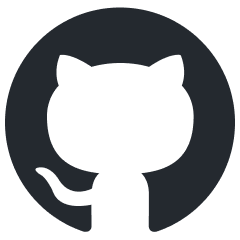
conversational-agent-langchain
FastAPI Backend for a Conversational Agent using Cohere, (Azure) OpenAI, Langchain & Langgraph and Qdrant as VectorDB
Stars: 148
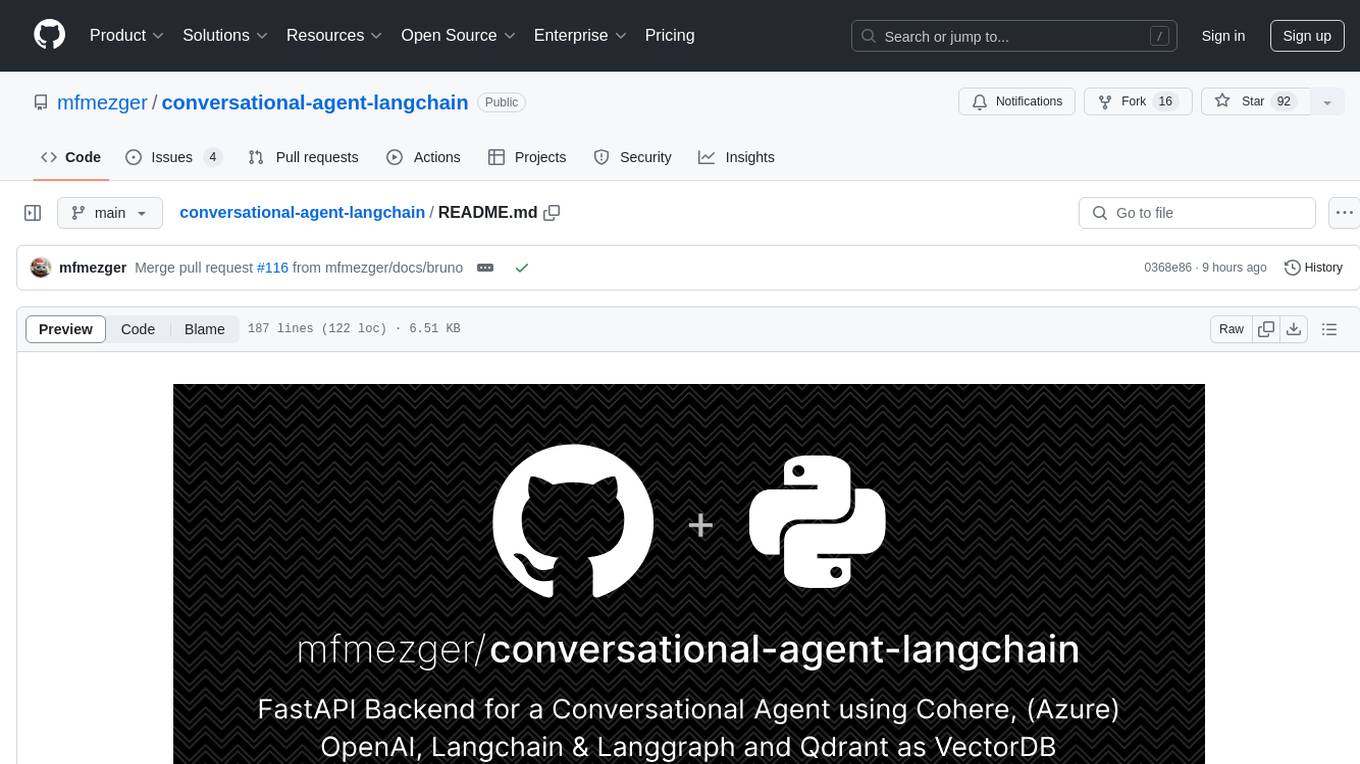
This repository contains a Rest-Backend for a Conversational Agent that allows embedding documents, semantic search, QA based on documents, and document processing with Large Language Models. It uses Aleph Alpha and OpenAI Large Language Models to generate responses to user queries, includes a vector database, and provides a REST API built with FastAPI. The project also features semantic search, secret management for API keys, installation instructions, and development guidelines for both backend and frontend components.
README:
This is a Rest-Backend for a Conversational Agent, that allows to embedd Documentes, search for them using Semantic Search, to QA based on Documents and do document processing with Large Language Models.
At the moment i am reworking to langgraph, therefore not all versions on main will work with all of the providers. I will update the providers in the next weeks. Please use the releases to get a working version.
- Complete Rework to Langgraph
- Adding Cohere
- Adding Ollama
If you want to use an Aleph Alpha only backend i would recommend my other backend: https://github.com/mfmezger/aleph-alpha-rag.
To run the complete system with docker use this command:
git clone https://github.com/mfmezger/conversational-agent-langchain.git
cd conversational-agent-langchain
Create a .env file from the .env-template and set the qdrant api key. For tests just set it to test. QDRANT_API_KEY="test"
Then start the system with
docker compose up -d
Then go to http://127.0.0.1:8001/docs or http://127.0.0.1:8001/redoc to see the API documentation.
Frontend: localhost:8501 Qdrant Dashboard: localhost:6333/dashboard
This project is a conversational agent that uses Aleph Alpha and OpenAI Large Language Models to generate responses to user queries. The agent also includes a vector database and a REST API built with FastAPI.
Features
- Uses Aleph Alpha and OpenAI Large Language Models to generate responses to user queries.
- Includes a vector database to store and retrieve information.
- Provides a REST API built with FastAPI for easy integration with other applications.
- Has a basic gui.
Semantic search is an advanced search technique that aims to understand the meaning and context of a user's query, rather than matching keywords. It involves natural language processing (NLP) and machine learning algorithms to analyze and interpret user intent, synonyms, relationships between words, and the structure of content. By considering these factors, semantic search improves the accuracy and relevance of search results, providing a more intuitive and personalized user experience.
Langchain is a library for natural language processing and machine learning. FastAPI is a modern, fast (high-performance) web framework for building APIs with Python 3.7+ based on standard Python type hints. A Vectordatabase is a database that stores vectors, which can be used for similarity searches and other machine learning tasks.
Two ways to manage your api keys are available, the easiest approach is to sent the api token in the request as the token. Another possiblity is to create a .env file and add the api token there. If you use OpenAI from Azure or OpenAI directly you need to set the correct parameters in the .env file.
On Linux or Mac you need to adjust your /etc/hosts file to include the following line:
127.0.0.1 qdrant
First install Python Dependencies:
You need to instal rye if you want to use it for syncing the requirements.lock file. Rye Installation.
rye sync
# or if you do not want to use rye
pip install -r requirements.lock
Start the complete system with:
docker compose up -d
To run the Qdrant Database local just run:
docker compose up qdrant
To run the Backend use this command in the root directory:
poetry run uvicorn agent.api:app --reload
To run the tests you can use this command:
poetry run coverage run -m pytest -o log_cli=true -vvv tests
To run the Frontend use this command in the root directory:
poetry run streamlit run gui.py --theme.base="dark"
mypy rag --explicit-package-bases
Qdrant Dashboard is available at http://127.0.0.1:6333/dashboard. There you need to enter the api key.
To use the Qdrant API you need to set the correct parameters in the .env file. QDRANT_API_KEY is the API key for the Qdrant API. And you need to change it in the qdrant.yaml file in the config folder.
If you want to ingest large amount of data i would recommend you use the scripts located in agent/ingestion.
To Test the API i would recommend Bruno. The API Requests are store in ConvAgentBruno folder.
For Tasks:
Click tags to check more tools for each tasksFor Jobs:
Alternative AI tools for conversational-agent-langchain
Similar Open Source Tools
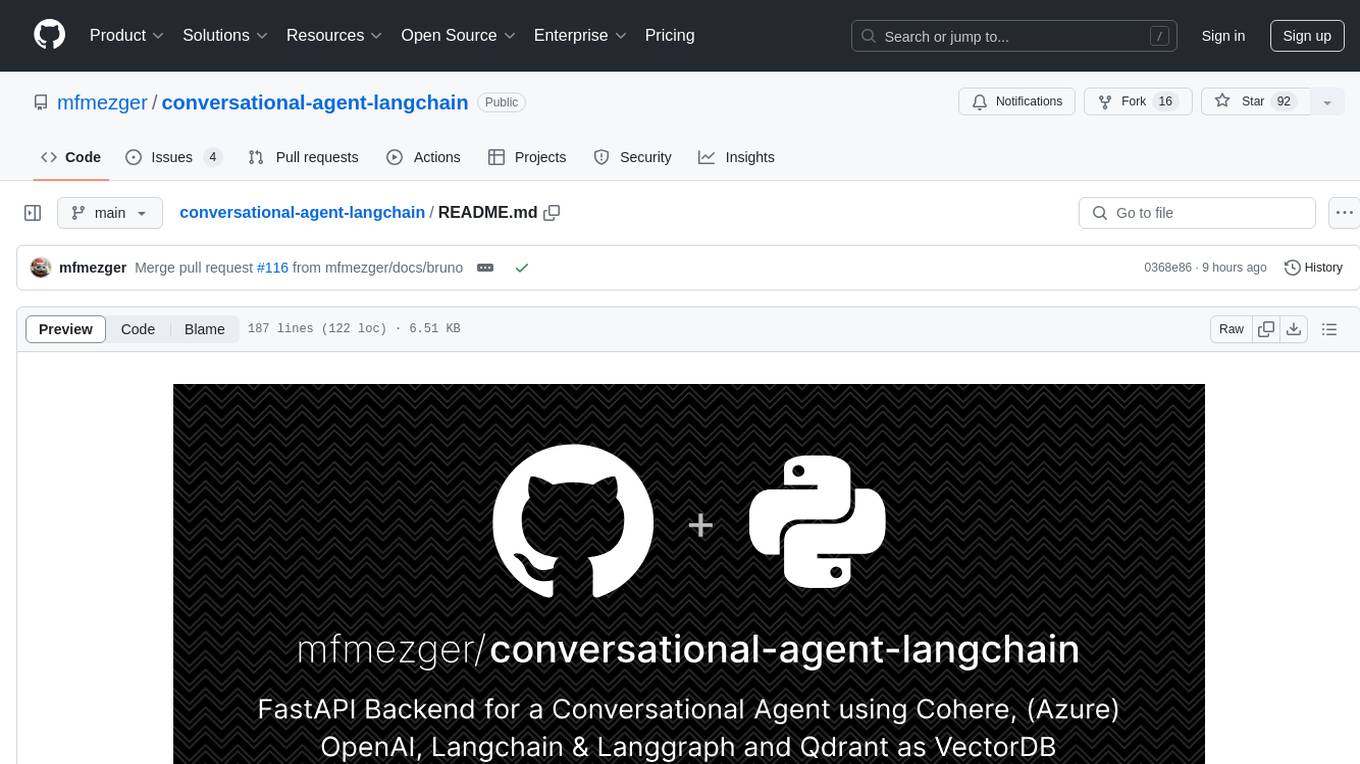
conversational-agent-langchain
This repository contains a Rest-Backend for a Conversational Agent that allows embedding documents, semantic search, QA based on documents, and document processing with Large Language Models. It uses Aleph Alpha and OpenAI Large Language Models to generate responses to user queries, includes a vector database, and provides a REST API built with FastAPI. The project also features semantic search, secret management for API keys, installation instructions, and development guidelines for both backend and frontend components.
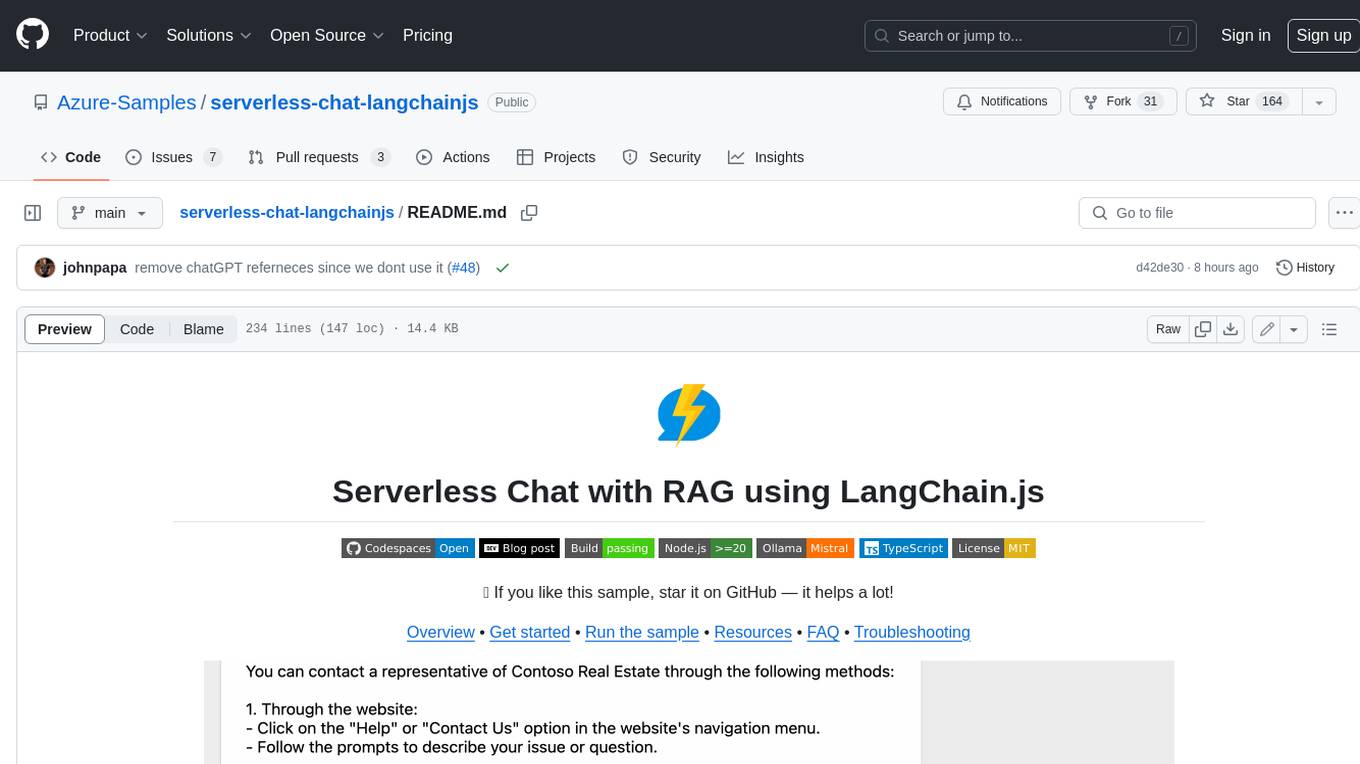
serverless-chat-langchainjs
This sample shows how to build a serverless chat experience with Retrieval-Augmented Generation using LangChain.js and Azure. The application is hosted on Azure Static Web Apps and Azure Functions, with Azure Cosmos DB for MongoDB vCore as the vector database. You can use it as a starting point for building more complex AI applications.
