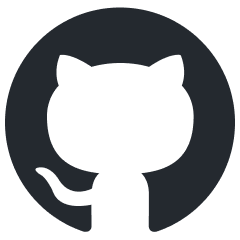
rlama
A powerful document AI question-answering tool that connects to your local Ollama models. Create, manage, and interact with RAG systems for all your document needs.
Stars: 905
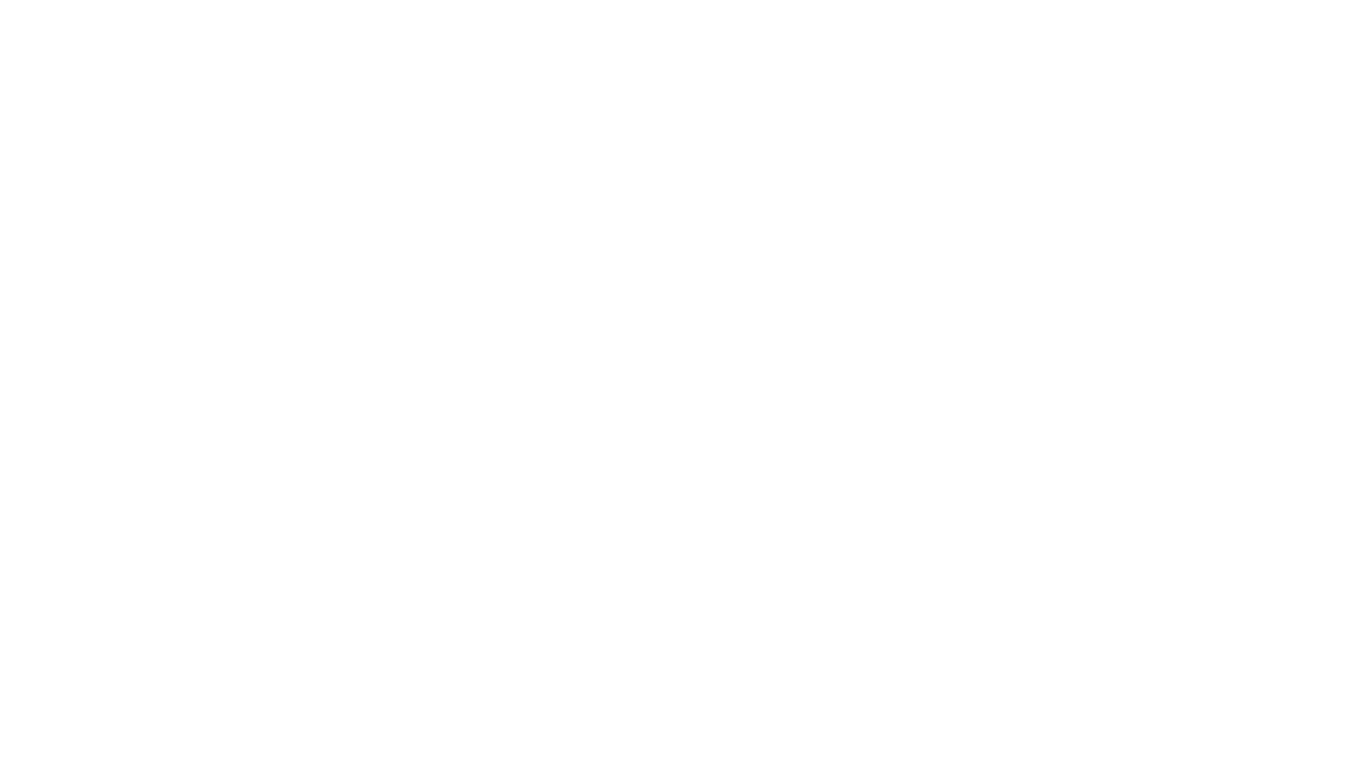
RLAMA is a powerful AI-driven question-answering tool that seamlessly integrates with local Ollama models. It enables users to create, manage, and interact with Retrieval-Augmented Generation (RAG) systems tailored to their documentation needs. RLAMA follows a clean architecture pattern with clear separation of concerns, focusing on lightweight and portable RAG capabilities with minimal dependencies. The tool processes documents, generates embeddings, stores RAG systems locally, and provides contextually-informed responses to user queries. Supported document formats include text, code, and various document types, with troubleshooting steps available for common issues like Ollama accessibility, text extraction problems, and relevance of answers.
README:
RLAMA is a powerful AI-driven question-answering tool for your documents, seamlessly integrating with your local Ollama models. It enables you to create, manage, and interact with Retrieval-Augmented Generation (RAG) systems tailored to your documentation needs.
- Vision & Roadmap
- Installation
-
Available Commands
- rag - Create a RAG system
- crawl-rag - Create a RAG system from a website
- wizard - Create a RAG system with interactive setup
- watch - Set up directory watching for a RAG system
- watch-off - Disable directory watching for a RAG system
- check-watched - Check a RAG's watched directory for new files
- web-watch - Set up website monitoring for a RAG system
- web-watch-off - Disable website monitoring for a RAG system
- check-web-watched - Check a RAG's monitored website for updates
- run - Use a RAG system
- api - Start API server
- list - List RAG systems
- delete - Delete a RAG system
- list-docs - List documents in a RAG
- list-chunks - Inspect document chunks
- view-chunk - View chunk details
- add-docs - Add documents to RAG
- crawl-add-docs - Add website content to RAG
- update-model - Change LLM model
- update - Update RLAMA
- version - Display version
- hf-browse - Browse GGUF models on Hugging Face
- run-hf - Run a Hugging Face GGUF model
- Uninstallation
- Supported Document Formats
- Troubleshooting
- Using OpenAI Models
RLAMA aims to become the definitive tool for creating local RAG systems that work seamlessly for everyone—from individual developers to large enterprises. Here's our strategic roadmap:
- ✅ Basic RAG System Creation: CLI tool for creating and managing RAG systems
- ✅ Document Processing: Support for multiple document formats (.txt, .md, .pdf, etc.)
- ✅ Document Chunking: Advanced semantic chunking with multiple strategies (fixed, semantic, hierarchical, hybrid)
- ✅ Vector Storage: Local storage of document embeddings
- ✅ Context Retrieval: Basic semantic search with configurable context size
- ✅ Ollama Integration: Seamless connection to Ollama models
- ✅ Cross-Platform Support: Works on Linux, macOS, and Windows
- ✅ Easy Installation: One-line installation script
- ✅ API Server: HTTP endpoints for integrating RAG capabilities in other applications
- ✅ Web Crawling: Create RAGs directly from websites
- ✅ Guided RAG Setup Wizard: Interactive interface for easy RAG creation
- ✅ Hugging Face Integration: Access to 45,000+ GGUF models from Hugging Face Hub
- [ ] Prompt Compression: Smart context summarization for limited context windows
- ✅ Adaptive Chunking: Dynamic content segmentation based on semantic boundaries and document structure
- ✅ Minimal Context Retrieval: Intelligent filtering to eliminate redundant content
- [ ] Parameter Optimization: Fine-tuned settings for different model sizes
- [ ] Multi-Model Embedding Support: Integration with various embedding models
- [ ] Hybrid Retrieval Techniques: Combining sparse and dense retrievers for better accuracy
- [ ] Embedding Evaluation Tools: Built-in metrics to measure retrieval quality
- [ ] Automated Embedding Cache: Smart caching to reduce computation for similar queries
- [ ] Lightweight Web Interface: Simple browser-based UI for the existing CLI backend
- [ ] Knowledge Graph Visualization: Interactive exploration of document connections
- [ ] Domain-Specific Templates: Pre-configured settings for different domains
- [ ] Multi-User Access Control: Role-based permissions for team environments
- [ ] Integration with Enterprise Systems: Connectors for SharePoint, Confluence, Google Workspace
- [ ] Knowledge Quality Monitoring: Detection of outdated or contradictory information
- [ ] System Integration API: Webhooks and APIs for embedding RLAMA in existing workflows
- [ ] AI Agent Creation Framework: Simplified system for building custom AI agents with RAG capabilities
- [ ] Multi-Step Retrieval: Using the LLM to refine search queries for complex questions
- [ ] Cross-Modal Retrieval: Support for image content understanding and retrieval
- [ ] Feedback-Based Optimization: Learning from user interactions to improve retrieval
- [ ] Knowledge Graphs & Symbolic Reasoning: Combining vector search with structured knowledge
RLAMA's core philosophy remains unchanged: to provide a simple, powerful, local RAG solution that respects privacy, minimizes resource requirements, and works seamlessly across platforms.
- Ollama installed and running
curl -fsSL https://raw.githubusercontent.com/dontizi/rlama/main/install.sh | sh
RLAMA is built with:
- Core Language: Go (chosen for performance, cross-platform compatibility, and single binary distribution)
- CLI Framework: Cobra (for command-line interface structure)
- LLM Integration: Ollama API (for embeddings and completions)
- Storage: Local filesystem-based storage (JSON files for simplicity and portability)
- Vector Search: Custom implementation of cosine similarity for embedding retrieval
RLAMA follows a clean architecture pattern with clear separation of concerns:
rlama/
├── cmd/ # CLI commands (using Cobra)
│ ├── root.go # Base command
│ ├── rag.go # Create RAG systems
│ ├── run.go # Query RAG systems
│ └── ...
├── internal/
│ ├── client/ # External API clients
│ │ └── ollama_client.go # Ollama API integration
│ ├── domain/ # Core domain models
│ │ ├── rag.go # RAG system entity
│ │ └── document.go # Document entity
│ ├── repository/ # Data persistence
│ │ └── rag_repository.go # Handles saving/loading RAGs
│ └── service/ # Business logic
│ ├── rag_service.go # RAG operations
│ ├── document_loader.go # Document processing
│ └── embedding_service.go # Vector embeddings
└── pkg/ # Shared utilities
└── vector/ # Vector operations
- Document Processing: Documents are loaded from the file system, parsed based on their type, and converted to plain text.
- Embedding Generation: Document text is sent to Ollama to generate vector embeddings.
- Storage: The RAG system (documents + embeddings) is stored in the user's home directory (~/.rlama).
- Query Process: When a user asks a question, it's converted to an embedding, compared against stored document embeddings, and relevant content is retrieved.
- Response Generation: Retrieved content and the question are sent to Ollama to generate a contextually-informed response.
┌─────────────┐ ┌─────────────┐ ┌─────────────┐
│ Documents │────>│ Document │────>│ Embedding │
│ (Input) │ │ Processing │ │ Generation │
└─────────────┘ └─────────────┘ └─────────────┘
│
▼
┌─────────────┐ ┌─────────────┐ ┌─────────────┐
│ Query │────>│ Vector │<────│ Vector Store│
│ Response │ │ Search │ │ (RAG System)│
└─────────────┘ └─────────────┘ └─────────────┘
▲ │
│ ▼
┌─────────────┐ ┌─────────────┐
│ Ollama │<────│ Context │
│ LLM │ │ Building │
└─────────────┘ └─────────────┘
RLAMA is designed to be lightweight and portable, focusing on providing RAG capabilities with minimal dependencies. The entire system runs locally, with the only external dependency being Ollama for LLM capabilities.
You can get help on all commands by using:
rlama --help
These flags can be used with any command:
--host string Ollama host (default: localhost)
--port string Ollama port (default: 11434)
RLAMA stores data in ~/.rlama
by default. To use a different location:
-
Command-line flag (highest priority):
# Use with any command rlama --data-dir /path/to/custom/directory run my-rag
-
Environment variable:
# Set the environment variable export RLAMA_DATA_DIR=/path/to/custom/directory rlama run my-rag
The precedence order is: command-line flag > environment variable > default location.
Creates a new RAG system by indexing all documents in the specified folder.
rlama rag [model] [rag-name] [folder-path]
Parameters:
-
model
: Name of the Ollama model to use (e.g., llama3, mistral, gemma) or a Hugging Face model using the formathf.co/username/repository[:quantization]
. -
rag-name
: Unique name to identify your RAG system. -
folder-path
: Path to the folder containing your documents.
Example:
# Using a standard Ollama model
rlama rag llama3 documentation ./docs
# Using a Hugging Face model
rlama rag hf.co/bartowski/Llama-3.2-1B-Instruct-GGUF my-rag ./docs
# Using a Hugging Face model with specific quantization
rlama rag hf.co/mlabonne/Meta-Llama-3.1-8B-Instruct-abliterated-GGUF:Q5_K_M my-rag ./docs
Creates a new RAG system by crawling a website and indexing its content.
rlama crawl-rag [model] [rag-name] [website-url]
Parameters:
-
model
: Name of the Ollama model to use (e.g., llama3, mistral, gemma). -
rag-name
: Unique name to identify your RAG system. -
website-url
: URL of the website to crawl and index.
Options:
-
--max-depth
: Maximum crawl depth (default: 2) -
--concurrency
: Number of concurrent crawlers (default: 5) -
--exclude-path
: Paths to exclude from crawling (comma-separated) -
--chunk-size
: Character count per chunk (default: 1000) -
--chunk-overlap
: Overlap between chunks in characters (default: 200) -
--chunking-strategy
: Chunking strategy to use (options: "fixed", "semantic", "hybrid", "hierarchical", default: "hybrid")
RLAMA offers multiple advanced chunking strategies to optimize document retrieval:
- Fixed: Traditional chunking with fixed size and overlap, respecting sentence boundaries when possible.
- Semantic: Intelligently splits documents based on semantic boundaries like headings, paragraphs, and natural topic shifts.
- Hybrid: Automatically selects the best strategy based on document type and content (markdown, HTML, code, or plain text).
- Hierarchical: For very long documents, creates a two-level chunking structure with major sections and sub-chunks.
The system automatically adapts to different document types:
- Markdown documents: Split by headers and sections
- HTML documents: Split by semantic HTML elements
- Code documents: Split by functions, classes, and logical blocks
- Plain text: Split by paragraphs with contextual overlap
Example:
# Create a new RAG from a documentation website
rlama crawl-rag llama3 docs-rag https://docs.example.com
# Customize crawling behavior
rlama crawl-rag llama3 blog-rag https://blog.example.com --max-depth=3 --exclude-path=/archive,/tags
# Create a RAG with semantic chunking
rlama rag llama3 documentation ./docs --chunking-strategy=semantic
# Use hierarchical chunking for large documents
rlama rag llama3 book-rag ./books --chunking-strategy=hierarchical
Provides an interactive step-by-step wizard for creating a new RAG system.
rlama wizard
The wizard guides you through:
- Naming your RAG
- Choosing an Ollama model
- Selecting document sources (local folder or website)
- Configuring chunking parameters
- Setting up file filtering
Example:
rlama wizard
# Follow the prompts to create your customized RAG
Configure a RAG system to automatically watch a directory for new files and add them to the RAG.
rlama watch [rag-name] [directory-path] [interval]
Parameters:
-
rag-name
: Name of the RAG system to watch. -
directory-path
: Path to the directory to watch for new files. -
interval
: Time in minutes to check for new files (use 0 to check only when the RAG is used).
