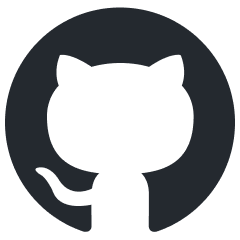
dbt-llm-agent
RAG based LLM chatbot for dbt projects
Stars: 76
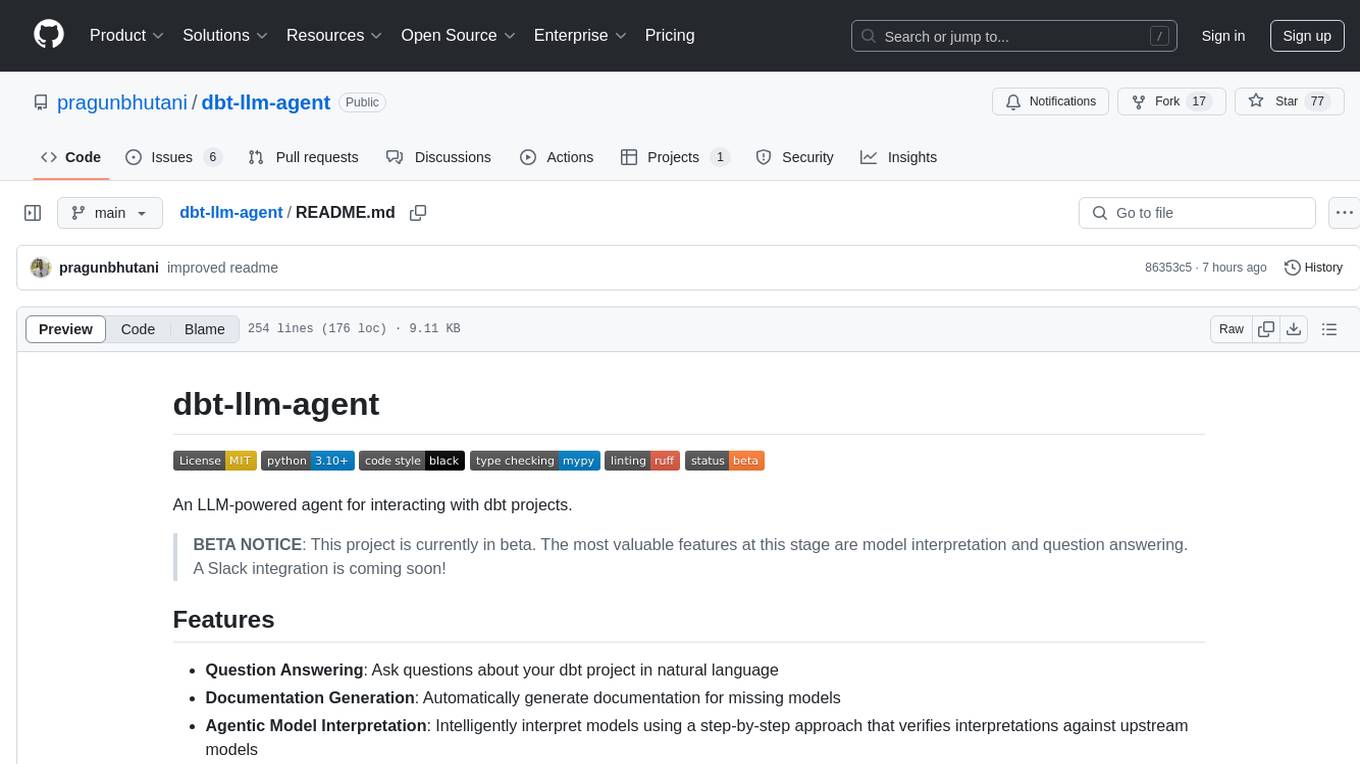
README:
An LLM-powered agent for interacting with dbt projects.
BETA NOTICE: This project is currently in beta. The most valuable features at this stage are model interpretation and question answering. A Slack integration is coming soon!
- Question Answering: Ask questions about your dbt project in natural language
- Documentation Generation: Automatically generate documentation for missing models
- Agentic Model Interpretation: Intelligently interpret models using a step-by-step approach that verifies interpretations against upstream models
- Postgres with pgvector: Store model embeddings in Postgres using pgvector (supports Supabase)
- dbt Model Selection: Use dbt's model selection syntax to specify which models to work with
- Question Tracking: Track questions, answers, and feedback for continuous improvement
- Coming Soon: Slack Integration: Ask questions and receive answers directly in Slack
The agent uses a combination of:
- dbt Project Parsing: Extract information from your dbt project including models, sources, and documentation
- PostgreSQL with pgvector: Store both structured metadata and vector embeddings for semantic search
- Model Selection: Selectively parse and embed models using dbt's selection syntax
- LLM Integration: Use large language models (like GPT-4) to generate responses and documentation
- Question Tracking: Store a history of questions, answers, and user feedback
-
Check Python Version: This project requires Python 3.10 or higher. You can check your Python version with:
python --version # or python3 --version
If you need to upgrade or install Python 3.10+, visit python.org/downloads.
-
Clone the repository:
git clone https://github.com/pragunbhutani/dbt-llm-agent.git cd dbt-llm-agent
-
Install dependencies: This project uses Poetry for dependency management.
# Install Poetry if you don't have it curl -sSL https://install.python-poetry.org | python3 - # Install dependencies poetry install
-
Set up PostgreSQL: You need a PostgreSQL database (version 11+) with the
pgvector
extension enabled. This database will store model metadata, embeddings, and question history.- Install PostgreSQL if you haven't already.
- Install
pgvector
. Follow the instructions at https://github.com/pgvector/pgvector. - Create a database for the agent (e.g.,
dbt_llm_agent
).
Quick setup commands for local PostgreSQL:
# Create database createdb dbt_llm_agent # Enable pgvector extension (run this in psql) psql -d dbt_llm_agent -c 'CREATE EXTENSION IF NOT EXISTS vector;'
-
Configure environment variables: Copy the example environment file and fill in your details:
cp .env.example .env
Edit the
.env
file with your:OPENAI_API_KEY
-
POSTGRES_URI
(database connection string) - dbt Cloud credentials (
DBT_CLOUD_...
) if usinginit cloud
. -
DBT_PROJECT_PATH
if usinginit local
orinit source
and not providing the path as an argument.
-
Initialize the database schema: Run the following command. This creates the necessary tables and enables the
pgvector
extension if needed.poetry run dbt-llm-agent init-db
To use the agent, you first need to load your dbt project's metadata into the database. Use the init
command:
poetry run dbt-llm-agent init <mode> [options]
There are three modes available:
Fetches the manifest.json
from the latest successful run in your dbt Cloud account. This provides the richest metadata, including compiled SQL.
-
Command:
poetry run dbt-llm-agent init cloud
-
Prerequisites:
- dbt Cloud account with successful job runs that generate artifacts.
- Environment variables set in
.env
:DBT_CLOUD_URL
DBT_CLOUD_ACCOUNT_ID
-
DBT_CLOUD_API_KEY
(User Token or Service Token)
-
Example:
# Ensure DBT_CLOUD_URL, DBT_CLOUD_ACCOUNT_ID, DBT_CLOUD_API_KEY are in .env poetry run dbt-llm-agent init cloud
Runs dbt compile
on your local dbt project and parses the generated manifest.json
from the target/
directory. Also provides rich metadata including compiled SQL.
-
Command:
poetry run dbt-llm-agent init local --project-path /path/to/your/dbt/project
-
Prerequisites:
- dbt project configured locally (
dbt_project.yml
,profiles.yml
etc.). - Ability to run
dbt compile
successfully in the project directory. - The dbt project path can be provided via the
--project-path
argument or theDBT_PROJECT_PATH
environment variable.
- dbt project configured locally (
-
Example:
# Using argument poetry run dbt-llm-agent init local --project-path /Users/me/code/my_dbt_project # Using environment variable (set DBT_PROJECT_PATH in .env) poetry run dbt-llm-agent init local
Parses your dbt project directly from the source .sql
and .yml
files. This mode does not capture compiled SQL or reliably determine data types.
-
Command:
poetry run dbt-llm-agent init source /path/to/your/dbt/project
-
Prerequisites:
- Access to the dbt project source code.
