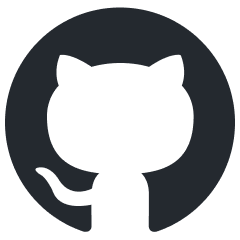
text-extract-api
Document (PDF, Word, PPTX ...) extraction and parse API using state of the art modern OCRs + Ollama supported models. Anonymize documents. Remove PII. Convert any document or picture to structured JSON or Markdown
Stars: 2068
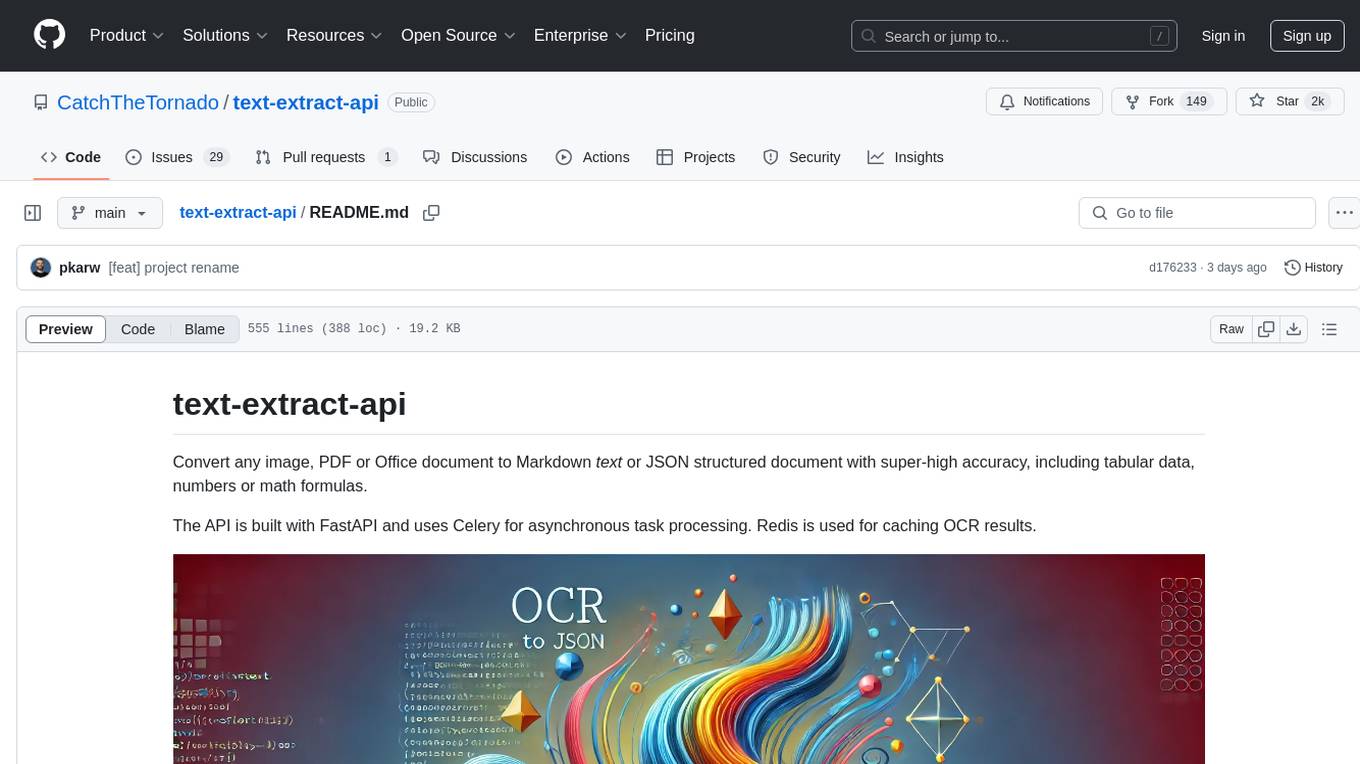
The text-extract-api is a powerful tool that allows users to convert images, PDFs, or Office documents to Markdown text or JSON structured documents with high accuracy. It is built using FastAPI and utilizes Celery for asynchronous task processing, with Redis for caching OCR results. The tool provides features such as PDF/Office to Markdown and JSON conversion, improving OCR results with LLama, removing Personally Identifiable Information from documents, distributed queue processing, caching using Redis, switchable storage strategies, and a CLI tool for task management. Users can run the tool locally or on cloud services, with support for GPU processing. The tool also offers an online demo for testing purposes.
README:
Convert any image, PDF or Office document to Markdown text or JSON structured document with super-high accuracy, including tabular data, numbers or math formulas.
The API is built with FastAPI and uses Celery for asynchronous task processing. Redis is used for caching OCR results.
-
No Cloud/external dependencies all you need: PyTorch based OCR (EasyOCR) + Ollama are shipped and configured via
docker-compose
no data is sent outside your dev/server environment, - PDF/Office to Markdown conversion with very high accuracy using different OCR strategies including llama3.2-vision, easyOCR
- PDF/Office to JSON conversion using Ollama supported models (eg. LLama 3.1)
- LLM Improving OCR results LLama is pretty good with fixing spelling and text issues in the OCR text
-
Removing PII This tool can be used for removing Personally Identifiable Information out of document - see
examples
- Distributed queue processing using Celery)
- Caching using Redis - the OCR results can be easily cached prior to LLM processing,
- Storage Strategies switchable storage strategies (Google Drive, Local File System ...)
- CLI tool for sending tasks and processing results
Converting MRI report to Markdown + JSON.
python client/cli.py ocr_upload --file examples/example-mri.pdf --prompt_file examples/example-mri-2-json-prompt.txt
Before running the example see getting started
Converting Invoice to JSON and remove PII
python client/cli.py ocr_upload --file examples/example-invoice.pdf --prompt_file examples/example-invoice-remove-pii.txt
Before running the example see getting started
You might want to run the app directly on your machine for development purposes OR to use for example Apple GPUs (which are not supported by Docker at the moment).
To have it up and running please execute the following steps:
Download and install Ollama Download and install Docker
To connect to an external Ollama instance, set the environment variable:
OLLAMA_HOST=http://address:port
, e.g.:OLLAMA_HOST=http(s)://127.0.0.1:5000If you want to disable the local Ollama model, use env
DISABLE_LOCAL_OLLAMA=1
, e.g.DISABLE_LOCAL_OLLAMA=1 make installNote: When local Ollama is disabled, ensure the required model is downloaded on the external instance.
Currently, the
DISABLE_LOCAL_OLLAMA
variable cannot be used to disable Ollama in Docker. As a workaround, remove theollama
service fromdocker-compose.yml
ordocker-compose.gpu.yml
.Support for using the variable in Docker environments will be added in a future release.
First, clone the repository and change current directory to it:
git clone https://github.com/CatchTheTornado/text-extract-api.git
cd text-extract-api
Be default application create virtual python env: .venv
. You can disable this functionality on local setup by adding DISABLE_VENV=1
before running script:
DISABLE_VENV=1 make install
DISABLE_VENV=1 make run
Configure environment variables:
cp .env.localhost.example .env.localhost
You might want to just use the defaults - should be fine. After ENV variables are set, just execute:
python3 -m venv .venv
source .venv/bin/activate
pip install -e .
chmod +x run.sh
run.sh
This command will install all the dependencies - including Redis (via Docker, so it is not entirely docker free method of running text-extract-api
anyways :)
(MAC) - Dependencies
brew update && brew install libmagic poppler pkg-config ghostscript ffmpeg automake autoconf
(Mac) - You need to startup the celery worker
source .venv/bin/activate && celery -A text_extract_api.celery_app worker --loglevel=info --pool=solo
Then you're good to go with running some CLI commands like:
python client/cli.py ocr_upload --file examples/example-mri.pdf --ocr_cache --prompt_file=examples/example-mri-remove-pii.txt
To have multiple tasks running at once - for concurrent processing please run the following command to start single worker process:
celery -A text_extract_api.tasks worker --loglevel=info --pool=solo & # to scale by concurrent processing please run this line as many times as many concurrent processess you want to have running
To try out the application with our hosted version you can skip the Getting started and try out the CLI tool against our cloud:
Open in the browser: demo.doctractor.com
... or run n the terminal:
python3 -m venv .venv
source .venv/bin/activate
pip install -e .
export OCR_UPLOAD_URL=https://doctractor:[email protected]/ocr/upload
export RESULT_URL=https://doctractor:[email protected]/ocr/result/
python client/cli.py ocr_upload --file examples/example-mri.pdf --ocr_cache --prompt_file=examples/example-mri-remove-pii.txt
Note: In the free demo we don't guarantee any processing times. The API is Open so please do not send any secret documents neither any documents containing personal information, If you do - you're doing it on your own risk and responsiblity.
In case of any questions, help requests or just feedback - please join us on Discord!
- Docker
- Docker Compose
git clone https://github.com/CatchTheTornado/text-extract-api.git
cd text-extract-api
You can use the make install
and make run
command to setup the Docker environment for text-extract-api
. You can find the manual steps required to do so described below.
Create .env
file in the root directory and set the necessary environment variables. You can use the .env.example
file as a template:
# defaults for docker instances
cp .env.example .env
or
# defaults for local run
cp .env.example.localhost .env
Then modify the variables inside the file:
#APP_ENV=production # sets the app into prod mode, otherwise dev mode with auto-reload on code changes
REDIS_CACHE_URL=redis://localhost:6379/1
STORAGE_PROFILE_PATH=./storage_profiles
LLAMA_VISION_PROMPT="You are OCR. Convert image to markdown."
# CLI settings
OCR_URL=http://localhost:8000/ocr/upload
OCR_UPLOAD_URL=http://localhost:8000/ocr/upload
OCR_REQUEST_URL=http://localhost:8000/ocr/request
RESULT_URL=http://localhost:8000/ocr/result/
CLEAR_CACHE_URL=http://localhost:8000/ocr/clear_cach
LLM_PULL_API_URL=http://localhost:8000/llm_pull
LLM_GENEREATE_API_URL=http://localhost:8000/llm_generate
CELERY_BROKER_URL=redis://localhost:6379/0
CELERY_RESULT_BACKEND=redis://localhost:6379/0
OLLAMA_HOST=http://localhost:11434
APP_ENV=development # Default to development mode
Note: In order to properly save the output files you might need to modify storage_profiles/default.yaml
to change the default storage path according to the volumes path defined in the docker-compose.yml
Build and run the Docker containers using Docker Compose:
docker-compose up --build
... for GPU support run:
docker-compose -f docker-compose.gpu.yml -p text-extract-api-gpu up --build
Note: While on Mac - Docker does not support Apple GPUs. In this case you might want to run the application natively without the Docker Compose please check how to run it natively with GPU support
This will start the following services:
- FastAPI App: Runs the FastAPI application.
- Celery Worker: Processes asynchronous OCR tasks.
- Redis: Caches OCR results.
- Ollama: Runs the Ollama model.
If the on-prem is too much hassle ask us about the hosted/cloud edition of text-extract-api, we can setup it you, billed just for the usage.
Note: While on Mac, you may need to create a virtual Python environment first:
python3 -m venv .venv
source .venv/bin/activate
# now you've got access to `python` and `pip` within your virutal env.
pip install -e . # install main project requirements
The project includes a CLI for interacting with the API. To make it work first run:
cd client
pip install -e .
You might want to test out different models supported by LLama
python client/cli.py llm_pull --model llama3.1
python client/cli.py llm_pull --model llama3.2-vision
These models are required for most features supported by text-extract-api
.
python client/cli.py ocr_upload --file examples/example-mri.pdf --ocr_cache
or alternatively
python client/cli.py ocr_request --file examples/example-mri.pdf --ocr_cache
The difference is just that the first call uses ocr/upload
- multipart form data upload, and the second one is a request to ocr/request
sending the file via base64 encoded JSON property - probable a better suit for smaller files.
Important note: To use LLM you must first run the llm_pull to get the specific model required by your requests.
For example you must run:
python client/cli.py llm_pull --model llama3.1
python client/cli.py llm_pull --model llama3.2-vision
and only after to run this specific prompt query:
python client/cli.py ocr_upload --file examples/example-mri.pdf --ocr_cache --prompt_file=examples/example-mri-remove-pii.txt --language en
Note: The language argument is used for the OCR strategy to load the model weights for the selected language. You can specify multiple languages as a list: en,de,pl
etc.
