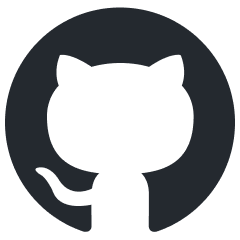
Mooncake
Mooncake is the serving platform for Kimi, a leading LLM service provided by Moonshot AI.
Stars: 2972
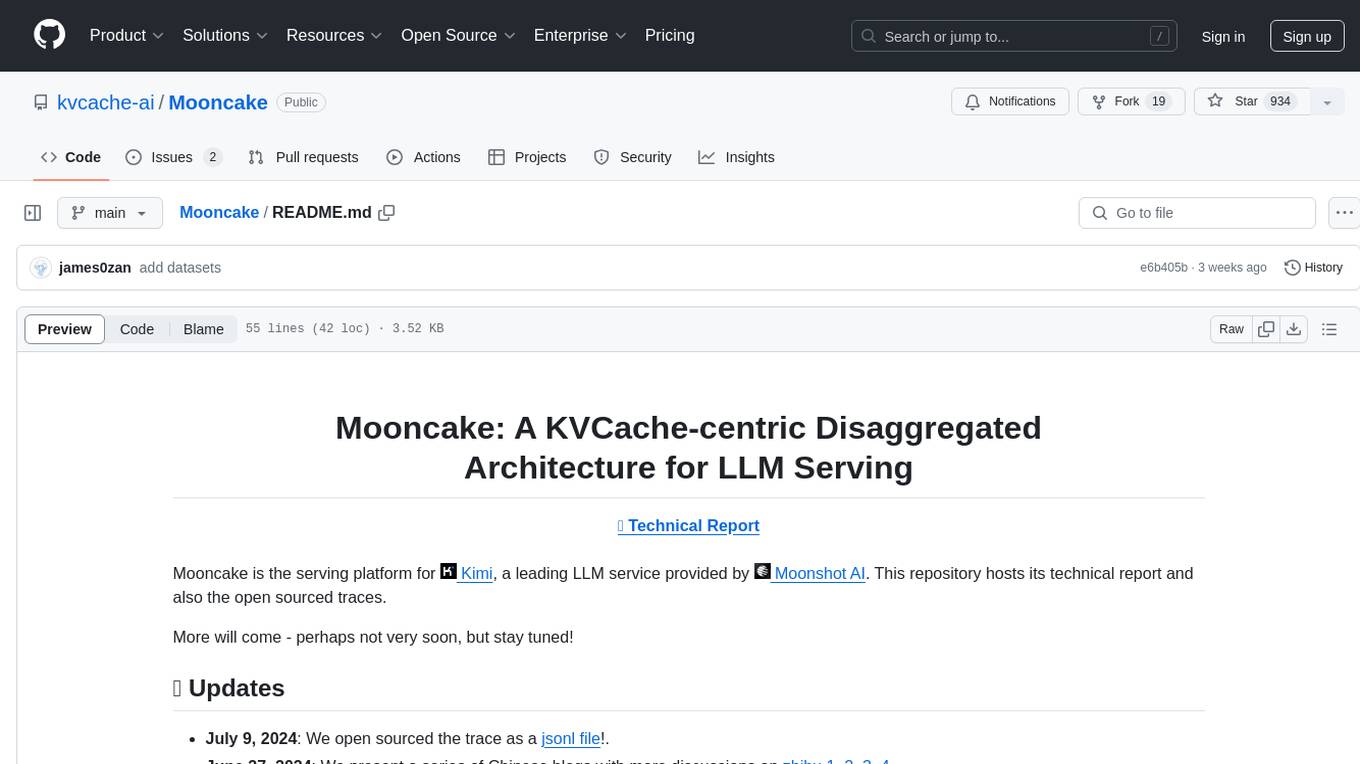
Mooncake is a serving platform for Kimi, a leading LLM service provided by Moonshot AI. It features a KVCache-centric disaggregated architecture that separates prefill and decoding clusters, leveraging underutilized CPU, DRAM, and SSD resources of the GPU cluster. Mooncake's scheduler balances throughput and latency-related SLOs, with a prediction-based early rejection policy for highly overloaded scenarios. It excels in long-context scenarios, achieving up to a 525% increase in throughput while handling 75% more requests under real workloads.
README:
Mooncake is the serving platform for Kimi, a leading LLM service provided by
Moonshot AI.
Now both the Transfer Engine and Mooncake Store are open-sourced!
This repository also hosts its technical report and the open sourced traces.
- Mar 7, 2025: We open sourced the Mooncake Store, a distributed KVCache based on Transfer Engine. vLLM's xPyD disaggregated prefilling & decoding based on Mooncake Store will be released soon.
- Feb 25, 2025: Mooncake receives the Best Paper Award at FAST 2025!
- Feb 21, 2025: The updated traces used in our FAST'25 paper have been released.
- Dec 16, 2024: vLLM officially supports Mooncake Transfer Engine for disaggregated prefilling and KV cache transfer.
- Nov 28, 2024: We open sourced the Transfer Engine, the central component of Mooncake. We also provide two demonstrations of Transfer Engine: a P2P Store and vLLM integration.
- July 9, 2024: We open sourced the trace as a jsonl file.
- June 27, 2024: We present a series of Chinese blogs with more discussions on zhihu 1, 2, 3, 4.
- June 26, 2024: Initial technical report release.
Mooncake features a KVCache-centric disaggregated architecture that separates the prefill and decoding clusters. It also leverages the underutilized CPU, DRAM, and SSD resources of the GPU cluster to implement a disaggregated cache of KVCache.
The core of Mooncake is its KVCache-centric scheduler, which balances maximizing overall effective throughput while meeting latency-related Service Level Objectives (SLOs) requirements. Unlike traditional studies that assume all requests will be processed, Mooncake faces challenges due to highly overloaded scenarios. To mitigate these, we developed a prediction-based early rejection policy. Experiments show that Mooncake excels in long-context scenarios. Compared to the baseline method, Mooncake can achieve up to a 525% increase in throughput in certain simulated scenarios while adhering to SLOs. Under real workloads, Mooncakeβs innovative architecture enables Kimi to handle 75% more requests.
- The bottom part of Mooncake is Transfer Engine, which supports rapid, reliable and flexible data transfer over TCP, RDMA, NVIDIA GPUDirect-based RDMA and and NVMe over Fabric (NVMe-of) protocols. Comparing with gloo (used by Distributed PyTorch) and TCP, Mooncake Transfer Engine has the lowest I/O latency.
- Based on Transfer Engine, we implemented the P2P Store library, supports sharing temporary objects (e.g., checkpoint files) among nodes in a cluster. It avoids bandwidth saturation on a single machine.
- Additionally, we modified vLLM so that Transfer Engine is integrated. It makes prefill-decode disaggregation more efficient by utilizing RDMA devices.
- Mooncake Store is based on Transfer Engine, which supports distributed pooled KVCache for vLLM's xPyD disaggregation.
Use Transfer Engine Standalone (Guide)
Transfer Engine is a high-performance data transfer framework. Transfer Engine provides a unified interface to transfer data from DRAM, VRAM or NVMe, while the technical details related to hardware are hidden. Transfer Engine supports TCP, RDMA (InfiniBand/RoCEv2/eRDMA/NVIDIA GPUDirect) and NVMe over Fabric (NVMe-of) protocols.
-
Efficient use of multiple RDMA NIC devices. Transfer Engine supports the use of multiple RDMA NIC devices to achieve the aggregation of transfer bandwidth.
-
Topology aware path selection. Transfer Engine can select optimal devices based on the location (NUMA affinity, etc.) of both source and destination.
-
More robust on temporary network error. Once transmission fails, Transfer Engine will try to use alternative paths for data delivery automatically.
With 40 GB of data (equivalent to the size of the KVCache generated by 128k tokens in the LLaMA3-70B model), Mooncake Transfer Engine delivers up to 87 GB/s and 190 GB/s of bandwidth in 4Γ200 Gbps and 8Γ400 Gbps RoCE networks respectively, which are about 2.4x and 4.6x faster than the TCP protocol.
P2P Store (Guide)
P2P Store is built on the Transfer Engine and supports sharing temporary objects between peer nodes in a cluster. P2P Store is ideal for scenarios like checkpoint transfer, where data needs to be rapidly and efficiently shared across a cluster. P2P Store has been used in the checkpoint transfer service of Moonshot AI.
-
Decentralized architecture. P2P Store leverages a pure client-side architecture with global metadata managed by the etcd service.
-
Efficient data distribution. Designed to enhance the efficiency of large-scale data distribution, P2P Store avoids bandwidth saturation issues by allowing replicated nodes to share data directly. This reduces the CPU/RDMA NIC pressures of data providers (e.g., trainers).
Thanks to the high performance of Transfer Engine, P2P Stores can also distribute objects with full utilization of hardware incoming bandwidth (e.g., A 25Gbps NIC was used in the following figure, and the throughput of get replica is about 3.1 GB/s).
Mooncake Store (Guide)
Mooncake Store is a distributed KVCache storage engine specialized for LLM inference. It offers object-level APIs (Put
, Get
and Remove
), and we will soon release an new vLLM integration to demonstrate xPyD disaggregation. Mooncake Store is the central component of the KVCache-centric disaggregated architecture.
vLLM Integration (Guide v0.2)
To optimize LLM inference, the vLLM community is working on supporting disaggregated prefilling (PR 10502). This feature allows separating the prefill phase from the decode phase in different processes. The vLLM uses nccl
and gloo
as the transport layer by default, but currently it cannot efficiently decouple both phases in different machines.
We have implemented vLLM integration, which uses Transfer Engine as the network layer instead of nccl
and gloo
, to support inter-node KVCache transfer (PR 10884). Transfer Engine provides simpler interfaces and more efficient use of RDMA devices.
We will soon release the new vLLM integration based on Mooncake Store, which supports xPyD prefill/decode disaggregation.
Update[Dec 16, 2024]: Here is the latest vLLM Integration (Guide v0.2) that is based on vLLM's main branch.
By supporting Topology Aware Path Selection and multi-card bandwidth aggregation, Mean TTFT of vLLM with Transfer Engine is up to 25% lower than traditional TCP-based transports. In the future, we will further improve TTFT through GPUDirect RDMA and zero-copy.
Backend/Setting | Output Token Throughput (tok/s) | Total Token Throughput (tok/s) | Mean TTFT (ms) | Median TTFT (ms) | P99 TTFT (ms) |
---|---|---|---|---|---|
Transfer Engine (RDMA) | 12.06 | 2042.74 | 1056.76 | 635.00 | 4006.59 |
TCP | 12.05 | 2041.13 | 1414.05 | 766.23 | 6035.36 |
- Click here to access detailed benchmark results.
More advanced features will coming soon, so stay tuned!
In order to install and use Mooncake, some preparation is required.
- RDMA Driver & SDK (e.g., Mellanox OFED).
- Linux-x86_64 with gcc, g++ (9.4+) and cmake (3.16+).
- Python (3.10 or above)
In addition, to support more features of Mooncake Transfer Engine, we recommend you to install the following components:
- CUDA 12.1 and above, including NVIDIA GPUDirect Storage Support, if you want to build with
-DUSE_CUDA
. You may install them from here.# Adding CUDA to PATH export PATH=/usr/local/cuda/bin:$PATH export LD_LIBRARY_PATH=/usr/local/cuda/lib64:$LD_LIBRARY_PATH export CUDA_PATH=/usr/local/cuda
- Go 1.20+, if you want to build with
-DWITH_P2P_STORE
. You may download it from here. - Rust Toolclain, if you want to build with
-DWITH_RUST_EXAMPLE
. -
hiredis
, if you want to build with-DUSE_REDIS
, so that you use Redis instead of etcd as metadata servers. -
curl
, if you want to build with-DUSE_HTTP
, so that you use HTTP instead of etcd as metadata servers.
-
Init source code
git clone https://github.com/kvcache-ai/Mooncake.git cd Mooncake
-
Install dependencies
bash dependencies.sh
-
Compile Mooncake and examples
mkdir build cd build cmake .. # (optional) Specify build options like -D make -j
Mooncake also supports Docker-based deployment, see Build Guide in detail.
- [x] First release of Mooncake and integrate with latest vLLM
- [ ] Share KV caches across multiple serving engines
- [ ] User and developer documentation
{
"timestamp": 27482,
"input_length": 6955,
"output_length": 52,
"hash_ids": [46, 47, 48, 49, 50, 51, 52, 53, 54, 55, 56, 57, 2353, 2354]
}
{
"timestamp": 30535,
"input_length": 6472,
"output_length": 26,
"hash_ids": [46, 47, 48, 49, 50, 51, 52, 53, 54, 55, 56, 57, 2366]
}
The above presents two samples from our trace dataset. The trace includes the timing of request arrivals, the number of input tokens, the number of output tokens, and the remapped block hash. To protect our customers' privacy, we applied several mechanisms to remove user-related information while preserving the dataset's utility for simulated evaluation. More descriptions of the trace (e.g., up to 50% cache hit ratio) can be found in Section 4 of the technical report.
Update[Feb 21, 2025]: The updated traces used in our FAST'25 paper have been released! Please refer to the paper's appendix (found here) for more details.