- The dbt project path can be provided via the argument or the
DBT_PROJECT_PATH
environment variable.
-
Example:
# Using argument poetry run dbt-llm-agent init source /Users/me/code/my_dbt_project # Using environment variable poetry run dbt-llm-agent init source
Note: The init
command replaces the older parse
command for loading project metadata.
You only need to run init
once initially, or again if your dbt project structure changes significantly. Use the --force
flag with init
to overwrite existing models in the database.
Once you've completed the setup and initialization, you've got the basics sorted! Now you can start using the agent's main features:
There are two main paths depending on whether your models already have documentation:
Generate vector embeddings for semantic search to enable question answering:
# Embed all models
poetry run dbt-llm-agent embed --select "*"
# Or embed specific models or tags
poetry run dbt-llm-agent embed --select "+tag:marts"
poetry run dbt-llm-agent embed --select "my_model"
First, use the LLM to interpret and generate descriptions for models and columns:
# Interpret a specific model and save the results
poetry run dbt-llm-agent interpret --select "fct_orders" --save
# Interpret all models in the staging layer, save, and embed
poetry run dbt-llm-agent interpret --select "tag:staging" --save --embed
The --save
flag stores the interpretations in the database, and --embed
automatically generates embeddings after interpretation.
Now that your models are embedded, you can ask questions about your dbt project:
poetry run dbt-llm-agent ask "What models are tagged as finance?"
poetry run dbt-llm-agent ask "Show me the columns in the customers model"
poetry run dbt-llm-agent ask "Explain the fct_orders model"
poetry run dbt-llm-agent ask "How is discount_amount calculated in the orders model?"
Help improve the agent by providing feedback on answers:
# List previous questions
poetry run dbt-llm-agent questions
# Provide positive feedback
poetry run dbt-llm-agent feedback 1 --useful
# Provide negative feedback with explanation
poetry run dbt-llm-agent feedback 2 --not-useful --text "Use this_other_model instead"
# Just provide text feedback without marking useful/not useful
poetry run dbt-llm-agent feedback 3 --text "This answer is correct but too verbose."
This feedback helps the agent improve its answers over time.
# List all models in your project
poetry run dbt-llm-agent list
# Get detailed information about a specific model
poetry run dbt-llm-agent model-details my_model_name
Contributions are welcome! Please follow standard fork-and-pull-request workflow.
For Tasks:
Click tags to check more tools for each tasksFor Jobs:
Alternative AI tools for dbt-llm-agent
Similar Open Source Tools
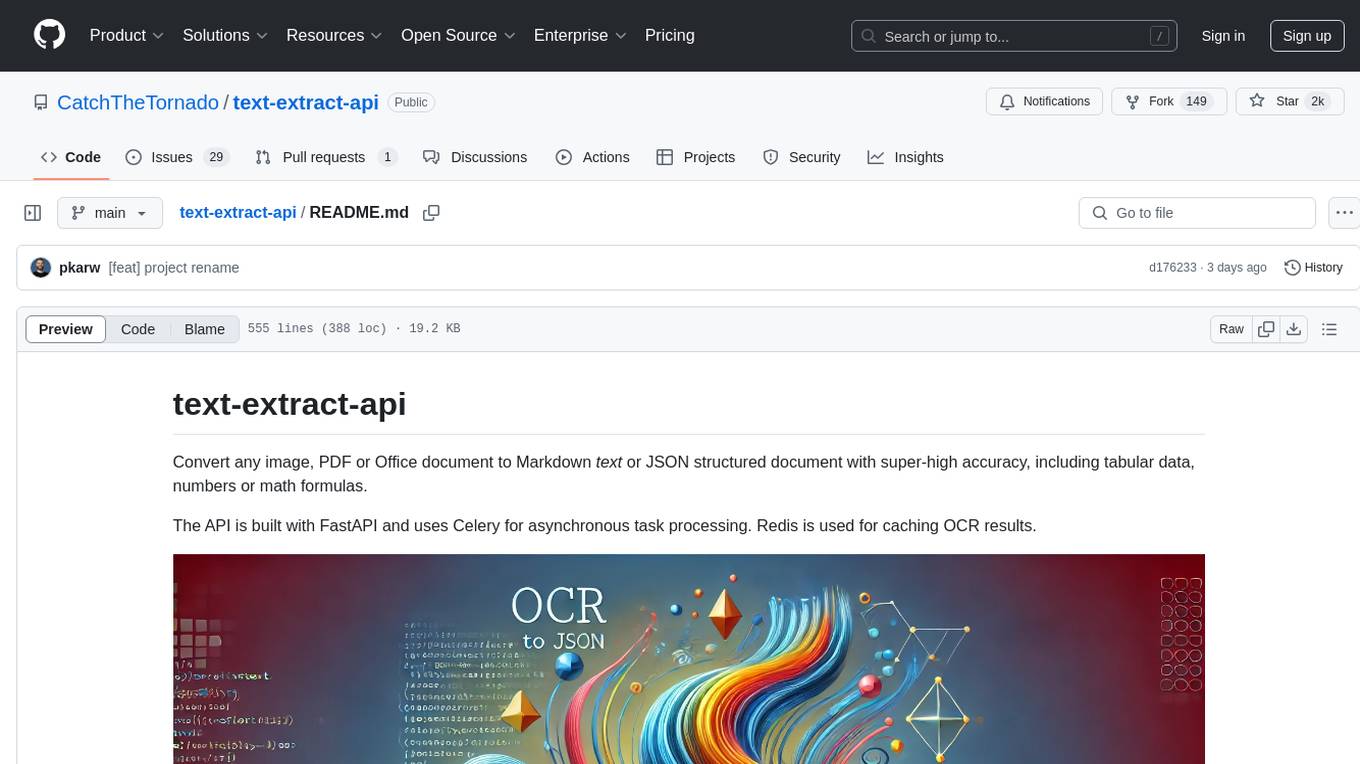
text-extract-api
The text-extract-api is a powerful tool that allows users to convert images, PDFs, or Office documents to Markdown text or JSON structured documents with high accuracy. It is built using FastAPI and utilizes Celery for asynchronous task processing, with Redis for caching OCR results. The tool provides features such as PDF/Office to Markdown and JSON conversion, improving OCR results with LLama, removing Personally Identifiable Information from documents, distributed queue processing, caching using Redis, switchable storage strategies, and a CLI tool for task management. Users can run the tool locally or on cloud services, with support for GPU processing. The tool also offers an online demo for testing purposes.
