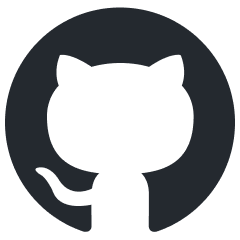
EasyInstruct
[ACL 2024] An Easy-to-use Instruction Processing Framework for LLMs.
Stars: 381
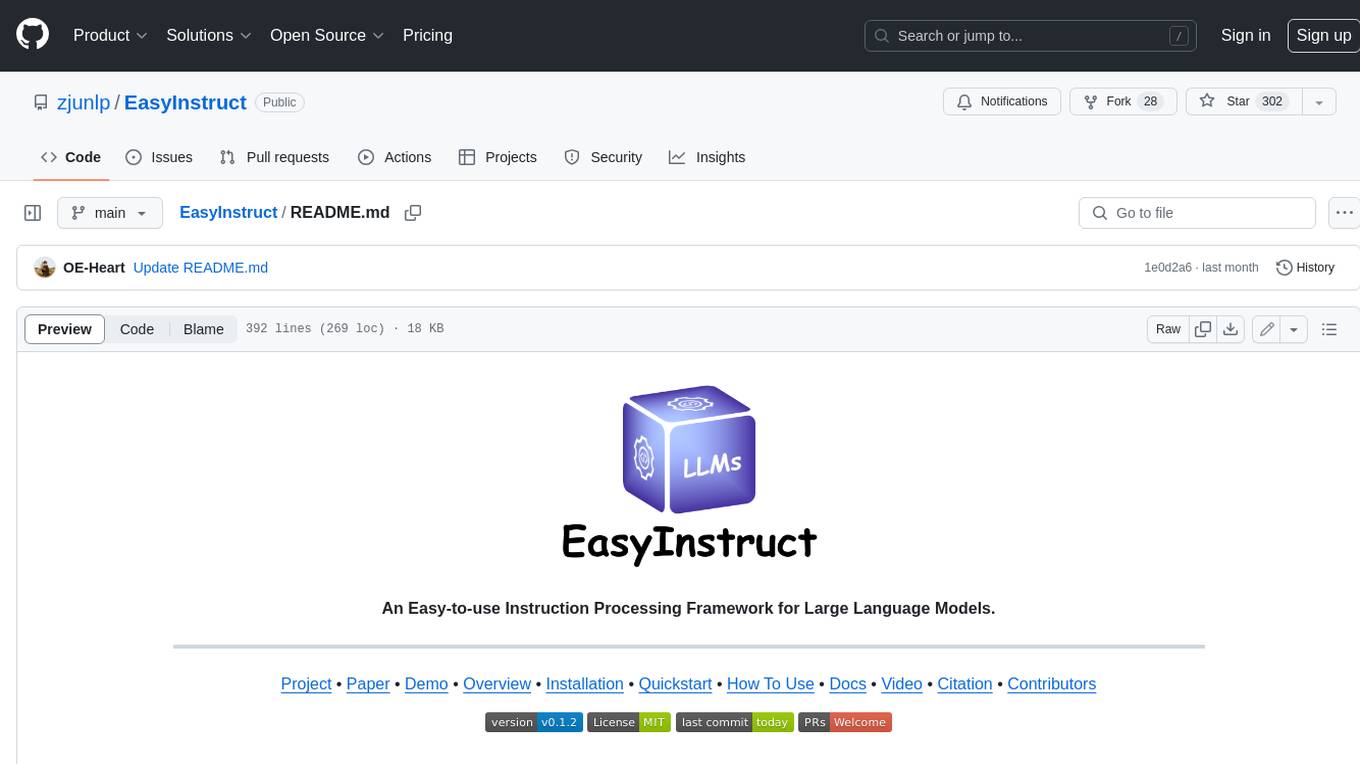
EasyInstruct is a Python package proposed as an easy-to-use instruction processing framework for Large Language Models (LLMs) like GPT-4, LLaMA, ChatGLM in your research experiments. EasyInstruct modularizes instruction generation, selection, and prompting, while also considering their combination and interaction.
README:

An Easy-to-use Instruction Processing Framework for Large Language Models.
Project • Paper • Demo • Overview • Installation • Quickstart • How To Use • Docs • Video • Citation • Contributors
- 2024-06-04, EasyInstruct is accepted by ACL 2024 System Demonstration Track. 🎉🎉
- 2024-02-06 We release a new paper: "EasyInstruct: An Easy-to-use Instruction Processing Framework for Large Language Models" with an HF demo EasyInstruct.
- 2024-02-06 We release a preliminary tool EasyDetect for hallucination detection, with a demo.
- 2024-02-05 We release version 0.1.2, supporting for new features and optimising the function interface.
- 2023-12-09 The paper "When Do Program-of-Thoughts Work for Reasoning?" (supported by EasyInstruct), is accepted by AAAI 2024!
- 2023-10-28 We release version 0.1.1, supporting for new features of instruction generation and instruction selection.
- 2023-08-09 We release version 0.0.6, supporting Cohere API calls.
- 2023-07-12 We release EasyEdit, an easy-to-use framework to edit Large Language Models.
Previous news
- 2023-5-23 We release version 0.0.5, removing requirement of llama-cpp-python.
- 2023-5-16 We release version 0.0.4, fixing some problems.
- 2023-4-21 We release version 0.0.3, check out our documentations for more details.
- 2023-3-25 We release version 0.0.2, suporting IndexPrompt, MMPrompt, IEPrompt and more LLMs
- 2023-3-13 We release version 0.0.1, supporting in-context learning, chain-of-thought with ChatGPT.
This repository is a subproject of KnowLM.
EasyInstruct is a Python package which is proposed as an easy-to-use instruction processing framework for Large Language Models(LLMs) like GPT-4, LLaMA, ChatGLM in your research experiments. EasyInstruct modularizes instruction generation, selection, and prompting, while also considering their combination and interaction.
-
The current supported instruction generation techniques are as follows:
Methods Description Self-Instruct The method that randomly samples a few instructions from a human-annotated seed tasks pool as demonstrations and prompts an LLM to generate more instructions and corresponding input-output pairs. Evol-Instruct The method that incrementally upgrades an initial set of instructions into more complex instructions by prompting an LLM with specific prompts. Backtranslation The method that creates an instruction following training instance by predicting an instruction that would be correctly answered by a portion of a document of the corpus. KG2Instruct The method that creates an instruction following training instance by predicting an instruction that would be correctly answered by a portion of a document of the corpus. -
The current supported instruction selection metrics are as follows:
Metrics Notation Description Length $Len$ The bounded length of every pair of instruction and response. Perplexity $PPL$ The exponentiated average negative log-likelihood of response. MTLD $MTLD$ Measure of textual lexical diversity, the mean length of sequential words in a text that maintains a minimum threshold TTR score. ROUGE $ROUGE$ Recall-Oriented Understudy for Gisting Evaluation, a set of metrics used for evaluating similarities between sentences. GPT score $GPT$ The score of whether the output is a good example of how AI Assistant should respond to the user's instruction, provided by ChatGPT. CIRS $CIRS$ The score using the abstract syntax tree to encode structural and logical attributes, to measure the correlation between code and reasoning abilities. -
API service providers and their corresponding LLM products that are currently available:
Model Description Default Version OpenAI GPT-3.5 A set of models that improve on GPT-3 and can understand as well as generate natural language or code. gpt-3.5-turbo
GPT-4 A set of models that improve on GPT-3.5 and can understand as well as generate natural language or code. gpt-4
Anthropic Claude A next-generation AI assistant based on Anthropic’s research into training helpful, honest, and harmless AI systems. claude-2.0
Claude-Instant A lighter, less expensive, and much faster option than Claude. claude-instant-1.2
Cohere Command A flagship text generation model of Cohere trained to follow user commands and to be instantly useful in practical business applications. command
Command-Light A light version of Command models that are faster but may produce lower-quality generated text. command-light
Installation from git repo branch:
pip install git+https://github.com/zjunlp/EasyInstruct@main
Installation for local development:
git clone https://github.com/zjunlp/EasyInstruct
cd EasyInstruct
pip install -e .
Installation using PyPI (not the latest version):
pip install easyinstruct -i https://pypi.org/simple
We provide two ways for users to quickly get started with EasyInstruct. You can either use the shell script or the Gradio app based on your specific needs.
Users can easily configure the parameters of EasyInstruct in a YAML-style file or just quickly use the default parameters in the configuration files we provide. Following is an example of the configuration file for Self-Instruct:
generator:
SelfInstructGenerator:
target_dir: data/generations/
data_format: alpaca
seed_tasks_path: data/seed_tasks.jsonl
generated_instructions_path: generated_instructions.jsonl
generated_instances_path: generated_instances.jsonl
num_instructions_to_generate: 100
engine: gpt-3.5-turbo
num_prompt_instructions: 8
More example configuration files can be found at configs.
Users should first specify the configuration file and provide their own OpenAI API key. Then, run the following shell script to launch the instruction generation or selection process.
config_file=""
openai_api_key=""
python demo/run.py \
--config $config_file\
--openai_api_key $openai_api_key \
We provide a Gradio app for users to quickly get started with EasyInstruct. You can run the following command to launch the Gradio app locally on the port 8080
(if available).
python demo/app.py
We also host a running gradio app in HuggingFace Spaces. You can try it out here.
Please refer to our documentations for more details.
The Generators
module streamlines the process of instruction data generation, allowing for the generation of instruction data based on seed data. You can choose the appropriate generator based on your specific needs.
BaseGenerator
is the base class for all generators.
You can also easily inherit this base class to customize your own generator class. Just override the
__init__
andgenerate
method.
SelfInstructGenerator
is the class for the instruction generation method of Self-Instruct. See Self-Instruct: Aligning Language Model with Self Generated Instructions for more details.
Example
from easyinstruct import SelfInstructGenerator
from easyinstruct.utils.api import set_openai_key
# Step1: Set your own API-KEY
set_openai_key("YOUR-KEY")
# Step2: Declare a generator class
generator = SelfInstructGenerator(num_instructions_to_generate=10)
# Step3: Generate self-instruct data
generator.generate()
BacktranslationGenerator
is the class for the instruction generation method of Instruction Backtranslation. See Self-Alignment with Instruction Backtranslation for more details.
Example
from easyinstruct import BacktranslationGenerator
from easyinstruct.utils.api import set_openai_key
# Step1: Set your own API-KEY
set_openai_key("YOUR-KEY")
# Step2: Declare a generator class
generator = BacktranslationGenerator(num_instructions_to_generate=10)
# Step3: Generate backtranslation data
generator.generate()
EvolInstructGenerator
is the class for the instruction generation method of EvolInstruct. See WizardLM: Empowering Large Language Models to Follow Complex Instructions for more details.
