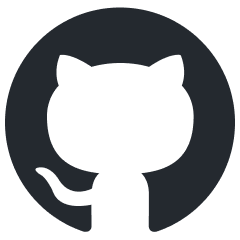
pentagi
✨ Fully autonomous AI Agents system capable of performing complex penetration testing tasks
Stars: 170
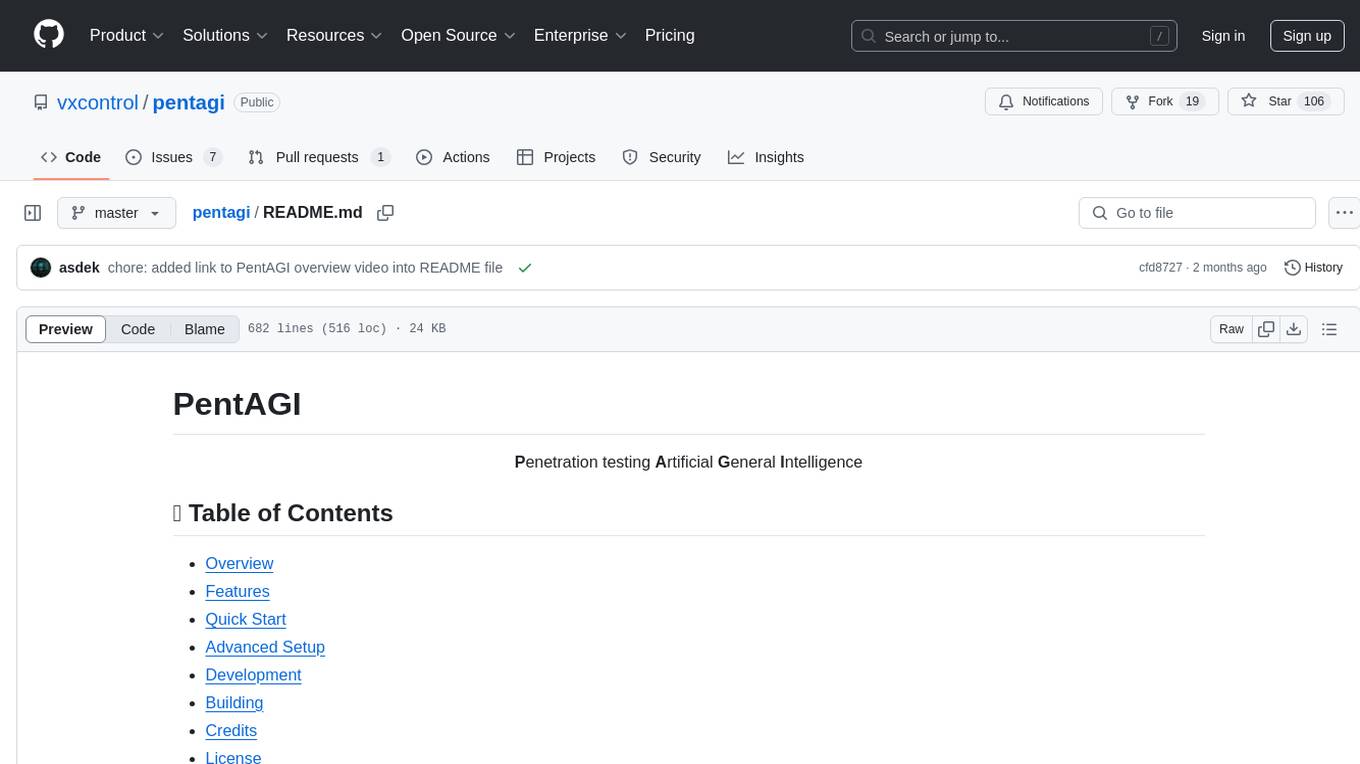
PentAGI is an innovative tool for automated security testing that leverages cutting-edge artificial intelligence technologies. It is designed for information security professionals, researchers, and enthusiasts who need a powerful and flexible solution for conducting penetration tests. The tool provides secure and isolated operations in a sandboxed Docker environment, fully autonomous AI-powered agent for penetration testing steps, a suite of 20+ professional security tools, smart memory system for storing research results, web intelligence for gathering information, integration with external search systems, team delegation system, comprehensive monitoring and reporting, modern interface, API integration, persistent storage, scalable architecture, self-hosted solution, flexible authentication, and quick deployment through Docker Compose.
README:
- Overview
- Features
- Quick Start
- Advanced Setup
- Development
- Testing LLM Agents
- Function Testing with ftester
- Building
- Credits
- License
PentAGI is an innovative tool for automated security testing that leverages cutting-edge artificial intelligence technologies. The project is designed for information security professionals, researchers, and enthusiasts who need a powerful and flexible solution for conducting penetration tests.
You can watch the video PentAGI overview:
- 🛡️ Secure & Isolated. All operations are performed in a sandboxed Docker environment with complete isolation.
- 🤖 Fully Autonomous. AI-powered agent that automatically determines and executes penetration testing steps.
- 🔬 Professional Pentesting Tools. Built-in suite of 20+ professional security tools including nmap, metasploit, sqlmap, and more.
- 🧠 Smart Memory System. Long-term storage of research results and successful approaches for future use.
- 🔍 Web Intelligence. Built-in browser via scraper for gathering latest information from web sources.
- 🔎 External Search Systems. Integration with advanced search APIs including Tavily, Traversaal, Perplexity, DuckDuckGo and Google Custom Search for comprehensive information gathering.
- 👥 Team of Specialists. Delegation system with specialized AI agents for research, development, and infrastructure tasks.
- 📊 Comprehensive Monitoring. Detailed logging and integration with Grafana/Prometheus for real-time system observation.
- 📝 Detailed Reporting. Generation of thorough vulnerability reports with exploitation guides.
- 📦 Smart Container Management. Automatic Docker image selection based on specific task requirements.
- 📱 Modern Interface. Clean and intuitive web UI for system management and monitoring.
- 🔌 API Integration. Support for REST and GraphQL APIs for seamless external system integration.
- 💾 Persistent Storage. All commands and outputs are stored in PostgreSQL with pgvector extension.
- 🎯 Scalable Architecture. Microservices-based design supporting horizontal scaling.
- 🏠 Self-Hosted Solution. Complete control over your deployment and data.
- 🔑 Flexible Authentication. Support for various LLM providers (OpenAI, Anthropic, Deep Infra, OpenRouter, DeepSeek) and custom configurations.
- ⚡ Quick Deployment. Easy setup through Docker Compose with comprehensive environment configuration.
flowchart TB
classDef person fill:#08427B,stroke:#073B6F,color:#fff
classDef system fill:#1168BD,stroke:#0B4884,color:#fff
classDef external fill:#666666,stroke:#0B4884,color:#fff
pentester["👤 Security Engineer
(User of the system)"]
pentagi["✨ PentAGI
(Autonomous penetration testing system)"]
target["🎯 target-system
(System under test)"]
llm["🧠 llm-provider
(OpenAI/Anthropic/Custom)"]
search["🔍 search-systems
(Google/DuckDuckGo/Tavily/Traversaal/Perplexity)"]
langfuse["📊 langfuse-ui
(LLM Observability Dashboard)"]
grafana["📈 grafana
(System Monitoring Dashboard)"]
pentester --> |Uses HTTPS| pentagi
pentester --> |Monitors AI HTTPS| langfuse
pentester --> |Monitors System HTTPS| grafana
pentagi --> |Tests Various protocols| target
pentagi --> |Queries HTTPS| llm
pentagi --> |Searches HTTPS| search
pentagi --> |Reports HTTPS| langfuse
pentagi --> |Reports HTTPS| grafana
class pentester person
class pentagi system
class target,llm,search,langfuse,grafana external
linkStyle default stroke:#ffffff,color:#ffffff
🔄 Container Architecture (click to expand)
graph TB
subgraph Core Services
UI[Frontend UI<br/>React + TypeScript]
API[Backend API<br/>Go + GraphQL]
DB[(Vector Store<br/>PostgreSQL + pgvector)]
MQ[Task Queue<br/>Async Processing]
Agent[AI Agents<br/>Multi-Agent System]
end
subgraph Monitoring
Grafana[Grafana<br/>Dashboards]
VictoriaMetrics[VictoriaMetrics<br/>Time-series DB]
Jaeger[Jaeger<br/>Distributed Tracing]
Loki[Loki<br/>Log Aggregation]
OTEL[OpenTelemetry<br/>Data Collection]
end
subgraph Analytics
Langfuse[Langfuse<br/>LLM Analytics]
ClickHouse[ClickHouse<br/>Analytics DB]
Redis[Redis<br/>Cache + Rate Limiter]
MinIO[MinIO<br/>S3 Storage]
end
subgraph Security Tools
Scraper[Web Scraper<br/>Isolated Browser]
PenTest[Security Tools<br/>20+ Pro Tools<br/>Sandboxed Execution]
end
UI --> |HTTP/WS| API
API --> |SQL| DB
API --> |Events| MQ
MQ --> |Tasks| Agent
Agent --> |Commands| Tools
Agent --> |Queries| DB
API --> |Telemetry| OTEL
OTEL --> |Metrics| VictoriaMetrics
OTEL --> |Traces| Jaeger
OTEL --> |Logs| Loki
Grafana --> |Query| VictoriaMetrics
Grafana --> |Query| Jaeger
Grafana --> |Query| Loki
API --> |Analytics| Langfuse
Langfuse --> |Store| ClickHouse
Langfuse --> |Cache| Redis
Langfuse --> |Files| MinIO
classDef core fill:#f9f,stroke:#333,stroke-width:2px,color:#000
classDef monitoring fill:#bbf,stroke:#333,stroke-width:2px,color:#000
classDef analytics fill:#bfb,stroke:#333,stroke-width:2px,color:#000
classDef tools fill:#fbb,stroke:#333,stroke-width:2px,color:#000
class UI,API,DB,MQ,Agent core
class Grafana,VictoriaMetrics,Jaeger,Loki,OTEL monitoring
class Langfuse,ClickHouse,Redis,MinIO analytics
class Scraper,PenTest tools
📊 Entity Relationship (click to expand)
erDiagram
Flow ||--o{ Task : contains
Task ||--o{ SubTask : contains
SubTask ||--o{ Action : contains
Action ||--o{ Artifact : produces
Action ||--o{ Memory : stores
Flow {
string id PK
string name "Flow name"
string description "Flow description"
string status "active/completed/failed"
json parameters "Flow parameters"
timestamp created_at
timestamp updated_at
}
Task {
string id PK
string flow_id FK
string name "Task name"
string description "Task description"
string status "pending/running/done/failed"
json result "Task results"
timestamp created_at
timestamp updated_at
}
SubTask {
string id PK
string task_id FK
string name "Subtask name"
string description "Subtask description"
string status "queued/running/completed/failed"
string agent_type "researcher/developer/executor"
json context "Agent context"
timestamp created_at
timestamp updated_at
}
Action {
string id PK
string subtask_id FK
string type "command/search/analyze/etc"
string status "success/failure"
json parameters "Action parameters"
json result "Action results"
timestamp created_at
}
Artifact {
string id PK
string action_id FK
string type "file/report/log"
string path "Storage path"
json metadata "Additional info"
timestamp created_at
}
Memory {
string id PK
string action_id FK
string type "observation/conclusion"
vector embedding "Vector representation"
text content "Memory content"
timestamp created_at
}
🤖 Agent Interaction (click to expand)
sequenceDiagram
participant O as Orchestrator
participant R as Researcher
participant D as Developer
participant E as Executor
participant VS as Vector Store
participant KB as Knowledge Base
Note over O,KB: Flow Initialization
O->>VS: Query similar tasks
VS-->>O: Return experiences
O->>KB: Load relevant knowledge
KB-->>O: Return context
Note over O,R: Research Phase
O->>R: Analyze target
R->>VS: Search similar cases
VS-->>R: Return patterns
R->>KB: Query vulnerabilities
KB-->>R: Return known issues
R->>VS: Store findings
R-->>O: Research results
Note over O,D: Planning Phase
O->>D: Plan attack
D->>VS: Query exploits
VS-->>D: Return techniques
D->>KB: Load tools info
KB-->>D: Return capabilities
D-->>O: Attack plan
Note over O,E: Execution Phase
O->>E: Execute plan
E->>KB: Load tool guides
KB-->>E: Return procedures
E->>VS: Store results
E-->>O: Execution status
🧠 Memory System (click to expand)
graph TB
subgraph "Long-term Memory"
VS[(Vector Store<br/>Embeddings DB)]
KB[Knowledge Base<br/>Domain Expertise]
Tools[Tools Knowledge<br/>Usage Patterns]
end
subgraph "Working Memory"
Context[Current Context<br/>Task State]
Goals[Active Goals<br/>Objectives]
State[System State<br/>Resources]
end
subgraph "Episodic Memory"
Actions[Past Actions<br/>Commands History]
Results[Action Results<br/>Outcomes]
Patterns[Success Patterns<br/>Best Practices]
end
Context --> |Query| VS
VS --> |Retrieve| Context
Goals --> |Consult| KB
KB --> |Guide| Goals
State --> |Record| Actions
Actions --> |Learn| Patterns
Patterns --> |Store| VS
Tools --> |Inform| State
Results --> |Update| Tools
VS --> |Enhance| KB
KB --> |Index| VS
classDef ltm fill:#f9f,stroke:#333,stroke-width:2px,color:#000
classDef wm fill:#bbf,stroke:#333,stroke-width:2px,color:#000
classDef em fill:#bfb,stroke:#333,stroke-width:2px,color:#000
class VS,KB,Tools ltm
class Context,Goals,State wm
class Actions,Results,Patterns em
The architecture of PentAGI is designed to be modular, scalable, and secure. Here are the key components:
-
Core Services
- Frontend UI: React-based web interface with TypeScript for type safety
- Backend API: Go-based REST and GraphQL APIs for flexible integration
- Vector Store: PostgreSQL with pgvector for semantic search and memory storage
- Task Queue: Async task processing system for reliable operation
- AI Agent: Multi-agent system with specialized roles for efficient testing
-
Monitoring Stack
- OpenTelemetry: Unified observability data collection and correlation
- Grafana: Real-time visualization and alerting dashboards
- VictoriaMetrics: High-performance time-series metrics storage
- Jaeger: End-to-end distributed tracing for debugging
- Loki: Scalable log aggregation and analysis
-
Analytics Platform
- Langfuse: Advanced LLM observability and performance analytics
- ClickHouse: Column-oriented analytics data warehouse
- Redis: High-speed caching and rate limiting
- MinIO: S3-compatible object storage for artifacts
-
Security Tools
- Web Scraper: Isolated browser environment for safe web interaction
- Pentesting Tools: Comprehensive suite of 20+ professional security tools
- Sandboxed Execution: All operations run in isolated containers
-
Memory Systems
- Long-term Memory: Persistent storage of knowledge and experiences
- Working Memory: Active context and goals for current operations
- Episodic Memory: Historical actions and success patterns
- Knowledge Base: Structured domain expertise and tool capabilities
The system uses Docker containers for isolation and easy deployment, with separate networks for core services, monitoring, and analytics to ensure proper security boundaries. Each component is designed to scale horizontally and can be configured for high availability in production environments.
- Docker and Docker Compose
- Minimum 4GB RAM
- 10GB free disk space
- Internet access for downloading images and updates
- Create a working directory or clone the repository:
mkdir pentagi && cd pentagi
- Copy
.env.example
to.env
or download it:
curl -o .env https://raw.githubusercontent.com/vxcontrol/pentagi/master/.env.example
- Fill in the required API keys in
.env
file.
# Required: At least one of these LLM providers
OPEN_AI_KEY=your_openai_key
ANTHROPIC_API_KEY=your_anthropic_key
# Optional: Additional search capabilities
GOOGLE_API_KEY=your_google_key
GOOGLE_CX_KEY=your_google_cx
TAVILY_API_KEY=your_tavily_key
TRAVERSAAL_API_KEY=your_traversaal_key
PERPLEXITY_API_KEY=your_perplexity_key
PERPLEXITY_MODEL=sonar-pro
PERPLEXITY_CONTEXT_SIZE=medium
- Change all security related environment variables in
.env
file to improve security.
Security related environment variables
-
COOKIE_SIGNING_SALT
- Salt for cookie signing, change to random value -
PUBLIC_URL
- Public URL of your server (eg.https://pentagi.example.com
) -
SERVER_SSL_CRT
andSERVER_SSL_KEY
- Custom paths to your existing SSL certificate and key for HTTPS (these paths should be used in the docker-compose.yml file to mount as volumes)
-
SCRAPER_PUBLIC_URL
- Public URL for scraper if you want to use different scraper server for public URLs -
SCRAPER_PRIVATE_URL
- Private URL for scraper (local scraper server in docker-compose.yml file to access it to local URLs)
-
PENTAGI_POSTGRES_USER
andPENTAGI_POSTGRES_PASSWORD
- PostgreSQL credentials
- Remove all inline comments from
.env
file if you want to use it in VSCode or other IDEs as a envFile option:
perl -i -pe 's/\s+#.*$//' .env
- Run the PentAGI stack:
curl -O https://raw.githubusercontent.com/vxcontrol/pentagi/master/docker-compose.yml
docker compose up -d
Visit localhost:8443 to access PentAGI Web UI (default is [email protected]
/ admin
)
[!NOTE] If you caught an error about
pentagi-network
orobservability-network
orlangfuse-network
you need to rundocker-compose.yml
firstly to create these networks and after that rundocker-compose-langfuse.yml
anddocker-compose-observability.yml
to use Langfuse and Observability services.You have to set at least one Language Model provider (OpenAI or Anthropic) to use PentAGI. Additional API keys for search engines are optional but recommended for better results.
LLM_SERVER_*
environment variables are experimental feature and will be changed in the future. Right now you can use them to specify custom LLM server URL and one model for all agent types.
PROXY_URL
is a global proxy URL for all LLM providers and external search systems. You can use it for isolation from external networks.The
docker-compose.yml
file runs the PentAGI service as root user because it needs access to docker.sock for container management. If you're using TCP/IP network connection to Docker instead of socket file, you can remove root privileges and use the defaultpentagi
user for better security.
For advanced configuration options and detailed setup instructions, please visit our documentation.
Langfuse provides advanced capabilities for monitoring and analyzing AI agent operations.
- Configure Langfuse environment variables in existing
.env
file.
Langfuse valuable environment variables
-
LANGFUSE_POSTGRES_USER
andLANGFUSE_POSTGRES_PASSWORD
- Langfuse PostgreSQL credentials -
LANGFUSE_CLICKHOUSE_USER
andLANGFUSE_CLICKHOUSE_PASSWORD
- ClickHouse credentials -
LANGFUSE_REDIS_AUTH
- Redis password
-
LANGFUSE_SALT
- Salt for hashing in Langfuse Web UI -
LANGFUSE_ENCRYPTION_KEY
- Encryption key (32 bytes in hex) -
LANGFUSE_NEXTAUTH_SECRET
- Secret key for NextAuth
-
LANGFUSE_INIT_USER_EMAIL
- Admin email -
LANGFUSE_INIT_USER_PASSWORD
- Admin password -
LANGFUSE_INIT_USER_NAME
- Admin username
-
LANGFUSE_INIT_PROJECT_PUBLIC_KEY
- Project public key (used from PentAGI side too) -
LANGFUSE_INIT_PROJECT_SECRET_KEY
- Project secret key (used from PentAGI side too)
-
LANGFUSE_S3_ACCESS_KEY_ID
- S3 access key ID -
LANGFUSE_S3_SECRET_ACCESS_KEY
- S3 secret access key
- Enable integration with Langfuse for PentAGI service in
.env
file.