Example:
# Set up directory watching to check every 60 minutes
rlama watch my-docs ./watched-folder 60
# Set up directory watching to only check when the RAG is used
rlama watch my-docs ./watched-folder 0
# Customize what files to watch
rlama watch my-docs ./watched-folder 30 --exclude-dir=node_modules,tmp --process-ext=.md,.txt
Disable automatic directory watching for a RAG system.
rlama watch-off [rag-name]
Parameters:
-
rag-name
: Name of the RAG system to disable watching.
Example:
rlama watch-off my-docs
Manually check a RAG's watched directory for new files and add them to the RAG.
rlama check-watched [rag-name]
Parameters:
-
rag-name
: Name of the RAG system to check.
Example:
rlama check-watched my-docs
Configure a RAG system to automatically monitor a website for updates and add new content to the RAG.
rlama web-watch [rag-name] [website-url] [interval]
Parameters:
-
rag-name
: Name of the RAG system to monitor. -
website-url
: URL of the website to monitor. -
interval
: Time in minutes between checks (use 0 to check only when the RAG is used).
Example:
# Set up website monitoring to check every 60 minutes
rlama web-watch my-docs https://example.com 60
# Set up website monitoring to only check when the RAG is used
rlama web-watch my-docs https://example.com 0
# Customize what content to monitor
rlama web-watch my-docs https://example.com 30 --exclude-path=/archive,/tags
Disable automatic website monitoring for a RAG system.
rlama web-watch-off [rag-name]
Parameters:
-
rag-name
: Name of the RAG system to disable monitoring.
Example:
rlama web-watch-off my-docs
Manually check a RAG's monitored website for new updates and add them to the RAG.
rlama check-web-watched [rag-name]
Parameters:
-
rag-name
: Name of the RAG system to check.
Example:
rlama check-web-watched my-docs
Starts an interactive session to interact with an existing RAG system.
rlama run [rag-name]
Parameters:
-
rag-name
: Name of the RAG system to use. -
--context-size
: (Optional) Number of context chunks to retrieve (default: 20)
Example:
rlama run documentation
> How do I install the project?
> What are the main features?
> exit
Context Size Tips:
- Smaller values (5-15) for faster responses with key information
- Medium values (20-40) for balanced performance
- Larger values (50+) for complex questions needing broad context
- Consider your model's context window limits
rlama run documentation --context-size=50 # Use 50 context chunks
Starts an HTTP API server that exposes RLAMA's functionality through RESTful endpoints.
rlama api [--port PORT]
Parameters:
-
--port
: (Optional) Port number to run the API server on (default: 11249)
Example:
rlama api --port 8080
Available Endpoints:
-
Query a RAG system -
POST /rag
curl -X POST http://localhost:11249/rag \ -H "Content-Type: application/json" \ -d '{ "rag_name": "documentation", "prompt": "How do I install the project?", "context_size": 20 }'
Request fields:
-
rag_name
(required): Name of the RAG system to query -
prompt
(required): Question or prompt to send to the RAG -
context_size
(optional): Number of chunks to include in context -
model
(optional): Override the model used by the RAG
-
-
Check server health -
GET /health
curl http://localhost:11249/health
Integration Example:
// Node.js example
const response = await fetch('http://localhost:11249/rag', {
method: 'POST',
headers: { 'Content-Type': 'application/json' },
body: JSON.stringify({
rag_name: 'my-docs',
prompt: 'Summarize the key features'
})
});
const data = await response.json();
console.log(data.response);
Displays a list of all available RAG systems.
rlama list
Permanently deletes a RAG system and all its indexed documents.
rlama delete [rag-name] [--force/-f]
Parameters:
-
rag-name
: Name of the RAG system to delete. -
--force
or-f
: (Optional) Delete without asking for confirmation.
Example:
rlama delete old-project
Or to delete without confirmation:
rlama delete old-project --force
Displays all documents in a RAG system with metadata.
rlama list-docs [rag-name]
Parameters:
-
rag-name
: Name of the RAG system
Example:
rlama list-docs documentation
List and filter document chunks in a RAG system with various options:
# Basic chunk listing
rlama list-chunks [rag-name]
# With content preview (shows first 100 characters)
rlama list-chunks [rag-name] --show-content
# Filter by document name/ID substring
rlama list-chunks [rag-name] --document=readme
# Combine options
rlama list-chunks [rag-name] --document=api --show-content
Options:
-
--show-content
: Display chunk content preview -
--document
: Filter by document name/ID substring
Output columns:
- Chunk ID (use with view-chunk command)
- Document Source
- Chunk Position (e.g., "2/5" for second of five chunks)
- Content Preview (if enabled)
- Created Date
Display detailed information about a specific chunk.
rlama view-chunk [rag-name] [chunk-id]
Parameters:
-
rag-name
: Name of the RAG system -
chunk-id
: Chunk identifier from list-chunks
Example:
rlama view-chunk documentation doc123_chunk_0
Add new documents to an existing RAG system.
rlama add-docs [rag-name] [folder-path] [flags]
Parameters:
-
rag-name
: Name of the RAG system -
folder-path
: Path to documents folder
Example:
rlama add-docs documentation ./new-docs --exclude-ext=.tmp
Add content from a website to an existing RAG system.
rlama crawl-add-docs [rag-name] [website-url]
Parameters:
-
rag-name
: Name of the RAG system -
website-url
: URL of the website to crawl and add to the RAG
Options:
-
--max-depth
: Maximum crawl depth (default: 2) -
--concurrency
: Number of concurrent crawlers (default: 5) -
--exclude-path
: Paths to exclude from crawling (comma-separated) -
--chunk-size
: Character count per chunk (default: 1000) -
--chunk-overlap
: Overlap between chunks in characters (default: 200)
Example:
# Add blog content to an existing RAG
rlama crawl-add-docs my-docs https://blog.example.com
# Customize crawling behavior
rlama crawl-add-docs knowledge-base https://docs.example.com --max-depth=1 --exclude-path=/api
Update the LLM model used by a RAG system.
rlama update-model [rag-name] [new-model]
Parameters:
-
rag-name
: Name of the RAG system -
new-model
: New Ollama model name
Example:
rlama update-model documentation deepseek-r1:7b-instruct
Checks if a new version of RLAMA is available and installs it.
rlama update [--force/-f]
Options:
-
--force
or-f
: (Optional) Update without asking for confirmation.
Displays the current version of RLAMA.
rlama --version
or
rlama -v
Search and browse GGUF models available on Hugging Face.
rlama hf-browse [search-term] [flags]
Parameters:
-
search-term
: (Optional) Term to search for (e.g., "llama3", "mistral")
Flags:
-
--open
: Open the search results in your default web browser -
--quant
: Specify quantization type to suggest (e.g., Q4_K_M, Q5_K_M) -
--limit
: Limit number of results (default: 10)
Examples:
# Search for GGUF models and show command-line help
rlama hf-browse "llama 3"
# Open browser with search results
rlama hf-browse mistral --open
# Search with specific quantization suggestion
rlama hf-browse phi --quant Q4_K_M
Run a Hugging Face GGUF model directly using Ollama. This is useful for testing models before creating a RAG system with them.
rlama run-hf [huggingface-model] [flags]
Parameters:
-
huggingface-model
: Hugging Face model path in the formatusername/repository
Flags:
-
--quant
: Quantization to use (e.g., Q4_K_M, Q5_K_M)
Examples:
# Try a model in chat mode
rlama run-hf bartowski/Llama-3.2-1B-Instruct-GGUF
# Specify quantization
rlama run-hf mlabonne/Meta-Llama-3.1-8B-Instruct-abliterated-GGUF --quant Q5_K_M
To uninstall RLAMA:
If you installed via go install
:
rlama uninstall
RLAMA stores its data in ~/.rlama
. To remove it:
rm -rf ~/.rlama
RLAMA supports many file formats:
-
Text:
.txt
,.md
,.html
,.json
,.csv
,.yaml
,.yml
,.xml
,.org
-
Code:
.go
,.py
,.js
,.java
,.c
,.cpp
,.cxx
,.h
,.rb
,.php
,.rs
,.swift
,.kt
,.ts
,.tsx
,.f
,.F
,.F90
,.el
,.svelte
-
Documents:
.pdf
,.docx
,.doc
,.rtf
,.odt
,.pptx
,.ppt
,.xlsx
,.xls
,.epub
Installing dependencies via install_deps.sh
is recommended to improve support for certain formats.
If you encounter connection errors to Ollama:
- Check that Ollama is running.
- By default, Ollama must be accessible at
http://localhost:11434
or the host and port specified by the OLLAMA_HOST environment variable. - If your Ollama instance is running on a different host or port, use the
--host
and--port
flags:rlama --host 192.168.1.100 --port 8000 list rlama --host my-ollama-server --port 11434 run my-rag
- Check Ollama logs for potential errors.
If you encounter problems with certain formats:
- Install dependencies via
./scripts/install_deps.sh
. - Verify that your system has the required tools (
pdftotext
,tesseract
, etc.).
If the answers are not relevant:
- Check that the documents are properly indexed with
rlama list
. - Make sure the content of the documents is properly extracted.
- Try rephrasing your question more precisely.
- Consider adjusting chunking parameters during RAG creation
For any other issues, please open an issue on the GitHub repository providing:
- The exact command used.
- The complete output of the command.
- Your operating system and architecture.
- The RLAMA version (
rlama --version
).
RLAMA provides multiple ways to connect to your Ollama instance:
-
Command-line flags (highest priority):
rlama --host 192.168.1.100 --port 8080 run my-rag
-
Environment variable:
# Format: "host:port" or just "host" export OLLAMA_HOST=remote-server:8080 rlama run my-rag
-
Default values (used if no other method is specified):
- Host:
localhost
- Port:
11434
- Host:
The precedence order is: command-line flags > environment variable > default values.
# Quick answers with minimal context
rlama run my-docs --context-size=10
# Deep analysis with maximum context
rlama run my-docs --context-size=50
# Balance between speed and depth
rlama run my-docs --context-size=30
rlama rag llama3 my-project ./code \
--exclude-dir=node_modules,dist \
--process-ext=.go,.ts \
--exclude-ext=.spec.ts
# List chunks with content preview
rlama list-chunks my-project --show-content
# Filter chunks from specific document
rlama list-chunks my-project --document=architecture
Get full command help:
rlama --help
Command-specific help:
rlama rag --help
rlama list-chunks --help
rlama update-model --help
All commands support the global --host
and --port
flags for custom Ollama connections.
The precedence order is: command-line flags > environment variable > default values.
RLAMA now supports using GGUF models directly from Hugging Face through Ollama's native integration:
# Search for GGUF models on Hugging Face
rlama hf-browse "llama 3"
# Open browser with search results
rlama hf-browse mistral --open
Before creating a RAG, you can test a Hugging Face model directly:
# Try a model in chat mode
rlama run-hf bartowski/Llama-3.2-1B-Instruct-GGUF
# Specify quantization
rlama run-hf mlabonne/Meta-Llama-3.1-8B-Instruct-abliterated-GGUF --quant Q5_K_M
Use Hugging Face models when creating RAG systems:
# Create a RAG with a Hugging Face model
rlama rag hf.co/bartowski/Llama-3.2-1B-Instruct-GGUF my-rag ./docs
# Use specific quantization
rlama rag hf.co/mlabonne/Meta-Llama-3.1-8B-Instruct-abliterated-GGUF:Q5_K_M my-rag ./docs
RLAMA now supports using OpenAI models for inference while keeping Ollama for embeddings:
-
Set your OpenAI API key:
export OPENAI_API_KEY="your-api-key"
-
Create a RAG system with an OpenAI model:
rlama rag gpt-4-turbo my-rag ./documents
-
Run your RAG as usual:
rlama run my-rag
Supported OpenAI models include:
- o3-mini
- gpt-4o and more...
Note: Only inference uses OpenAI API. Document embeddings still use Ollama for processing.
RLAMA allows you to create API profiles to manage multiple API keys for different providers:
# Create a profile for your OpenAI account
rlama profile add openai-work openai "sk-your-api-key"
# Create another profile for a different account
rlama profile add openai-personal openai "sk-your-personal-api-key"
# View all available profiles
rlama profile list
# Delete a profile
rlama profile delete openai-old
When creating a new RAG:
# Create a RAG with a specific profile
rlama rag gpt-4 my-rag ./documents --profile openai-work
When updating an existing RAG:
# Update a RAG to use a different model and profile
rlama update-model my-rag gpt-4-turbo --profile openai-personal
Benefits of using profiles:
- Manage multiple API keys for different projects
- Easily switch between different accounts
- Keep API keys secure (stored in ~/.rlama/profiles)
- Track which profile was used last and when
For Tasks:
Click tags to check more tools for each tasksFor Jobs:
Alternative AI tools for rlama
Similar Open Source Tools
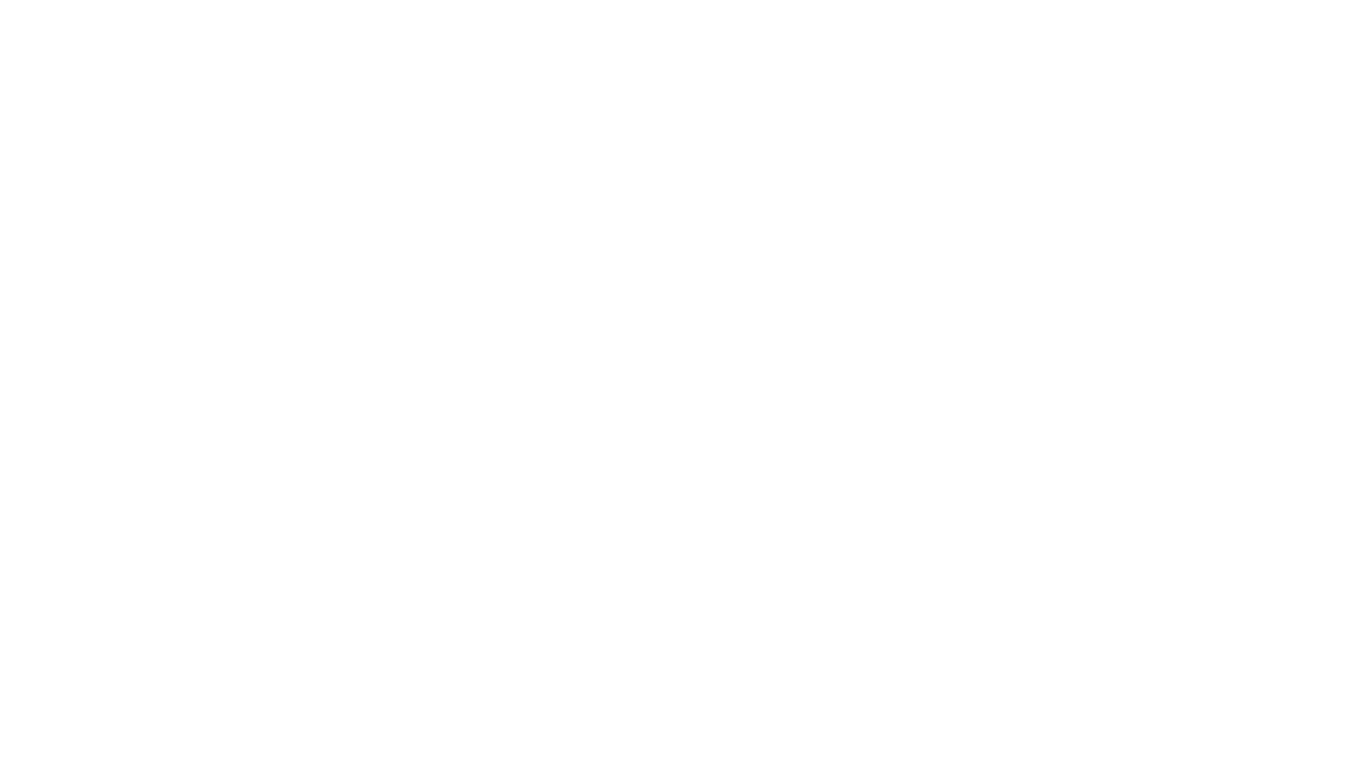
rlama
RLAMA is a powerful AI-driven question-answering tool that seamlessly integrates with local Ollama models. It enables users to create, manage, and interact with Retrieval-Augmented Generation (RAG) systems tailored to their documentation needs. RLAMA follows a clean architecture pattern with clear separation of concerns, focusing on lightweight and portable RAG capabilities with minimal dependencies. The tool processes documents, generates embeddings, stores RAG systems locally, and provides contextually-informed responses to user queries. Supported document formats include text, code, and various document types, with troubleshooting steps available for common issues like Ollama accessibility, text extraction problems, and relevance of answers.