The ocr
command can store the results using the storage_profiles
:
-
storage_profile: Used to save the result - the
default
profile (./storage_profiles/default.yaml
) is used by default; if empty file is not saved -
storage_filename: Outputting filename - relative path of the
root_path
set in the storage profile - by default a relative path to/storage
folder; can use placeholders for dynamic formatting:{file_name}
,{file_extension}
,{Y}
,{mm}
,{dd}
- for date formatting,{HH}
,{MM}
,{SS}
- for time formatting
python client/cli.py ocr_upload --file examples/example-mri.pdf --ocr_cache --prompt_file=examples/example-mri-remove-pii.txt --storage_filename "invoices/{Y}/{file_name}-{Y}-{mm}-{dd}.md"
python client/cli.py result --task_id {your_task_id_from_upload_step}
python client/cli.py list_files
to use specific (in this case google drive
) storage profile run:
python client/cli.py list_files --storage_profile gdrive
python client/cli.py load_file --file_name "invoices/2024/example-invoice-2024-10-31-16-33.md"
python client/cli.py delete_file --file_name "invoices/2024/example-invoice-2024-10-31-16-33.md" --storage_profile gdrive
or for default profile (local file system):
python client/cli.py delete_file --file_name "invoices/2024/example-invoice-2024-10-31-16-33.md"
python client/cli.py clear_cache
python llm_generate --prompt "Your prompt here"
You might want to use the decdicated API clients to use text-extract-api
There's a dedicated API client for Typescript - text-extract-api-client and the npm
package by the same name:
npm install text-extract-api-client
Usage:
import { ApiClient, OcrRequest } from 'text-extract-api-client';
const apiClient = new ApiClient('https://api.doctractor.com/', 'doctractor', 'Aekie2ao');
const formData = new FormData();
formData.append('file', fileInput.files[0]);
formData.append('prompt', 'Convert file to JSON and return only JSON'); // if not provided, no LLM transformation will gonna happen - just the OCR
formData.append('strategy', 'llama_vision');
formData.append('model', 'llama3.1')
formData.append('ocr_cache', 'true');
apiClient.uploadFile(formData).then(response => {
console.log(response);
});
- URL: /ocr/upload
- Method: POST
-
Parameters:
- file: PDF, image or Office file to be processed.
-
strategy: OCR strategy to use (
llama_vision
oreasyocr
). - ocr_cache: Whether to cache the OCR result (true or false).
- prompt: When provided, will be used for Ollama processing the OCR result
- model: When provided along with the prompt - this model will be used for LLM processing
-
storage_profile: Used to save the result - the
default
profile (./storage_profiles/default.yaml
) is used by default; if empty file is not saved -
storage_filename: Outputting filename - relative path of the
root_path
set in the storage profile - by default a relative path to/storage
folder; can use placeholders for dynamic formatting:{file_name}
,{file_extension}
,{Y}
,{mm}
,{dd}
- for date formatting,{HH}
,{MM}
,{SS}
- for time formatting -
language: One or many (
en
oren,pl,de
) language codes for the OCR to load the language weights
Example:
curl -X POST -H "Content-Type: multipart/form-data" -F "file=@examples/example-mri.pdf" -F "strategy=easyocr" -F "ocr_cache=true" -F "prompt=" -F "model=" "http://localhost:8000/ocr/upload"
- URL: /ocr/request
- Method: POST
-
Parameters (JSON body):
- file: Base64 encoded PDF file content.
-
strategy: OCR strategy to use (
llama_vision
oreasyocr
). - ocr_cache: Whether to cache the OCR result (true or false).
- prompt: When provided, will be used for Ollama processing the OCR result.
- model: When provided along with the prompt - this model will be used for LLM processing.
-
storage_profile: Used to save the result - the
default
profile (/storage_profiles/default.yaml
) is used by default; if empty file is not saved. -
storage_filename: Outputting filename - relative path of the
root_path
set in the storage profile - by default a relative path to/storage
folder; can use placeholders for dynamic formatting:{file_name}
,{file_extension}
,{Y}
,{mm}
,{dd}
- for date formatting,{HH}
,{MM}
,{SS}
- for time formatting. -
language: One or many (
en
oren,pl,de
) language codes for the OCR to load the language weights
Example:
curl -X POST "http://localhost:8000/ocr/request" -H "Content-Type: application/json" -d '{
"file": "<base64-encoded-file-content>",
"strategy": "easyocr",
"ocr_cache": true,
"prompt": "",
"model": "llama3.1",
"storage_profile": "default",
"storage_filename": "example.pdf"
}'
- URL: /ocr/result/{task_id}
- Method: GET
-
Parameters:
- task_id: Task ID returned by the OCR endpoint.
Example:
curl -X GET "http://localhost:8000/ocr/result/{task_id}"
- URL: /ocr/clear_cache
- Method: POST
Example:
curl -X POST "http://localhost:8000/ocr/clear_cache"
- URL: /llm/pull
- Method: POST
-
Parameters:
- model: Pull the model you are to use first
Example:
curl -X POST "http://localhost:8000/llm/pull" -H "Content-Type: application/json" -d '{"model": "llama3.1"}'
- URL: /llm/generate
- Method: POST
-
Parameters:
- prompt: Prompt for the Ollama model.
- model: Model you like to query
Example:
curl -X POST "http://localhost:8000/llm/generate" -H "Content-Type: application/json" -d '{"prompt": "Your prompt here", "model":"llama3.1"}'
- URL: /storage/list
- Method: GET
-
Parameters:
-
storage_profile: Name of the storage profile to use for listing files (default:
default
).
-
storage_profile: Name of the storage profile to use for listing files (default:
- URL: /storage/load
- Method: GET
-
Parameters:
- file_name: File name to load from the storage
-
storage_profile: Name of the storage profile to use for listing files (default:
default
).
- URL: /storage/delete
- Method: DELETE
-
Parameters:
- file_name: File name to load from the storage
-
storage_profile: Name of the storage profile to use for listing files (default:
default
).
The tool can automatically save the results using different storage strategies and storage profiles. Storage profiles are set in the /storage_profiles
by a yaml configuration files.
strategy: local_filesystem
settings:
root_path: /storage # The root path where the files will be stored - mount a proper folder in the docker file to match it
subfolder_names_format: "" # eg: by_months/{Y}-{mm}/
create_subfolders: true
strategy: google_drive
settings:
## how to enable GDrive API: https://developers.google.com/drive/api/quickstart/python?hl=pl
service_account_file: /storage/client_secret_269403342997-290pbjjlb06nbof78sjaj7qrqeakp3t0.apps.googleusercontent.com.json
folder_id:
Where the service_account_file
is a json
file with authorization credentials. Please read on how to enable Google Drive API and prepare this authorization file here.
Note: Service Account is different account that the one you're using for Google workspace (files will not be visible in the UI)
strategy: aws_s3
settings:
bucket_name: ${AWS_S3_BUCKET_NAME}
region: ${AWS_REGION}
access_key: ${AWS_ACCESS_KEY_ID}
secret_access_key: ${AWS_SECRET_ACCESS_KEY}
-
Access Key Ownership
The access key must belong to an IAM user or role with permissions for S3 operations. -
IAM Policy Example
The IAM policy attached to the user or role must allow the necessary actions. Below is an example of a policy granting access to an S3 bucket:{ "Version": "2012-10-17", "Statement": [ { "Effect": "Allow", "Action": [ "s3:PutObject", "s3:GetObject", "s3:ListBucket", "s3:DeleteObject" ], "Resource": [ "arn:aws:s3:::your-bucket-name", "arn:aws:s3:::your-bucket-name/*" ] } ] }
Next, populate the appropriate .env
file (e.g., .env, .env.localhost) with the required AWS credentials:
AWS_ACCESS_KEY_ID=your-access-key-id
AWS_SECRET_ACCESS_KEY=your-secret-access-key
AWS_REGION=your-region
AWS_S3_BUCKET_NAME=your-bucket-name
This project is licensed under the MIT License. See the LICENSE file for details.
In case of any questions please contact us at: [email protected]
For Tasks:
Click tags to check more tools for each tasksFor Jobs:
Alternative AI tools for text-extract-api
Similar Open Source Tools
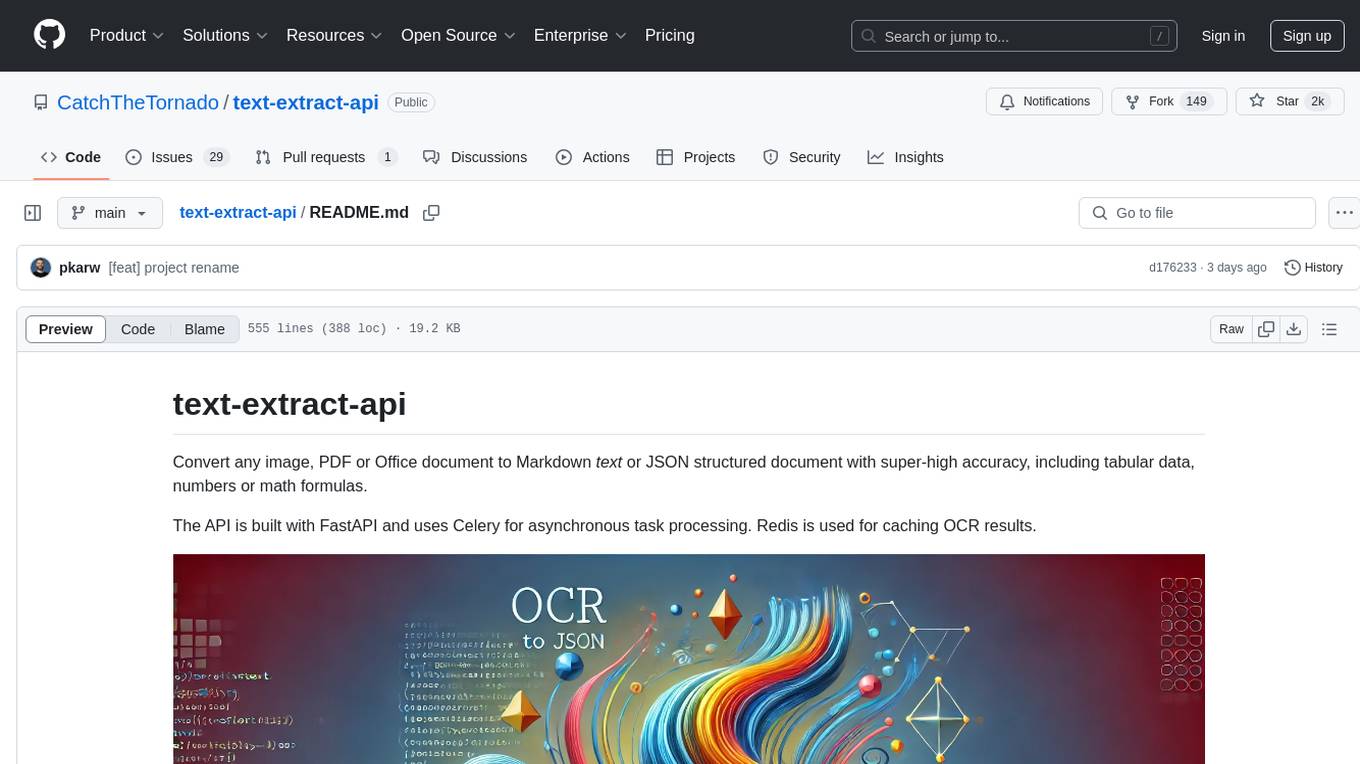
text-extract-api
The text-extract-api is a powerful tool that allows users to convert images, PDFs, or Office documents to Markdown text or JSON structured documents with high accuracy. It is built using FastAPI and utilizes Celery for asynchronous task processing, with Redis for caching OCR results. The tool provides features such as PDF/Office to Markdown and JSON conversion, improving OCR results with LLama, removing Personally Identifiable Information from documents, distributed queue processing, caching using Redis, switchable storage strategies, and a CLI tool for task management. Users can run the tool locally or on cloud services, with support for GPU processing. The tool also offers an online demo for testing purposes.
