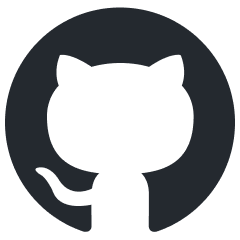
SageAttention
Quantized Attention that achieves speedups of 2.1-3.1x and 2.7-5.1x compared to FlashAttention2 and xformers, respectively, without lossing end-to-end metrics across various models.
Stars: 897
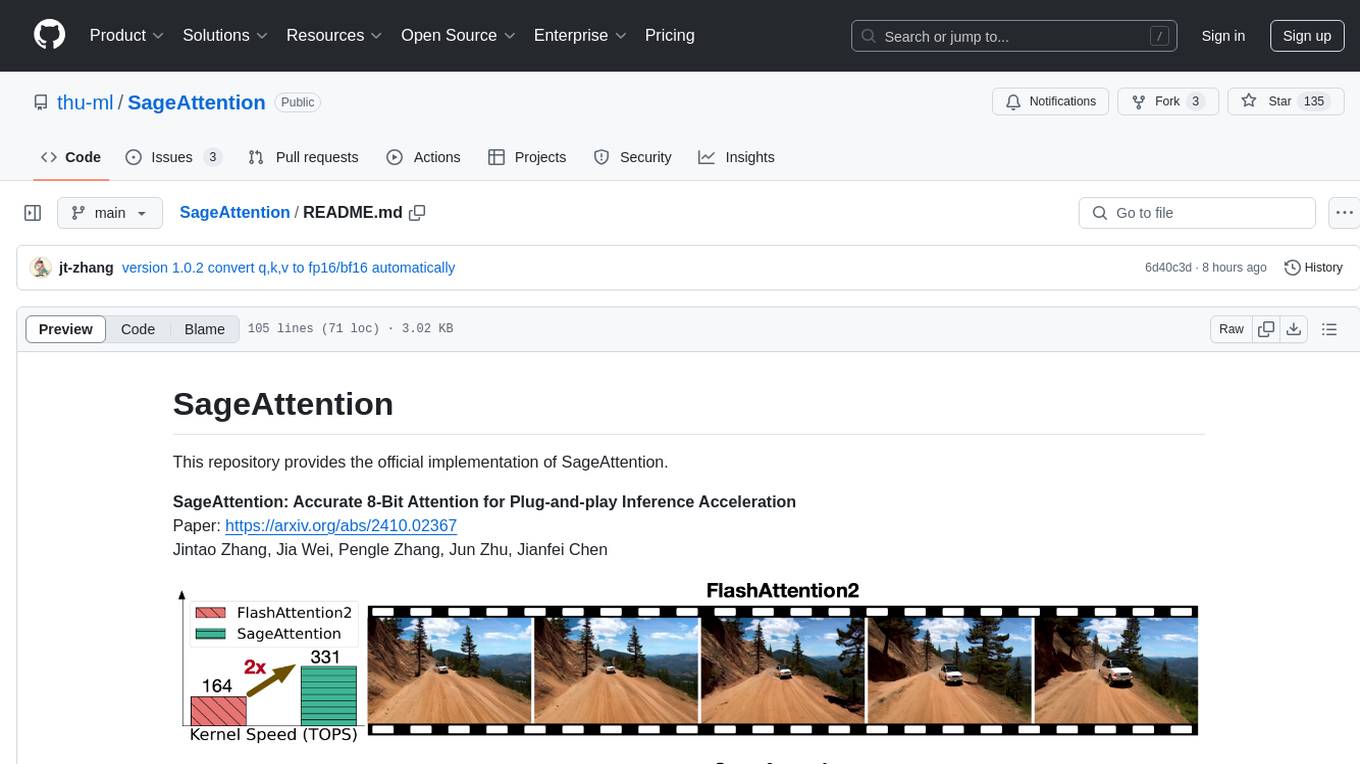
SageAttention is an official implementation of an accurate 8-bit attention mechanism for plug-and-play inference acceleration. It is optimized for RTX4090 and RTX3090 GPUs, providing performance improvements for specific GPU architectures. The tool offers a technique called 'smooth_k' to ensure accuracy in processing FP16/BF16 data. Users can easily replace 'scaled_dot_product_attention' with SageAttention for faster video processing.
README:
We are continuously updating more features. You could Star and Watch our repository to stay updated.
This repository provides the official implementation of SageAttention and SageAttention2.
SageAttention: Accurate 8-Bit Attention for Plug-and-play Inference Acceleration
Paper: https://arxiv.org/abs/2410.02367
Jintao Zhang, Jia Wei, Haofeng Huang, Pengle Zhang, Jun Zhu, Jianfei Chen
SageAttention2: Efficient Attention with Thorough Outlier Smoothing and Per-thread INT4 Quantization
Paper: https://arxiv.org/abs/2411.10958
Jintao Zhang, Haofeng Huang, Pengle Zhang, Jia Wei, Jun Zhu, Jianfei Chen
This is a beta release of SageAttention2. We welcome any feedback on accuracy, performance issues, bugs, feature requests, or suggestions. Please feel free to open an issue or launch a pull request!
Current Features:
- INT8 quantization for $QK^\top$ with support for varying granularities
- FP8 quantization for $PV$
- FP32 buffer for $PV$ to improve accuracy in FP8 MMA
- Support
torch.compile
with non-cudagraphs mode and distributed inference
For a stable version, please use the branch of SageAttention-1 branch.
- News [2024-12-20]: Update the SageAttention2 Paper.
- News [2024-12-20]: We are excited to announce the release of SageAttention 2.0.1 Beta! In this version, we introduce a new feature: per-thread quantization, which offers finer granularity while maintaining hardware efficiency.
- News [2024-11-21]: SageAttention 2.0.0 beta is released! Now SageAttention has measured speedup on L20, L40, A100, A800, and A6000 other than RTX3090 and RTX4090.
-
News [2024-11-12]: Support for
sageattn_varlen
is available now. -
News [2024-11-11]: Support for different sequence lengths between
q
andk,v
,(batch_size, head_num, seq_len, head_dim)
or(batch_size, seq_len, head_num, head_dim)
input shapes, andgroup-query attention
is available now.
python>=3.9
torch>=2.3.0
triton>=3.0.0
-
CUDA>=12.4
if you want to use fp8 elseCUDA>=12.0
-
flash-attn
for benchmarking
For the stable version or Triton-only version, refer to SageAttention-1 and install using pip:
pip install sageattention==1.0.6
To use SageAttention 2.0.1, please compile from source:
git clone https://github.com/thu-ml/SageAttention.git
cd sageattention
python setup.py install # or pip install -e .
Note: Currently, SageAttention is optimized for excellent performance on RTX4090, RTX3090, L20, and L40 GPUs. On A100, A800, and A6000 GPUs, performance is best with a
head_dim=128
, whilehead_dim=64
is less optimal. Similarly, performance on the Hopper architecture is currently not optimal. We are actively working to enhance performance in these configurations.
from sageattention import sageattn
attn_output = sageattn(q, k, v, tensor_layout="HND", is_causal=False)
-
q, k, v
are FP16/BF16 dtype with the shape(batch_size, head_num, seq_len, head_dim)
using defaulttensor_layout="HND"
. For shape(batch_size, seq_len, head_num, head_dim)
, settensor_layout="NHD"
. -
is_causal
determines the use of a causal mask.
-
sageattn
: Automatically selects the optimal kernel based on the GPU to achieve a good performance-accuracy trade-off. -
sageattn_qk_int8_pv_fp16_triton
: INT8 quantization for $QK^\top$ and FP16 for $PV$ using Triton backend. -
sageattn_qk_int8_pv_fp16_cuda
: INT8 quantization for $QK^\top$ and FP16 for $PV$ using CUDA backend. -
sageattn_qk_int8_pv_fp8_cuda
: INT8 quantization for $QK^\top$ and FP8 for $PV$ using CUDA backend. -
sageattn_varlen
: INT8 quantization for $QK^\top$ and FP16 for $PV$ using Triton backend. Support for varying sequence lengths within the same batch.
For optimal speed and accuracy performance on custom devices and models, we strongly recommend referring to the this file for detailed guidance.
Note: Support for different sequence lengths between
q
andk,v
andgroup-query attention
is available.
We can replace scaled_dot_product_attention
easily.
We will take CogvideoX as an example:
Add the following codes and run
from sageattention import sageattn
import torch.nn.functional as F
F.scaled_dot_product_attention = sageattn
Specifically,
cd example
python sageattn_cogvideo.py --compile
You can get a lossless video in ./example
faster than by using python original_cogvideo.py --compile
Note: Not all models works with
F.scaled_dot_product_attention = sageattn
. Technically, you should replace the original Attention by modifying theAttention Class
of the target model. For image and video models, we suggest only replacing the attention in DiT.
8+8
means the kernel with INT8 quantization for $QK^\top$ and FP8 quantization for $PV$. 8+16
uses FP16 with FP16 accumulator for $PV$.
Note: The TOPS results refer only to the Attention Kernel, excluding the quantization and smoothing.
If you use this code or find our work valuable, please cite:
@inproceedings{zhang2025sageattention,
title={SageAttention: Accurate 8-Bit Attention for Plug-and-play Inference Acceleration},
author={Zhang, Jintao and Wei, Jia and Zhang, Pengle and Zhu, Jun and Chen, Jianfei},
booktitle={International Conference on Learning Representations (ICLR)},
year={2025}
}
@misc{zhang2024sageattention2,
title={SageAttention2: Efficient Attention with Thorough Outlier Smoothing and Per-thread INT4 Quantization},
author={Jintao Zhang and Haofeng Huang and Pengle Zhang and Jia Wei and Jun Zhu and Jianfei Chen},
year={2024},
eprint={2411.10958},
archivePrefix={arXiv},
primaryClass={cs.LG},
url={https://arxiv.org/abs/2411.10958},
}
For Tasks:
Click tags to check more tools for each tasksFor Jobs:
Alternative AI tools for SageAttention
Similar Open Source Tools
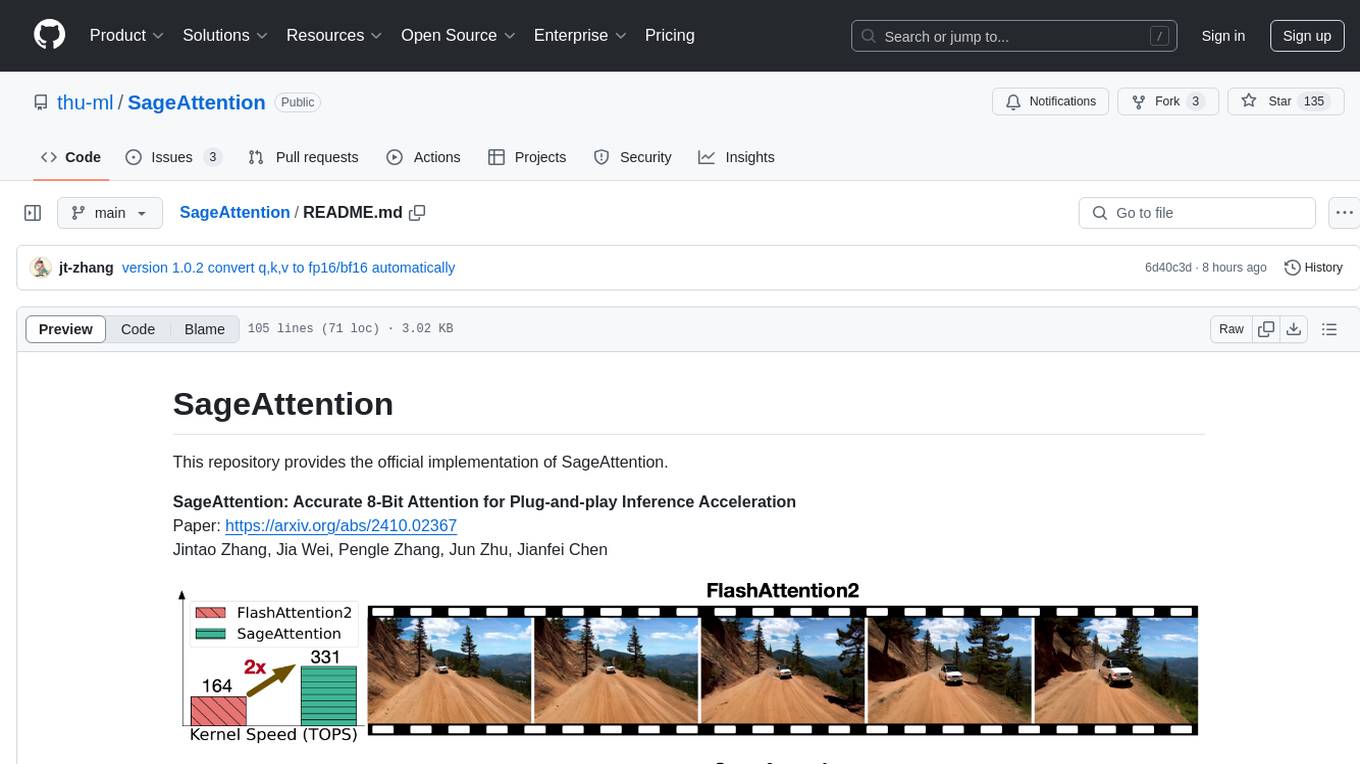
SageAttention
SageAttention is an official implementation of an accurate 8-bit attention mechanism for plug-and-play inference acceleration. It is optimized for RTX4090 and RTX3090 GPUs, providing performance improvements for specific GPU architectures. The tool offers a technique called 'smooth_k' to ensure accuracy in processing FP16/BF16 data. Users can easily replace 'scaled_dot_product_attention' with SageAttention for faster video processing.
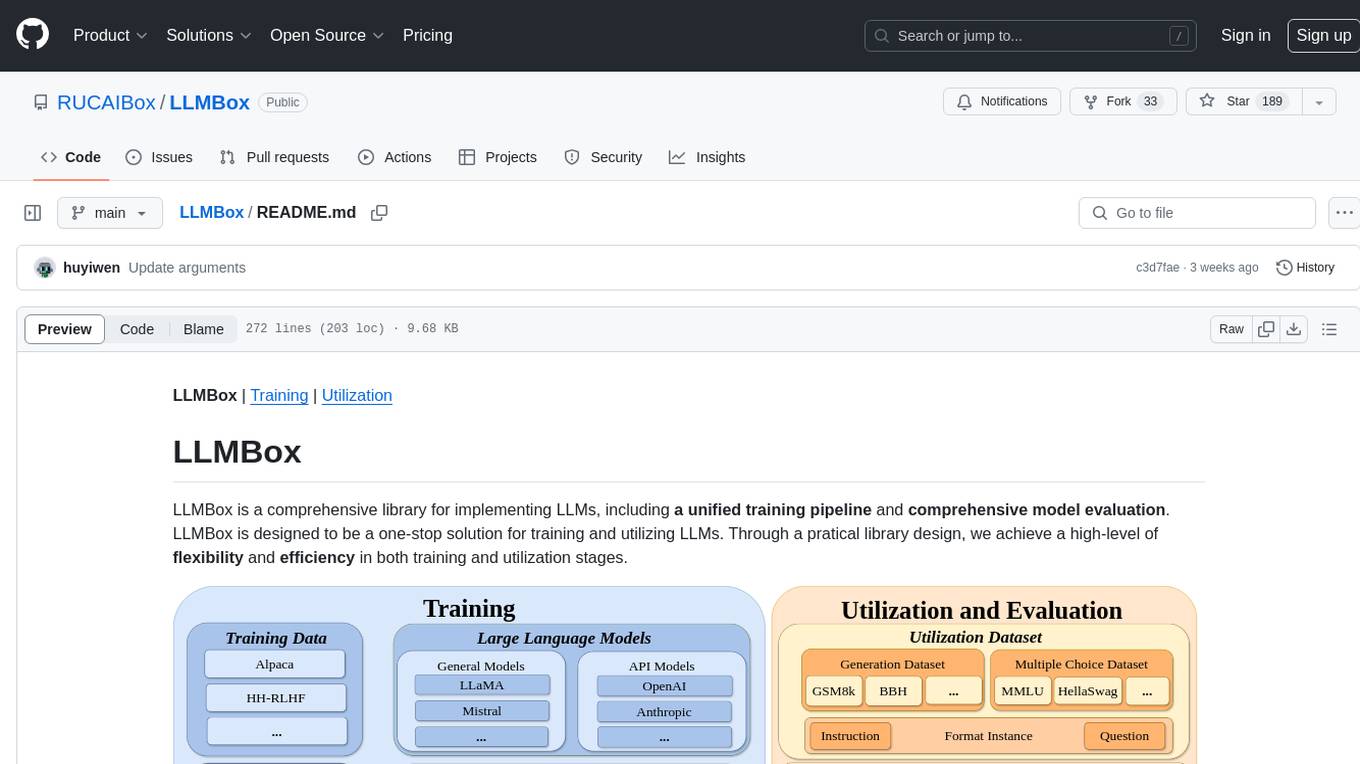
LLMBox
LLMBox is a comprehensive library designed for implementing Large Language Models (LLMs) with a focus on a unified training pipeline and comprehensive model evaluation. It serves as a one-stop solution for training and utilizing LLMs, offering flexibility and efficiency in both training and utilization stages. The library supports diverse training strategies, comprehensive datasets, tokenizer vocabulary merging, data construction strategies, parameter efficient fine-tuning, and efficient training methods. For utilization, LLMBox provides comprehensive evaluation on various datasets, in-context learning strategies, chain-of-thought evaluation, evaluation methods, prefix caching for faster inference, support for specific LLM models like vLLM and Flash Attention, and quantization options. The tool is suitable for researchers and developers working with LLMs for natural language processing tasks.
