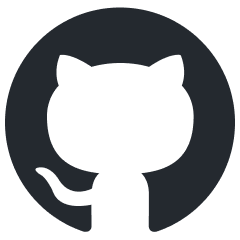
forge
AI enabled shell
Stars: 94
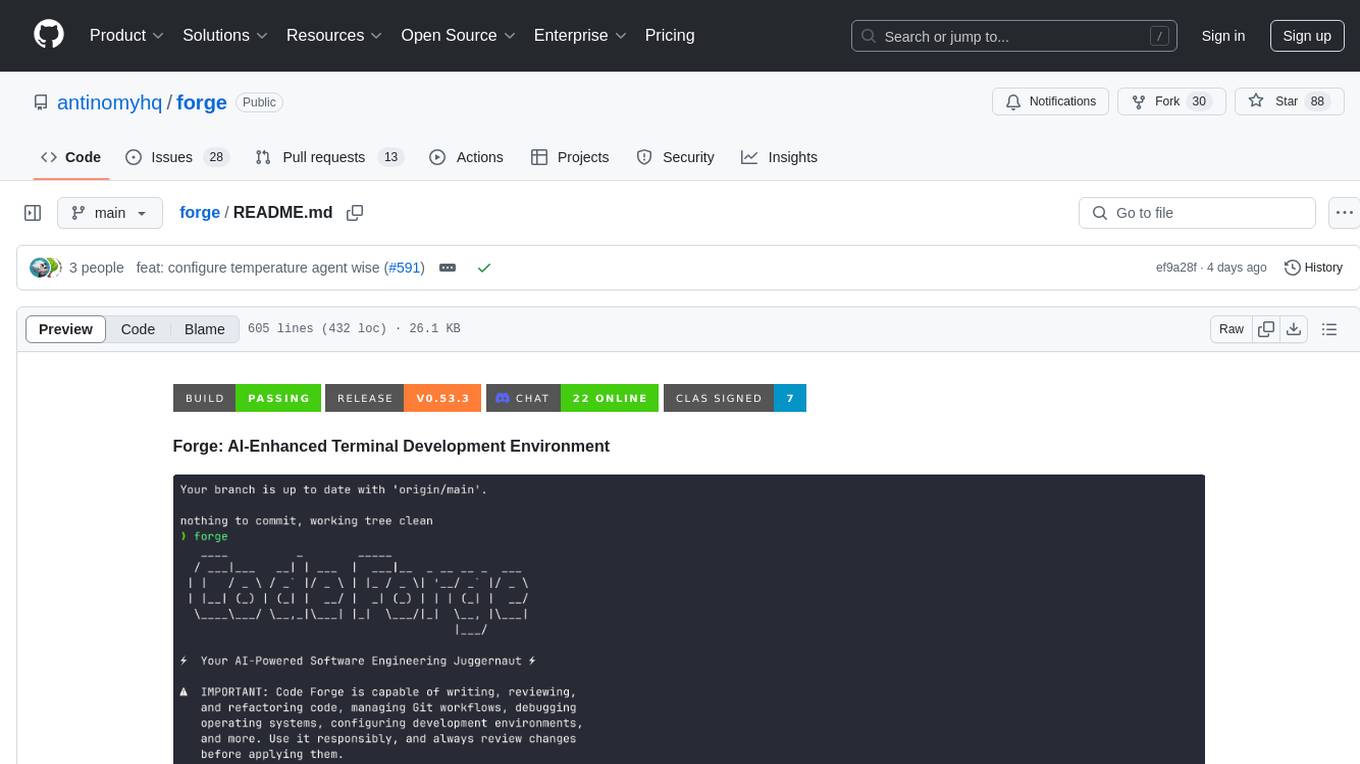
README:
Forge is a comprehensive coding agent that integrates AI capabilities with your development environment, offering sophisticated assistance while maintaining the efficiency of your existing workflow. ✨
Install Forge globally using npm:
# Install Forge globally using npm
npm install -g @antinomyhq/forge
# Or run directly without installation using npx
npx @antinomyhq/forge
This method works on Windows, macOS, and Linux, providing a consistent installation experience across all platforms. 💻
-
Create a
.env
file in your home directory with your API credentials:# Your API key for accessing AI models OPENROUTER_API_KEY=<Enter your Open Router Key> # Optional: Set a custom URL for OpenAI-compatible providers OPENAI_URL=https://custom-openai-provider.com/v1 # Optional: Set a custom URL for Anthropic ANTHROPIC_URL=https://custom-anthropic-provider.com/v1
You can get a Key at Open Router 🔑
-
Launch Code Forge: Type
@
and press[tab]
to tag files. You can also use and define custom slash commands. 🖥️
For comprehensive documentation on all features and capabilities, please visit the documentation site.
Join our vibrant Discord community to connect with other Code-Forge users and contributors, get help with your projects, share ideas, and provide feedback! 🌟
Your support drives Code-Forge's continued evolution! By starring our GitHub repository, you:
- Help others discover this powerful tool 🔍
- Motivate our development team 💪
- Enable us to prioritize new features 🛠️
- Strengthen our open-source community 🌱
