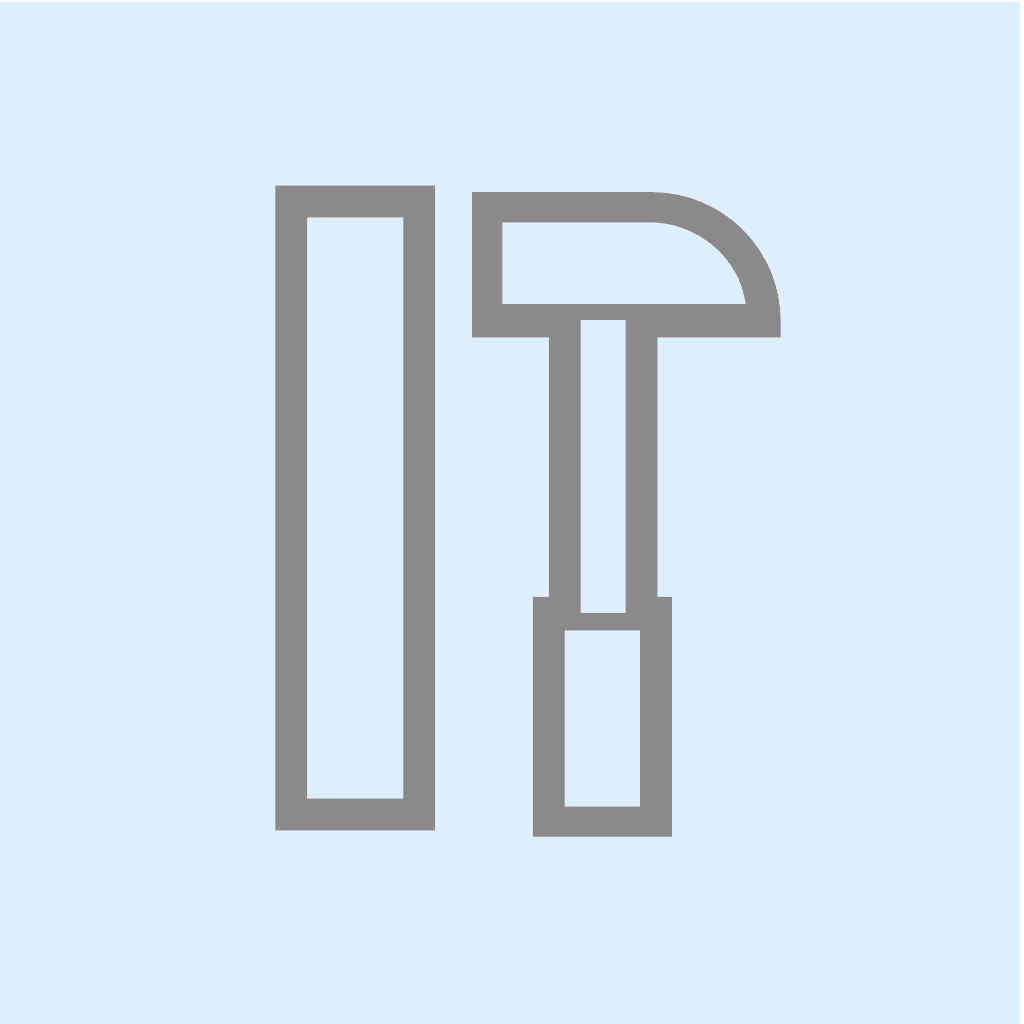
ENOVA
A deployment, monitoring and autoscaling service towards serverless LLM serving.
Stars: 124
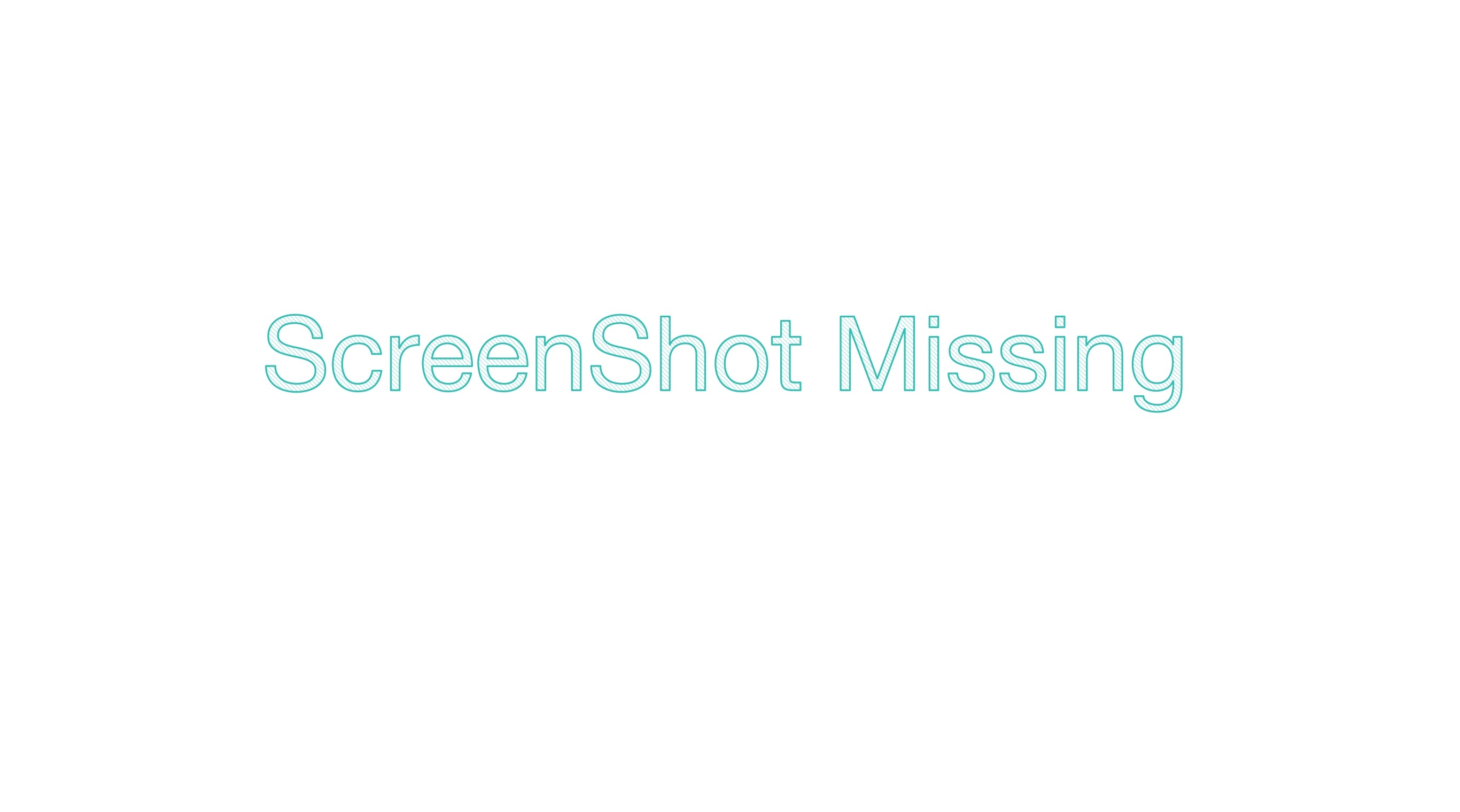
ENOVA is an open-source service for Large Language Model (LLM) deployment, monitoring, injection, and auto-scaling. It addresses challenges in deploying stable serverless LLM services on GPU clusters with auto-scaling by deconstructing the LLM service execution process and providing configuration recommendations and performance detection. Users can build and deploy LLM with few command lines, recommend optimal computing resources, experience LLM performance, observe operating status, achieve load balancing, and more. ENOVA ensures stable operation, cost-effectiveness, efficiency, and strong scalability of LLM services.
README:
ENOVA is an open-source service for LLM deployment, monitoring, injection, and auto-scaling. With the increasing popularity of large language model (LLM) backend systems, deploying stable serverless LLM services on GPU clusters with auto-scaling has become essential. However, challenges arise due to the diversity and co-location of applications in GPU clusters, leading to low service quality and GPU utilization.
To address these issues, ENOVA deconstructs the LLM service execution process and incorporates a configuration recommendation module for automatic deployment on any GPU cluster and a performance detection module for auto-scaling. Additionally, ENOVA features a deployment execution engine for efficient GPU cluster scheduling.
With ENOVA, users can:
- Build and deploy LLM with only few command lines
- Recommend optimal computing resources and operating parameter configurations for LLM
- Quick experience LLM performance with ENOVA by request injection offered
- In-depth observation of LLM operating status and abnormal self-healing
- Achieve load balancing through autoscaling
Here are ENOVA's core technical points and values:
- Configuration Recommendation: ENOVA can automatically identify various LLMs (open-source or fine-tuned), and recommend the most suitable parameter configurations for deploying the model, such as GPU type, maximal batch size, replicas, weights etc.
- Performance Detection: ENOVA enables real-time monitoring of service quality and abnormal usage of computational resources.
- Deep Observability: By conducting in-depth observation of the entire chain of task execution of large models, we can provide the best guidance for maximizing model performance and optimizing the utilization of computing resources.
- Deployment & Execution: Achieve rapid deployment and model serving, aiming to achieve autoscaling objectives.
Based on the aforementioned capabilities of ENOVA, we can ensure that LLM services with ENOVA are:
- Stable: Achieve a high availability rate of over 99%, ensuring stable operation without downtime.
- Cost-effective: Increase resource utilization by over 50% and enhance comprehensive GPU memory utilization from 40% to 90%.
- Efficient: Boost deployment efficiency by over 10 times and run LLMs with lower latency and higher throughputs
- Strong Scalability: ENOVA can automatically cluster different task types, thus adapting to applications in many fields.
We can demonstrate the powerful capabilities of E NOVA in model deployment and performance monitoring by swiftly running an open-source AI model on your GPUs and conducting request injection tests.
- OS: Linux
- Docker
- Python: >=3.10
- GPU: Nvidia GPUs with compute capability 7.0 or higher
[!NOTE]
If the above conditions are not met, the installation and operation of ENOVA may fail. If you do not have available GPU resources, we recommend that you use the free GPU resources on Google Colab to install and experience ENOVA.
# Create a new Python environment
conda create -n enova_env python=3.10
conda activate enova_env
# Install ENOVA
# Source: https://pypi.python.org/simple/
pip install enova_instrumentation_llmo
pip install enova
- To verify the installation, run:
enova -h
The expected output is:
Usage: enova [OPTIONS] COMMAND [ARGS]...
███████╗███╗ ██╗ ██████╗ ██╗ ██╗ █████╗
██╔════╝████╗ ██║██╔═══██╗██║ ██║██╔══██╗
█████╗ ██╔██╗ ██║██║ ██║██║ ██║███████║
██╔══╝ ██║╚██╗██║██║ ██║╚██╗ ██╔╝██╔══██║
███████╗██║ ╚████║╚██████╔╝ ╚████╔╝ ██║ ██║
╚══════╝╚═╝ ╚═══╝ ╚═════╝ ╚═══╝ ╚═╝ ╚═╝
ENOVA is an open-source llm deployment, monitoring, injection and auto-scaling service.
It provides a set of commands to deploy stable serverless serving of LLM on GPU clusters with auto-scaling.
Options:
-v, --version Show the version and exit.
-h, --help Show this message and exit.
Commands:
algo Run the autoscaling service.
app Start ENOVA application server.
enode Deploy the target LLM and launch the LLM API service.
injector Run the autoscaling service.
mon Run the monitors of LLM server
pilot Start an all-in-one LLM server with deployment, monitoring,...
webui Build agent at this page based on the launched LLM API service.
- Start an all-in-one LLM server with deployment, monitoring, injection and auto-scaling service:
enova pilot run --model mistralai/Mistral-7B-Instruct-v0.1
# openai
enova pilot run --model mistralai/Mistral-7B-Instruct-v0.1 --vllm_mode openai
Use proxy to download LLMs:
enova pilot run --model mistralai/Mistral-7B-Instruct-v0.1 --hf_proxy xxx
[!TIP]
- The default port of LLM service is 9199.
- The default port of grafana server is 32827.
- The default port of LLM webUI server is 8501.
- The default port of ENOVA application server is 8182.