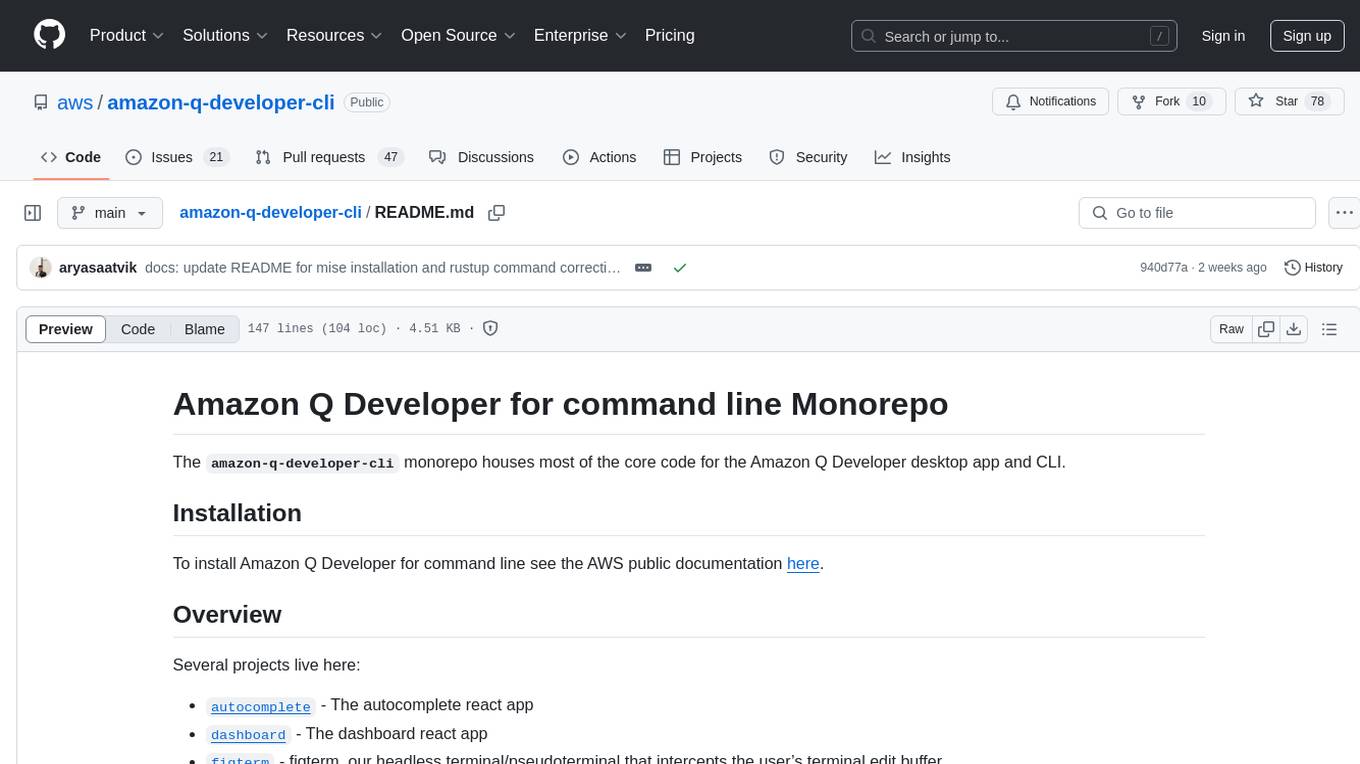
amazon-q-developer-cli
The `amazon-q-developer-cli` monorepo houses core code for the Amazon Q Developer desktop app and CLI. It includes projects like autocomplete, dashboard, figterm, q CLI, fig_desktop, fig_input_method, VSCode plugin, and JetBrains plugin. The repo also contains build scripts, internal rust crates, internal npm packages, protocol buffer message specification, and integration tests. The architecture involves different components communicating via IPC.
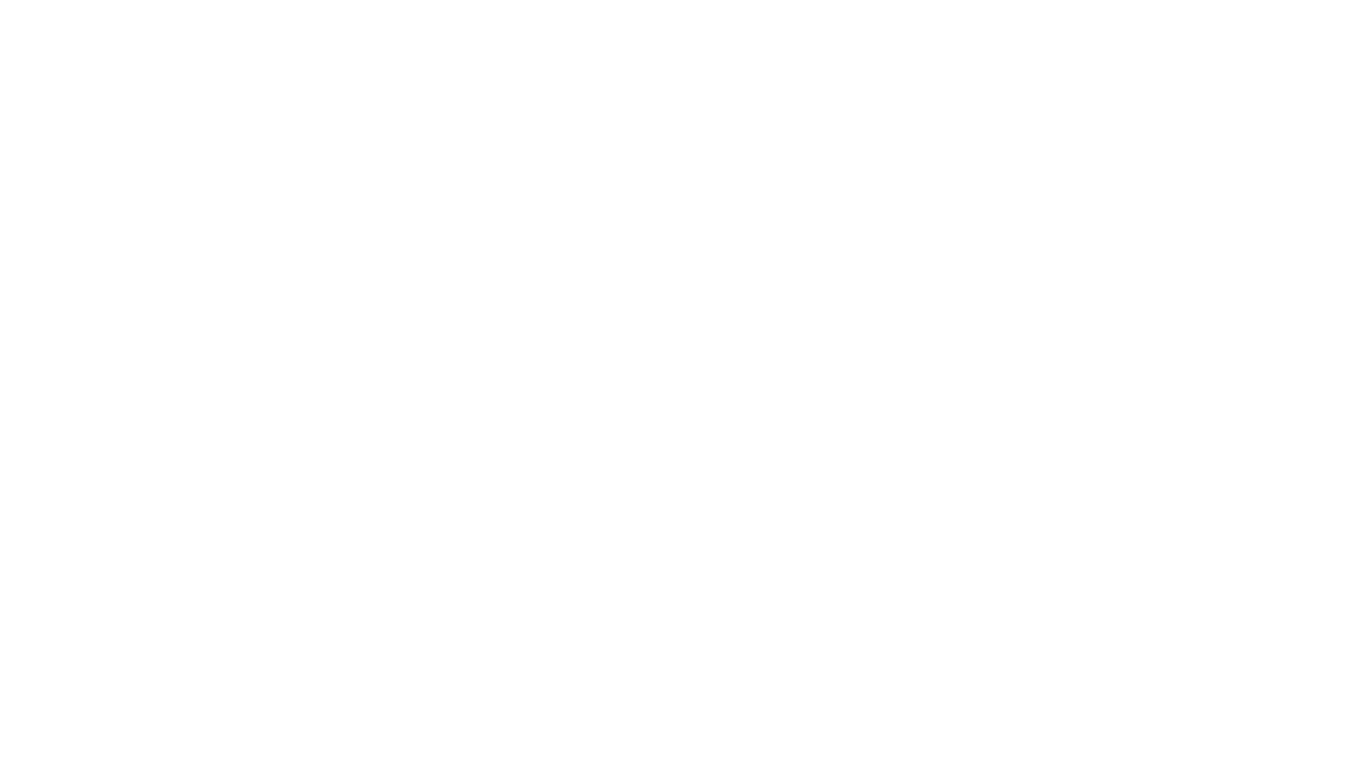
rlama
RLAMA is a powerful AI-driven question-answering tool that seamlessly integrates with local Ollama models. It enables users to create, manage, and interact with Retrieval-Augmented Generation (RAG) systems tailored to their documentation needs. RLAMA follows a clean architecture pattern with clear separation of concerns, focusing on lightweight and portable RAG capabilities with minimal dependencies. The tool processes documents, generates embeddings, stores RAG systems locally, and provides contextually-informed responses to user queries. Supported document formats include text, code, and various document types, with troubleshooting steps available for common issues like Ollama accessibility, text extraction problems, and relevance of answers.
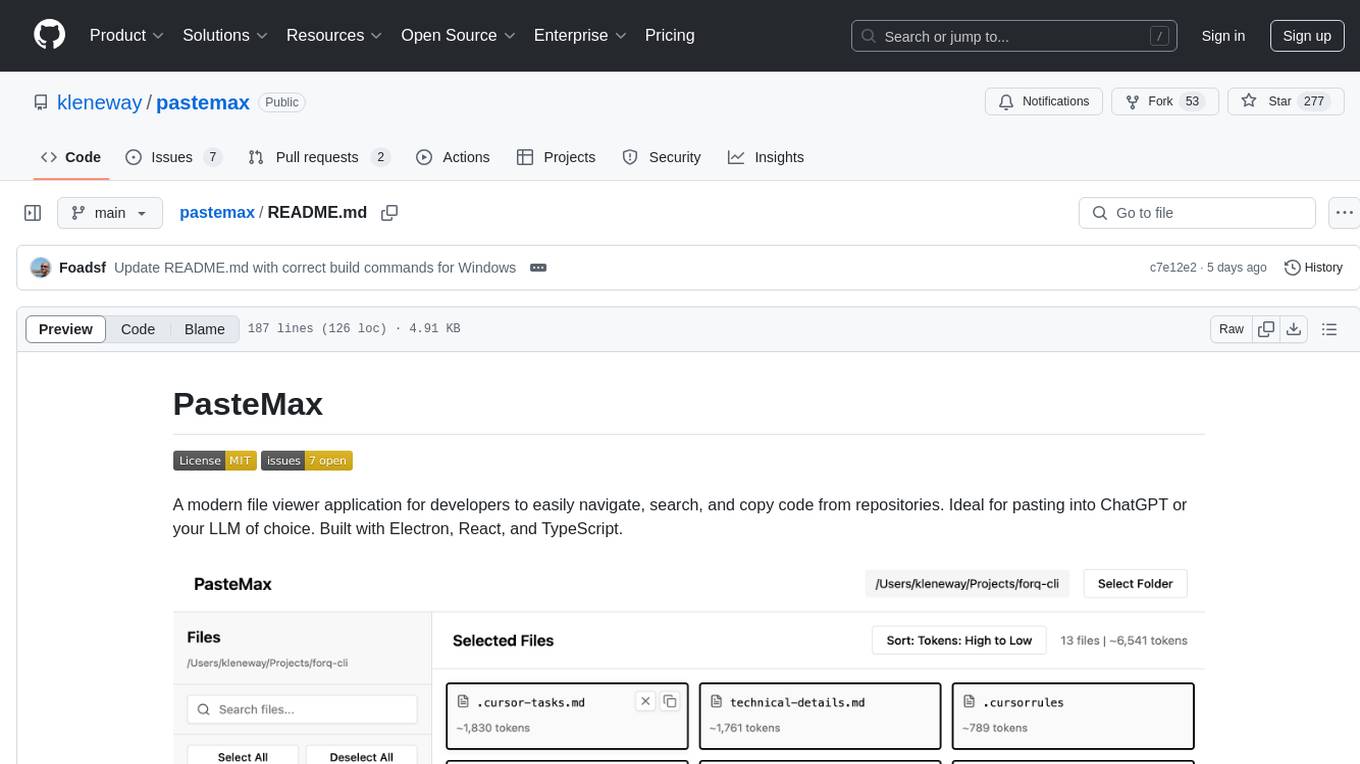
pastemax
PasteMax is a modern file viewer application designed for developers to easily navigate, search, and copy code from repositories. It provides features such as file tree navigation, token counting, search capabilities, selection management, sorting options, dark mode, binary file detection, and smart file exclusion. Built with Electron, React, and TypeScript, PasteMax is ideal for pasting code into ChatGPT or other language models. Users can download the application or build it from source, and customize file exclusions. Troubleshooting steps are provided for common issues, and contributions to the project are welcome under the MIT License.
