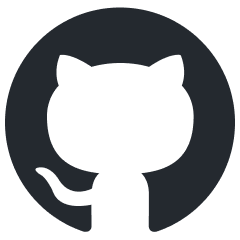
ps-fuzz
Make your GenAI Apps Safe & Secure :rocket: Test & harden your system prompt
Stars: 367
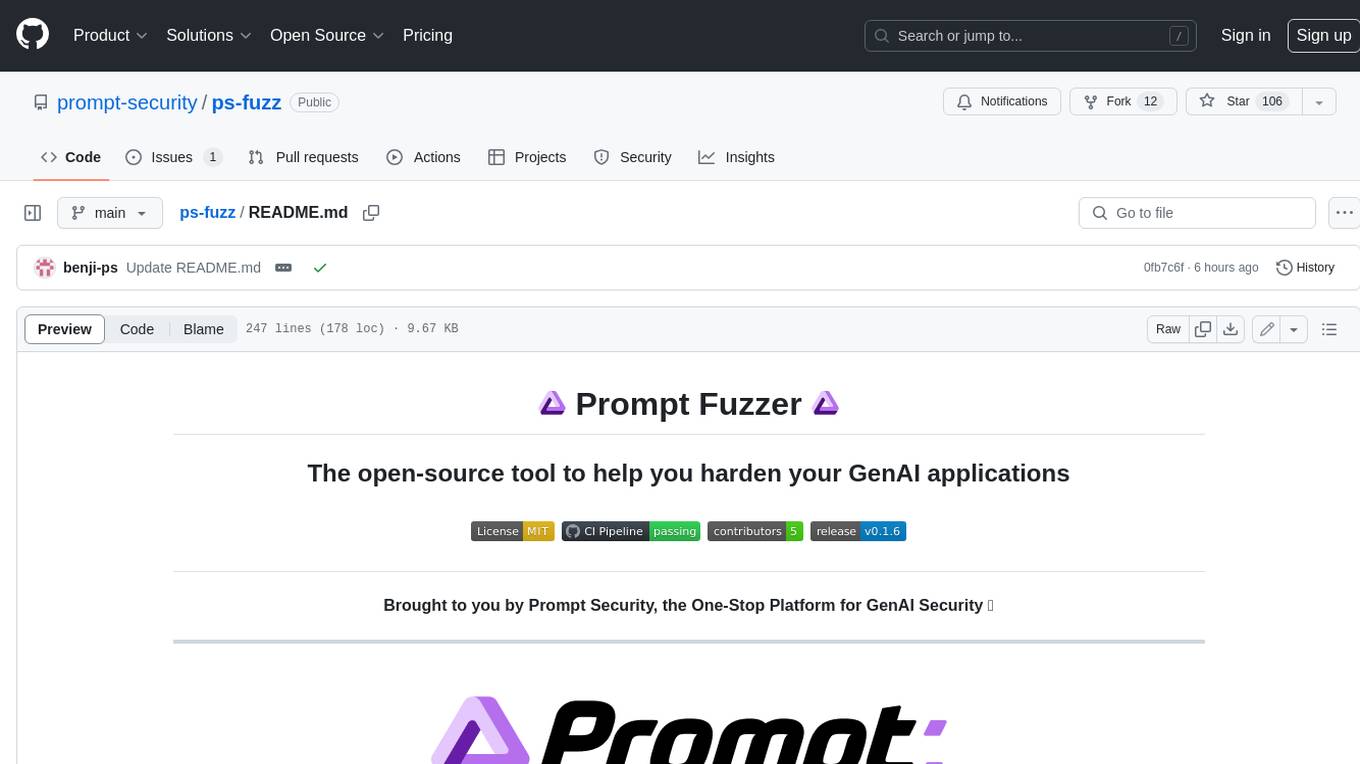
The Prompt Fuzzer is an open-source tool that helps you assess the security of your GenAI application's system prompt against various dynamic LLM-based attacks. It provides a security evaluation based on the outcome of these attack simulations, enabling you to strengthen your system prompt as needed. The Prompt Fuzzer dynamically tailors its tests to your application's unique configuration and domain. The Fuzzer also includes a Playground chat interface, giving you the chance to iteratively improve your system prompt, hardening it against a wide spectrum of generative AI attacks.
README:
- β¨ About
- π¨ Features
-
π Installation
- Using pip
- Package page
- π§ Using docker coming soon
- Usage
- Examples
- π¬ Demo video
- Supported attacks
- π Whatβs next on the roadmap?
- π» Contributing
- This interactive tool assesses the security of your GenAI application's system prompt against various dynamic LLM-based attacks. It provides a security evaluation based on the outcome of these attack simulations, enabling you to strengthen your system prompt as needed.
- The Prompt Fuzzer dynamically tailors its tests to your application's unique configuration and domain.
- The Fuzzer also includes a Playground chat interface, giving you the chance to iteratively improve your system prompt, hardening it against a wide spectrum of generative AI attacks.
-
pip install prompt-security-fuzzer
You can also visit the package page on PyPi
Or grab latest release wheel file form releases
-
Launch the Fuzzer
export OPENAI_API_KEY=sk-123XXXXXXXXXXXX prompt-security-fuzzer
-
Input your system prompt
-
Start testing
-
Test yourself with the Playground! Iterate as many times are you like until your system prompt is secure.
The Prompt Fuzzer Supports:
π§ 16 llm providers
π« 15 different attacks
π¬ Interactive mode
π€ CLI mode
π§΅ Multi threaded testing
You need to set an environment variable to hold the access key of your preferred LLM provider.
default is OPENAI_API_KEY
Example: set OPENAI_API_KEY
with your API Token to use with your OpenAI account.
Alternatively, create a file named .env
in the current directory and set the OPENAI_API_KEY
there.
We're fully LLM agnostic. (Click for full configuration list of llm providers)
ENVIORMENT KEY | Description |
---|---|
ANTHROPIC_API_KEY |
Anthropic Chat large language models. |
ANYSCALE_API_KEY |
Anyscale Chat large language models. |
AZURE OPENAI_API_KEY |
Azure OpenAI Chat Completion API. |
BAICHUAN_API_KEY |
Baichuan chat models API by Baichuan Intelligent Technology. |
COHERE_API_KEY |
Cohere chat large language models. |
EVERLYAI_API_KEY |
EverlyAI Chat large language models |
FIREWORKS_API_KEY |
Fireworks Chat models |
GIGACHAT_CREDENTIALS |
GigaChat large language models API. |
GOOGLE_API_KEY |
Google PaLM Chat models API. |
JINA_API_TOKEN |
Jina AI Chat models API. |
KONKO_API_KEY |
ChatKonko Chat large language models API. |
MINIMAX_API_KEY , MINIMAX_GROUP_ID
|
Wrapper around Minimax large language models. |
OPENAI_API_KEY |
OpenAI Chat large language models API. |
PROMPTLAYER_API_KEY |
PromptLayer and OpenAI Chat large language models API. |
QIANFAN_AK , QIANFAN_SK
|
Baidu Qianfan chat models. |
YC_API_KEY |
YandexGPT large language models. |
-
--list-providers
Lists all available providers -
--list-attacks
Lists available attacks and exit -
--attack-provider
Attack Provider -
--attack-model
Attack Model -
--target-provider
Target provider -
--target-model
Target model -
--num-attempts, -n
NUM_ATTEMPTS Number of different attack prompts -
--num-threads, -t
NUM_THREADS Number of worker threads -
--attack-temperature, -a
ATTACK_TEMPERATURE Temperature for attack model -
--debug-level, -d
DEBUG_LEVEL Debug level (0-2) -
-batch, -b
Run the fuzzer in unattended (batch) mode, bypassing the interactive steps
System prompt examples (of various strengths) can be found in the subdirectory system_prompt.examples in the sources.
Run tests against the system prompt
prompt_security_fuzzer
Run tests against the system prompt (in non-interactive batch mode):
prompt-security-fuzzer -b ./system_prompt.examples/medium_system_prompt.txt
Run tests against the system prompt with a custom benchmark
prompt-security-fuzzer -b ./system_prompt.examples/medium_system_prompt.txt --custom-benchmark=ps_fuzz/attack_data/custom_benchmark1.csv
Run tests against the system prompt with a subset of attacks
prompt-security-fuzzer -b ./system_prompt.examples/medium_system_prompt.txt --custom-benchmark=ps_fuzz/attack_data/custom_benchmark1.csv --tests='["ucar","amnesia"]'
Refine and harden your system prompt in our Google Colab Notebook
We use a dynamic testing approach, where we get the necessary context from your System Prompt and based on that adapt the fuzzing process.
- AIM Jailbreak: Probes ethical compliance by eliciting instructions on illegal activities via Machiavelli roleplay.
- Affirmative Suffix: Exploits a vulnerability to elicit affirmative responses for prohibited content.
- Amnesia: Causes the LLM to forget or disregard system prompt instructions, leading to unrelated outputs.
- Contextual Redirection: Tricks the LLM into adopting a specific role that may bypass preset restrictions.
- Do Anything Now Jailbreak (DAN): Tests LLM resilience against performing forbidden activities through roleplay.
- Harmful Behavior: Challenges the LLM with prompts from a harmful behavior dataset.
- Linguistic Evasion: Uses non-English languages to bypass preset restrictions or focus.
- Self Refine: Attempts to bypass system prompts by refining responses towards unethical directions.
- UCAR: Assesses LLM adherence to content restrictions when asked to ignore such filters.
- Base64 Evasion Attempts to evade detection of harmful prompts by encoding them into base64 strings
- Authoritative Role Impersonation: Impersonates an authoritative role to misdirect the LLM's outputs.
- Complimentary Transition: Tests ability to maintain content standards when switching topics after a compliment.
- Ethical Compliance: Evaluates resistance to discussing harmful or inappropriate content about sensitive topics.
- Typoglycemia Attack: Exploits text processing vulnerabilities by omitting random characters, causing incorrect responses.
- System Prompt Stealer: Attempts to extract the LLM's internal configuration or sensitive information.
- Broken: Attack type attempts that LLM succumbed to.
- Resilient: Attack type attempts that LLM resisted.
- Errors: Attack type attempts that had inconclusive results.
- [X] Google Colab Notebook
- [X] Adjust the output evaluation mechanism for prompt dataset testing
- [ ] Continue adding new GenAI attack types
- [ ] Enhaced reporting capabilites
- [ ] Hardening recommendations
Turn this into a community project! We want this to be useful to everyone building GenAI applications. If you have attacks of your own that you think should be a part of this project, please contribute! This is how: https://github.com/prompt-security/ps-fuzz/blob/main/CONTRIBUTING.md
Interested in contributing to the development of our tools? Great! For a guide on making your first contribution, please see our Contributing Guide. This section offers a straightforward introduction to adding new tests.
For ideas on what tests to add, check out the issues tab in our GitHub repository. Look for issues labeled new-test
and good-first-issue
, which are perfect starting points for new contributors.