- Check Deployed LLM service via ENOVA Application Server:
http://localhost:8182/instance
- Test the Deployed LLM service with an prompt:
Use WebUI:
http://localhost:8501
Use Shell:
curl -X POST http://localhost:9199/generate \
-d '{
"prompt": "San Francisco is a",
"max_tokens": 1024,
"temperature": 0.9,
"top_p": 0.9
}'
# openai
curl http://localhost:9199/v1/completions \
-H "Content-Type: application/json" \
-d '{
"model": "mistralai/Mistral-7B-Instruct-v0.1",
"prompt": "San Francisco is a",
"max_tokens": 128,
"temperature": 0
}'
- Monitor the LLM Service Quality via ENOVA Application Server:
http://localhost:8182/instance
- Stop all service
enova pilot stop --service all
In addition to offering an all-in-one solution for service deployment, monitoring, and autoscaling, ENOVA also provides support for single modules.
The LLM deployment service facilitates the deployment of LLMs and provides a stable API for accessing LLMs.
enova enode run --model mistralai/Mistral-7B-Instruct-v0.1
[!NOTE]
The LLM server is launched with default vllm backend. OpenAI API and Generate API are both supported. vllm config can be specified using command-line parameters like:
enova enode run --model mistralai/Mistral-7B-Instruct-v0.1 --host 127.0.0.1 --port 9199
This service features a WebUI page for dialog interaction, where the serving host and port for the LLM server, and the host and port for the WebUI service, are configurable parameters.
enova webui run --serving_host 127.0.0.1 --serving_port 9199 --host 127.0.0.1 --port 8501
The autoscaling service is automatically launched and managed by escaler module.
We implemented a request injection module using JMeter to simulate real user requests for evaluating LLM performance. The module allows simulation of request arrival probabilities using two modes: Poisson distribution and normal distribution. Further details on the injection operation are available at:
http://localhost:8182/instance
The monitoring system is designed for monitoring and autoscaling, which contains real-time data collection, storage, and consumption. We can manage the LLM monitoring service via:
- Start llm monitoring service
enova mon start
- Check service status
enova mon status
- Stop monitoring service
enova mon stop
Monitoring metrics are collected using the DCGM exporter, Prometheus exporters, and the OpenTelemetry collector. A brief description is provided in the following tables. For more details, please refer to the Grafana dashboard.
Metric Type | Metric Description |
---|---|
API Service | The number of requests sent to LLM services per second |
API Service | The number of requests processed by LLM services per second |
API Service | The number of requests successfully processed per second |
API Service | The success rate of requests processed by LLM services per second |
API Service | The number of requests being processed by LLM services |
API Service | The average execution time per request processed by LLM services |
API Service | The average request size of requests per second |
API Service | The average response size of requests per second |
LLM Performance | The average prompt throughput per second |
LLM Performance | The average generation throughput per second |
LLM Performance | The number of requests being processed by the deployed LLM |
LLM Performance | The number of requests being pended by the deployed LLM |
LLM Performance | The utilization ratio of memory allocated for KV cache |
GPU Utilization | DCGM Metrics, like DCGM_FI_DEV_GPU_UTIL. |
More detailed metrics can be viewed on our application server. In the process of deploying the all-in-one llm service with ENOVA, we also created the corresponding indicator dashboard in Grafana and supported viewing of more detailed trace data.
- URL:http://localhost:32827/dashboards
- Default user account:admin
- Password:grafana
@inproceedings{tao2024ENOVA,
title={ENOVA: Autoscaling towards Cost-effective and Stable Serverless LLM Serving},
author={Tao Huang and Pengfei Chen and Kyoka Gong and Jocky Hawk and Zachary Bright and Wenxin Xie and Kecheng Huang and Zhi Ji},
booktitle={arXiv preprint arXiv:},
year={2024}
}
We use Slack workspace for the collaboration on building ENOVA.
- Slack workspace
- Browse our website for more informations
For Tasks:
Click tags to check more tools for each tasksFor Jobs:
Alternative AI tools for ENOVA
Similar Open Source Tools
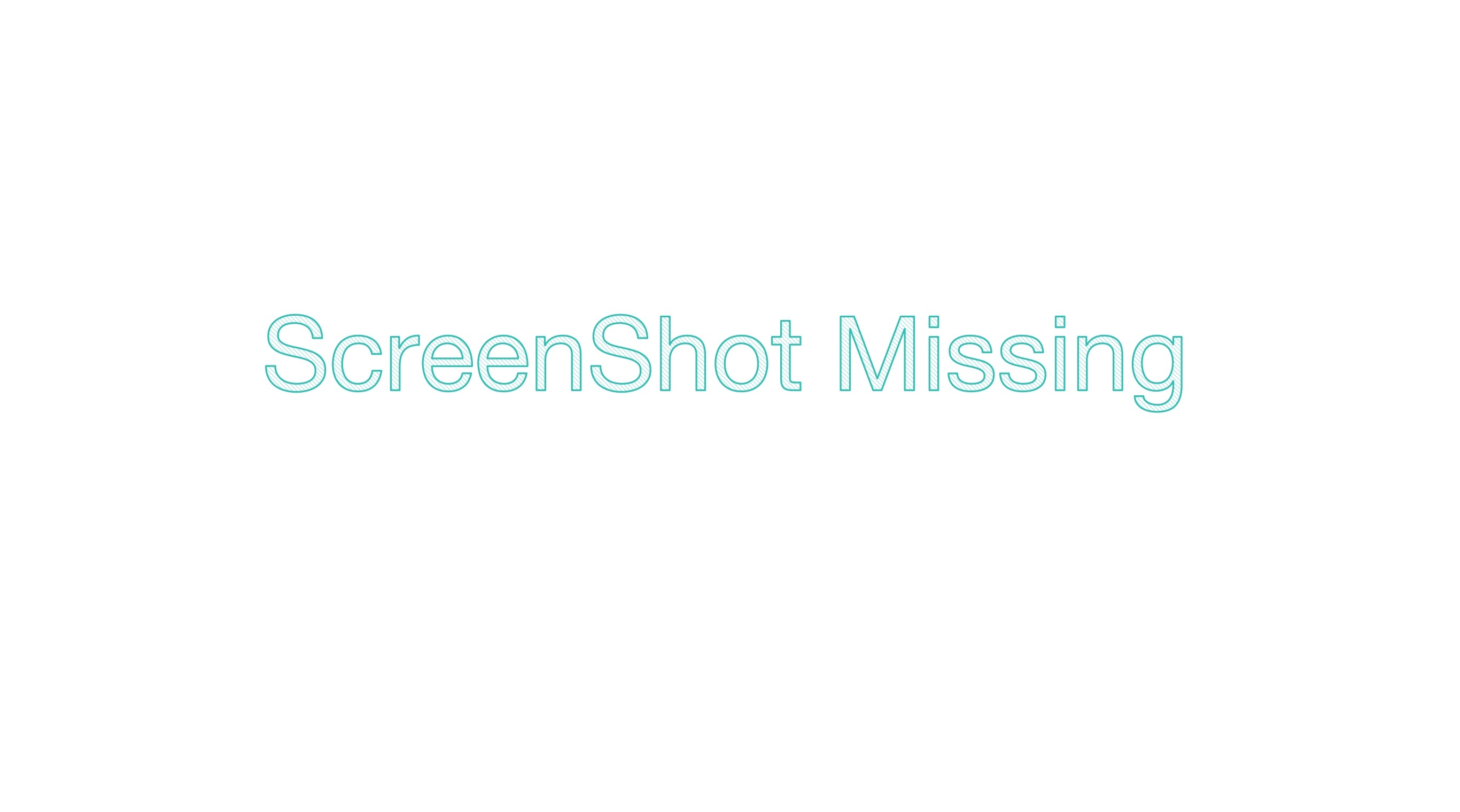
ENOVA
ENOVA is an open-source service for Large Language Model (LLM) deployment, monitoring, injection, and auto-scaling. It addresses challenges in deploying stable serverless LLM services on GPU clusters with auto-scaling by deconstructing the LLM service execution process and providing configuration recommendations and performance detection. Users can build and deploy LLM with few command lines, recommend optimal computing resources, experience LLM performance, observe operating status, achieve load balancing, and more. ENOVA ensures stable operation, cost-effectiveness, efficiency, and strong scalability of LLM services.
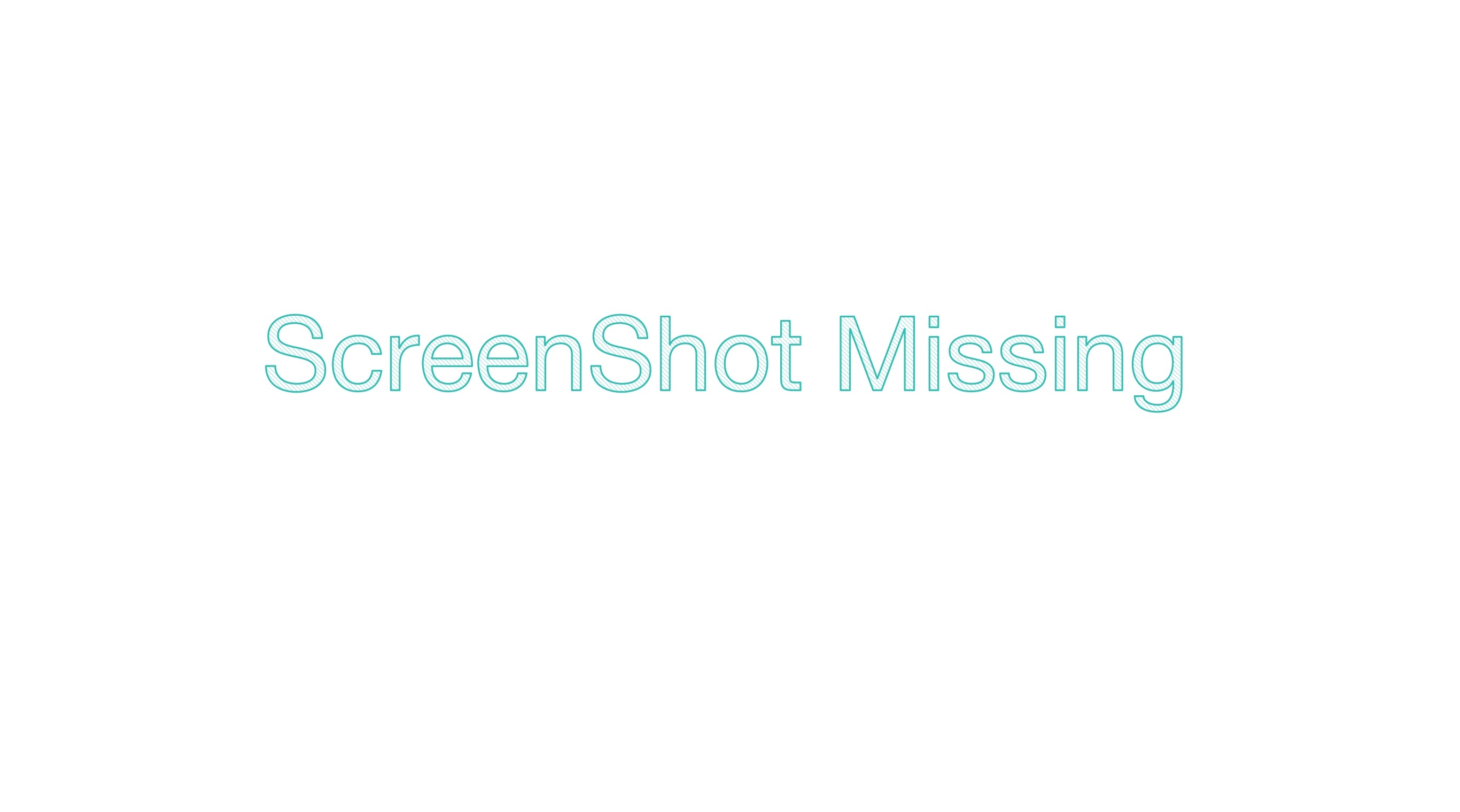
nous
Nous is an open-source TypeScript platform for autonomous AI agents and LLM based workflows. It aims to automate processes, support requests, review code, assist with refactorings, and more. The platform supports various integrations, multiple LLMs/services, CLI and web interface, human-in-the-loop interactions, flexible deployment options, observability with OpenTelemetry tracing, and specific agents for code editing, software engineering, and code review. It offers advanced features like reasoning/planning, memory and function call history, hierarchical task decomposition, and control-loop function calling options. Nous is designed to be a flexible platform for the TypeScript community to expand and support different use cases and integrations.