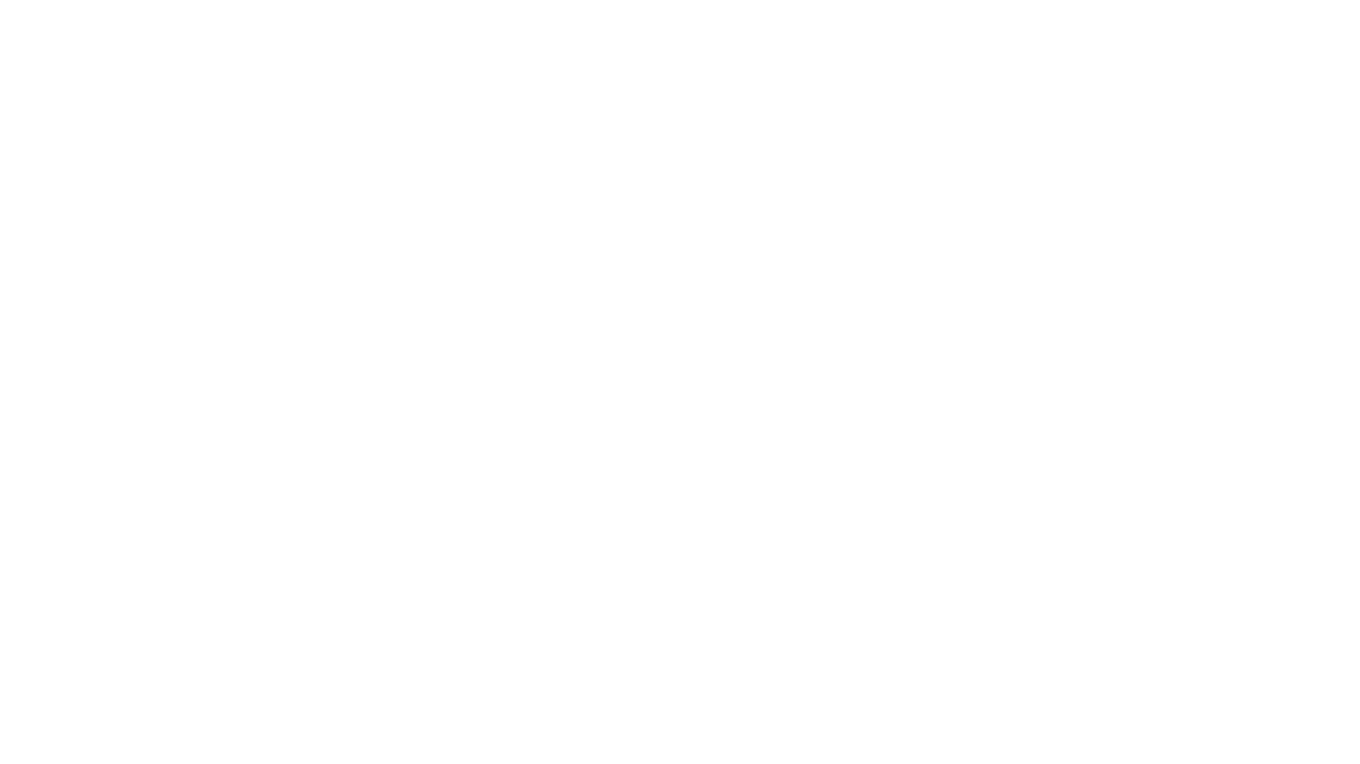
TalkWithGemini
Talk With Gemini is a web application that allows users to deploy their private Gemini application for free with one click. It supports Gemini Pro and Gemini Pro Vision models. The application features talk mode for direct communication with Gemini, visual recognition for understanding picture content, full Markdown support, automatic compression of chat records, privacy and security with local data storage, well-designed UI with responsive design, fast loading speed, and multi-language support. The tool is designed to be user-friendly and versatile for various deployment options and language preferences.
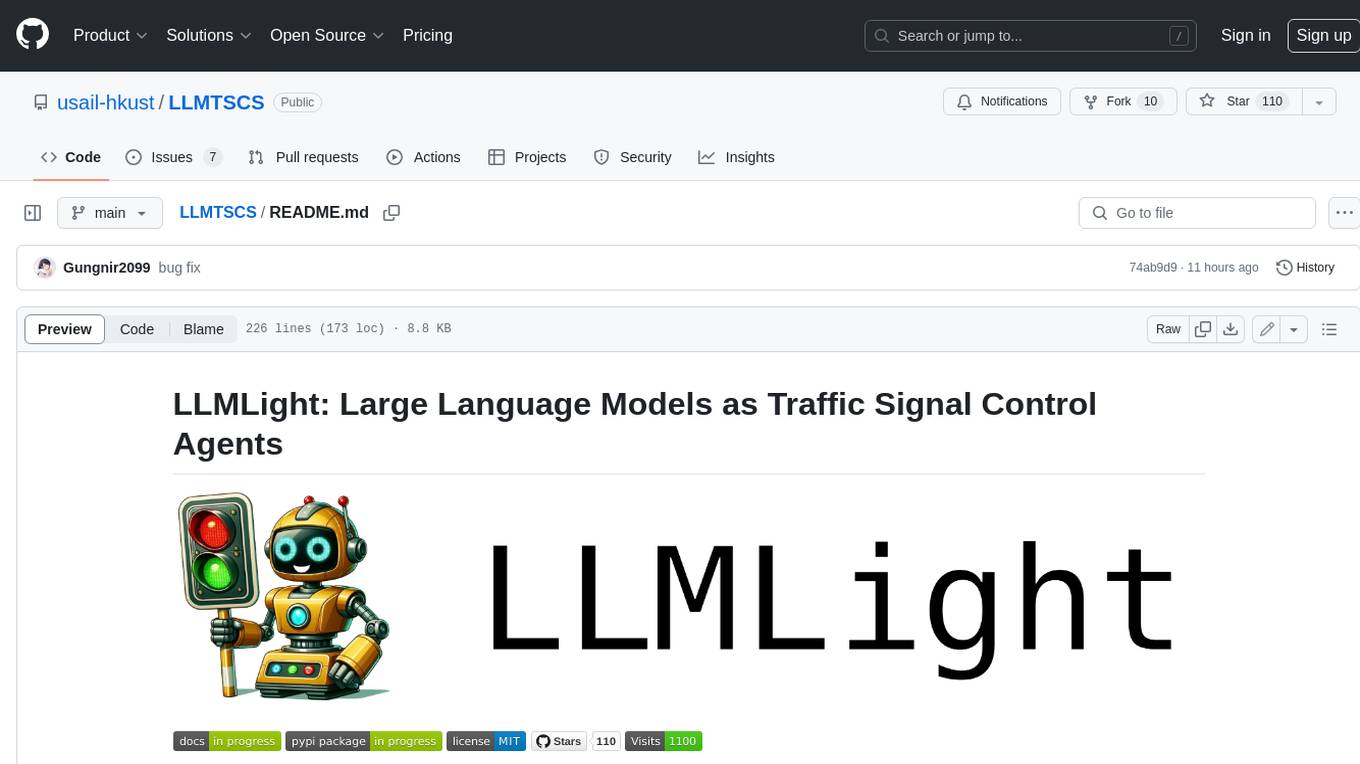
LLMTSCS
LLMLight is a novel framework that employs Large Language Models (LLMs) as decision-making agents for Traffic Signal Control (TSC). The framework leverages the advanced generalization capabilities of LLMs to engage in a reasoning and decision-making process akin to human intuition for effective traffic control. LLMLight has been demonstrated to be remarkably effective, generalizable, and interpretable against various transportation-based and RL-based baselines on nine real-world and synthetic datasets.
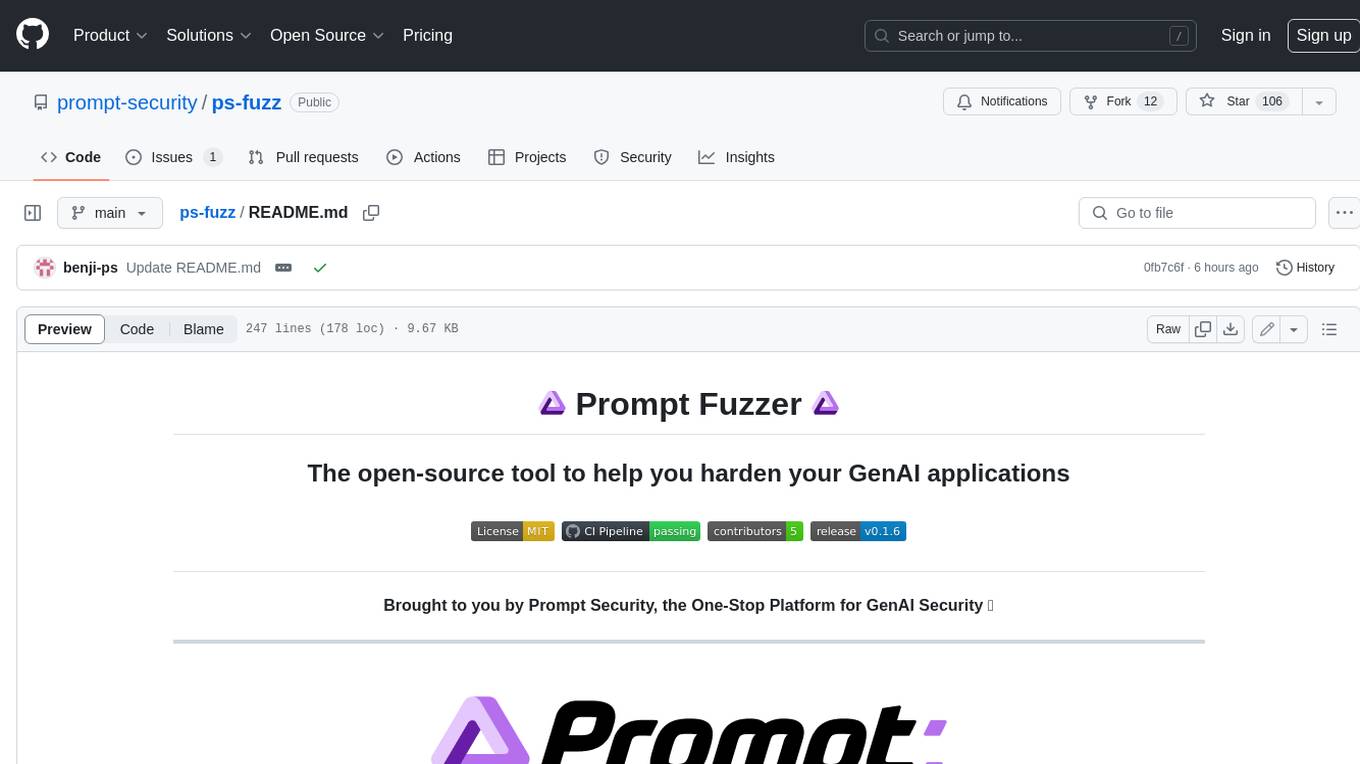
ps-fuzz
The Prompt Fuzzer is an open-source tool that helps you assess the security of your GenAI application's system prompt against various dynamic LLM-based attacks. It provides a security evaluation based on the outcome of these attack simulations, enabling you to strengthen your system prompt as needed. The Prompt Fuzzer dynamically tailors its tests to your application's unique configuration and domain. The Fuzzer also includes a Playground chat interface, giving you the chance to iteratively improve your system prompt, hardening it against a wide spectrum of generative AI attacks.