For Tasks:
Click tags to check more tools for each tasksFor Jobs:
Alternative AI tools for ps-fuzz
Similar Open Source Tools
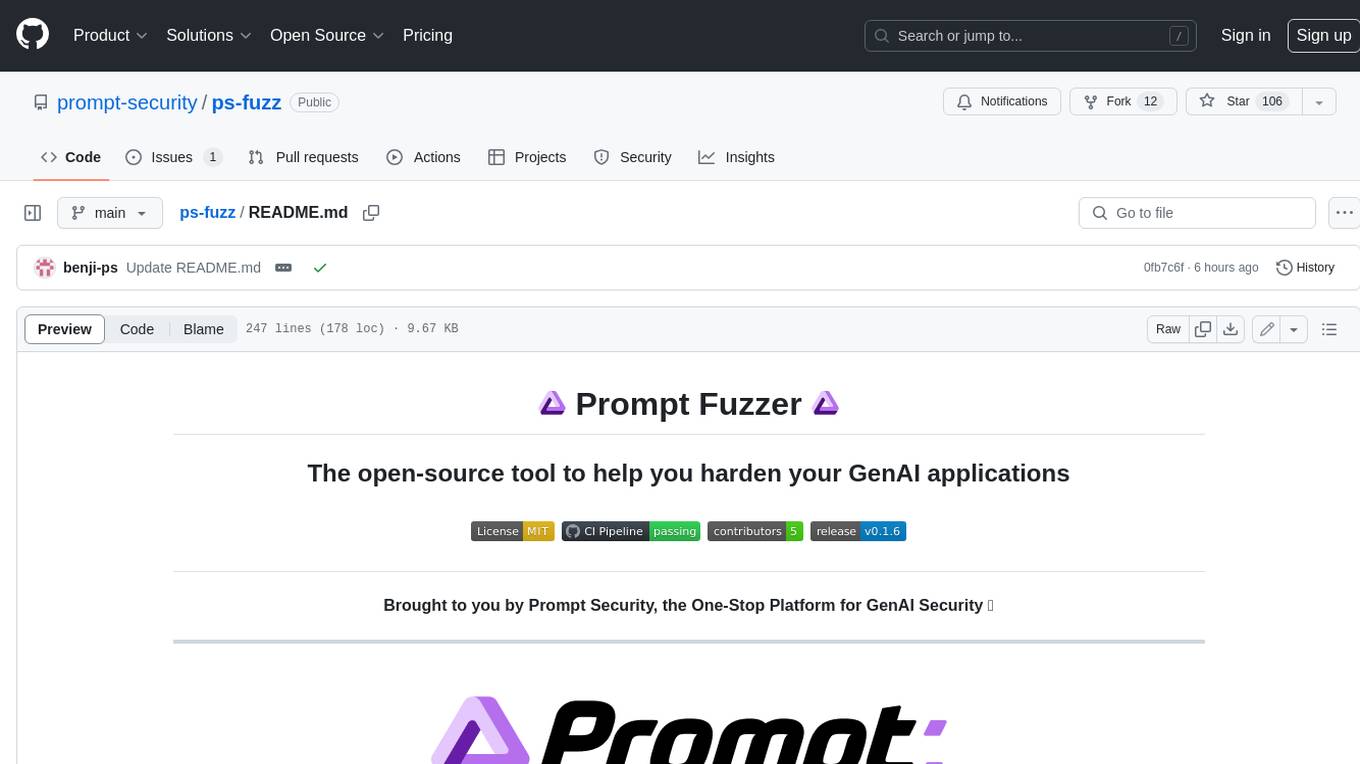
ps-fuzz
The Prompt Fuzzer is an open-source tool that helps you assess the security of your GenAI application's system prompt against various dynamic LLM-based attacks. It provides a security evaluation based on the outcome of these attack simulations, enabling you to strengthen your system prompt as needed. The Prompt Fuzzer dynamically tailors its tests to your application's unique configuration and domain. The Fuzzer also includes a Playground chat interface, giving you the chance to iteratively improve your system prompt, hardening it against a wide spectrum of generative AI attacks.
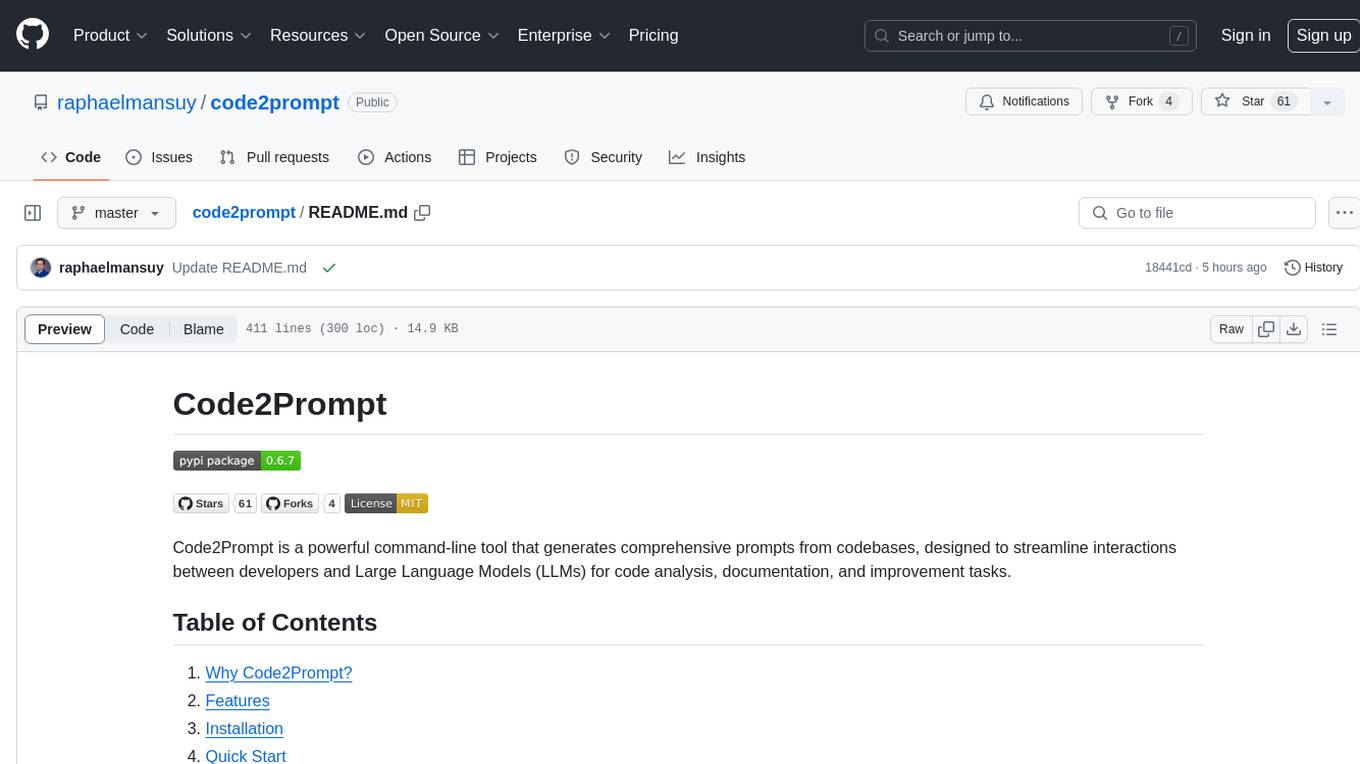
code2prompt
Code2Prompt is a powerful command-line tool that generates comprehensive prompts from codebases, designed to streamline interactions between developers and Large Language Models (LLMs) for code analysis, documentation, and improvement tasks. It bridges the gap between codebases and LLMs by converting projects into AI-friendly prompts, enabling users to leverage AI for various software development tasks. The tool offers features like holistic codebase representation, intelligent source tree generation, customizable prompt templates, smart token management, Gitignore integration, flexible file handling, clipboard-ready output, multiple output options, and enhanced code readability.
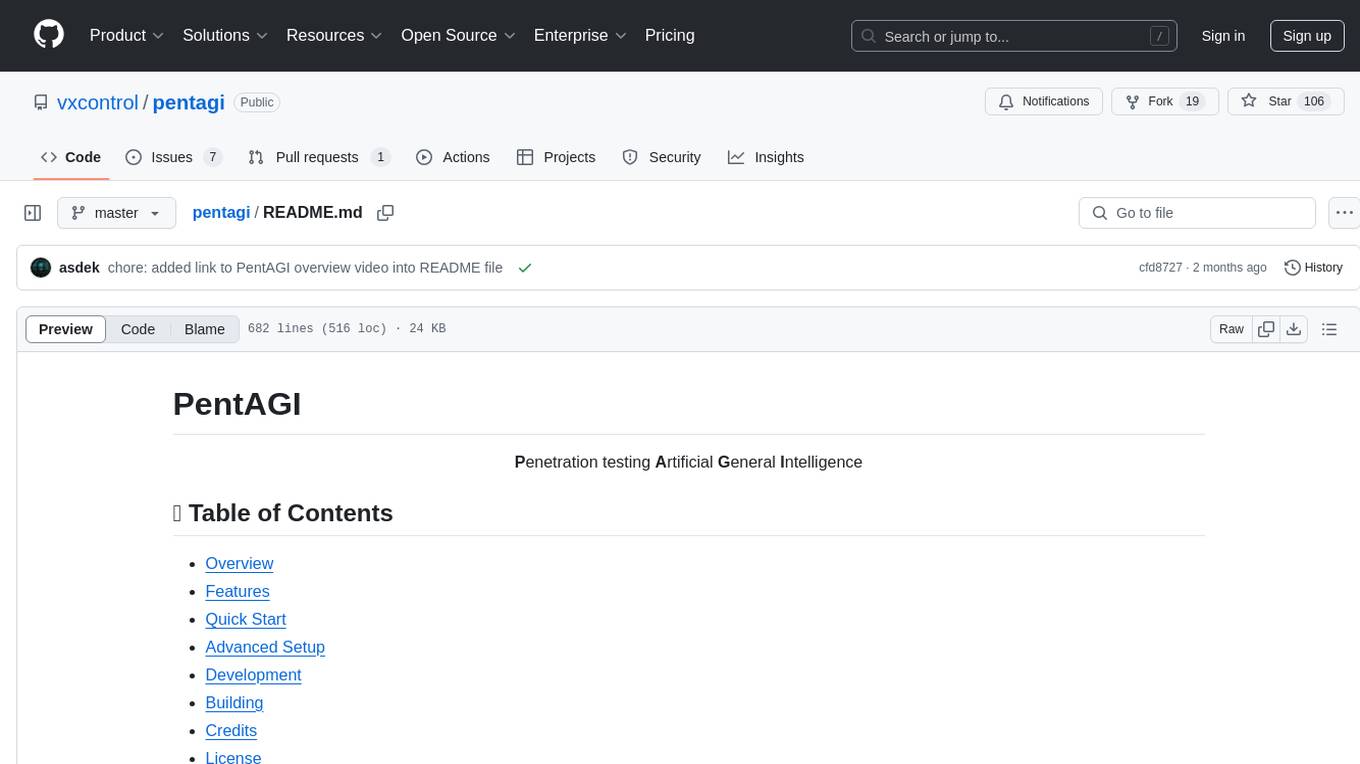
pentagi
PentAGI is an innovative tool for automated security testing that leverages cutting-edge artificial intelligence technologies. It is designed for information security professionals, researchers, and enthusiasts who need a powerful and flexible solution for conducting penetration tests. The tool provides secure and isolated operations in a sandboxed Docker environment, fully autonomous AI-powered agent for penetration testing steps, a suite of 20+ professional security tools, smart memory system for storing research results, web intelligence for gathering information, integration with external search systems, team delegation system, comprehensive monitoring and reporting, modern interface, API integration, persistent storage, scalable architecture, self-hosted solution, flexible authentication, and quick deployment through Docker Compose.
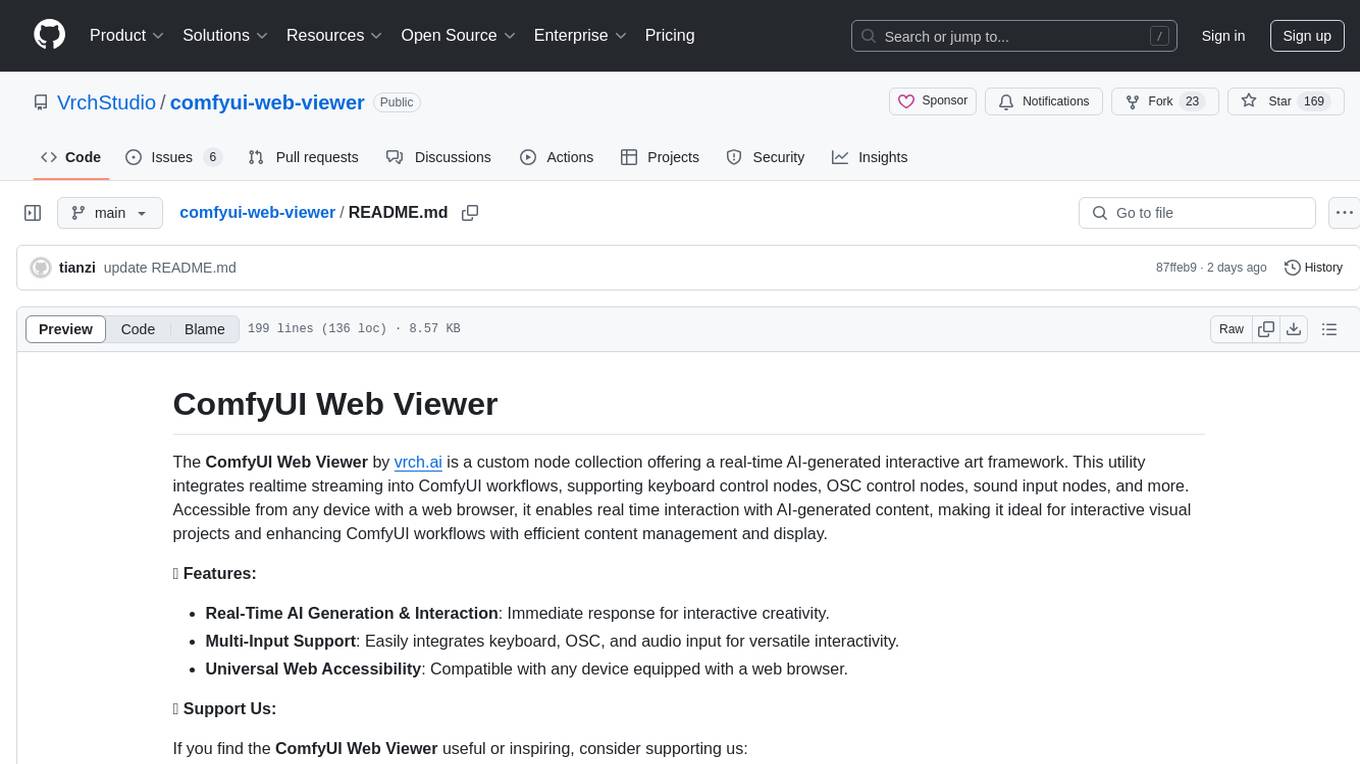
comfyui-web-viewer
The ComfyUI Web Viewer by vrch.ai is a real-time AI-generated interactive art framework that integrates realtime streaming into ComfyUI workflows. It supports keyboard control nodes, OSC control nodes, sound input nodes, and more, accessible from any device with a web browser. It enables real-time interaction with AI-generated content, ideal for interactive visual projects and enhancing ComfyUI workflows with efficient content management and display.
