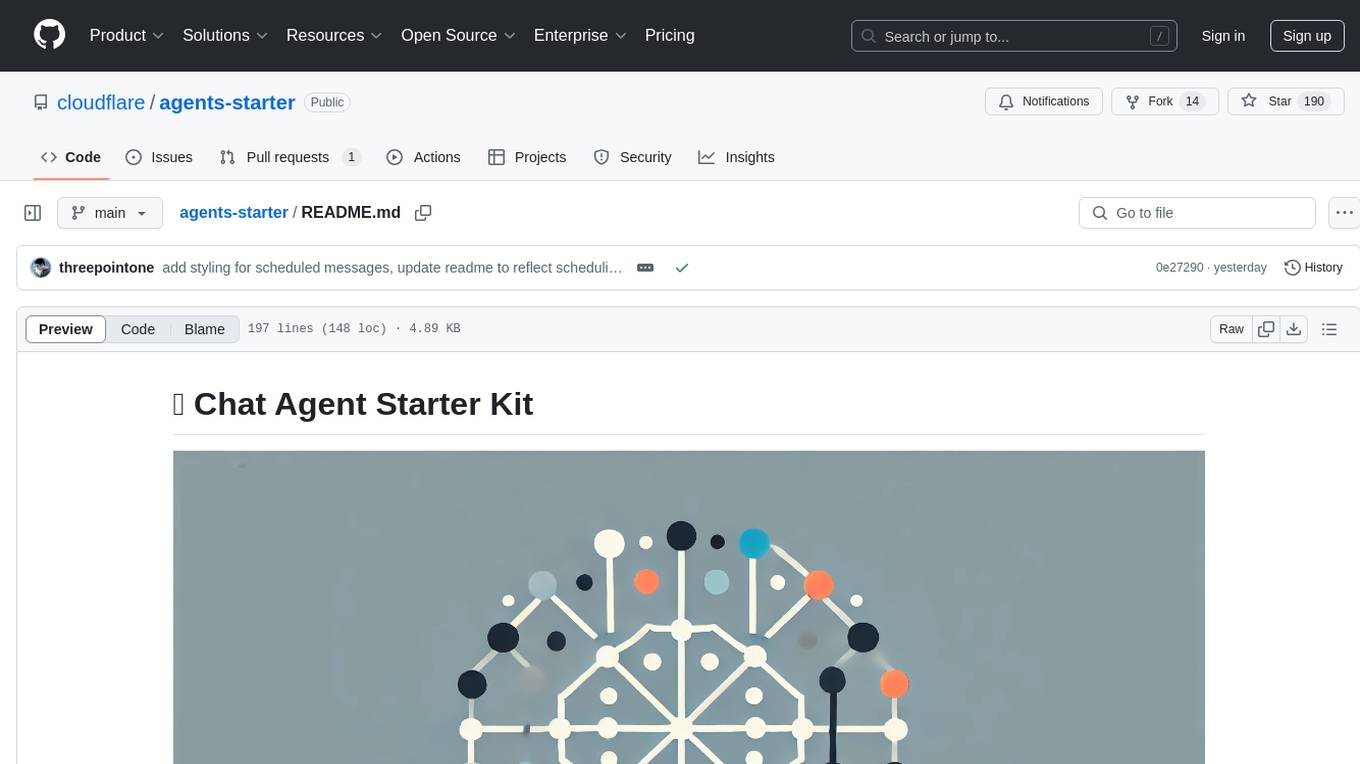
agents-starter
A starter template for building AI-powered chat agents using Cloudflare's Agent platform, powered by agents-sdk. It provides a foundation for creating interactive chat experiences with AI, complete with a modern UI and tool integration capabilities. Features include interactive chat interface with AI, built-in tool system with human-in-the-loop confirmation, advanced task scheduling, dark/light theme support, real-time streaming responses, state management, and chat history. Prerequisites include a Cloudflare account and OpenAI API key. The project structure includes components for chat UI implementation, chat agent logic, tool definitions, and helper functions. Customization guide covers adding new tools, modifying the UI, and example use cases for customer support, development assistant, data analysis assistant, personal productivity assistant, and scheduling assistant.
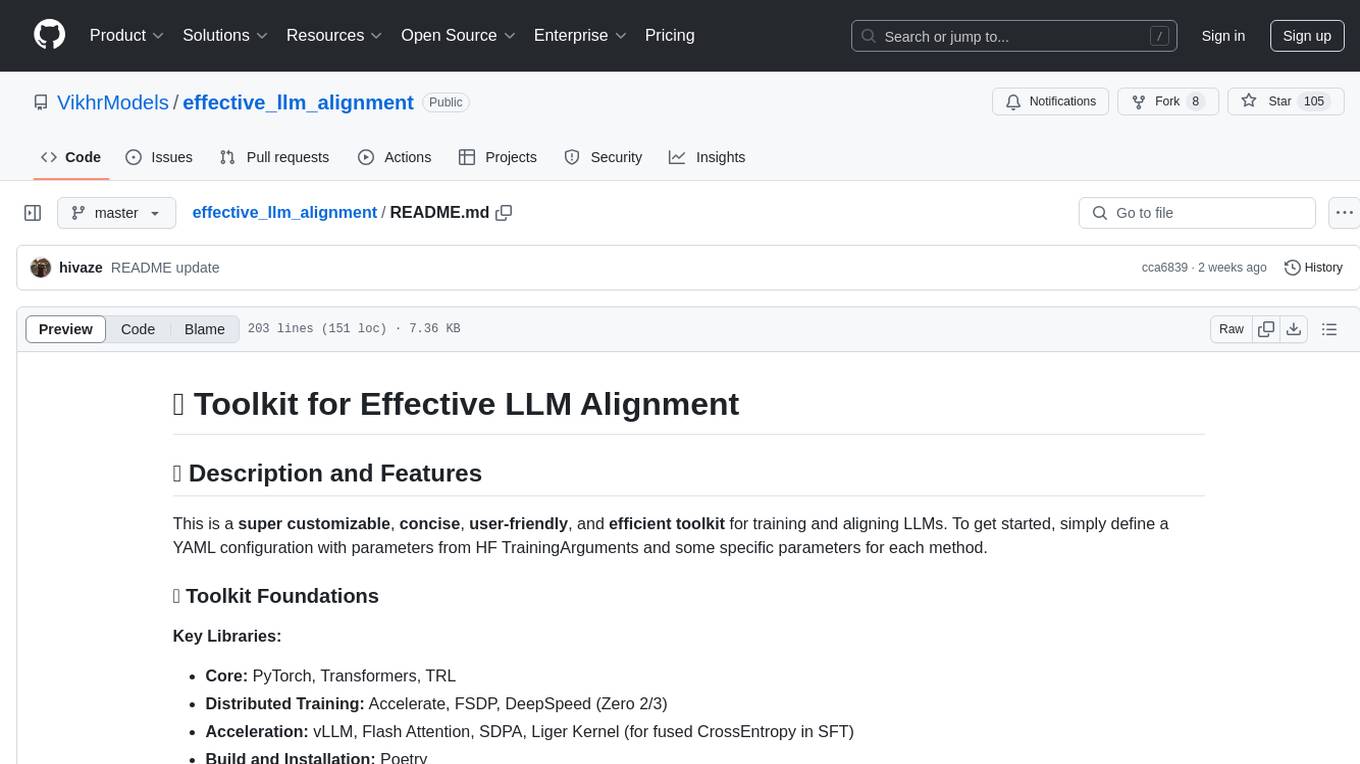
effective_llm_alignment
This is a super customizable, concise, user-friendly, and efficient toolkit for training and aligning LLMs. It provides support for various methods such as SFT, Distillation, DPO, ORPO, CPO, SimPO, SMPO, Non-pair Reward Modeling, Special prompts basket format, Rejection Sampling, Scoring using RM, Effective FAISS Map-Reduce Deduplication, LLM scoring using RM, NER, CLIP, Classification, and STS. The toolkit offers key libraries like PyTorch, Transformers, TRL, Accelerate, FSDP, DeepSpeed, and tools for result logging with wandb or clearml. It allows mixing datasets, generation and logging in wandb/clearml, vLLM batched generation, and aligns models using the SMPO method.