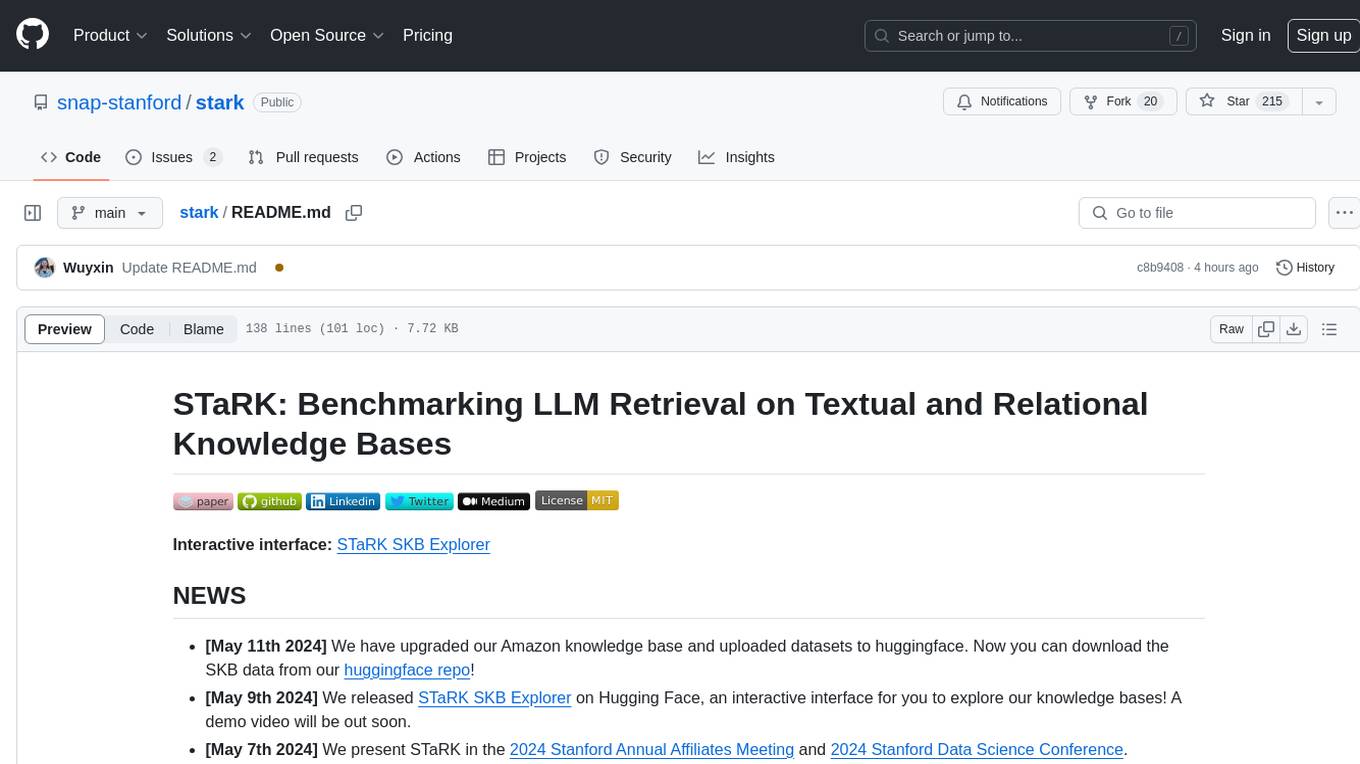
stark
STaRK is a large-scale semi-structure retrieval benchmark on Textual and Relational Knowledge Bases. It provides natural-sounding and practical queries crafted to incorporate rich relational information and complex textual properties, closely mirroring real-life scenarios. The benchmark aims to assess how effectively large language models can handle the interplay between textual and relational requirements in queries, using three diverse knowledge bases constructed from public sources.
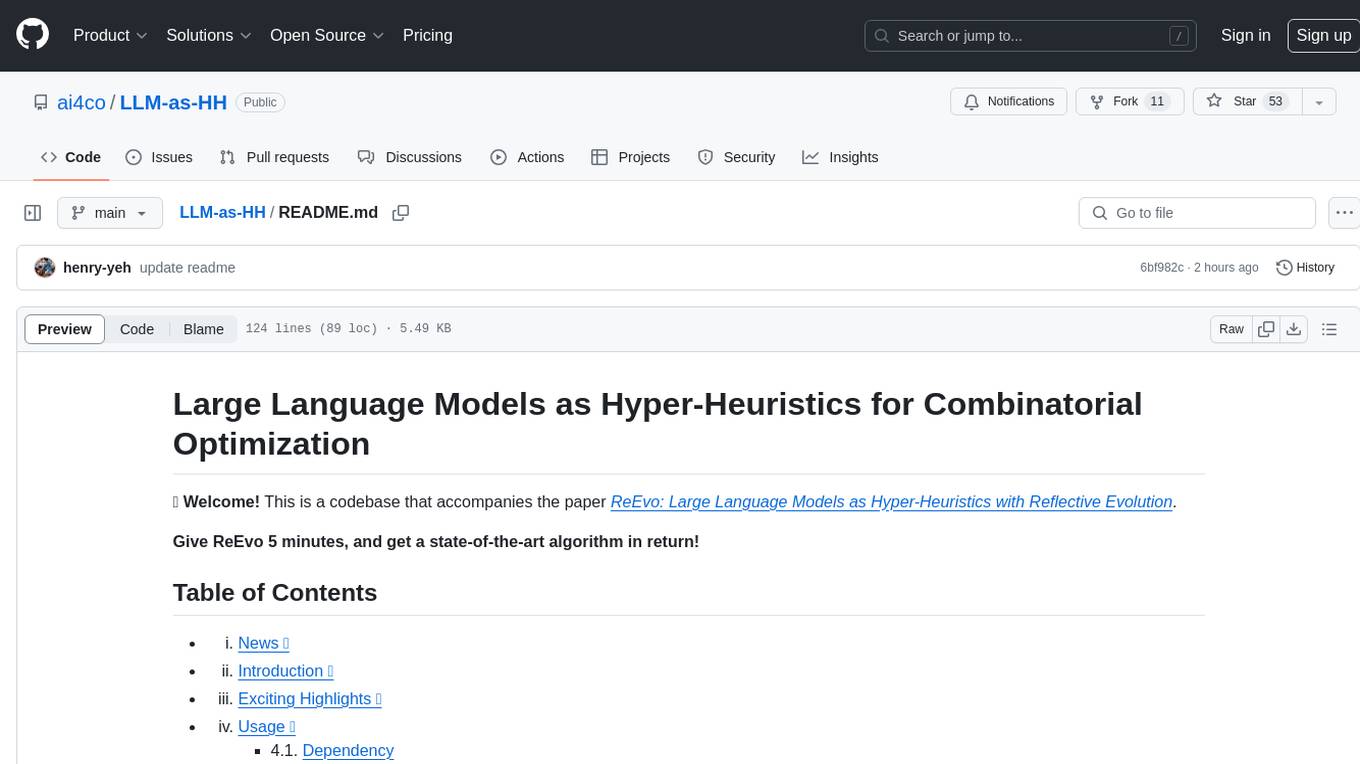
LLM-as-HH
LLM-as-HH is a codebase that accompanies the paper ReEvo: Large Language Models as Hyper-Heuristics with Reflective Evolution. It introduces Language Hyper-Heuristics (LHHs) that leverage LLMs for heuristic generation with minimal manual intervention and open-ended heuristic spaces. Reflective Evolution (ReEvo) is presented as a searching framework that emulates the reflective design approach of human experts while surpassing human capabilities with scalable LLM inference, Internet-scale domain knowledge, and powerful evolutionary search. The tool can improve various algorithms on problems like Traveling Salesman Problem, Capacitated Vehicle Routing Problem, Orienteering Problem, Multiple Knapsack Problems, Bin Packing Problem, and Decap Placement Problem in both black-box and white-box settings.
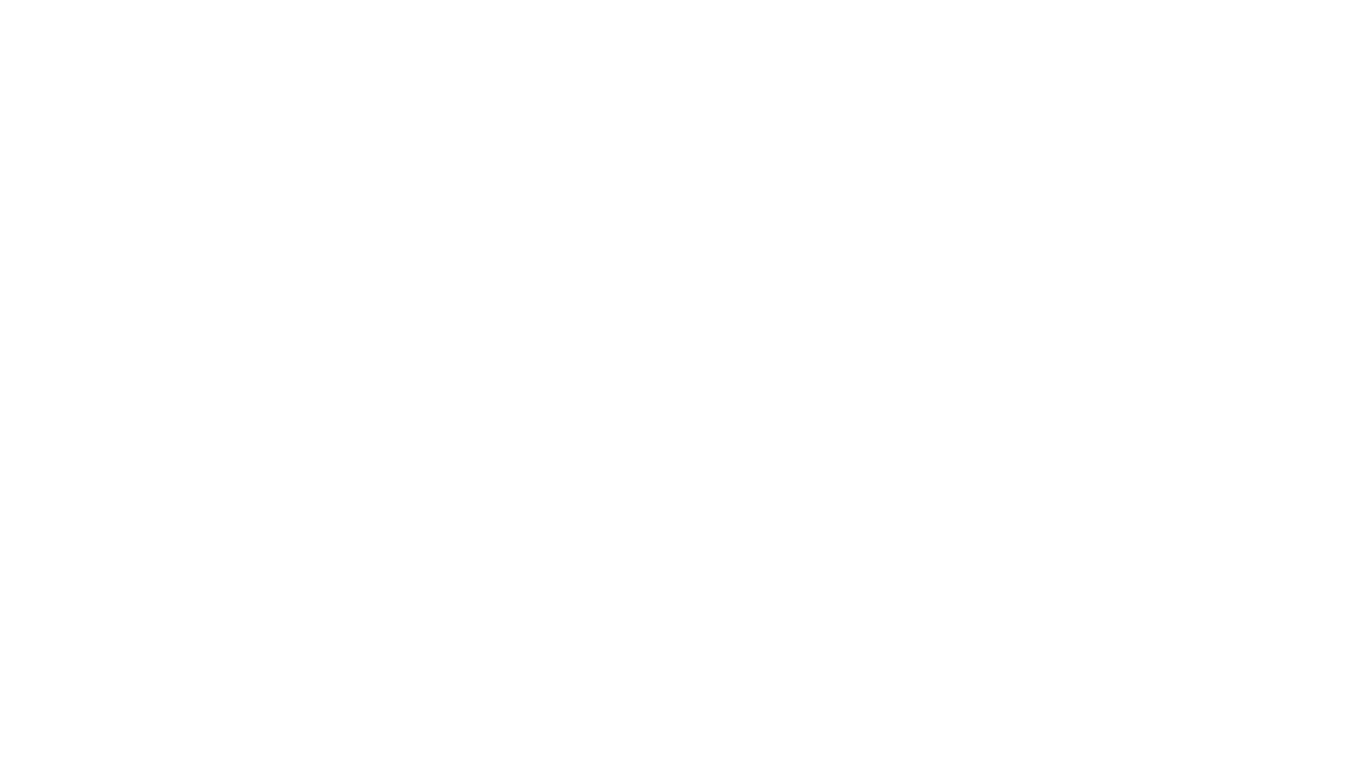
upgini
Upgini is an intelligent data search engine with a Python library that helps users find and add relevant features to their ML pipeline from various public, community, and premium external data sources. It automates the optimization of connected data sources by generating an optimal set of machine learning features using large language models, GraphNNs, and recurrent neural networks. The tool aims to simplify feature search and enrichment for external data to make it a standard approach in machine learning pipelines. It democratizes access to data sources for the data science community.
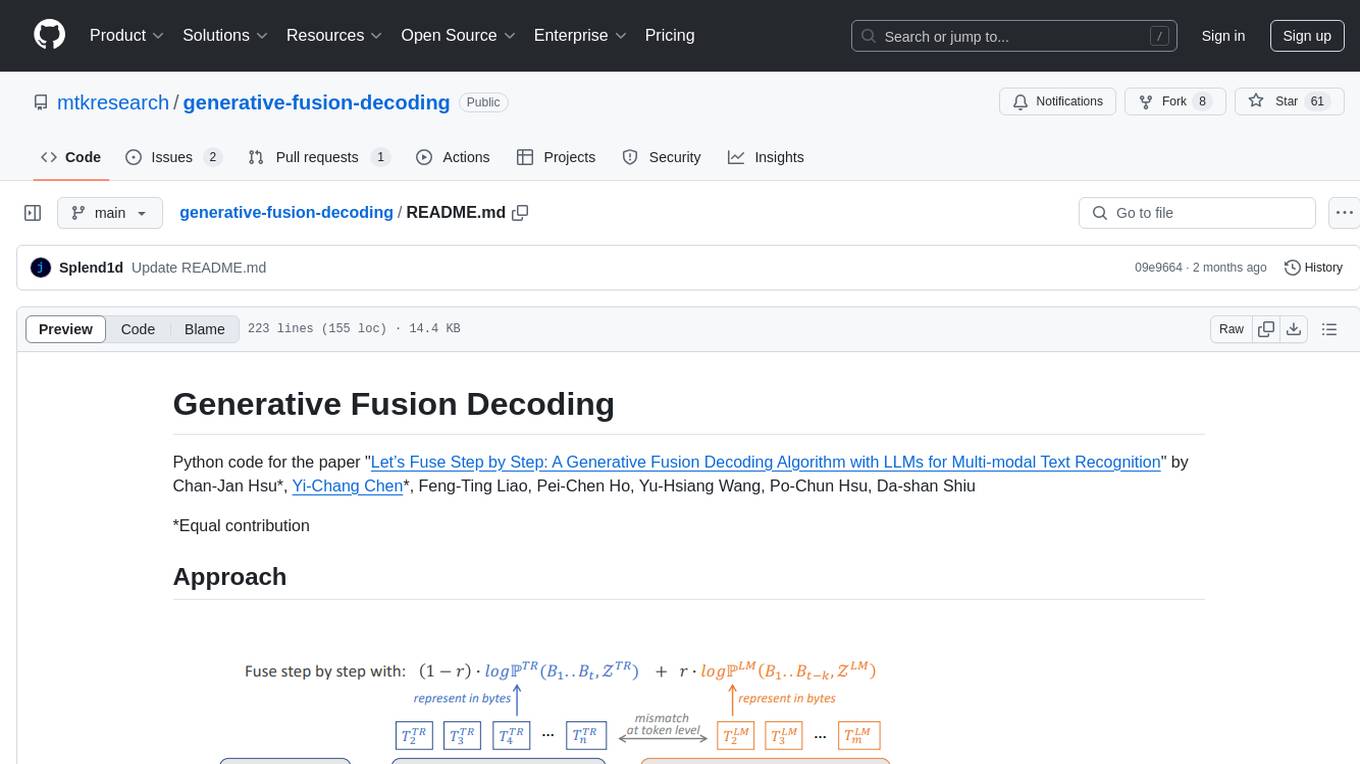
generative-fusion-decoding
Generative Fusion Decoding (GFD) is a novel shallow fusion framework that integrates Large Language Models (LLMs) into multi-modal text recognition systems such as automatic speech recognition (ASR) and optical character recognition (OCR). GFD operates across mismatched token spaces of different models by mapping text token space to byte token space, enabling seamless fusion during the decoding process. It simplifies the complexity of aligning different model sample spaces, allows LLMs to correct errors in tandem with the recognition model, increases robustness in long-form speech recognition, and enables fusing recognition models deficient in Chinese text recognition with LLMs extensively trained on Chinese. GFD significantly improves performance in ASR and OCR tasks, offering a unified solution for leveraging existing pre-trained models through step-by-step fusion.