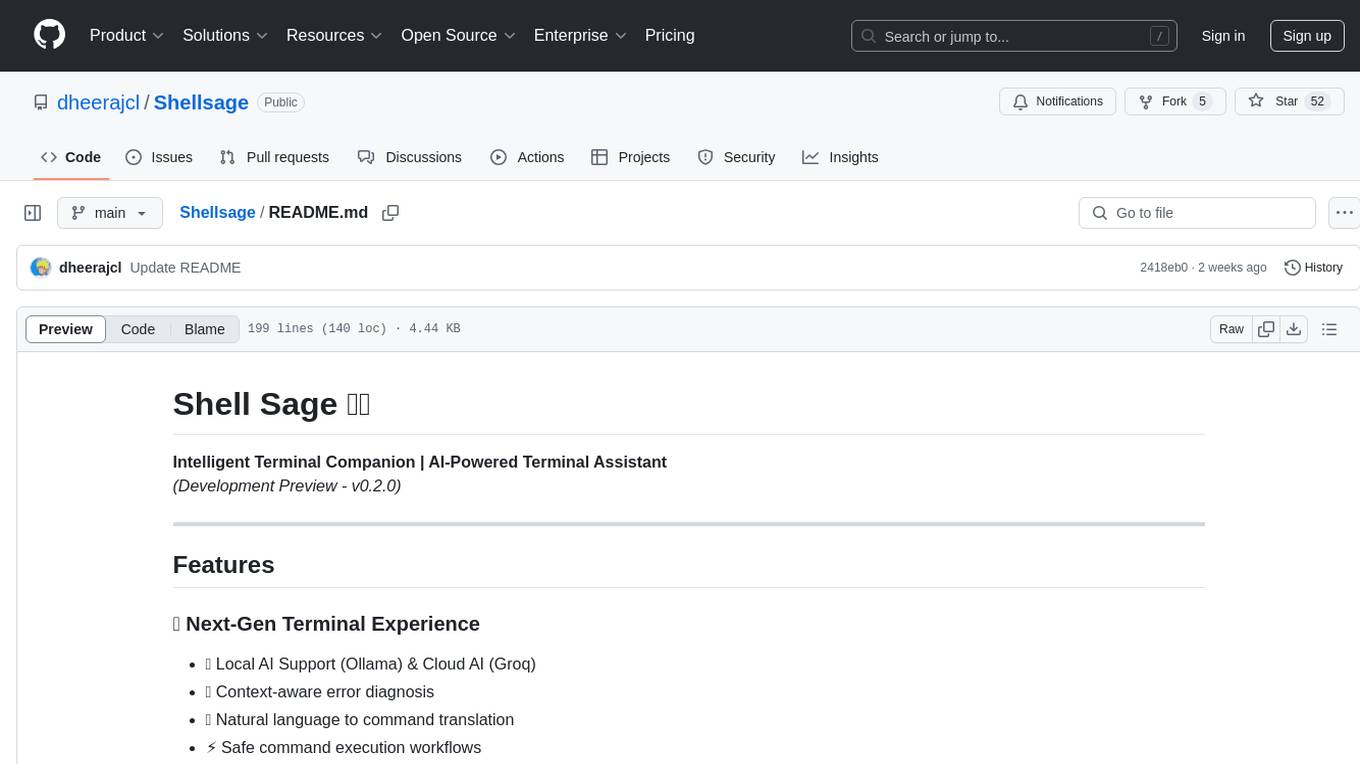
Shellsage
Shell Sage is an intelligent terminal companion and AI-powered terminal assistant that enhances the terminal experience with features like local and cloud AI support, context-aware error diagnosis, natural language to command translation, and safe command execution workflows. It offers interactive workflows, supports various API providers, and allows for custom model selection. Users can configure the tool for local or API mode, select specific models, and switch between modes easily. Currently in alpha development, Shell Sage has known limitations like limited Windows support and occasional false positives in error detection. The roadmap includes improvements like better context awareness, Windows PowerShell integration, Tmux integration, and CI/CD error pattern database.
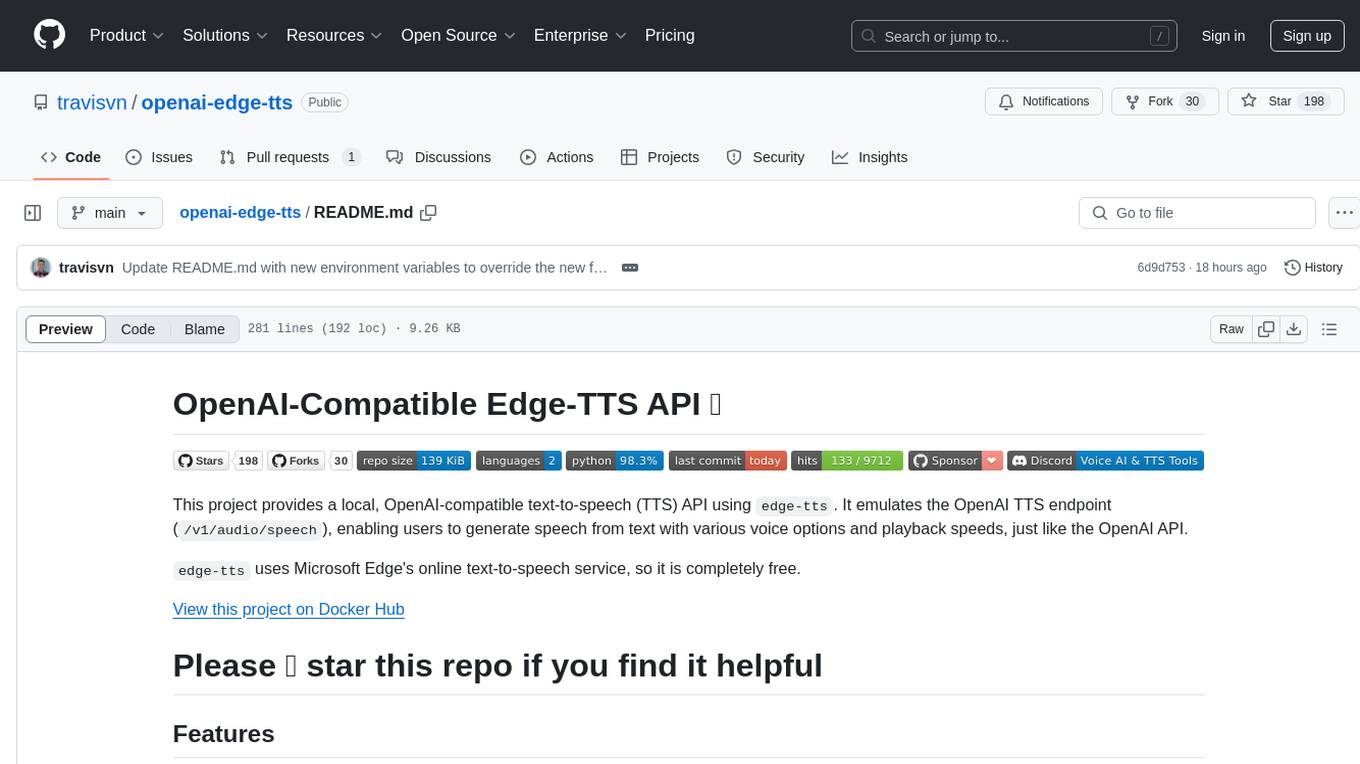
openai-edge-tts
This project provides a local, OpenAI-compatible text-to-speech (TTS) API using `edge-tts`. It emulates the OpenAI TTS endpoint (`/v1/audio/speech`), enabling users to generate speech from text with various voice options and playback speeds, just like the OpenAI API. `edge-tts` uses Microsoft Edge's online text-to-speech service, making it completely free. The project supports multiple audio formats, adjustable playback speed, and voice selection options, providing a flexible and customizable TTS solution for users.
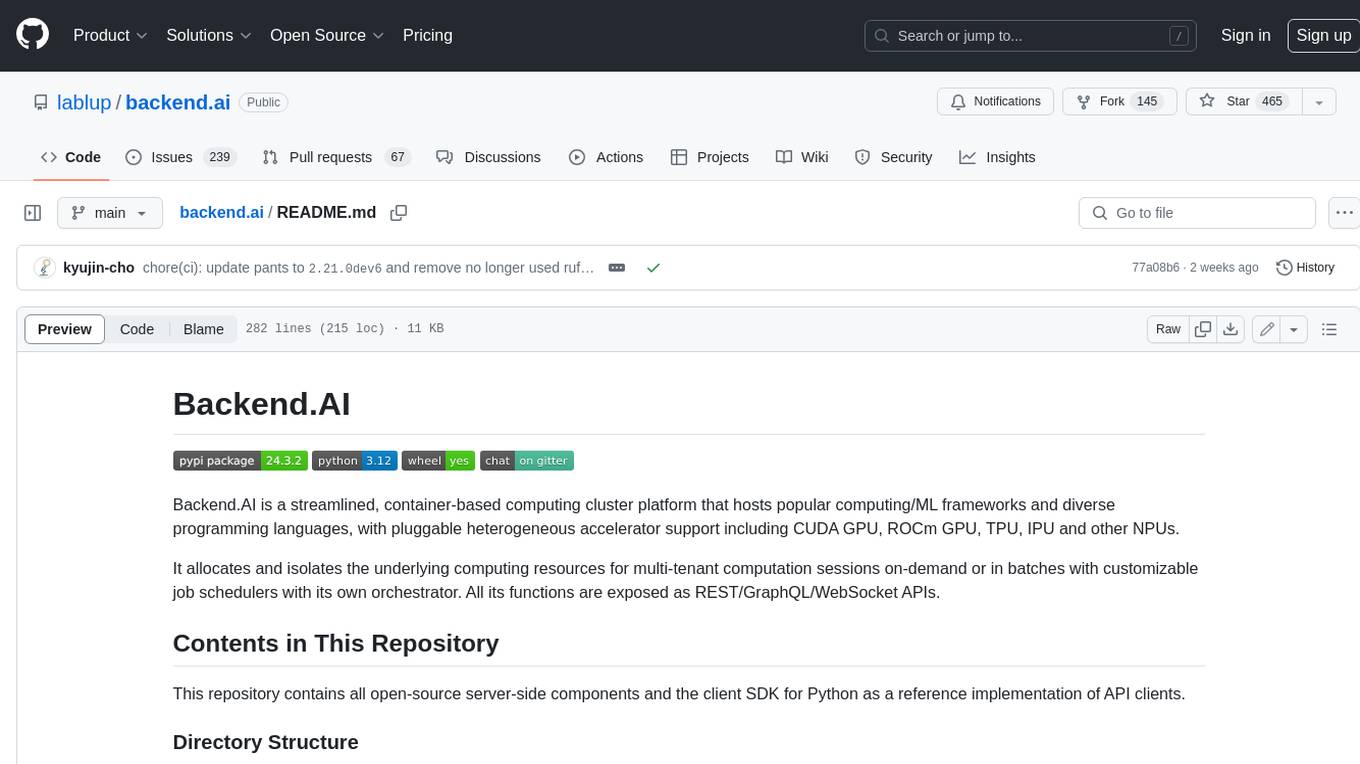
backend.ai
Backend.AI is a streamlined, container-based computing cluster platform that hosts popular computing/ML frameworks and diverse programming languages, with pluggable heterogeneous accelerator support including CUDA GPU, ROCm GPU, TPU, IPU and other NPUs. It allocates and isolates the underlying computing resources for multi-tenant computation sessions on-demand or in batches with customizable job schedulers with its own orchestrator. All its functions are exposed as REST/GraphQL/WebSocket APIs.
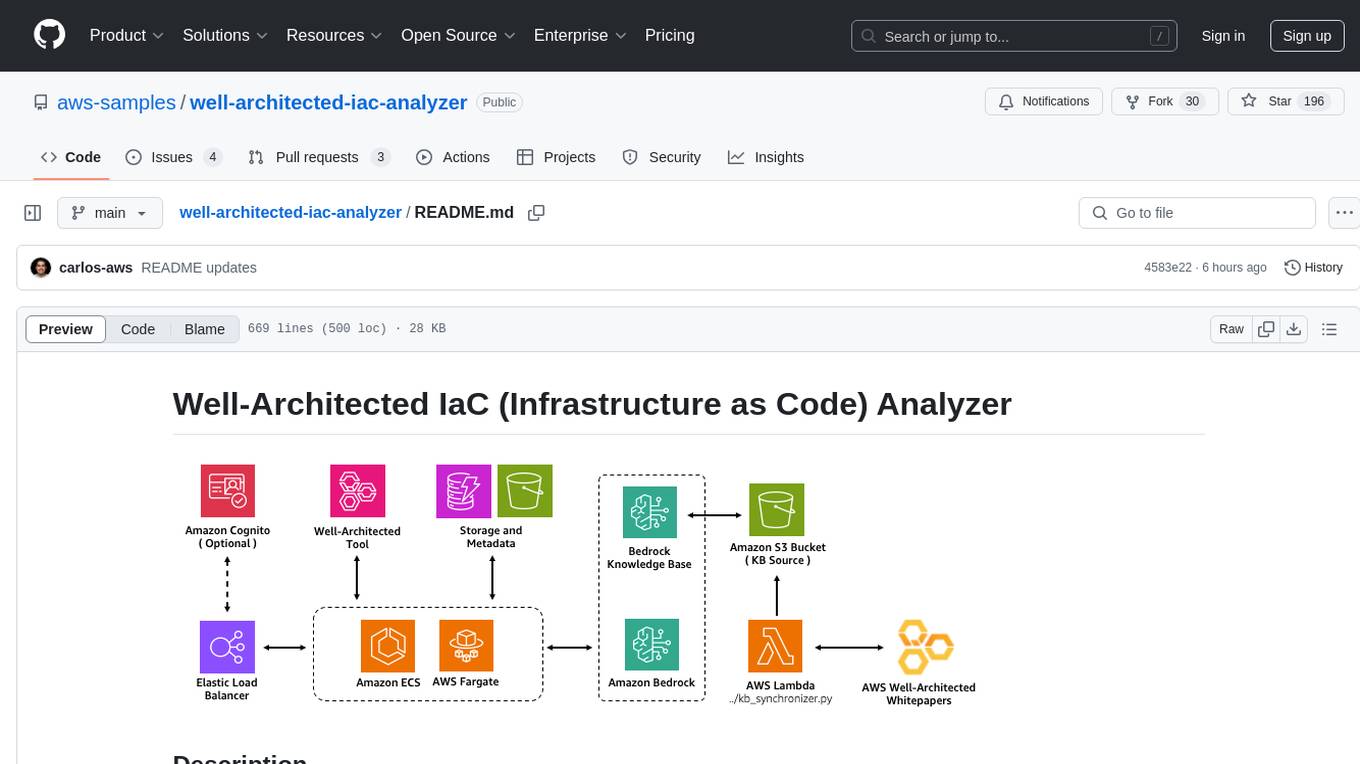
well-architected-iac-analyzer
Well-Architected Infrastructure as Code (IaC) Analyzer is a project demonstrating how generative AI can evaluate infrastructure code for alignment with best practices. It features a modern web application allowing users to upload IaC documents, complete IaC projects, or architecture diagrams for assessment. The tool provides insights into infrastructure code alignment with AWS best practices, offers suggestions for improving cloud architecture designs, and can generate IaC templates from architecture diagrams. Users can analyze CloudFormation, Terraform, or AWS CDK templates, architecture diagrams in PNG or JPEG format, and complete IaC projects with supporting documents. Real-time analysis against Well-Architected best practices, integration with AWS Well-Architected Tool, and export of analysis results and recommendations are included.
