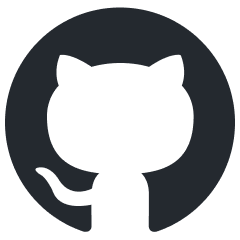
yomo
π¦ Stateful Serverless Framework for Geo-distributed Edge AI Infra. with function calling support, write once, run on any model.
Stars: 1731
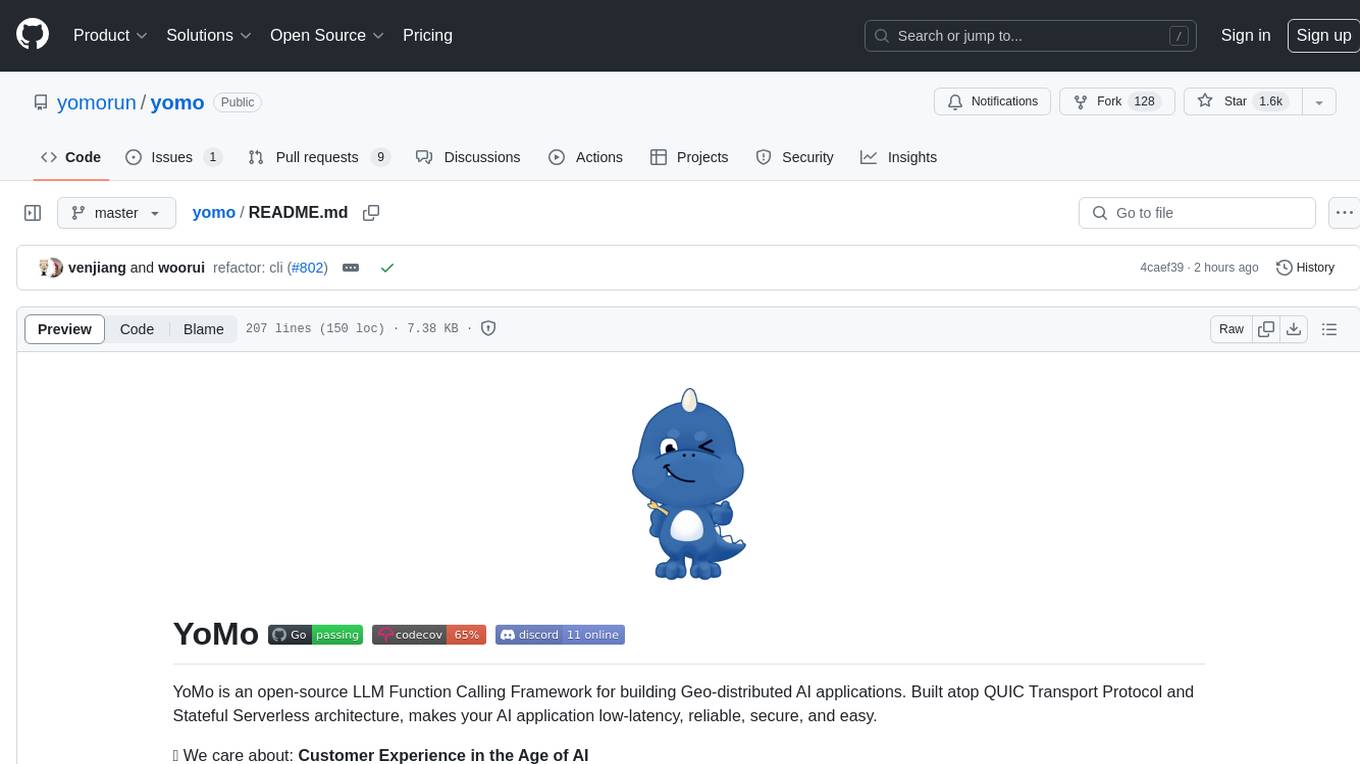
YoMo is an open-source LLM Function Calling Framework for building Geo-distributed AI applications. It is built atop QUIC Transport Protocol and Stateful Serverless architecture, making AI applications low-latency, reliable, secure, and easy. The framework focuses on providing low-latency, secure, stateful serverless functions that can be distributed geographically to bring AI inference closer to end users. It offers features such as low-latency communication, security with TLS v1.3, stateful serverless functions for faster GPU processing, geo-distributed architecture, and a faster-than-real-time codec called Y3. YoMo enables developers to create and deploy stateful serverless functions for AI inference in a distributed manner, ensuring quick responses to user queries from various locations worldwide.
README:
YoMo is an open-source LLM Function Calling Framework for building Geo-distributed AI applications. Built atop QUIC Transport Protocol and Stateful Serverless architecture, makes your AI application low-latency, reliable, secure, and easy.
π We care about: Customer Experience in the Age of AI
Features | |
---|---|
β‘οΈ | Low-latency Guaranteed by implementing atop QUIC QUIC |
π | Security TLS v1.3 on every data packet by design |
πΈ | Stateful Serverless Make your GPU serverless 10x faster |
π | Geo-Distributed Architecture Brings AI inference closer to end users |
π | Y3 a faster than real-time codec |
Let's implement a function calling with sfn-currency-converter
:
curl -fsSL https://get.yomo.run | sh
Verify if the CLI was installed successfully
yomo version
Prepare the configuration as my-agent.yaml
name: ai-zipper
host: 0.0.0.0
port: 9000
auth:
type: token
token: SECRET_TOKEN
bridge:
ai:
server:
addr: 0.0.0.0:8000 ## Restful API endpoint
provider: openai ## LLM API Service we will use
providers:
azopenai:
api_endpoint: https://<RESOURCE>.openai.azure.com
deployment_id: <DEPLOYMENT_ID>
api_key: <API_KEY>
api_version: <API_VERSION>
openai:
api_key: sk-xxxxxxxxxxxxxxxxxxxxxxxxxxx
model: gpt-4-1106-preview
gemini:
api_key: <GEMINI_API_KEY>
cloudflare_azure:
endpoint: https://gateway.ai.cloudflare.com/v1/<CF_GATEWAY_ID>/<CF_GATEWAY_NAME>
api_key: <AZURE_API_KEY>
resource: <AZURE_OPENAI_RESOURCE>
deployment_id: <AZURE_OPENAI_DEPLOYMENT_ID>
api_version: 2023-12-01-preview
Start the server:
YOMO_LOG_LEVEL=debug yomo serve -c my-agent.yaml
First, let's define what this function do and how's the parameters required, these will be combined to prompt when invoking LLM.
type Parameter struct {
Domain string `json:"domain" jsonschema:"description=Domain of the website,example=example.com"`
}
func Description() string {
return `if user asks ip or network latency of a domain, you should return the result of the giving domain. try your best to dissect user expressions to infer the right domain names`
}
func InputSchema() any {
return &Parameter{}
}
Create a Stateful Serverless Function to get the IP and Latency of a domain:
func Handler(ctx serverless.Context) {
var msg Parameter
ctx.ReadLLMArguments(&msg)
// get ip of the domain
ips, _ := net.LookupIP(msg.Domain)
// get ip[0] ping latency
pinger, _ := ping.NewPinger(ips[0].String())
pinger.Count = 3
pinger.Run()
stats := pinger.Statistics()
val := fmt.Sprintf("domain %s has ip %s with average latency %s", msg.Domain, ips[0], stats.AvgRtt)
ctx.WriteLLMResult(val)
}
Finally, let's run it
$ yomo run app.go
time=2024-03-19T21:43:30.583+08:00 level=INFO msg="connected to zipper" component=StreamFunction sfn_id=B0ttNSEKLSgMjXidB11K1 sfn_name=fn-get-ip-from-domain zipper_addr=localhost:9000
time=2024-03-19T21:43:30.584+08:00 level=INFO msg="register ai function success" component=StreamFunction sfn_id=B0ttNSEKLSgMjXidB11K1 sfn_name=fn-get-ip-from-domain zipper_addr=localhost:9000 name=fn-get-ip-from-domain tag=16
$ curl -i http://127.0.0.1:9000/v1/chat/completions -H "Content-Type: application/json" -d '{
"messages": [
{
"role": "system",
"content": "You are a test assistant."
},
{
"role": "user",
"content": "Compare website speed between Nike and Puma"
}
],
"stream": false
}'
HTTP/1.1 200 OK
Content-Length: 944
Connection: keep-alive
Content-Type: application/json
Date: Tue, 19 Mar 2024 13:30:14 GMT
Keep-Alive: timeout=4
Proxy-Connection: keep-alive
{
"Content": "Based on the data provided for the domains nike.com and puma.com which include IP addresses and average latencies, we can infer the following about their website speeds:
- Nike.com has an IP address of 13.225.183.84 with an average latency of 65.568333 milliseconds.
- Puma.com has an IP address of 151.101.194.132 with an average latency of 54.563666 milliseconds.
Comparing these latencies, Puma.com is faster than Nike.com as it has a lower average latency.
Please be aware, however, that website speed can be influenced by many factors beyond latency, such as server processing time, content size, and delivery networks among others. To get a more comprehensive understanding of website speed, you would need to consider additional metrics and possibly conductreal-time speed tests.",
"FinishReason": "stop"
}
Full LLM Function Calling Codes
Read more about YoMo at yomo.run/docs.
YoMo β€οΈ Vercel, our documentation website is
Itβs no secret that todayβs users want instant AI inference, every AI
application is more powerful when it response quickly. But, currently, when we
talk about distribution
, it represents distribution in data center. The AI model is
far away from their users from all over the world.
If an application can be deployed anywhere close to their end users, solve the problem, this is Geo-distributed System Architecture:
First off, thank you for considering making contributions. It's people like you that make YoMo better. There are many ways in which you can participate in the project, for example:
- File a bug report. Be sure to include information like what version of YoMo you are using, what your operating system is, and steps to recreate the bug.
- Suggest a new feature.
- Read our contributing guidelines to learn about what types of contributions we are looking for.
- We have also adopted a code of conduct that we expect project participants to adhere to.
For Tasks:
Click tags to check more tools for each tasksFor Jobs:
Alternative AI tools for yomo
Similar Open Source Tools
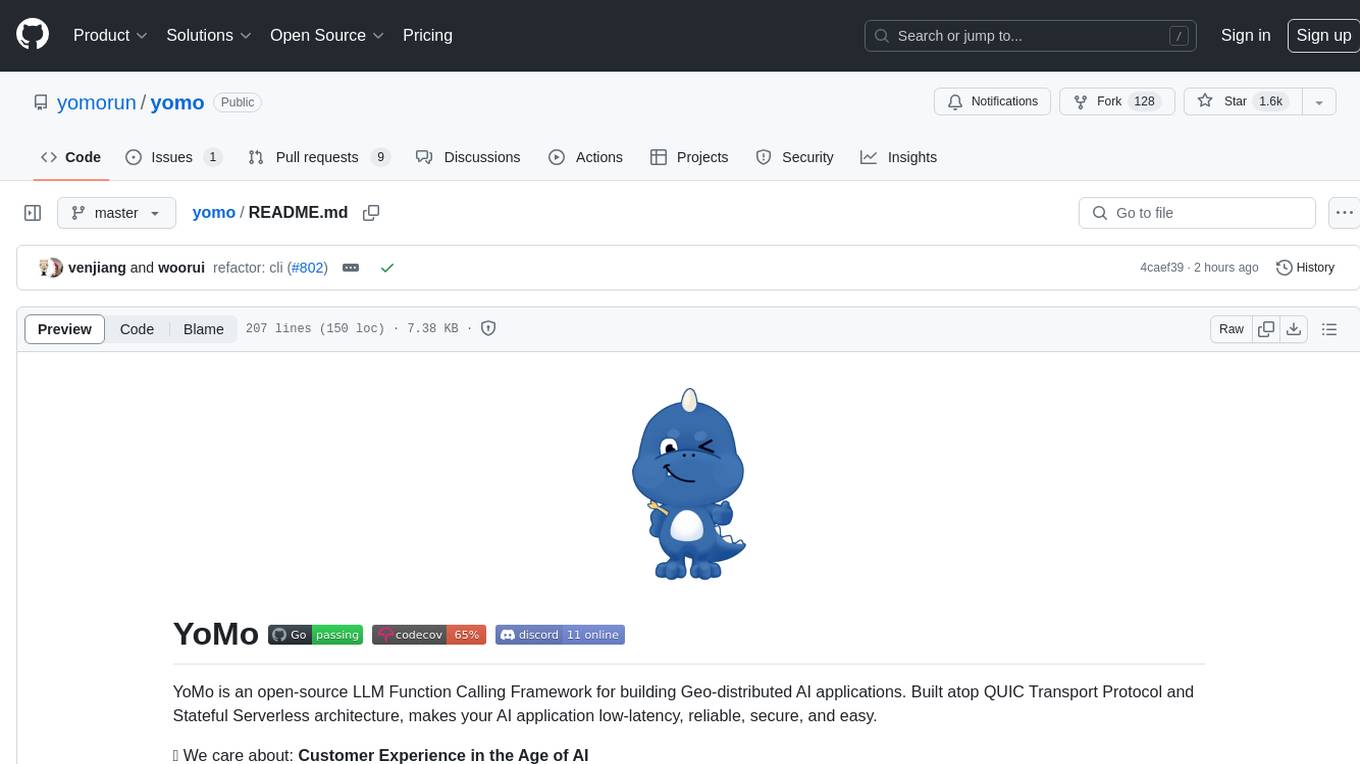
yomo
YoMo is an open-source LLM Function Calling Framework for building Geo-distributed AI applications. It is built atop QUIC Transport Protocol and Stateful Serverless architecture, making AI applications low-latency, reliable, secure, and easy. The framework focuses on providing low-latency, secure, stateful serverless functions that can be distributed geographically to bring AI inference closer to end users. It offers features such as low-latency communication, security with TLS v1.3, stateful serverless functions for faster GPU processing, geo-distributed architecture, and a faster-than-real-time codec called Y3. YoMo enables developers to create and deploy stateful serverless functions for AI inference in a distributed manner, ensuring quick responses to user queries from various locations worldwide.