LANGFUSE_BASE_URL=http://langfuse-web:3000
LANGFUSE_PROJECT_ID= # default: value from ${LANGFUSE_INIT_PROJECT_ID}
LANGFUSE_PUBLIC_KEY= # default: value from ${LANGFUSE_INIT_PROJECT_PUBLIC_KEY}
LANGFUSE_SECRET_KEY= # default: value from ${LANGFUSE_INIT_PROJECT_SECRET_KEY}
- Run the Langfuse stack:
curl -O https://raw.githubusercontent.com/vxcontrol/pentagi/master/docker-compose-langfuse.yml
docker compose -f docker-compose.yml -f docker-compose-langfuse.yml up -d
Visit localhost:4000 to access Langfuse Web UI with credentials from .env
file:
-
LANGFUSE_INIT_USER_EMAIL
- Admin email -
LANGFUSE_INIT_USER_PASSWORD
- Admin password
For detailed system operation tracking, integration with monitoring tools is available.
- Enable integration with OpenTelemetry and all observability services for PentAGI in
.env
file.
OTEL_HOST=otelcol:8148
- Run the observability stack:
curl -O https://raw.githubusercontent.com/vxcontrol/pentagi/master/docker-compose-observability.yml
docker compose -f docker-compose.yml -f docker-compose-observability.yml up -d
Visit localhost:3000 to access Grafana Web UI.
[!NOTE] If you want to use Observability stack with Langfuse, you need to enable integration in
.env
file to setLANGFUSE_OTEL_EXPORTER_OTLP_ENDPOINT
tohttp://otelcol:4318
.And you need to run both stacks
docker compose -f docker-compose.yml -f docker-compose-langfuse.yml -f docker-compose-observability.yml up -d
to have all services running.Also you can register aliases for these commands in your shell to run it faster:
alias pentagi="docker compose -f docker-compose.yml -f docker-compose-langfuse.yml -f docker-compose-observability.yml" alias pentagi-up="docker compose -f docker-compose.yml -f docker-compose-langfuse.yml -f docker-compose-observability.yml up -d" alias pentagi-down="docker compose -f docker-compose.yml -f docker-compose-langfuse.yml -f docker-compose-observability.yml down"```
OAuth integration with GitHub and Google allows users to authenticate using their existing accounts on these platforms. This provides several benefits:
- Simplified login process without need to create separate credentials
- Enhanced security through trusted identity providers
- Access to user profile information from GitHub/Google accounts
- Seamless integration with existing development workflows
For using GitHub OAuth you need to create a new OAuth application in your GitHub account and set the GITHUB_CLIENT_ID
and GITHUB_CLIENT_SECRET
in .env
file.
For using Google OAuth you need to create a new OAuth application in your Google account and set the GOOGLE_CLIENT_ID
and GOOGLE_CLIENT_SECRET
in .env
file.
- golang
- nodejs
- docker
- postgres
- commitlint
Run once cd backend && go mod download
to install needed packages.
For generating swagger files have to run
swag init -g ../../pkg/server/router.go -o pkg/server/docs/ --parseDependency --parseInternal --parseDepth 2 -d cmd/pentagi
before installing swag
package via
go install github.com/swaggo/swag/cmd/[email protected]
For generating graphql resolver files have to run
go run github.com/99designs/gqlgen --config ./gqlgen/gqlgen.yml
after that you can see the generated files in pkg/graph
folder.
For generating ORM methods (database package) from sqlc configuration
docker run --rm -v $(pwd):/src -w /src --network pentagi-network -e DATABASE_URL="{URL}" sqlc/sqlc generate -f sqlc/sqlc.yml
For generating Langfuse SDK from OpenAPI specification
fern generate --local
and to install fern-cli
npm install -g fern-api
For running tests cd backend && go test -v ./...
Run once cd frontend && npm install
to install needed packages.
For generating graphql files have to run npm run graphql:generate
which using graphql-codegen.ts
file.
Be sure that you have graphql-codegen
installed globally:
npm install -g graphql-codegen
After that you can run:
-
npm run prettier
to check if your code is formatted correctly -
npm run prettier:fix
to fix it -
npm run lint
to check if your code is linted correctly -
npm run lint:fix
to fix it
For generating SSL certificates you need to run npm run ssl:generate
which using generate-ssl.ts
file or it will be generated automatically when you run npm run dev
.
Edit the configuration for backend
in .vscode/launch.json
file:
-
DATABASE_URL
- PostgreSQL database URL (eg.postgres://postgres:postgres@localhost:5432/pentagidb?sslmode=disable
) -
DOCKER_HOST
- Docker SDK API (eg. for macOSDOCKER_HOST=unix:///Users/<my-user>/Library/Containers/com.docker.docker/Data/docker.raw.sock
) more info
Optional:
-
SERVER_PORT
- Port to run the server (default:8443
) -
SERVER_USE_SSL
- Enable SSL for the server (default:false
)
Edit the configuration for frontend
in .vscode/launch.json
file:
-
VITE_API_URL
- Backend API URL. Omit the URL scheme (e.g.,localhost:8080
NOThttp://localhost:8080
) -
VITE_USE_HTTPS
- Enable SSL for the server (default:false
) -
VITE_PORT
- Port to run the server (default:8000
) -
VITE_HOST
- Host to run the server (default:0.0.0.0
)
Run the command(s) in backend
folder:
- Use
.env
file to set environment variables like asource .env
- Run
go run cmd/pentagi/main.go
to start the server
[!NOTE] The first run can take a while as dependencies and docker images need to be downloaded to setup the backend environment.
Run the command(s) in frontend
folder:
- Run
npm install
to install the dependencies - Run
npm run dev
to run the web app - Run
npm run build
to build the web app
Open your browser and visit the web app URL.
PentAGI includes a powerful utility called ctester
for testing and validating LLM agent capabilities. This tool helps ensure your LLM provider configurations work correctly with different agent types, allowing you to optimize model selection for each specific agent role.
The utility features parallel testing of multiple agents, detailed reporting, and flexible configuration options.
- Parallel Testing: Tests multiple agents simultaneously for faster results
- Comprehensive Test Suite: Evaluates basic completion, JSON responses, function calling, and more
- Detailed Reporting: Generates markdown reports with success rates and performance metrics
- Flexible Configuration: Test specific agents or test groups as needed
If you've cloned the repository and have Go installed:
# Default configuration with .env file
cd backend
go run cmd/ctester/*.go -verbose
# Custom provider configuration
go run cmd/ctester/*.go -config ../examples/configs/openrouter.provider.yml -verbose
# Generate a report file
go run cmd/ctester/*.go -config ../examples/configs/deepinfra.provider.yml -report ../test-report.md
# Test specific agent types only
go run cmd/ctester/*.go -agents simple,simple_json,agent -verbose
# Test specific test groups only
go run cmd/ctester/*.go -tests "Simple Completion,System User Prompts" -verbose
If you prefer to use the pre-built Docker image without setting up a development environment:
# Using Docker to test with default environment
docker run --rm -v $(pwd)/.env:/opt/pentagi/.env vxcontrol/pentagi /opt/pentagi/bin/ctester -verbose
# Test with your custom provider configuration
docker run --rm \
-v $(pwd)/.env:/opt/pentagi/.env \
-v $(pwd)/my-config.yml:/opt/pentagi/config.yml \
vxcontrol/pentagi /opt/pentagi/bin/ctester -config /opt/pentagi/config.yml -verbose
# Generate a detailed report
docker run --rm \
-v $(pwd)/.env:/opt/pentagi/.env \
-v $(pwd):/opt/pentagi/output \
vxcontrol/pentagi /opt/pentagi/bin/ctester -report /opt/pentagi/output/report.md
The Docker image comes with pre-configured provider files for OpenRouter or DeepInfra or DeepSeek:
# Test with OpenRouter configuration
docker run --rm \
-v $(pwd)/.env:/opt/pentagi/.env \
vxcontrol/pentagi /opt/pentagi/bin/ctester -config /opt/pentagi/conf/openrouter.provider.yml
# Test with DeepInfra configuration
docker run --rm \
-v $(pwd)/.env:/opt/pentagi/.env \
vxcontrol/pentagi /opt/pentagi/bin/ctester -config /opt/pentagi/conf/deepinfra.provider.yml
# Test with DeepSeek configuration
docker run --rm \
-v $(pwd)/.env:/opt/pentagi/.env \
vxcontrol/pentagi /opt/pentagi/bin/ctester -config /opt/pentagi/conf/deepseek.provider.yml
To use these configurations, your .env
file only needs to contain:
LLM_SERVER_URL=https://openrouter.ai/api/v1 # or https://api.deepinfra.com/v1/openai or https://api.deepseek.com
LLM_SERVER_KEY=your_api_key
LLM_SERVER_MODEL= # Leave empty, as models are specified in the config
LLM_SERVER_CONFIG_PATH=/opt/pentagi/conf/openrouter.provider.yml # or deepinfra.provider.yml or deepseek.provider.yml
If you already have a running PentAGI container and want to test the current configuration:
# Run ctester in an existing container using current environment variables
docker exec -it pentagi /opt/pentagi/bin/ctester -verbose
# Generate a report file inside the container
docker exec -it pentagi /opt/pentagi/bin/ctester -report /opt/pentagi/data/agent-test-report.md
# Access the report from the host
docker cp pentagi:/opt/pentagi/data/agent-test-report.md ./
The utility accepts several options:
-
-env <path>
- Path to environment file (default:.env
) -
-config <path>
- Path to custom provider config (default: fromLLM_SERVER_CONFIG_PATH
env variable) -
-report <path>
- Path to write the report file (optional) -
-agents <list>
- Comma-separated list of agent types to test (default:all
) -
-tests <list>
- Comma-separated list of test groups to run (default:all
) -
-verbose
- Enable verbose output with detailed test results for each agent
Provider configuration defines which models to use for different agent types:
simple:
model: "provider/model-name"
temperature: 0.7
top_p: 0.95
n: 1
max_tokens: 4000
simple_json:
model: "provider/model-name"
temperature: 0.7
top_p: 1.0
n: 1
max_tokens: 4000
json: true
# ... other agent types ...
- Create a baseline: Run tests with default configuration
- Experiment: Try different models for each agent type
- Compare results: Look for the best success rate and performance
- Deploy optimal configuration: Use in production with your optimized setup
This tool helps ensure your AI agents are using the most effective models for their specific tasks, improving reliability while optimizing costs.
PentAGI includes a versatile utility called ftester
for debugging, testing, and developing specific functions and AI agent behaviors. While ctester
focuses on testing LLM model capabilities, ftester
allows you to directly invoke individual system functions and AI agent components with precise control over execution context.
- Direct Function Access: Test individual functions without running the entire system
- Mock Mode: Test functions without a live PentAGI deployment using built-in mocks
- Interactive Input: Fill function arguments interactively for exploratory testing
- Detailed Output: Color-coded terminal output with formatted responses and errors
- Context-Aware Testing: Debug AI agents within the context of specific flows, tasks, and subtasks
- Observability Integration: All function calls are logged to Langfuse and Observability stack
Run ftester with specific function and arguments directly from the command line:
# Basic usage with mock mode
cd backend
go run cmd/ftester/main.go [function_name] -[arg1] [value1] -[arg2] [value2]
# Example: Test terminal command in mock mode
go run cmd/ftester/main.go terminal -command "ls -la" -message "List files"
# Using a real flow context
go run cmd/ftester/main.go -flow 123 terminal -command "whoami" -message "Check user"
# Testing AI agent in specific task/subtask context
go run cmd/ftester/main.go -flow 123 -task 456 -subtask 789 pentester -message "Find vulnerabilities"
Run ftester without arguments for a guided interactive experience:
# Start interactive mode
go run cmd/ftester/main.go [function_name]
# For example, to interactively fill browser tool arguments
go run cmd/ftester/main.go browser
Available Functions (click to expand)
- terminal: Execute commands in a container and return the output
- file: Perform file operations (read, write, list) in a container
- browser: Access websites and capture screenshots
- google: Search the web using Google Custom Search
- duckduckgo: Search the web using DuckDuckGo
- tavily: Search using Tavily AI search engine
- traversaal: Search using Traversaal AI search engine
- perplexity: Search using Perplexity AI
- search_in_memory: Search for information in vector database
- search_guide: Find guidance documents in vector database
- search_answer: Find answers to questions in vector database
- search_code: Find code examples in vector database
- advice: Get expert advice from an AI agent
- coder: Request code generation or modification
- maintenance: Run system maintenance tasks
- memorist: Store and organize information in vector database
- pentester: Perform security tests and vulnerability analysis
- search: Complex search across multiple sources
- describe: Show information about flows, tasks, and subtasks
Debugging Flow Context (click to expand)
The describe
function provides detailed information about tasks and subtasks within a flow. This is particularly useful for diagnosing issues when PentAGI encounters problems or gets stuck.
# List all flows in the system
go run cmd/ftester/main.go describe
# Show all tasks and subtasks for a specific flow
go run cmd/ftester/main.go -flow 123 describe
# Show detailed information for a specific task
go run cmd/ftester/main.go -flow 123 -task 456 describe
# Show detailed information for a specific subtask
go run cmd/ftester/main.go -flow 123 -task 456 -subtask 789 describe
# Show verbose output with full descriptions and results
go run cmd/ftester/main.go -flow 123 describe -verbose
This function allows you to identify the exact point where a flow might be stuck and resume processing by directly invoking the appropriate agent function.
Function Help and Discovery (click to expand)
Each function has a help mode that shows available parameters:
# Get help for a specific function
go run cmd/ftester/main.go [function_name] -help
# Examples:
go run cmd/ftester/main.go terminal -help
go run cmd/ftester/main.go browser -help
go run cmd/ftester/main.go describe -help
You can also run ftester without arguments to see a list of all available functions:
go run cmd/ftester/main.go
Output Format (click to expand)
The ftester
utility uses color-coded output to make interpretation easier:
- Blue headers: Section titles and key names
- Cyan [INFO]: General information messages
- Green [SUCCESS]: Successful operations
- Red [ERROR]: Error messages
- Yellow [WARNING]: Warning messages
- Yellow [MOCK]: Indicates mock mode operation
- Magenta values: Function arguments and results
JSON and Markdown responses are automatically formatted for readability.
Advanced Usage Scenarios (click to expand)
When PentAGI gets stuck in a flow:
- Pause the flow through the UI
- Use
describe
to identify the current task and subtask - Directly invoke the agent function with the same task/subtask IDs
- Examine the detailed output to identify the issue
- Resume the flow or manually intervene as needed
Verify that API keys and external services are configured correctly:
# Test Google search API configuration
go run cmd/ftester/main.go google -query "pentesting tools"
# Test browser access to external websites
go run cmd/ftester/main.go browser -url "https://example.com"
When developing new prompt templates or agent behaviors:
- Create a test flow in the UI
- Use ftester to directly invoke the agent with different prompts
- Observe responses and adjust prompts accordingly
- Check Langfuse for detailed traces of all function calls
Ensure containers are properly configured:
go run cmd/ftester/main.go -flow 123 terminal -command "env | grep -i proxy" -message "Check proxy settings"
Docker Container Usage (click to expand)
If you have PentAGI running in Docker, you can use ftester from within the container:
# Run ftester inside the running PentAGI container
docker exec -it pentagi /opt/pentagi/bin/ftester [arguments]
# Examples:
docker exec -it pentagi /opt/pentagi/bin/ftester -flow 123 describe
docker exec -it pentagi /opt/pentagi/bin/ftester -flow 123 terminal -command "ps aux" -message "List processes"
This is particularly useful for production deployments where you don't have a local development environment.
Integration with Observability Tools (click to expand)
All function calls made through ftester are logged to:
- Langfuse: Captures the entire AI agent interaction chain, including prompts, responses, and function calls
- OpenTelemetry: Records metrics, traces, and logs for system performance analysis
- Terminal Output: Provides immediate feedback on function execution
To access detailed logs:
- Check Langfuse UI for AI agent traces (typically at
http://localhost:4000
) - Use Grafana dashboards for system metrics (typically at
http://localhost:3000
) - Examine terminal output for immediate function results and errors
The main utility accepts several options:
-
-env <path>
- Path to environment file (optional, default:.env
) -
-provider <type>
- Provider type to use (default:custom
, options:openai
,anthropic
,custom
) -
-flow <id>
- Flow ID for testing (0 means using mocks, default:0
) -
-task <id>
- Task ID for agent context (optional) -
-subtask <id>
- Subtask ID for agent context (optional)
Function-specific arguments are passed after the function name using -name value
format.
docker build -t local/pentagi:latest .
[!NOTE] You can use
docker buildx
to build the image for different platforms like adocker buildx build --platform linux/amd64 -t local/pentagi:latest .
You need to change image name in docker-compose.yml file to
local/pentagi:latest
and rundocker compose up -d
to start the server or usebuild
key option in docker-compose.yml file.