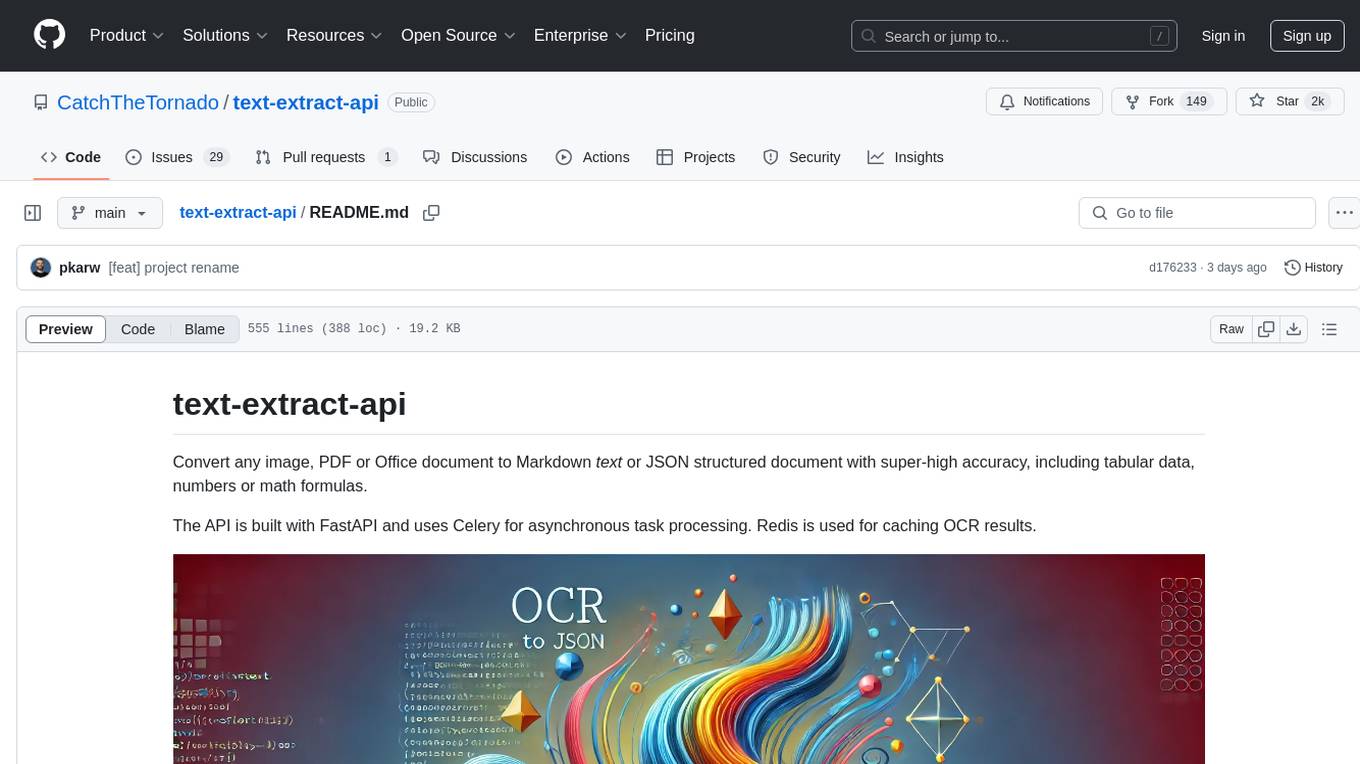
text-extract-api
The text-extract-api is a powerful tool that allows users to convert images, PDFs, or Office documents to Markdown text or JSON structured documents with high accuracy. It is built using FastAPI and utilizes Celery for asynchronous task processing, with Redis for caching OCR results. The tool provides features such as PDF/Office to Markdown and JSON conversion, improving OCR results with LLama, removing Personally Identifiable Information from documents, distributed queue processing, caching using Redis, switchable storage strategies, and a CLI tool for task management. Users can run the tool locally or on cloud services, with support for GPU processing. The tool also offers an online demo for testing purposes.
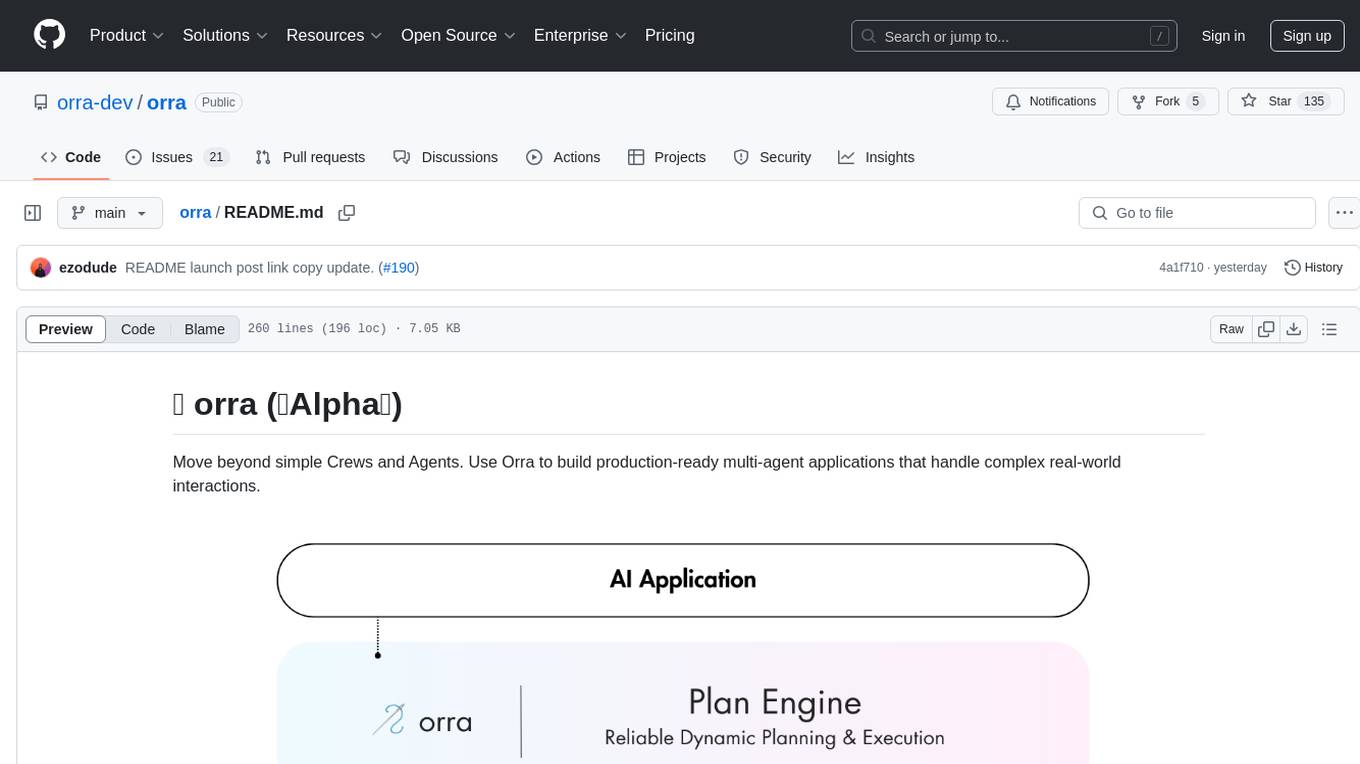
orra
Orra is a tool for building production-ready multi-agent applications that handle complex real-world interactions. It coordinates tasks across existing stack, agents, and tools run as services using intelligent reasoning. With features like smart pre-evaluated execution plans, domain grounding, durable execution, and automatic service health monitoring, Orra enables users to go fast with tools as services and revert state to handle failures. It provides real-time status tracking and webhook result delivery, making it ideal for developers looking to move beyond simple crews and agents.
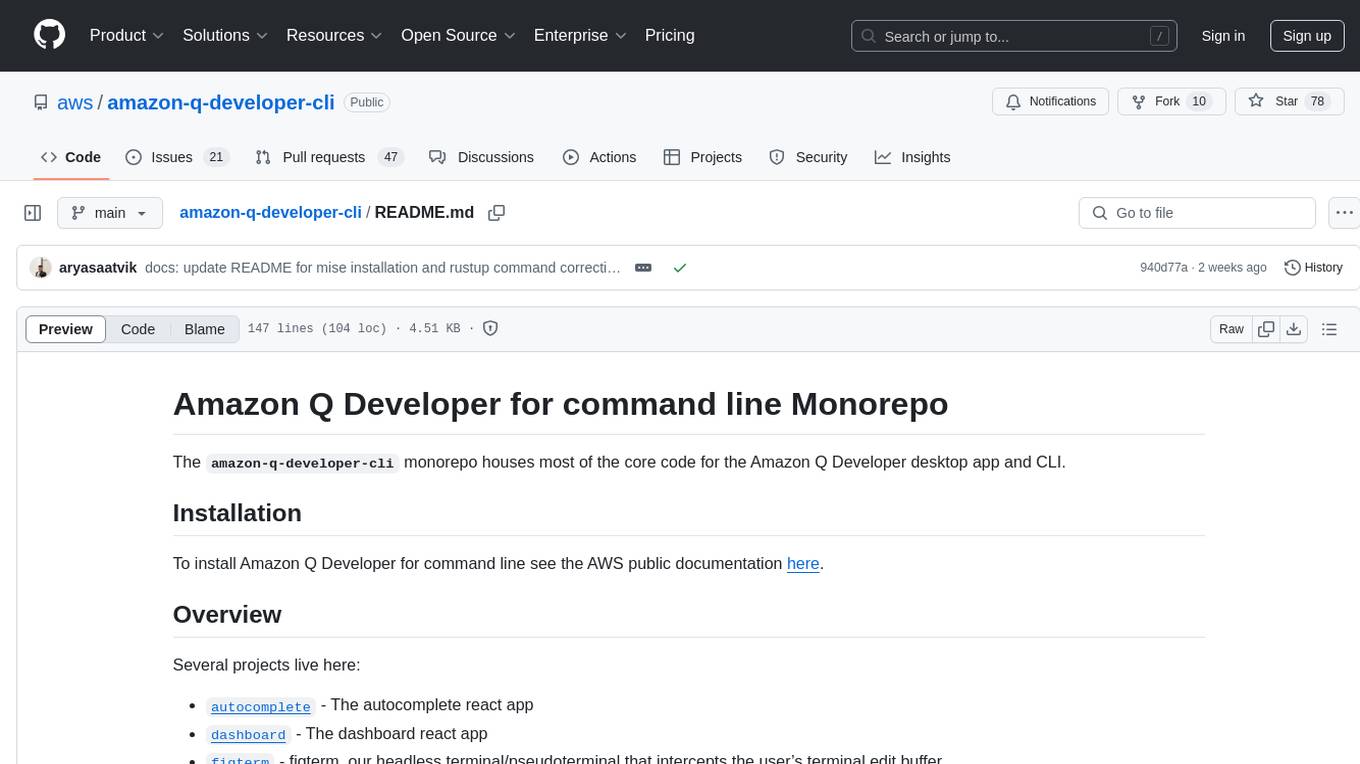
amazon-q-developer-cli
The `amazon-q-developer-cli` monorepo houses core code for the Amazon Q Developer desktop app and CLI. It includes projects like autocomplete, dashboard, figterm, q CLI, fig_desktop, fig_input_method, VSCode plugin, and JetBrains plugin. The repo also contains build scripts, internal rust crates, internal npm packages, protocol buffer message specification, and integration tests. The architecture involves different components communicating via IPC.
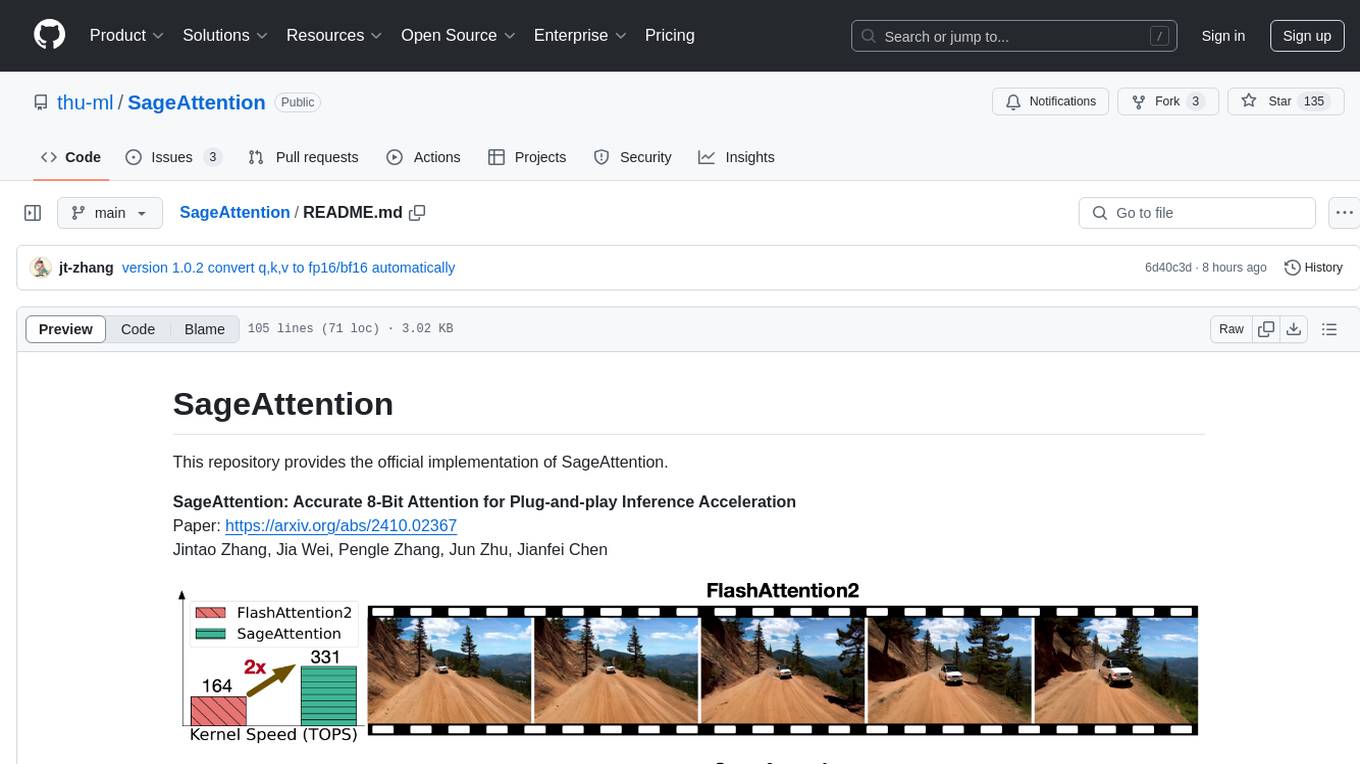
SageAttention
SageAttention is an official implementation of an accurate 8-bit attention mechanism for plug-and-play inference acceleration. It is optimized for RTX4090 and RTX3090 GPUs, providing performance improvements for specific GPU architectures. The tool offers a technique called 'smooth_k' to ensure accuracy in processing FP16/BF16 data. Users can easily replace 'scaled_dot_product_attention' with SageAttention for faster video processing.