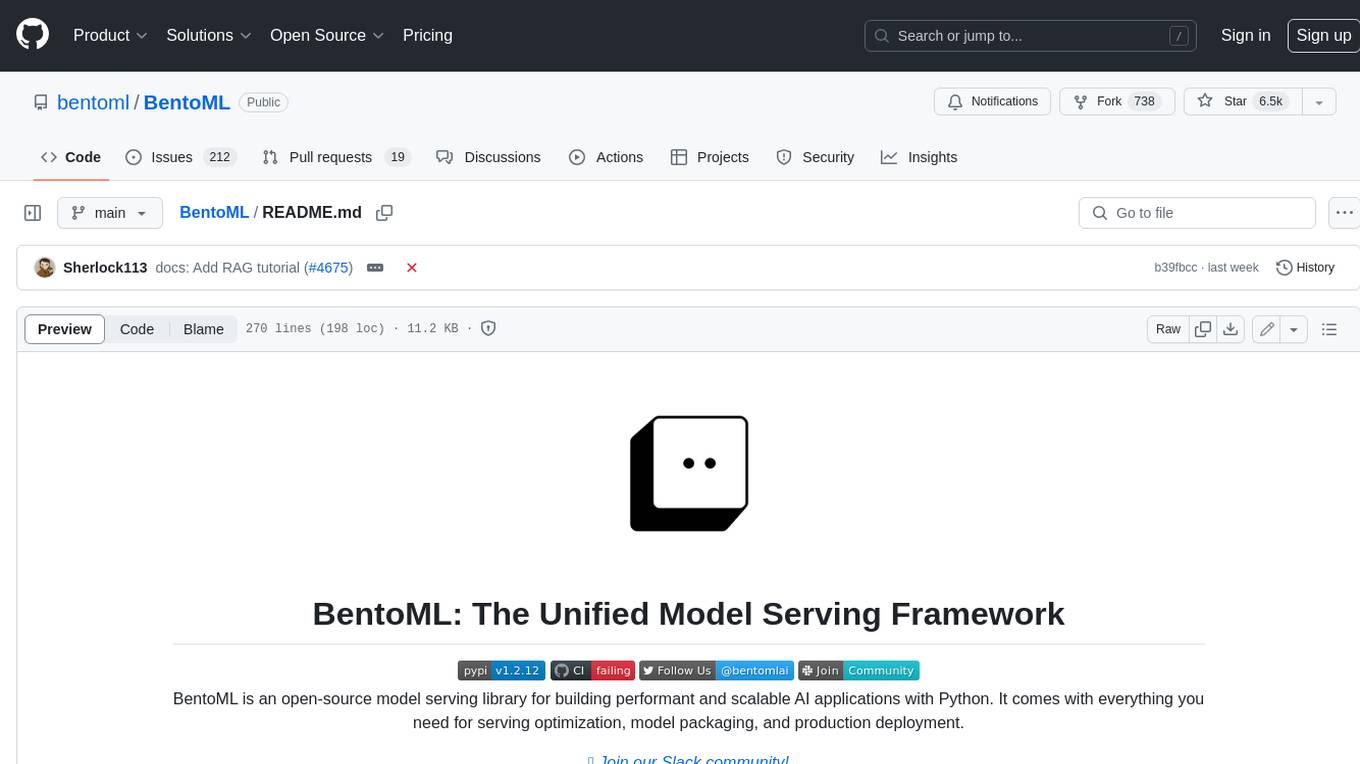
BentoML
BentoML is an open-source model serving library for building performant and scalable AI applications with Python. It comes with everything you need for serving optimization, model packaging, and production deployment.
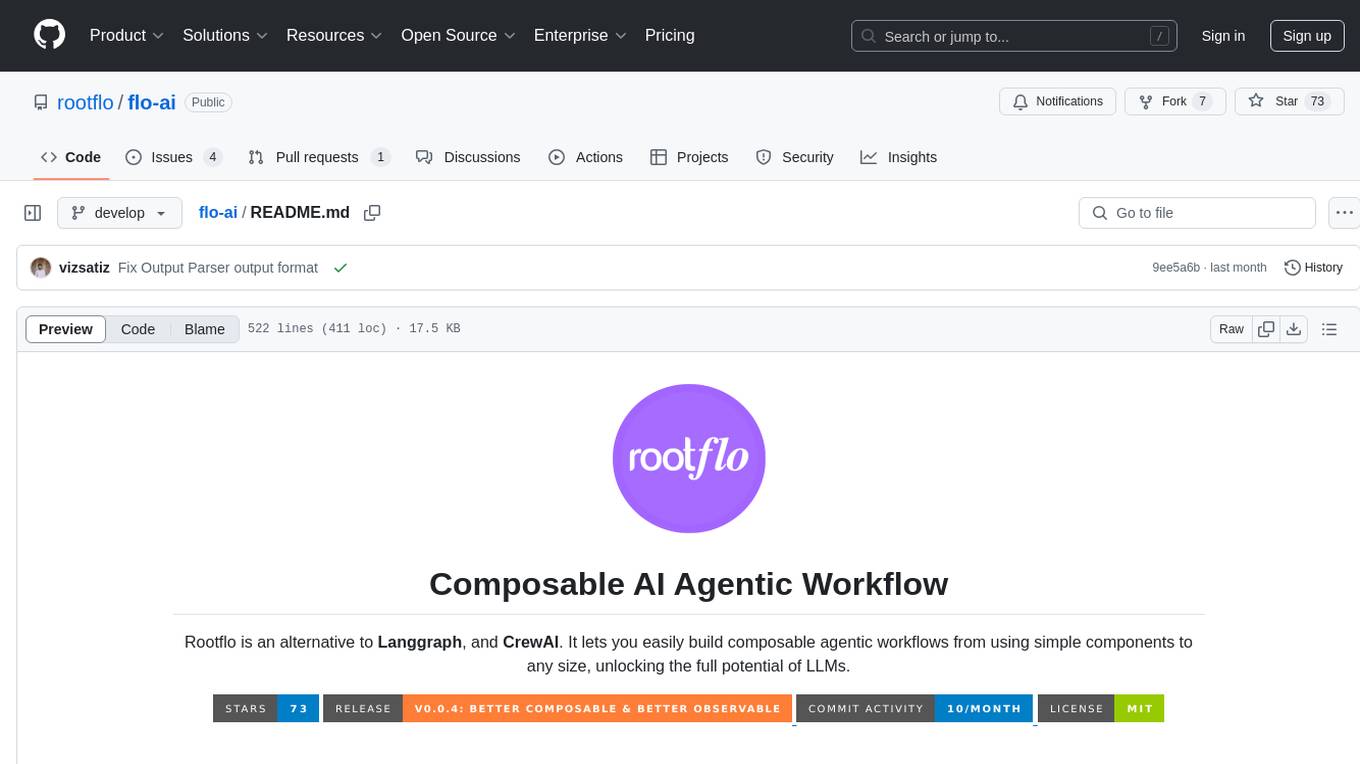
flo-ai
Flo AI is a Python framework that enables users to build production-ready AI agents and teams with minimal code. It allows users to compose complex AI architectures using pre-built components while maintaining the flexibility to create custom components. The framework supports composable, production-ready, YAML-first, and flexible AI systems. Users can easily create AI agents and teams, manage teams of AI agents working together, and utilize built-in support for Retrieval-Augmented Generation (RAG) and compatibility with Langchain tools. Flo AI also provides tools for output parsing and formatting, tool logging, data collection, and JSON output collection. It is MIT Licensed and offers detailed documentation, tutorials, and examples for AI engineers and teams to accelerate development, maintainability, scalability, and testability of AI systems.
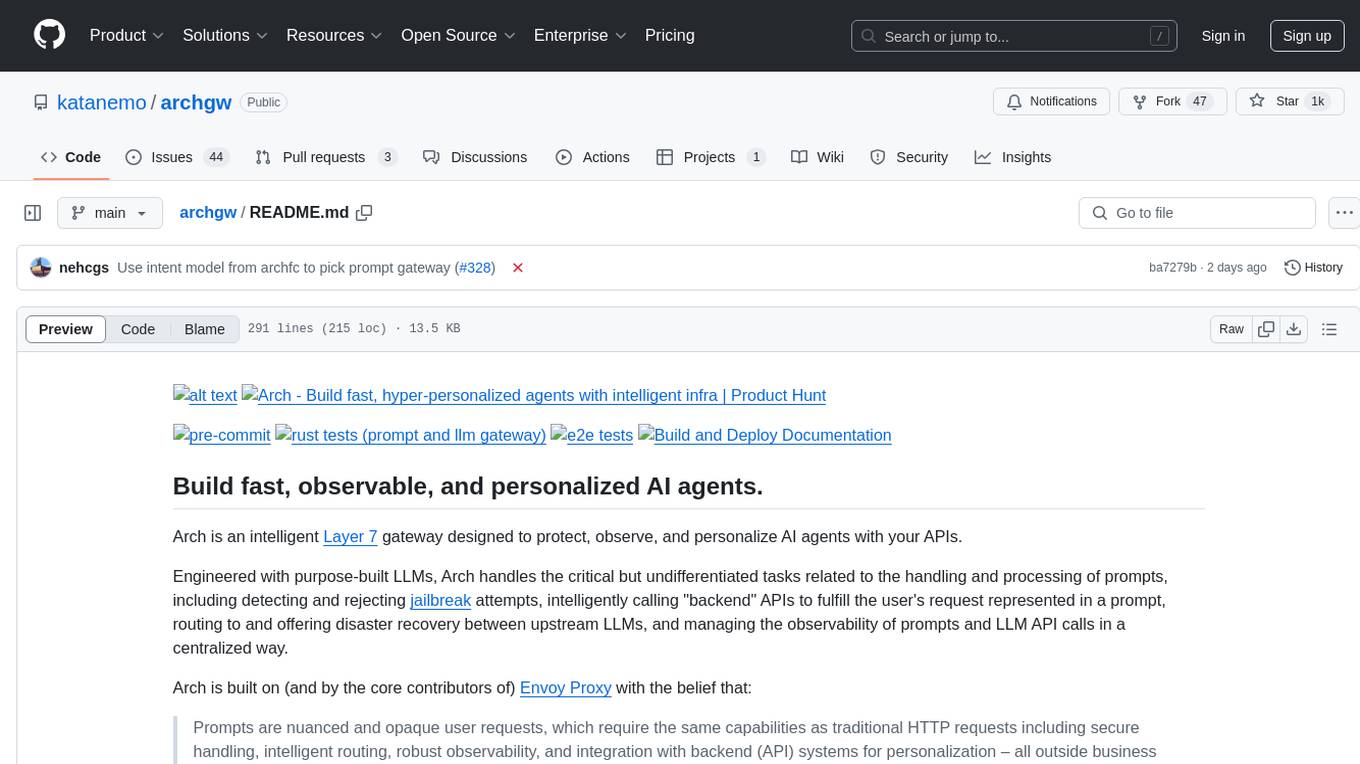
archgw
Arch is an intelligent Layer 7 gateway designed to protect, observe, and personalize AI agents with APIs. It handles tasks related to prompts, including detecting jailbreak attempts, calling backend APIs, routing between LLMs, and managing observability. Built on Envoy Proxy, it offers features like function calling, prompt guardrails, traffic management, and observability. Users can build fast, observable, and personalized AI agents using Arch to improve speed, security, and personalization of GenAI apps.