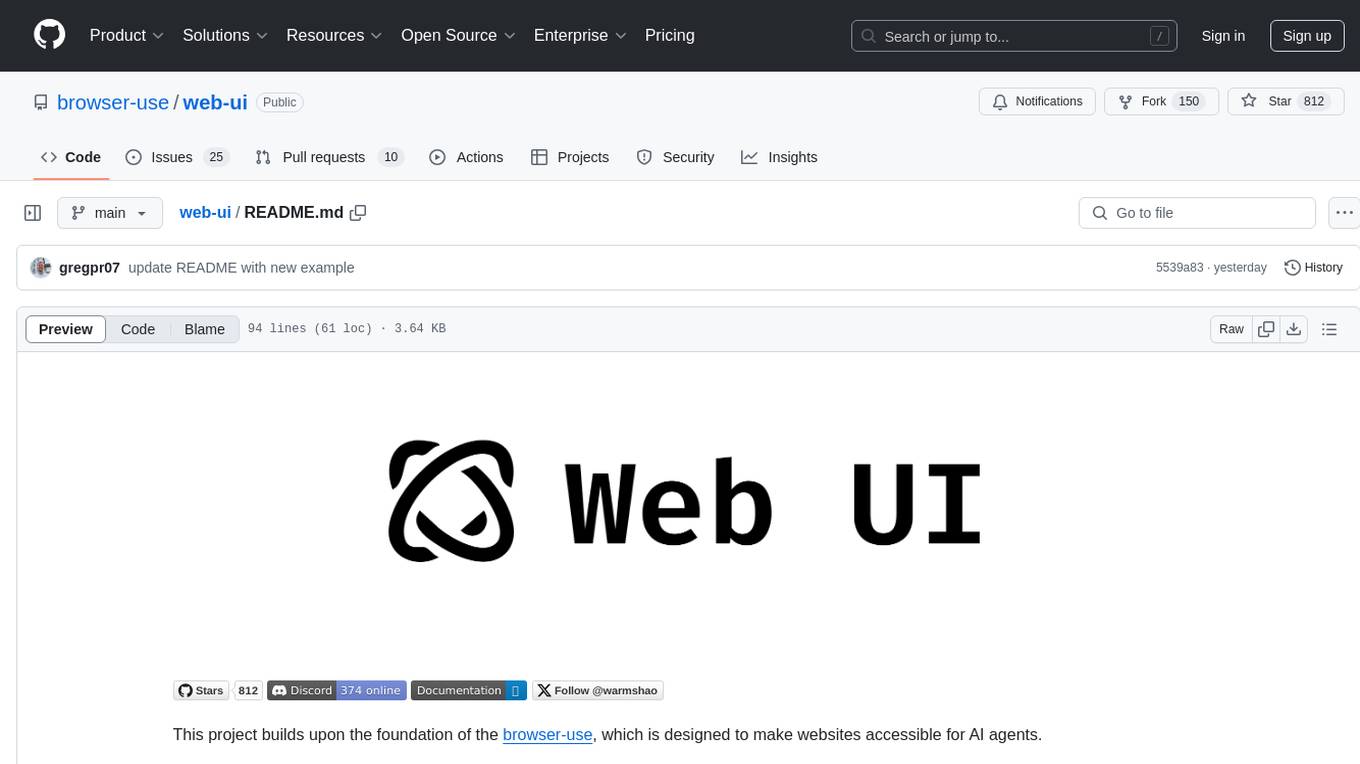
web-ui
WebUI is a user-friendly tool built on Gradio that enhances website accessibility for AI agents. It supports various Large Language Models (LLMs) and allows custom browser integration for seamless interaction. The tool eliminates the need for re-login and authentication challenges, offering high-definition screen recording capabilities.
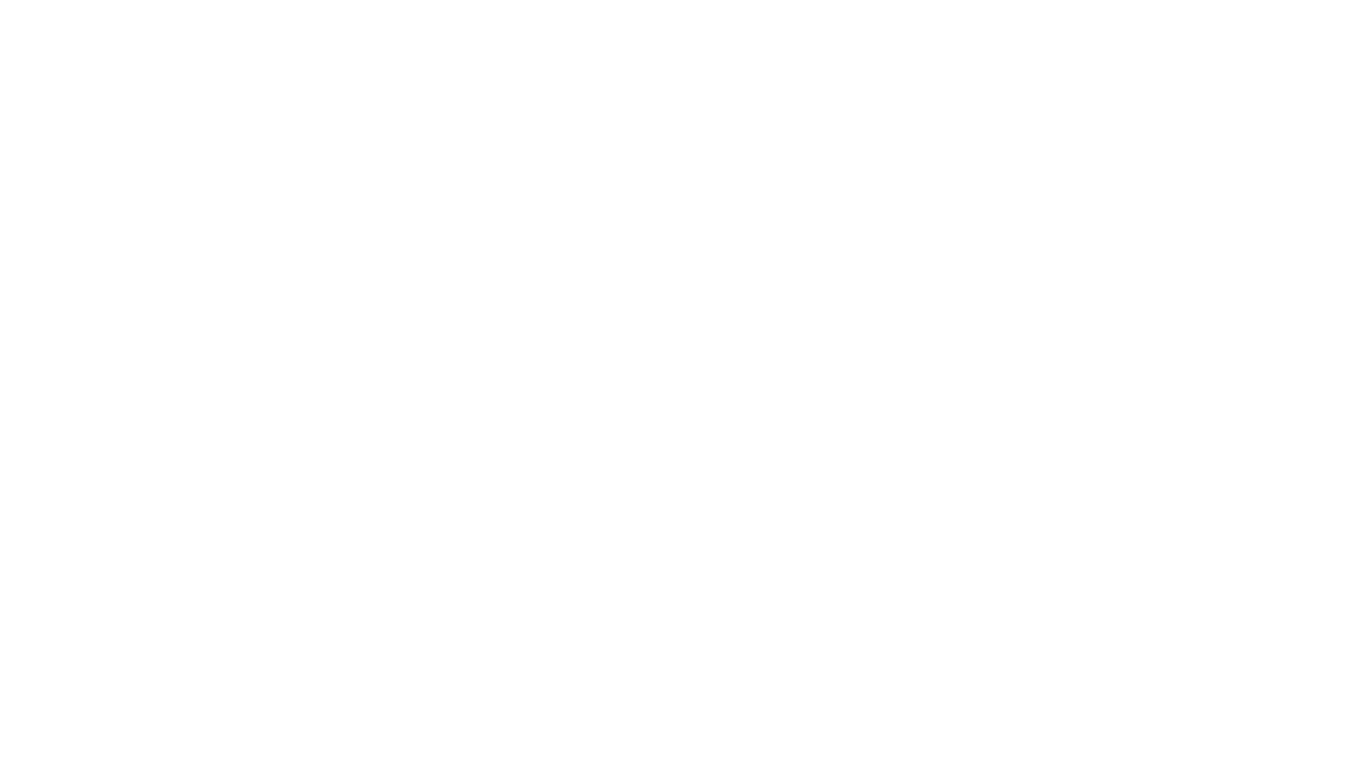
rlama
RLAMA is a powerful AI-driven question-answering tool that seamlessly integrates with local Ollama models. It enables users to create, manage, and interact with Retrieval-Augmented Generation (RAG) systems tailored to their documentation needs. RLAMA follows a clean architecture pattern with clear separation of concerns, focusing on lightweight and portable RAG capabilities with minimal dependencies. The tool processes documents, generates embeddings, stores RAG systems locally, and provides contextually-informed responses to user queries. Supported document formats include text, code, and various document types, with troubleshooting steps available for common issues like Ollama accessibility, text extraction problems, and relevance of answers.
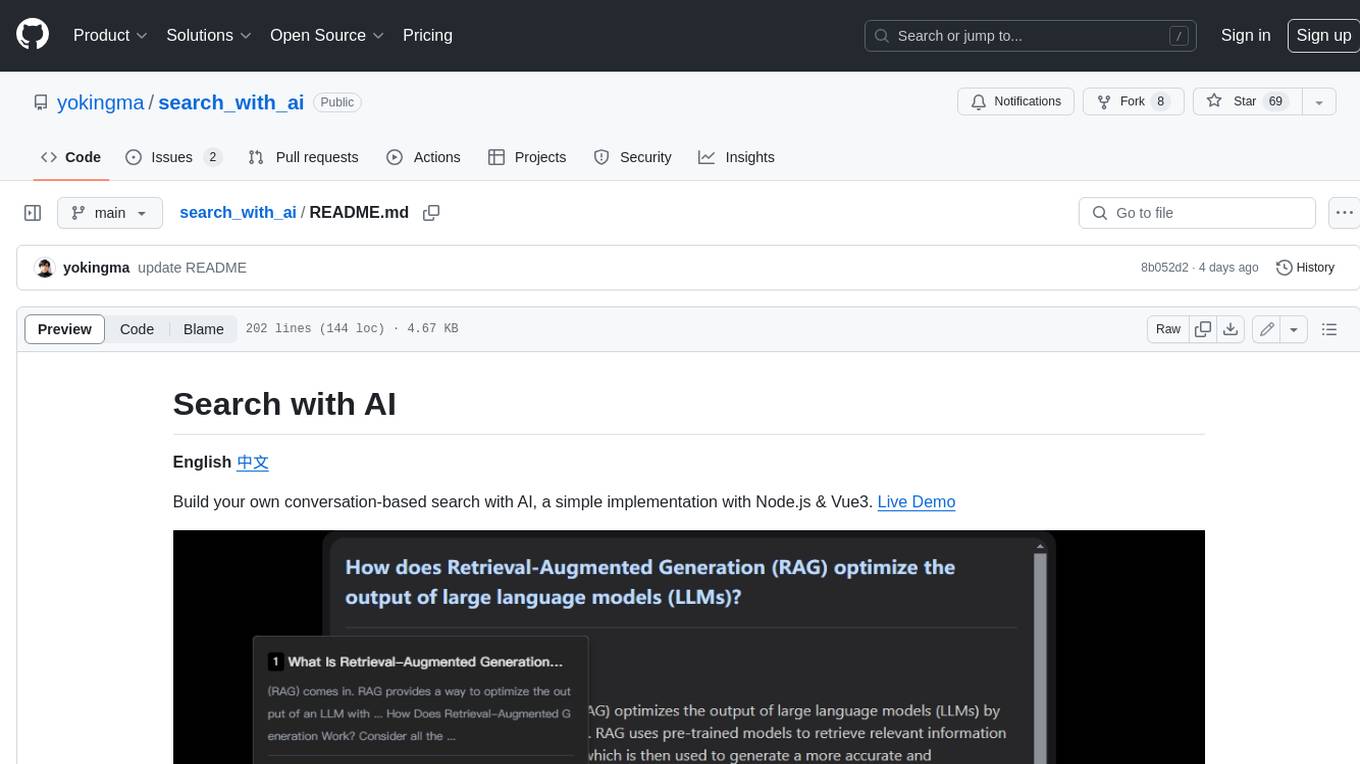
search_with_ai
Build your own conversation-based search with AI, a simple implementation with Node.js & Vue3. Live Demo Features: * Built-in support for LLM: OpenAI, Google, Lepton, Ollama(Free) * Built-in support for search engine: Bing, Sogou, Google, SearXNG(Free) * Customizable pretty UI interface * Support dark mode * Support mobile display * Support local LLM with Ollama * Support i18n * Support Continue Q&A with contexts.