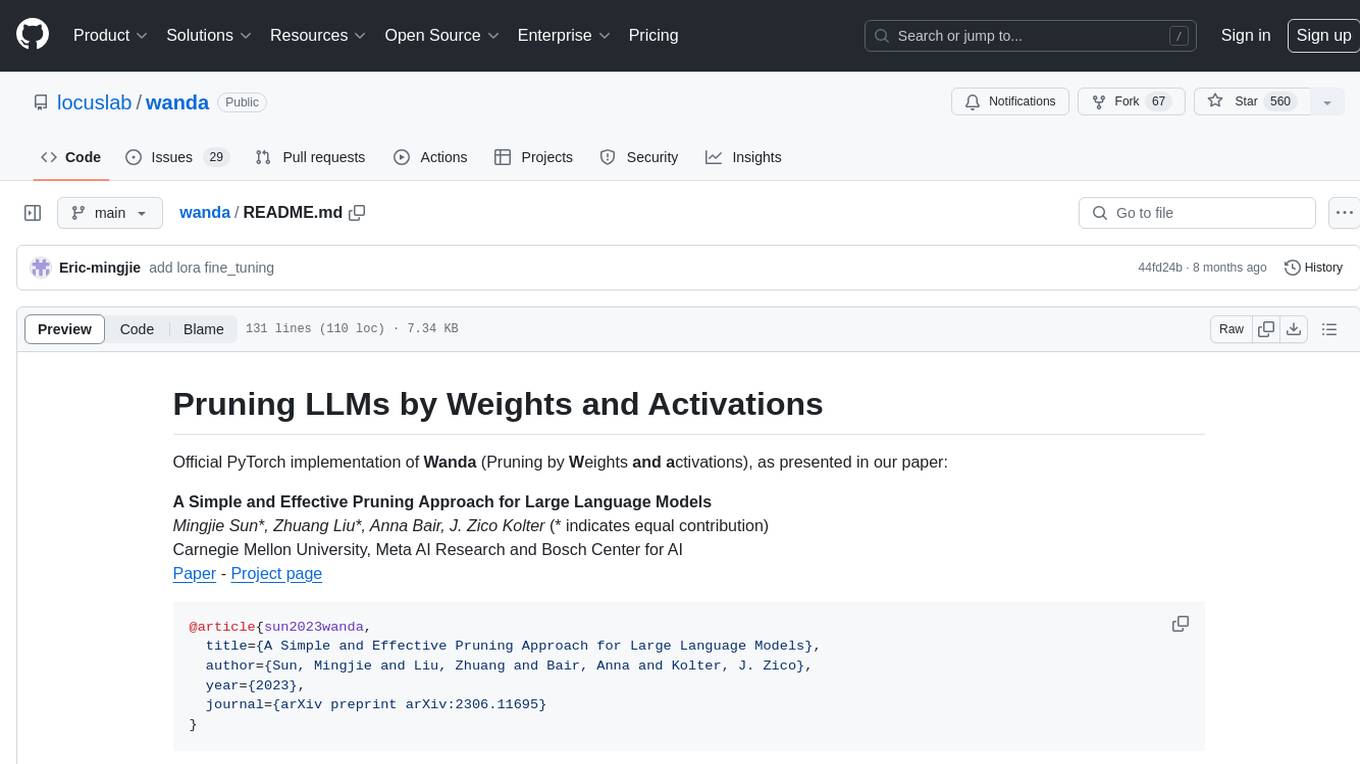
wanda
Official PyTorch implementation of Wanda (Pruning by Weights and Activations), a simple and effective pruning approach for large language models. The pruning approach removes weights on a per-output basis, by the product of weight magnitudes and input activation norms. The repository provides support for various features such as LLaMA-2, ablation study on OBS weight update, zero-shot evaluation, and speedup evaluation. Users can replicate main results from the paper using provided bash commands. The tool aims to enhance the efficiency and performance of language models through structured and unstructured sparsity techniques.
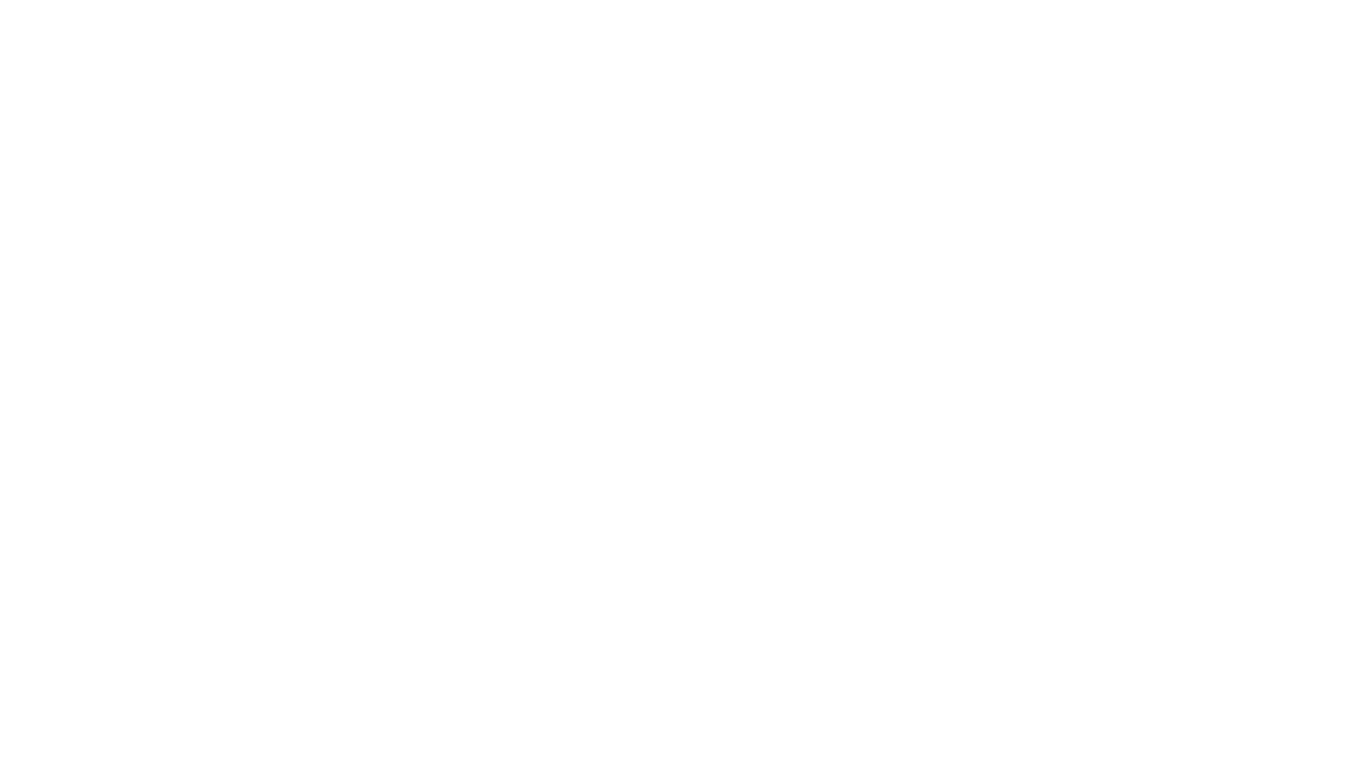
shellChatGPT
ShellChatGPT is a shell wrapper for OpenAI's ChatGPT, DALL-E, Whisper, and TTS, featuring integration with LocalAI, Ollama, Gemini, Mistral, Groq, and GitHub Models. It provides text and chat completions, vision, reasoning, and audio models, voice-in and voice-out chatting mode, text editor interface, markdown rendering support, session management, instruction prompt manager, integration with various service providers, command line completion, file picker dialogs, color scheme personalization, stdin and text file input support, and compatibility with Linux, FreeBSD, MacOS, and Termux for a responsive experience.
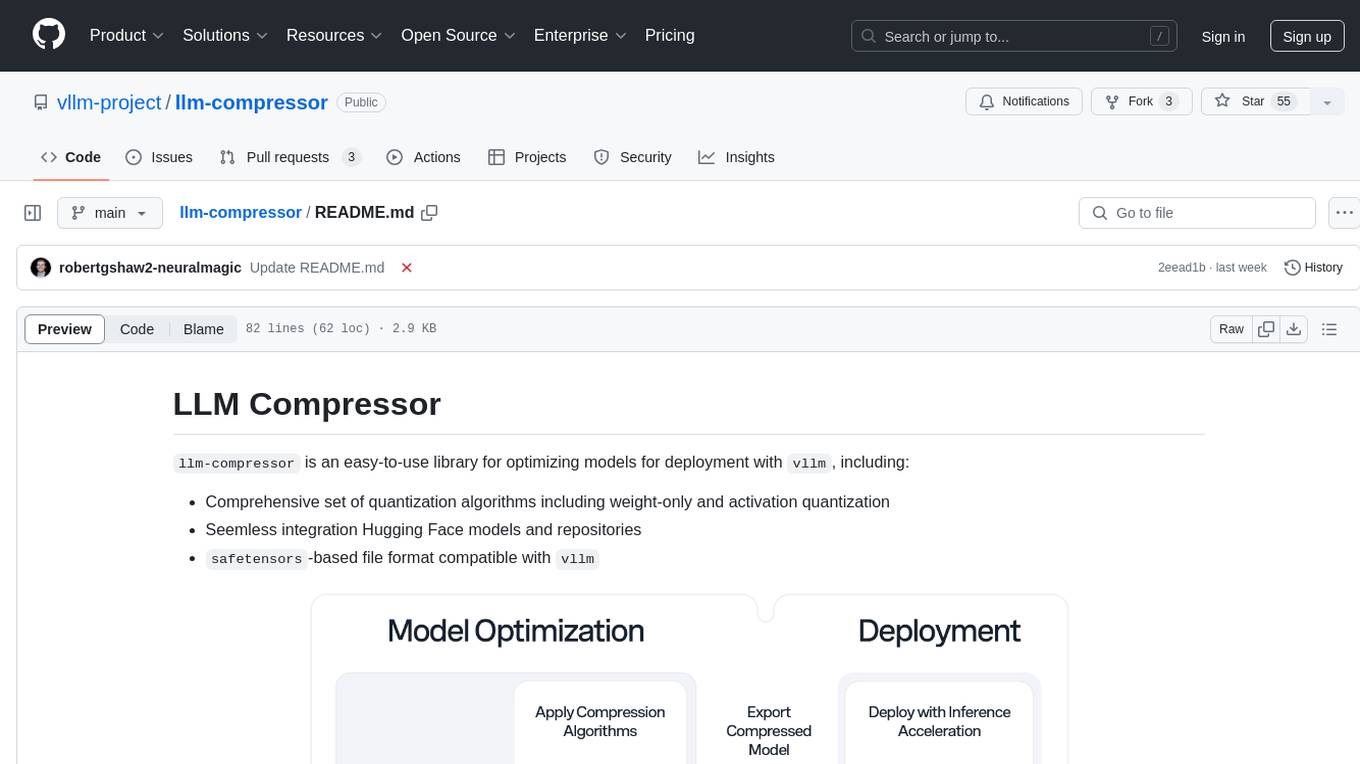
llm-compressor
llm-compressor is an easy-to-use library for optimizing models for deployment with vllm. It provides a comprehensive set of quantization algorithms, seamless integration with Hugging Face models and repositories, and supports mixed precision, activation quantization, and sparsity. Supported algorithms include PTQ, GPTQ, SmoothQuant, and SparseGPT. Installation can be done via git clone and local pip install. Compression can be easily applied by selecting an algorithm and calling the oneshot API. The library also offers end-to-end examples for model compression. Contributions to the code, examples, integrations, and documentation are appreciated.
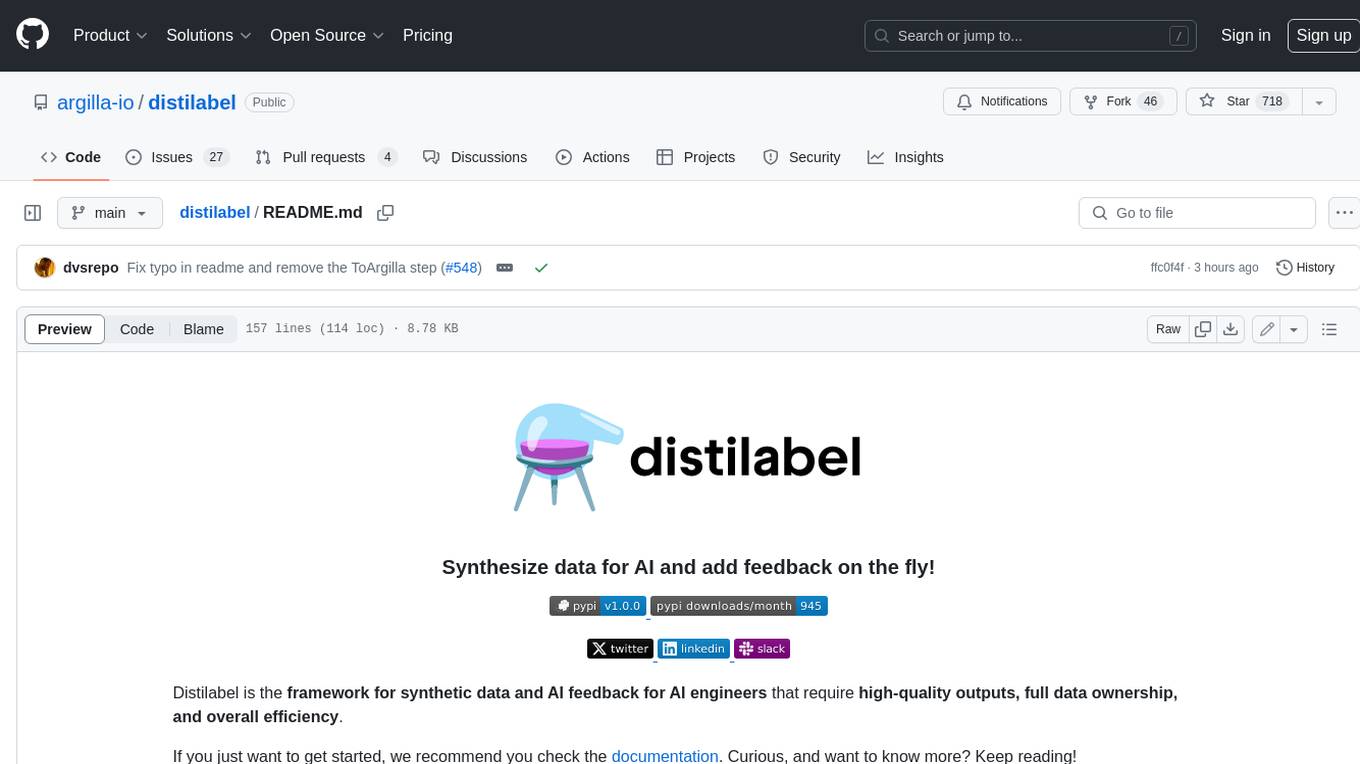
distilabel
Distilabel is a framework for synthetic data and AI feedback for AI engineers that require high-quality outputs, full data ownership, and overall efficiency. It helps you synthesize data and provide AI feedback to improve the quality of your AI models. With Distilabel, you can: * **Synthesize data:** Generate synthetic data to train your AI models. This can help you to overcome the challenges of data scarcity and bias. * **Provide AI feedback:** Get feedback from AI models on your data. This can help you to identify errors and improve the quality of your data. * **Improve your AI output quality:** By using Distilabel to synthesize data and provide AI feedback, you can improve the quality of your AI models and get better results.