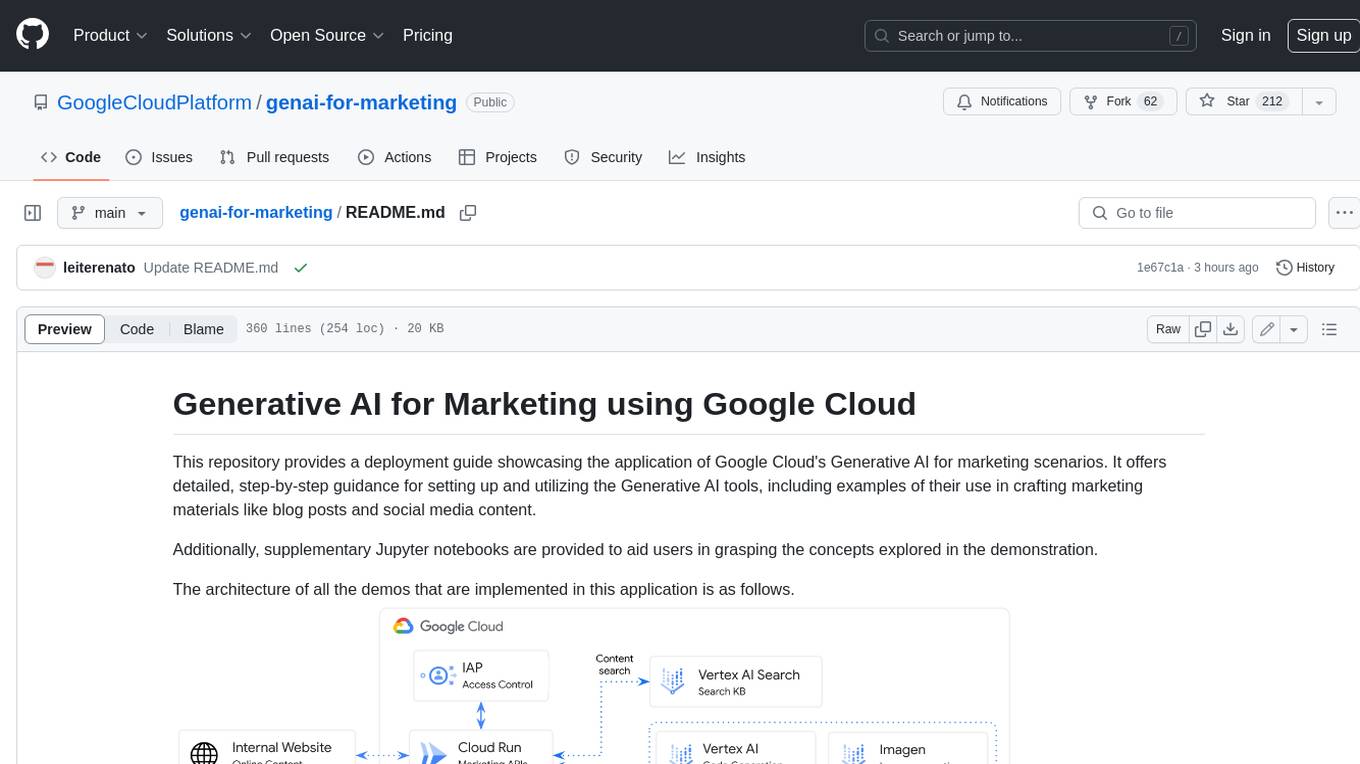
genai-for-marketing
This repository provides a deployment guide for utilizing Google Cloud's Generative AI tools in marketing scenarios. It includes step-by-step instructions, examples of crafting marketing materials, and supplementary Jupyter notebooks. The demos cover marketing insights, audience analysis, trendspotting, content search, content generation, and workspace integration. Users can access and visualize marketing data, analyze trends, improve search experience, and generate compelling content. The repository structure includes backend APIs, frontend code, sample notebooks, templates, and installation scripts.
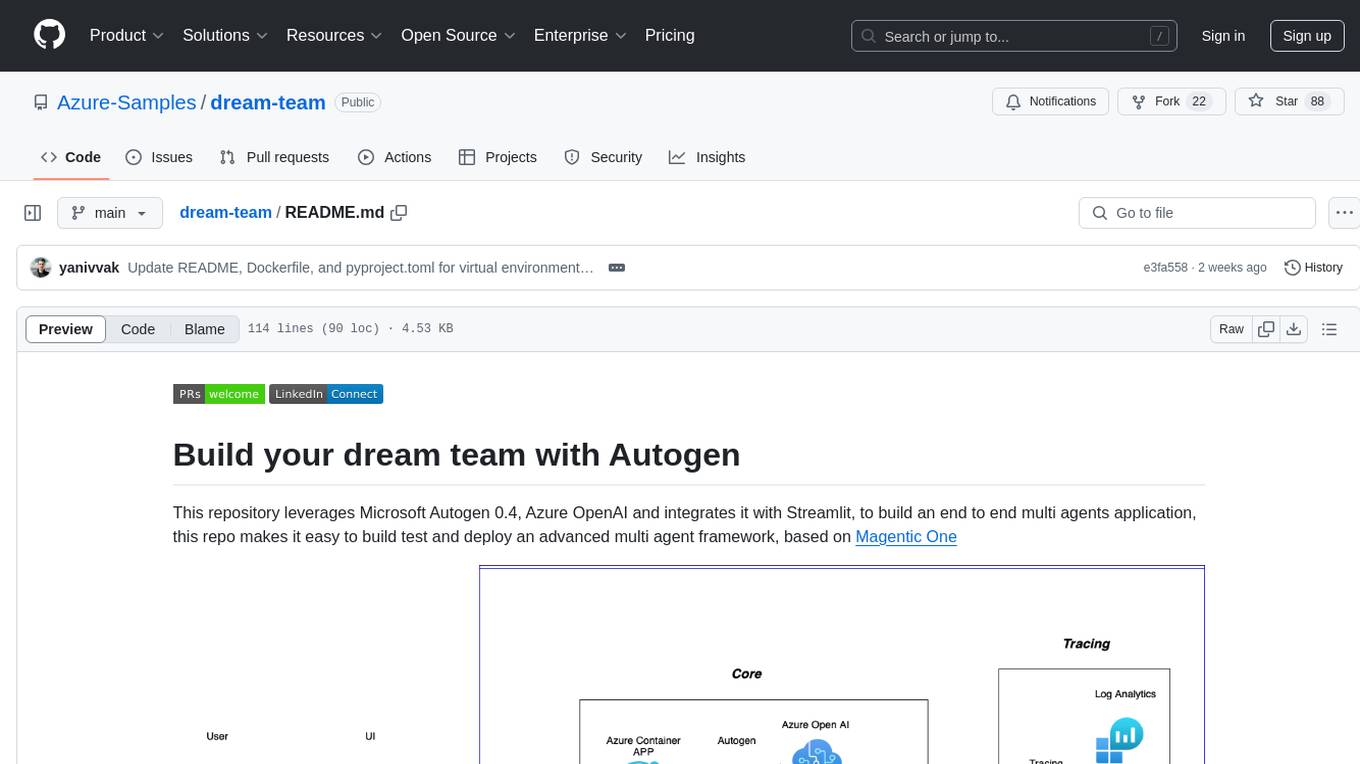
dream-team
Build your dream team with Autogen is a repository that leverages Microsoft Autogen 0.4, Azure OpenAI, and Streamlit to create an end-to-end multi-agent application. It provides an advanced multi-agent framework based on Magentic One, with features such as a friendly UI, single-line deployment, secure code execution, managed identities, and observability & debugging tools. Users can deploy Azure resources and the app with simple commands, work locally with virtual environments, install dependencies, update configurations, and run the application. The repository also offers resources for learning more about building applications with Autogen.
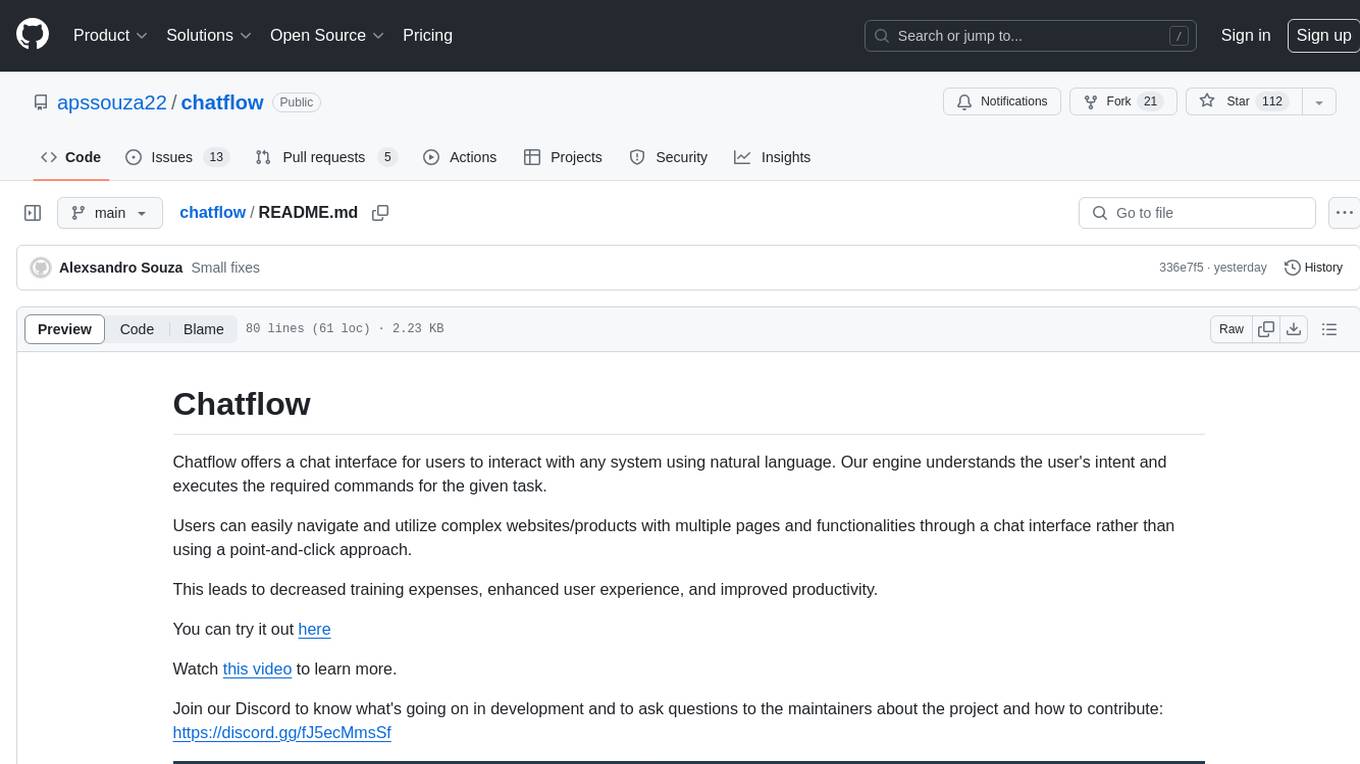
chatflow
Chatflow is a tool that provides a chat interface for users to interact with systems using natural language. The engine understands user intent and executes commands for tasks, allowing easy navigation of complex websites/products. This approach enhances user experience, reduces training costs, and boosts productivity.
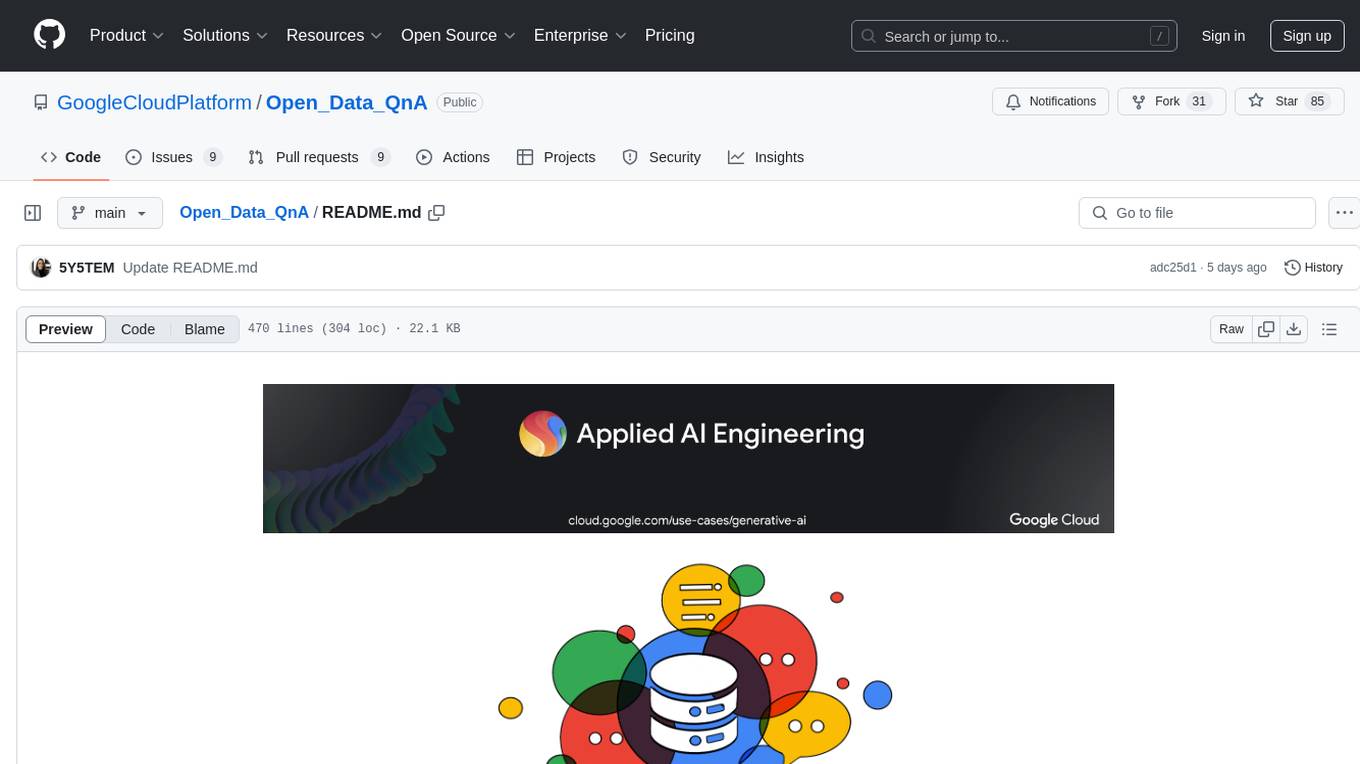
Open_Data_QnA
Open Data QnA is a Python library that allows users to interact with their PostgreSQL or BigQuery databases in a conversational manner, without needing to write SQL queries. The library leverages Large Language Models (LLMs) to bridge the gap between human language and database queries, enabling users to ask questions in natural language and receive informative responses. It offers features such as conversational querying with multiturn support, table grouping, multi schema/dataset support, SQL generation, query refinement, natural language responses, visualizations, and extensibility. The library is built on a modular design and supports various components like Database Connectors, Vector Stores, and Agents for SQL generation, validation, debugging, descriptions, embeddings, responses, and visualizations.
