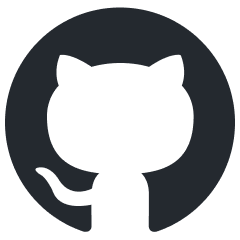
llm_benchmarks
A collection of benchmarks and datasets for evaluating LLM.
Stars: 94
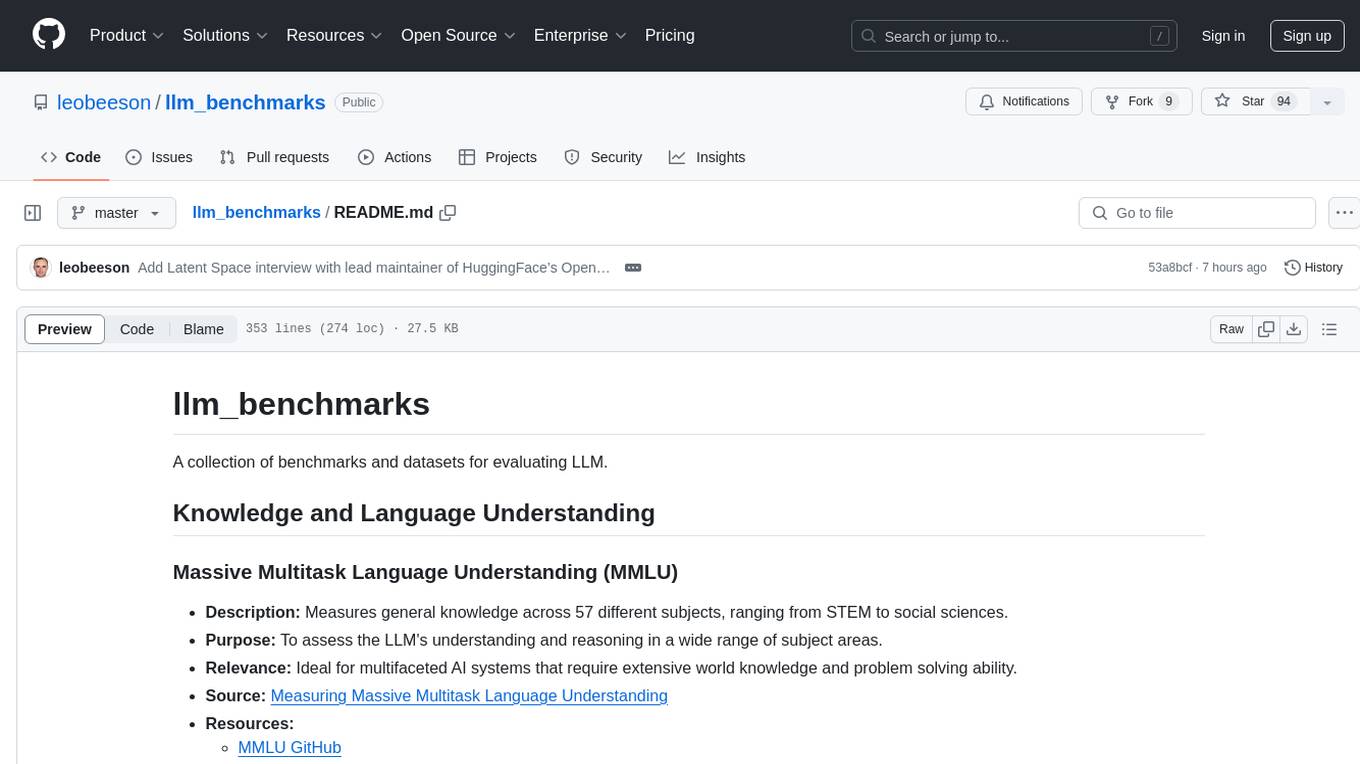
llm_benchmarks is a collection of benchmarks and datasets for evaluating Large Language Models (LLMs). It includes various tasks and datasets to assess LLMs' knowledge, reasoning, language understanding, and conversational abilities. The repository aims to provide comprehensive evaluation resources for LLMs across different domains and applications, such as education, healthcare, content moderation, coding, and conversational AI. Researchers and developers can leverage these benchmarks to test and improve the performance of LLMs in various real-world scenarios.
README:
A collection of benchmarks and datasets for evaluating LLM.
- Description: Measures general knowledge across 57 different subjects, ranging from STEM to social sciences.
- Purpose: To assess the LLM's understanding and reasoning in a wide range of subject areas.
- Relevance: Ideal for multifaceted AI systems that require extensive world knowledge and problem solving ability.
- Source: Measuring Massive Multitask Language Understanding
- Resources:
- Description: Tests LLMs on grade-school science questions, requiring both deep general knowledge and reasoning abilities.
- Purpose: To evaluate the ability to answer complex science questions that require logical reasoning.
- Relevance: Useful for educational AI applications, automated tutoring systems, and general knowledge assessments.
- Source: Think you have Solved Question Answering? Try ARC, the AI2 Reasoning Challenge
- Resources:
- Description: A collection of various language tasks from multiple datasets, designed to measure overall language understanding.
- Purpose: To provide a comprehensive assessment of language understanding abilities in different contexts.
- Relevance: Crucial for applications requiring advanced language processing, such as chatbots and content analysis.
- Source: GLUE: A Multi-Task Benchmark and Analysis Platform for Natural Language Understanding
- Resources:
- Description: A collection of real-world questions people have Googled, paired with relevant Wikipedia pages to extract answers.
- Purpose: To test the ability to find accurate short and long answers from web-based sources.
- Relevance: Essential for search engines, information retrieval systems, and AI-driven question-answering tools.
- Source: Natural Questions: A Benchmark for Question Answering Research
- Resources:
- Description: A collection of passages testing the ability of language models to understand and predict text based on long-range context.
- Purpose: To assess the models' comprehension of narratives and their predictive abilities in text generation.
- Relevance: Important for AI applications in narrative analysis, content creation, and long-form text understanding.
- Source: The LAMBADA Dataset: Word prediction requiring a broad discourse context
- Resources:
- Description: Tests natural language inference by requiring LLMs to complete passages in a way that requires understanding intricate details.
- Purpose: To evaluate the model's ability to generate contextually appropriate text continuations.
- Relevance: Useful in content creation, dialogue systems, and applications requiring advanced text generation capabilities.
- Source: HellaSwag: Can a Machine Really Finish Your Sentence?
- Resources:
- Description: A benchmark consisting of 433K sentence pairs across various genres of English data, testing natural language inference.
- Purpose: To assess the ability of LLMs to assign correct labels to hypothesis statements based on premises.
- Relevance: Vital for systems requiring advanced text comprehension and inference, such as automated reasoning and text analytics tools.
- Source: A Broad-Coverage Challenge Corpus for Sentence Understanding through Inference
- Resources:
- Description: An advanced version of the GLUE benchmark, comprising more challenging and diverse language tasks.
- Purpose: To evaluate deeper aspects of language understanding and reasoning.
- Relevance: Important for sophisticated AI systems requiring advanced language processing capabilities.
- Source: SuperGLUE: A Stickier Benchmark for General-Purpose Language Understanding Systems
- Resources:
- Description: A reading comprehension test with questions from sources like Wikipedia, demanding contextual analysis.
- Purpose: To assess the ability to sift through context and find accurate answers in complex texts.
- Relevance: Suitable for AI systems in knowledge extraction, research, and detailed content analysis.
- Source: TriviaQA: A Large Scale Distantly Supervised Challenge Dataset for Reading Comprehension
- Resources:
- Description: A large set of problems based on the Winograd Schema Challenge, testing context understanding in sentences.
- Purpose: To evaluate the ability of LLMs to grasp nuanced context and subtle variations in text.
- Relevance: Crucial for models dealing with narrative analysis, content personalization, and advanced text interpretation.
- Source: WinoGrande: An Adversarial Winograd Schema Challenge at Scale
- Resources:
- Description: Consists of multiple-choice questions mainly in natural sciences like physics, chemistry, and biology.
- Purpose: To test the ability to answer science-based questions, often with additional supporting text.
- Relevance: Useful for educational tools, especially in science education and knowledge testing platforms.
- Source: Crowdsourcing Multiple Choice Science Questions
- Resources:
- Description: A set of 8.5K grade-school math problems that require basic to intermediate math operations.
- Purpose: To test LLMs’ ability to work through multistep math problems.
- Relevance: Useful for assessing AI’s capability in solving basic mathematical problems, valuable in educational contexts.
- Source: Training Verifiers to Solve Math Word Problems
- Resources:
- Description: An adversarially-created reading comprehension benchmark requiring models to navigate through references and execute operations like addition or sorting.
- Purpose: To evaluate the ability of models to understand complex texts and perform discrete operations.
- Relevance: Useful in advanced educational tools and text analysis systems requiring logical reasoning.
- Source: DROP: A Reading Comprehension Benchmark Requiring Discrete Reasoning Over Paragraphs
- Resources::
- Description: Evaluates counterfactual reasoning abilities of LLMs, focusing on "what if" scenarios.
- Purpose: To assess models' ability to understand and reason about alternate scenarios based on given data.
- Relevance: Important for AI applications in strategic planning, decision-making, and scenario analysis.
- Source: CRASS: A Novel Data Set and Benchmark to Test Counterfactual Reasoning of Large Language Models
- Resources:
- Description: A set of reading comprehension questions derived from English exams given to Chinese students.
- Purpose: To test LLMs' understanding of complex reading material and their ability to answer examination-level questions.
- Relevance: Useful in language learning applications and educational systems for exam preparation.
- Source: RACE: Large-scale ReAding Comprehension Dataset From Examinations
- Resources:
- Description: A subset of BIG-Bench focusing on the most challenging tasks requiring multi-step reasoning.
- Purpose: To challenge LLMs with complex tasks demanding advanced reasoning skills.
- Relevance: Important for evaluating the upper limits of AI capabilities in complex reasoning and problem-solving.
- Source: Challenging BIG-Bench Tasks and Whether Chain-of-Thought Can Solve Them
- Resources:
- Description: A collection of standardized tests, including GRE, GMAT, SAT, LSAT, and civil service exams.
- Purpose: To evaluate LLMs' reasoning abilities and problem-solving skills across various academic and professional scenarios.
- Relevance: Useful for assessing AI capabilities in standardized testing and professional qualification contexts.
- Source: AGIEval: A Human-Centric Benchmark for Evaluating Foundation Models
- Resources:
- Description: A collection of over 15,000 real yes/no questions from Google searches, paired with Wikipedia passages.
- Purpose: To test the ability of LLMs to infer correct answers from contextual information that may not be explicit.
- Relevance: Crucial for question-answering systems and knowledge-based AI applications where accurate inference is key.
- Source: BoolQ: Exploring the Surprising Difficulty of Natural Yes/No Questions
- Resources:
- Description: Tailored for evaluating the proficiency of chat assistants in sustaining multi-turn conversations.
- Purpose: To test the ability of models to engage in coherent and contextually relevant dialogues over multiple turns.
- Relevance: Essential for developing sophisticated conversational agents and chatbots.
- Source: Judging LLM-as-a-Judge with MT-Bench and Chatbot Arena
- Resources:
- Description: Features 14,000 dialogues with 100,000 question-answer pairs, simulating student-teacher interactions.
- Purpose: To challenge LLMs with context-dependent, sometimes unanswerable questions within dialogues.
- Relevance: Useful for conversational AI, educational software, and context-aware information systems.
- Source: QuAC : Question Answering in Context
- Resources:
- Description: Contains full doctor-patient conversations and associated clinical notes from various medical domains.
- Purpose: To challenge models to accurately generate clinical notes based on conversational data.
- Relevance: Vital for AI applications in healthcare, especially in automated documentation and medical analysis.
- Source:: ACI-BENCH: a Novel Ambient Clinical Intelligence Dataset for Benchmarking Automatic Visit Note Generation
- Resources::
- Description: A large-scale collection of natural language questions and answers derived from real web queries.
- Purpose: To test the ability of models to accurately understand and respond to real-world queries.
- Relevance: Crucial for search engines, question-answering systems, and other consumer-facing AI applications.
- Source: MS MARCO: A Human Generated MAchine Reading COmprehension Dataset
- Resources:
- Description: A benchmark for summarizing relevant spans of meetings in response to specific queries.
- Purpose: To evaluate the ability of models to extract and summarize important information from meeting content.
- Relevance: Useful for business intelligence tools, meeting analysis applications, and automated summarization systems.
- Source: QMSum: A New Benchmark for Query-based Multi-domain Meeting Summarization
- Resources:
- Description: Tests knowledge and understanding of the physical world through hypothetical scenarios and solutions.
- Purpose: To measure the model’s capability in handling physical interaction scenarios.
- Relevance: Important for AI applications in robotics, physical simulations, and practical problem-solving systems.
- Source:: PIQA: Reasoning about Physical Commonsense in Natural Language
- Resources:
- Description: A dataset of toxic and benign statements about minority groups, focusing on implicit hate speech.
- Purpose: To test a model's ability to both identify and avoid generating toxic content.
- Relevance: Crucial for content moderation systems, community management, and AI ethics research.
- Source: ToxiGen: A Large-Scale Machine-Generated Dataset for Adversarial and Implicit Hate Speech Detection
- Resources:
- Description: Evaluates language models' alignment with ethical standards such as helpfulness, honesty, and harmlessness.
- Purpose: To assess the ethical responses of models in interaction scenarios.
- Relevance: Vital for ensuring AI systems promote positive interactions and adhere to ethical standards.
- Source: A General Language Assistant as a Laboratory for Alignment
- Resources:
- Description: A benchmark for evaluating the truthfulness of LLMs in generating answers to questions prone to false beliefs and biases.
- Purpose: To test the ability of models to provide accurate and unbiased information.
- Relevance: Important for AI systems where delivering accurate and unbiased information is critical, such as in educational or advisory roles.
- Source: TruthfulQA: Measuring How Models Mimic Human Falsehoods
- Resources:
- Description: A framework for evaluating the safety of chat-optimized models in conversational settings.
- Purpose: To assess potential harmful content, IP leakage, and security breaches in AI-driven conversations.
- Relevance: Crucial for developing safe and secure conversational AI applications, particularly in sensitive domains.
- Source: A Framework for Automated Measurement of Responsible AI Harms in Generative AI Applications
- Description: Evaluates LLMs' ability to understand and work with code across various tasks like code completion and translation.
- Purpose: To assess code intelligence, including understanding, fixing, and explaining code.
- Relevance: Essential for applications in software development, code analysis, and technical documentation.
- Source: CodeXGLUE: A Machine Learning Benchmark Dataset for Code Understanding and Generation
- Reference:
- Description: Contains programming challenges for evaluating LLMs' ability to write functional code based on instructions.
- Purpose: To test the generation of correct and efficient code from given requirements.
- Relevance: Important for automated code generation tools, programming assistants, and coding education platforms.
- Source: Evaluating Large Language Models Trained on Code
- Resources:
- Description: Includes 1,000 Python programming problems suitable for entry-level programmers.
- Purpose: To evaluate proficiency in solving basic programming tasks and understanding of Python.
- Relevance: Useful for beginner-level coding education, automated code generation, and entry-level programming testing.
- Source: Program Synthesis with Large Language Models
- Resources:
- Source: Judging LLM-as-a-Judge with MT-Bench and Chatbot Arena
- Abstract: Evaluating large language model (LLM) based chat assistants is challenging due to their broad capabilities and the inadequacy of existing benchmarks in measuring human preferences. To address this, we explore using strong LLMs as judges to evaluate these models on more open-ended questions. We examine the usage and limitations of LLM-as-a-judge, including position, verbosity, and self-enhancement biases, as well as limited reasoning ability, and propose solutions to mitigate some of them. We then verify the agreement between LLM judges and human preferences by introducing two benchmarks: MT-bench, a multi-turn question set; and Chatbot Arena, a crowdsourced battle platform. Our results reveal that strong LLM judges like GPT-4 can match both controlled and crowdsourced human preferences well, achieving over 80% agreement, the same level of agreement between humans. Hence, LLM-as-a-judge is a scalable and explainable way to approximate human preferences, which are otherwise very expensive to obtain. Additionally, we show our benchmark and traditional benchmarks complement each other by evaluating several variants of LLaMA and Vicuna. The MT-bench questions, 3K expert votes, and 30K conversations with human preferences are publicly available at this https URL.
-
Insights:
- Use MT-bench questions and prompts to evaluate your models with LLM-as-a-judge. MT-bench is a set of challenging multi-turn open-ended questions for evaluating chat assistants. To automate the evaluation process, we prompt strong LLMs like GPT-4 to act as judges and assess the quality of the models' responses.
- Resources:
- Source: Unified Multi-Dimensional Automatic Evaluation for Open-Domain Conversations with Large Language Models
- Abstract: We propose LLM-Eval, a unified multi-dimensional automatic evaluation method for open-domain conversations with large language models (LLMs). Existing evaluation methods often rely on human annotations, ground-truth responses, or multiple LLM prompts, which can be expensive and time-consuming. To address these issues, we design a single prompt-based evaluation method that leverages a unified evaluation schema to cover multiple dimensions of conversation quality in a single model call. We extensively evaluate the performance of LLM-Eval on various benchmark datasets, demonstrating its effectiveness, efficiency, and adaptability compared to state-of-the-art evaluation methods. Our analysis also highlights the importance of choosing suitable LLMs and decoding strategies for accurate evaluation results. LLM-Eval offers a versatile and robust solution for evaluating open-domain conversation systems, streamlining the evaluation process and providing consistent performance across diverse scenarios.
-
Insights:
- Top-shelve LLM (e.g. GPT4, Claude) correlate better with human score than metric-based eval measures.