This project is made possible thanks to the following research and developments:
Copyright (c) PentAGI Development Team. MIT License
For Tasks:
Click tags to check more tools for each tasksFor Jobs:
Alternative AI tools for pentagi
Similar Open Source Tools
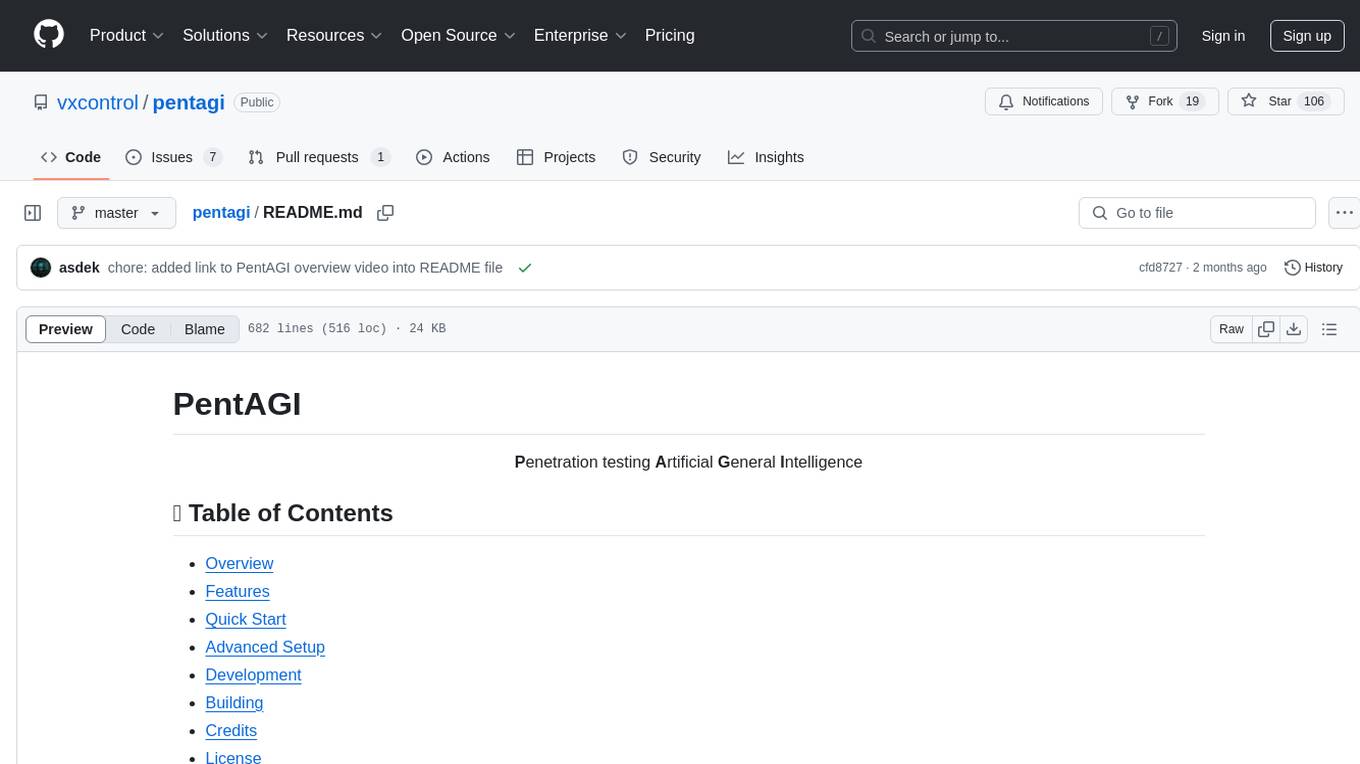
pentagi
PentAGI is an innovative tool for automated security testing that leverages cutting-edge artificial intelligence technologies. It is designed for information security professionals, researchers, and enthusiasts who need a powerful and flexible solution for conducting penetration tests. The tool provides secure and isolated operations in a sandboxed Docker environment, fully autonomous AI-powered agent for penetration testing steps, a suite of 20+ professional security tools, smart memory system for storing research results, web intelligence for gathering information, integration with external search systems, team delegation system, comprehensive monitoring and reporting, modern interface, API integration, persistent storage, scalable architecture, self-hosted solution, flexible authentication, and quick deployment through Docker Compose.
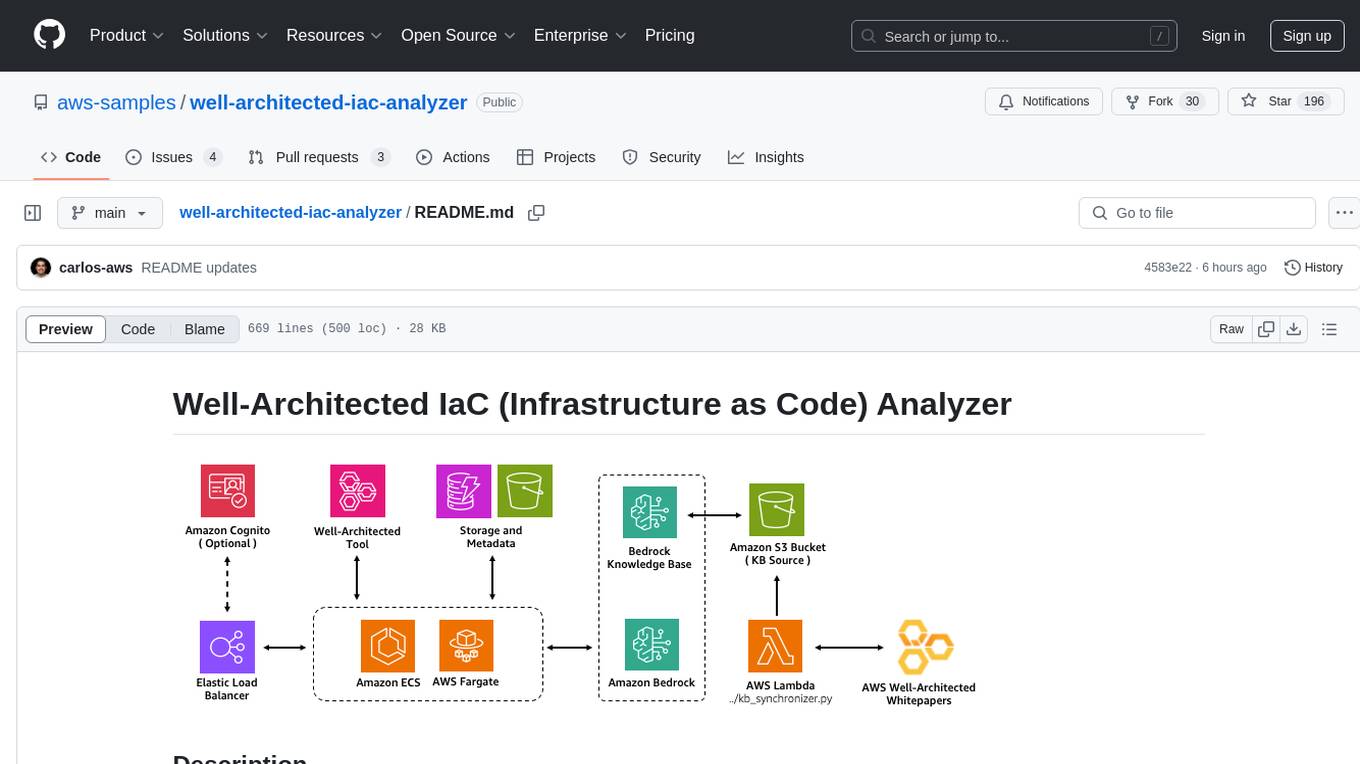
well-architected-iac-analyzer
Well-Architected Infrastructure as Code (IaC) Analyzer is a project demonstrating how generative AI can evaluate infrastructure code for alignment with best practices. It features a modern web application allowing users to upload IaC documents, complete IaC projects, or architecture diagrams for assessment. The tool provides insights into infrastructure code alignment with AWS best practices, offers suggestions for improving cloud architecture designs, and can generate IaC templates from architecture diagrams. Users can analyze CloudFormation, Terraform, or AWS CDK templates, architecture diagrams in PNG or JPEG format, and complete IaC projects with supporting documents. Real-time analysis against Well-Architected best practices, integration with AWS Well-Architected Tool, and export of analysis results and recommendations are included.
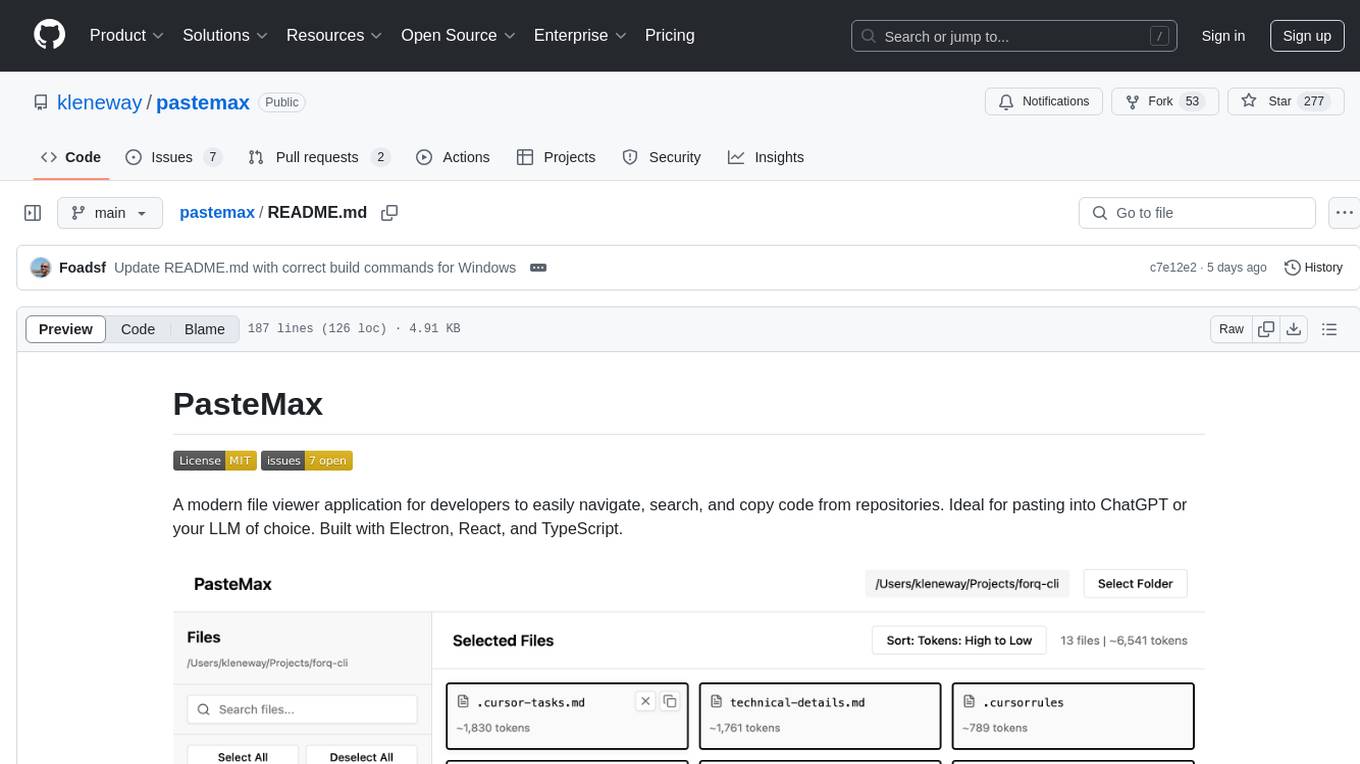
pastemax
PasteMax is a modern file viewer application designed for developers to easily navigate, search, and copy code from repositories. It provides features such as file tree navigation, token counting, search capabilities, selection management, sorting options, dark mode, binary file detection, and smart file exclusion. Built with Electron, React, and TypeScript, PasteMax is ideal for pasting code into ChatGPT or other language models. Users can download the application or build it from source, and customize file exclusions. Troubleshooting steps are provided for common issues, and contributions to the project are welcome under the MIT License.
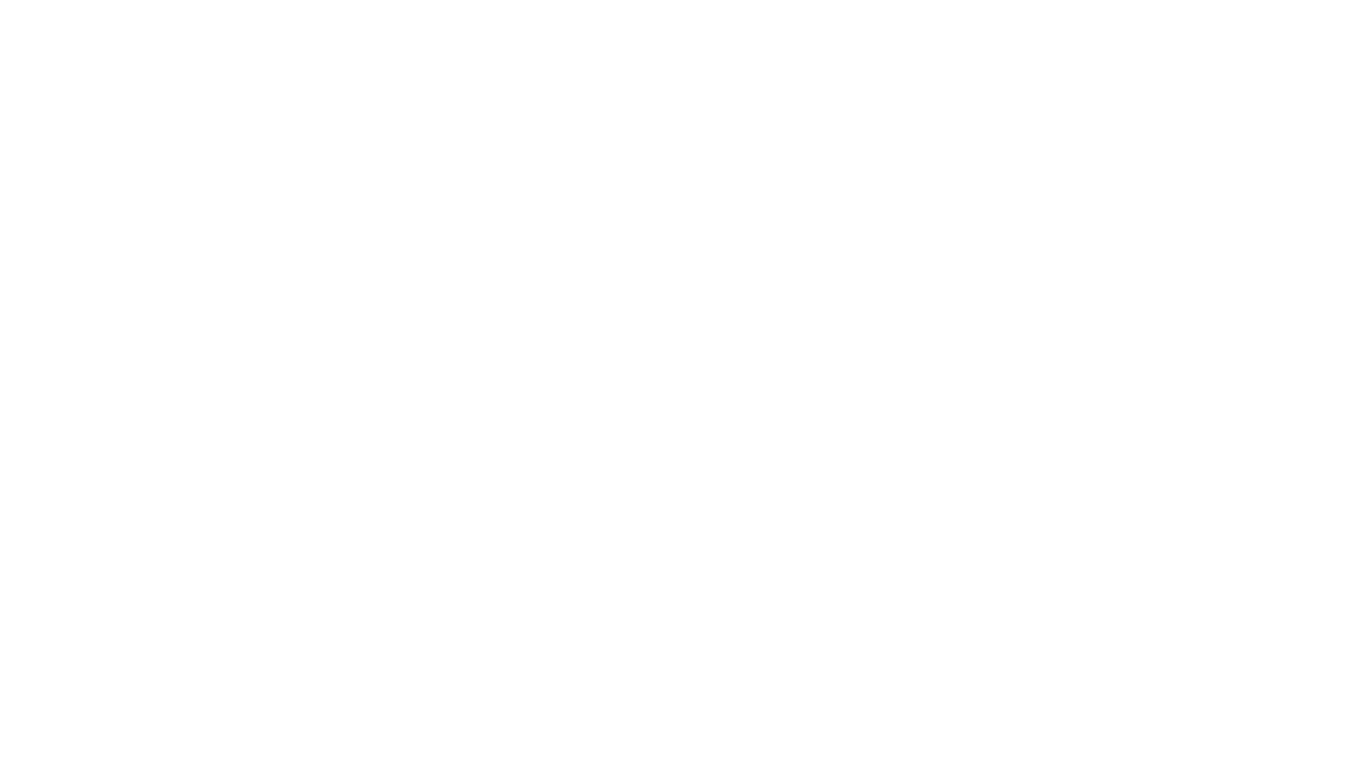
Zero
Zero is an open-source AI email solution that allows users to self-host their email app while integrating external services like Gmail. It aims to modernize and enhance emails through AI agents, offering features like open-source transparency, AI-driven enhancements, data privacy, self-hosting freedom, unified inbox, customizable UI, and developer-friendly extensibility. Built with modern technologies, Zero provides a reliable tech stack including Next.js, React, TypeScript, TailwindCSS, Node.js, Drizzle ORM, and PostgreSQL. Users can set up Zero using standard setup or Dev Container setup for VS Code users, with detailed environment setup instructions for Better Auth, Google OAuth, and optional GitHub OAuth. Database setup involves starting a local PostgreSQL instance, setting up database connection, and executing database commands for dependencies, tables, migrations, and content viewing.
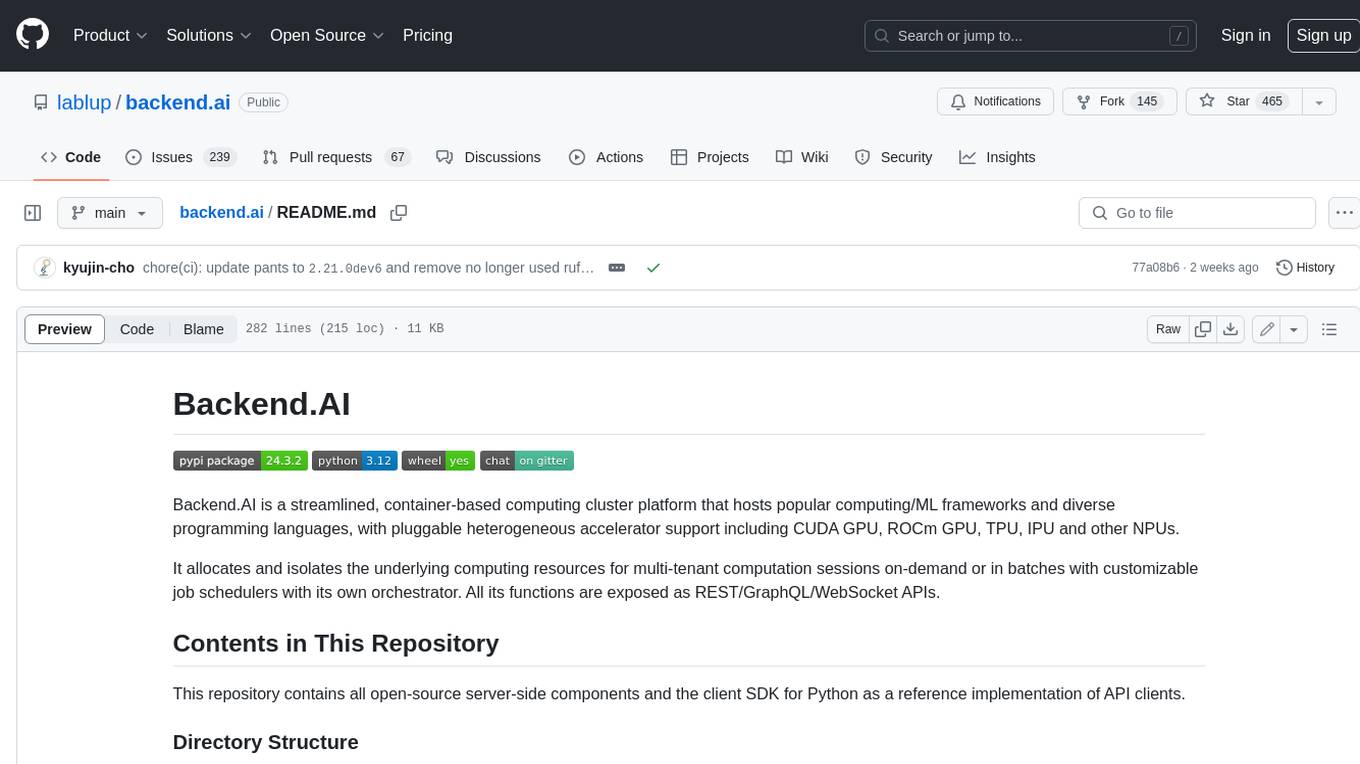
backend.ai
Backend.AI is a streamlined, container-based computing cluster platform that hosts popular computing/ML frameworks and diverse programming languages, with pluggable heterogeneous accelerator support including CUDA GPU, ROCm GPU, TPU, IPU and other NPUs. It allocates and isolates the underlying computing resources for multi-tenant computation sessions on-demand or in batches with customizable job schedulers with its own orchestrator. All its functions are exposed as REST/GraphQL/WebSocket APIs.
