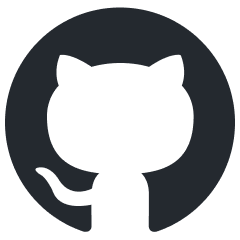
basiclingua-LLM-Based-NLP
LLM Based NLP Library.
Stars: 77
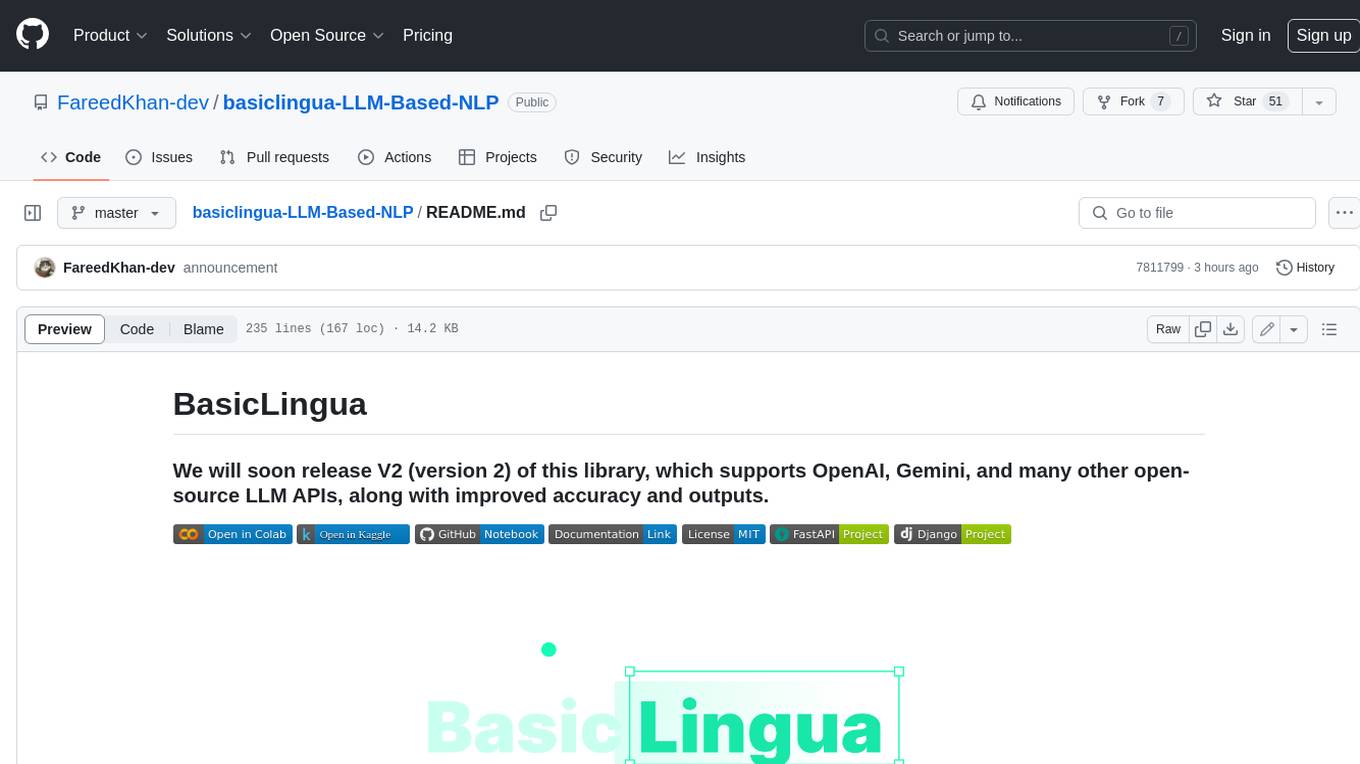
BasicLingua is a Python library that provides functionalities for linguistic tasks such as tokenization, stemming, lemmatization, and many others. It is based on the Gemini Language Model, which has demonstrated promising results in dealing with text data. BasicLingua can be used as an API or through a web demo. It is available under the MIT license and can be used in various projects.
README:
Created By: Fareed Khan
Basiclingua is a LLM based Python library that provides functionalities for linguistic tasks such as pattern extractions, intent recognition and many others, (Imagination is the limit).
- Why Building this Project?
- BasicLingua Architecture
- Evaluation Metrics
- AI Powered / Manual Documentation
- Tools and Technologies Used
- Updates
- Demo Video
- File Structure
- Installation / Update
- Initialization
- Supported LLMs
- Usage
- Features of the library
- Playground
- Acknowledgements
Natural Language Processing (NLP) has always been a challenging domain, requiring significant human effort and costs. Additionally, the data is becoming increasingly complex. By 2025, unstructured data, including vast amounts of text data, is expected to account for over 90% of the total data generated globally. Handling such data is expensive due to its ever-changing structure. However, recent advancements in AI, such as Large Language Models (LLMs), can process and understand text data more quickly and accurately than the human eye.
Our proposed solution, BasicLingua, is an NLP library in Python built on top of LLMs like LLaMA-3 and many others. It handles NLP tasks by processing only relevant text information, reducing costs, and providing efficient outputs similar to other text processing Python packages. BasicLingua is versatile and can be applied across various domains, from finance to healthcare, and any other field where text information is crucial. It offers endless possibilities and features, supported by AI-powered documentation. With the continuous growth of LLMs, such as LLaMA-3.1 and more recent powerful vision or text LLMs, the BasicLingua ecosystem has the potential to expand significantly.
One of the biggest challenges in building this architecture was ensuring the cost associated with using the features was minimal. To address this, we use effective prompt engineering
techniques to optimize the performance of the models and reduce the cost of using the library.
Here is one of our spellcheck
feature that uses OpenAI
model to correct spelling mistakes in text, the backend engineering is shown below:
The spellcheck feature takes the user input and passes it to the selected model, which then corrects the spelling mistakes in the text but only returns the corrected words which are then replaced in the original text. This approach ensures that only the necessary corrections are made, reducing the cost of using the feature.
Our library has been tested on a wide range of text data, including clinical notes, documents, and general text.
Dataset Category | Dataset Name | Description | Size | Source |
---|---|---|---|---|
Clinical Notes | MIMIC-IV | Medical Information Mart for Intensive Care | Random 3000 notes | MIMIC-IV |
Documents | Wikipedia Articles | Wikipedia articles on various topics | Random 1000 articles | Wikipedia |
General Text | AI generated text | Text generated by OpenAI and Gemini models | Random 5000 text samples | OpenAI, Gemini |
We evaluated the library for various linguistic tasks, benchmarking it against OpenAI, Gemini, and AnyScale models. The evaluation metrics used are as follows:
Task Name | Evaluation Metric | OpenAI (GPT-3.5) | Gemini (Gemini-1.0) | AnyScale (Llama-3-70b) |
---|---|---|---|---|
Information Extraction | Average F1 Score | 0.85 | 0.64 | 0.77 |
Analysis | Average Accuracy | 0.90 | 0.74 | 0.81 |
Summarization | ROUGE Score | 0.78 | 0.68 | 0.72 |
Coreference & Disambiguation | Average F1 Score | 0.89 | 0.73 | 0.84 |
Pre-Processing | Average Accuracy | 0.88 | 0.73 | 0.82 |
The evaluation metrics show that the library performs well across different tasks, with OpenAI achieving the highest scores in most tasks. We evaluated the library for various linguistic tasks, benchmarking it against OpenAI, Gemini, and AnyScale models. The evaluation metrics used are as follows:
Task Name | Evaluation Metric | OpenAI (GPT-3.5) | Gemini (Gemini-1.0) | AnyScale (Llama-3-70b) |
---|---|---|---|---|
Information Extraction | Average F1 Score | 0.85 | 0.64 | 0.77 |
Analysis | Average Accuracy | 0.90 | 0.74 | 0.81 |
Summarization | ROUGE Score | 0.78 | 0.68 | 0.72 |
Coreference & Disambiguation | Average F1 Score | 0.89 | 0.73 | 0.84 |
Pre-Processing | Average Accuracy | 0.88 | 0.73 | 0.82 |
The evaluation metrics show that the library performs well across different tasks, with OpenAI achieving the highest scores in most tasks. However, AnyScale's Llama-3-70b, an open-source model, demonstrates strong performance, closely matching the accuracy of other closed-source models. This is particularly noteworthy given its cost-effectiveness and accessibility. Additionally, it is anticipated that the upcoming Llama-3.1 will likely surpass the accuracy of the closed-source LLMs once it is integrated into version 3 of this library.
Given that our NLP library can handle a wide range of domain-related tasks, it is crucial to provide an AI-powered documentation for BasicLingua. This documentation allows developers to ask questions related to the library, and it responds promptly by offering the exact features they need, along with examples. Additionally, it can answer other common queries, such as how to get started with the library and much more.
Given that our NLP library is based on LLMs, it is crucial to provide an AI-powered documentation for BasicLingua. Makes the library more efficient to use and understand.
AI Documentation Webapp is available at -
You can also access the manual documentation within your code editor which contains parameters details, return types and examples of each function and more.
- Programming Language: Python
- Libraries: OpenAI, Google generative ai, AnyScale, Streamlit
- Deployment: Streamlit Sharing, PyPi
- Version Control: Git, GitHub
- Documentation: AI-Powered Documentation
- IDE: Jupyter Notebook, Visual Studio Code
-
2024/4/20
We have released the second version of the library. The new version includes additional features and new LLMs for text and vision tasks. Our library now supportsOpenAI
,Gemini
, andAnyScale
models for various linguistic tasks. We have also improved the performance of the library and added more functionalities to make it more versatile and user-friendly. -
2024/4/16
We have added the AI-powered documentation for the library. The documentation is now available for use. We are currently working on improving the documentation and adding more features to the library. -
2024/3/3
We have released the first version of the library. The library is now available for use. We are currently working on the documentation and the next version of the library. We are also working on the integration of the library with other LLMs. -
2024/1/10
We have released the baby version of this library containing limited number of pre-processing features.
Project demo video showcasing some of the functionalities provided by the library.
The project files are organized as follows:
├── Demo
│ ├── demo_video.mp4 <-- Demo Video
├── Document <-- Containing the pdf of the project overview document
├── Tutorial
│ ├── Guide.ipynb <-- Jupyter Notebook for the library guide
├── backend-engineering
│ ├── OpenAI.py <-- OpenAI Backend Engineering
│ ├── Gemini.py <-- Gemini Backend Engineering
│ ├── AnyScale.py <-- AnyScale Backend Engineering (Open-Source LLMs)
├── images <-- Images used in the README
├── LICENSE <-- MIT License
├── README.md <-- Project README
Before installing BasicLingua, ensure that you have Python installed on your system. BasicLingua tested on Python 3.9
or greater. Earlier version may work but not guaranteed. To check your Python version, run the following command in your terminal:
python --version
If you don't have Python installed or need to upgrade, visit the official Python website to download and install the latest version.
Once Python is set up, you can install BasicLingua using pip
:
pip install basiclingua
or you can upgrade to the latest version using:
pip install --upgrade basiclingua
After installing BasicLingua, you need to import the models you want to use. You can choose to import specific models or all available models.
Import a Specific Model
# Importing OpenAI Model
from basiclingua import OpenAILingua
# Importing Google Gemini Model
from basiclingua import GeminiLingua
# Importing Anyscale Model
from basiclingua import AnyScaleLingua
Import All Models at Once
from basiclingua import OpenAILingua, GeminiLingua, AnyScaleLingua
Option 1 - Since this project is for AI Challenge 2024,you can contact us at [email protected] to get the API keys for OpenAI, Gemini and AnyScale for testing purposes.
Option 2 - You can create your own API keys from the respective platforms.
- Get your OpenAI API Key from OpenAI Platform
- Get your Gemini api key from Gemini Platform
- Get anyscale api key from AnyScale Platform
Before using any model, you must set the API key for that specific platform. This is a mandatory step. Each model class has a constructor that takes the API key and optional additional parameters, such as model names.
For Initializing OpenAI
# Initializing OpenAI Model
openai_model = OpenAILingua(
api_key="YOUR_OPENAI_API_KEY", # Your OpenAI API Key
model_name='gpt-3.5-turbo-0125', # Text Model Name
vision_model_name='gpt-4-turbo' # Vision Model Name
)
Default models are gpt-3.5-turbo-0125
and gpt-4-turbo
for text and vision respectively.
For Initializing Gemini
# Initializing Gemini Model
gemini_model = GeminiLingua(
api_key="YOUR_GEMINI_API_KEY", # Your Gemini API Key
model_name='gemini-1.0-pro-latest', # Text Model Name
vision_model_name='models/gemini-1.5-pro-latest' # Vision Model Name
)
Default models are gemini-1.0-pro-latest
and gemini-1.5-pro-latest
for text and vision respectively.
For Initializing AnyScale (LLaMA-3, Mistral etc)
# Initializing AnyScale Model
anyscale_model = AnyScaleLingua(
api_key="YOUR_ANY_SCALE_API_KEY", # Your AnyScale API Key
model_name="meta-llama/Llama-3-70b-chat-hf" # Text Model Name
)
Default model is meta-llama/Llama-3-70b-chat-hf
.
For OpenAILingua
, all text and vision models are supported as available on the OpenAI Platform.
For GeminiLingua
, all text and vision models are supported as available on the Gemini Platform.
A complete list of open-source supported models under AnyScaleLingua
is available on the AnyScale Platform.
Default models are:
Source | Text Model | Vision Model | Embedding Model |
---|---|---|---|
OpenAI | gpt-3.5-turbo-0125 |
gpt-4-turbo |
text-embedding-3-large |
Gemini | gemini-1.0-pro-latest |
gemini-1.5-pro-latest |
models/embedding-001 |
AnyScale | meta-llama/Llama-3-70b-chat-hf |
meta-llama/Llama-3-70b-chat-hf |
thenlper/gte-large |
The library provides a wide range of functionalities for linguistic tasks some of which are mentioned below. You can use our AI-powered documentation to learn more about the functionalities provided by the library.
Entity extraction is crucial for transforming unstructured text into structured data, enabling efficient analysis and automation in fields like finance, healthcare, and cybersecurity. However, it can be challenging due to the complexity and ambiguity of language, which often requires intricate regex or NLP techniques.