Please kindly cite our paper if you find the paper or the traces are useful:@article{qin2024mooncake,
title = {Mooncake: A KVCache-centric Disaggregated Architecture for LLM Serving},
author = {Ruoyu Qin, Zheming Li, Weiran He, Mingxing Zhang, Yongwei Wu, Weimin Zheng, and Xinran Xu},
year = {2024},
url = {https://arxiv.org/abs/2407.00079}
}
@inproceedings {qin2025mooncake,
author = {Ruoyu Qin and Zheming Li and Weiran He and Jialei Cui and Feng Ren and Mingxing Zhang and Yongwei Wu and Weimin Zheng and Xinran Xu},
title = {Mooncake: Trading More Storage for Less Computation {\textemdash} A {KVCache-centric} Architecture for Serving {LLM} Chatbot},
booktitle = {23rd USENIX Conference on File and Storage Technologies (FAST 25)},
year = {2025},
isbn = {978-1-939133-45-8},
address = {Santa Clara, CA},
pages = {155--170},
url = {https://www.usenix.org/conference/fast25/presentation/qin},
publisher = {USENIX Association},
month = feb
}
For Tasks:
Click tags to check more tools for each tasksFor Jobs:
Alternative AI tools for Mooncake
Similar Open Source Tools
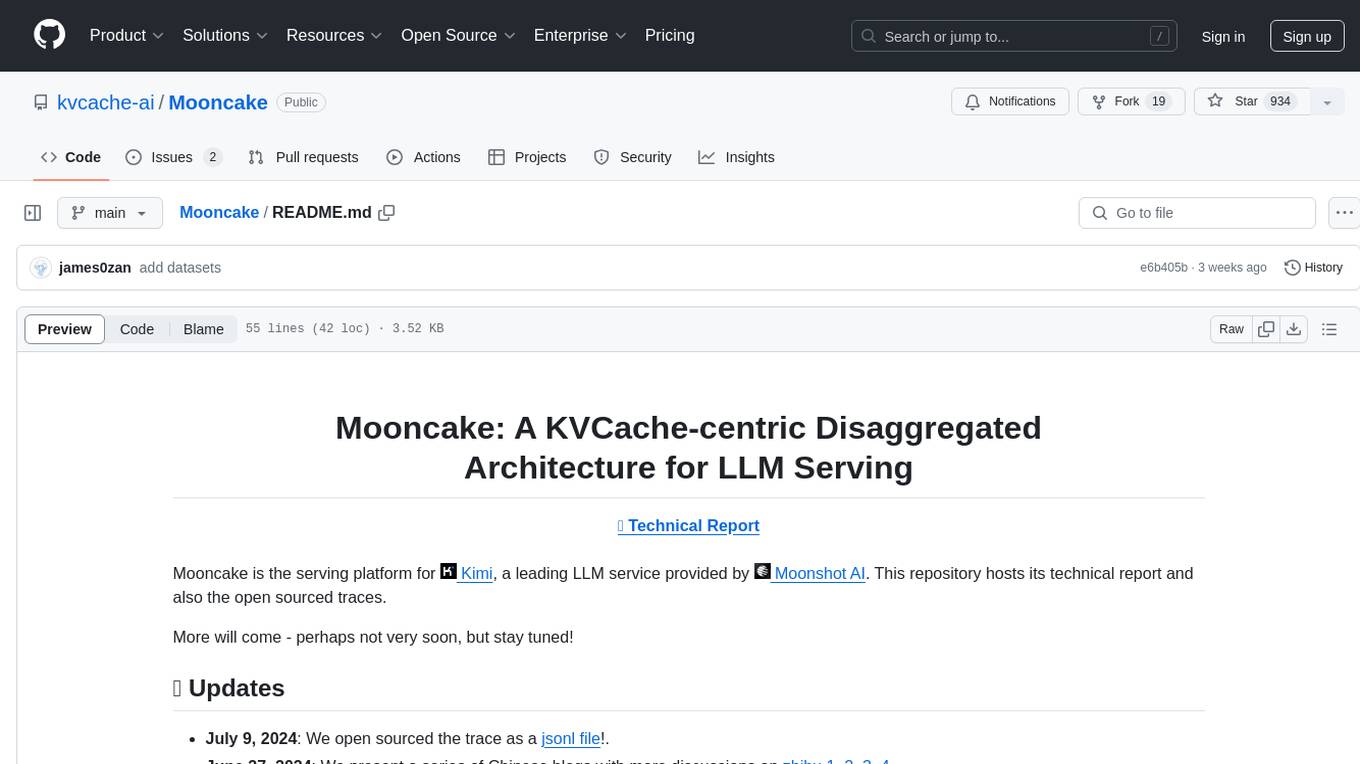
Mooncake
Mooncake is a serving platform for Kimi, a leading LLM service provided by Moonshot AI. It features a KVCache-centric disaggregated architecture that separates prefill and decoding clusters, leveraging underutilized CPU, DRAM, and SSD resources of the GPU cluster. Mooncake's scheduler balances throughput and latency-related SLOs, with a prediction-based early rejection policy for highly overloaded scenarios. It excels in long-context scenarios, achieving up to a 525% increase in throughput while handling 75% more requests under real workloads.
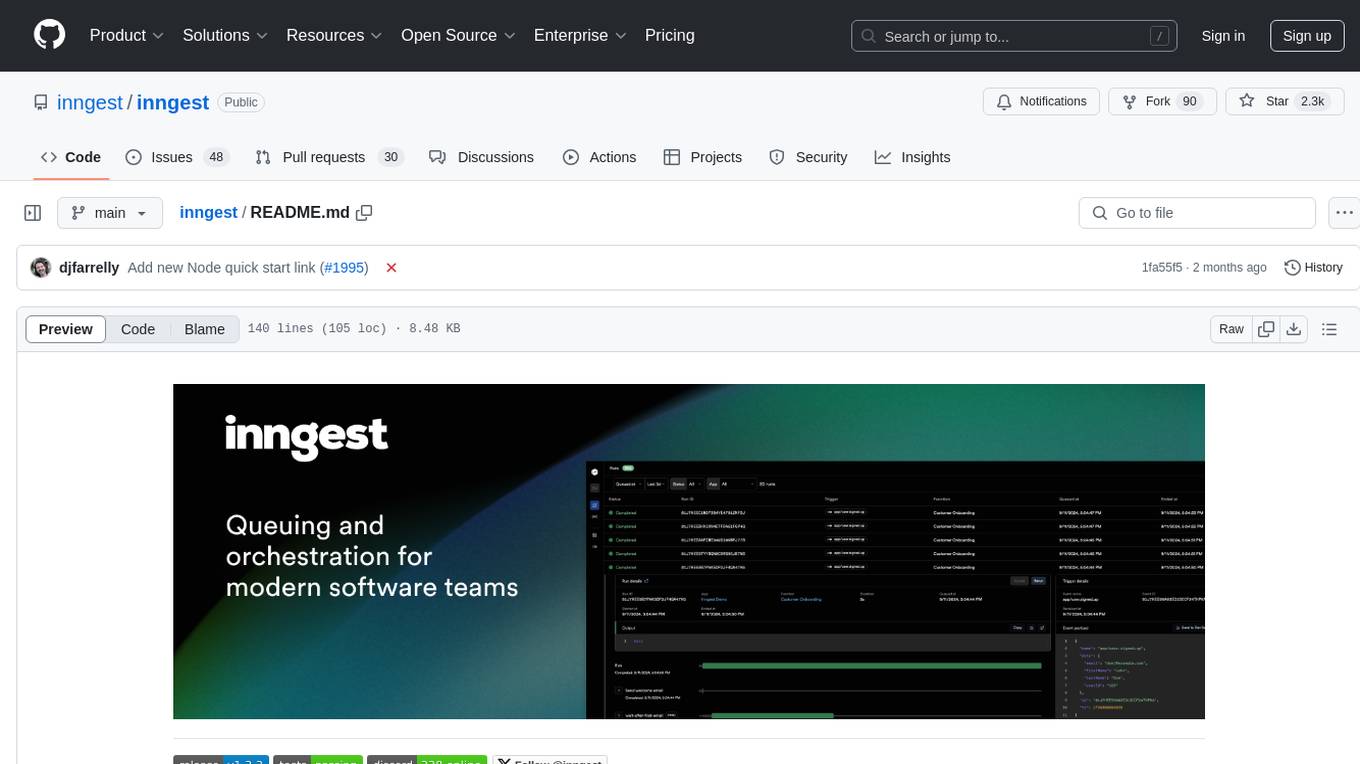
inngest
Inngest is a platform that offers durable functions to replace queues, state management, and scheduling for developers. It allows writing reliable step functions faster without dealing with infrastructure. Developers can create durable functions using various language SDKs, run a local development server, deploy functions to their infrastructure, sync functions with the Inngest Platform, and securely trigger functions via HTTPS. Inngest Functions support retrying, scheduling, and coordinating operations through triggers, flow control, and steps, enabling developers to build reliable workflows with robust support for various operations.
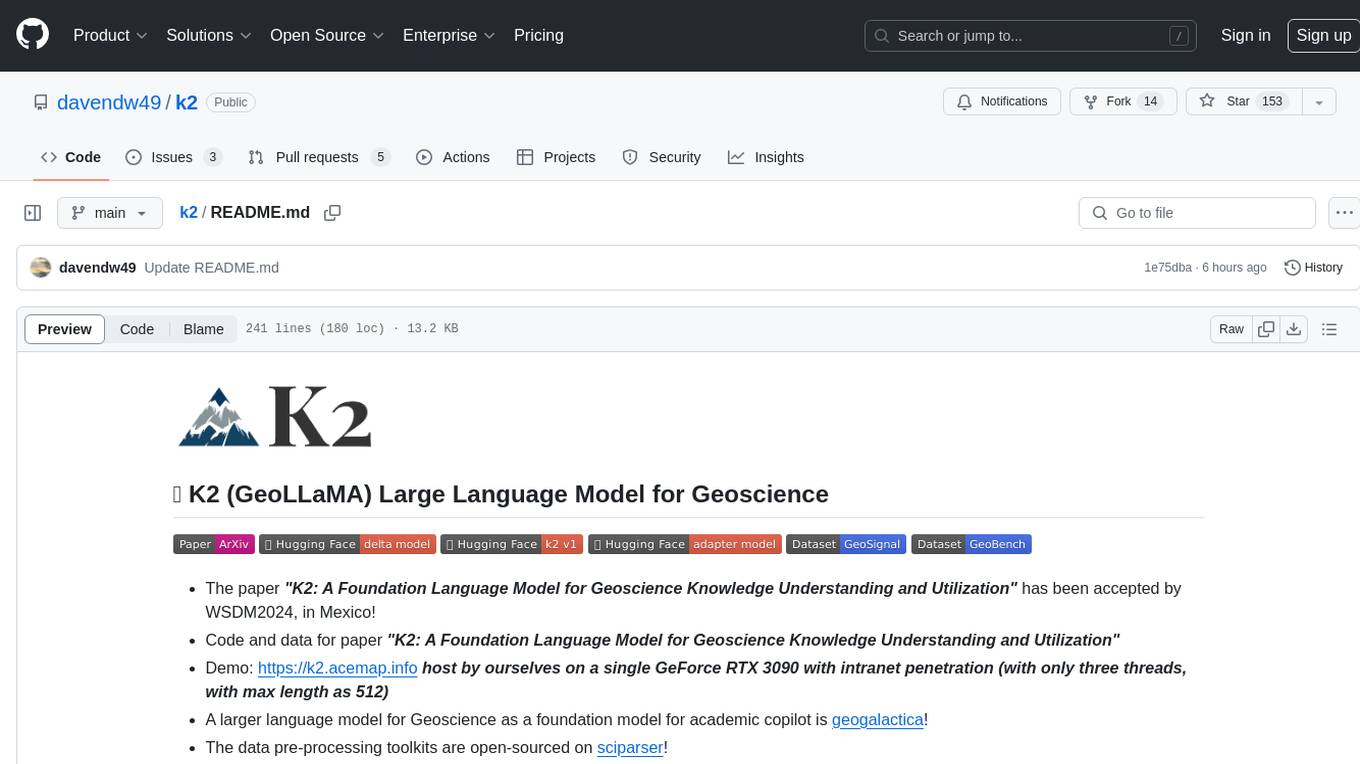
k2
K2 (GeoLLaMA) is a large language model for geoscience, trained on geoscience literature and fine-tuned with knowledge-intensive instruction data. It outperforms baseline models on objective and subjective tasks. The repository provides K2 weights, core data of GeoSignal, GeoBench benchmark, and code for further pretraining and instruction tuning. The model is available on Hugging Face for use. The project aims to create larger and more powerful geoscience language models in the future.