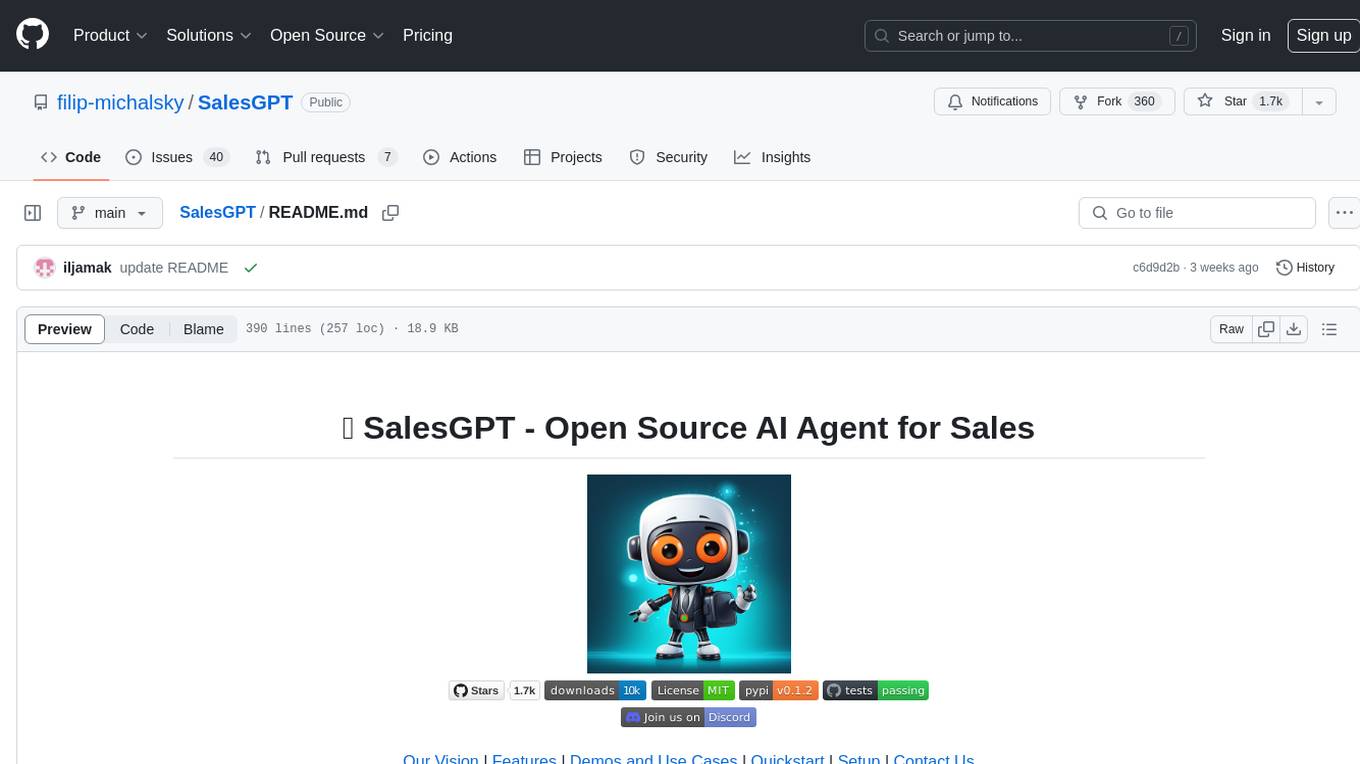
SalesGPT
SalesGPT is an open-source AI agent designed for sales, utilizing context-awareness and LLMs to work across various communication channels like voice, email, and texting. It aims to enhance sales conversations by understanding the stage of the conversation and providing tools like product knowledge base to reduce errors. The agent can autonomously generate payment links, handle objections, and close sales. It also offers features like automated email communication, meeting scheduling, and integration with various LLMs for customization. SalesGPT is optimized for low latency in voice channels and ensures human supervision where necessary. The tool provides enterprise-grade security and supports LangSmith tracing for monitoring and evaluation of intelligent agents built on LLM frameworks.
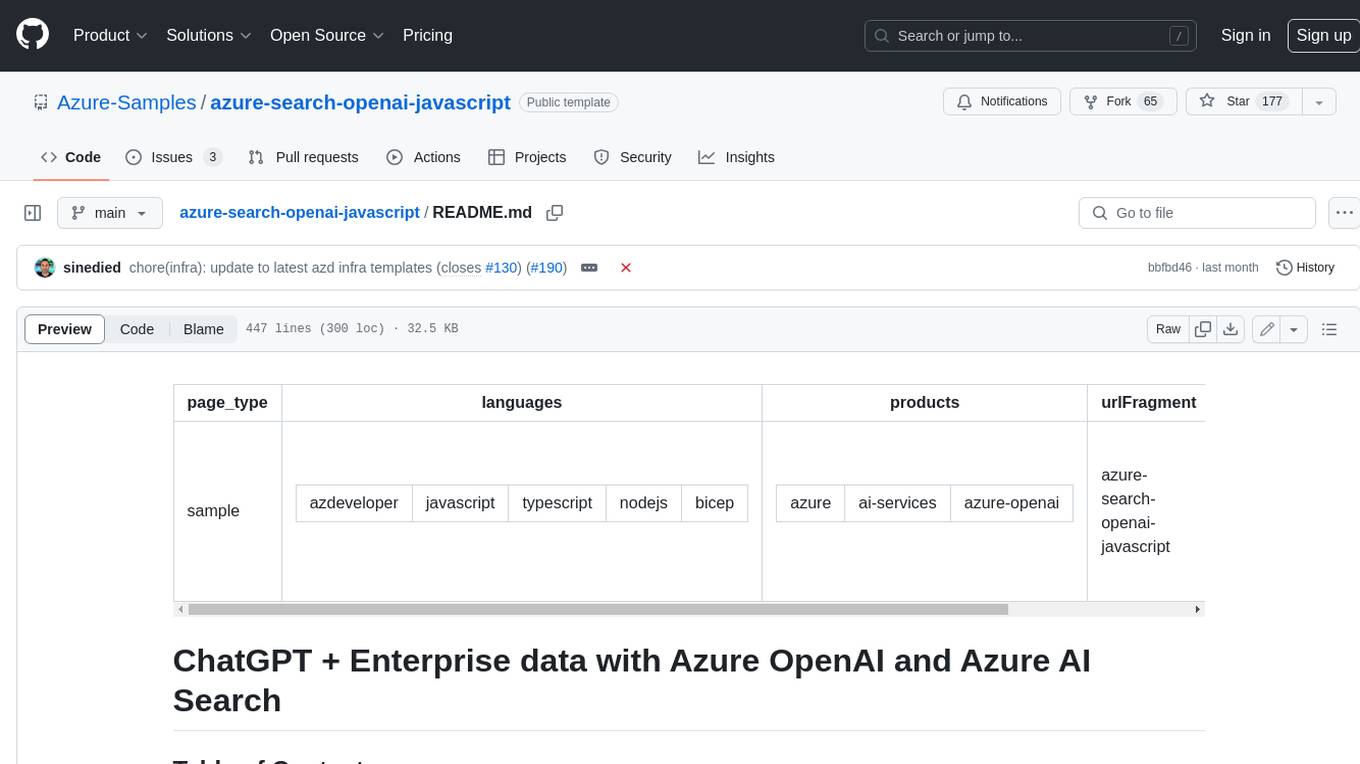
azure-search-openai-javascript
This sample demonstrates a few approaches for creating ChatGPT-like experiences over your own data using the Retrieval Augmented Generation pattern. It uses Azure OpenAI Service to access the ChatGPT model (gpt-35-turbo), and Azure AI Search for data indexing and retrieval.
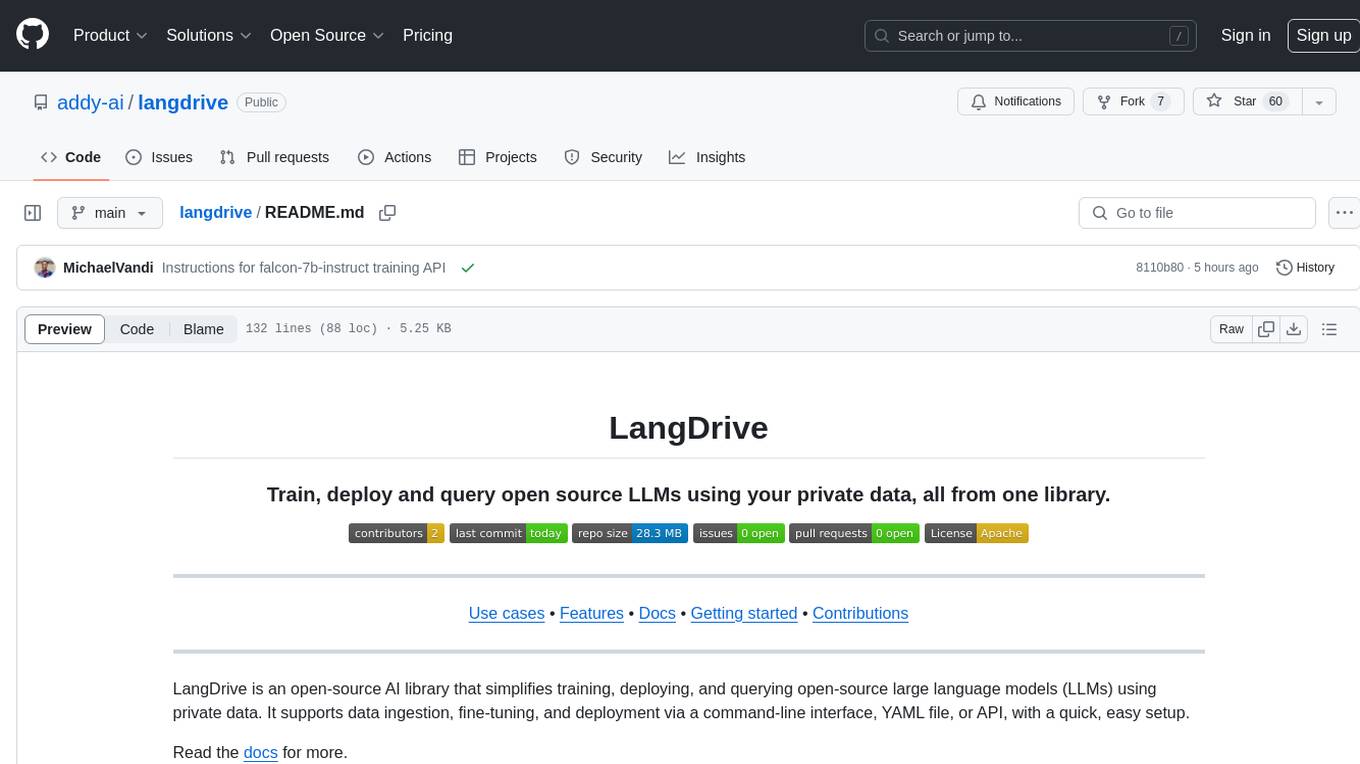
langdrive
LangDrive is an open-source AI library that simplifies training, deploying, and querying open-source large language models (LLMs) using private data. It supports data ingestion, fine-tuning, and deployment via a command-line interface, YAML file, or API, with a quick, easy setup. Users can build AI applications such as question/answering systems, chatbots, AI agents, and content generators. The library provides features like data connectors for ingestion, fine-tuning of LLMs, deployment to Hugging Face hub, inference querying, data utilities for CRUD operations, and APIs for model access. LangDrive is designed to streamline the process of working with LLMs and making AI development more accessible.
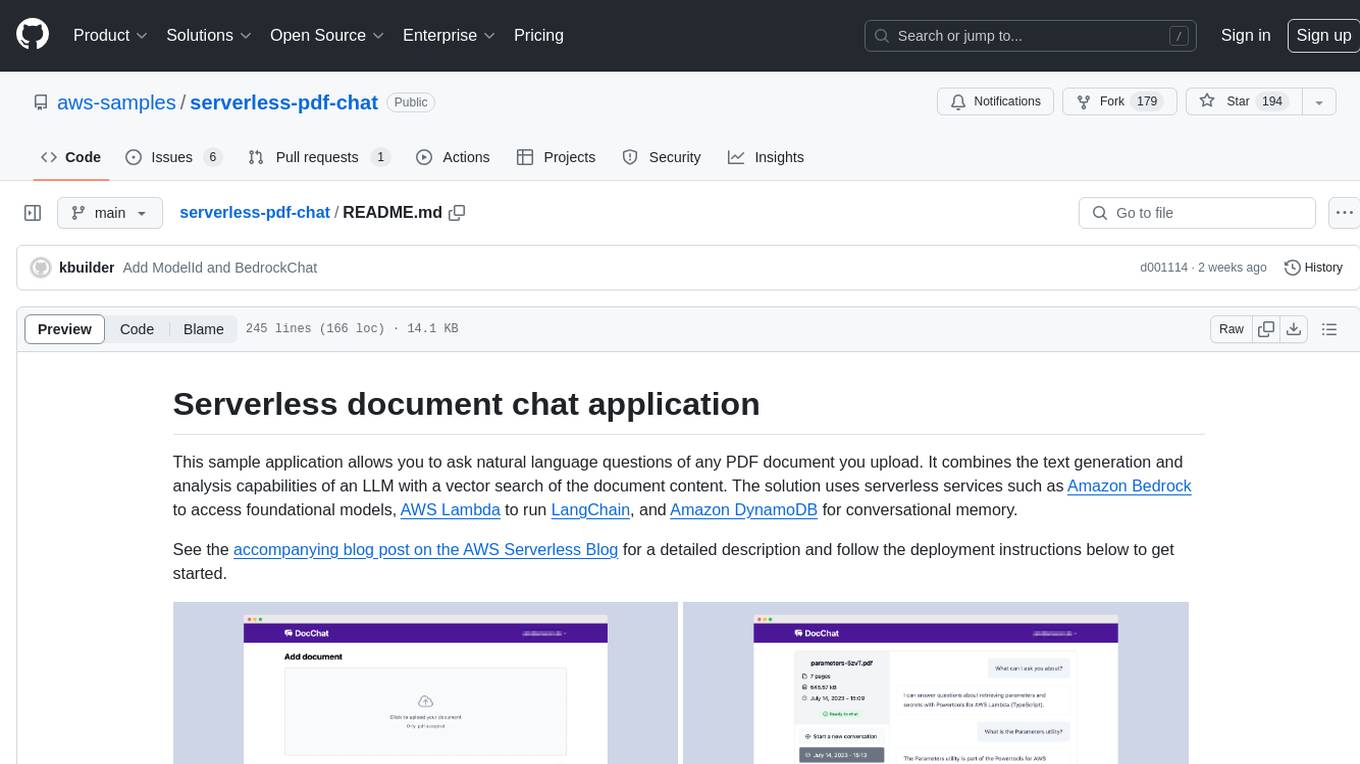
serverless-pdf-chat
The serverless-pdf-chat repository contains a sample application that allows users to ask natural language questions of any PDF document they upload. It leverages serverless services like Amazon Bedrock, AWS Lambda, and Amazon DynamoDB to provide text generation and analysis capabilities. The application architecture involves uploading a PDF document to an S3 bucket, extracting metadata, converting text to vectors, and using a LangChain to search for information related to user prompts. The application is not intended for production use and serves as a demonstration and educational tool.
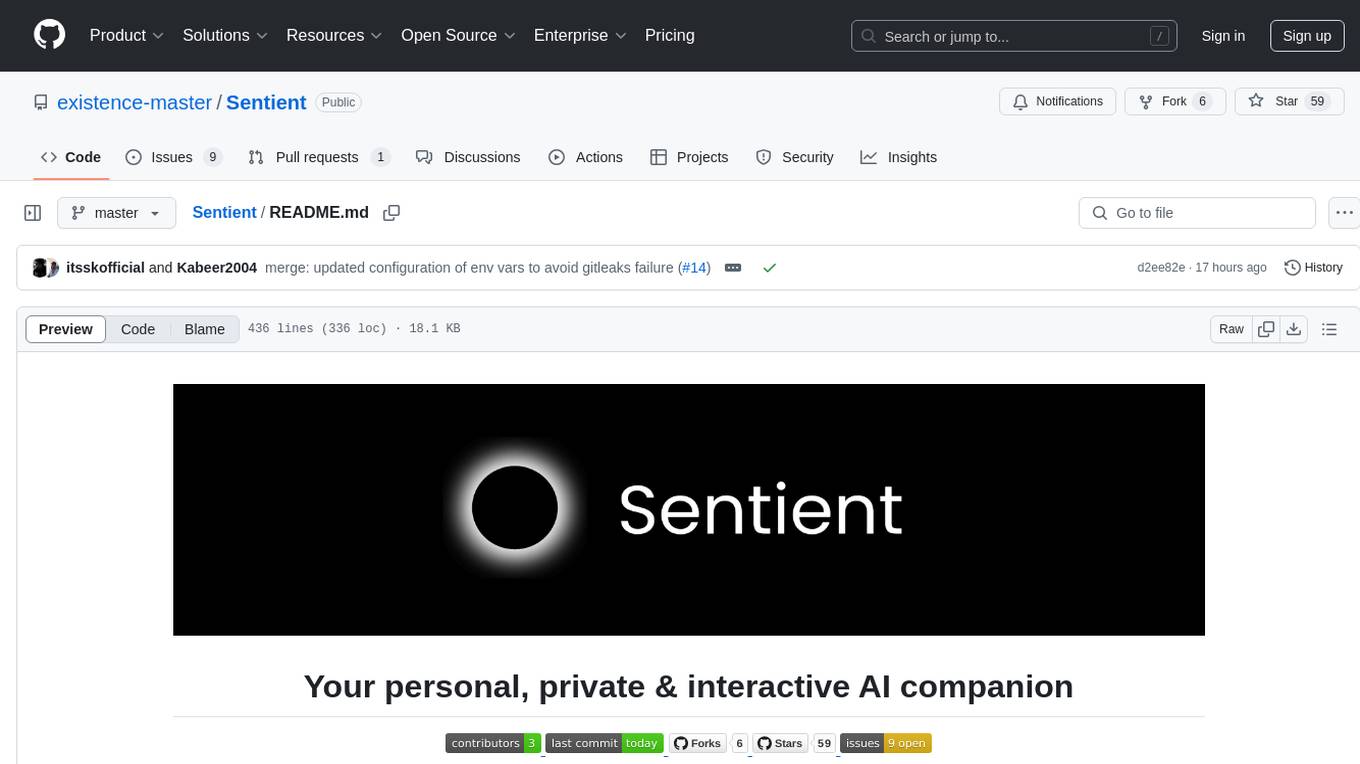
Sentient
Sentient is a personal, private, and interactive AI companion developed by Existence. The project aims to build a completely private AI companion that is deeply personalized and context-aware of the user. It utilizes automation and privacy to create a true companion for humans. The tool is designed to remember information about the user and use it to respond to queries and perform various actions. Sentient features a local and private environment, MBTI personality test, integrations with LinkedIn, Reddit, and more, self-managed graph memory, web search capabilities, multi-chat functionality, and auto-updates for the app. The project is built using technologies like ElectronJS, Next.js, TailwindCSS, FastAPI, Neo4j, and various APIs.