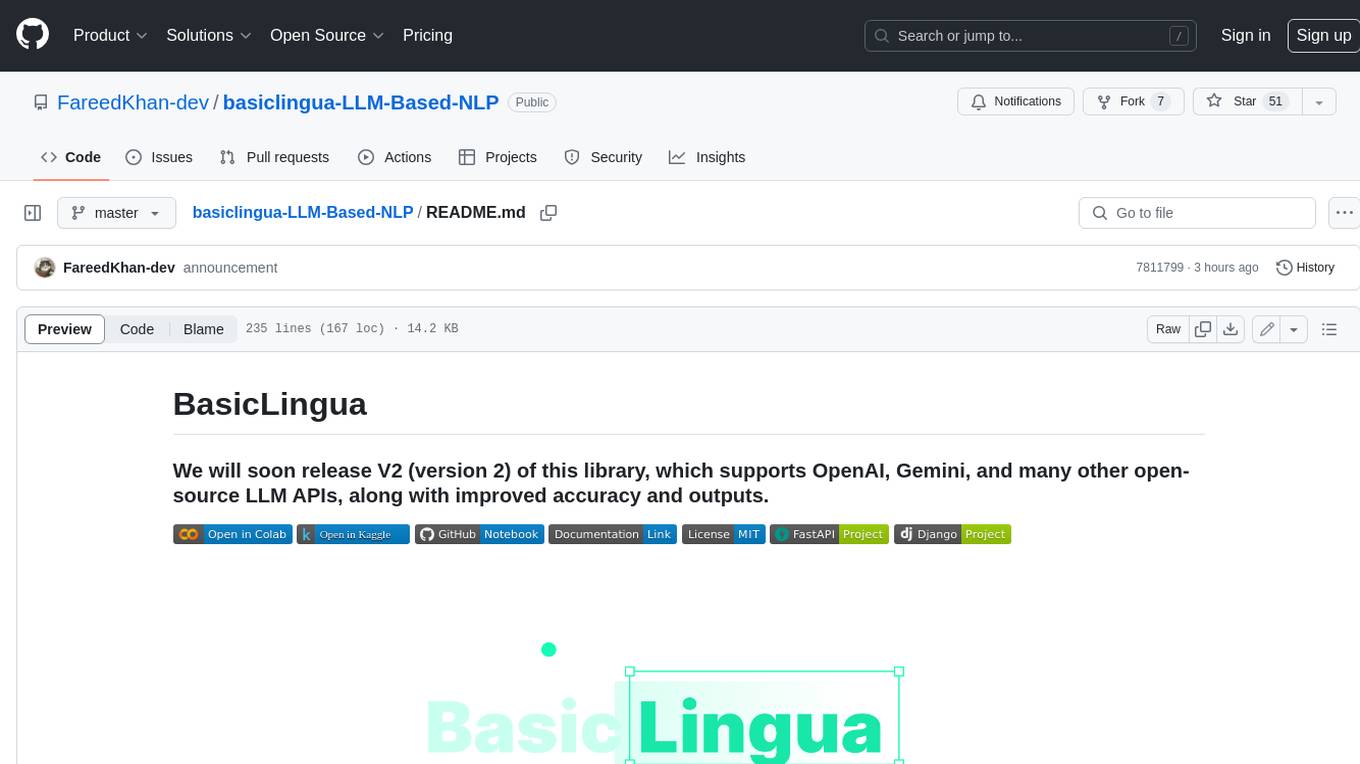
basiclingua-LLM-Based-NLP
BasicLingua is a Python library that provides functionalities for linguistic tasks such as tokenization, stemming, lemmatization, and many others. It is based on the Gemini Language Model, which has demonstrated promising results in dealing with text data. BasicLingua can be used as an API or through a web demo. It is available under the MIT license and can be used in various projects.
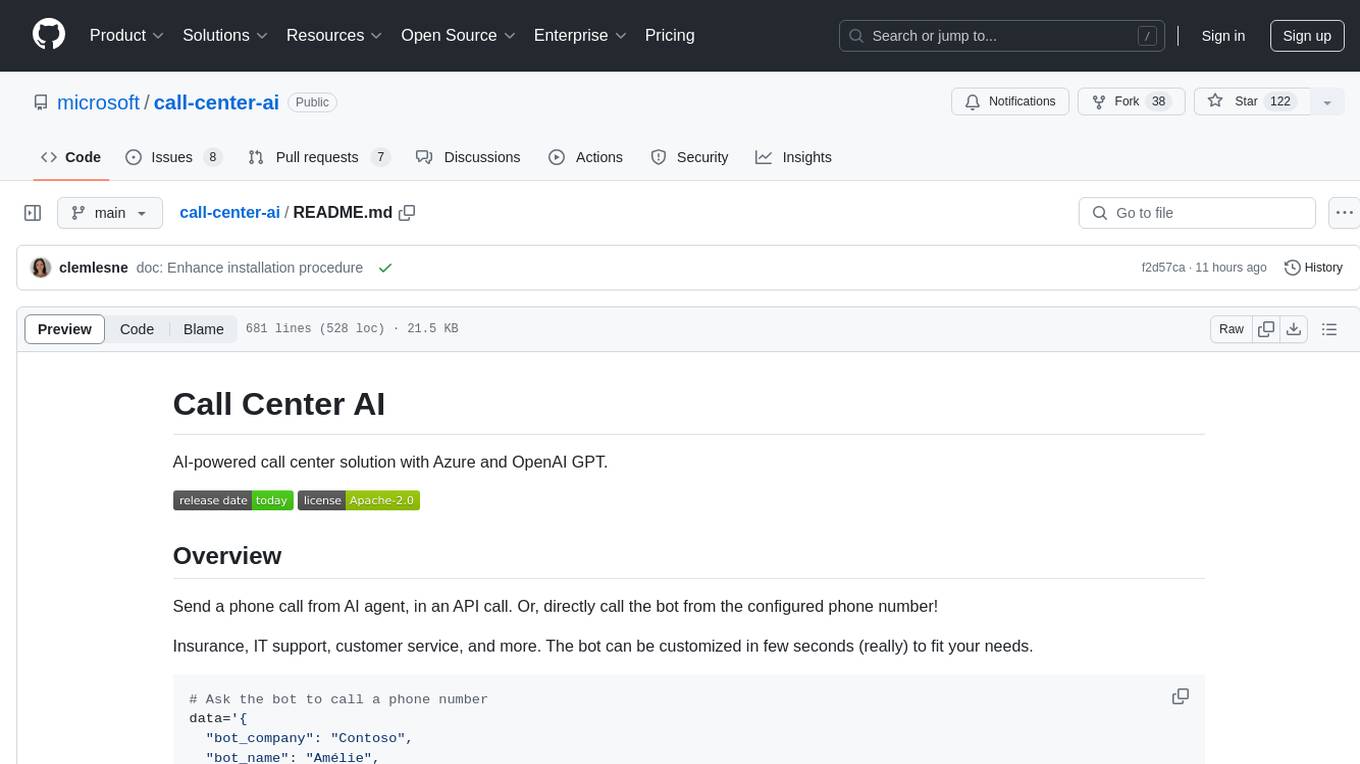
call-center-ai
Call Center AI is an AI-powered call center solution leveraging Azure and OpenAI GPT. It allows for AI agent-initiated phone calls or direct calls to the bot from a configured phone number. The bot is customizable for various industries like insurance, IT support, and customer service, with features such as accessing claim information, conversation history, language change, SMS sending, and more. The project is a proof of concept showcasing the integration of Azure Communication Services, Azure Cognitive Services, and Azure OpenAI for an automated call center solution.
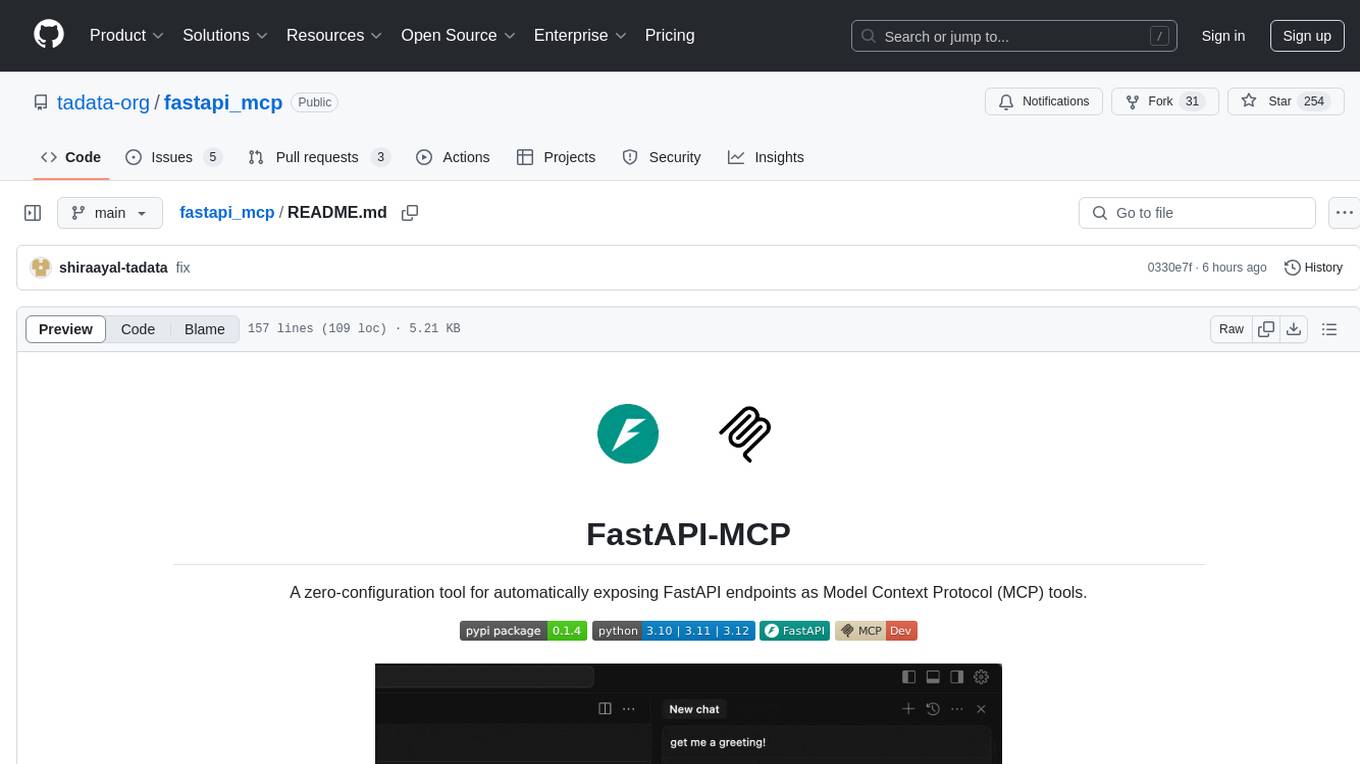
fastapi_mcp
FastAPI-MCP is a zero-configuration tool that automatically exposes FastAPI endpoints as Model Context Protocol (MCP) tools. It allows for direct integration with FastAPI apps, automatic discovery and conversion of endpoints to MCP tools, preservation of request and response schemas, documentation preservation similar to Swagger, and the ability to extend with custom MCP tools. Users can easily add an MCP server to their FastAPI application and customize the server creation and configuration. The tool supports connecting to the MCP server using SSE or mcp-proxy stdio for different MCP clients. FastAPI-MCP is developed and maintained by Tadata Inc.
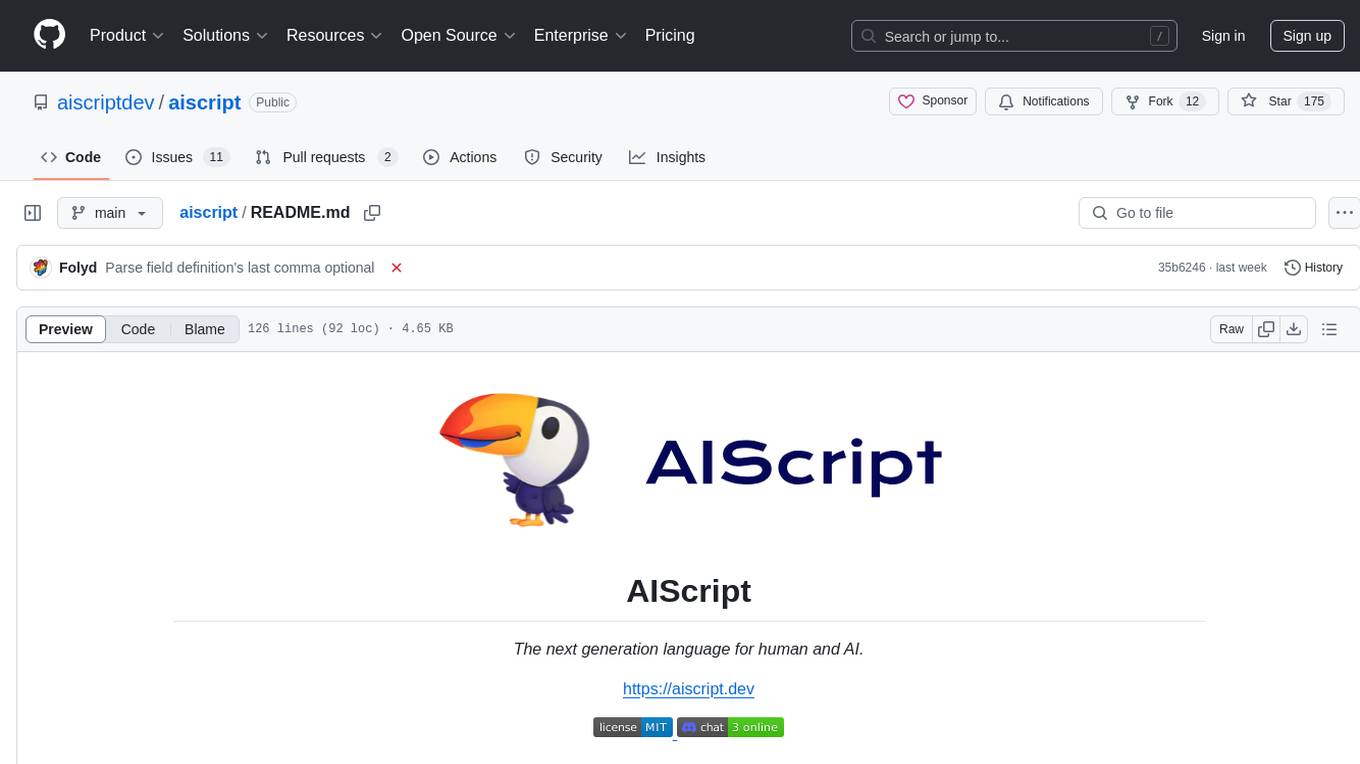
aiscript
AIScript is a unique programming language and web framework written in Rust, designed to help developers effortlessly build AI applications. It combines the strengths of Python, JavaScript, and Rust to create an intuitive, powerful, and easy-to-use tool. The language features first-class functions, built-in AI primitives, dynamic typing with static type checking, data validation, error handling inspired by Rust, a rich standard library, and automatic garbage collection. The web framework offers an elegant route DSL, automatic parameter validation, OpenAPI schema generation, database modules, authentication capabilities, and more. AIScript excels in AI-powered APIs, prototyping, microservices, data validation, and building internal tools.