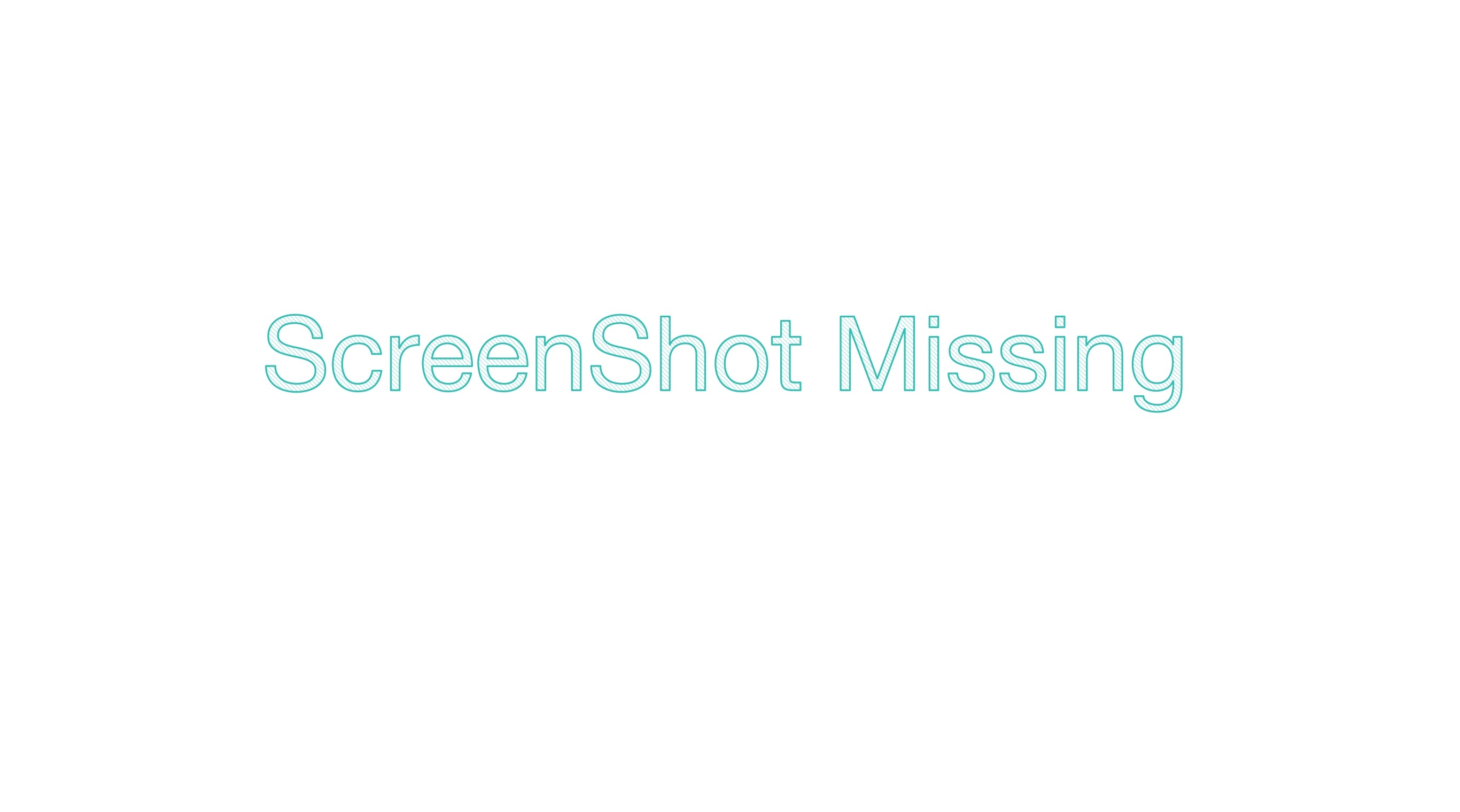
vertex-ai-mlops
Vertex AI is a platform for end-to-end model development. It consist of core components that make the processes of MLOps possible for design patterns of all types.
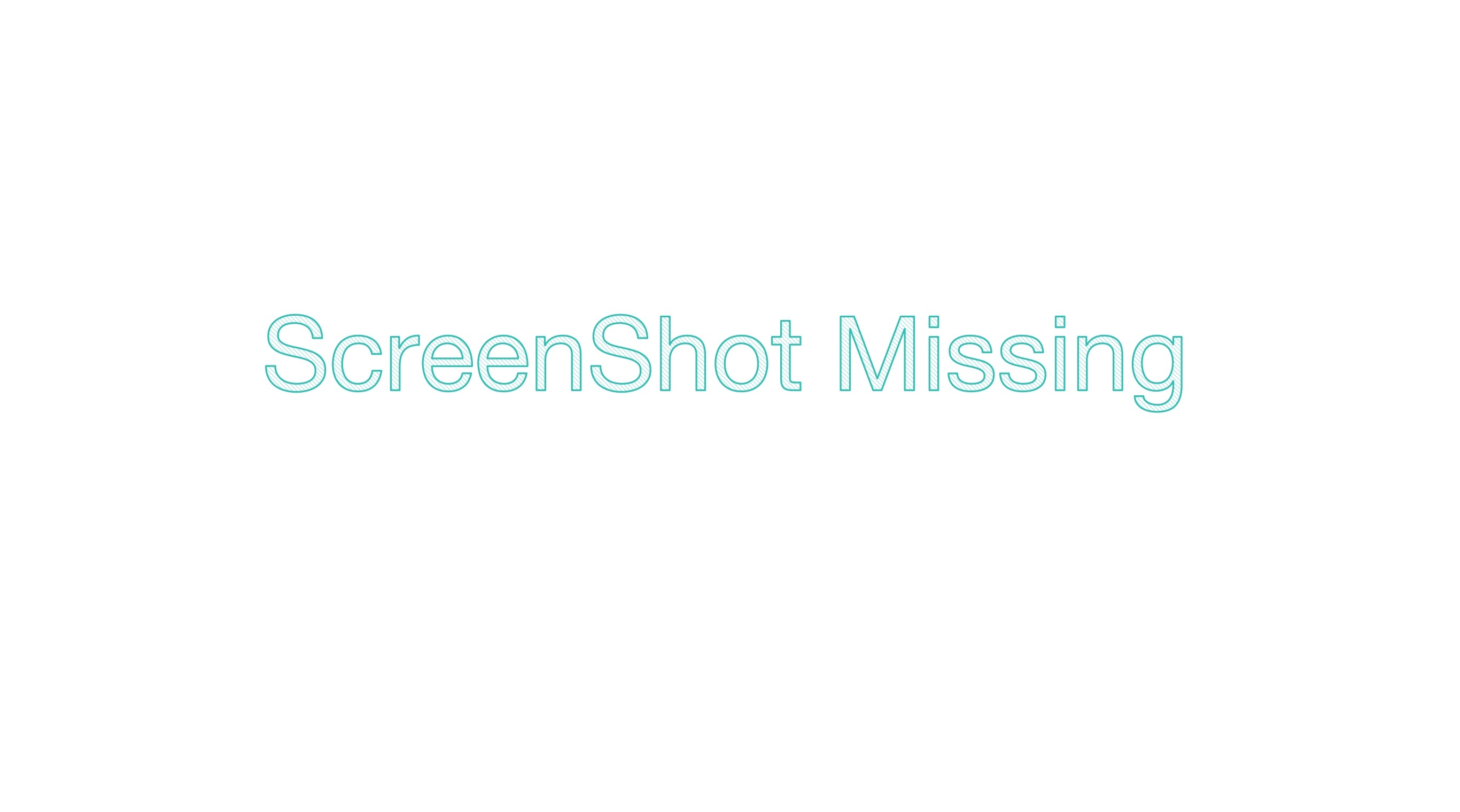
Reflection_Tuning
Reflection-Tuning is a project focused on improving the quality of instruction-tuning data through a reflection-based method. It introduces Selective Reflection-Tuning, where the student model can decide whether to accept the improvements made by the teacher model. The project aims to generate high-quality instruction-response pairs by defining specific criteria for the oracle model to follow and respond to. It also evaluates the efficacy and relevance of instruction-response pairs using the r-IFD metric. The project provides code for reflection and selection processes, along with data and model weights for both V1 and V2 methods.
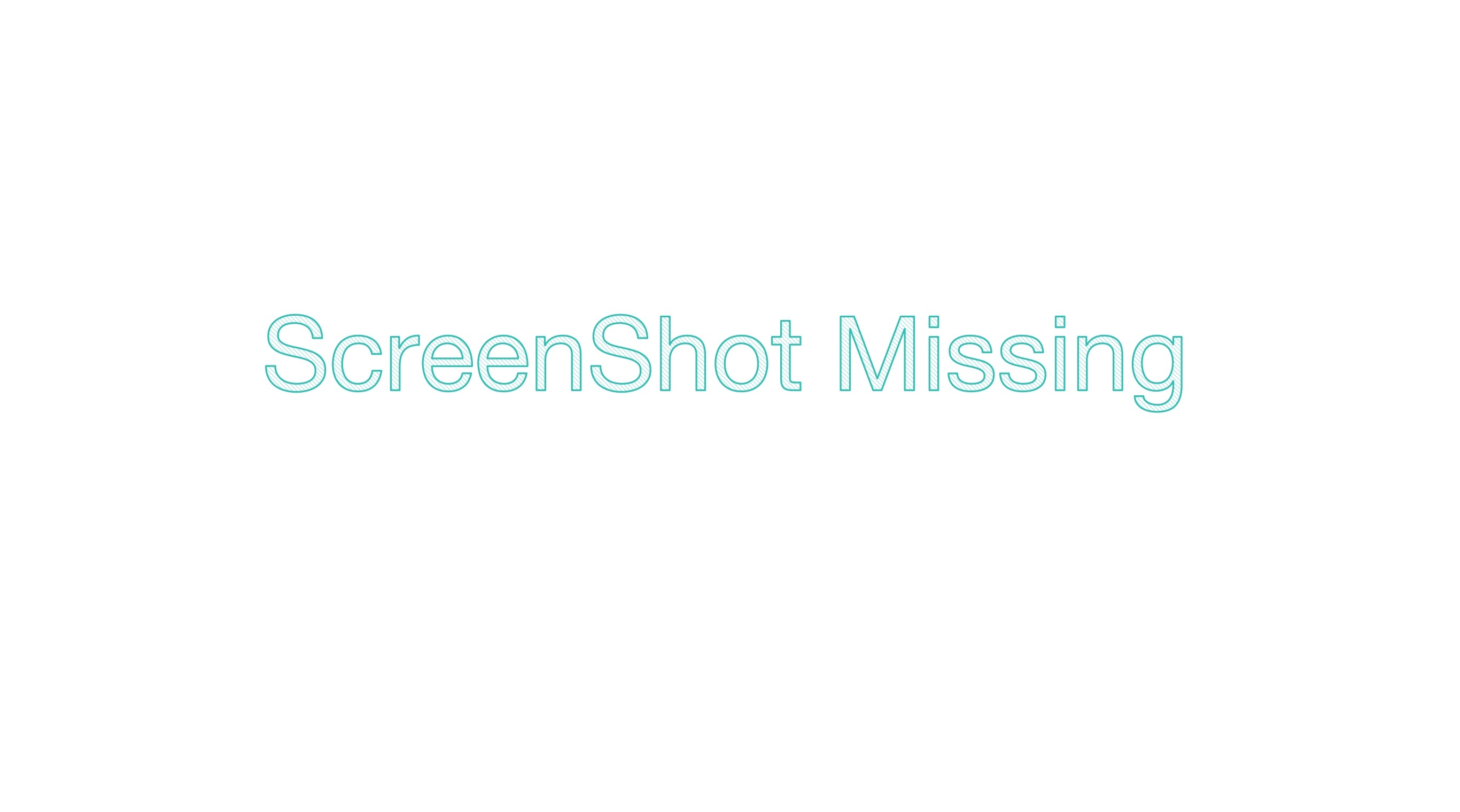
NeMo-Curator
NeMo Curator is a GPU-accelerated open-source framework designed for efficient large language model data curation. It provides scalable dataset preparation for tasks like foundation model pretraining, domain-adaptive pretraining, supervised fine-tuning, and parameter-efficient fine-tuning. The library leverages GPUs with Dask and RAPIDS to accelerate data curation, offering customizable and modular interfaces for pipeline expansion and model convergence. Key features include data download, text extraction, quality filtering, deduplication, downstream-task decontamination, distributed data classification, and PII redaction. NeMo Curator is suitable for curating high-quality datasets for large language model training.