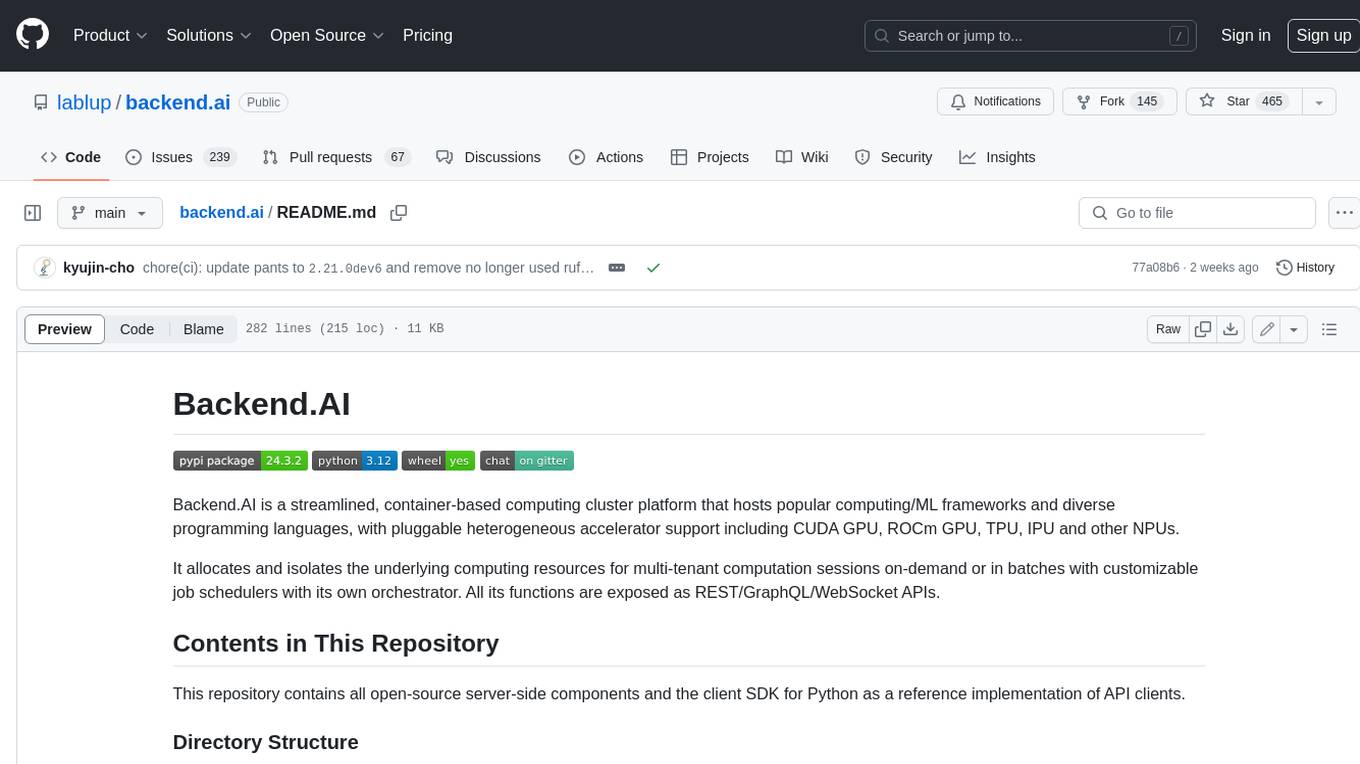
backend.ai
Backend.AI is a streamlined, container-based computing cluster platform that hosts popular computing/ML frameworks and diverse programming languages, with pluggable heterogeneous accelerator support including CUDA GPU, ROCm GPU, TPU, IPU and other NPUs. It allocates and isolates the underlying computing resources for multi-tenant computation sessions on-demand or in batches with customizable job schedulers with its own orchestrator. All its functions are exposed as REST/GraphQL/WebSocket APIs.
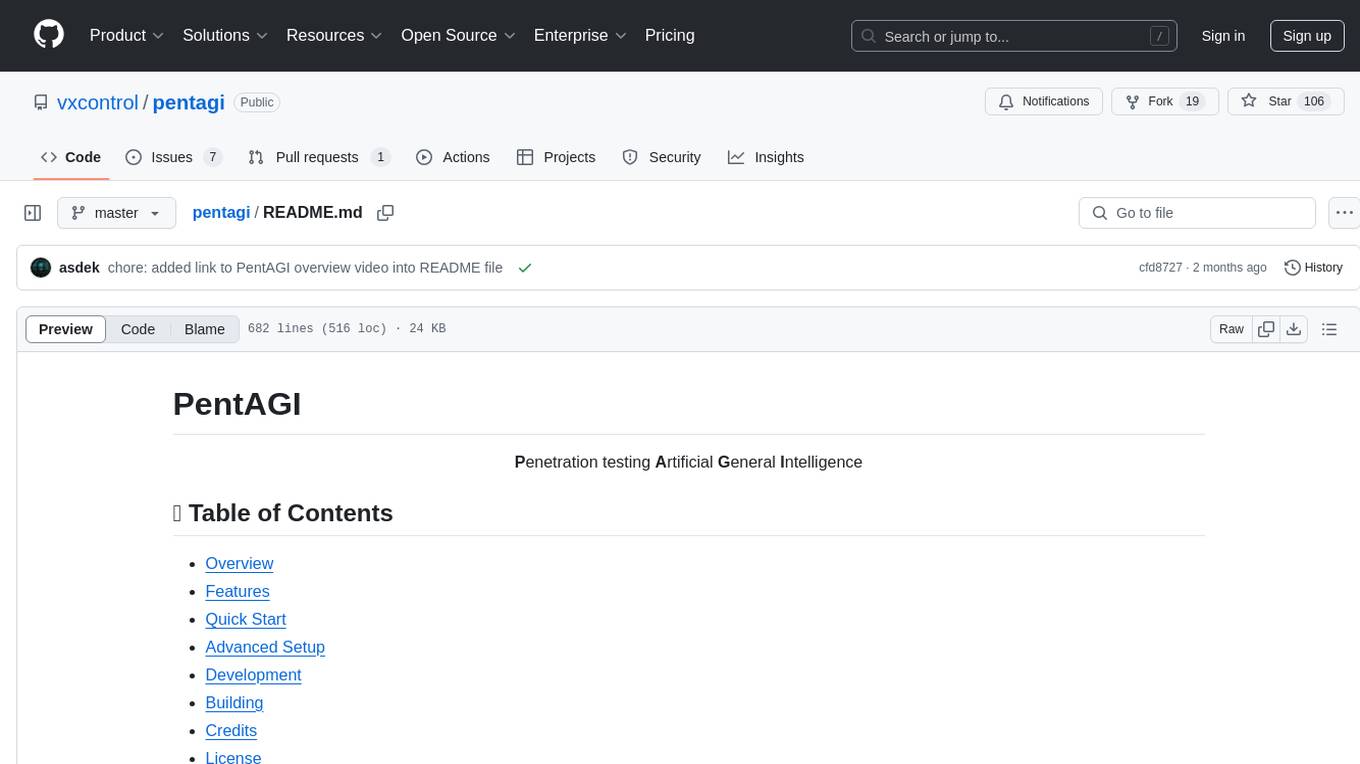
pentagi
PentAGI is an innovative tool for automated security testing that leverages cutting-edge artificial intelligence technologies. It is designed for information security professionals, researchers, and enthusiasts who need a powerful and flexible solution for conducting penetration tests. The tool provides secure and isolated operations in a sandboxed Docker environment, fully autonomous AI-powered agent for penetration testing steps, a suite of 20+ professional security tools, smart memory system for storing research results, web intelligence for gathering information, integration with external search systems, team delegation system, comprehensive monitoring and reporting, modern interface, API integration, persistent storage, scalable architecture, self-hosted solution, flexible authentication, and quick deployment through Docker Compose.
