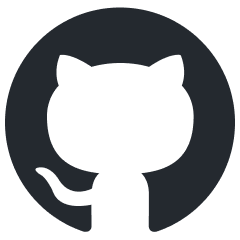
serverless-chat-langchainjs
Build your own serverless AI Chat with Retrieval-Augmented-Generation using LangChain.js, TypeScript and Azure
Stars: 771
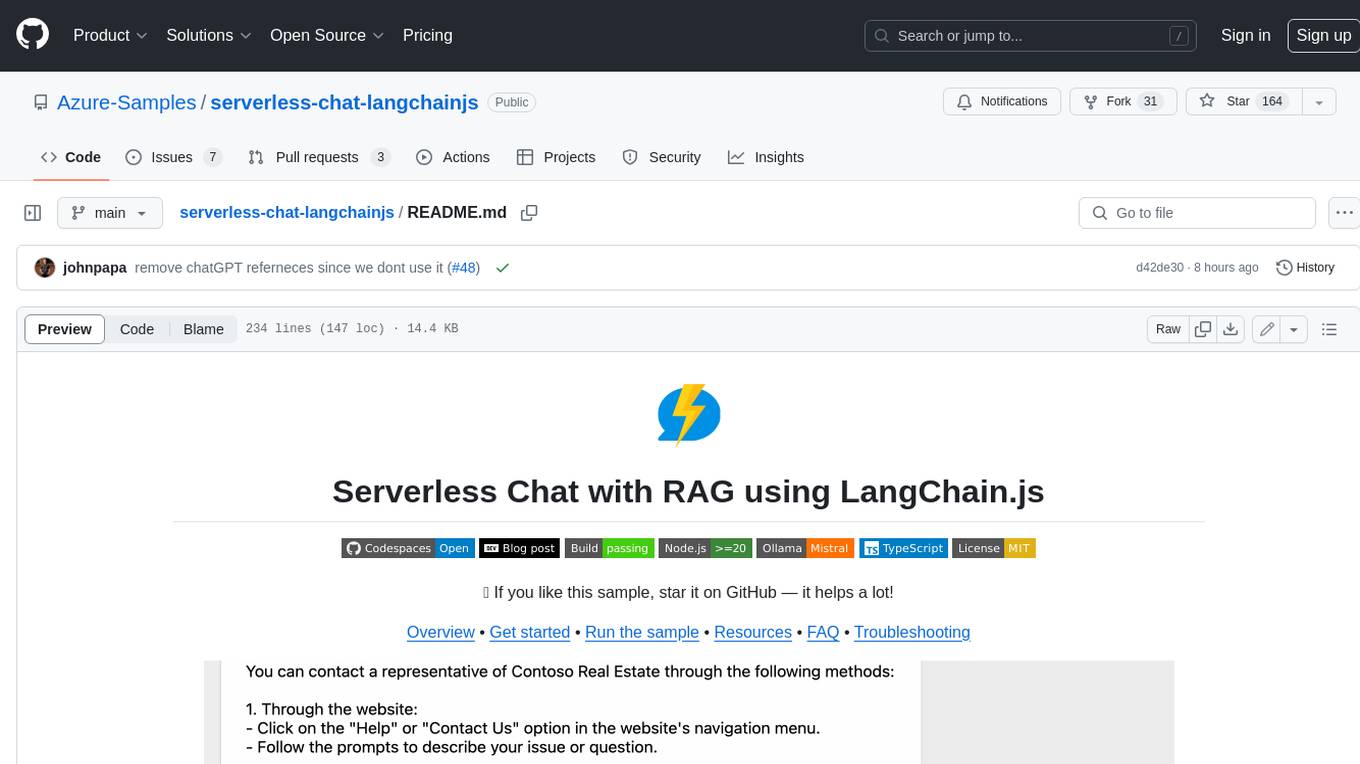
This sample shows how to build a serverless chat experience with Retrieval-Augmented Generation using LangChain.js and Azure. The application is hosted on Azure Static Web Apps and Azure Functions, with Azure Cosmos DB for MongoDB vCore as the vector database. You can use it as a starting point for building more complex AI applications.
README:

⭐ If you like this sample, star it on GitHub — it helps a lot!
Overview • Get started • Run the sample • Resources • FAQ • Troubleshooting
This sample shows how to build a serverless AI chat experience with Retrieval-Augmented Generation using LangChain.js and Azure. The application is hosted on Azure Static Web Apps and Azure Functions, with Azure Cosmos DB for NoSQL as the vector database. You can use it as a starting point for building more complex AI applications.
[!TIP] You can test this application locally without any cost using Ollama. Follow the instructions in the Local Development section to get started.
Building AI applications can be complex and time-consuming, but using LangChain.js and Azure serverless technologies allows to greatly simplify the process. This application is a chatbot that uses a set of enterprise documents to generate responses to user queries.
We provide sample data to make this sample ready to try, but feel free to replace it with your own. We use a fictitious company called Contoso Real Estate, and the experience allows its customers to ask support questions about the usage of its products. The sample data includes a set of documents that describes its terms of service, privacy policy and a support guide.
This application is made from multiple components:
-
A web app made with a single chat web component built with Lit and hosted on Azure Static Web Apps. The code is located in the
packages/webapp
folder. -
A serverless API built with Azure Functions and using LangChain.js to ingest the documents and generate responses to the user chat queries. The code is located in the
packages/api
folder. -
A database to store chat sessions and the text extracted from the documents and the vectors generated by LangChain.js, using Azure Cosmos DB for NoSQL.
-
A file storage to store the source documents, using Azure Blob Storage.
We use the HTTP protocol for AI chat apps to communicate between the web app and the API.
- Serverless Architecture: Utilizes Azure Functions and Azure Static Web Apps for a fully serverless deployment.
- Retrieval-Augmented Generation (RAG): Combines the power of Azure Cosmos DB and LangChain.js to provide relevant and accurate responses.
- Chat Sessions History: Maintains a personal chat history for each user, allowing them to revisit previous conversations.
- Scalable and Cost-Effective: Leverages Azure's serverless offerings to provide a scalable and cost-effective solution.
- Local Development: Supports local development using Ollama for testing without any cloud costs.
There are multiple ways to get started with this project.
The quickest way is to use GitHub Codespaces that provides a preconfigured environment for you. Alternatively, you can set up your local environment following the instructions below.
[!IMPORTANT] If you want to run this sample entirely locally using Ollama, you have to follow the instructions in the local environment section.
You need to install following tools to work on your local machine:
- Node.js LTS
- Azure Developer CLI
- Git
-
PowerShell 7+ (for Windows users only)
-
Important: Ensure you can run
pwsh.exe
from a PowerShell command. If this fails, you likely need to upgrade PowerShell. - Instead of Powershell, you can also use Git Bash or WSL to run the Azure Developer CLI commands.
-
Important: Ensure you can run
- Azure Functions Core Tools (should be installed automatically with NPM, only install manually if the API fails to start)
Then you can get the project code:
- Fork the project to create your own copy of this repository.
- On your forked repository, select the Code button, then the Local tab, and copy the URL of your forked repository.
git clone <your-repo-url>
You can run this project directly in your browser by using GitHub Codespaces, which will open a web-based VS Code:
A similar option to Codespaces is VS Code Dev Containers, that will open the project in your local VS Code instance using the Dev Containers extension.
You will also need to have Docker installed on your machine to run the container.
There are multiple ways to run this sample: locally using Ollama or Azure OpenAI models, or by deploying it to Azure.
- Azure account. If you're new to Azure, get an Azure account for free to get free Azure credits to get started. If you're a student, you can also get free credits with Azure for Students.
- Azure subscription with access enabled for the Azure OpenAI service. You can request access with this form.
-
Azure account permissions:
- Your Azure account must have
Microsoft.Authorization/roleAssignments/write
permissions, such as Role Based Access Control Administrator, User Access Administrator, or Owner. If you don't have subscription-level permissions, you must be granted RBAC for an existing resource group and deploy to that existing group. - Your Azure account also needs
Microsoft.Resources/deployments/write
permissions on the subscription level.
- Your Azure account must have
See the cost estimation details for running this sample on Azure.
- Open a terminal and navigate to the root of the project.
- Authenticate with Azure by running
azd auth login
. - Run
azd up
to deploy the application to Azure. This will provision Azure resources, deploy this sample, and build the search index based on the files found in the./data
folder.- You will be prompted to select a base location for the resources. If you're unsure of which location to choose, select
eastus2
. - By default, the OpenAI resource will be deployed to
eastus2
. You can set a different location withazd env set AZURE_OPENAI_RESOURCE_GROUP_LOCATION <location>
. Currently only a short list of locations is accepted. That location list is based on the OpenAI model availability table and may become outdated as availability changes.
- You will be prompted to select a base location for the resources. If you're unsure of which location to choose, select
The deployment process will take a few minutes. Once it's done, you'll see the URL of the web app in the terminal.
You can now open the web app in your browser and start chatting with the bot.
When deploying the sample in an enterprise context, you may want to enforce tighter security restrictions to protect your data and resources. See the enhance security guide for more information.
To clean up all the Azure resources created by this sample:
- Run
azd down --purge
- When asked if you are sure you want to continue, enter
y
The resource group and all the resources will be deleted.
If you have a machine with enough resources, you can run this sample entirely locally without using any cloud resources. To do that, you first have to install Ollama and then run the following commands to download the models on your machine:
ollama pull llama3.1:latest
ollama pull nomic-embed-text:latest
[!NOTE] The
llama3.1
model with download a few gigabytes of data, so it can take some time depending on your internet connection.
After that you have to install the NPM dependencies:
npm install
Then you can start the application by running the following command which will start the web app and the API locally:
npm start
Then, open a new terminal running concurrently and run the following command to upload the PDF documents from the /data
folder to the API:
npm run upload:docs
This only has to be done once, unless you want to add more documents.
You can now open the URL http://localhost:8000
in your browser to start chatting with the bot.
[!NOTE] While local models usually works well enough to answer the questions, sometimes they may not be able to follow perfectly the advanced formatting instructions for the citations and follow-up questions. This is expected, and a limitation of using smaller local models.
First you need to provision the Azure resources needed to run the sample. Follow the instructions in the Deploy the sample to Azure section to deploy the sample to Azure, then you'll be able to run the sample locally using the deployed Azure resources.
Once your deployment is complete, you should see a .env
file in the packages/api
folder. This file contains the environment variables needed to run the application using Azure resources.
To run the sample, you can then use the same commands as for the Ollama setup. This will start the web app and the API locally:
npm start
Open the URL http://localhost:8000
in your browser to start chatting with the bot.
Note that the documents are uploaded automatically when deploying the sample to Azure with azd up
.
[!TIP] You can switch back to using Ollama models by simply deleting the
packages/api/.env
file and starting the application again. To regenerate the.env
file, you can runazd env get-values > packages/api/.env
.
Here are some resources to learn more about the technologies used in this sample:
- LangChain.js documentation
- Generative AI with JavaScript
- Generative AI For Beginners
- Azure OpenAI Service
- Azure Cosmos DB for NoSQL
- Ask YouTube: LangChain.js + Azure Quickstart sample
- Chat + Enterprise data with Azure OpenAI and Azure AI Search
- Revolutionize your Enterprise Data with Chat: Next-gen Apps w/ Azure OpenAI and AI Search
You can also find more Azure AI samples here.
You can find answers to frequently asked questions in the FAQ.
If you have any issue when running or deploying this sample, please check the troubleshooting guide. If you can't find a solution to your problem, please open an issue in this repository.
For more detailed guidance on how to use this sample, please refer to the tutorial.
This project welcomes contributions and suggestions. Most contributions require you to agree to a Contributor License Agreement (CLA) declaring that you have the right to, and actually do, grant us the rights to use your contribution. For details, visit https://cla.opensource.microsoft.com.
When you submit a pull request, a CLA bot will automatically determine whether you need to provide a CLA and decorate the PR appropriately (e.g., status check, comment). Simply follow the instructions provided by the bot. You will only need to do this once across all repos using our CLA.
This project has adopted the Microsoft Open Source Code of Conduct. For more information see the Code of Conduct FAQ or contact [email protected] with any additional questions or comments.
This project may contain trademarks or logos for projects, products, or services. Authorized use of Microsoft trademarks or logos is subject to and must follow Microsoft's Trademark & Brand Guidelines. Use of Microsoft trademarks or logos in modified versions of this project must not cause confusion or imply Microsoft sponsorship. Any use of third-party trademarks or logos are subject to those third-party's policies.
For Tasks:
Click tags to check more tools for each tasksFor Jobs:
Alternative AI tools for serverless-chat-langchainjs
Similar Open Source Tools
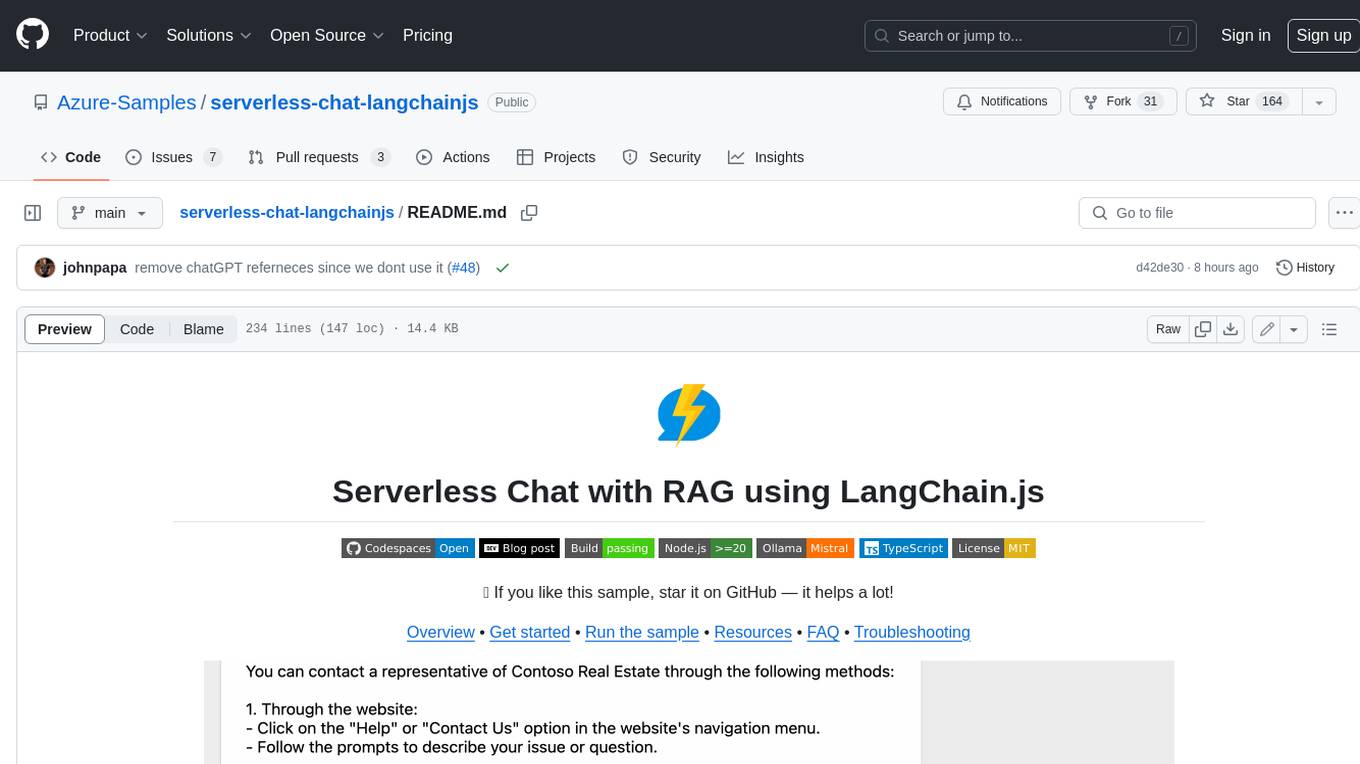
serverless-chat-langchainjs
This sample shows how to build a serverless chat experience with Retrieval-Augmented Generation using LangChain.js and Azure. The application is hosted on Azure Static Web Apps and Azure Functions, with Azure Cosmos DB for MongoDB vCore as the vector database. You can use it as a starting point for building more complex AI applications.