Example
from easyinstruct import EvolInstructGenerator
from easyinstruct.utils.api import set_openai_key
# Step1: Set your own API-KEY
set_openai_key("YOUR-KEY")
# Step2: Declare a generator class
generator = EvolInstructGenerator(num_instructions_to_generate=10)
# Step3: Generate evolution data
generator.generate()
KG2InstructGenerator
is the class for the instruction generation method of KG2Instruct. See InstructIE: A Chinese Instruction-based Information Extraction Dataset for more details.
The Selectors
module standardizes the instruction selection process, enabling the extraction of high-quality instruction datasets from raw, unprocessed instruction data. The raw data can be sourced from publicly available instruction datasets or generated by the framework itself. You can choose the appropriate selector based on your specific needs.
BaseSelector
is the base class for all selectors.
You can also easily inherit this base class to customize your own selector class. Just override the
__init__
and__process__
method.
Deduplicator
is the class for eliminating duplicate instruction samples that could adversely affect both pre-training stability and the performance of LLMs.Deduplicator
can also enables efficient use and optimization of storage space.
LengthSelector
is the class for selecting instruction samples based on the length of the instruction. Instructions that are too long or too short can affect data quality and are not conducive to instruction tuning.
RougeSelector
is the class for selecting instruction samples based on the ROUGE metric which is often used for evaluating the quality of automated generation of text.
GPTScoreSelector
is the class for selecting instruction samples based on the GPT score, which reflects whether the output is a good example of how AI Assistant should respond to the user's instruction, provided by ChatGPT.
PPLSelector
is the class for selecting instruction samples based on the perplexity, which is the exponentiated average negative log-likelihood of response.
MTLDSelector
is the class for selecting instruction samples based on the MTLD, which is short for Measure of Textual Lexical Diversity.
CodeSelector
is the class for selecting code instruction samples based on the Complexity-Impacted Reasoning Score (CIRS), which combines structural and logical attributes, to measure the correlation between code and reasoning abilities. See When Do Program-of-Thoughts Work for Reasoning? for more details.
Example
from easyinstruct import CodeSelector
# Step1: Specify your source file of code instructions
src_file = "data/code_example.json"
# Step2: Declare a code selecter class
selector = CodeSelector(
source_file_path=src_file,
target_dir="data/selections/",
manually_partion_data=True,
min_boundary = 0.125,
max_boundary = 0.5,
automatically_partion_data = True,
k_means_cluster_number = 2,
)
# Step3: Process the code instructions
selector.process()
MultiSelector
is the class for combining multiple appropricate selectors based on your specific needs.
The Prompts
module standardizes the instruction prompting step, where user requests are constructed as instruction prompts and sent to specific LLMs to obtain responses. You can choose the appropriate prompting method based on your specific needs.
Please check out link for more detials.
The Engines
module standardizes the instruction execution process, enabling the execution of instruction prompts on specific locally deployed LLMs. You can choose the appropriate engine based on your specific needs.
Please check out link for more detials.
Please cite our repository if you use EasyInstruct in your work.
@article{ou2024easyinstruct,
title={EasyInstruct: An Easy-to-use Instruction Processing Framework for Large Language Models},
author={Ou, Yixin and Zhang, Ningyu and Gui, Honghao and Xu, Ziwen and Qiao, Shuofei and Bi, Zhen and Chen, Huajun},
journal={arXiv preprint arXiv:2402.03049},
year={2024}
}
@misc{knowlm,
author = {Ningyu Zhang and Jintian Zhang and Xiaohan Wang and Honghao Gui and Kangwei Liu and Yinuo Jiang and Xiang Chen and Shengyu Mao and Shuofei Qiao and Yuqi Zhu and Zhen Bi and Jing Chen and Xiaozhuan Liang and Yixin Ou and Runnan Fang and Zekun Xi and Xin Xu and Lei Li and Peng Wang and Mengru Wang and Yunzhi Yao and Bozhong Tian and Yin Fang and Guozhou Zheng and Huajun Chen},
title = {KnowLM: An Open-sourced Knowledgeable Large Langugae Model Framework},
year = {2023},
url = {http://knowlm.zjukg.cn/},
}
@article{bi2023program,
title={When do program-of-thoughts work for reasoning?},
author={Bi, Zhen and Zhang, Ningyu and Jiang, Yinuo and Deng, Shumin and Zheng, Guozhou and Chen, Huajun},
journal={arXiv preprint arXiv:2308.15452},
year={2023}
}
We will offer long-term maintenance to fix bugs, solve issues and meet new requests. So if you have any problems, please put issues to us.
Other Related Projects
🙌 We would like to express our heartfelt gratitude for the contribution of Self-Instruct to our project, as we have utilized portions of their source code in our project.
For Tasks:
Click tags to check more tools for each tasksFor Jobs:
Alternative AI tools for EasyInstruct
Similar Open Source Tools
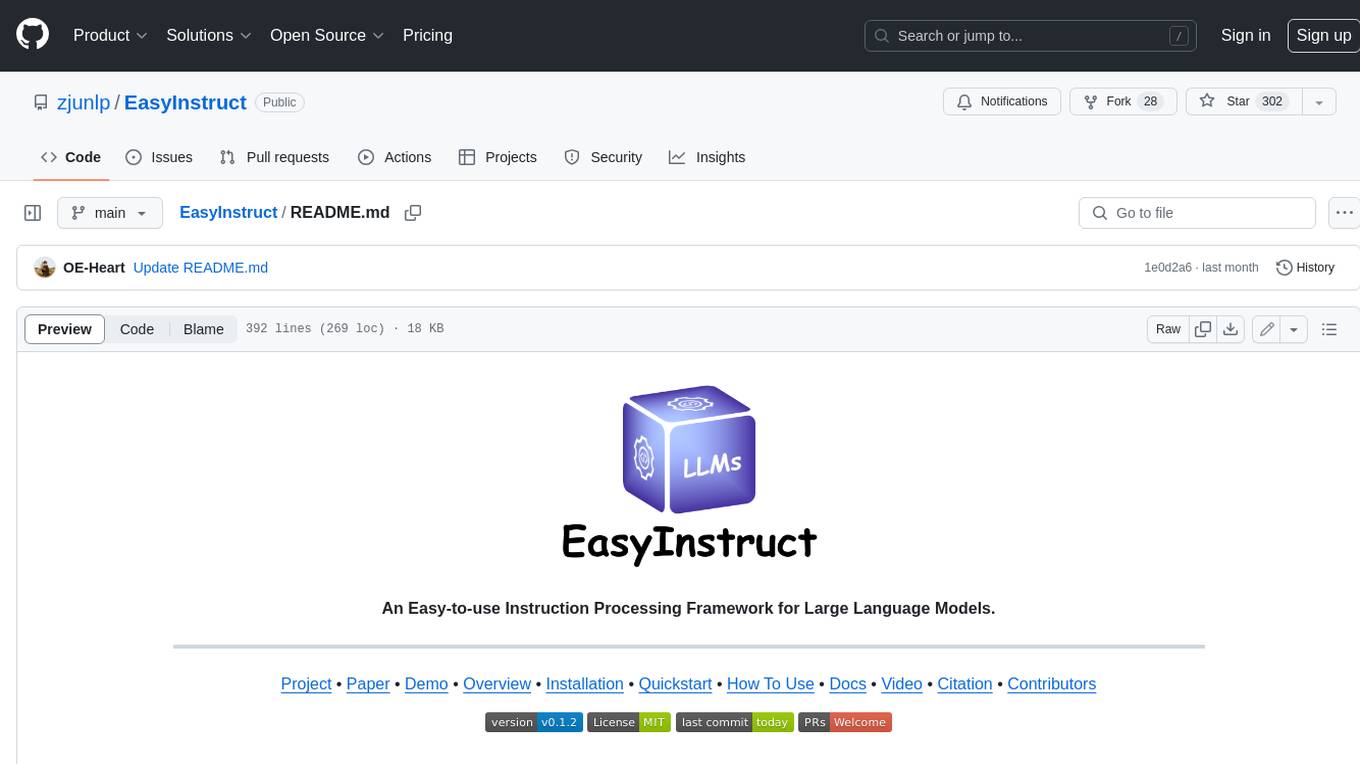
EasyInstruct
EasyInstruct is a Python package proposed as an easy-to-use instruction processing framework for Large Language Models (LLMs) like GPT-4, LLaMA, ChatGLM in your research experiments. EasyInstruct modularizes instruction generation, selection, and prompting, while also considering their combination and interaction.
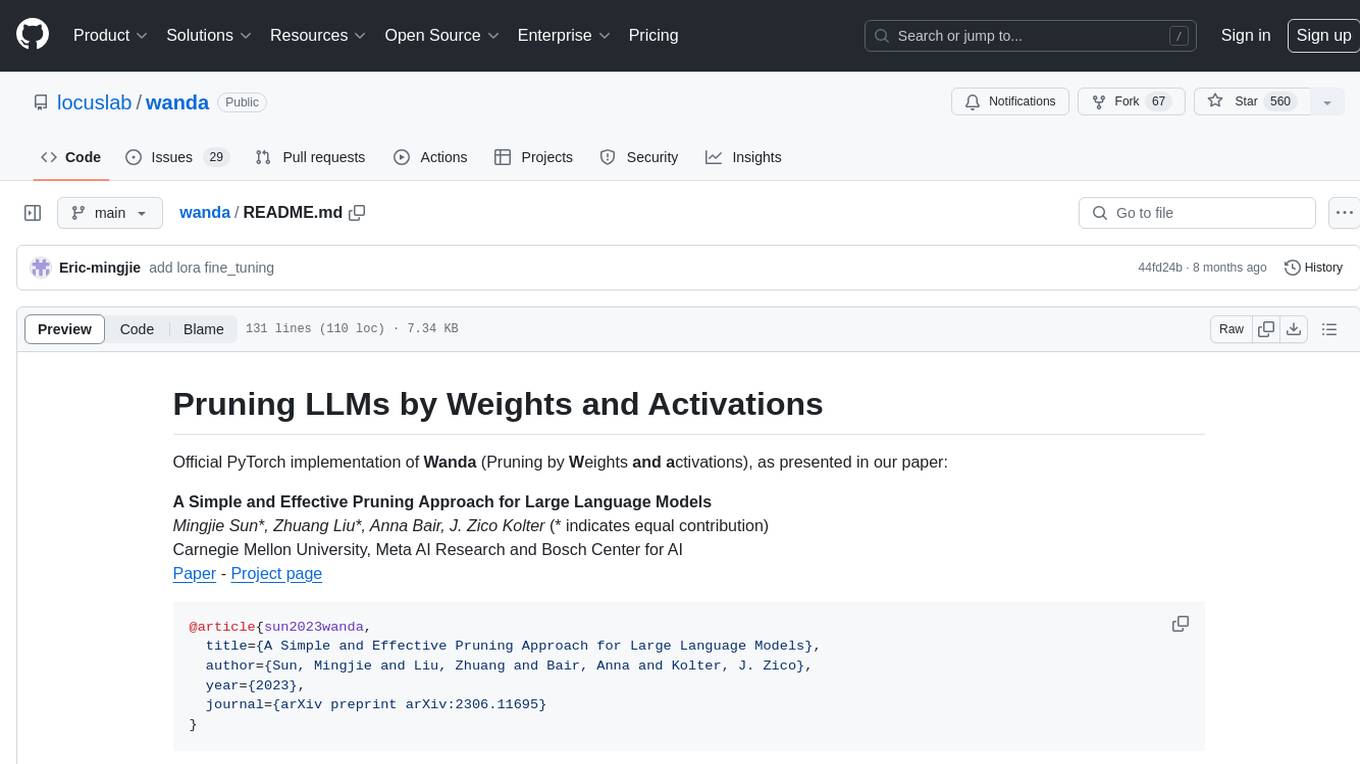
wanda
Official PyTorch implementation of Wanda (Pruning by Weights and Activations), a simple and effective pruning approach for large language models. The pruning approach removes weights on a per-output basis, by the product of weight magnitudes and input activation norms. The repository provides support for various features such as LLaMA-2, ablation study on OBS weight update, zero-shot evaluation, and speedup evaluation. Users can replicate main results from the paper using provided bash commands. The tool aims to enhance the efficiency and performance of language models through structured and unstructured sparsity techniques.