For Tasks:
Click tags to check more tools for each tasksFor Jobs:
Alternative AI tools for forge
Similar Open Source Tools
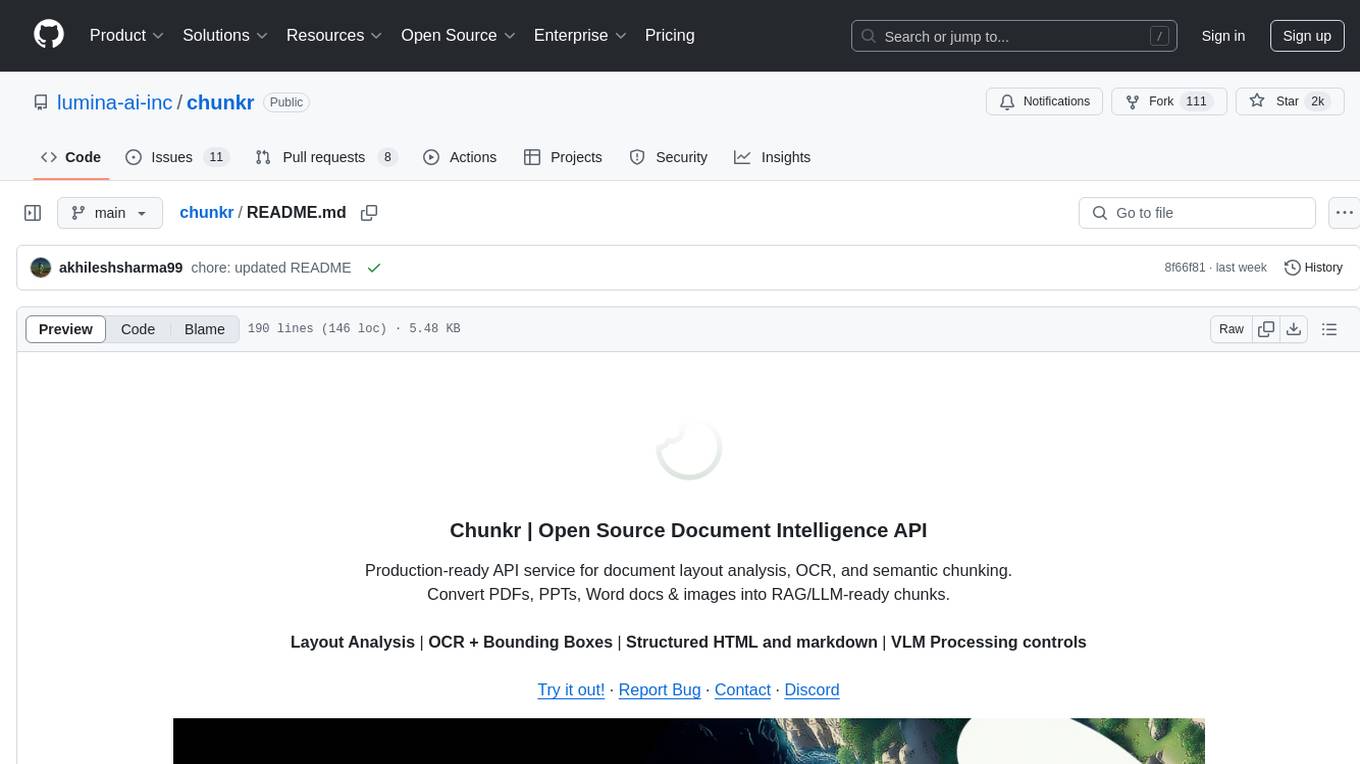
chunkr
Chunkr is an open-source document intelligence API that provides a production-ready service for document layout analysis, OCR, and semantic chunking. It allows users to convert PDFs, PPTs, Word docs, and images into RAG/LLM-ready chunks. The API offers features such as layout analysis, OCR with bounding boxes, structured HTML and markdown output, and VLM processing controls. Users can interact with Chunkr through a Python SDK, enabling them to upload documents, process them, and export results in various formats. The tool also supports self-hosted deployment options using Docker Compose or Kubernetes, with configurations for different AI models like OpenAI, Google AI Studio, and OpenRouter. Chunkr is dual-licensed under the GNU Affero General Public License v3.0 (AGPL-3.0) and a commercial license, providing flexibility for different usage scenarios.
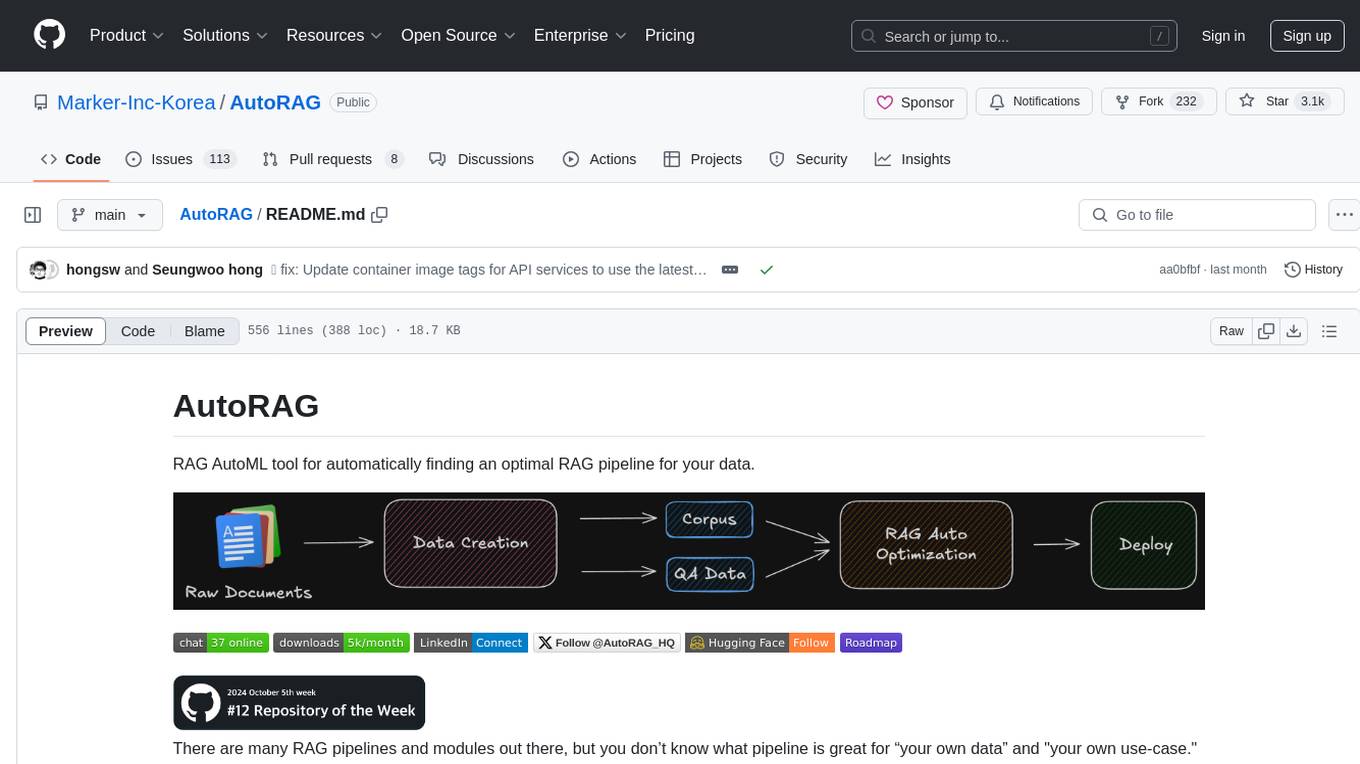
AutoRAG
AutoRAG is an AutoML tool designed to automatically find the optimal RAG pipeline for your data. It simplifies the process of evaluating various RAG modules to identify the best pipeline for your specific use-case. The tool supports easy evaluation of different module combinations, making it efficient to find the most suitable RAG pipeline for your needs. AutoRAG also offers a cloud beta version to assist users in running and optimizing the tool, along with building RAG evaluation datasets for a starting price of $9.99 per optimization.