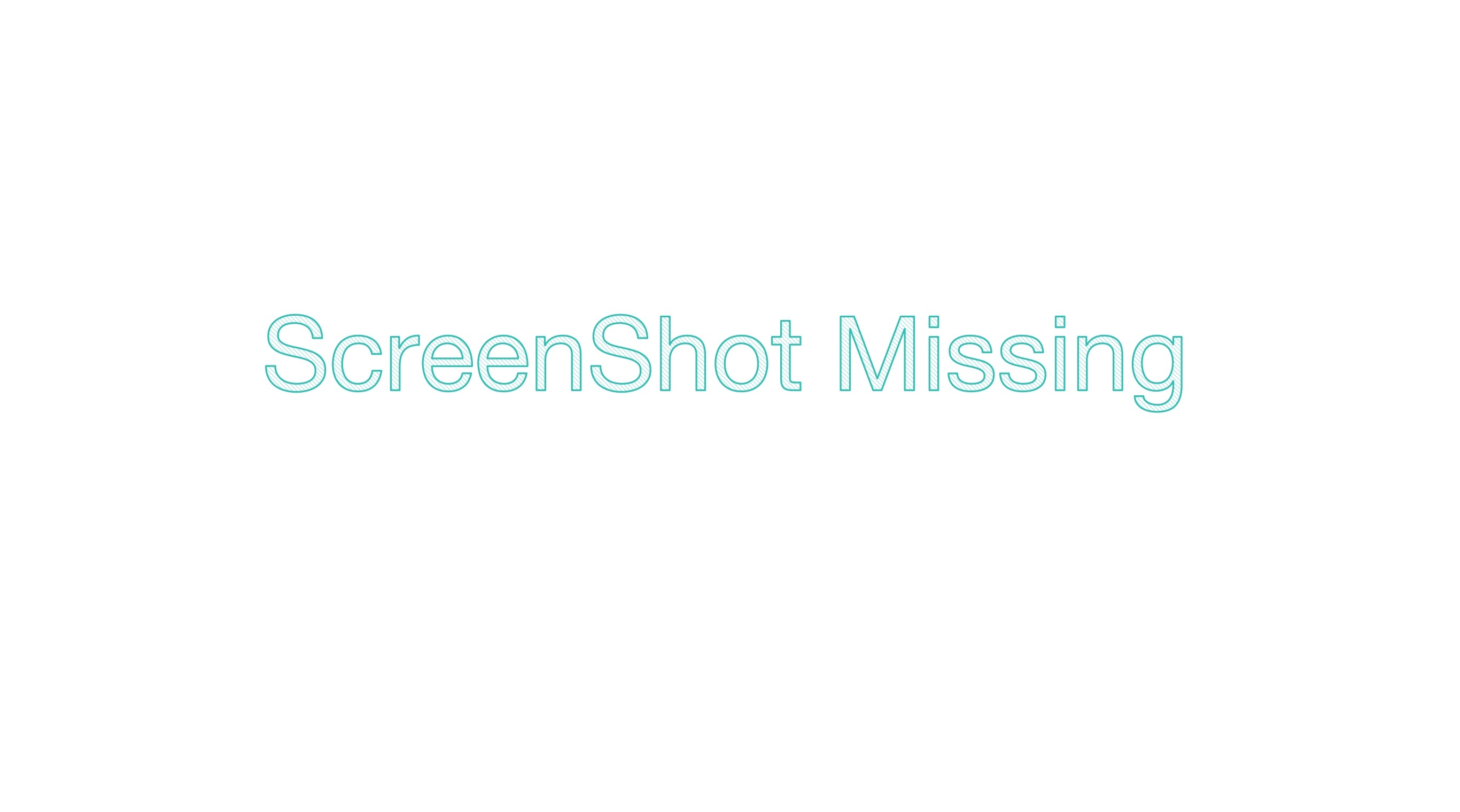
evalverse
Evalverse is an open-source project designed to support Large Language Model (LLM) evaluation needs. It provides a standardized and user-friendly solution for processing and managing LLM evaluations, catering to AI research engineers and scientists. Evalverse supports various evaluation methods, insightful reports, and no-code evaluation processes. Users can access unified evaluation with submodules, request evaluations without code via Slack bot, and obtain comprehensive reports with scores, rankings, and visuals. The tool allows for easy comparison of scores across different models and swift addition of new evaluation tools.
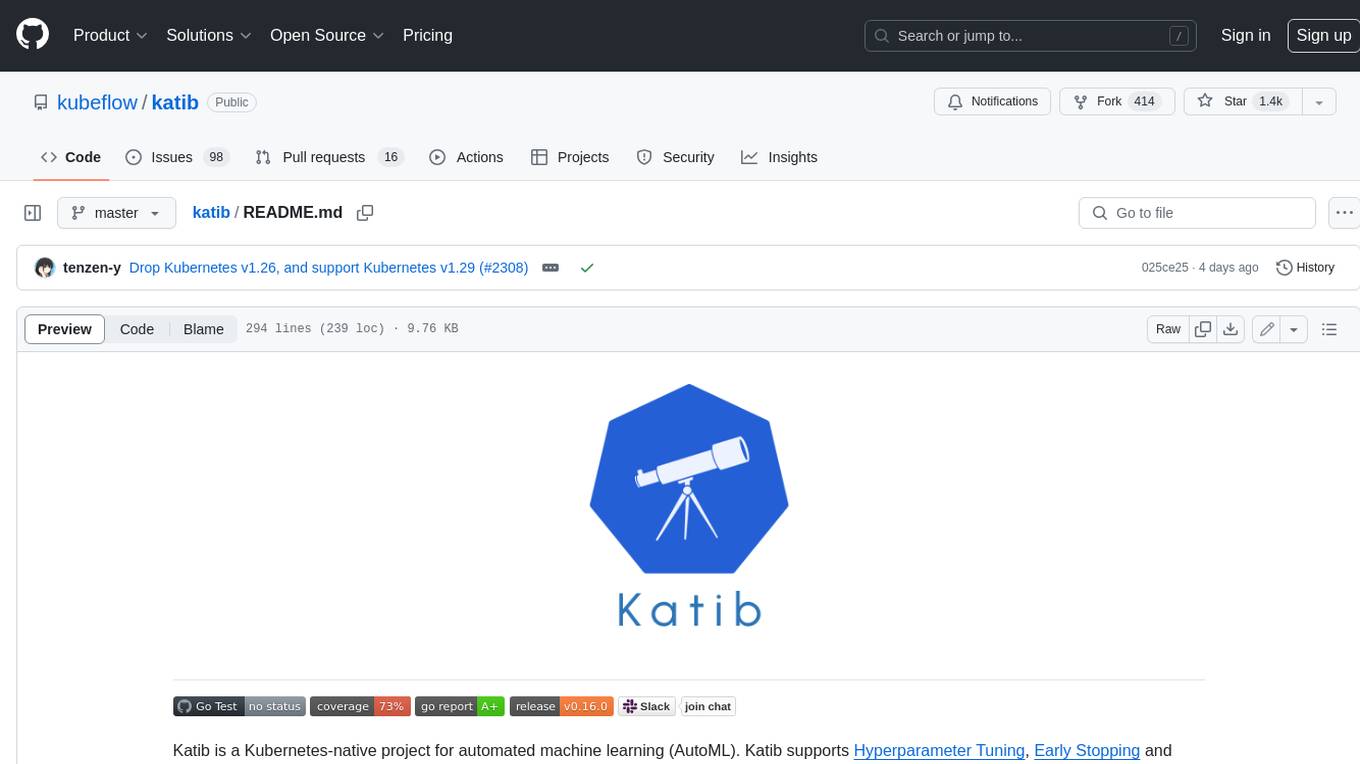
katib
Katib is a Kubernetes-native project for automated machine learning (AutoML). Katib supports Hyperparameter Tuning, Early Stopping and Neural Architecture Search. Katib is the project which is agnostic to machine learning (ML) frameworks. It can tune hyperparameters of applications written in any language of the users’ choice and natively supports many ML frameworks, such as TensorFlow, Apache MXNet, PyTorch, XGBoost, and others. Katib can perform training jobs using any Kubernetes Custom Resources with out of the box support for Kubeflow Training Operator, Argo Workflows, Tekton Pipelines and many more.
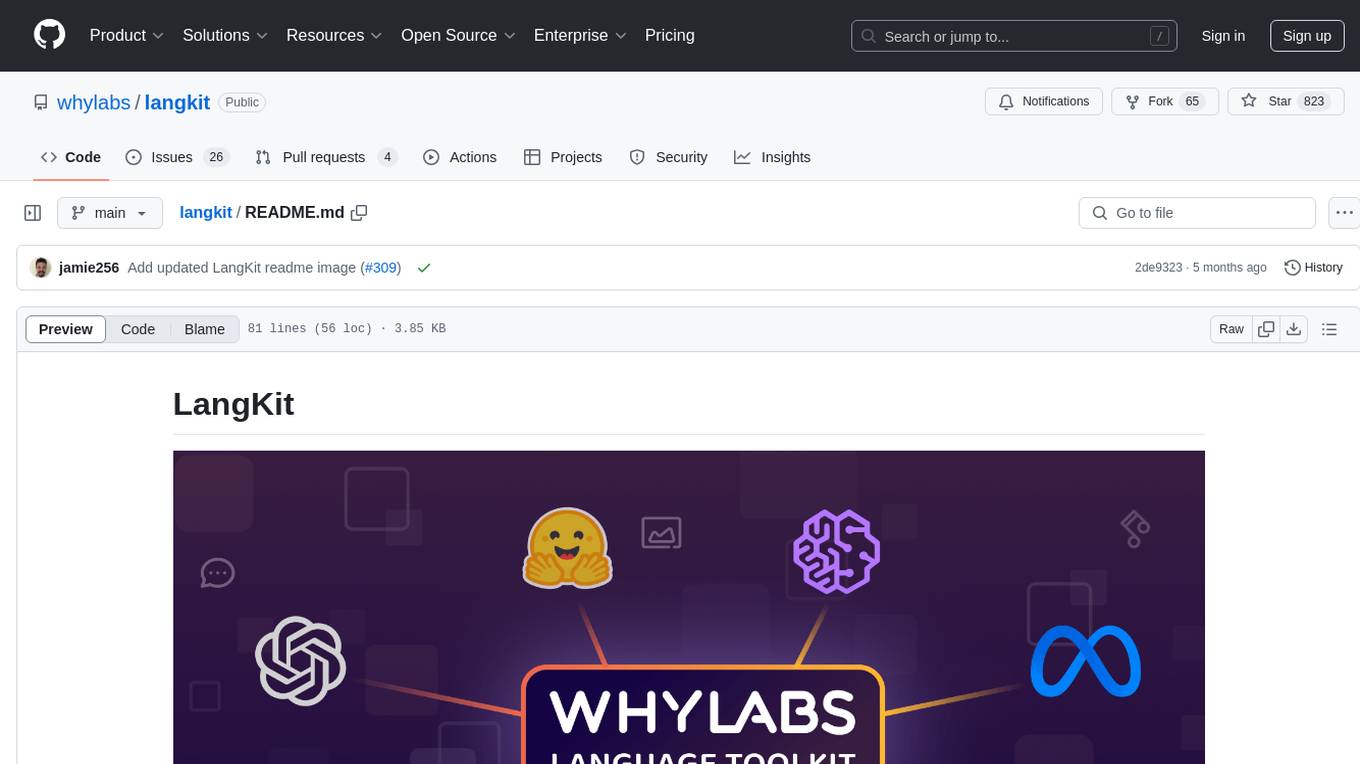
langkit
LangKit is an open-source text metrics toolkit for monitoring language models. It offers methods for extracting signals from input/output text, compatible with whylogs. Features include text quality, relevance, security, sentiment, toxicity analysis. Installation via PyPI. Modules contain UDFs for whylogs. Benchmarks show throughput on AWS instances. FAQs available.