Regex-based entity extraction is time-consuming due to its detailed pattern definitions, but with our approach, you only need to define the pattern name to extract entities with minimal effort. Here's an example of extracting ICD (International Classification of Diseases) codes:
# ClinicalNote with complex structure and formatting
user_input = """Patient John, last name: Doe; 45 yrs
Symptoms: fatigue + frequent urination (possible diabetes); dizziness
Diagnosis - Type 2 Diabetes (E11.9), Hypertension (I10)
Prescribed: Metformin @ 500mg/day; Amlodipine, twice a day
Allergic: PCN (penicillin)
Family history of diabetes and HBP (high blood pressure)
Additional notes: testing for cholesterol and kidney function
Patient was advised to monitor blood sugar levels regularly.
Mentioned: Father - Type 2 Diabetes; Mother - Hypertension
Description - T2 Diabetes without complications; Essential Hypertension."""
# Define the patterns to extract
patterns = "ICD-10 Codes, Diseases, Medications, Allergies, Symptoms, Family History, Descriptions"
# Using OpenAI to extract entities
openai_entities = anyscale_model.extract_patterns(user_input, patterns=patterns)
# Displaying the extracted entities
print(openai_entities)
######## Output ########
{
"ICD-10 Codes": ["E11.9", "I10"],
"Diseases": ["Type 2 Diabetes", "Hypertension"],
"Medications": ["Metformin", "Amlodipine"],
"Allergies": ["Penicillin"],
"Symptoms": ["fatigue", "frequent urination", "dizziness"],
"Family History": ["Father with Type 2 Diabetes", "Mother with Hypertension"],
"Descriptions": [
"Type 2 Diabetes without complications",
"Essential (primary) hypertension",
"testing for cholesterol and kidney function"
]
}
######## Output ########
Similarly, Text coreference is difficult because it involves figuring out which words refer to the same person or thing in a sentence or a text. It requires a deep understanding of context and the way language is used to connect different parts of a text.
Here's an example of how BasicLingua
can help you resolve coreferences in a text:
# User input with complex co-references
user_input = """
Jane and her colleague Tom were preparing for the upcoming meeting with the new clients. She had worked on the presentation slides, while he focused on the data analysis.
When the day of the meeting arrived, Jane noticed that the projector was not working properly, so she asked Tom to check it out.
He found that it needed a new cable, but they had none in the office. They had to improvise with a laptop. During the presentation, Jane felt nervous because the setup wasn't ideal, but Tom reassured her that everything would be fine.
The clients appreciated their efforts, and both Jane and Tom were relieved when the meeting concluded successfully. As they left, Jane told Tom that she was grateful for his support.
"""
# Using AnyScale model to resolve coreferences
anyscale_coref = anyscale_model.text_coreference(user_input)
# Displaying the resolved coreferences
print("AnyScale Coreference:", anyscale_coref)
######## Output ########
{
"she": "Jane",
"he": "Tom",
"they": ["Jane", "Tom"],
"her": "Jane",
"him": "Tom"
}
######## Output ########
There are many other functionalities provided by the library that can help you with various linguistic tasks. You can refer to our AI-powered documentation that can help you understand the functionalities of the library and how to use them effectively.
There are more than 20 functionalities provided by the library. But due to their effectiveness across different domains, we have created an AI-powered documentation to help you understand the functionalities in a more broadened way.
Function Name | Python Function Name | Parameters | Returns |
---|---|---|---|
Extract Patterns | extract_patterns |
user_input , patterns
|
A JSON of extracted patterns from the input sentence |
Detect NER | detect_ner |
user_input , ner_tags
|
A JSON of detected Named Entity Recognition (NER) entities |
Text Intent | text_intent |
user_input |
A list of identified intents from the input sentence |
. . . | . . . | . . . | . . . |
You can explore more by chatting with our Documentation Chatbot to get a better understanding of the functionalities provided by the library.
Since this library is available under the MIT license
, you can use it in your projects. All the backend code is available in the backend-engineering folder.
- Rohan Anil et al., "Gemini: A Family of Highly Capable Multimodal Models", arXiv, April 2024. DOI: 10.48550/arXiv.2312.11805
- OpenAI Team. (2024). OpenAI GPT-3.5: The Next Evolution of Language Models. OpenAI Blog. https://openai.com/blog/gpt-3-5
- Meta AI. (2024, April 18). Introducing Meta Llama 3: The most capable openly available LLM to date. Meta AI Blog, from https://ai.meta.com/blog/meta-llama-3
- Ye, Q., Axmed, M., Pryzant, R., & Khani, F. (2024). Prompt engineering a prompt engineer. arXiv. https://doi.org/10.48550/arXiv.2311.05661
For Tasks:
Click tags to check more tools for each tasksFor Jobs:
Alternative AI tools for basiclingua-LLM-Based-NLP
Similar Open Source Tools
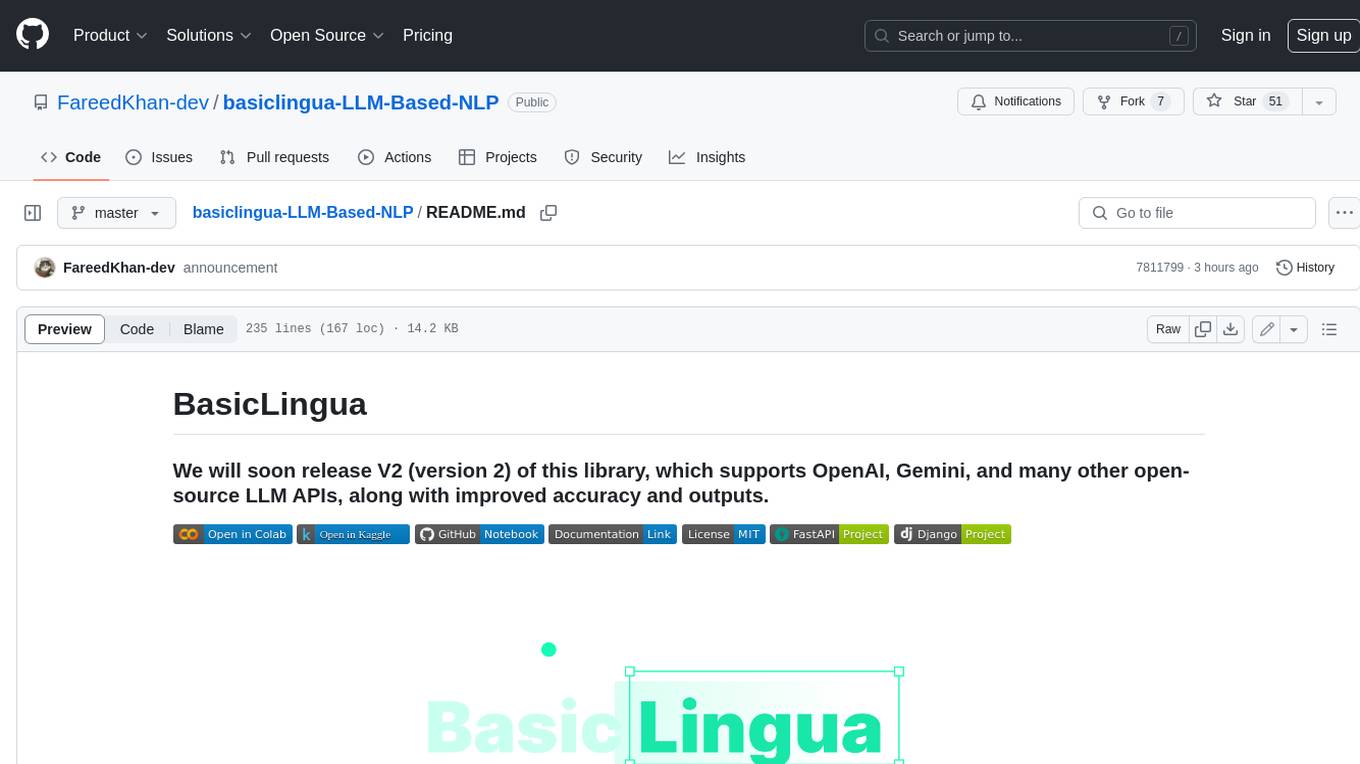
basiclingua-LLM-Based-NLP
BasicLingua is a Python library that provides functionalities for linguistic tasks such as tokenization, stemming, lemmatization, and many others. It is based on the Gemini Language Model, which has demonstrated promising results in dealing with text data. BasicLingua can be used as an API or through a web demo. It is available under the MIT license and can be used in various projects.
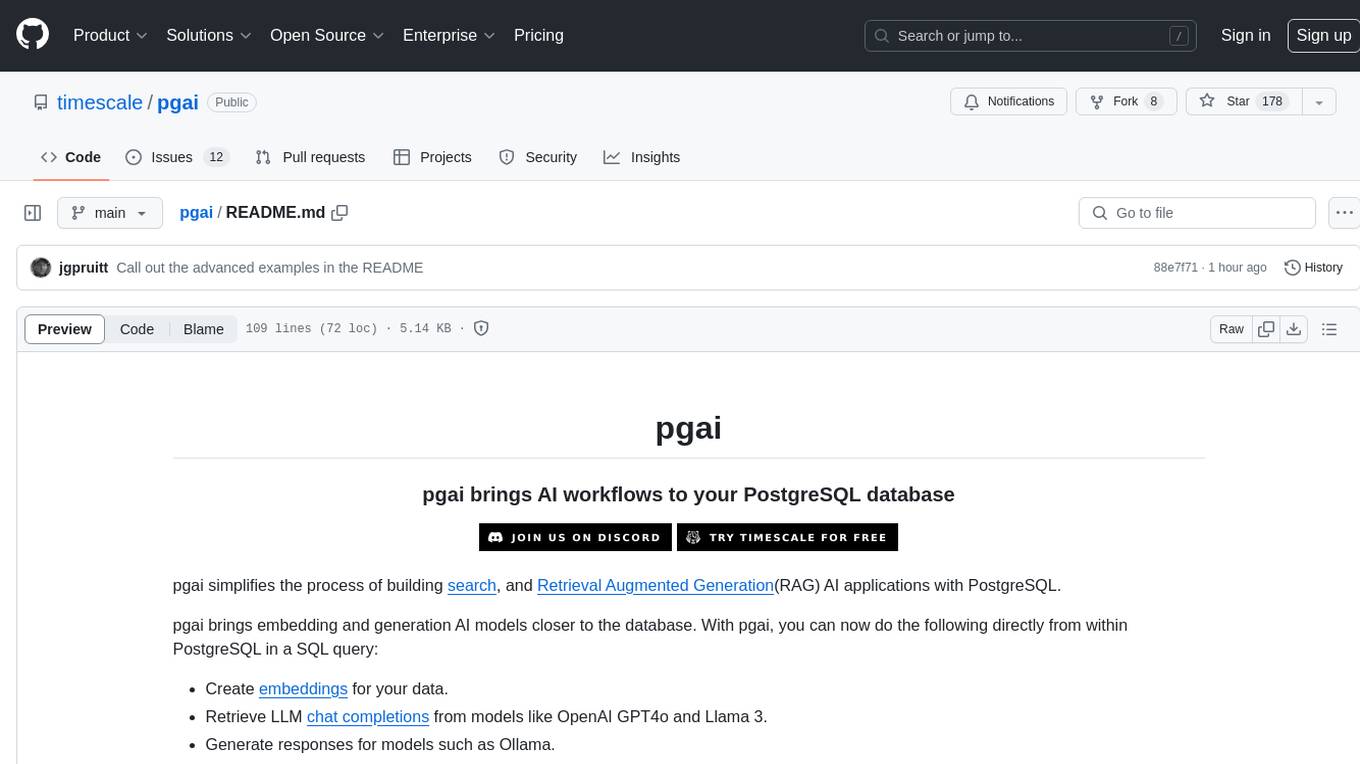
pgai
pgai simplifies the process of building search and Retrieval Augmented Generation (RAG) AI applications with PostgreSQL. It brings embedding and generation AI models closer to the database, allowing users to create embeddings, retrieve LLM chat completions, reason over data for classification, summarization, and data enrichment directly from within PostgreSQL in a SQL query. The tool requires an OpenAI API key and a PostgreSQL client to enable AI functionality in the database. Users can install pgai from source, run it in a pre-built Docker container, or enable it in a Timescale Cloud service. The tool provides functions to handle API keys using psql or Python, and offers various AI functionalities like tokenizing, detokenizing, embedding, chat completion, and content moderation.