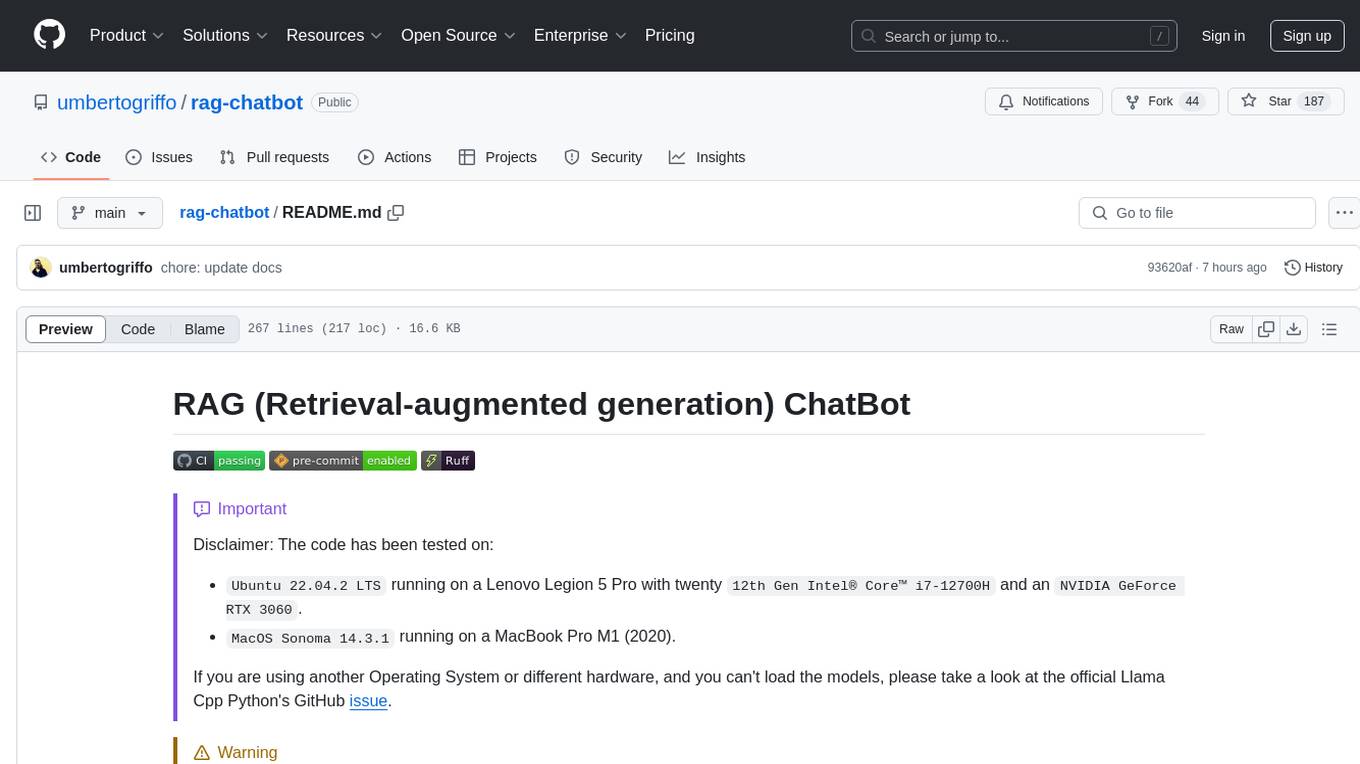
rag-chatbot
The RAG ChatBot project combines Lama.cpp, Chroma, and Streamlit to build a Conversation-aware Chatbot and a Retrieval-augmented generation (RAG) ChatBot. The RAG Chatbot works by taking a collection of Markdown files as input and provides answers based on the context provided by those files. It utilizes a Memory Builder component to load Markdown pages, divide them into sections, calculate embeddings, and save them in an embedding database. The chatbot retrieves relevant sections from the database, rewrites questions for optimal retrieval, and generates answers using a local language model. It also remembers previous interactions for more accurate responses. Various strategies are implemented to deal with context overflows, including creating and refining context, hierarchical summarization, and async hierarchical summarization.
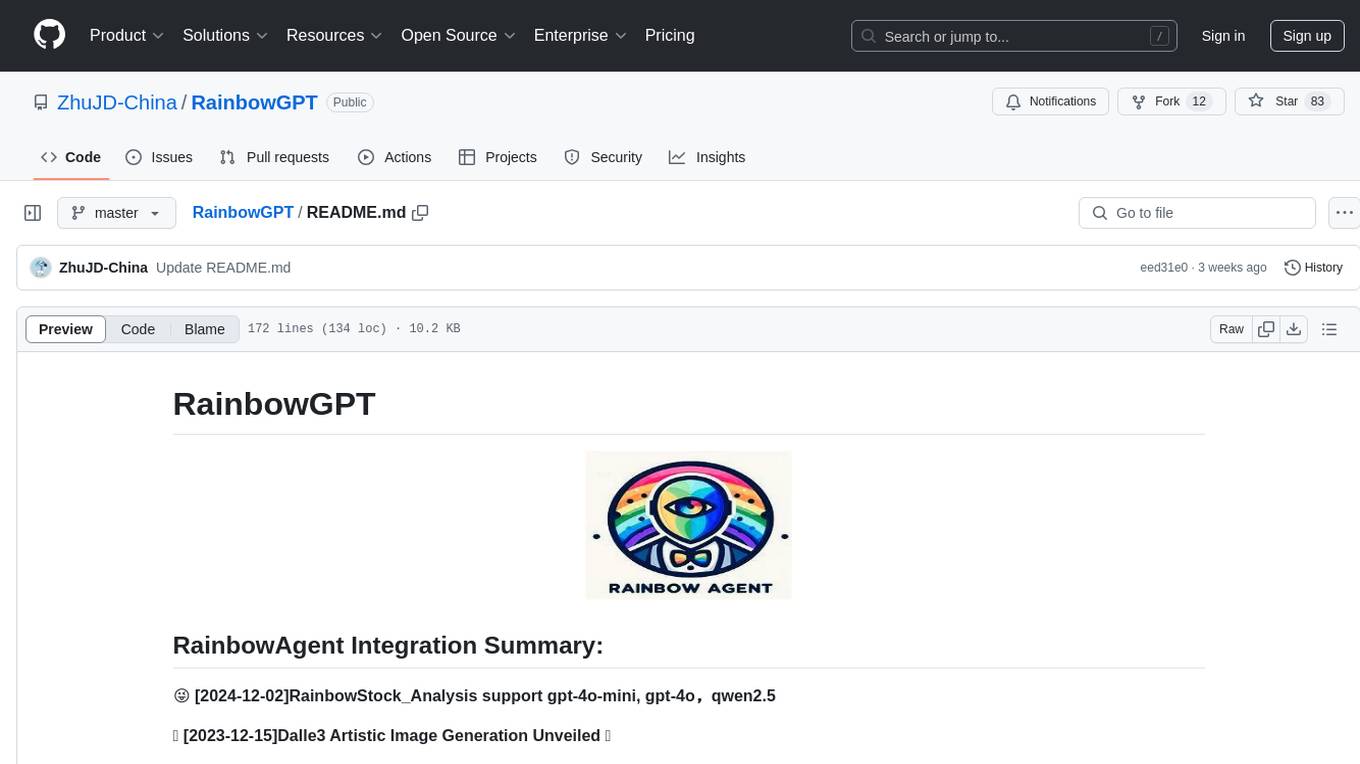
RainbowGPT
RainbowGPT is a versatile tool that offers a range of functionalities, including Stock Analysis for financial decision-making, MySQL Management for database navigation, and integration of AI technologies like GPT-4 and ChatGlm3. It provides a user-friendly interface suitable for all skill levels, ensuring seamless information flow and continuous expansion of emerging technologies. The tool enhances adaptability, creativity, and insight, making it a valuable asset for various projects and tasks.