- Source: JudgeLM: Fine-tuned Large Language Models are Scalable Judges
- Abstract: Evaluating Large Language Models (LLMs) in open-ended scenarios is challenging because existing benchmarks and metrics can not measure them comprehensively. To address this problem, we propose to fine-tune LLMs as scalable judges (JudgeLM) to evaluate LLMs efficiently and effectively in open-ended benchmarks. We first propose a comprehensive, large-scale, high-quality dataset containing task seeds, LLMs-generated answers, and GPT-4-generated judgments for fine-tuning high-performance judges, as well as a new benchmark for evaluating the judges. We train JudgeLM at different scales from 7B, 13B, to 33B parameters, and conduct a systematic analysis of its capabilities and behaviors. We then analyze the key biases in fine-tuning LLM as a judge and consider them as position bias, knowledge bias, and format bias. To address these issues, JudgeLM introduces a bag of techniques including swap augmentation, reference support, and reference drop, which clearly enhance the judge's performance. JudgeLM obtains the state-of-the-art judge performance on both the existing PandaLM benchmark and our proposed new benchmark. Our JudgeLM is efficient and the JudgeLM-7B only needs 3 minutes to judge 5K samples with 8 A100 GPUs. JudgeLM obtains high agreement with the teacher judge, achieving an agreement exceeding 90% that even surpasses human-to-human agreement. JudgeLM also demonstrates extended capabilities in being judges of the single answer, multimodal models, multiple answers, and multi-turn chat.
-
Insights:
- Relatively small models (e.g 7b models) can be fine-tuned to be reliable judges of other models.
- Source: Prometheus: Inducing Fine-grained Evaluation Capability in Language Models
- Abstract: Recently, using a powerful proprietary Large Language Model (LLM) (e.g., GPT-4) as an evaluator for long-form responses has become the de facto standard. However, for practitioners with large-scale evaluation tasks and custom criteria in consideration (e.g., child-readability), using proprietary LLMs as an evaluator is unreliable due to the closed-source nature, uncontrolled versioning, and prohibitive costs. In this work, we propose Prometheus, a fully open-source LLM that is on par with GPT-4's evaluation capabilities when the appropriate reference materials (reference answer, score rubric) are accompanied. We first construct the Feedback Collection, a new dataset that consists of 1K fine-grained score rubrics, 20K instructions, and 100K responses and language feedback generated by GPT-4. Using the Feedback Collection, we train Prometheus, a 13B evaluator LLM that can assess any given long-form text based on customized score rubric provided by the user. Experimental results show that Prometheus scores a Pearson correlation of 0.897 with human evaluators when evaluating with 45 customized score rubrics, which is on par with GPT-4 (0.882), and greatly outperforms ChatGPT (0.392). Furthermore, measuring correlation with GPT-4 with 1222 customized score rubrics across four benchmarks (MT Bench, Vicuna Bench, Feedback Bench, Flask Eval) shows similar trends, bolstering Prometheus's capability as an evaluator LLM. Lastly, Prometheus achieves the highest accuracy on two human preference benchmarks (HHH Alignment & MT Bench Human Judgment) compared to open-sourced reward models explicitly trained on human preference datasets, highlighting its potential as an universal reward model.
-
Insights:
- A scoring rubric and a reference answer vastly improve correlation with human scores.
-
Latent Space - Benchmarks 201: Why Leaderboards > Arenas >> LLM-as-Judge
-
Summary:
- The OpenLLM Leaderboard, maintained by Clémentine Fourrier, is a standardized and reproducible way to evaluate language models' performance.
- The leaderboard initially gained popularity in summer 2023 and has had over 2 million unique visitors and 300,000 active community members.
- The recent update to the leaderboard (v2) includes six benchmarks to address model overfitting and to provide more room for improved performance.
- LLMs are not recommended as judges due to issues like mode collapse and positional bias.
- If LLMs must be used as judges, open LLMs like Prometheus or JudgeLM are suggested for reproducibility.
- The LMSys Arena is another platform for AI engineers, but its rankings are not reproducible and may not accurately reflect model capabilities.
-
Summary:
For Tasks:
Click tags to check more tools for each tasksFor Jobs:
Alternative AI tools for llm_benchmarks
Similar Open Source Tools
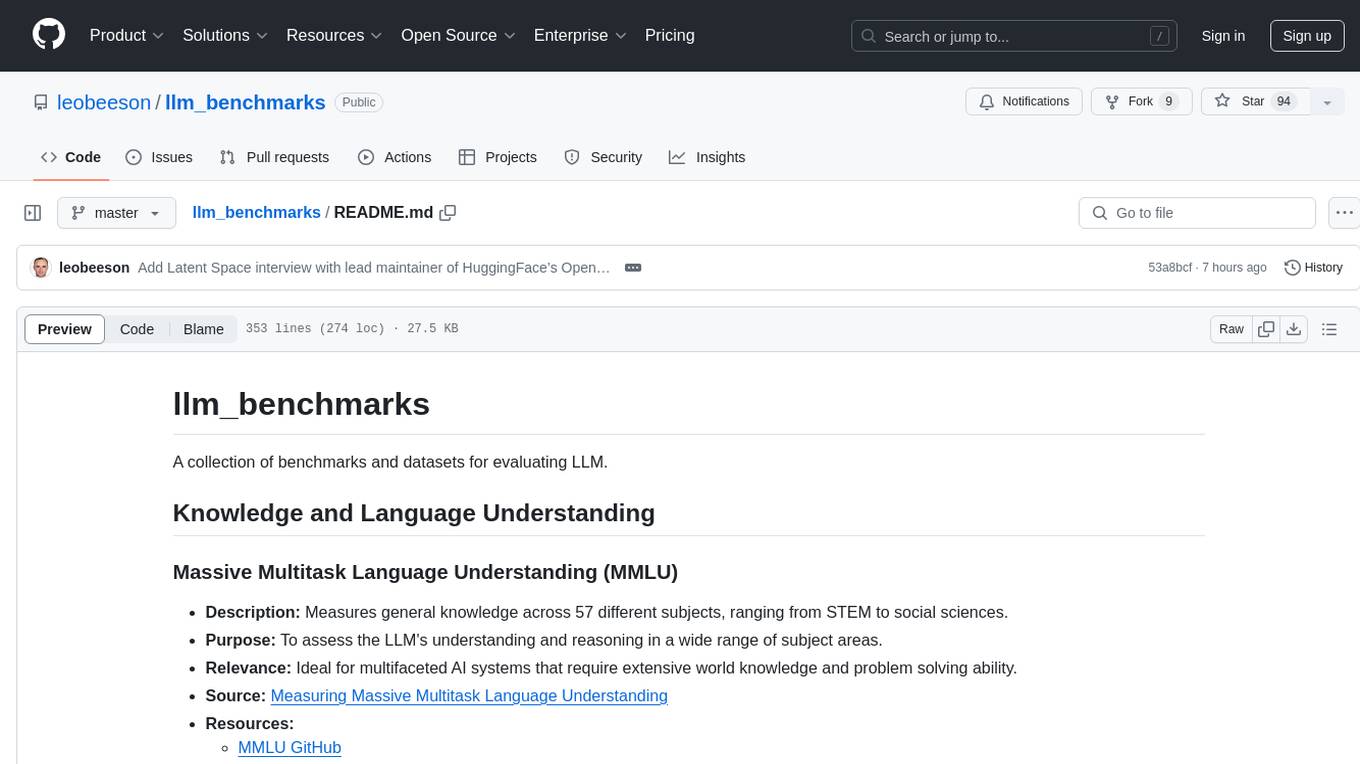
llm_benchmarks
llm_benchmarks is a collection of benchmarks and datasets for evaluating Large Language Models (LLMs). It includes various tasks and datasets to assess LLMs' knowledge, reasoning, language understanding, and conversational abilities. The repository aims to provide comprehensive evaluation resources for LLMs across different domains and applications, such as education, healthcare, content moderation, coding, and conversational AI. Researchers and developers can leverage these benchmarks to test and improve the performance of LLMs in various real-world scenarios.
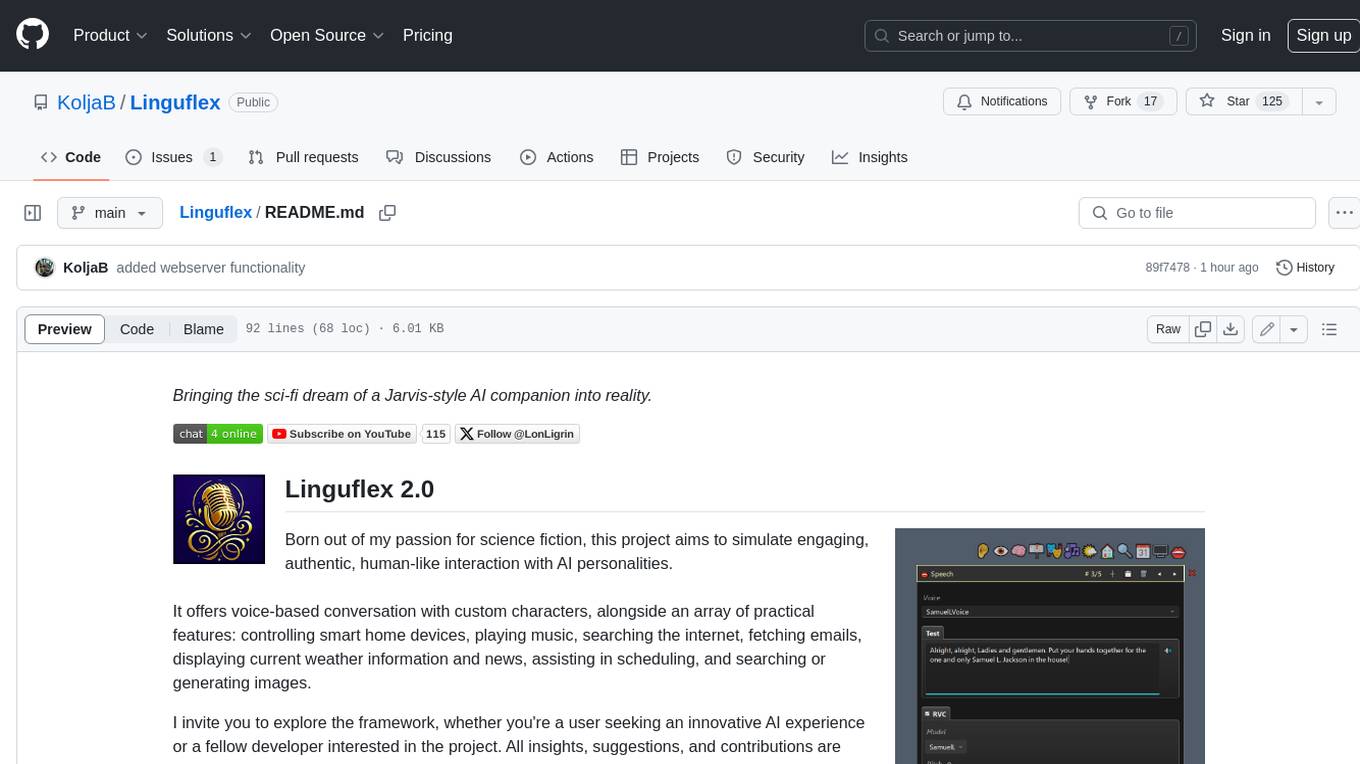
Linguflex
Linguflex is a project that aims to simulate engaging, authentic, human-like interaction with AI personalities. It offers voice-based conversation with custom characters, alongside an array of practical features such as controlling smart home devices, playing music, searching the internet, fetching emails, displaying current weather information and news, assisting in scheduling, and searching or generating images.
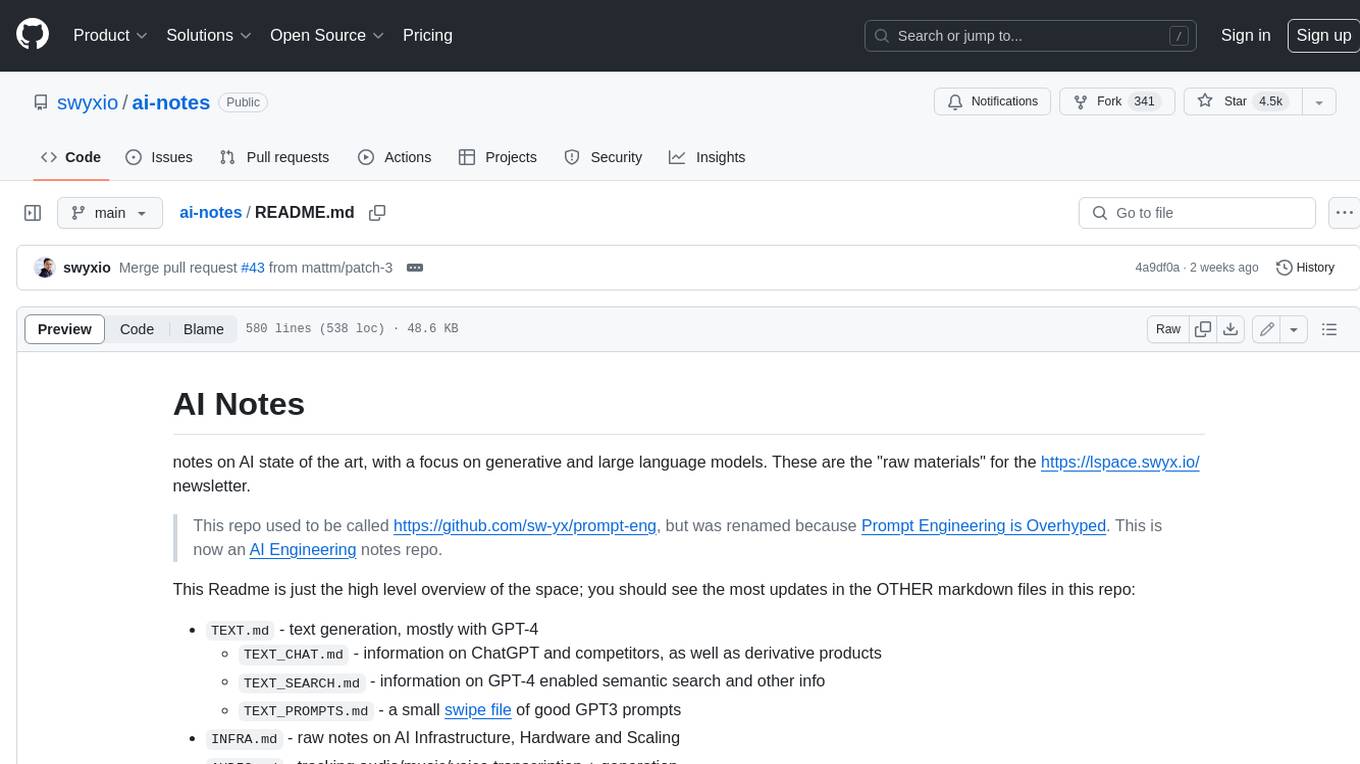
ai-notes
Notes on AI state of the art, with a focus on generative and large language models. These are the "raw materials" for the https://lspace.swyx.io/ newsletter. This repo used to be called https://github.com/sw-yx/prompt-eng, but was renamed because Prompt Engineering is Overhyped. This is now an AI Engineering notes repo.
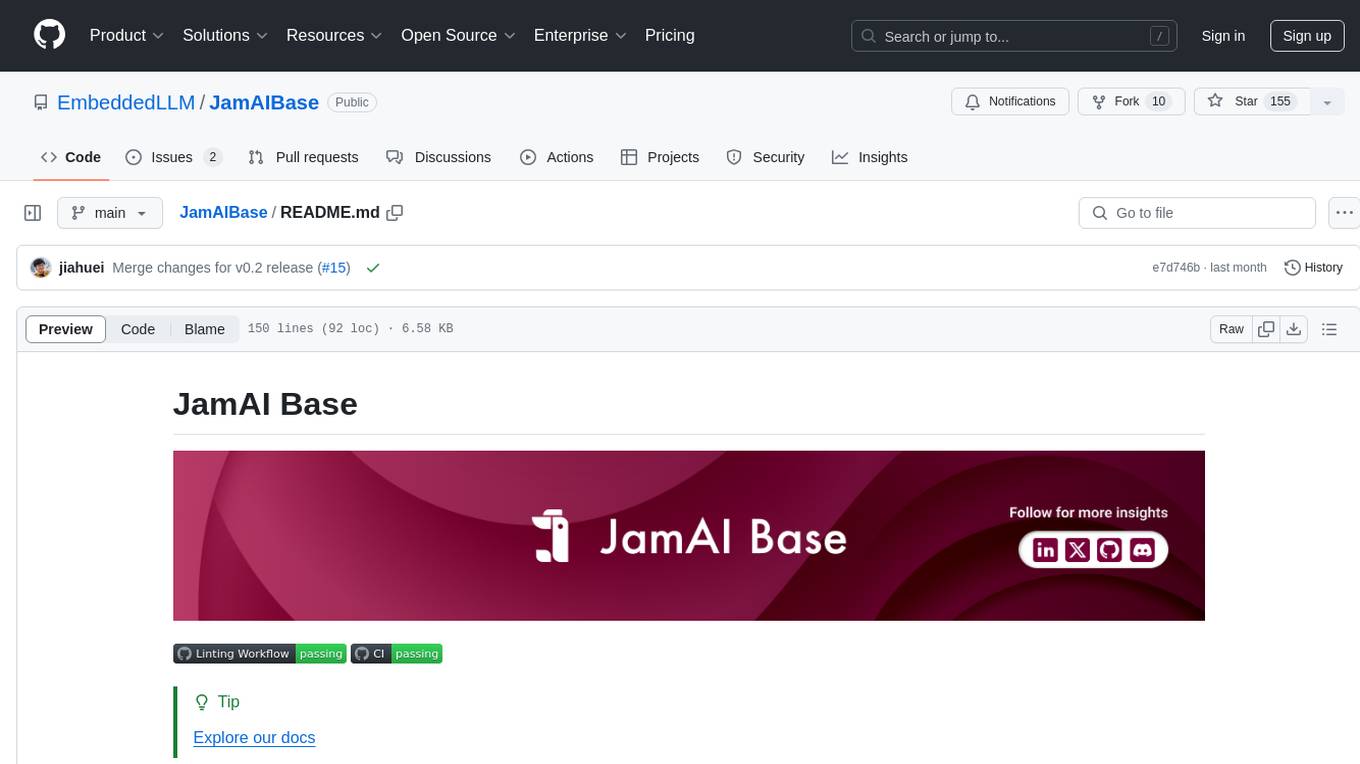
JamAIBase
JamAI Base is an open-source platform integrating SQLite and LanceDB databases with managed memory and RAG capabilities. It offers built-in LLM, vector embeddings, and reranker orchestration accessible through a spreadsheet-like UI and REST API. Users can transform static tables into dynamic entities, facilitate real-time interactions, manage structured data, and simplify chatbot development. The tool focuses on ease of use, scalability, flexibility, declarative paradigm, and innovative RAG techniques, making complex data operations accessible to users with varying technical expertise.