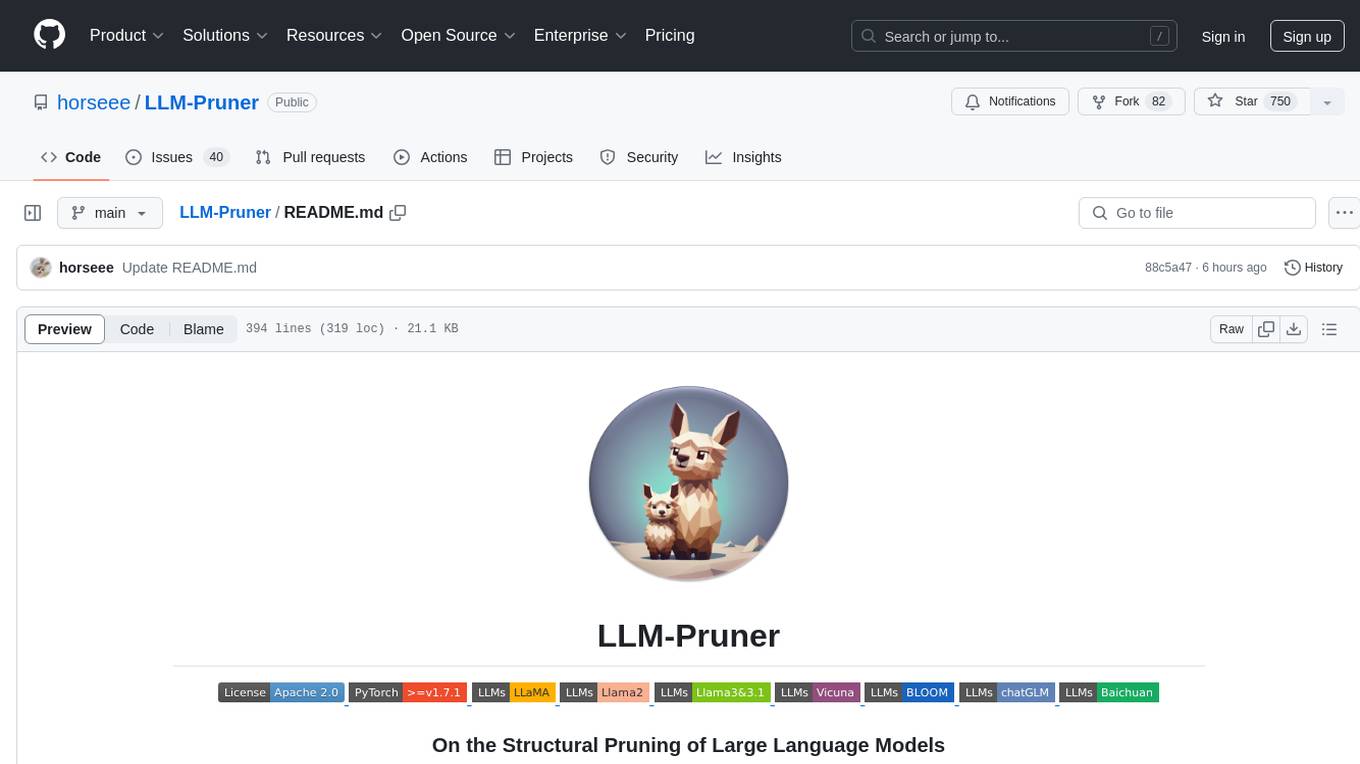
LLM-Pruner
LLM-Pruner is a tool for structural pruning of large language models, allowing task-agnostic compression while retaining multi-task solving ability. It supports automatic structural pruning of various LLMs with minimal human effort. The tool is efficient, requiring only 3 minutes for pruning and 3 hours for post-training. Supported LLMs include Llama-3.1, Llama-3, Llama-2, LLaMA, BLOOM, Vicuna, and Baichuan. Updates include support for new LLMs like GQA and BLOOM, as well as fine-tuning results achieving high accuracy. The tool provides step-by-step instructions for pruning, post-training, and evaluation, along with a Gradio interface for text generation. Limitations include issues with generating repetitive or nonsensical tokens in compressed models and manual operations for certain models.
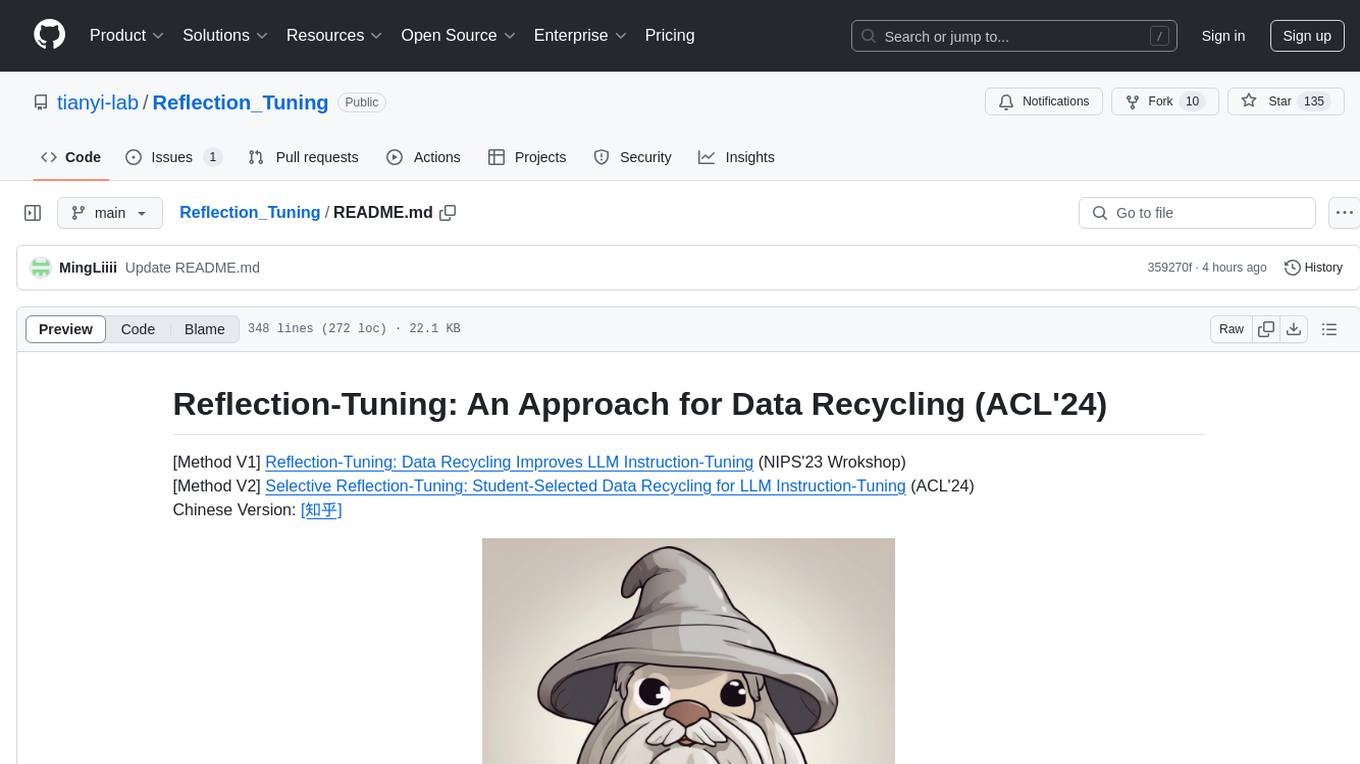
Reflection_Tuning
Reflection-Tuning is a project focused on improving the quality of instruction-tuning data through a reflection-based method. It introduces Selective Reflection-Tuning, where the student model can decide whether to accept the improvements made by the teacher model. The project aims to generate high-quality instruction-response pairs by defining specific criteria for the oracle model to follow and respond to. It also evaluates the efficacy and relevance of instruction-response pairs using the r-IFD metric. The project provides code for reflection and selection processes, along with data and model weights for both V1 and V2 methods.
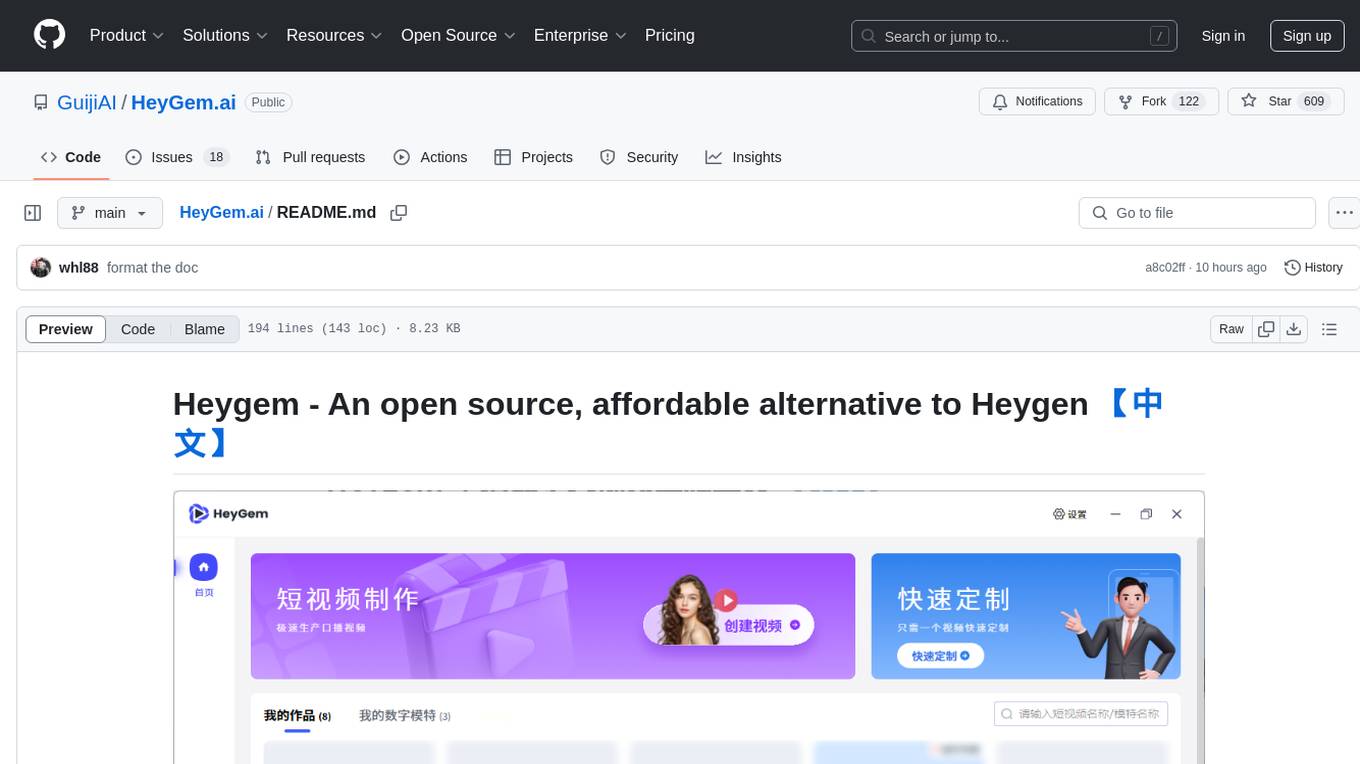
HeyGem.ai
Heygem is an open-source, affordable alternative to Heygen, offering a fully offline video synthesis tool for Windows systems. It enables precise appearance and voice cloning, allowing users to digitalize their image and drive virtual avatars through text and voice for video production. With core features like efficient video synthesis and multi-language support, Heygem ensures a user-friendly experience with fully offline operation and support for multiple models. The tool leverages advanced AI algorithms for voice cloning, automatic speech recognition, and computer vision technology to enhance the virtual avatar's performance and synchronization.
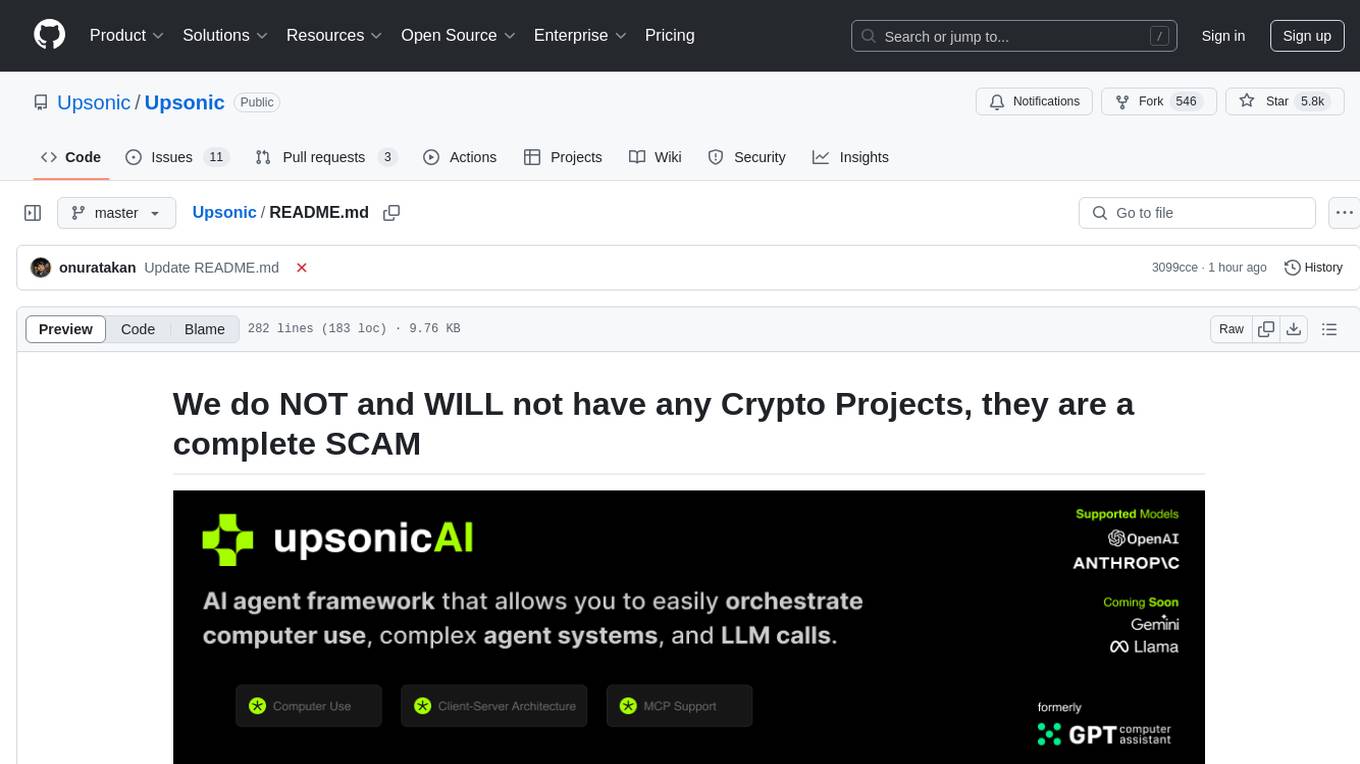
Upsonic
Upsonic offers a cutting-edge enterprise-ready framework for orchestrating LLM calls, agents, and computer use to complete tasks cost-effectively. It provides reliable systems, scalability, and a task-oriented structure for real-world cases. Key features include production-ready scalability, task-centric design, MCP server support, tool-calling server, computer use integration, and easy addition of custom tools. The framework supports client-server architecture and allows seamless deployment on AWS, GCP, or locally using Docker.