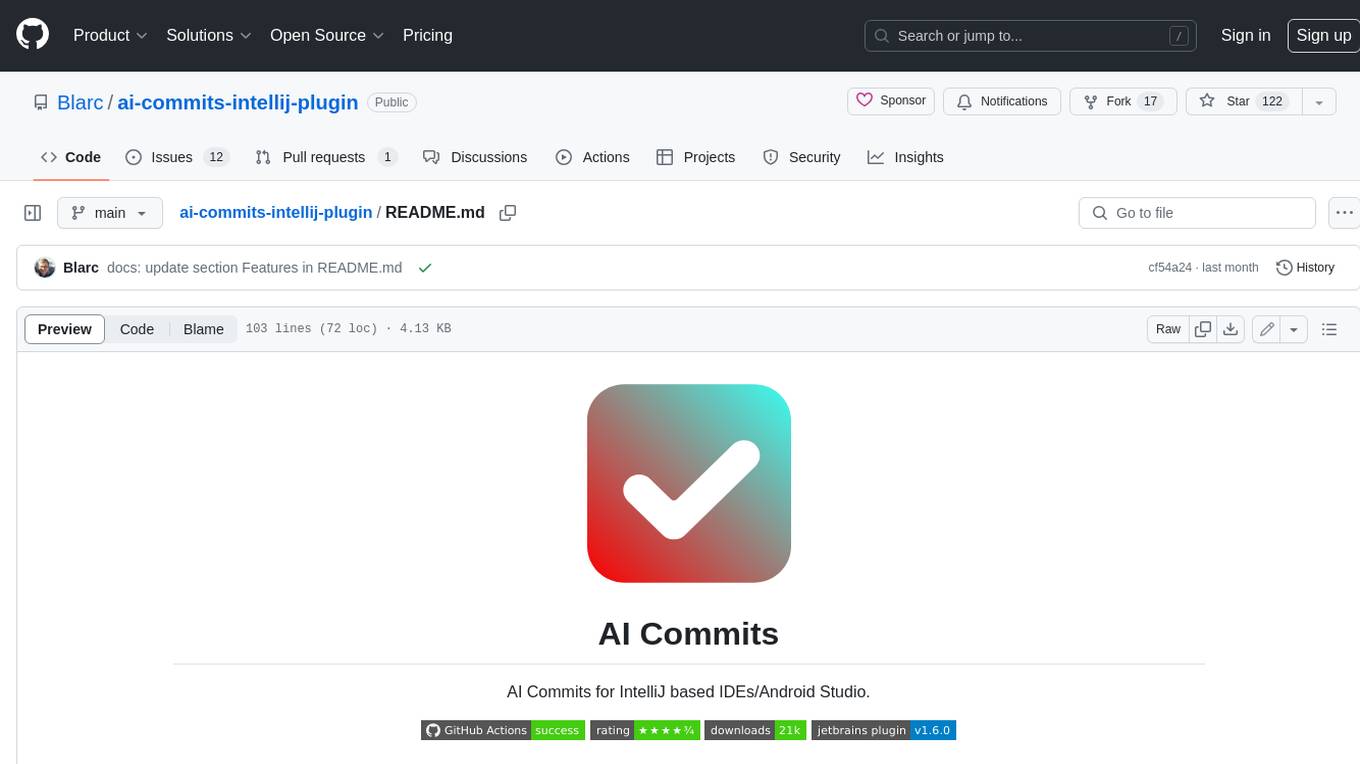
ai-commits-intellij-plugin
AI Commits is a plugin for IntelliJ-based IDEs and Android Studio that generates commit messages using git diff and OpenAI. It offers features such as generating commit messages from diff using OpenAI API, computing diff only from selected files and lines in the commit dialog, creating custom prompts for commit message generation, using predefined variables and hints to customize prompts, choosing any of the models available in OpenAI API, setting OpenAI network proxy, and setting custom OpenAI compatible API endpoint.
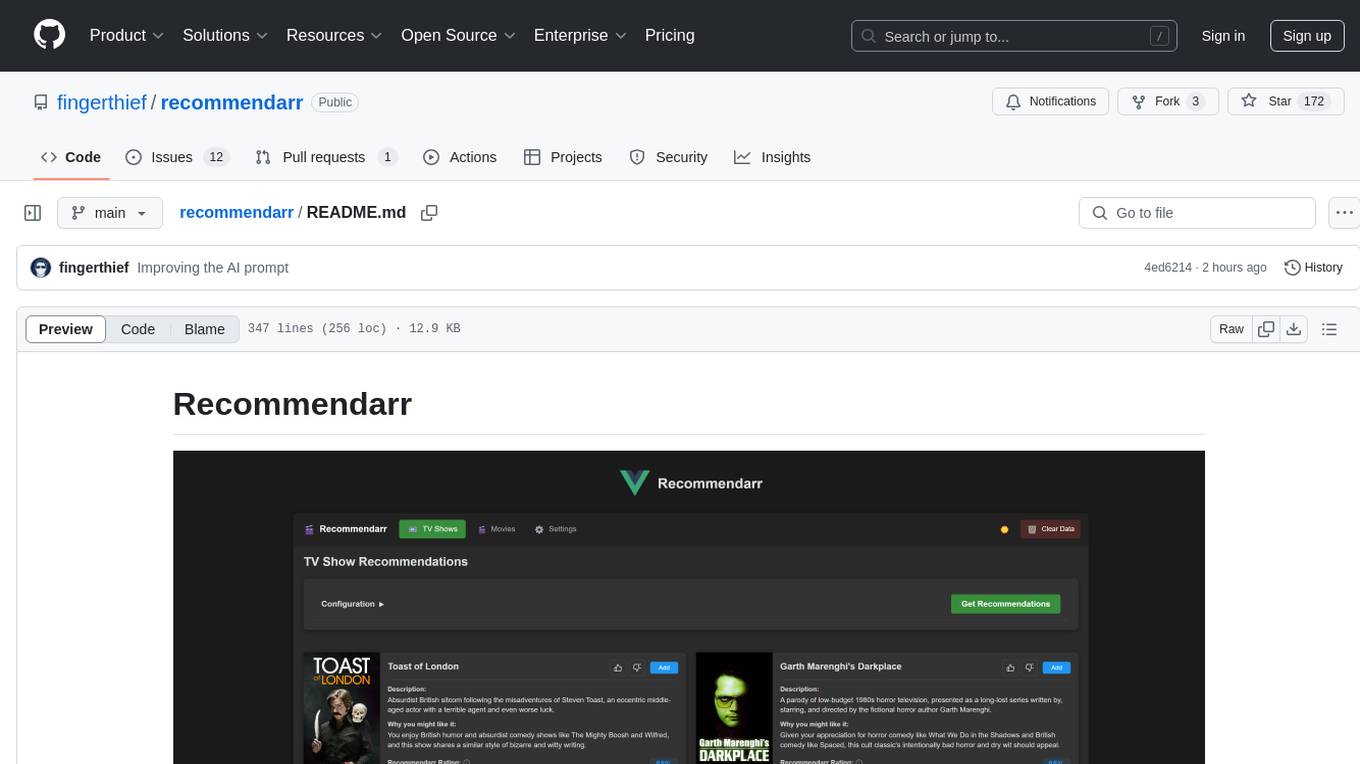
recommendarr
Recommendarr is a tool that generates personalized TV show and movie recommendations based on your Sonarr, Radarr, Plex, and Jellyfin libraries using AI. It offers AI-powered recommendations, media server integration, flexible AI support, watch history analysis, customization options, and dark/light mode toggle. Users can connect their media libraries and watch history services, configure AI service settings, and get personalized recommendations based on genre, language, and mood/vibe preferences. The tool works with any OpenAI-compatible API and offers various recommended models for different cost options and performance levels. It provides personalized suggestions, detailed information, filter options, watch history analysis, and one-click adding of recommended content to Sonarr/Radarr.
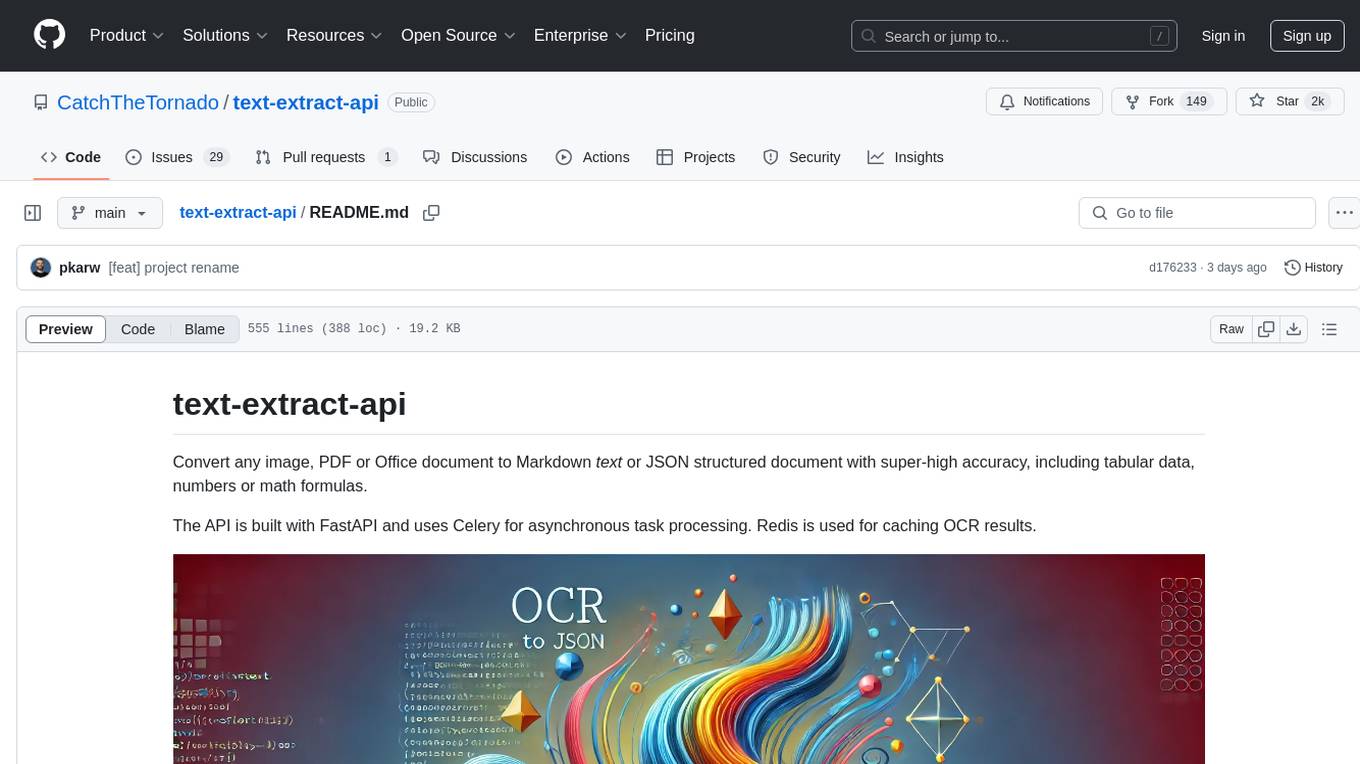
text-extract-api
The text-extract-api is a powerful tool that allows users to convert images, PDFs, or Office documents to Markdown text or JSON structured documents with high accuracy. It is built using FastAPI and utilizes Celery for asynchronous task processing, with Redis for caching OCR results. The tool provides features such as PDF/Office to Markdown and JSON conversion, improving OCR results with LLama, removing Personally Identifiable Information from documents, distributed queue processing, caching using Redis, switchable storage strategies, and a CLI tool for task management. Users can run the tool locally or on cloud services, with support for GPU processing. The tool also offers an online demo for testing purposes.
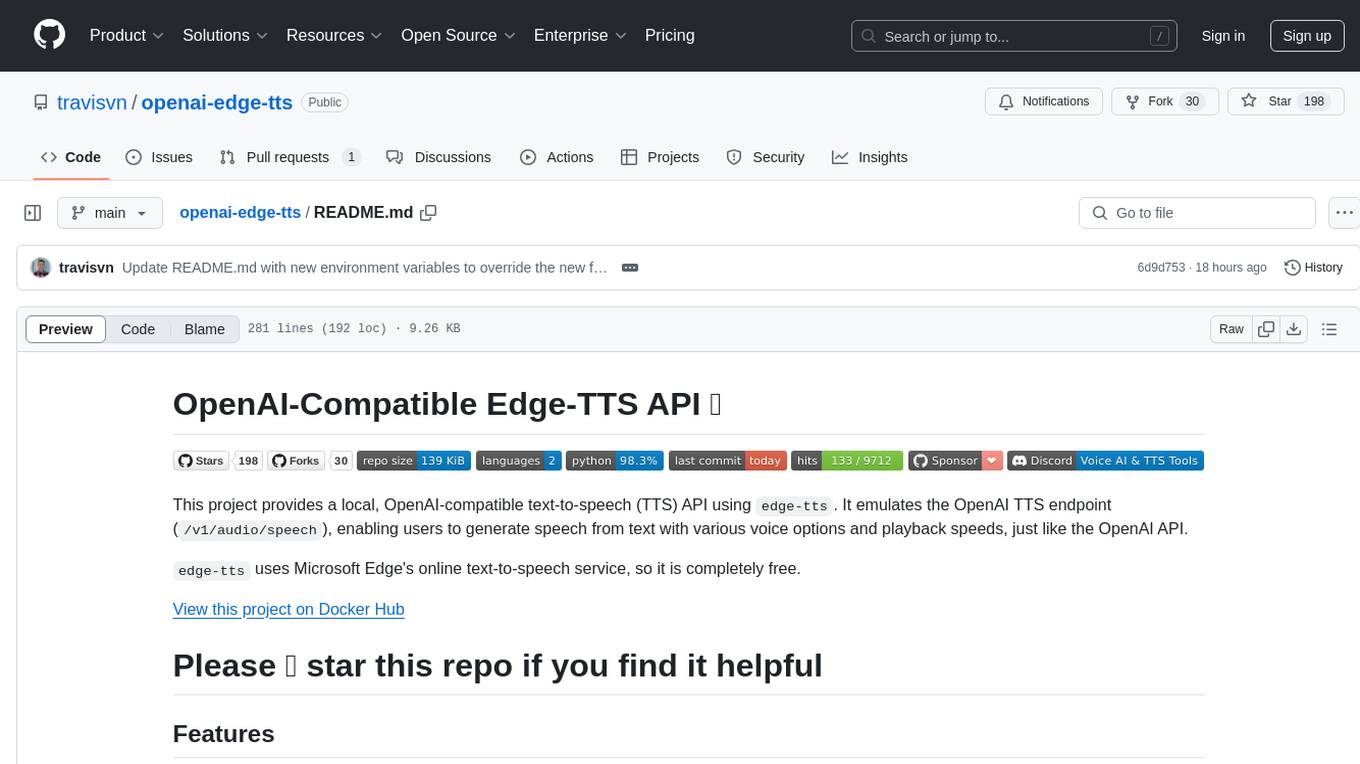
openai-edge-tts
This project provides a local, OpenAI-compatible text-to-speech (TTS) API using `edge-tts`. It emulates the OpenAI TTS endpoint (`/v1/audio/speech`), enabling users to generate speech from text with various voice options and playback speeds, just like the OpenAI API. `edge-tts` uses Microsoft Edge's online text-to-speech service, making it completely free. The project supports multiple audio formats, adjustable playback speed, and voice selection options, providing a flexible and customizable TTS solution for users.
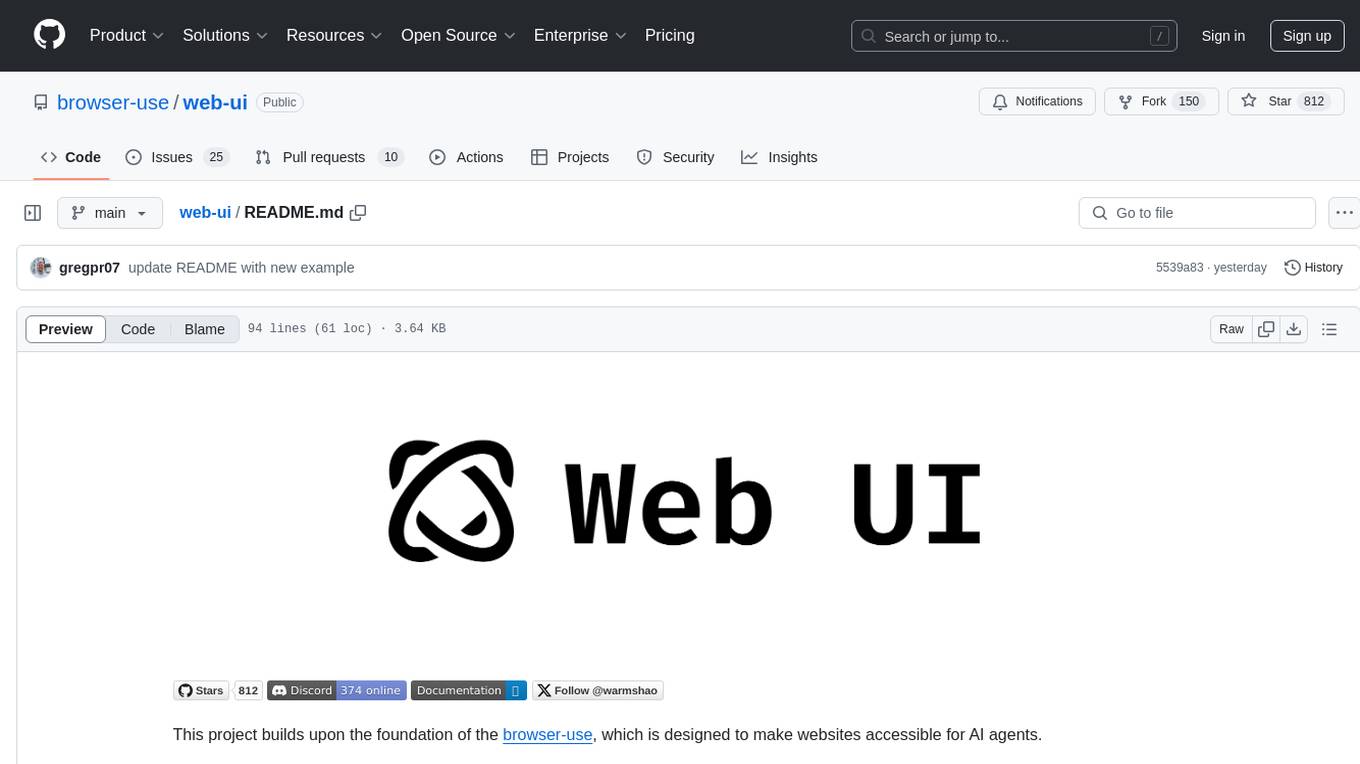
web-ui
WebUI is a user-friendly tool built on Gradio that enhances website accessibility for AI agents. It supports various Large Language Models (LLMs) and allows custom browser integration for seamless interaction. The tool eliminates the need for re-login and authentication challenges, offering high-definition screen recording capabilities.