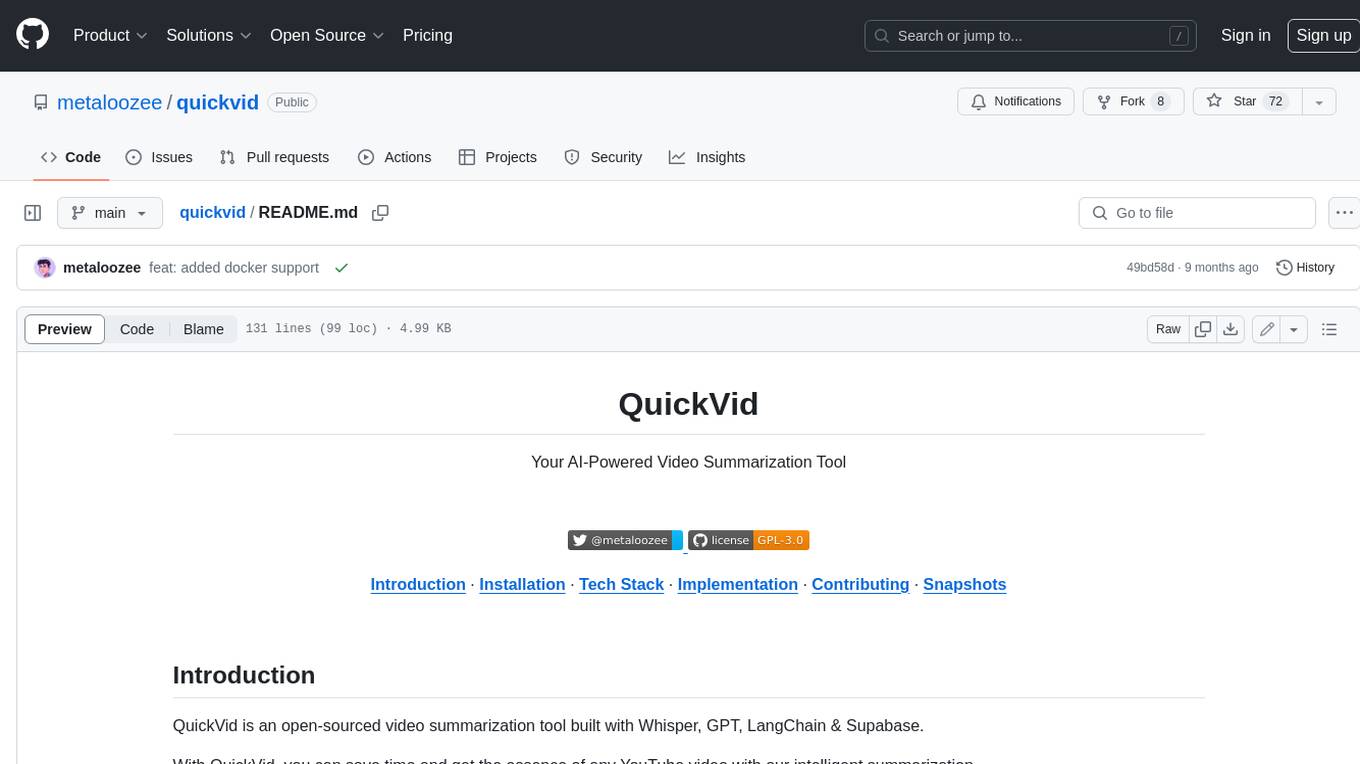
quickvid
QuickVid is an open-source video summarization tool that uses AI to generate summaries of YouTube videos. It is built with Whisper, GPT, LangChain, and Supabase. QuickVid can be used to save time and get the essence of any YouTube video with intelligent summarization.
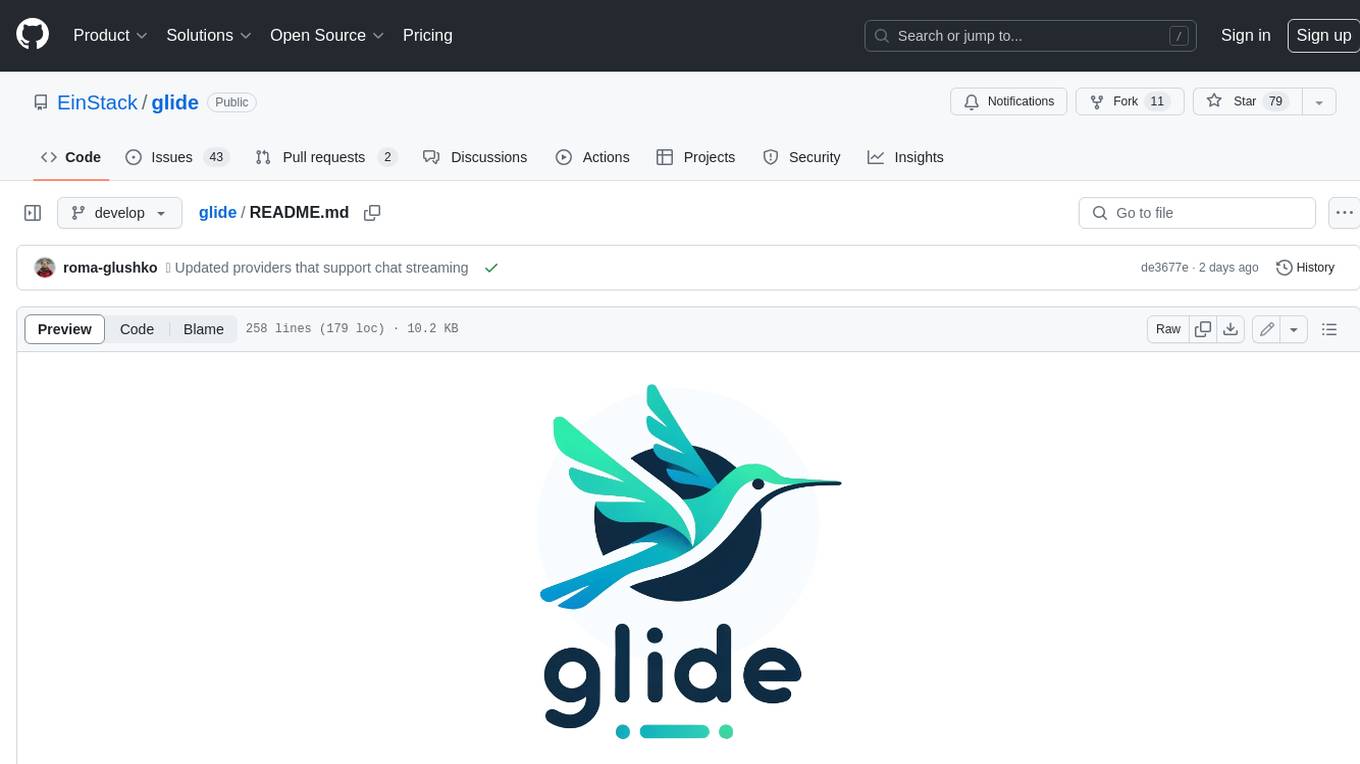
glide
Glide is a cloud-native LLM gateway that provides a unified REST API for accessing various large language models (LLMs) from different providers. It handles LLMOps tasks such as model failover, caching, key management, and more, making it easy to integrate LLMs into applications. Glide supports popular LLM providers like OpenAI, Anthropic, Azure OpenAI, AWS Bedrock (Titan), Cohere, Google Gemini, OctoML, and Ollama. It offers high availability, performance, and observability, and provides SDKs for Python and NodeJS to simplify integration.