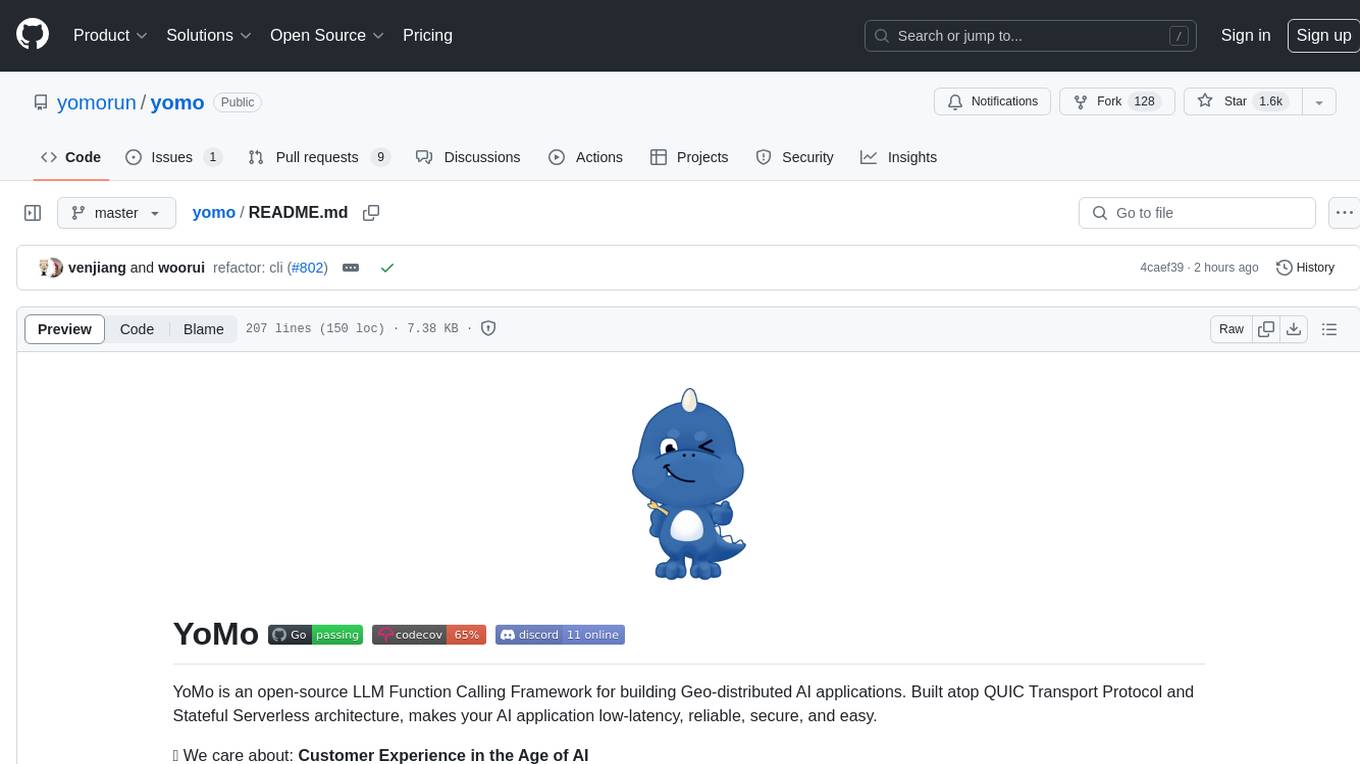
yomo
YoMo is an open-source LLM Function Calling Framework for building Geo-distributed AI applications. It is built atop QUIC Transport Protocol and Stateful Serverless architecture, making AI applications low-latency, reliable, secure, and easy. The framework focuses on providing low-latency, secure, stateful serverless functions that can be distributed geographically to bring AI inference closer to end users. It offers features such as low-latency communication, security with TLS v1.3, stateful serverless functions for faster GPU processing, geo-distributed architecture, and a faster-than-real-time codec called Y3. YoMo enables developers to create and deploy stateful serverless functions for AI inference in a distributed manner, ensuring quick responses to user queries from various locations worldwide.
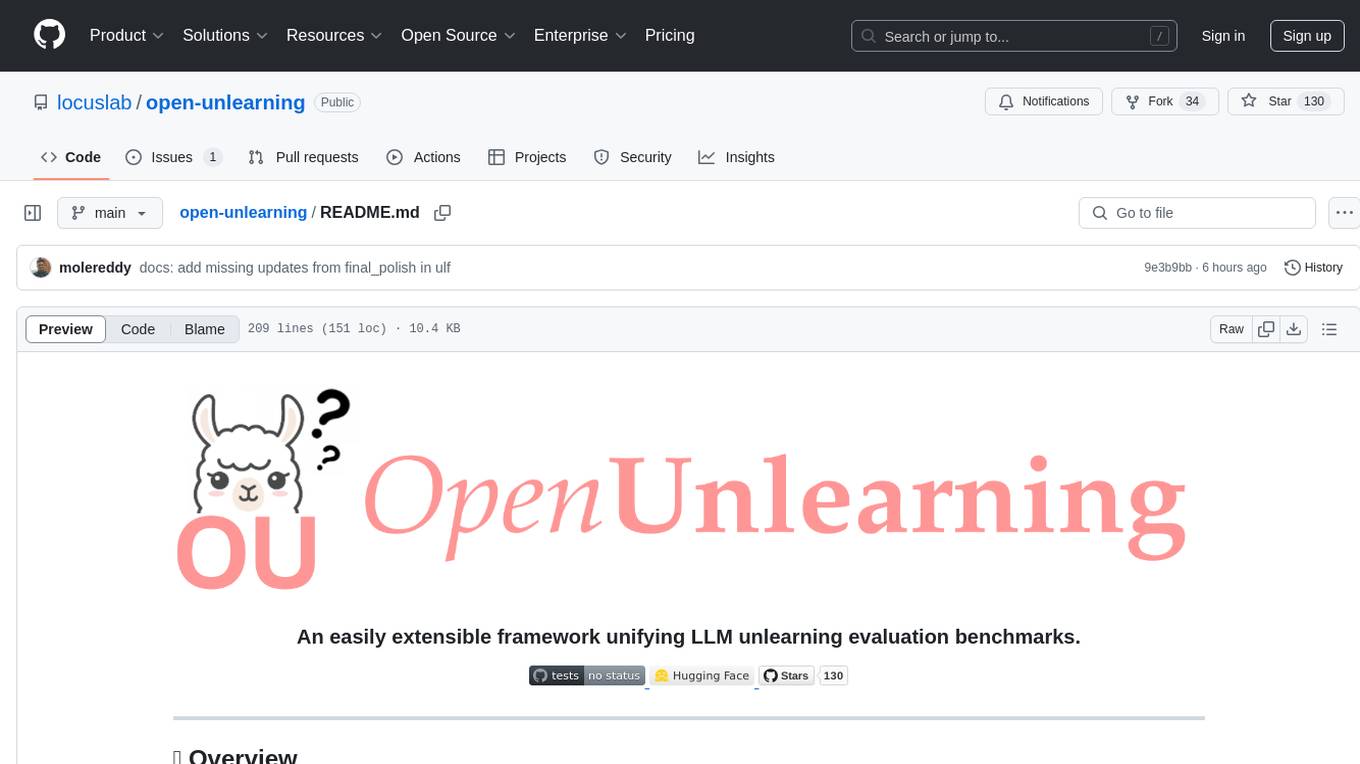
open-unlearning
OpenUnlearning is an easily extensible framework that unifies LLM unlearning evaluation benchmarks. It provides efficient implementations of TOFU and MUSE unlearning benchmarks, supporting 5 unlearning methods, 3+ datasets, 6+ evaluation metrics, and 7+ LLMs. Users can easily extend the framework to incorporate more variants, collaborate by adding new benchmarks, unlearning methods, datasets, and evaluation metrics, and drive progress in the field.
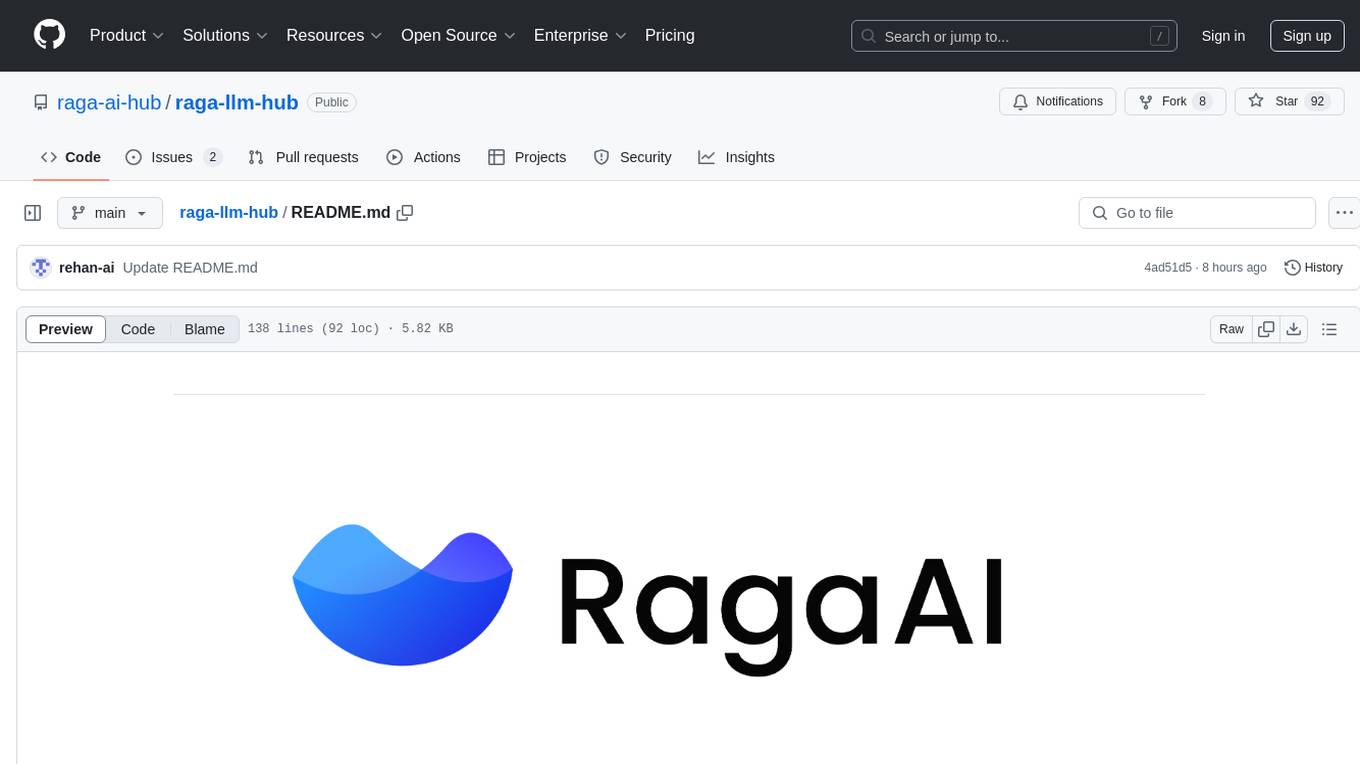
raga-llm-hub
Raga LLM Hub is a comprehensive evaluation toolkit for Language and Learning Models (LLMs) with over 100 meticulously designed metrics. It allows developers and organizations to evaluate and compare LLMs effectively, establishing guardrails for LLMs and Retrieval Augmented Generation (RAG) applications. The platform assesses aspects like Relevance & Understanding, Content Quality, Hallucination, Safety & Bias, Context Relevance, Guardrails, and Vulnerability scanning, along with Metric-Based Tests for quantitative analysis. It helps teams identify and fix issues throughout the LLM lifecycle, revolutionizing reliability and trustworthiness.
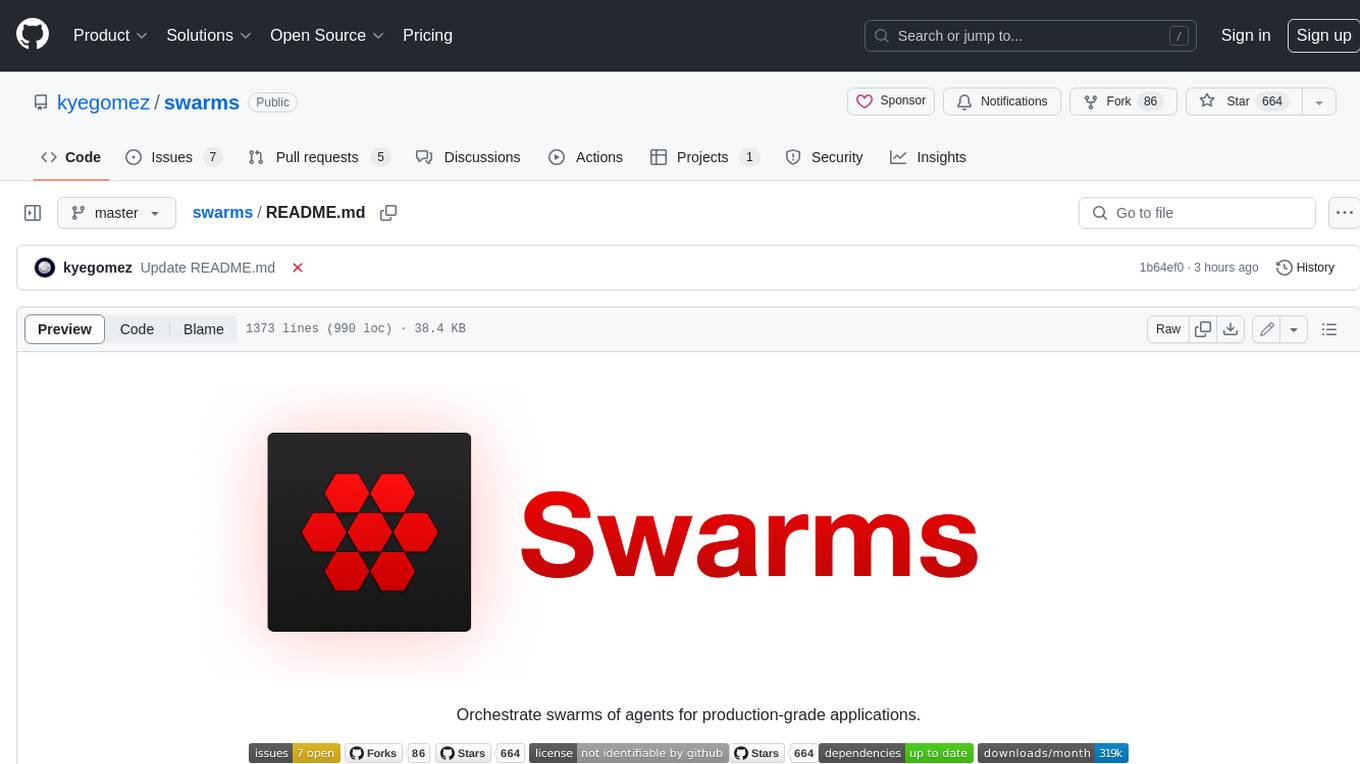
swarms
Swarms provides simple, reliable, and agile tools to create your own Swarm tailored to your specific needs. Currently, Swarms is being used in production by RBC, John Deere, and many AI startups.