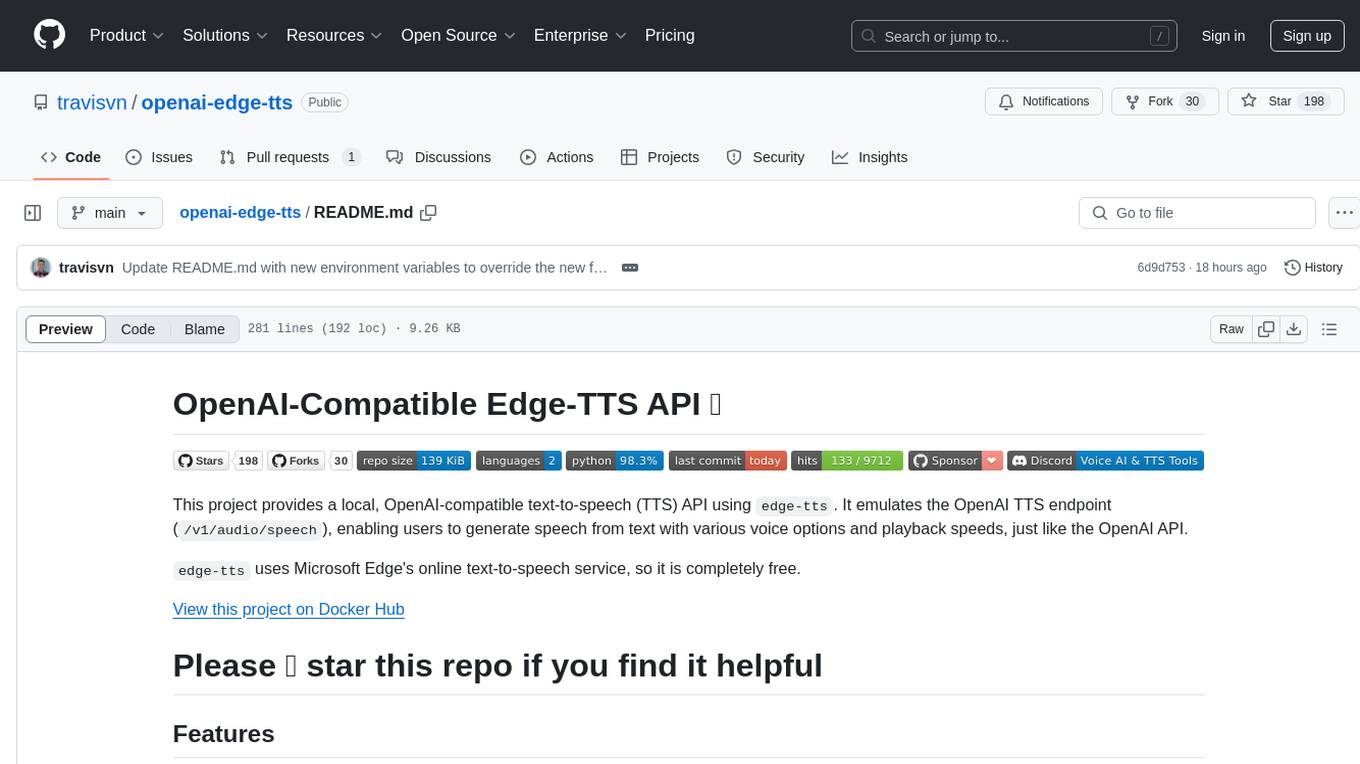
openai-edge-tts
This project provides a local, OpenAI-compatible text-to-speech (TTS) API using `edge-tts`. It emulates the OpenAI TTS endpoint (`/v1/audio/speech`), enabling users to generate speech from text with various voice options and playback speeds, just like the OpenAI API. `edge-tts` uses Microsoft Edge's online text-to-speech service, making it completely free. The project supports multiple audio formats, adjustable playback speed, and voice selection options, providing a flexible and customizable TTS solution for users.
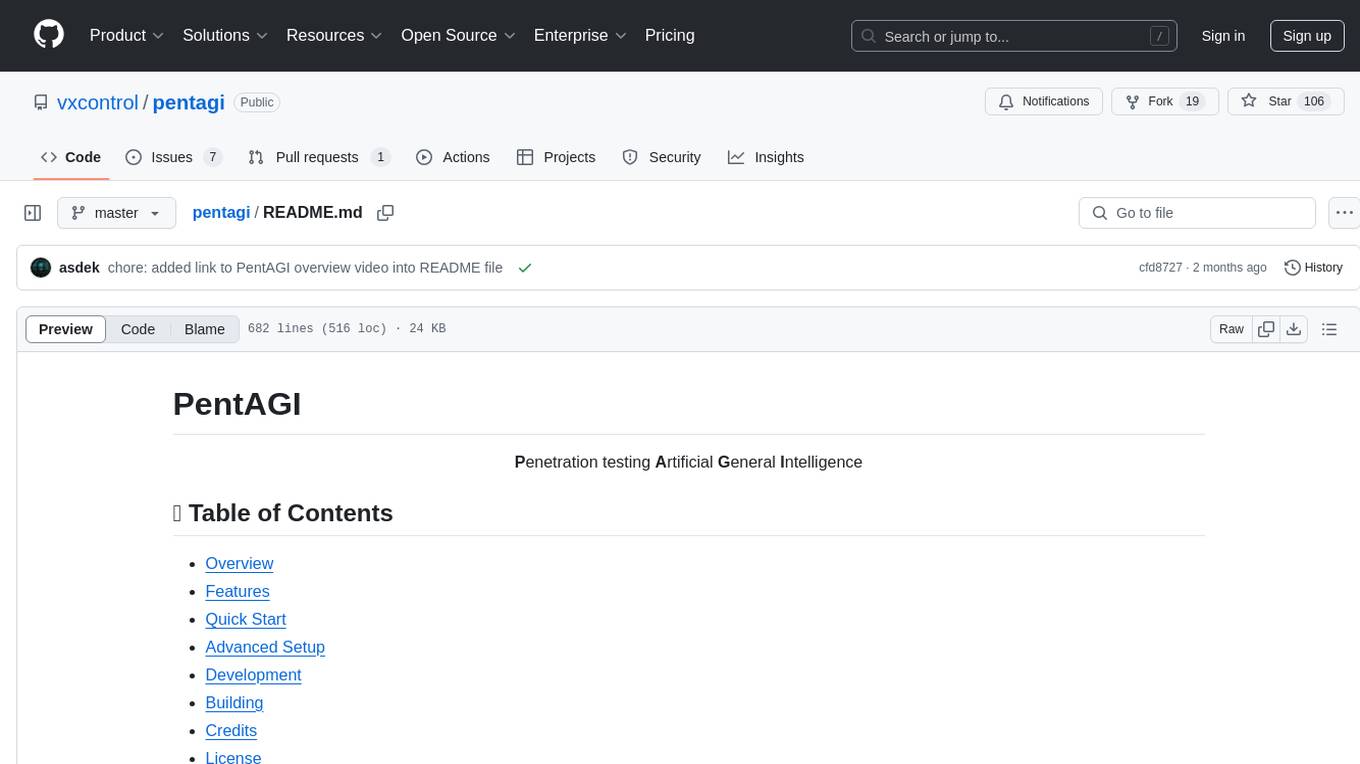
pentagi
PentAGI is an innovative tool for automated security testing that leverages cutting-edge artificial intelligence technologies. It is designed for information security professionals, researchers, and enthusiasts who need a powerful and flexible solution for conducting penetration tests. The tool provides secure and isolated operations in a sandboxed Docker environment, fully autonomous AI-powered agent for penetration testing steps, a suite of 20+ professional security tools, smart memory system for storing research results, web intelligence for gathering information, integration with external search systems, team delegation system, comprehensive monitoring and reporting, modern interface, API integration, persistent storage, scalable architecture, self-hosted solution, flexible authentication, and quick deployment through Docker Compose.
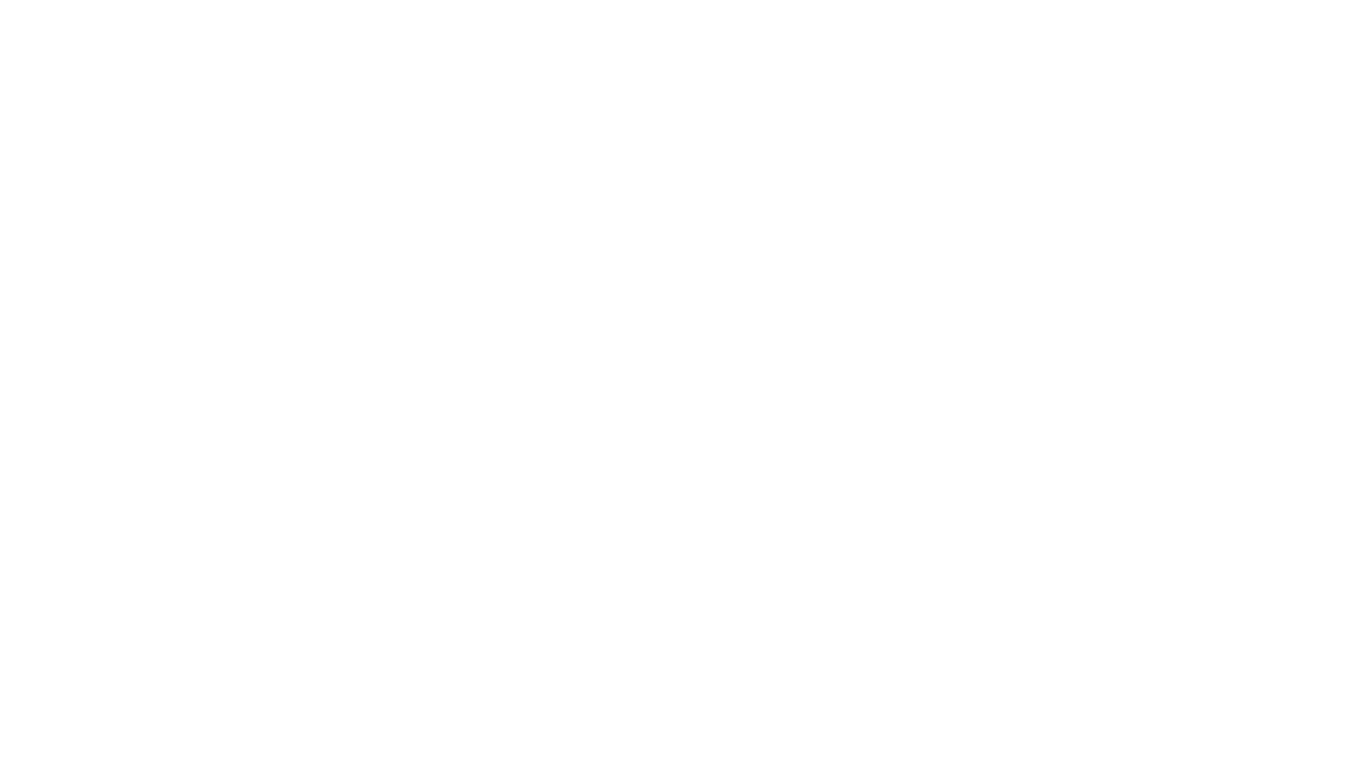
Zero
Zero is an open-source AI email solution that allows users to self-host their email app while integrating external services like Gmail. It aims to modernize and enhance emails through AI agents, offering features like open-source transparency, AI-driven enhancements, data privacy, self-hosting freedom, unified inbox, customizable UI, and developer-friendly extensibility. Built with modern technologies, Zero provides a reliable tech stack including Next.js, React, TypeScript, TailwindCSS, Node.js, Drizzle ORM, and PostgreSQL. Users can set up Zero using standard setup or Dev Container setup for VS Code users, with detailed environment setup instructions for Better Auth, Google OAuth, and optional GitHub OAuth. Database setup involves starting a local PostgreSQL instance, setting up database connection, and executing database commands for dependencies, tables, migrations, and content viewing.
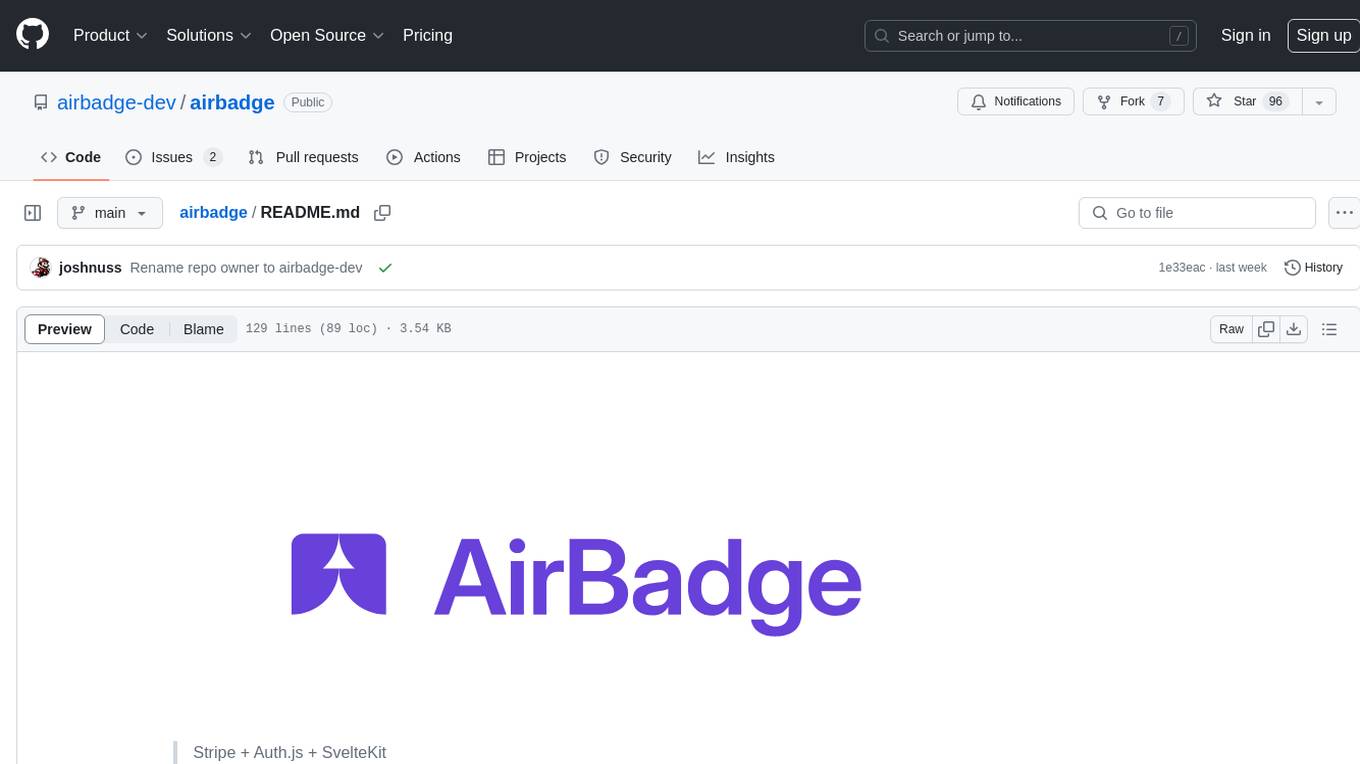
airbadge
Airbadge is a Stripe addon for Auth.js that simplifies the process of creating a SaaS site by integrating payment, authentication, gating, self-service account management, webhook handling, trials & free plans, session data, and more. It allows users to launch a SaaS app without writing any authentication or payment code. The project is open source and free to use with optional paid features under the BSL License.