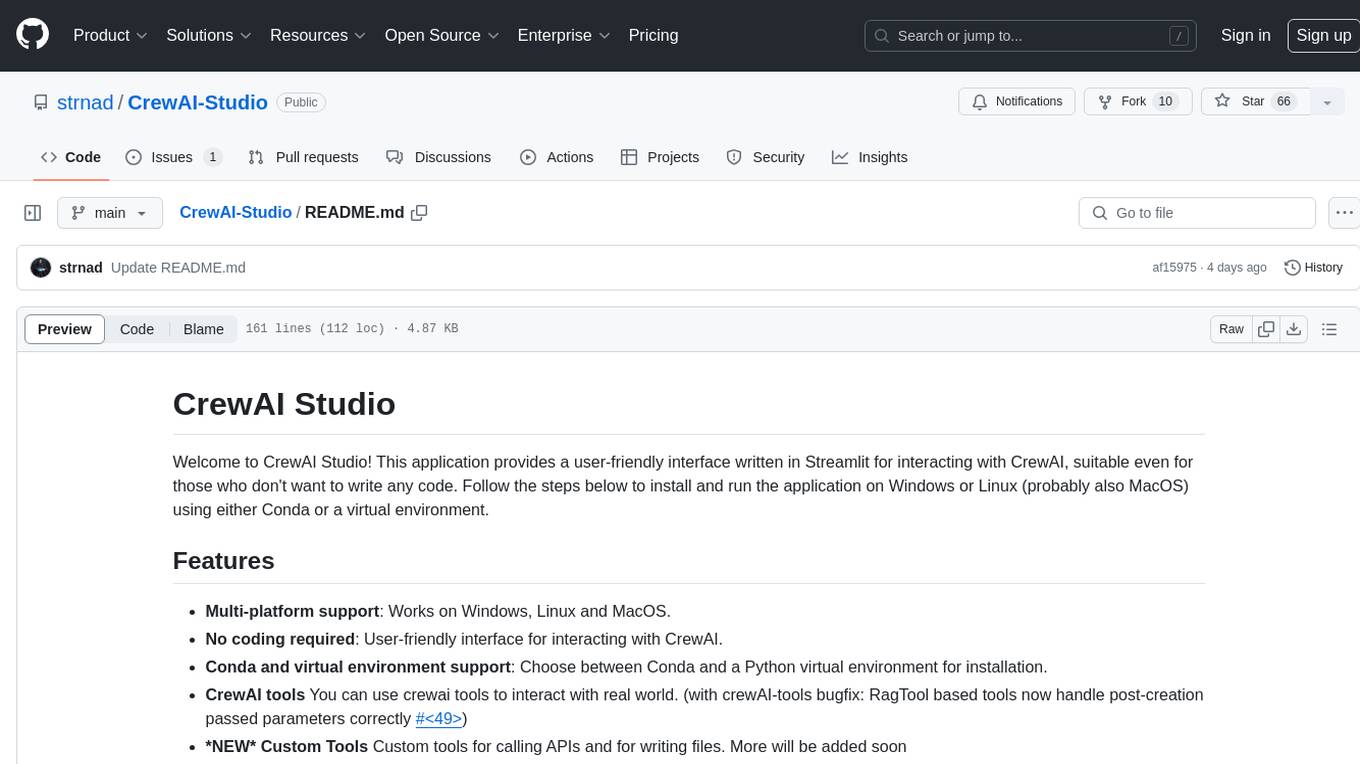
CrewAI-Studio
CrewAI Studio is an application with a user-friendly interface for interacting with CrewAI, offering support for multiple platforms and various backend providers. It allows users to run crews in the background, export single-page apps, and use custom tools for APIs and file writing. The roadmap includes features like better import/export, human input, chat functionality, automatic crew creation, and multiuser environment support.
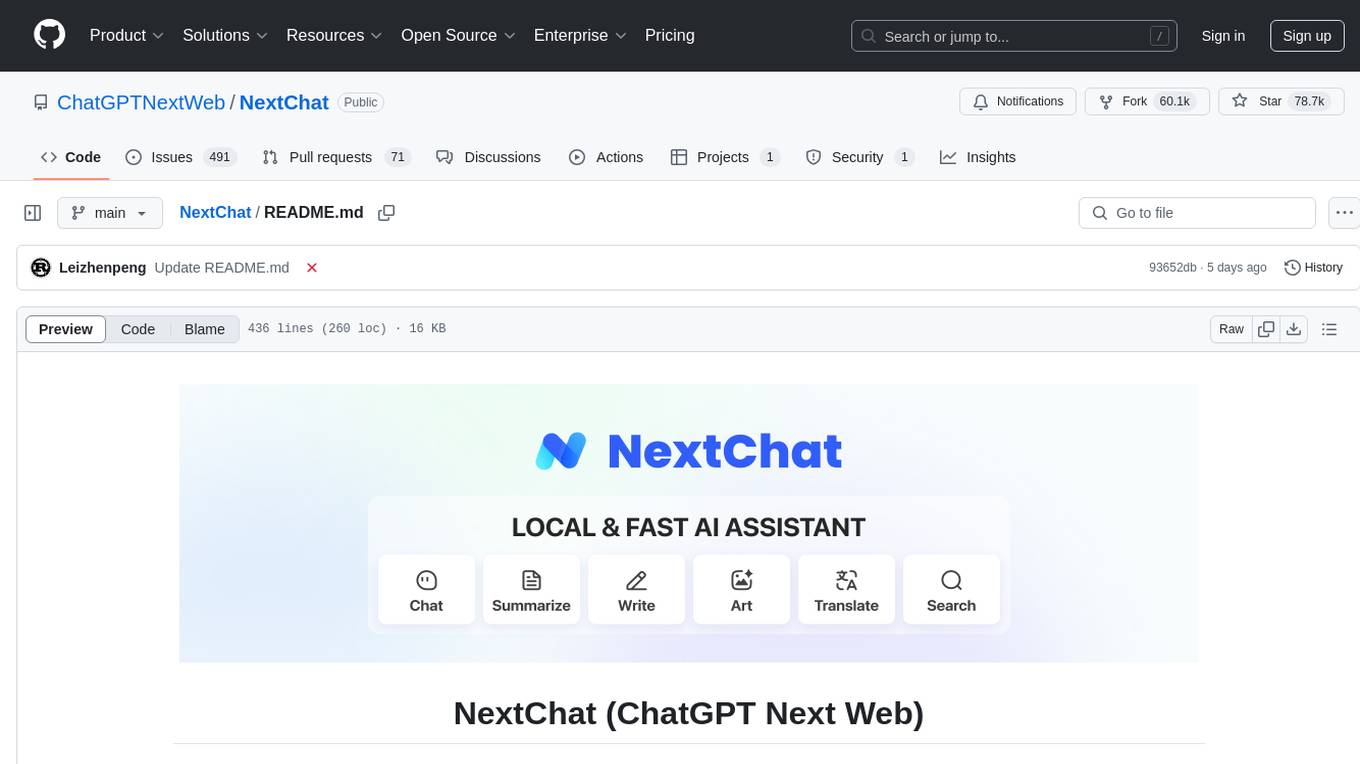
NextChat
NextChat is a well-designed cross-platform ChatGPT web UI tool that supports Claude, GPT4, and Gemini Pro. It offers a compact client for Linux, Windows, and MacOS, with features like self-deployed LLMs compatibility, privacy-first data storage, markdown support, responsive design, and fast loading speed. Users can create, share, and debug chat tools with prompt templates, access various prompts, compress chat history, and use multiple languages. The tool also supports enterprise-level privatization and customization deployment, with features like brand customization, resource integration, permission control, knowledge integration, security auditing, private deployment, and continuous updates.