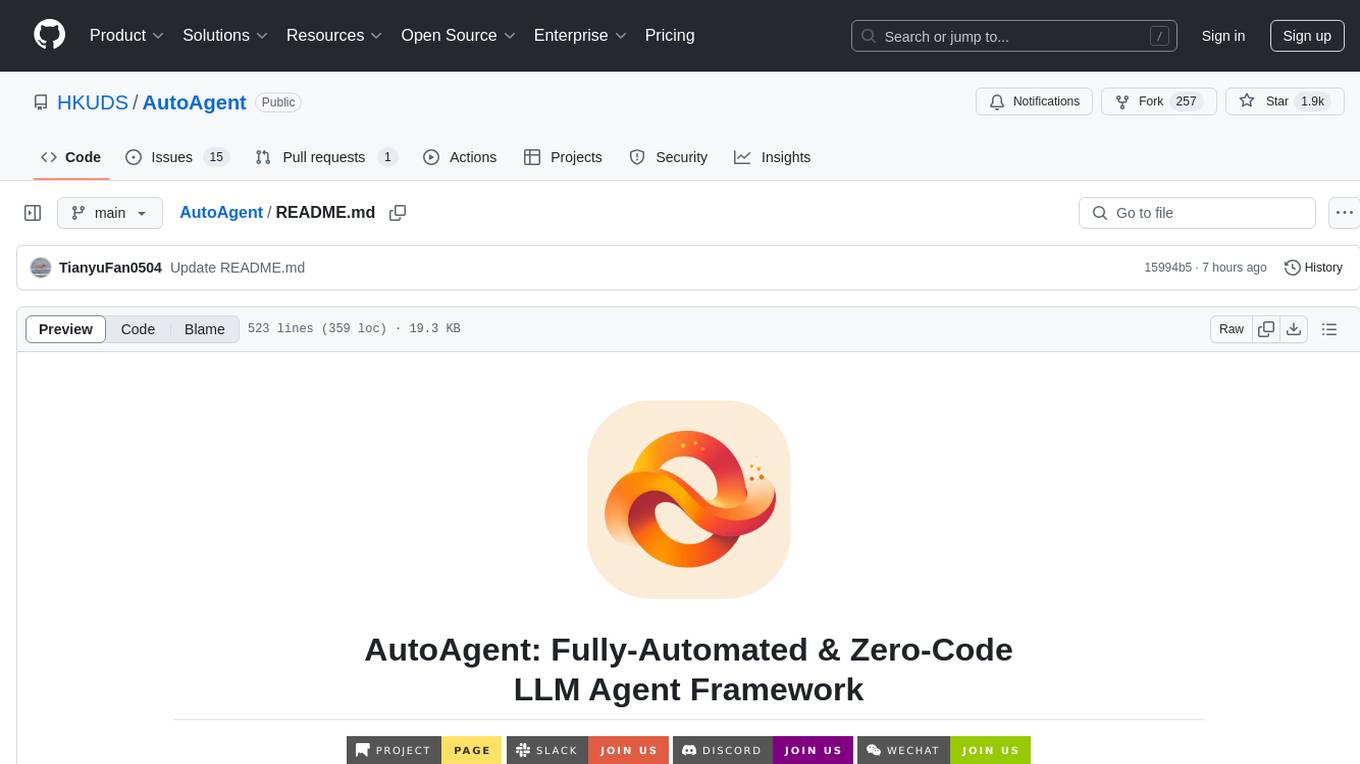
AutoAgent
AutoAgent is a fully-automated and zero-code framework that enables users to create and deploy LLM agents through natural language alone. It is a top performer on the GAIA Benchmark, equipped with a native self-managing vector database, and allows for easy creation of tools, agents, and workflows without any coding. AutoAgent seamlessly integrates with a wide range of LLMs and supports both function-calling and ReAct interaction modes. It is designed to be dynamic, extensible, customized, and lightweight, serving as a personal AI assistant.
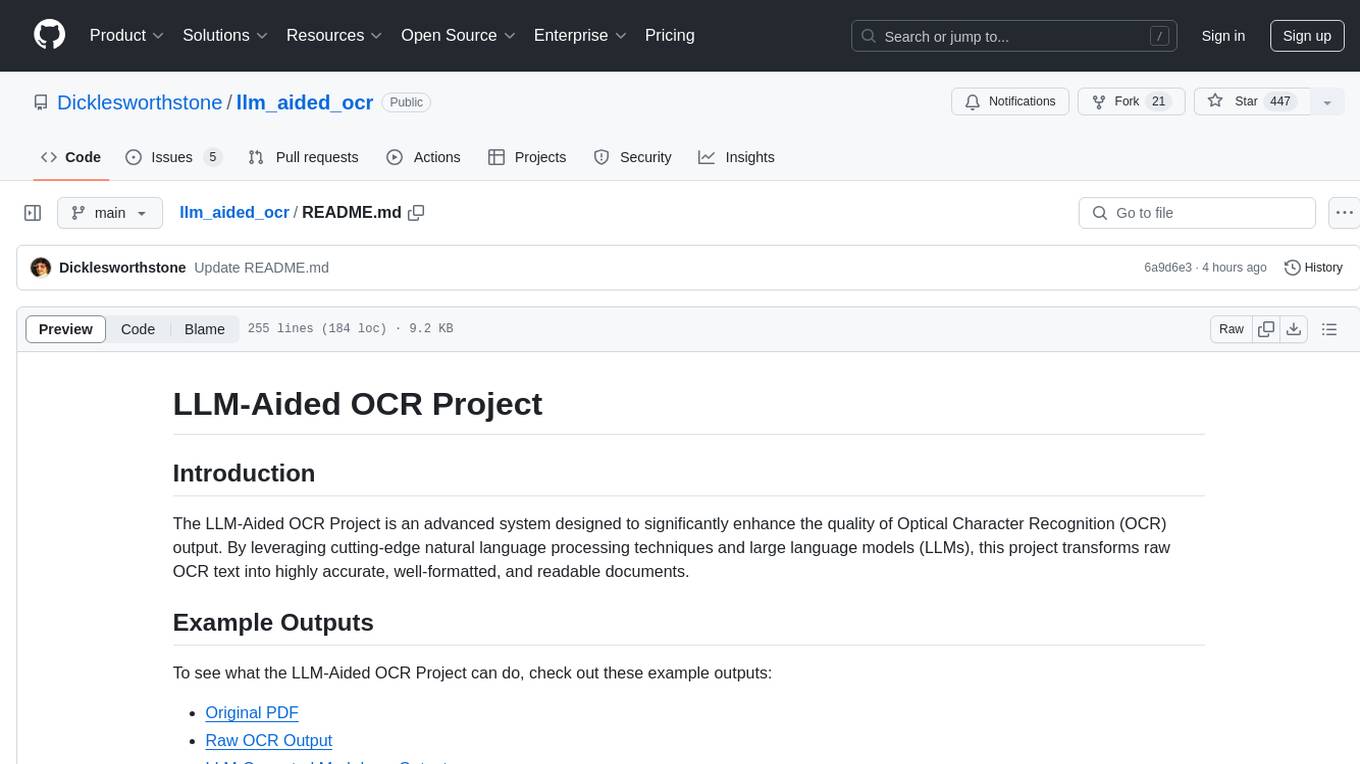
llm_aided_ocr
The LLM-Aided OCR Project is an advanced system that enhances Optical Character Recognition (OCR) output by leveraging natural language processing techniques and large language models. It offers features like PDF to image conversion, OCR using Tesseract, error correction using LLMs, smart text chunking, markdown formatting, duplicate content removal, quality assessment, support for local and cloud-based LLMs, asynchronous processing, detailed logging, and GPU acceleration. The project provides detailed technical overview, text processing pipeline, LLM integration, token management, quality assessment, logging, configuration, and customization. It requires Python 3.12+, Tesseract OCR engine, PDF2Image library, PyTesseract, and optional OpenAI or Anthropic API support for cloud-based LLMs. The installation process involves setting up the project, installing dependencies, and configuring environment variables. Users can place a PDF file in the project directory, update input file path, and run the script to generate post-processed text. The project optimizes processing with concurrent processing, context preservation, and adaptive token management. Configuration settings include choosing between local or API-based LLMs, selecting API provider, specifying models, and setting context size for local LLMs. Output files include raw OCR output and LLM-corrected text. Limitations include performance dependency on LLM quality and time-consuming processing for large documents.
For similar tasks
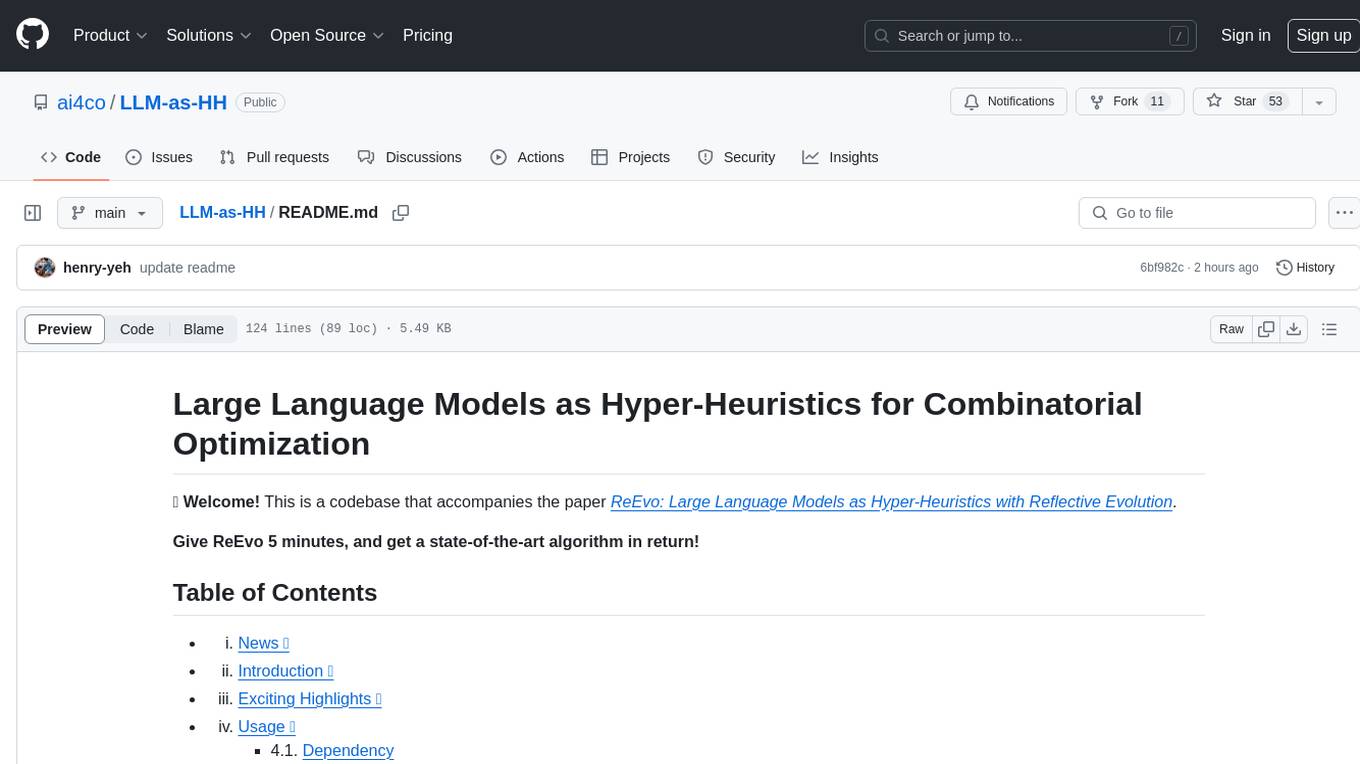
LLM-as-HH
LLM-as-HH is a codebase that accompanies the paper ReEvo: Large Language Models as Hyper-Heuristics with Reflective Evolution. It introduces Language Hyper-Heuristics (LHHs) that leverage LLMs for heuristic generation with minimal manual intervention and open-ended heuristic spaces. Reflective Evolution (ReEvo) is presented as a searching framework that emulates the reflective design approach of human experts while surpassing human capabilities with scalable LLM inference, Internet-scale domain knowledge, and powerful evolutionary search. The tool can improve various algorithms on problems like Traveling Salesman Problem, Capacitated Vehicle Routing Problem, Orienteering Problem, Multiple Knapsack Problems, Bin Packing Problem, and Decap Placement Problem in both black-box and white-box settings.