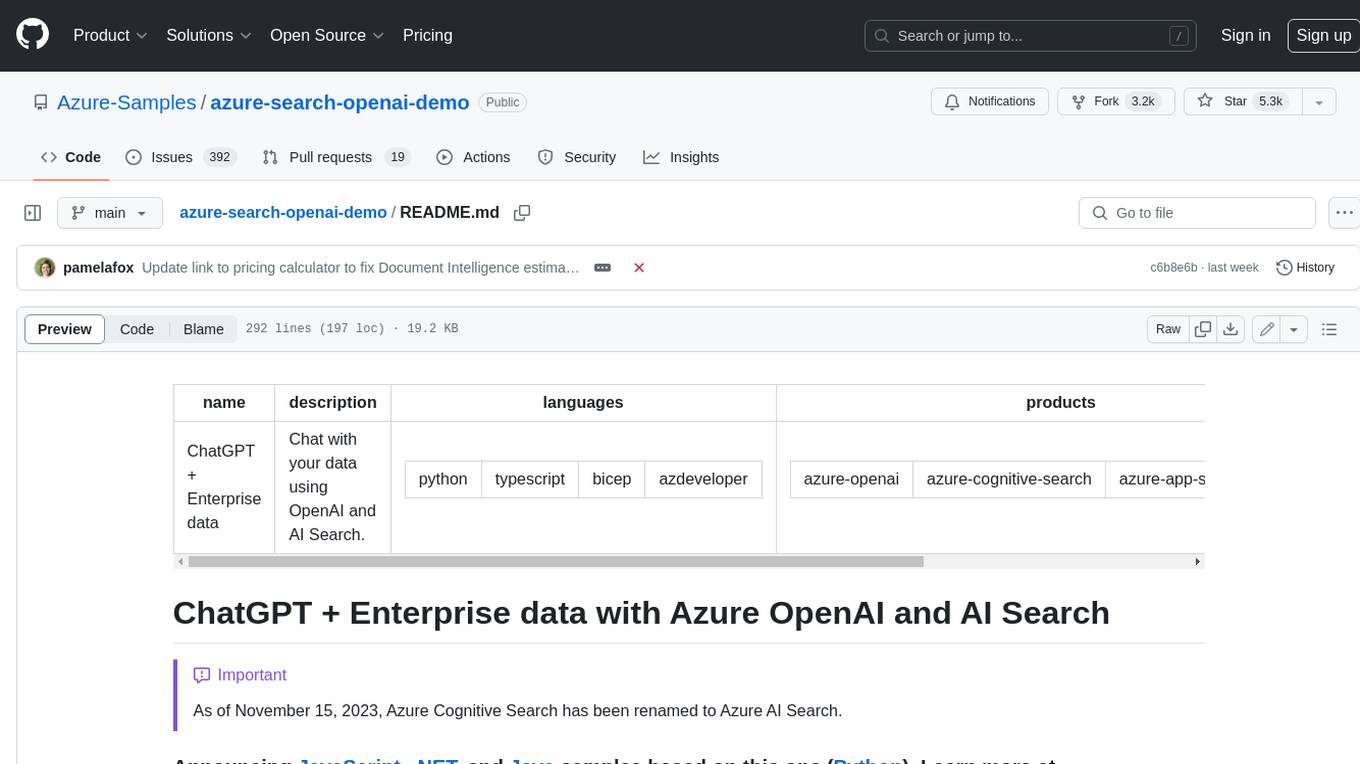
azure-search-openai-demo
This sample demonstrates a few approaches for creating ChatGPT-like experiences over your own data using the Retrieval Augmented Generation pattern. It uses Azure OpenAI Service to access a GPT model (gpt-35-turbo), and Azure AI Search for data indexing and retrieval. The repo includes sample data so it's ready to try end to end. In this sample application we use a fictitious company called Contoso Electronics, and the experience allows its employees to ask questions about the benefits, internal policies, as well as job descriptions and roles.
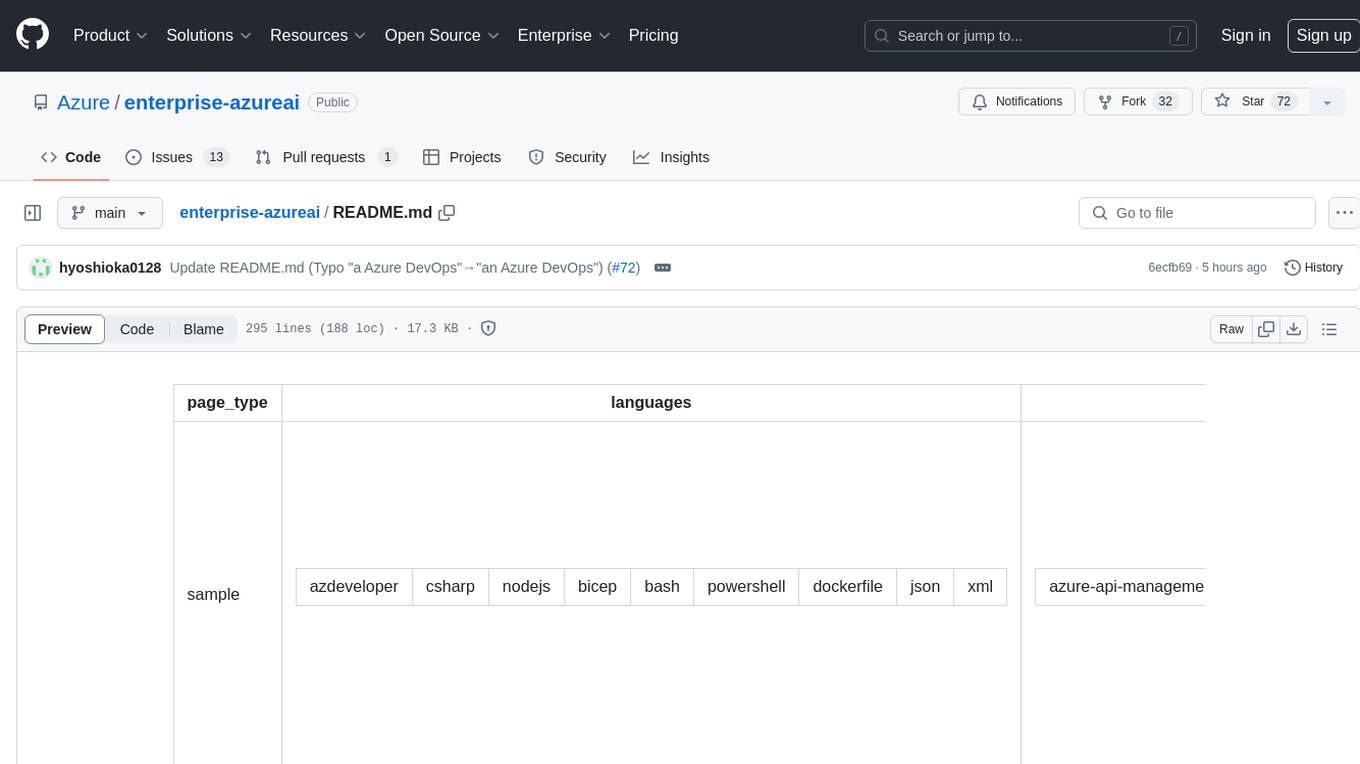
enterprise-azureai
Azure OpenAI Service is a central capability with Azure API Management, providing guidance and tools for organizations to implement Azure OpenAI in a production environment with an emphasis on cost control, secure access, and usage monitoring. It includes infrastructure-as-code templates, CI/CD pipelines, secure access management, usage monitoring, load balancing, streaming requests, and end-to-end samples like ChatApp and Azure Dashboards.
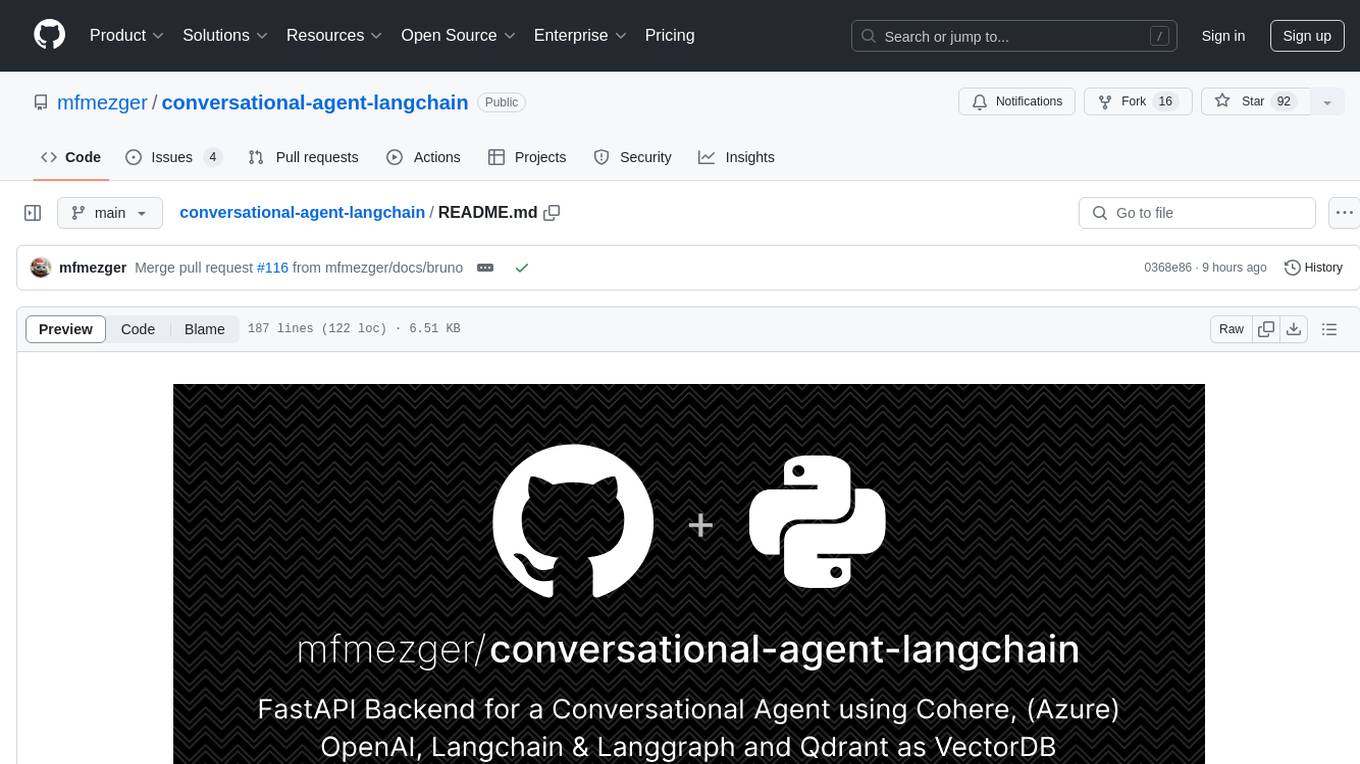
conversational-agent-langchain
This repository contains a Rest-Backend for a Conversational Agent that allows embedding documents, semantic search, QA based on documents, and document processing with Large Language Models. It uses Aleph Alpha and OpenAI Large Language Models to generate responses to user queries, includes a vector database, and provides a REST API built with FastAPI. The project also features semantic search, secret management for API keys, installation instructions, and development guidelines for both backend and frontend components.
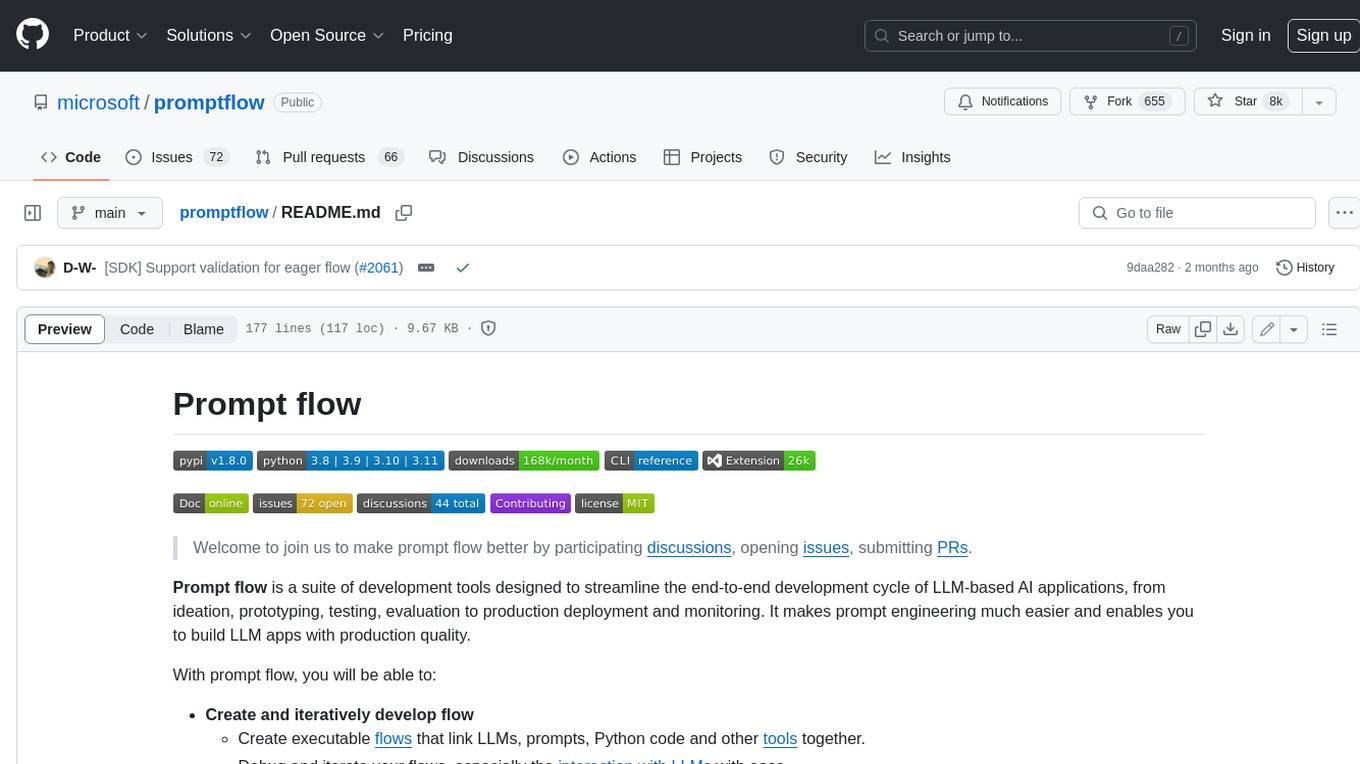
promptflow
**Prompt flow** is a suite of development tools designed to streamline the end-to-end development cycle of LLM-based AI applications, from ideation, prototyping, testing, evaluation to production deployment and monitoring. It makes prompt engineering much easier and enables you to build LLM apps with production quality.
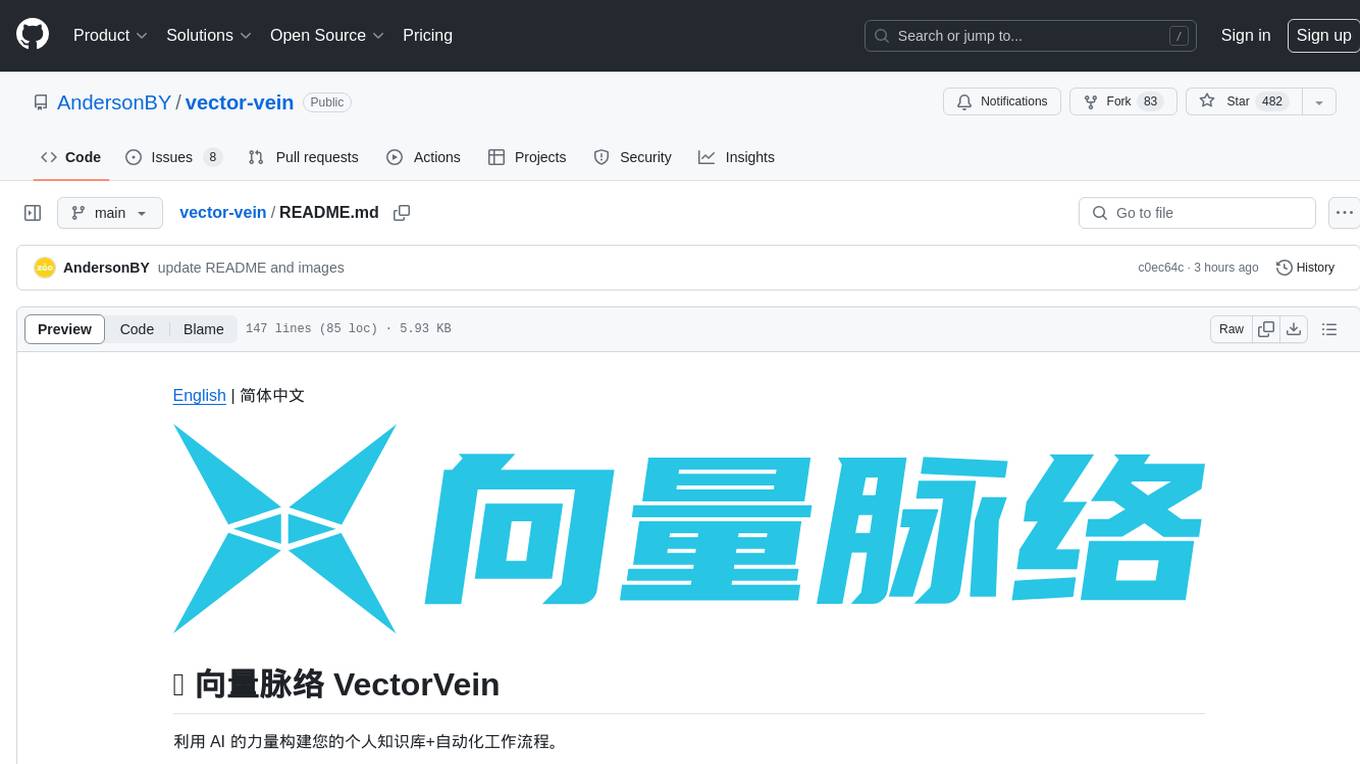
vector-vein
VectorVein is a no-code AI workflow software inspired by LangChain and langflow, aiming to combine the powerful capabilities of large language models and enable users to achieve intelligent and automated daily workflows through simple drag-and-drop actions. Users can create powerful workflows without the need for programming, automating all tasks with ease. The software allows users to define inputs, outputs, and processing methods to create customized workflow processes for various tasks such as translation, mind mapping, summarizing web articles, and automatic categorization of customer reviews.