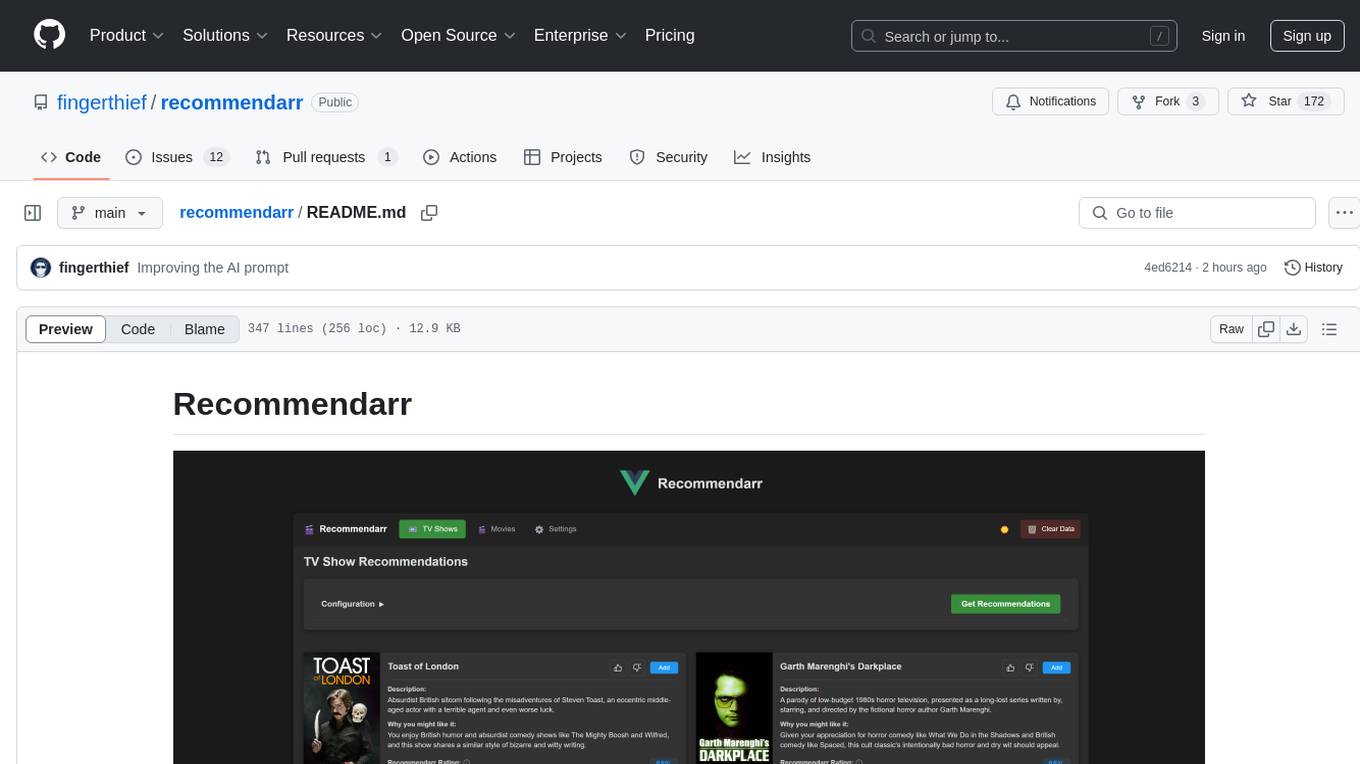
recommendarr
Recommendarr is a tool that generates personalized TV show and movie recommendations based on your Sonarr, Radarr, Plex, and Jellyfin libraries using AI. It offers AI-powered recommendations, media server integration, flexible AI support, watch history analysis, customization options, and dark/light mode toggle. Users can connect their media libraries and watch history services, configure AI service settings, and get personalized recommendations based on genre, language, and mood/vibe preferences. The tool works with any OpenAI-compatible API and offers various recommended models for different cost options and performance levels. It provides personalized suggestions, detailed information, filter options, watch history analysis, and one-click adding of recommended content to Sonarr/Radarr.
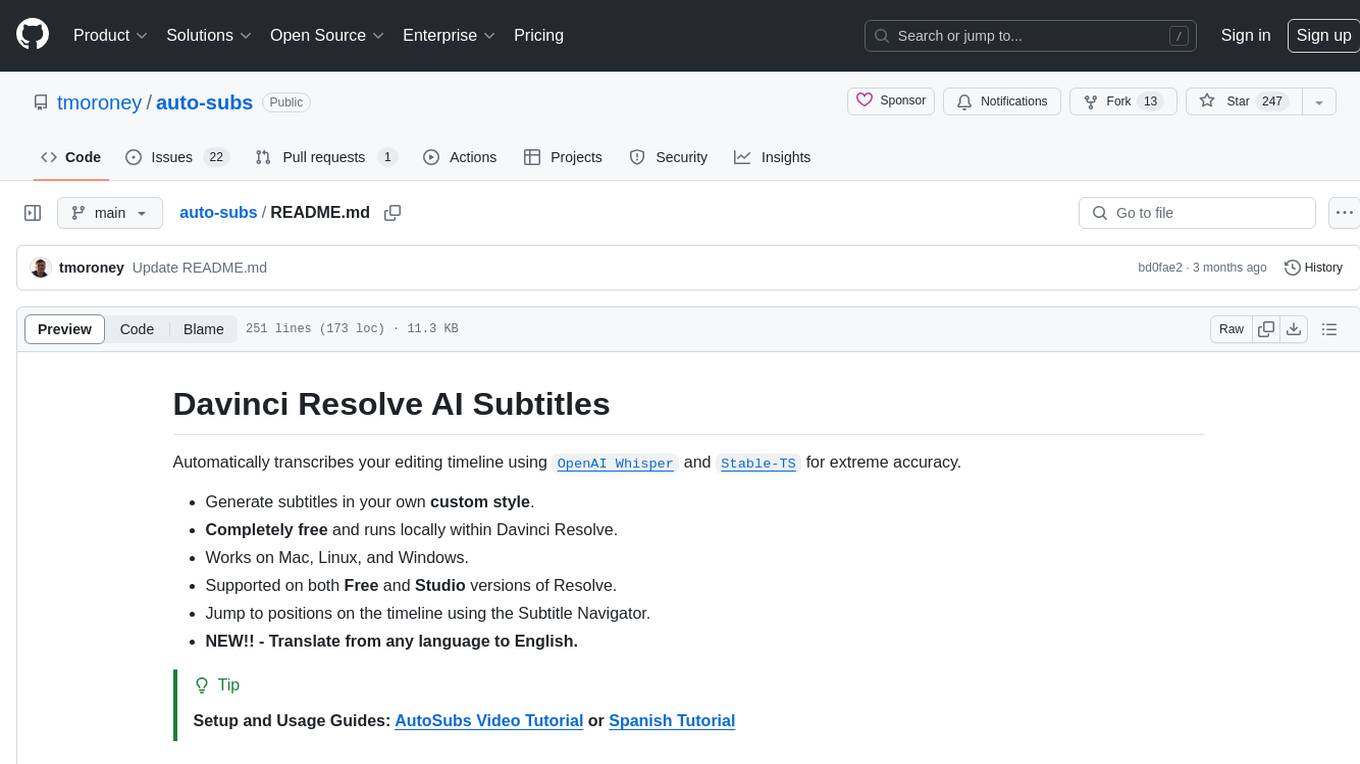
auto-subs
Auto-subs is a tool designed to automatically transcribe editing timelines using OpenAI Whisper and Stable-TS for extreme accuracy. It generates subtitles in a custom style, is completely free, and runs locally within Davinci Resolve. It works on Mac, Linux, and Windows, supporting both Free and Studio versions of Resolve. Users can jump to positions on the timeline using the Subtitle Navigator and translate from any language to English. The tool provides a user-friendly interface for creating and customizing subtitles for video content.