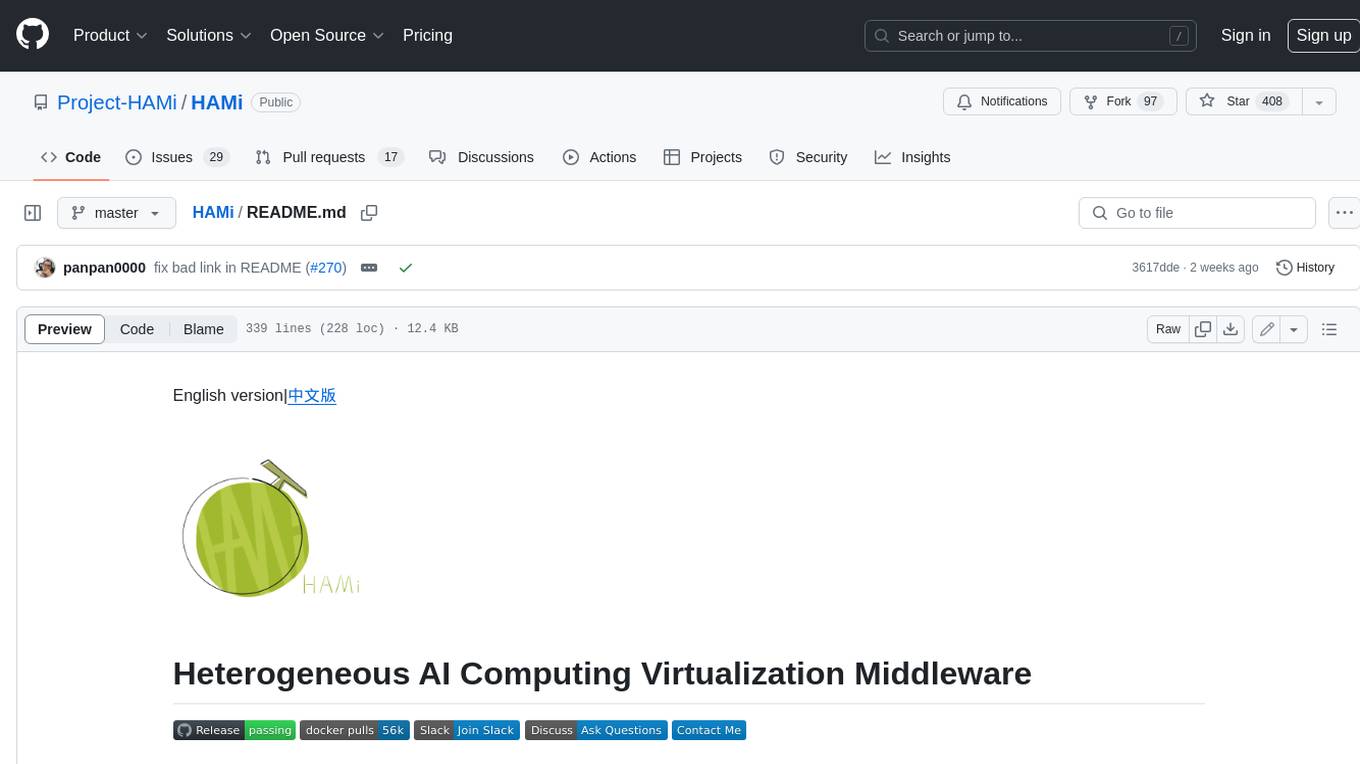
HAMi
HAMi is a Heterogeneous AI Computing Virtualization Middleware designed to manage Heterogeneous AI Computing Devices in a Kubernetes cluster. It allows for device sharing, device memory control, device type specification, and device UUID specification. The tool is easy to use and does not require modifying task YAML files. It includes features like hard limits on device memory, partial device allocation, streaming multiprocessor limits, and core usage specification. HAMi consists of components like a mutating webhook, scheduler extender, device plugins, and in-container virtualization techniques. It is suitable for scenarios requiring device sharing, specific device memory allocation, GPU balancing, low utilization optimization, and scenarios needing multiple small GPUs. The tool requires prerequisites like NVIDIA drivers, CUDA version, nvidia-docker, Kubernetes version, glibc version, and helm. Users can install, upgrade, and uninstall HAMi, submit tasks, and monitor cluster information. The tool's roadmap includes supporting additional AI computing devices, video codec processing, and Multi-Instance GPUs (MIG).
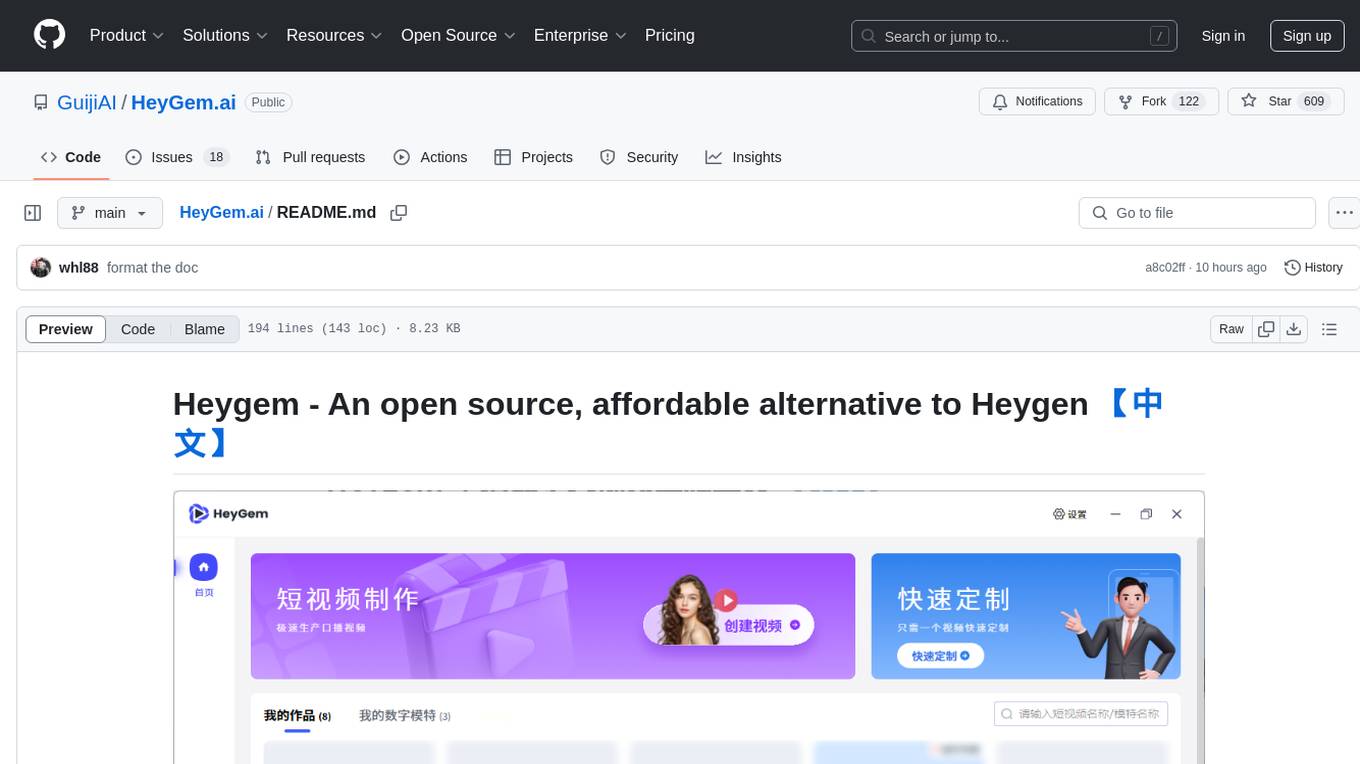
HeyGem.ai
Heygem is an open-source, affordable alternative to Heygen, offering a fully offline video synthesis tool for Windows systems. It enables precise appearance and voice cloning, allowing users to digitalize their image and drive virtual avatars through text and voice for video production. With core features like efficient video synthesis and multi-language support, Heygem ensures a user-friendly experience with fully offline operation and support for multiple models. The tool leverages advanced AI algorithms for voice cloning, automatic speech recognition, and computer vision technology to enhance the virtual avatar's performance and synchronization.
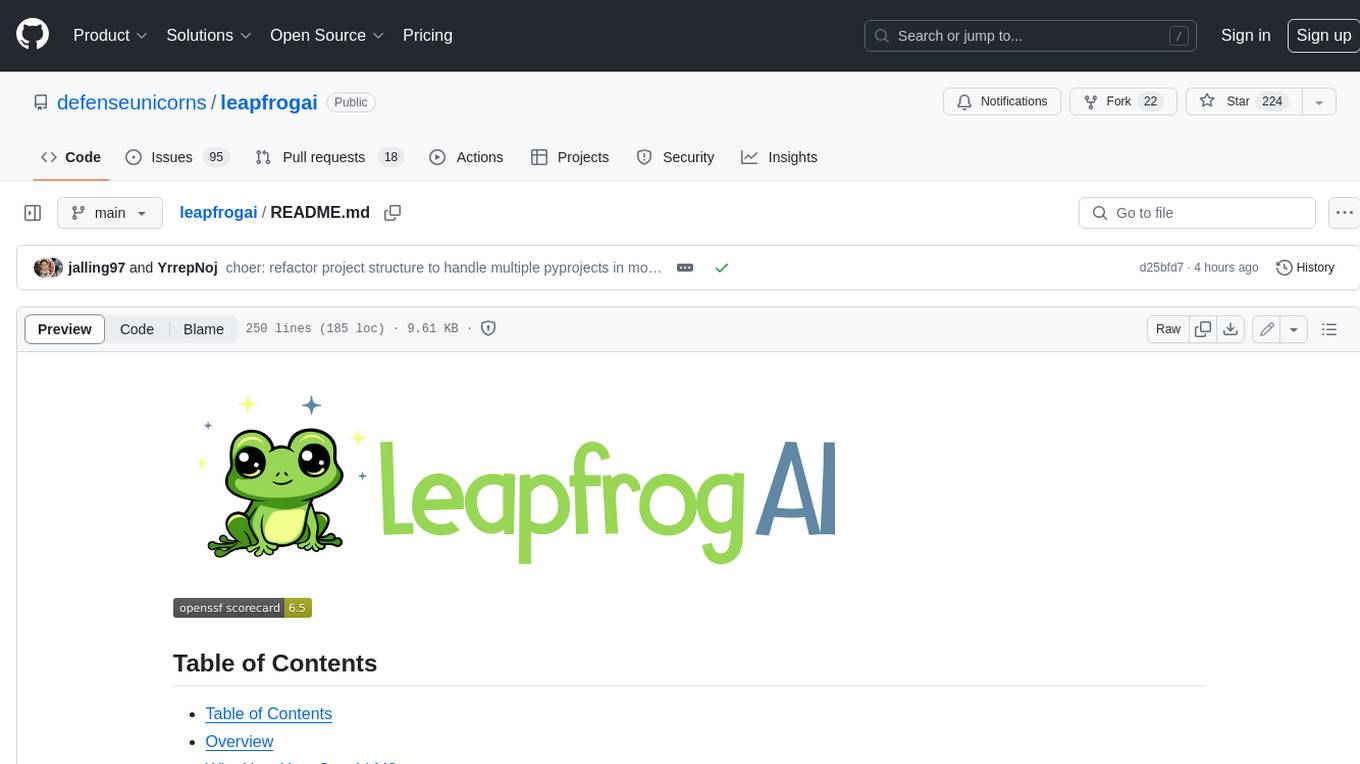
leapfrogai
LeapfrogAI is a self-hosted AI platform designed to be deployed in air-gapped resource-constrained environments. It brings sophisticated AI solutions to these environments by hosting all the necessary components of an AI stack, including vector databases, model backends, API, and UI. LeapfrogAI's API closely matches that of OpenAI, allowing tools built for OpenAI/ChatGPT to function seamlessly with a LeapfrogAI backend. It provides several backends for various use cases, including llama-cpp-python, whisper, text-embeddings, and vllm. LeapfrogAI leverages Chainguard's apko to harden base python images, ensuring the latest supported Python versions are used by the other components of the stack. The LeapfrogAI SDK provides a standard set of protobuffs and python utilities for implementing backends and gRPC. LeapfrogAI offers UI options for common use-cases like chat, summarization, and transcription. It can be deployed and run locally via UDS and Kubernetes, built out using Zarf packages. LeapfrogAI is supported by a community of users and contributors, including Defense Unicorns, Beast Code, Chainguard, Exovera, Hypergiant, Pulze, SOSi, United States Navy, United States Air Force, and United States Space Force.