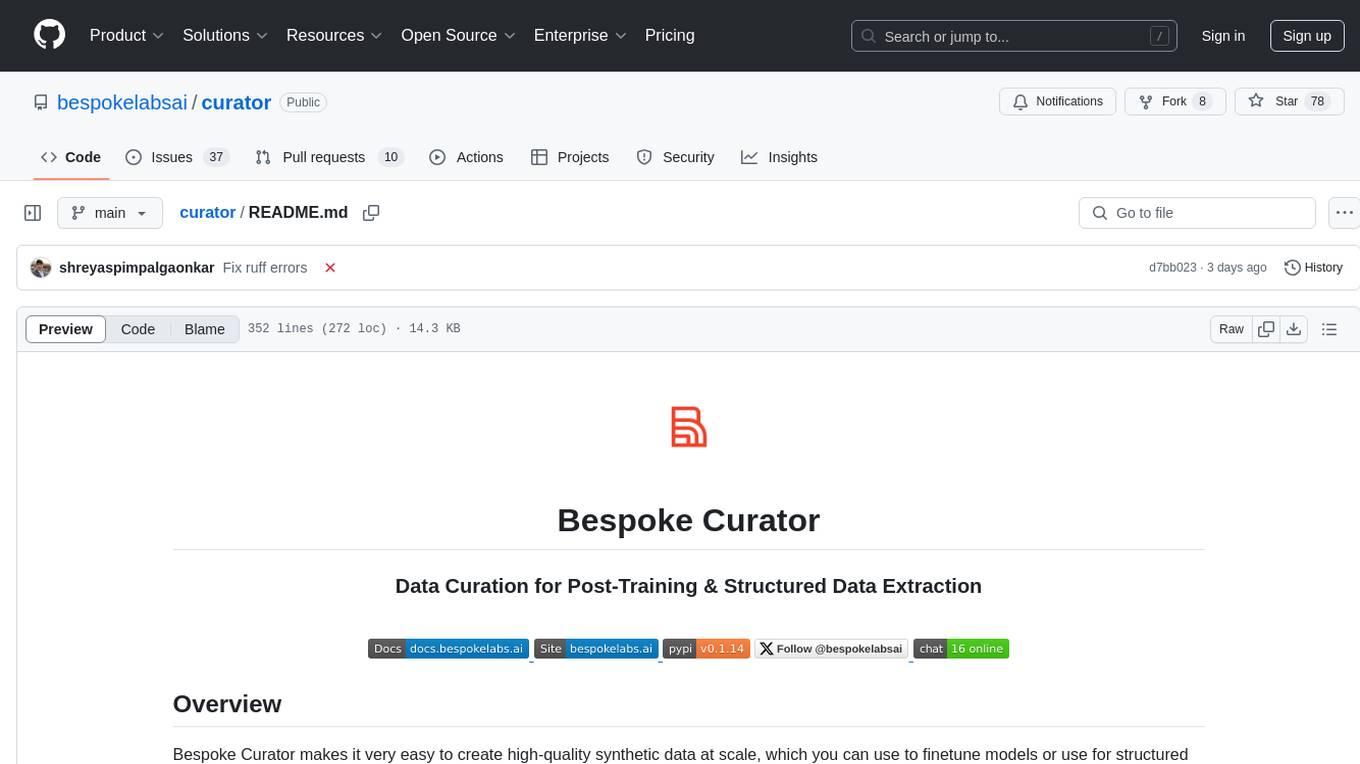
curator
Bespoke Curator is an open-source tool for data curation and structured data extraction. It provides a Python library for generating synthetic data at scale, with features like programmability, performance optimization, caching, and integration with HuggingFace Datasets. The tool includes a Curator Viewer for dataset visualization and offers a rich set of functionalities for creating and refining data generation strategies.
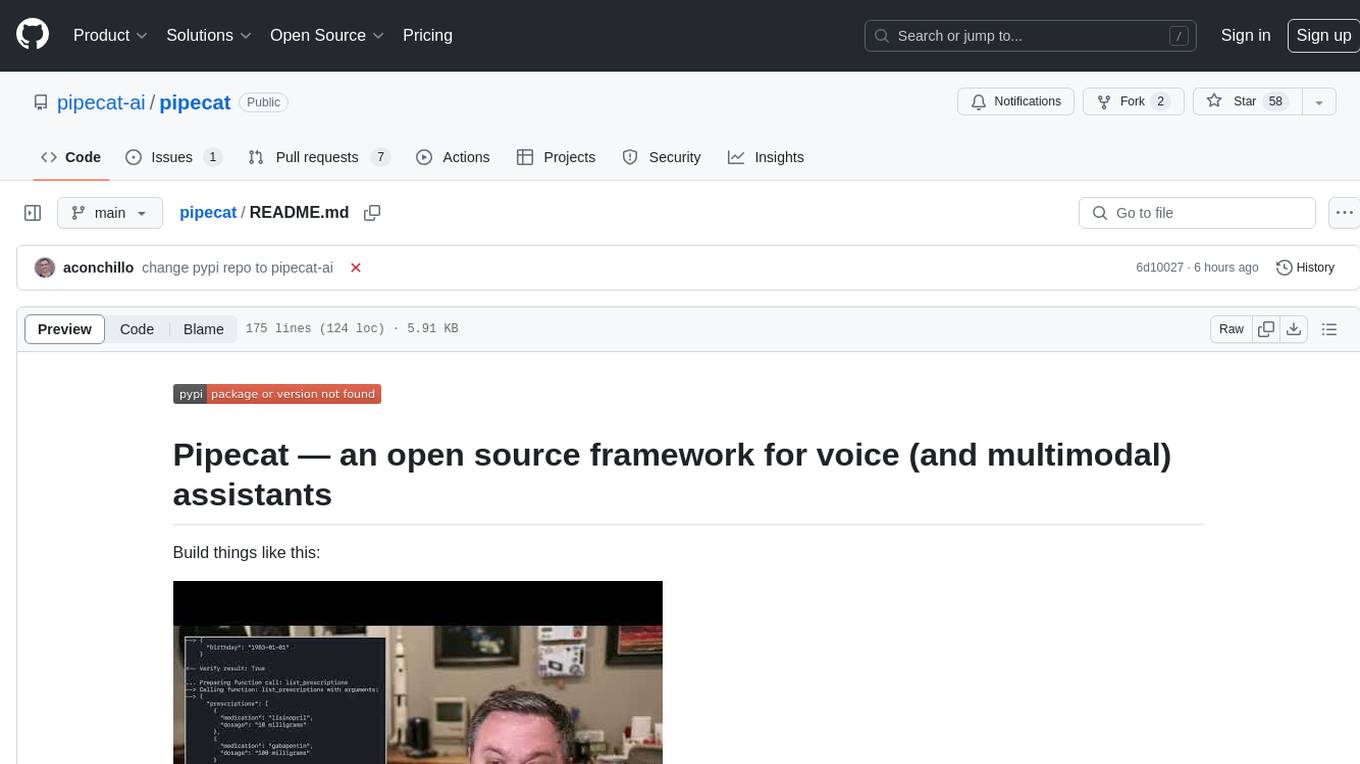
pipecat
Pipecat is an open-source framework designed for building generative AI voice bots and multimodal assistants. It provides code building blocks for interacting with AI services, creating low-latency data pipelines, and transporting audio, video, and events over the Internet. Pipecat supports various AI services like speech-to-text, text-to-speech, image generation, and vision models. Users can implement new services and contribute to the framework. Pipecat aims to simplify the development of applications like personal coaches, meeting assistants, customer support bots, and more by providing a complete framework for integrating AI services.
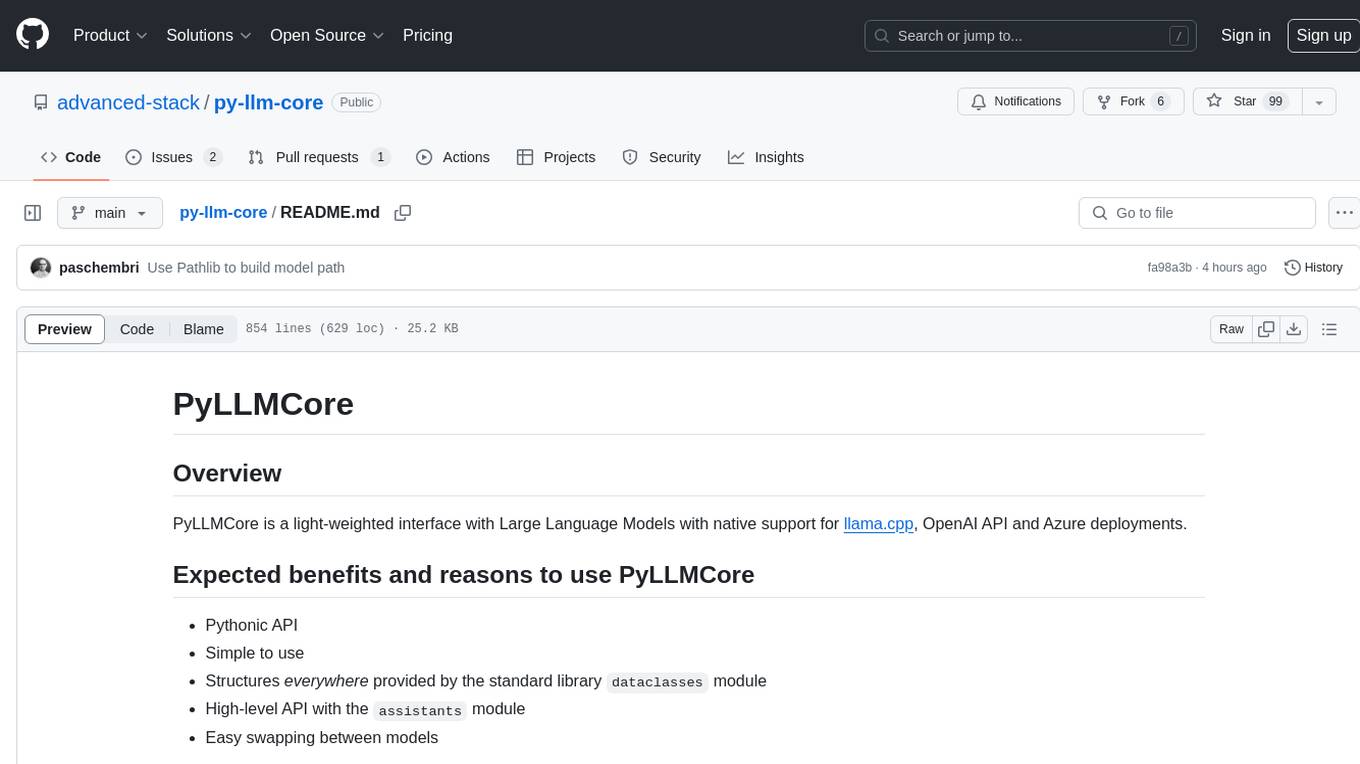
py-llm-core
PyLLMCore is a light-weighted interface with Large Language Models with native support for llama.cpp, OpenAI API, and Azure deployments. It offers a Pythonic API that is simple to use, with structures provided by the standard library dataclasses module. The high-level API includes the assistants module for easy swapping between models. PyLLMCore supports various models including those compatible with llama.cpp, OpenAI, and Azure APIs. It covers use cases such as parsing, summarizing, question answering, hallucinations reduction, context size management, and tokenizing. The tool allows users to interact with language models for tasks like parsing text, summarizing content, answering questions, reducing hallucinations, managing context size, and tokenizing text.