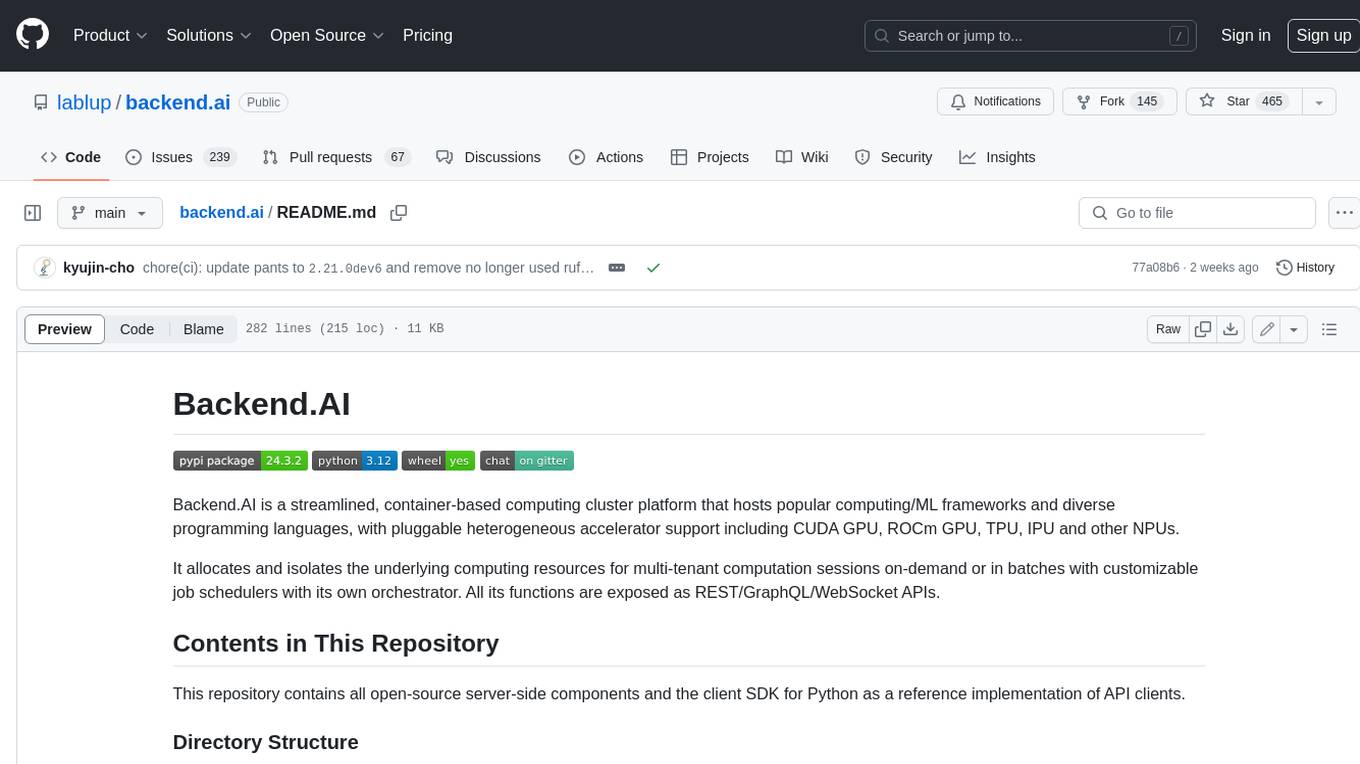
backend.ai
Backend.AI is a streamlined, container-based computing cluster platform that hosts popular computing/ML frameworks and diverse programming languages, with pluggable heterogeneous accelerator support including CUDA GPU, ROCm GPU, TPU, IPU and other NPUs. It allocates and isolates the underlying computing resources for multi-tenant computation sessions on-demand or in batches with customizable job schedulers with its own orchestrator. All its functions are exposed as REST/GraphQL/WebSocket APIs.
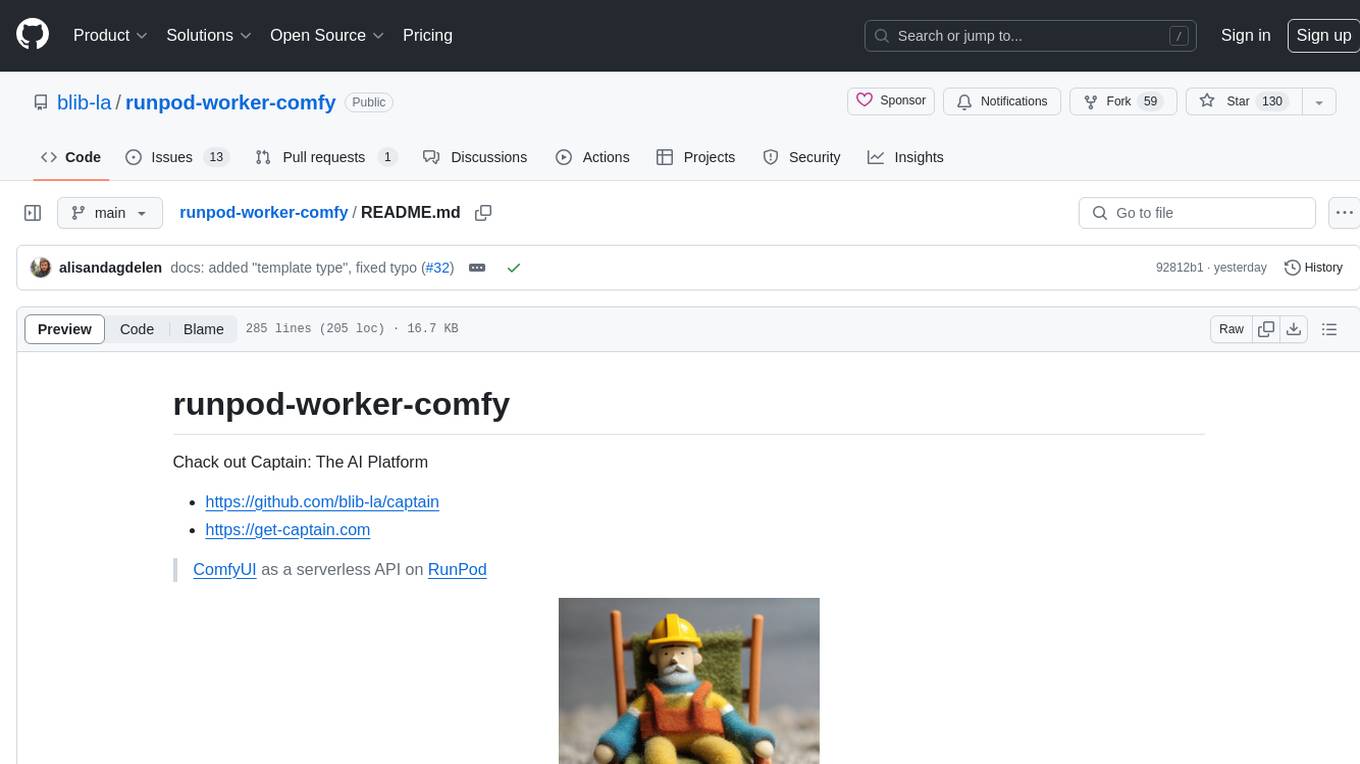
runpod-worker-comfy
runpod-worker-comfy is a serverless API tool that allows users to run any ComfyUI workflow to generate an image. Users can provide input images as base64-encoded strings, and the generated image can be returned as a base64-encoded string or uploaded to AWS S3. The tool is built on Ubuntu + NVIDIA CUDA and provides features like built-in checkpoints and VAE models. Users can configure environment variables to upload images to AWS S3 and interact with the RunPod API to generate images. The tool also supports local testing and deployment to Docker hub using Github Actions.
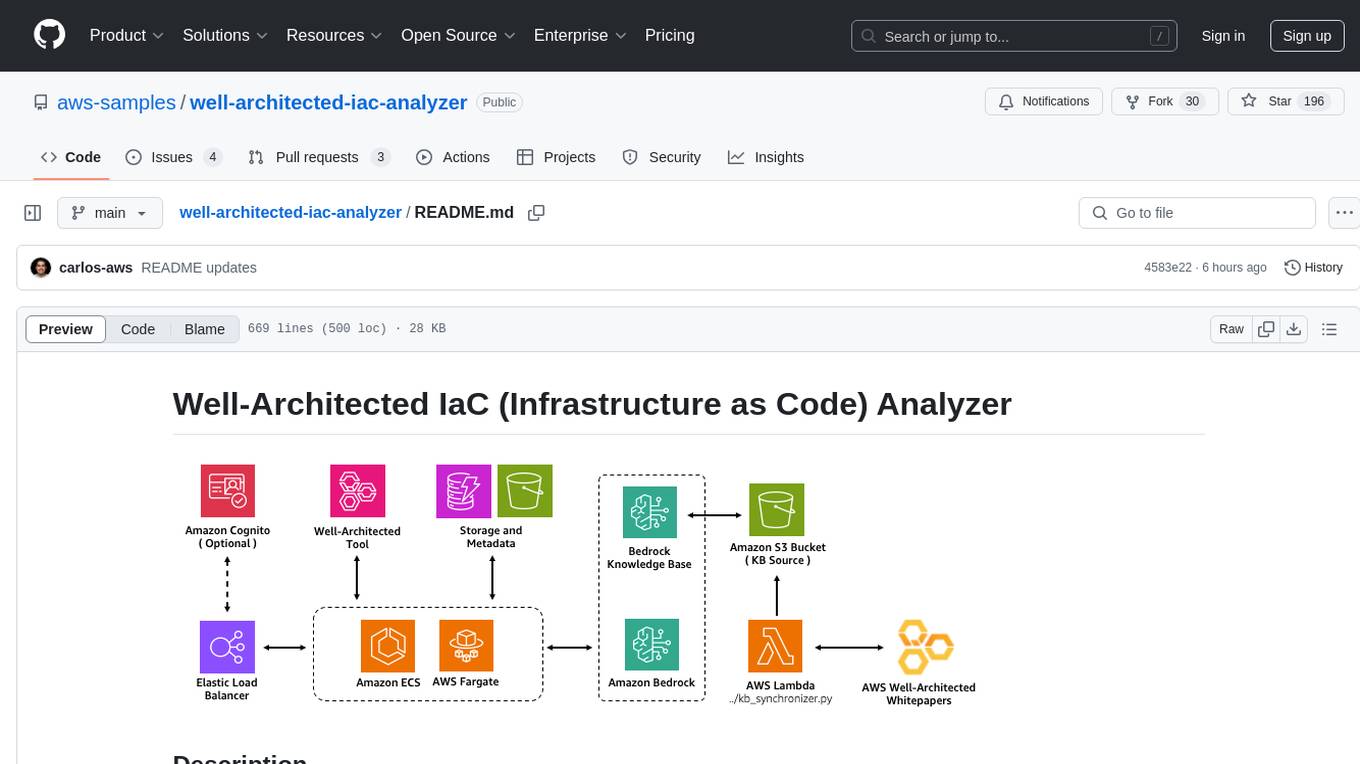
well-architected-iac-analyzer
Well-Architected Infrastructure as Code (IaC) Analyzer is a project demonstrating how generative AI can evaluate infrastructure code for alignment with best practices. It features a modern web application allowing users to upload IaC documents, complete IaC projects, or architecture diagrams for assessment. The tool provides insights into infrastructure code alignment with AWS best practices, offers suggestions for improving cloud architecture designs, and can generate IaC templates from architecture diagrams. Users can analyze CloudFormation, Terraform, or AWS CDK templates, architecture diagrams in PNG or JPEG format, and complete IaC projects with supporting documents. Real-time analysis against Well-Architected best practices, integration with AWS Well-Architected Tool, and export of analysis results and recommendations are included.