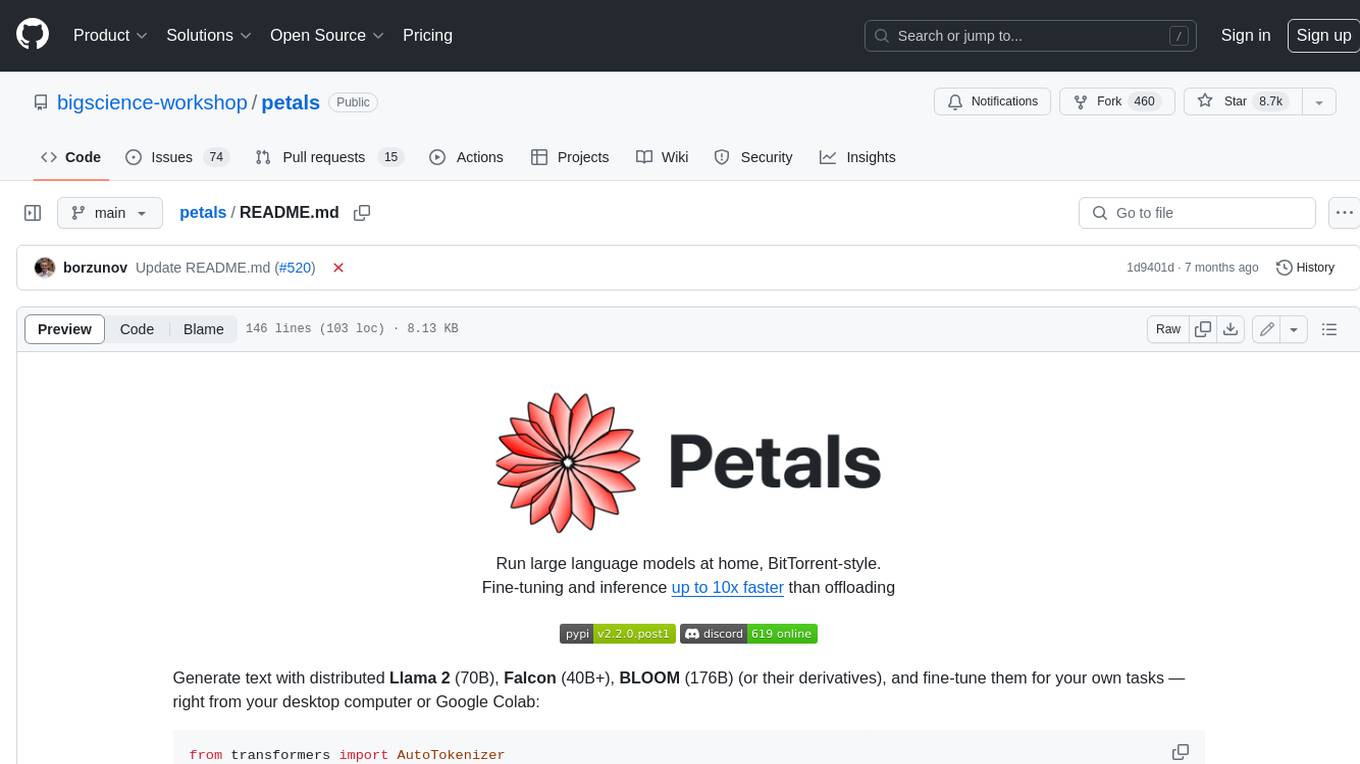
petals
Petals is a tool that allows users to run large language models at home in a BitTorrent-style manner. It enables fine-tuning and inference up to 10x faster than offloading. Users can generate text with distributed models like Llama 2, Falcon, and BLOOM, and fine-tune them for specific tasks directly from their desktop computer or Google Colab. Petals is a community-run system that relies on people sharing their GPUs to increase its capacity and offer a distributed network for hosting model layers.
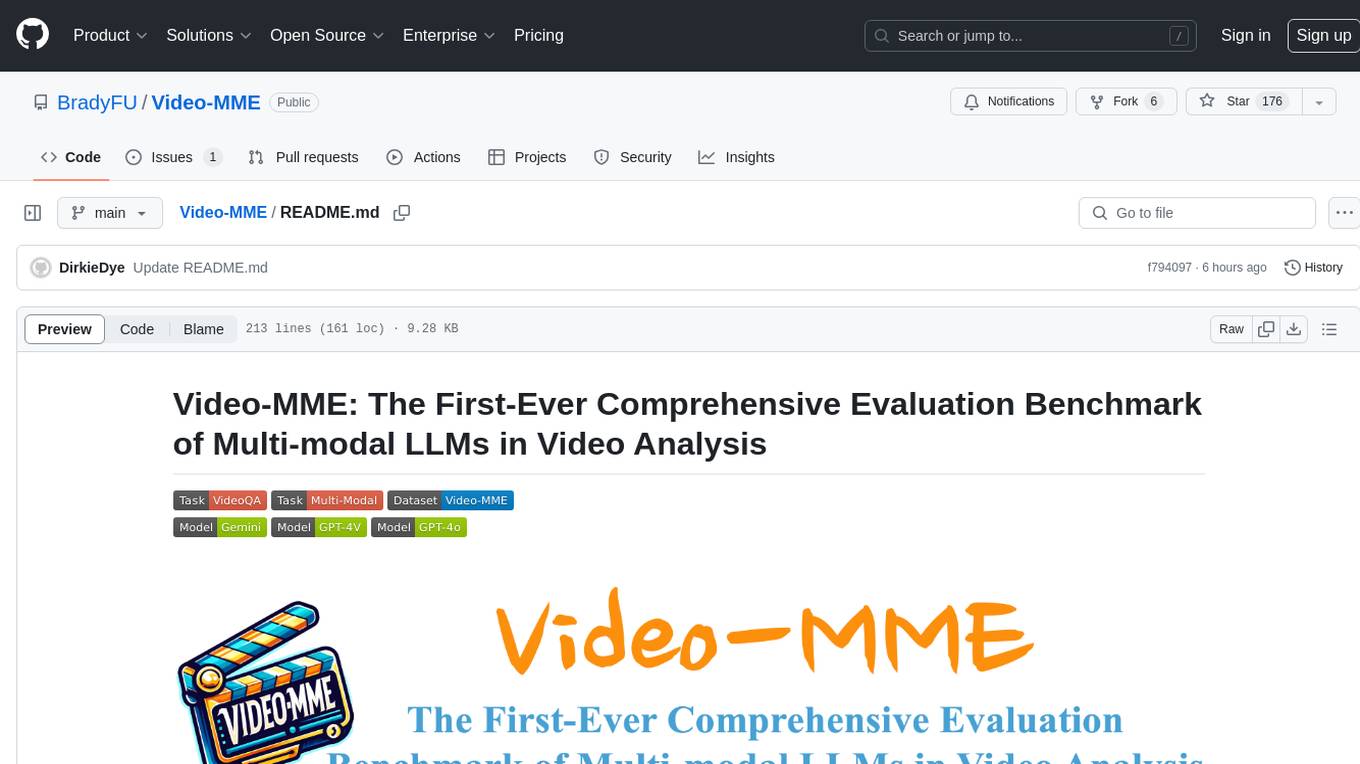
Video-MME
Video-MME is the first-ever comprehensive evaluation benchmark of Multi-modal Large Language Models (MLLMs) in Video Analysis. It assesses the capabilities of MLLMs in processing video data, covering a wide range of visual domains, temporal durations, and data modalities. The dataset comprises 900 videos with 256 hours and 2,700 human-annotated question-answer pairs. It distinguishes itself through features like duration variety, diversity in video types, breadth in data modalities, and quality in annotations.
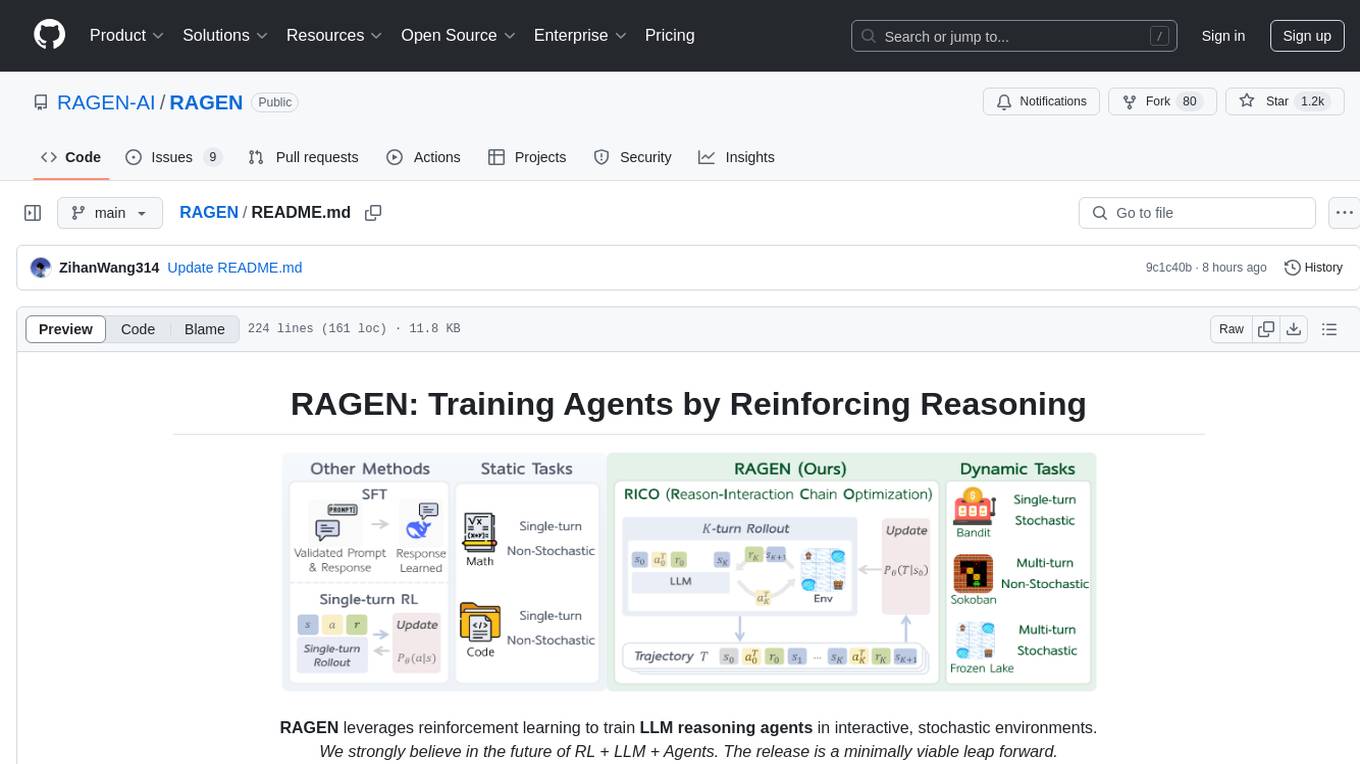
RAGEN
RAGEN is a reinforcement learning framework designed to train reasoning-capable large language model (LLM) agents in interactive, stochastic environments. It addresses challenges such as multi-turn interactions and stochastic environments through a Markov Decision Process (MDP) formulation, Reason-Interaction Chain Optimization (RICO) algorithm, and progressive reward normalization strategies. The framework consists of MDP formulation, RICO algorithm with rollout and update stages, and reward normalization strategies to stabilize training. RAGEN aims to optimize reasoning and action strategies for LLM agents operating in complex environments.
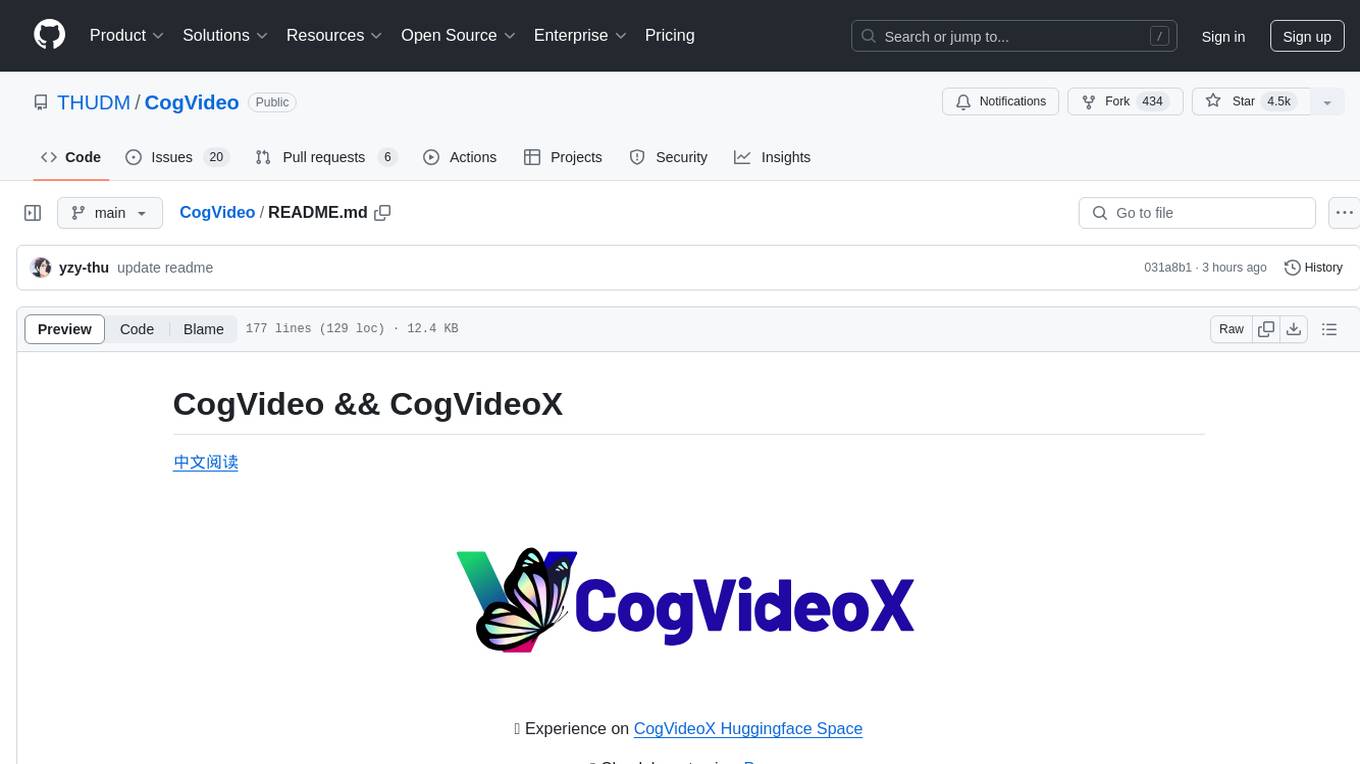
CogVideo
CogVideo is an open-source repository that provides pretrained text-to-video models for generating videos based on input text. It includes models like CogVideoX-2B and CogVideo, offering powerful video generation capabilities. The repository offers tools for inference, fine-tuning, and model conversion, along with demos showcasing the model's capabilities through CLI, web UI, and online experiences. CogVideo aims to facilitate the creation of high-quality videos from textual descriptions, catering to a wide range of applications.