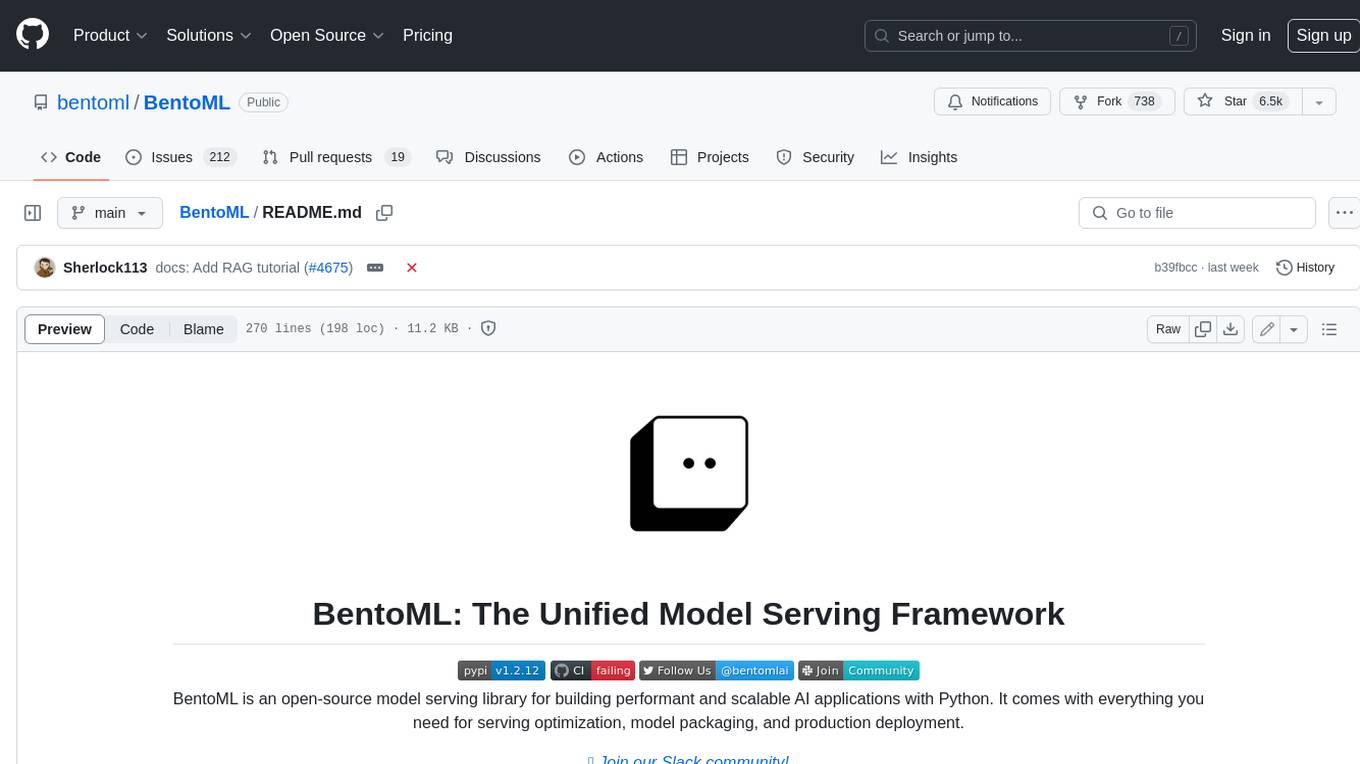
BentoML
BentoML is an open-source model serving library for building performant and scalable AI applications with Python. It comes with everything you need for serving optimization, model packaging, and production deployment.
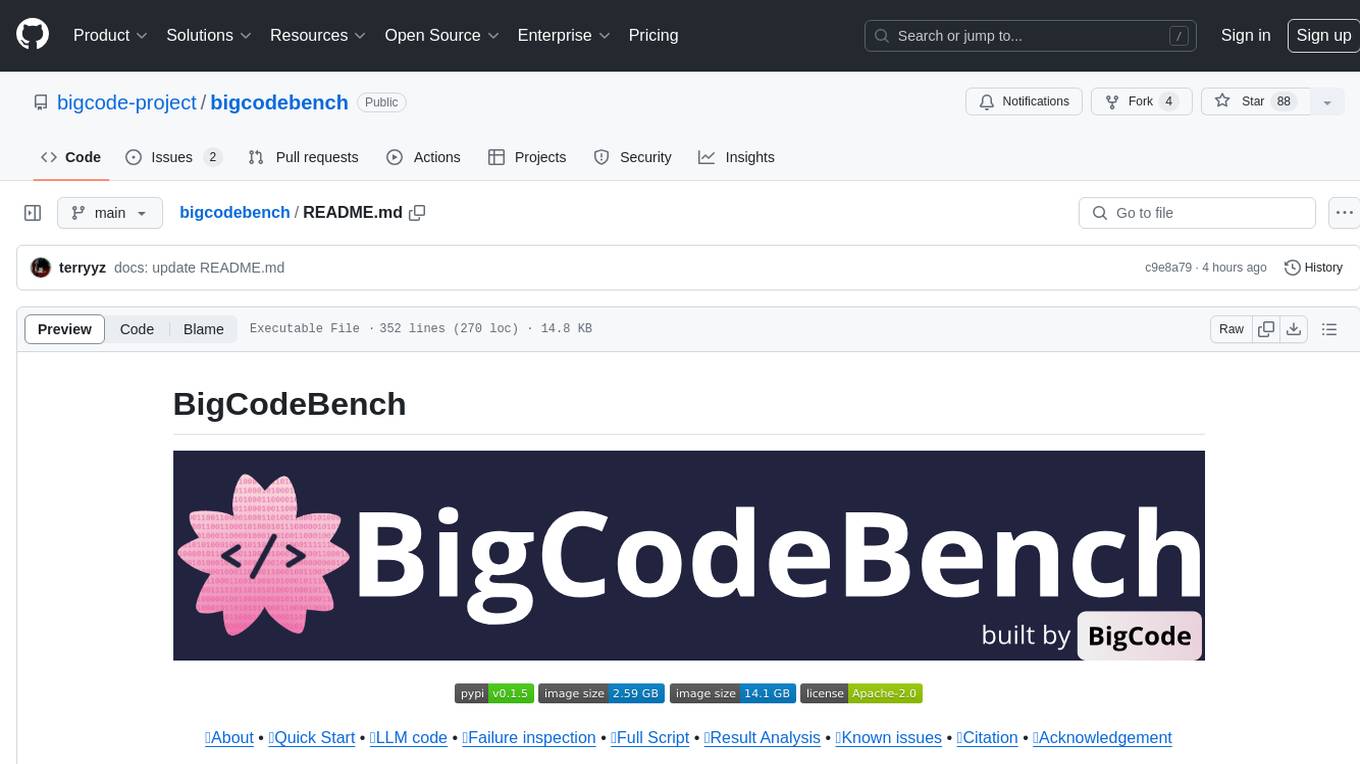
bigcodebench
BigCodeBench is an easy-to-use benchmark for code generation with practical and challenging programming tasks. It aims to evaluate the true programming capabilities of large language models (LLMs) in a more realistic setting. The benchmark is designed for HumanEval-like function-level code generation tasks, but with much more complex instructions and diverse function calls. BigCodeBench focuses on the evaluation of LLM4Code with diverse function calls and complex instructions, providing precise evaluation & ranking and pre-generated samples to accelerate code intelligence research. It inherits the design of the EvalPlus framework but differs in terms of execution environment and test evaluation.
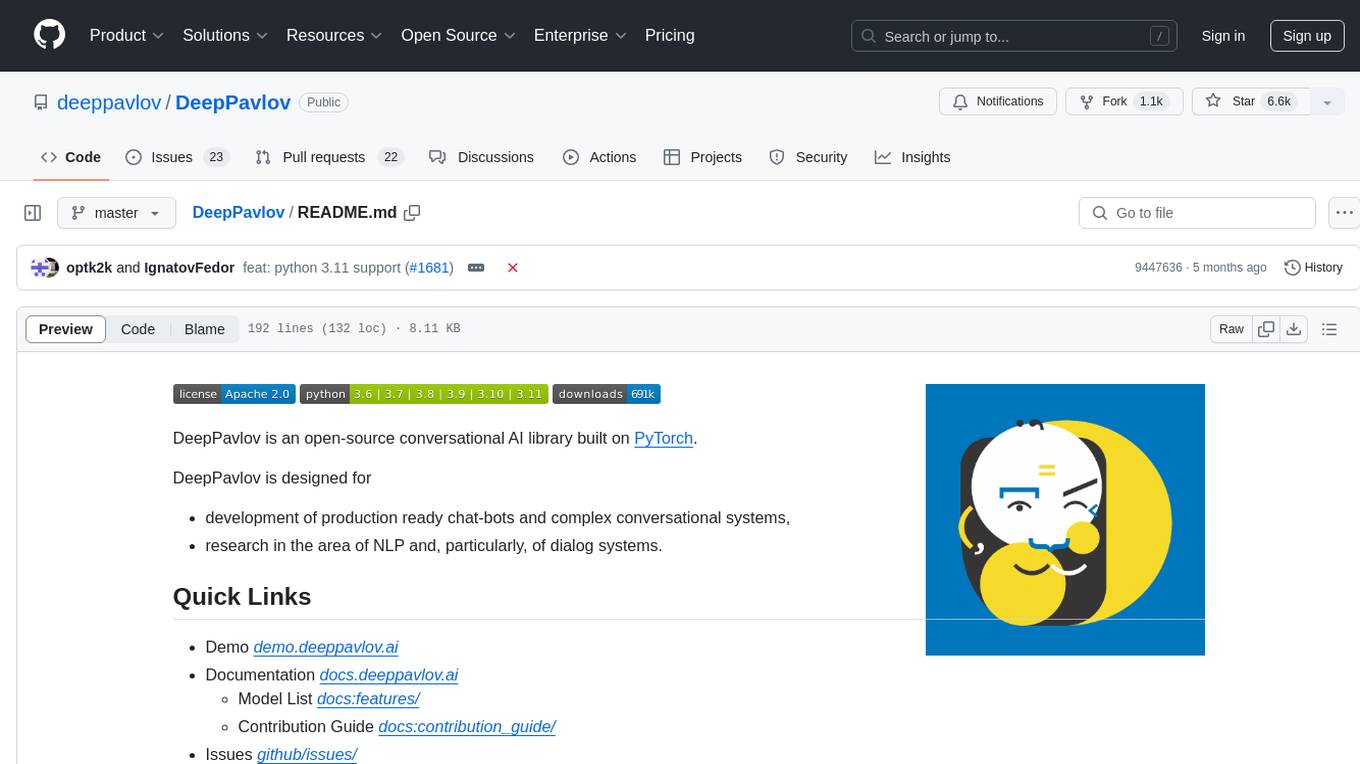
DeepPavlov
DeepPavlov is an open-source conversational AI library built on PyTorch. It is designed for the development of production-ready chatbots and complex conversational systems, as well as for research in the area of NLP and dialog systems. The library offers a wide range of models for tasks such as Named Entity Recognition, Intent/Sentence Classification, Question Answering, Sentence Similarity/Ranking, Syntactic Parsing, and more. DeepPavlov also provides embeddings like BERT, ELMo, and FastText for various languages, along with AutoML capabilities and integrations with REST API, Socket API, and Amazon AWS.
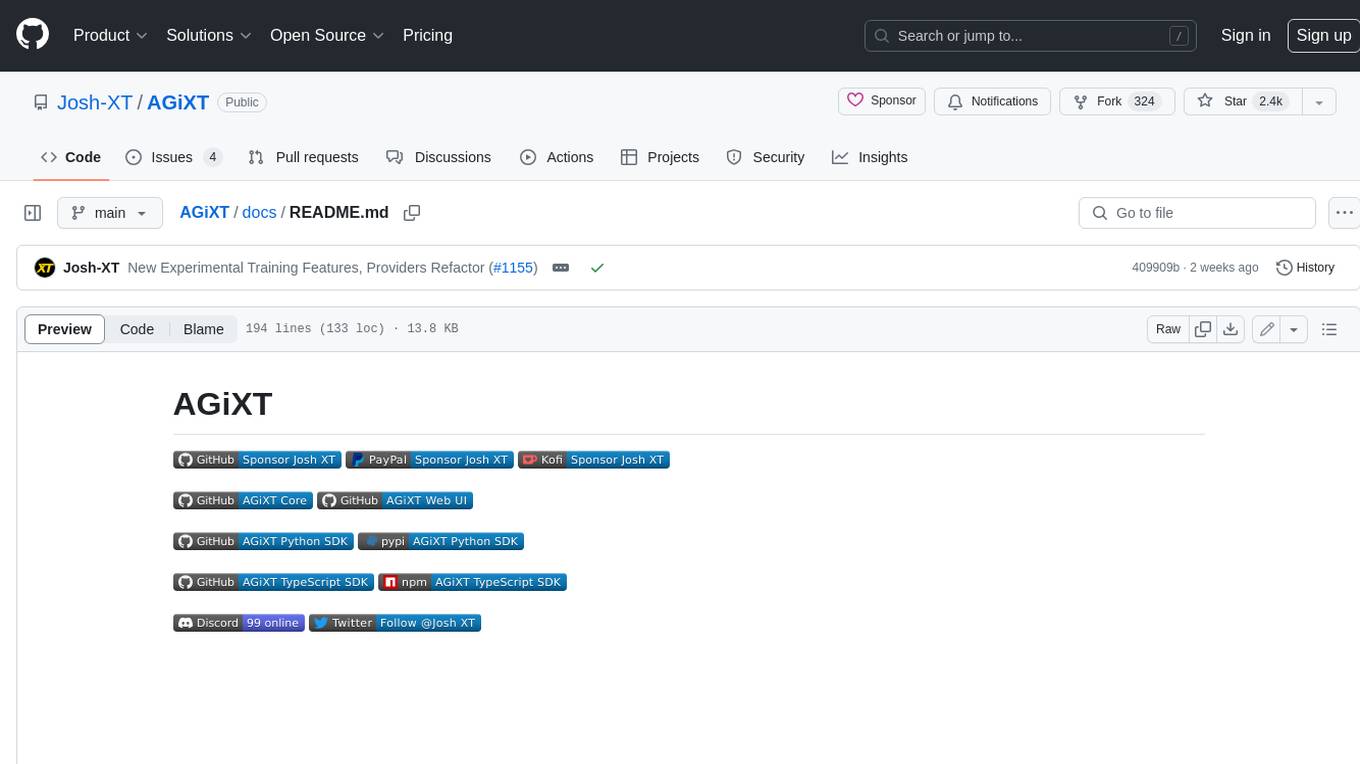
AGiXT
AGiXT is a dynamic Artificial Intelligence Automation Platform engineered to orchestrate efficient AI instruction management and task execution across a multitude of providers. Our solution infuses adaptive memory handling with a broad spectrum of commands to enhance AI's understanding and responsiveness, leading to improved task completion. The platform's smart features, like Smart Instruct and Smart Chat, seamlessly integrate web search, planning strategies, and conversation continuity, transforming the interaction between users and AI. By leveraging a powerful plugin system that includes web browsing and command execution, AGiXT stands as a versatile bridge between AI models and users. With an expanding roster of AI providers, code evaluation capabilities, comprehensive chain management, and platform interoperability, AGiXT is consistently evolving to drive a multitude of applications, affirming its place at the forefront of AI technology.
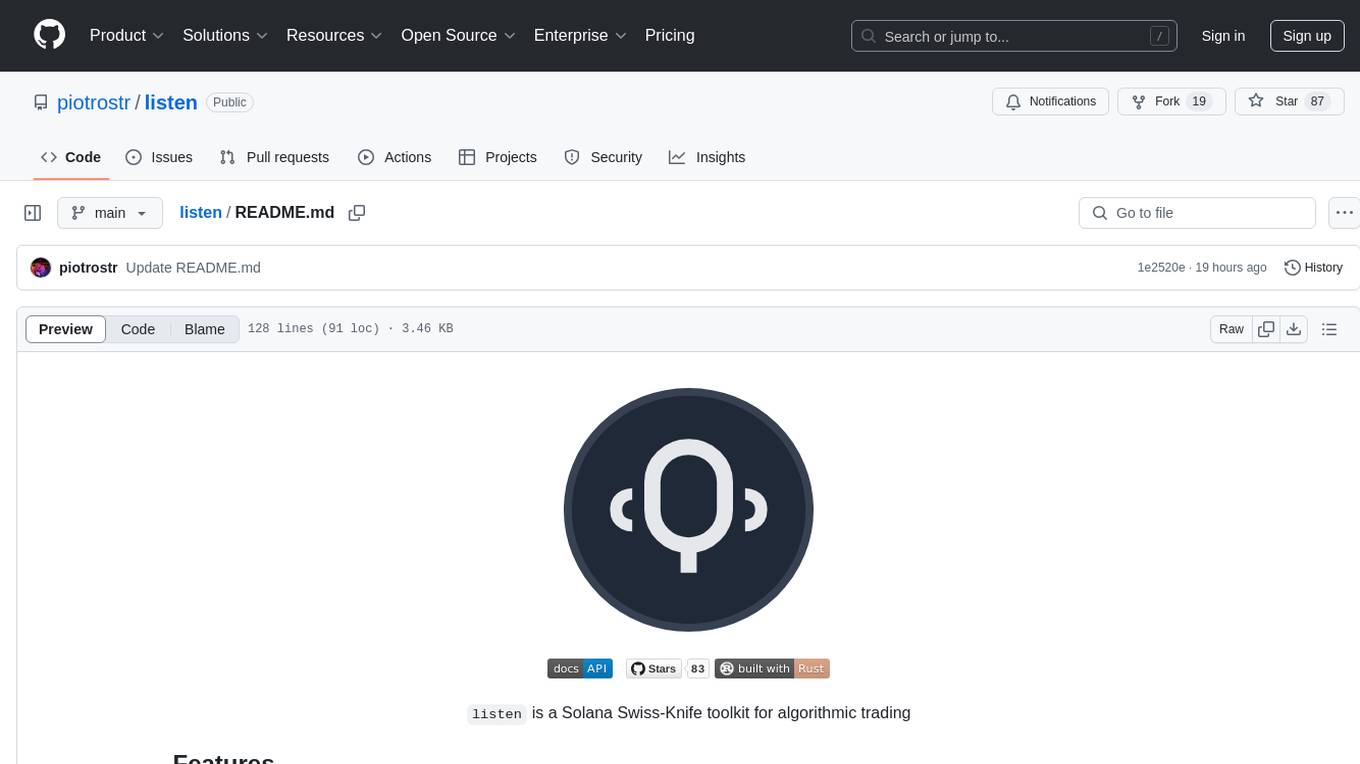
listen
Listen is a Solana Swiss-Knife toolkit for algorithmic trading, offering real-time transaction monitoring, multi-DEX swap execution, fast transactions with Jito MEV bundles, price tracking, token management utilities, and performance monitoring. It includes tools for grabbing data from unofficial APIs and works with the $arc rig framework for AI Agents to interact with the Solana blockchain. The repository provides miscellaneous tools for analysis and data retrieval, with the core functionality in the `src` directory.