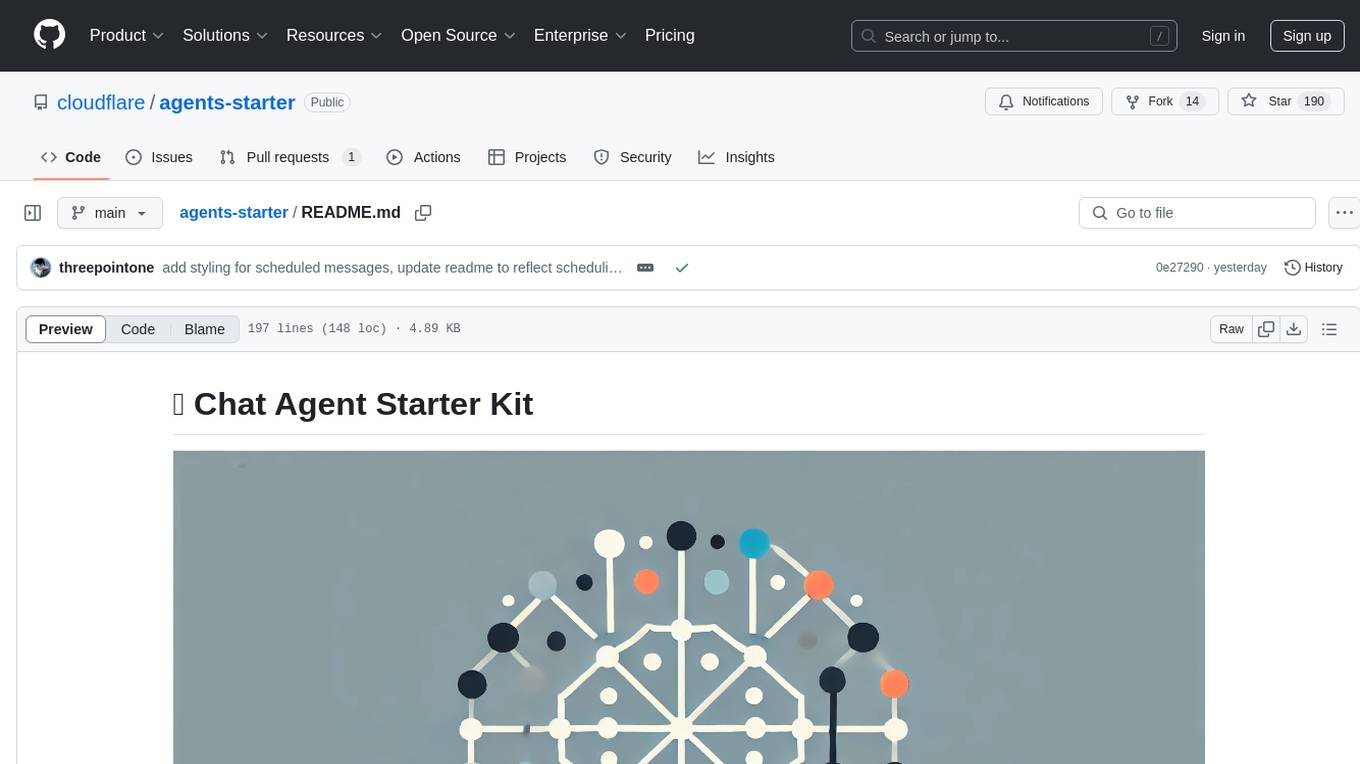
agents-starter
A starter template for building AI-powered chat agents using Cloudflare's Agent platform, powered by agents-sdk. It provides a foundation for creating interactive chat experiences with AI, complete with a modern UI and tool integration capabilities. Features include interactive chat interface with AI, built-in tool system with human-in-the-loop confirmation, advanced task scheduling, dark/light theme support, real-time streaming responses, state management, and chat history. Prerequisites include a Cloudflare account and OpenAI API key. The project structure includes components for chat UI implementation, chat agent logic, tool definitions, and helper functions. Customization guide covers adding new tools, modifying the UI, and example use cases for customer support, development assistant, data analysis assistant, personal productivity assistant, and scheduling assistant.
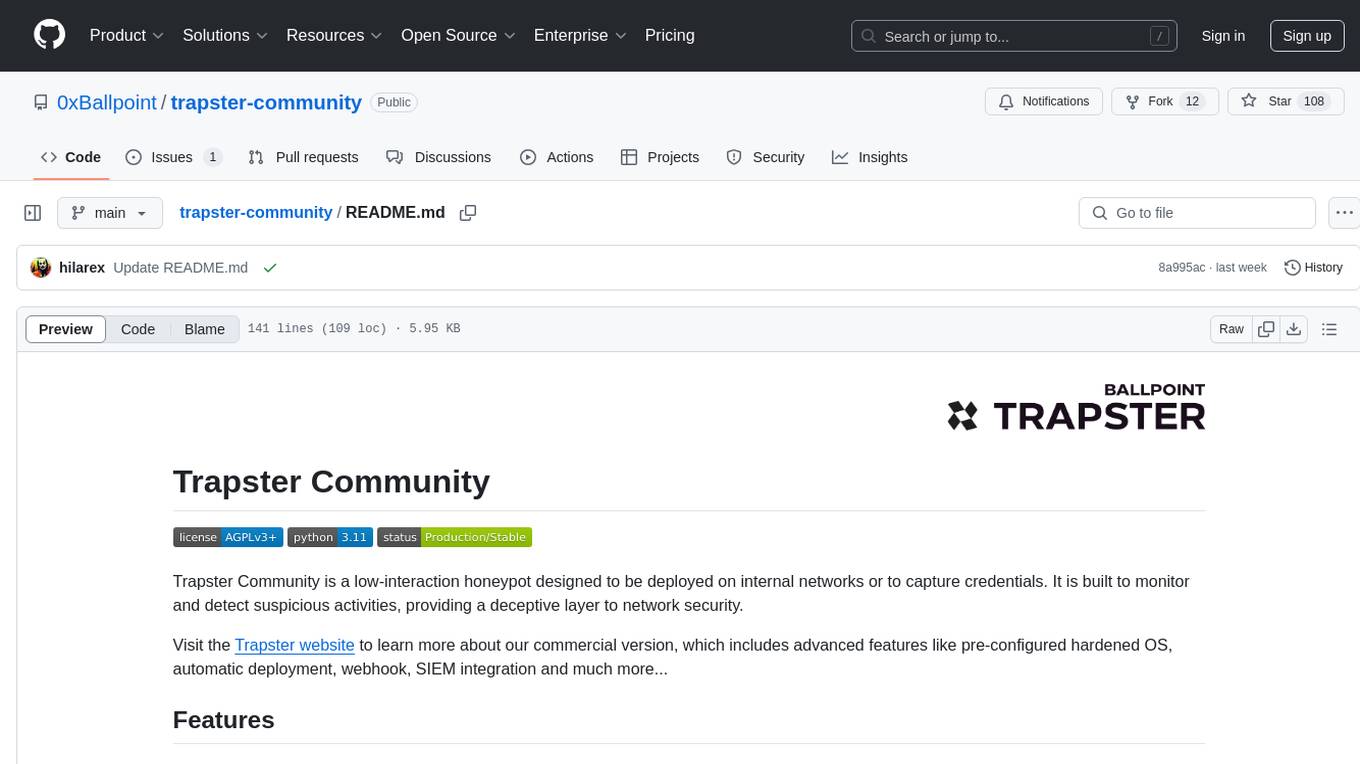
trapster-community
Trapster Community is a low-interaction honeypot designed for internal networks or credential capture. It monitors and detects suspicious activities, providing deceptive security layer. Features include mimicking network services, asynchronous framework, easy configuration, expandable services, and HTTP honeypot engine with AI capabilities. Supported protocols include DNS, HTTP/HTTPS, FTP, LDAP, MSSQL, POSTGRES, RDP, SNMP, SSH, TELNET, VNC, and RSYNC. The tool generates various types of logs and offers HTTP engine with AI capabilities to emulate websites using YAML configuration. Contributions are welcome under AGPLv3+ license.
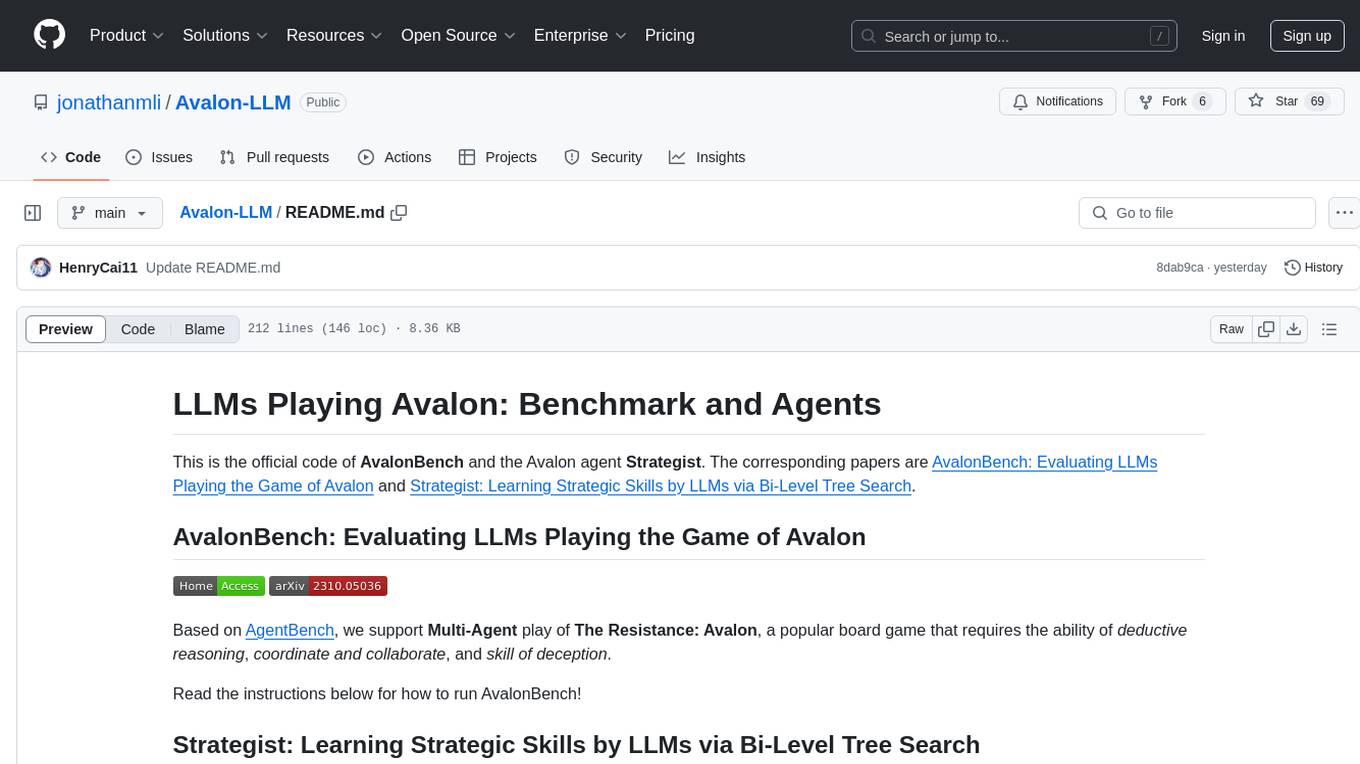
Avalon-LLM
Avalon-LLM is a repository containing the official code for AvalonBench and the Avalon agent Strategist. AvalonBench evaluates Large Language Models (LLMs) playing The Resistance: Avalon, a board game requiring deductive reasoning, coordination, collaboration, and deception skills. Strategist utilizes LLMs to learn strategic skills through self-improvement, including high-level strategic evaluation and low-level execution guidance. The repository provides instructions for running AvalonBench, setting up Strategist, and conducting experiments with different agents in the game environment.
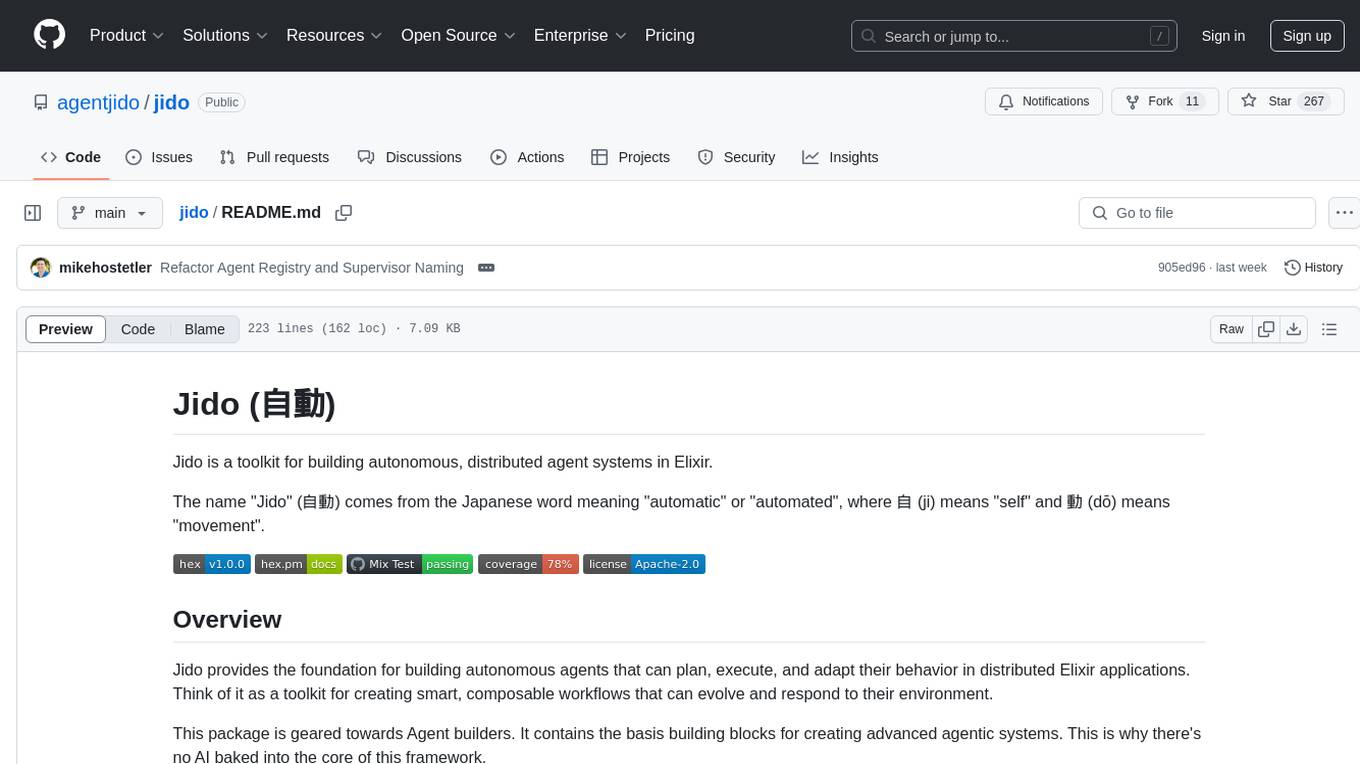
jido
Jido is a toolkit for building autonomous, distributed agent systems in Elixir. It provides the foundation for creating smart, composable workflows that can evolve and respond to their environment. Geared towards Agent builders, it contains core state primitives, composable actions, agent data structures, real-time sensors, signal system, skills, and testing tools. Jido is designed for multi-node Elixir clusters and offers rich helpers for unit and property-based testing.