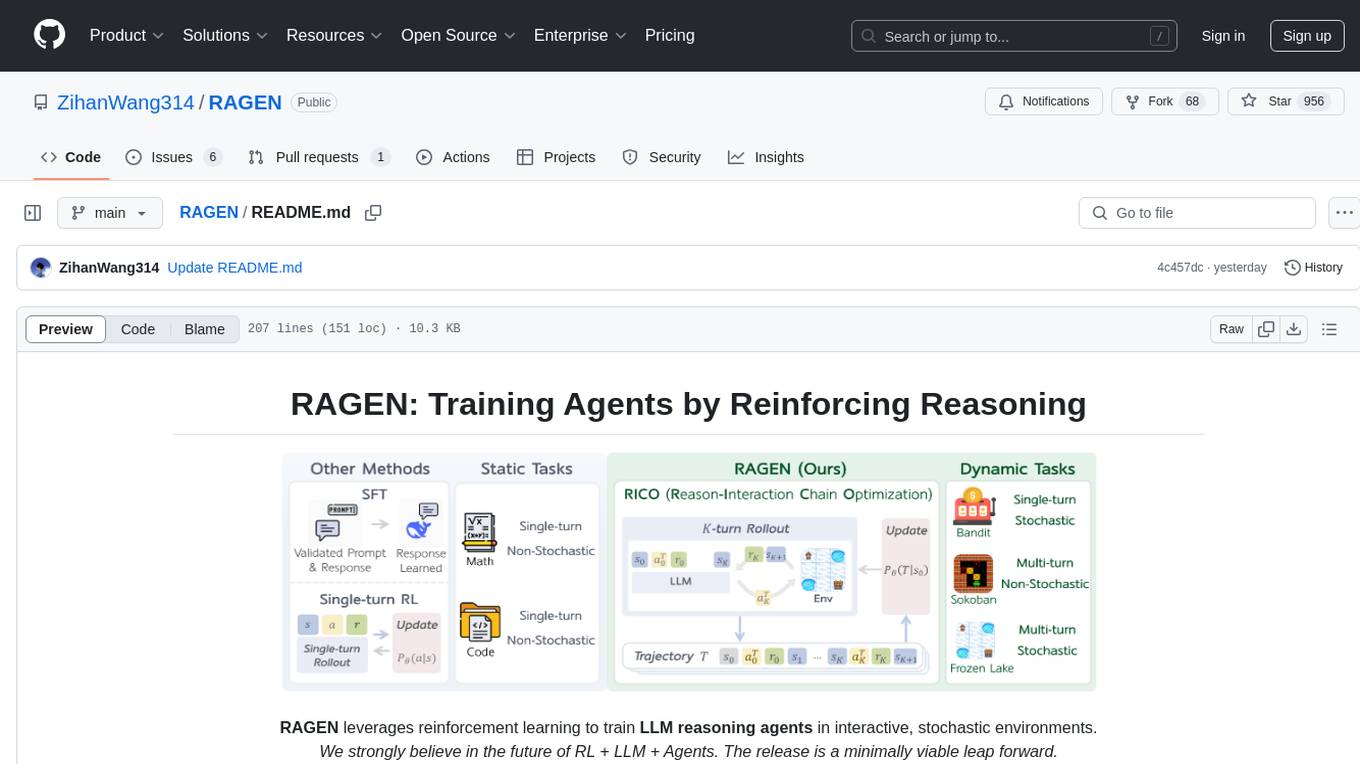
RAGEN
RAGEN is a reinforcement learning framework designed to train reasoning-capable large language model (LLM) agents in interactive, stochastic environments. It addresses challenges such as multi-turn interactions and stochastic environments through a Markov Decision Process (MDP) formulation, Reason-Interaction Chain Optimization (RICO) algorithm, and progressive reward normalization strategies. The framework enables LLMs to reason and interact with the environment, optimizing entire trajectories for long-horizon reasoning while maintaining computational efficiency.
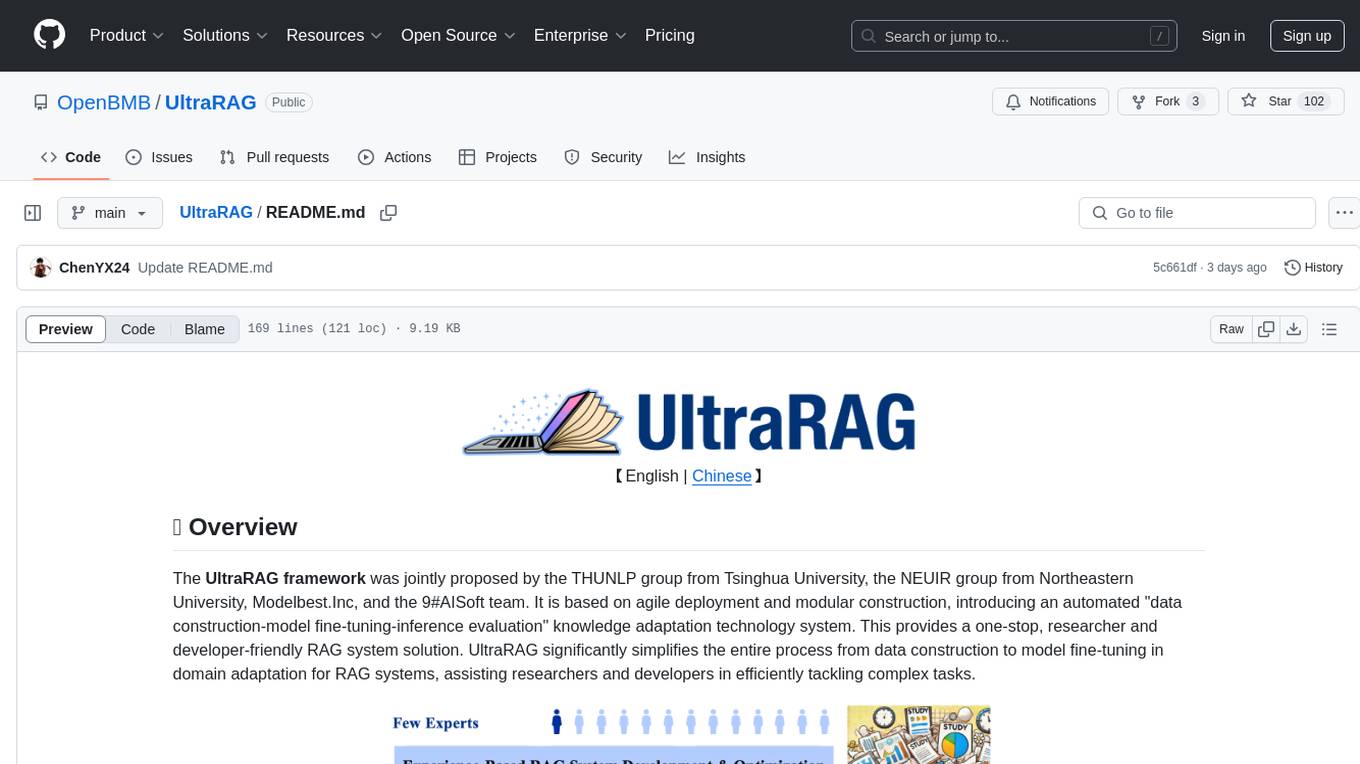
UltraRAG
The UltraRAG framework is a researcher and developer-friendly RAG system solution that simplifies the process from data construction to model fine-tuning in domain adaptation. It introduces an automated knowledge adaptation technology system, supporting no-code programming, one-click synthesis and fine-tuning, multidimensional evaluation, and research-friendly exploration work integration. The architecture consists of Frontend, Service, and Backend components, offering flexibility in customization and optimization. Performance evaluation in the legal field shows improved results compared to VanillaRAG, with specific metrics provided. The repository is licensed under Apache-2.0 and encourages citation for support.
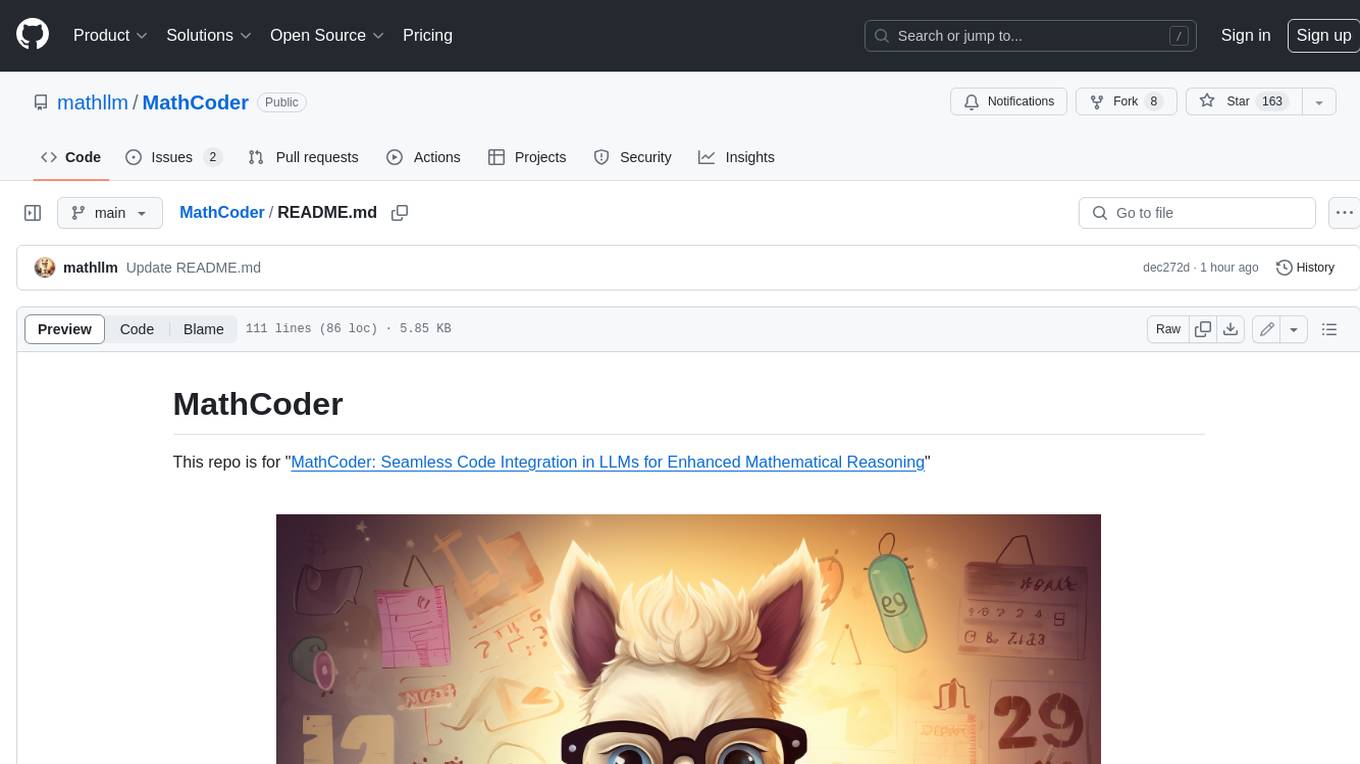
MathCoder
MathCoder is a repository focused on enhancing mathematical reasoning by fine-tuning open-source language models to use code for modeling and deriving math equations. It introduces MathCodeInstruct dataset with solutions interleaving natural language, code, and execution results. The repository provides MathCoder models capable of generating code-based solutions for challenging math problems, achieving state-of-the-art scores on MATH and GSM8K datasets. It offers tools for model deployment, inference, and evaluation, along with a citation for referencing the work.