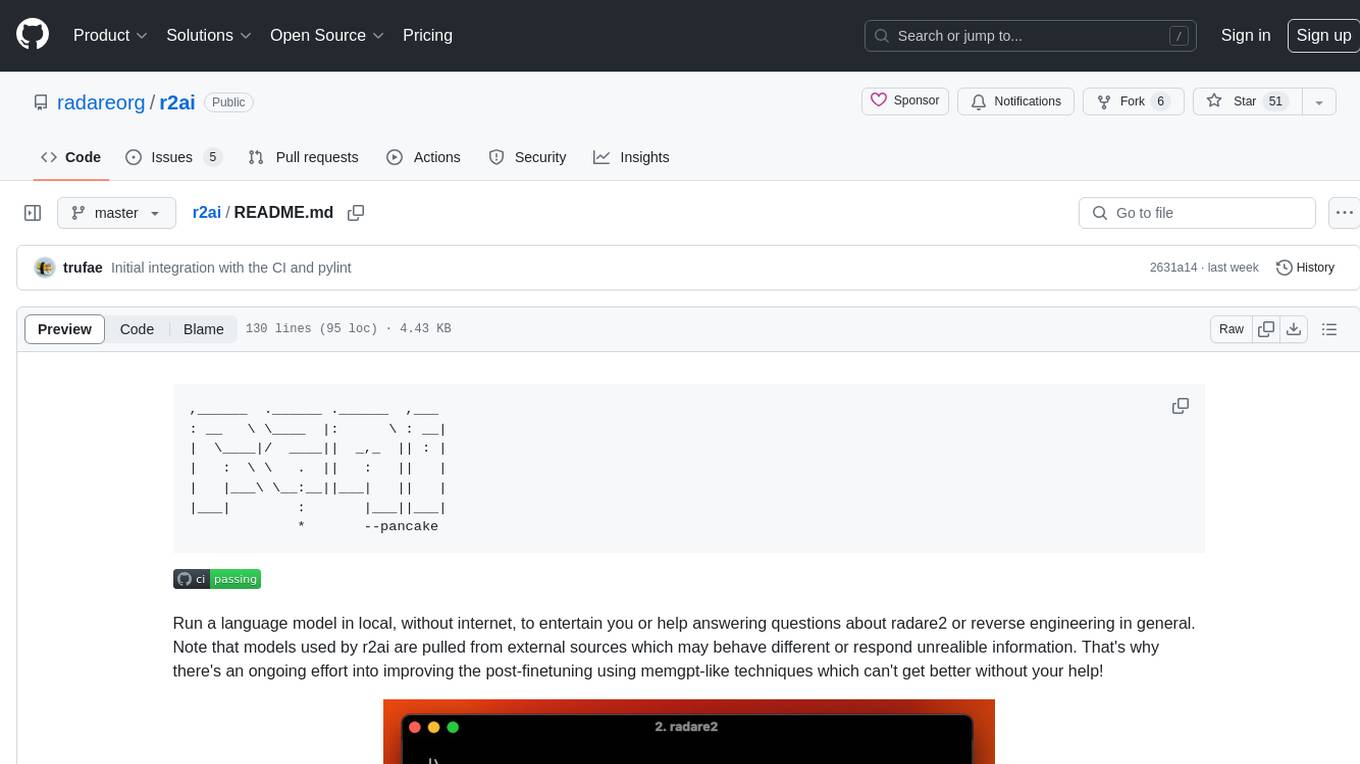
r2ai
r2ai is a tool designed to run a language model locally without internet access. It can be used to entertain users or assist in answering questions related to radare2 or reverse engineering. The tool allows users to prompt the language model, index large codebases, slurp file contents, embed the output of an r2 command, define different system-level assistant roles, set environment variables, and more. It is accessible as an r2lang-python plugin and can be scripted from various languages. Users can use different models, adjust query templates dynamically, load multiple models, and make them communicate with each other.
For similar tasks
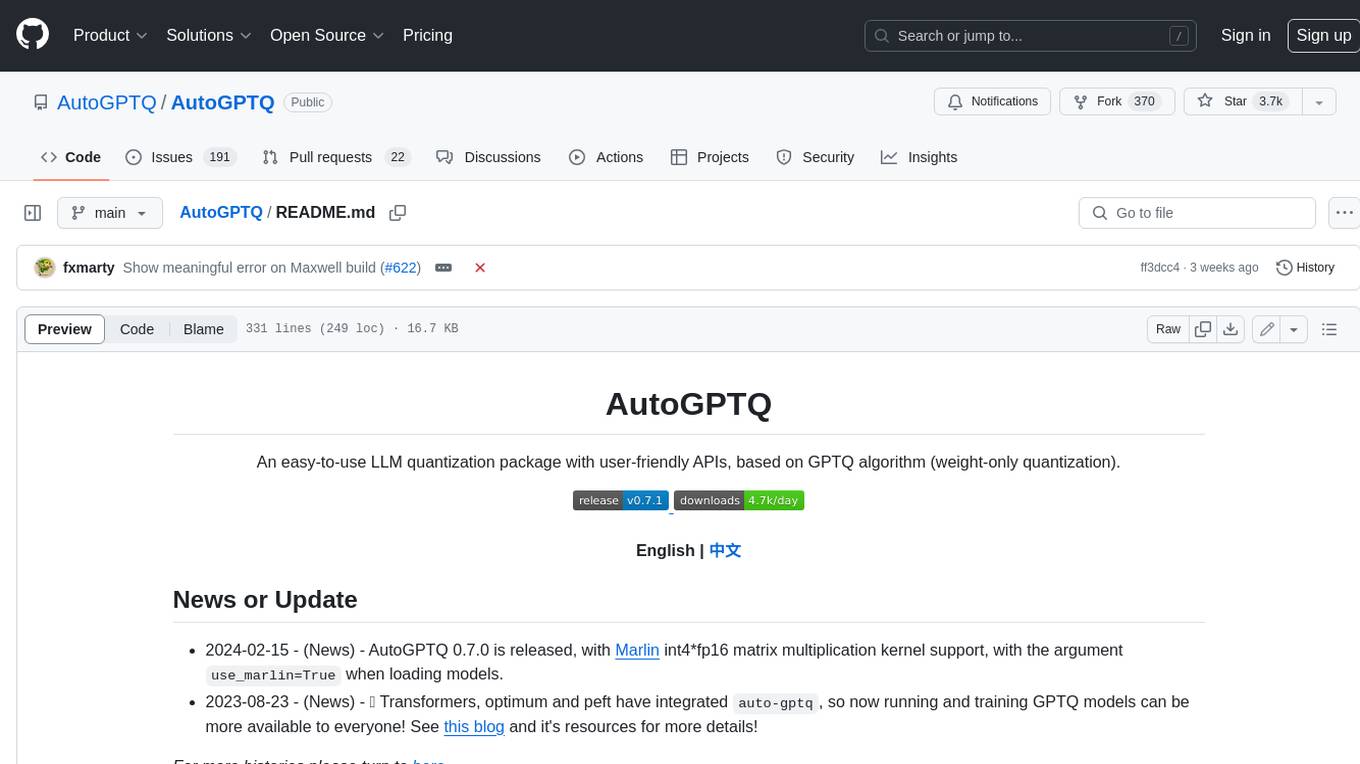
AutoGPTQ
AutoGPTQ is an easy-to-use LLM quantization package with user-friendly APIs, based on GPTQ algorithm (weight-only quantization). It provides a simple and efficient way to quantize large language models (LLMs) to reduce their size and computational cost while maintaining their performance. AutoGPTQ supports a wide range of LLM models, including GPT-2, GPT-J, OPT, and BLOOM. It also supports various evaluation tasks, such as language modeling, sequence classification, and text summarization. With AutoGPTQ, users can easily quantize their LLM models and deploy them on resource-constrained devices, such as mobile phones and embedded systems.
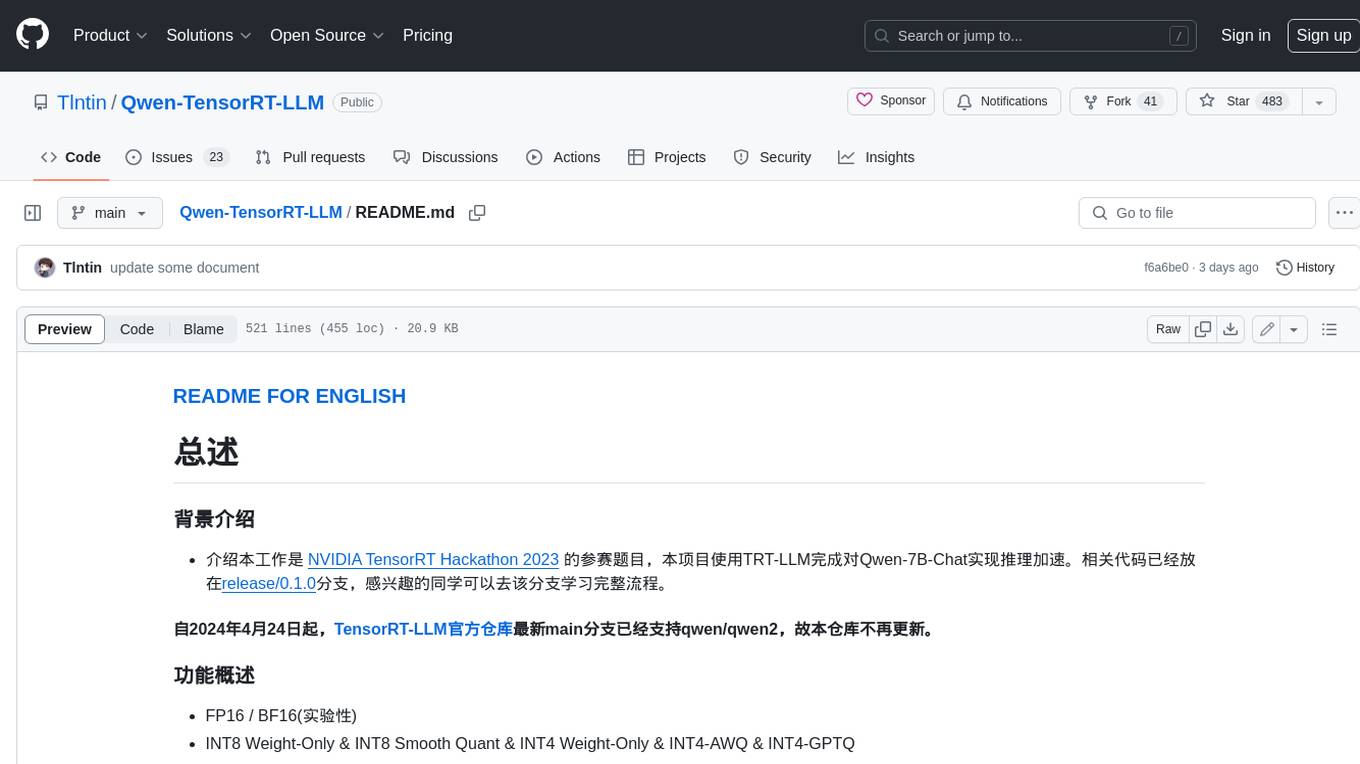
Qwen-TensorRT-LLM
Qwen-TensorRT-LLM is a project developed for the NVIDIA TensorRT Hackathon 2023, focusing on accelerating inference for the Qwen-7B-Chat model using TRT-LLM. The project offers various functionalities such as FP16/BF16 support, INT8 and INT4 quantization options, Tensor Parallel for multi-GPU parallelism, web demo setup with gradio, Triton API deployment for maximum throughput/concurrency, fastapi integration for openai requests, CLI interaction, and langchain support. It supports models like qwen2, qwen, and qwen-vl for both base and chat models. The project also provides tutorials on Bilibili and blogs for adapting Qwen models in NVIDIA TensorRT-LLM, along with hardware requirements and quick start guides for different model types and quantization methods.
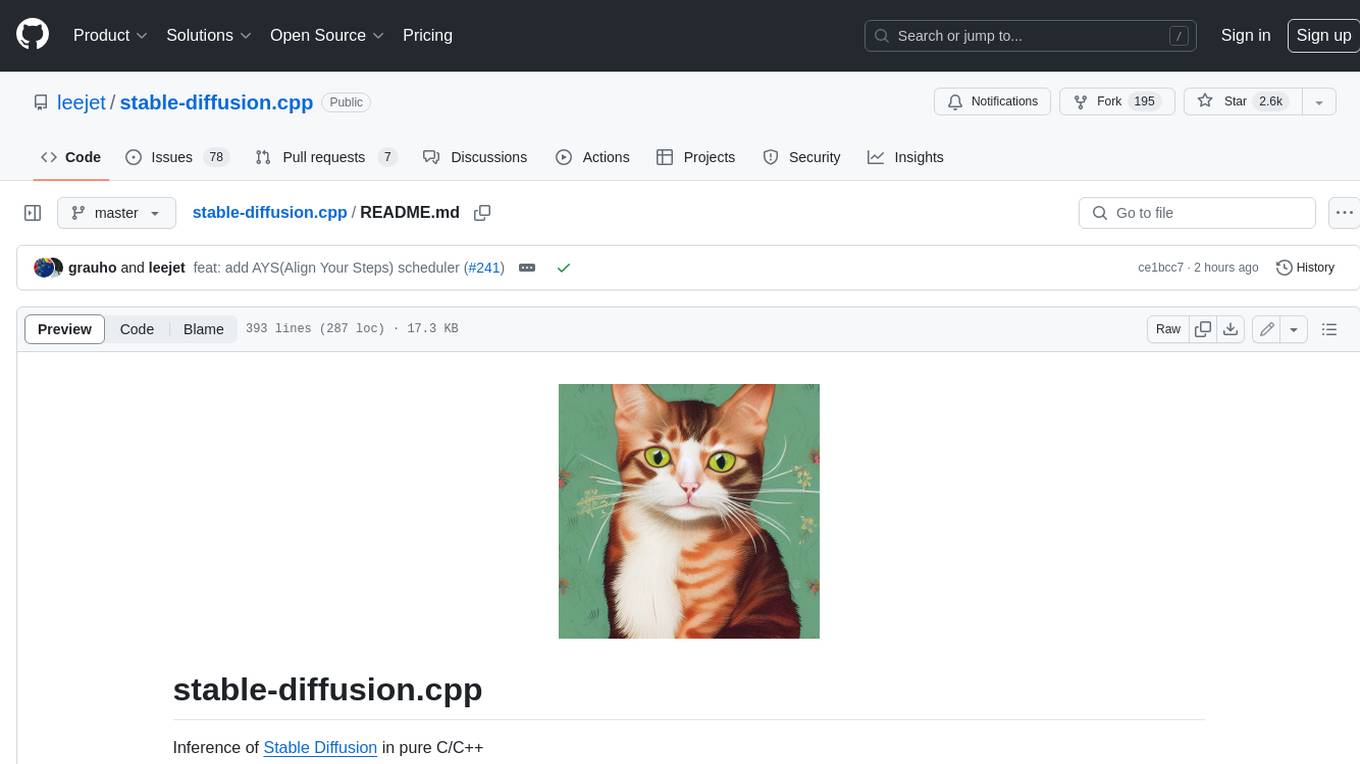
stable-diffusion.cpp
The stable-diffusion.cpp repository provides an implementation for inferring stable diffusion in pure C/C++. It offers features such as support for different versions of stable diffusion, lightweight and dependency-free implementation, various quantization support, memory-efficient CPU inference, GPU acceleration, and more. Users can download the built executable program or build it manually. The repository also includes instructions for downloading weights, building from scratch, using different acceleration methods, running the tool, converting weights, and utilizing various features like Flash Attention, ESRGAN upscaling, PhotoMaker support, and more. Additionally, it mentions future TODOs and provides information on memory requirements, bindings, UIs, contributors, and references.