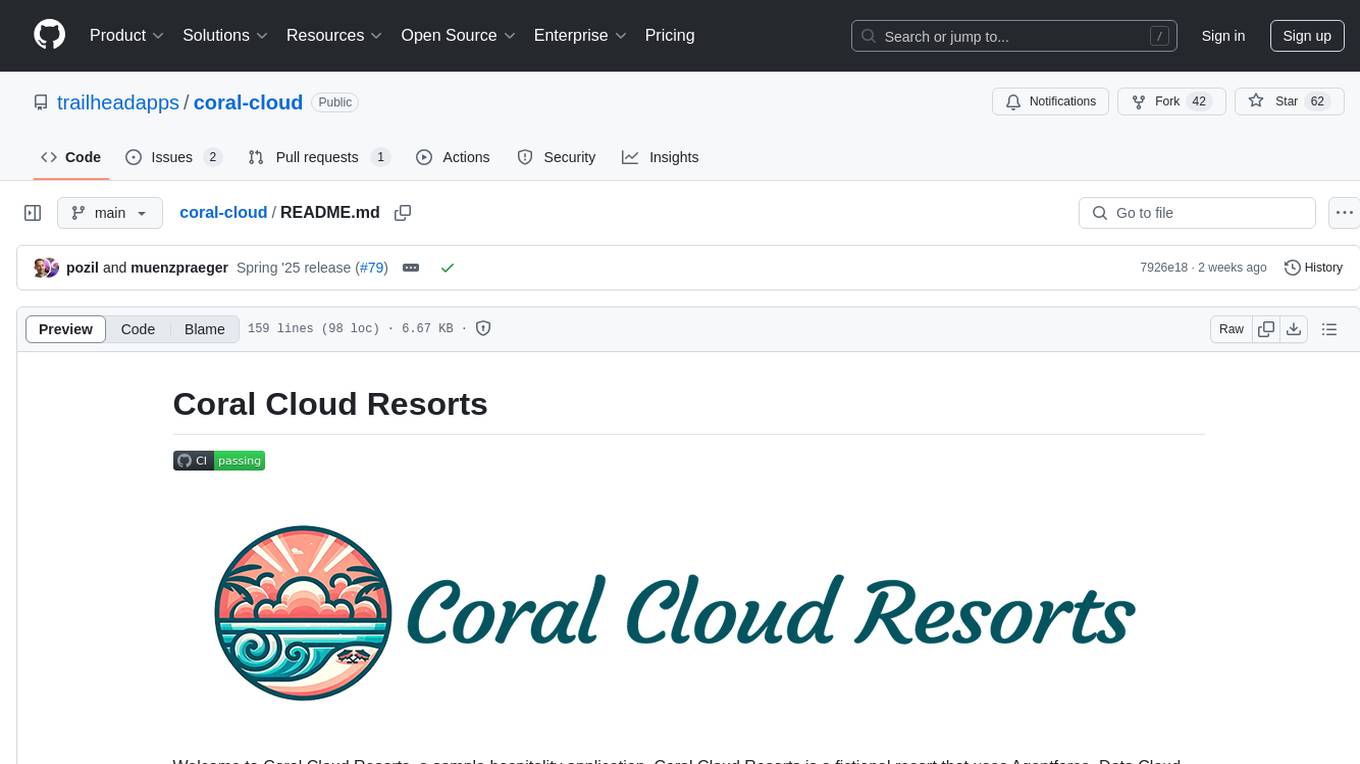
coral-cloud
Coral Cloud Resorts is a sample hospitality application that showcases Data Cloud, Agents, and Prompts. It provides highly personalized guest experiences through smart automation, content generation, and summarization. The app requires licenses for Data Cloud, Agents, Prompt Builder, and Einstein for Sales. Users can activate features, deploy metadata, assign permission sets, import sample data, and troubleshoot common issues. Additionally, the repository offers integration with modern web development tools like Prettier, ESLint, and pre-commit hooks for code formatting and linting.
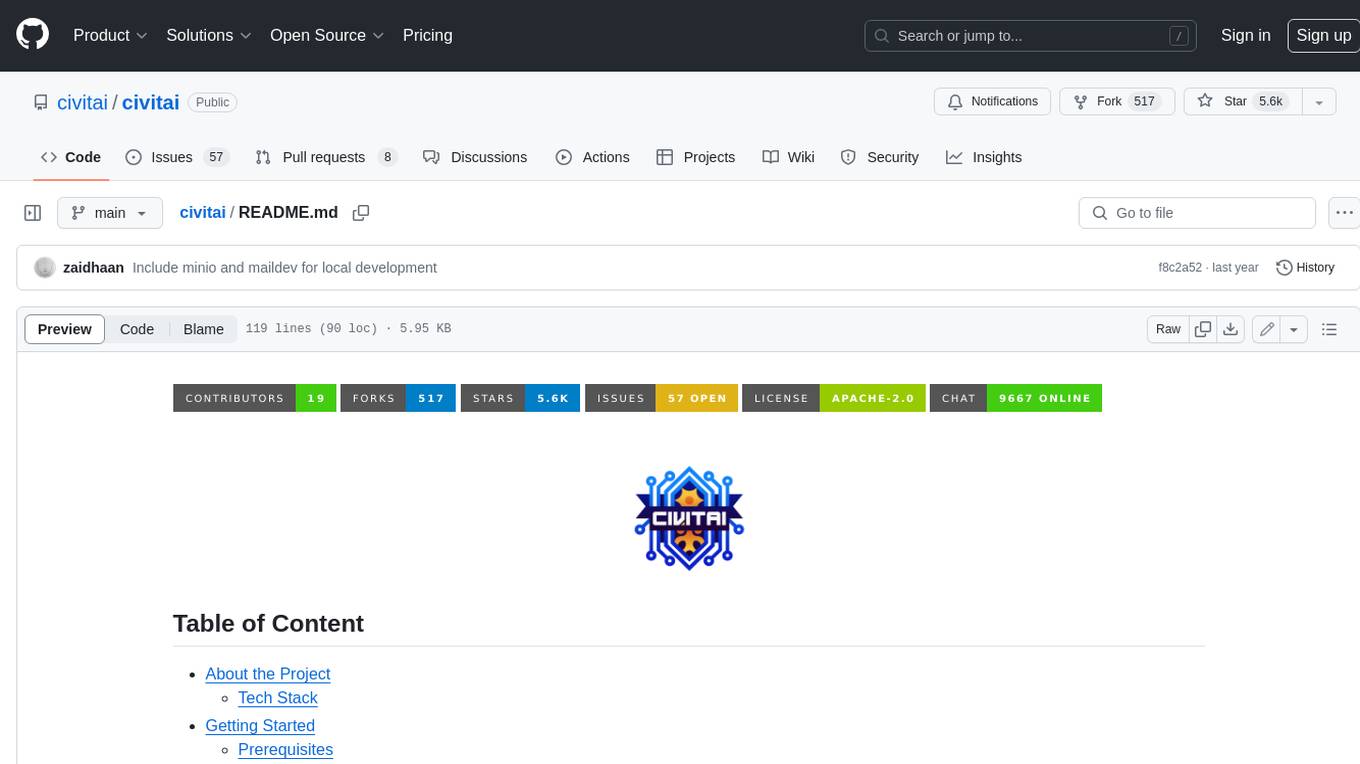
civitai
Civitai is a platform where people can share their stable diffusion models (textual inversions, hypernetworks, aesthetic gradients, VAEs, and any other crazy stuff people do to customize their AI generations), collaborate with others to improve them, and learn from each other's work. The platform allows users to create an account, upload their models, and browse models that have been shared by others. Users can also leave comments and feedback on each other's models to facilitate collaboration and knowledge sharing.
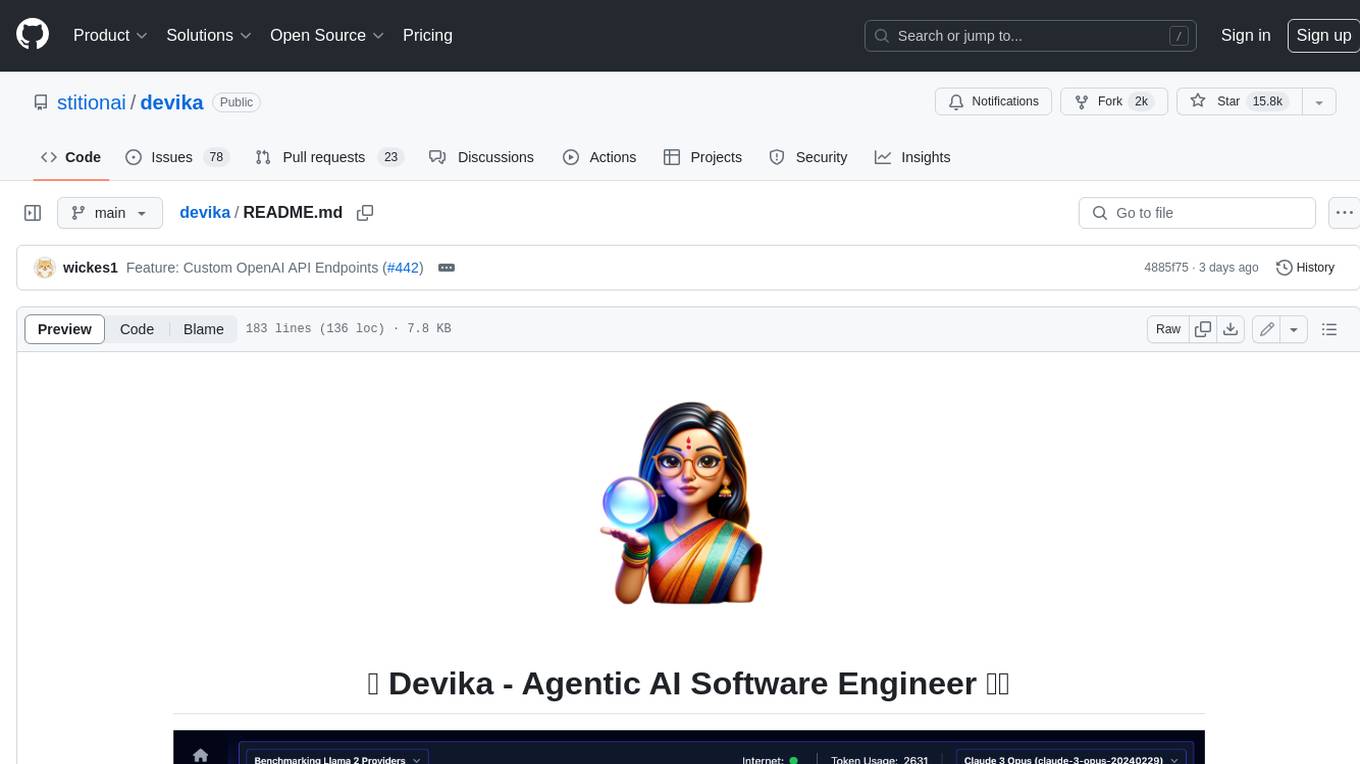
devika
Devika is an advanced AI software engineer that can understand high-level human instructions, break them down into steps, research relevant information, and write code to achieve the given objective. Devika utilizes large language models, planning and reasoning algorithms, and web browsing abilities to intelligently develop software. Devika aims to revolutionize the way we build software by providing an AI pair programmer who can take on complex coding tasks with minimal human guidance. Whether you need to create a new feature, fix a bug, or develop an entire project from scratch, Devika is here to assist you.
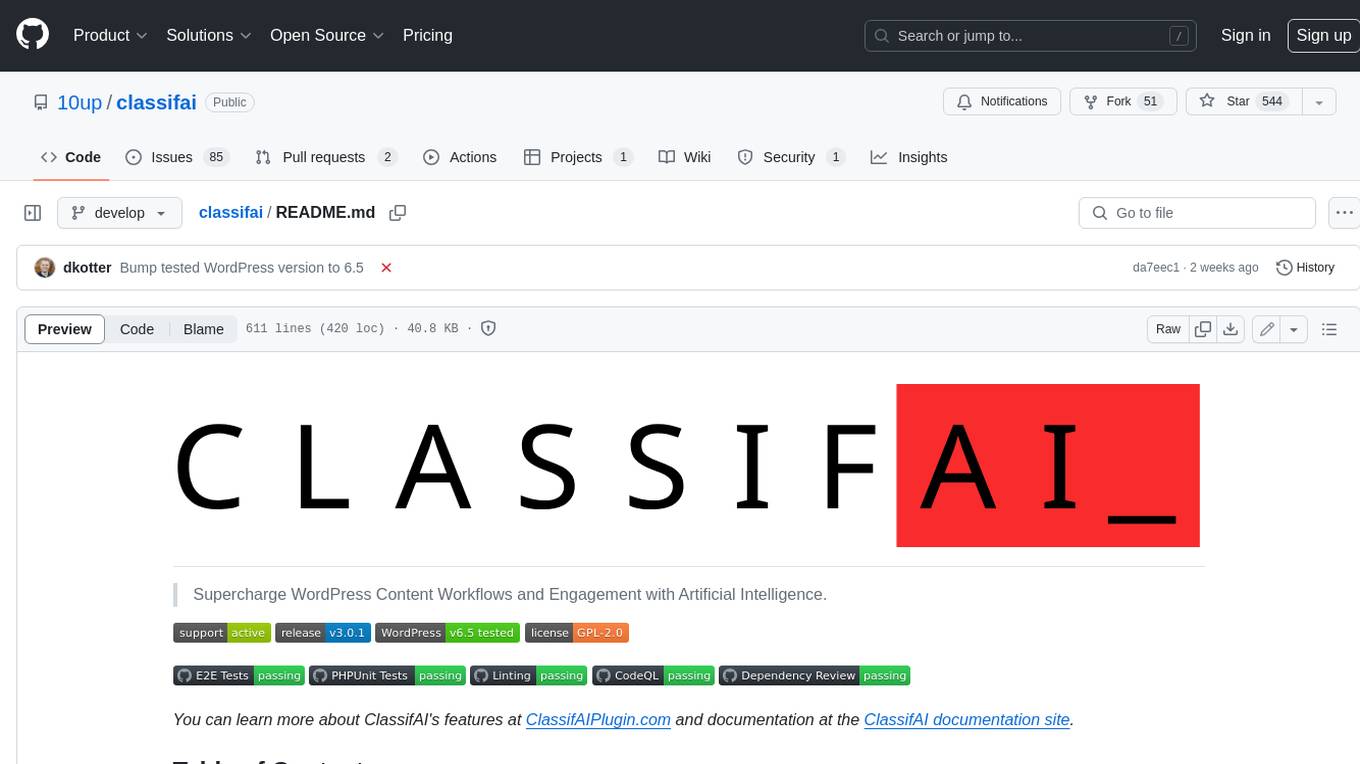
classifai
Supercharge WordPress Content Workflows and Engagement with Artificial Intelligence. Tap into leading cloud-based services like OpenAI, Microsoft Azure AI, Google Gemini and IBM Watson to augment your WordPress-powered websites. Publish content faster while improving SEO performance and increasing audience engagement. ClassifAI integrates Artificial Intelligence and Machine Learning technologies to lighten your workload and eliminate tedious tasks, giving you more time to create original content that matters.