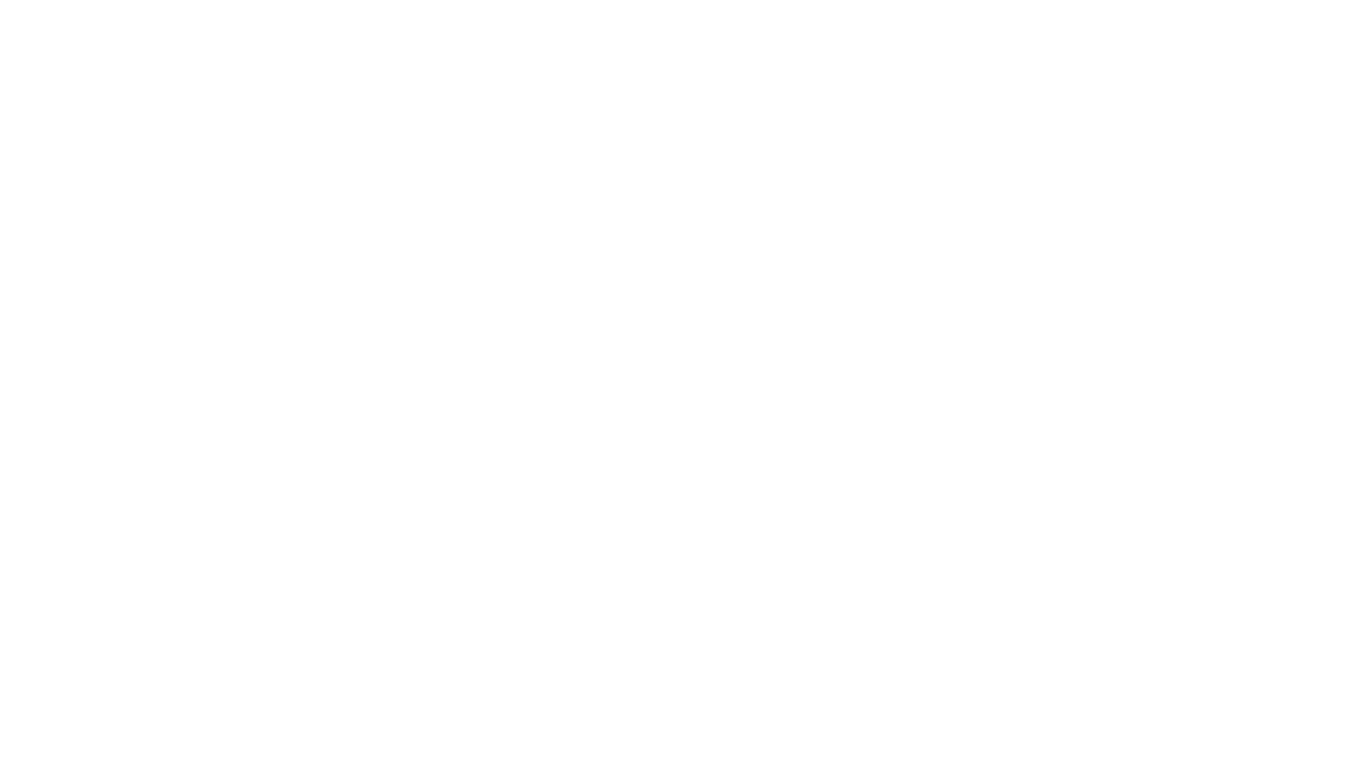
LinguaHaru
Next-generation AI translation tool that provides high-quality, precise translations for various common file formats with a single click. It is based on cutting-edge large language models, offering exceptional translation quality with minimal operation, supporting multiple document formats and languages. Features include multi-format compatibility, global language translation, one-click rapid translation, flexible translation engines, and LAN sharing for efficient collaborative work.
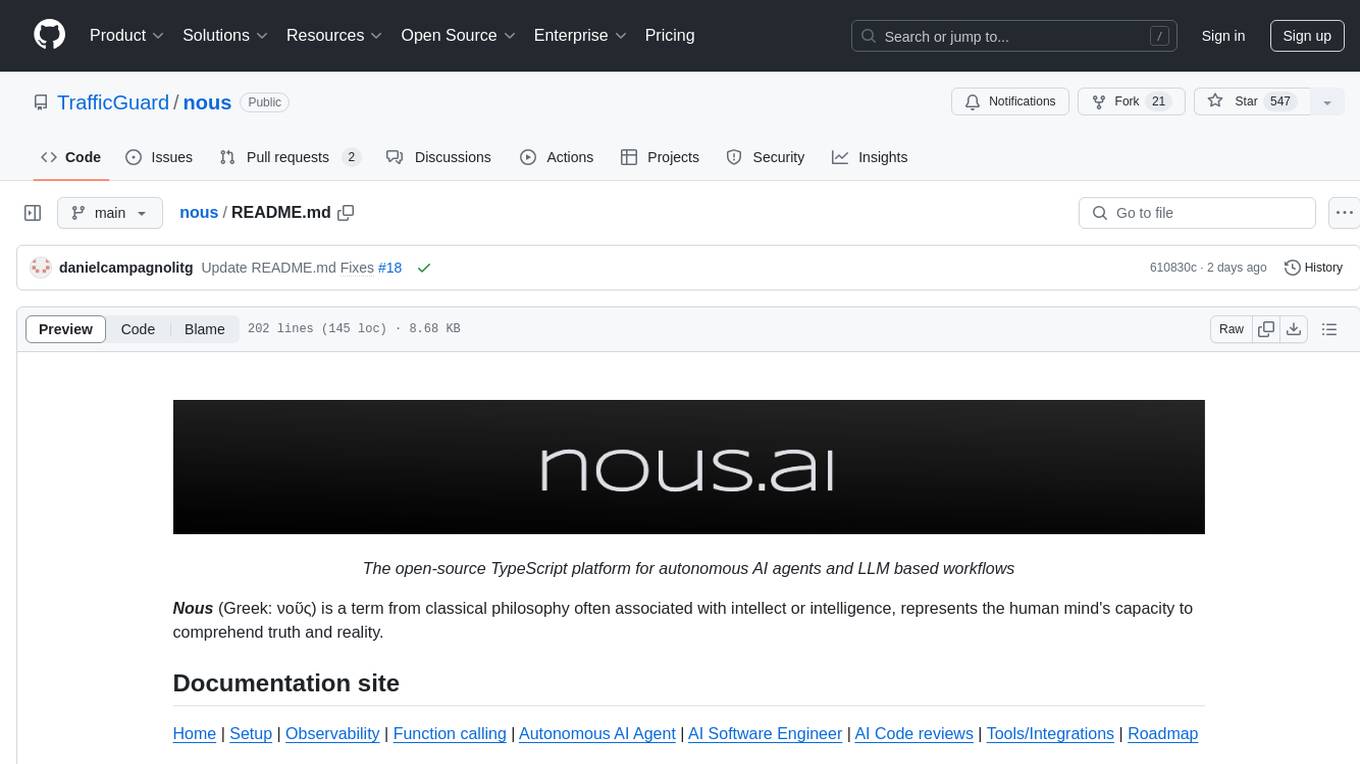
nous
Nous is an open-source TypeScript platform for autonomous AI agents and LLM based workflows. It aims to automate processes, support requests, review code, assist with refactorings, and more. The platform supports various integrations, multiple LLMs/services, CLI and web interface, human-in-the-loop interactions, flexible deployment options, observability with OpenTelemetry tracing, and specific agents for code editing, software engineering, and code review. It offers advanced features like reasoning/planning, memory and function call history, hierarchical task decomposition, and control-loop function calling options. Nous is designed to be a flexible platform for the TypeScript community to expand and support different use cases and integrations.
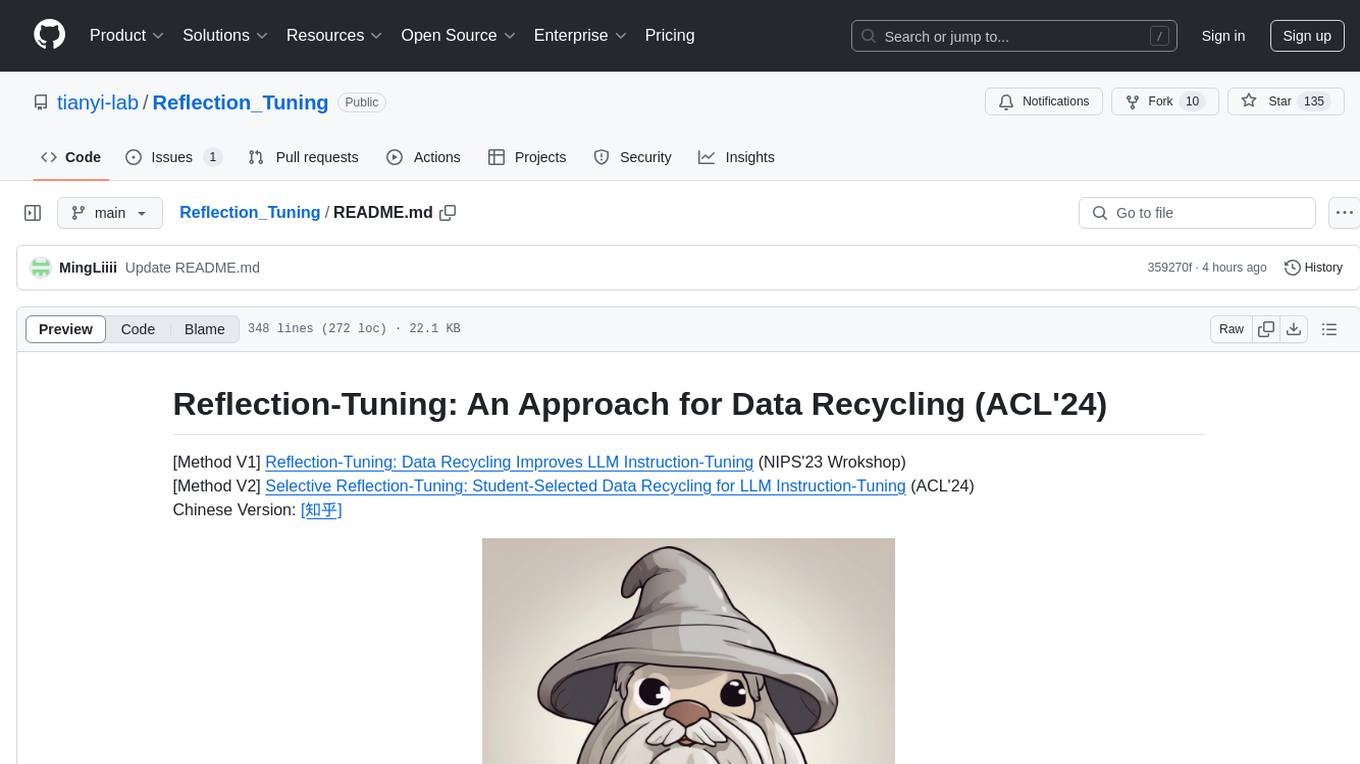
Reflection_Tuning
Reflection-Tuning is a project focused on improving the quality of instruction-tuning data through a reflection-based method. It introduces Selective Reflection-Tuning, where the student model can decide whether to accept the improvements made by the teacher model. The project aims to generate high-quality instruction-response pairs by defining specific criteria for the oracle model to follow and respond to. It also evaluates the efficacy and relevance of instruction-response pairs using the r-IFD metric. The project provides code for reflection and selection processes, along with data and model weights for both V1 and V2 methods.
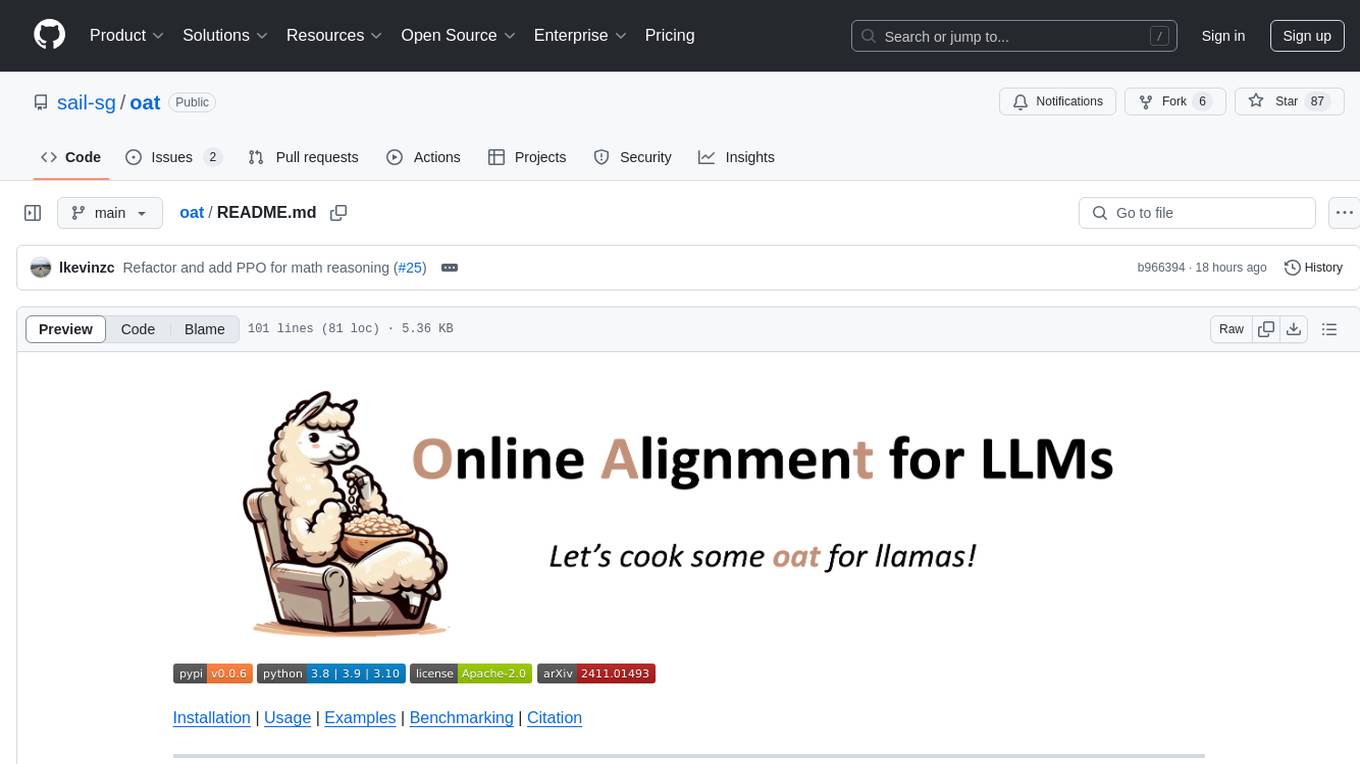
oat
Oat is a simple and efficient framework for running online LLM alignment algorithms. It implements a distributed Actor-Learner-Oracle architecture, with components optimized using state-of-the-art tools. Oat simplifies the experimental pipeline of LLM alignment by serving an Oracle online for preference data labeling and model evaluation. It provides a variety of oracles for simulating feedback and supports verifiable rewards. Oat's modular structure allows for easy inheritance and modification of classes, enabling rapid prototyping and experimentation with new algorithms. The framework implements cutting-edge online algorithms like PPO for math reasoning and various online exploration algorithms.