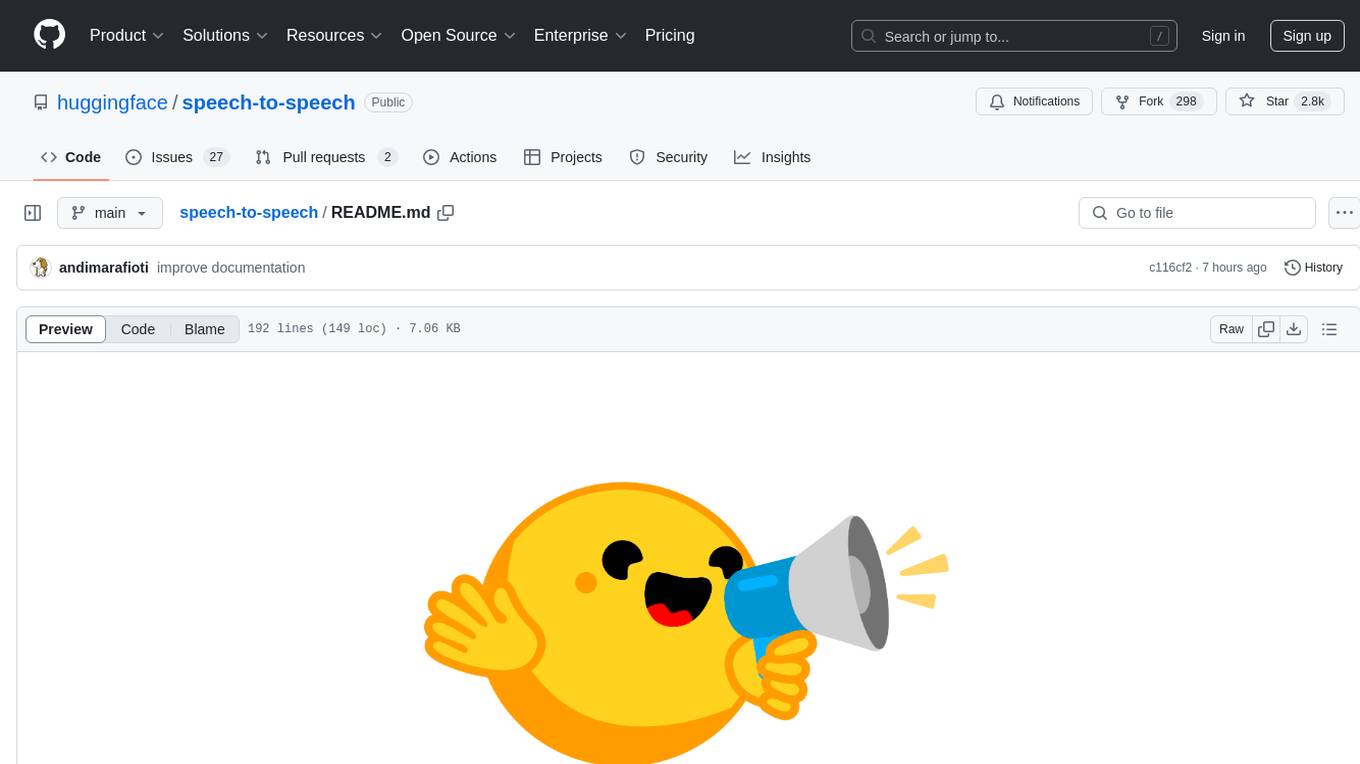
speech-to-speech
This repository implements a speech-to-speech cascaded pipeline with consecutive parts including Voice Activity Detection (VAD), Speech to Text (STT), Language Model (LM), and Text to Speech (TTS). It aims to provide a fully open and modular approach by leveraging models available on the Transformers library via the Hugging Face hub. The code is designed for easy modification, with each component implemented as a class. Users can run the pipeline either on a server/client approach or locally, with detailed setup and usage instructions provided in the readme.
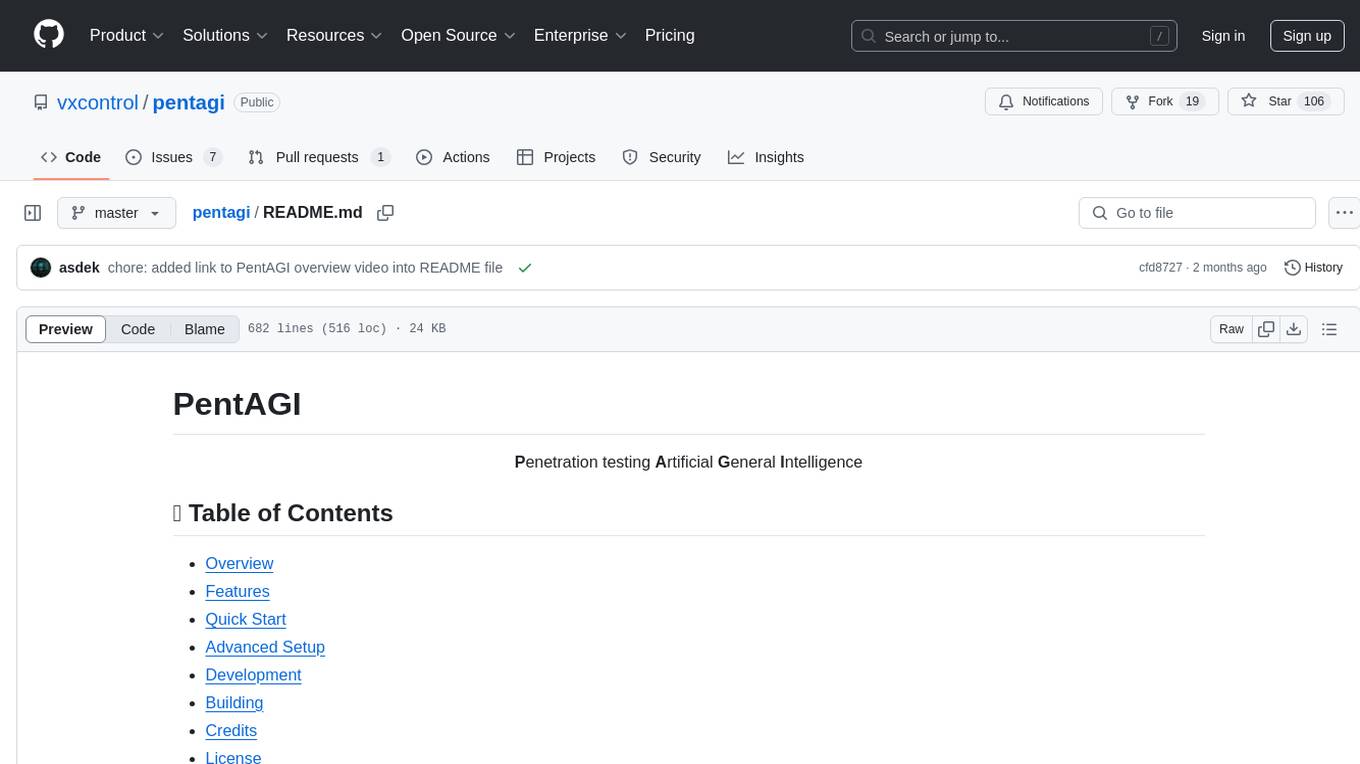
pentagi
PentAGI is an innovative tool for automated security testing that leverages cutting-edge artificial intelligence technologies. It is designed for information security professionals, researchers, and enthusiasts who need a powerful and flexible solution for conducting penetration tests. The tool provides secure and isolated operations in a sandboxed Docker environment, fully autonomous AI-powered agent for penetration testing steps, a suite of 20+ professional security tools, smart memory system for storing research results, web intelligence for gathering information, integration with external search systems, team delegation system, comprehensive monitoring and reporting, modern interface, API integration, persistent storage, scalable architecture, self-hosted solution, flexible authentication, and quick deployment through Docker Compose.
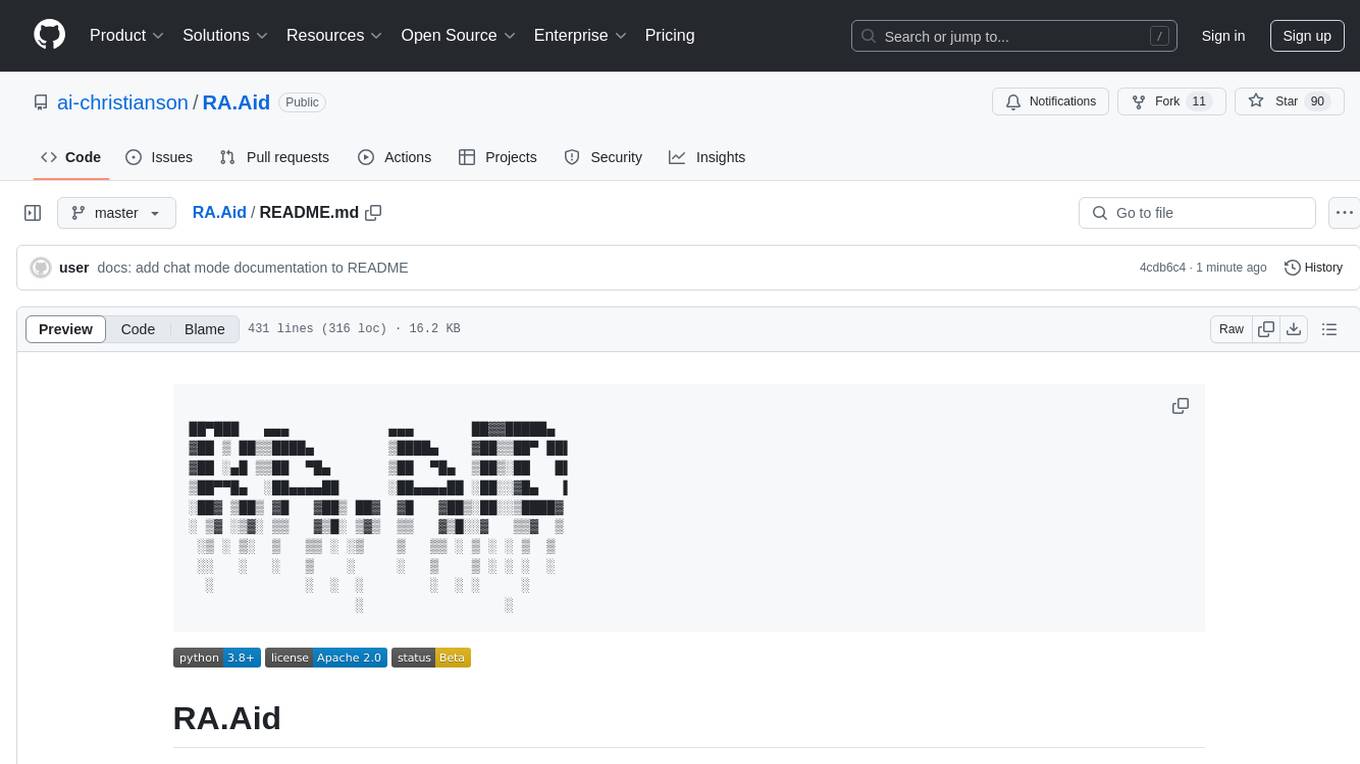
RA.Aid
RA.Aid is an AI software development agent powered by `aider` and advanced reasoning models like `o1`. It combines `aider`'s code editing capabilities with LangChain's agent-based task execution framework to provide an intelligent assistant for research, planning, and implementation of multi-step development tasks. It handles complex programming tasks by breaking them down into manageable steps, running shell commands automatically, and leveraging expert reasoning models like OpenAI's o1. RA.Aid is designed for everyday software development, offering features such as multi-step task planning, automated command execution, and the ability to handle complex programming tasks beyond single-shot code edits.
For similar tasks
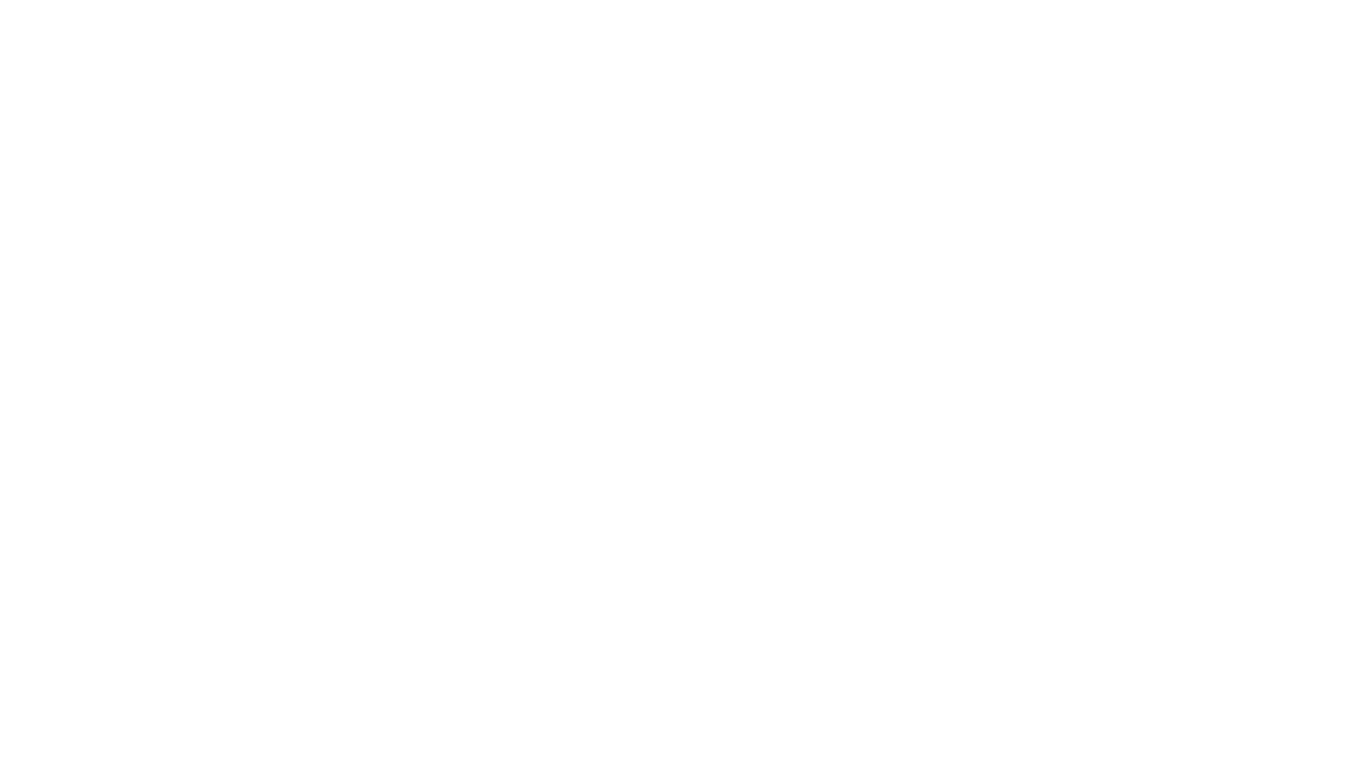
rlama
RLAMA is a powerful AI-driven question-answering tool that seamlessly integrates with local Ollama models. It enables users to create, manage, and interact with Retrieval-Augmented Generation (RAG) systems tailored to their documentation needs. RLAMA follows a clean architecture pattern with clear separation of concerns, focusing on lightweight and portable RAG capabilities with minimal dependencies. The tool processes documents, generates embeddings, stores RAG systems locally, and provides contextually-informed responses to user queries. Supported document formats include text, code, and various document types, with troubleshooting steps available for common issues like Ollama accessibility, text extraction problems, and relevance of answers.