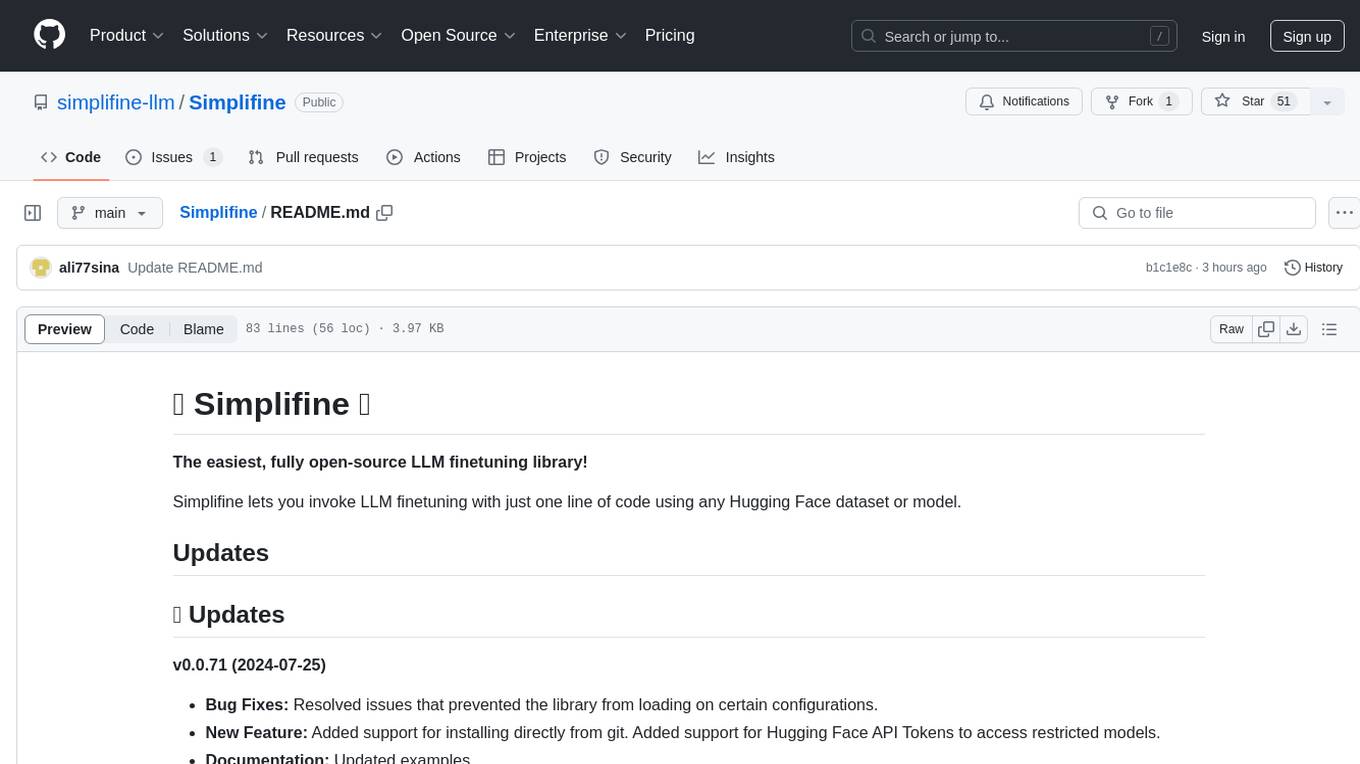
Simplifine
Simplifine is an open-source library designed for easy LLM finetuning, enabling users to perform tasks such as supervised fine tuning, question-answer finetuning, contrastive loss for embedding tasks, multi-label classification finetuning, and more. It provides features like WandB logging, in-built evaluation tools, automated finetuning parameters, and state-of-the-art optimization techniques. The library offers bug fixes, new features, and documentation updates in its latest version. Users can install Simplifine via pip or directly from GitHub. The project welcomes contributors and provides comprehensive documentation and support for users.
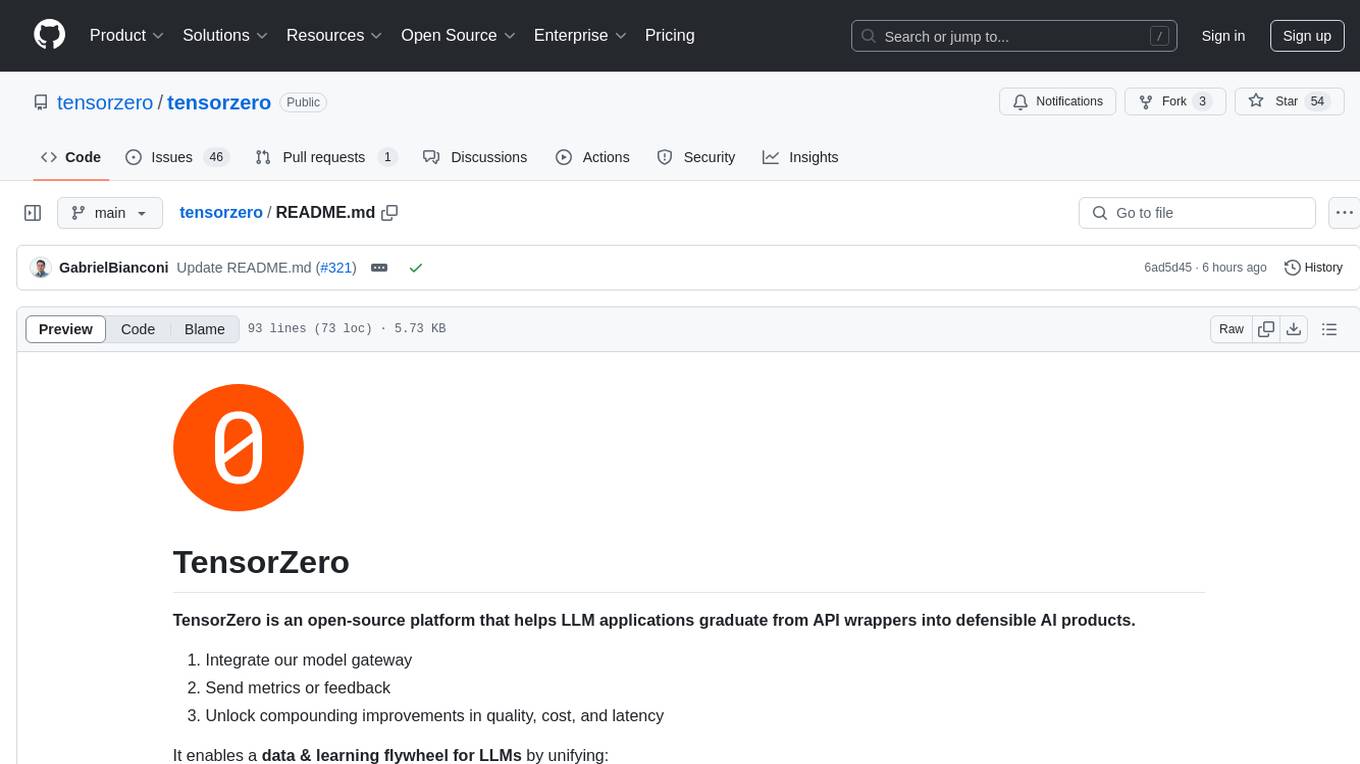
tensorzero
TensorZero is an open-source platform that helps LLM applications graduate from API wrappers into defensible AI products. It enables a data & learning flywheel for LLMs by unifying inference, observability, optimization, and experimentation. The platform includes a high-performance model gateway, structured schema-based inference, observability, experimentation, and data warehouse for analytics. TensorZero Recipes optimize prompts and models, and the platform supports experimentation features and GitOps orchestration for deployment.
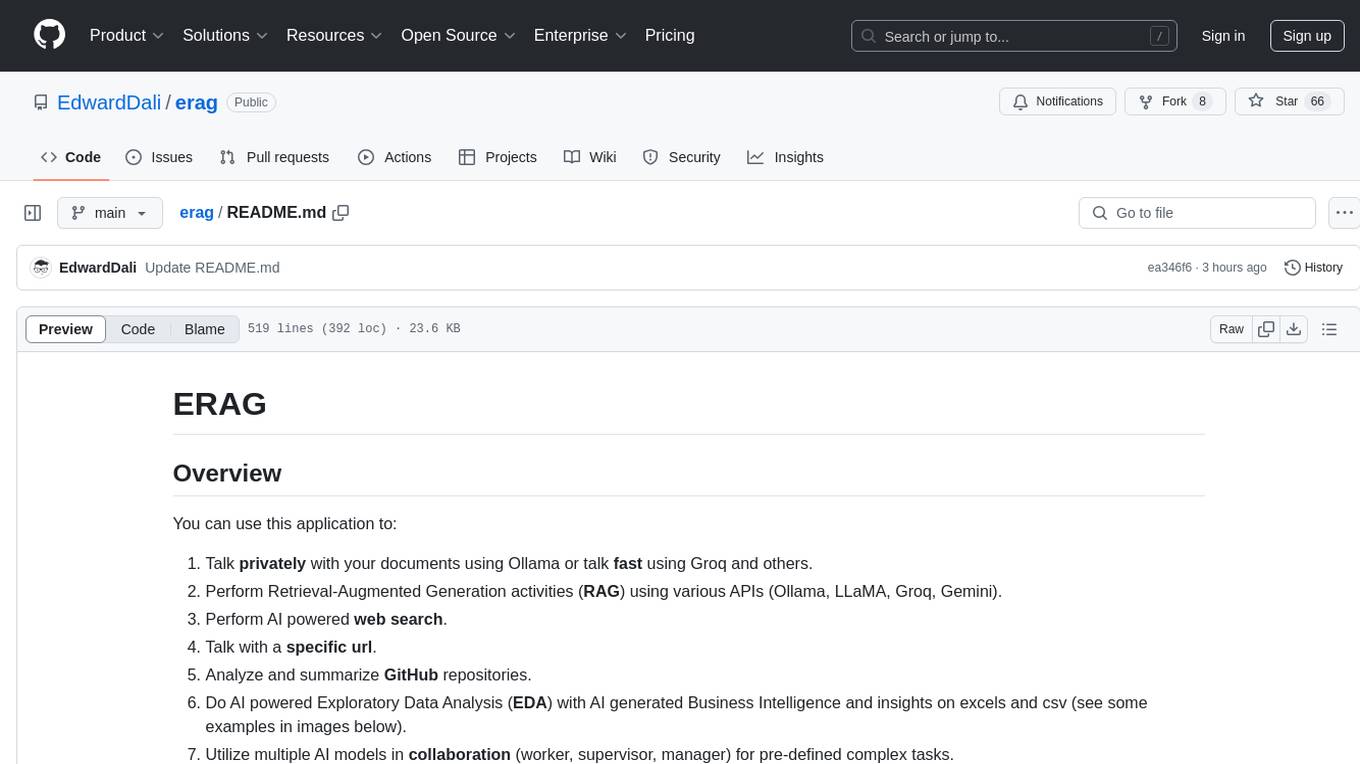
erag
ERAG is an advanced system that combines lexical, semantic, text, and knowledge graph searches with conversation context to provide accurate and contextually relevant responses. This tool processes various document types, creates embeddings, builds knowledge graphs, and uses this information to answer user queries intelligently. It includes modules for interacting with web content, GitHub repositories, and performing exploratory data analysis using various language models.
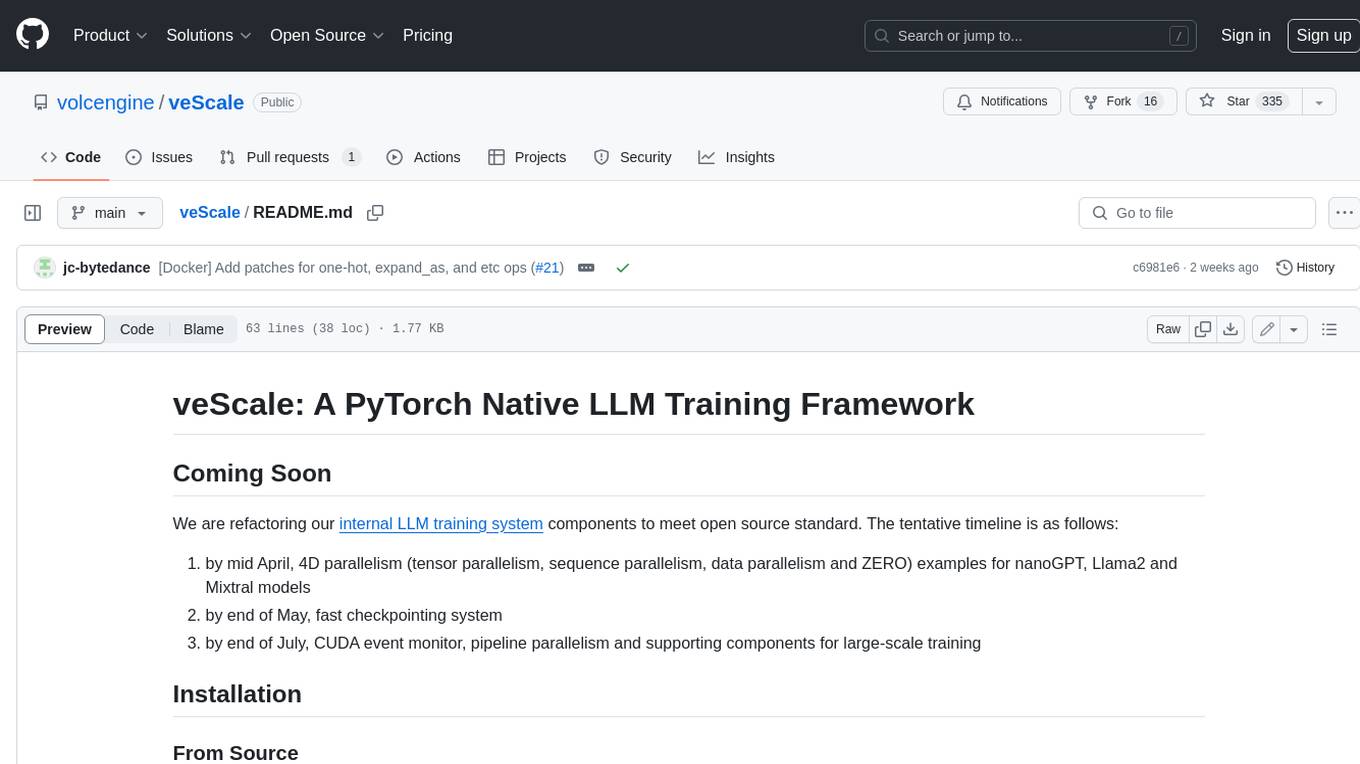
veScale
veScale is a PyTorch Native LLM Training Framework. It provides a set of tools and components to facilitate the training of large language models (LLMs) using PyTorch. veScale includes features such as 4D parallelism, fast checkpointing, and a CUDA event monitor. It is designed to be scalable and efficient, and it can be used to train LLMs on a variety of hardware platforms.