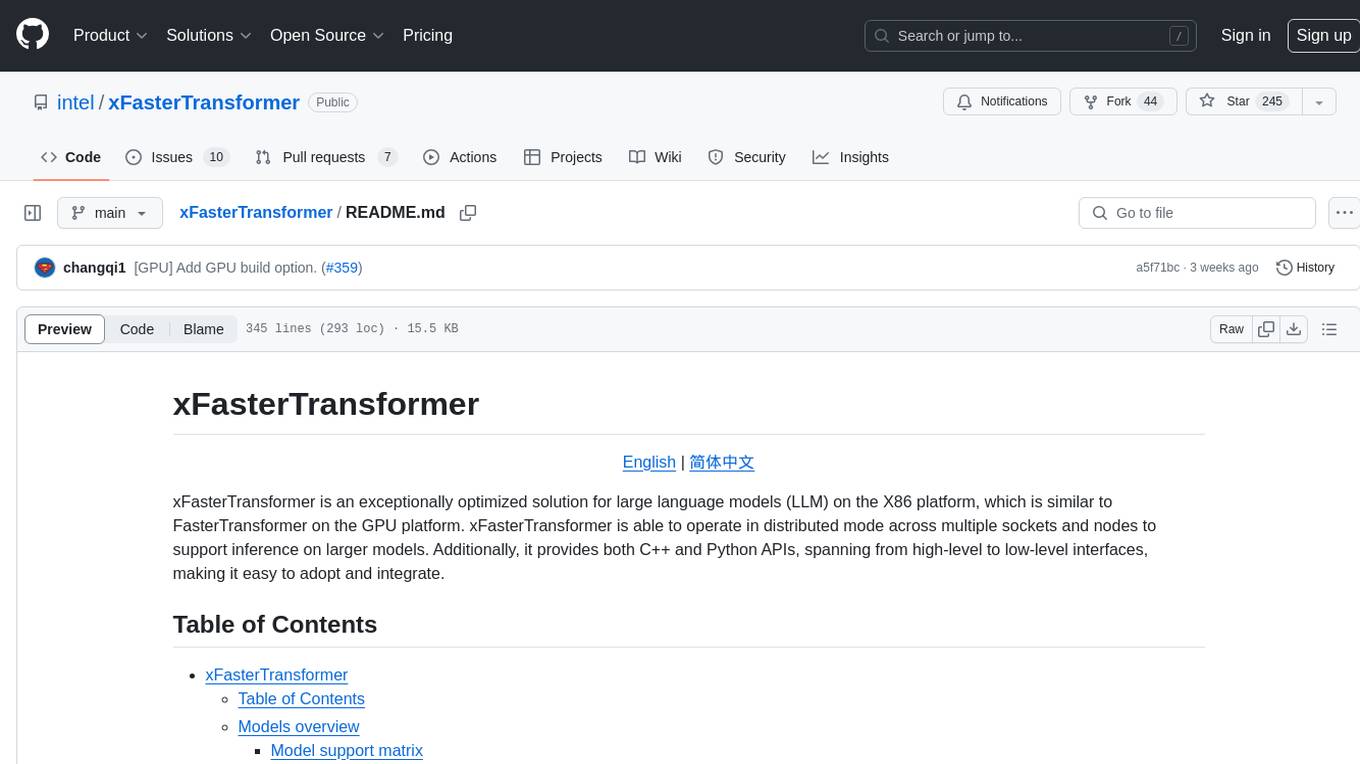
xFasterTransformer
xFasterTransformer is an optimized solution for Large Language Models (LLMs) on the X86 platform, providing high performance and scalability for inference on mainstream LLM models. It offers C++ and Python APIs for easy integration, along with example codes and benchmark scripts. Users can prepare models in a different format, convert them, and use the APIs for tasks like encoding input prompts, generating token ids, and serving inference requests. The tool supports various data types and models, and can run in single or multi-rank modes using MPI. A web demo based on Gradio is available for popular LLM models like ChatGLM and Llama2. Benchmark scripts help evaluate model inference performance quickly, and MLServer enables serving with REST and gRPC interfaces.
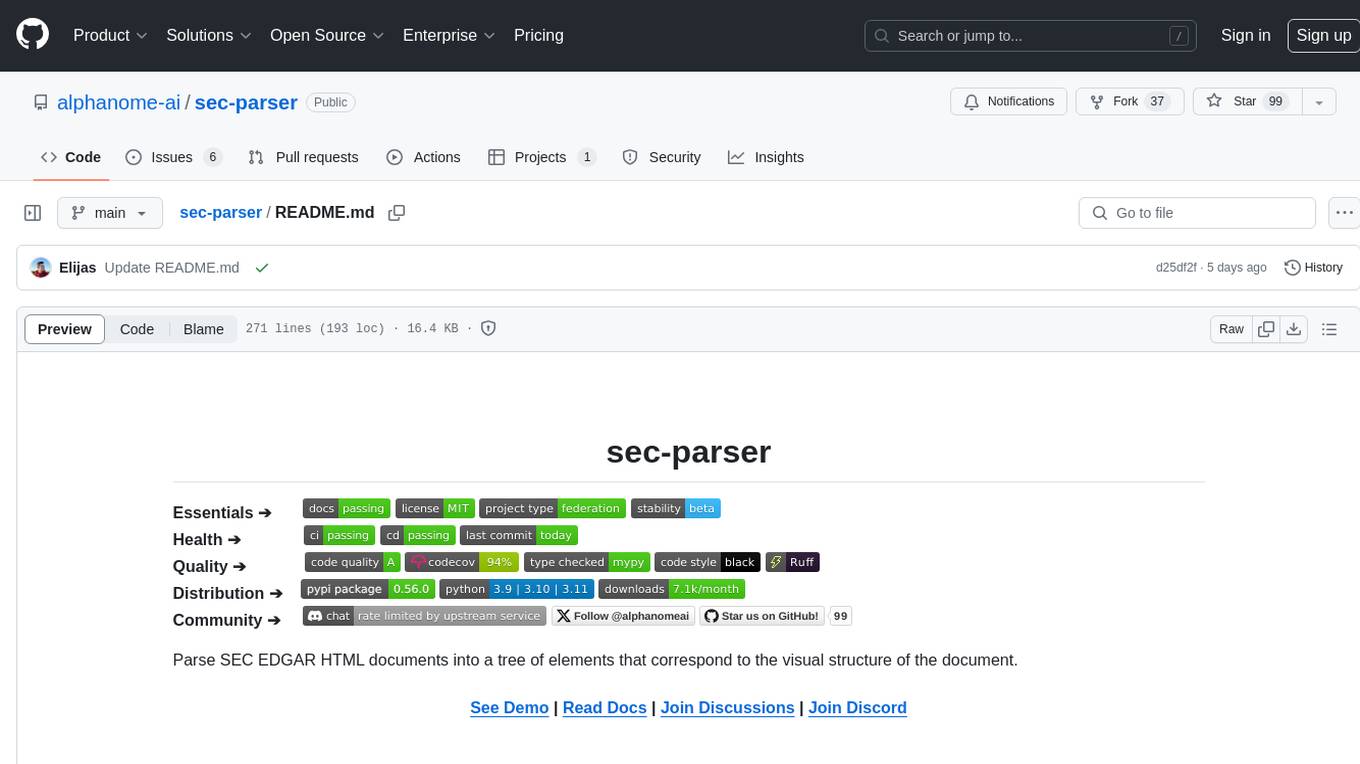
sec-parser
The `sec-parser` project simplifies extracting meaningful information from SEC EDGAR HTML documents by organizing them into semantic elements and a tree structure. It helps in parsing SEC filings for financial and regulatory analysis, analytics and data science, AI and machine learning, causal AI, and large language models. The tool is especially beneficial for AI, ML, and LLM applications by streamlining data pre-processing and feature extraction.
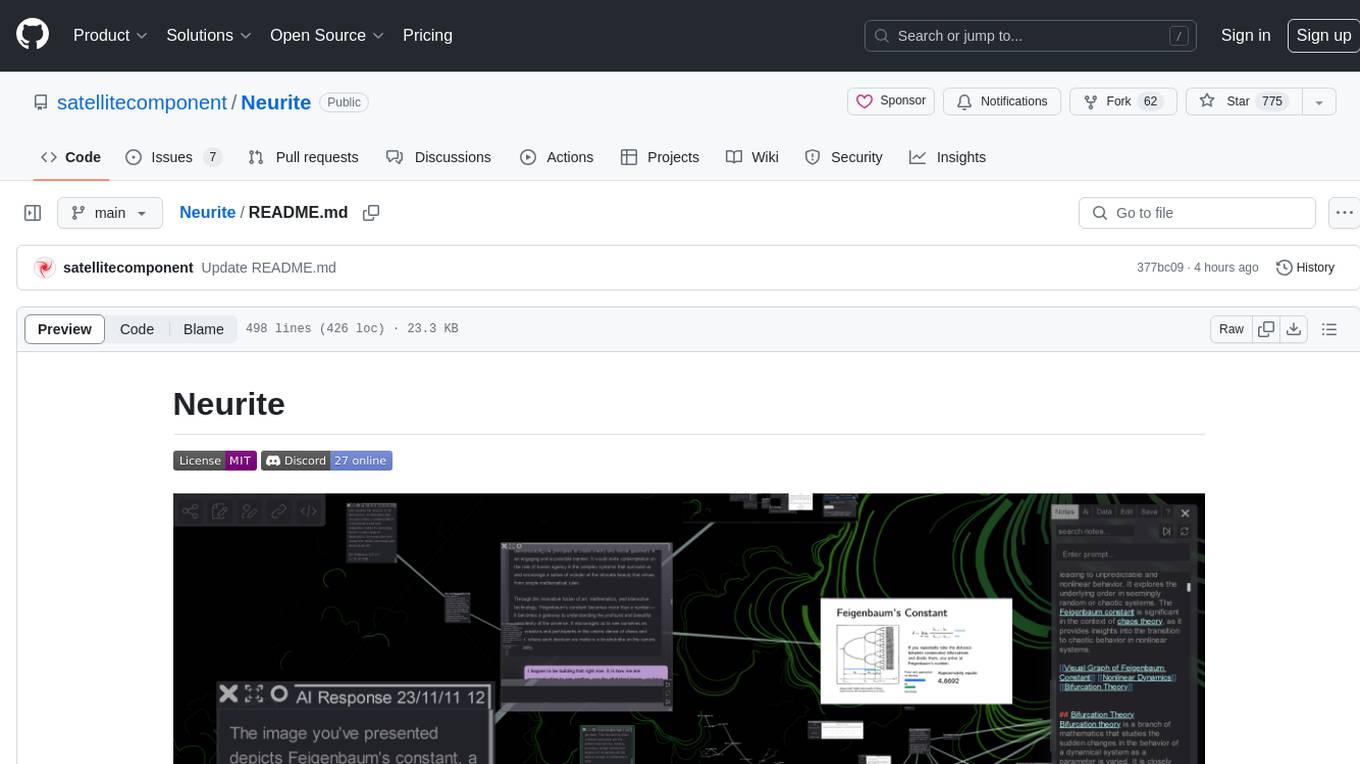
Neurite
Neurite is an innovative project that combines chaos theory and graph theory to create a digital interface that explores hidden patterns and connections for creative thinking. It offers a unique workspace blending fractals with mind mapping techniques, allowing users to navigate the Mandelbrot set in real-time. Nodes in Neurite represent various content types like text, images, videos, code, and AI agents, enabling users to create personalized microcosms of thoughts and inspirations. The tool supports synchronized knowledge management through bi-directional synchronization between mind-mapping and text-based hyperlinking. Neurite also features FractalGPT for modular conversation with AI, local AI capabilities for multi-agent chat networks, and a Neural API for executing code and sequencing animations. The project is actively developed with plans for deeper fractal zoom, advanced control over node placement, and experimental features.