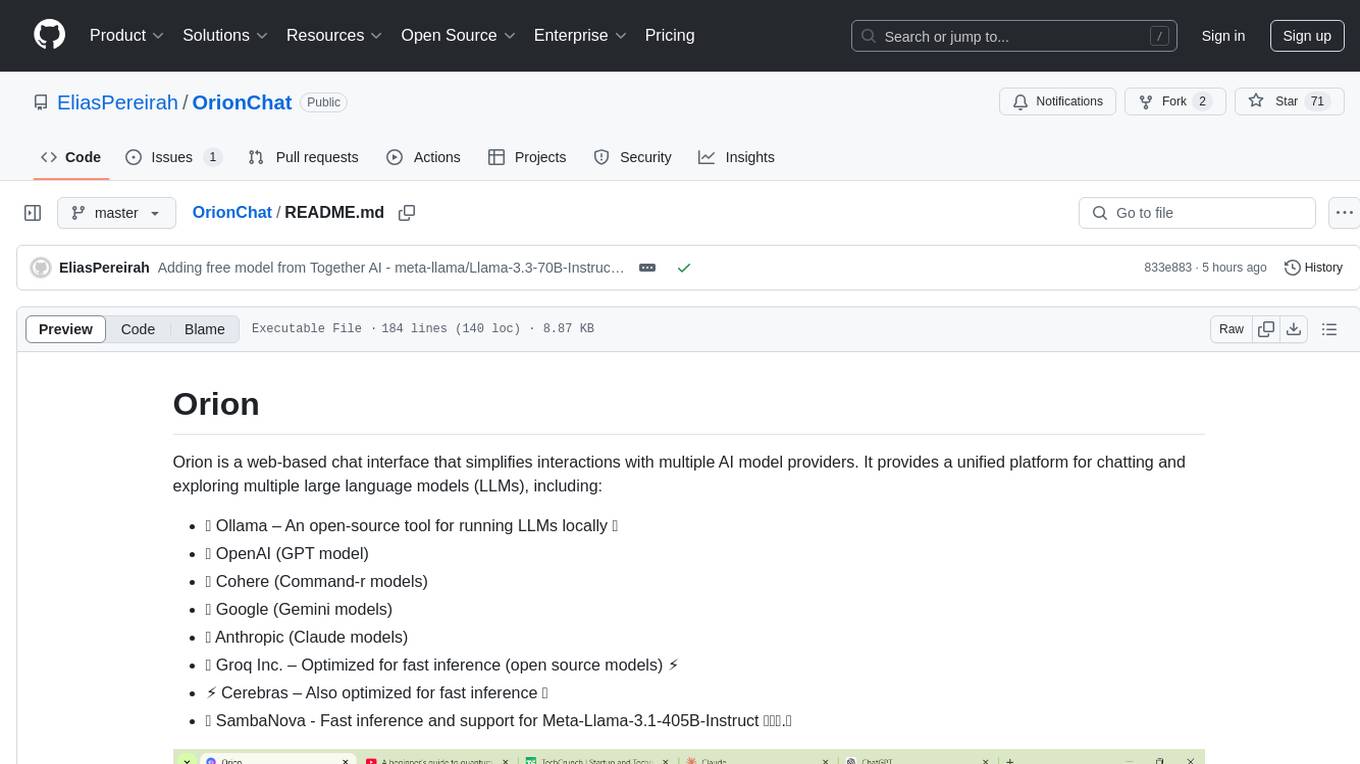
OrionChat
Orion is a web-based chat interface that simplifies interactions with multiple AI model providers. It provides a unified platform for chatting and exploring various large language models (LLMs) such as Ollama, OpenAI (GPT model), Cohere (Command-r models), Google (Gemini models), Anthropic (Claude models), Groq Inc., Cerebras, and SambaNova. Users can easily navigate and assess different AI models through an intuitive, user-friendly interface. Orion offers features like browser-based access, code execution with Google Gemini, text-to-speech (TTS), speech-to-text (STT), seamless integration with multiple AI models, customizable system prompts, language translation tasks, document uploads for analysis, and more. API keys are stored locally, and requests are sent directly to official providers' APIs without external proxies.
For similar tasks
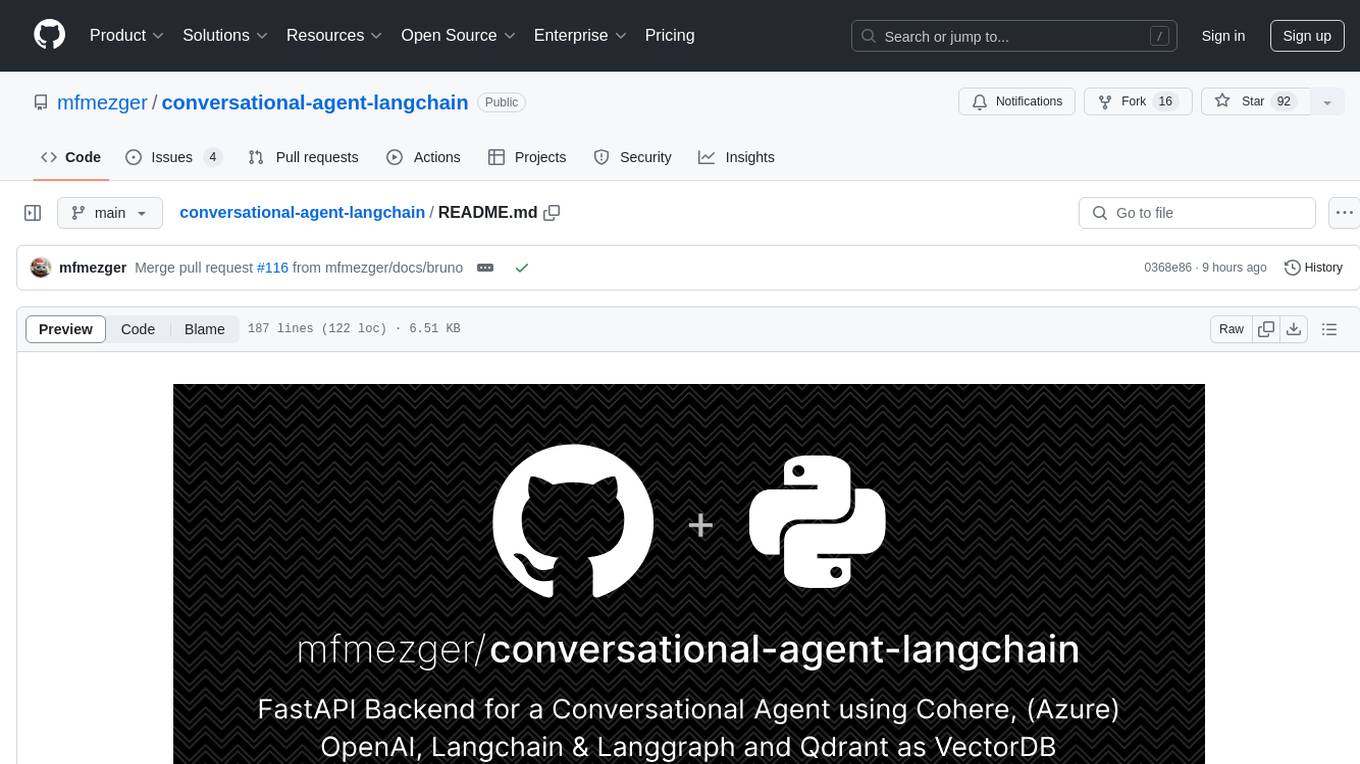
conversational-agent-langchain
This repository contains a Rest-Backend for a Conversational Agent that allows embedding documents, semantic search, QA based on documents, and document processing with Large Language Models. It uses Aleph Alpha and OpenAI Large Language Models to generate responses to user queries, includes a vector database, and provides a REST API built with FastAPI. The project also features semantic search, secret management for API keys, installation instructions, and development guidelines for both backend and frontend components.
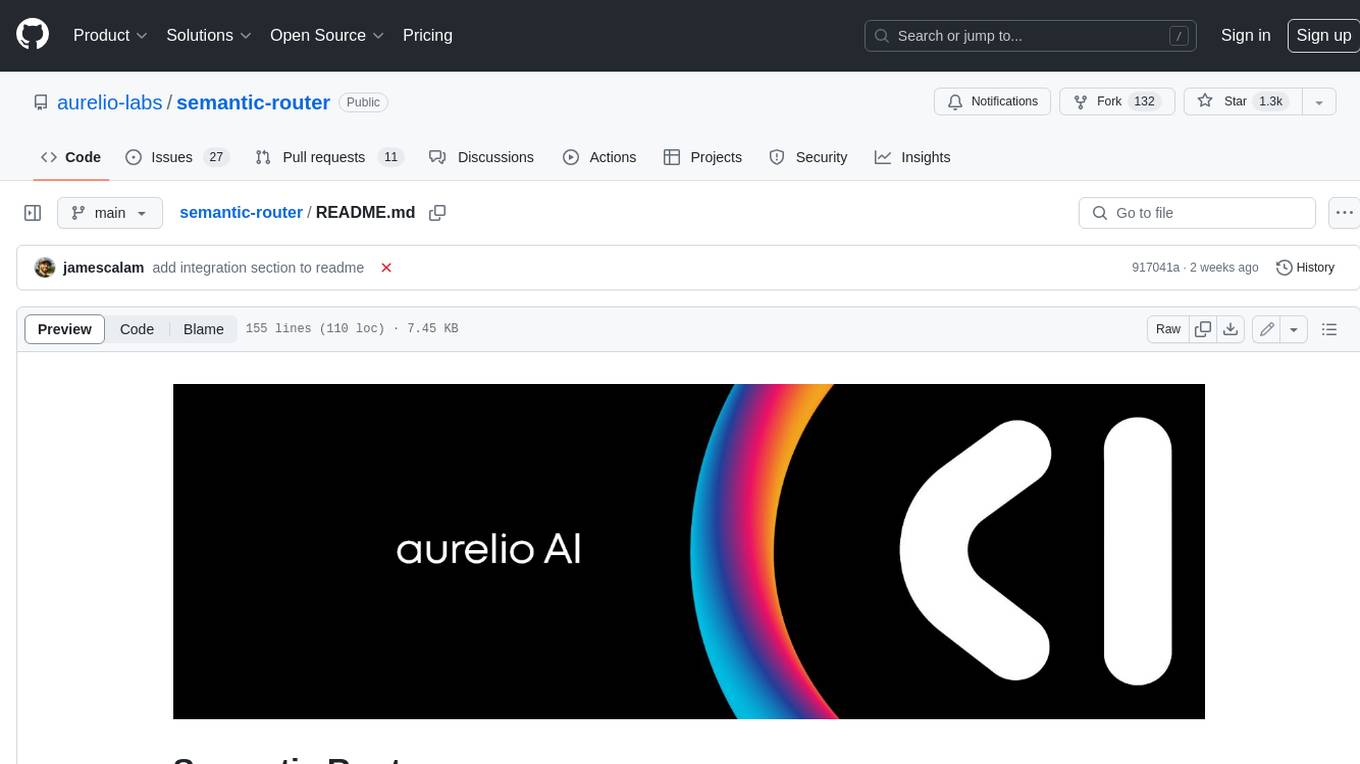
semantic-router
Semantic Router is a superfast decision-making layer for your LLMs and agents. Rather than waiting for slow LLM generations to make tool-use decisions, we use the magic of semantic vector space to make those decisions — _routing_ our requests using _semantic_ meaning.
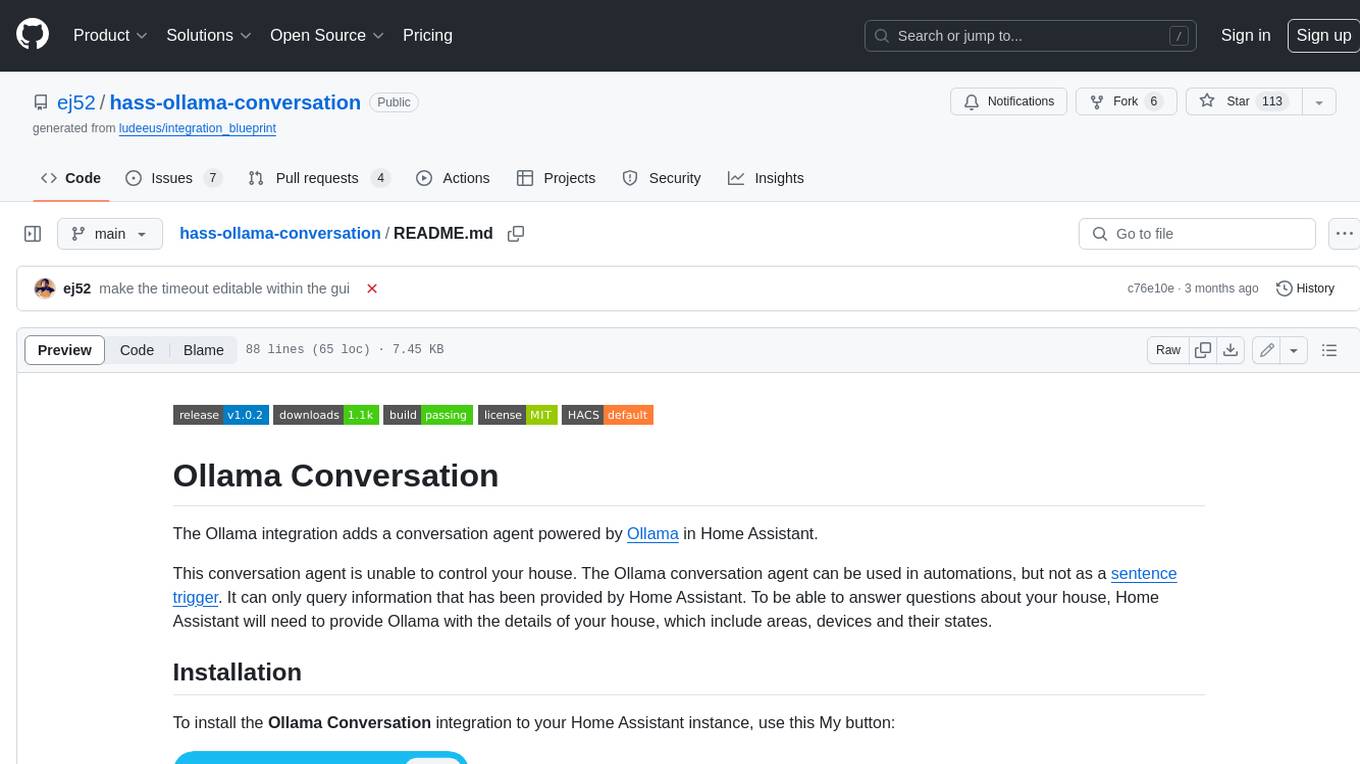
hass-ollama-conversation
The Ollama Conversation integration adds a conversation agent powered by Ollama in Home Assistant. This agent can be used in automations to query information provided by Home Assistant about your house, including areas, devices, and their states. Users can install the integration via HACS and configure settings such as API timeout, model selection, context size, maximum tokens, and other parameters to fine-tune the responses generated by the AI language model. Contributions to the project are welcome, and discussions can be held on the Home Assistant Community platform.