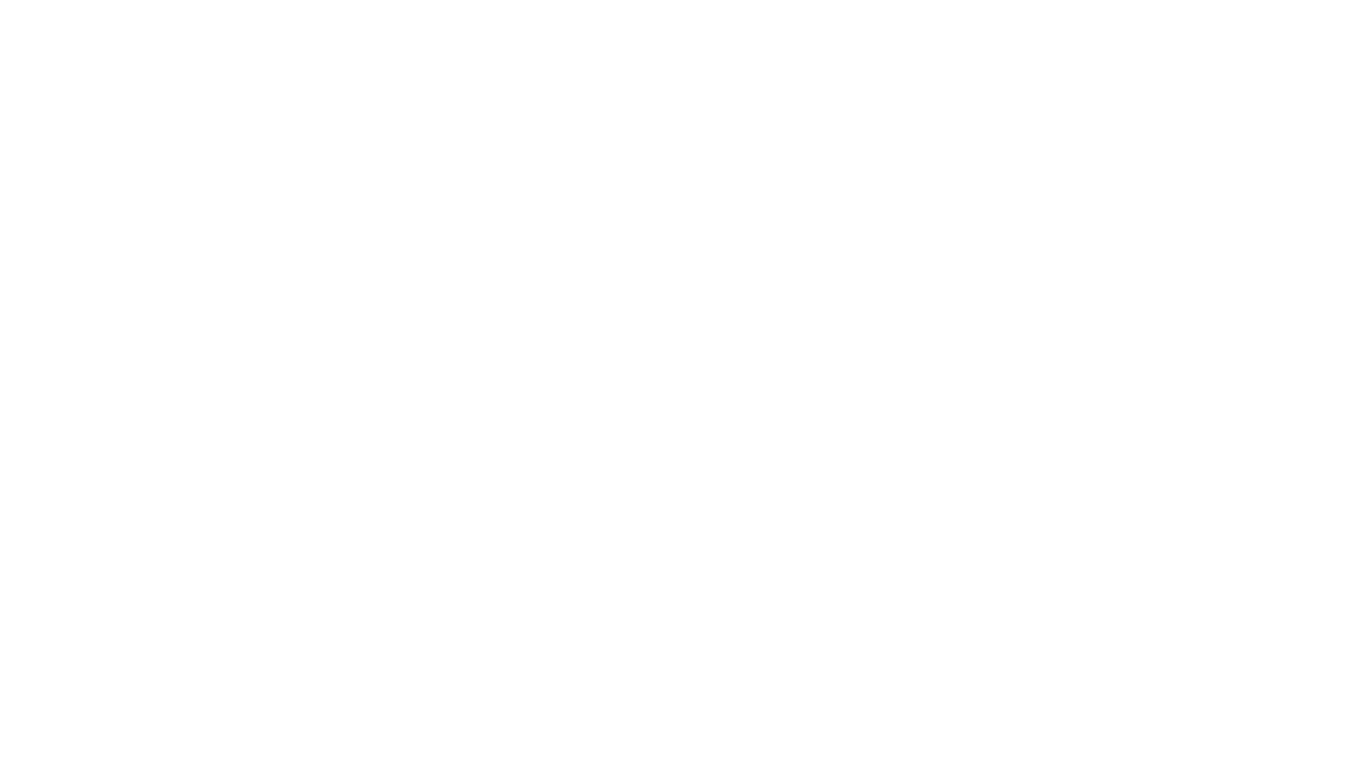
Zero
Zero is an open-source AI email solution that allows users to self-host their email app while integrating external services like Gmail. It aims to modernize and enhance emails through AI agents, offering features like open-source transparency, AI-driven enhancements, data privacy, self-hosting freedom, unified inbox, customizable UI, and developer-friendly extensibility. Built with modern technologies, Zero provides a reliable tech stack including Next.js, React, TypeScript, TailwindCSS, Node.js, Drizzle ORM, and PostgreSQL. Users can set up Zero using standard setup or Dev Container setup for VS Code users, with detailed environment setup instructions for Better Auth, Google OAuth, and optional GitHub OAuth. Database setup involves starting a local PostgreSQL instance, setting up database connection, and executing database commands for dependencies, tables, migrations, and content viewing.
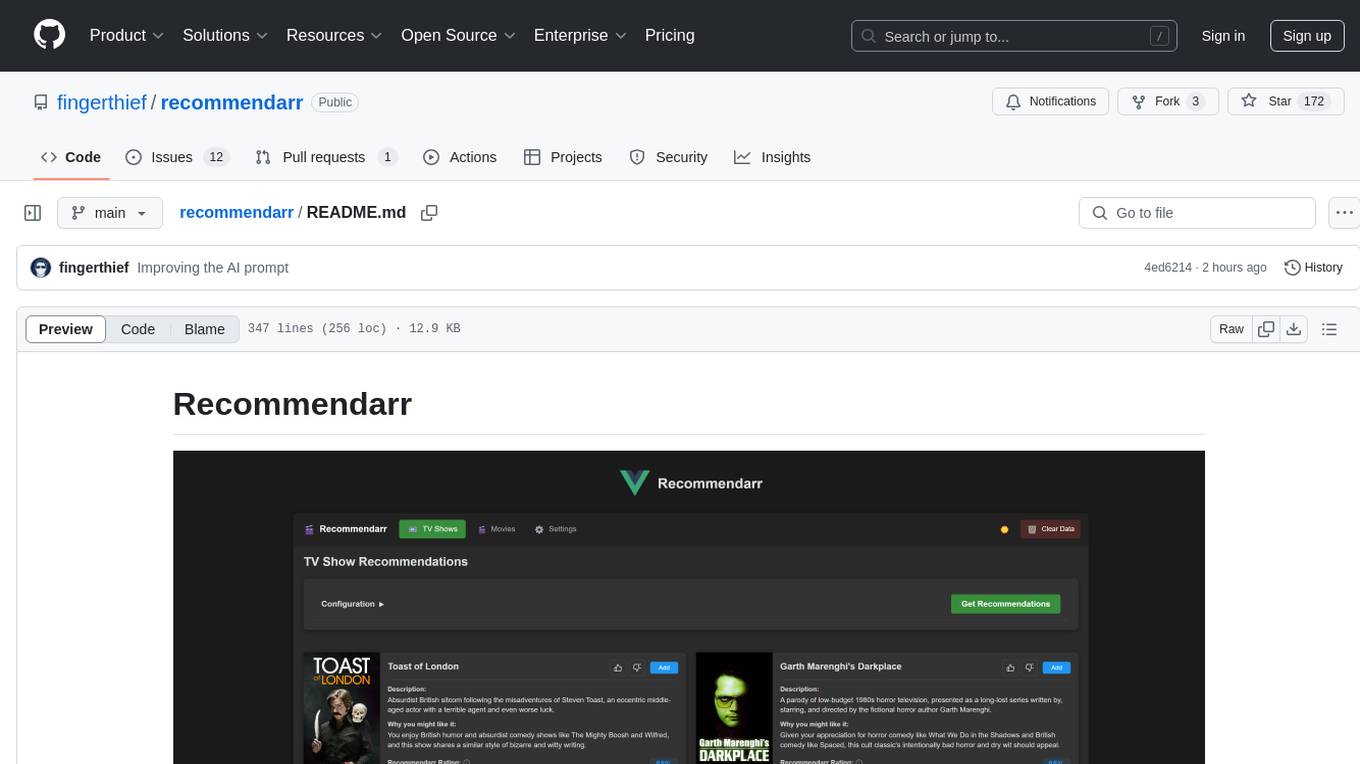
recommendarr
Recommendarr is a tool that generates personalized TV show and movie recommendations based on your Sonarr, Radarr, Plex, and Jellyfin libraries using AI. It offers AI-powered recommendations, media server integration, flexible AI support, watch history analysis, customization options, and dark/light mode toggle. Users can connect their media libraries and watch history services, configure AI service settings, and get personalized recommendations based on genre, language, and mood/vibe preferences. The tool works with any OpenAI-compatible API and offers various recommended models for different cost options and performance levels. It provides personalized suggestions, detailed information, filter options, watch history analysis, and one-click adding of recommended content to Sonarr/Radarr.
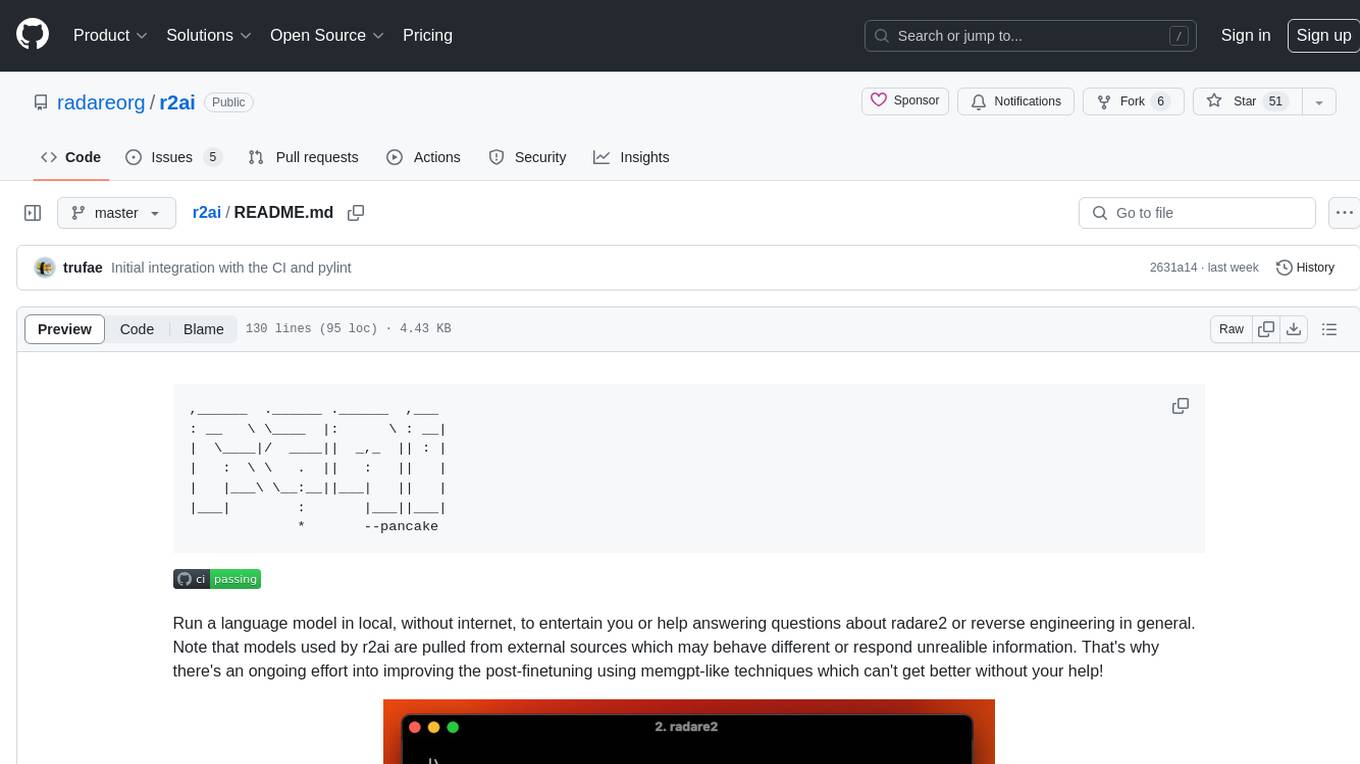
r2ai
r2ai is a tool designed to run a language model locally without internet access. It can be used to entertain users or assist in answering questions related to radare2 or reverse engineering. The tool allows users to prompt the language model, index large codebases, slurp file contents, embed the output of an r2 command, define different system-level assistant roles, set environment variables, and more. It is accessible as an r2lang-python plugin and can be scripted from various languages. Users can use different models, adjust query templates dynamically, load multiple models, and make them communicate with each other.