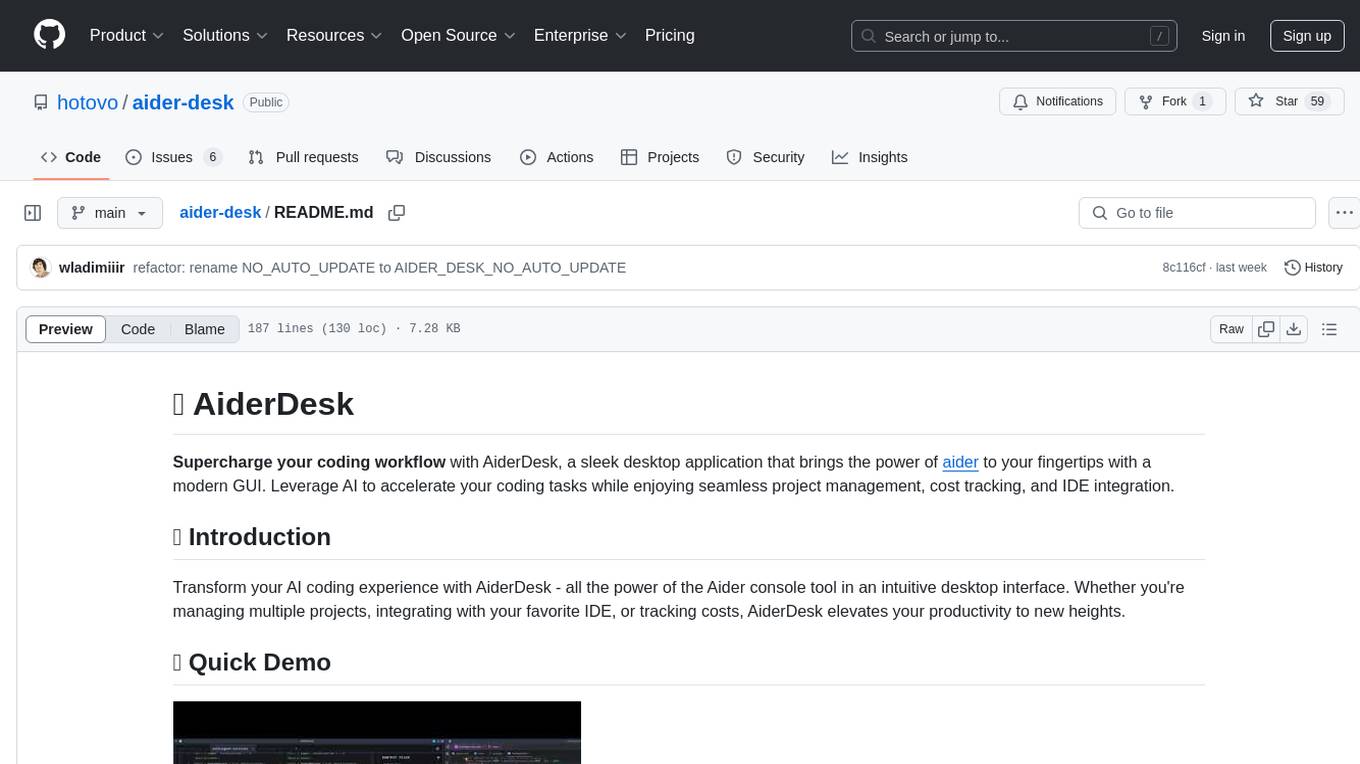
aider-desk
AiderDesk is a desktop application that enhances coding workflow by leveraging AI capabilities. It offers an intuitive GUI, project management, IDE integration, MCP support, settings management, cost tracking, structured messages, visual file management, model switching, code diff viewer, one-click reverts, and easy sharing. Users can install it by downloading the latest release and running the executable. AiderDesk also supports Python version detection and auto update disabling. It includes features like multiple project management, context file management, model switching, chat mode selection, question answering, cost tracking, MCP server integration, and MCP support for external tools and context. Development setup involves cloning the repository, installing dependencies, running in development mode, and building executables for different platforms. Contributions from the community are welcome following specific guidelines.
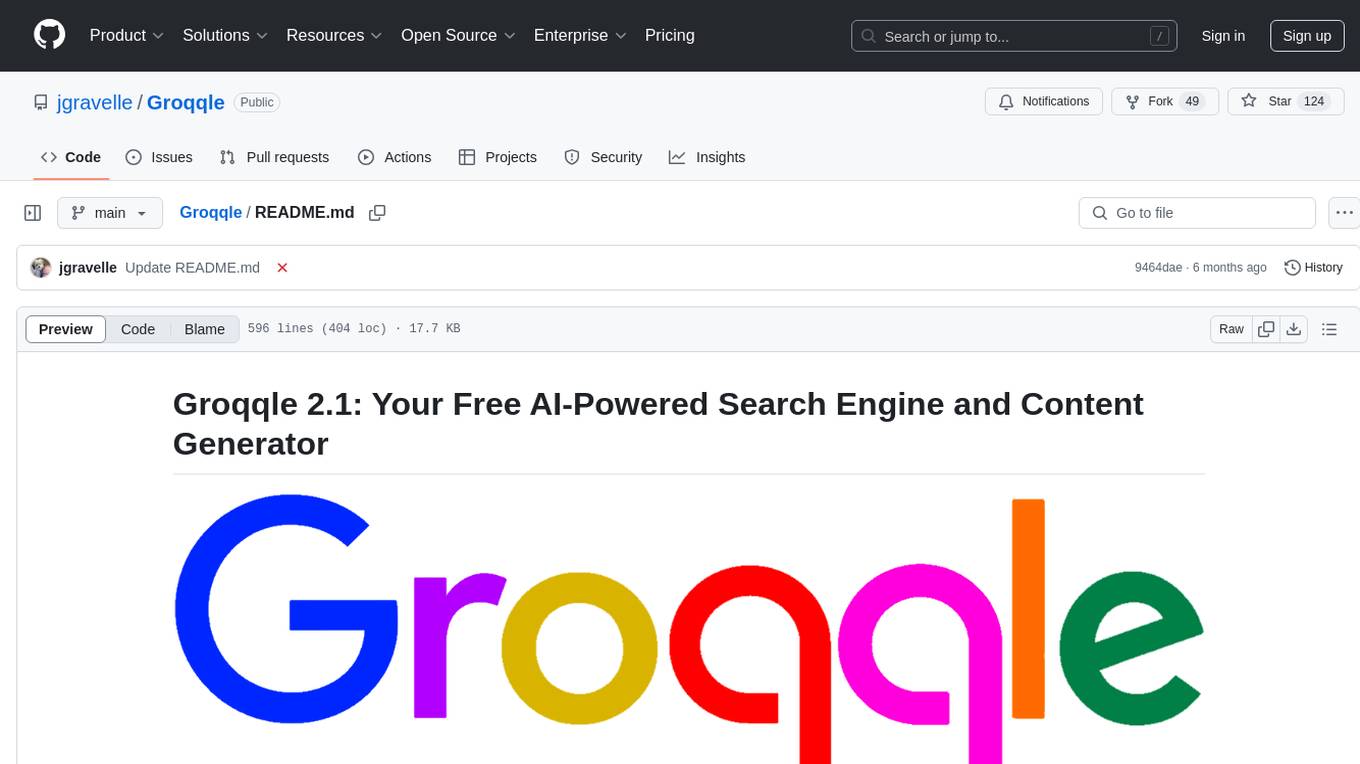
Groqqle
Groqqle 2.1 is a revolutionary, free AI web search and API that instantly returns ORIGINAL content derived from source articles, websites, videos, and even foreign language sources, for ANY target market of ANY reading comprehension level! It combines the power of large language models with advanced web and news search capabilities, offering a user-friendly web interface, a robust API, and now a powerful Groqqle_web_tool for seamless integration into your projects. Developers can instantly incorporate Groqqle into their applications, providing a powerful tool for content generation, research, and analysis across various domains and languages.
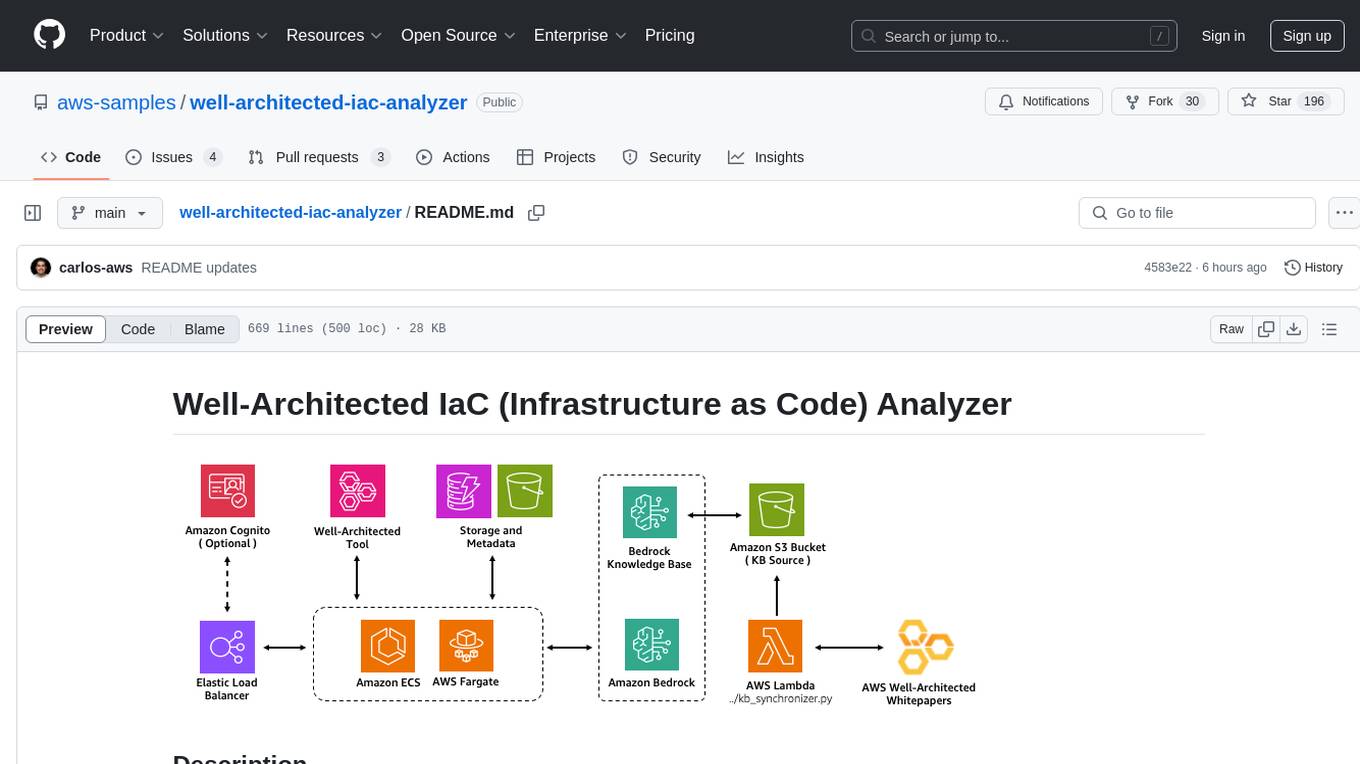
well-architected-iac-analyzer
Well-Architected Infrastructure as Code (IaC) Analyzer is a project demonstrating how generative AI can evaluate infrastructure code for alignment with best practices. It features a modern web application allowing users to upload IaC documents, complete IaC projects, or architecture diagrams for assessment. The tool provides insights into infrastructure code alignment with AWS best practices, offers suggestions for improving cloud architecture designs, and can generate IaC templates from architecture diagrams. Users can analyze CloudFormation, Terraform, or AWS CDK templates, architecture diagrams in PNG or JPEG format, and complete IaC projects with supporting documents. Real-time analysis against Well-Architected best practices, integration with AWS Well-Architected Tool, and export of analysis results and recommendations are included.