For similar jobs
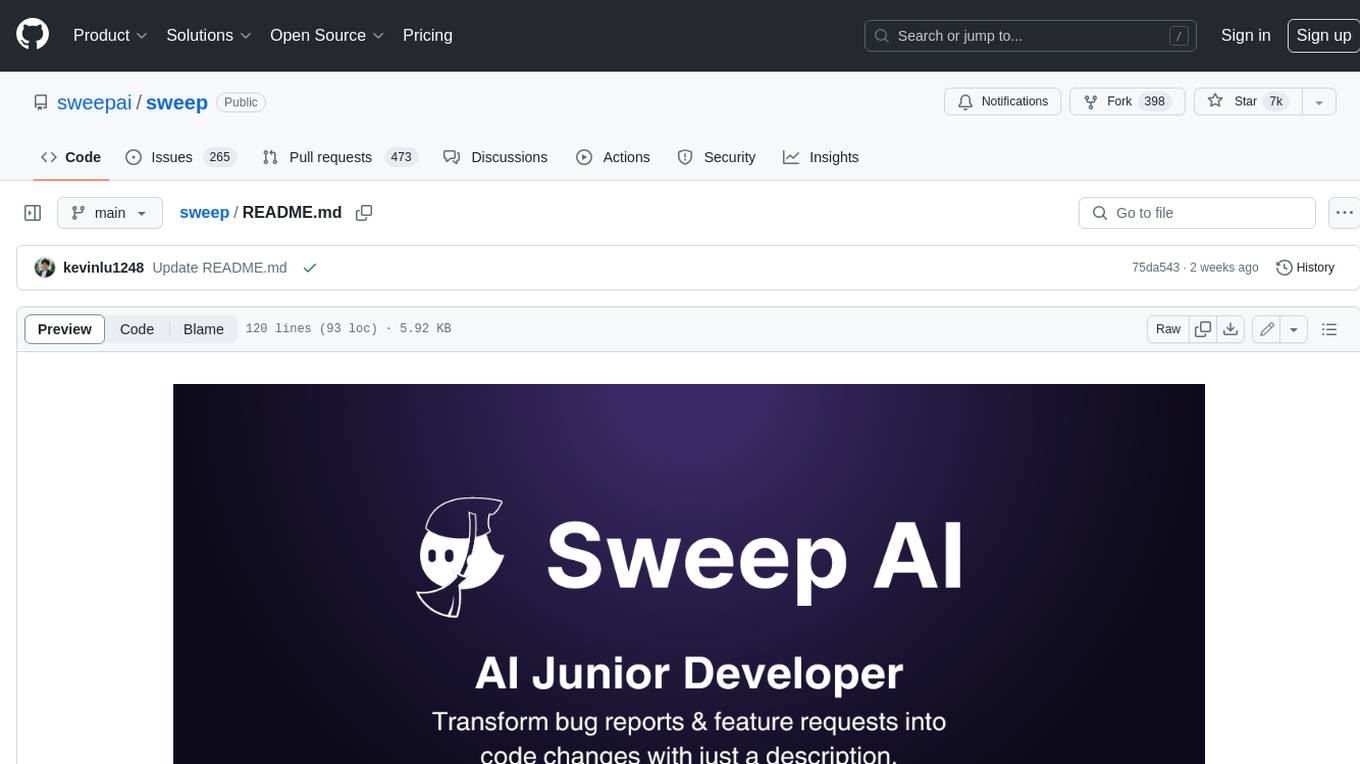
sweep
Sweep is an AI junior developer that turns bugs and feature requests into code changes. It automatically handles developer experience improvements like adding type hints and improving test coverage.
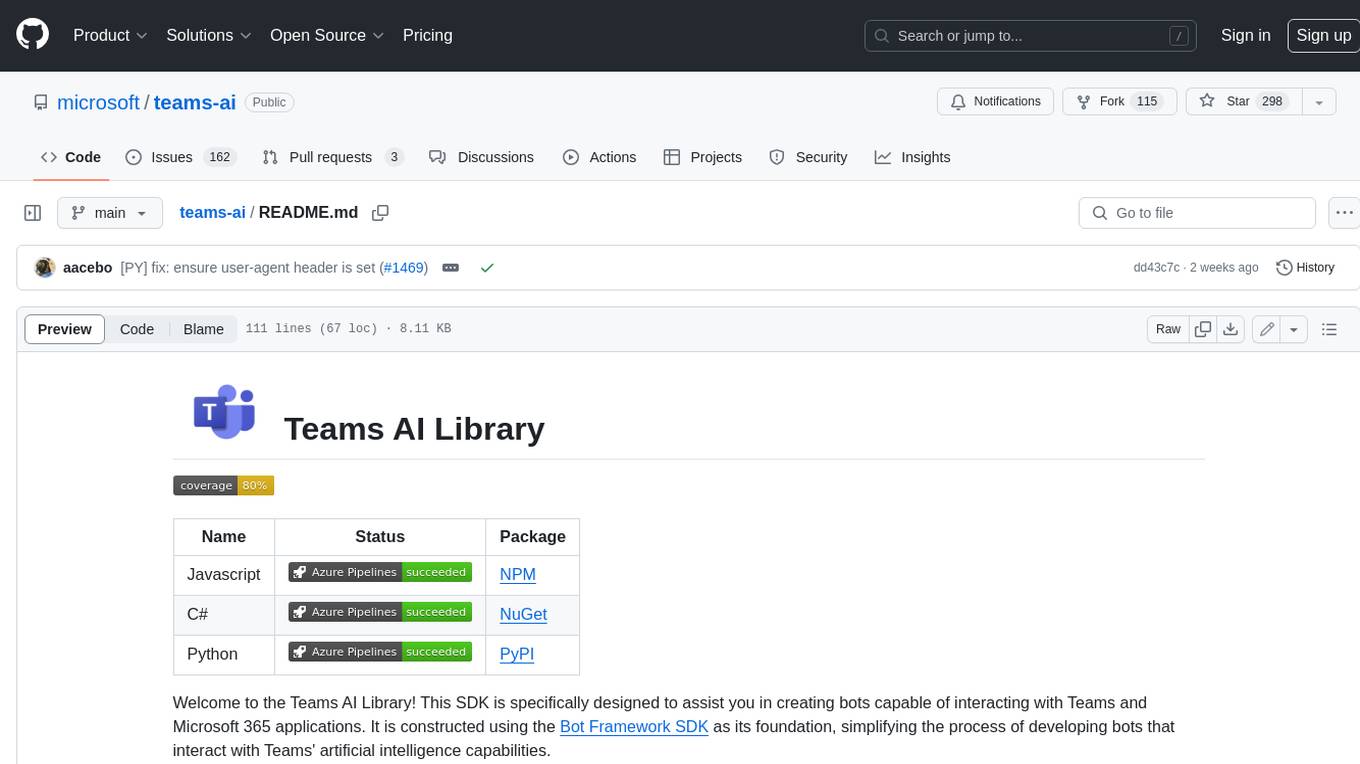
teams-ai
The Teams AI Library is a software development kit (SDK) that helps developers create bots that can interact with Teams and Microsoft 365 applications. It is built on top of the Bot Framework SDK and simplifies the process of developing bots that interact with Teams' artificial intelligence capabilities. The SDK is available for JavaScript/TypeScript, .NET, and Python.
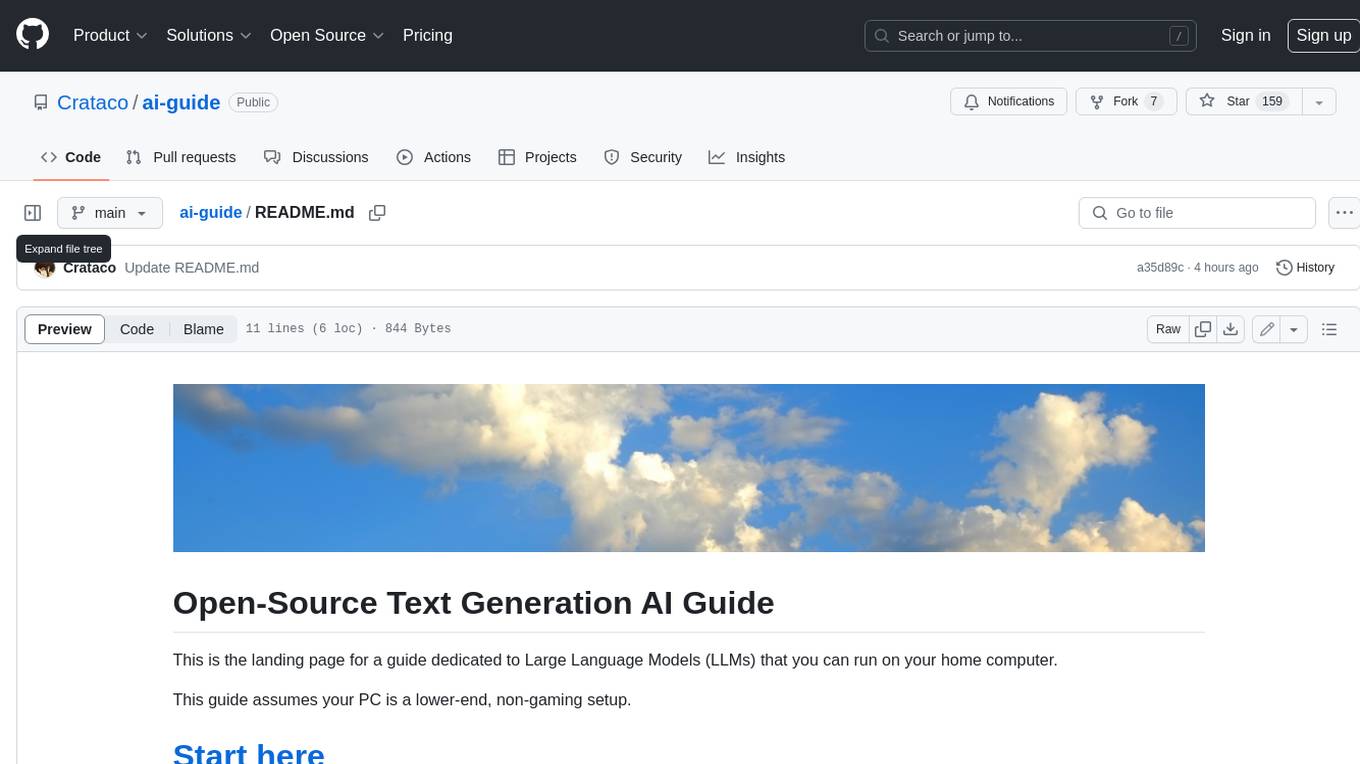
ai-guide
This guide is dedicated to Large Language Models (LLMs) that you can run on your home computer. It assumes your PC is a lower-end, non-gaming setup.
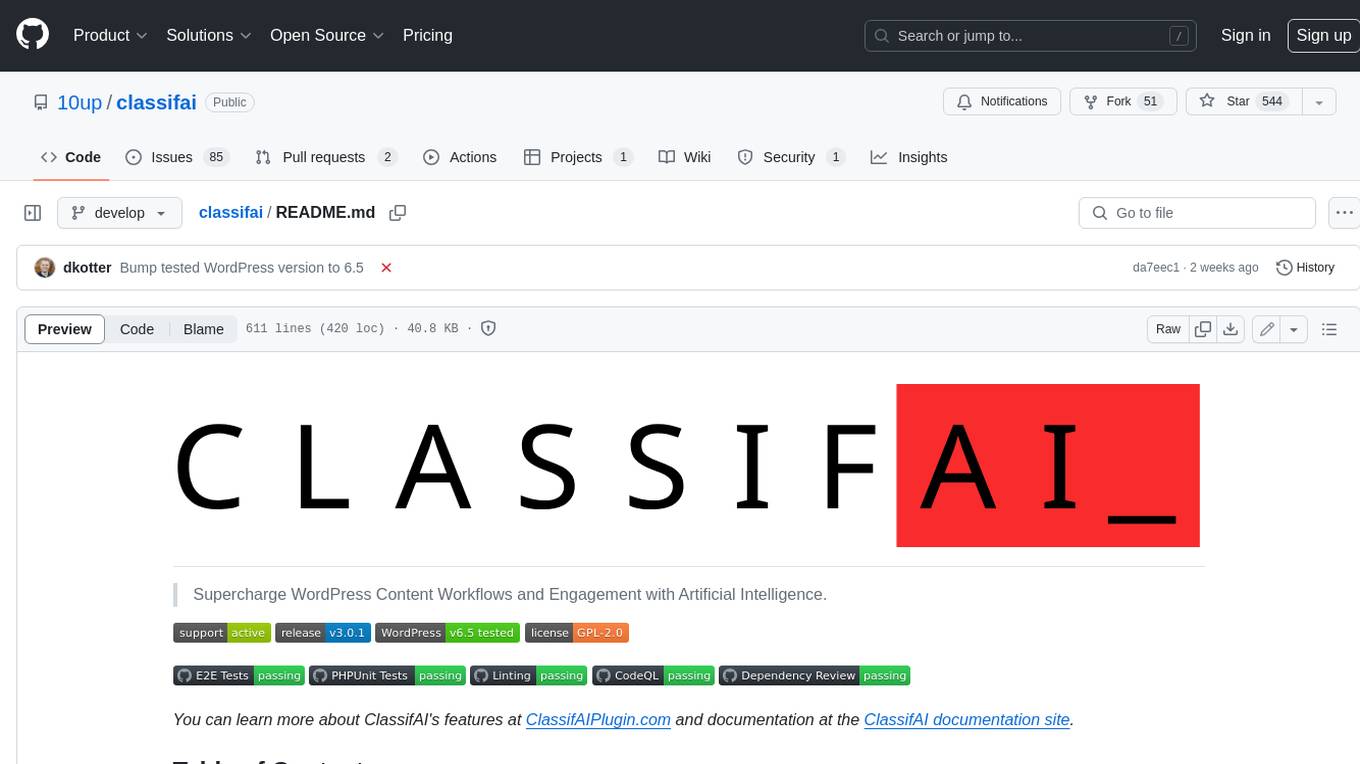
classifai
Supercharge WordPress Content Workflows and Engagement with Artificial Intelligence. Tap into leading cloud-based services like OpenAI, Microsoft Azure AI, Google Gemini and IBM Watson to augment your WordPress-powered websites. Publish content faster while improving SEO performance and increasing audience engagement. ClassifAI integrates Artificial Intelligence and Machine Learning technologies to lighten your workload and eliminate tedious tasks, giving you more time to create original content that matters.