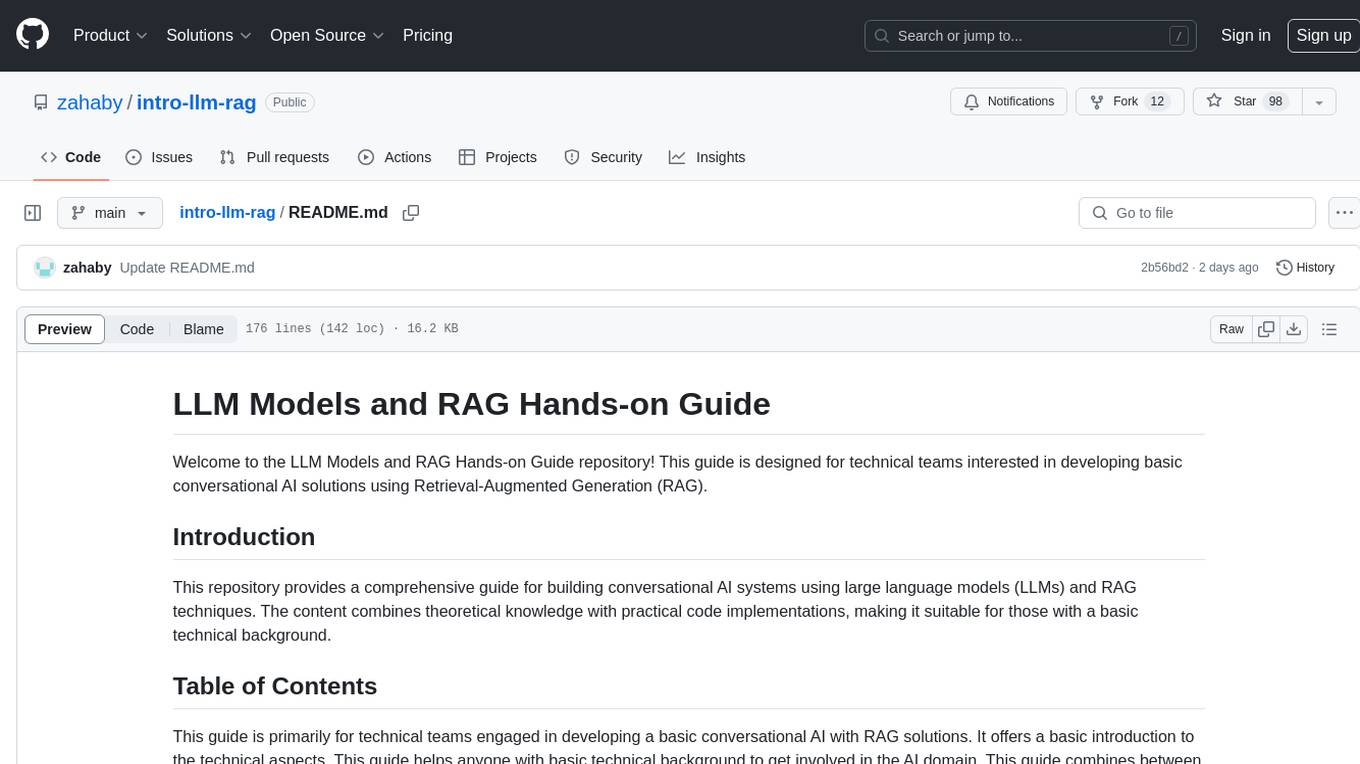
intro-llm-rag
This repository serves as a comprehensive guide for technical teams interested in developing conversational AI solutions using Retrieval-Augmented Generation (RAG) techniques. It covers theoretical knowledge and practical code implementations, making it suitable for individuals with a basic technical background. The content includes information on large language models (LLMs), transformers, prompt engineering, embeddings, vector stores, and various other key concepts related to conversational AI. The repository also provides hands-on examples for two different use cases, along with implementation details and performance analysis.
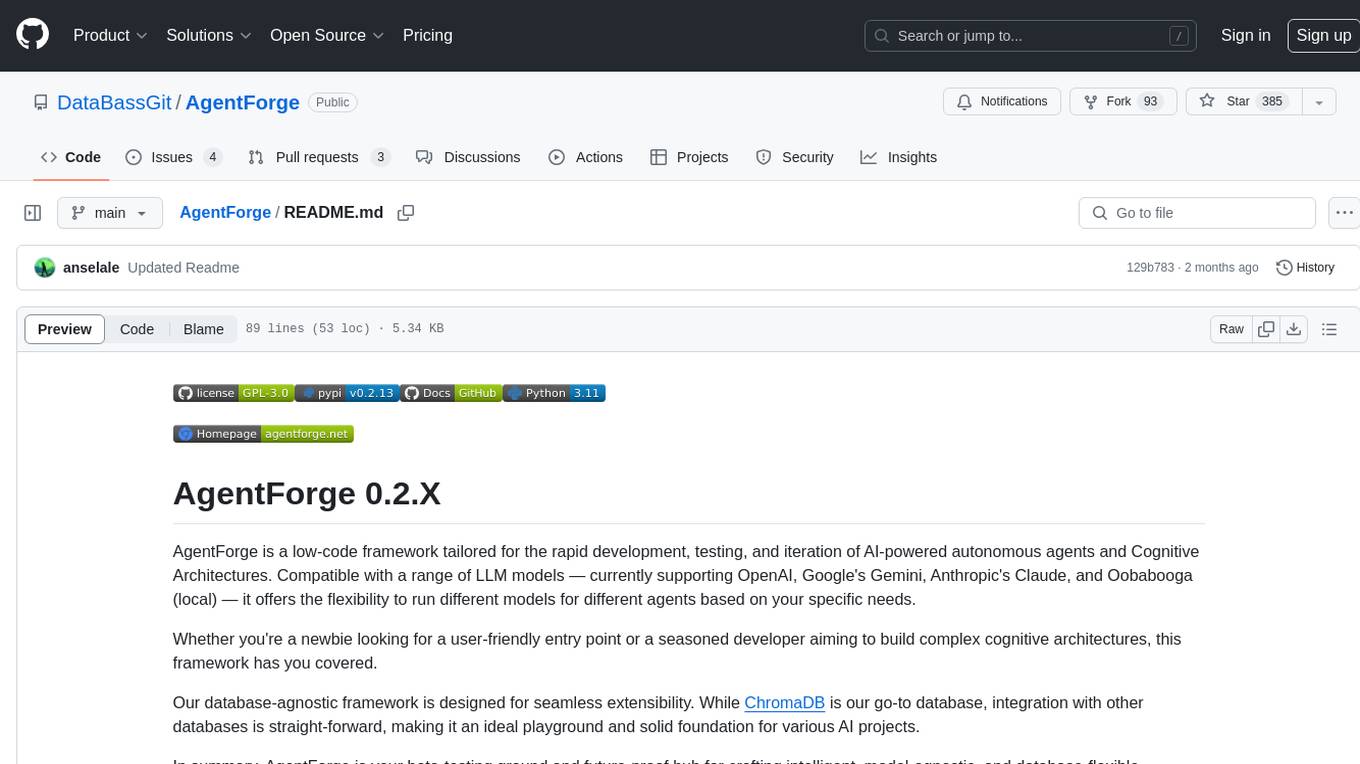
AgentForge
AgentForge is a low-code framework tailored for the rapid development, testing, and iteration of AI-powered autonomous agents and Cognitive Architectures. It is compatible with a range of LLM models and offers flexibility to run different models for different agents based on specific needs. The framework is designed for seamless extensibility and database-flexibility, making it an ideal playground for various AI projects. AgentForge is a beta-testing ground and future-proof hub for crafting intelligent, model-agnostic autonomous agents.
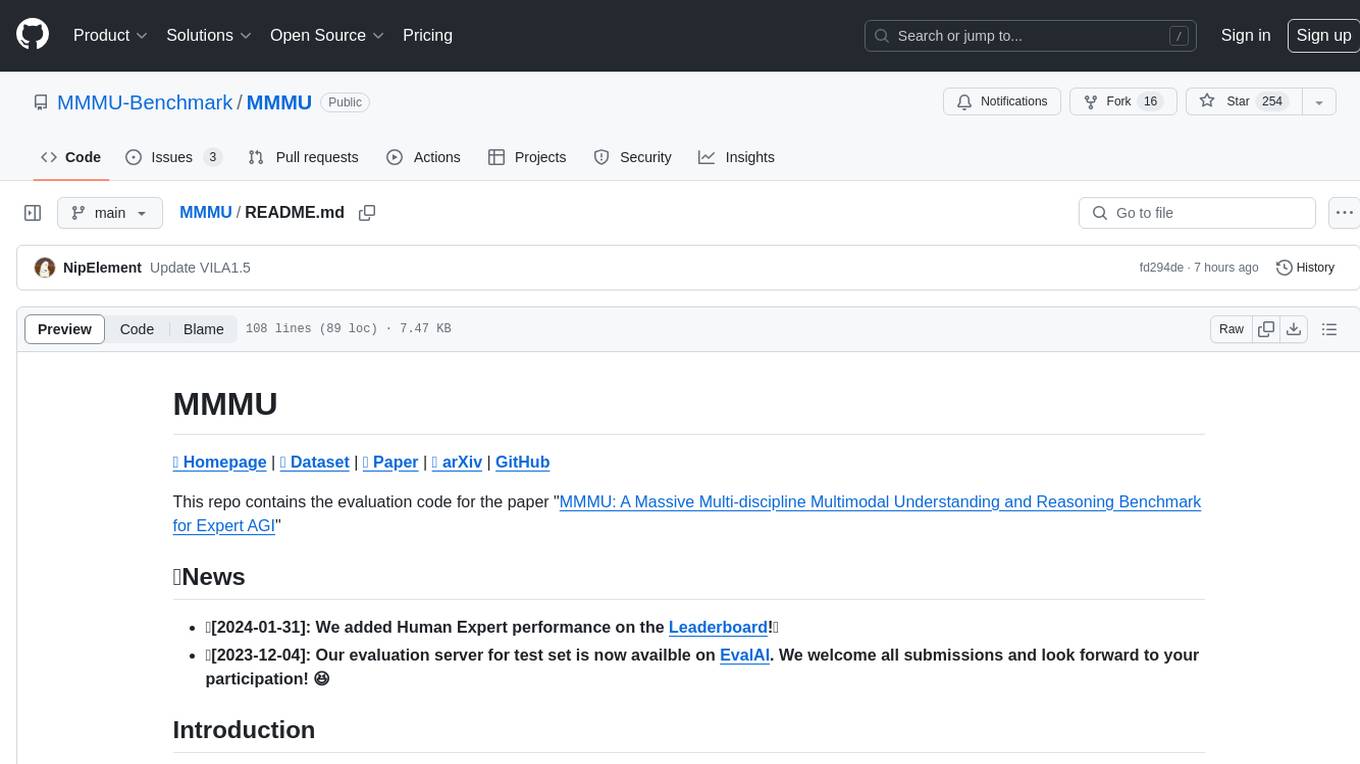
MMMU
MMMU is a benchmark designed to evaluate multimodal models on college-level subject knowledge tasks, covering 30 subjects and 183 subfields with 11.5K questions. It focuses on advanced perception and reasoning with domain-specific knowledge, challenging models to perform tasks akin to those faced by experts. The evaluation of various models highlights substantial challenges, with room for improvement to stimulate the community towards expert artificial general intelligence (AGI).
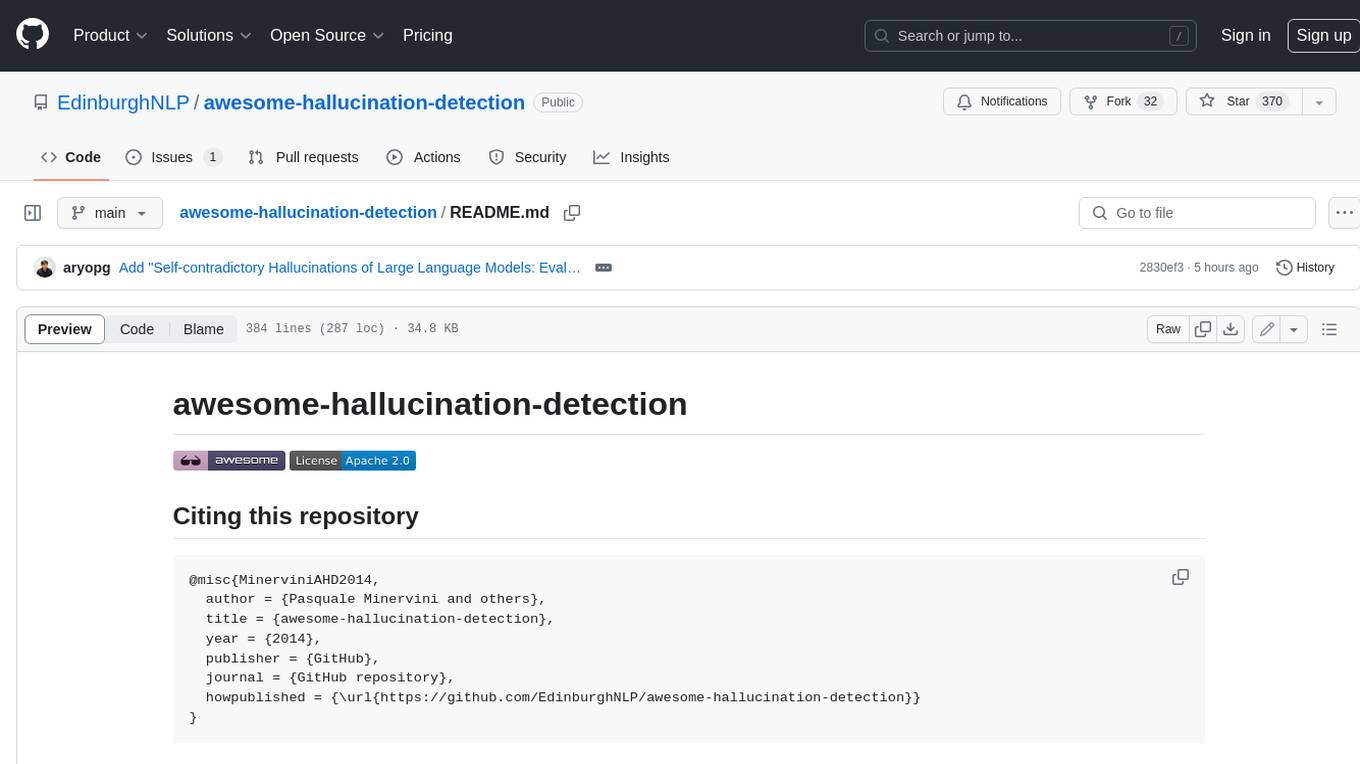
awesome-hallucination-detection
This repository provides a curated list of papers, datasets, and resources related to the detection and mitigation of hallucinations in large language models (LLMs). Hallucinations refer to the generation of factually incorrect or nonsensical text by LLMs, which can be a significant challenge for their use in real-world applications. The resources in this repository aim to help researchers and practitioners better understand and address this issue.
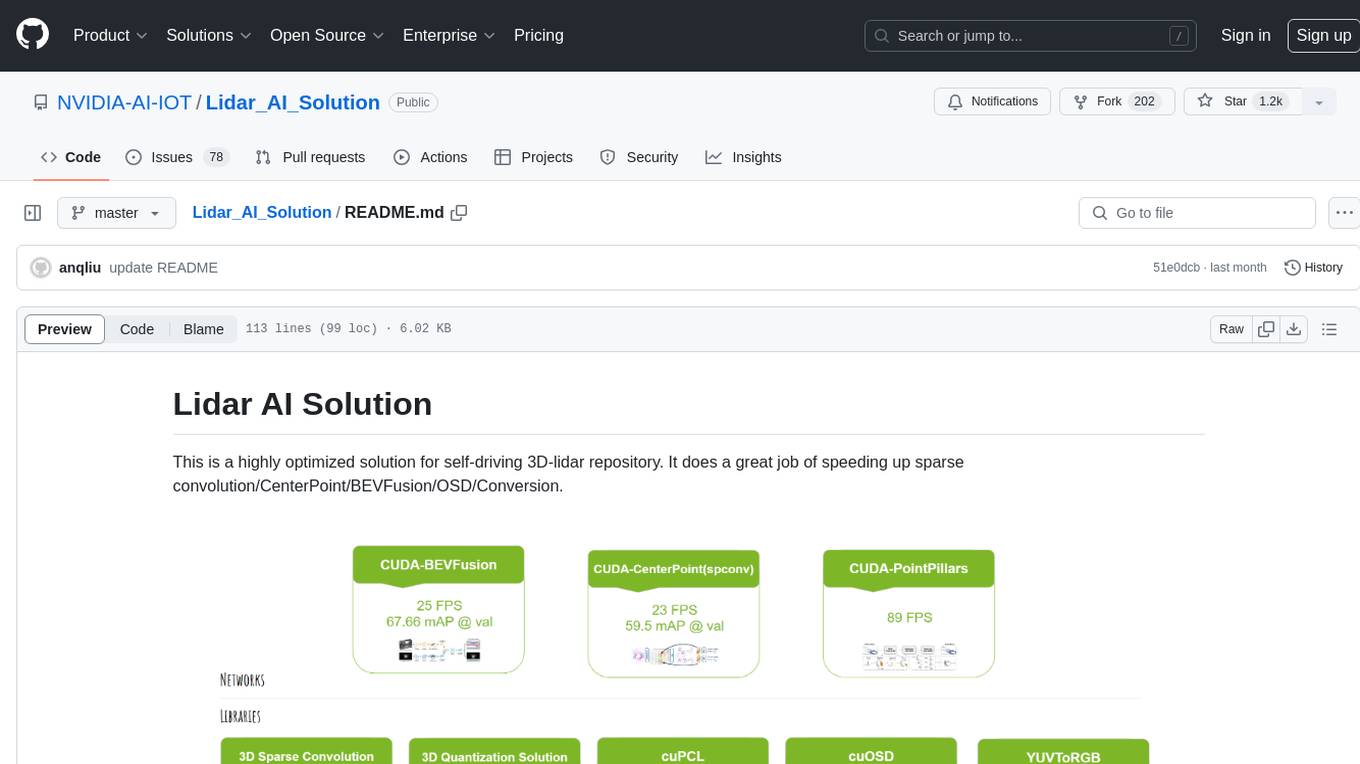
Lidar_AI_Solution
Lidar AI Solution is a highly optimized repository for self-driving 3D lidar, providing solutions for sparse convolution, BEVFusion, CenterPoint, OSD, and Conversion. It includes CUDA and TensorRT implementations for various tasks such as 3D sparse convolution, BEVFusion, CenterPoint, PointPillars, V2XFusion, cuOSD, cuPCL, and YUV to RGB conversion. The repository offers easy-to-use solutions, high accuracy, low memory usage, and quantization options for different tasks related to self-driving technology.