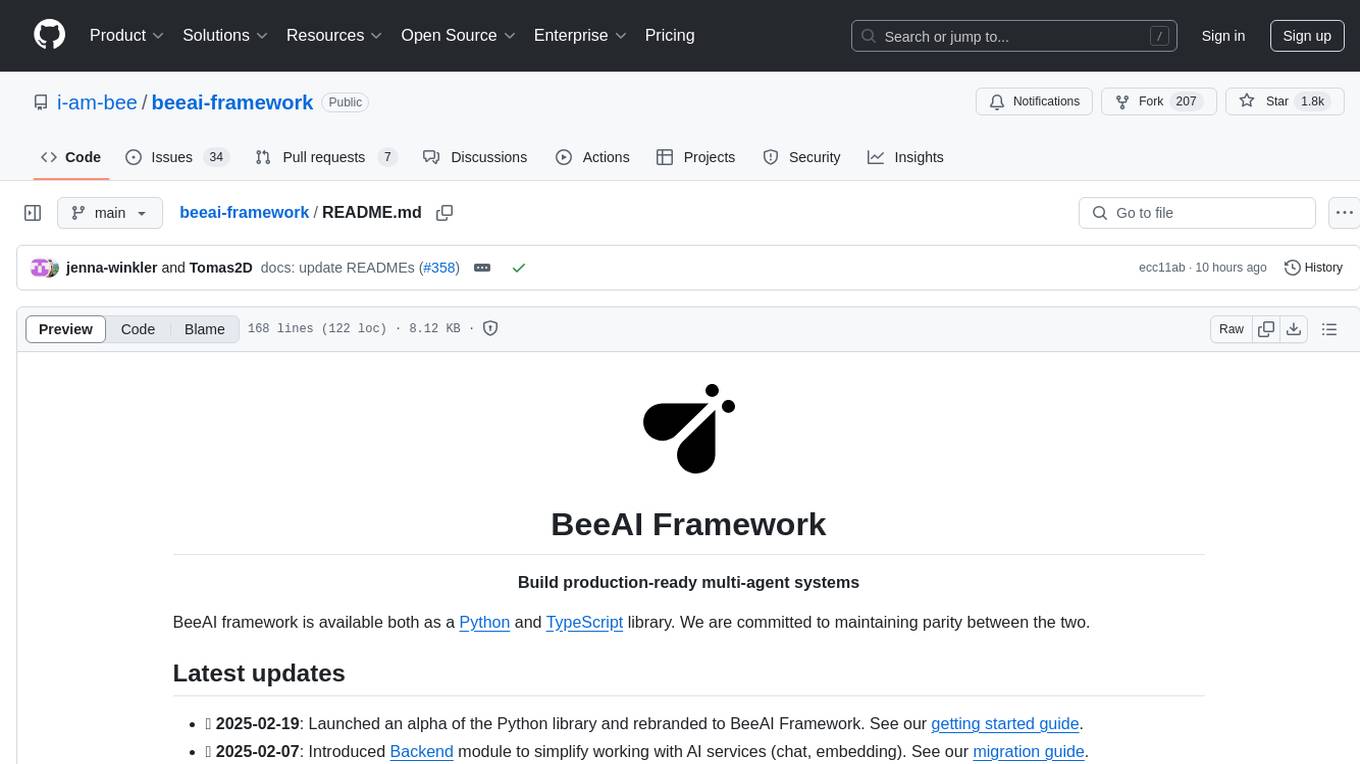
beeai-framework
BeeAI Framework is a versatile tool for building production-ready multi-agent systems. It offers flexibility in orchestrating agents, seamless integration with various models and tools, and production-grade controls for scaling. The framework supports Python and TypeScript libraries, enabling users to implement simple to complex multi-agent patterns, connect with AI services, and optimize token usage and resource management.
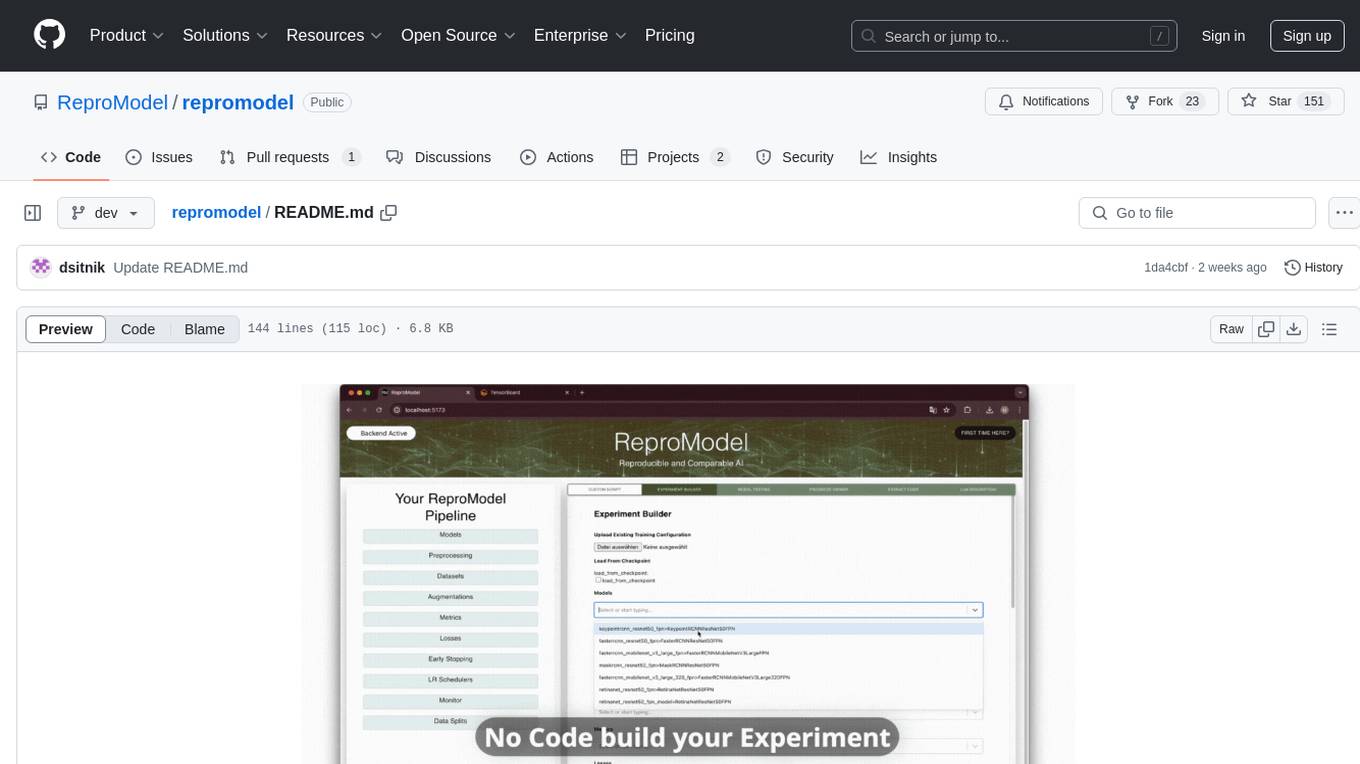
repromodel
ReproModel is an open-source toolbox designed to boost AI research efficiency by enabling researchers to reproduce, compare, train, and test AI models faster. It provides standardized models, dataloaders, and processing procedures, allowing researchers to focus on new datasets and model development. With a no-code solution, users can access benchmark and SOTA models and datasets, utilize training visualizations, extract code for publication, and leverage an LLM-powered automated methodology description writer. The toolbox helps researchers modularize development, compare pipeline performance reproducibly, and reduce time for model development, computation, and writing. Future versions aim to facilitate building upon state-of-the-art research by loading previously published study IDs with verified code, experiments, and results stored in the system.
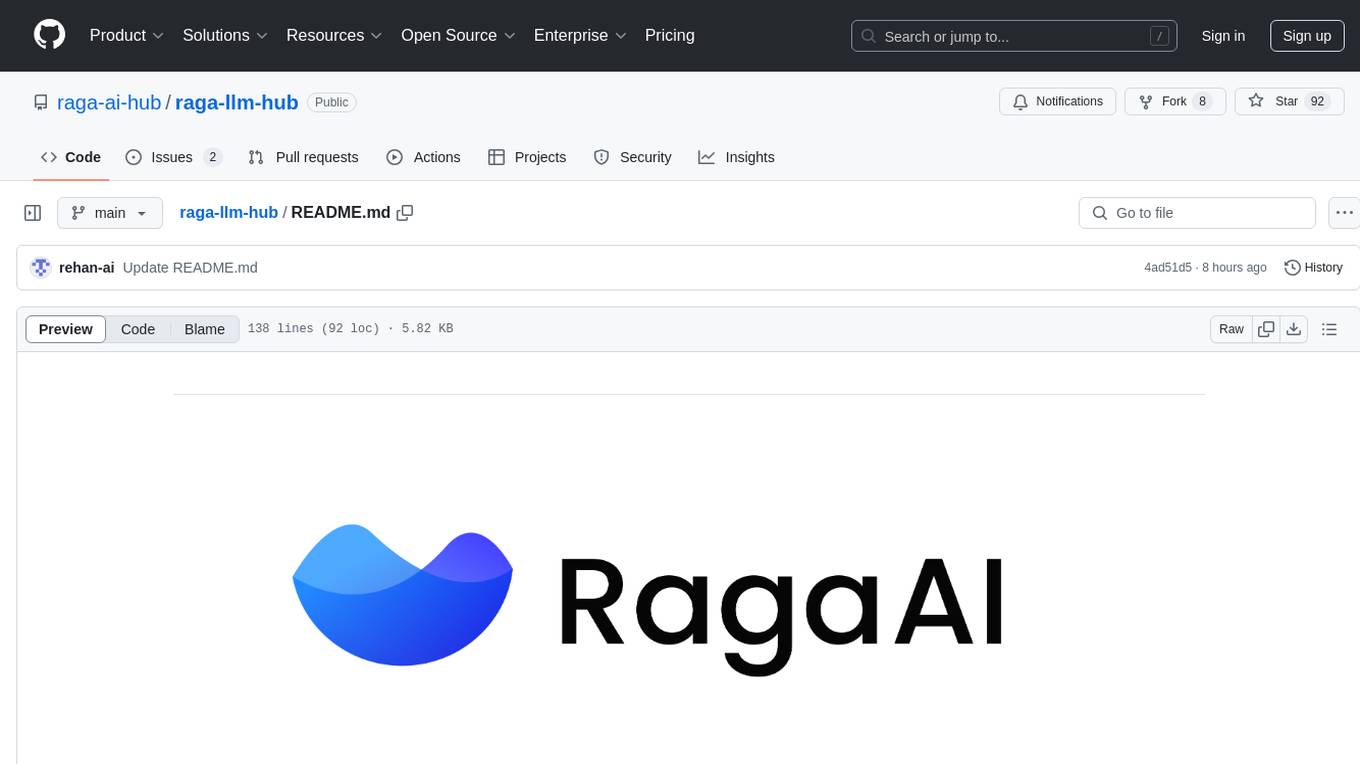
raga-llm-hub
Raga LLM Hub is a comprehensive evaluation toolkit for Language and Learning Models (LLMs) with over 100 meticulously designed metrics. It allows developers and organizations to evaluate and compare LLMs effectively, establishing guardrails for LLMs and Retrieval Augmented Generation (RAG) applications. The platform assesses aspects like Relevance & Understanding, Content Quality, Hallucination, Safety & Bias, Context Relevance, Guardrails, and Vulnerability scanning, along with Metric-Based Tests for quantitative analysis. It helps teams identify and fix issues throughout the LLM lifecycle, revolutionizing reliability and trustworthiness.
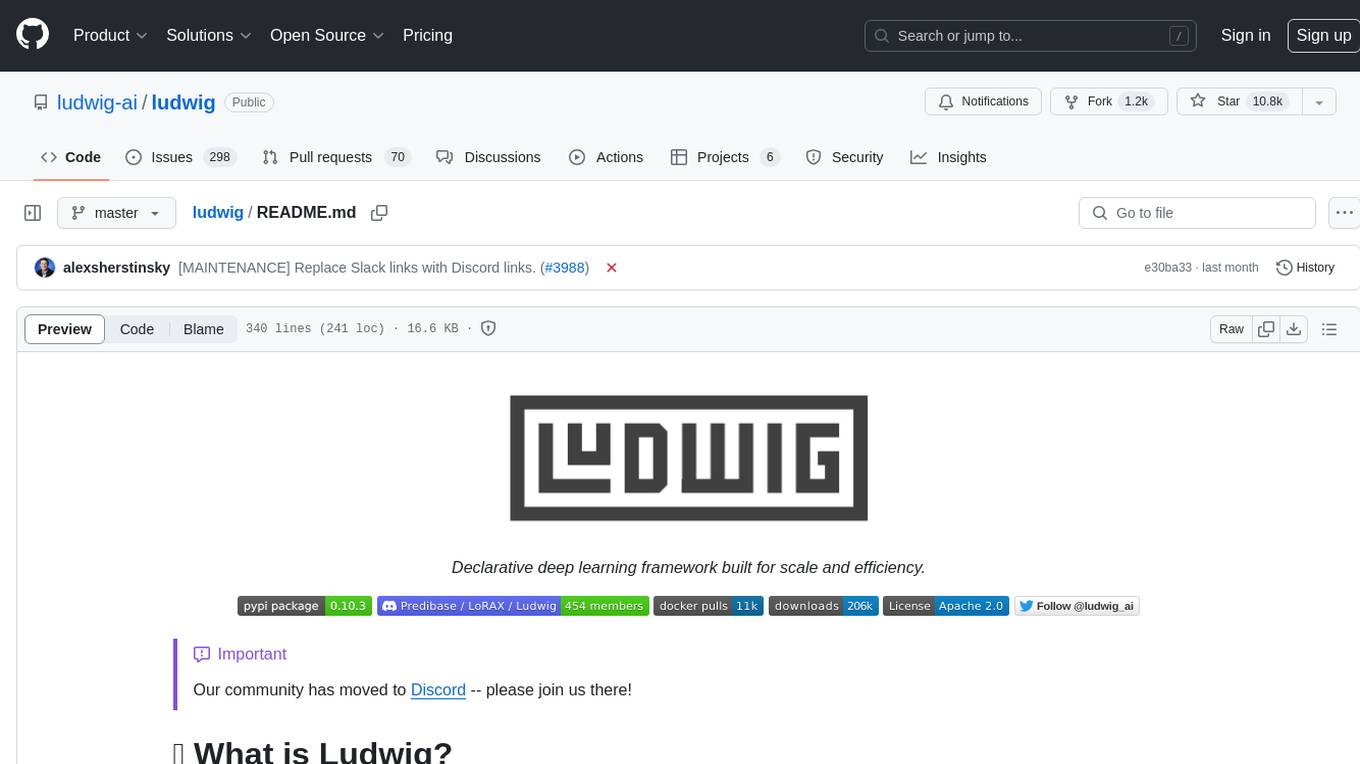
ludwig
Ludwig is a declarative deep learning framework designed for scale and efficiency. It is a low-code framework that allows users to build custom AI models like LLMs and other deep neural networks with ease. Ludwig offers features such as optimized scale and efficiency, expert level control, modularity, and extensibility. It is engineered for production with prebuilt Docker containers, support for running with Ray on Kubernetes, and the ability to export models to Torchscript and Triton. Ludwig is hosted by the Linux Foundation AI & Data.