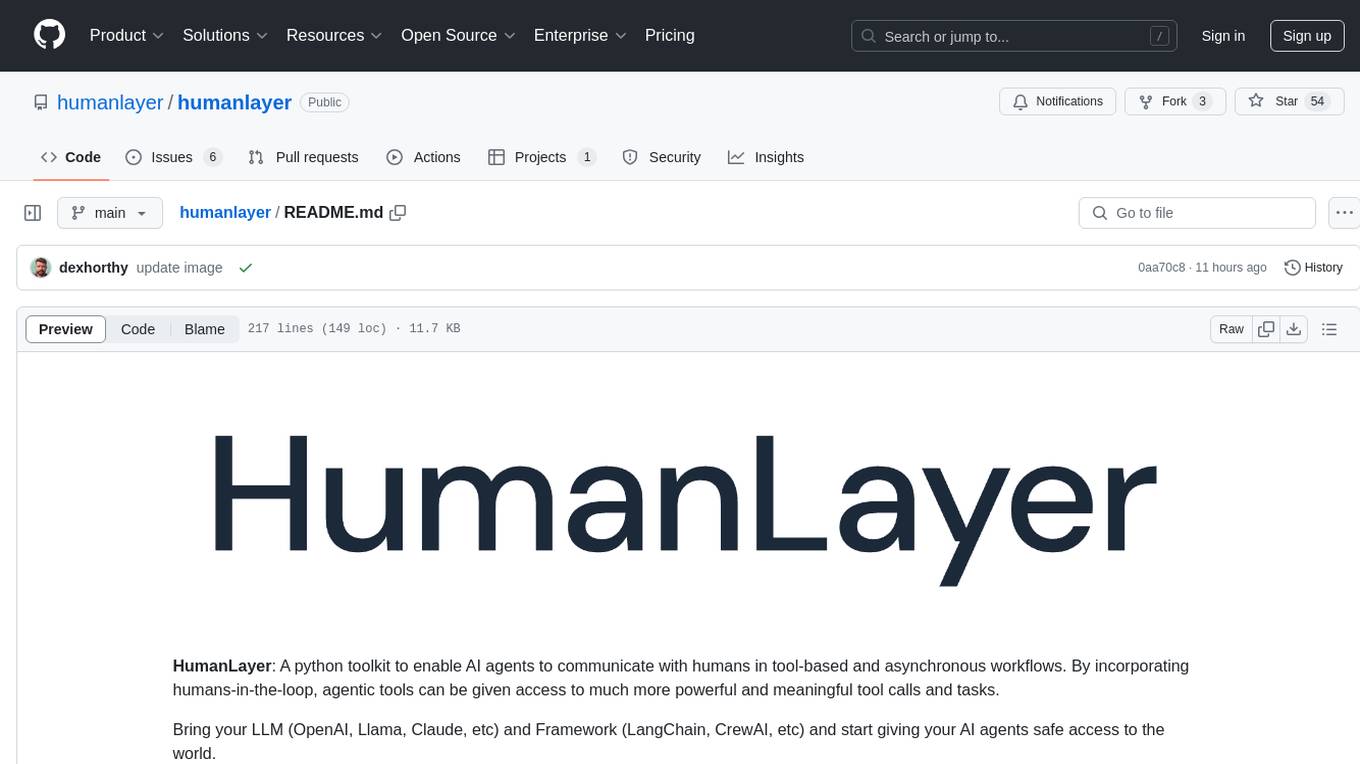
humanlayer
HumanLayer is a Python toolkit designed to enable AI agents to interact with humans in tool-based and asynchronous workflows. By incorporating humans-in-the-loop, agentic tools can access more powerful and meaningful tasks. The toolkit provides features like requiring human approval for function calls, human as a tool for contacting humans, omni-channel contact capabilities, granular routing, and support for various LLMs and orchestration frameworks. HumanLayer aims to ensure human oversight of high-stakes function calls, making AI agents more reliable and safe in executing impactful tasks.
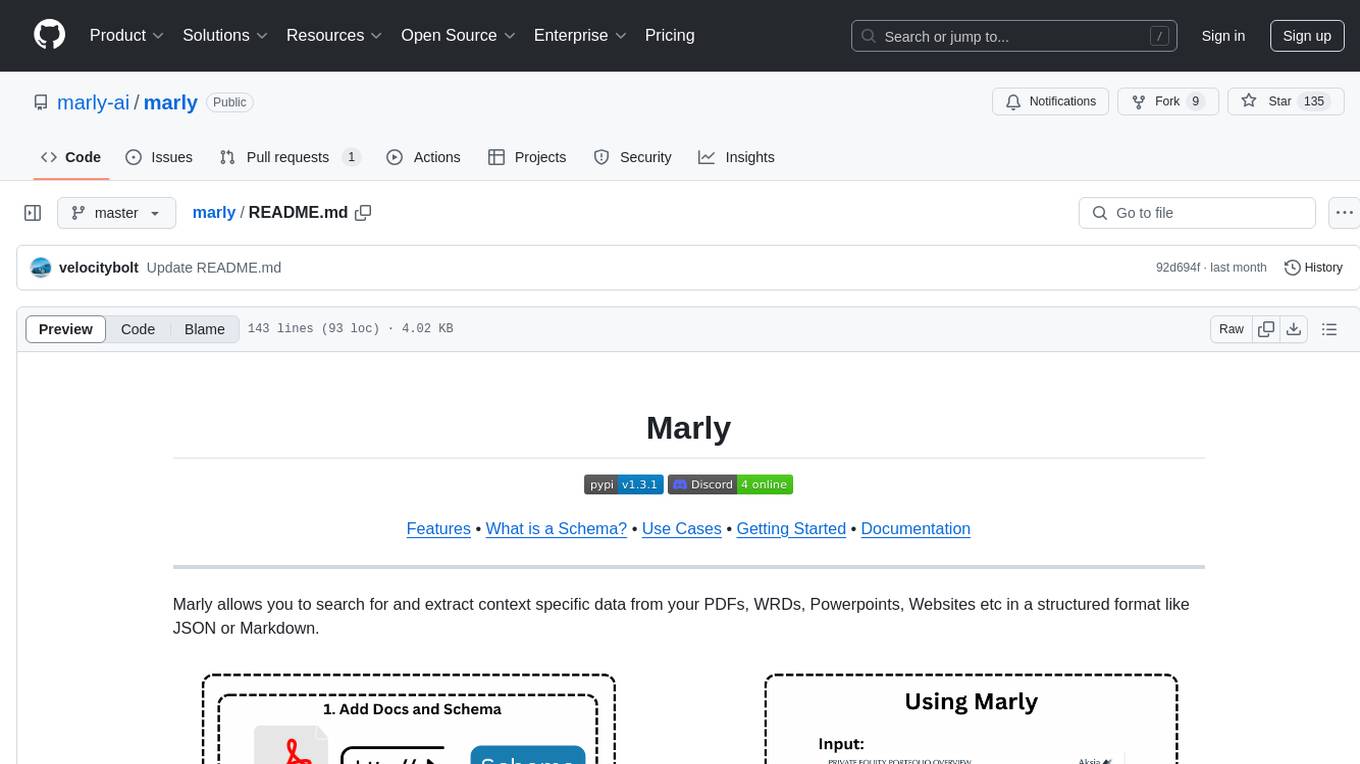
marly
Marly is a tool that allows users to search for and extract context-specific data from various types of documents such as PDFs, Word files, Powerpoints, and websites. It provides the ability to extract data in structured formats like JSON or Markdown, making it easy to integrate into workflows. Marly supports multi-schema and multi-document extraction, offers built-in caching for rapid repeat extractions, and ensures no vendor lock-in by allowing flexibility in choosing model providers.
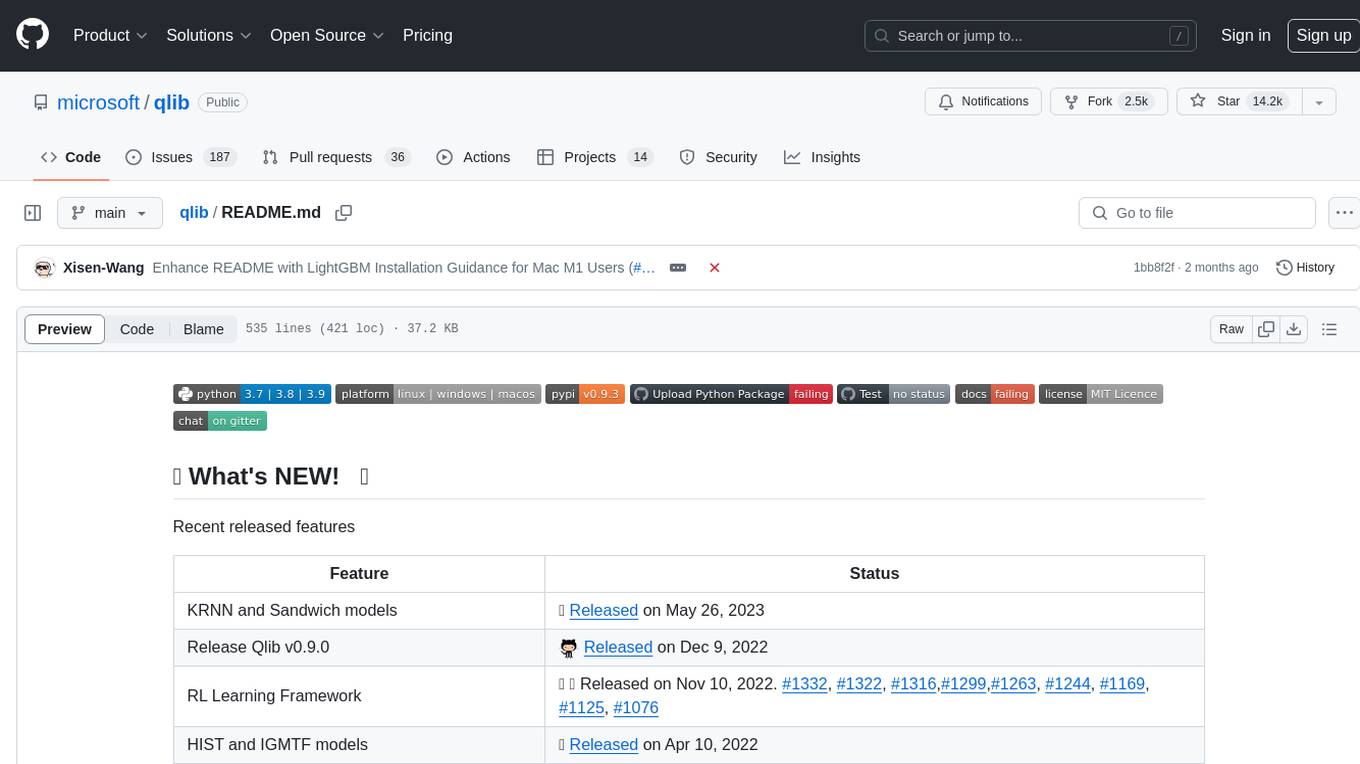
qlib
Qlib is an open-source, AI-oriented quantitative investment platform that supports diverse machine learning modeling paradigms, including supervised learning, market dynamics modeling, and reinforcement learning. It covers the entire chain of quantitative investment, from alpha seeking to order execution. The platform empowers researchers to explore ideas and implement productions using AI technologies in quantitative investment. Qlib collaboratively solves key challenges in quantitative investment by releasing state-of-the-art research works in various paradigms. It provides a full ML pipeline for data processing, model training, and back-testing, enabling users to perform tasks such as forecasting market patterns, adapting to market dynamics, and modeling continuous investment decisions.
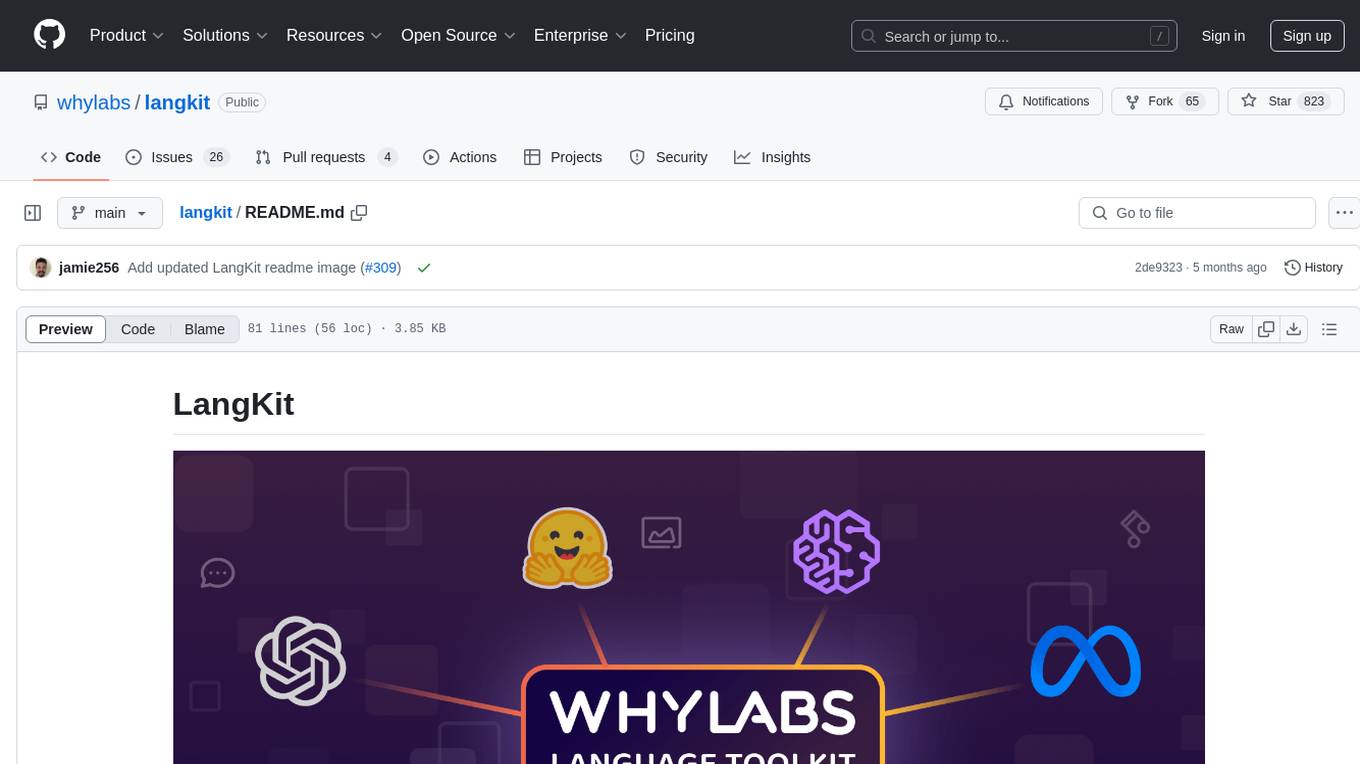
langkit
LangKit is an open-source text metrics toolkit for monitoring language models. It offers methods for extracting signals from input/output text, compatible with whylogs. Features include text quality, relevance, security, sentiment, toxicity analysis. Installation via PyPI. Modules contain UDFs for whylogs. Benchmarks show throughput on AWS instances. FAQs available.