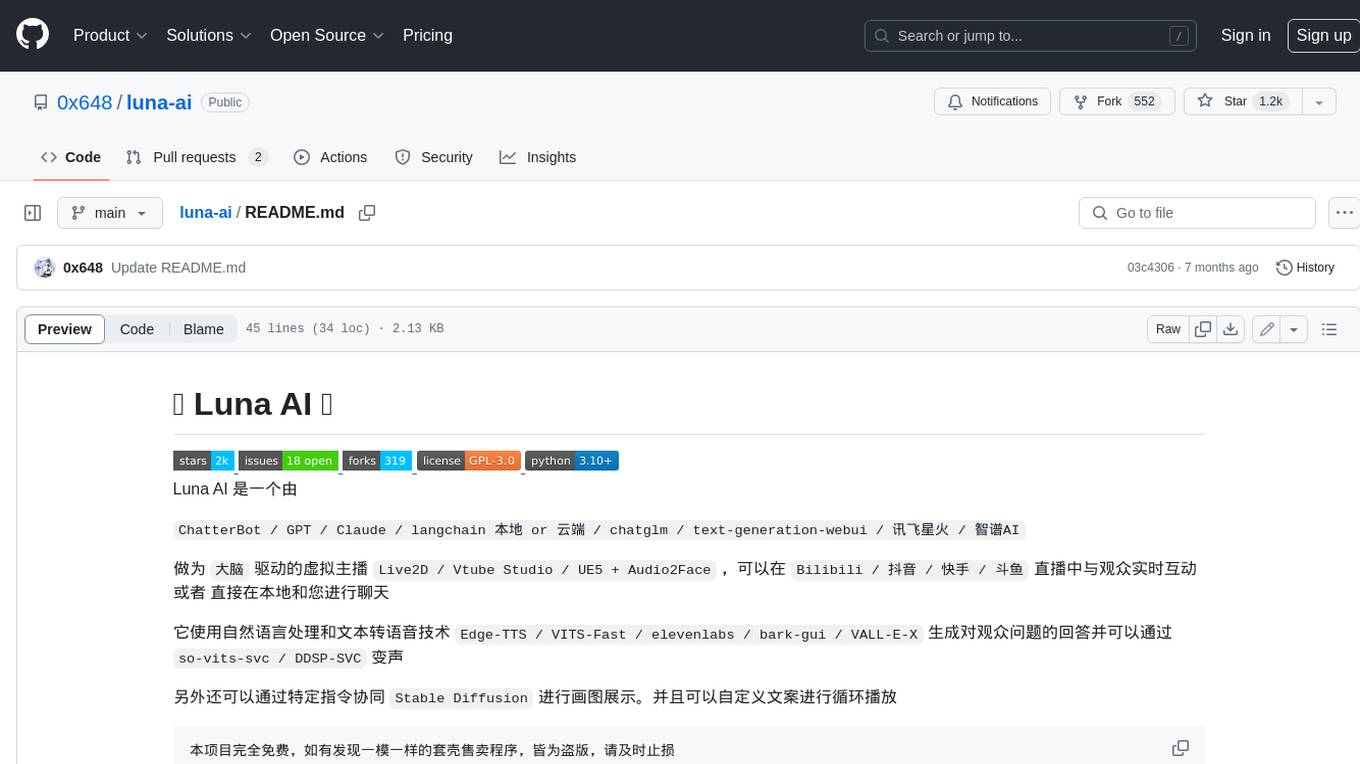
luna-ai
Luna AI is a virtual streamer driven by a 'brain' composed of ChatterBot, GPT, Claude, langchain, chatglm, text-generation-webui, 讯飞星火, 智谱AI. It can interact with viewers in real-time during live streams on platforms like Bilibili, Douyin, Kuaishou, Douyu, or chat with you locally. Luna AI uses natural language processing and text-to-speech technologies like Edge-TTS, VITS-Fast, elevenlabs, bark-gui, VALL-E-X to generate responses to viewer questions and can change voice using so-vits-svc, DDSP-SVC. It can also collaborate with Stable Diffusion for drawing displays and loop custom texts. This project is completely free, and any identical copycat selling programs are pirated, please stop them promptly.
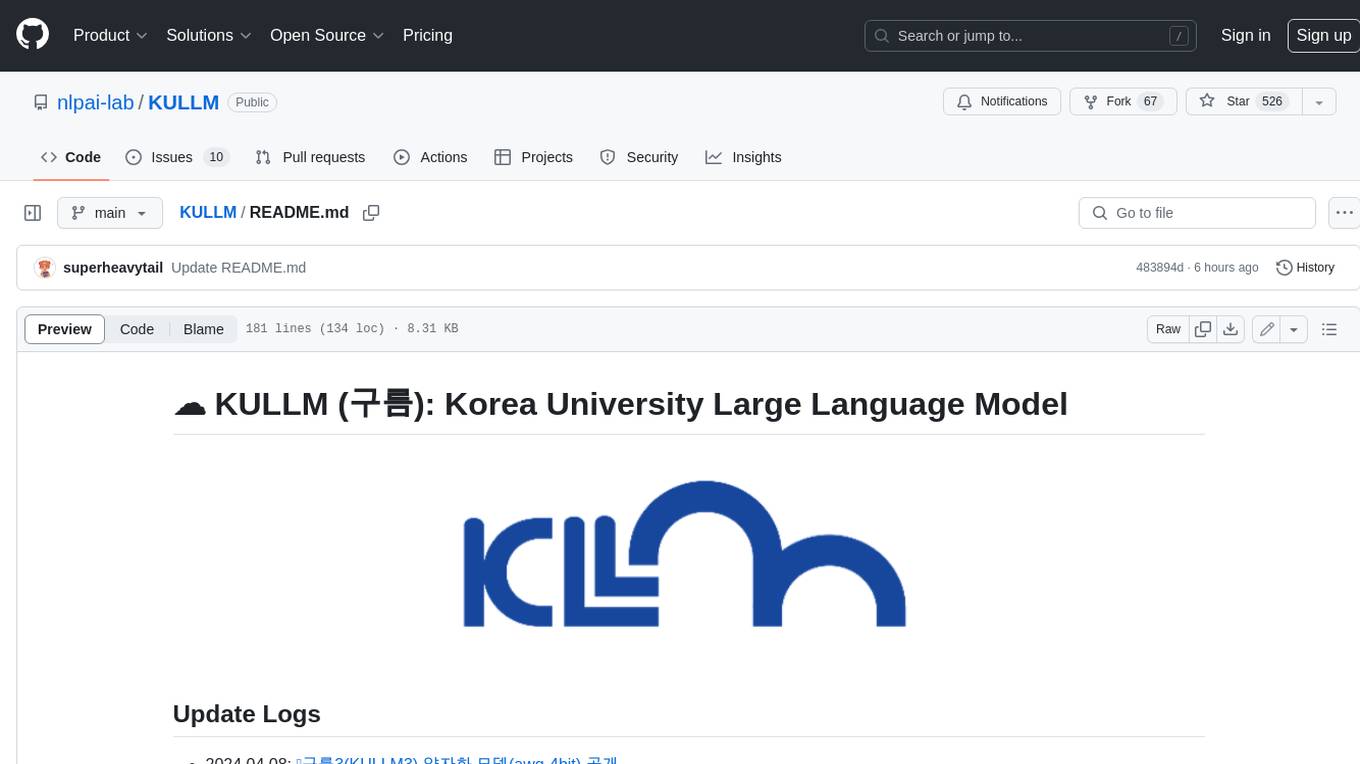
KULLM
KULLM (구름) is a Korean Large Language Model developed by Korea University NLP & AI Lab and HIAI Research Institute. It is based on the upstage/SOLAR-10.7B-v1.0 model and has been fine-tuned for instruction. The model has been trained on 8×A100 GPUs and is capable of generating responses in Korean language. KULLM exhibits hallucination and repetition phenomena due to its decoding strategy. Users should be cautious as the model may produce inaccurate or harmful results. Performance may vary in benchmarks without a fixed system prompt.
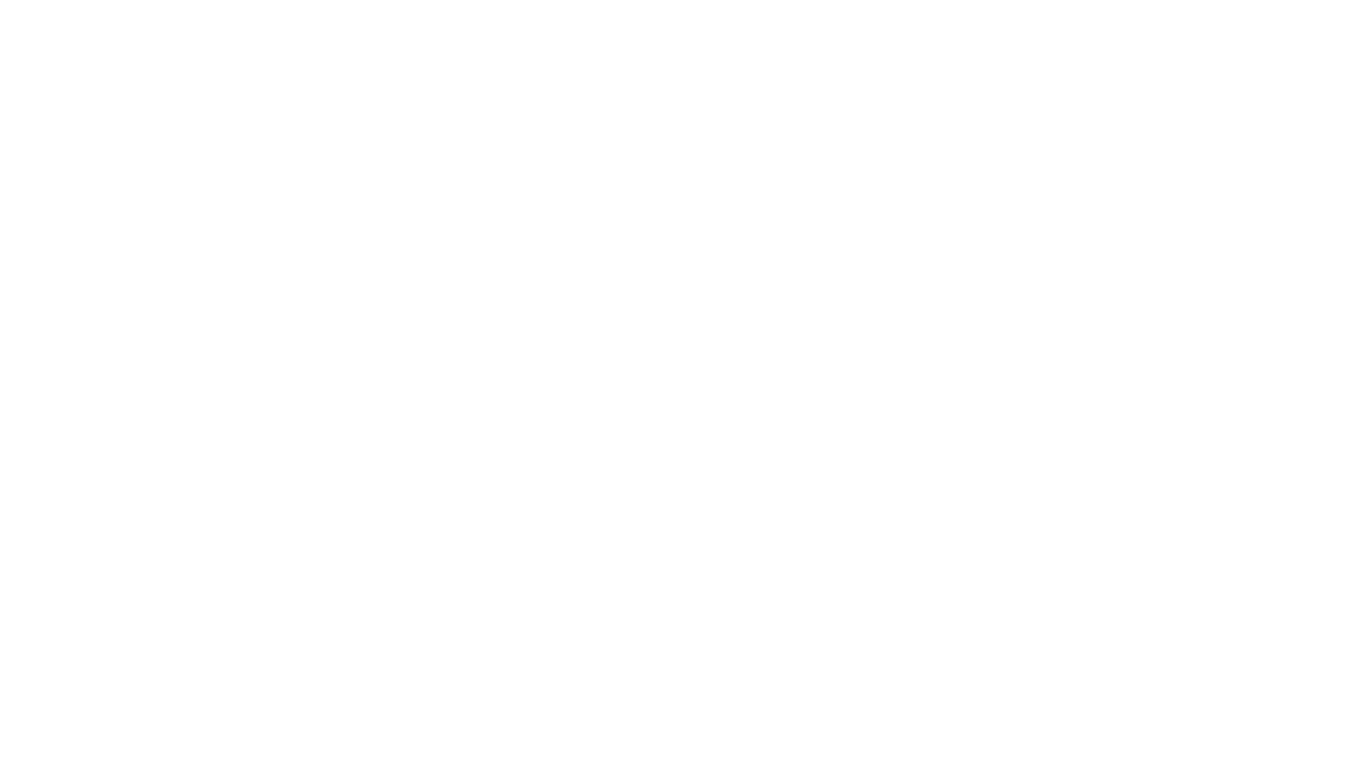
cria
Cria is a Python library designed for running Large Language Models with minimal configuration. It provides an easy and concise way to interact with LLMs, offering advanced features such as custom models, streams, message history management, and running multiple models in parallel. Cria simplifies the process of using LLMs by providing a straightforward API that requires only a few lines of code to get started. It also handles model installation automatically, making it efficient and user-friendly for various natural language processing tasks.
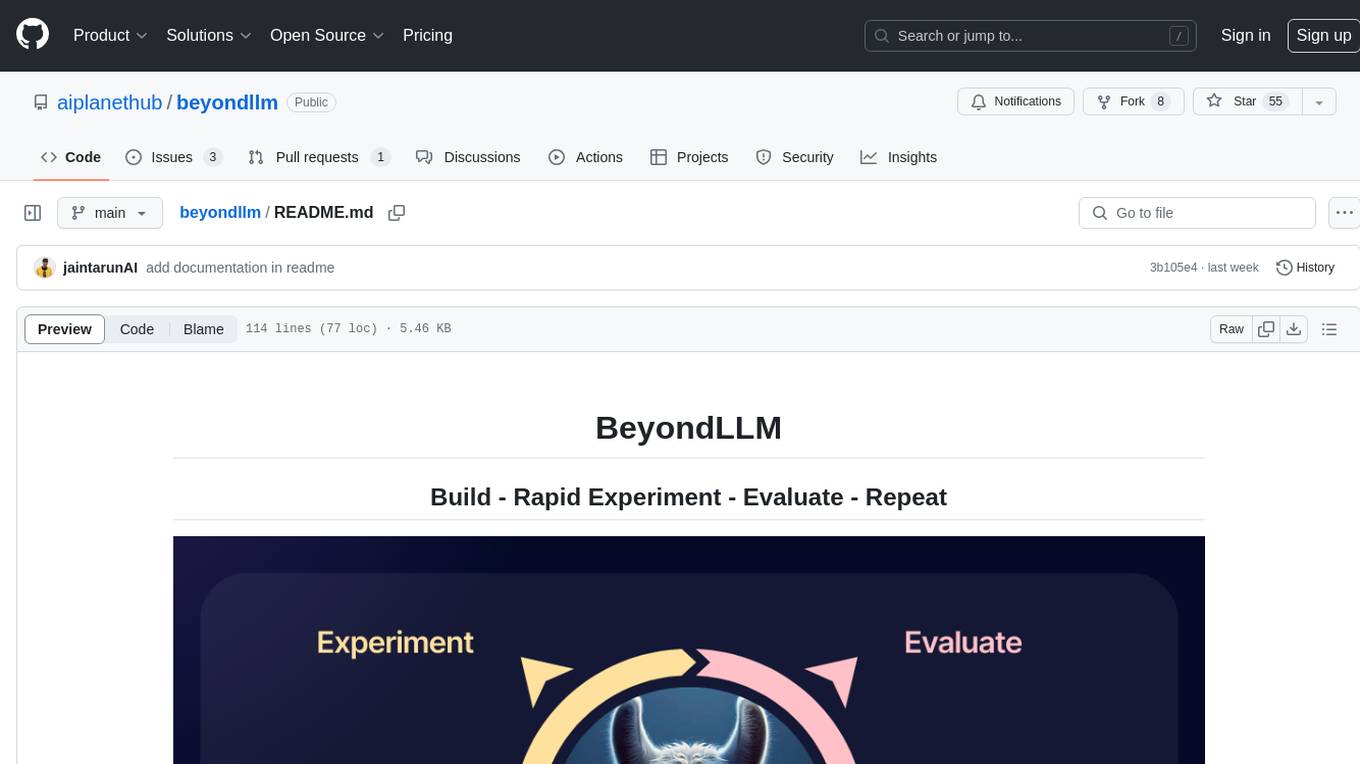
beyondllm
Beyond LLM offers an all-in-one toolkit for experimentation, evaluation, and deployment of Retrieval-Augmented Generation (RAG) systems. It simplifies the process with automated integration, customizable evaluation metrics, and support for various Large Language Models (LLMs) tailored to specific needs. The aim is to reduce LLM hallucination risks and enhance reliability.