For similar tasks
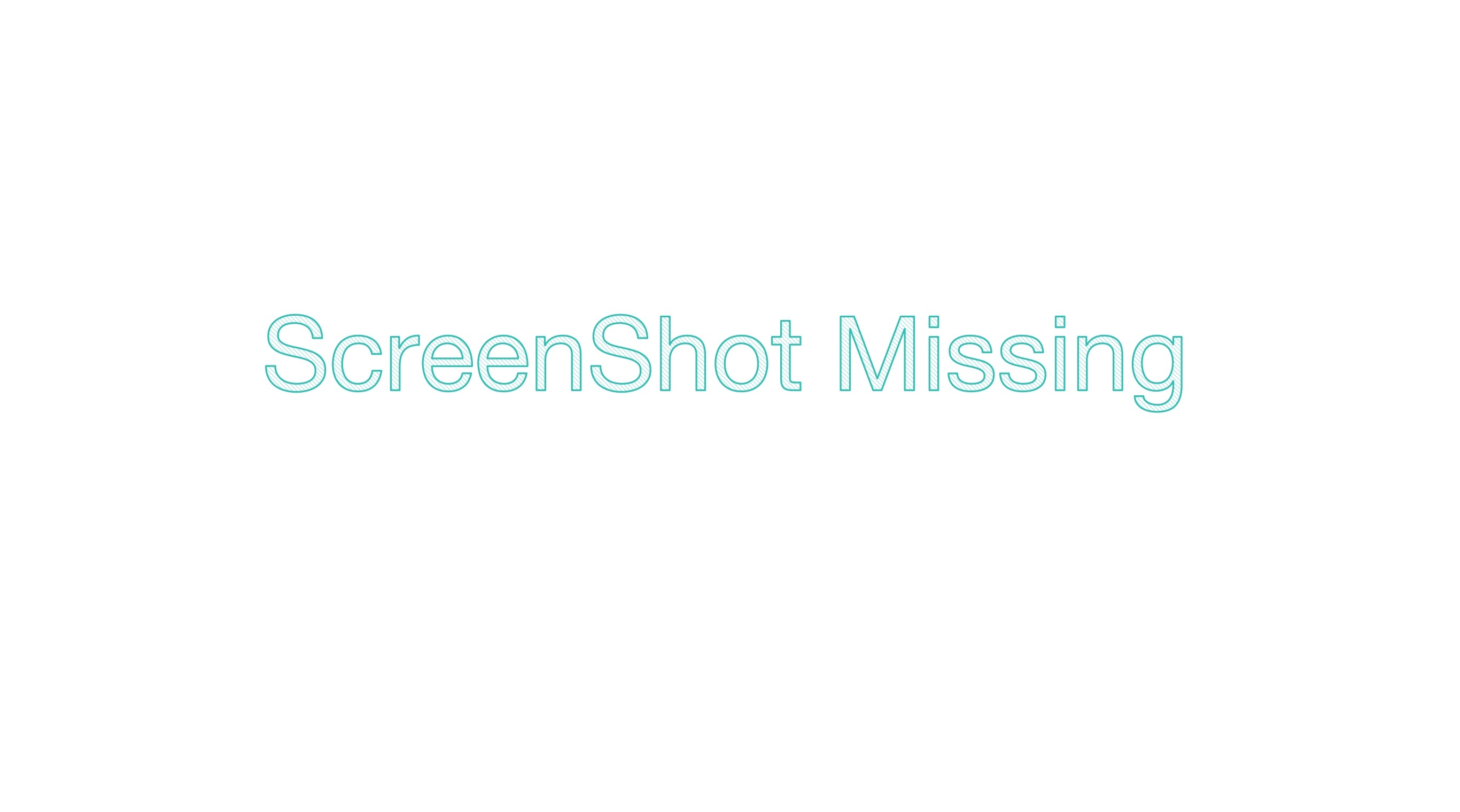
ENOVA
ENOVA is an open-source service for Large Language Model (LLM) deployment, monitoring, injection, and auto-scaling. It addresses challenges in deploying stable serverless LLM services on GPU clusters with auto-scaling by deconstructing the LLM service execution process and providing configuration recommendations and performance detection. Users can build and deploy LLM with few command lines, recommend optimal computing resources, experience LLM performance, observe operating status, achieve load balancing, and more. ENOVA ensures stable operation, cost-effectiveness, efficiency, and strong scalability of LLM services.
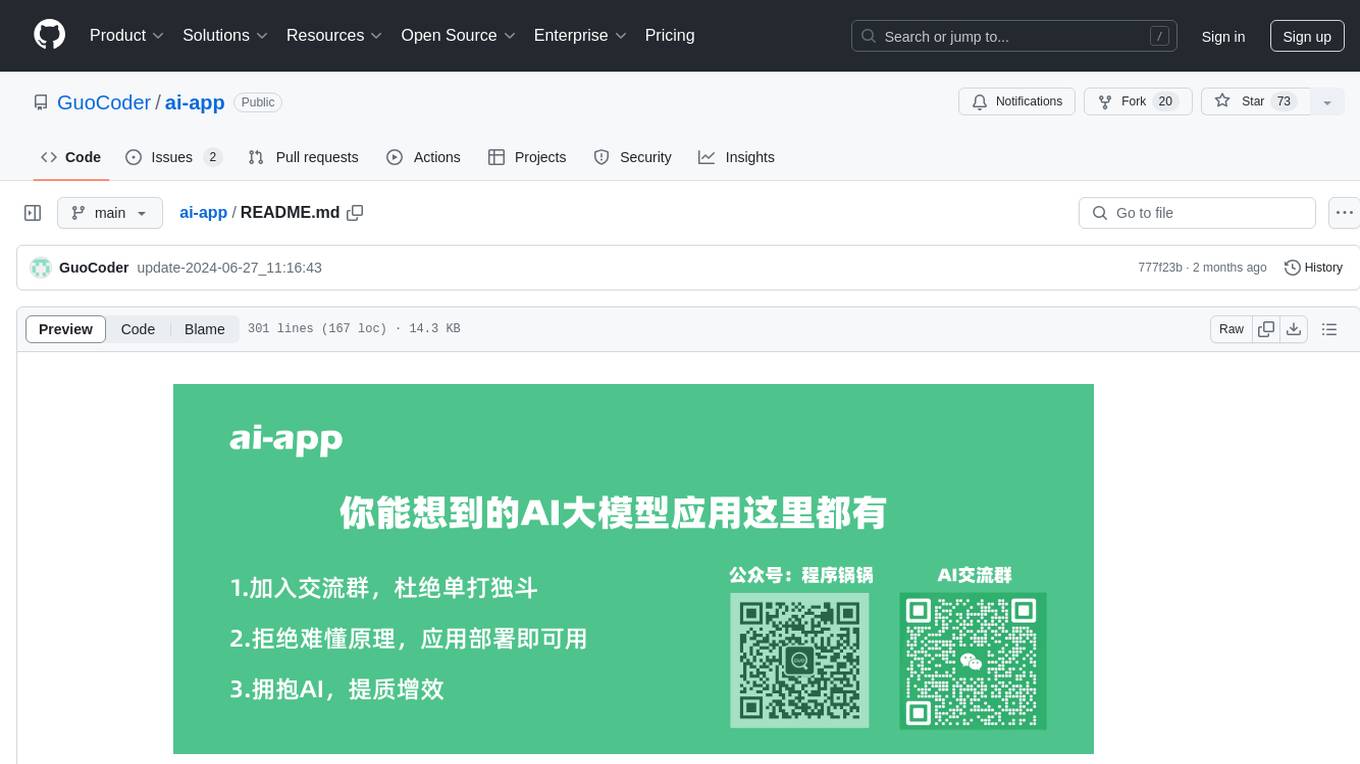
ai-app
The 'ai-app' repository is a comprehensive collection of tools and resources related to artificial intelligence, focusing on topics such as server environment setup, PyCharm and Anaconda installation, large model deployment and training, Transformer principles, RAG technology, vector databases, AI image, voice, and music generation, and AI Agent frameworks. It also includes practical guides and tutorials on implementing various AI applications. The repository serves as a valuable resource for individuals interested in exploring different aspects of AI technology.
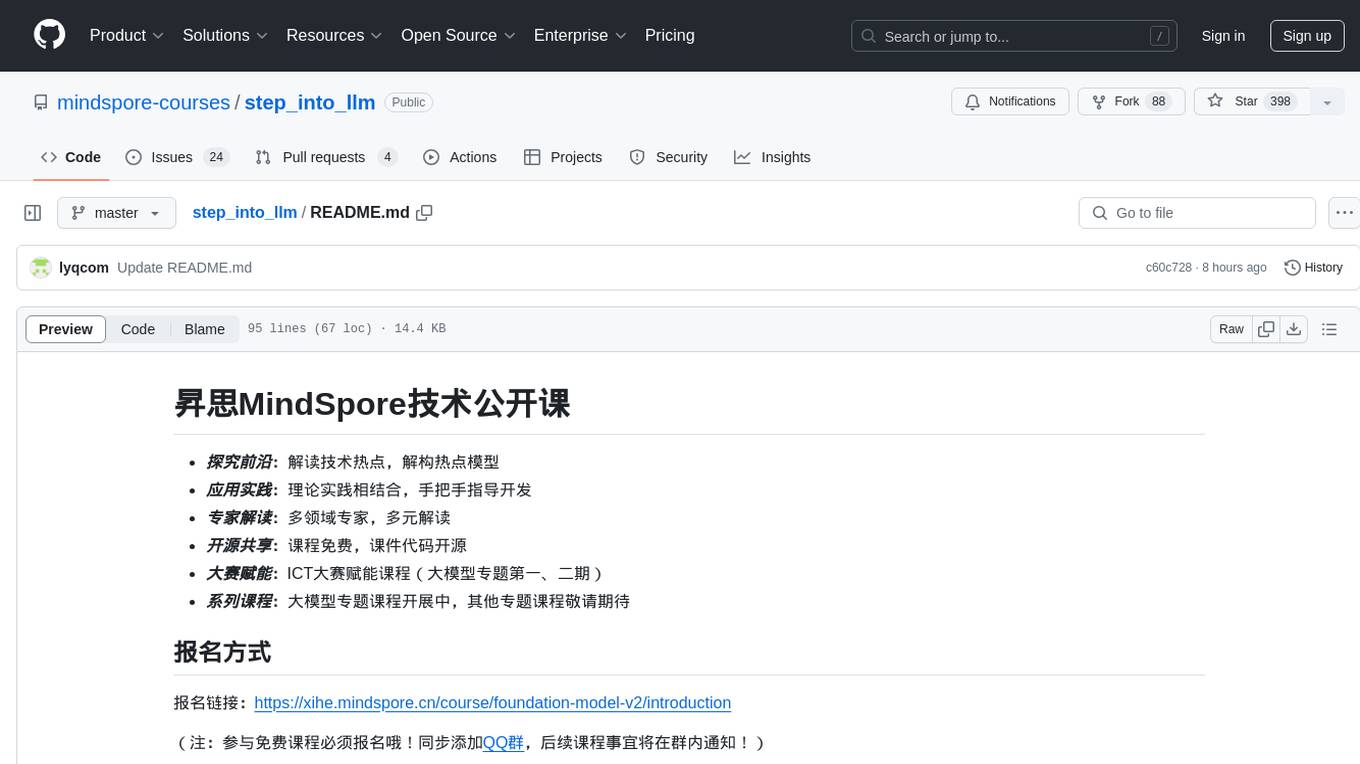
step_into_llm
The 'step_into_llm' repository is dedicated to the 昇思MindSpore technology open class, which focuses on exploring cutting-edge technologies, combining theory with practical applications, expert interpretations, open sharing, and empowering competitions. The repository contains course materials, including slides and code, for the ongoing second phase of the course. It covers various topics related to large language models (LLMs) such as Transformer, BERT, GPT, GPT2, and more. The course aims to guide developers interested in LLMs from theory to practical implementation, with a special emphasis on the development and application of large models.