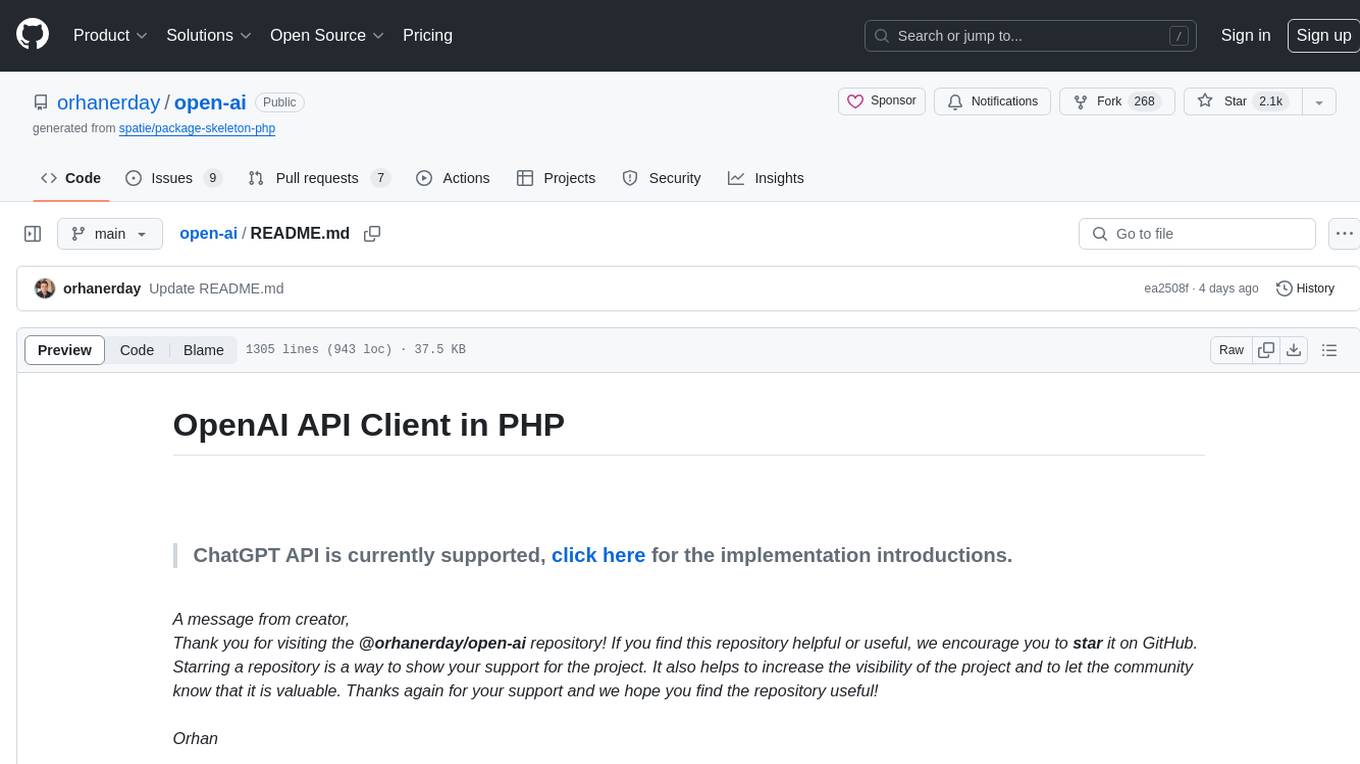
open-ai
Open AI is a powerful tool for artificial intelligence research and development. It provides a wide range of machine learning models and algorithms, making it easier for developers to create innovative AI applications. With Open AI, users can explore cutting-edge technologies such as natural language processing, computer vision, and reinforcement learning. The platform offers a user-friendly interface and comprehensive documentation to support users in building and deploying AI solutions. Whether you are a beginner or an experienced AI practitioner, Open AI offers the tools and resources you need to accelerate your AI projects and stay ahead in the rapidly evolving field of artificial intelligence.
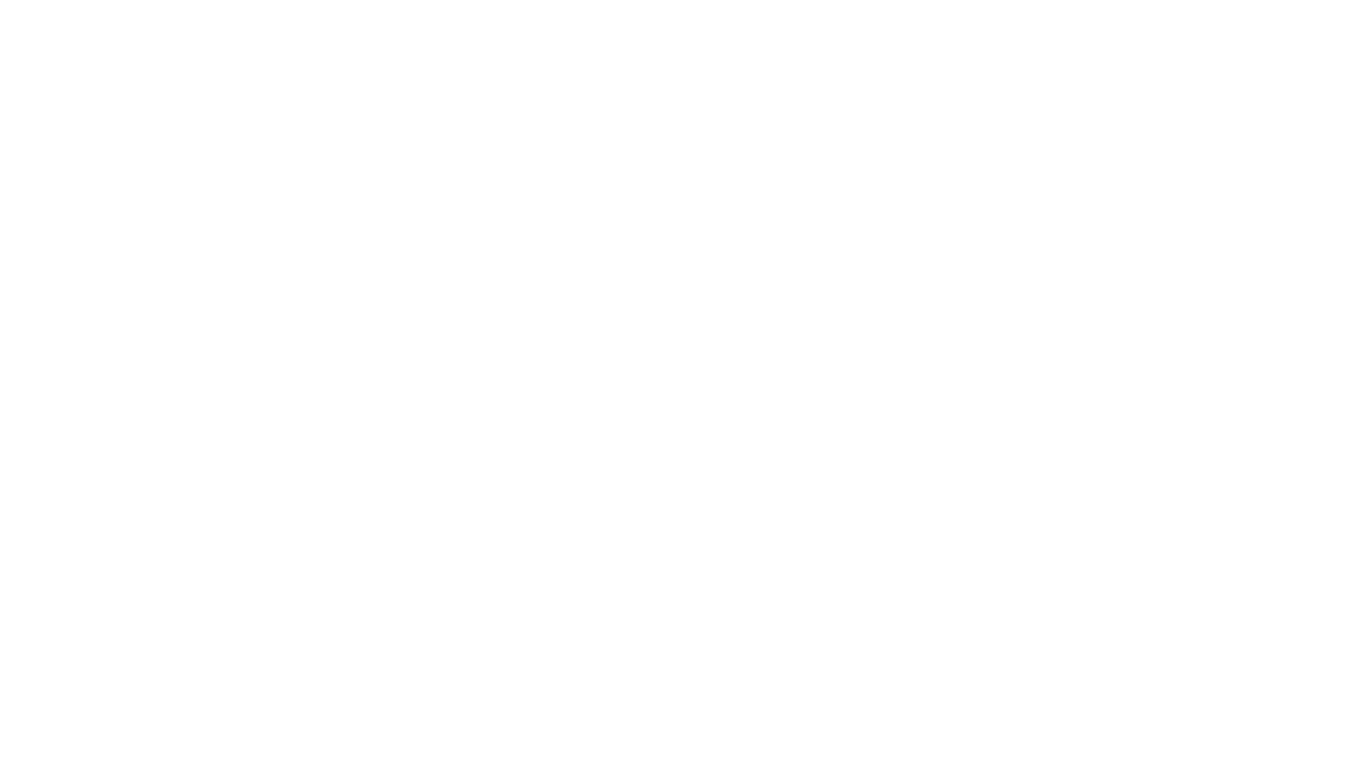
universal
The Universal Numbers Library is a header-only C++ template library designed for universal number arithmetic, offering alternatives to native integer and floating-point for mixed-precision algorithm development and optimization. It tailors arithmetic types to the application's precision and dynamic range, enabling improved application performance and energy efficiency. The library provides fast implementations of special IEEE-754 formats like quarter precision, half-precision, and quad precision, as well as vendor-specific extensions. It supports static and elastic integers, decimals, fixed-points, rationals, linear floats, tapered floats, logarithmic, interval, and adaptive-precision integers, rationals, and floats. The library is suitable for AI, DSP, HPC, and HFT algorithms.