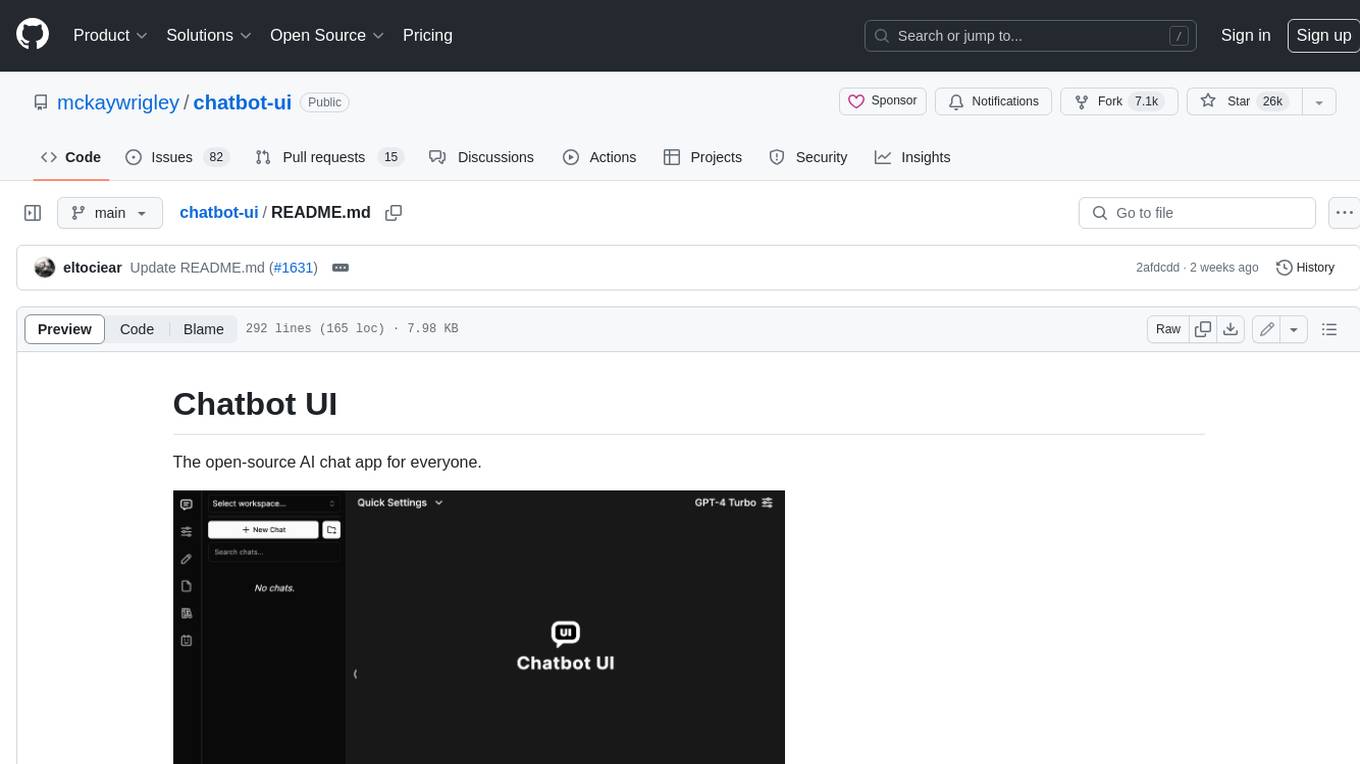
chatbot-ui
Chatbot UI is an open-source AI chat app that allows users to create and deploy their own AI chatbots. It is easy to use and can be customized to fit any need. Chatbot UI is perfect for businesses, developers, and anyone who wants to create a chatbot.
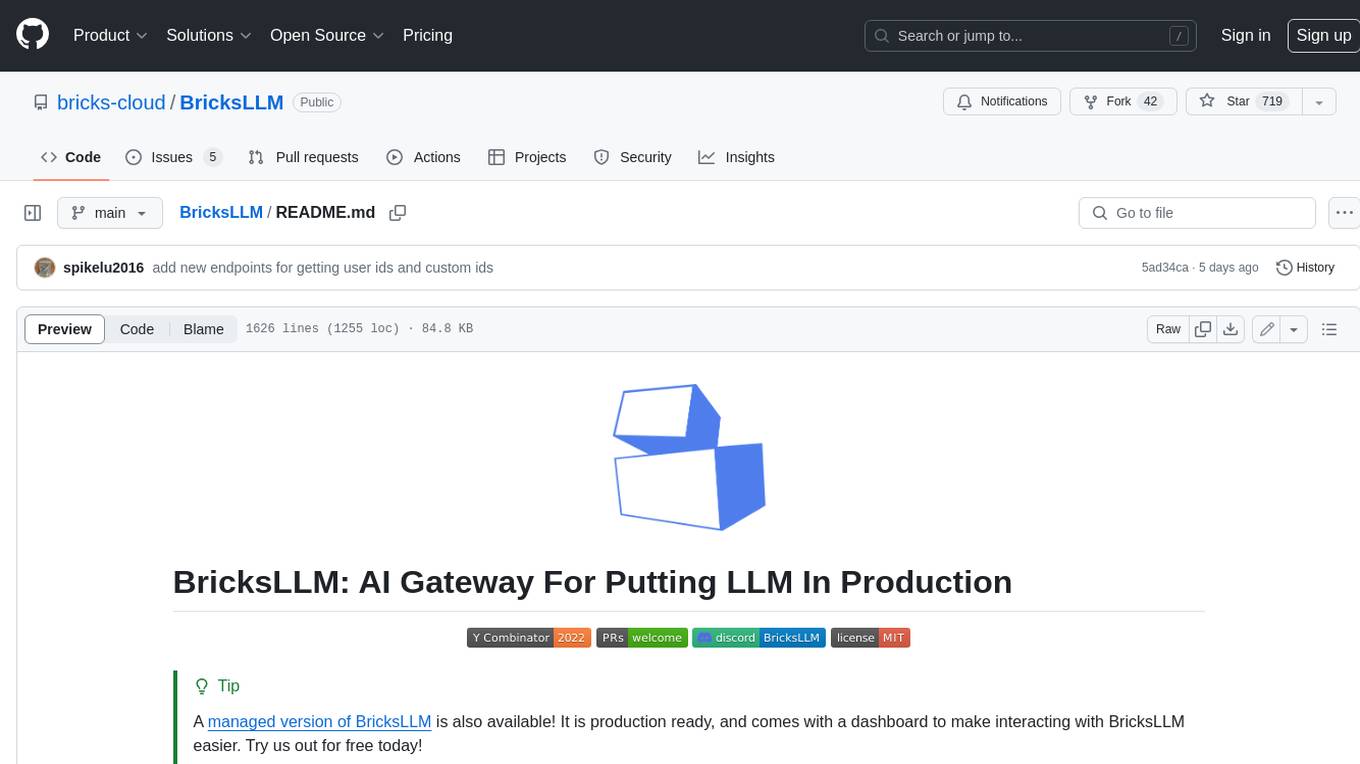
BricksLLM
BricksLLM is a cloud native AI gateway written in Go. Currently, it provides native support for OpenAI, Anthropic, Azure OpenAI and vLLM. BricksLLM aims to provide enterprise level infrastructure that can power any LLM production use cases. Here are some use cases for BricksLLM: * Set LLM usage limits for users on different pricing tiers * Track LLM usage on a per user and per organization basis * Block or redact requests containing PIIs * Improve LLM reliability with failovers, retries and caching * Distribute API keys with rate limits and cost limits for internal development/production use cases * Distribute API keys with rate limits and cost limits for students
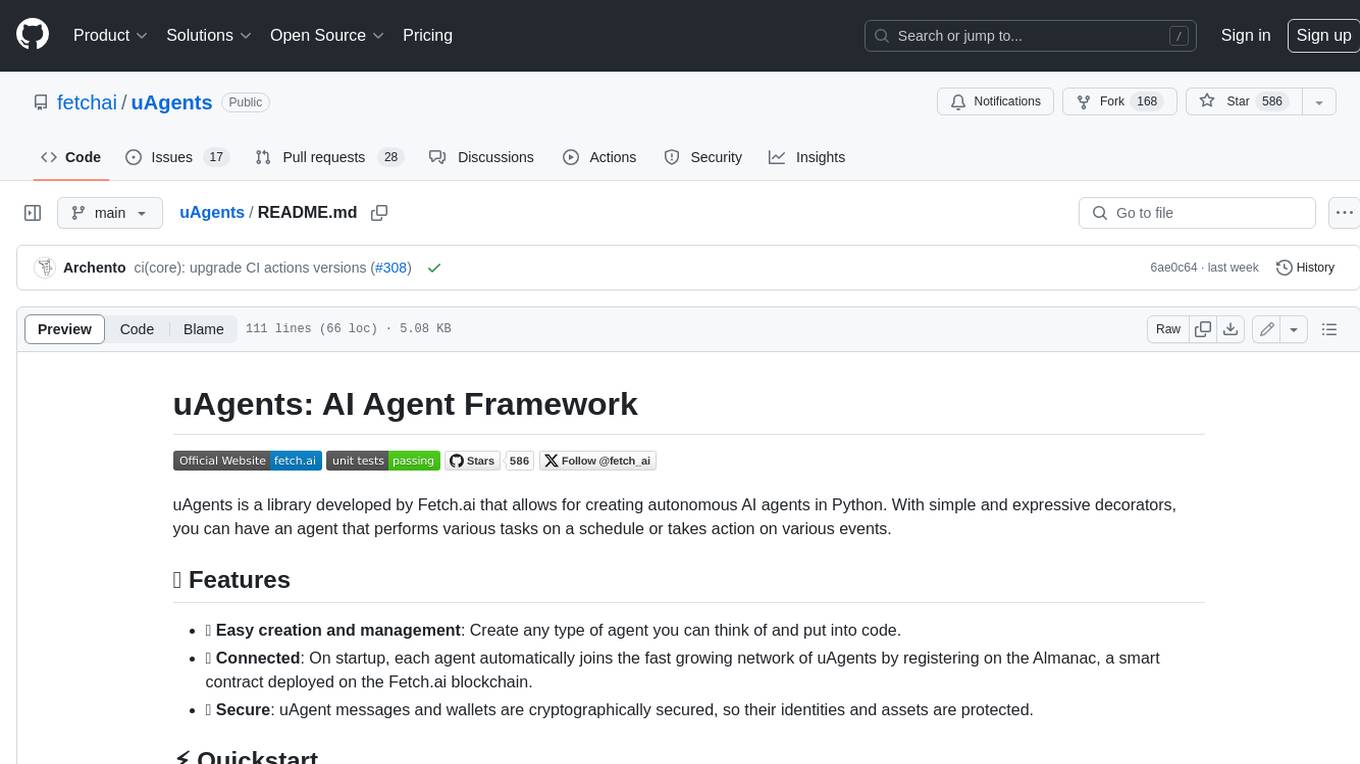
uAgents
uAgents is a Python library developed by Fetch.ai that allows for the creation of autonomous AI agents. These agents can perform various tasks on a schedule or take action on various events. uAgents are easy to create and manage, and they are connected to a fast-growing network of other uAgents. They are also secure, with cryptographically secured messages and wallets.
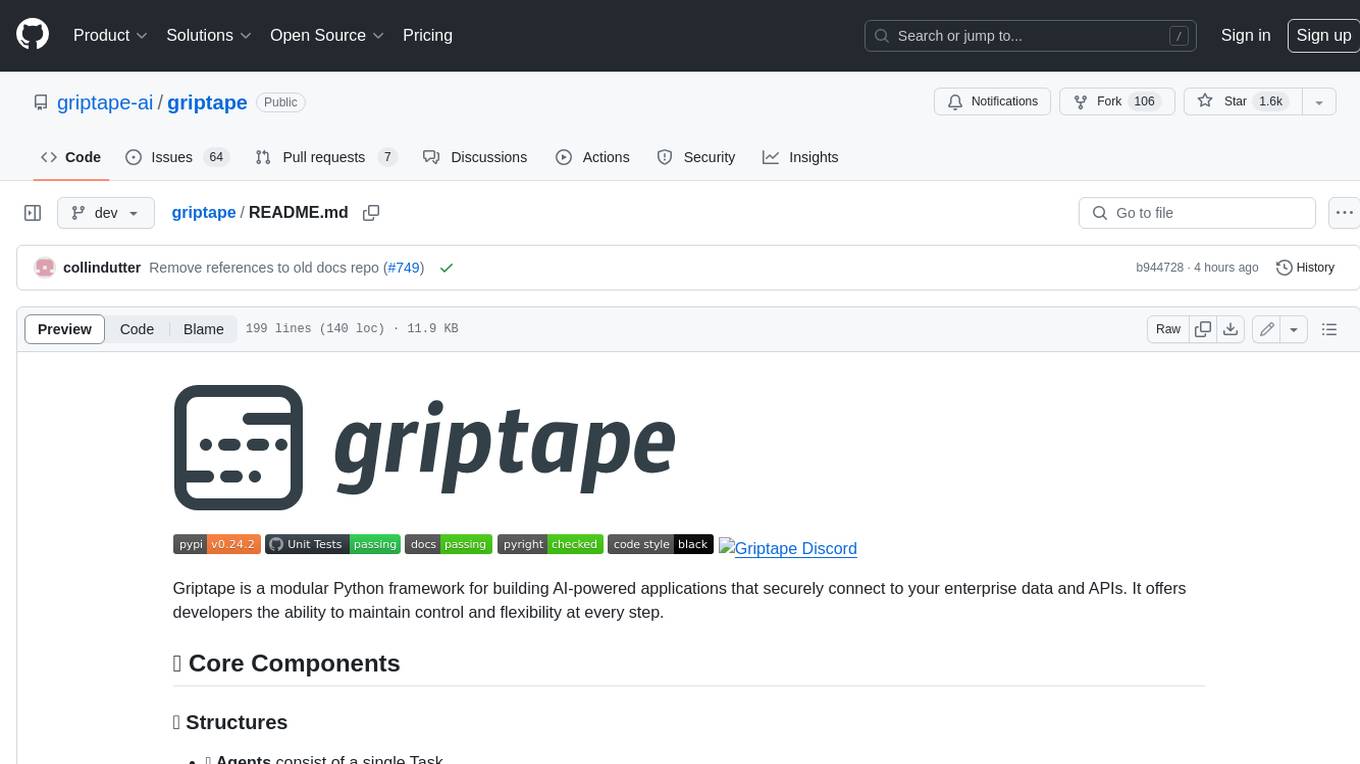
griptape
Griptape is a modular Python framework for building AI-powered applications that securely connect to your enterprise data and APIs. It offers developers the ability to maintain control and flexibility at every step. Griptape's core components include Structures (Agents, Pipelines, and Workflows), Tasks, Tools, Memory (Conversation Memory, Task Memory, and Meta Memory), Drivers (Prompt and Embedding Drivers, Vector Store Drivers, Image Generation Drivers, Image Query Drivers, SQL Drivers, Web Scraper Drivers, and Conversation Memory Drivers), Engines (Query Engines, Extraction Engines, Summary Engines, Image Generation Engines, and Image Query Engines), and additional components (Rulesets, Loaders, Artifacts, Chunkers, and Tokenizers). Griptape enables developers to create AI-powered applications with ease and efficiency.