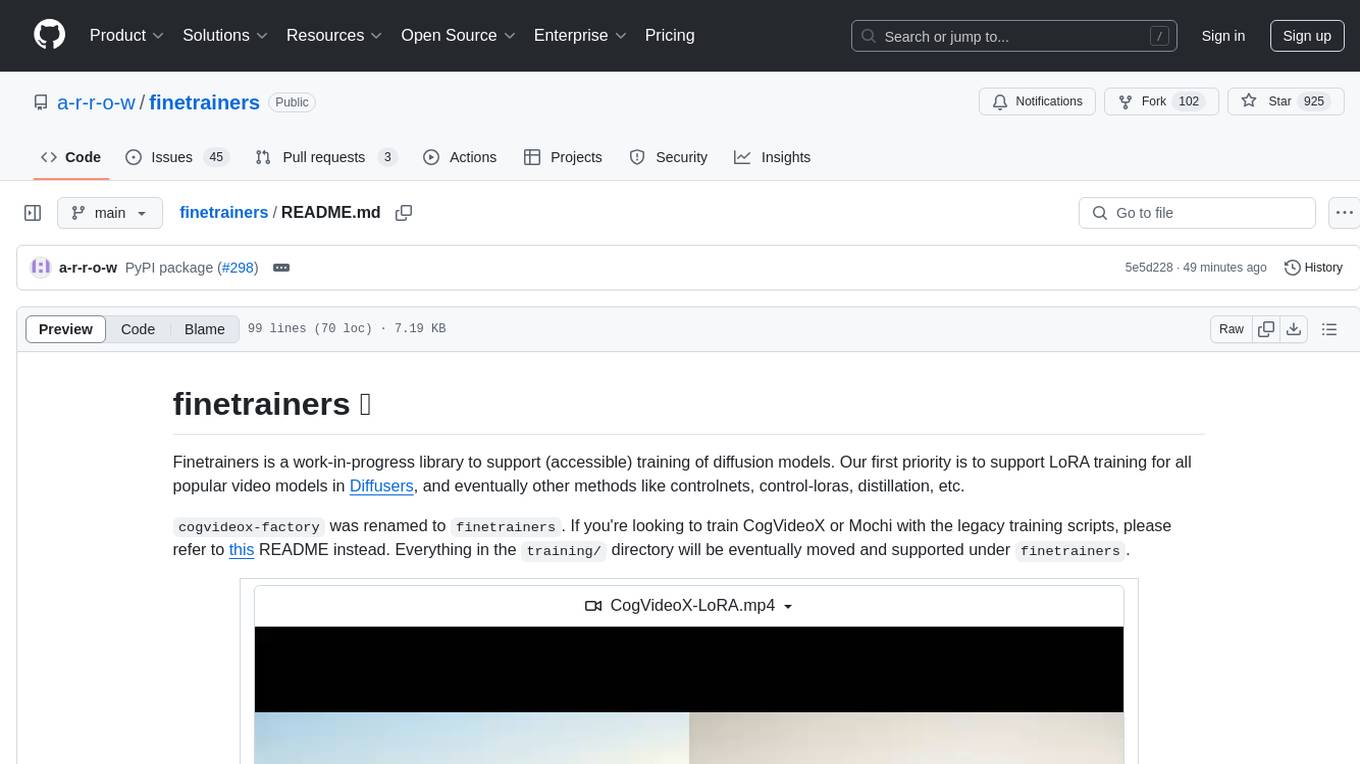
finetrainers
FineTrainers is a work-in-progress library designed to support the training of video models, with a focus on LoRA training for popular video models in Diffusers. It aims to eventually extend support to other methods like controlnets, control-loras, distillation, etc. The library provides tools for training custom models, handling big datasets, and supporting multi-backend distributed training. It also offers tooling for curating small and high-quality video datasets for fine-tuning.
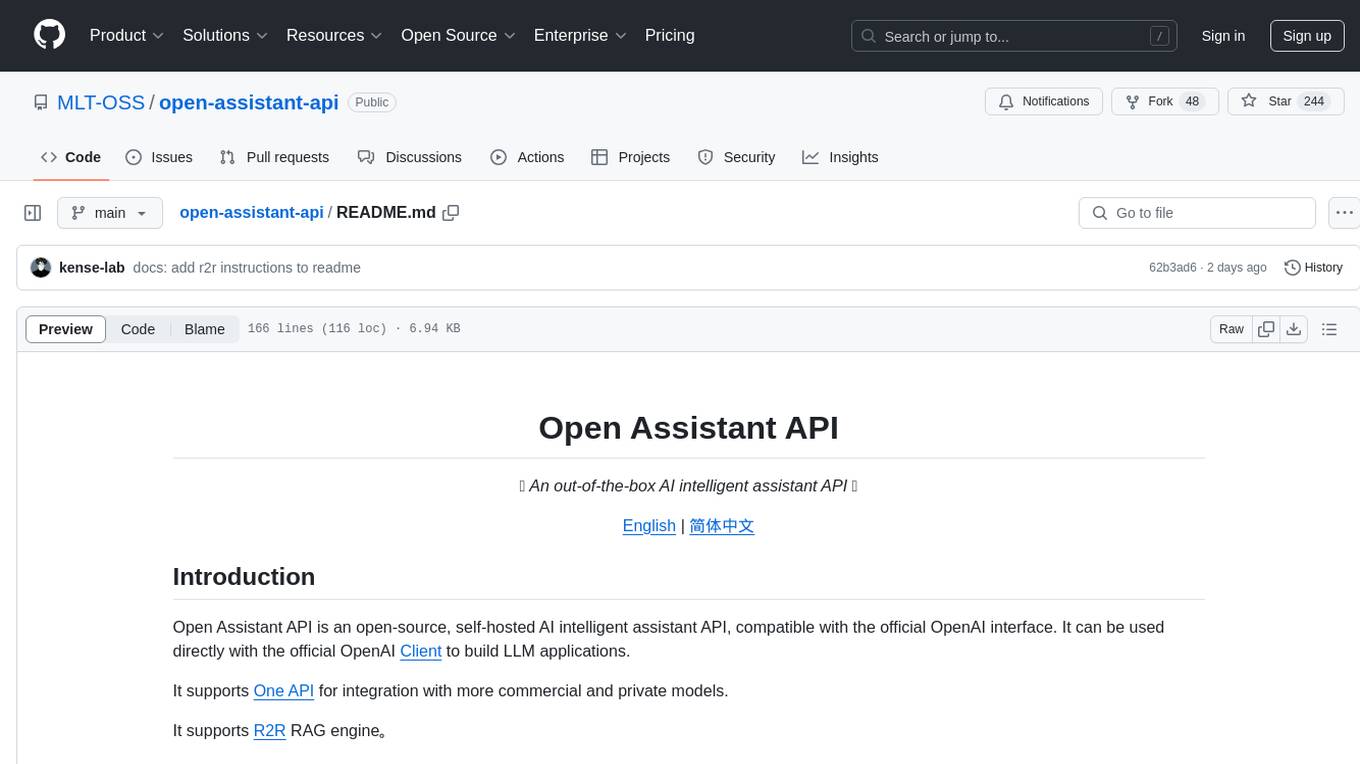
open-assistant-api
Open Assistant API is an open-source, self-hosted AI intelligent assistant API compatible with the official OpenAI interface. It supports integration with more commercial and private models, R2R RAG engine, internet search, custom functions, built-in tools, code interpreter, multimodal support, LLM support, and message streaming output. Users can deploy the service locally and expand existing features. The API provides user isolation based on tokens for SaaS deployment requirements and allows integration of various tools to enhance its capability to connect with the external world.
For similar tasks
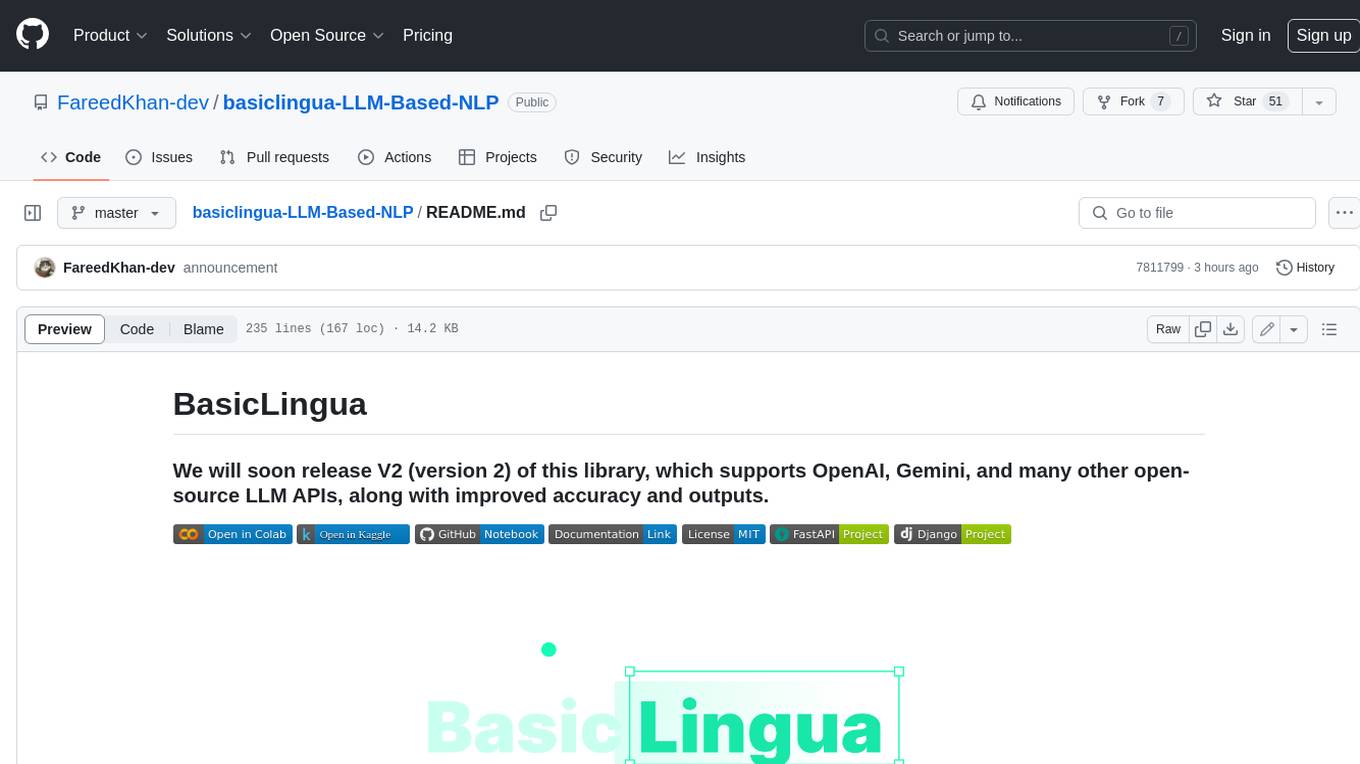
basiclingua-LLM-Based-NLP
BasicLingua is a Python library that provides functionalities for linguistic tasks such as tokenization, stemming, lemmatization, and many others. It is based on the Gemini Language Model, which has demonstrated promising results in dealing with text data. BasicLingua can be used as an API or through a web demo. It is available under the MIT license and can be used in various projects.