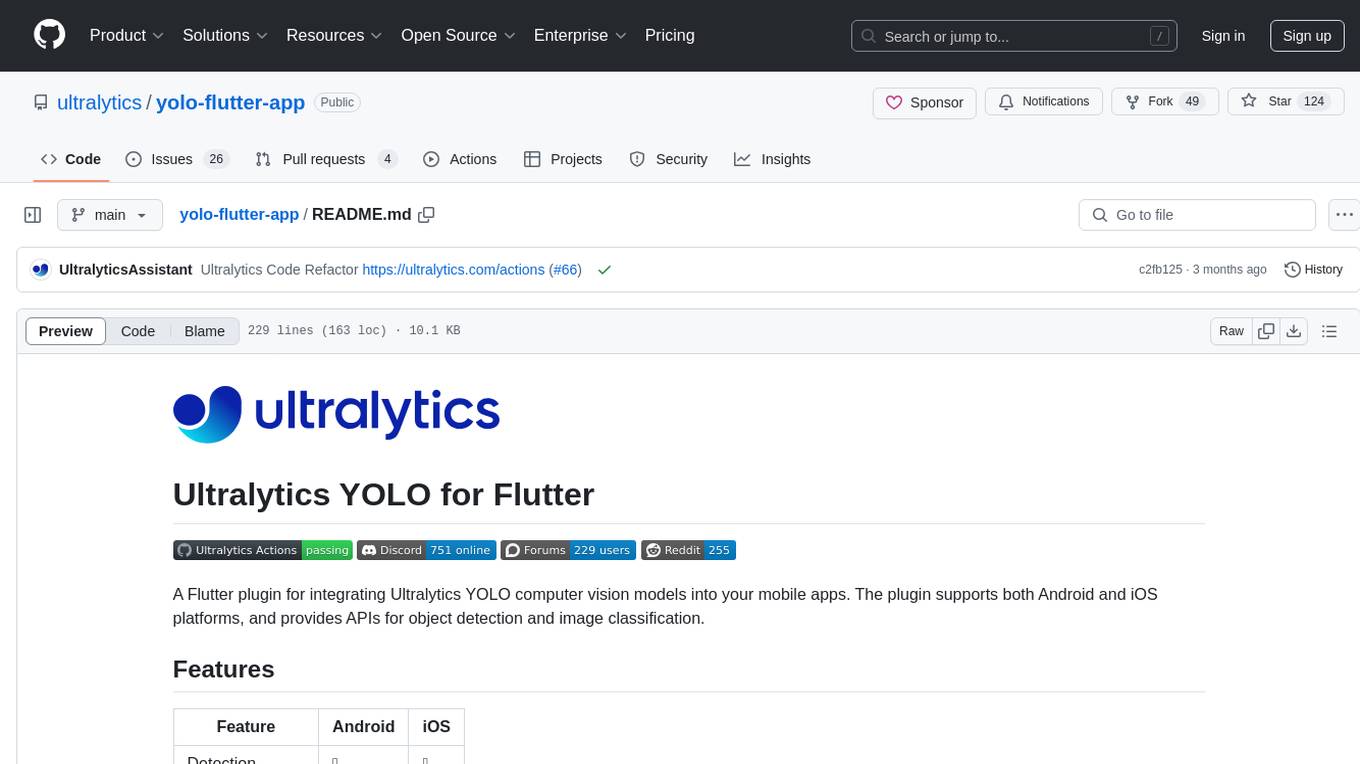
yolo-flutter-app
Ultralytics YOLO for Flutter is a Flutter plugin that allows you to integrate Ultralytics YOLO computer vision models into your mobile apps. It supports both Android and iOS platforms, providing APIs for object detection and image classification. The plugin leverages Flutter Platform Channels for seamless communication between the client and host, handling all processing natively. Before using the plugin, you need to export the required models in `.tflite` and `.mlmodel` formats. The plugin provides support for tasks like detection and classification, with specific instructions for Android and iOS platforms. It also includes features like camera preview and methods for object detection and image classification on images. Ultralytics YOLO thrives on community collaboration and offers different licensing paths for open-source and commercial use cases.