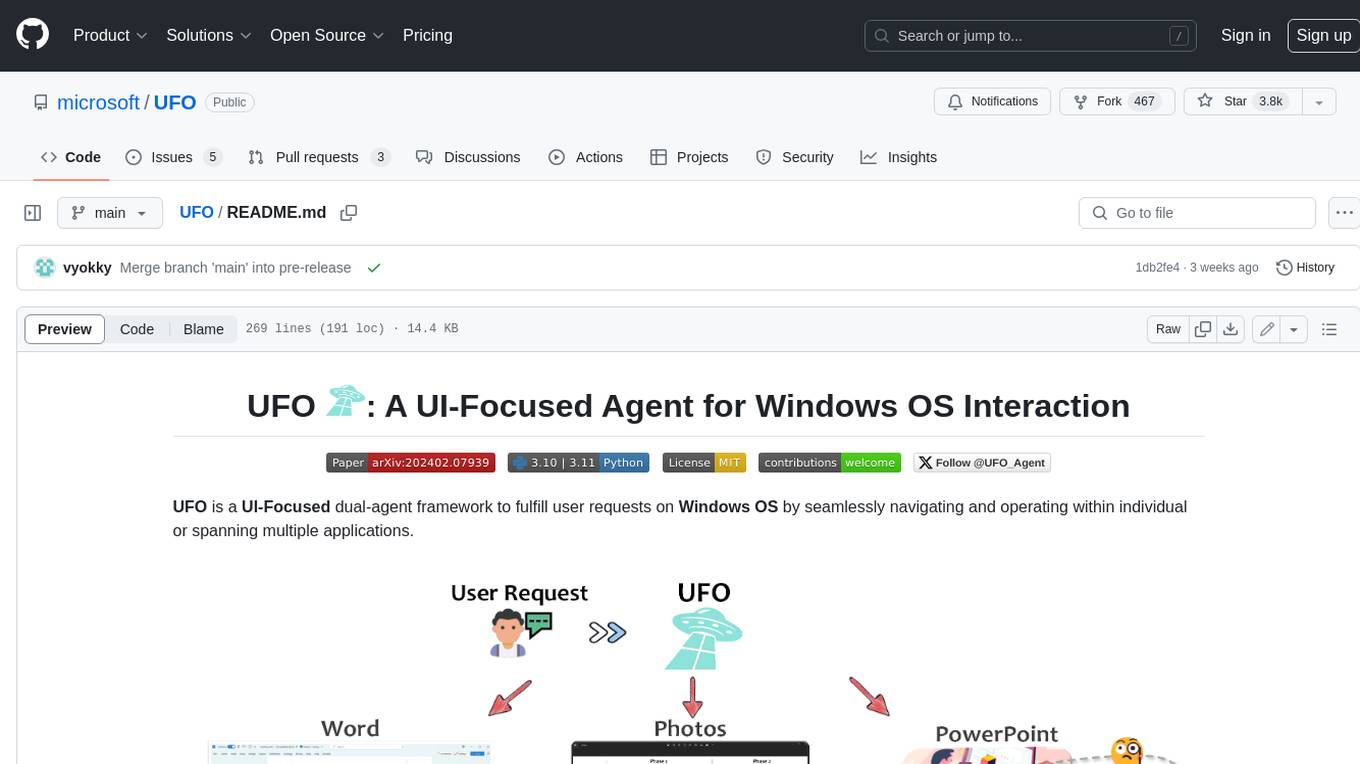
UFO
UFO is a UI-focused dual-agent framework to fulfill user requests on Windows OS by seamlessly navigating and operating within individual or spanning multiple applications.
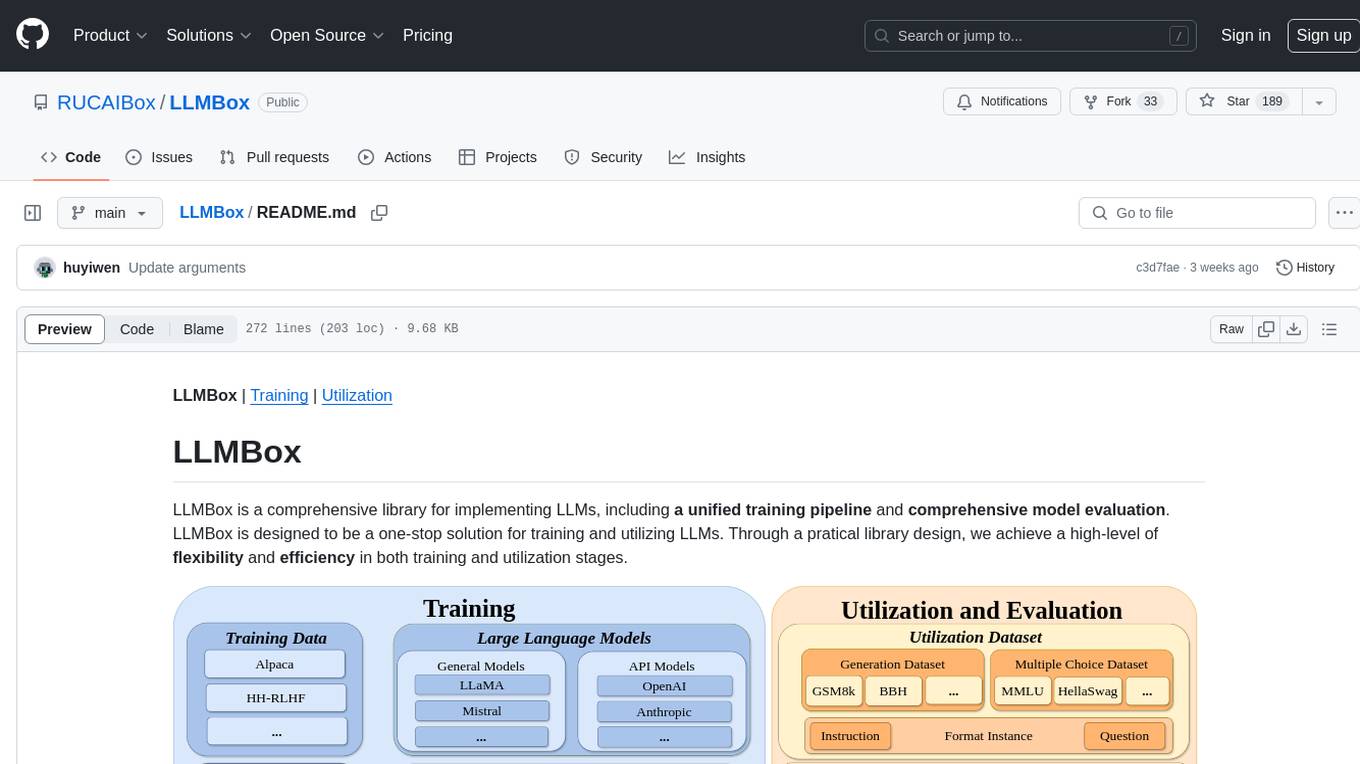
LLMBox
LLMBox is a comprehensive library designed for implementing Large Language Models (LLMs) with a focus on a unified training pipeline and comprehensive model evaluation. It serves as a one-stop solution for training and utilizing LLMs, offering flexibility and efficiency in both training and utilization stages. The library supports diverse training strategies, comprehensive datasets, tokenizer vocabulary merging, data construction strategies, parameter efficient fine-tuning, and efficient training methods. For utilization, LLMBox provides comprehensive evaluation on various datasets, in-context learning strategies, chain-of-thought evaluation, evaluation methods, prefix caching for faster inference, support for specific LLM models like vLLM and Flash Attention, and quantization options. The tool is suitable for researchers and developers working with LLMs for natural language processing tasks.
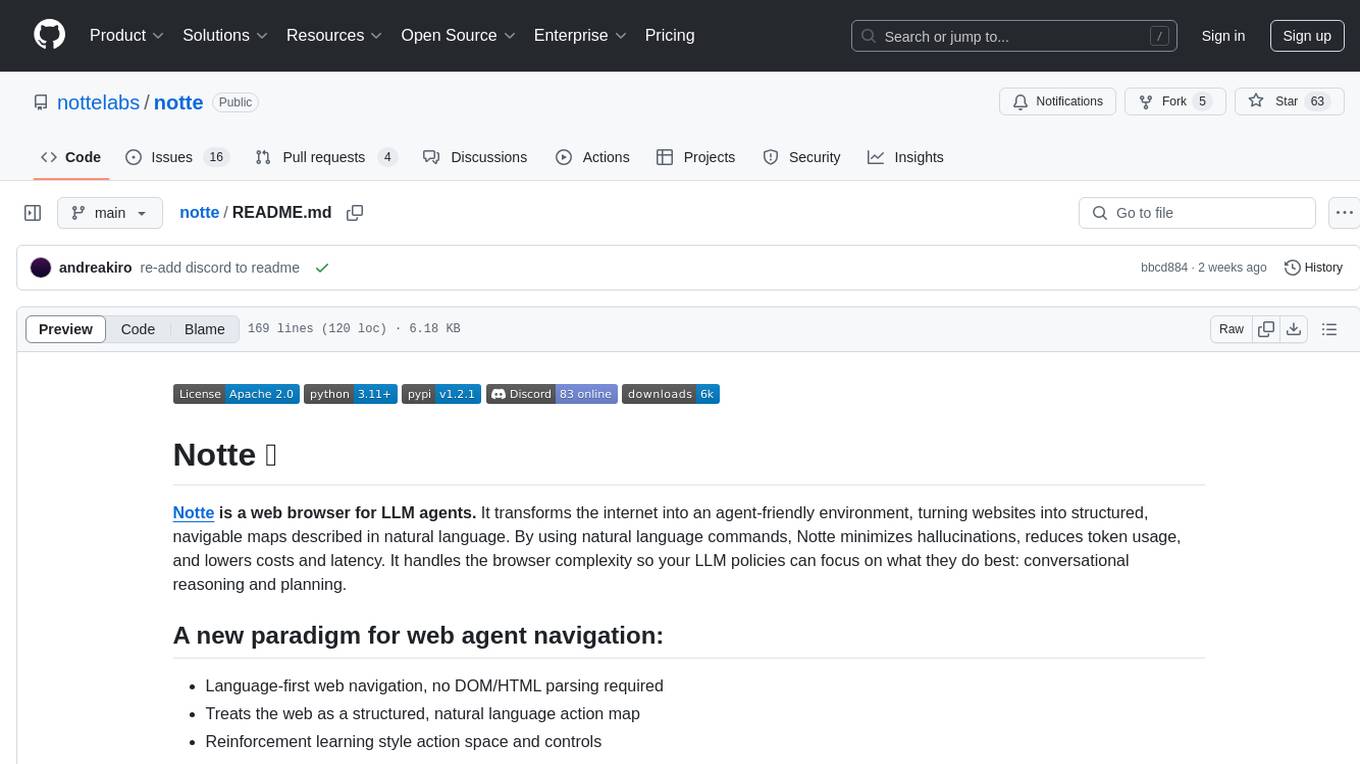
notte
Notte is a web browser designed specifically for LLM agents, providing a language-first web navigation experience without the need for DOM/HTML parsing. It transforms websites into structured, navigable maps described in natural language, enabling users to interact with the web using natural language commands. By simplifying browser complexity, Notte allows LLM policies to focus on conversational reasoning and planning, reducing token usage, costs, and latency. The tool supports various language model providers and offers a reinforcement learning style action space and controls for full navigation control.