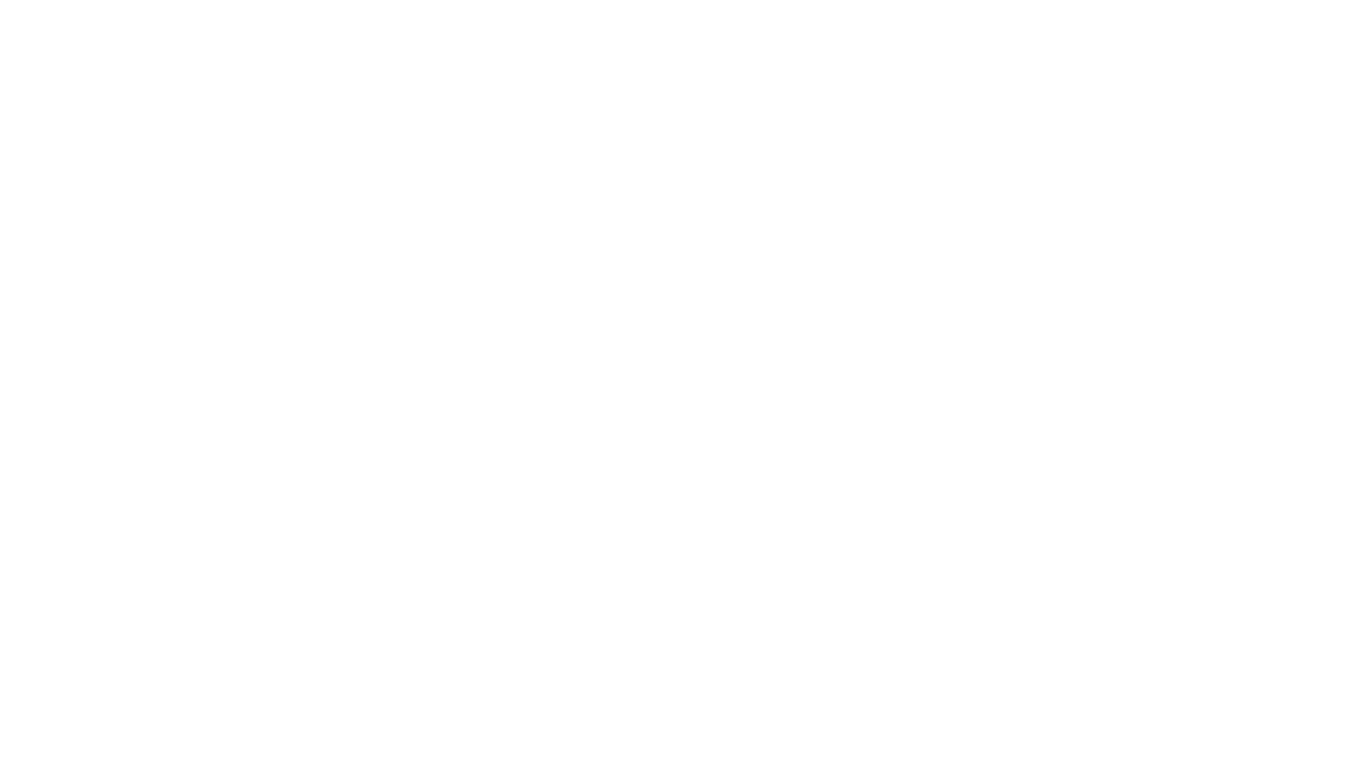
LMOps
LMOps is a research initiative focusing on fundamental research and technology for building AI products with foundation models, particularly enabling AI capabilities with Large Language Models (LLMs) and Generative AI models. The project explores various aspects such as prompt optimization, longer context handling, LLM alignment, acceleration of LLMs, LLM customization, and understanding in-context learning. It also includes tools like Promptist for automatic prompt optimization, Structured Prompting for efficient long-sequence prompts consumption, and X-Prompt for extensible prompts beyond natural language. Additionally, LLMA accelerators are developed to speed up LLM inference by referencing and copying text spans from documents. The project aims to advance technologies that facilitate prompting language models and enhance the performance of LLMs in various scenarios.
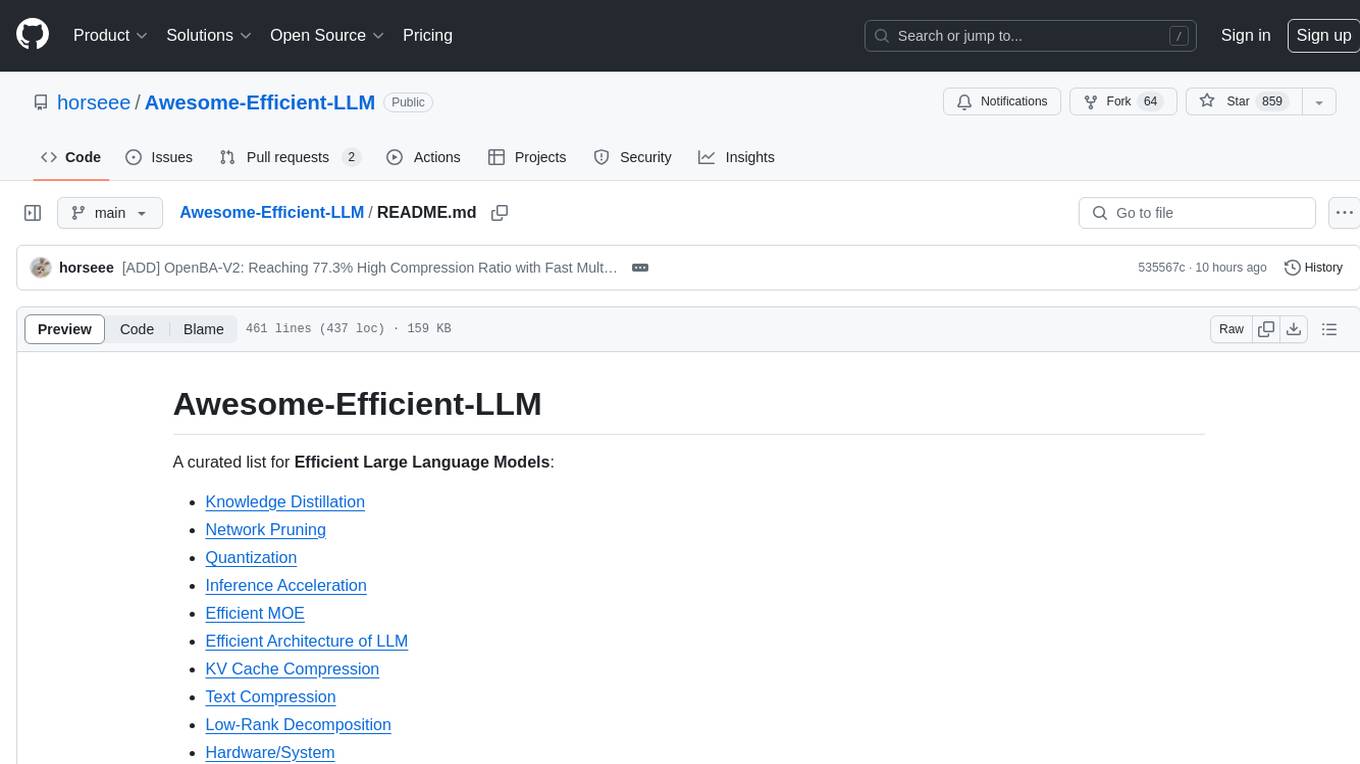
Awesome-Efficient-LLM
Awesome-Efficient-LLM is a curated list focusing on efficient large language models. It includes topics such as knowledge distillation, network pruning, quantization, inference acceleration, efficient MOE, efficient architecture of LLM, KV cache compression, text compression, low-rank decomposition, hardware/system, tuning, and survey. The repository provides a collection of papers and projects related to improving the efficiency of large language models through various techniques like sparsity, quantization, and compression.
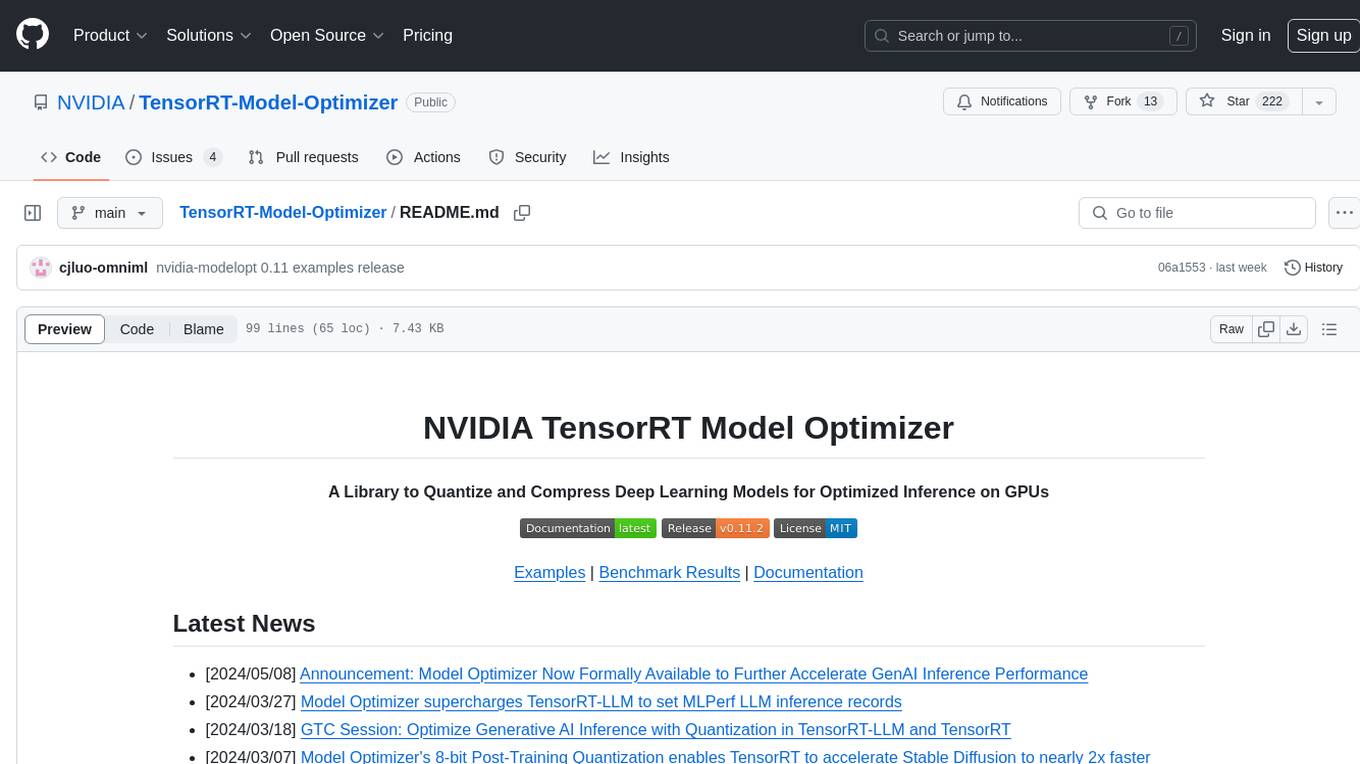
TensorRT-Model-Optimizer
The NVIDIA TensorRT Model Optimizer is a library designed to quantize and compress deep learning models for optimized inference on GPUs. It offers state-of-the-art model optimization techniques including quantization and sparsity to reduce inference costs for generative AI models. Users can easily stack different optimization techniques to produce quantized checkpoints from torch or ONNX models. The quantized checkpoints are ready for deployment in inference frameworks like TensorRT-LLM or TensorRT, with planned integrations for NVIDIA NeMo and Megatron-LM. The tool also supports 8-bit quantization with Stable Diffusion for enterprise users on NVIDIA NIM. Model Optimizer is available for free on NVIDIA PyPI, and this repository serves as a platform for sharing examples, GPU-optimized recipes, and collecting community feedback.
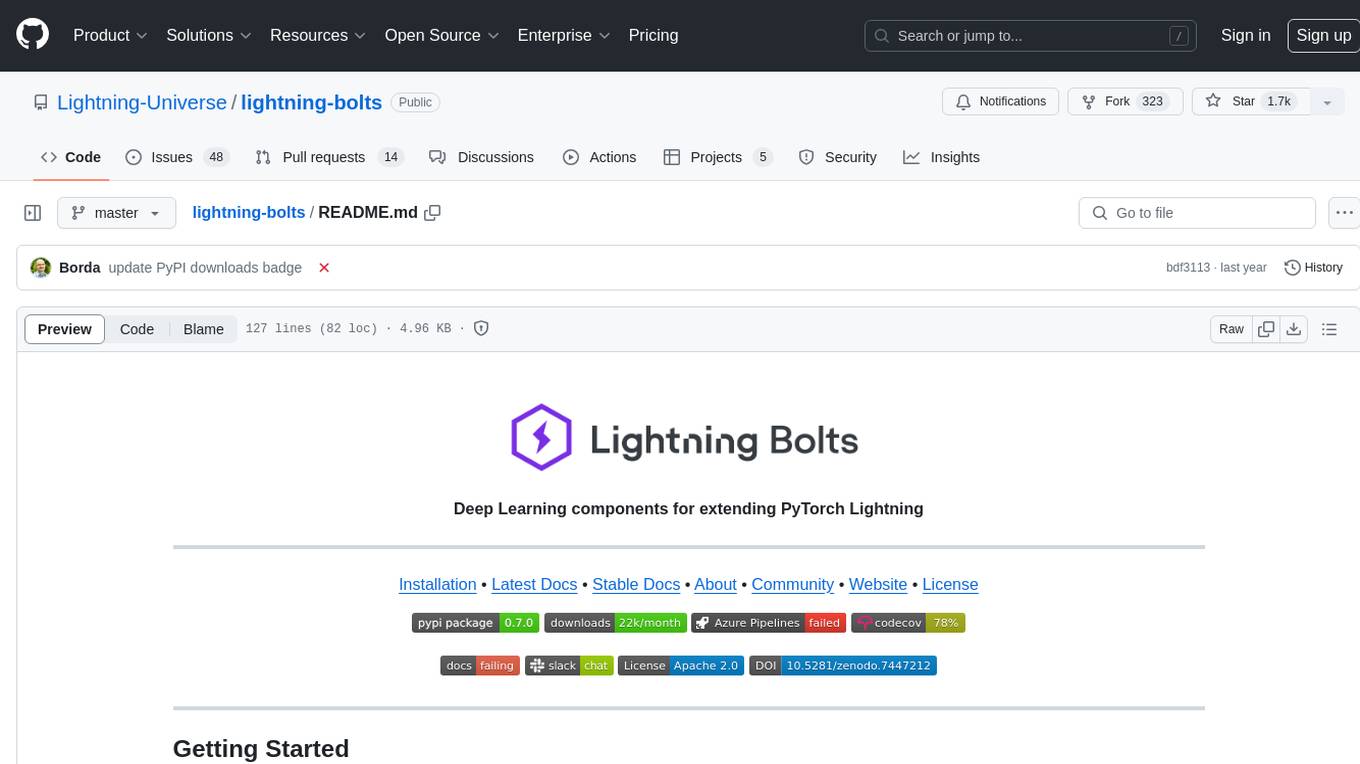
lightning-bolts
Bolts package provides a variety of components to extend PyTorch Lightning, such as callbacks & datasets, for applied research and production. Users can accelerate Lightning training with the Torch ORT Callback to optimize ONNX graph for faster training & inference. Additionally, users can introduce sparsity with the SparseMLCallback to accelerate inference by leveraging the DeepSparse engine. Specific research implementations are encouraged, with contributions that help train SSL models and integrate with Lightning Flash for state-of-the-art models in applied research.