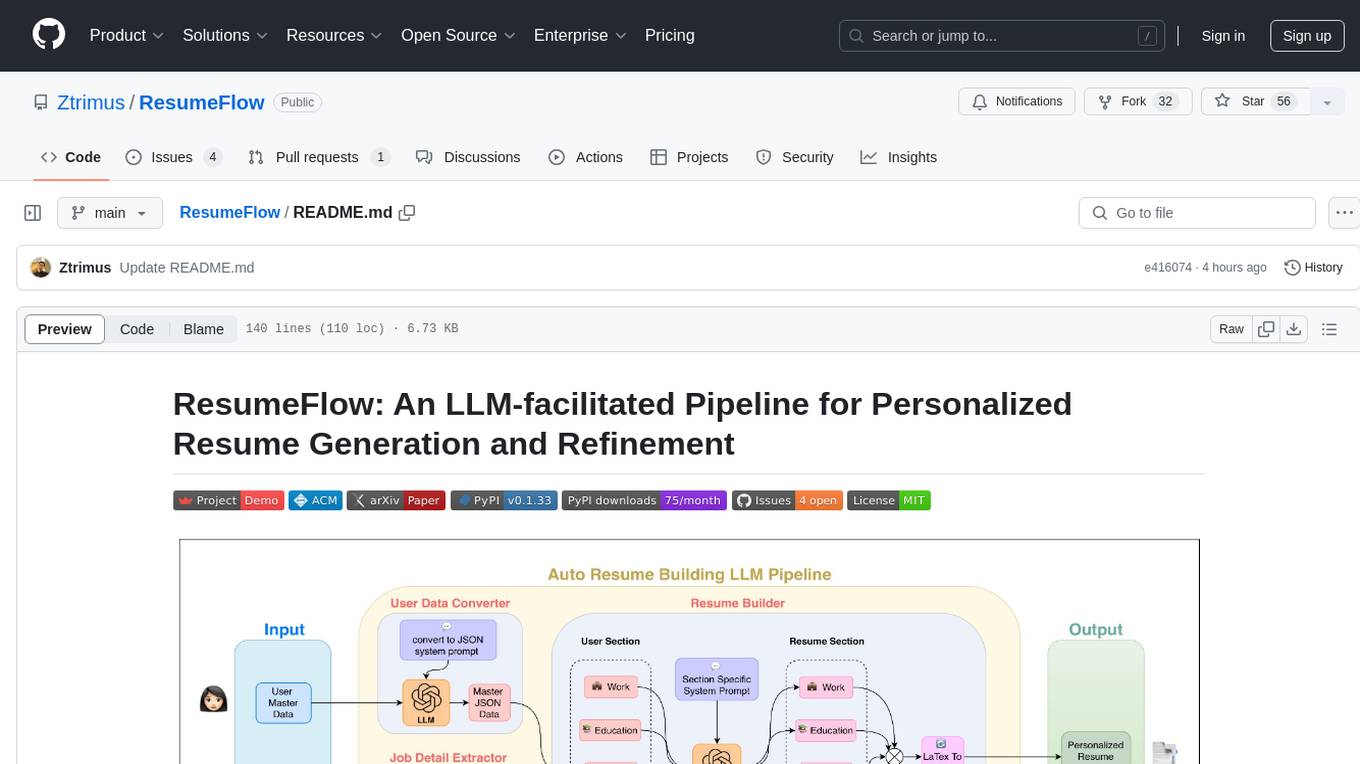
ResumeFlow
ResumeFlow is an automated system that leverages Large Language Models (LLMs) to streamline the job application process. By integrating LLM technology, the tool aims to automate various stages of job hunting, making it easier for users to apply for jobs. Users can access ResumeFlow as a web tool, install it as a Python package, or download the source code from GitHub. The tool requires Python 3.11.6 or above and an LLM API key from OpenAI or Gemini Pro for usage. ResumeFlow offers functionalities such as generating curated resumes and cover letters based on job URLs and user's master resume data.
For similar tasks
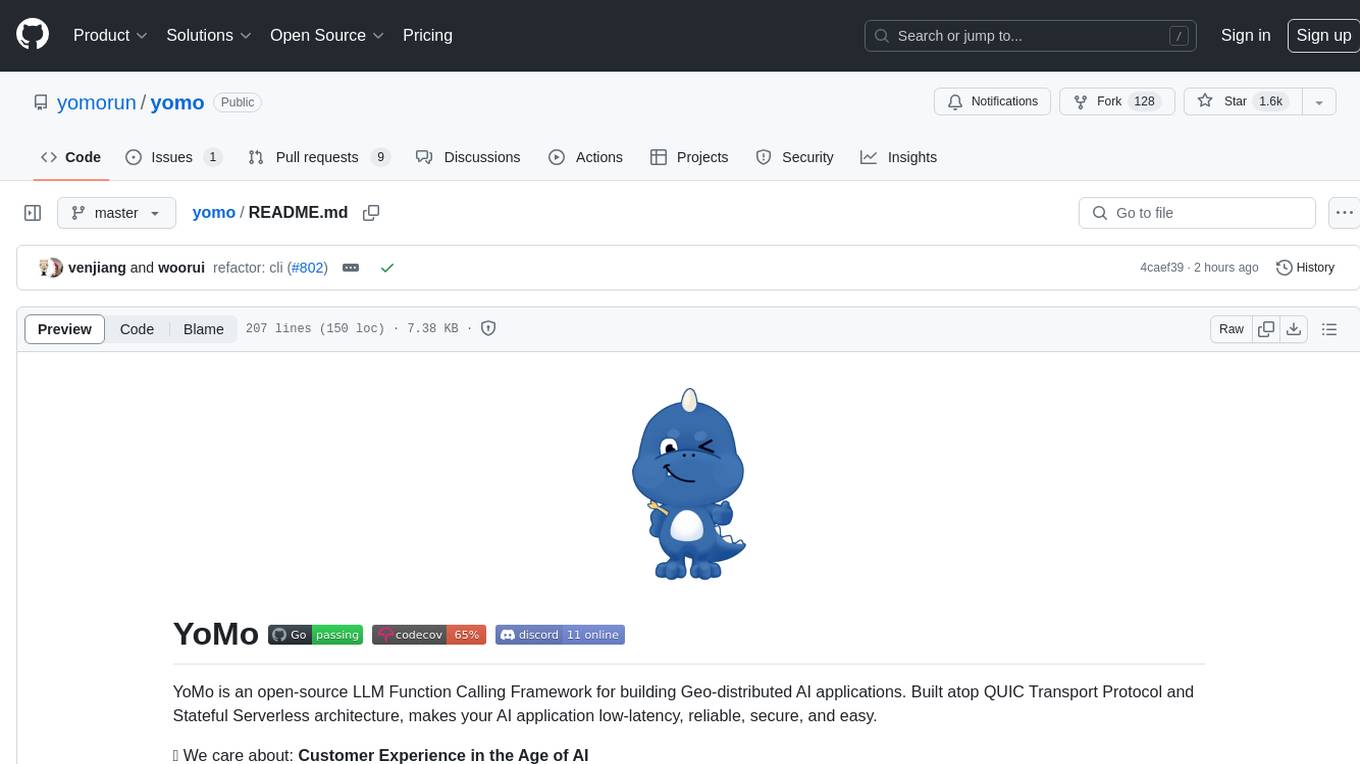
yomo
YoMo is an open-source LLM Function Calling Framework for building Geo-distributed AI applications. It is built atop QUIC Transport Protocol and Stateful Serverless architecture, making AI applications low-latency, reliable, secure, and easy. The framework focuses on providing low-latency, secure, stateful serverless functions that can be distributed geographically to bring AI inference closer to end users. It offers features such as low-latency communication, security with TLS v1.3, stateful serverless functions for faster GPU processing, geo-distributed architecture, and a faster-than-real-time codec called Y3. YoMo enables developers to create and deploy stateful serverless functions for AI inference in a distributed manner, ensuring quick responses to user queries from various locations worldwide.
For similar jobs
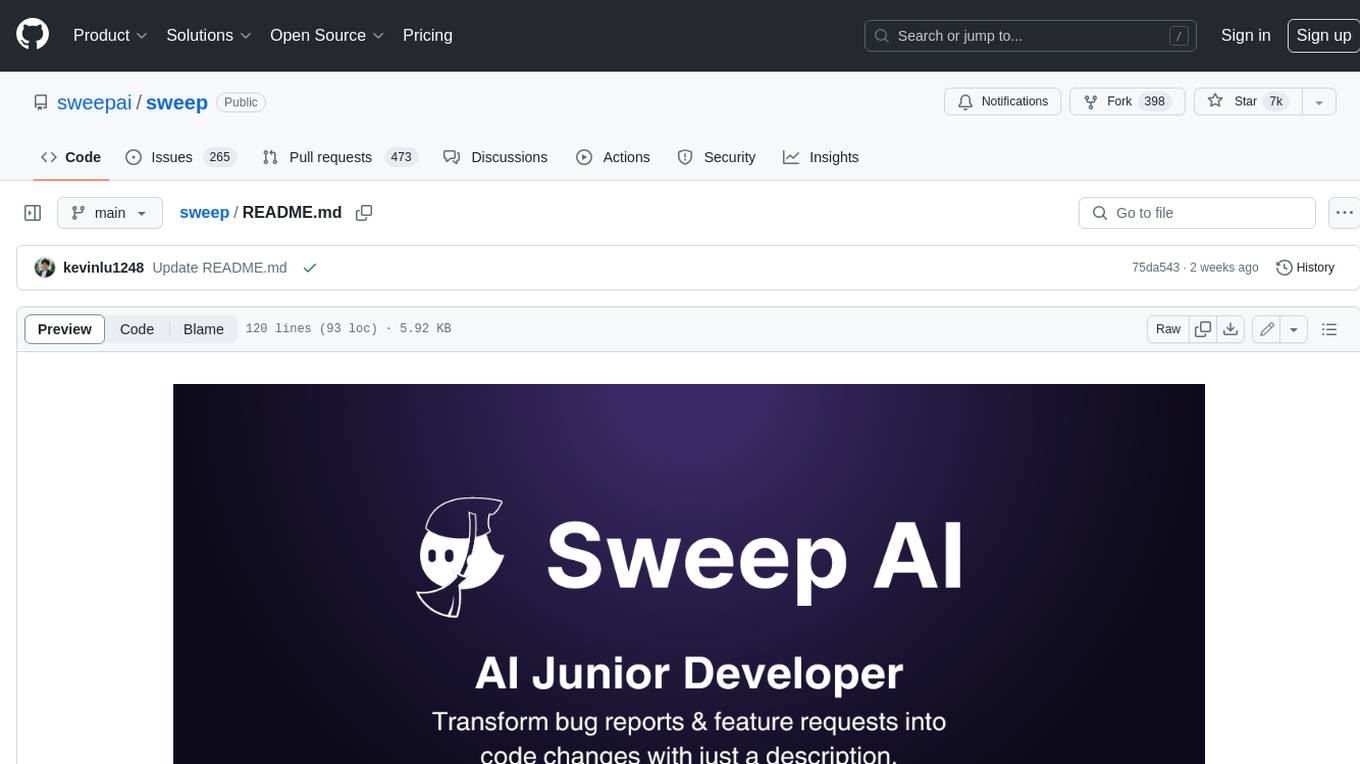
sweep
Sweep is an AI junior developer that turns bugs and feature requests into code changes. It automatically handles developer experience improvements like adding type hints and improving test coverage.