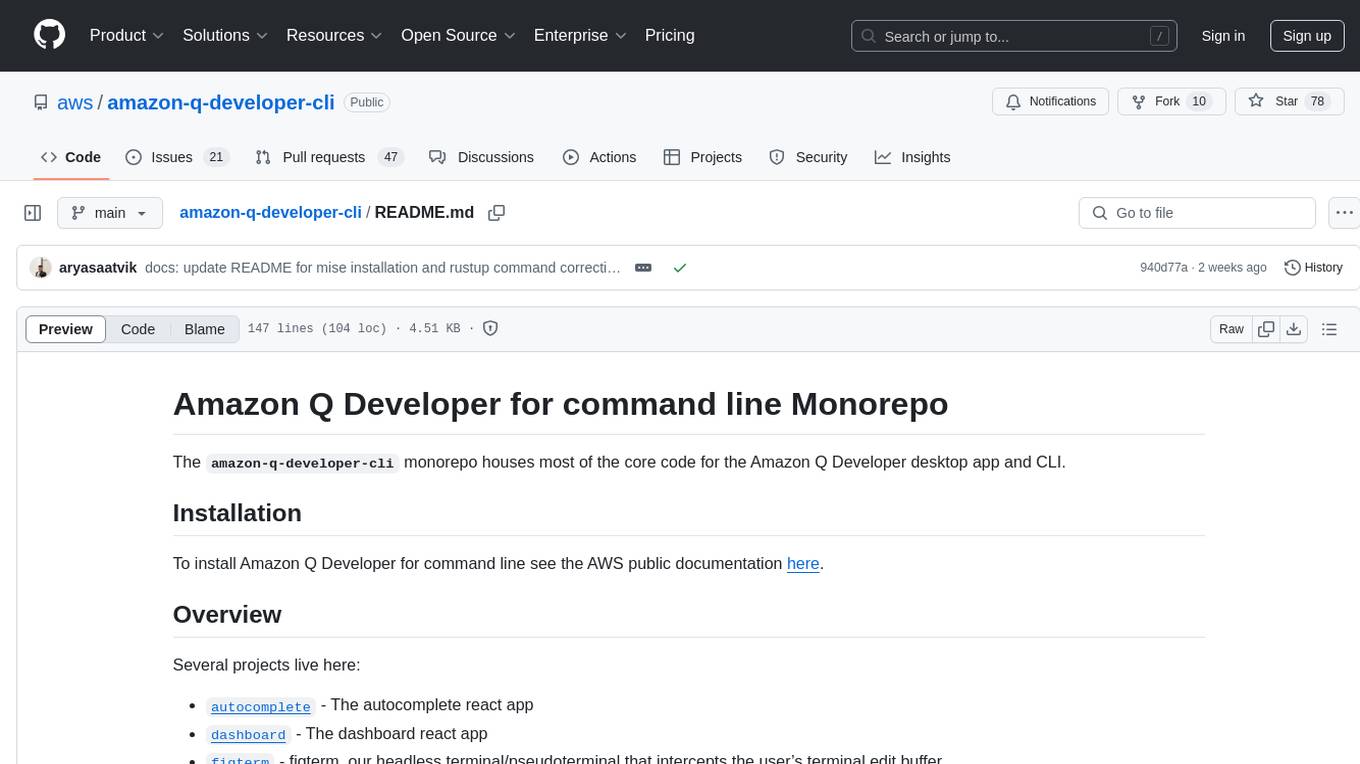
amazon-q-developer-cli
The `amazon-q-developer-cli` monorepo houses core code for the Amazon Q Developer desktop app and CLI. It includes projects like autocomplete, dashboard, figterm, q CLI, fig_desktop, fig_input_method, VSCode plugin, and JetBrains plugin. The repo also contains build scripts, internal rust crates, internal npm packages, protocol buffer message specification, and integration tests. The architecture involves different components communicating via IPC.
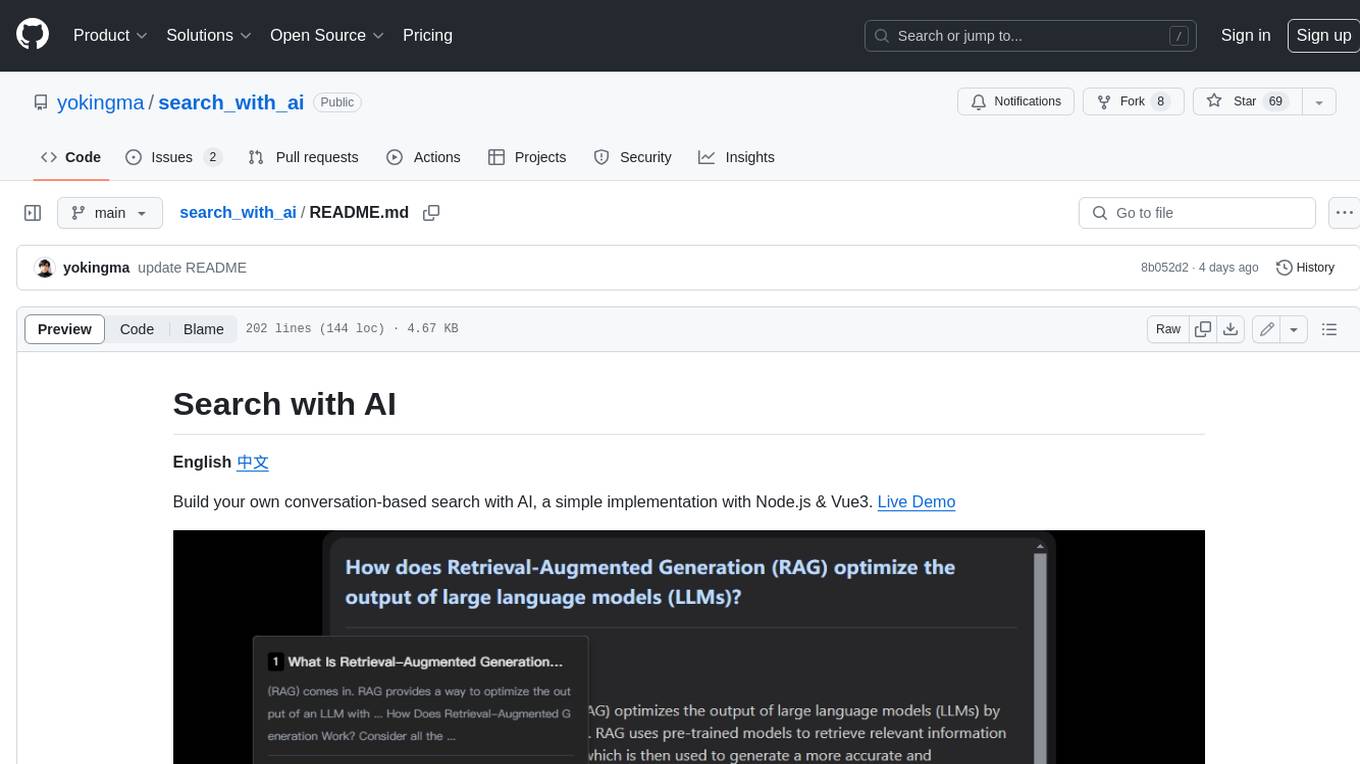
search_with_ai
Build your own conversation-based search with AI, a simple implementation with Node.js & Vue3. Live Demo Features: * Built-in support for LLM: OpenAI, Google, Lepton, Ollama(Free) * Built-in support for search engine: Bing, Sogou, Google, SearXNG(Free) * Customizable pretty UI interface * Support dark mode * Support mobile display * Support local LLM with Ollama * Support i18n * Support Continue Q&A with contexts.
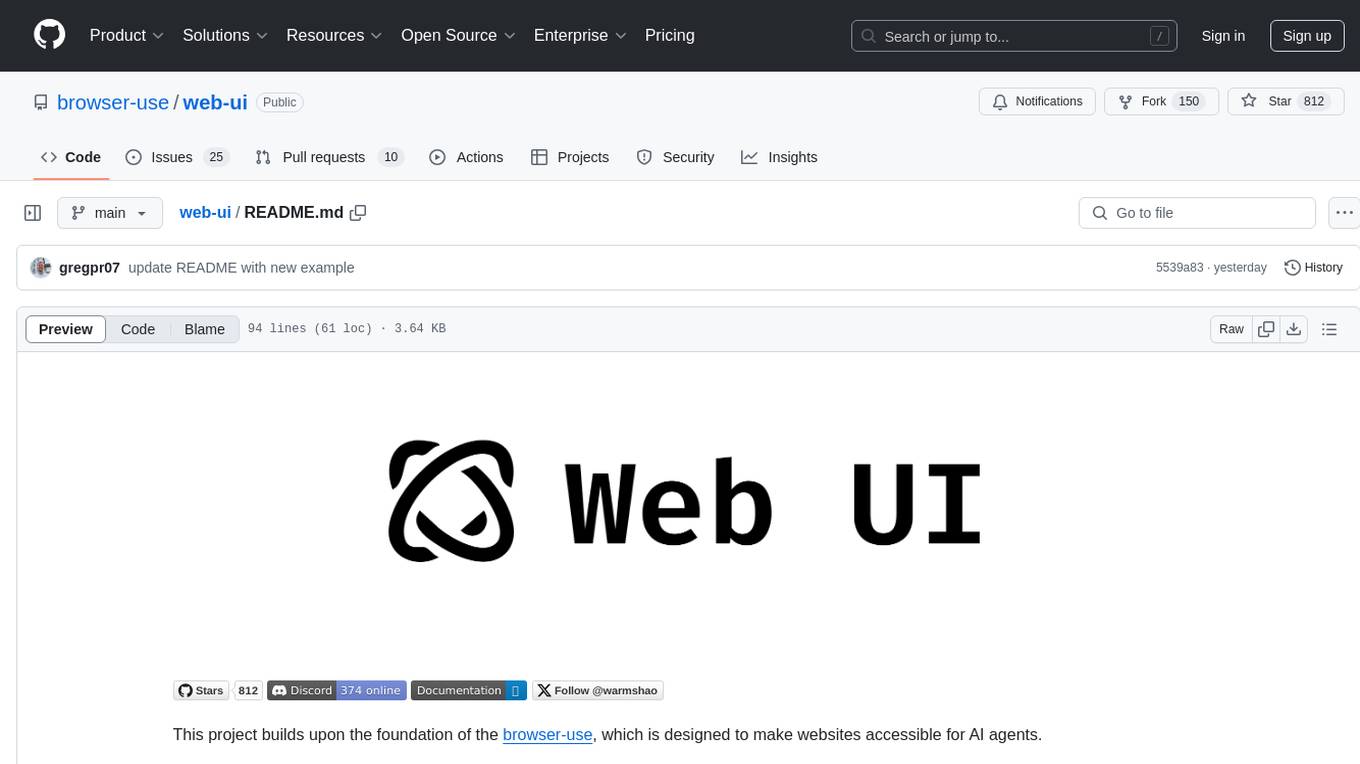
web-ui
WebUI is a user-friendly tool built on Gradio that enhances website accessibility for AI agents. It supports various Large Language Models (LLMs) and allows custom browser integration for seamless interaction. The tool eliminates the need for re-login and authentication challenges, offering high-definition screen recording capabilities.
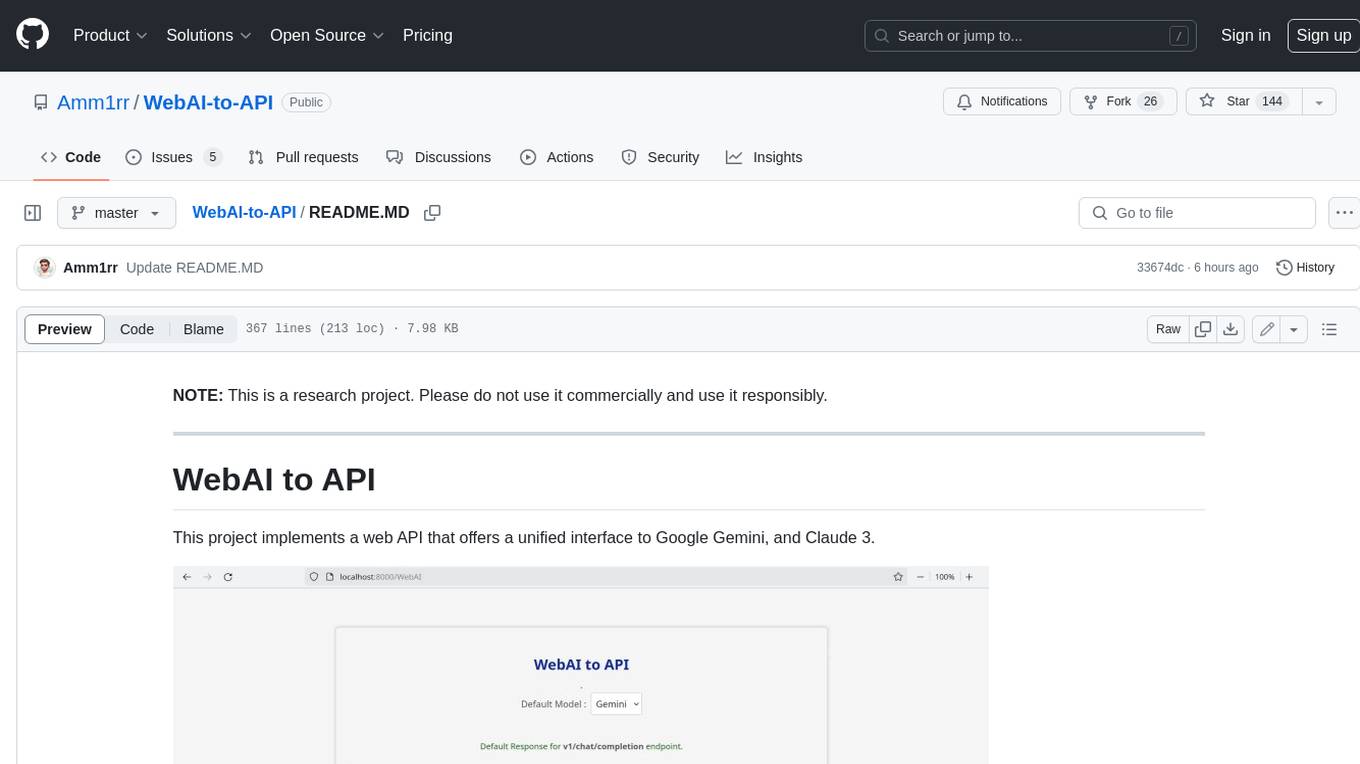
WebAI-to-API
This project implements a web API that offers a unified interface to Google Gemini and Claude 3. It provides a self-hosted, lightweight, and scalable solution for accessing these AI models through a streaming API. The API supports both Claude and Gemini models, allowing users to interact with them in real-time. The project includes a user-friendly web UI for configuration and documentation, making it easy to get started and explore the capabilities of the API.