For similar jobs
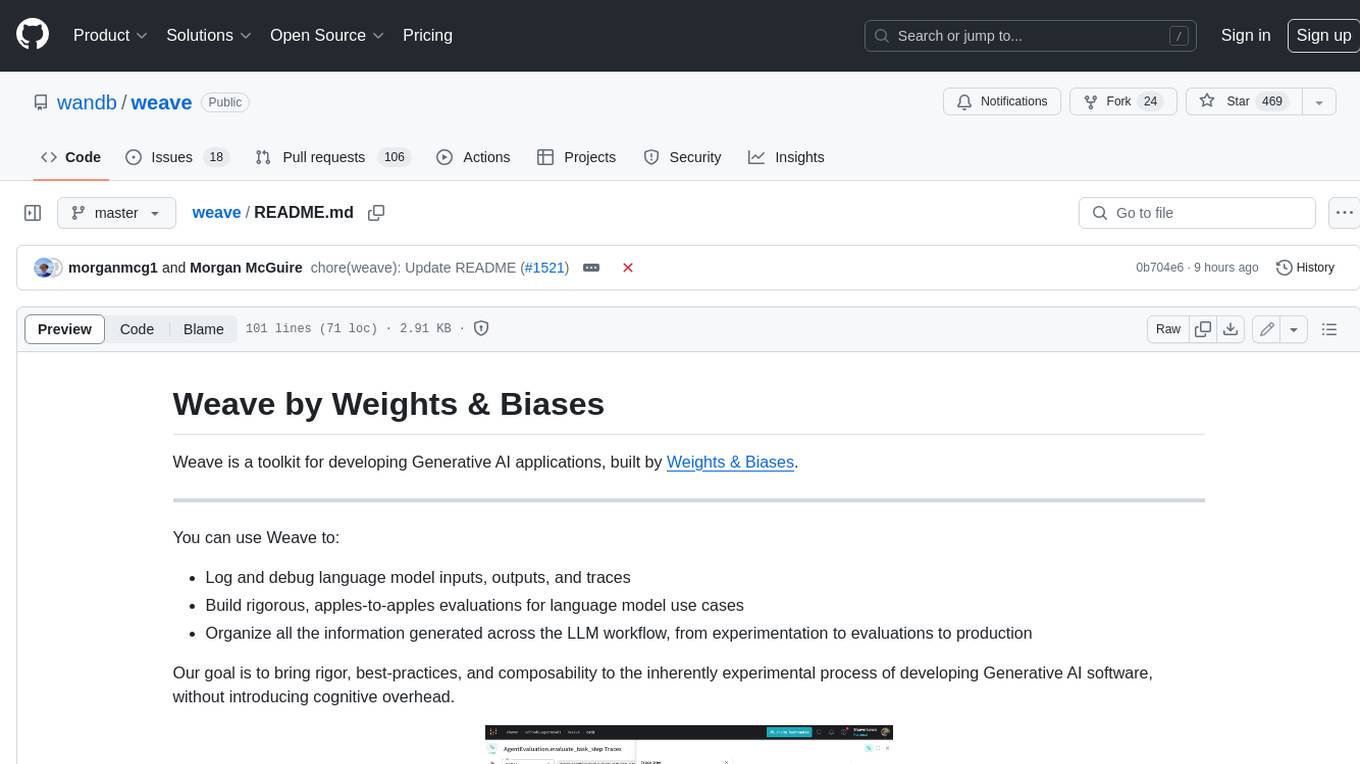
weave
Weave is a toolkit for developing Generative AI applications, built by Weights & Biases. With Weave, you can log and debug language model inputs, outputs, and traces; build rigorous, apples-to-apples evaluations for language model use cases; and organize all the information generated across the LLM workflow, from experimentation to evaluations to production. Weave aims to bring rigor, best-practices, and composability to the inherently experimental process of developing Generative AI software, without introducing cognitive overhead.
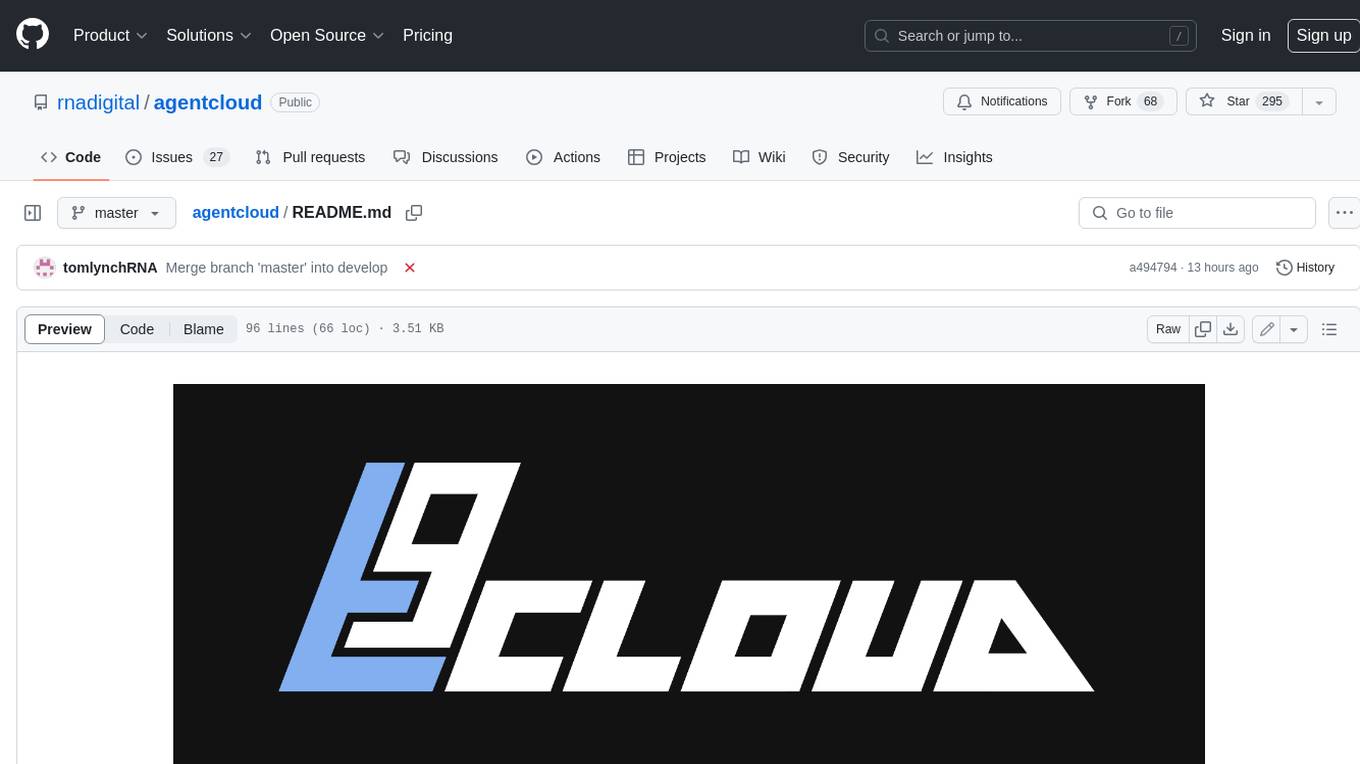
agentcloud
AgentCloud is an open-source platform that enables companies to build and deploy private LLM chat apps, empowering teams to securely interact with their data. It comprises three main components: Agent Backend, Webapp, and Vector Proxy. To run this project locally, clone the repository, install Docker, and start the services. The project is licensed under the GNU Affero General Public License, version 3 only. Contributions and feedback are welcome from the community.
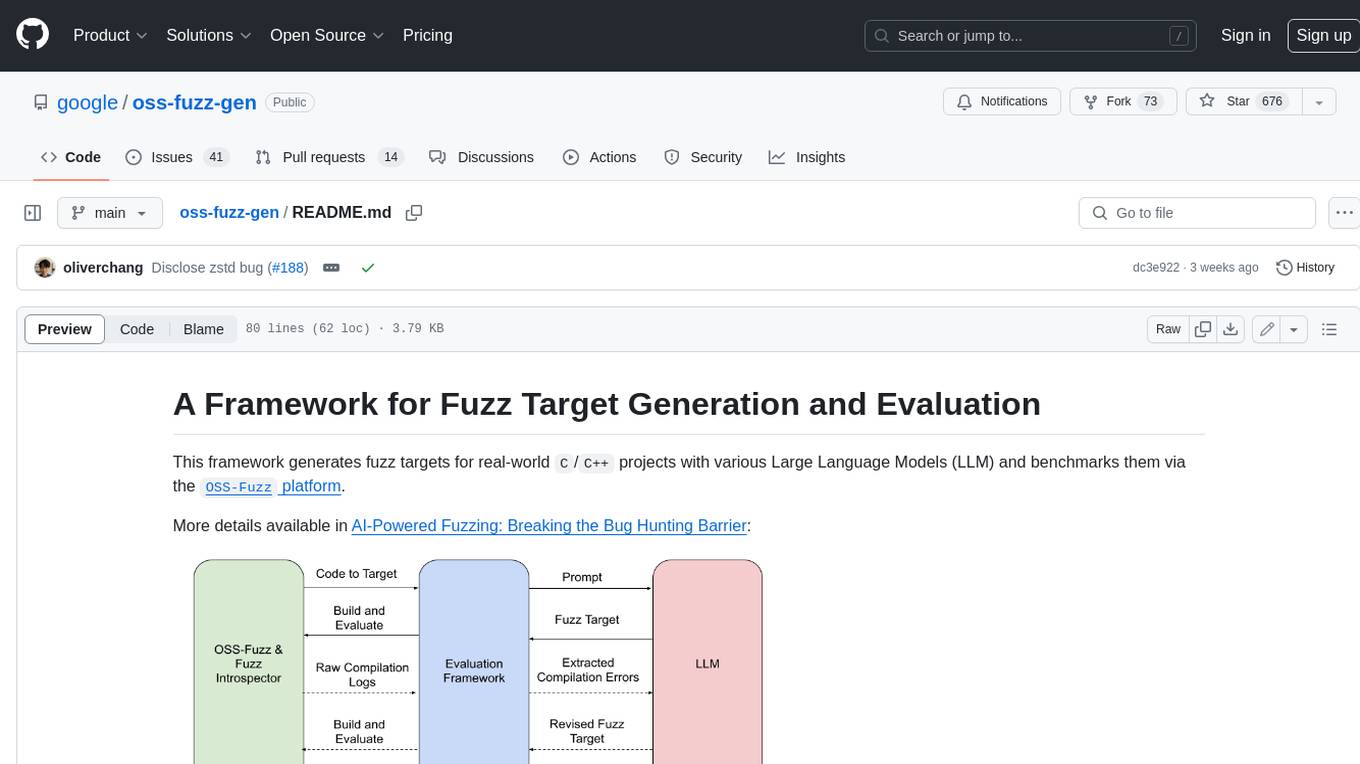
oss-fuzz-gen
This framework generates fuzz targets for real-world `C`/`C++` projects with various Large Language Models (LLM) and benchmarks them via the `OSS-Fuzz` platform. It manages to successfully leverage LLMs to generate valid fuzz targets (which generate non-zero coverage increase) for 160 C/C++ projects. The maximum line coverage increase is 29% from the existing human-written targets.
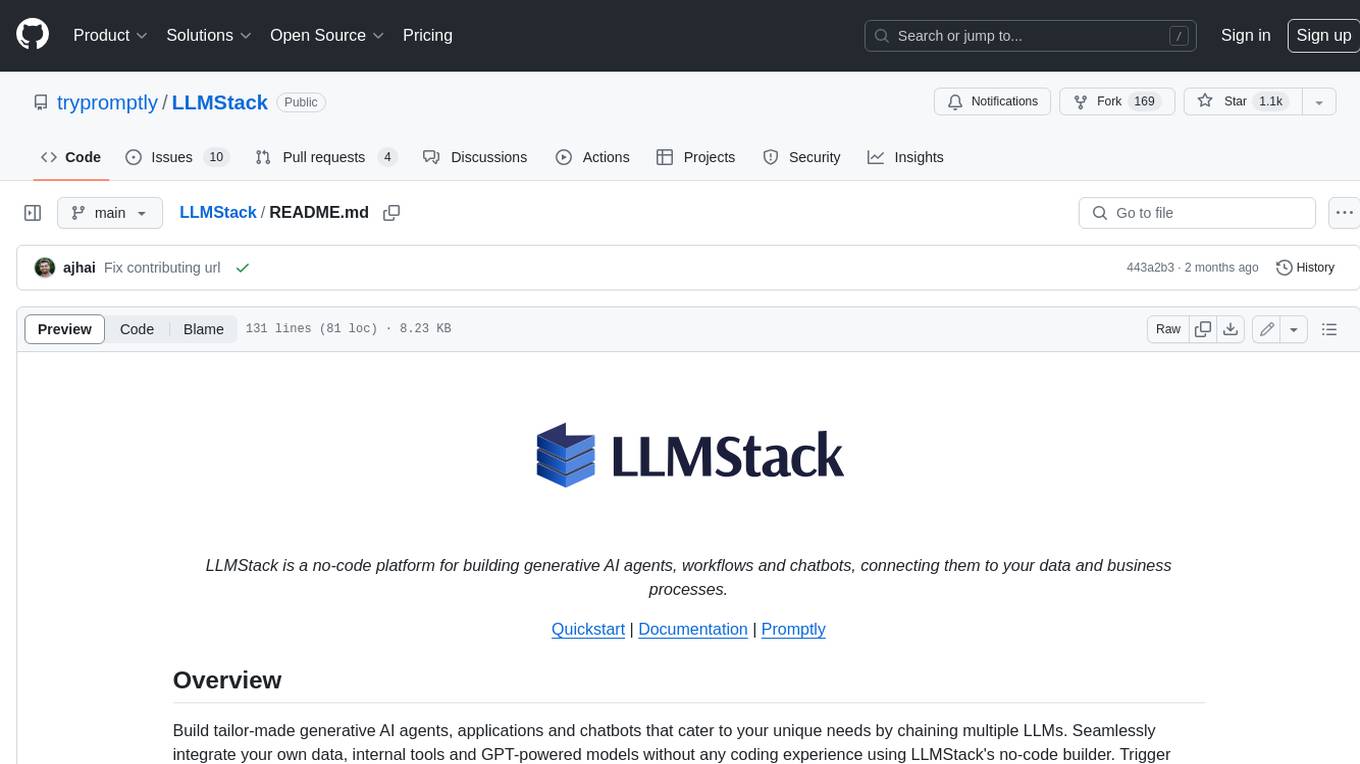
LLMStack
LLMStack is a no-code platform for building generative AI agents, workflows, and chatbots. It allows users to connect their own data, internal tools, and GPT-powered models without any coding experience. LLMStack can be deployed to the cloud or on-premise and can be accessed via HTTP API or triggered from Slack or Discord.