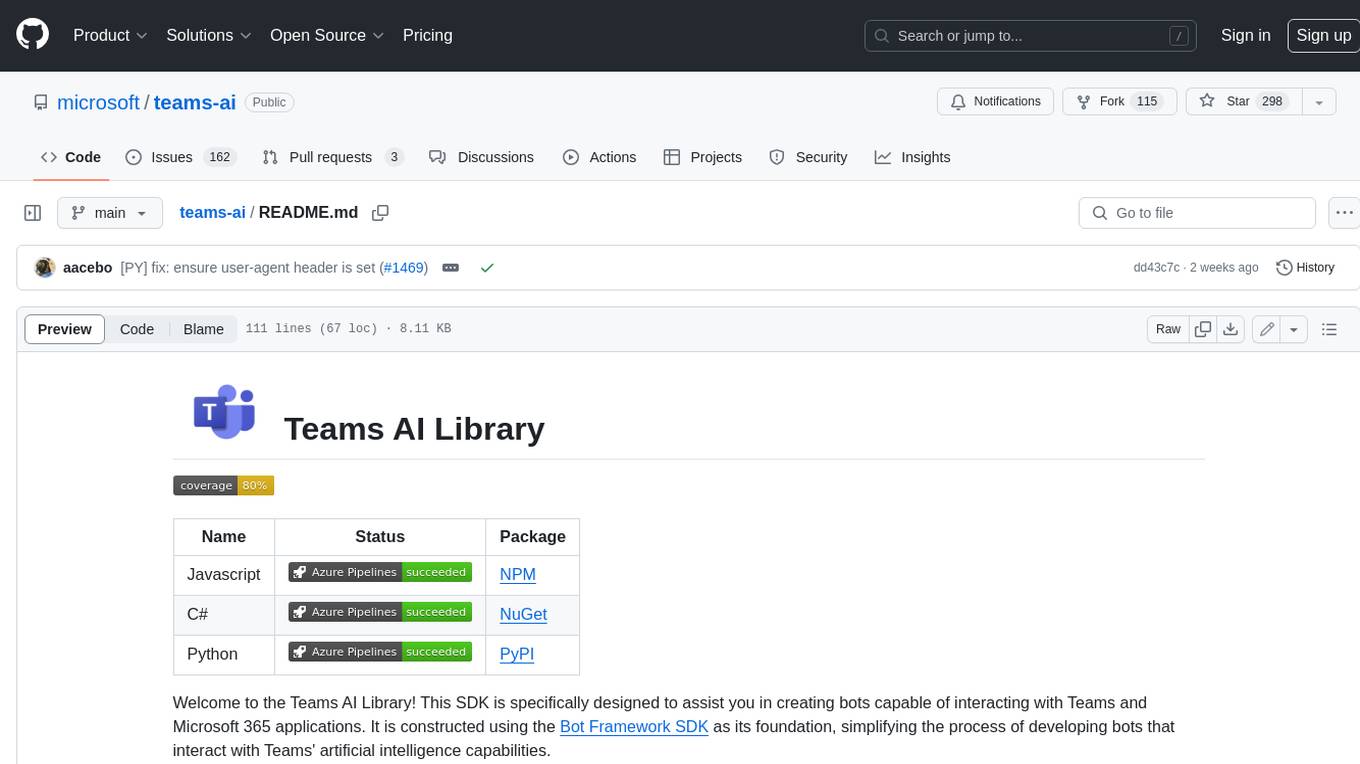
teams-ai
The Teams AI Library is a software development kit (SDK) that helps developers create bots that can interact with Teams and Microsoft 365 applications. It is built on top of the Bot Framework SDK and simplifies the process of developing bots that interact with Teams' artificial intelligence capabilities. The SDK is available for JavaScript/TypeScript, .NET, and Python.
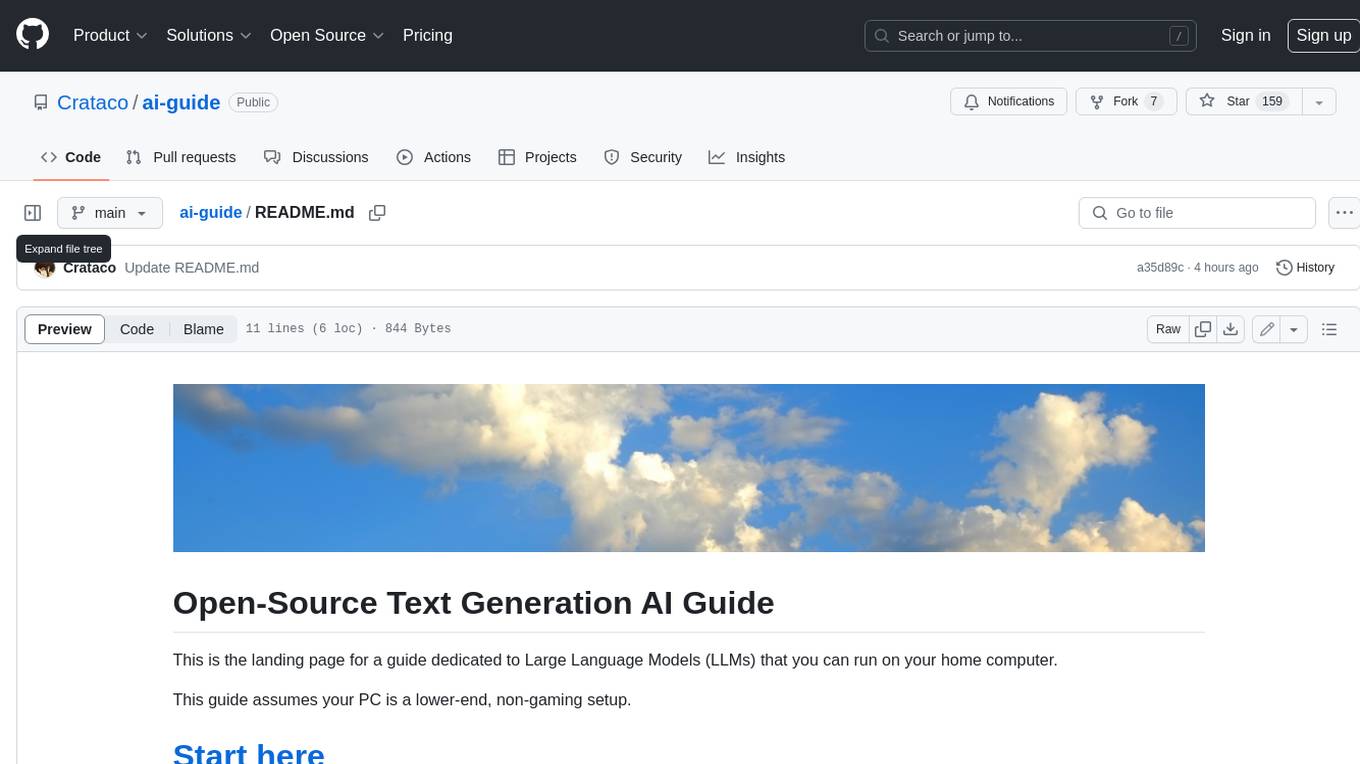
ai-guide
This guide is dedicated to Large Language Models (LLMs) that you can run on your home computer. It assumes your PC is a lower-end, non-gaming setup.
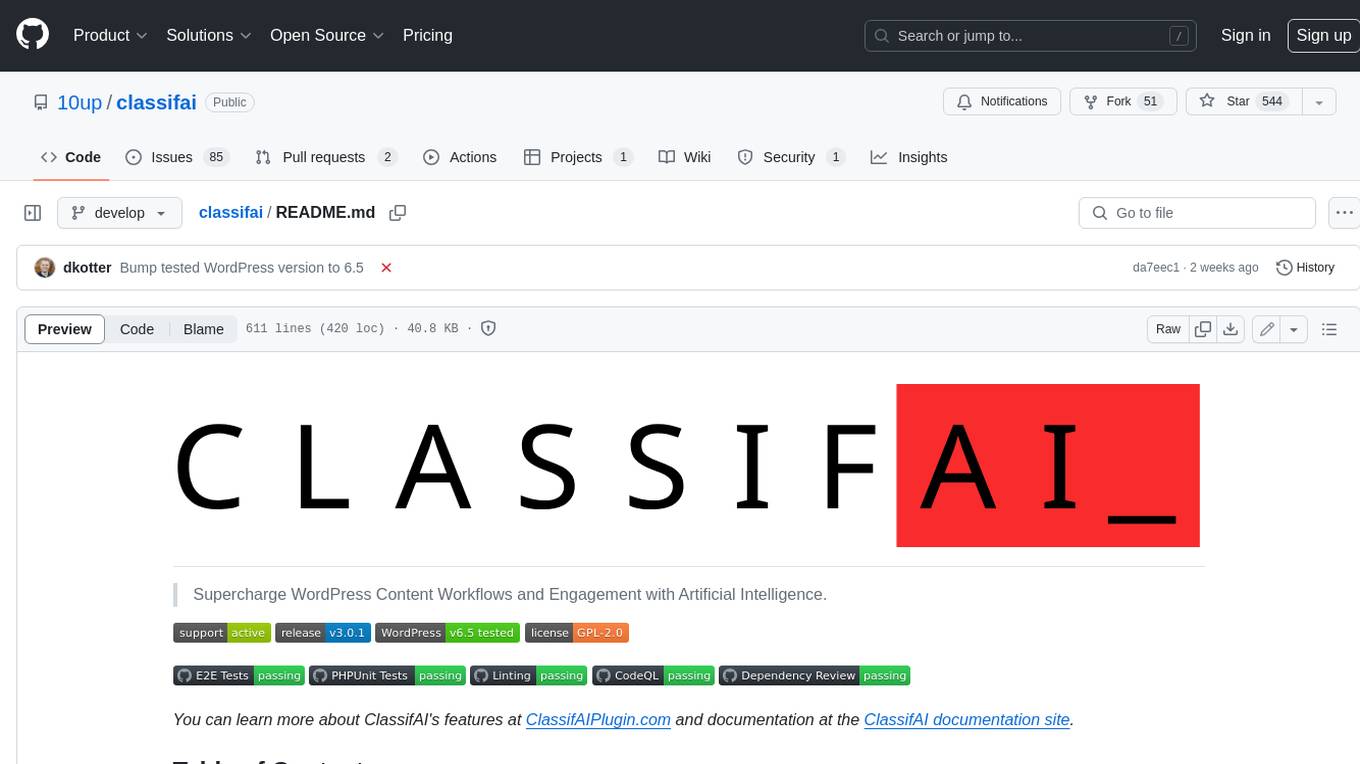
classifai
Supercharge WordPress Content Workflows and Engagement with Artificial Intelligence. Tap into leading cloud-based services like OpenAI, Microsoft Azure AI, Google Gemini and IBM Watson to augment your WordPress-powered websites. Publish content faster while improving SEO performance and increasing audience engagement. ClassifAI integrates Artificial Intelligence and Machine Learning technologies to lighten your workload and eliminate tedious tasks, giving you more time to create original content that matters.
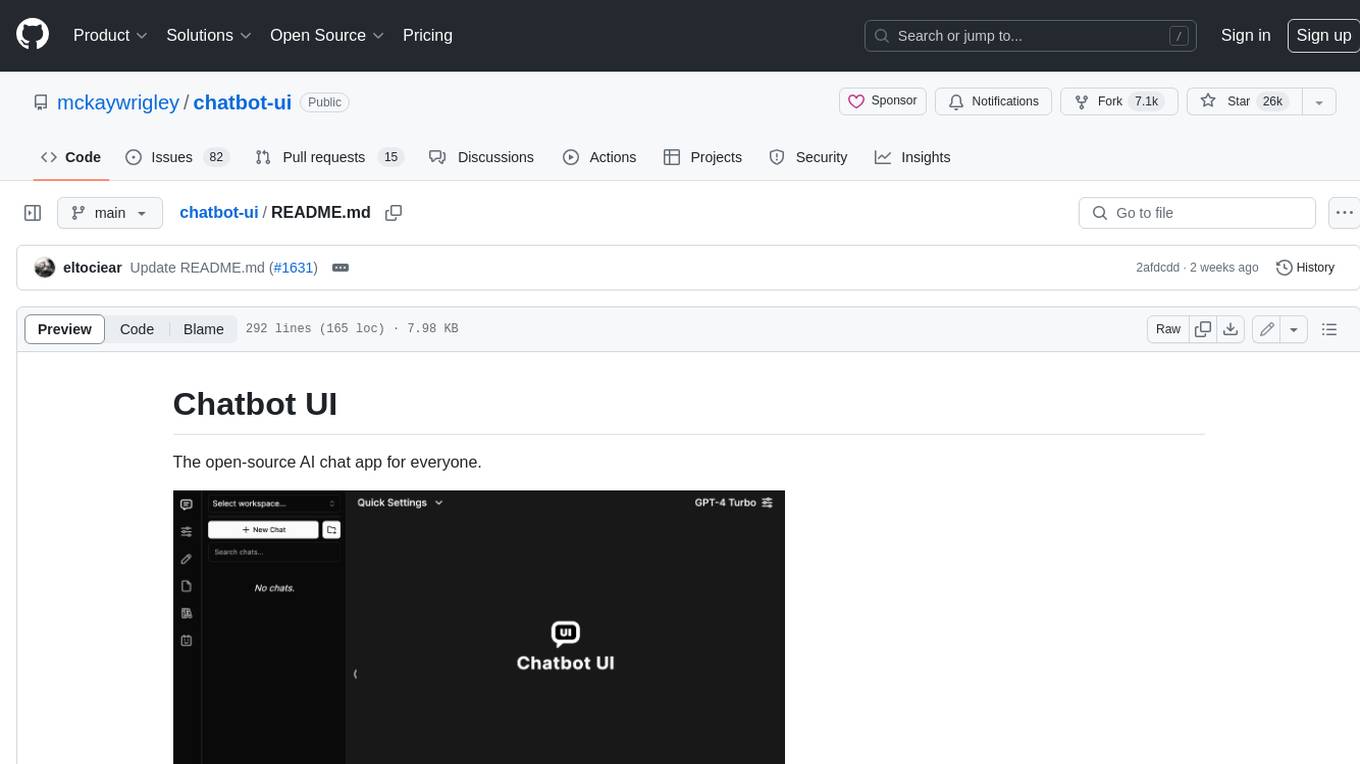
chatbot-ui
Chatbot UI is an open-source AI chat app that allows users to create and deploy their own AI chatbots. It is easy to use and can be customized to fit any need. Chatbot UI is perfect for businesses, developers, and anyone who wants to create a chatbot.