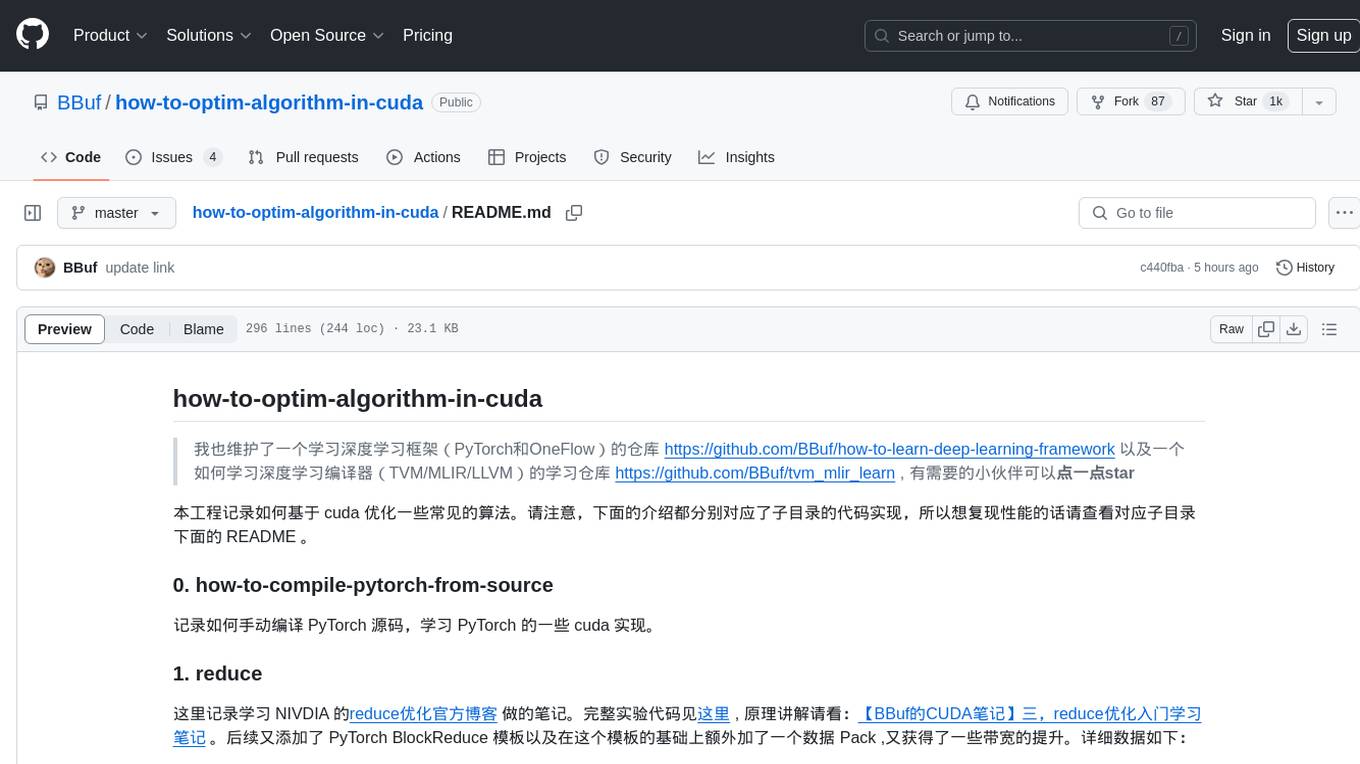
how-to-optim-algorithm-in-cuda
This repository documents how to optimize common algorithms based on CUDA. It includes subdirectories with code implementations for specific optimizations. The optimizations cover topics such as compiling PyTorch from source, NVIDIA's reduce optimization, OneFlow's elementwise template, fast atomic add for half data types, upsample nearest2d optimization in OneFlow, optimized indexing in PyTorch, OneFlow's softmax kernel, linear attention optimization, and more. The repository also includes learning resources related to deep learning frameworks, compilers, and optimization techniques.
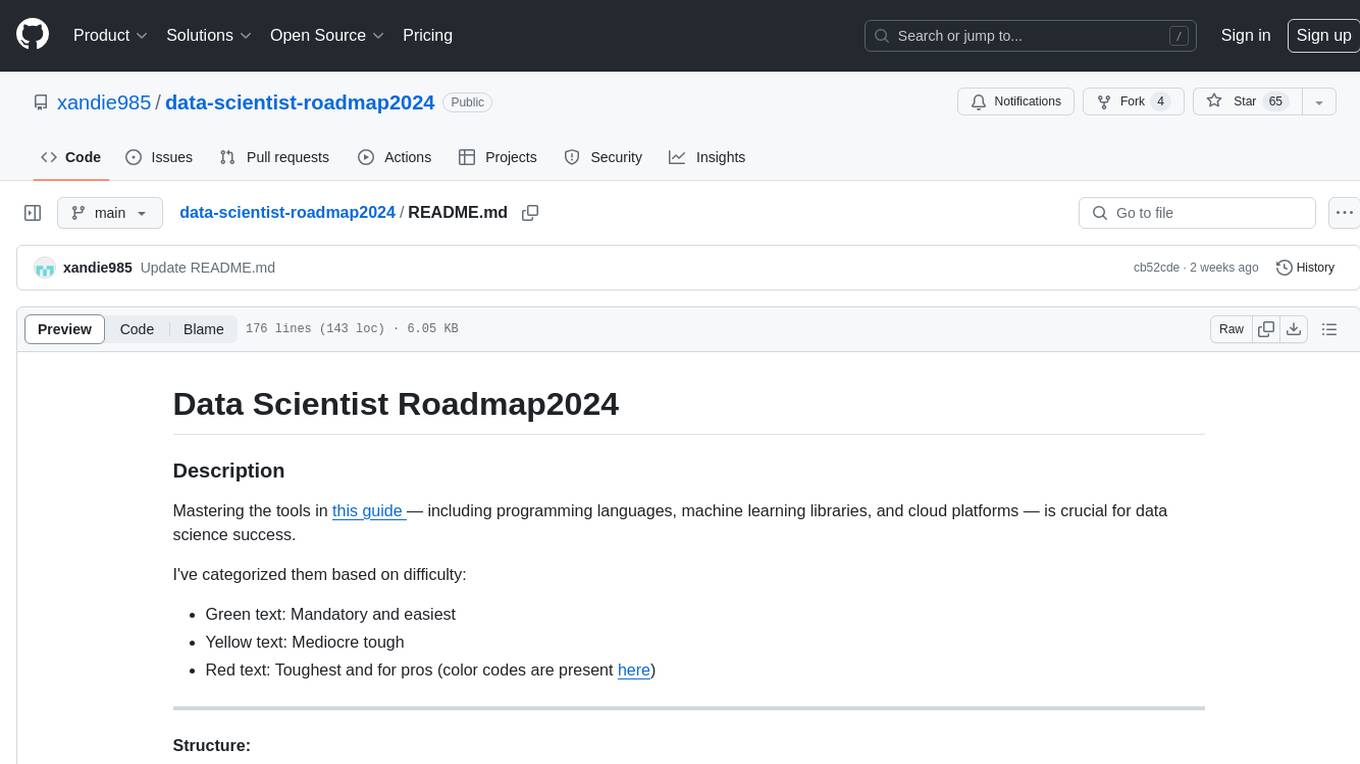
data-scientist-roadmap2024
The Data Scientist Roadmap2024 provides a comprehensive guide to mastering essential tools for data science success. It includes programming languages, machine learning libraries, cloud platforms, and concepts categorized by difficulty. The roadmap covers a wide range of topics from programming languages to machine learning techniques, data visualization tools, and DevOps/MLOps tools. It also includes web development frameworks and specific concepts like supervised and unsupervised learning, NLP, deep learning, reinforcement learning, and statistics. Additionally, it delves into DevOps tools like Airflow and MLFlow, data visualization tools like Tableau and Matplotlib, and other topics such as ETL processes, optimization algorithms, and financial modeling.
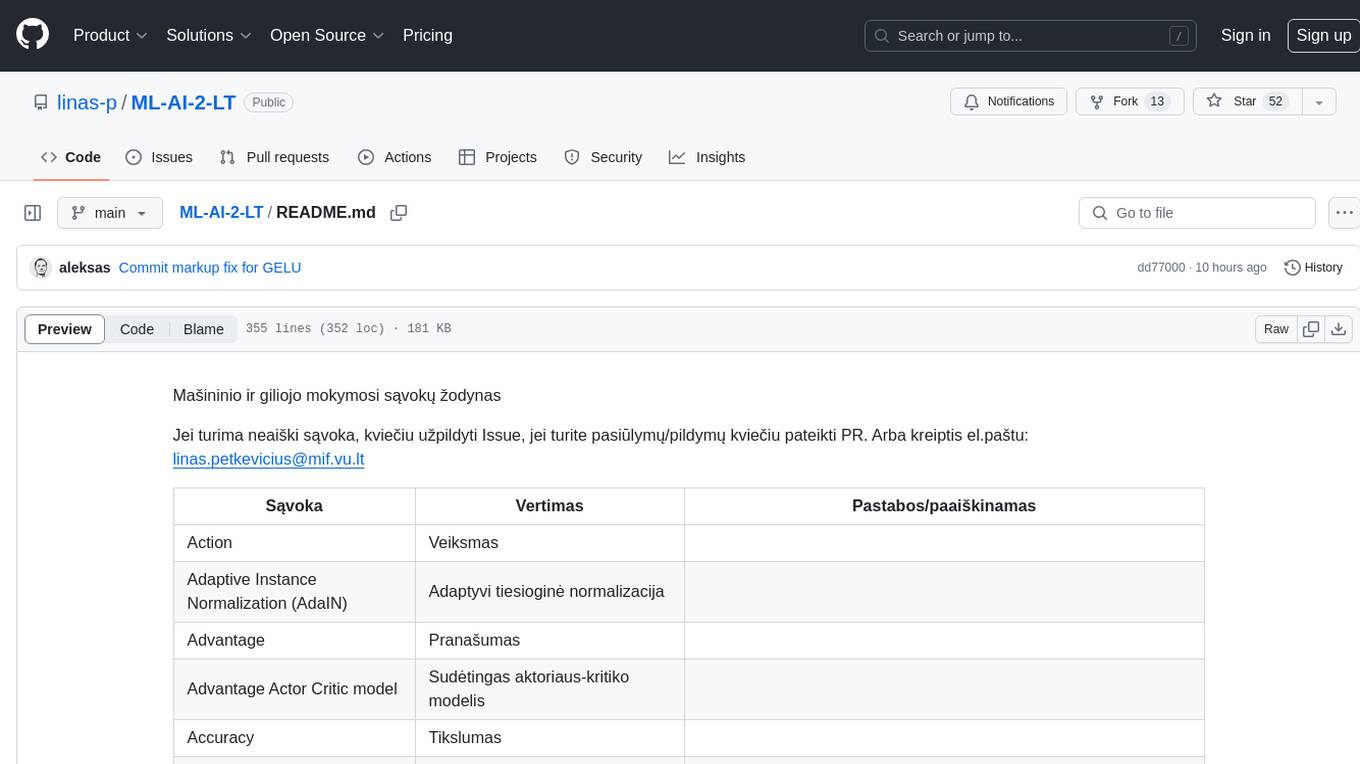
ML-AI-2-LT
ML-AI-2-LT is a repository that serves as a glossary for machine learning and deep learning concepts. It contains translations and explanations of various terms related to artificial intelligence, including definitions and notes. Users can contribute by filling issues for unclear concepts or by submitting pull requests with suggestions or additions. The repository aims to provide a comprehensive resource for understanding key terminology in the field of AI and machine learning.