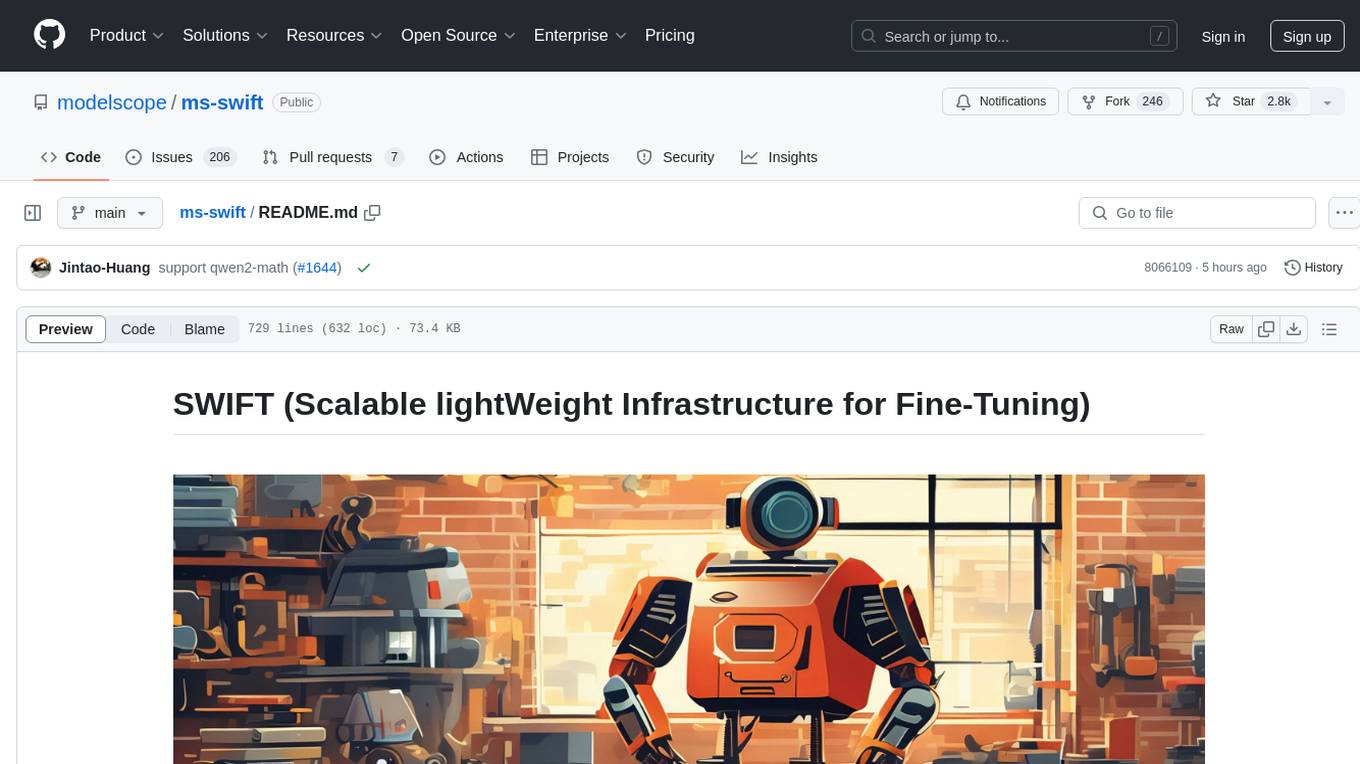
ms-swift
ms-swift is an official framework provided by the ModelScope community for fine-tuning and deploying large language models and multi-modal large models. It supports training, inference, evaluation, quantization, and deployment of over 400 large models and 100+ multi-modal large models. The framework includes various training technologies and accelerates inference, evaluation, and deployment modules. It offers a Gradio-based Web-UI interface and best practices for easy application of large models. ms-swift supports a wide range of model types, dataset types, hardware support, lightweight training methods, distributed training techniques, quantization training, RLHF training, multi-modal training, interface training, plugin and extension support, inference acceleration engines, model evaluation, and model quantization.
For similar jobs
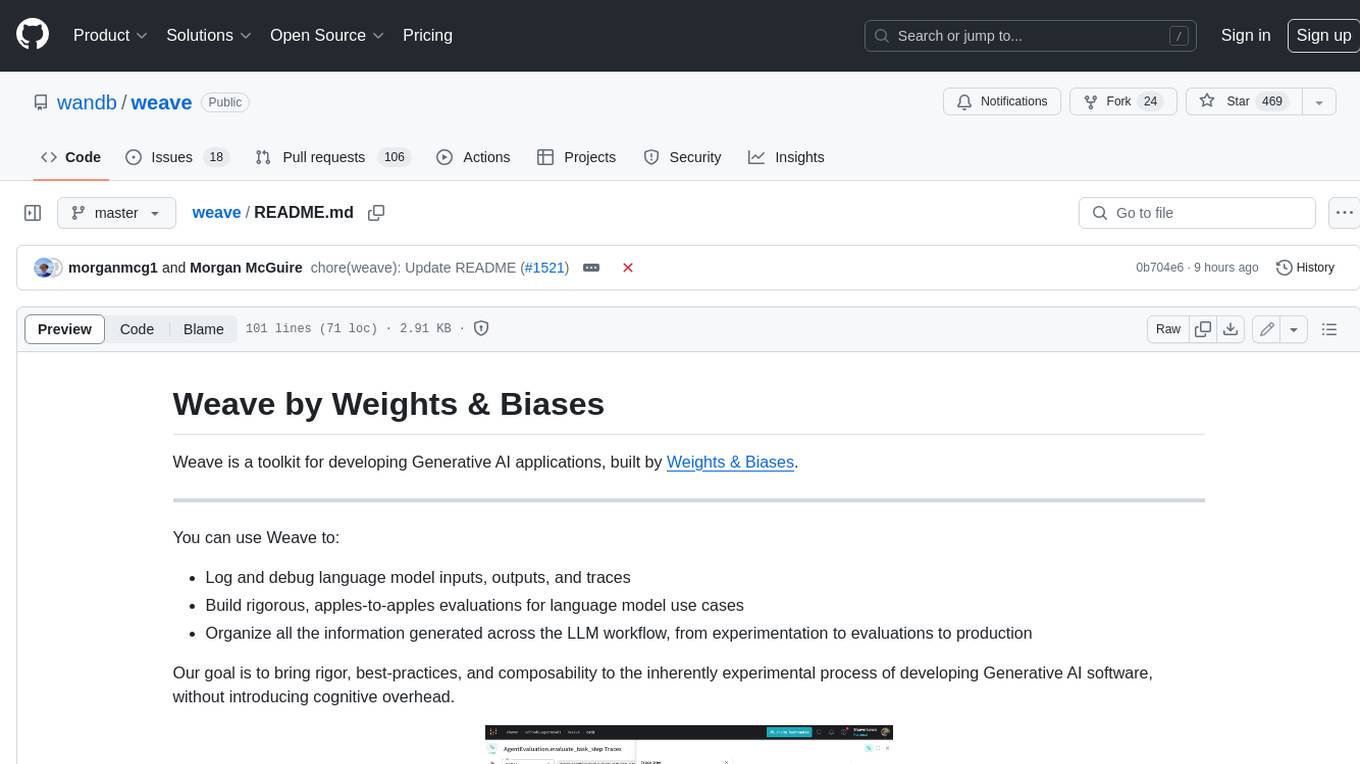
weave
Weave is a toolkit for developing Generative AI applications, built by Weights & Biases. With Weave, you can log and debug language model inputs, outputs, and traces; build rigorous, apples-to-apples evaluations for language model use cases; and organize all the information generated across the LLM workflow, from experimentation to evaluations to production. Weave aims to bring rigor, best-practices, and composability to the inherently experimental process of developing Generative AI software, without introducing cognitive overhead.
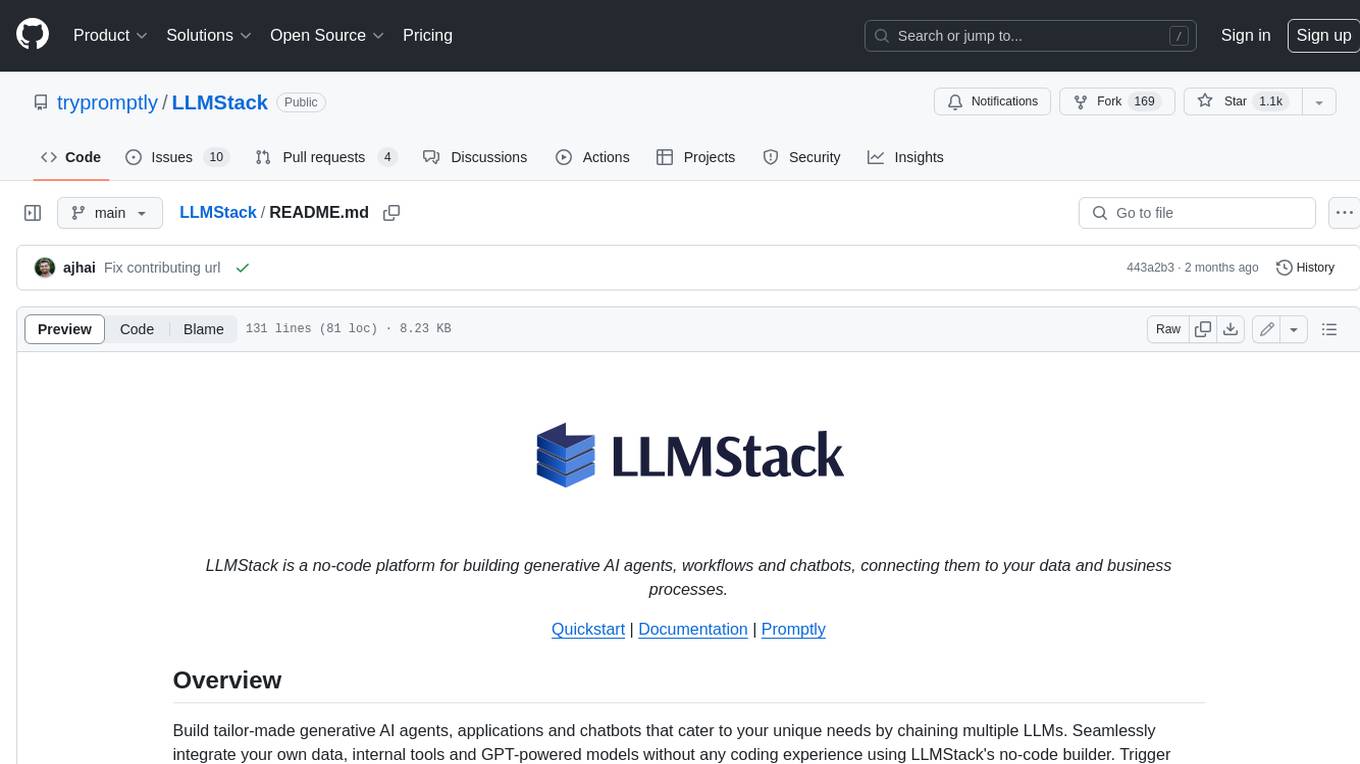
LLMStack
LLMStack is a no-code platform for building generative AI agents, workflows, and chatbots. It allows users to connect their own data, internal tools, and GPT-powered models without any coding experience. LLMStack can be deployed to the cloud or on-premise and can be accessed via HTTP API or triggered from Slack or Discord.
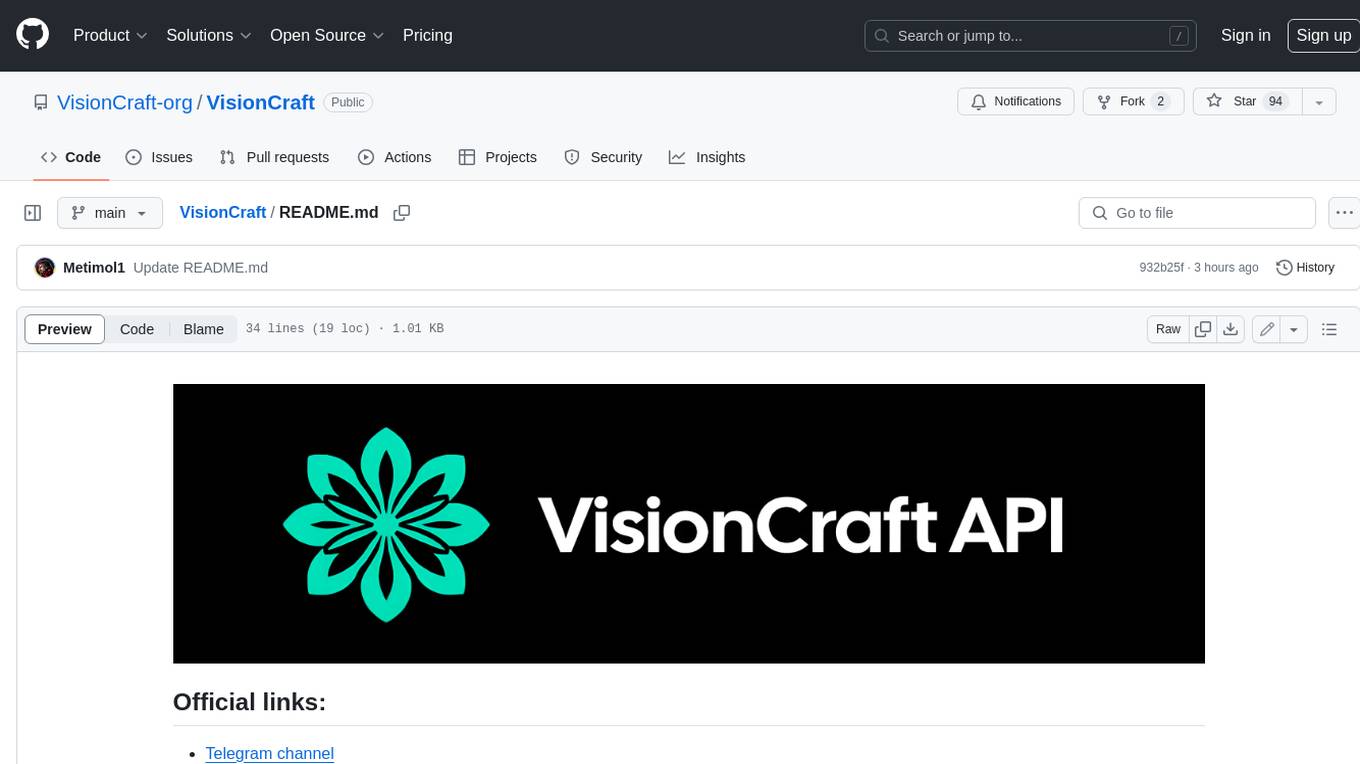
VisionCraft
The VisionCraft API is a free API for using over 100 different AI models. From images to sound.
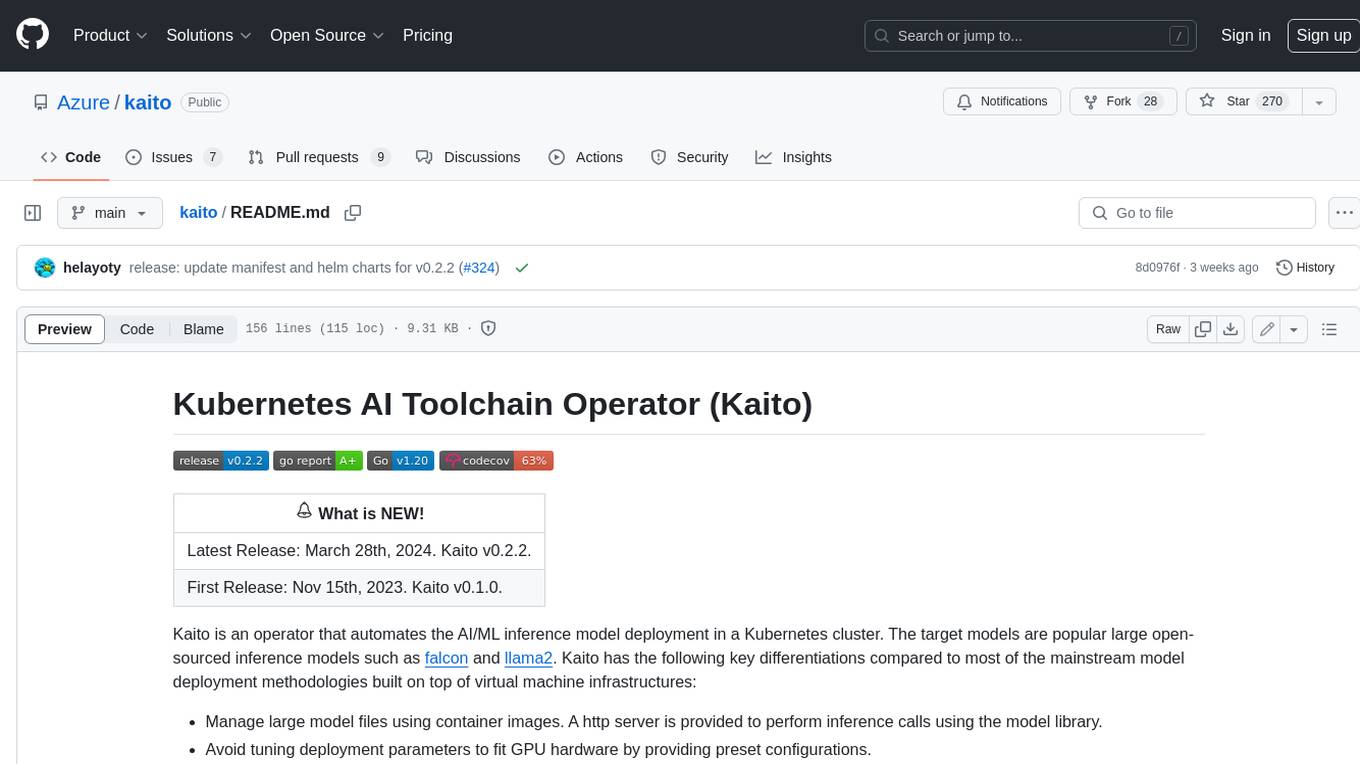
kaito
Kaito is an operator that automates the AI/ML inference model deployment in a Kubernetes cluster. It manages large model files using container images, avoids tuning deployment parameters to fit GPU hardware by providing preset configurations, auto-provisions GPU nodes based on model requirements, and hosts large model images in the public Microsoft Container Registry (MCR) if the license allows. Using Kaito, the workflow of onboarding large AI inference models in Kubernetes is largely simplified.