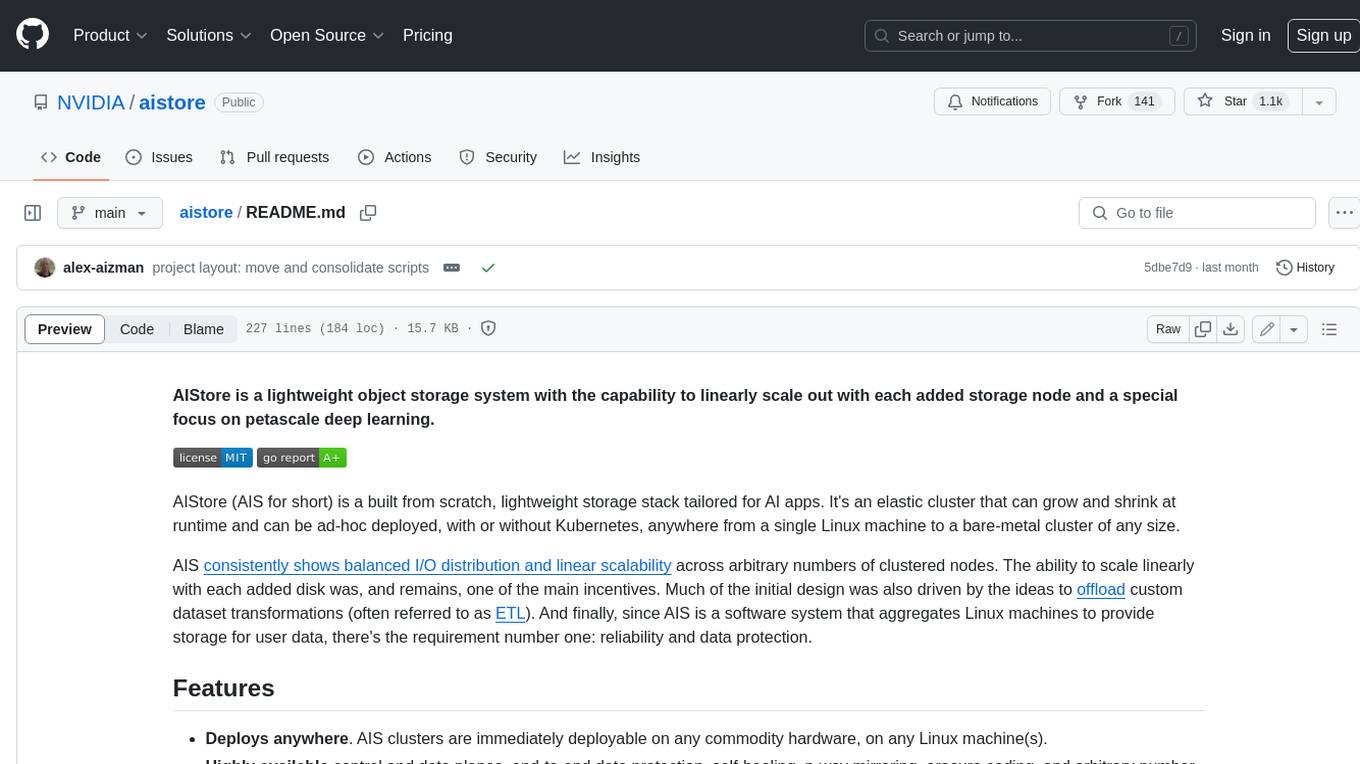
aistore
AIStore is a lightweight object storage system designed for AI applications. It is highly scalable, reliable, and easy to use. AIStore can be deployed on any commodity hardware, and it can be used to store and manage large datasets for deep learning and other AI applications.
For similar tasks
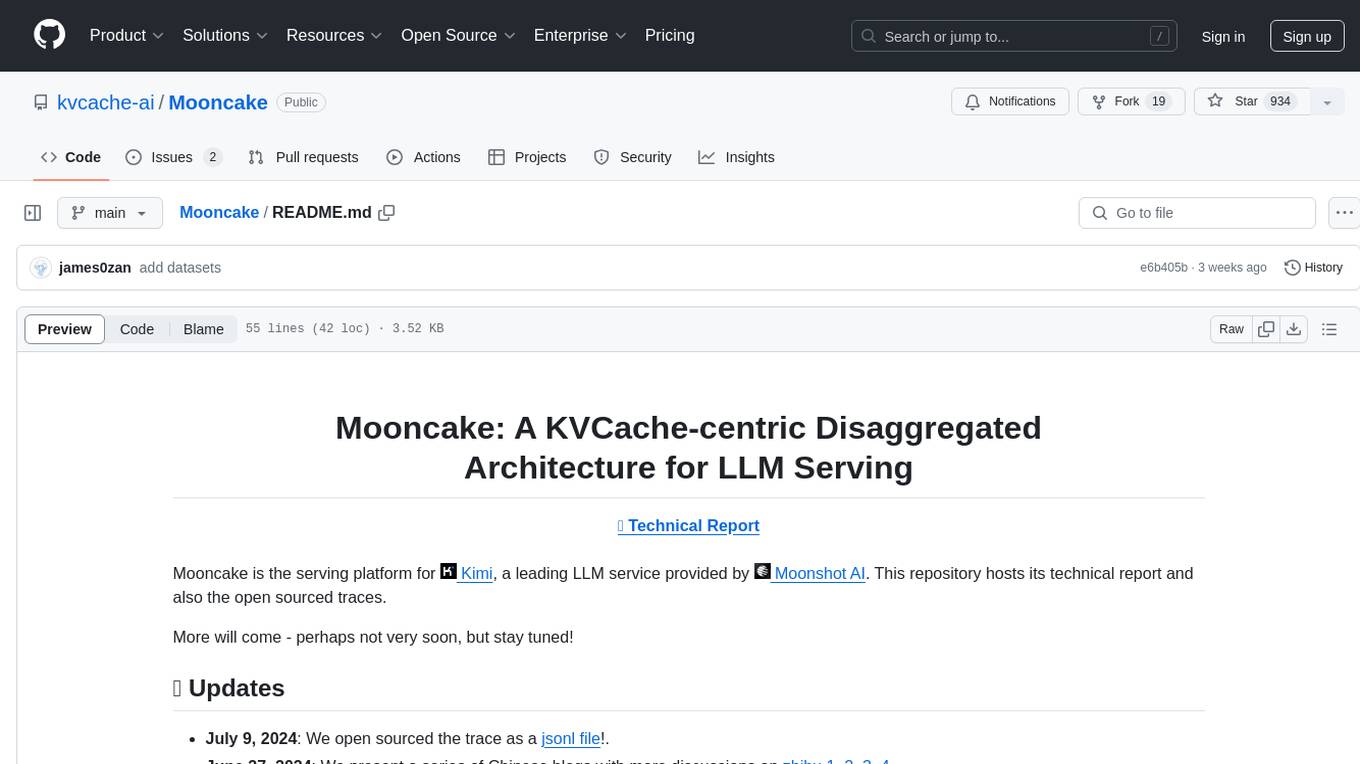
Mooncake
Mooncake is a serving platform for Kimi, a leading LLM service provided by Moonshot AI. It features a KVCache-centric disaggregated architecture that separates prefill and decoding clusters, leveraging underutilized CPU, DRAM, and SSD resources of the GPU cluster. Mooncake's scheduler balances throughput and latency-related SLOs, with a prediction-based early rejection policy for highly overloaded scenarios. It excels in long-context scenarios, achieving up to a 525% increase in throughput while handling 75% more requests under real workloads.
For similar jobs
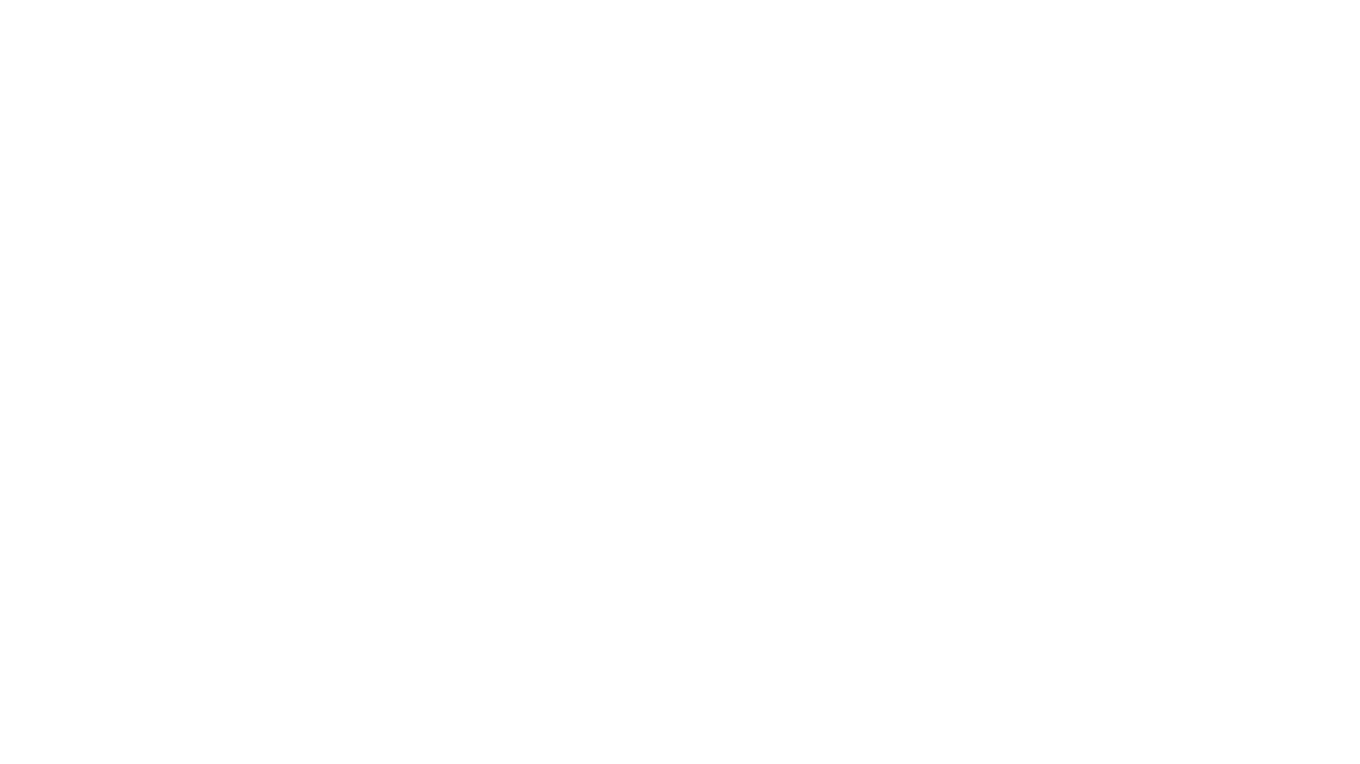
AirGo
AirGo is a front and rear end separation, multi user, multi protocol proxy service management system, simple and easy to use. It supports vless, vmess, shadowsocks, and hysteria2.