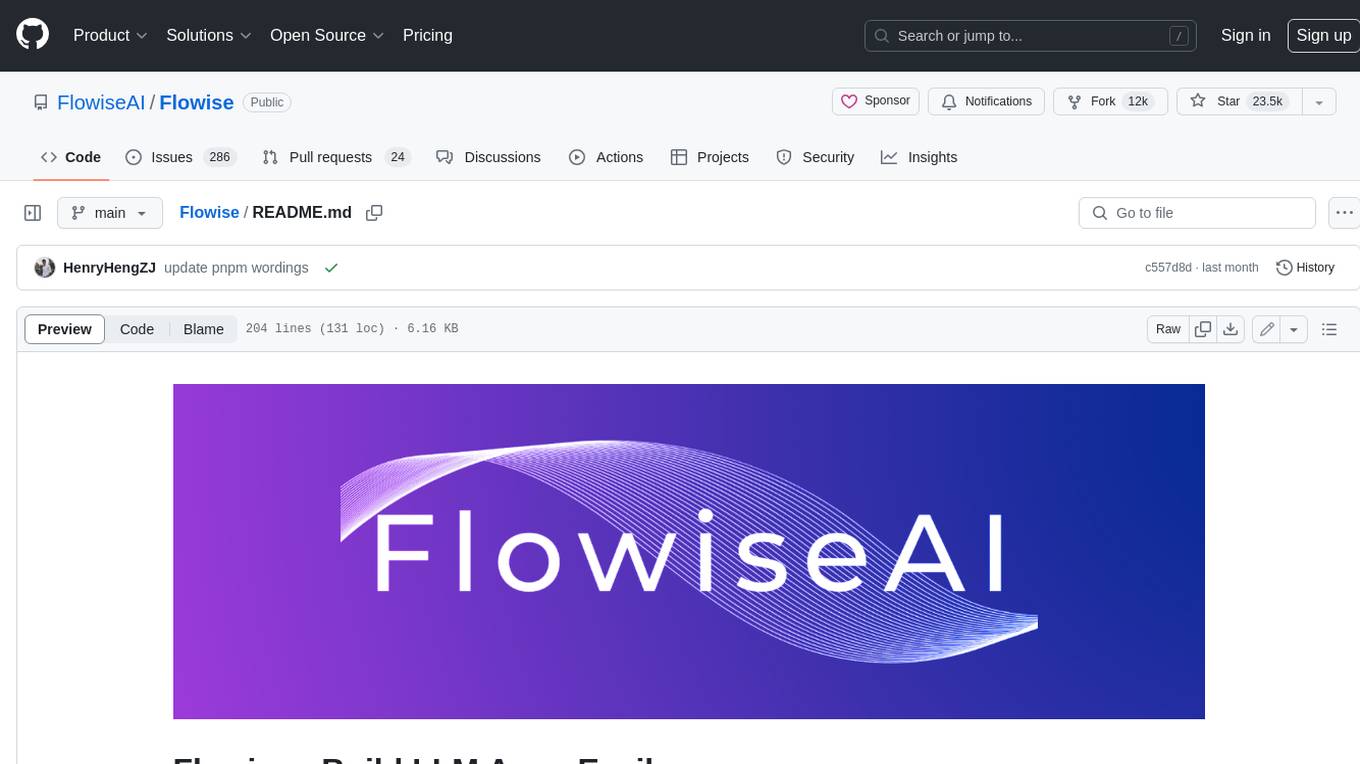
Flowise
Flowise is a tool that allows users to build customized LLM flows with a drag-and-drop UI. It is open-source and self-hostable, and it supports various deployments, including AWS, Azure, Digital Ocean, GCP, Railway, Render, HuggingFace Spaces, Elestio, Sealos, and RepoCloud. Flowise has three different modules in a single mono repository: server, ui, and components. The server module is a Node backend that serves API logics, the ui module is a React frontend, and the components module contains third-party node integrations. Flowise supports different environment variables to configure your instance, and you can specify these variables in the .env file inside the packages/server folder.
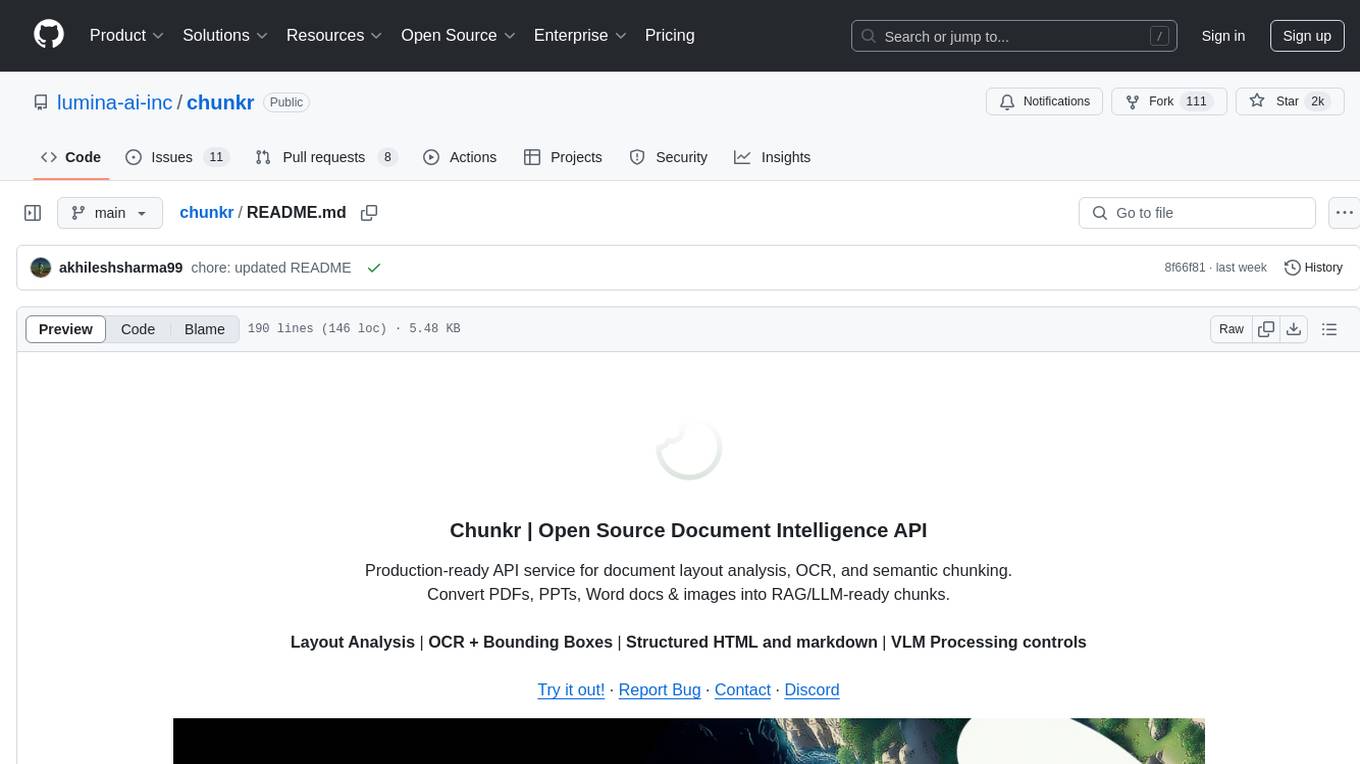
chunkr
Chunkr is an open-source document intelligence API that provides a production-ready service for document layout analysis, OCR, and semantic chunking. It allows users to convert PDFs, PPTs, Word docs, and images into RAG/LLM-ready chunks. The API offers features such as layout analysis, OCR with bounding boxes, structured HTML and markdown output, and VLM processing controls. Users can interact with Chunkr through a Python SDK, enabling them to upload documents, process them, and export results in various formats. The tool also supports self-hosted deployment options using Docker Compose or Kubernetes, with configurations for different AI models like OpenAI, Google AI Studio, and OpenRouter. Chunkr is dual-licensed under the GNU Affero General Public License v3.0 (AGPL-3.0) and a commercial license, providing flexibility for different usage scenarios.
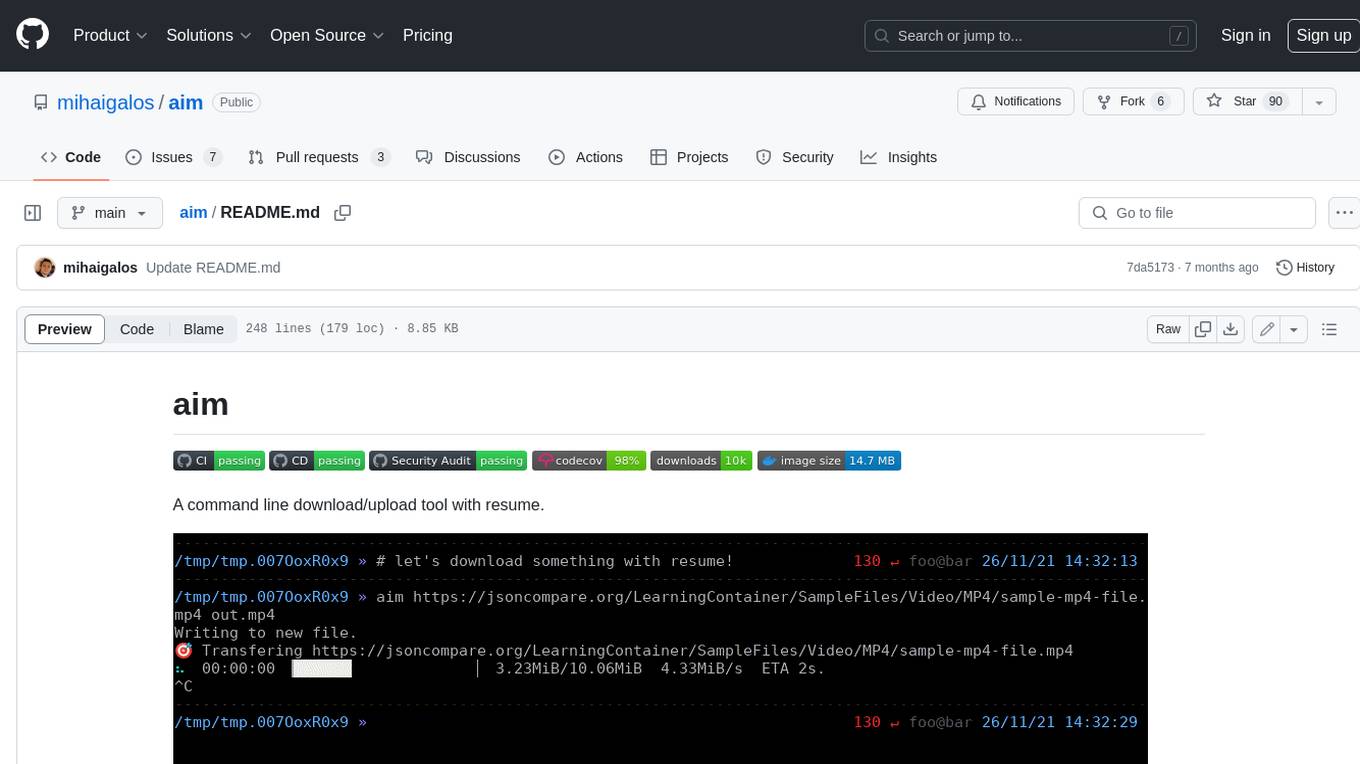
aim
Aim is a command-line tool for downloading and uploading files with resume support. It supports various protocols including HTTP, FTP, SFTP, SSH, and S3. Aim features an interactive mode for easy navigation and selection of files, as well as the ability to share folders over HTTP for easy access from other devices. Additionally, it offers customizable progress indicators and output formats, and can be integrated with other commands through piping. Aim can be installed via pre-built binaries or by compiling from source, and is also available as a Docker image for platform-independent usage.
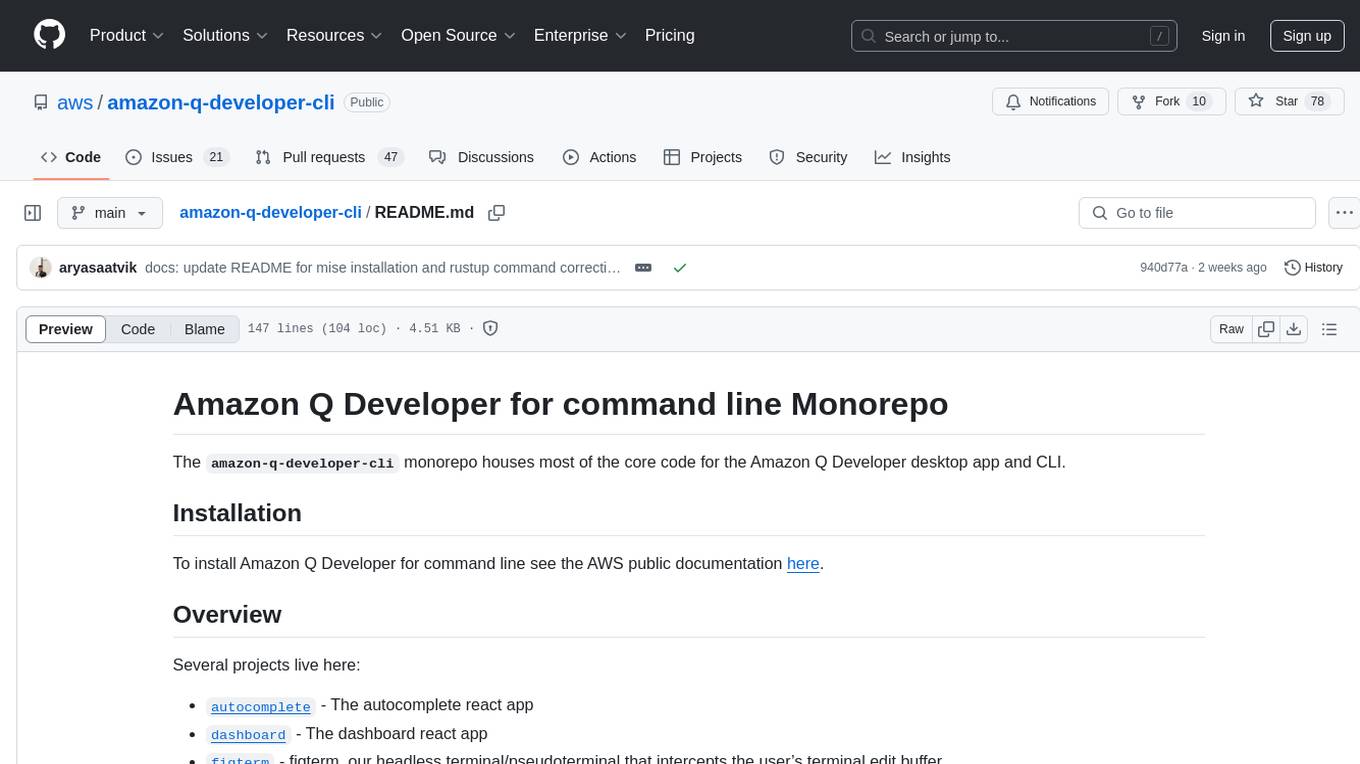
amazon-q-developer-cli
The `amazon-q-developer-cli` monorepo houses core code for the Amazon Q Developer desktop app and CLI. It includes projects like autocomplete, dashboard, figterm, q CLI, fig_desktop, fig_input_method, VSCode plugin, and JetBrains plugin. The repo also contains build scripts, internal rust crates, internal npm packages, protocol buffer message specification, and integration tests. The architecture involves different components communicating via IPC.
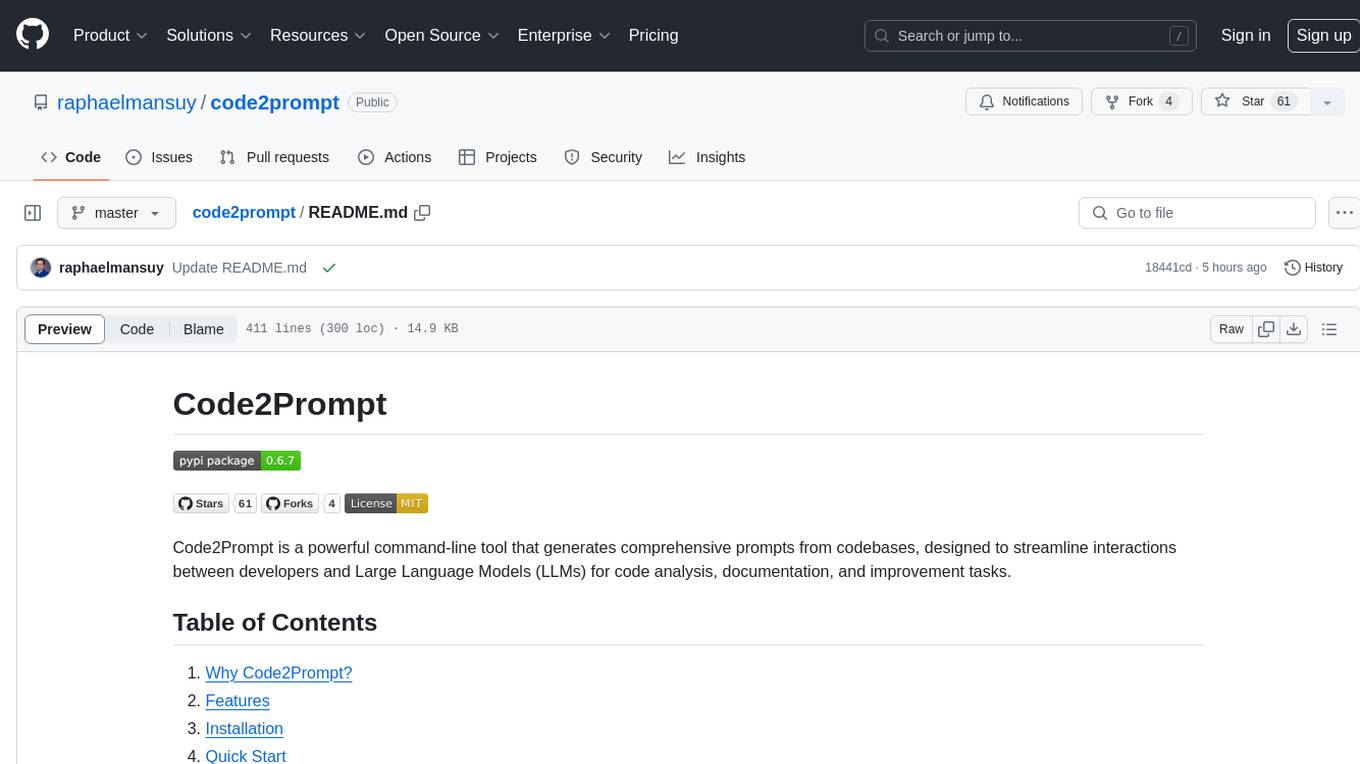
code2prompt
Code2Prompt is a powerful command-line tool that generates comprehensive prompts from codebases, designed to streamline interactions between developers and Large Language Models (LLMs) for code analysis, documentation, and improvement tasks. It bridges the gap between codebases and LLMs by converting projects into AI-friendly prompts, enabling users to leverage AI for various software development tasks. The tool offers features like holistic codebase representation, intelligent source tree generation, customizable prompt templates, smart token management, Gitignore integration, flexible file handling, clipboard-ready output, multiple output options, and enhanced code readability.