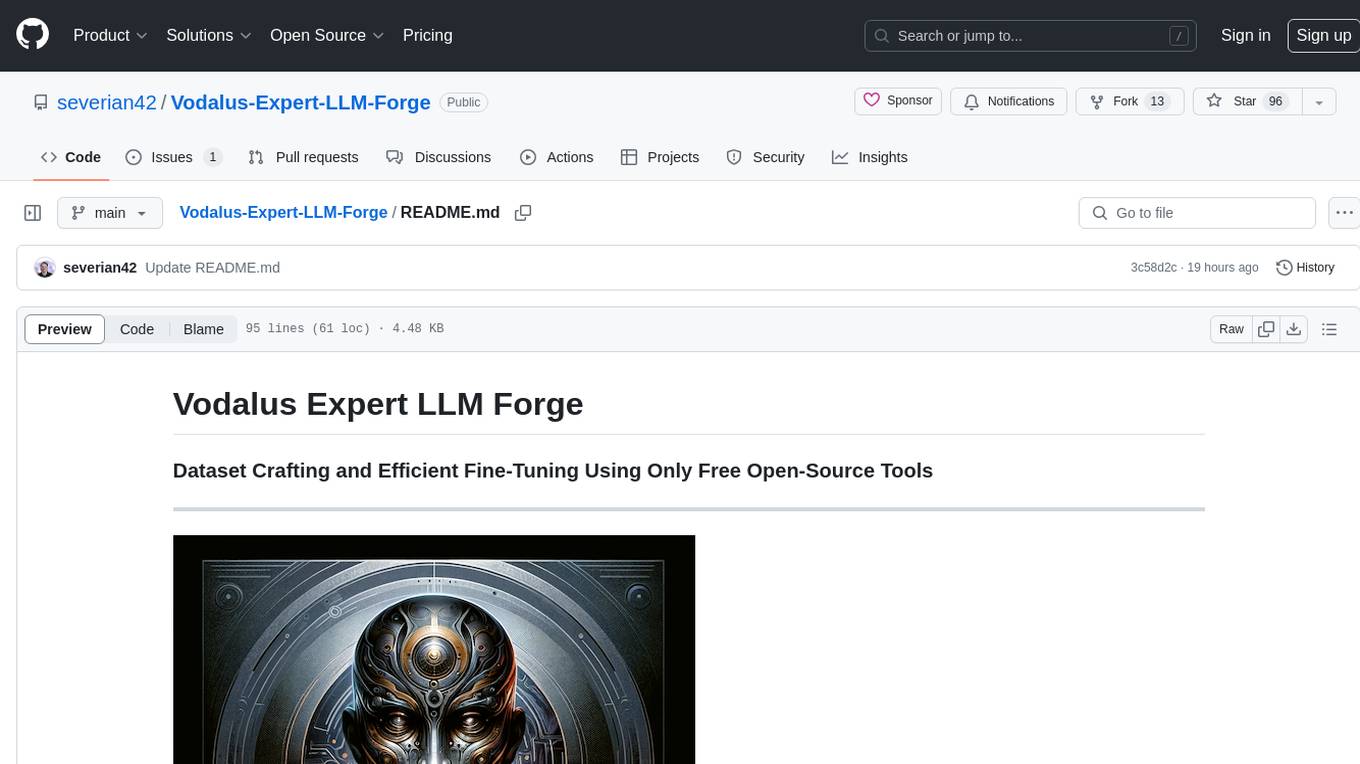
Vodalus-Expert-LLM-Forge
Vodalus Expert LLM Forge is a tool designed for crafting datasets and efficiently fine-tuning models using free open-source tools. It includes components for data generation, LLM interaction, RAG engine integration, model training, fine-tuning, and quantization. The tool is suitable for users at all levels and is accompanied by comprehensive documentation. Users can generate synthetic data, interact with LLMs, train models, and optimize performance for local execution. The tool provides detailed guides and instructions for setup, usage, and customization.
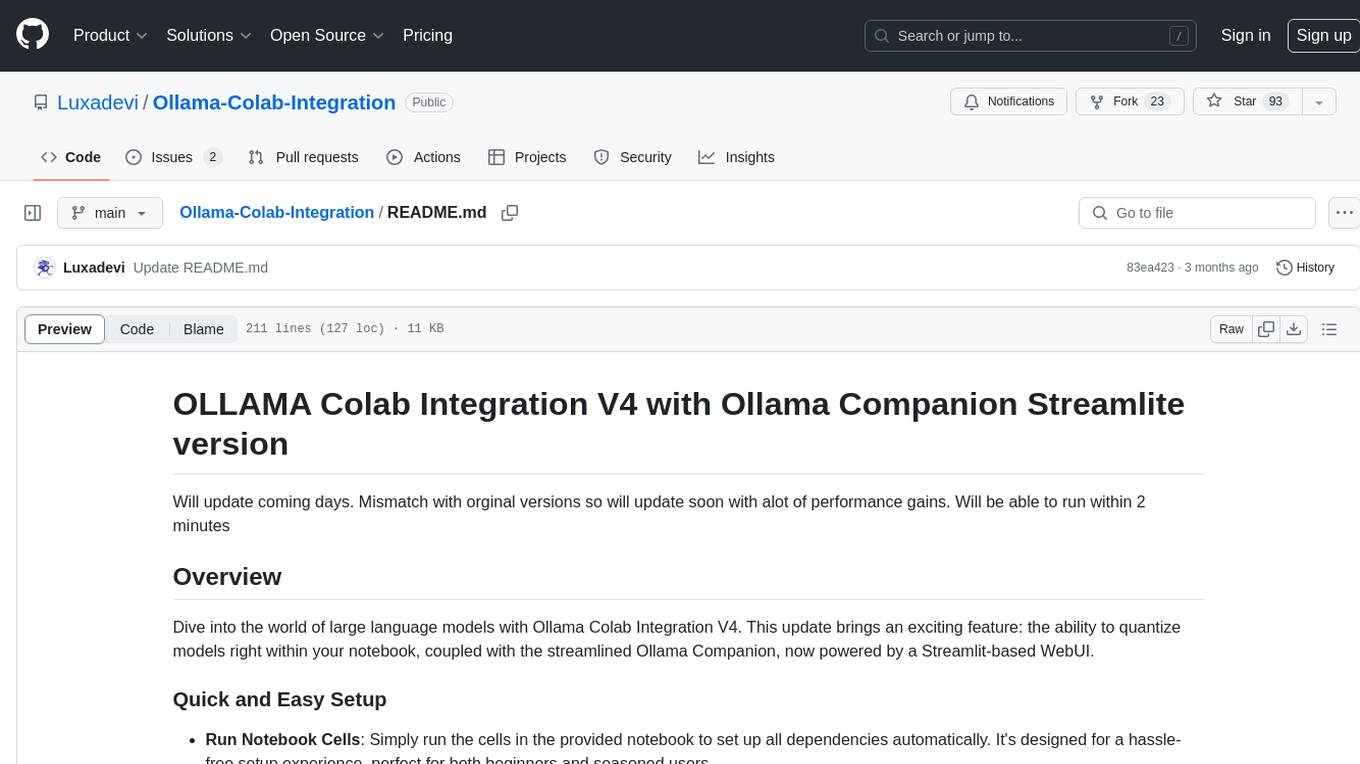
Ollama-Colab-Integration
Ollama Colab Integration V4 is a tool designed to enhance the interaction and management of large language models. It allows users to quantize models within their notebook environment, access a variety of models through a user-friendly interface, and manage public endpoints efficiently. The tool also provides features like LiteLLM proxy control, model insights, and customizable model file templating. Users can troubleshoot model loading issues, CPU fallback strategies, and manage VRAM and RAM effectively. Additionally, the tool offers functionalities for downloading model files from Hugging Face, model conversion with high precision, model quantization using Q and Kquants, and securely uploading converted models to Hugging Face.
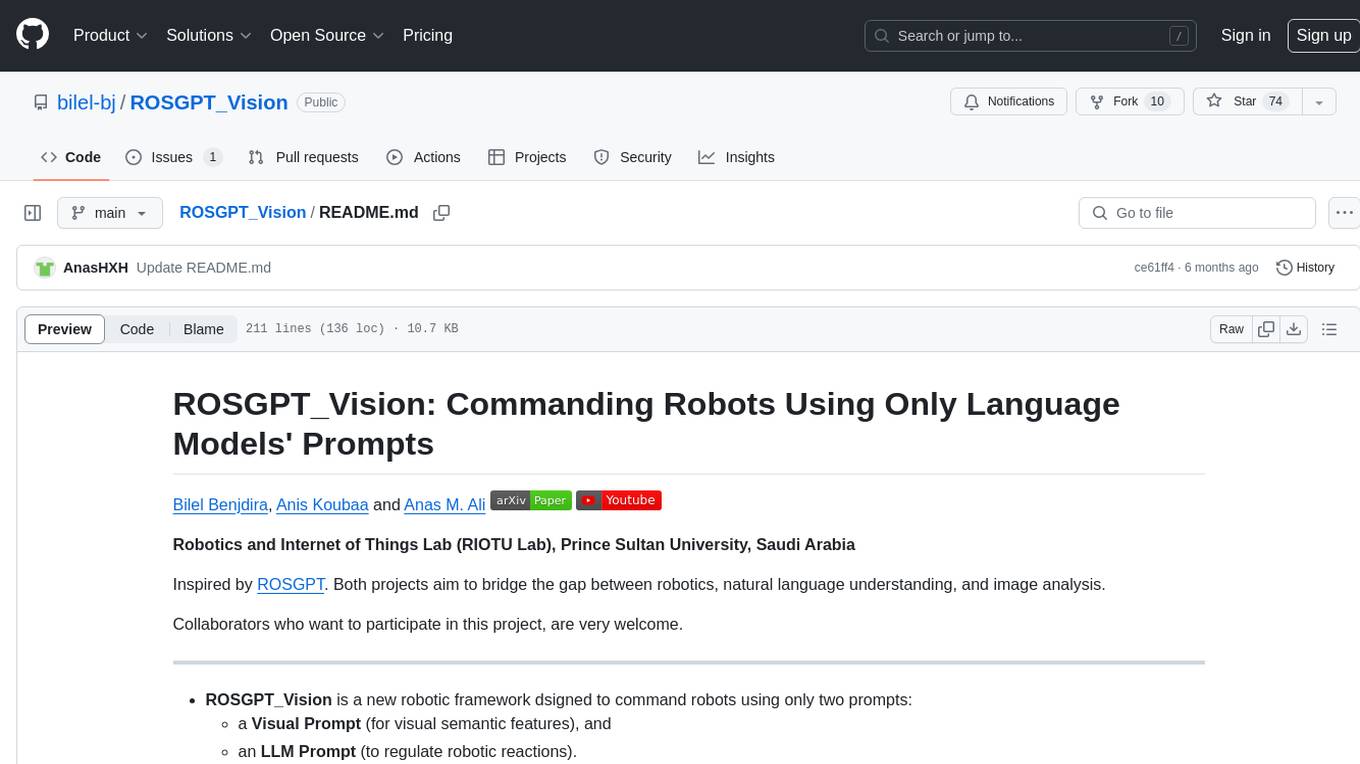
ROSGPT_Vision
ROSGPT_Vision is a new robotic framework designed to command robots using only two prompts: a Visual Prompt for visual semantic features and an LLM Prompt to regulate robotic reactions. It is based on the Prompting Robotic Modalities (PRM) design pattern and is used to develop CarMate, a robotic application for monitoring driver distractions and providing real-time vocal notifications. The framework leverages state-of-the-art language models to facilitate advanced reasoning about image data and offers a unified platform for robots to perceive, interpret, and interact with visual data through natural language. LangChain is used for easy customization of prompts, and the implementation includes the CarMate application for driver monitoring and assistance.
For similar tasks
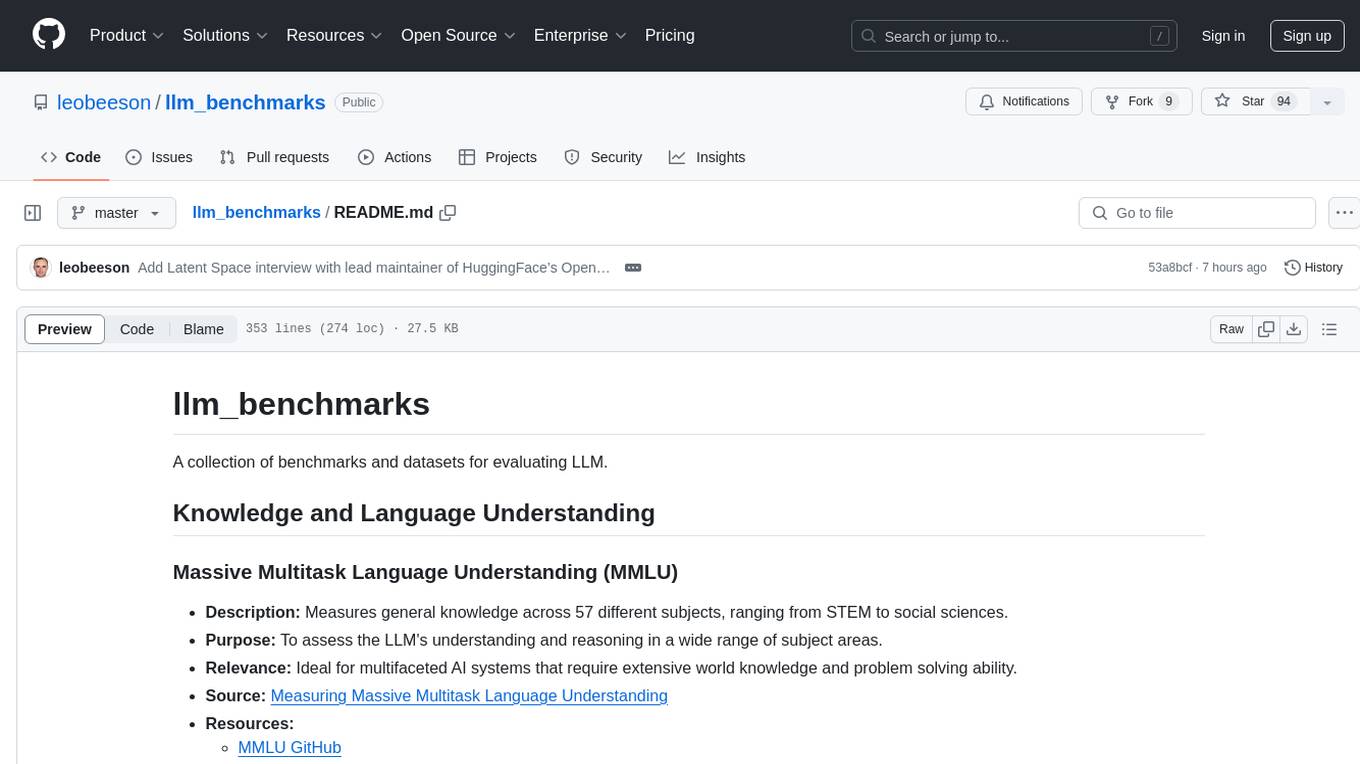
llm_benchmarks
llm_benchmarks is a collection of benchmarks and datasets for evaluating Large Language Models (LLMs). It includes various tasks and datasets to assess LLMs' knowledge, reasoning, language understanding, and conversational abilities. The repository aims to provide comprehensive evaluation resources for LLMs across different domains and applications, such as education, healthcare, content moderation, coding, and conversational AI. Researchers and developers can leverage these benchmarks to test and improve the performance of LLMs in various real-world scenarios.
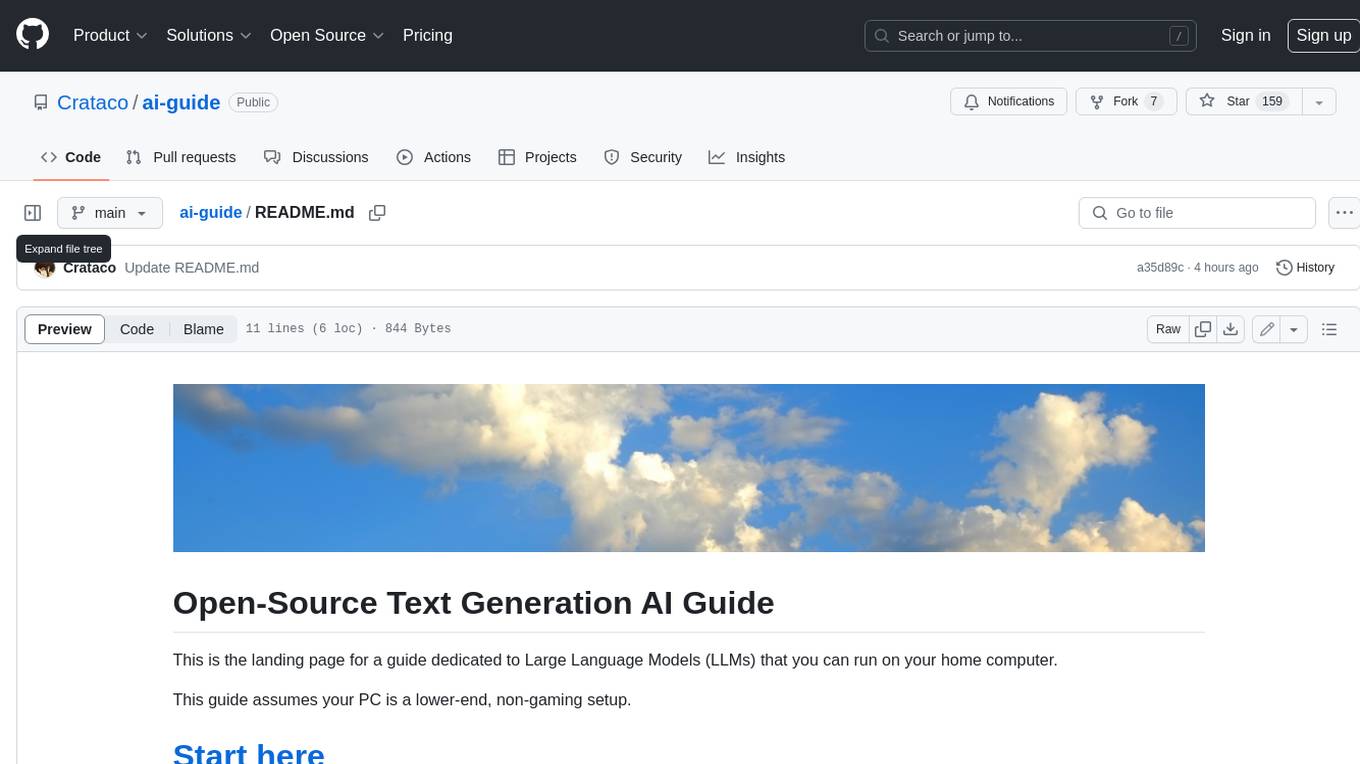
ai-guide
This guide is dedicated to Large Language Models (LLMs) that you can run on your home computer. It assumes your PC is a lower-end, non-gaming setup.