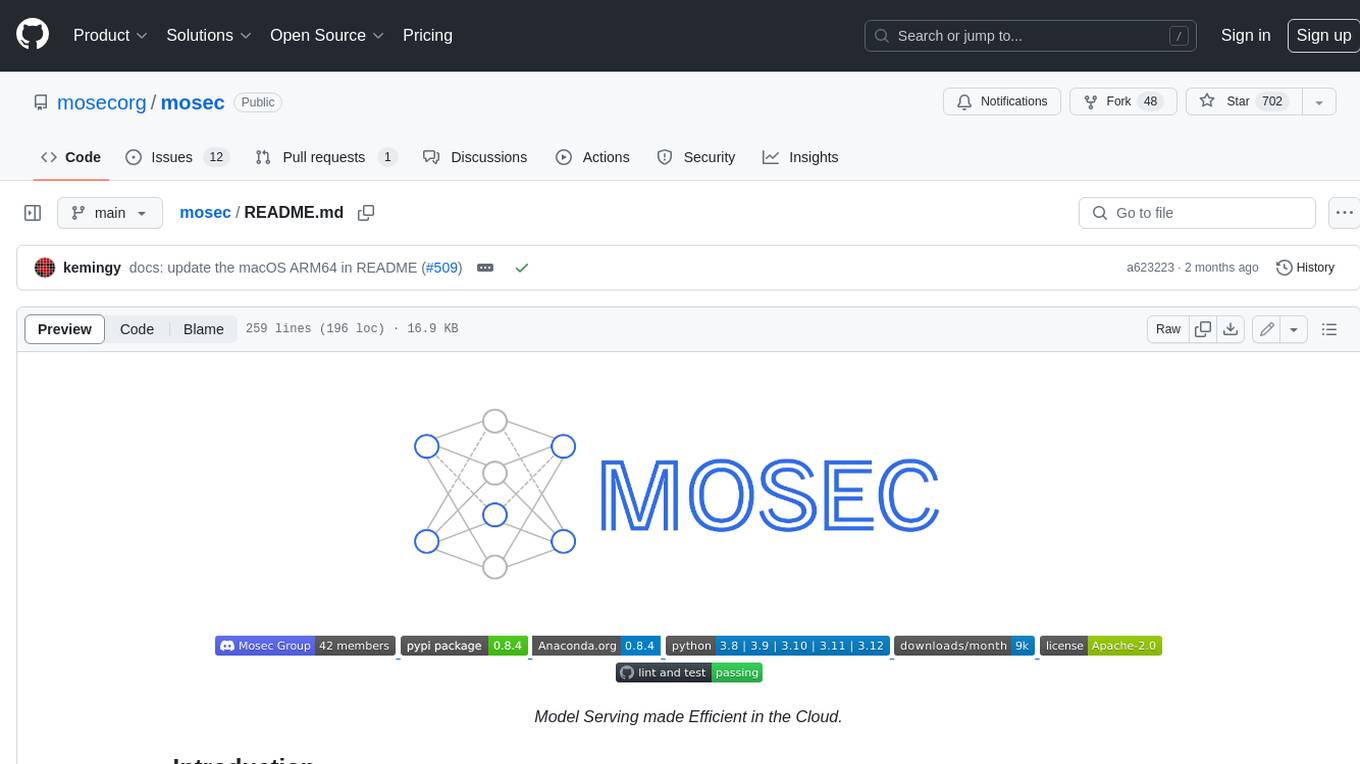
mosec
Mosec is a high-performance and flexible model serving framework for building ML model-enabled backend and microservices. It bridges the gap between any machine learning models you just trained and the efficient online service API. * **Highly performant** : web layer and task coordination built with Rust π¦, which offers blazing speed in addition to efficient CPU utilization powered by async I/O * **Ease of use** : user interface purely in Python π, by which users can serve their models in an ML framework-agnostic manner using the same code as they do for offline testing * **Dynamic batching** : aggregate requests from different users for batched inference and distribute results back * **Pipelined stages** : spawn multiple processes for pipelined stages to handle CPU/GPU/IO mixed workloads * **Cloud friendly** : designed to run in the cloud, with the model warmup, graceful shutdown, and Prometheus monitoring metrics, easily managed by Kubernetes or any container orchestration systems * **Do one thing well** : focus on the online serving part, users can pay attention to the model optimization and business logic
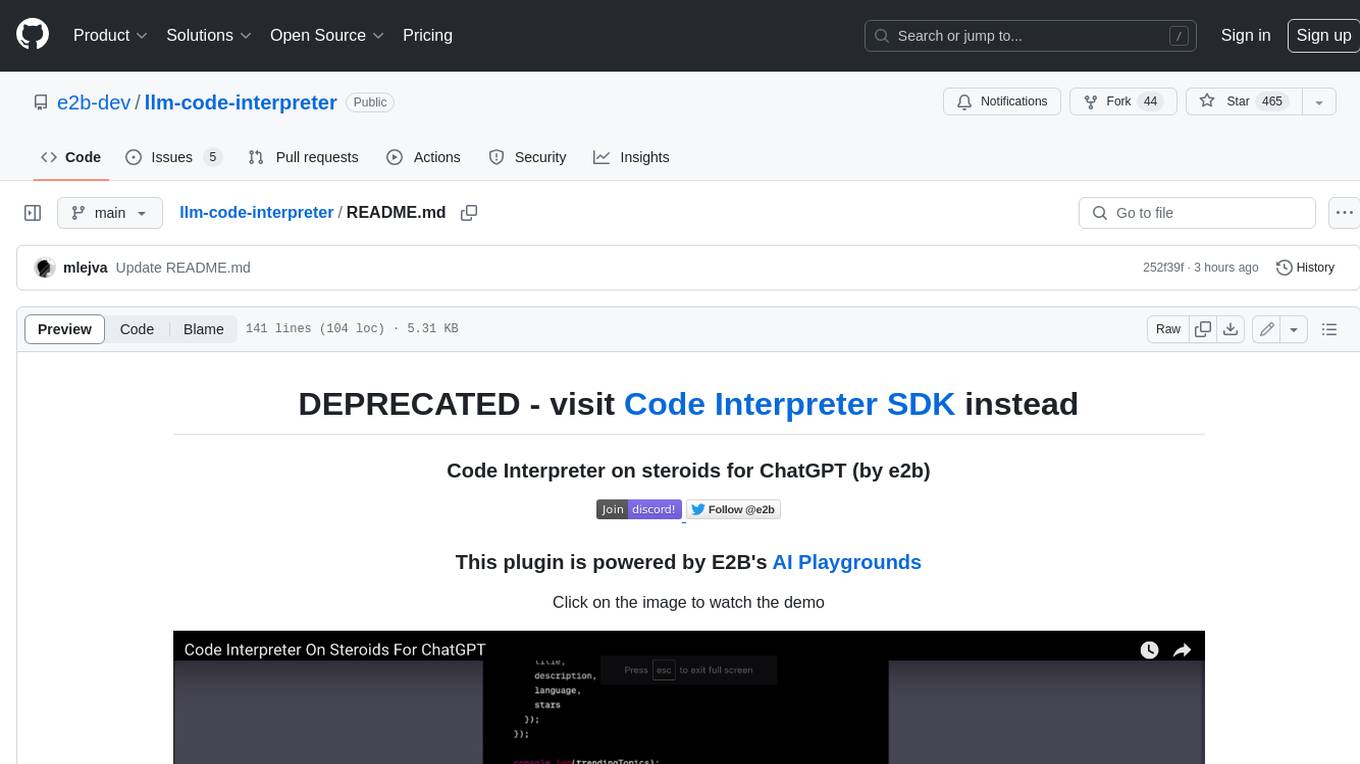
llm-code-interpreter
The 'llm-code-interpreter' repository is a deprecated plugin that provides a code interpreter on steroids for ChatGPT by E2B. It gives ChatGPT access to a sandboxed cloud environment with capabilities like running any code, accessing Linux OS, installing programs, using filesystem, running processes, and accessing the internet. The plugin exposes commands to run shell commands, read files, and write files, enabling various possibilities such as running different languages, installing programs, starting servers, deploying websites, and more. It is powered by the E2B API and is designed for agents to freely experiment within a sandboxed environment.
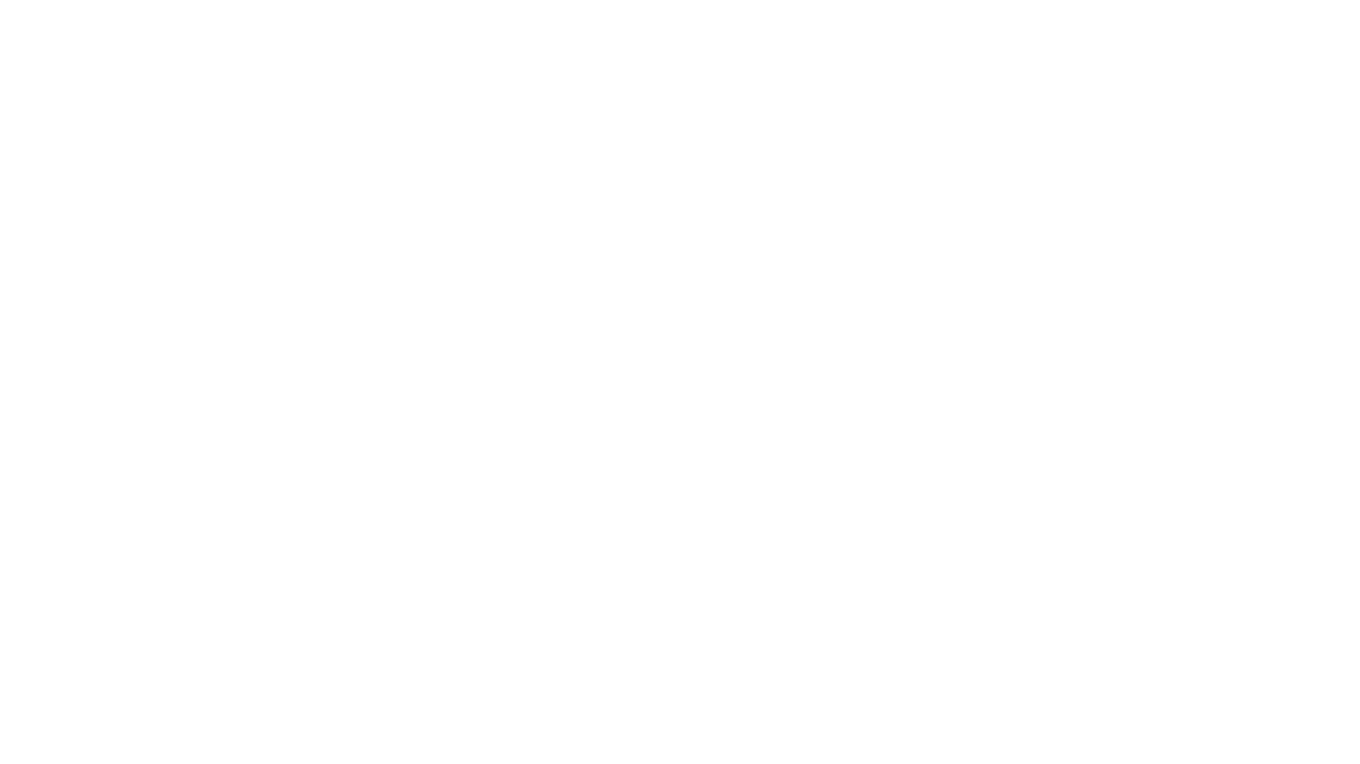
pezzo
Pezzo is a fully cloud-native and open-source LLMOps platform that allows users to observe and monitor AI operations, troubleshoot issues, save costs and latency, collaborate, manage prompts, and deliver AI changes instantly. It supports various clients for prompt management, observability, and caching. Users can run the full Pezzo stack locally using Docker Compose, with prerequisites including Node.js 18+, Docker, and a GraphQL Language Feature Support VSCode Extension. Contributions are welcome, and the source code is available under the Apache 2.0 License.
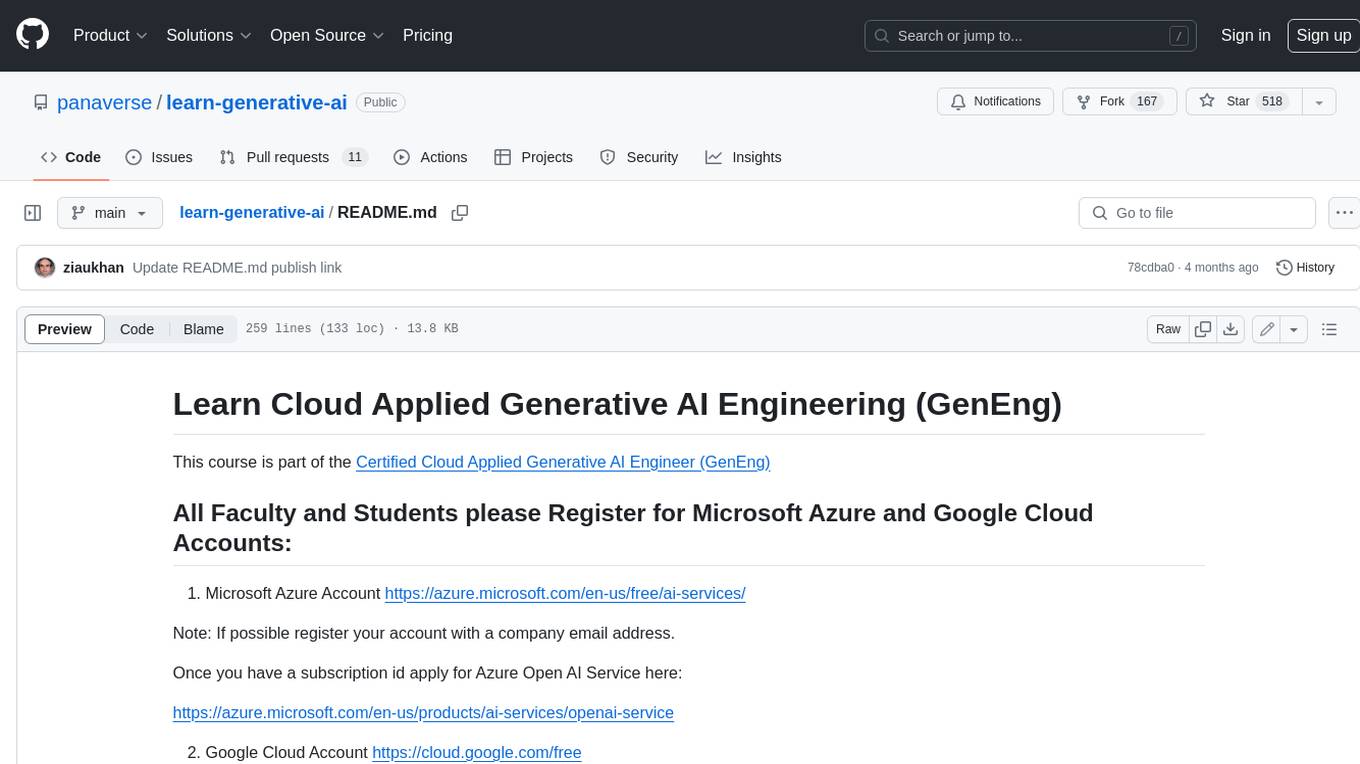
learn-generative-ai
Learn Cloud Applied Generative AI Engineering (GenEng) is a course focusing on the application of generative AI technologies in various industries. The course covers topics such as the economic impact of generative AI, the role of developers in adopting and integrating generative AI technologies, and the future trends in generative AI. Students will learn about tools like OpenAI API, LangChain, and Pinecone, and how to build and deploy Large Language Models (LLMs) for different applications. The course also explores the convergence of generative AI with Web 3.0 and its potential implications for decentralized intelligence.