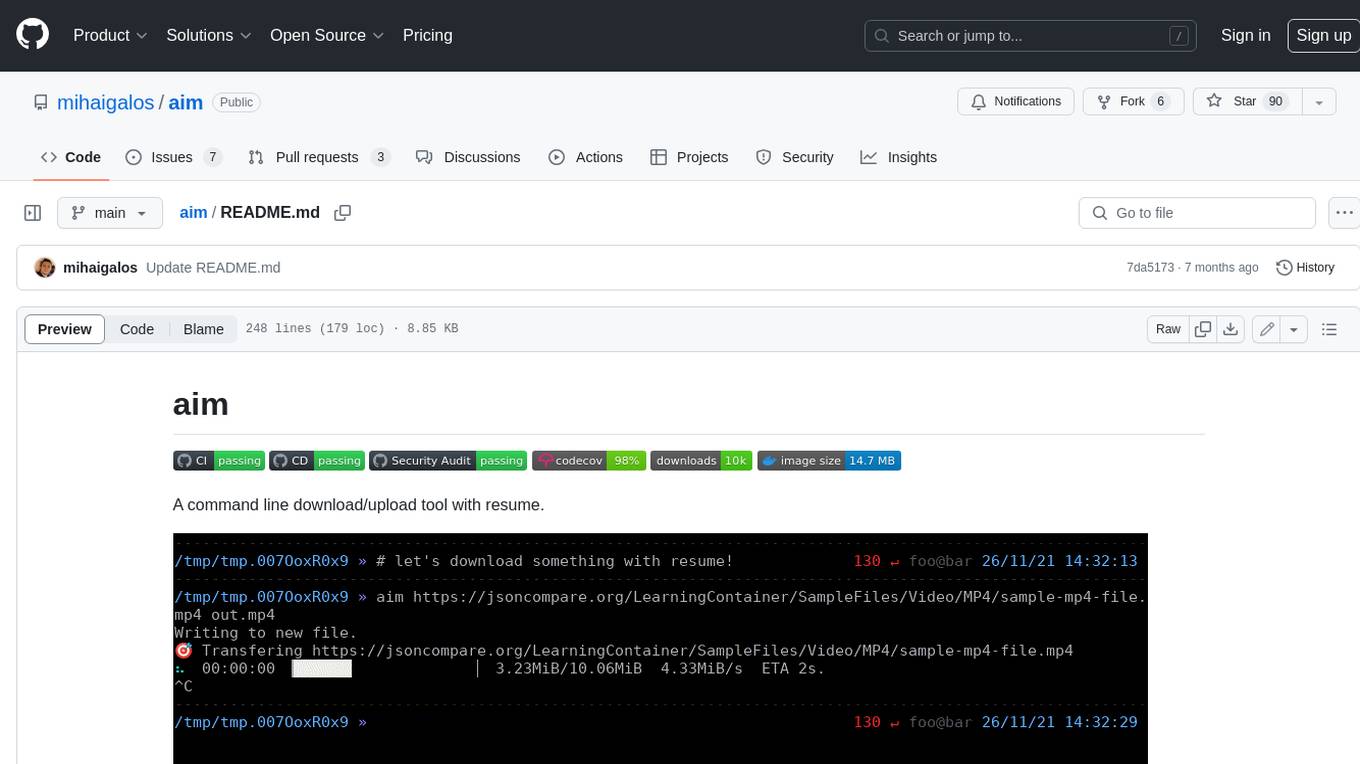
aim
Aim is a command-line tool for downloading and uploading files with resume support. It supports various protocols including HTTP, FTP, SFTP, SSH, and S3. Aim features an interactive mode for easy navigation and selection of files, as well as the ability to share folders over HTTP for easy access from other devices. Additionally, it offers customizable progress indicators and output formats, and can be integrated with other commands through piping. Aim can be installed via pre-built binaries or by compiling from source, and is also available as a Docker image for platform-independent usage.
For similar tasks
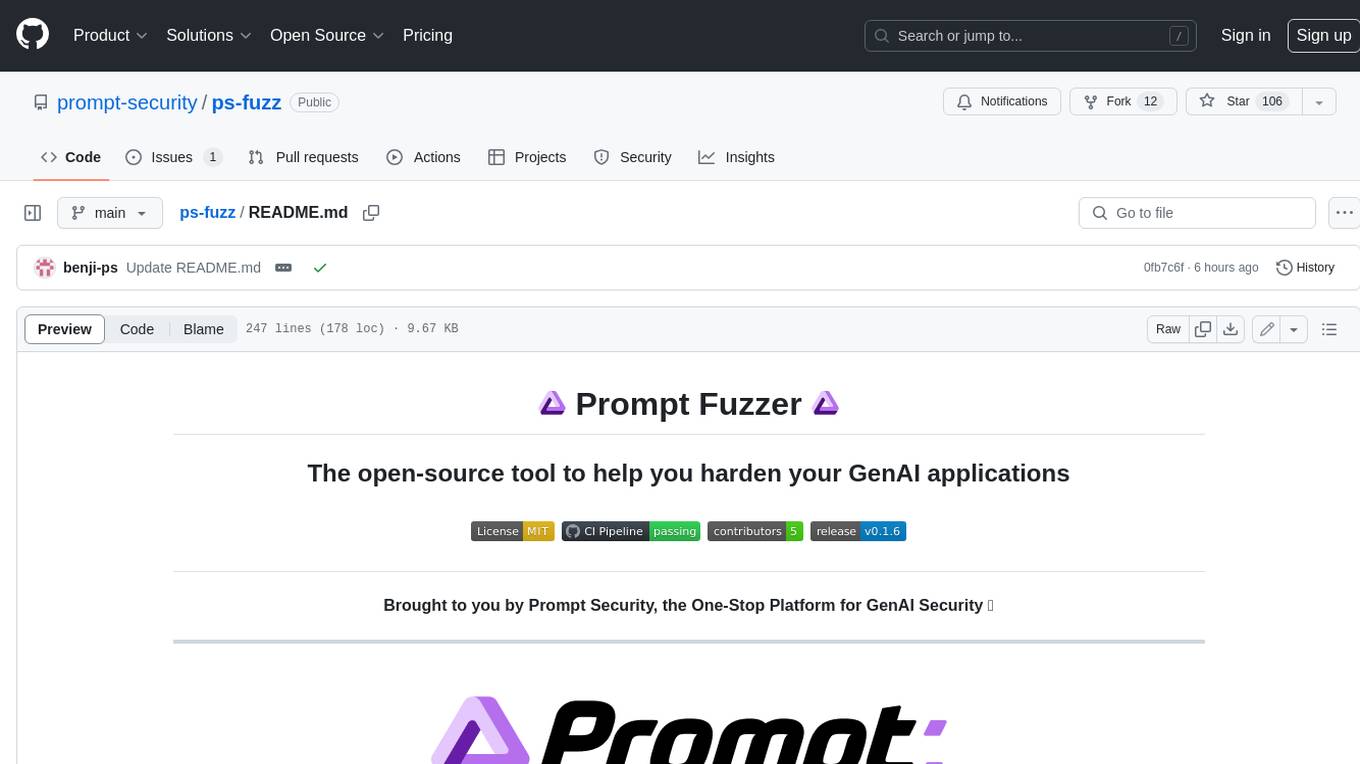
ps-fuzz
The Prompt Fuzzer is an open-source tool that helps you assess the security of your GenAI application's system prompt against various dynamic LLM-based attacks. It provides a security evaluation based on the outcome of these attack simulations, enabling you to strengthen your system prompt as needed. The Prompt Fuzzer dynamically tailors its tests to your application's unique configuration and domain. The Fuzzer also includes a Playground chat interface, giving you the chance to iteratively improve your system prompt, hardening it against a wide spectrum of generative AI attacks.
For similar jobs
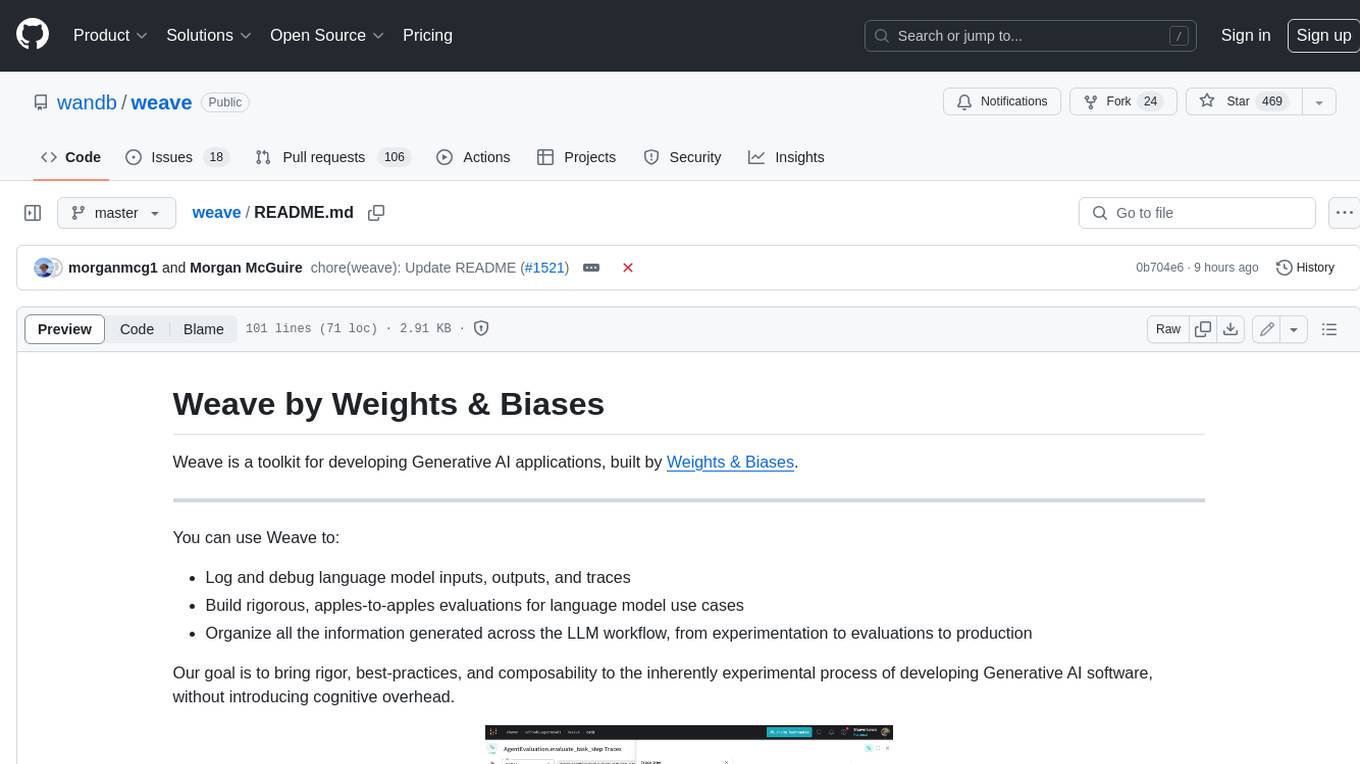
weave
Weave is a toolkit for developing Generative AI applications, built by Weights & Biases. With Weave, you can log and debug language model inputs, outputs, and traces; build rigorous, apples-to-apples evaluations for language model use cases; and organize all the information generated across the LLM workflow, from experimentation to evaluations to production. Weave aims to bring rigor, best-practices, and composability to the inherently experimental process of developing Generative AI software, without introducing cognitive overhead.