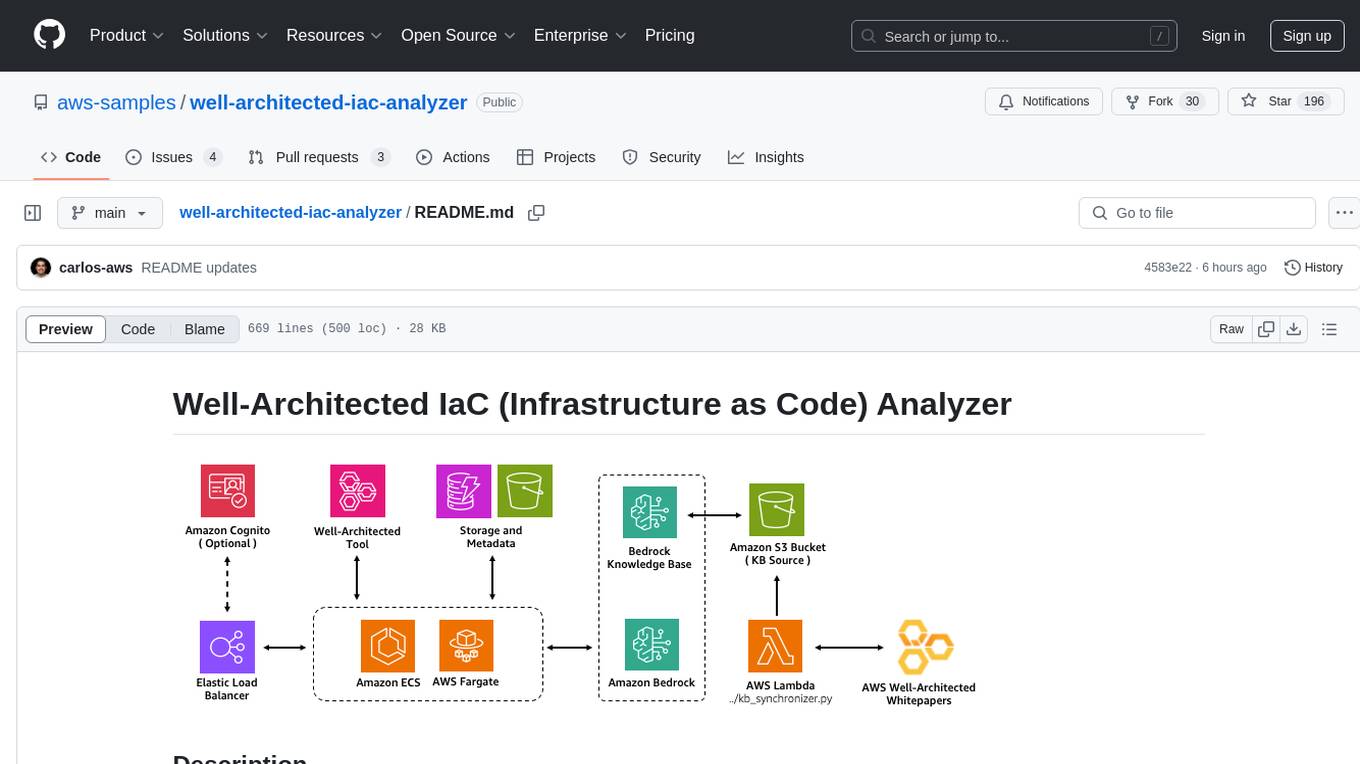
well-architected-iac-analyzer
Well-Architected Infrastructure as Code (IaC) Analyzer is a project demonstrating how generative AI can evaluate infrastructure code for alignment with best practices. It features a modern web application allowing users to upload IaC documents, complete IaC projects, or architecture diagrams for assessment. The tool provides insights into infrastructure code alignment with AWS best practices, offers suggestions for improving cloud architecture designs, and can generate IaC templates from architecture diagrams. Users can analyze CloudFormation, Terraform, or AWS CDK templates, architecture diagrams in PNG or JPEG format, and complete IaC projects with supporting documents. Real-time analysis against Well-Architected best practices, integration with AWS Well-Architected Tool, and export of analysis results and recommendations are included.
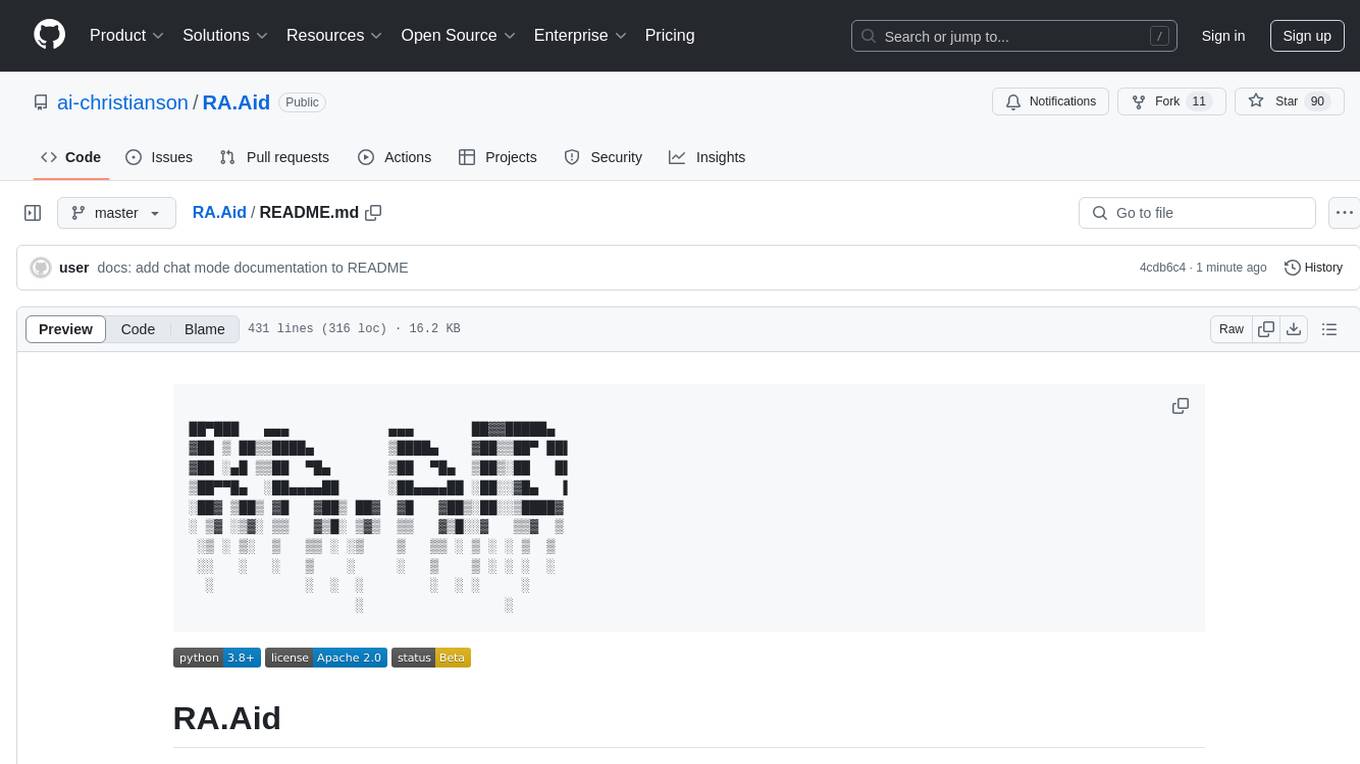
RA.Aid
RA.Aid is an AI software development agent powered by `aider` and advanced reasoning models like `o1`. It combines `aider`'s code editing capabilities with LangChain's agent-based task execution framework to provide an intelligent assistant for research, planning, and implementation of multi-step development tasks. It handles complex programming tasks by breaking them down into manageable steps, running shell commands automatically, and leveraging expert reasoning models like OpenAI's o1. RA.Aid is designed for everyday software development, offering features such as multi-step task planning, automated command execution, and the ability to handle complex programming tasks beyond single-shot code edits.