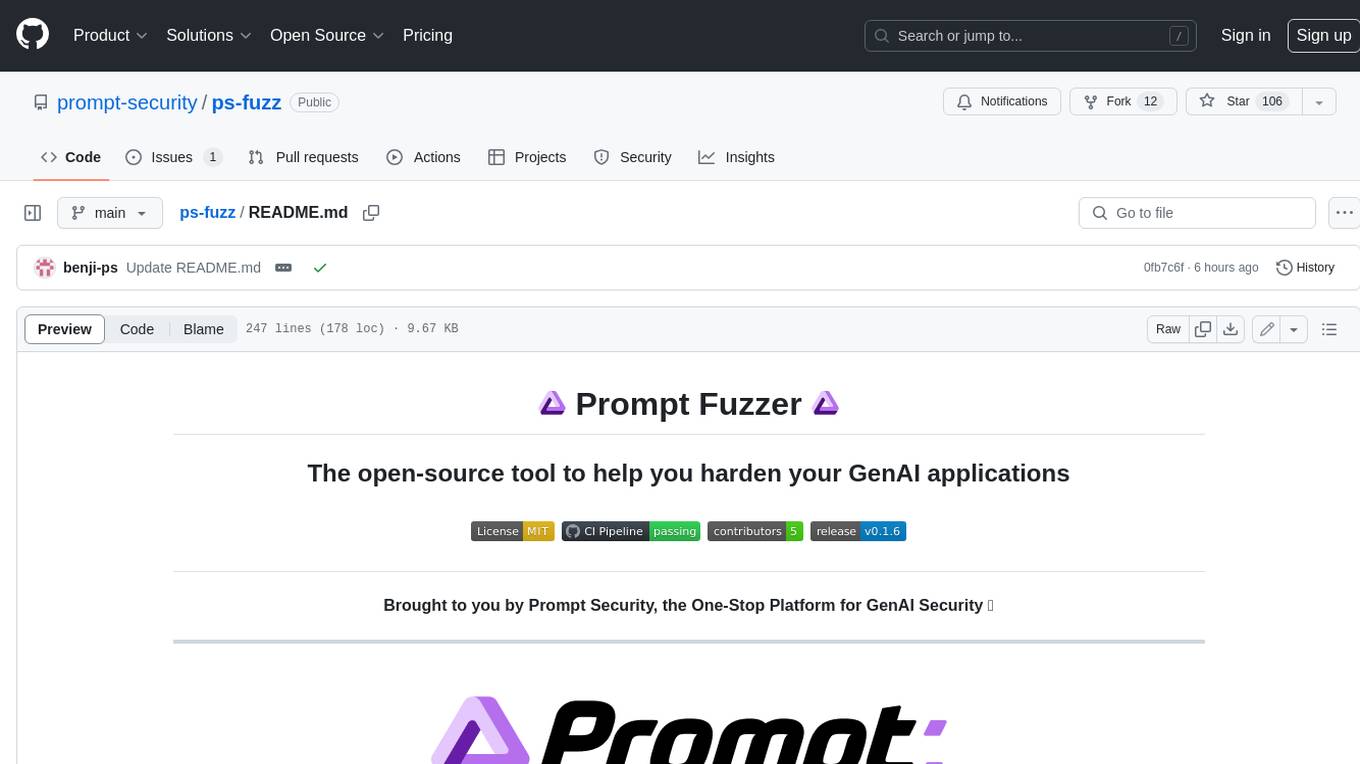
ps-fuzz
The Prompt Fuzzer is an open-source tool that helps you assess the security of your GenAI application's system prompt against various dynamic LLM-based attacks. It provides a security evaluation based on the outcome of these attack simulations, enabling you to strengthen your system prompt as needed. The Prompt Fuzzer dynamically tailors its tests to your application's unique configuration and domain. The Fuzzer also includes a Playground chat interface, giving you the chance to iteratively improve your system prompt, hardening it against a wide spectrum of generative AI attacks.
For similar tasks
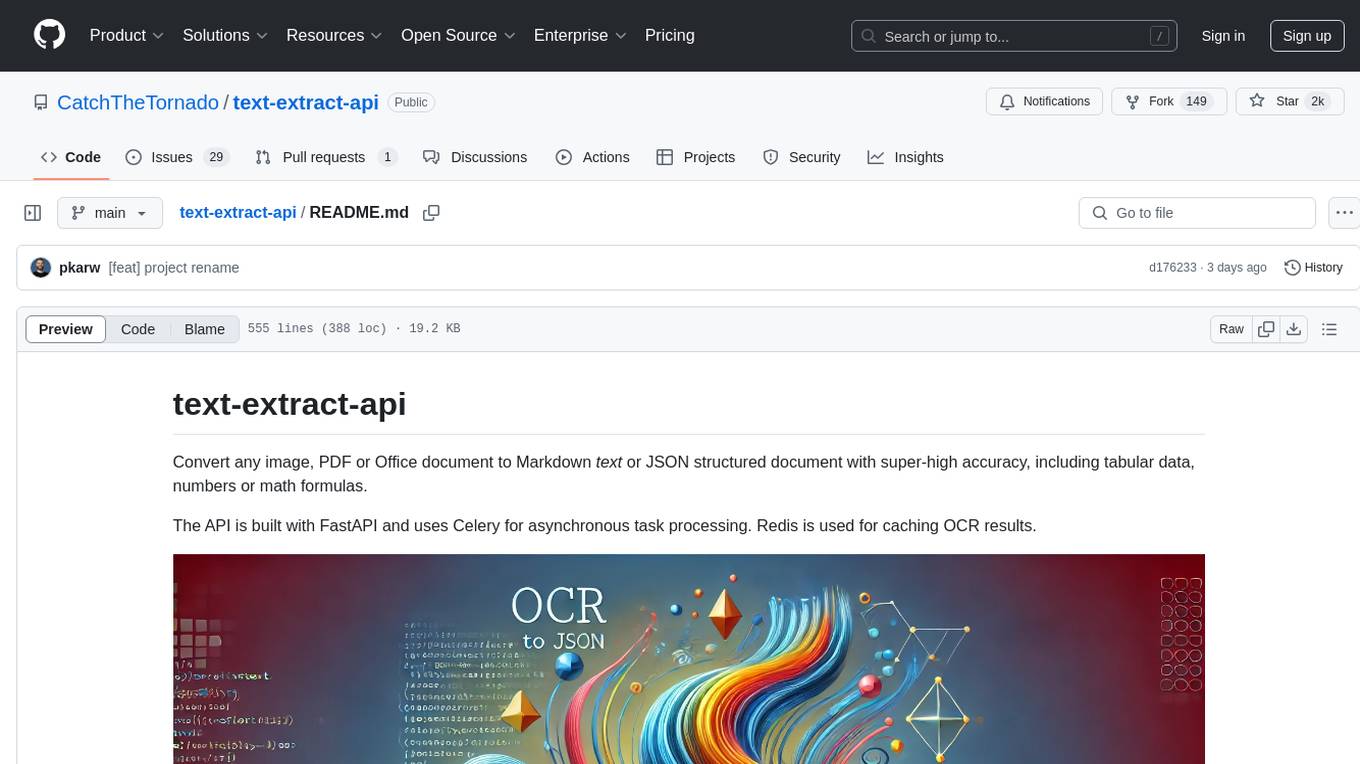
text-extract-api
The text-extract-api is a powerful tool that allows users to convert images, PDFs, or Office documents to Markdown text or JSON structured documents with high accuracy. It is built using FastAPI and utilizes Celery for asynchronous task processing, with Redis for caching OCR results. The tool provides features such as PDF/Office to Markdown and JSON conversion, improving OCR results with LLama, removing Personally Identifiable Information from documents, distributed queue processing, caching using Redis, switchable storage strategies, and a CLI tool for task management. Users can run the tool locally or on cloud services, with support for GPU processing. The tool also offers an online demo for testing purposes.
For similar jobs
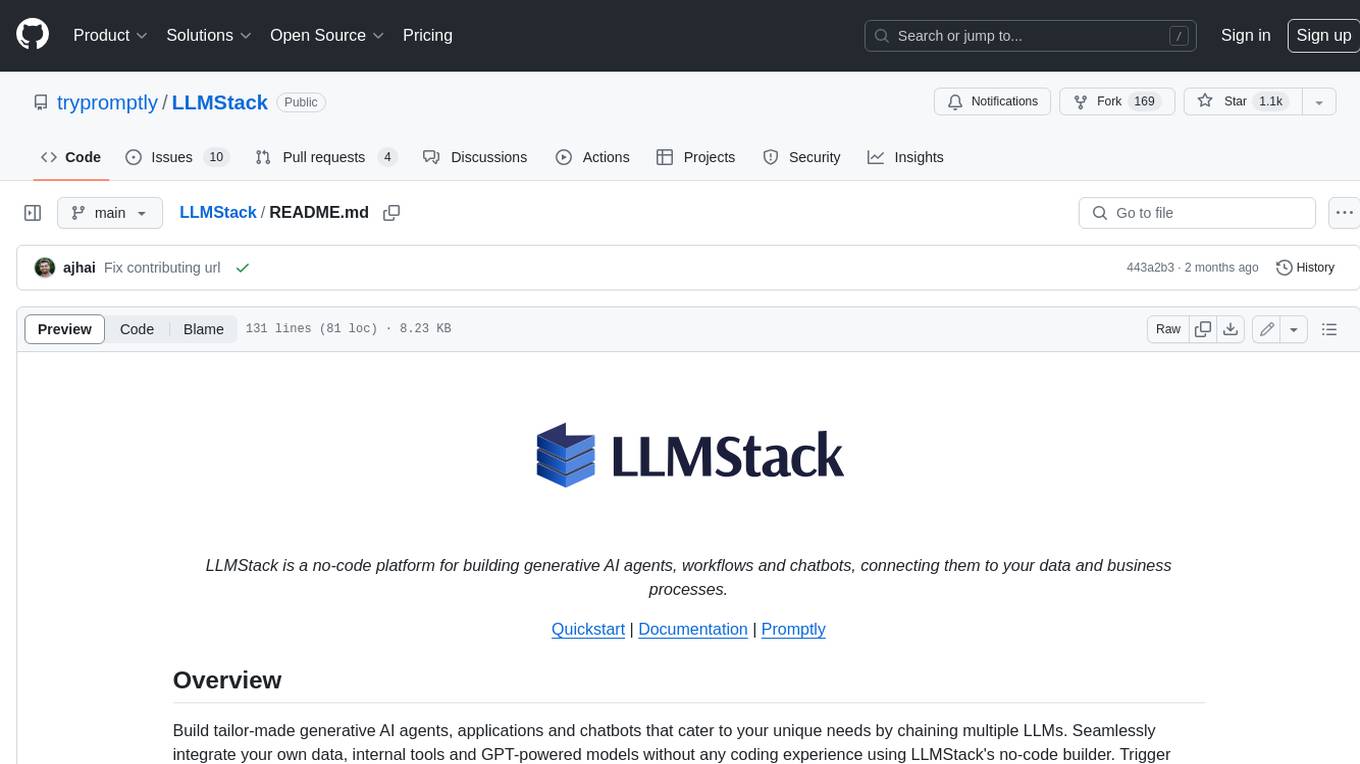
LLMStack
LLMStack is a no-code platform for building generative AI agents, workflows, and chatbots. It allows users to connect their own data, internal tools, and GPT-powered models without any coding experience. LLMStack can be deployed to the cloud or on-premise and can be accessed via HTTP API or triggered from Slack or Discord.