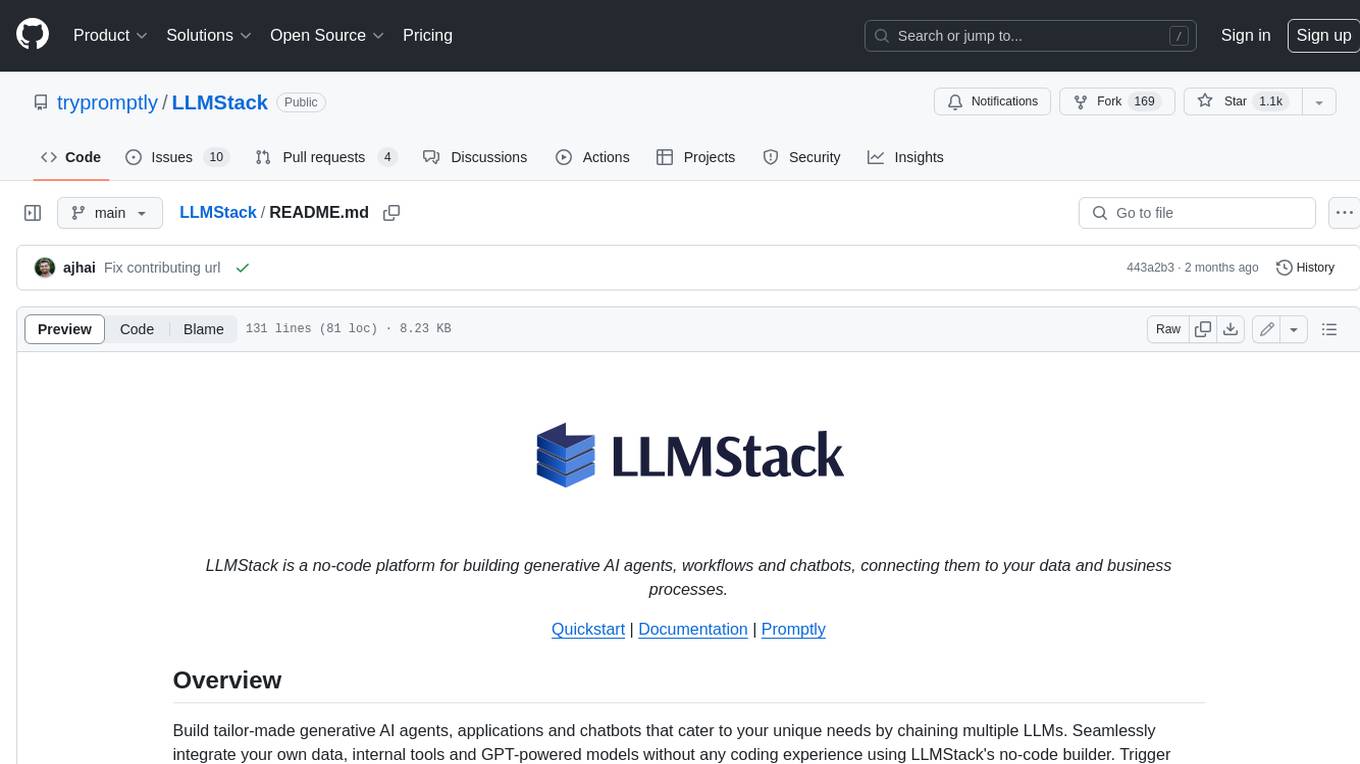
LLMStack
LLMStack is a no-code platform for building generative AI agents, workflows, and chatbots. It allows users to connect their own data, internal tools, and GPT-powered models without any coding experience. LLMStack can be deployed to the cloud or on-premise and can be accessed via HTTP API or triggered from Slack or Discord.
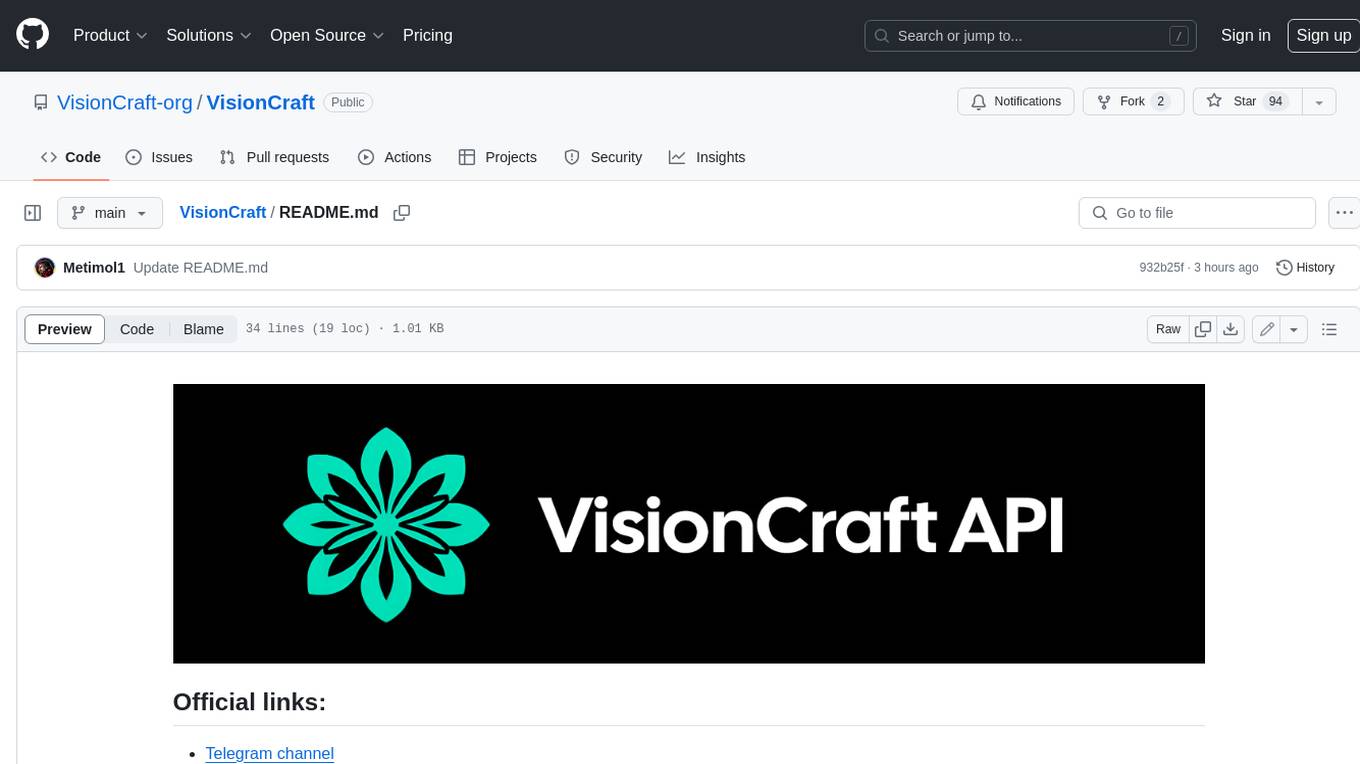
VisionCraft
The VisionCraft API is a free API for using over 100 different AI models. From images to sound.
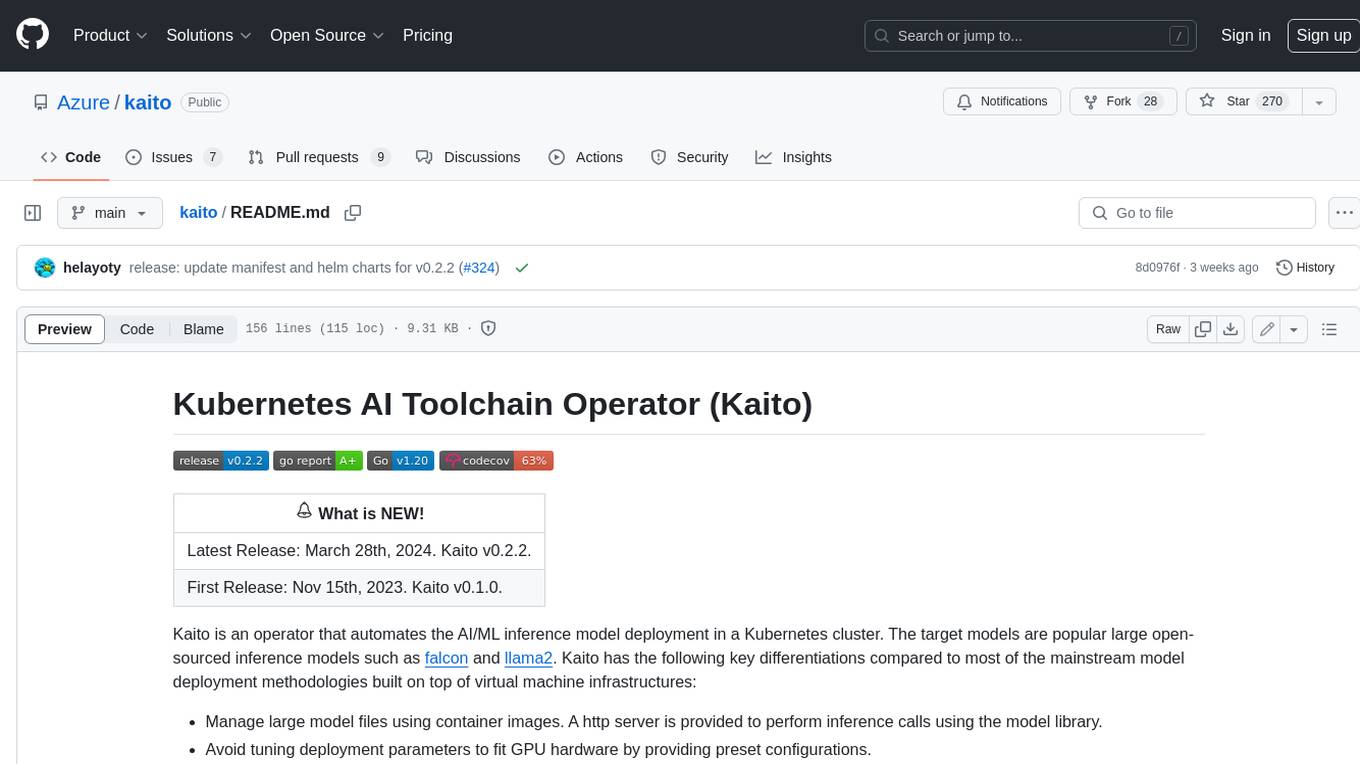
kaito
Kaito is an operator that automates the AI/ML inference model deployment in a Kubernetes cluster. It manages large model files using container images, avoids tuning deployment parameters to fit GPU hardware by providing preset configurations, auto-provisions GPU nodes based on model requirements, and hosts large model images in the public Microsoft Container Registry (MCR) if the license allows. Using Kaito, the workflow of onboarding large AI inference models in Kubernetes is largely simplified.
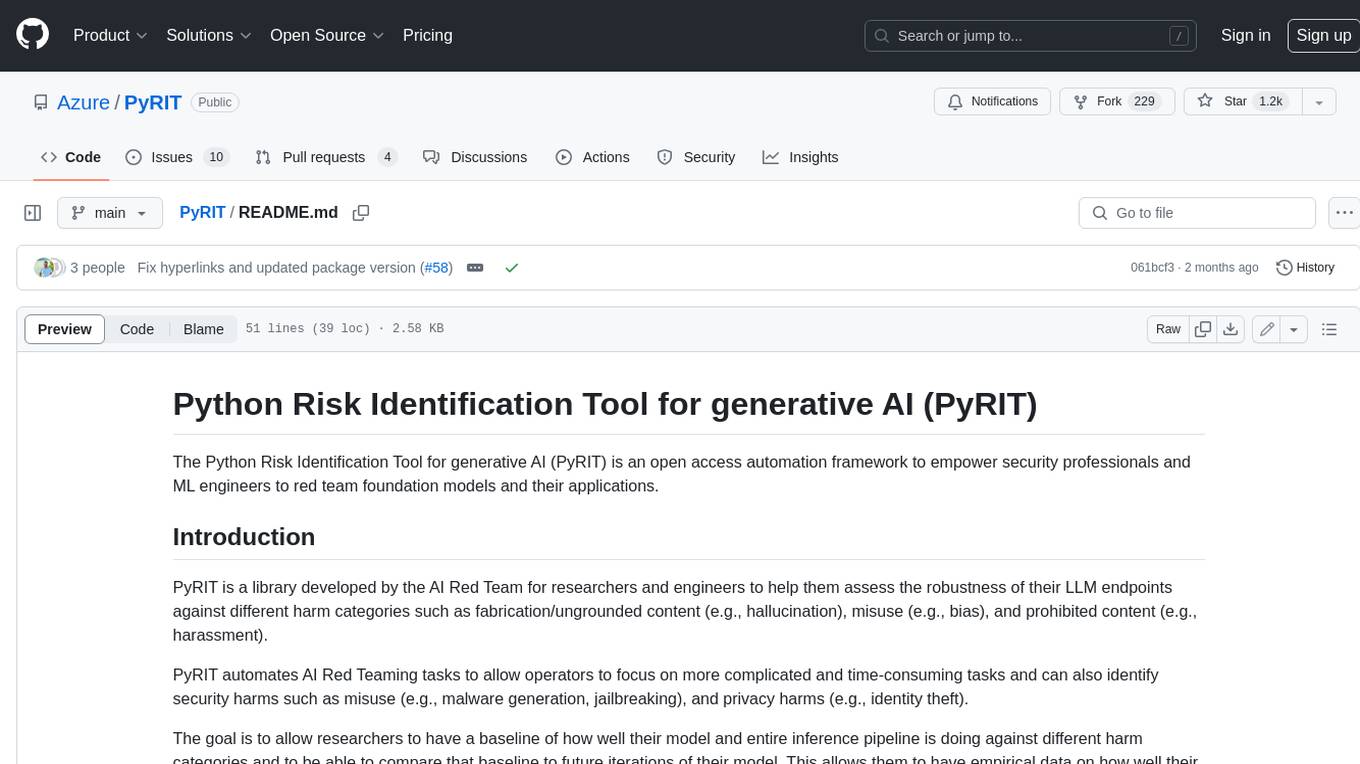
PyRIT
PyRIT is an open access automation framework designed to empower security professionals and ML engineers to red team foundation models and their applications. It automates AI Red Teaming tasks to allow operators to focus on more complicated and time-consuming tasks and can also identify security harms such as misuse (e.g., malware generation, jailbreaking), and privacy harms (e.g., identity theft). The goal is to allow researchers to have a baseline of how well their model and entire inference pipeline is doing against different harm categories and to be able to compare that baseline to future iterations of their model. This allows them to have empirical data on how well their model is doing today, and detect any degradation of performance based on future improvements.