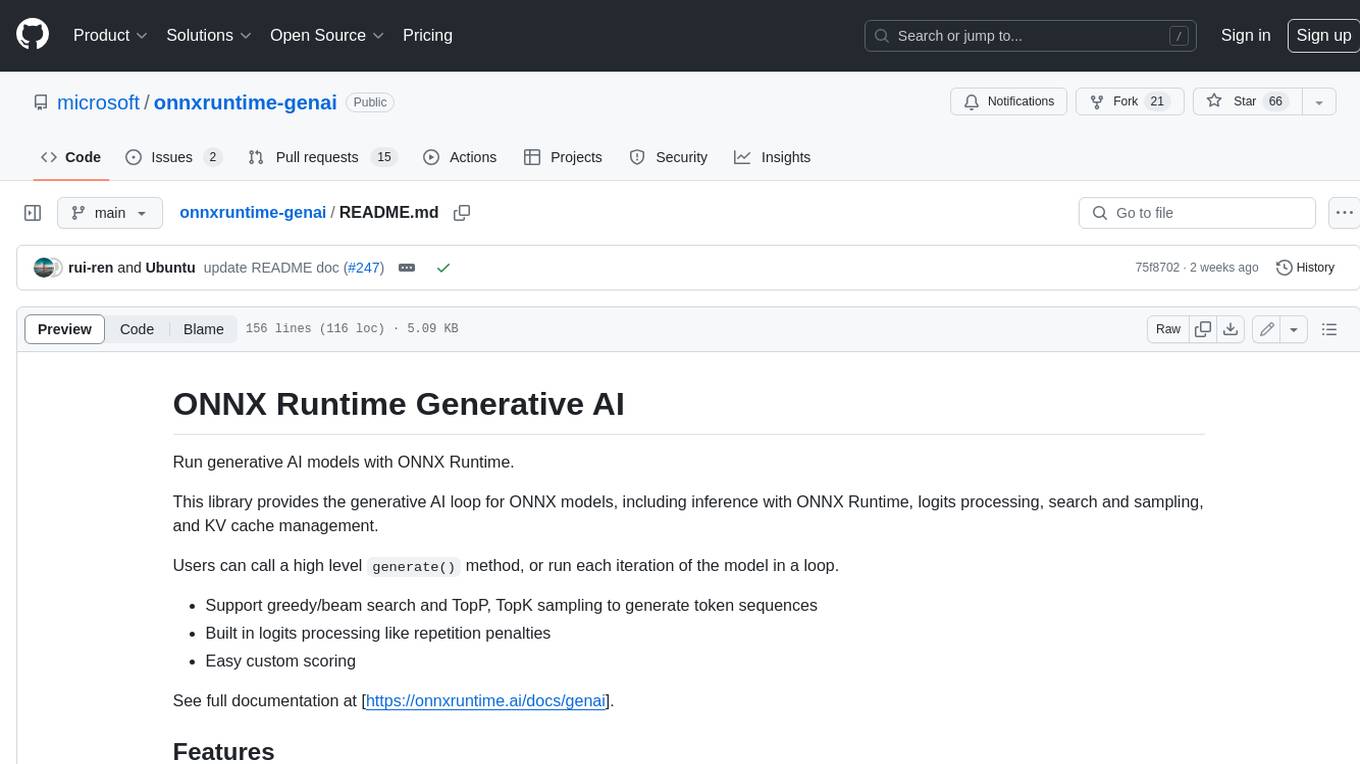
onnxruntime-genai
ONNX Runtime Generative AI is a library that provides the generative AI loop for ONNX models, including inference with ONNX Runtime, logits processing, search and sampling, and KV cache management. Users can call a high level `generate()` method, or run each iteration of the model in a loop. It supports greedy/beam search and TopP, TopK sampling to generate token sequences, has built in logits processing like repetition penalties, and allows for easy custom scoring.
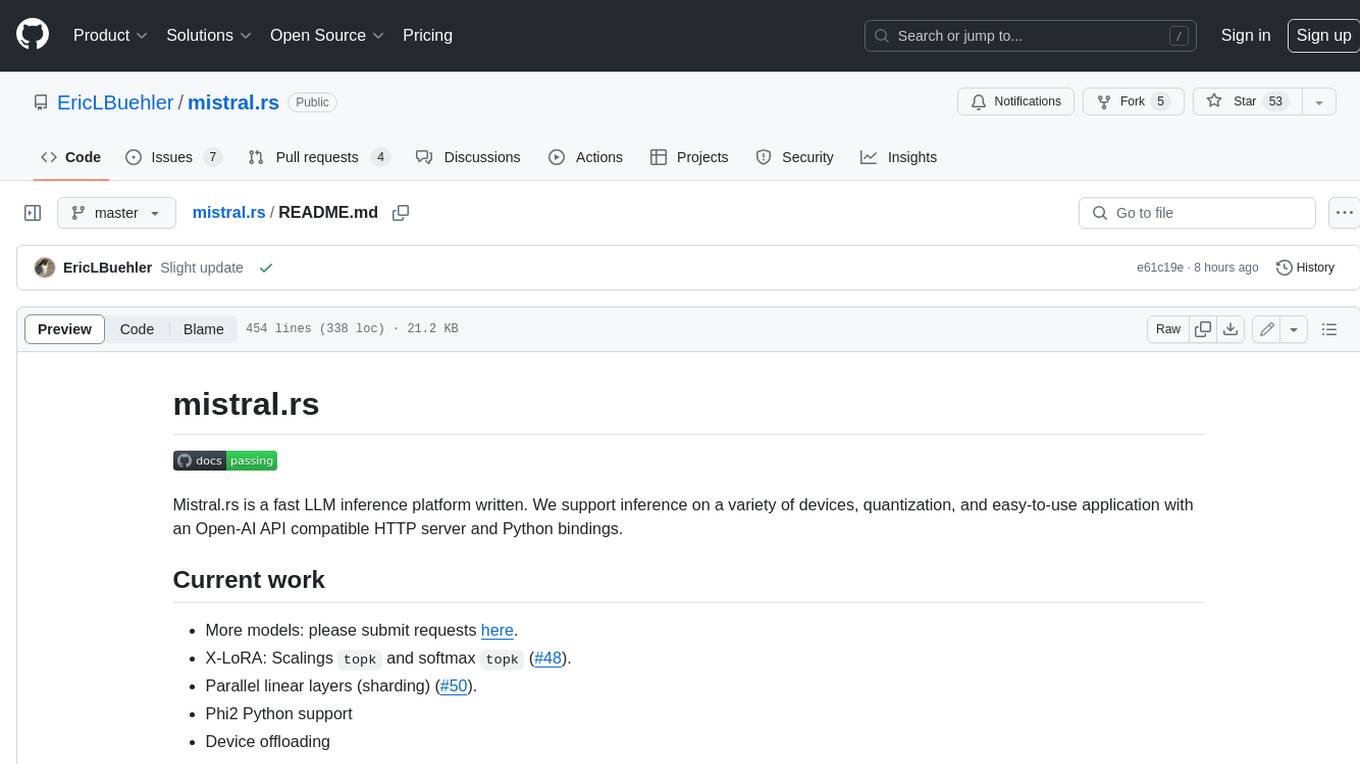
mistral.rs
Mistral.rs is a fast LLM inference platform written in Rust. We support inference on a variety of devices, quantization, and easy-to-use application with an Open-AI API compatible HTTP server and Python bindings.
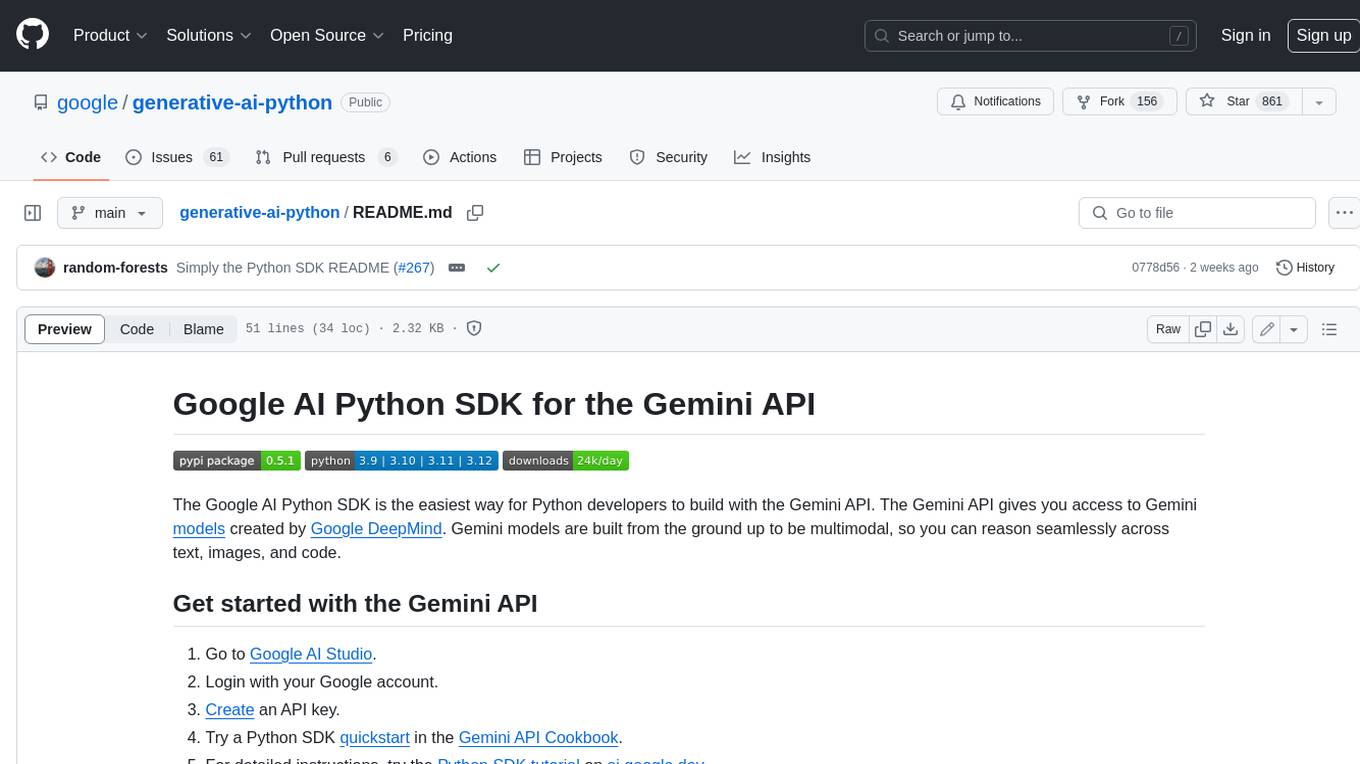
generative-ai-python
The Google AI Python SDK is the easiest way for Python developers to build with the Gemini API. The Gemini API gives you access to Gemini models created by Google DeepMind. Gemini models are built from the ground up to be multimodal, so you can reason seamlessly across text, images, and code.
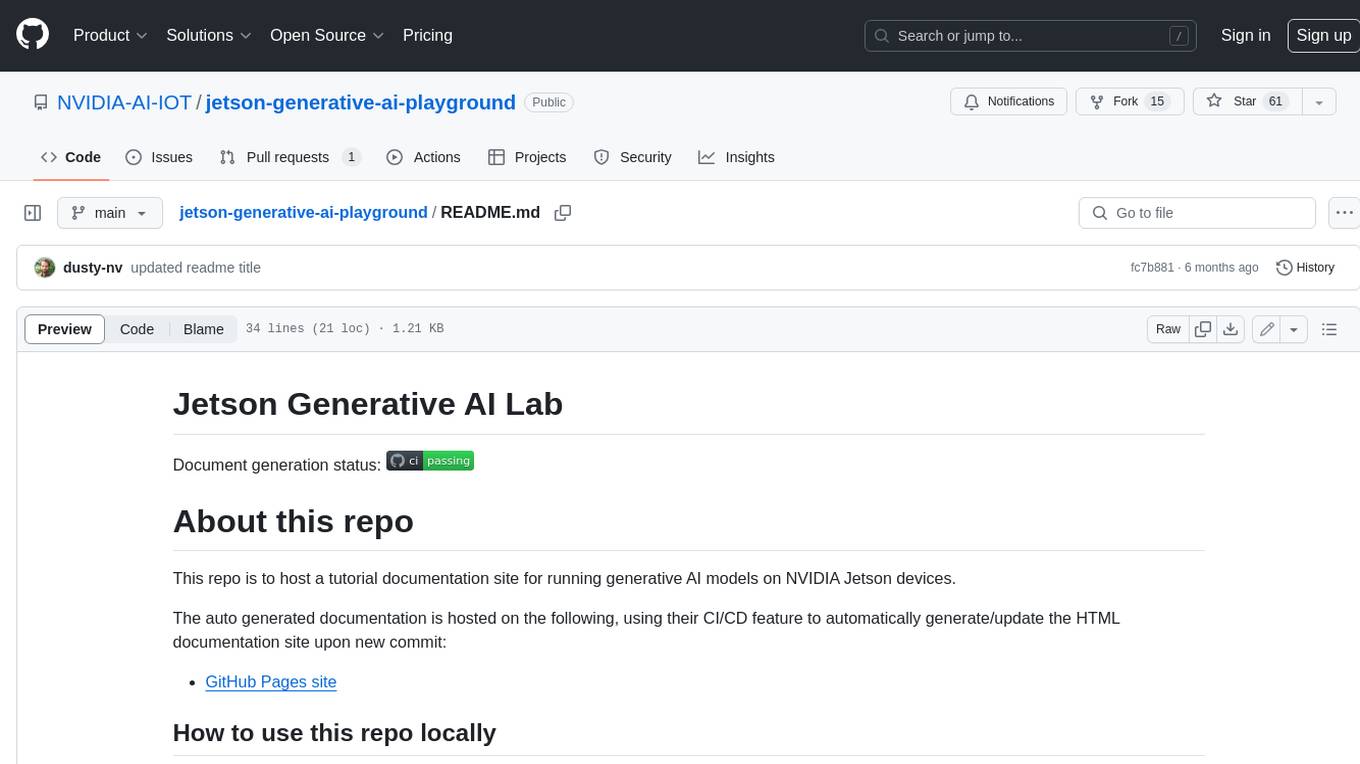
jetson-generative-ai-playground
This repo hosts tutorial documentation for running generative AI models on NVIDIA Jetson devices. The documentation is auto-generated and hosted on GitHub Pages using their CI/CD feature to automatically generate/update the HTML documentation site upon new commits.