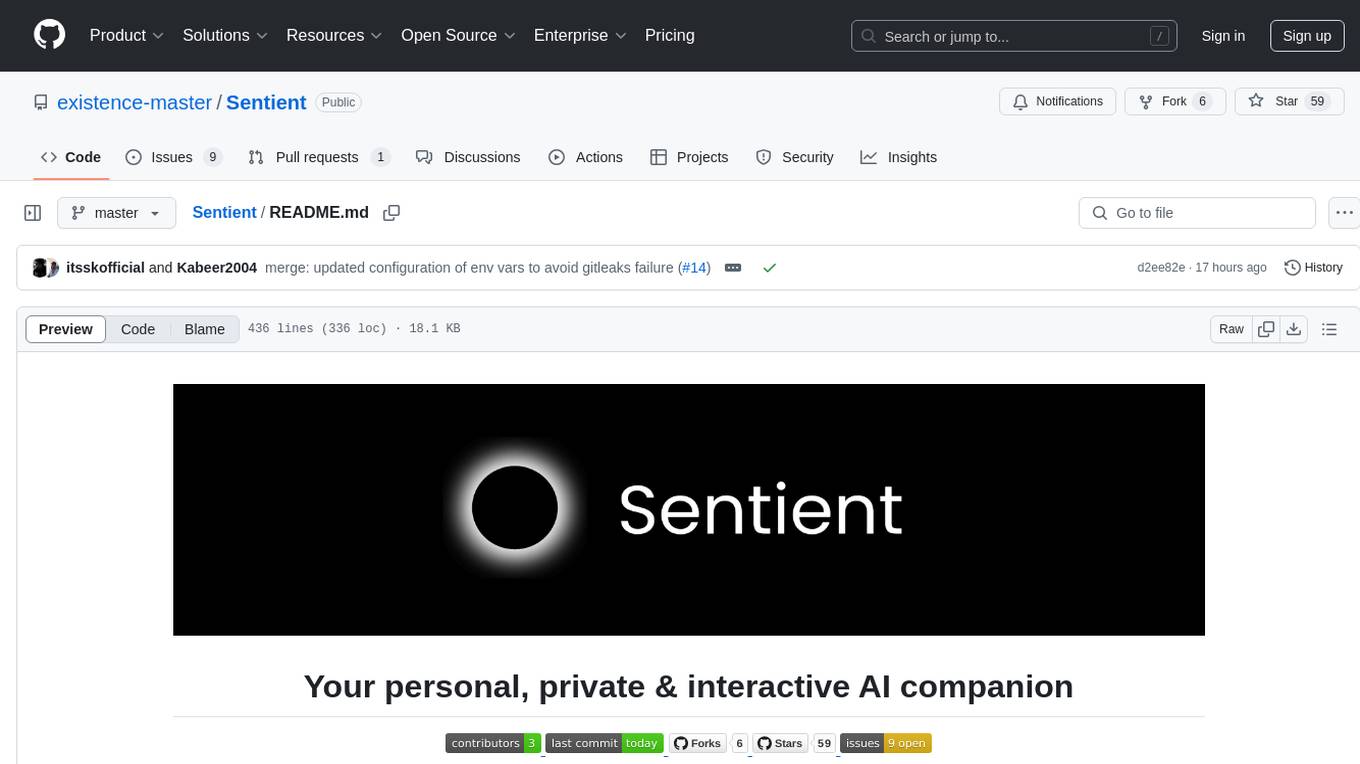
Sentient
Sentient is a personal, private, and interactive AI companion developed by Existence. The project aims to build a completely private AI companion that is deeply personalized and context-aware of the user. It utilizes automation and privacy to create a true companion for humans. The tool is designed to remember information about the user and use it to respond to queries and perform various actions. Sentient features a local and private environment, MBTI personality test, integrations with LinkedIn, Reddit, and more, self-managed graph memory, web search capabilities, multi-chat functionality, and auto-updates for the app. The project is built using technologies like ElectronJS, Next.js, TailwindCSS, FastAPI, Neo4j, and various APIs.
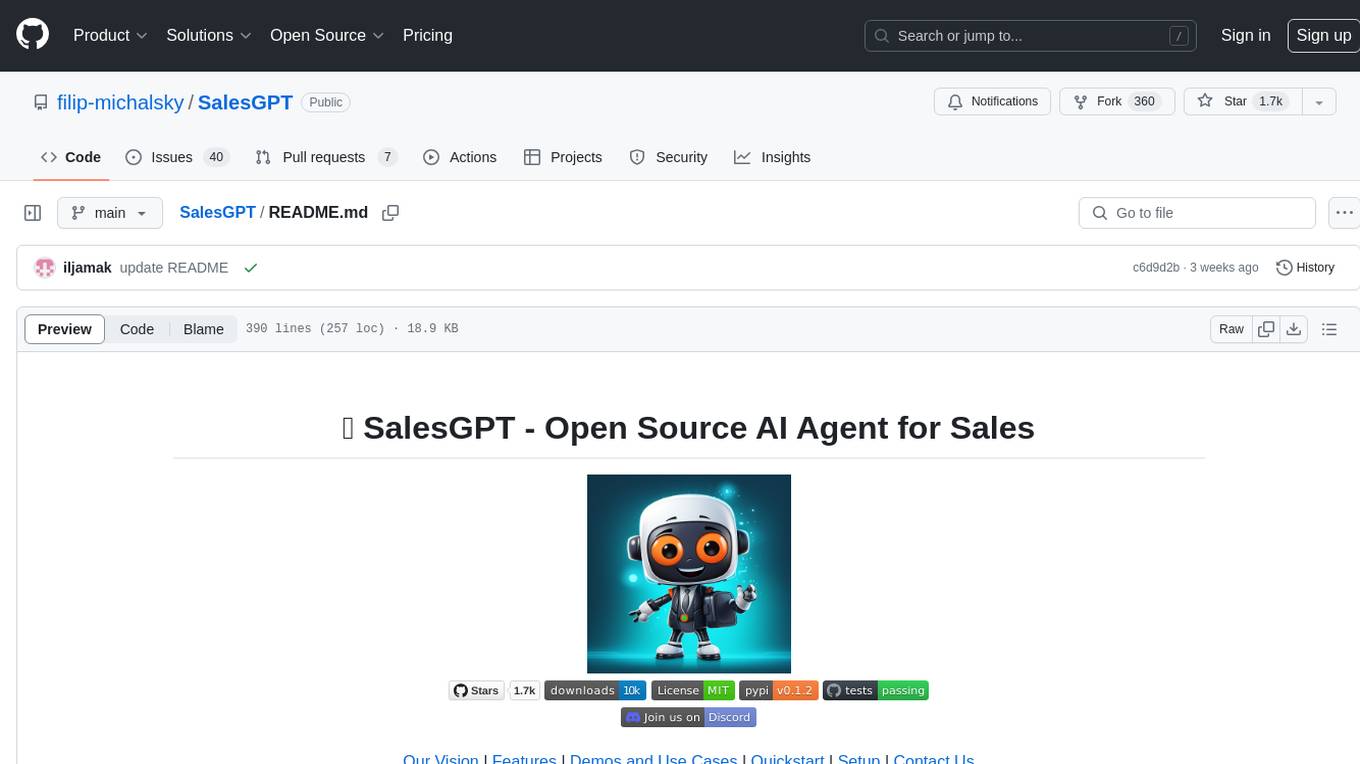
SalesGPT
SalesGPT is an open-source AI agent designed for sales, utilizing context-awareness and LLMs to work across various communication channels like voice, email, and texting. It aims to enhance sales conversations by understanding the stage of the conversation and providing tools like product knowledge base to reduce errors. The agent can autonomously generate payment links, handle objections, and close sales. It also offers features like automated email communication, meeting scheduling, and integration with various LLMs for customization. SalesGPT is optimized for low latency in voice channels and ensures human supervision where necessary. The tool provides enterprise-grade security and supports LangSmith tracing for monitoring and evaluation of intelligent agents built on LLM frameworks.
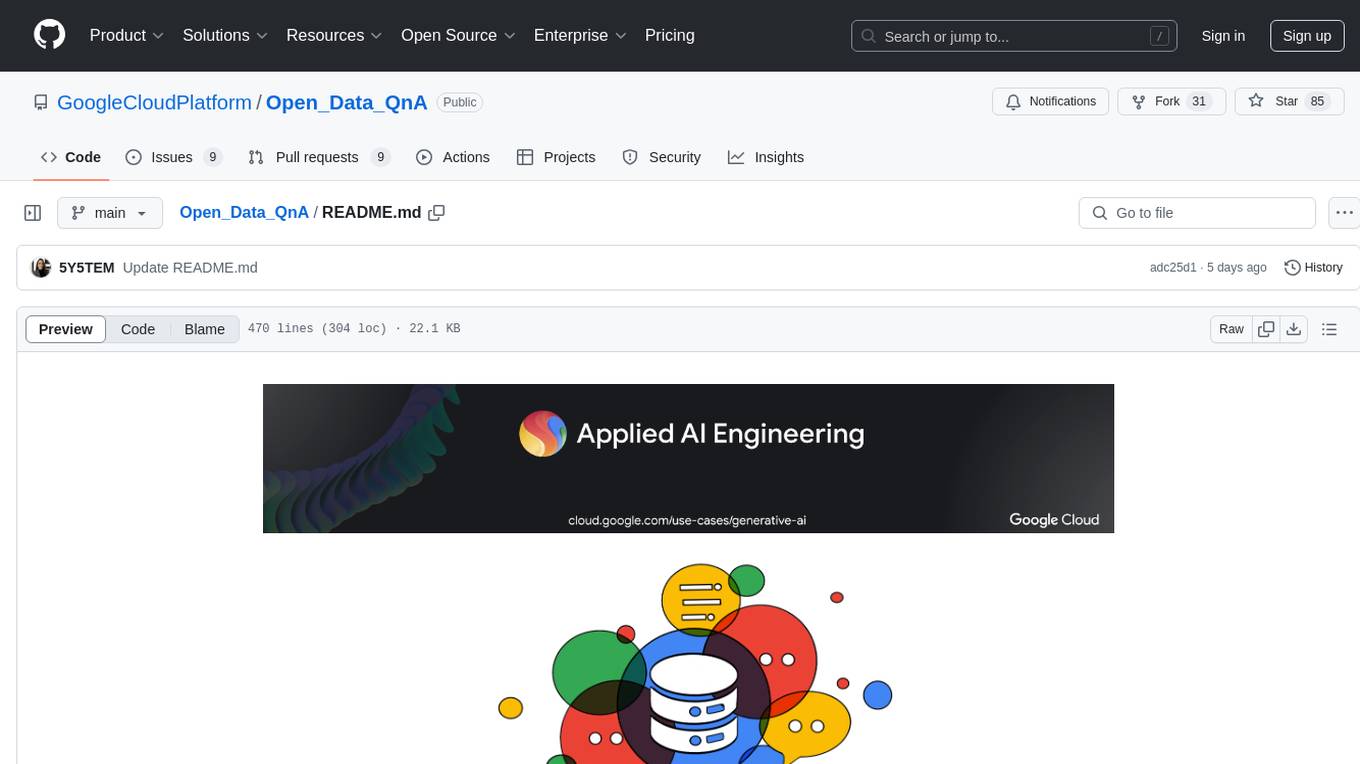
Open_Data_QnA
Open Data QnA is a Python library that allows users to interact with their PostgreSQL or BigQuery databases in a conversational manner, without needing to write SQL queries. The library leverages Large Language Models (LLMs) to bridge the gap between human language and database queries, enabling users to ask questions in natural language and receive informative responses. It offers features such as conversational querying with multiturn support, table grouping, multi schema/dataset support, SQL generation, query refinement, natural language responses, visualizations, and extensibility. The library is built on a modular design and supports various components like Database Connectors, Vector Stores, and Agents for SQL generation, validation, debugging, descriptions, embeddings, responses, and visualizations.
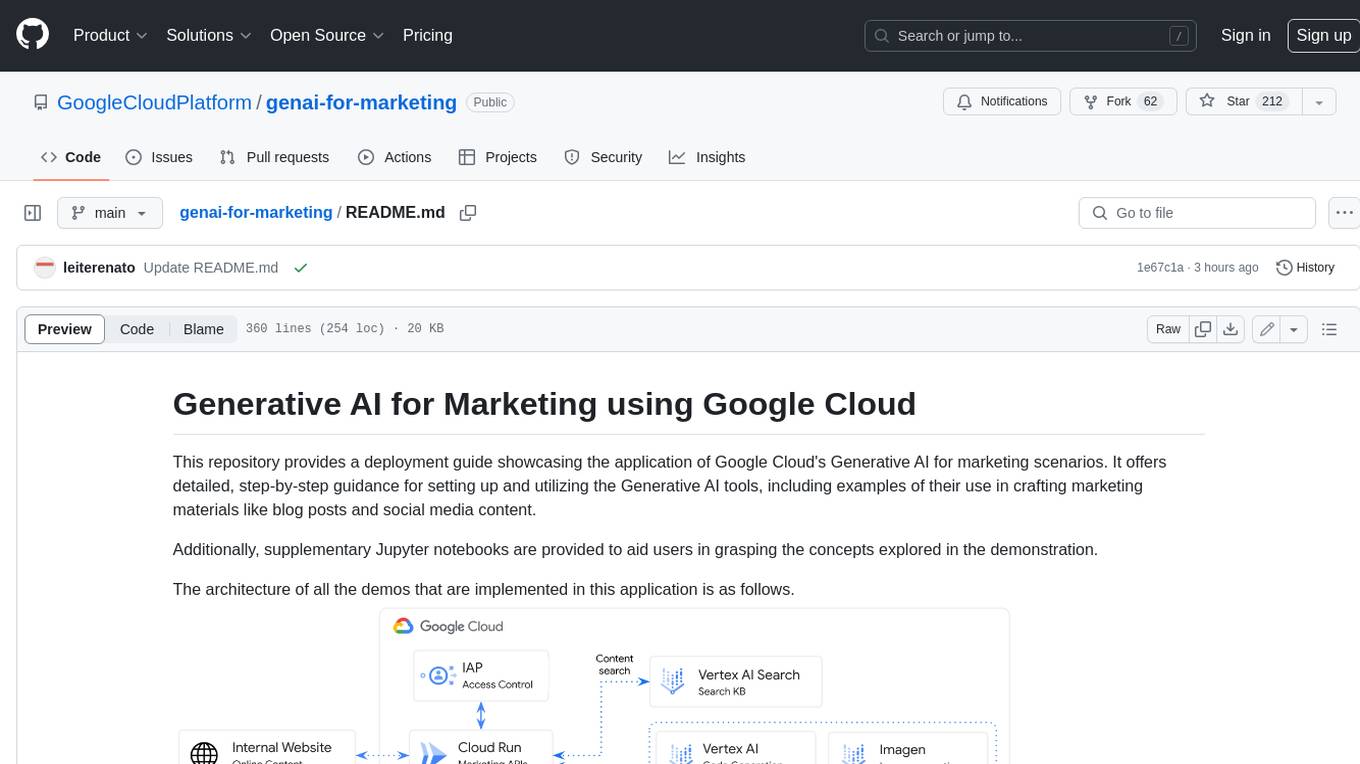
genai-for-marketing
This repository provides a deployment guide for utilizing Google Cloud's Generative AI tools in marketing scenarios. It includes step-by-step instructions, examples of crafting marketing materials, and supplementary Jupyter notebooks. The demos cover marketing insights, audience analysis, trendspotting, content search, content generation, and workspace integration. Users can access and visualize marketing data, analyze trends, improve search experience, and generate compelling content. The repository structure includes backend APIs, frontend code, sample notebooks, templates, and installation scripts.