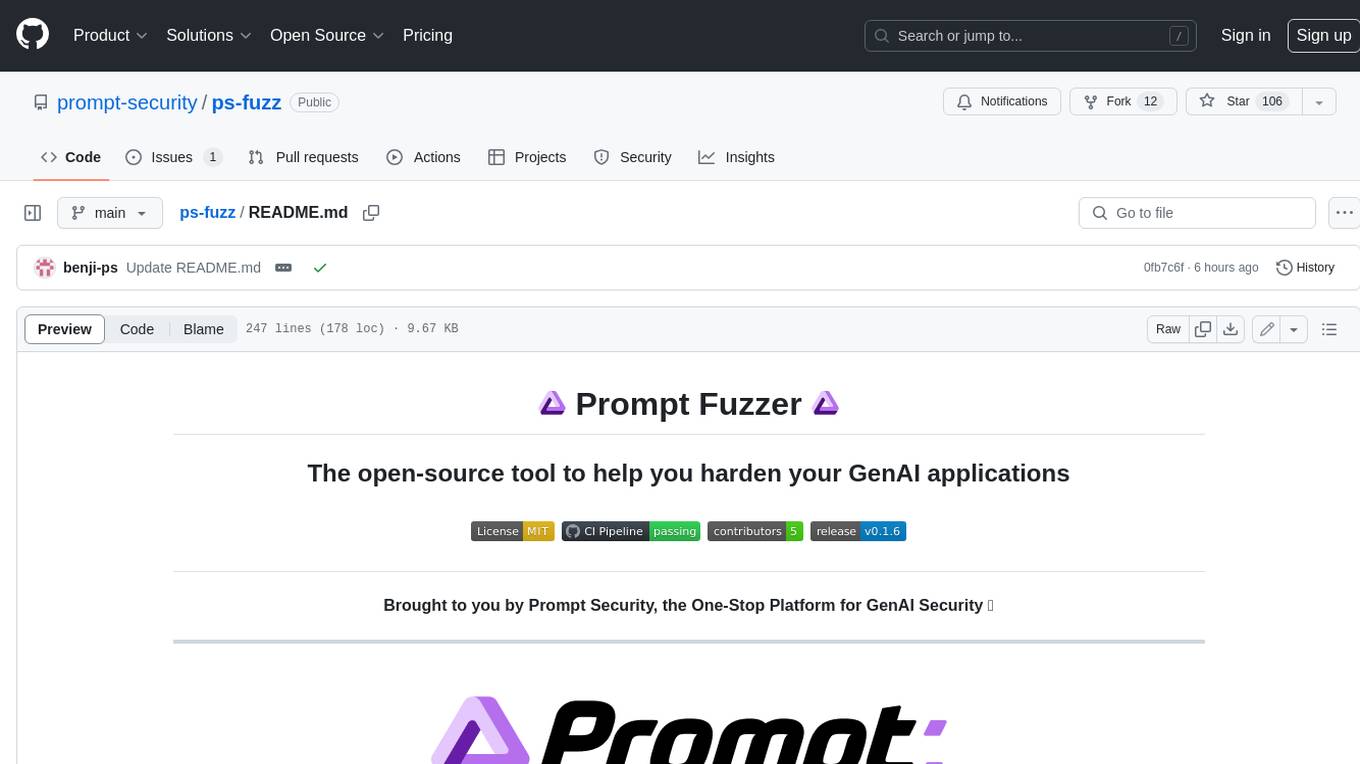
ps-fuzz
The Prompt Fuzzer is an open-source tool that helps you assess the security of your GenAI application's system prompt against various dynamic LLM-based attacks. It provides a security evaluation based on the outcome of these attack simulations, enabling you to strengthen your system prompt as needed. The Prompt Fuzzer dynamically tailors its tests to your application's unique configuration and domain. The Fuzzer also includes a Playground chat interface, giving you the chance to iteratively improve your system prompt, hardening it against a wide spectrum of generative AI attacks.
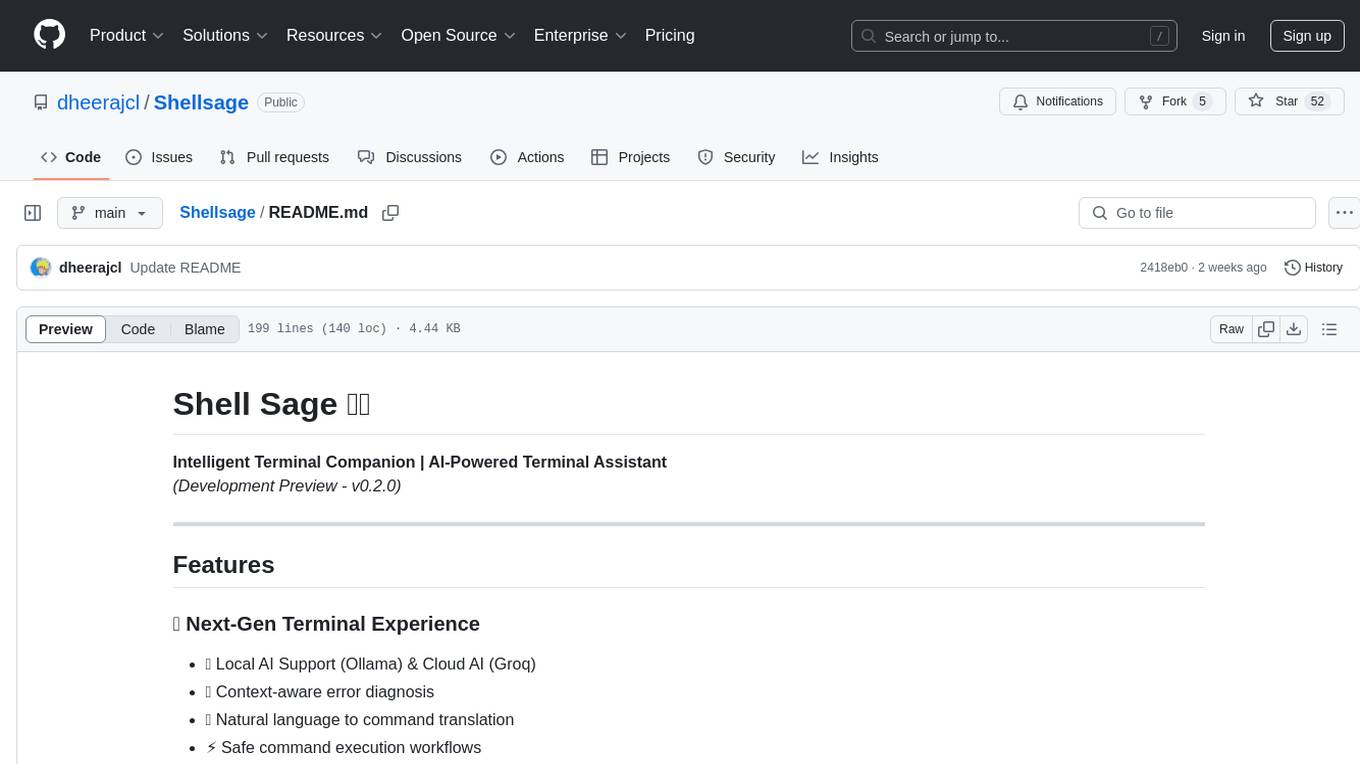
Shellsage
Shell Sage is an intelligent terminal companion and AI-powered terminal assistant that enhances the terminal experience with features like local and cloud AI support, context-aware error diagnosis, natural language to command translation, and safe command execution workflows. It offers interactive workflows, supports various API providers, and allows for custom model selection. Users can configure the tool for local or API mode, select specific models, and switch between modes easily. Currently in alpha development, Shell Sage has known limitations like limited Windows support and occasional false positives in error detection. The roadmap includes improvements like better context awareness, Windows PowerShell integration, Tmux integration, and CI/CD error pattern database.