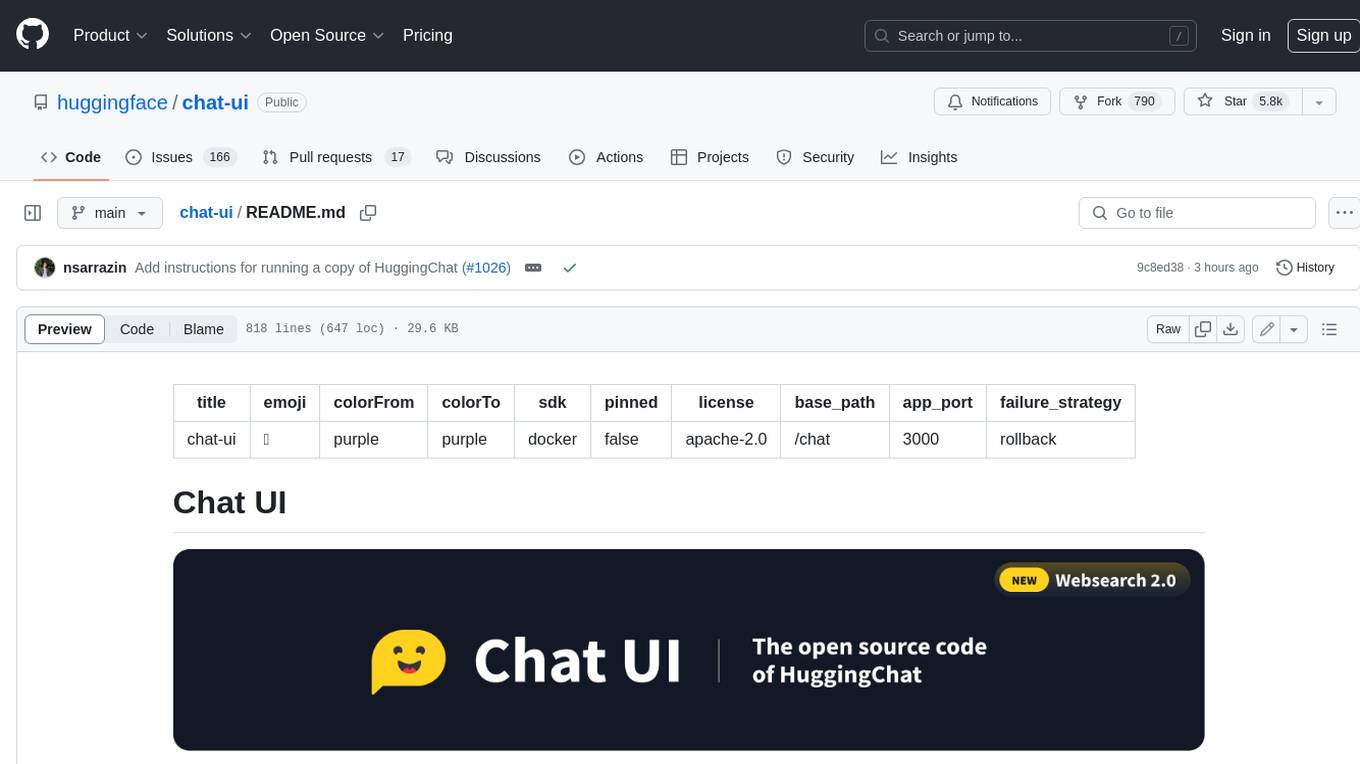
chat-ui
A chat interface using open source models, eg OpenAssistant or Llama. It is a SvelteKit app and it powers the HuggingChat app on hf.co/chat.
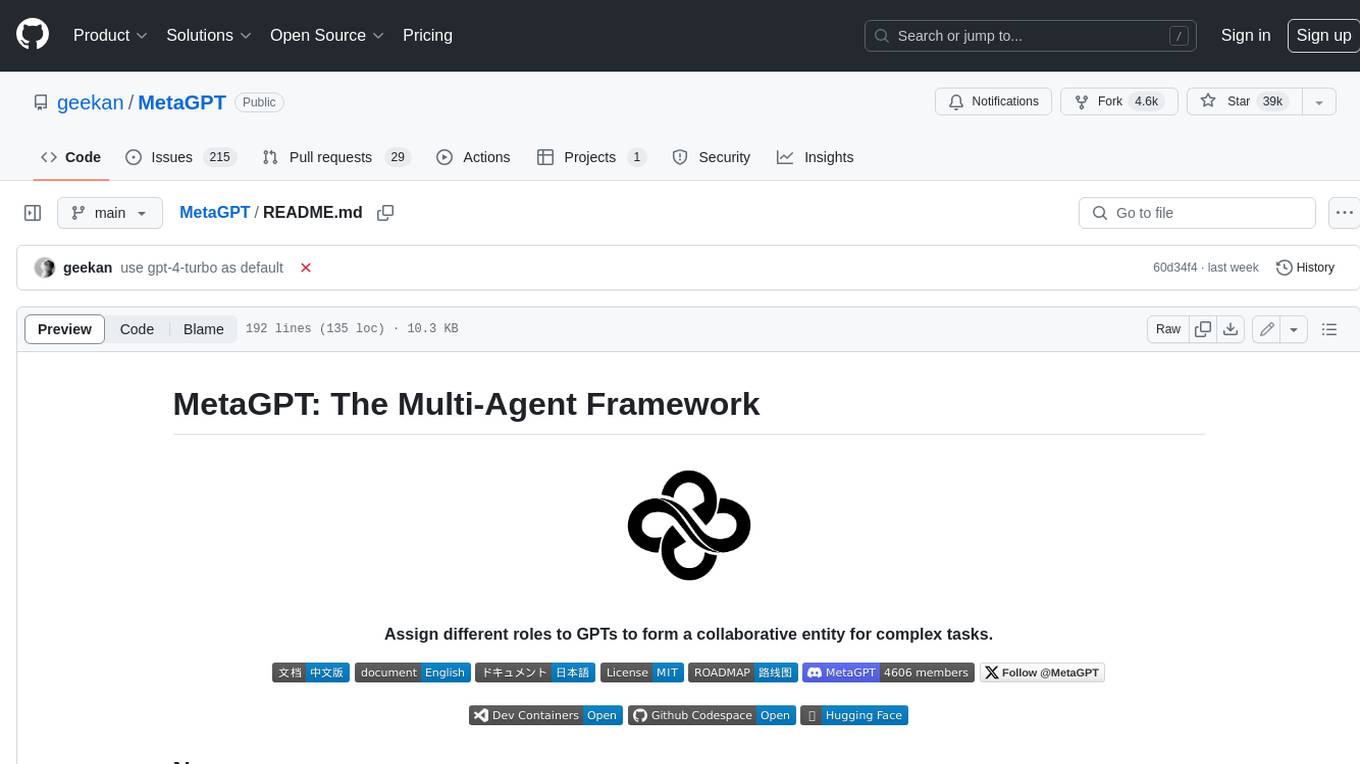
MetaGPT
MetaGPT is a multi-agent framework that enables GPT to work in a software company, collaborating to tackle more complex tasks. It assigns different roles to GPTs to form a collaborative entity for complex tasks. MetaGPT takes a one-line requirement as input and outputs user stories, competitive analysis, requirements, data structures, APIs, documents, etc. Internally, MetaGPT includes product managers, architects, project managers, and engineers. It provides the entire process of a software company along with carefully orchestrated SOPs. MetaGPT's core philosophy is "Code = SOP(Team)", materializing SOP and applying it to teams composed of LLMs.
For similar jobs
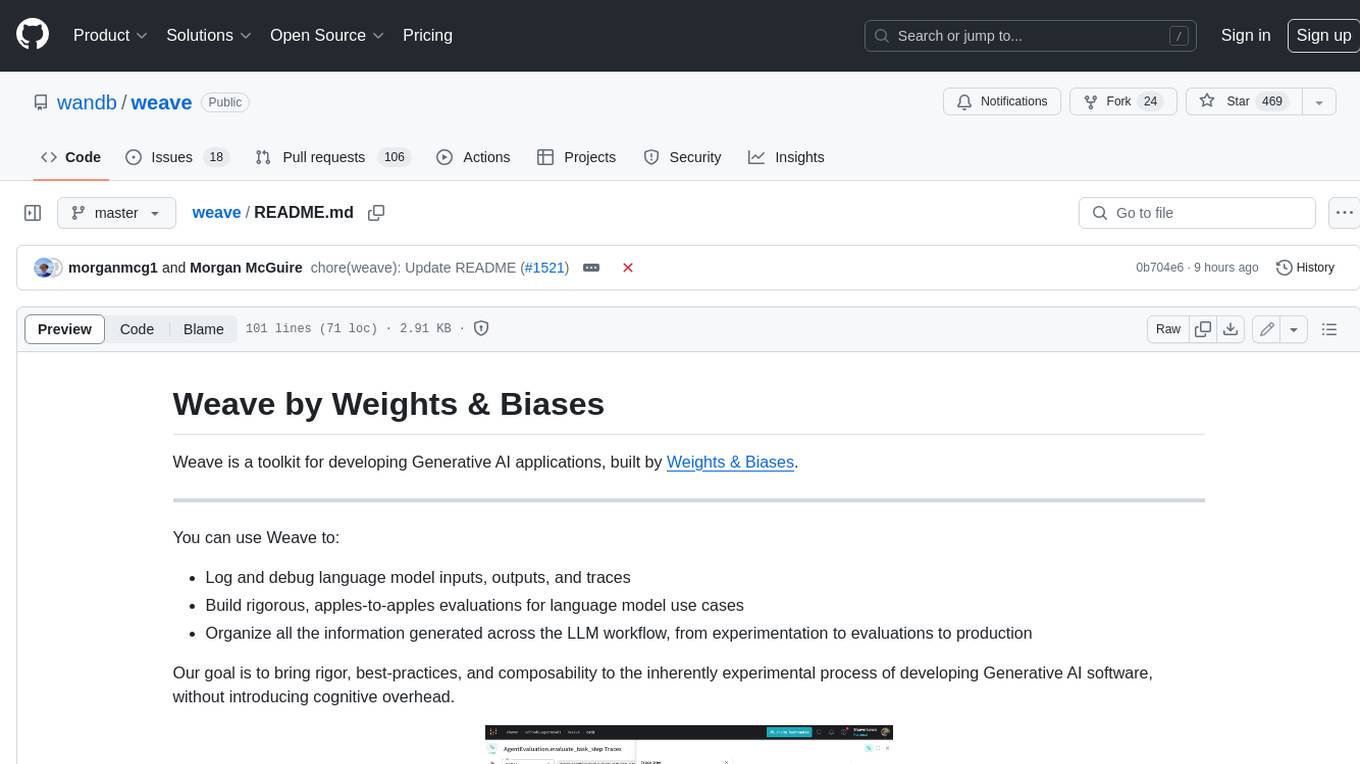
weave
Weave is a toolkit for developing Generative AI applications, built by Weights & Biases. With Weave, you can log and debug language model inputs, outputs, and traces; build rigorous, apples-to-apples evaluations for language model use cases; and organize all the information generated across the LLM workflow, from experimentation to evaluations to production. Weave aims to bring rigor, best-practices, and composability to the inherently experimental process of developing Generative AI software, without introducing cognitive overhead.
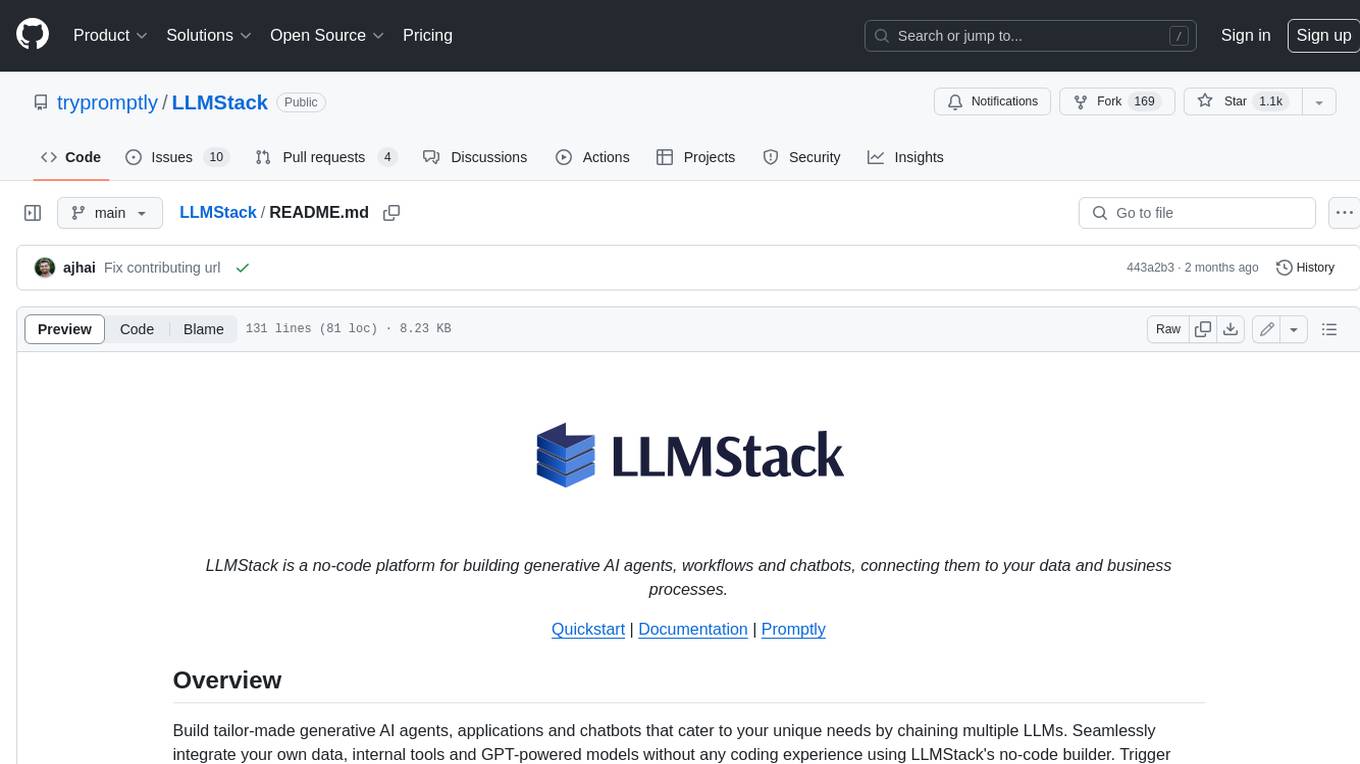
LLMStack
LLMStack is a no-code platform for building generative AI agents, workflows, and chatbots. It allows users to connect their own data, internal tools, and GPT-powered models without any coding experience. LLMStack can be deployed to the cloud or on-premise and can be accessed via HTTP API or triggered from Slack or Discord.
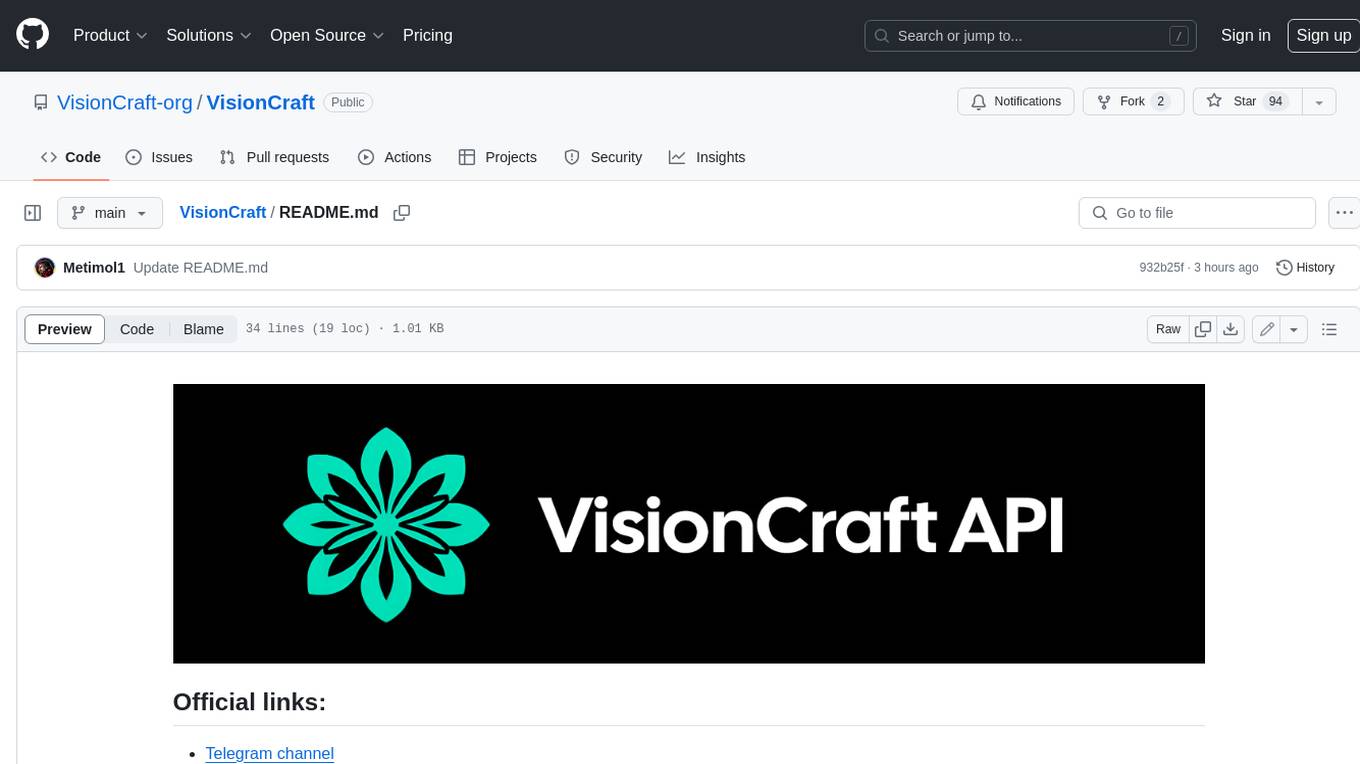
VisionCraft
The VisionCraft API is a free API for using over 100 different AI models. From images to sound.