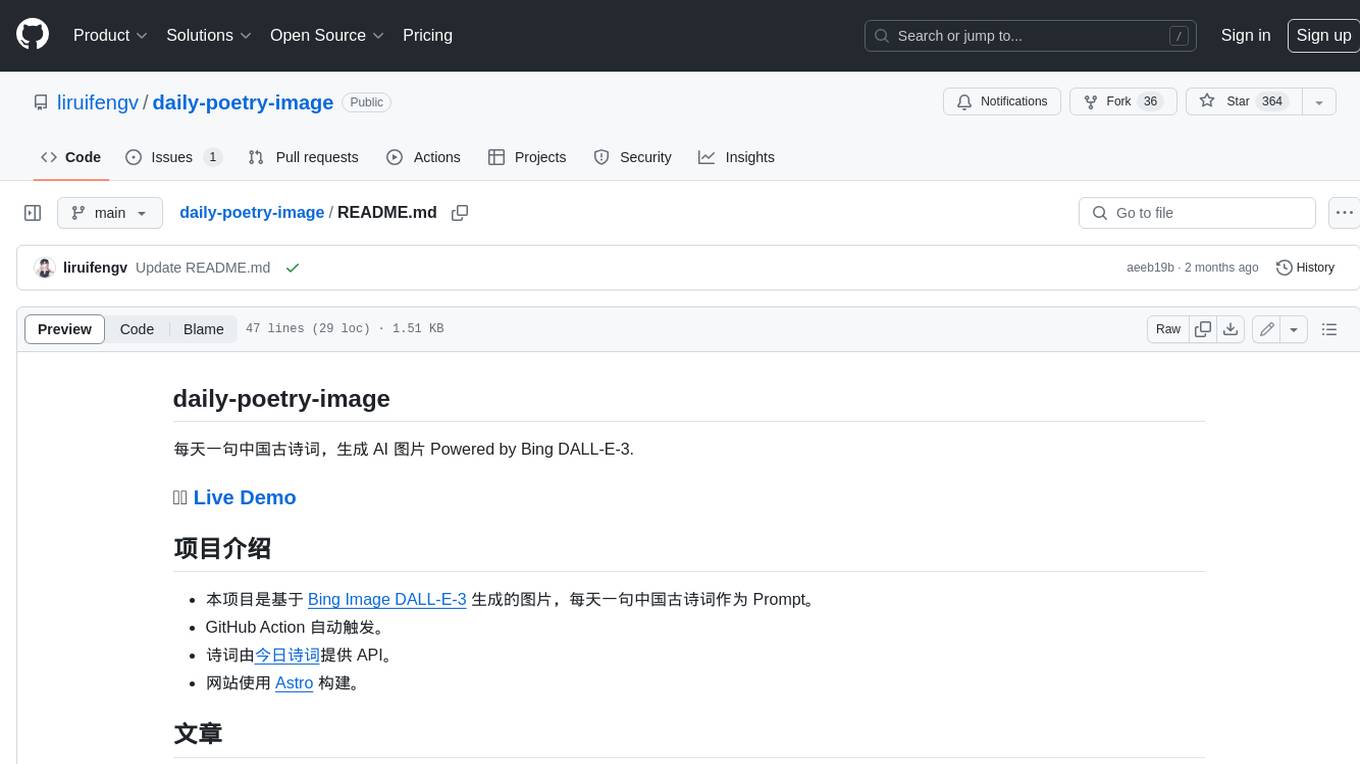
daily-poetry-image
Daily Chinese ancient poetry and AI-generated images powered by Bing DALL-E-3. GitHub Action triggers the process automatically. Poetry is provided by Today's Poem API. The website is built with Astro.
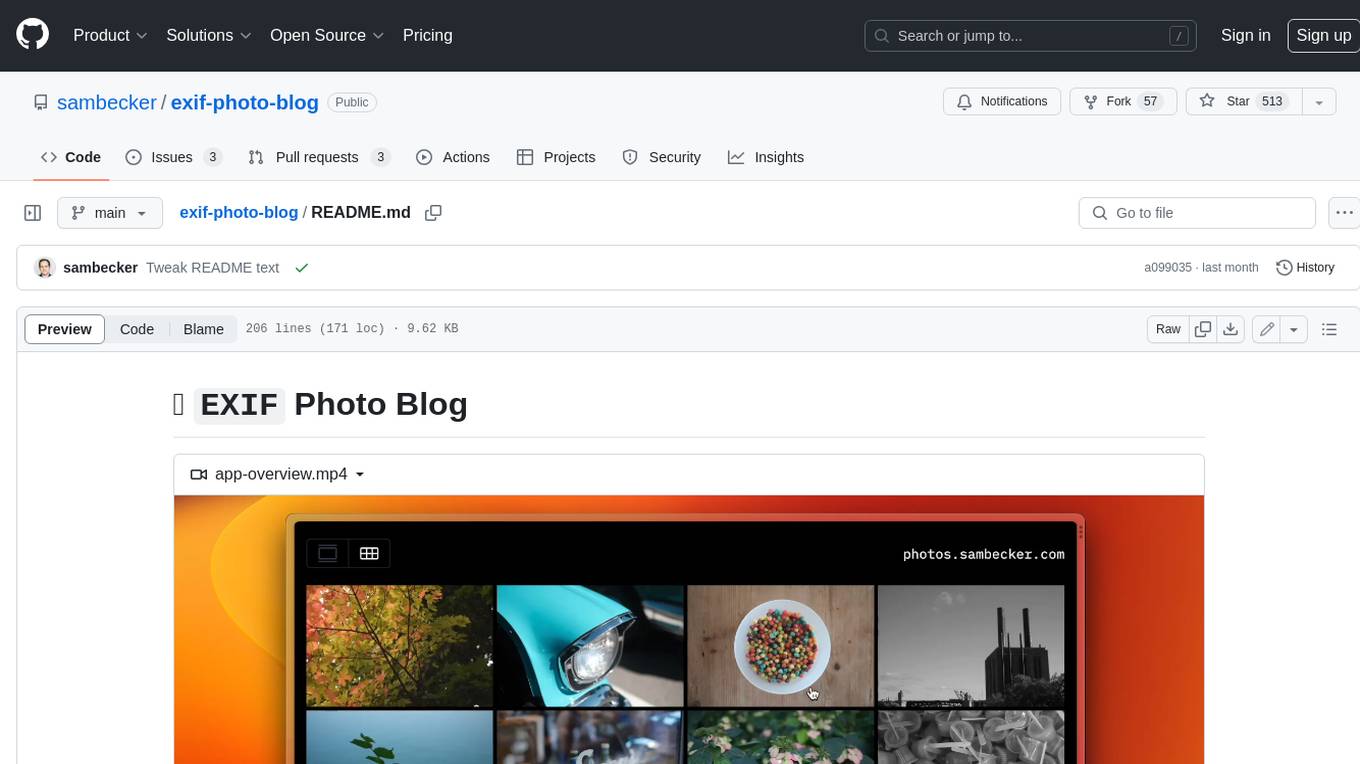
exif-photo-blog
EXIF Photo Blog is a full-stack photo blog application built with Next.js, Vercel, and Postgres. It features built-in authentication, photo upload with EXIF extraction, photo organization by tag, infinite scroll, light/dark mode, automatic OG image generation, a CMD-K menu with photo search, experimental support for AI-generated descriptions, and support for Fujifilm simulations. The application is easy to deploy to Vercel with just a few clicks and can be customized with a variety of environment variables.
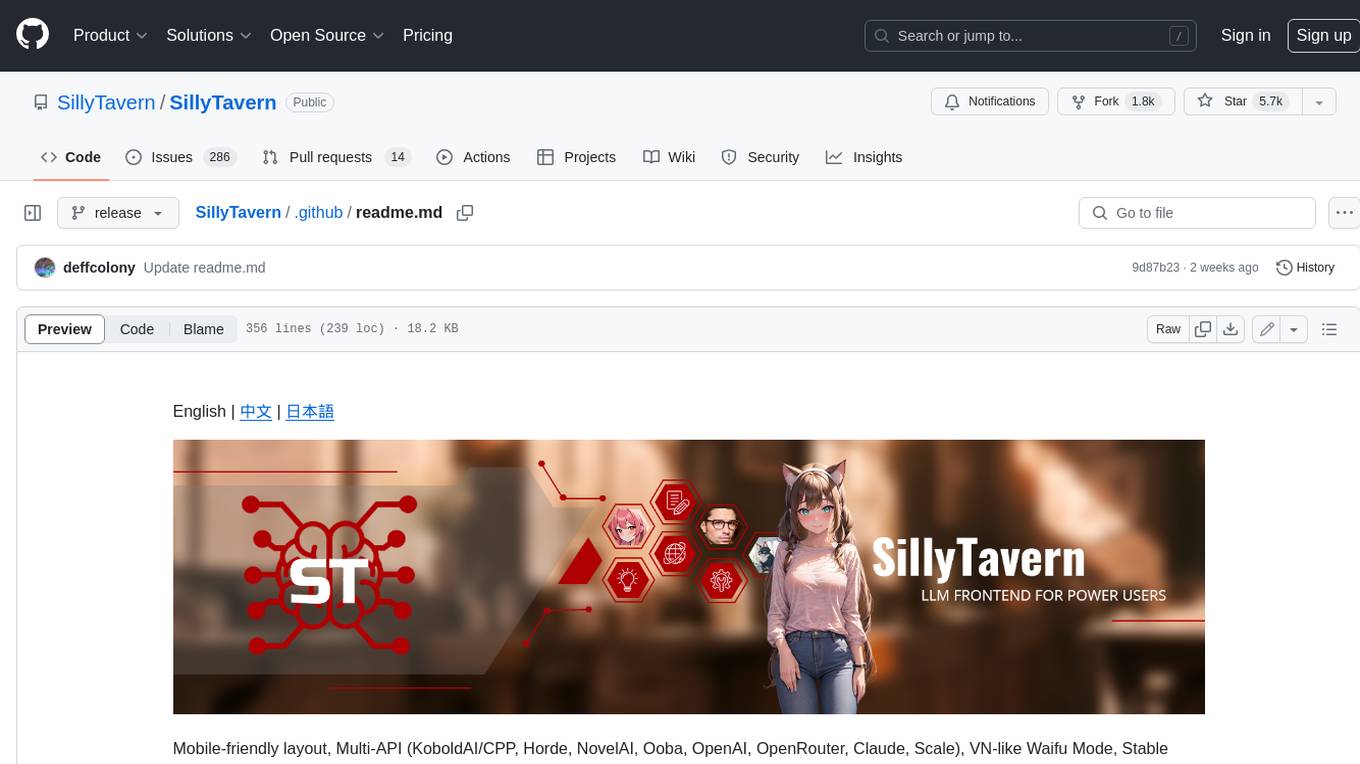
SillyTavern
SillyTavern is a user interface you can install on your computer (and Android phones) that allows you to interact with text generation AIs and chat/roleplay with characters you or the community create. SillyTavern is a fork of TavernAI 1.2.8 which is under more active development and has added many major features. At this point, they can be thought of as completely independent programs.
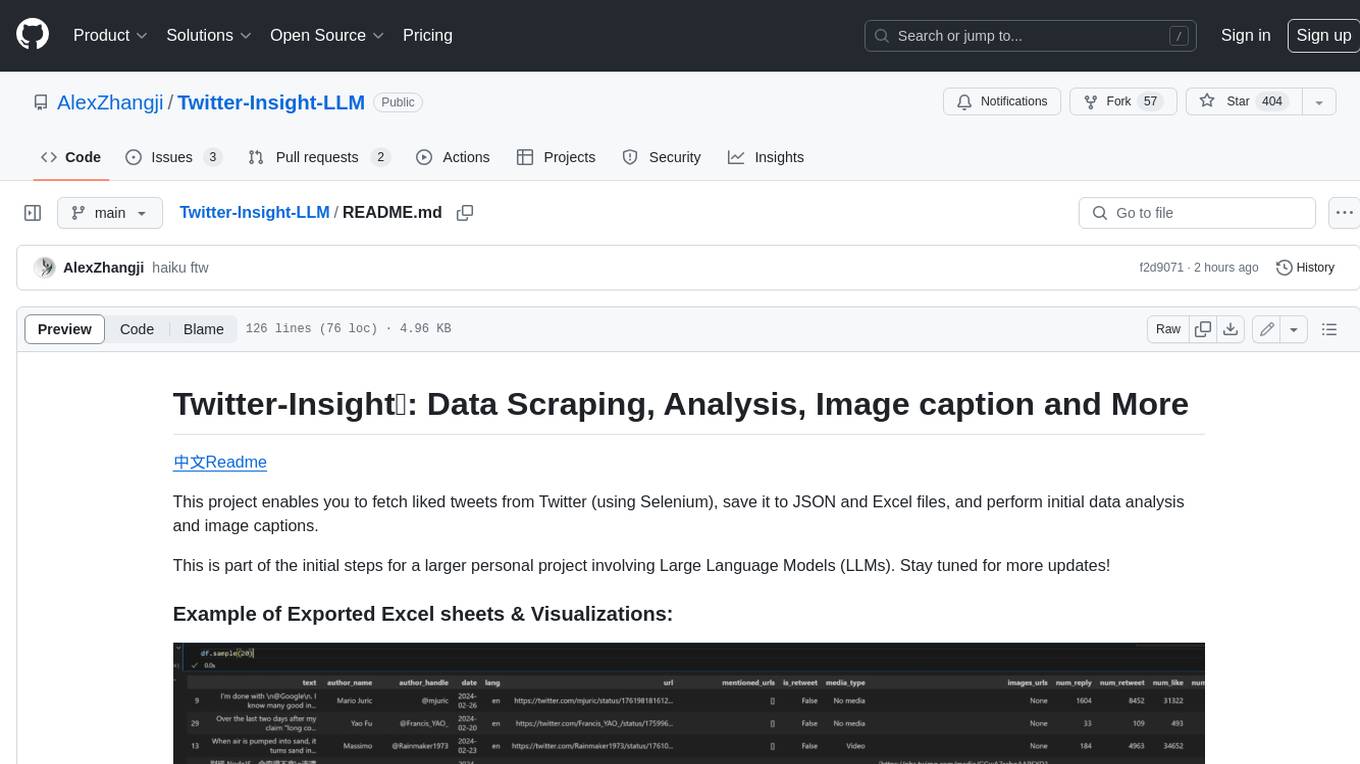
Twitter-Insight-LLM
This project enables you to fetch liked tweets from Twitter (using Selenium), save it to JSON and Excel files, and perform initial data analysis and image captions. This is part of the initial steps for a larger personal project involving Large Language Models (LLMs).