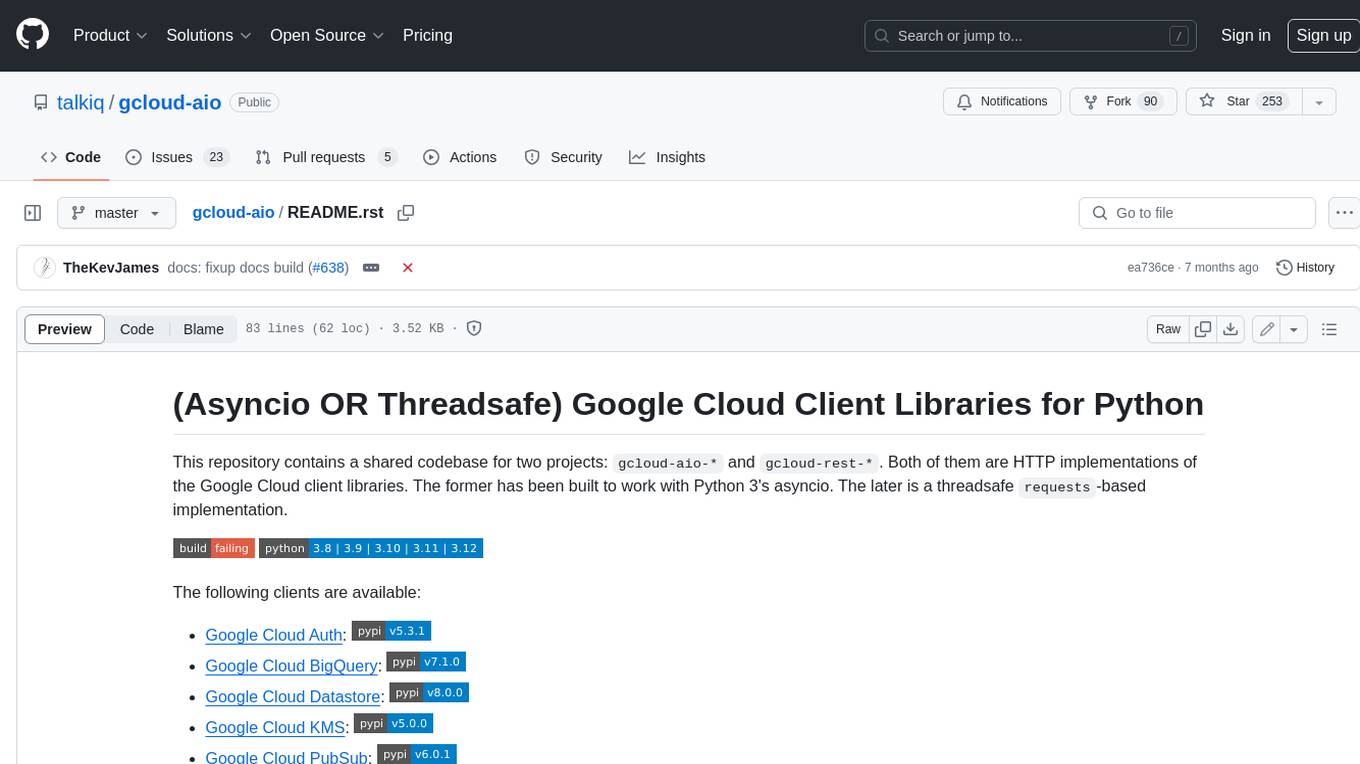
gcloud-aio
This repository contains shared codebase for two projects: gcloud-aio and gcloud-rest. gcloud-aio is built for Python 3's asyncio, while gcloud-rest is a threadsafe requests-based implementation. It provides clients for Google Cloud services like Auth, BigQuery, Datastore, KMS, PubSub, Storage, and Task Queue. Users can install the library using pip and refer to the documentation for usage details. Developers can contribute to the project by following the contribution guide.
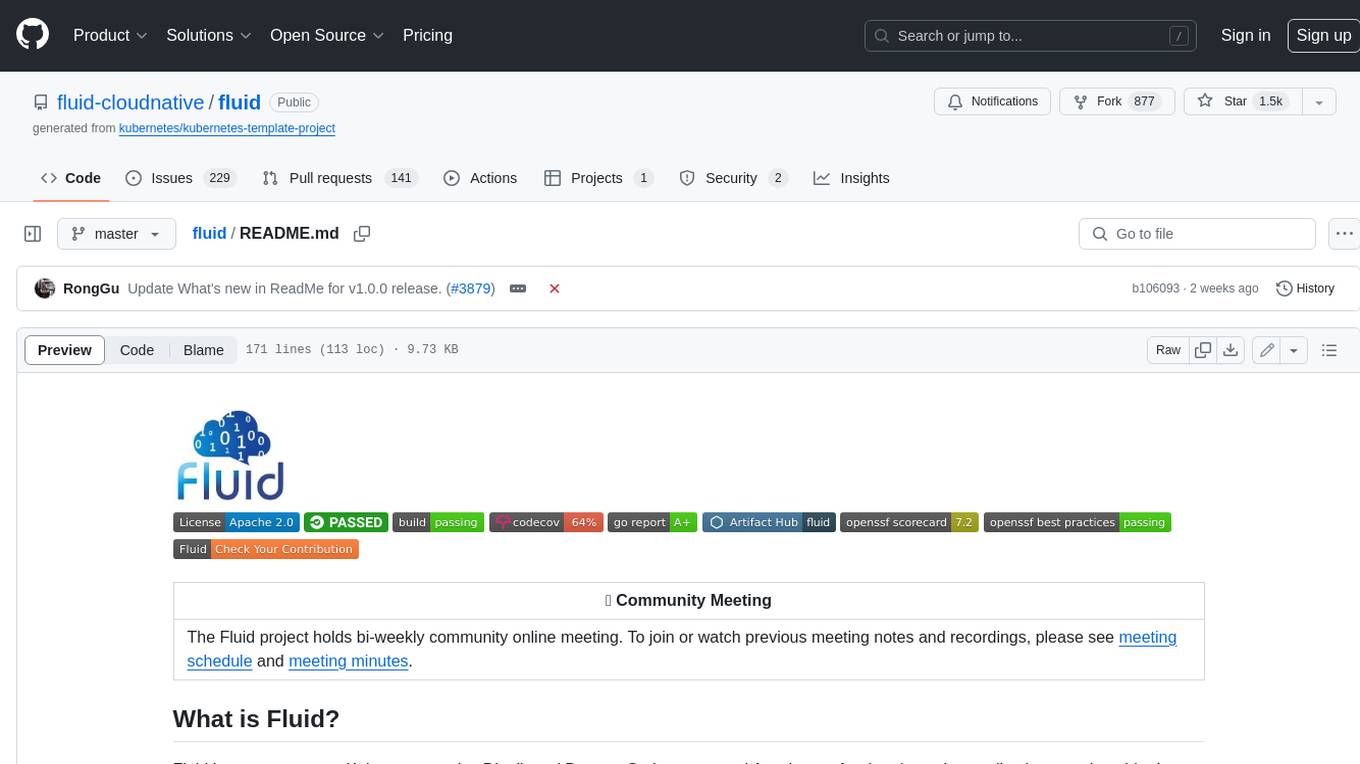
fluid
Fluid is an open source Kubernetes-native Distributed Dataset Orchestrator and Accelerator for data-intensive applications, such as big data and AI applications. It implements dataset abstraction, scalable cache runtime, automated data operations, elasticity and scheduling, and is runtime platform agnostic. Key concepts include Dataset and Runtime. Prerequisites include Kubernetes version > 1.16, Golang 1.18+, and Helm 3. The tool offers features like accelerating remote file accessing, machine learning, accelerating PVC, preloading dataset, and on-the-fly dataset cache scaling. Contributions are welcomed, and the project is under the Apache 2.0 license with a vendor-neutral approach.
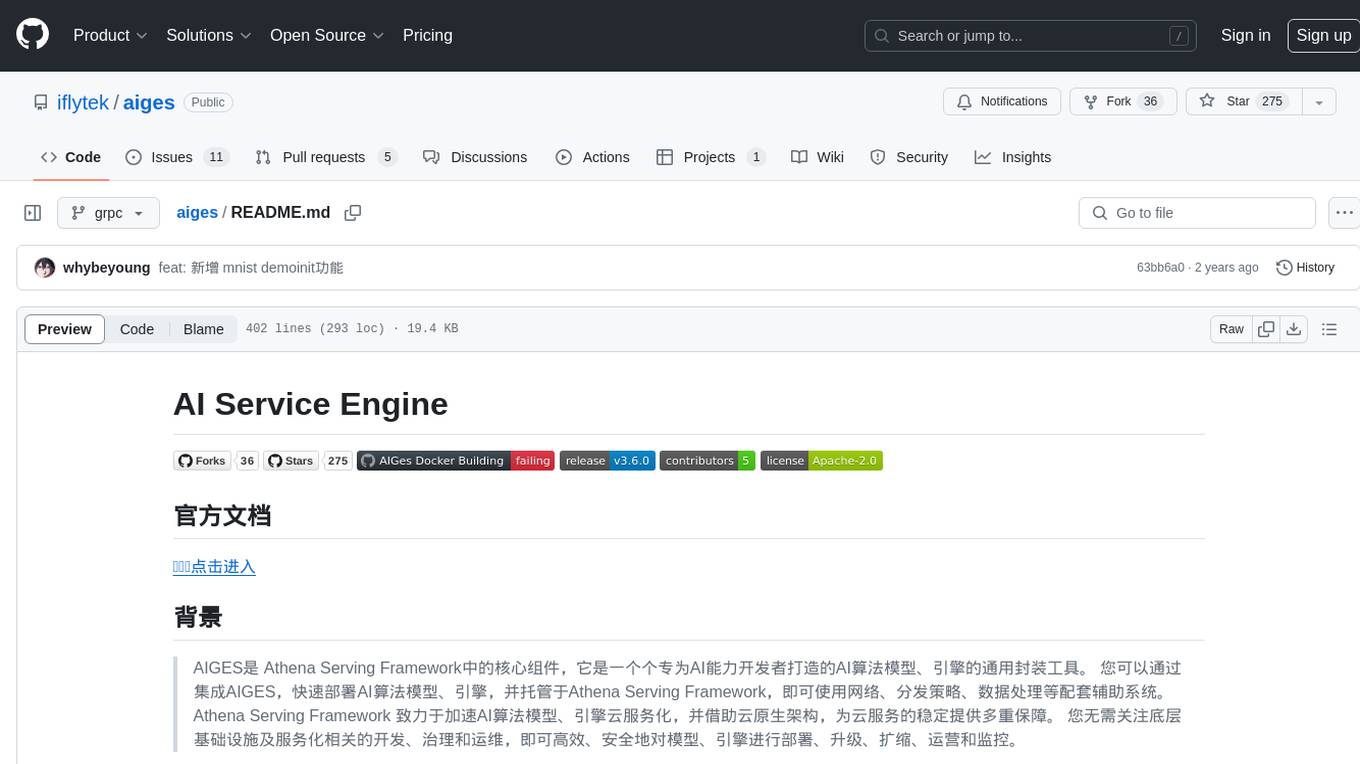
aiges
AIGES is a core component of the Athena Serving Framework, designed as a universal encapsulation tool for AI developers to deploy AI algorithm models and engines quickly. By integrating AIGES, you can deploy AI algorithm models and engines rapidly and host them on the Athena Serving Framework, utilizing supporting auxiliary systems for networking, distribution strategies, data processing, etc. The Athena Serving Framework aims to accelerate the cloud service of AI algorithm models and engines, providing multiple guarantees for cloud service stability through cloud-native architecture. You can efficiently and securely deploy, upgrade, scale, operate, and monitor models and engines without focusing on underlying infrastructure and service-related development, governance, and operations.