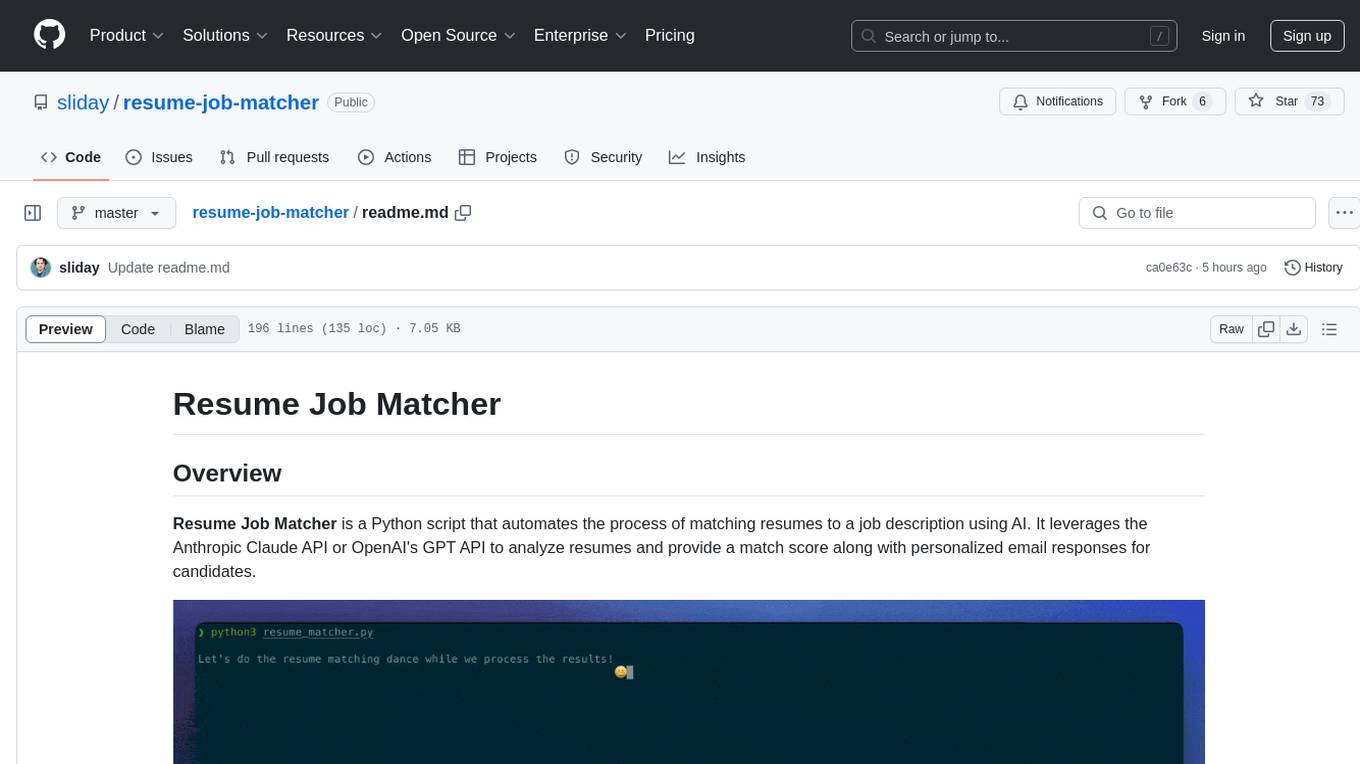
resume-job-matcher
Resume Job Matcher is a Python script that automates the process of matching resumes to a job description using AI. It leverages the Anthropic Claude API or OpenAI's GPT API to analyze resumes and provide a match score along with personalized email responses for candidates. The tool offers comprehensive resume processing, advanced AI-powered analysis, in-depth evaluation & scoring, comprehensive analytics & reporting, enhanced candidate profiling, and robust system management. Users can customize font presets, generate PDF versions of unified resumes, adjust logging level, change scoring model, modify AI provider, and adjust AI model. The final score for each resume is calculated based on AI-generated match score and resume quality score, ensuring content relevance and presentation quality are considered. Troubleshooting tips, best practices, contribution guidelines, and required Python packages are provided.
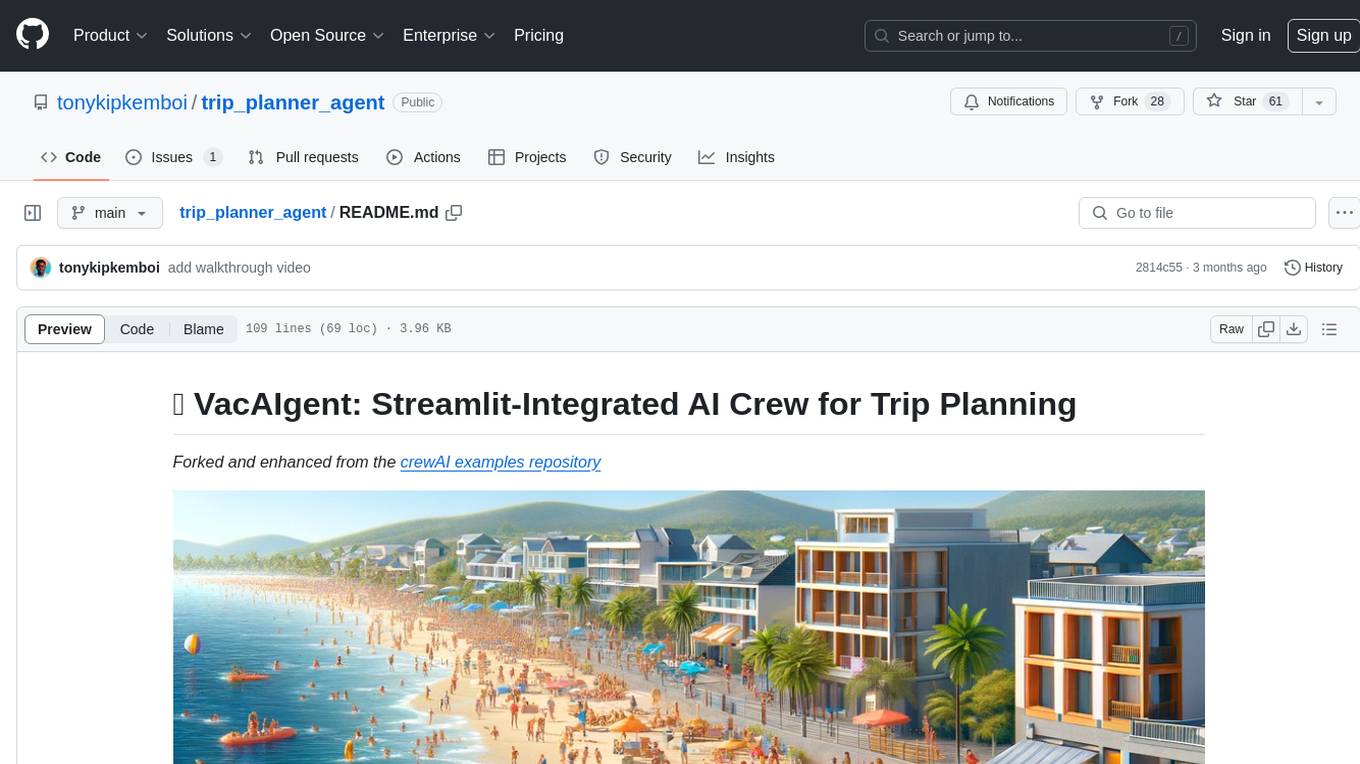
trip_planner_agent
VacAIgent is an AI tool that automates and enhances trip planning by leveraging the CrewAI framework. It integrates a user-friendly Streamlit interface for interactive travel planning. Users can input preferences and receive tailored travel plans with the help of autonomous AI agents. The tool allows for collaborative decision-making on cities and crafting complete itineraries based on specified preferences, all accessible via a streamlined Streamlit user interface. VacAIgent can be customized to use different AI models like GPT-3.5 or local models like Ollama for enhanced privacy and customization.