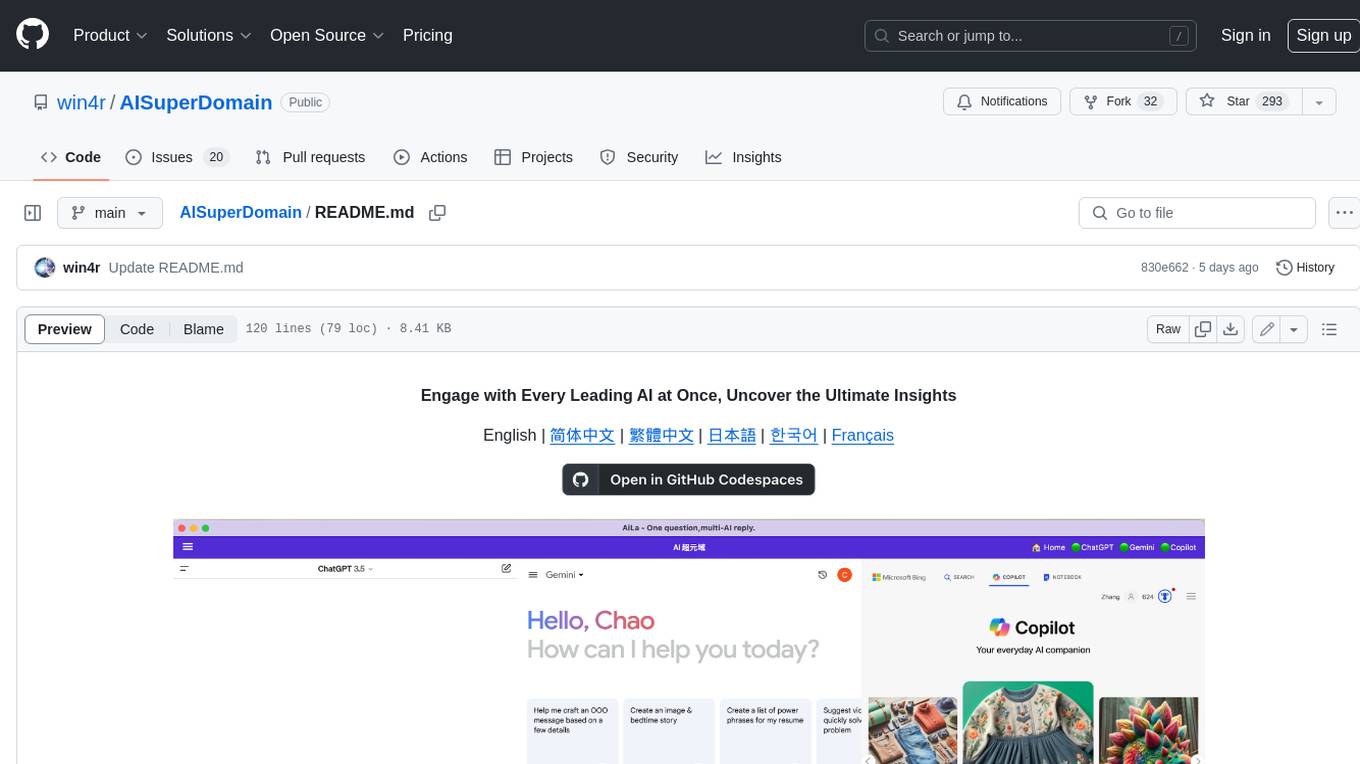
AISuperDomain
Aila Desktop Application is a powerful tool that integrates multiple leading AI models into a single desktop application. It allows users to interact with various AI models simultaneously, providing diverse responses and insights to their inquiries. With its user-friendly interface and customizable features, Aila empowers users to engage with AI seamlessly and efficiently. Whether you're a researcher, student, or professional, Aila can enhance your AI interactions and streamline your workflow.
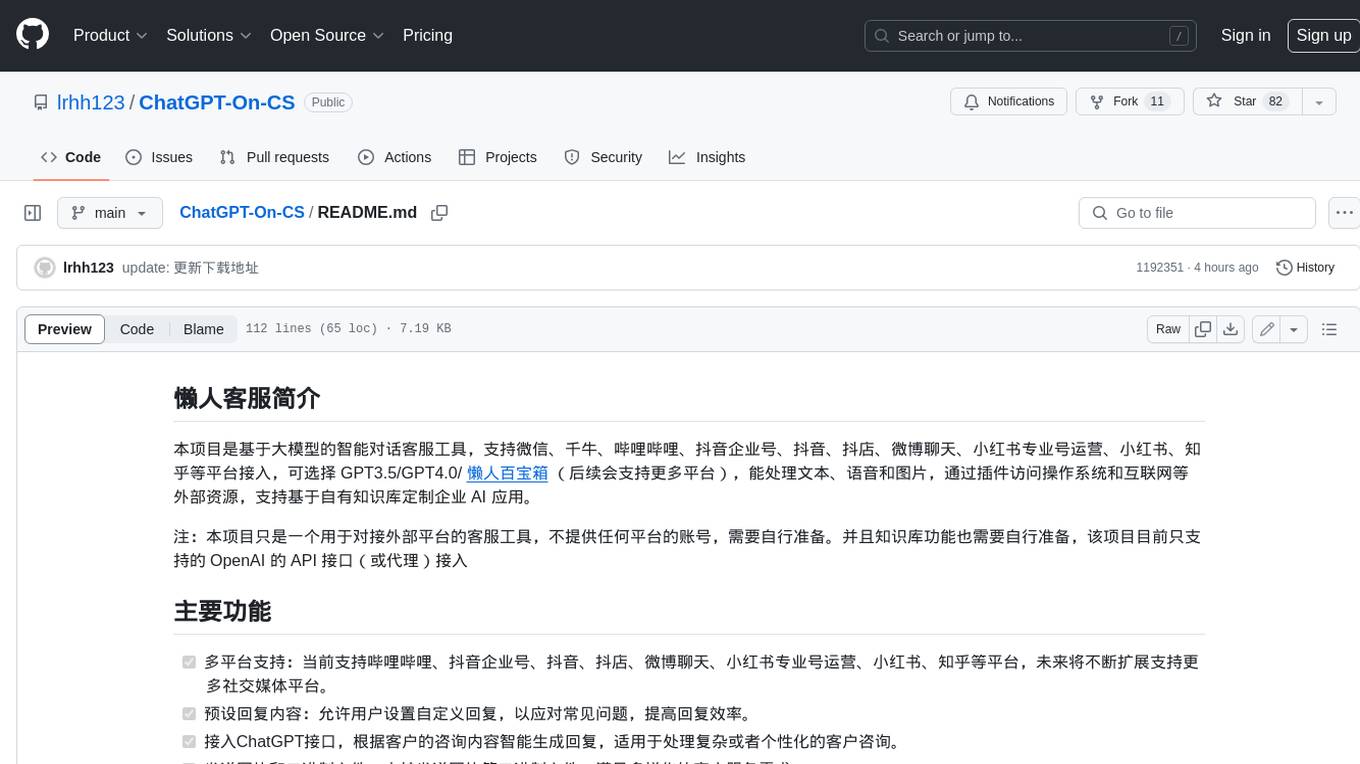
ChatGPT-On-CS
This project is an intelligent dialogue customer service tool based on a large model, which supports access to platforms such as WeChat, Qianniu, Bilibili, Douyin Enterprise, Douyin, Doudian, Weibo chat, Xiaohongshu professional account operation, Xiaohongshu, Zhihu, etc. You can choose GPT3.5/GPT4.0/ Lazy Treasure Box (more platforms will be supported in the future), which can process text, voice and pictures, and access external resources such as operating systems and the Internet through plug-ins, and support enterprise AI applications customized based on their own knowledge base.
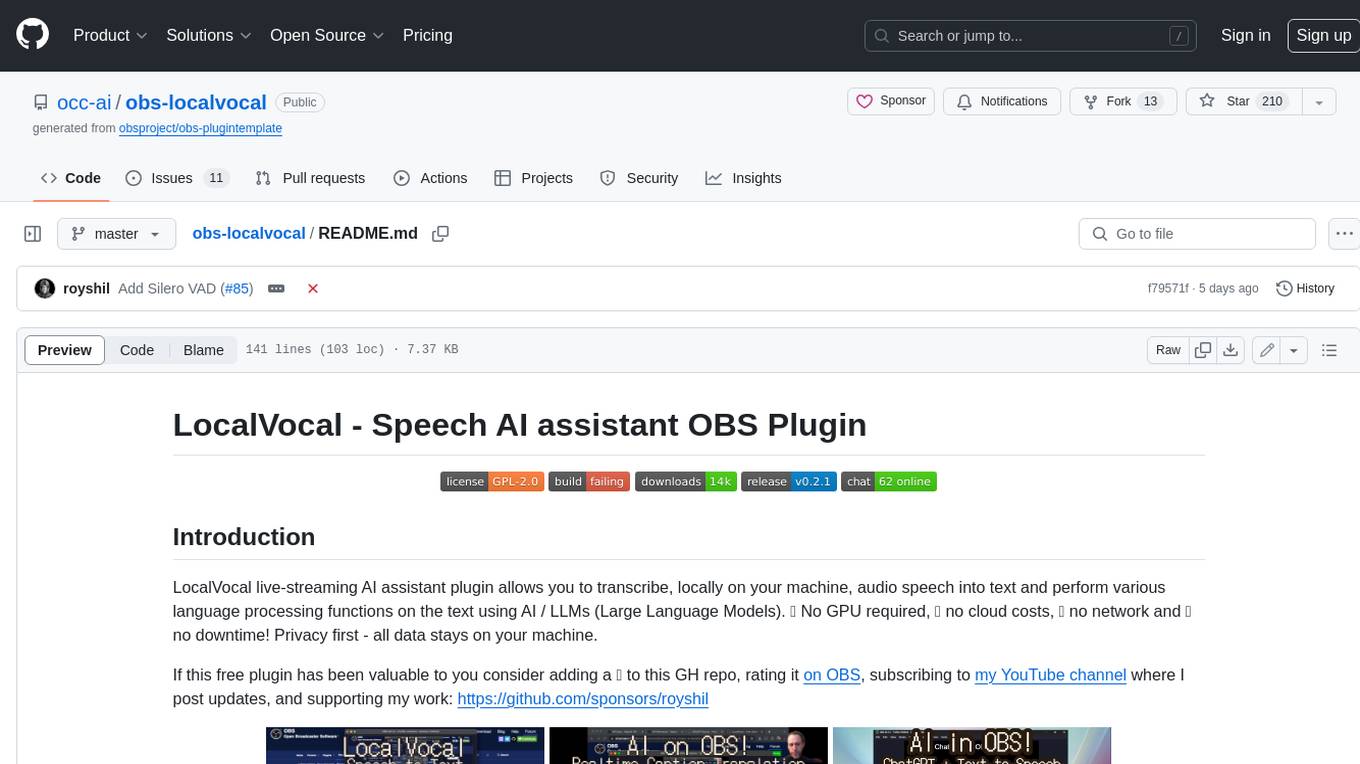
obs-localvocal
LocalVocal is a live-streaming AI assistant plugin for OBS that allows you to transcribe audio speech into text and perform various language processing functions on the text using AI / LLMs (Large Language Models). It's privacy-first, with all data staying on your machine, and requires no GPU, cloud costs, network, or downtime.