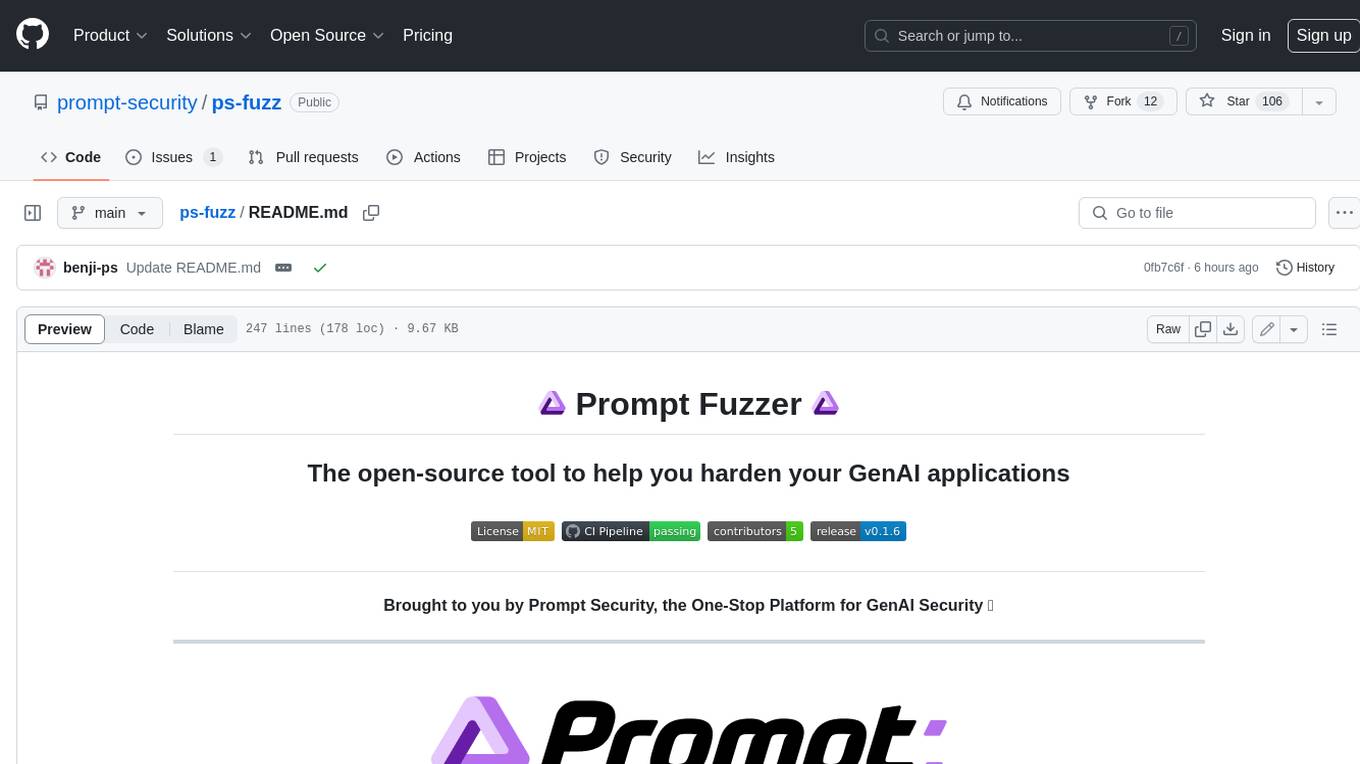
ps-fuzz
The Prompt Fuzzer is an open-source tool that helps you assess the security of your GenAI application's system prompt against various dynamic LLM-based attacks. It provides a security evaluation based on the outcome of these attack simulations, enabling you to strengthen your system prompt as needed. The Prompt Fuzzer dynamically tailors its tests to your application's unique configuration and domain. The Fuzzer also includes a Playground chat interface, giving you the chance to iteratively improve your system prompt, hardening it against a wide spectrum of generative AI attacks.
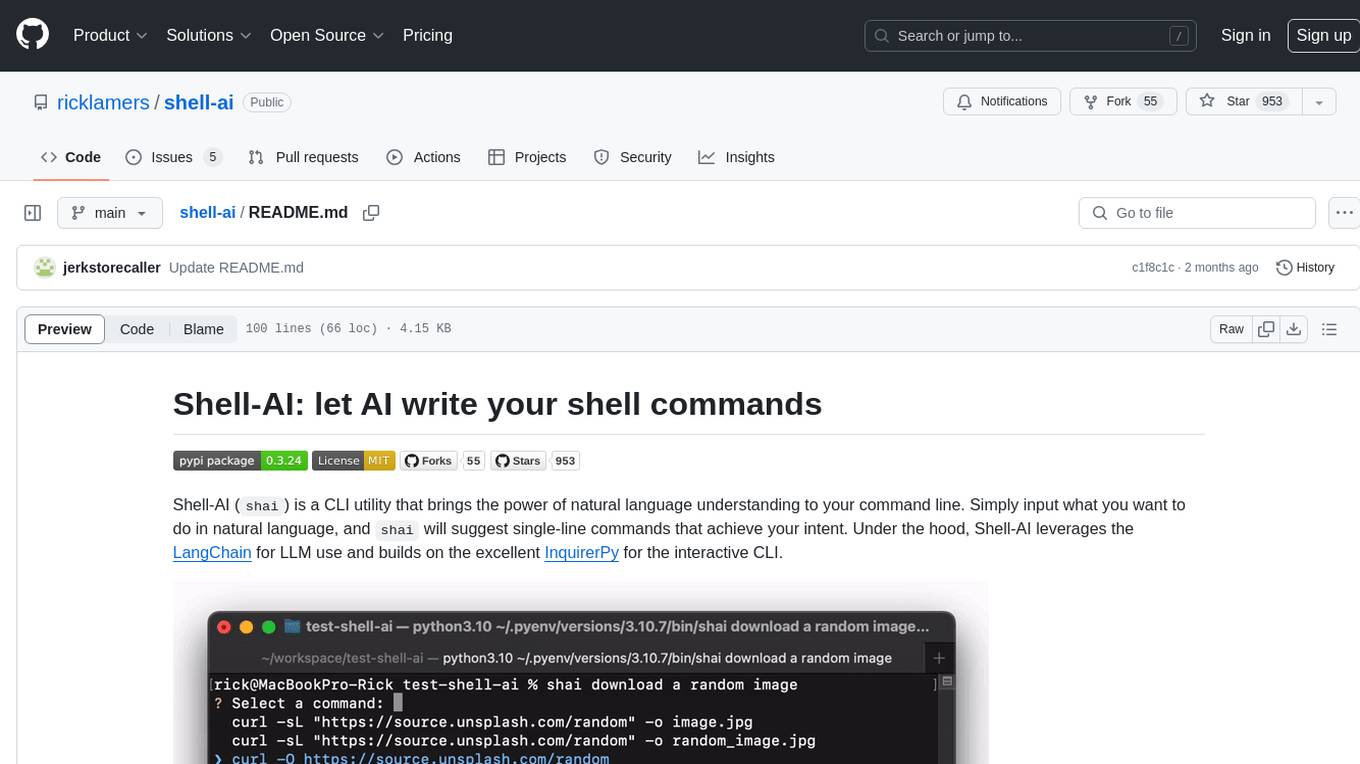
shell-ai
Shell-AI (`shai`) is a CLI utility that enables users to input commands in natural language and receive single-line command suggestions. It leverages natural language understanding and interactive CLI tools to enhance command line interactions. Users can describe tasks in plain English and receive corresponding command suggestions, making it easier to execute commands efficiently. Shell-AI supports cross-platform usage and is compatible with Azure OpenAI deployments, offering a user-friendly and efficient way to interact with the command line.