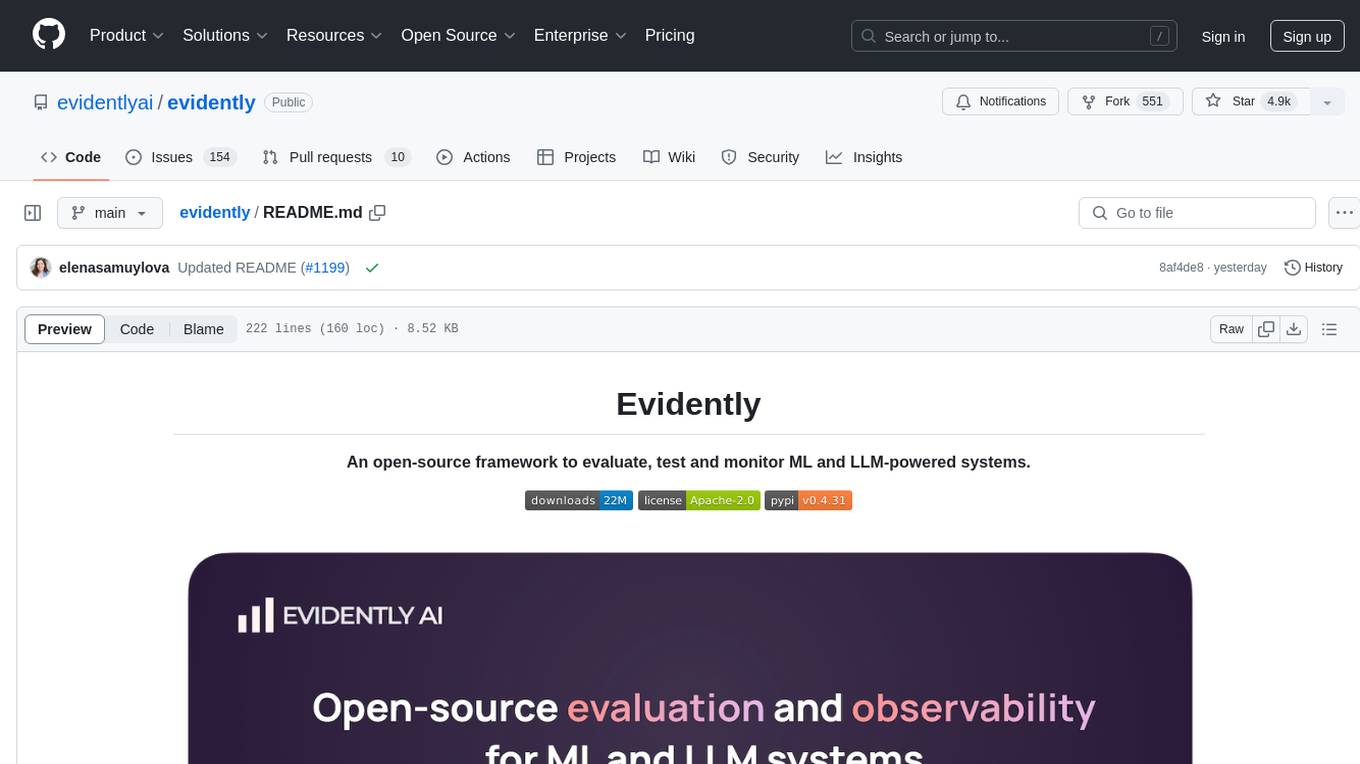
evidently
Evidently is an open-source Python library designed for evaluating, testing, and monitoring machine learning (ML) and large language model (LLM) powered systems. It offers a wide range of functionalities, including working with tabular, text data, and embeddings, supporting predictive and generative systems, providing over 100 built-in metrics for data drift detection and LLM evaluation, allowing for custom metrics and tests, enabling both offline evaluations and live monitoring, and offering an open architecture for easy data export and integration with existing tools. Users can utilize Evidently for one-off evaluations using Reports or Test Suites in Python, or opt for real-time monitoring through the Dashboard service.
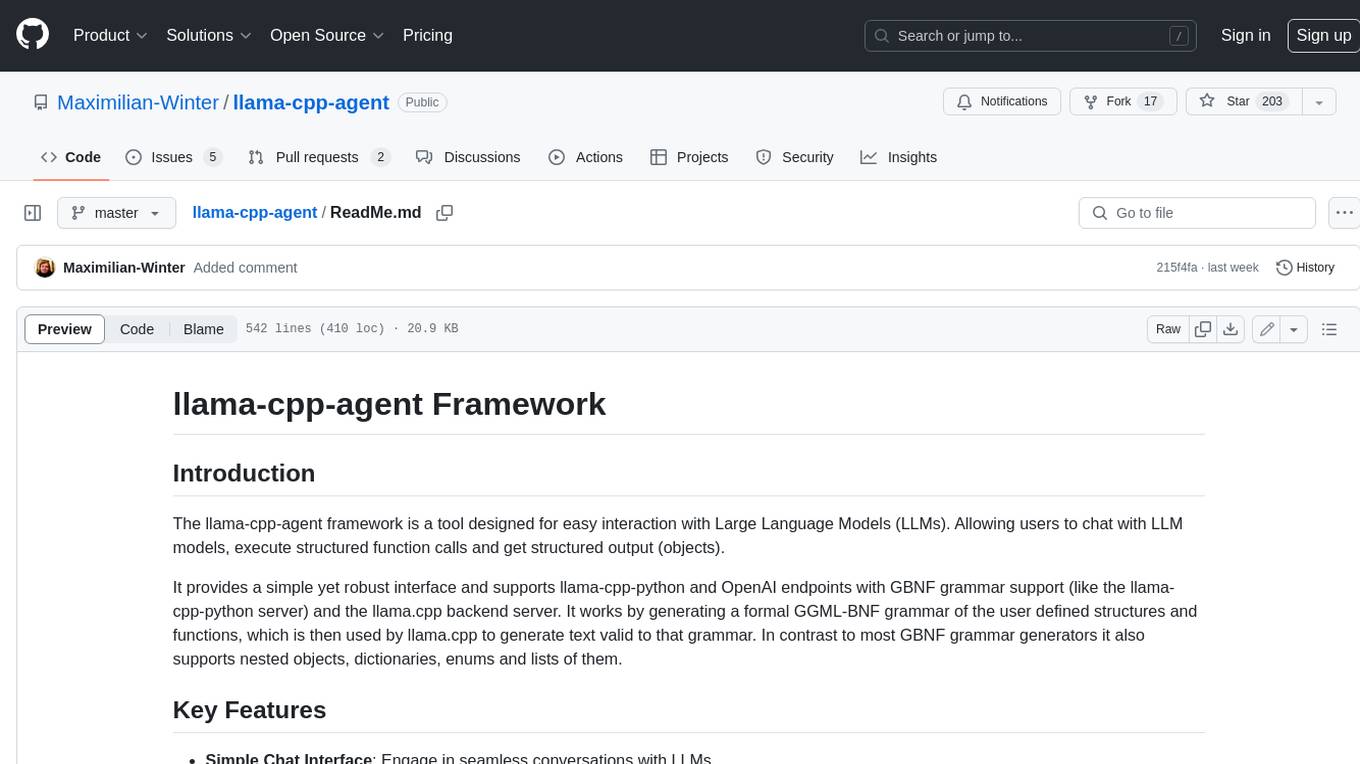
llama-cpp-agent
The llama-cpp-agent framework is a tool designed for easy interaction with Large Language Models (LLMs). Allowing users to chat with LLM models, execute structured function calls and get structured output (objects). It provides a simple yet robust interface and supports llama-cpp-python and OpenAI endpoints with GBNF grammar support (like the llama-cpp-python server) and the llama.cpp backend server. It works by generating a formal GGML-BNF grammar of the user defined structures and functions, which is then used by llama.cpp to generate text valid to that grammar. In contrast to most GBNF grammar generators it also supports nested objects, dictionaries, enums and lists of them.
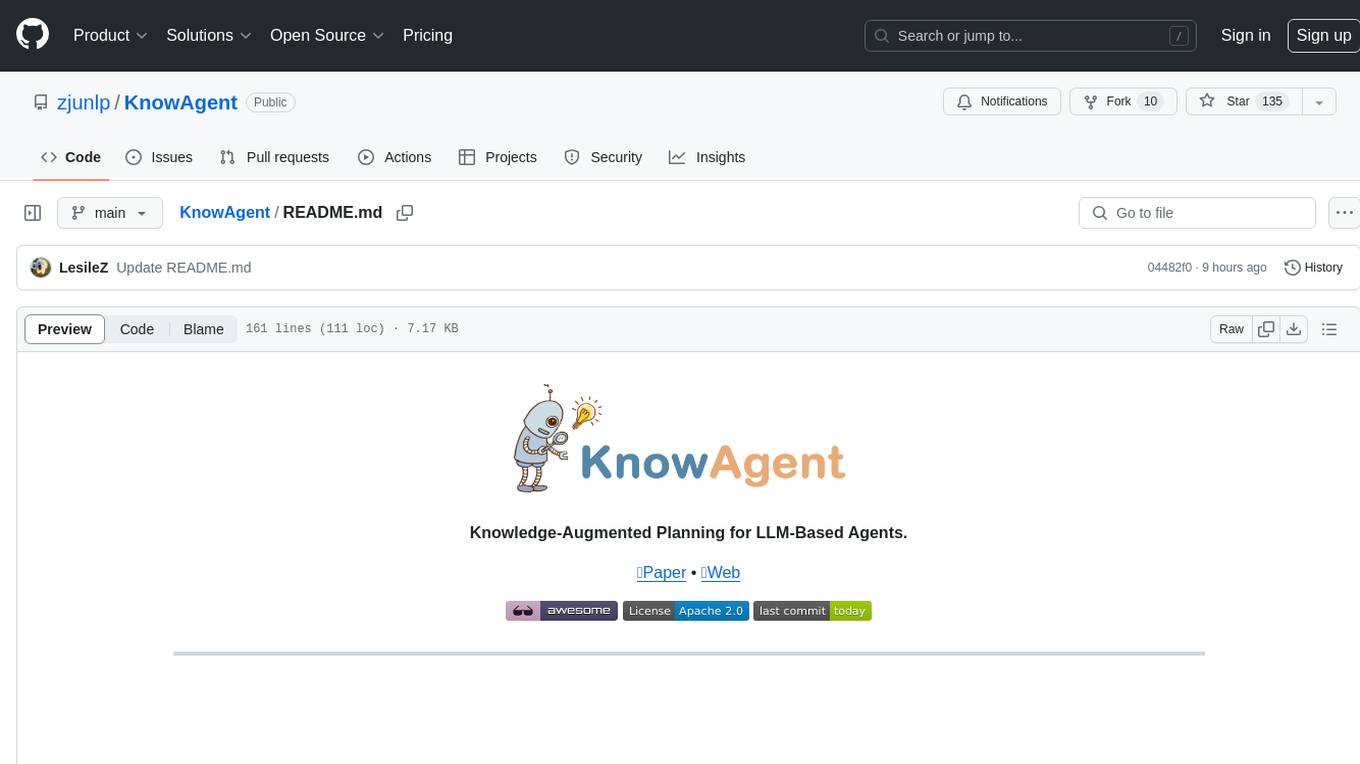
KnowAgent
KnowAgent is a tool designed for Knowledge-Augmented Planning for LLM-Based Agents. It involves creating an action knowledge base, converting action knowledge into text for model understanding, and a knowledgeable self-learning phase to continually improve the model's planning abilities. The tool aims to enhance agents' potential for application in complex situations by leveraging external reservoirs of information and iterative processes.