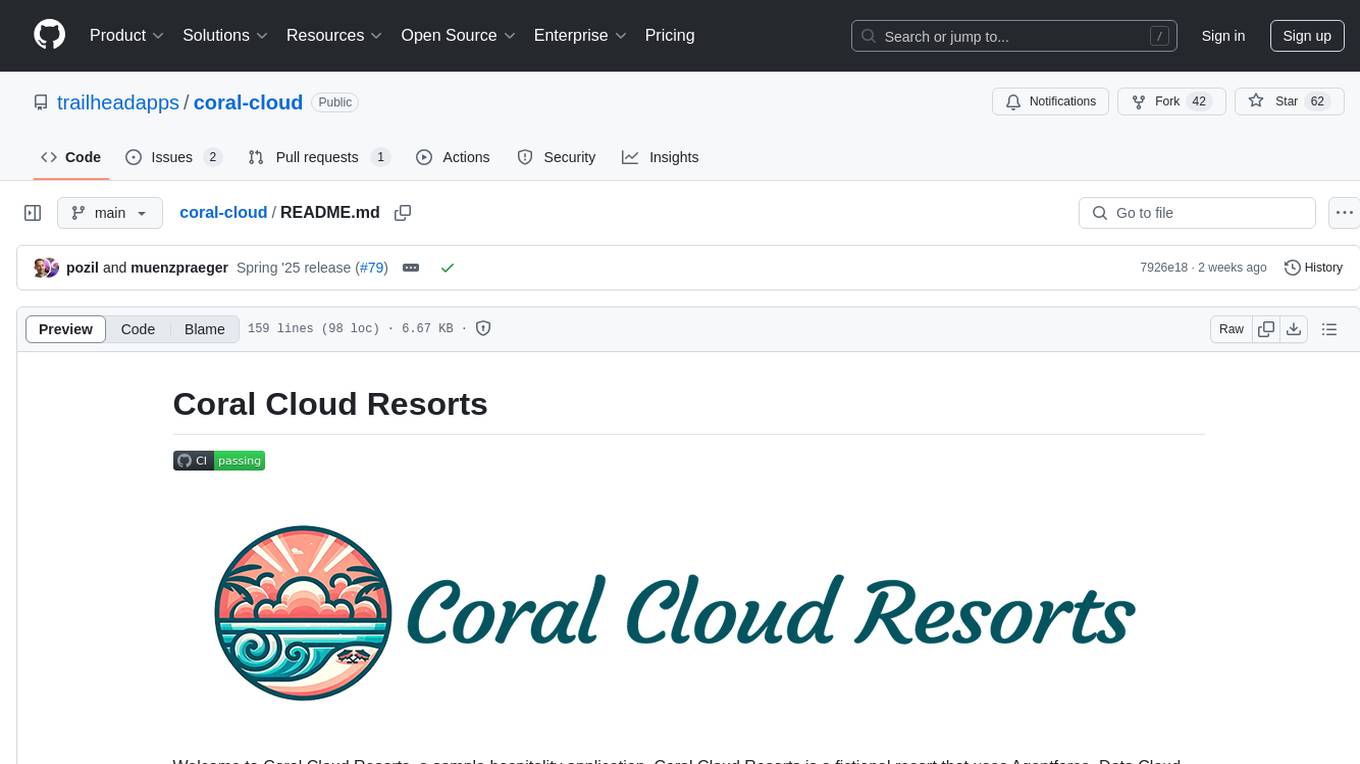
coral-cloud
Coral Cloud Resorts is a sample hospitality application that showcases Data Cloud, Agents, and Prompts. It provides highly personalized guest experiences through smart automation, content generation, and summarization. The app requires licenses for Data Cloud, Agents, Prompt Builder, and Einstein for Sales. Users can activate features, deploy metadata, assign permission sets, import sample data, and troubleshoot common issues. Additionally, the repository offers integration with modern web development tools like Prettier, ESLint, and pre-commit hooks for code formatting and linting.
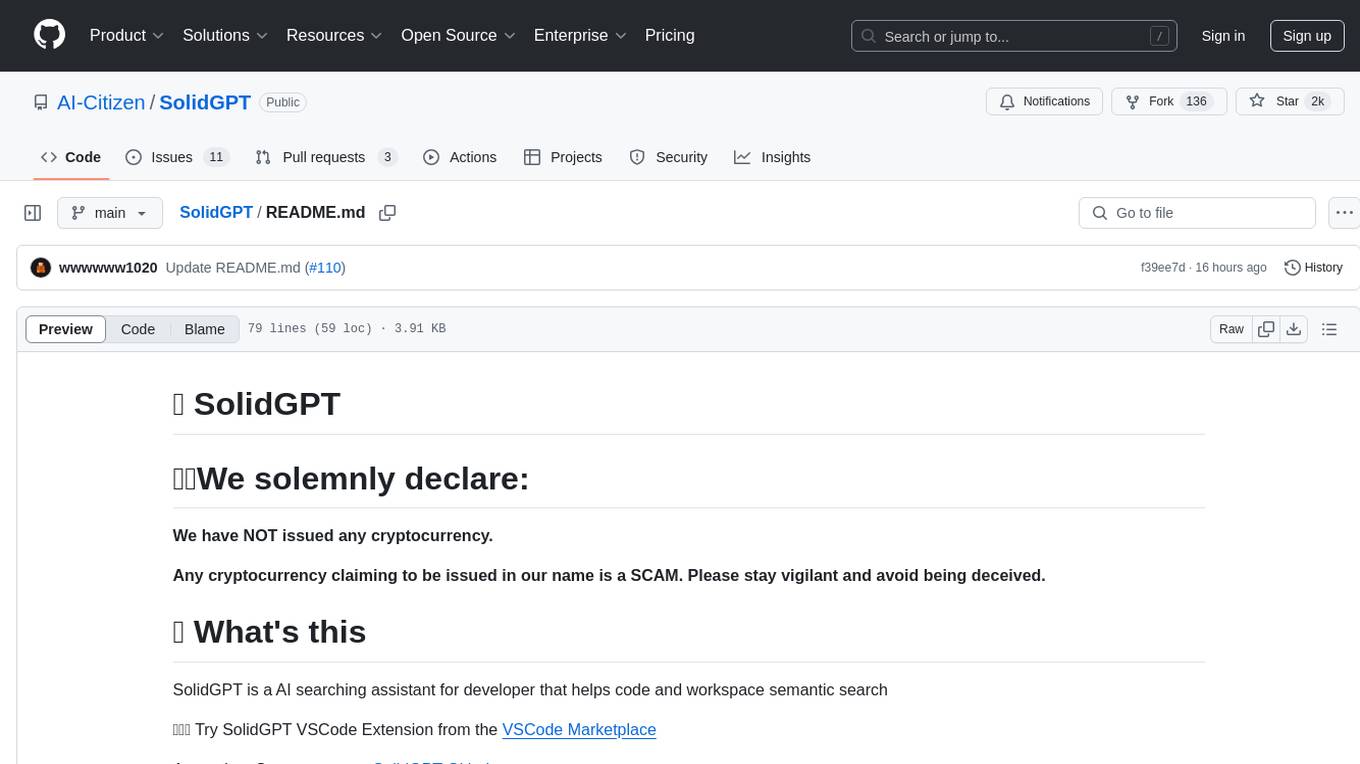
SolidGPT
SolidGPT is an AI searching assistant for developers that helps with code and workspace semantic search. It provides features such as talking to your codebase, asking questions about your codebase, semantic search and summary in Notion, and getting questions answered from your codebase and Notion without context switching. The tool ensures data safety by not collecting users' data and uses the OpenAI series model API.
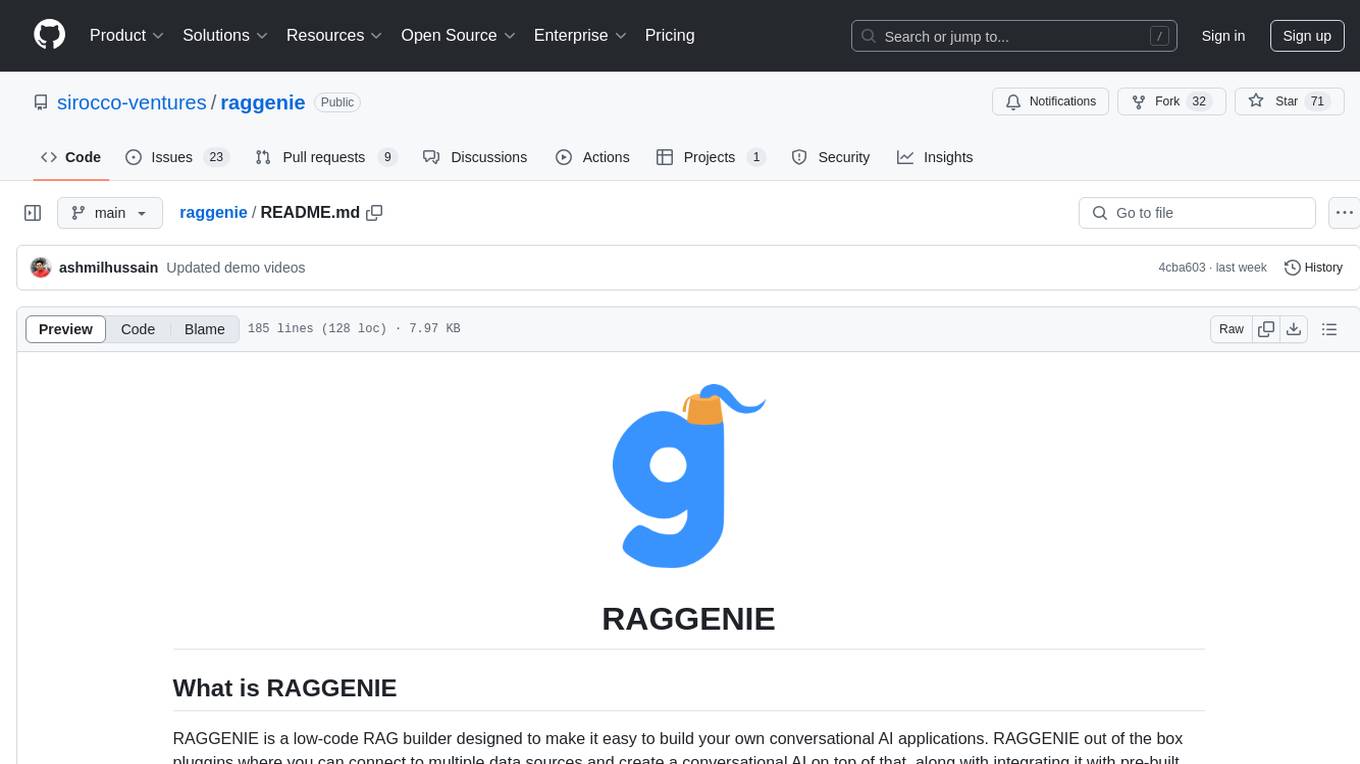
raggenie
RAGGENIE is a low-code RAG builder tool designed to simplify the creation of conversational AI applications. It offers out-of-the-box plugins for connecting to various data sources and building conversational AI on top of them, including integration with pre-built agents for actions. The tool is open-source under the MIT license, with a current focus on making it easy to build RAG applications and future plans for maintenance, monitoring, and transitioning applications from pilots to production.
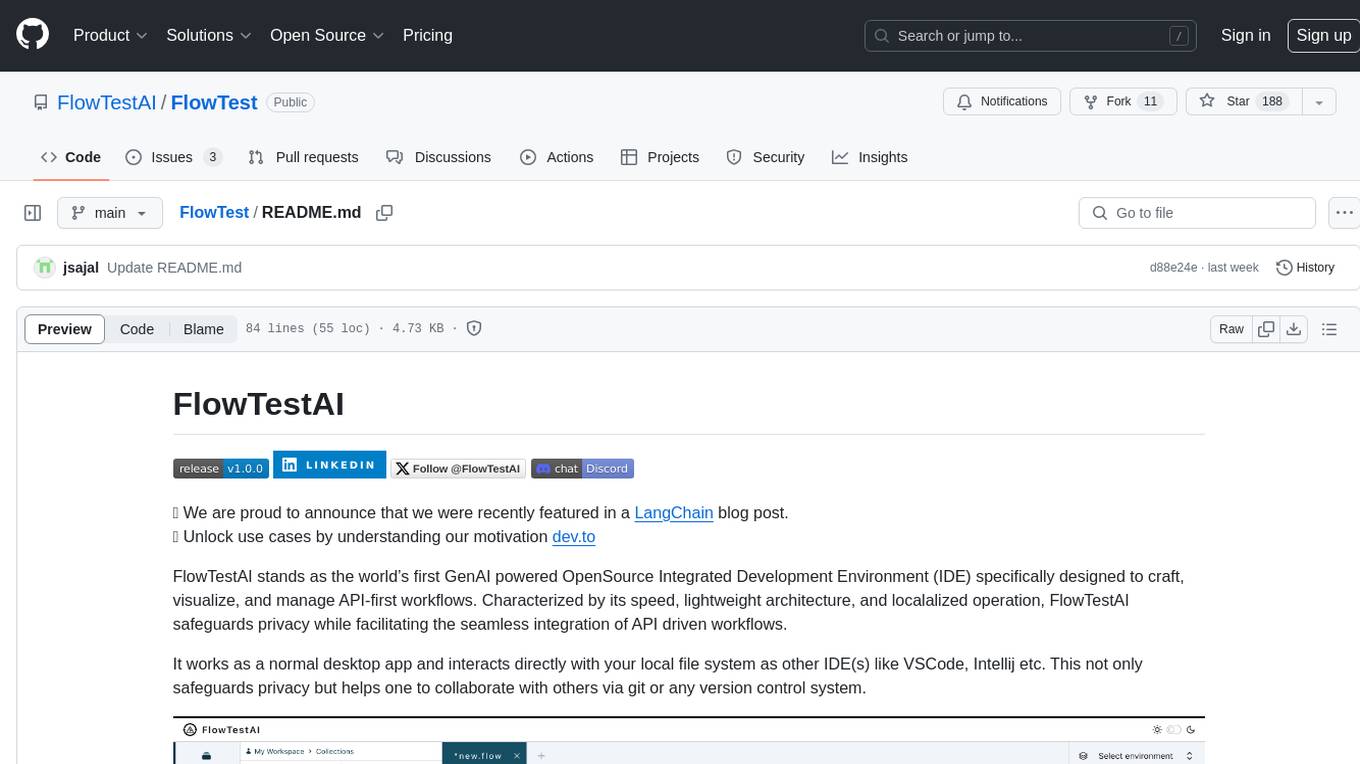
FlowTest
FlowTestAI is the world’s first GenAI powered OpenSource Integrated Development Environment (IDE) designed for crafting, visualizing, and managing API-first workflows. It operates as a desktop app, interacting with the local file system, ensuring privacy and enabling collaboration via version control systems. The platform offers platform-specific binaries for macOS, with versions for Windows and Linux in development. It also features a CLI for running API workflows from the command line interface, facilitating automation and CI/CD processes.