For similar tasks
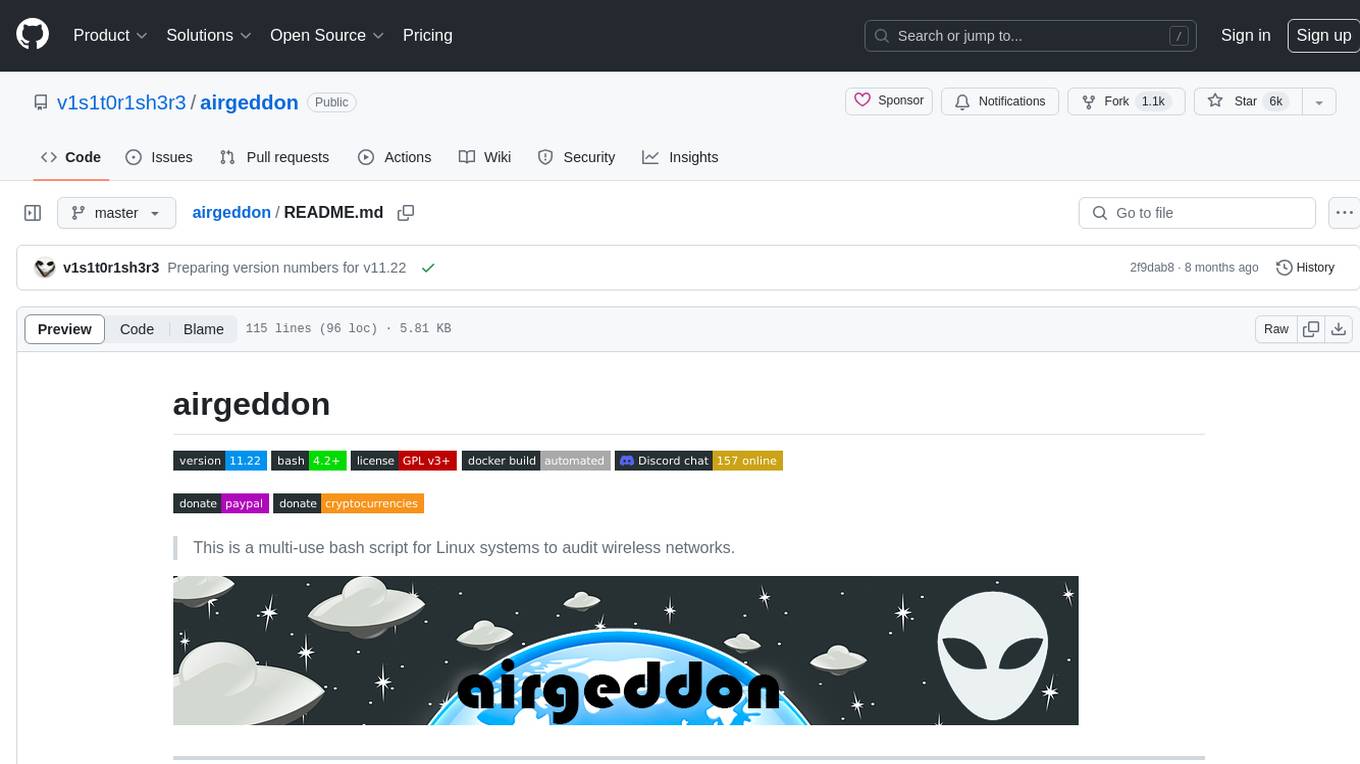
airgeddon
Airgeddon is a versatile bash script designed for Linux systems to conduct wireless network audits. It provides a comprehensive set of features and tools for auditing and securing wireless networks. The script is user-friendly and offers functionalities such as scanning, capturing handshakes, deauth attacks, and more. Airgeddon is regularly updated and supported, making it a valuable tool for both security professionals and enthusiasts.
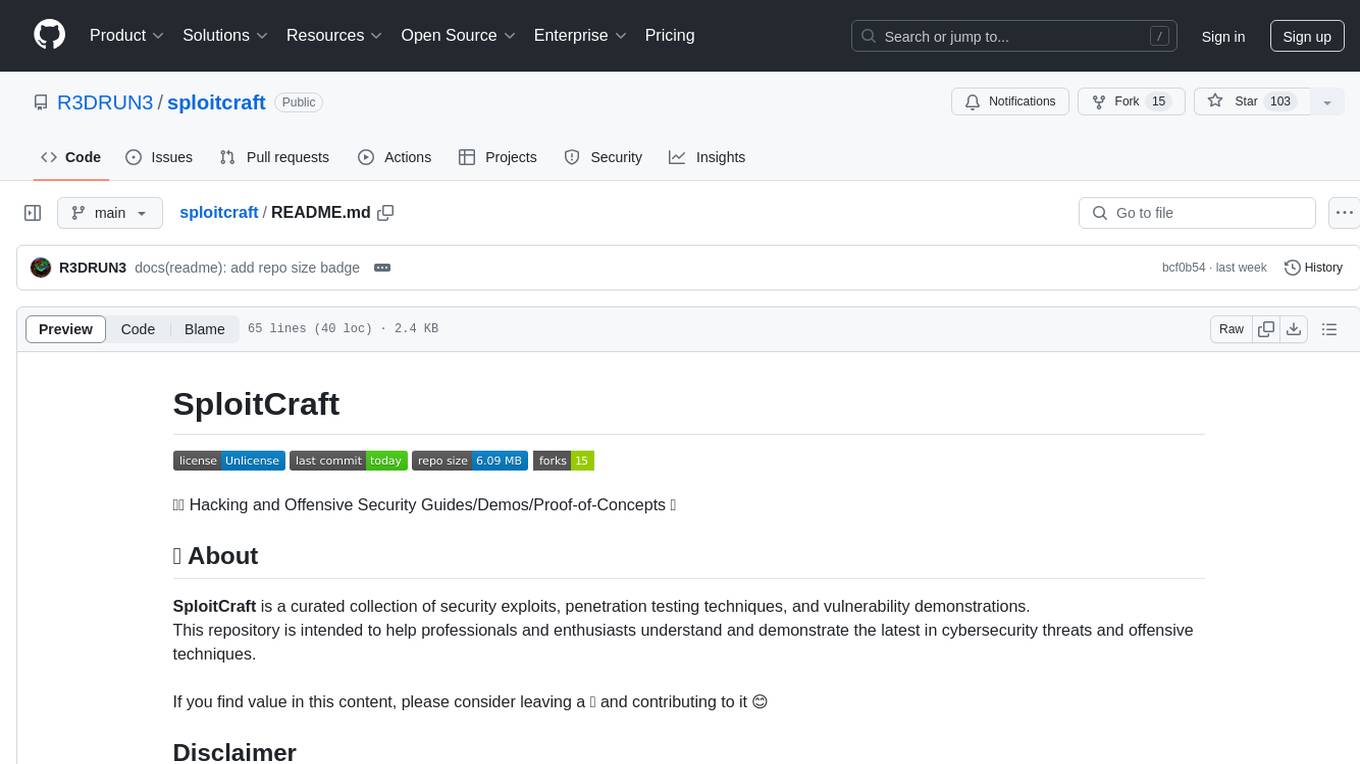
sploitcraft
SploitCraft is a curated collection of security exploits, penetration testing techniques, and vulnerability demonstrations intended to help professionals and enthusiasts understand and demonstrate the latest in cybersecurity threats and offensive techniques. The repository is organized into folders based on specific topics, each containing directories and detailed READMEs with step-by-step instructions. Contributions from the community are welcome, with a focus on adding new proof of concepts or expanding existing ones while adhering to the current structure and format of the repository.
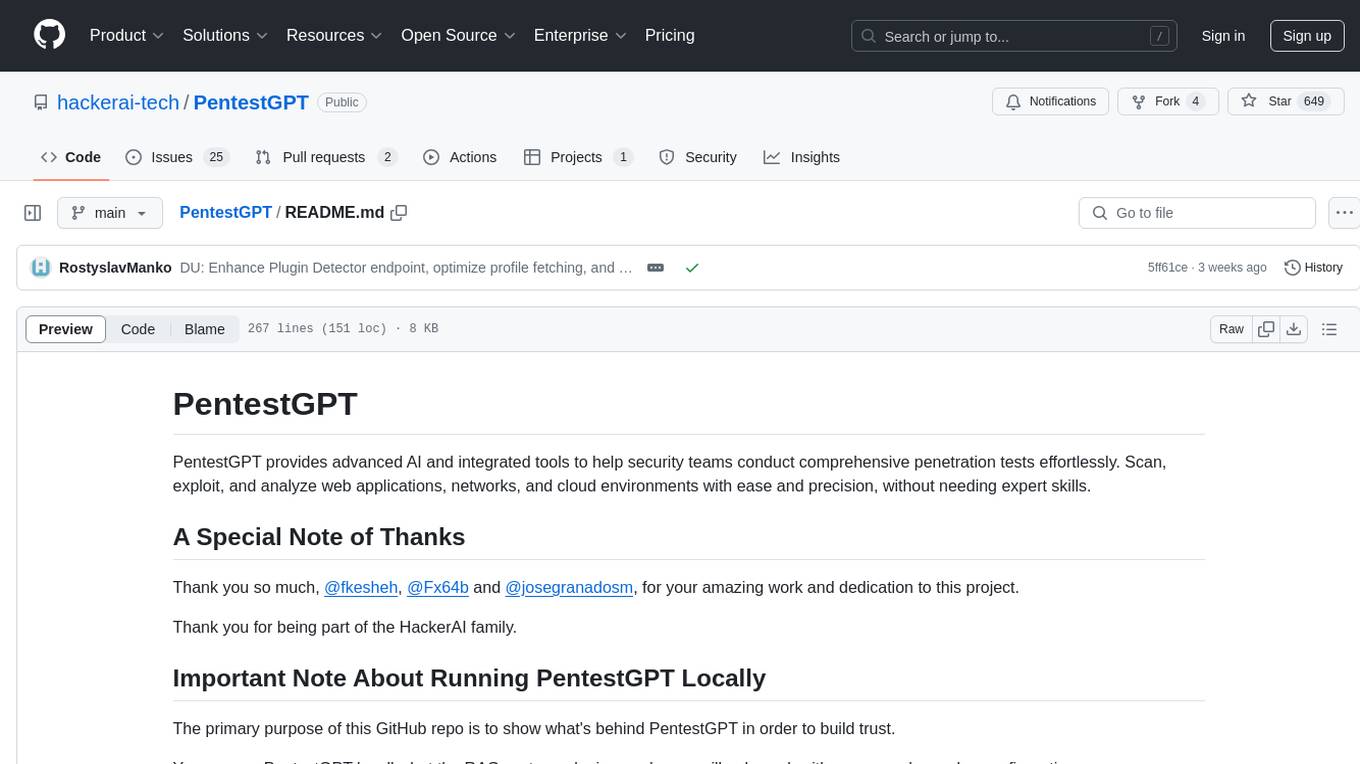
PentestGPT
PentestGPT provides advanced AI and integrated tools to help security teams conduct comprehensive penetration tests effortlessly. Scan, exploit, and analyze web applications, networks, and cloud environments with ease and precision, without needing expert skills. The tool utilizes Supabase for data storage and management, and Vercel for hosting the frontend. It offers a local quickstart guide for running the tool locally and a hosted quickstart guide for deploying it in the cloud. PentestGPT aims to simplify the penetration testing process for security professionals and enthusiasts alike.
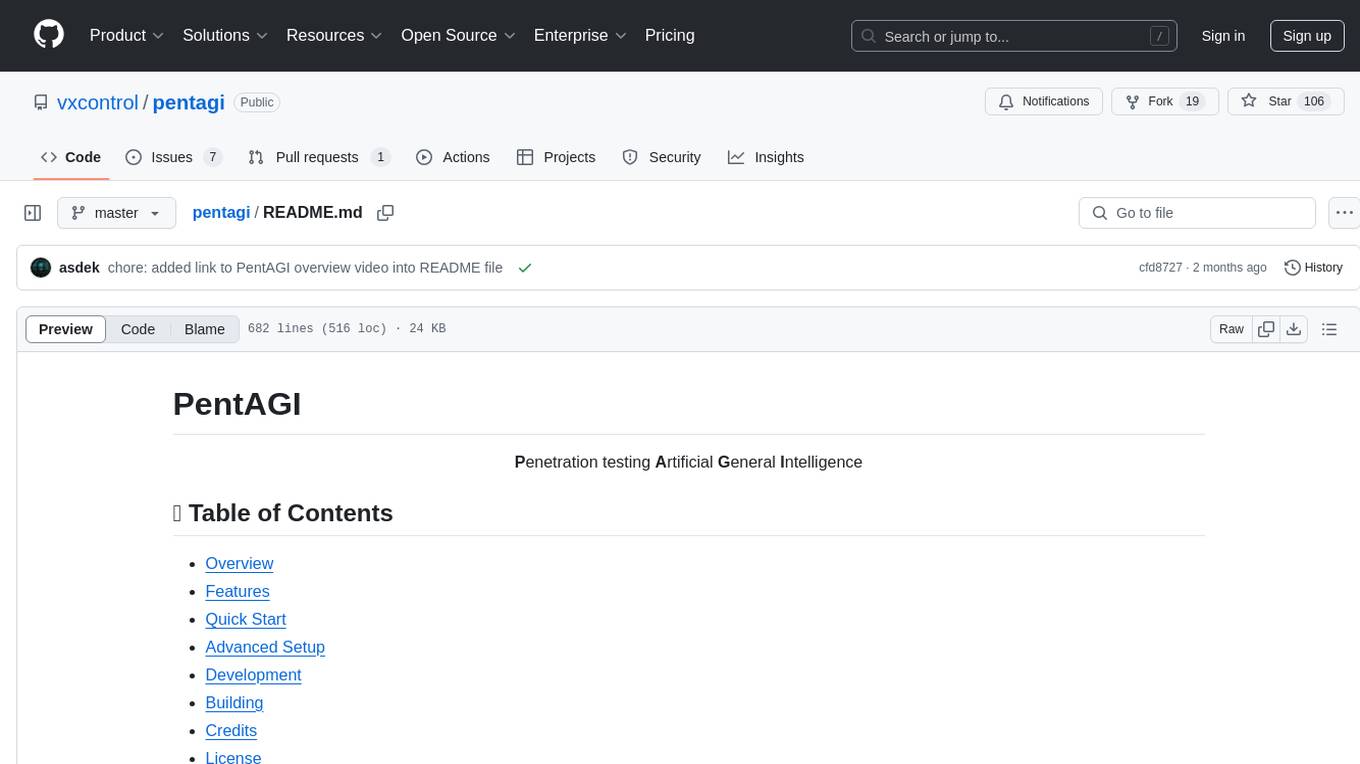
pentagi
PentAGI is an innovative tool for automated security testing that leverages cutting-edge artificial intelligence technologies. It is designed for information security professionals, researchers, and enthusiasts who need a powerful and flexible solution for conducting penetration tests. The tool provides secure and isolated operations in a sandboxed Docker environment, fully autonomous AI-powered agent for penetration testing steps, a suite of 20+ professional security tools, smart memory system for storing research results, web intelligence for gathering information, integration with external search systems, team delegation system, comprehensive monitoring and reporting, modern interface, API integration, persistent storage, scalable architecture, self-hosted solution, flexible authentication, and quick deployment through Docker Compose.
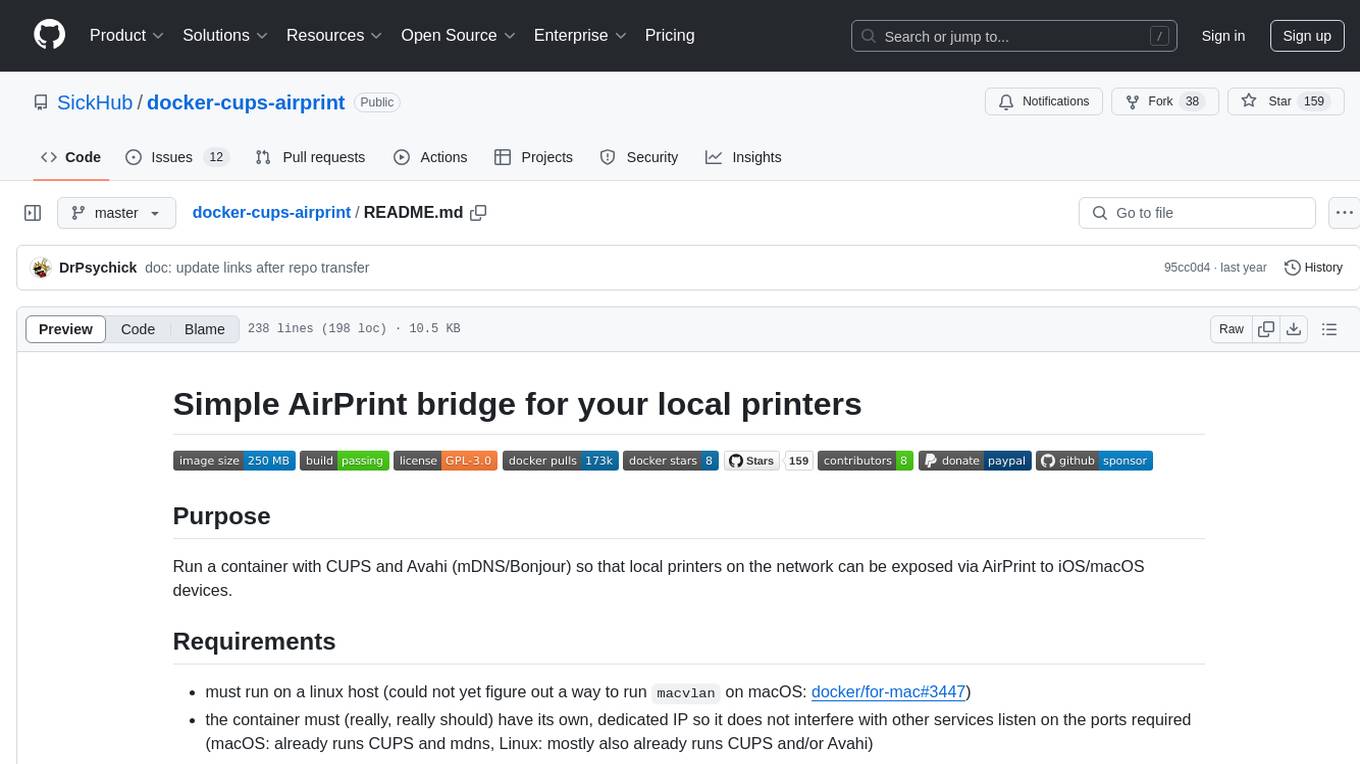
docker-cups-airprint
This repository provides a Docker image that acts as an AirPrint bridge for local printers, allowing them to be exposed to iOS/macOS devices. It runs a container with CUPS and Avahi to facilitate this functionality. Users must have CUPS drivers available for their printers. The tool requires a Linux host and a dedicated IP for the container to avoid interference with other services. It supports setting up printers through environment variables and offers options for automated configuration via command line, web interface, or files. The repository includes detailed instructions on setting up and testing the AirPrint bridge.