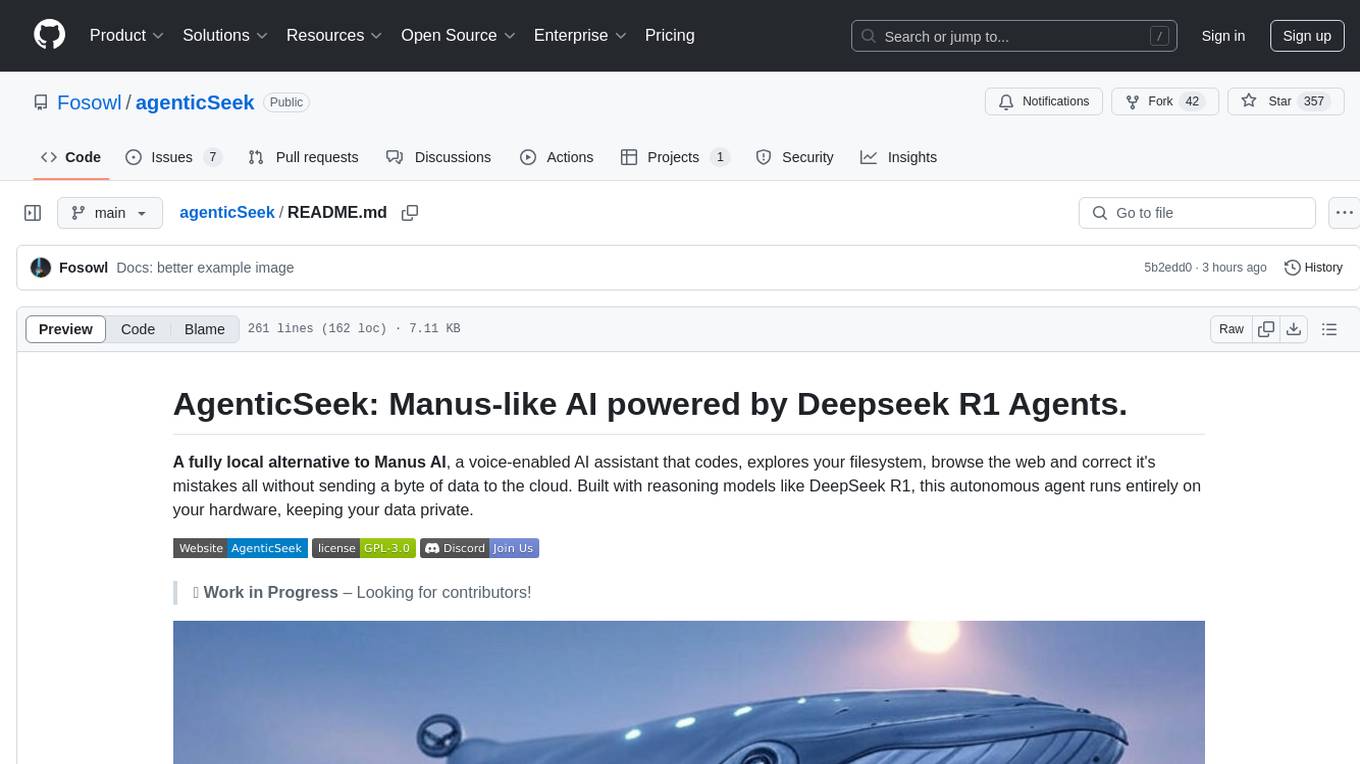
agenticSeek
AgenticSeek is a voice-enabled AI assistant powered by DeepSeek R1 agents, offering a fully local alternative to cloud-based AI services. It allows users to interact with their filesystem, code in multiple languages, and perform various tasks autonomously. The tool is equipped with memory to remember user preferences and past conversations, and it can divide tasks among multiple agents for efficient execution. AgenticSeek prioritizes privacy by running entirely on the user's hardware without sending data to the cloud.
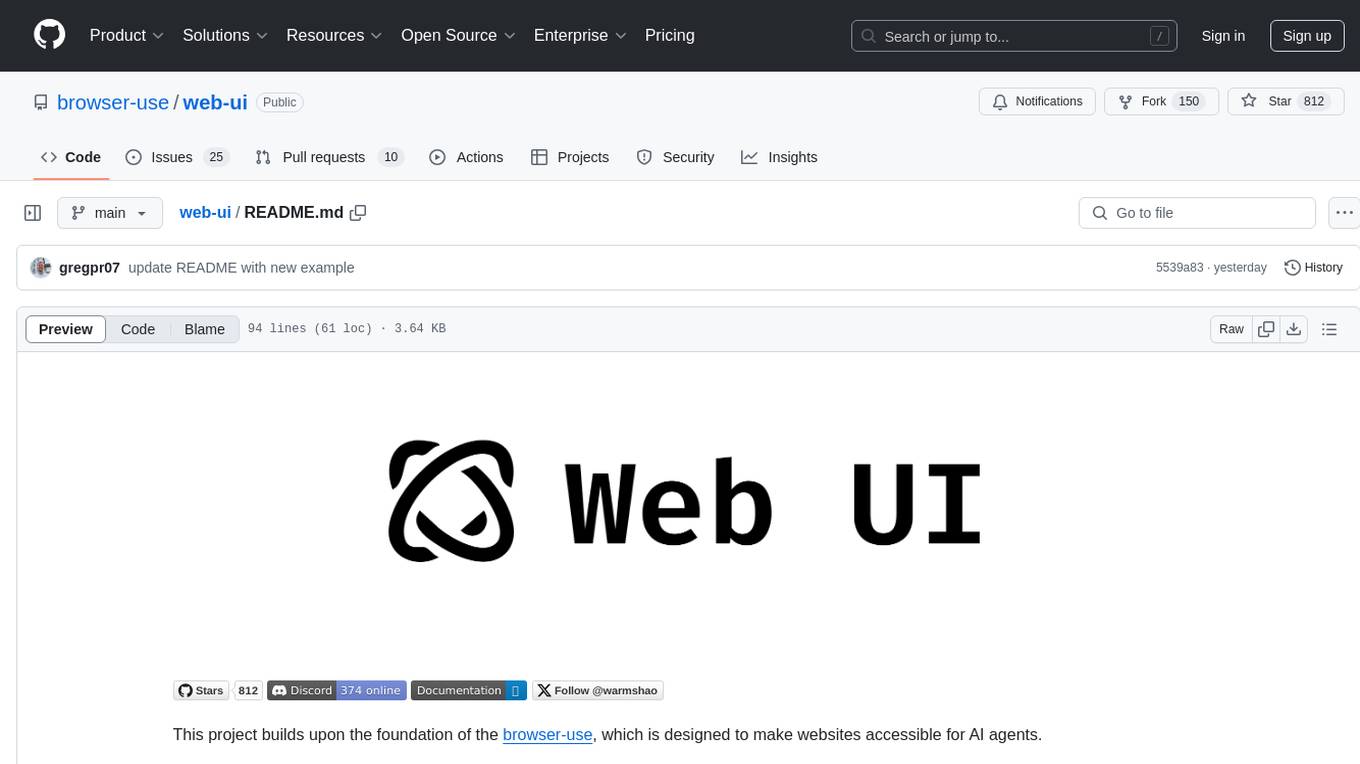
web-ui
WebUI is a user-friendly tool built on Gradio that enhances website accessibility for AI agents. It supports various Large Language Models (LLMs) and allows custom browser integration for seamless interaction. The tool eliminates the need for re-login and authentication challenges, offering high-definition screen recording capabilities.