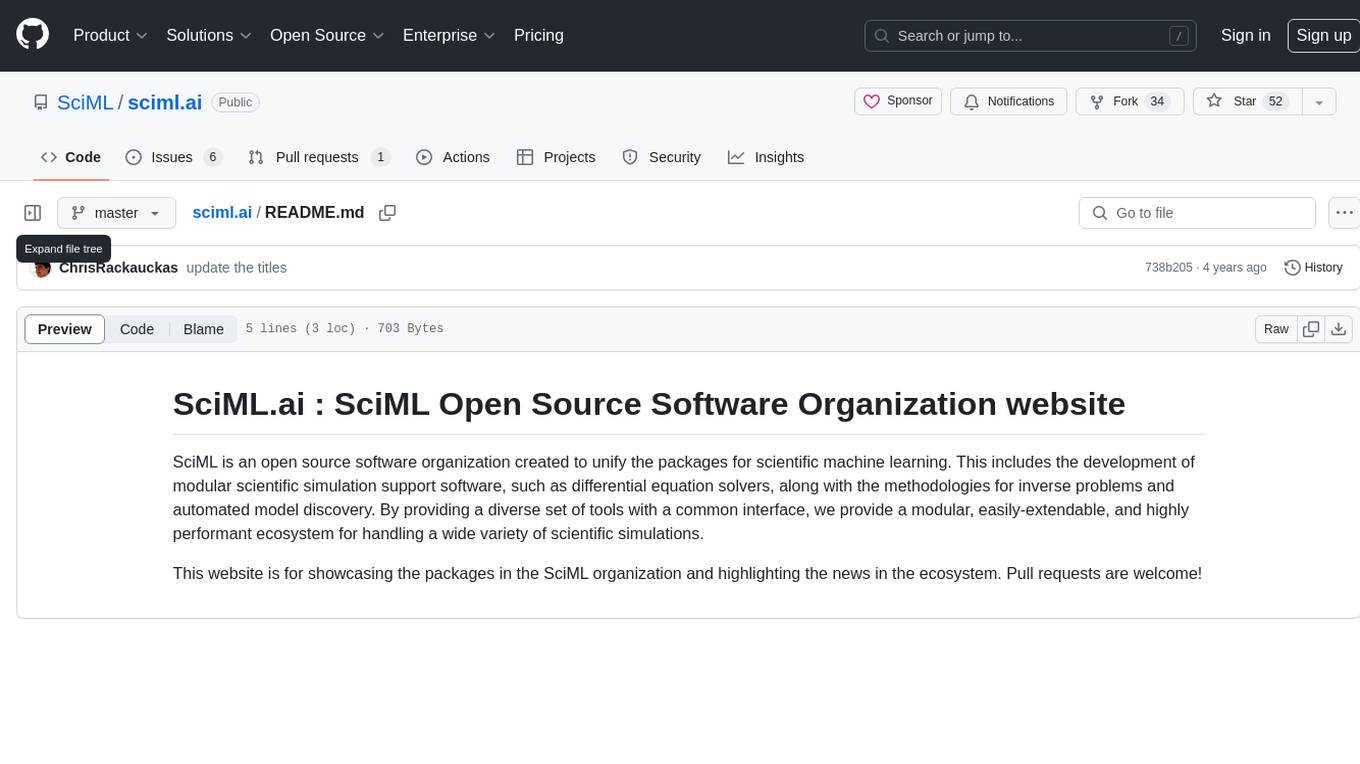
sciml.ai
SciML.ai is an open source software organization dedicated to unifying packages for scientific machine learning. It focuses on developing modular scientific simulation support software, including differential equation solvers, inverse problems methodologies, and automated model discovery. The organization aims to provide a diverse set of tools with a common interface, creating a modular, easily-extendable, and highly performant ecosystem for scientific simulations. The website serves as a platform to showcase SciML organization's packages and share news within the ecosystem. Pull requests are encouraged for contributions.
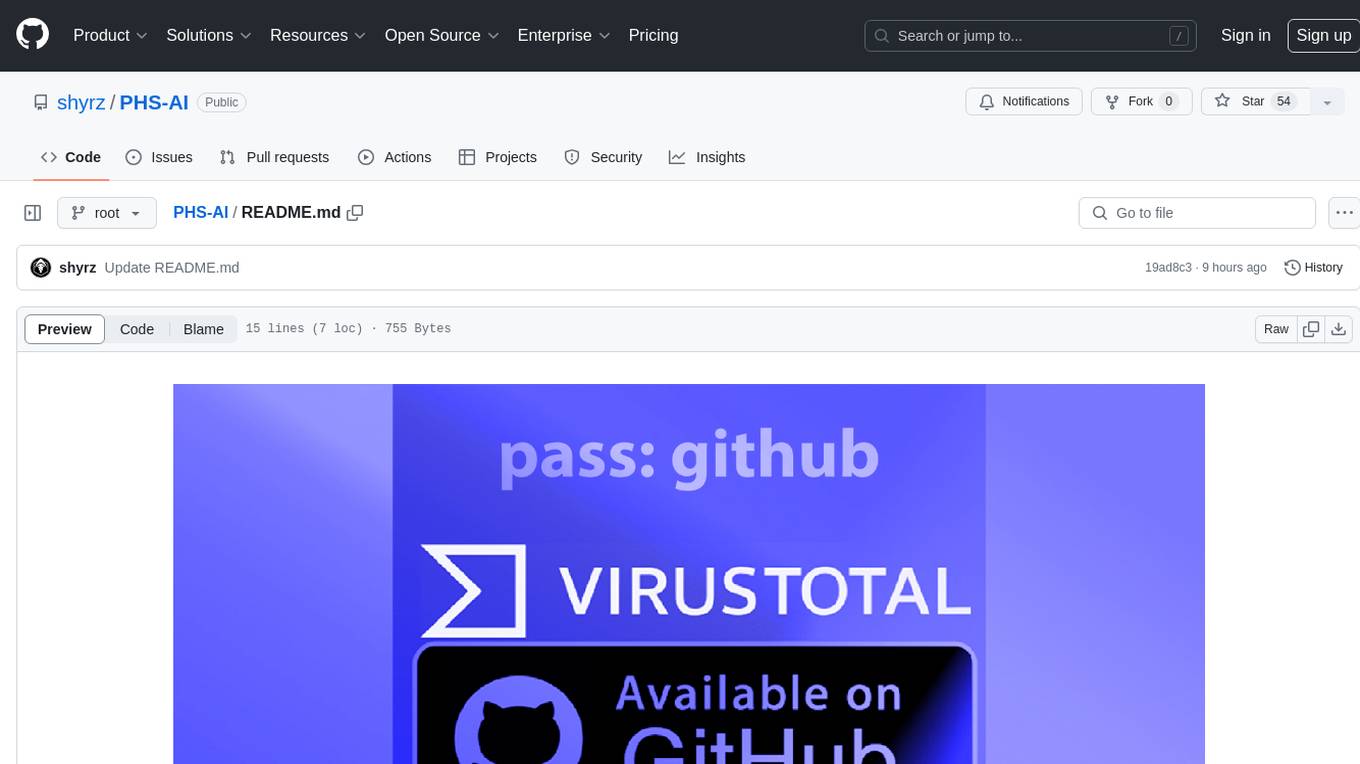
PHS-AI
PHS-AI is a project that provides functionality as is, without any warranties or commitments. Users are advised to exercise caution when using the code and conduct thorough testing before deploying in a production environment. The author assumes no responsibility for any losses or damages incurred through the use of this code. Feedback and contributions to improve the project are always welcome.