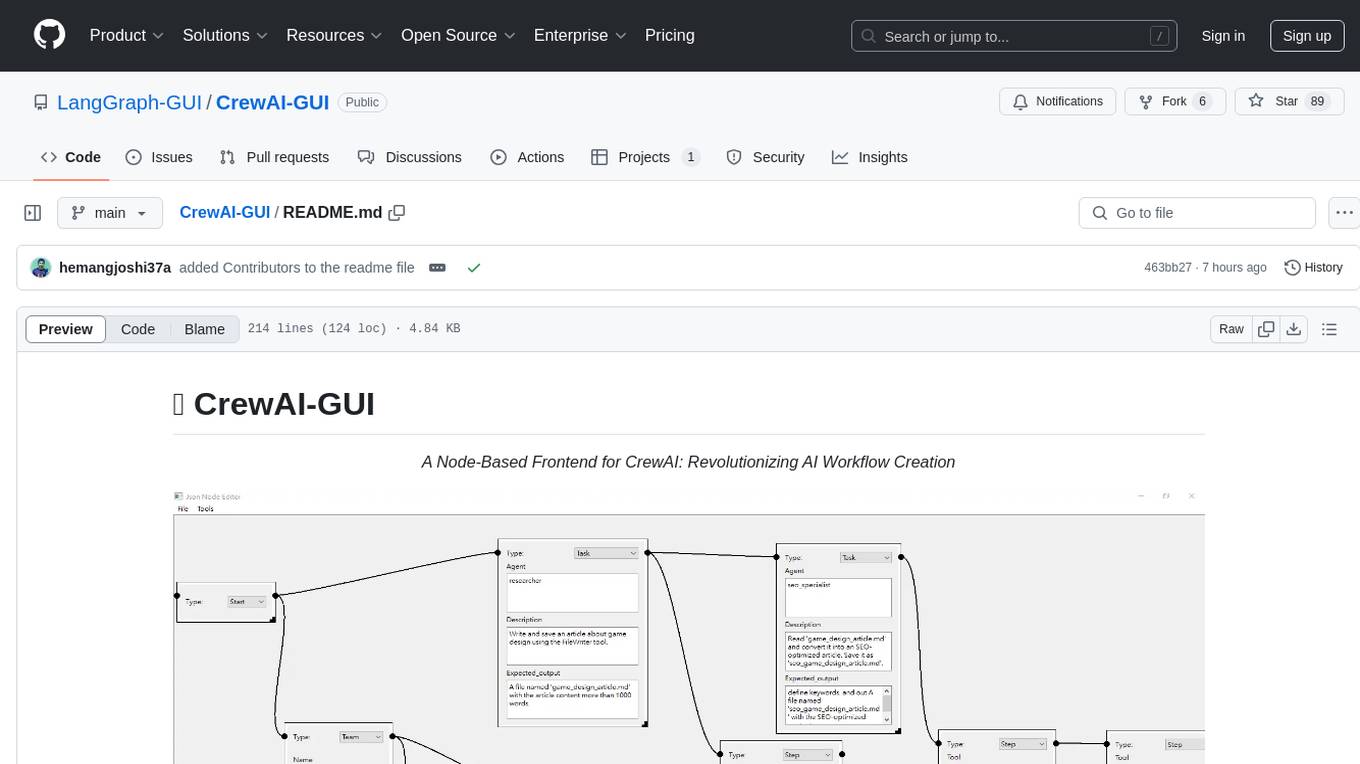
CrewAI-GUI
CrewAI-GUI is a Node-Based Frontend tool designed to revolutionize AI workflow creation. It empowers users to design complex AI agent interactions through an intuitive drag-and-drop interface, export designs to JSON for modularity and reusability, and supports both GPT-4 API and Ollama for flexible AI backend. The tool ensures cross-platform compatibility, allowing users to create AI workflows on Windows, Linux, or macOS efficiently.
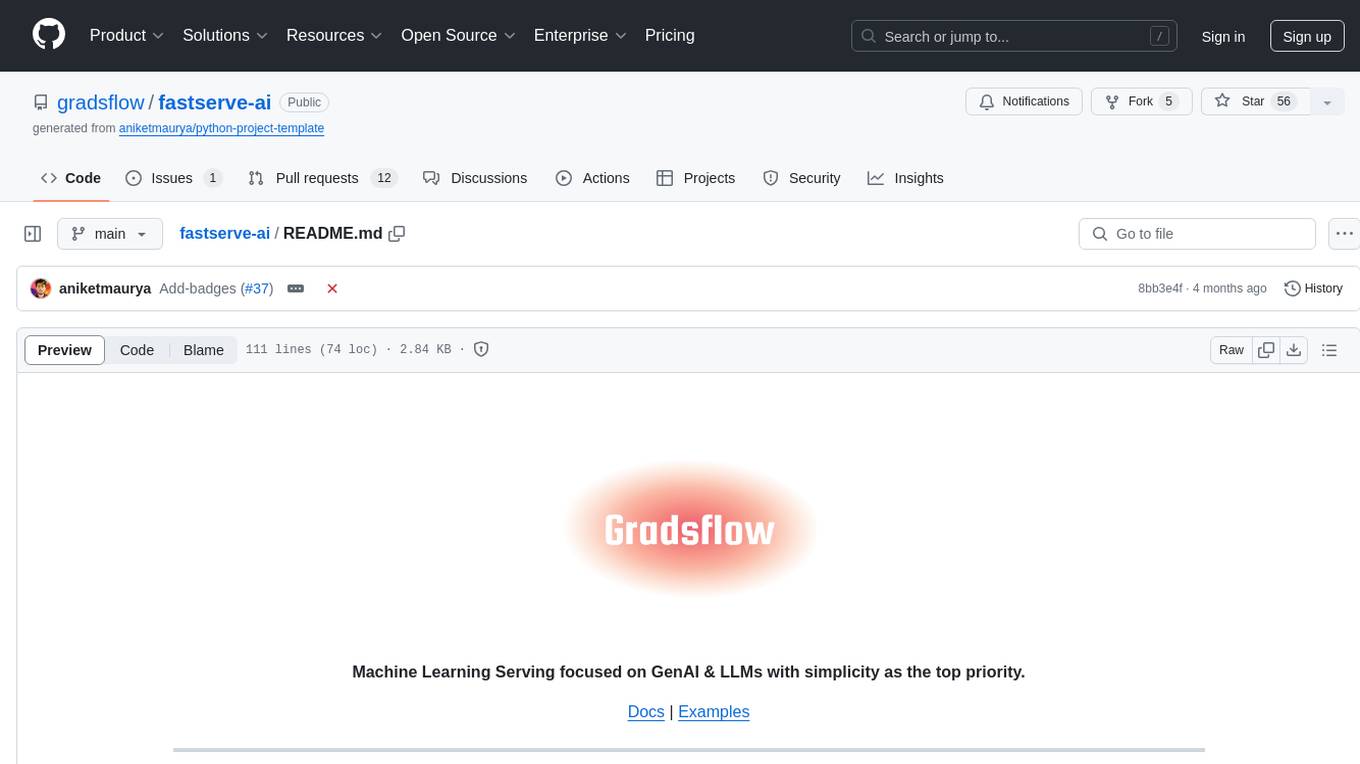
fastserve-ai
FastServe-AI is a machine learning serving tool focused on GenAI & LLMs with simplicity as the top priority. It allows users to easily serve custom models by implementing the 'handle' method for 'FastServe'. The tool provides a FastAPI server for custom models and can be deployed using Lightning AI Studio. Users can install FastServe-AI via pip and run it to serve their own GPT-like LLM models in minutes.