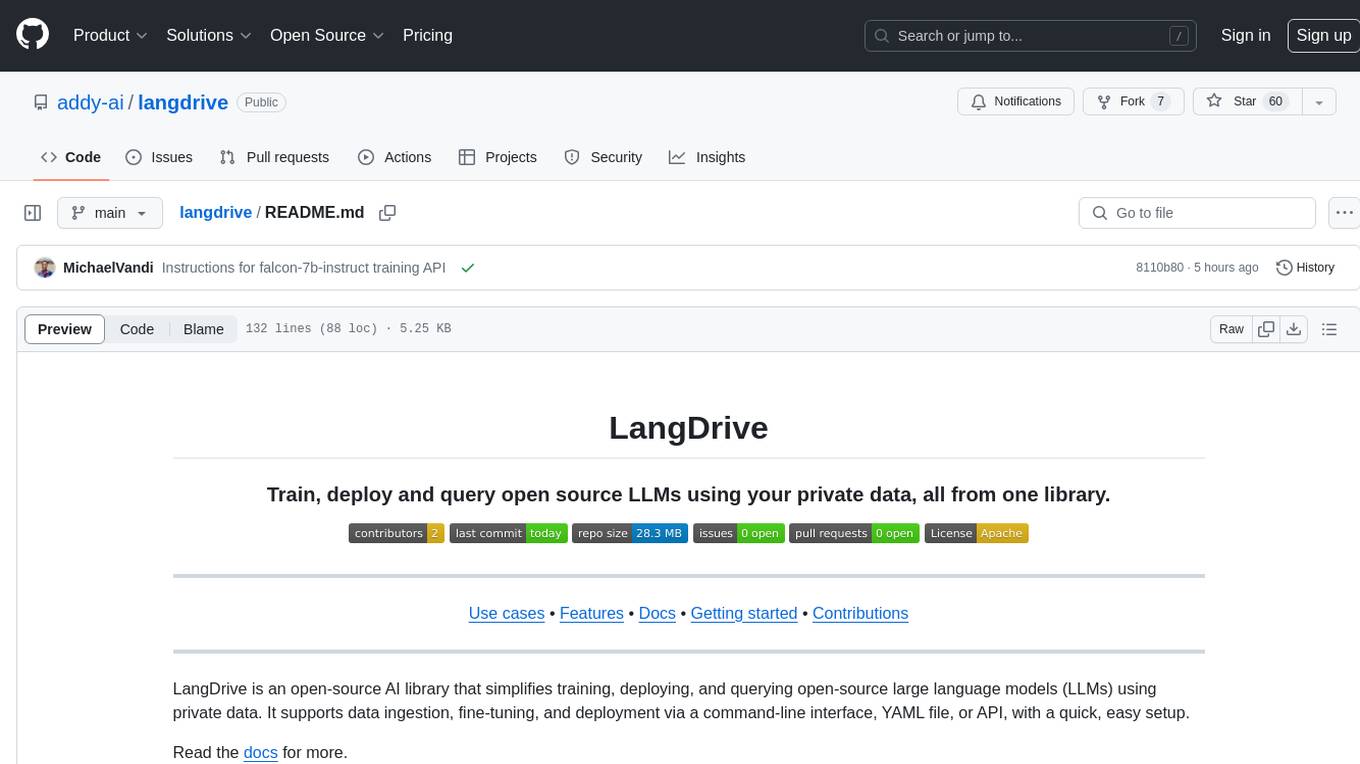
langdrive
LangDrive is an open-source AI library that simplifies training, deploying, and querying open-source large language models (LLMs) using private data. It supports data ingestion, fine-tuning, and deployment via a command-line interface, YAML file, or API, with a quick, easy setup. Users can build AI applications such as question/answering systems, chatbots, AI agents, and content generators. The library provides features like data connectors for ingestion, fine-tuning of LLMs, deployment to Hugging Face hub, inference querying, data utilities for CRUD operations, and APIs for model access. LangDrive is designed to streamline the process of working with LLMs and making AI development more accessible.
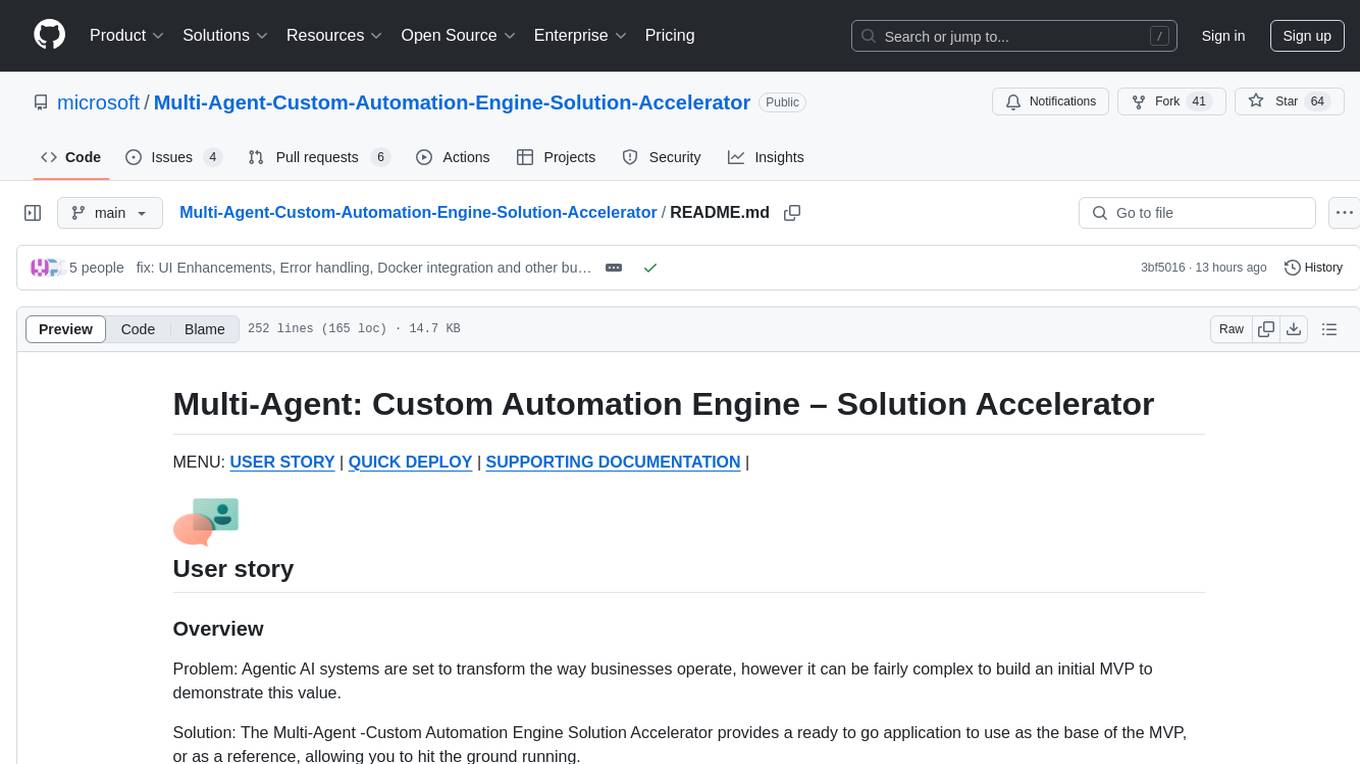
Multi-Agent-Custom-Automation-Engine-Solution-Accelerator
The Multi-Agent -Custom Automation Engine Solution Accelerator is an AI-driven orchestration system that manages a group of AI agents to accomplish tasks based on user input. It uses a FastAPI backend to handle HTTP requests, processes them through various specialized agents, and stores stateful information using Azure Cosmos DB. The system allows users to focus on what matters by coordinating activities across an organization, enabling GenAI to scale, and is applicable to most industries. It is intended for developing and deploying custom AI solutions for specific customers, providing a foundation to accelerate building out multi-agent systems.
For similar tasks
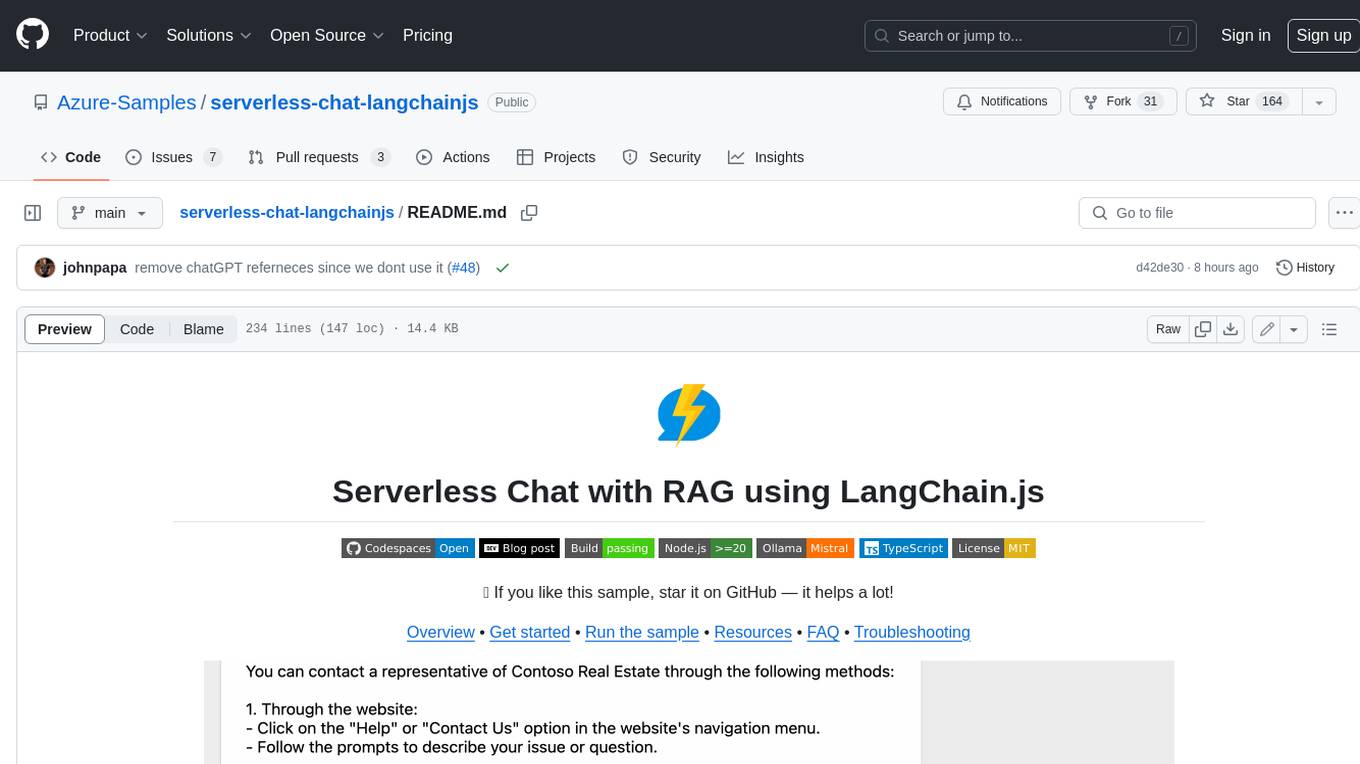
serverless-chat-langchainjs
This sample shows how to build a serverless chat experience with Retrieval-Augmented Generation using LangChain.js and Azure. The application is hosted on Azure Static Web Apps and Azure Functions, with Azure Cosmos DB for MongoDB vCore as the vector database. You can use it as a starting point for building more complex AI applications.
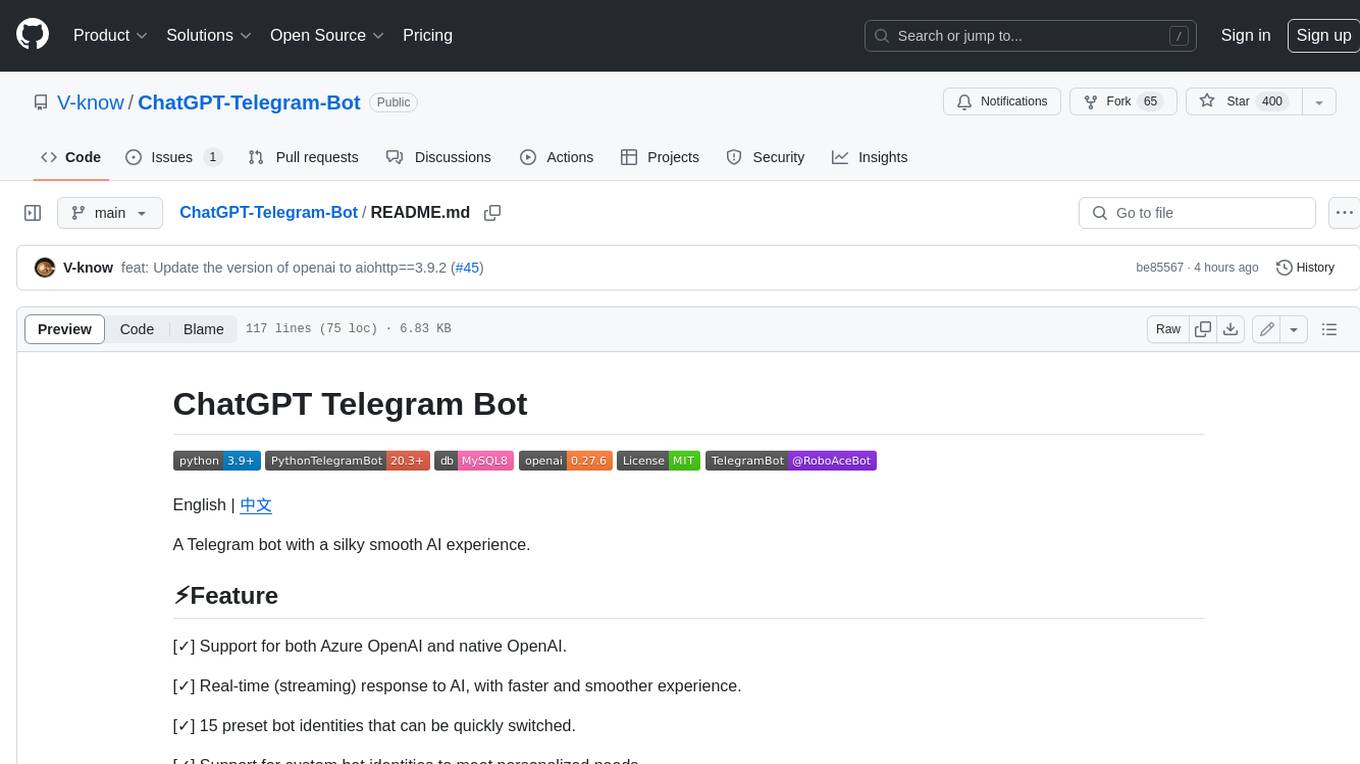
ChatGPT-Telegram-Bot
ChatGPT Telegram Bot is a Telegram bot that provides a smooth AI experience. It supports both Azure OpenAI and native OpenAI, and offers real-time (streaming) response to AI, with a faster and smoother experience. The bot also has 15 preset bot identities that can be quickly switched, and supports custom bot identities to meet personalized needs. Additionally, it supports clearing the contents of the chat with a single click, and restarting the conversation at any time. The bot also supports native Telegram bot button support, making it easy and intuitive to implement required functions. User level division is also supported, with different levels enjoying different single session token numbers, context numbers, and session frequencies. The bot supports English and Chinese on UI, and is containerized for easy deployment.
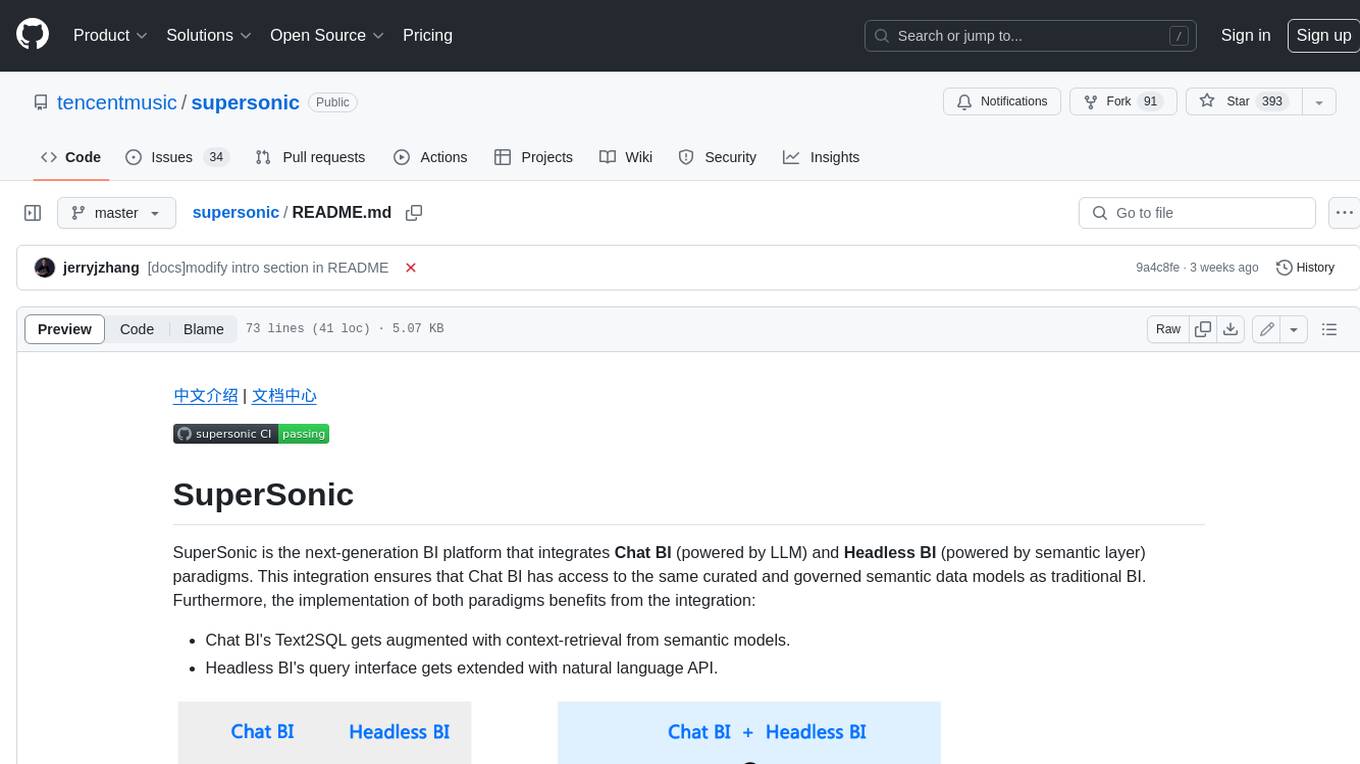
supersonic
SuperSonic is a next-generation BI platform that integrates Chat BI (powered by LLM) and Headless BI (powered by semantic layer) paradigms. This integration ensures that Chat BI has access to the same curated and governed semantic data models as traditional BI. Furthermore, the implementation of both paradigms benefits from the integration: * Chat BI's Text2SQL gets augmented with context-retrieval from semantic models. * Headless BI's query interface gets extended with natural language API. SuperSonic provides a Chat BI interface that empowers users to query data using natural language and visualize the results with suitable charts. To enable such experience, the only thing necessary is to build logical semantic models (definition of metric/dimension/tag, along with their meaning and relationships) through a Headless BI interface. Meanwhile, SuperSonic is designed to be extensible and composable, allowing custom implementations to be added and configured with Java SPI. The integration of Chat BI and Headless BI has the potential to enhance the Text2SQL generation in two dimensions: 1. Incorporate data semantics (such as business terms, column values, etc.) into the prompt, enabling LLM to better understand the semantics and reduce hallucination. 2. Offload the generation of advanced SQL syntax (such as join, formula, etc.) from LLM to the semantic layer to reduce complexity. With these ideas in mind, we develop SuperSonic as a practical reference implementation and use it to power our real-world products. Additionally, to facilitate further development we decide to open source SuperSonic as an extensible framework.