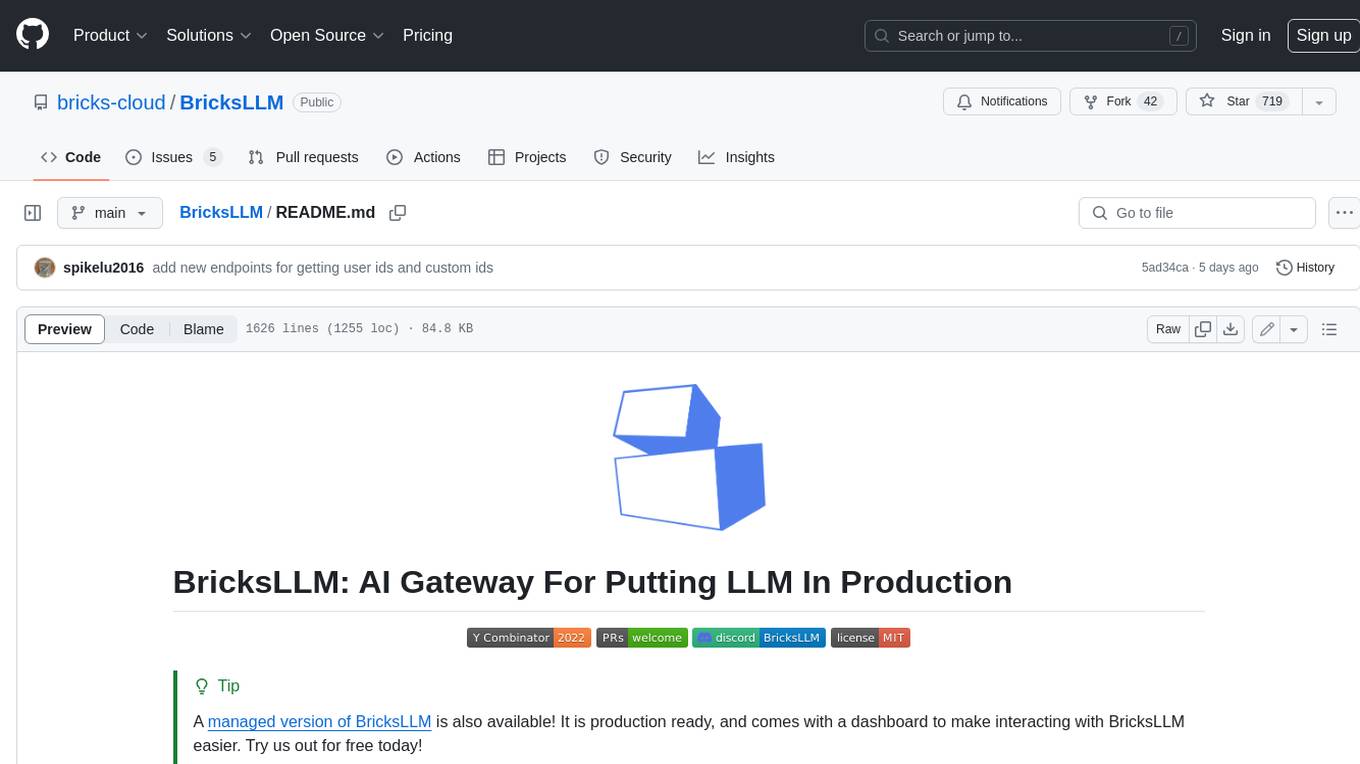
BricksLLM
BricksLLM is a cloud native AI gateway written in Go. Currently, it provides native support for OpenAI, Anthropic, Azure OpenAI and vLLM. BricksLLM aims to provide enterprise level infrastructure that can power any LLM production use cases. Here are some use cases for BricksLLM: * Set LLM usage limits for users on different pricing tiers * Track LLM usage on a per user and per organization basis * Block or redact requests containing PIIs * Improve LLM reliability with failovers, retries and caching * Distribute API keys with rate limits and cost limits for internal development/production use cases * Distribute API keys with rate limits and cost limits for students
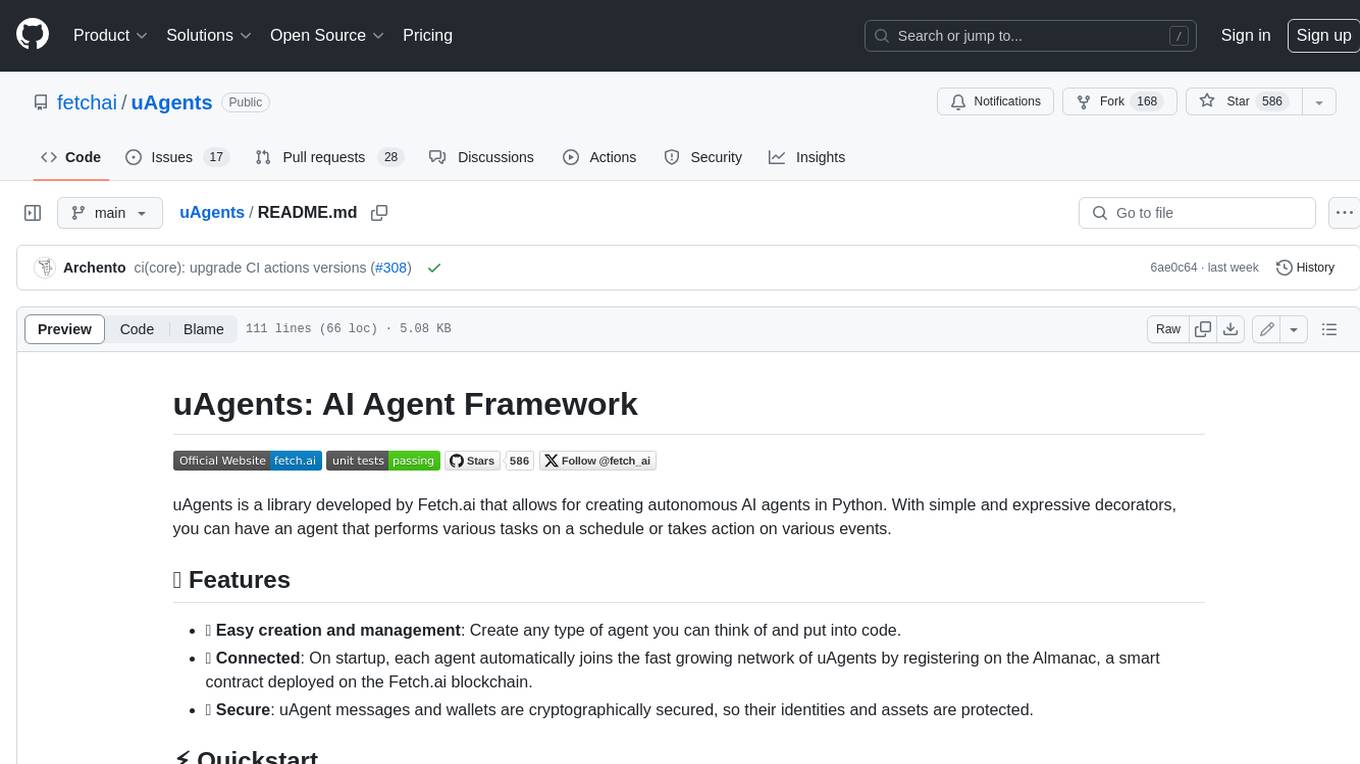
uAgents
uAgents is a Python library developed by Fetch.ai that allows for the creation of autonomous AI agents. These agents can perform various tasks on a schedule or take action on various events. uAgents are easy to create and manage, and they are connected to a fast-growing network of other uAgents. They are also secure, with cryptographically secured messages and wallets.
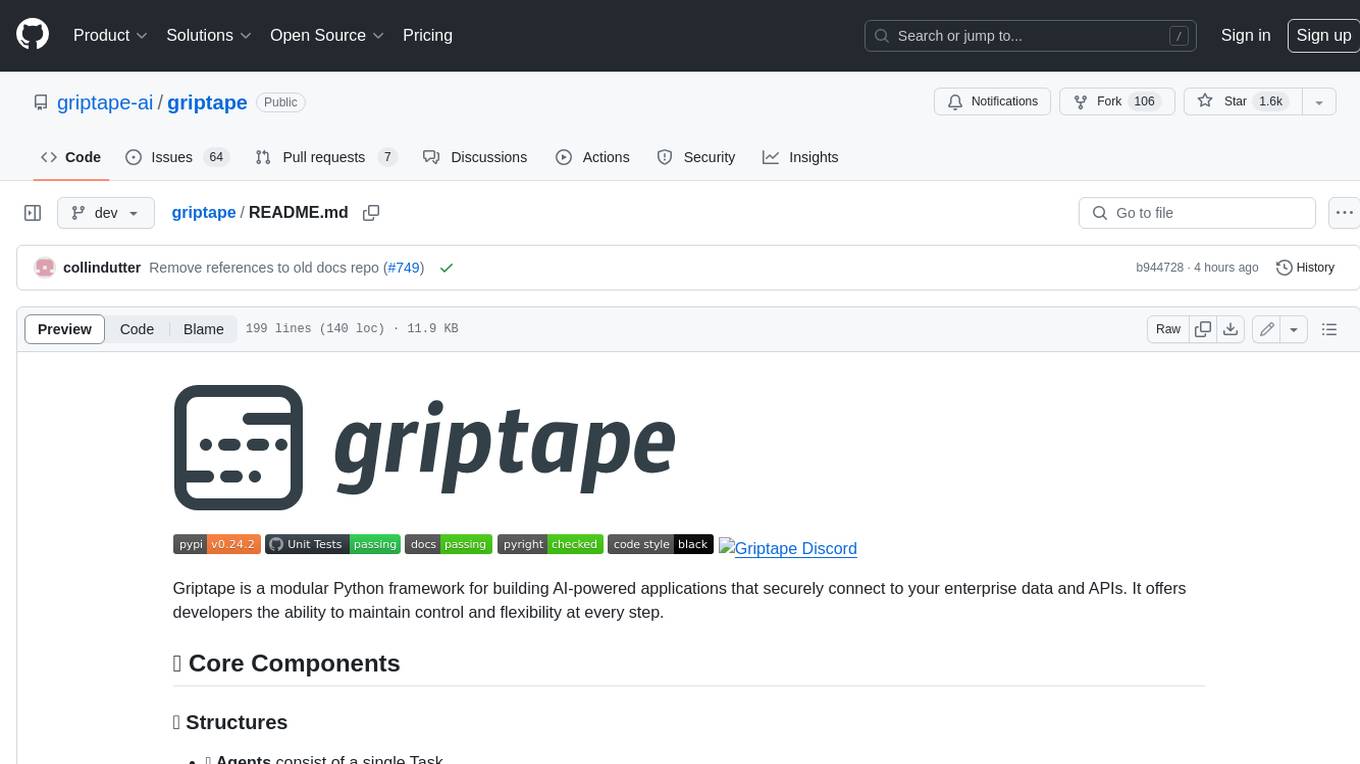
griptape
Griptape is a modular Python framework for building AI-powered applications that securely connect to your enterprise data and APIs. It offers developers the ability to maintain control and flexibility at every step. Griptape's core components include Structures (Agents, Pipelines, and Workflows), Tasks, Tools, Memory (Conversation Memory, Task Memory, and Meta Memory), Drivers (Prompt and Embedding Drivers, Vector Store Drivers, Image Generation Drivers, Image Query Drivers, SQL Drivers, Web Scraper Drivers, and Conversation Memory Drivers), Engines (Query Engines, Extraction Engines, Summary Engines, Image Generation Engines, and Image Query Engines), and additional components (Rulesets, Loaders, Artifacts, Chunkers, and Tokenizers). Griptape enables developers to create AI-powered applications with ease and efficiency.