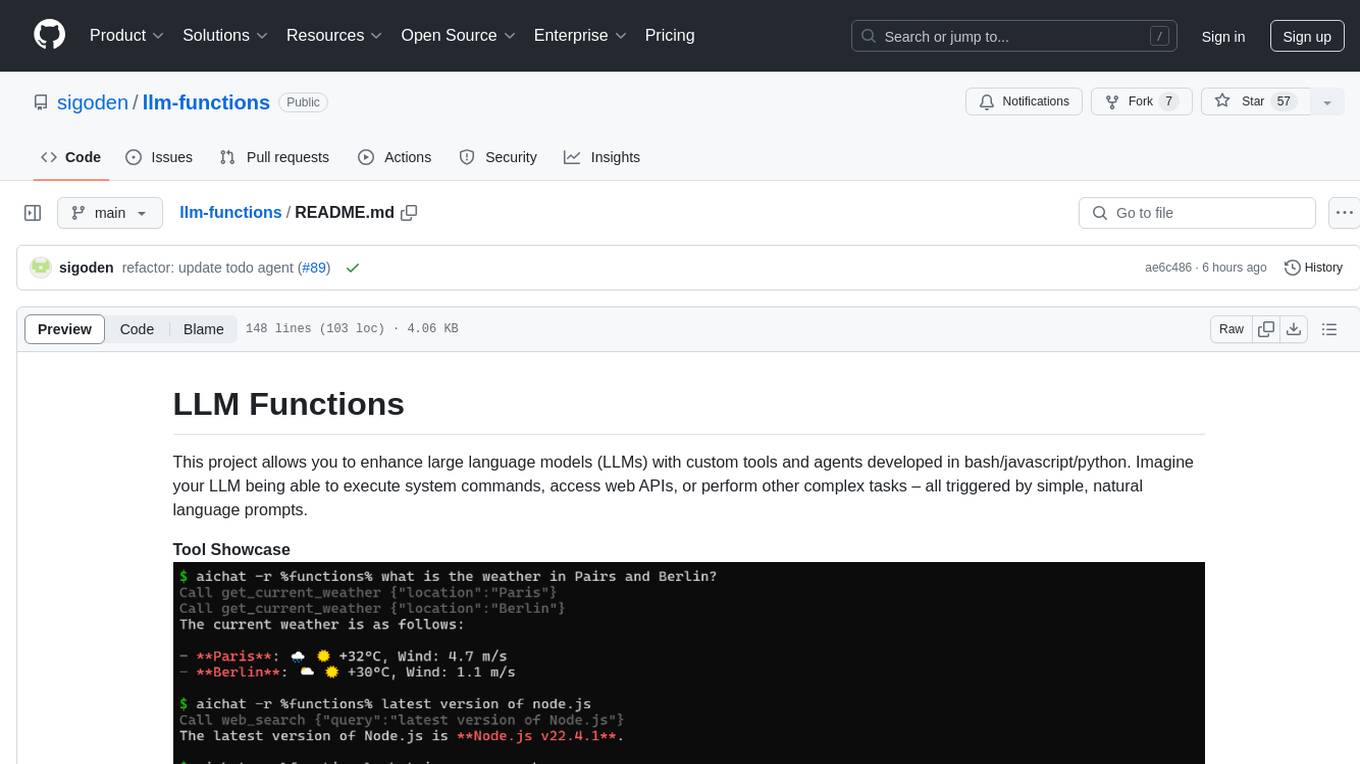
llm-functions
LLM Functions is a project that enables the enhancement of large language models (LLMs) with custom tools and agents developed in bash, javascript, and python. Users can create tools for their LLM to execute system commands, access web APIs, or perform other complex tasks triggered by natural language prompts. The project provides a framework for building tools and agents, with tools being functions written in the user's preferred language and automatically generating JSON declarations based on comments. Agents combine prompts, function callings, and knowledge (RAG) to create conversational AI agents. The project is designed to be user-friendly and allows users to easily extend the capabilities of their language models.
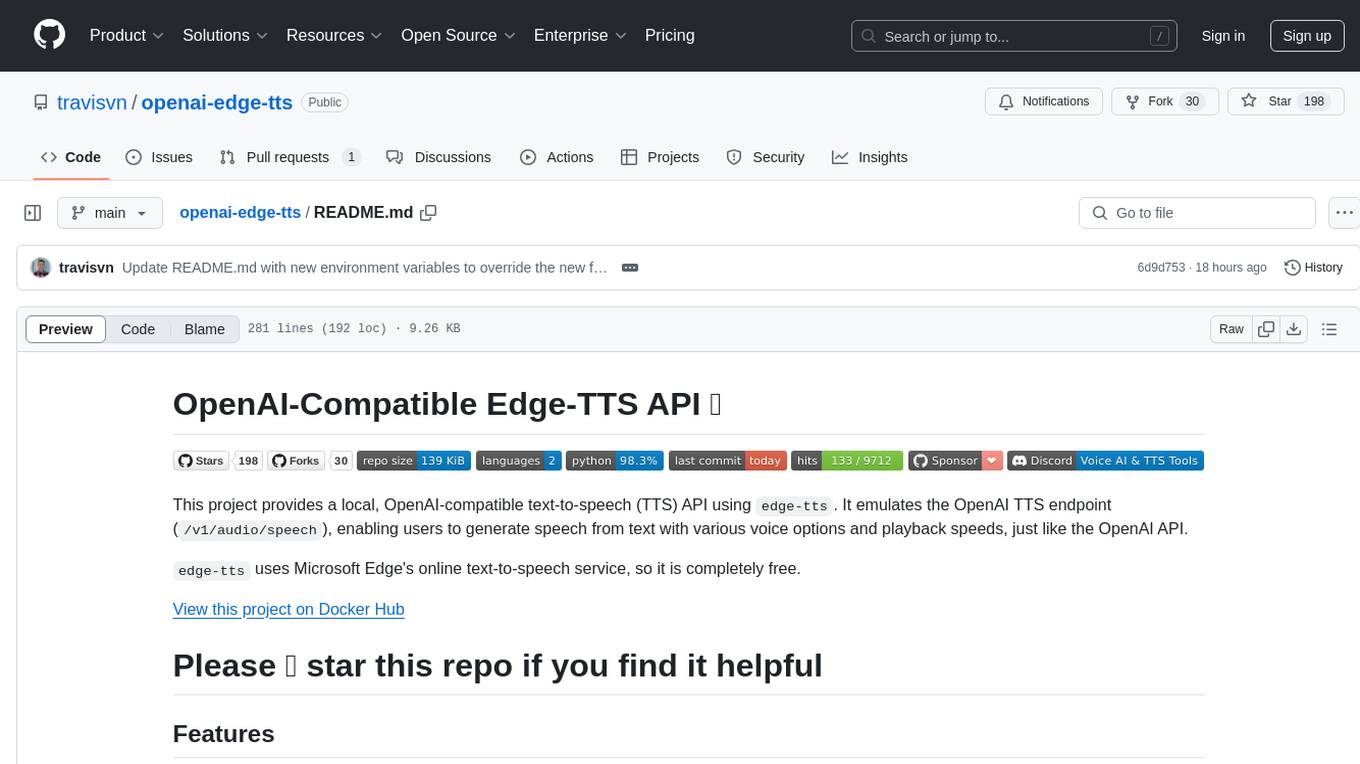
openai-edge-tts
This project provides a local, OpenAI-compatible text-to-speech (TTS) API using `edge-tts`. It emulates the OpenAI TTS endpoint (`/v1/audio/speech`), enabling users to generate speech from text with various voice options and playback speeds, just like the OpenAI API. `edge-tts` uses Microsoft Edge's online text-to-speech service, making it completely free. The project supports multiple audio formats, adjustable playback speed, and voice selection options, providing a flexible and customizable TTS solution for users.