For similar jobs
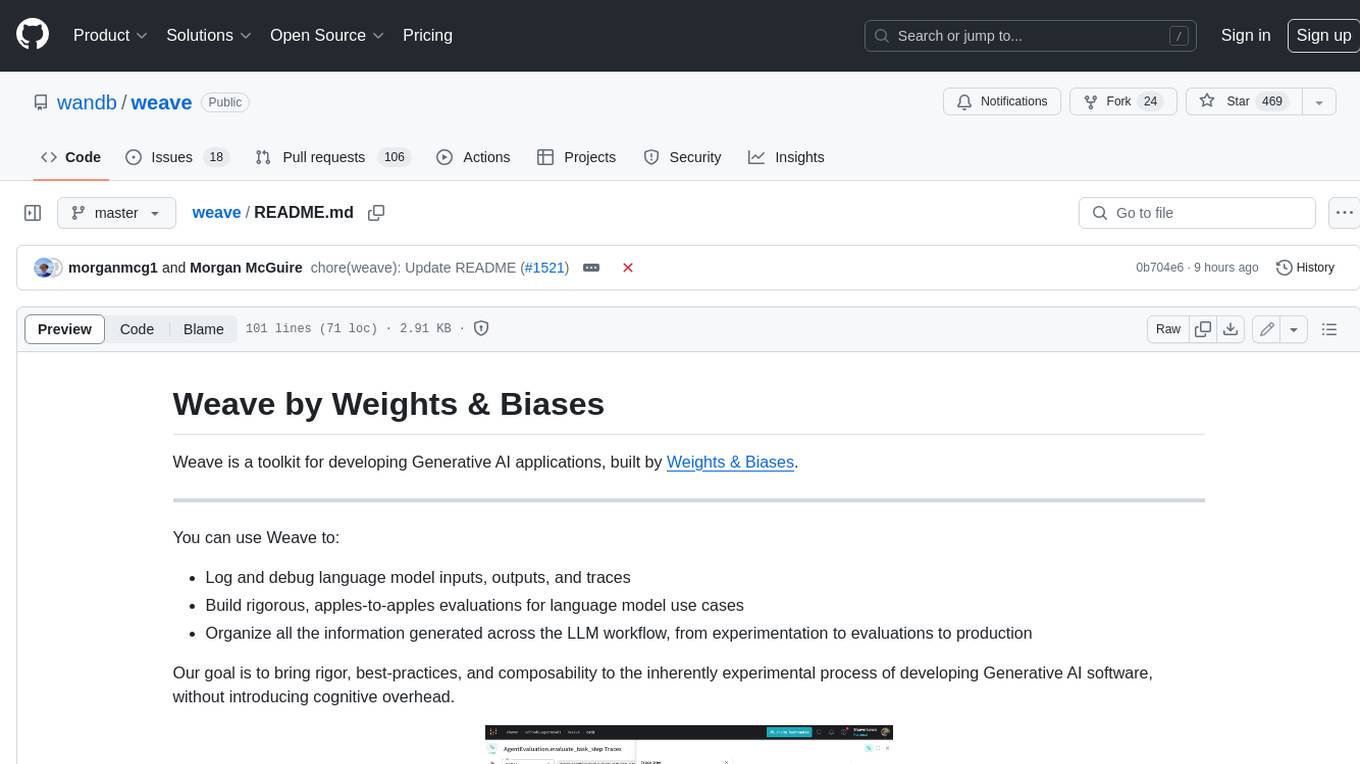
weave
Weave is a toolkit for developing Generative AI applications, built by Weights & Biases. With Weave, you can log and debug language model inputs, outputs, and traces; build rigorous, apples-to-apples evaluations for language model use cases; and organize all the information generated across the LLM workflow, from experimentation to evaluations to production. Weave aims to bring rigor, best-practices, and composability to the inherently experimental process of developing Generative AI software, without introducing cognitive overhead.
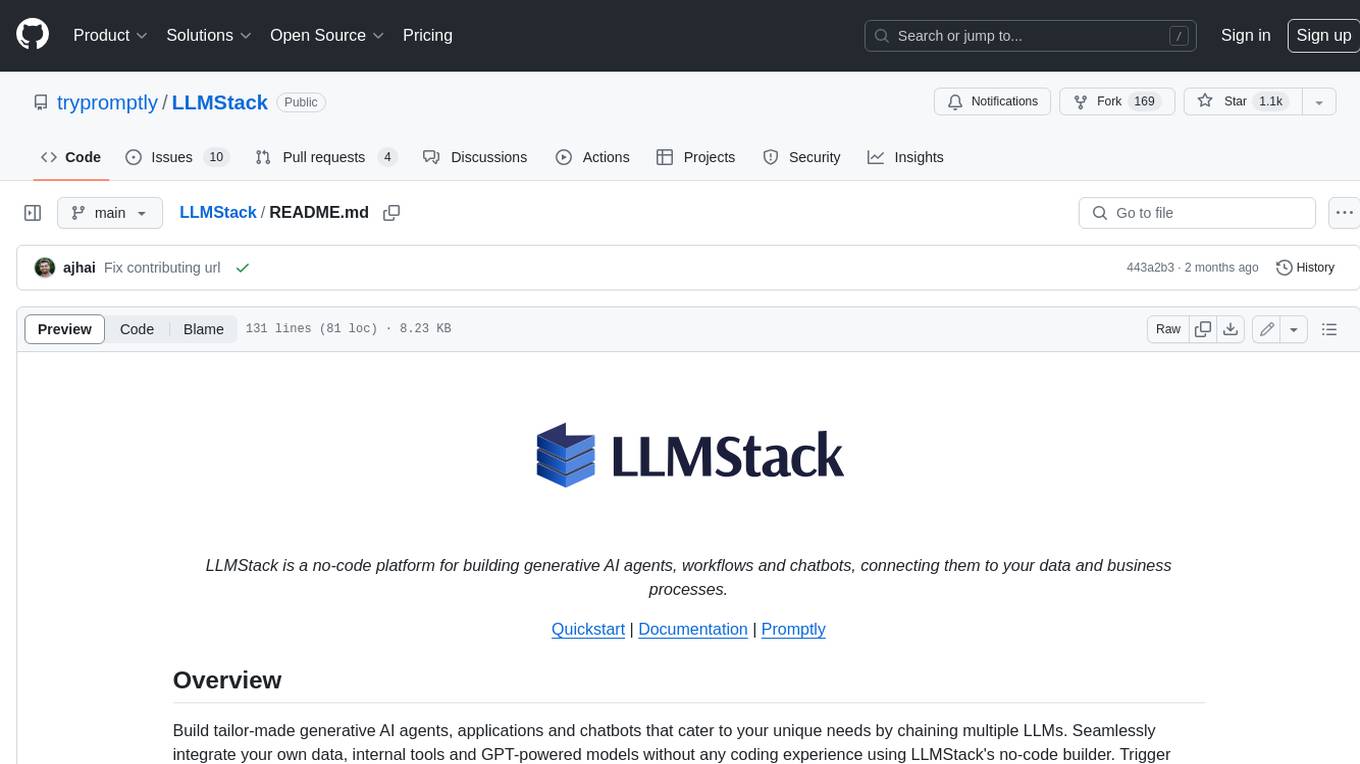
LLMStack
LLMStack is a no-code platform for building generative AI agents, workflows, and chatbots. It allows users to connect their own data, internal tools, and GPT-powered models without any coding experience. LLMStack can be deployed to the cloud or on-premise and can be accessed via HTTP API or triggered from Slack or Discord.
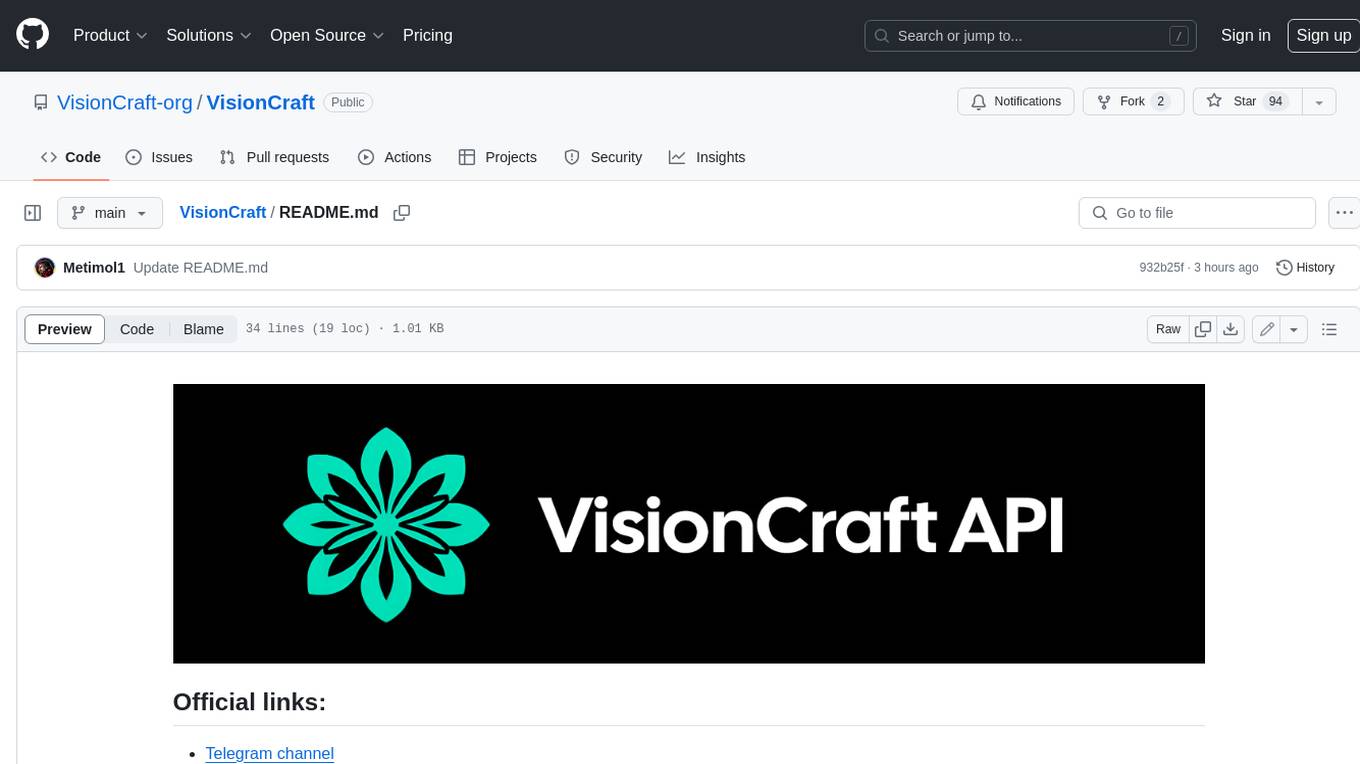
VisionCraft
The VisionCraft API is a free API for using over 100 different AI models. From images to sound.