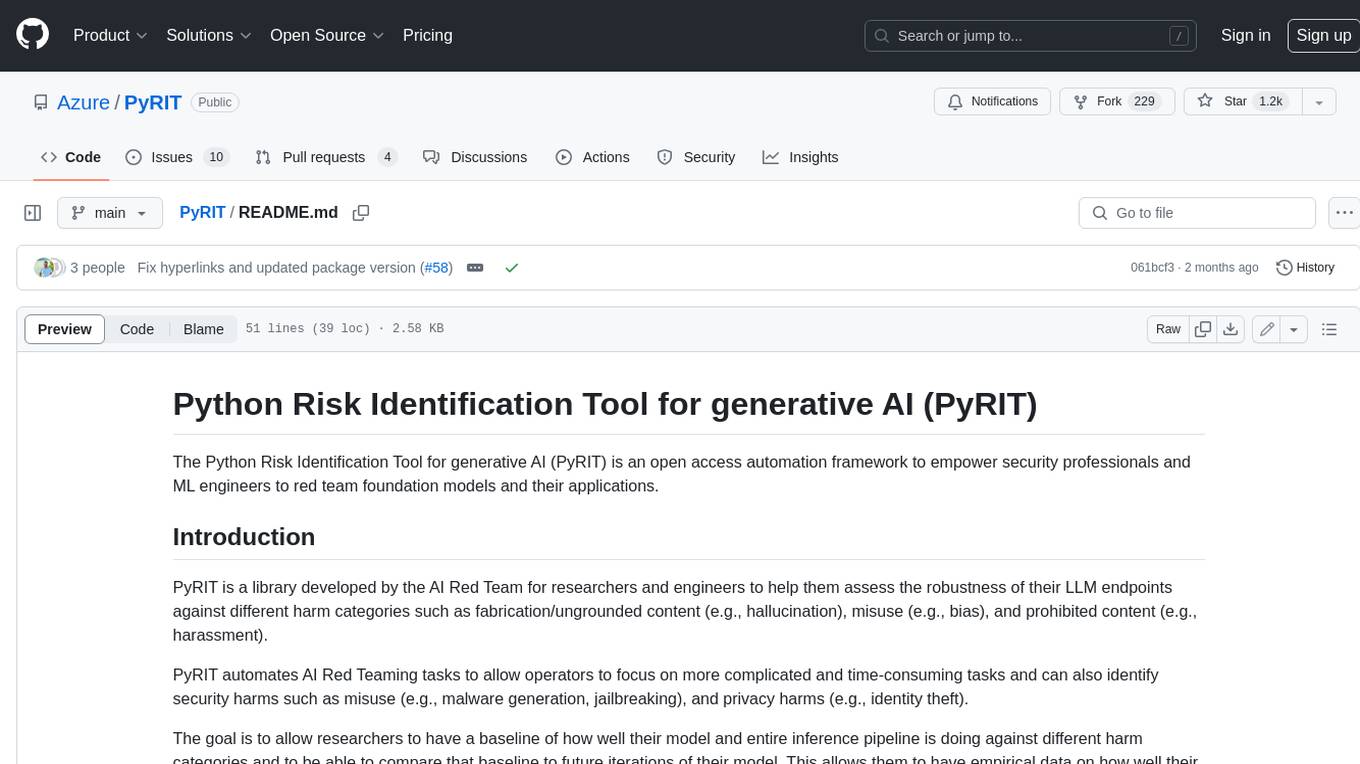
PyRIT
PyRIT is an open access automation framework designed to empower security professionals and ML engineers to red team foundation models and their applications. It automates AI Red Teaming tasks to allow operators to focus on more complicated and time-consuming tasks and can also identify security harms such as misuse (e.g., malware generation, jailbreaking), and privacy harms (e.g., identity theft). The goal is to allow researchers to have a baseline of how well their model and entire inference pipeline is doing against different harm categories and to be able to compare that baseline to future iterations of their model. This allows them to have empirical data on how well their model is doing today, and detect any degradation of performance based on future improvements.
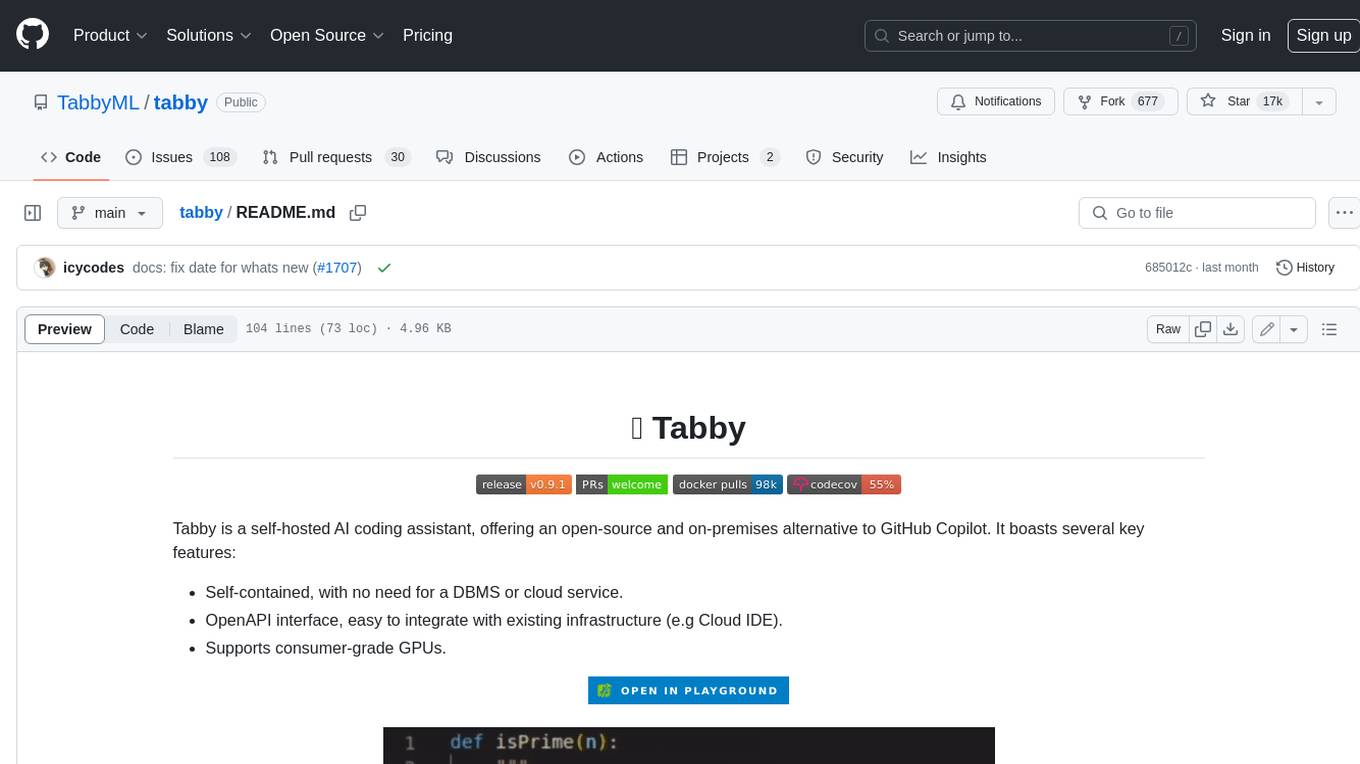
tabby
Tabby is a self-hosted AI coding assistant, offering an open-source and on-premises alternative to GitHub Copilot. It boasts several key features: * Self-contained, with no need for a DBMS or cloud service. * OpenAPI interface, easy to integrate with existing infrastructure (e.g Cloud IDE). * Supports consumer-grade GPUs.
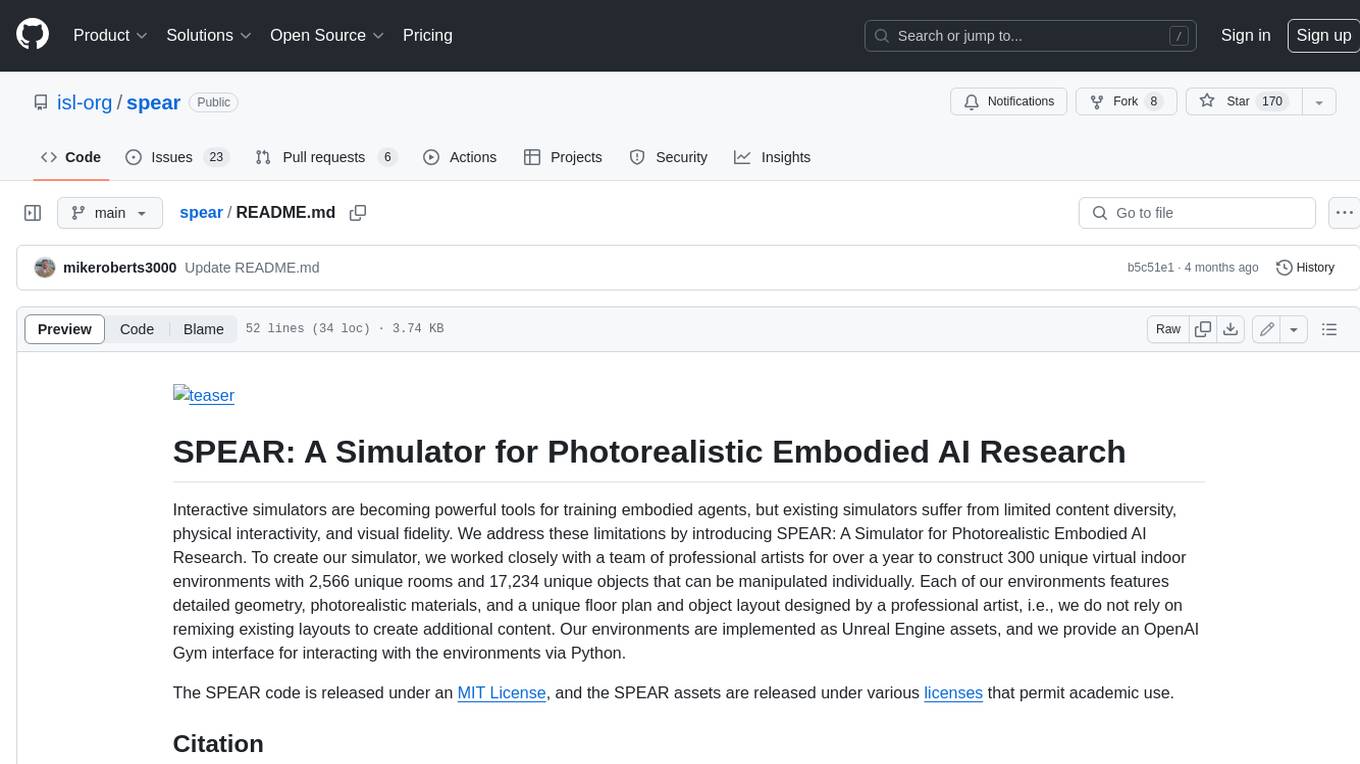
spear
SPEAR (Simulator for Photorealistic Embodied AI Research) is a powerful tool for training embodied agents. It features 300 unique virtual indoor environments with 2,566 unique rooms and 17,234 unique objects that can be manipulated individually. Each environment is designed by a professional artist and features detailed geometry, photorealistic materials, and a unique floor plan and object layout. SPEAR is implemented as Unreal Engine assets and provides an OpenAI Gym interface for interacting with the environments via Python.
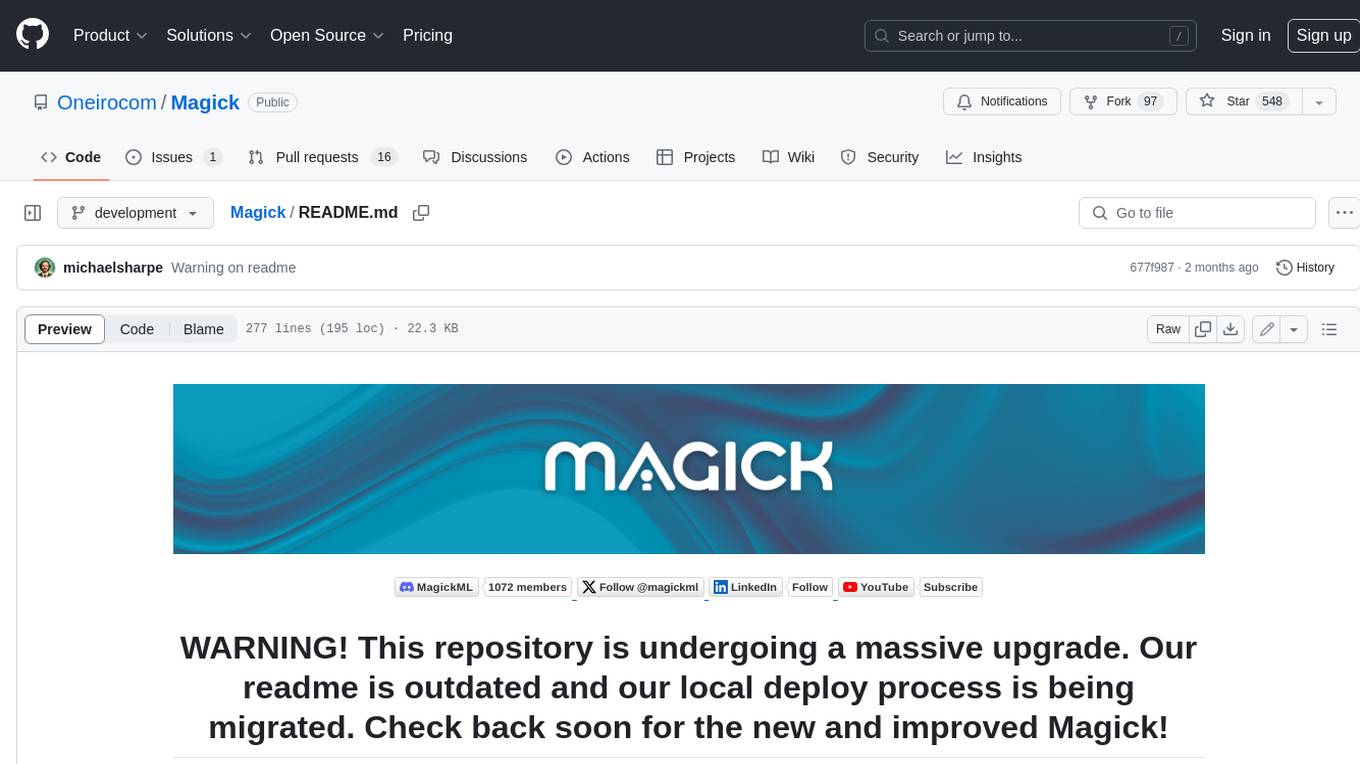
Magick
Magick is a groundbreaking visual AIDE (Artificial Intelligence Development Environment) for no-code data pipelines and multimodal agents. Magick can connect to other services and comes with nodes and templates well-suited for intelligent agents, chatbots, complex reasoning systems and realistic characters.