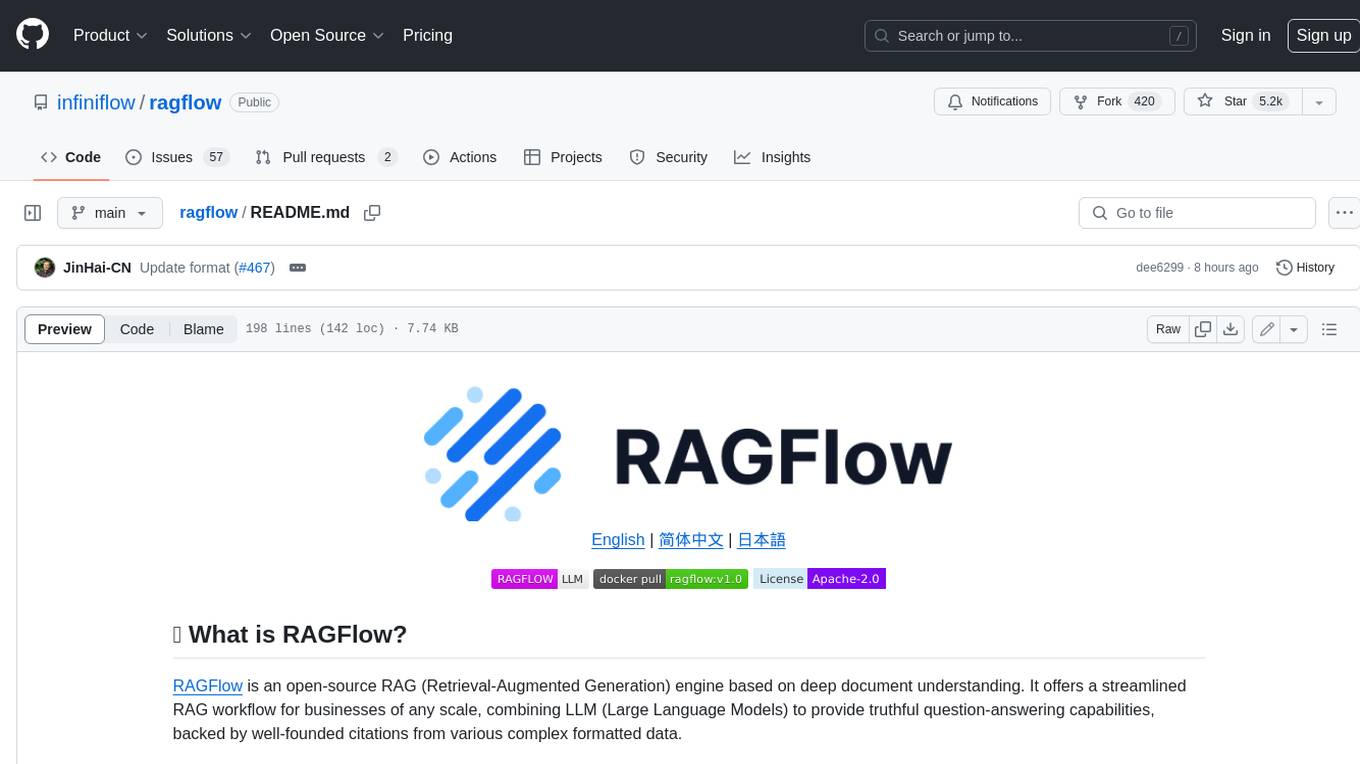
ragflow
RAGFlow is an open-source Retrieval-Augmented Generation (RAG) engine that combines deep document understanding with Large Language Models (LLMs) to provide accurate question-answering capabilities. It offers a streamlined RAG workflow for businesses of all sizes, enabling them to extract knowledge from unstructured data in various formats, including Word documents, slides, Excel files, images, and more. RAGFlow's key features include deep document understanding, template-based chunking, grounded citations with reduced hallucinations, compatibility with heterogeneous data sources, and an automated and effortless RAG workflow. It supports multiple recall paired with fused re-ranking, configurable LLMs and embedding models, and intuitive APIs for seamless integration with business applications.
For similar tasks
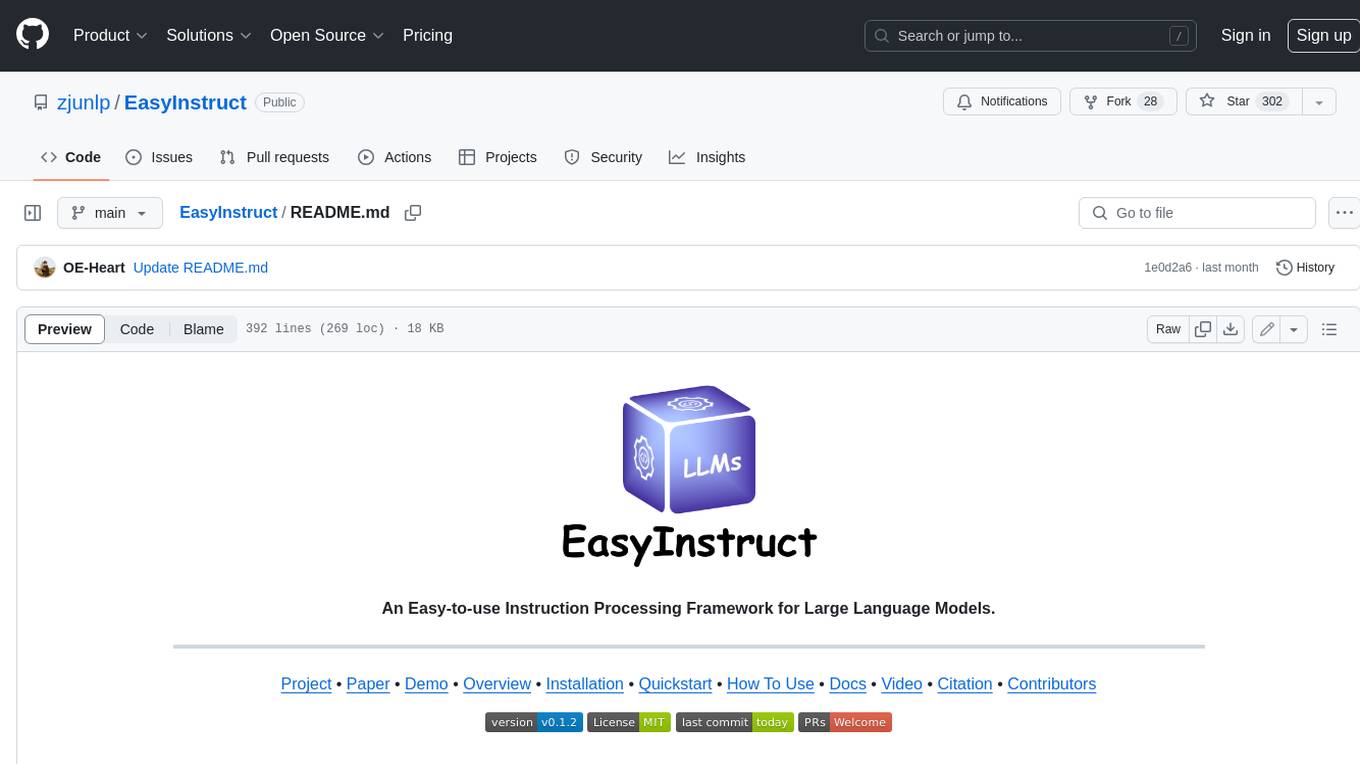
EasyInstruct
EasyInstruct is a Python package proposed as an easy-to-use instruction processing framework for Large Language Models (LLMs) like GPT-4, LLaMA, ChatGLM in your research experiments. EasyInstruct modularizes instruction generation, selection, and prompting, while also considering their combination and interaction.
For similar jobs
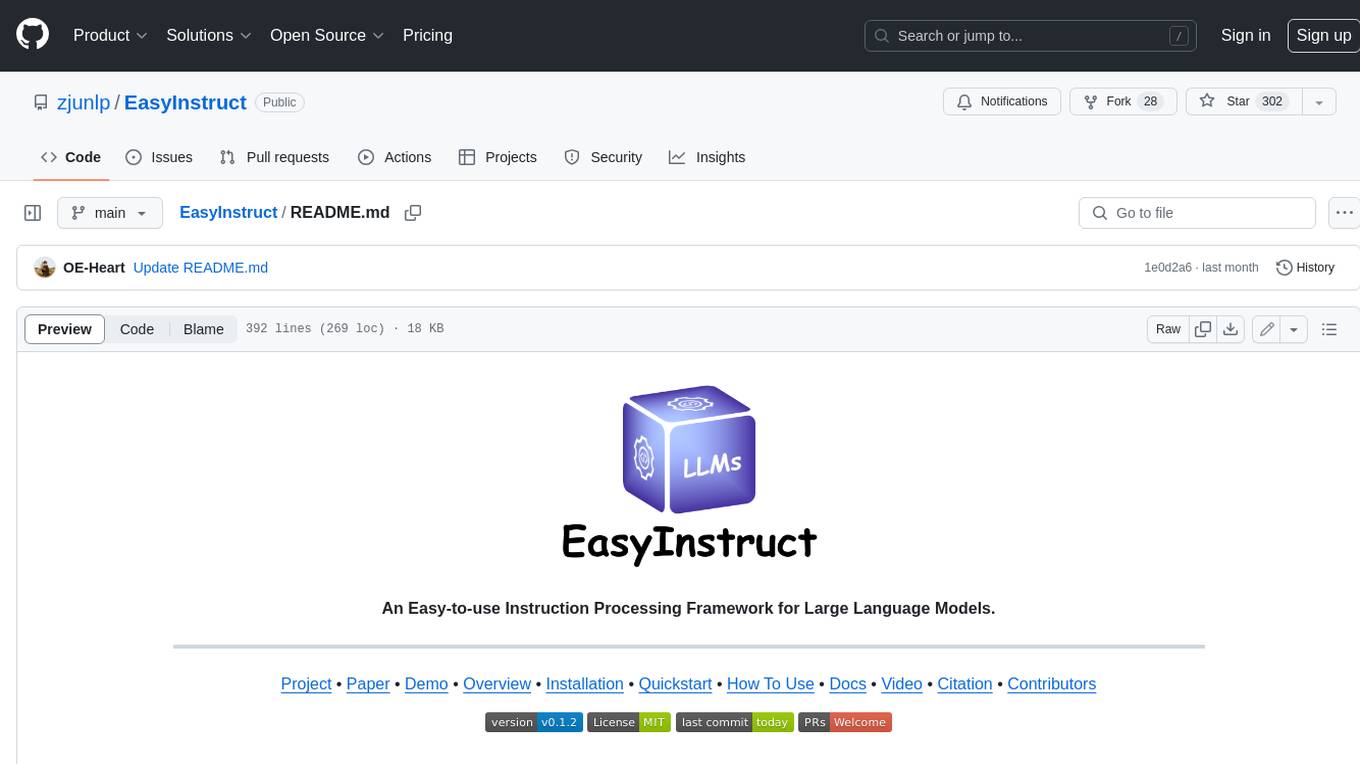
EasyInstruct
EasyInstruct is a Python package proposed as an easy-to-use instruction processing framework for Large Language Models (LLMs) like GPT-4, LLaMA, ChatGLM in your research experiments. EasyInstruct modularizes instruction generation, selection, and prompting, while also considering their combination and interaction.