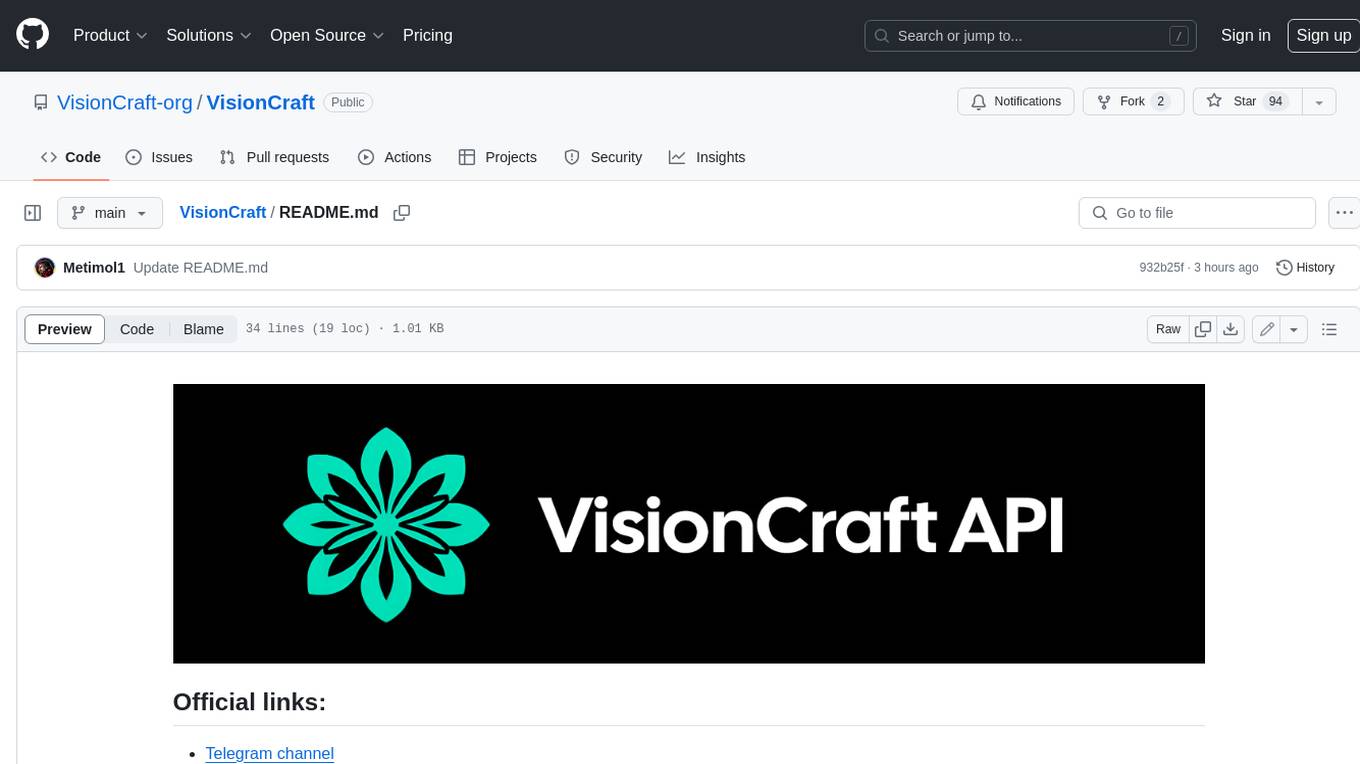
VisionCraft
The VisionCraft API is a free API for using over 100 different AI models. From images to sound.
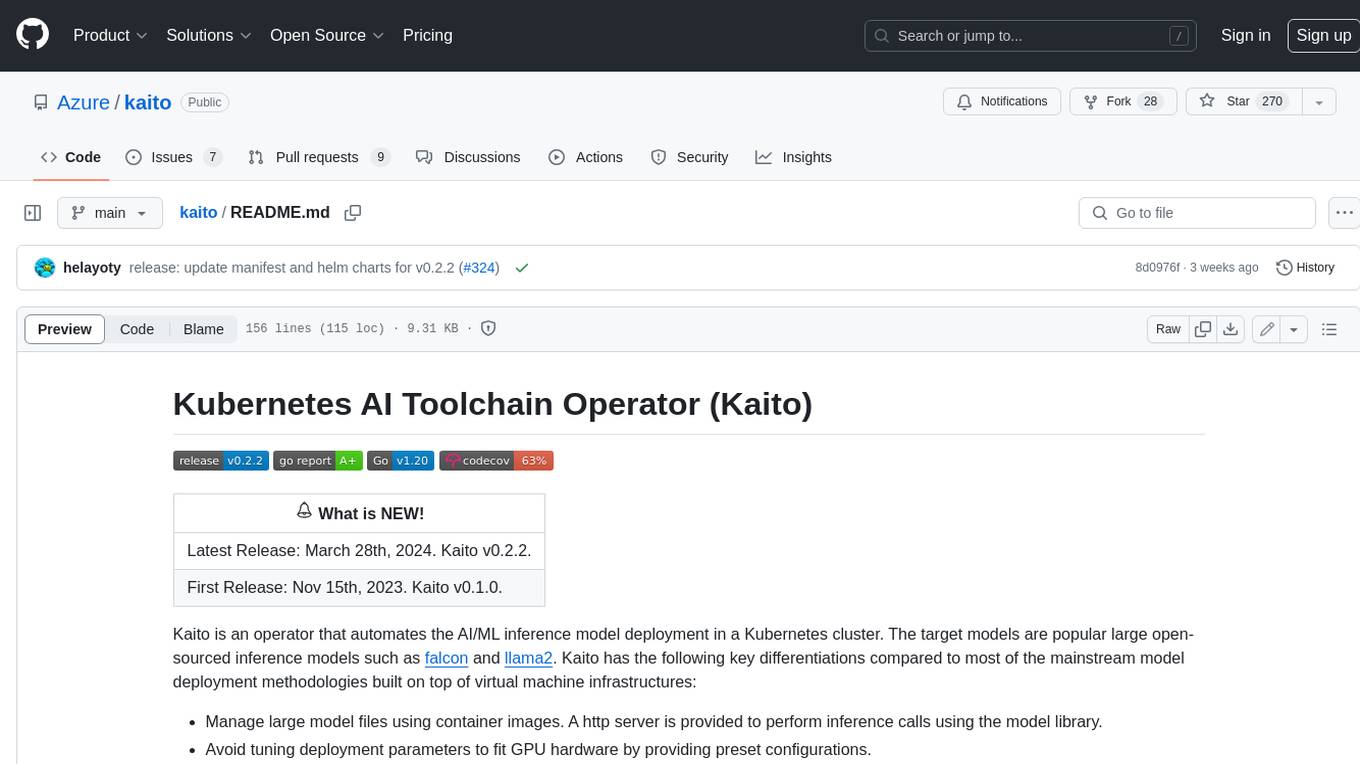
kaito
Kaito is an operator that automates the AI/ML inference model deployment in a Kubernetes cluster. It manages large model files using container images, avoids tuning deployment parameters to fit GPU hardware by providing preset configurations, auto-provisions GPU nodes based on model requirements, and hosts large model images in the public Microsoft Container Registry (MCR) if the license allows. Using Kaito, the workflow of onboarding large AI inference models in Kubernetes is largely simplified.
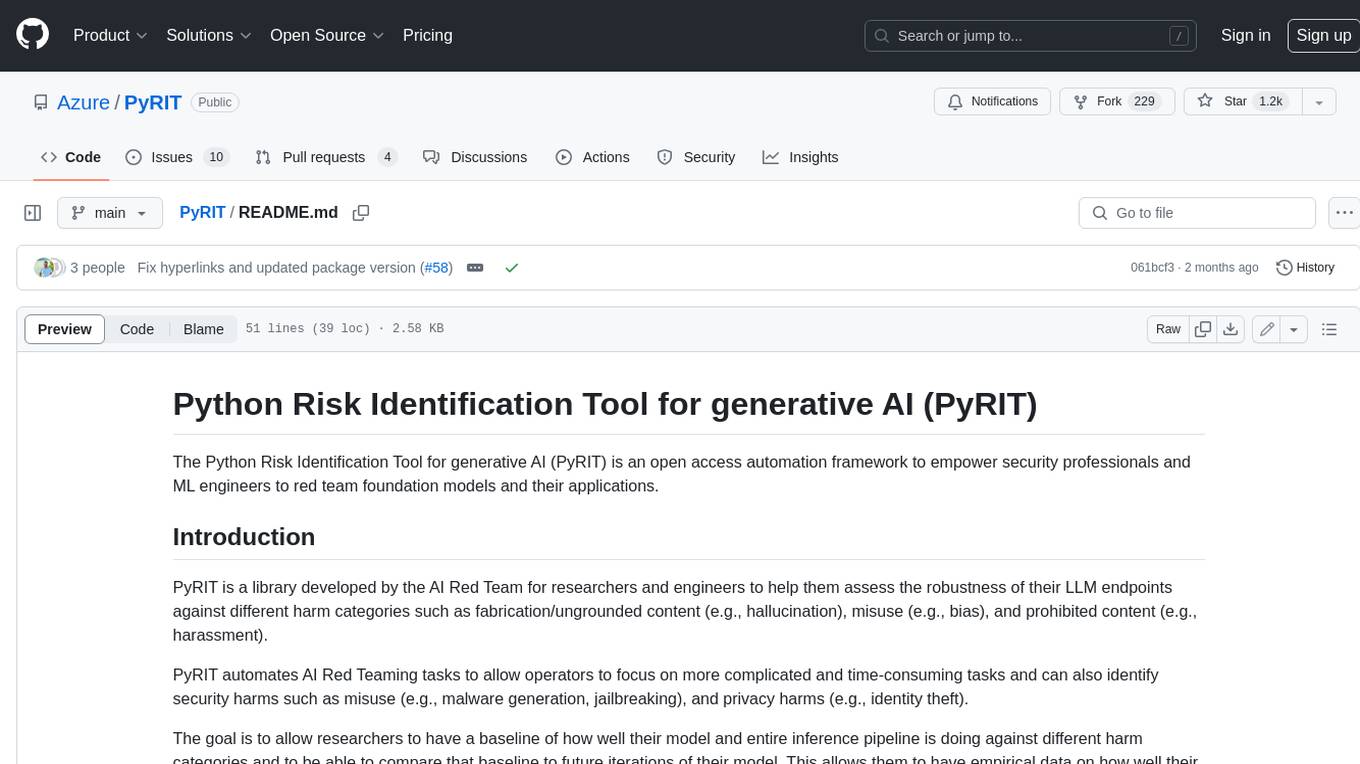
PyRIT
PyRIT is an open access automation framework designed to empower security professionals and ML engineers to red team foundation models and their applications. It automates AI Red Teaming tasks to allow operators to focus on more complicated and time-consuming tasks and can also identify security harms such as misuse (e.g., malware generation, jailbreaking), and privacy harms (e.g., identity theft). The goal is to allow researchers to have a baseline of how well their model and entire inference pipeline is doing against different harm categories and to be able to compare that baseline to future iterations of their model. This allows them to have empirical data on how well their model is doing today, and detect any degradation of performance based on future improvements.
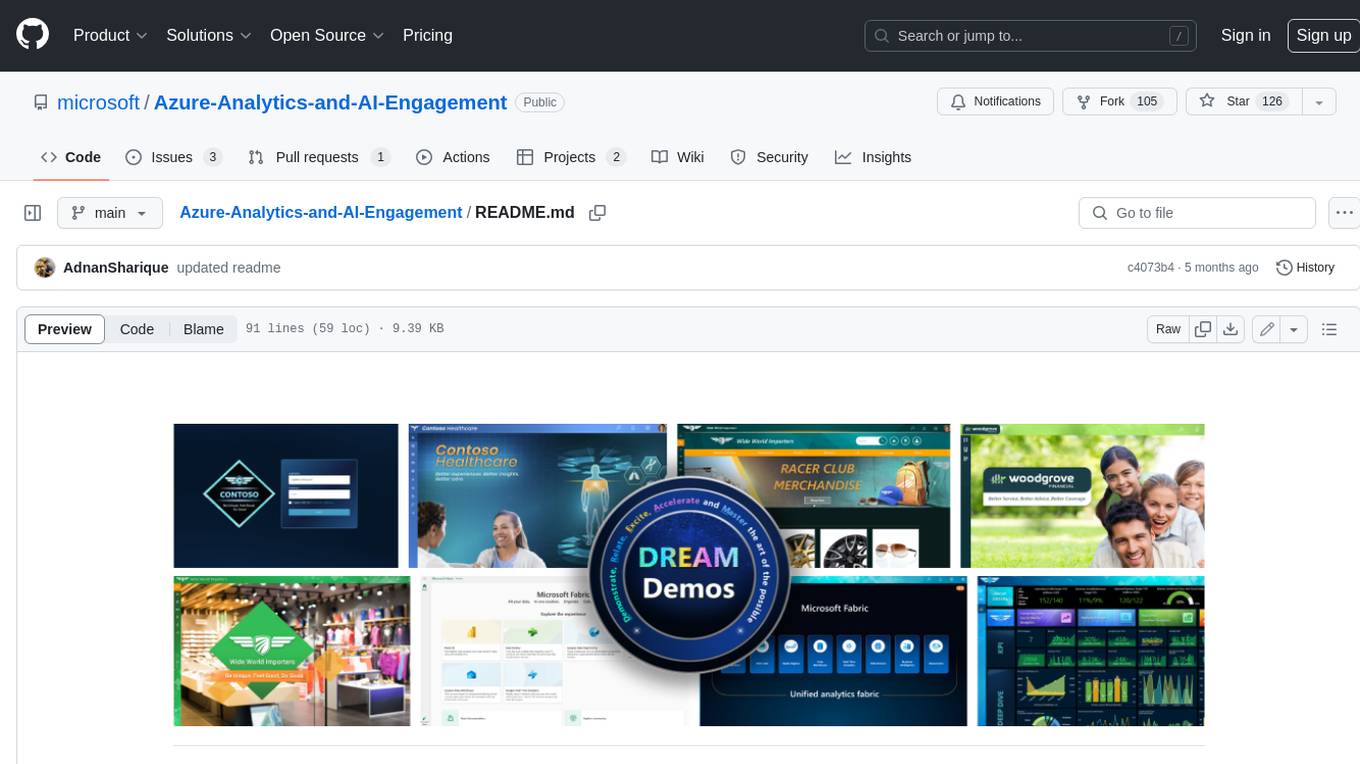
Azure-Analytics-and-AI-Engagement
The Azure-Analytics-and-AI-Engagement repository provides packaged Industry Scenario DREAM Demos with ARM templates (Containing a demo web application, Power BI reports, Synapse resources, AML Notebooks etc.) that can be deployed in a customer’s subscription using the CAPE tool within a matter of few hours. Partners can also deploy DREAM Demos in their own subscriptions using DPoC.