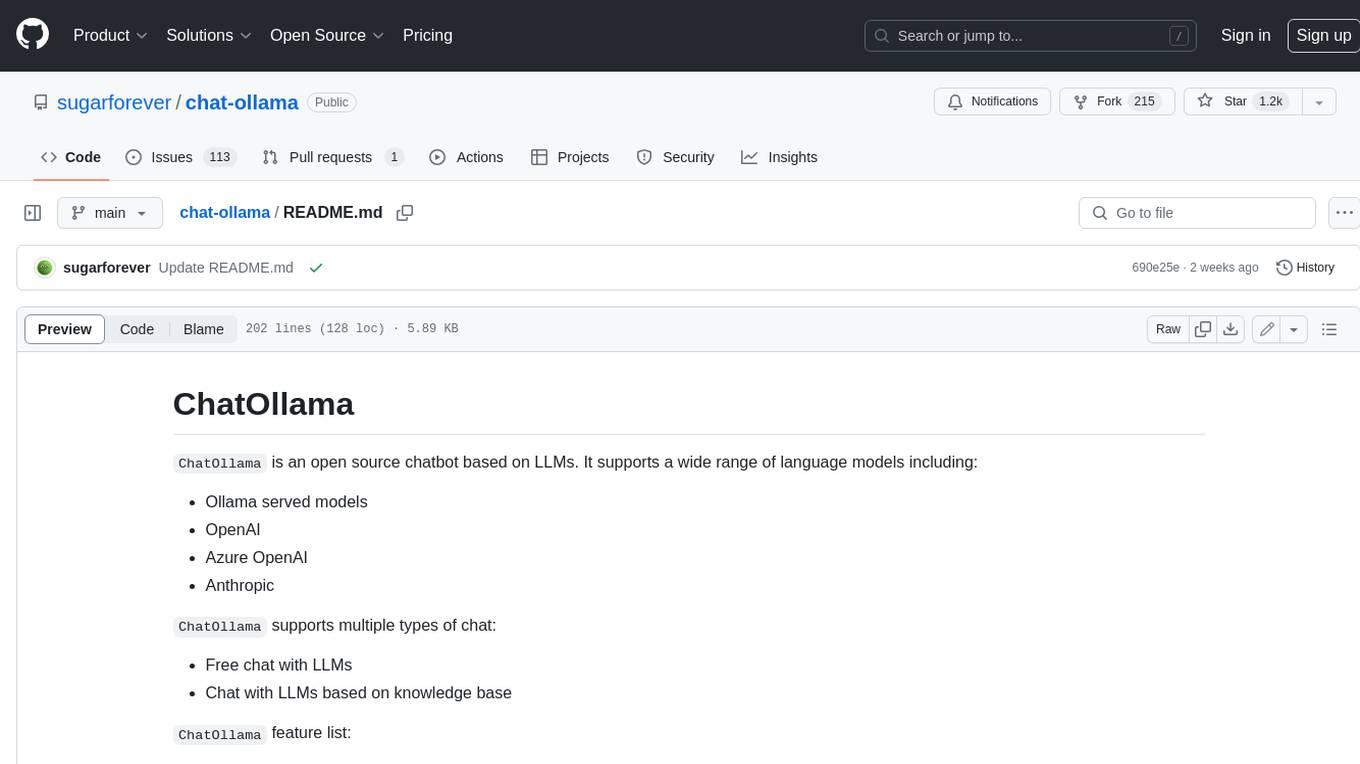
chat-ollama
ChatOllama is an open-source chatbot based on LLMs (Large Language Models). It supports a wide range of language models, including Ollama served models, OpenAI, Azure OpenAI, and Anthropic. ChatOllama supports multiple types of chat, including free chat with LLMs and chat with LLMs based on a knowledge base. Key features of ChatOllama include Ollama models management, knowledge bases management, chat, and commercial LLMs API keys management.
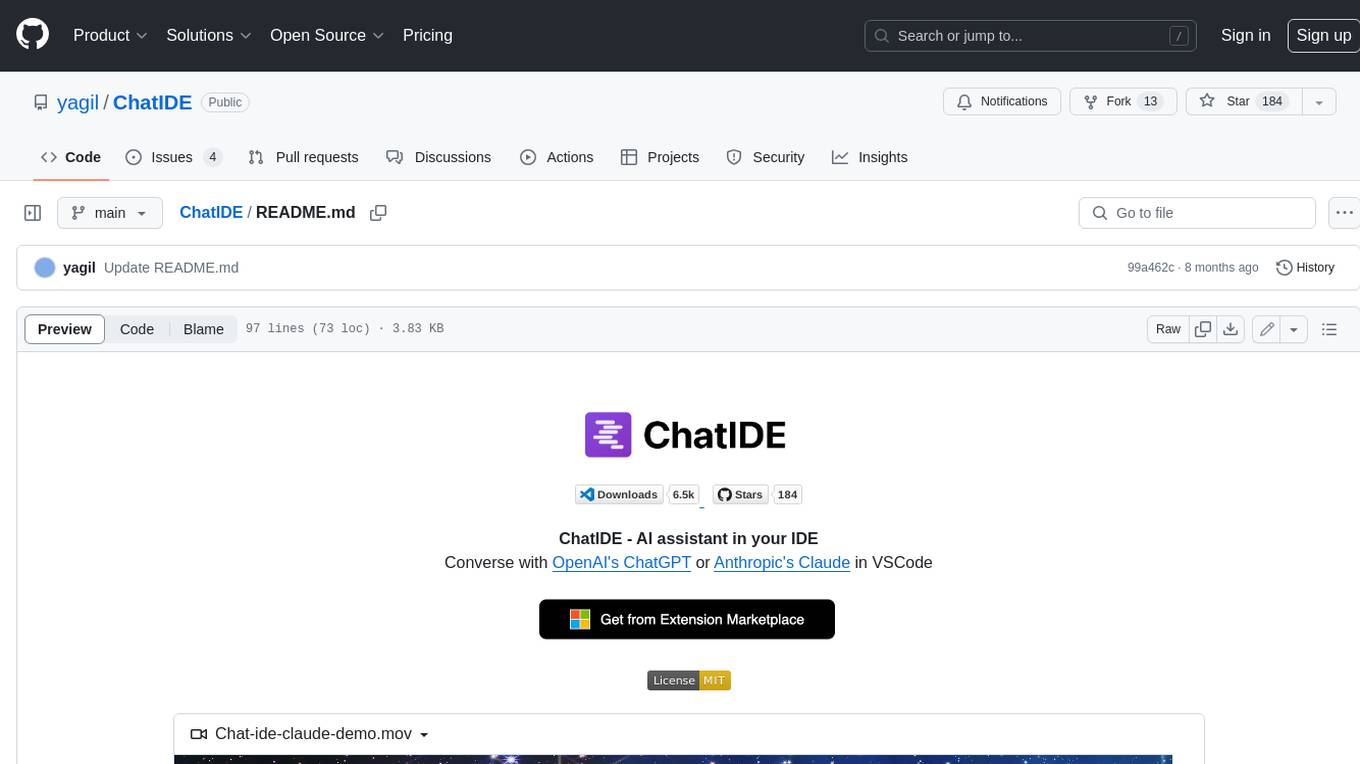
ChatIDE
ChatIDE is an AI assistant that integrates with your IDE, allowing you to converse with OpenAI's ChatGPT or Anthropic's Claude within your development environment. It provides a seamless way to access AI-powered assistance while coding, enabling you to get real-time help, generate code snippets, debug errors, and brainstorm ideas without leaving your IDE.
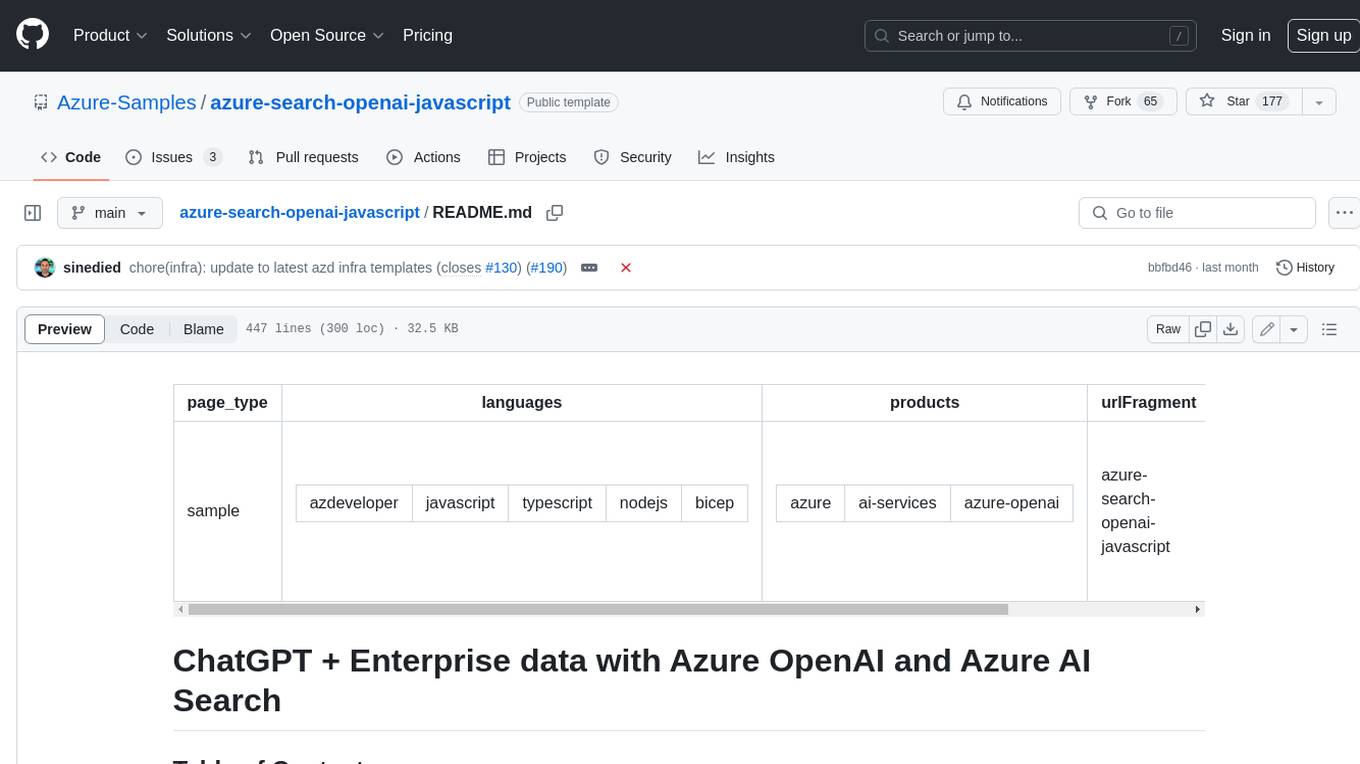
azure-search-openai-javascript
This sample demonstrates a few approaches for creating ChatGPT-like experiences over your own data using the Retrieval Augmented Generation pattern. It uses Azure OpenAI Service to access the ChatGPT model (gpt-35-turbo), and Azure AI Search for data indexing and retrieval.
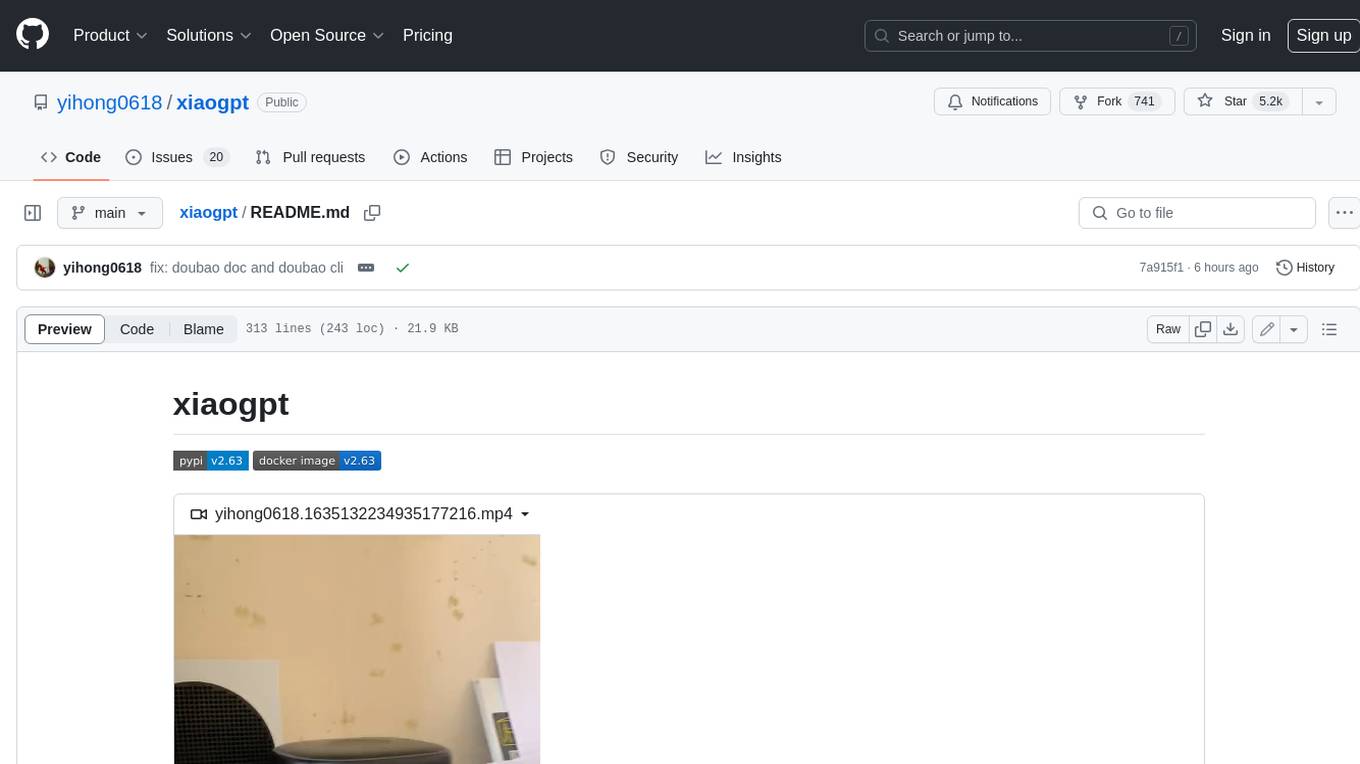
xiaogpt
xiaogpt is a tool that allows you to play ChatGPT and other LLMs with Xiaomi AI Speaker. It supports ChatGPT, New Bing, ChatGLM, Gemini, Doubao, and Tongyi Qianwen. You can use it to ask questions, get answers, and have conversations with AI assistants. xiaogpt is easy to use and can be set up in a few minutes. It is a great way to experience the power of AI and have fun with your Xiaomi AI Speaker.