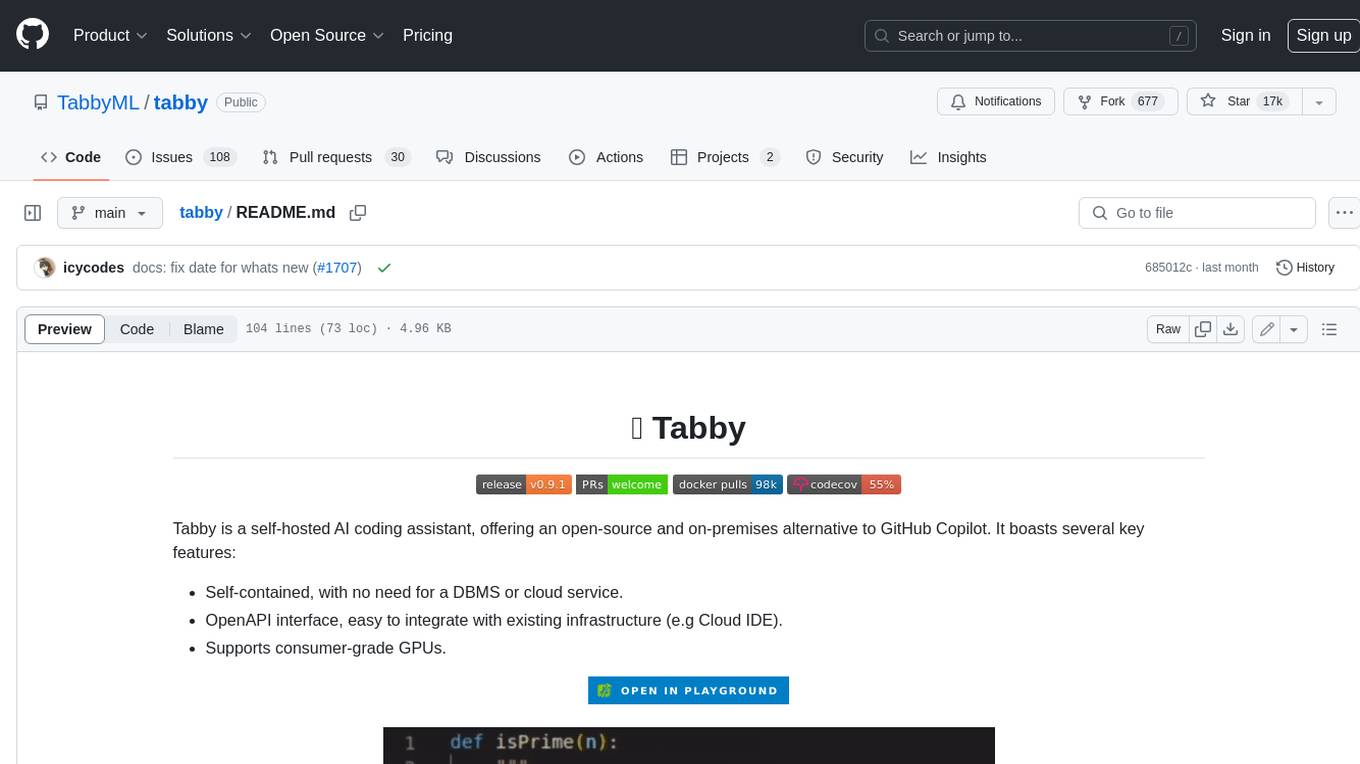
tabby
Tabby is a self-hosted AI coding assistant, offering an open-source and on-premises alternative to GitHub Copilot. It boasts several key features: * Self-contained, with no need for a DBMS or cloud service. * OpenAPI interface, easy to integrate with existing infrastructure (e.g Cloud IDE). * Supports consumer-grade GPUs.
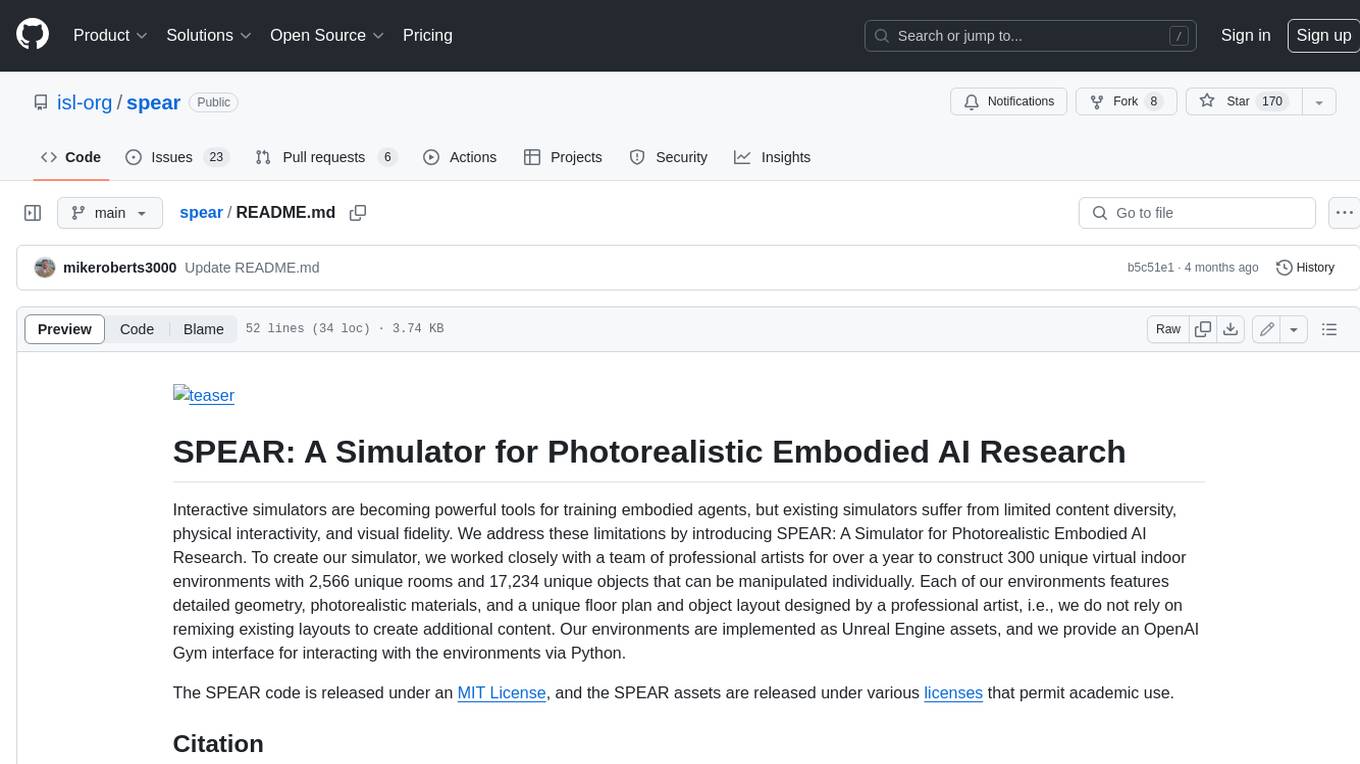
spear
SPEAR (Simulator for Photorealistic Embodied AI Research) is a powerful tool for training embodied agents. It features 300 unique virtual indoor environments with 2,566 unique rooms and 17,234 unique objects that can be manipulated individually. Each environment is designed by a professional artist and features detailed geometry, photorealistic materials, and a unique floor plan and object layout. SPEAR is implemented as Unreal Engine assets and provides an OpenAI Gym interface for interacting with the environments via Python.
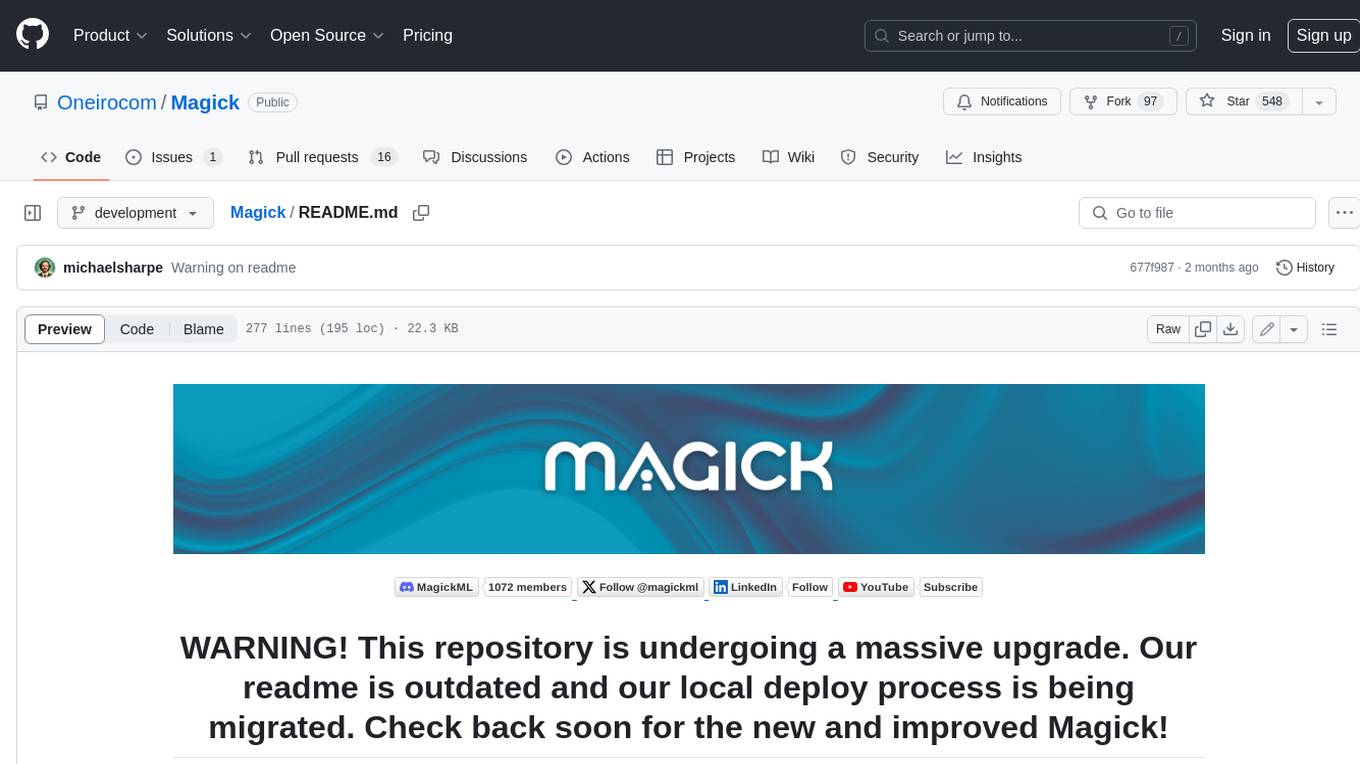
Magick
Magick is a groundbreaking visual AIDE (Artificial Intelligence Development Environment) for no-code data pipelines and multimodal agents. Magick can connect to other services and comes with nodes and templates well-suited for intelligent agents, chatbots, complex reasoning systems and realistic characters.