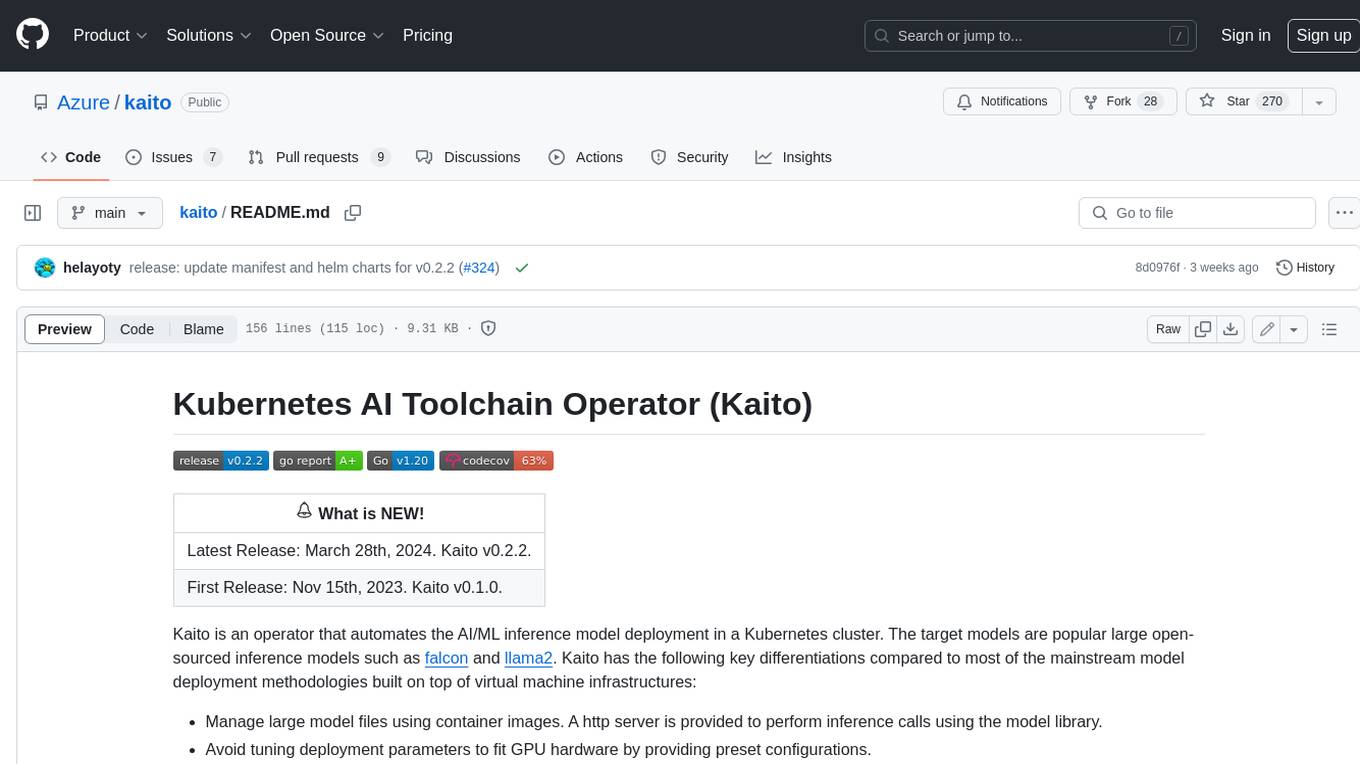
kaito
Kaito is an operator that automates the AI/ML inference model deployment in a Kubernetes cluster. It manages large model files using container images, avoids tuning deployment parameters to fit GPU hardware by providing preset configurations, auto-provisions GPU nodes based on model requirements, and hosts large model images in the public Microsoft Container Registry (MCR) if the license allows. Using Kaito, the workflow of onboarding large AI inference models in Kubernetes is largely simplified.
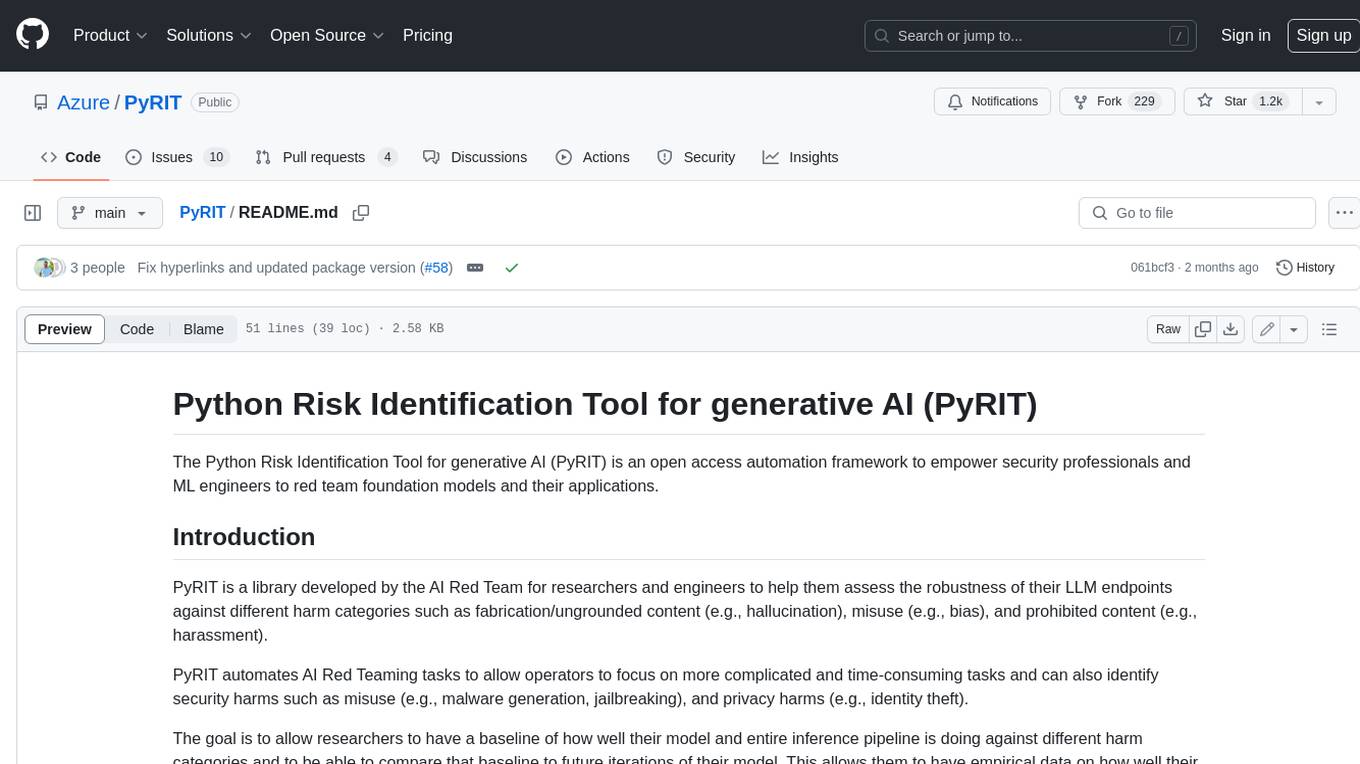
PyRIT
PyRIT is an open access automation framework designed to empower security professionals and ML engineers to red team foundation models and their applications. It automates AI Red Teaming tasks to allow operators to focus on more complicated and time-consuming tasks and can also identify security harms such as misuse (e.g., malware generation, jailbreaking), and privacy harms (e.g., identity theft). The goal is to allow researchers to have a baseline of how well their model and entire inference pipeline is doing against different harm categories and to be able to compare that baseline to future iterations of their model. This allows them to have empirical data on how well their model is doing today, and detect any degradation of performance based on future improvements.
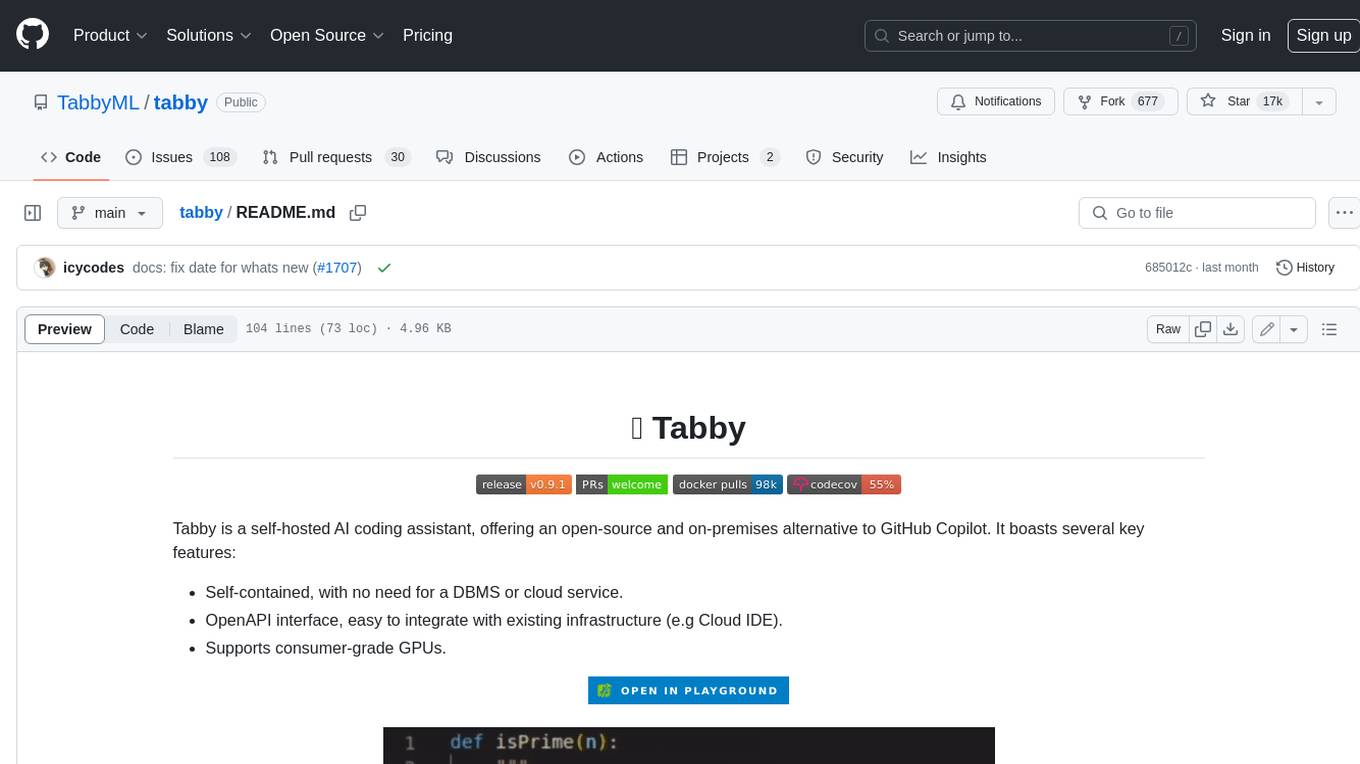
tabby
Tabby is a self-hosted AI coding assistant, offering an open-source and on-premises alternative to GitHub Copilot. It boasts several key features: * Self-contained, with no need for a DBMS or cloud service. * OpenAPI interface, easy to integrate with existing infrastructure (e.g Cloud IDE). * Supports consumer-grade GPUs.
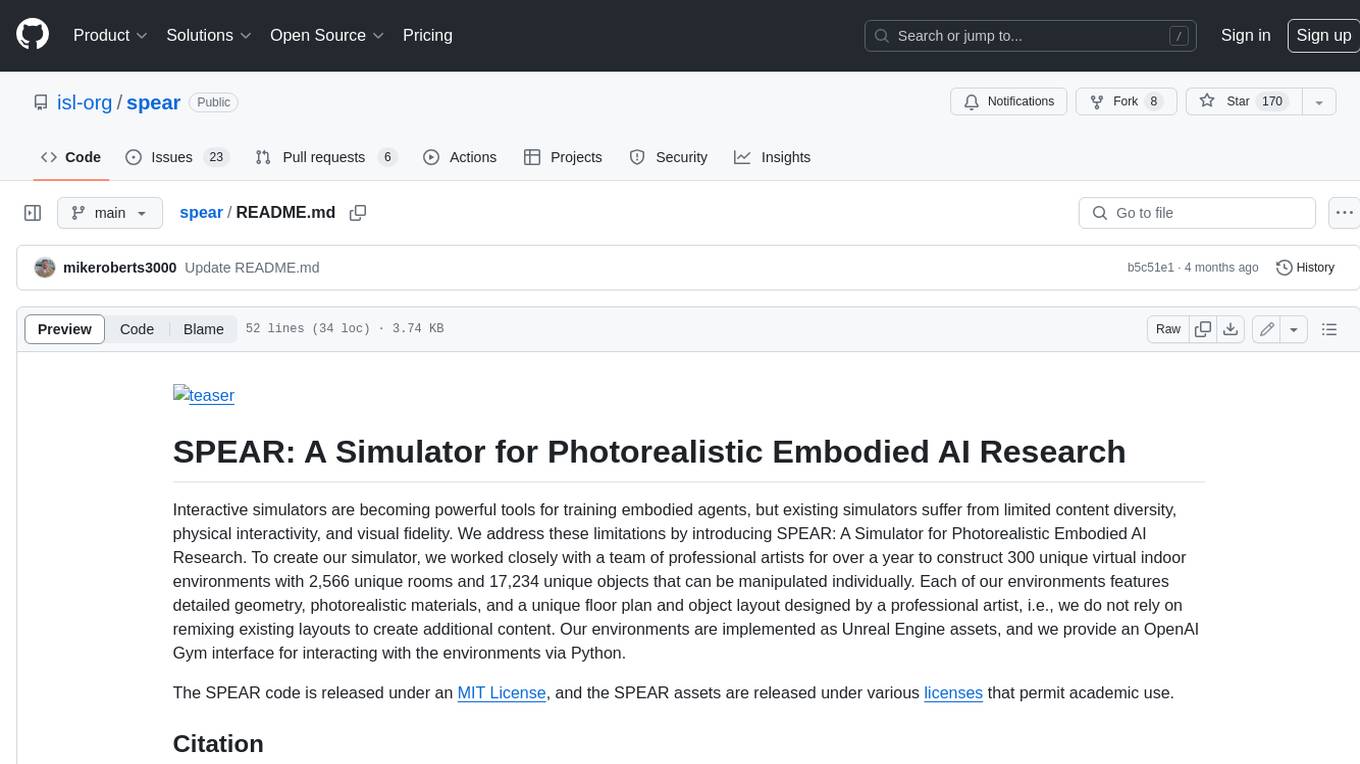
spear
SPEAR (Simulator for Photorealistic Embodied AI Research) is a powerful tool for training embodied agents. It features 300 unique virtual indoor environments with 2,566 unique rooms and 17,234 unique objects that can be manipulated individually. Each environment is designed by a professional artist and features detailed geometry, photorealistic materials, and a unique floor plan and object layout. SPEAR is implemented as Unreal Engine assets and provides an OpenAI Gym interface for interacting with the environments via Python.
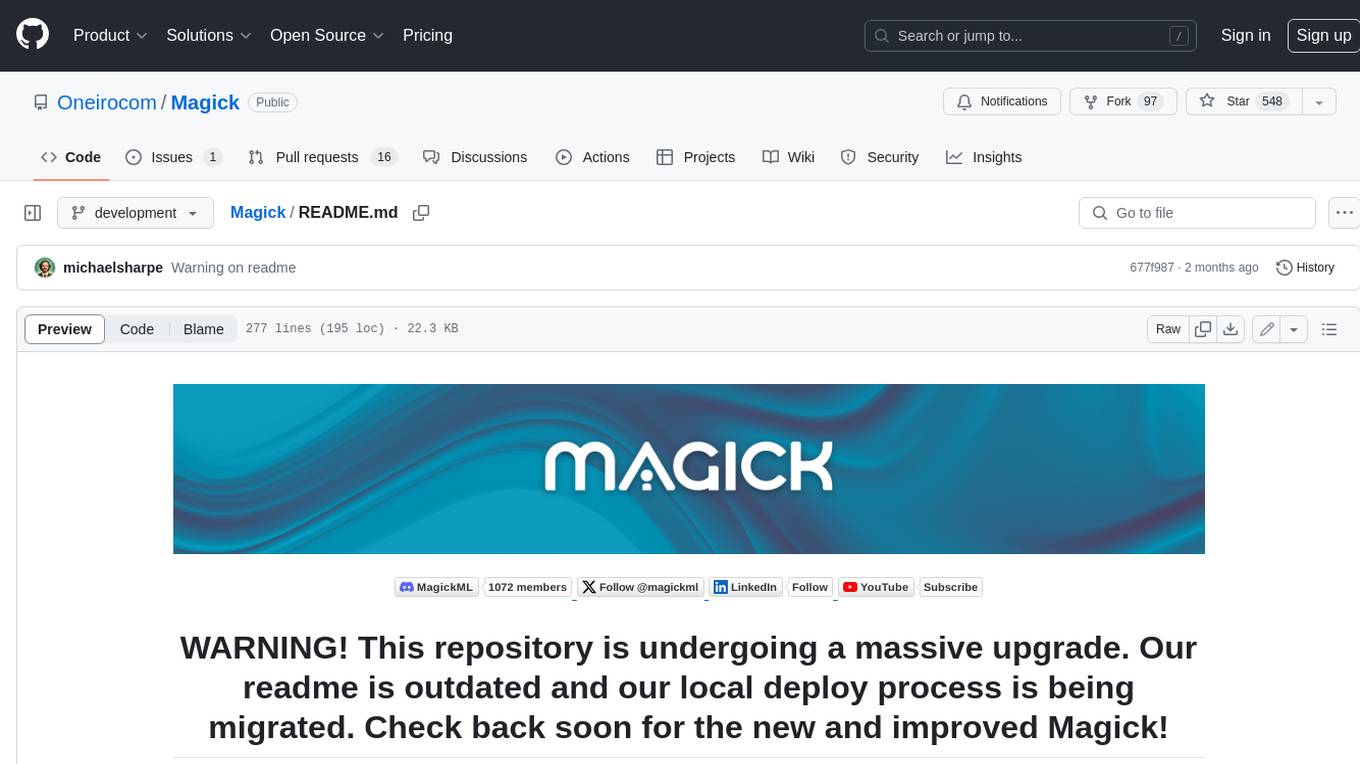
Magick
Magick is a groundbreaking visual AIDE (Artificial Intelligence Development Environment) for no-code data pipelines and multimodal agents. Magick can connect to other services and comes with nodes and templates well-suited for intelligent agents, chatbots, complex reasoning systems and realistic characters.