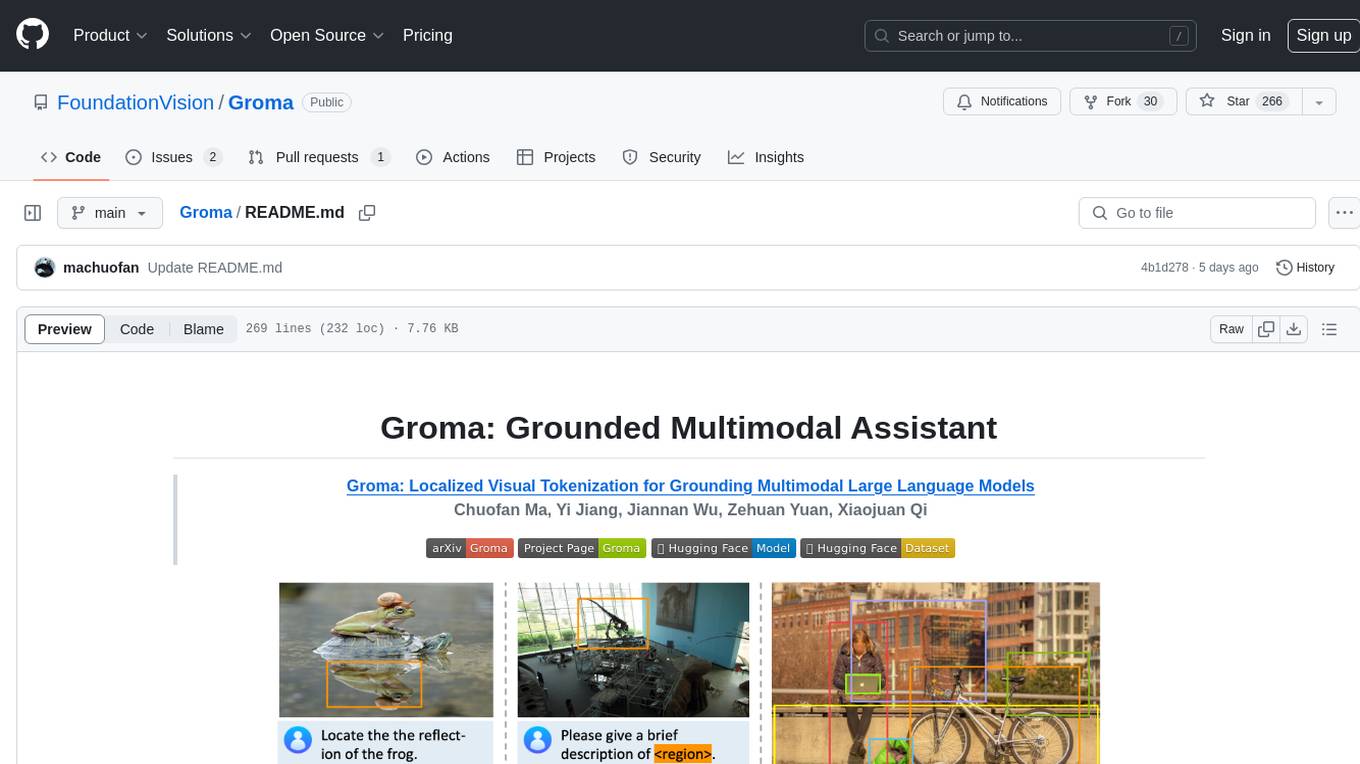
Groma
Groma is a grounded multimodal assistant that excels in region understanding and visual grounding. It can process user-defined region inputs and generate contextually grounded long-form responses. The tool presents a unique paradigm for multimodal large language models, focusing on visual tokenization for localization. Groma achieves state-of-the-art performance in referring expression comprehension benchmarks. The tool provides pretrained model weights and instructions for data preparation, training, inference, and evaluation. Users can customize training by starting from intermediate checkpoints. Groma is designed to handle tasks related to detection pretraining, alignment pretraining, instruction finetuning, instruction following, and more.
For similar jobs
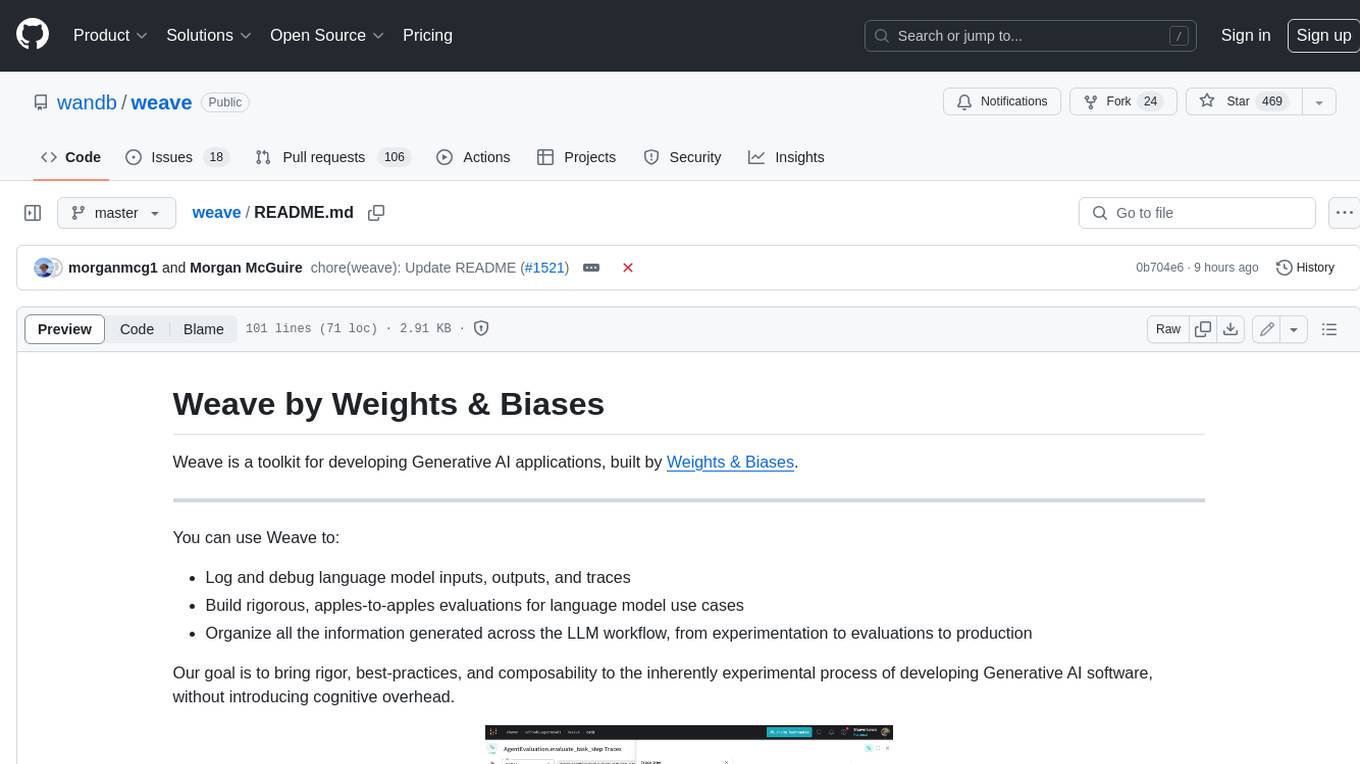
weave
Weave is a toolkit for developing Generative AI applications, built by Weights & Biases. With Weave, you can log and debug language model inputs, outputs, and traces; build rigorous, apples-to-apples evaluations for language model use cases; and organize all the information generated across the LLM workflow, from experimentation to evaluations to production. Weave aims to bring rigor, best-practices, and composability to the inherently experimental process of developing Generative AI software, without introducing cognitive overhead.
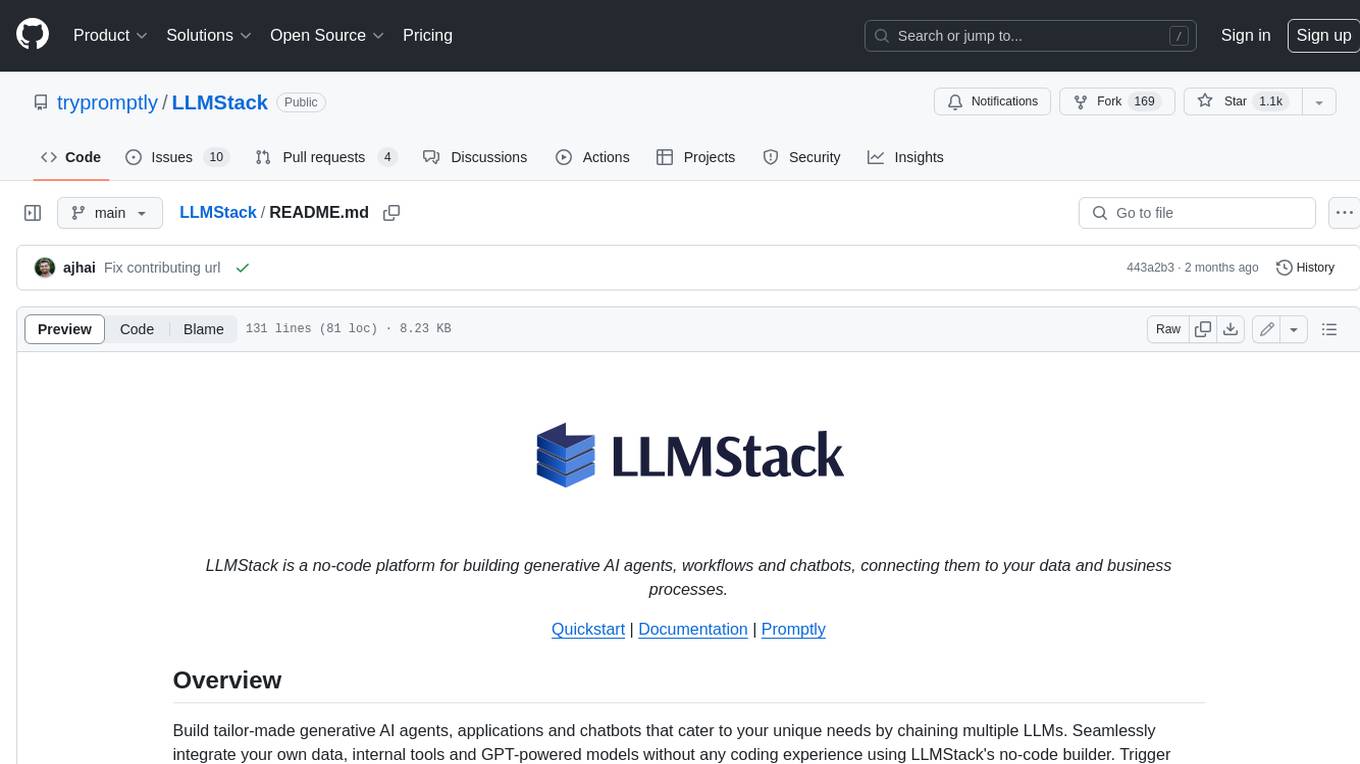
LLMStack
LLMStack is a no-code platform for building generative AI agents, workflows, and chatbots. It allows users to connect their own data, internal tools, and GPT-powered models without any coding experience. LLMStack can be deployed to the cloud or on-premise and can be accessed via HTTP API or triggered from Slack or Discord.
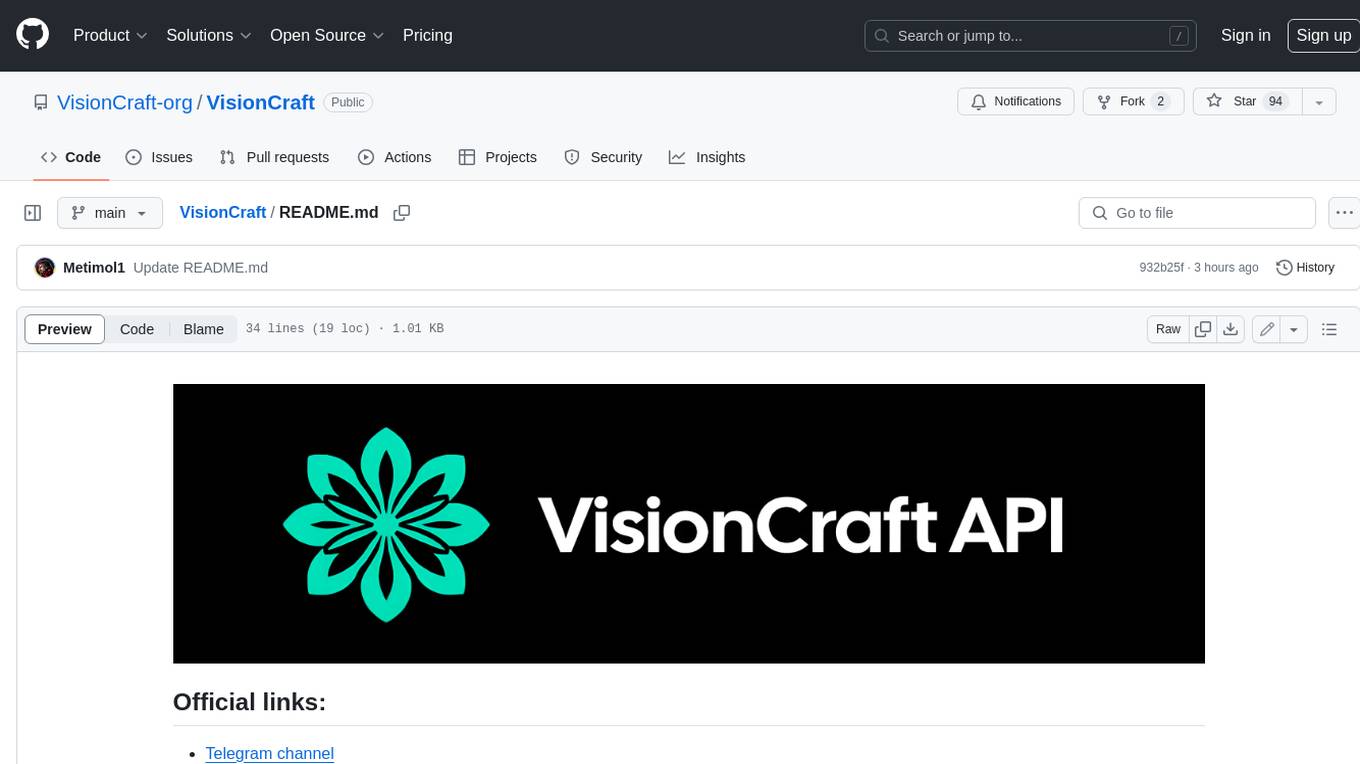
VisionCraft
The VisionCraft API is a free API for using over 100 different AI models. From images to sound.
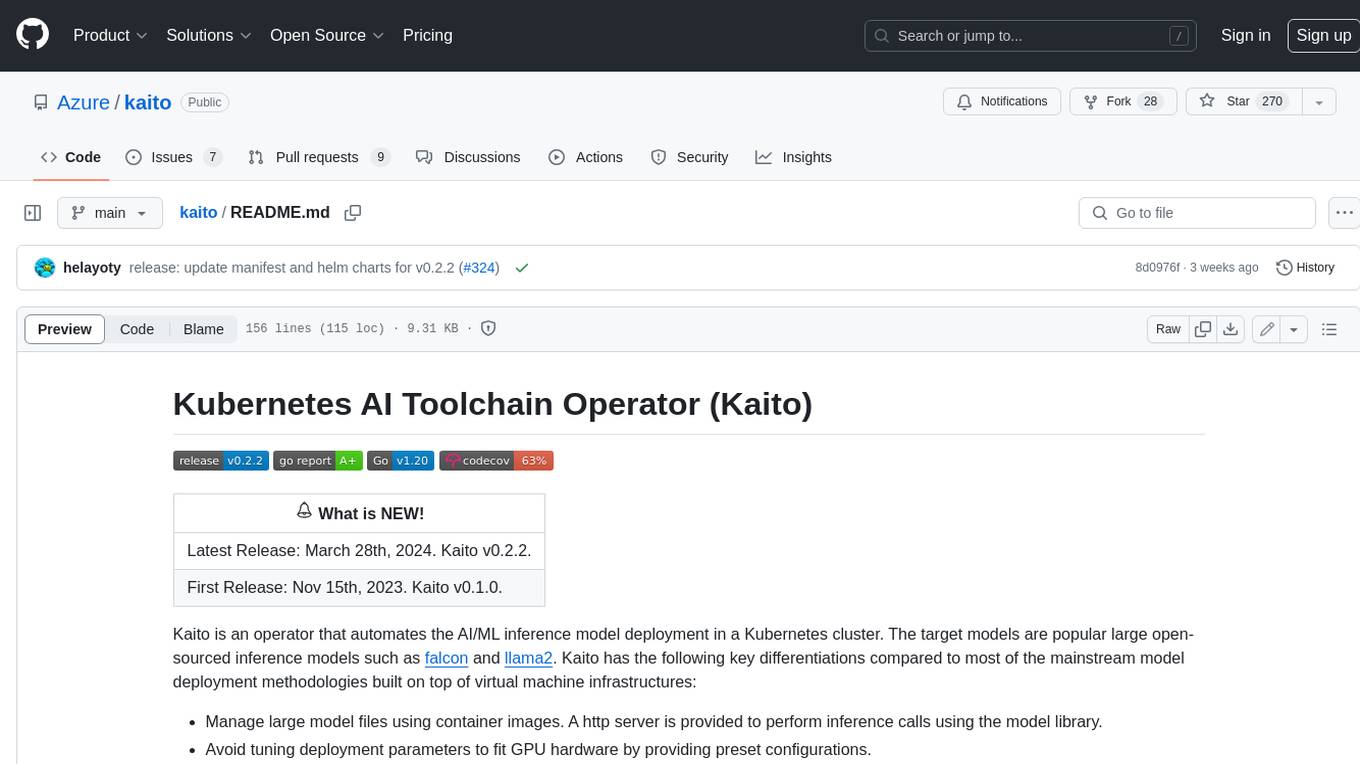
kaito
Kaito is an operator that automates the AI/ML inference model deployment in a Kubernetes cluster. It manages large model files using container images, avoids tuning deployment parameters to fit GPU hardware by providing preset configurations, auto-provisions GPU nodes based on model requirements, and hosts large model images in the public Microsoft Container Registry (MCR) if the license allows. Using Kaito, the workflow of onboarding large AI inference models in Kubernetes is largely simplified.