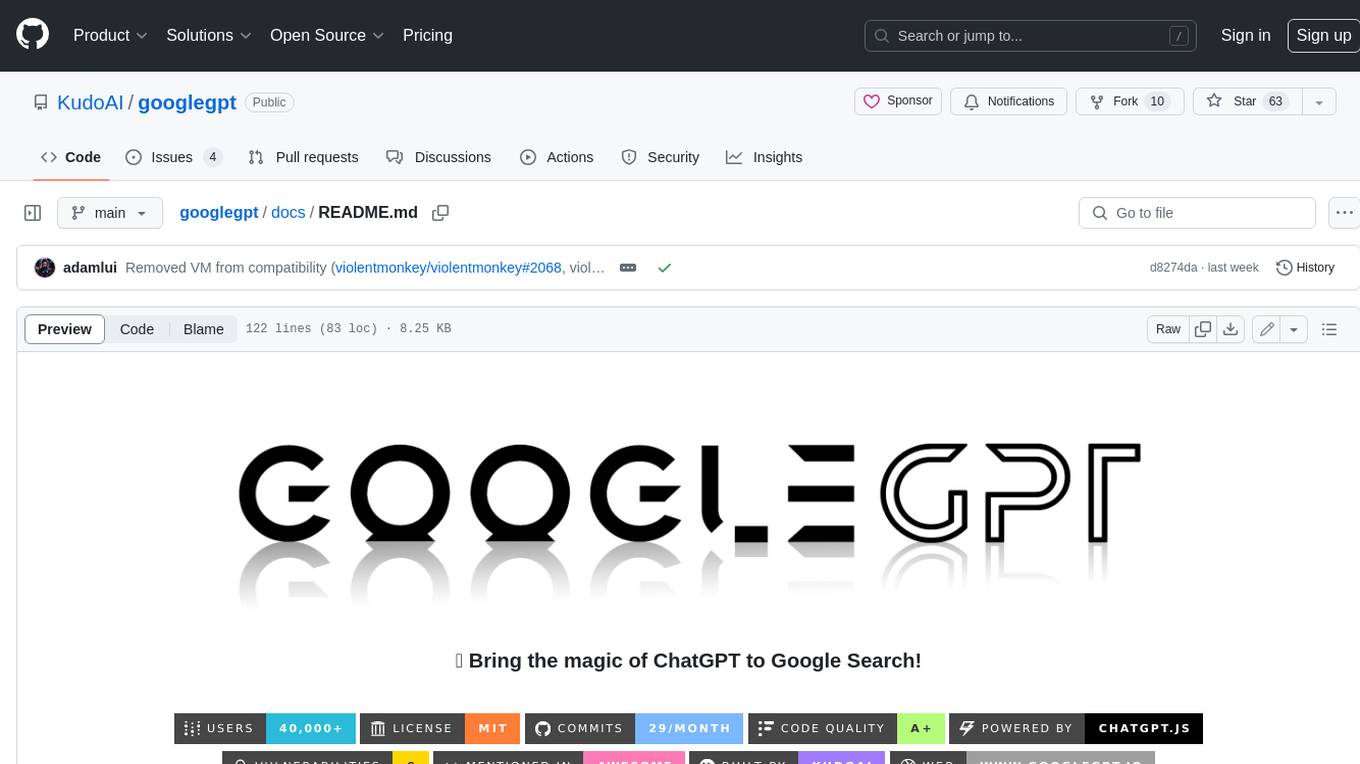
googlegpt
GoogleGPT is a browser extension that brings the power of ChatGPT to Google Search. With GoogleGPT, you can ask ChatGPT questions and get answers directly in your search results. You can also use GoogleGPT to generate text, translate languages, and more. GoogleGPT is compatible with all major browsers, including Chrome, Firefox, Edge, and Safari.
For similar jobs
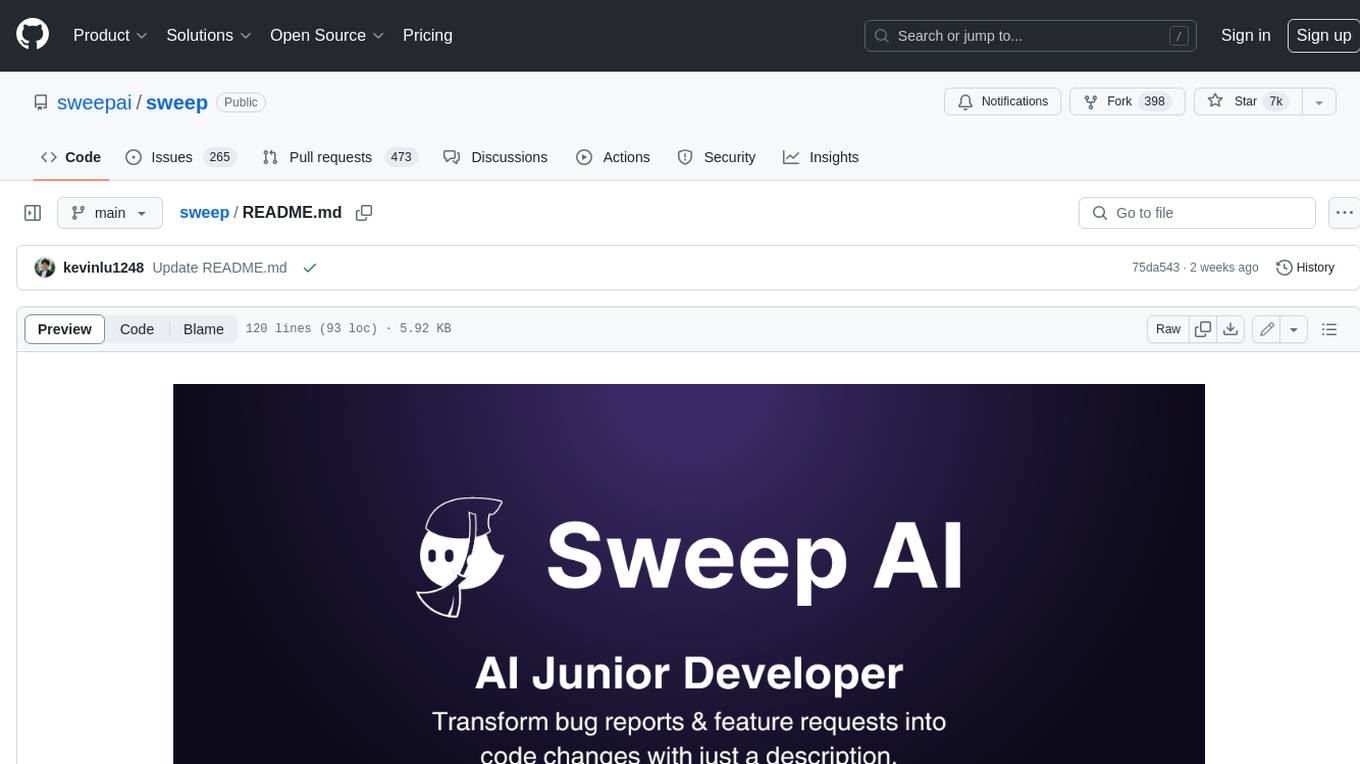
sweep
Sweep is an AI junior developer that turns bugs and feature requests into code changes. It automatically handles developer experience improvements like adding type hints and improving test coverage.
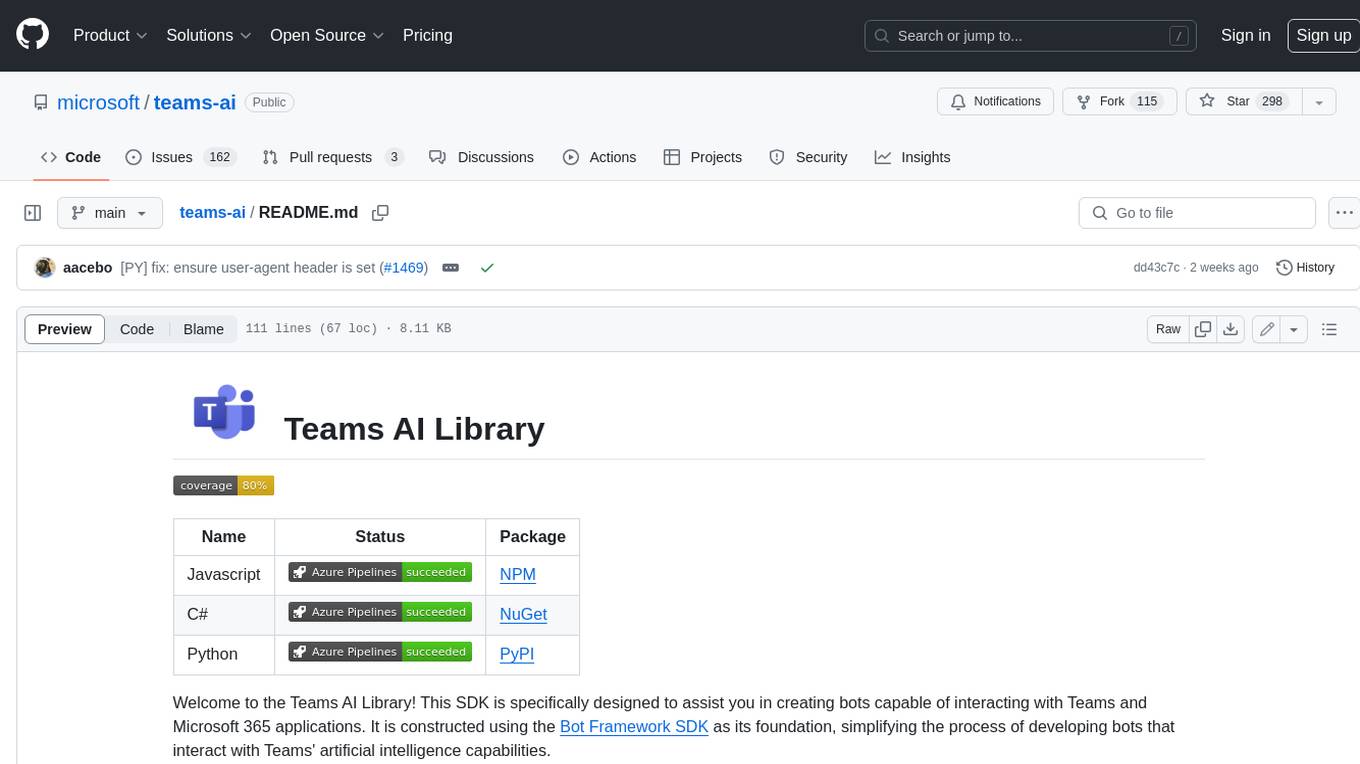
teams-ai
The Teams AI Library is a software development kit (SDK) that helps developers create bots that can interact with Teams and Microsoft 365 applications. It is built on top of the Bot Framework SDK and simplifies the process of developing bots that interact with Teams' artificial intelligence capabilities. The SDK is available for JavaScript/TypeScript, .NET, and Python.
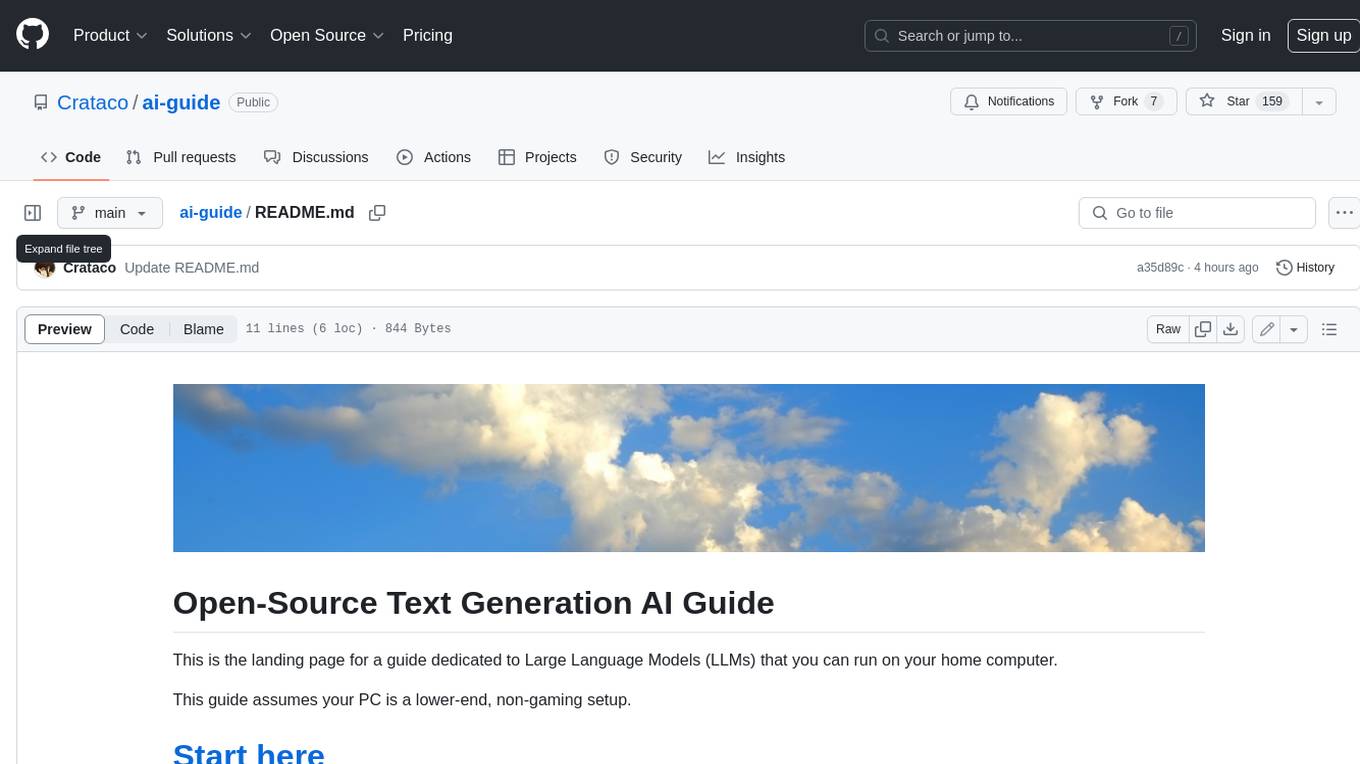
ai-guide
This guide is dedicated to Large Language Models (LLMs) that you can run on your home computer. It assumes your PC is a lower-end, non-gaming setup.
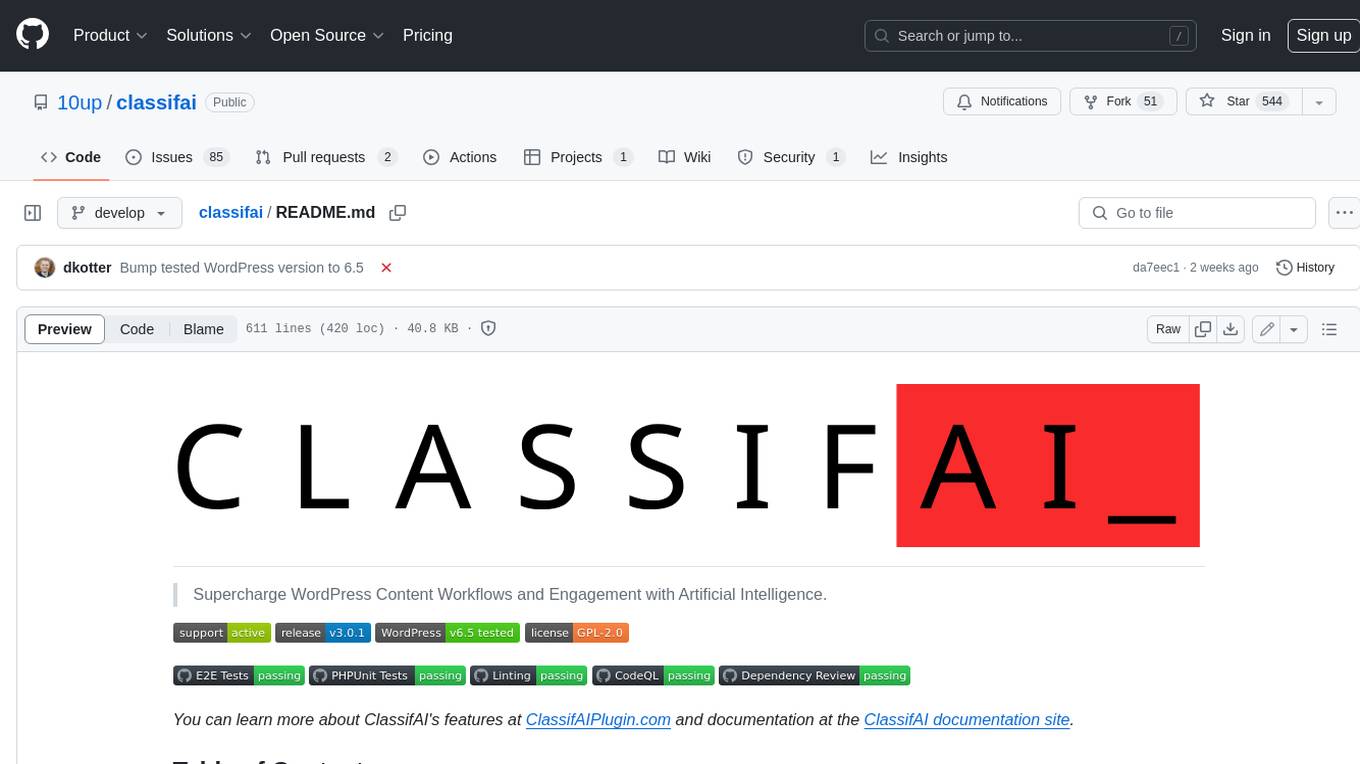
classifai
Supercharge WordPress Content Workflows and Engagement with Artificial Intelligence. Tap into leading cloud-based services like OpenAI, Microsoft Azure AI, Google Gemini and IBM Watson to augment your WordPress-powered websites. Publish content faster while improving SEO performance and increasing audience engagement. ClassifAI integrates Artificial Intelligence and Machine Learning technologies to lighten your workload and eliminate tedious tasks, giving you more time to create original content that matters.