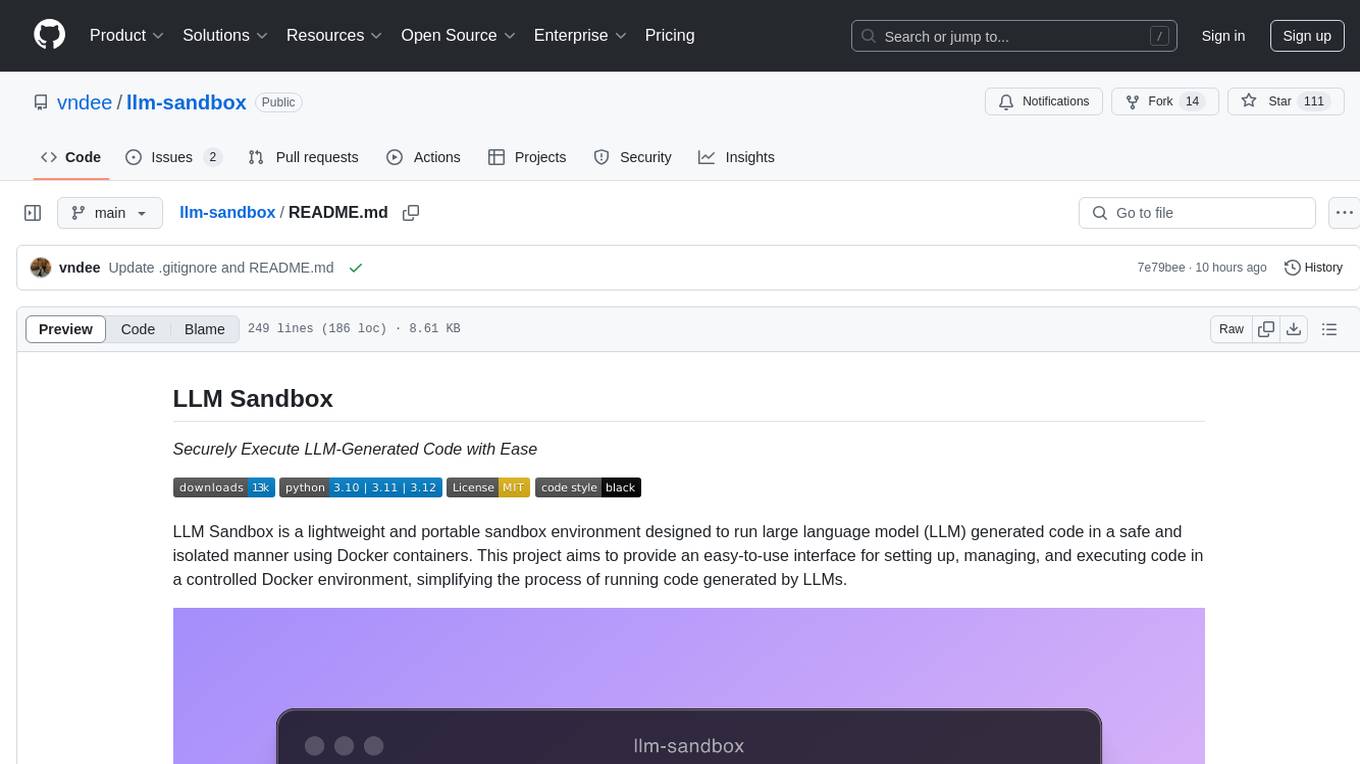
llm-sandbox
LLM Sandbox is a lightweight and portable sandbox environment designed to securely execute large language model (LLM) generated code in a safe and isolated manner using Docker containers. It provides an easy-to-use interface for setting up, managing, and executing code in a controlled Docker environment, simplifying the process of running code generated by LLMs. The tool supports multiple programming languages, offers flexibility with predefined Docker images or custom Dockerfiles, and allows scalability with support for Kubernetes and remote Docker hosts.
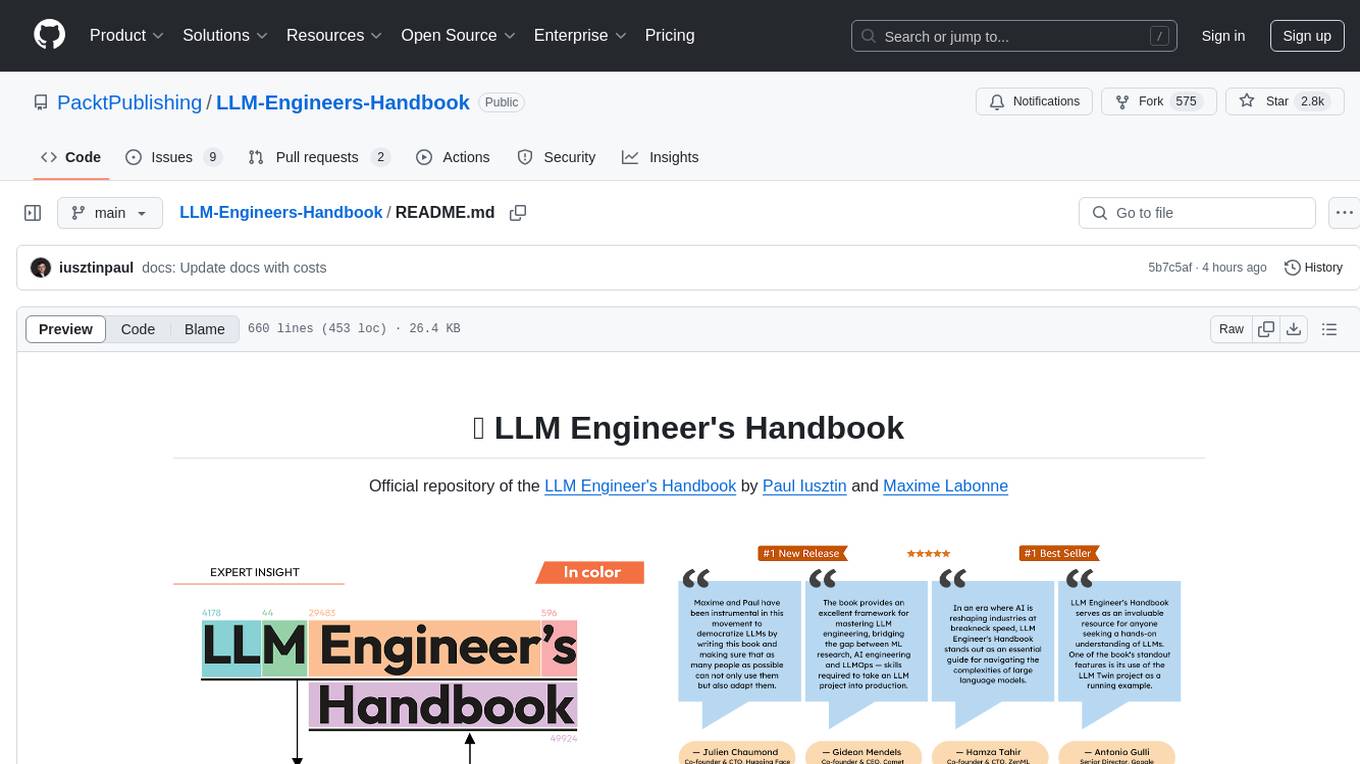
LLM-Engineers-Handbook
The LLM Engineer's Handbook is an official repository containing a comprehensive guide on creating an end-to-end LLM-based system using best practices. It covers data collection & generation, LLM training pipeline, a simple RAG system, production-ready AWS deployment, comprehensive monitoring, and testing and evaluation framework. The repository includes detailed instructions on setting up local and cloud dependencies, project structure, installation steps, infrastructure setup, pipelines for data processing, training, and inference, as well as QA, tests, and running the project end-to-end.
For similar jobs
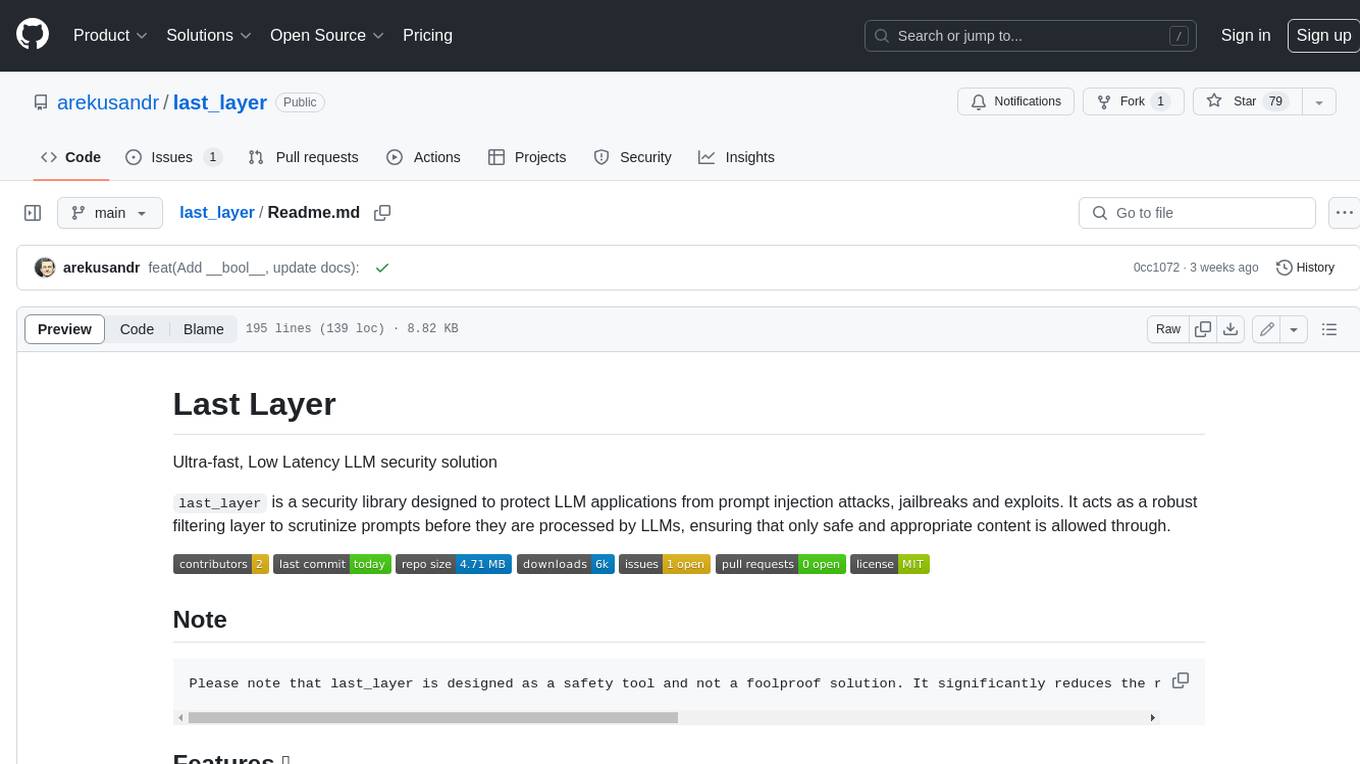
last_layer
last_layer is a security library designed to protect LLM applications from prompt injection attacks, jailbreaks, and exploits. It acts as a robust filtering layer to scrutinize prompts before they are processed by LLMs, ensuring that only safe and appropriate content is allowed through. The tool offers ultra-fast scanning with low latency, privacy-focused operation without tracking or network calls, compatibility with serverless platforms, advanced threat detection mechanisms, and regular updates to adapt to evolving security challenges. It significantly reduces the risk of prompt-based attacks and exploits but cannot guarantee complete protection against all possible threats.
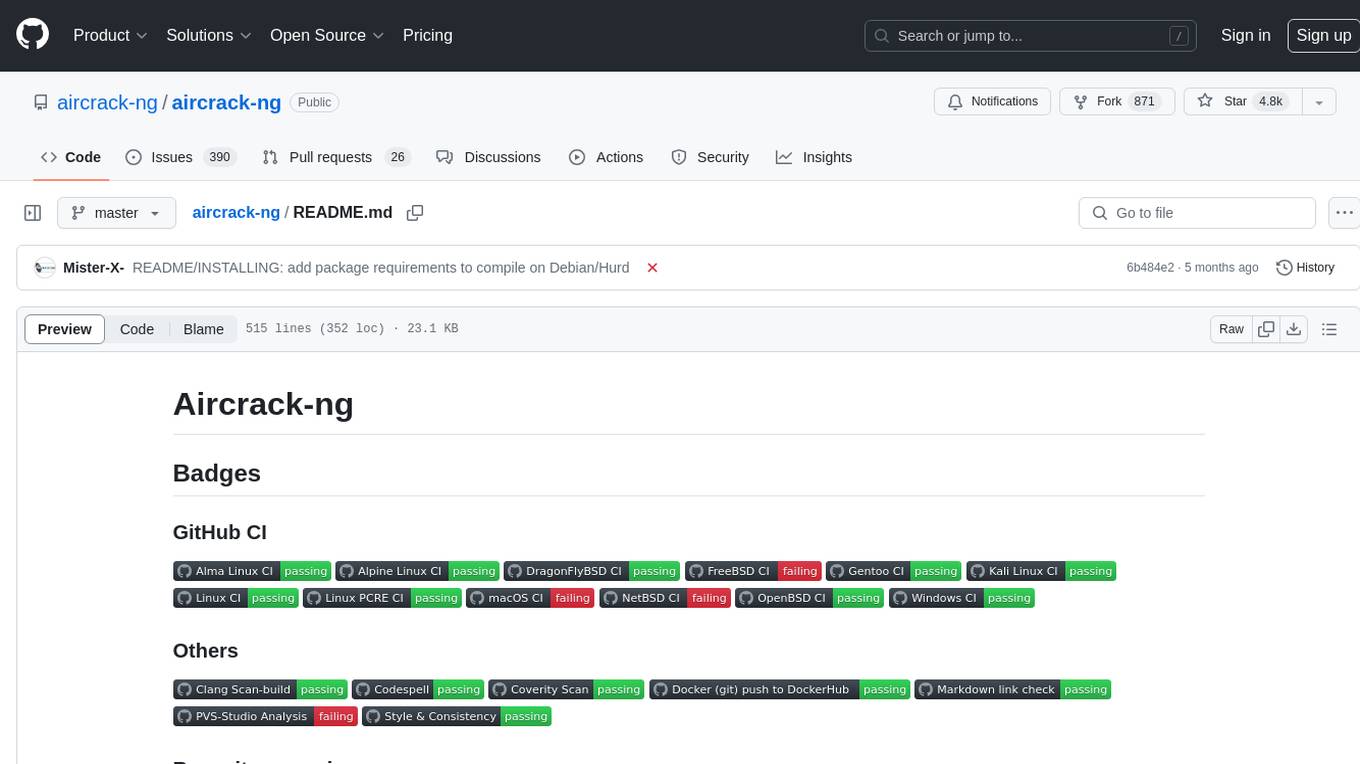
aircrack-ng
Aircrack-ng is a comprehensive suite of tools designed to evaluate the security of WiFi networks. It covers various aspects of WiFi security, including monitoring, attacking (replay attacks, deauthentication, fake access points), testing WiFi cards and driver capabilities, and cracking WEP and WPA PSK. The tools are command line-based, allowing for extensive scripting and have been utilized by many GUIs. Aircrack-ng primarily works on Linux but also supports Windows, macOS, FreeBSD, OpenBSD, NetBSD, Solaris, and eComStation 2.
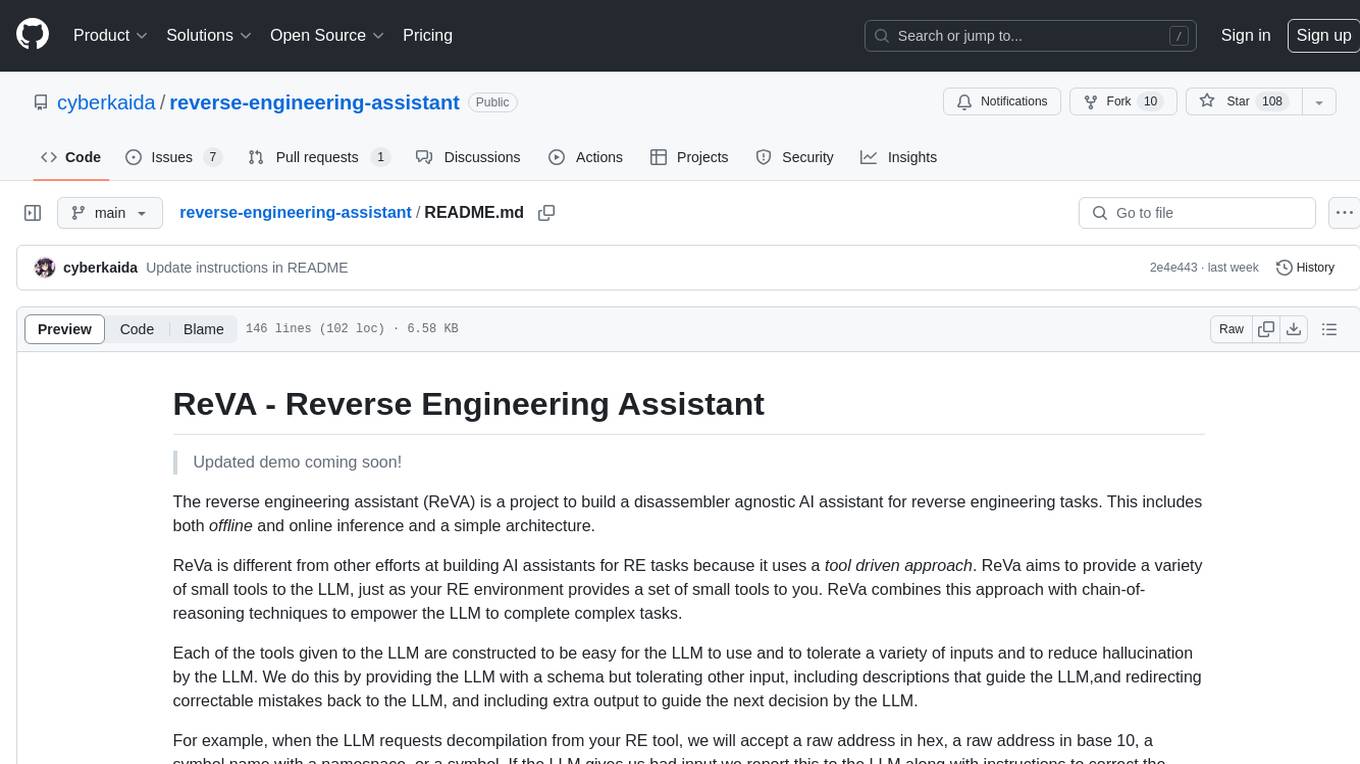
reverse-engineering-assistant
ReVA (Reverse Engineering Assistant) is a project aimed at building a disassembler agnostic AI assistant for reverse engineering tasks. It utilizes a tool-driven approach, providing small tools to the user to empower them in completing complex tasks. The assistant is designed to accept various inputs, guide the user in correcting mistakes, and provide additional context to encourage exploration. Users can ask questions, perform tasks like decompilation, class diagram generation, variable renaming, and more. ReVA supports different language models for online and local inference, with easy configuration options. The workflow involves opening the RE tool and program, then starting a chat session to interact with the assistant. Installation includes setting up the Python component, running the chat tool, and configuring the Ghidra extension for seamless integration. ReVA aims to enhance the reverse engineering process by breaking down actions into small parts, including the user's thoughts in the output, and providing support for monitoring and adjusting prompts.