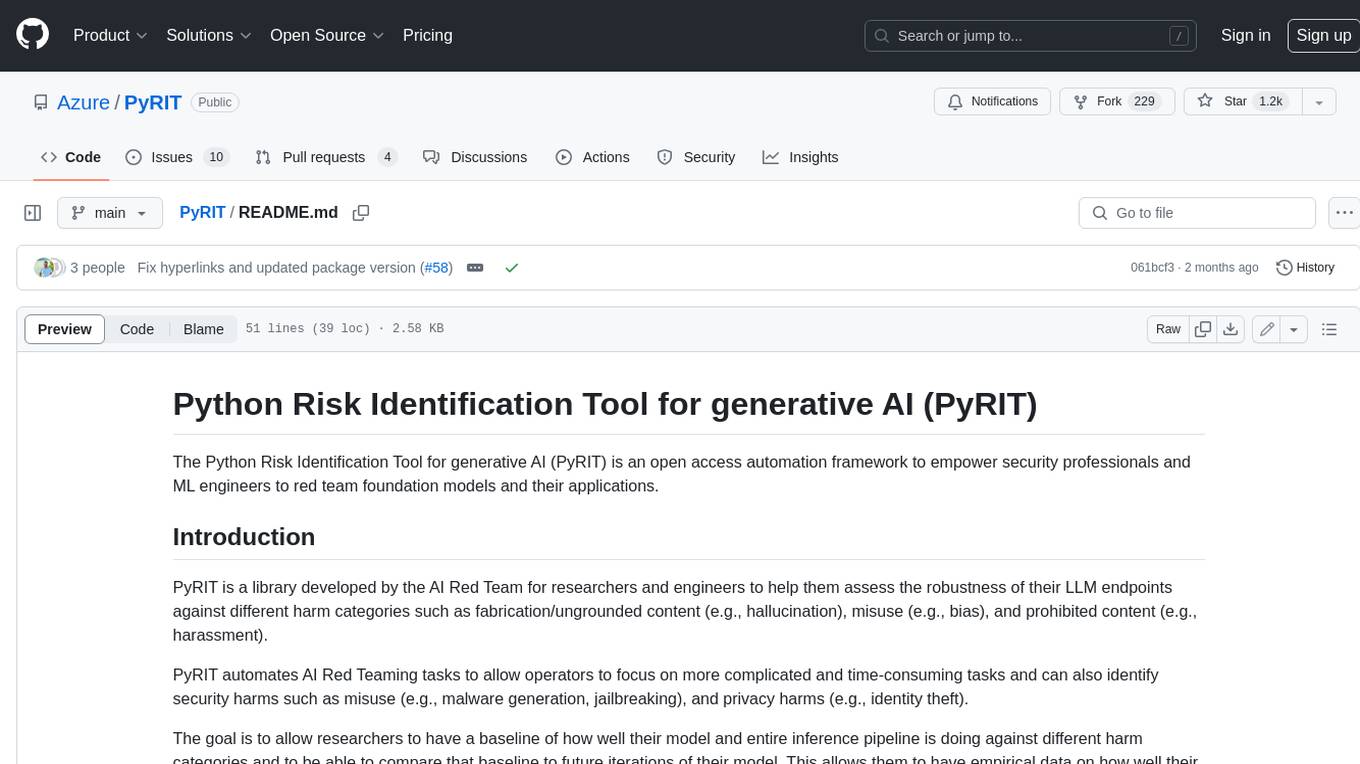
PyRIT
PyRIT is an open access automation framework designed to empower security professionals and ML engineers to red team foundation models and their applications. It automates AI Red Teaming tasks to allow operators to focus on more complicated and time-consuming tasks and can also identify security harms such as misuse (e.g., malware generation, jailbreaking), and privacy harms (e.g., identity theft). The goal is to allow researchers to have a baseline of how well their model and entire inference pipeline is doing against different harm categories and to be able to compare that baseline to future iterations of their model. This allows them to have empirical data on how well their model is doing today, and detect any degradation of performance based on future improvements.
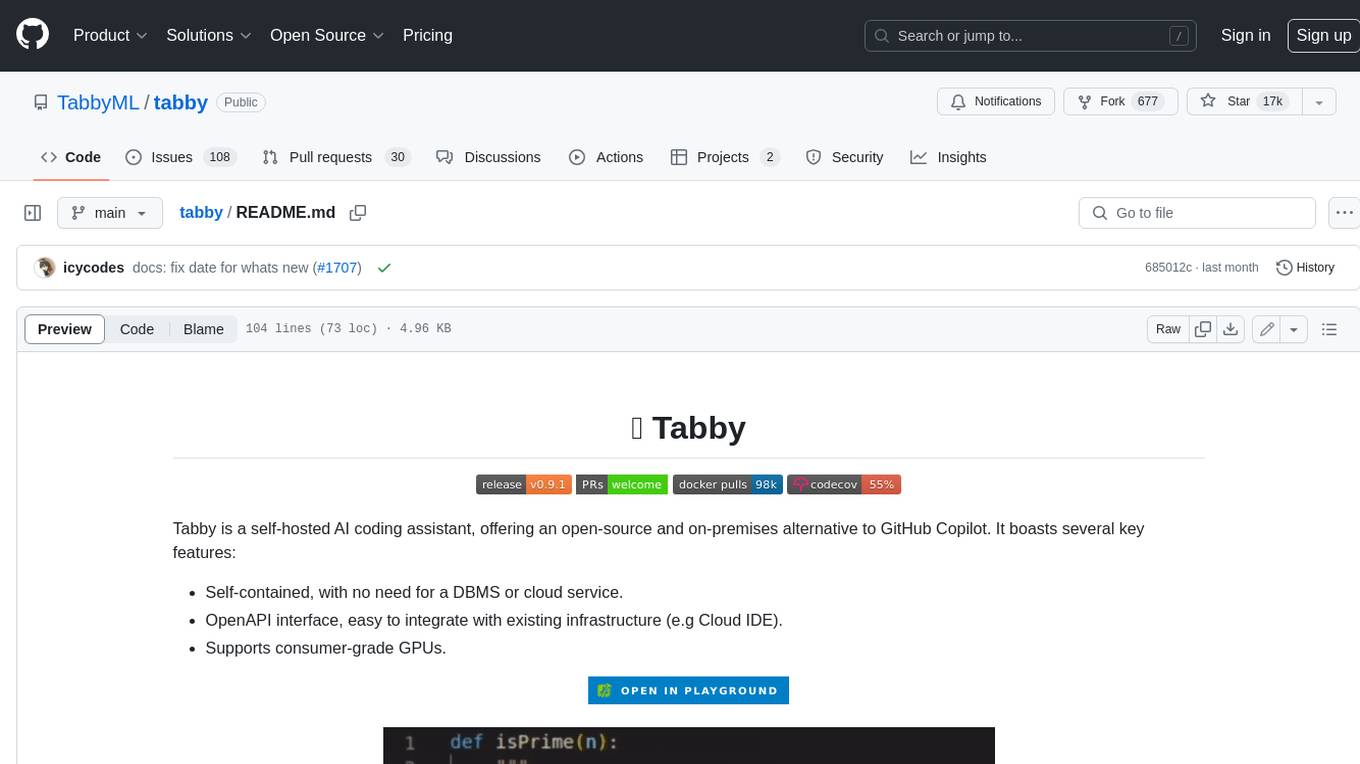
tabby
Tabby is a self-hosted AI coding assistant, offering an open-source and on-premises alternative to GitHub Copilot. It boasts several key features: * Self-contained, with no need for a DBMS or cloud service. * OpenAPI interface, easy to integrate with existing infrastructure (e.g Cloud IDE). * Supports consumer-grade GPUs.
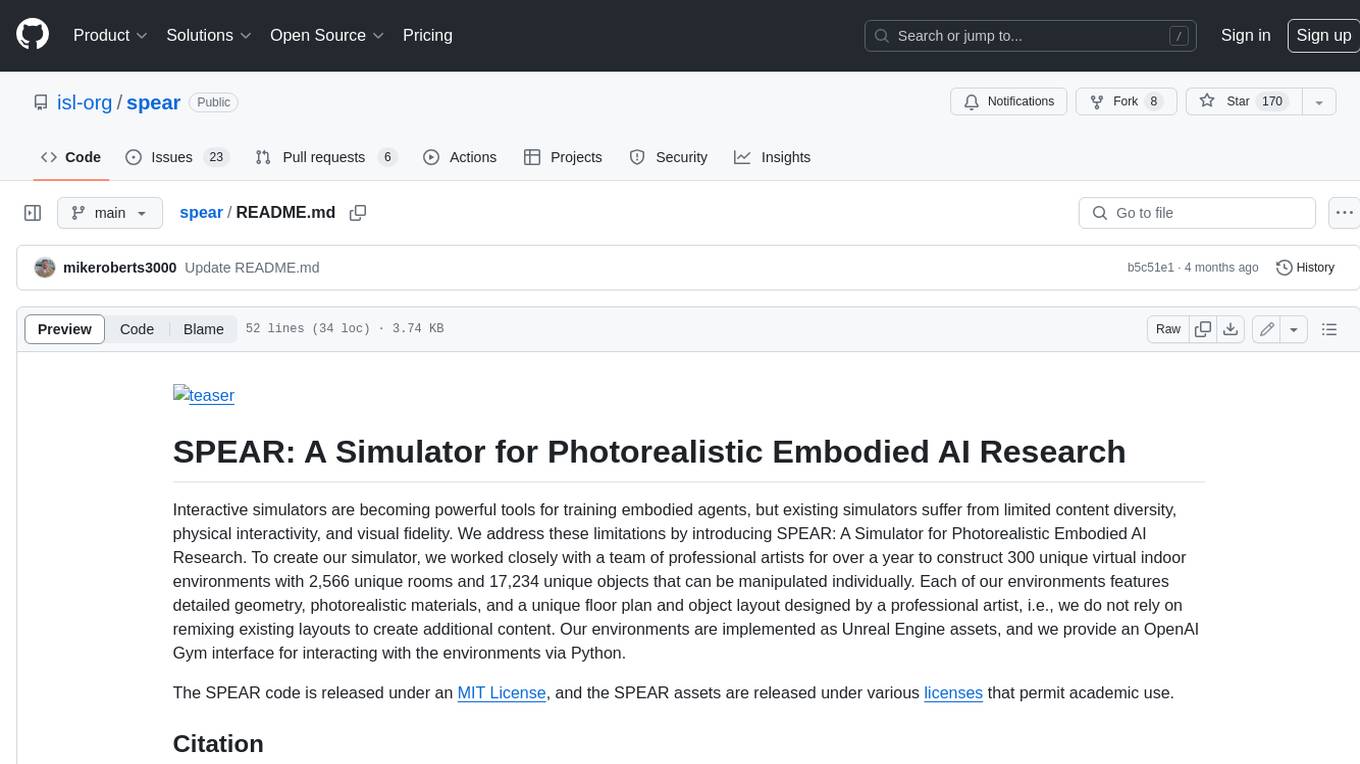
spear
SPEAR (Simulator for Photorealistic Embodied AI Research) is a powerful tool for training embodied agents. It features 300 unique virtual indoor environments with 2,566 unique rooms and 17,234 unique objects that can be manipulated individually. Each environment is designed by a professional artist and features detailed geometry, photorealistic materials, and a unique floor plan and object layout. SPEAR is implemented as Unreal Engine assets and provides an OpenAI Gym interface for interacting with the environments via Python.
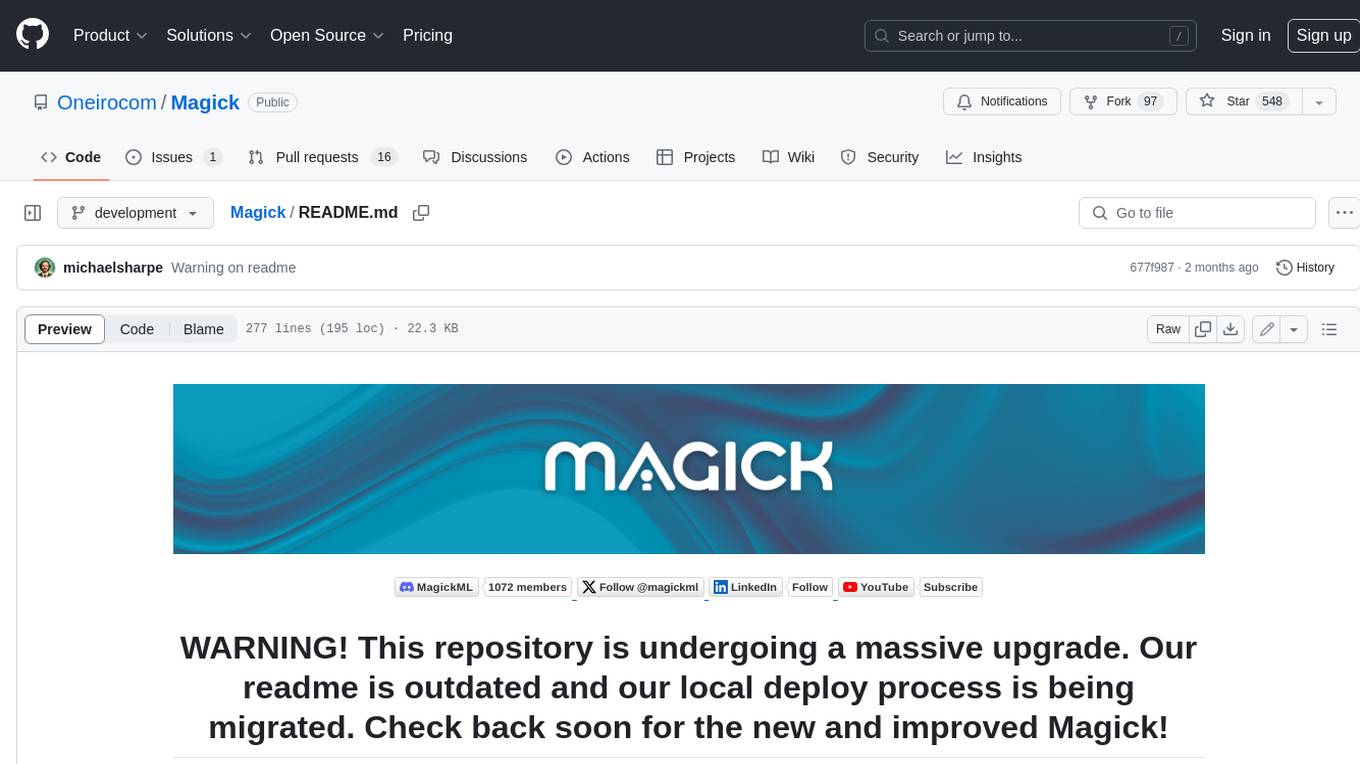
Magick
Magick is a groundbreaking visual AIDE (Artificial Intelligence Development Environment) for no-code data pipelines and multimodal agents. Magick can connect to other services and comes with nodes and templates well-suited for intelligent agents, chatbots, complex reasoning systems and realistic characters.