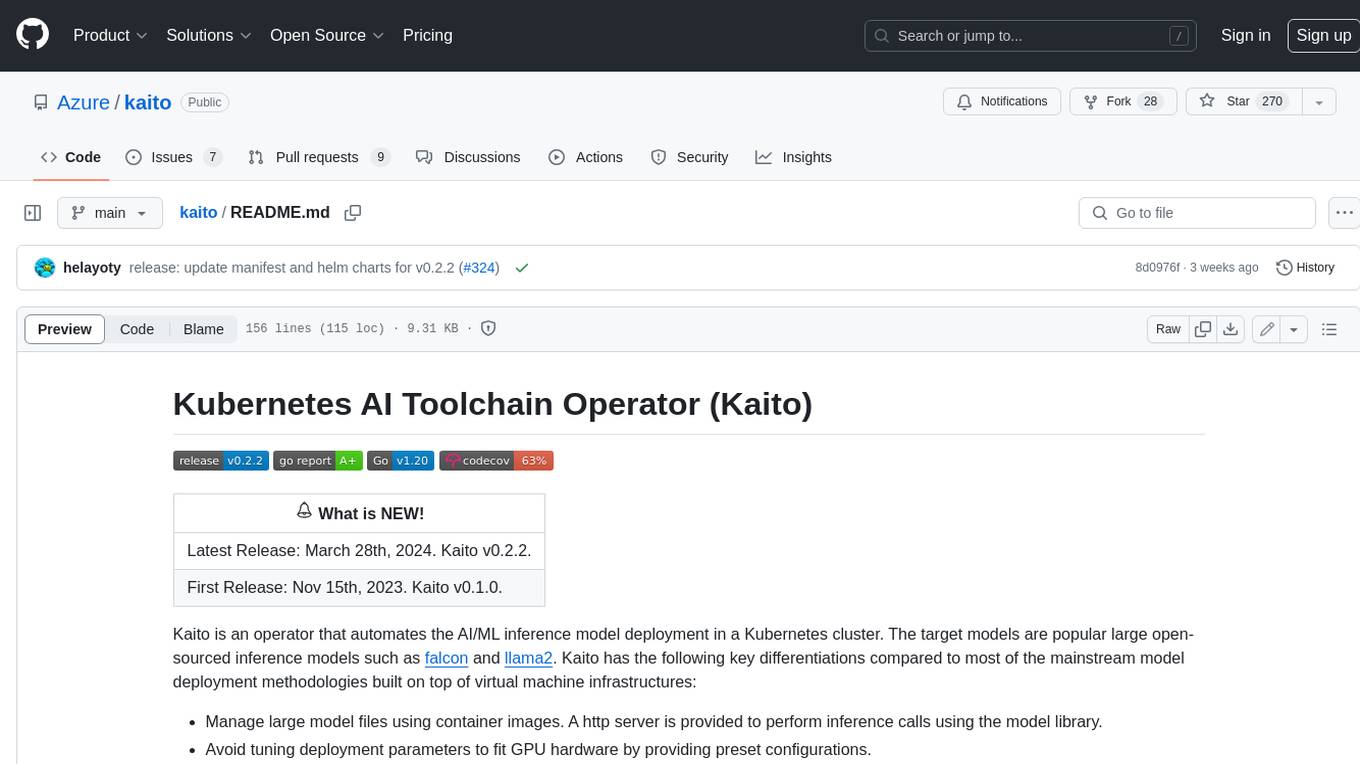
kaito
Kaito is an operator that automates the AI/ML inference model deployment in a Kubernetes cluster. It manages large model files using container images, avoids tuning deployment parameters to fit GPU hardware by providing preset configurations, auto-provisions GPU nodes based on model requirements, and hosts large model images in the public Microsoft Container Registry (MCR) if the license allows. Using Kaito, the workflow of onboarding large AI inference models in Kubernetes is largely simplified.
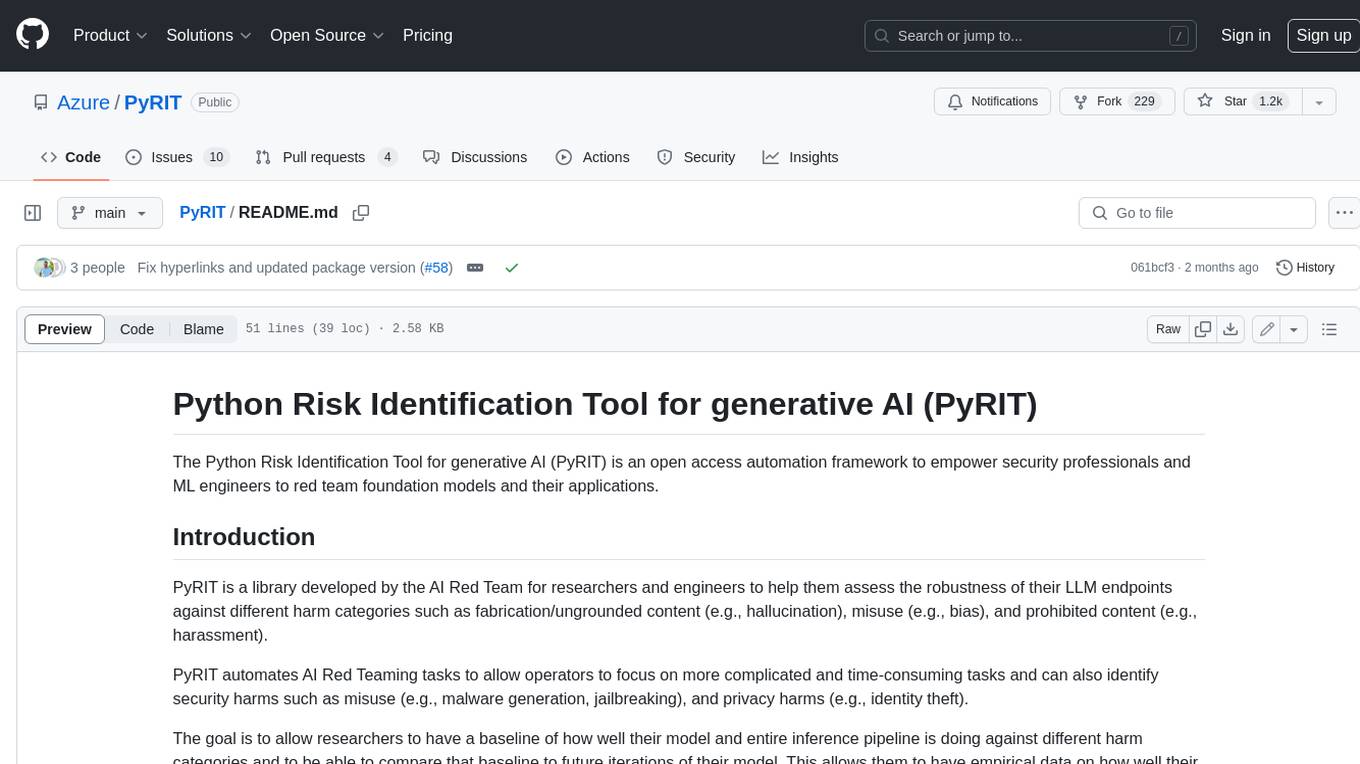
PyRIT
PyRIT is an open access automation framework designed to empower security professionals and ML engineers to red team foundation models and their applications. It automates AI Red Teaming tasks to allow operators to focus on more complicated and time-consuming tasks and can also identify security harms such as misuse (e.g., malware generation, jailbreaking), and privacy harms (e.g., identity theft). The goal is to allow researchers to have a baseline of how well their model and entire inference pipeline is doing against different harm categories and to be able to compare that baseline to future iterations of their model. This allows them to have empirical data on how well their model is doing today, and detect any degradation of performance based on future improvements.