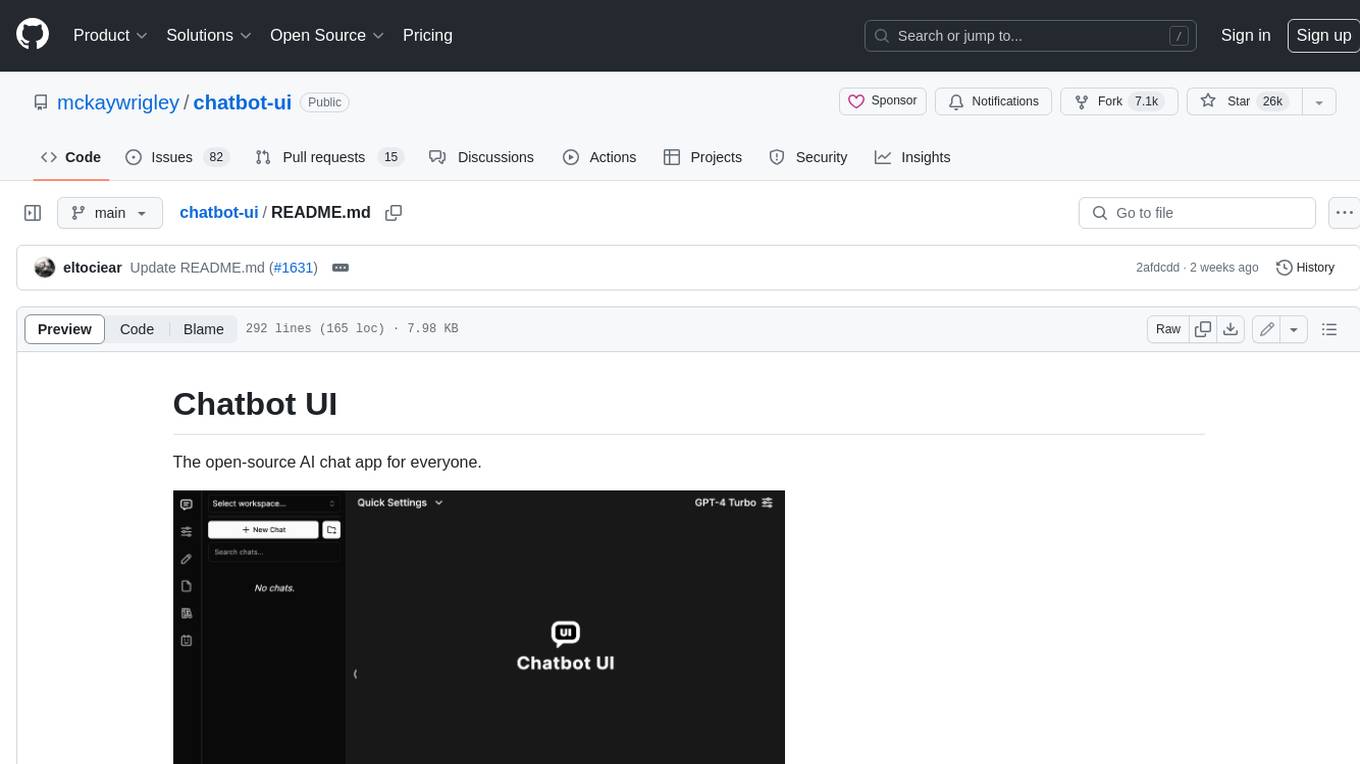
chatbot-ui
Chatbot UI is an open-source AI chat app that allows users to create and deploy their own AI chatbots. It is easy to use and can be customized to fit any need. Chatbot UI is perfect for businesses, developers, and anyone who wants to create a chatbot.
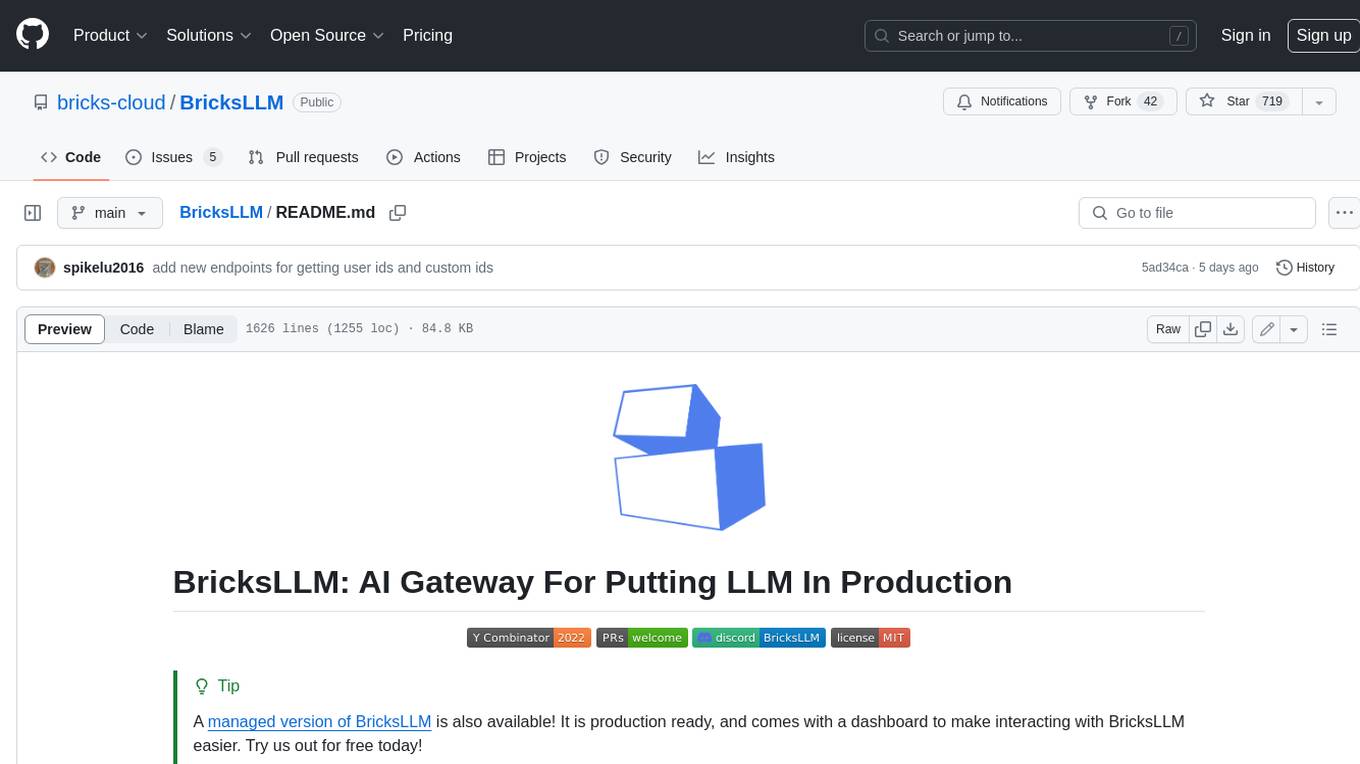
BricksLLM
BricksLLM is a cloud native AI gateway written in Go. Currently, it provides native support for OpenAI, Anthropic, Azure OpenAI and vLLM. BricksLLM aims to provide enterprise level infrastructure that can power any LLM production use cases. Here are some use cases for BricksLLM: * Set LLM usage limits for users on different pricing tiers * Track LLM usage on a per user and per organization basis * Block or redact requests containing PIIs * Improve LLM reliability with failovers, retries and caching * Distribute API keys with rate limits and cost limits for internal development/production use cases * Distribute API keys with rate limits and cost limits for students
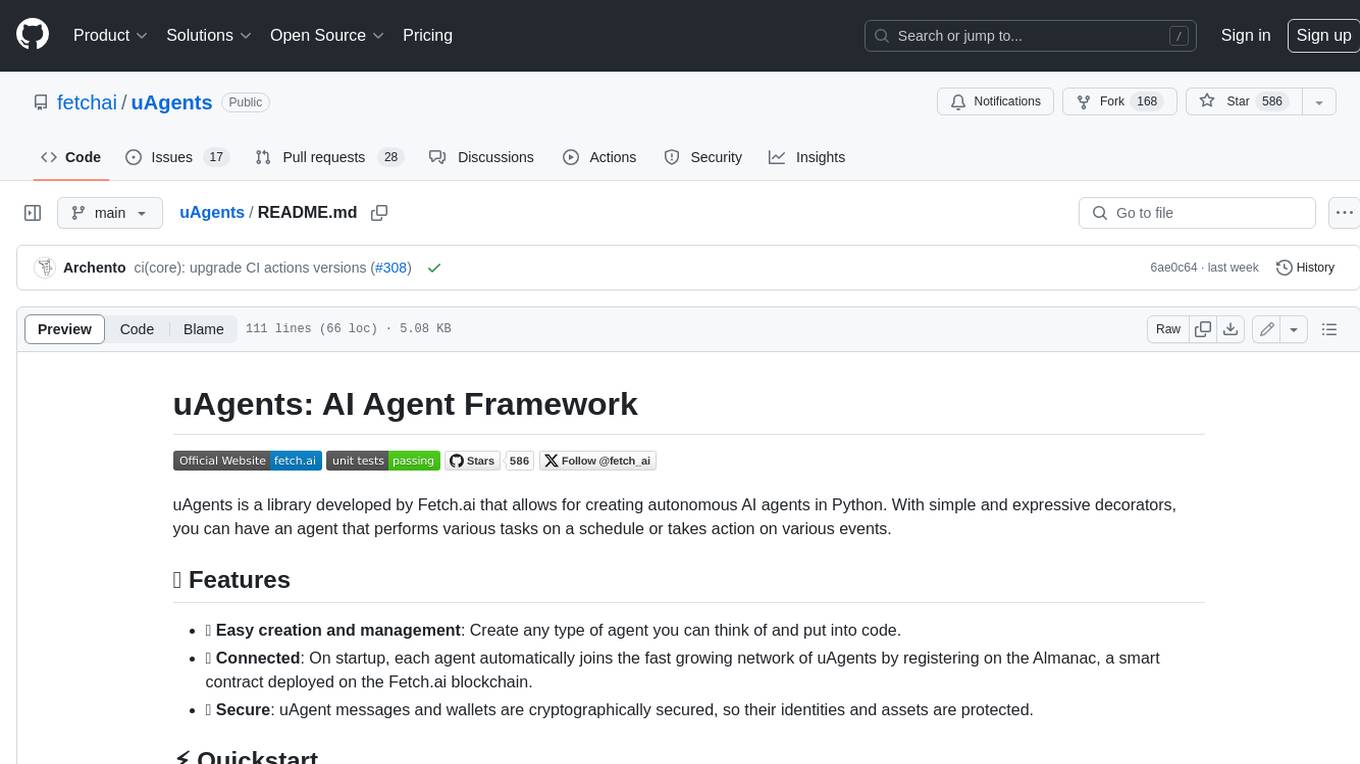
uAgents
uAgents is a Python library developed by Fetch.ai that allows for the creation of autonomous AI agents. These agents can perform various tasks on a schedule or take action on various events. uAgents are easy to create and manage, and they are connected to a fast-growing network of other uAgents. They are also secure, with cryptographically secured messages and wallets.
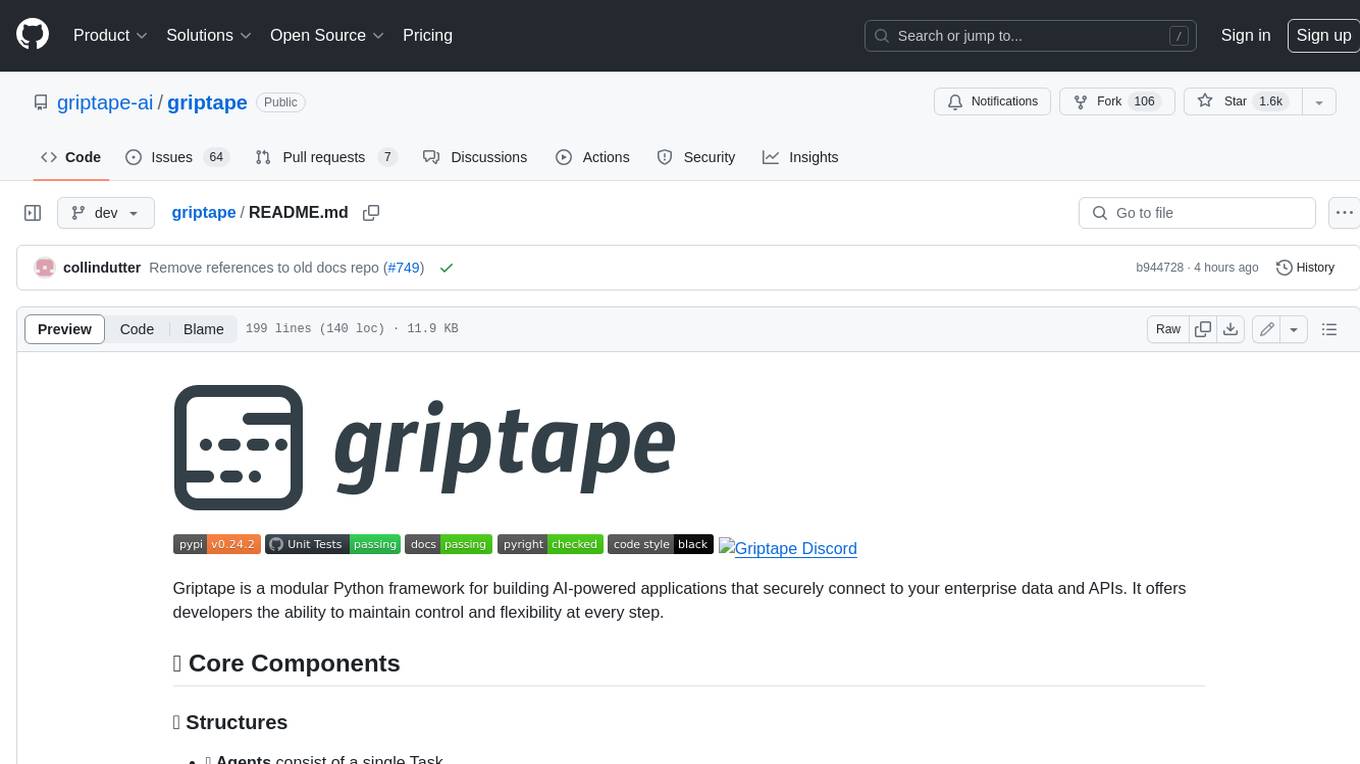
griptape
Griptape is a modular Python framework for building AI-powered applications that securely connect to your enterprise data and APIs. It offers developers the ability to maintain control and flexibility at every step. Griptape's core components include Structures (Agents, Pipelines, and Workflows), Tasks, Tools, Memory (Conversation Memory, Task Memory, and Meta Memory), Drivers (Prompt and Embedding Drivers, Vector Store Drivers, Image Generation Drivers, Image Query Drivers, SQL Drivers, Web Scraper Drivers, and Conversation Memory Drivers), Engines (Query Engines, Extraction Engines, Summary Engines, Image Generation Engines, and Image Query Engines), and additional components (Rulesets, Loaders, Artifacts, Chunkers, and Tokenizers). Griptape enables developers to create AI-powered applications with ease and efficiency.