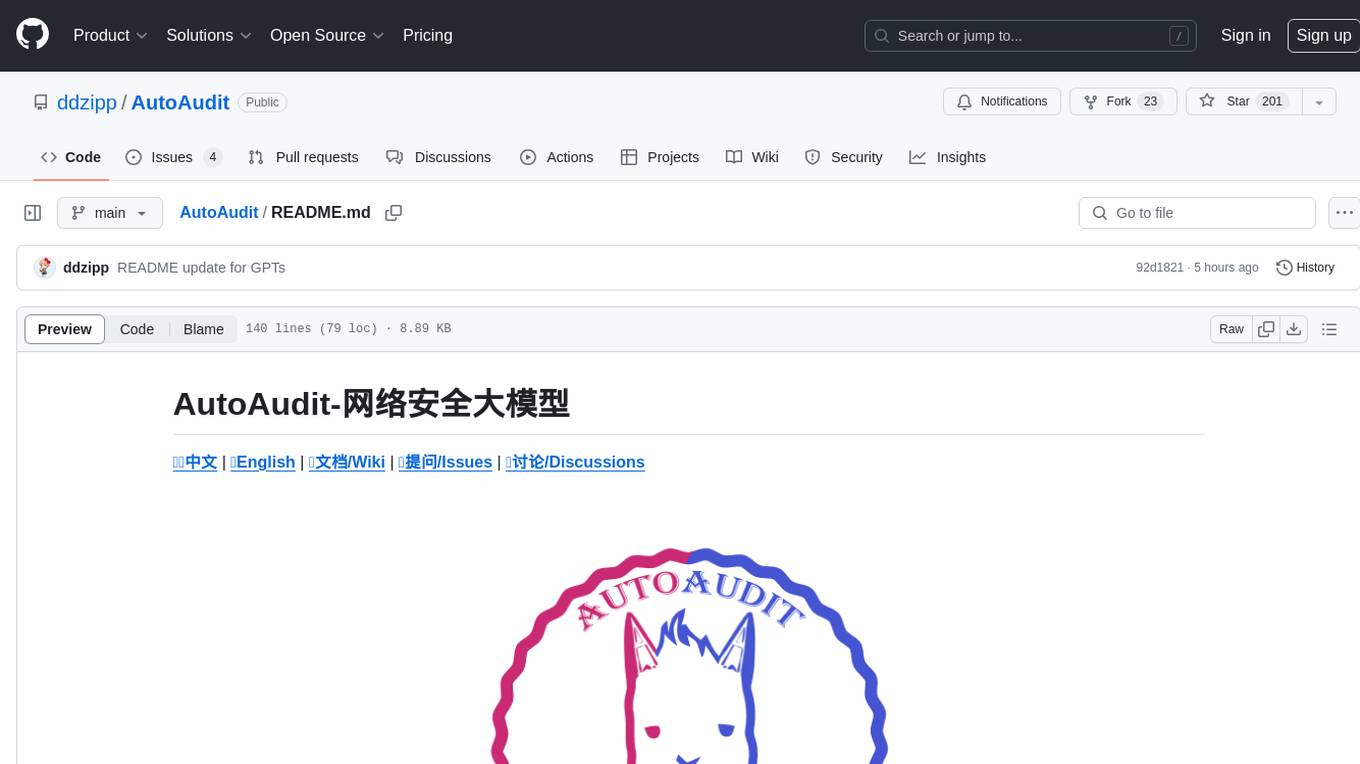
AutoAudit
AutoAudit is an open-source large language model specifically designed for the field of network security. It aims to provide powerful natural language processing capabilities for security auditing and network defense, including analyzing malicious code, detecting network attacks, and predicting security vulnerabilities. By coupling AutoAudit with ClamAV, a security scanning platform has been created for practical security audit applications. The tool is intended to assist security professionals with accurate and fast analysis and predictions to combat evolving network threats.
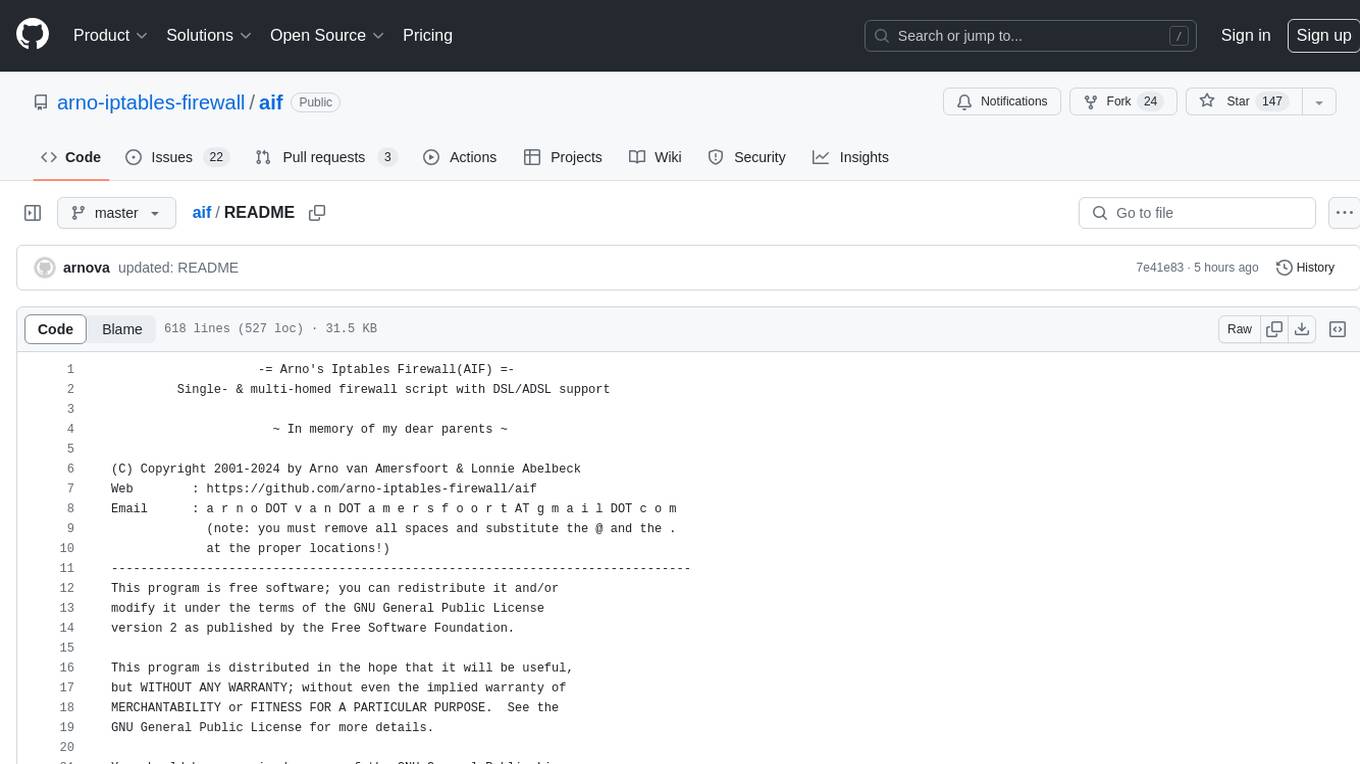
aif
Arno's Iptables Firewall (AIF) is a single- & multi-homed firewall script with DSL/ADSL support. It is a free software distributed under the GNU GPL License. The script provides a comprehensive set of configuration files and plugins for setting up and managing firewall rules, including support for NAT, load balancing, and multirouting. It offers detailed instructions for installation and configuration, emphasizing security best practices and caution when modifying settings. The script is designed to protect against hostile attacks by blocking all incoming traffic by default and allowing users to configure specific rules for open ports and network interfaces.
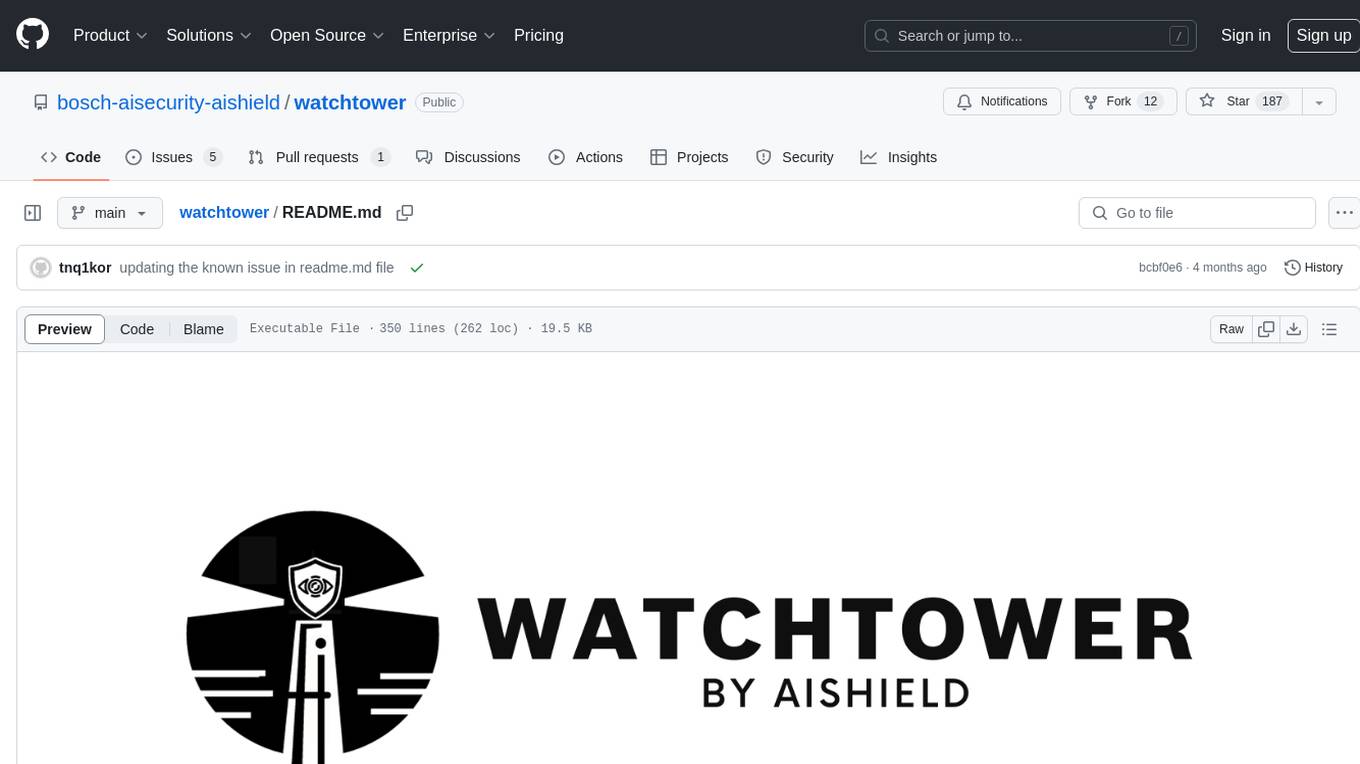
watchtower
AIShield Watchtower is a tool designed to fortify the security of AI/ML models and Jupyter notebooks by automating model and notebook discoveries, conducting vulnerability scans, and categorizing risks into 'low,' 'medium,' 'high,' and 'critical' levels. It supports scanning of public GitHub repositories, Hugging Face repositories, AWS S3 buckets, and local systems. The tool generates comprehensive reports, offers a user-friendly interface, and aligns with industry standards like OWASP, MITRE, and CWE. It aims to address the security blind spots surrounding Jupyter notebooks and AI models, providing organizations with a tailored approach to enhancing their security efforts.
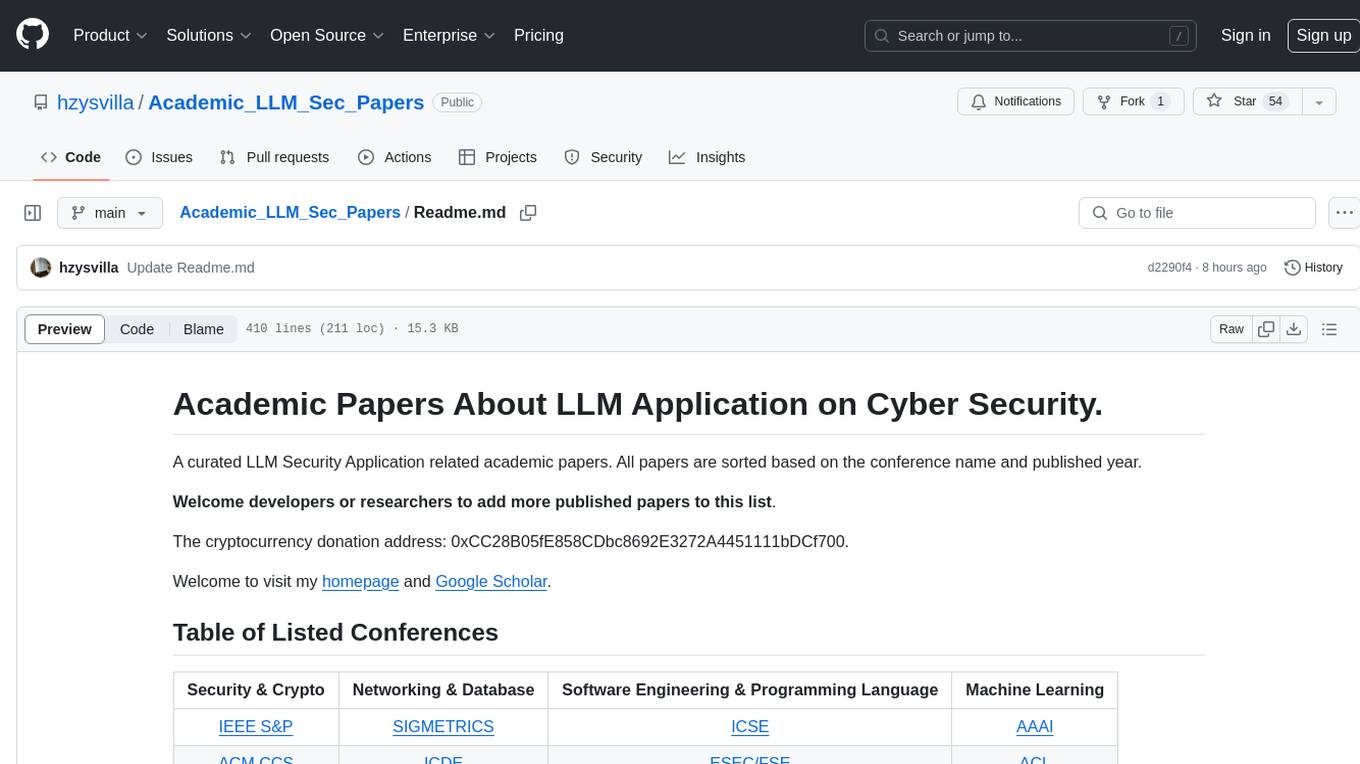
Academic_LLM_Sec_Papers
Academic_LLM_Sec_Papers is a curated collection of academic papers related to LLM Security Application. The repository includes papers sorted by conference name and published year, covering topics such as large language models for blockchain security, software engineering, machine learning, and more. Developers and researchers are welcome to contribute additional published papers to the list. The repository also provides information on listed conferences and journals related to security, networking, software engineering, and cryptography. The papers cover a wide range of topics including privacy risks, ethical concerns, vulnerabilities, threat modeling, code analysis, fuzzing, and more.
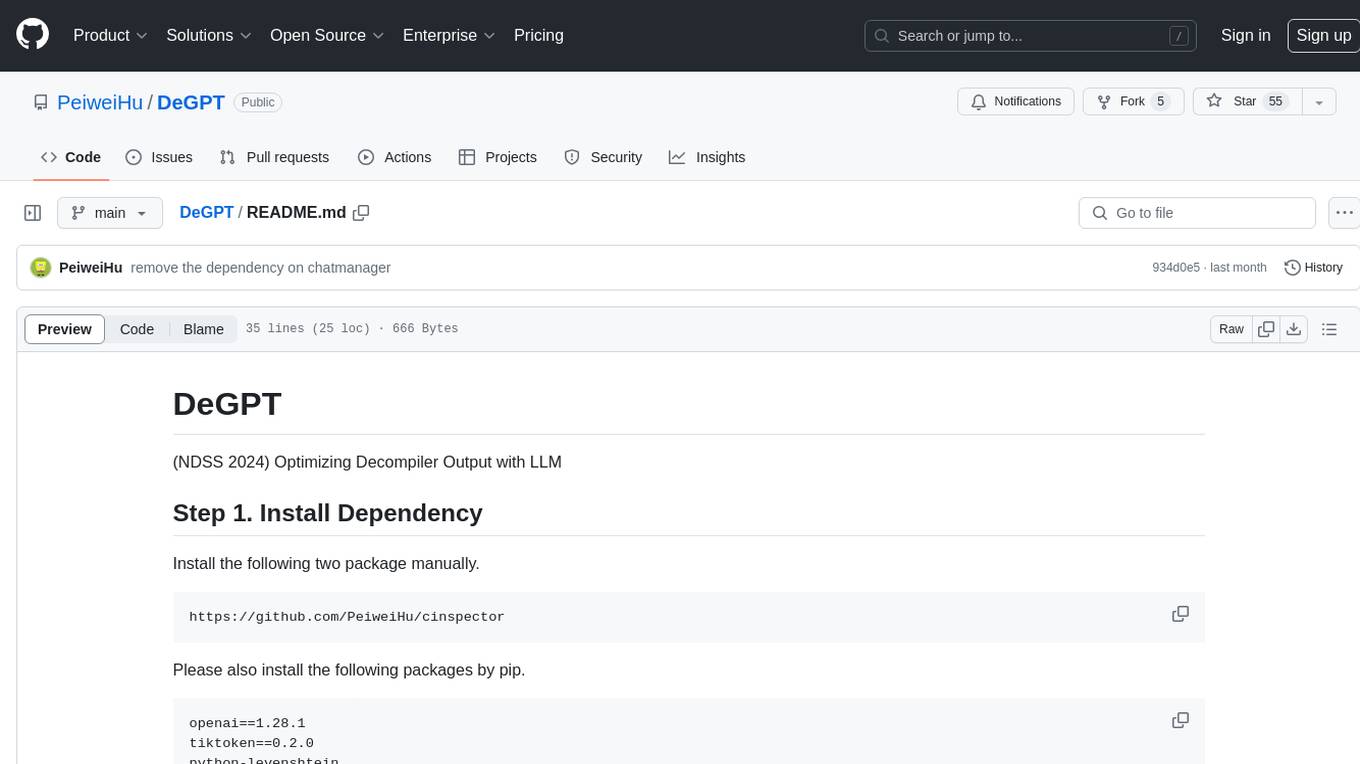
DeGPT
DeGPT is a tool designed to optimize decompiler output using Large Language Models (LLM). It requires manual installation of specific packages and setting up API key for OpenAI. The tool provides functionality to perform optimization on decompiler output by running specific scripts.