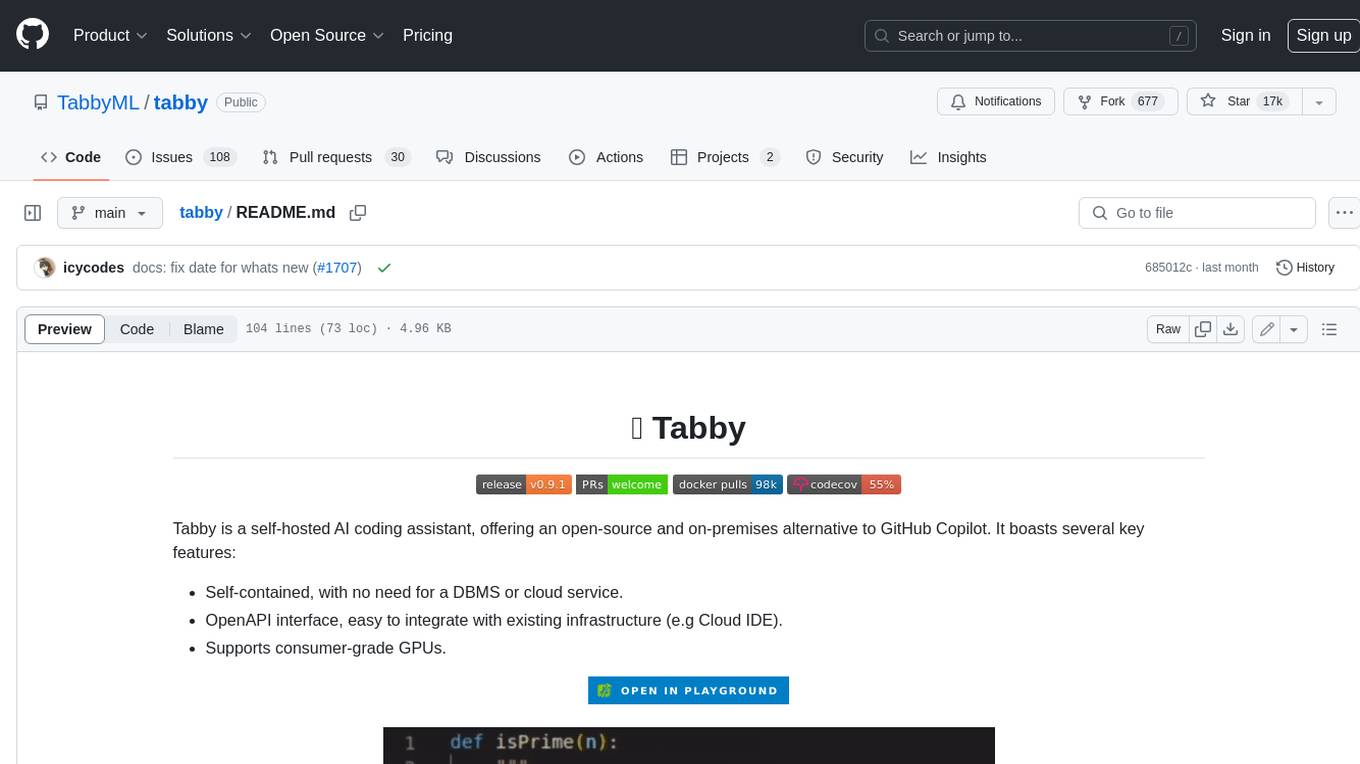
tabby
Tabby is a self-hosted AI coding assistant, offering an open-source and on-premises alternative to GitHub Copilot. It boasts several key features: * Self-contained, with no need for a DBMS or cloud service. * OpenAPI interface, easy to integrate with existing infrastructure (e.g Cloud IDE). * Supports consumer-grade GPUs.
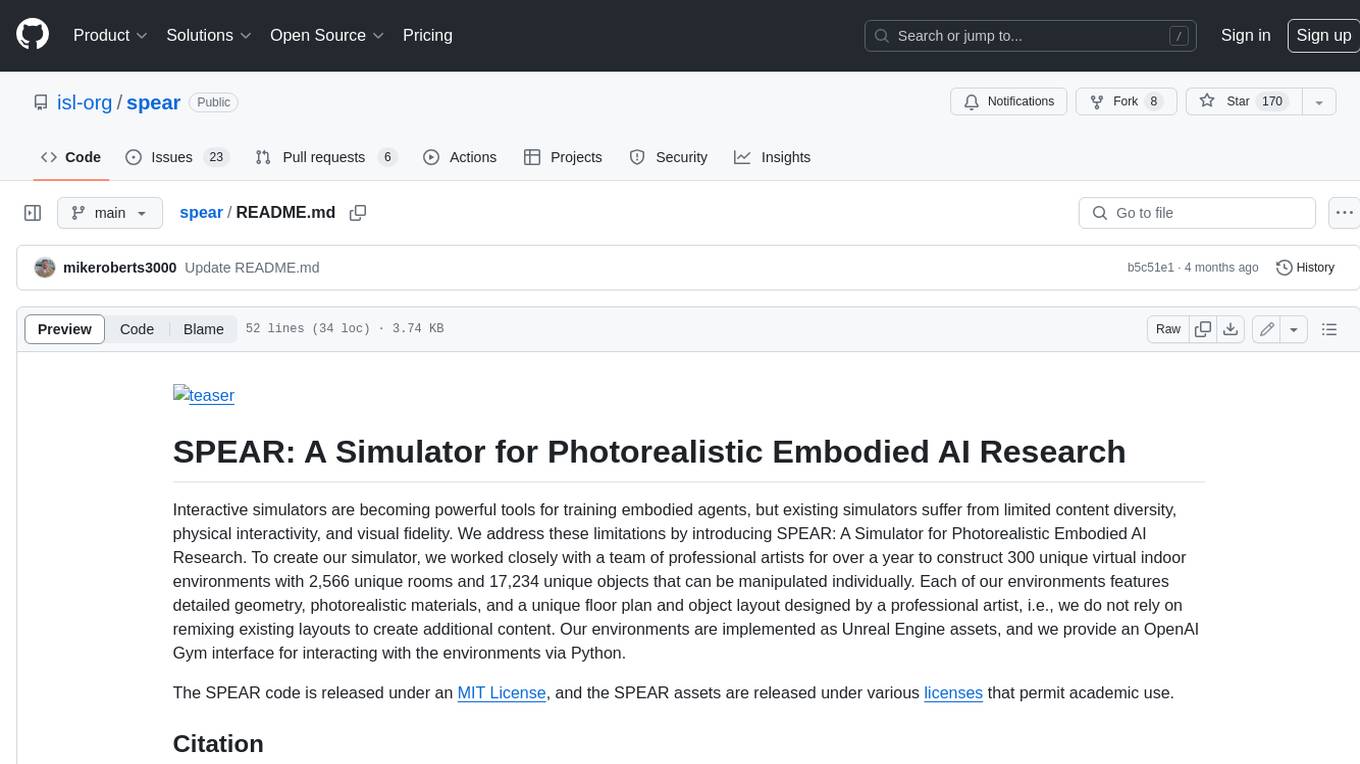
spear
SPEAR (Simulator for Photorealistic Embodied AI Research) is a powerful tool for training embodied agents. It features 300 unique virtual indoor environments with 2,566 unique rooms and 17,234 unique objects that can be manipulated individually. Each environment is designed by a professional artist and features detailed geometry, photorealistic materials, and a unique floor plan and object layout. SPEAR is implemented as Unreal Engine assets and provides an OpenAI Gym interface for interacting with the environments via Python.
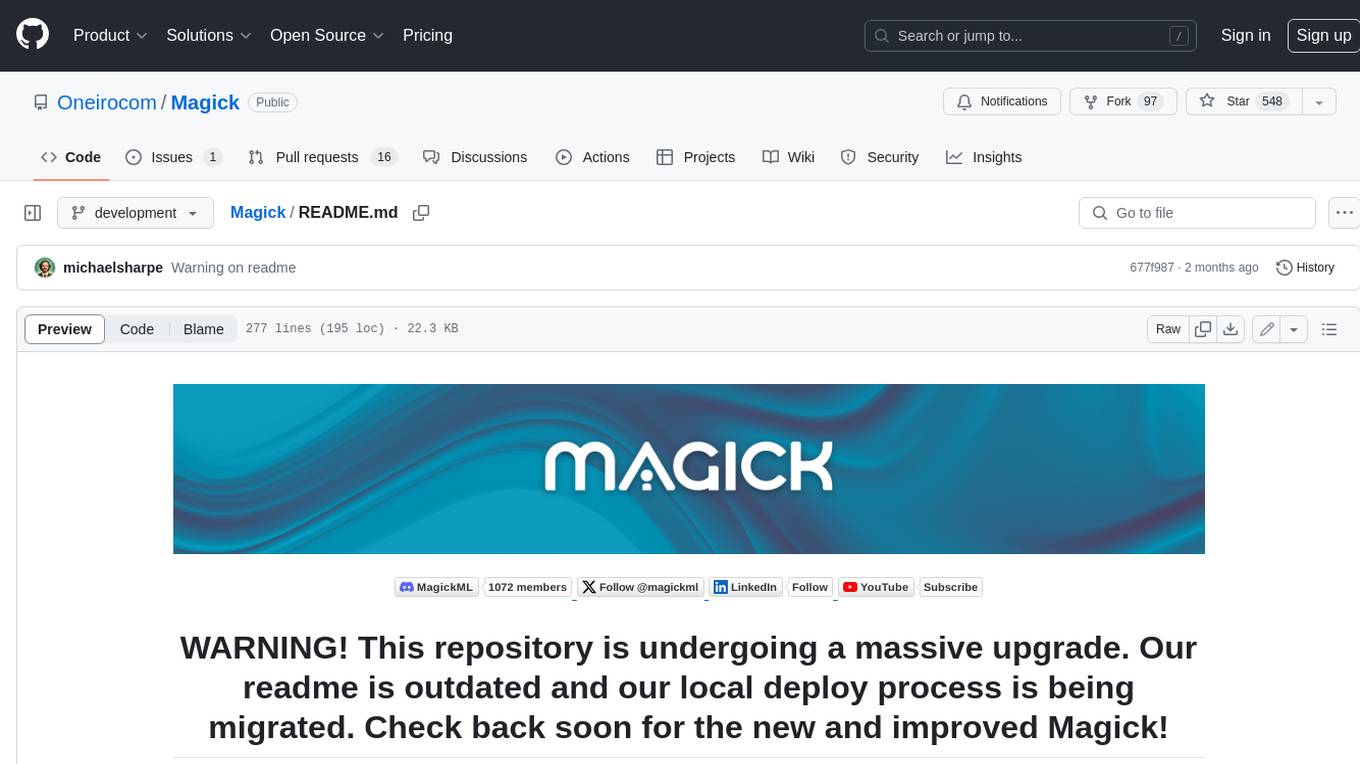
Magick
Magick is a groundbreaking visual AIDE (Artificial Intelligence Development Environment) for no-code data pipelines and multimodal agents. Magick can connect to other services and comes with nodes and templates well-suited for intelligent agents, chatbots, complex reasoning systems and realistic characters.