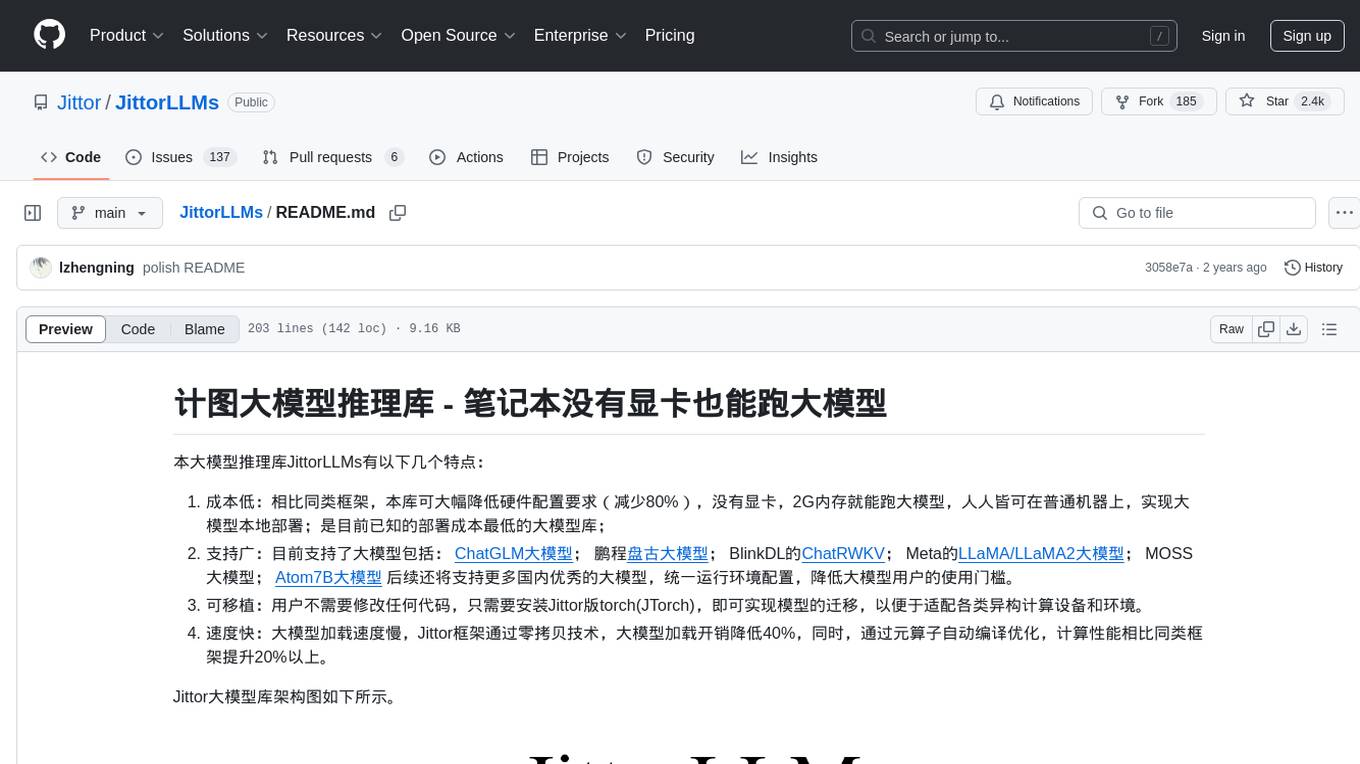
JittorLLMs
JittorLLMs is a large model inference library that allows running large models on machines with low hardware requirements. It significantly reduces hardware configuration demands, enabling deployment on ordinary machines with 2GB of memory. It supports various large models and provides a unified environment configuration for users. Users can easily migrate models without modifying any code by installing Jittor version of torch (JTorch). The framework offers fast model loading speed, optimized computation performance, and portability across different computing devices and environments.
For similar jobs
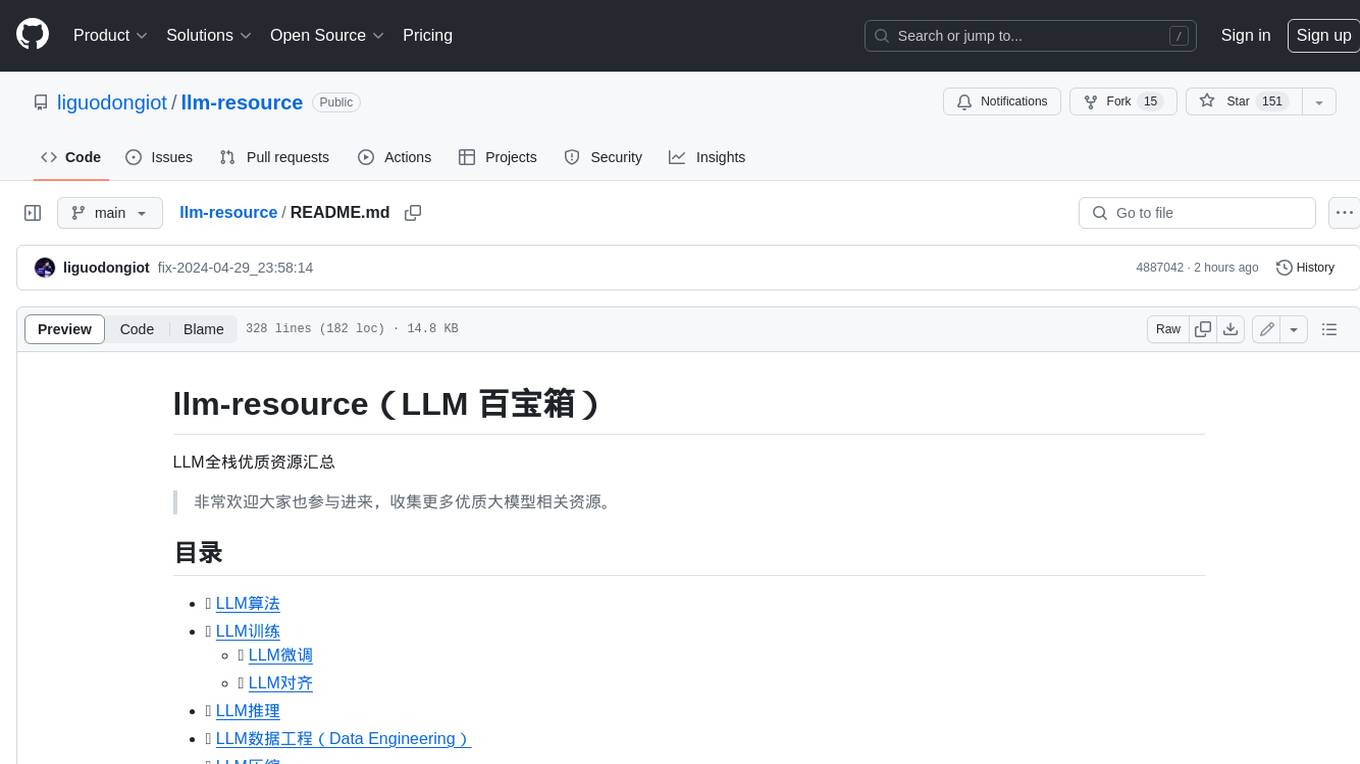
llm-resource
llm-resource is a comprehensive collection of high-quality resources for Large Language Models (LLM). It covers various aspects of LLM including algorithms, training, fine-tuning, alignment, inference, data engineering, compression, evaluation, prompt engineering, AI frameworks, AI basics, AI infrastructure, AI compilers, LLM application development, LLM operations, AI systems, and practical implementations. The repository aims to gather and share valuable resources related to LLM for the community to benefit from.
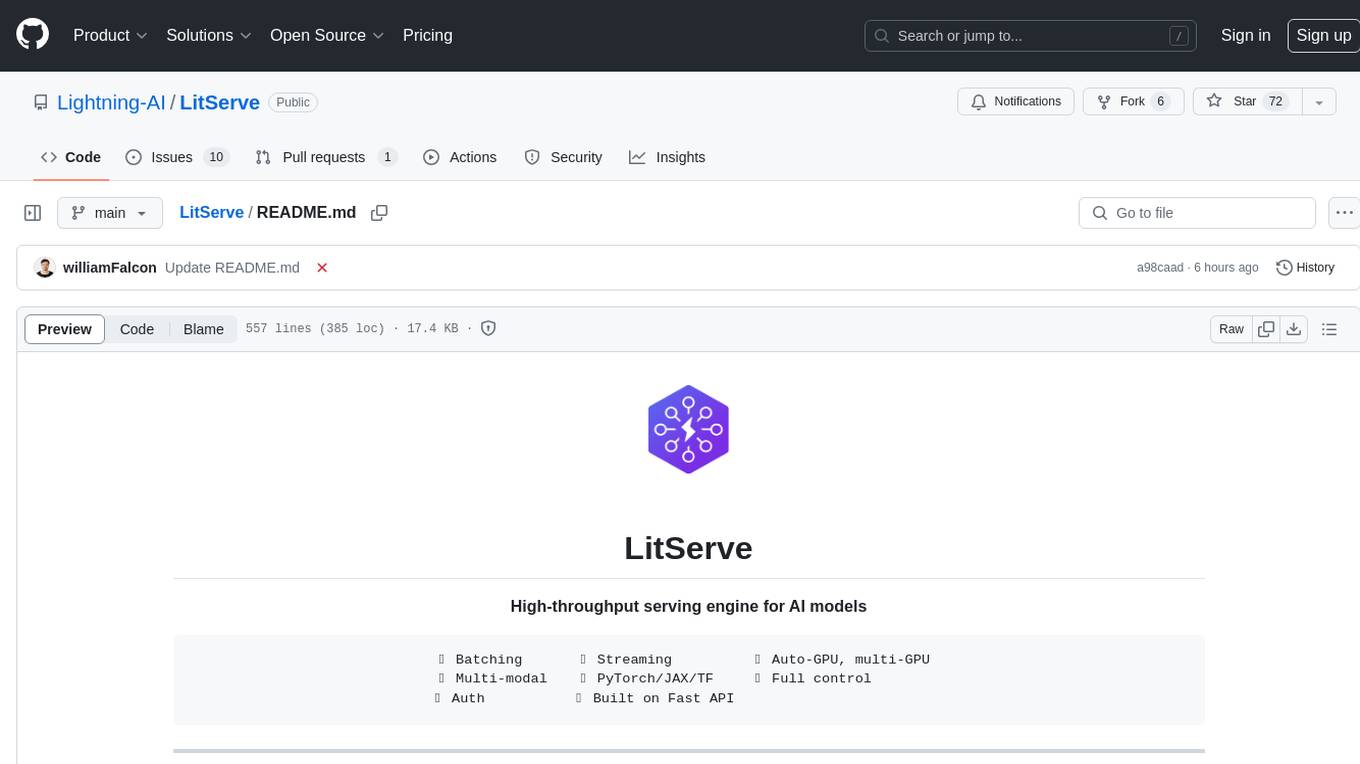
LitServe
LitServe is a high-throughput serving engine designed for deploying AI models at scale. It generates an API endpoint for models, handles batching, streaming, and autoscaling across CPU/GPUs. LitServe is built for enterprise scale with a focus on minimal, hackable code-base without bloat. It supports various model types like LLMs, vision, time-series, and works with frameworks like PyTorch, JAX, Tensorflow, and more. The tool allows users to focus on model performance rather than serving boilerplate, providing full control and flexibility.
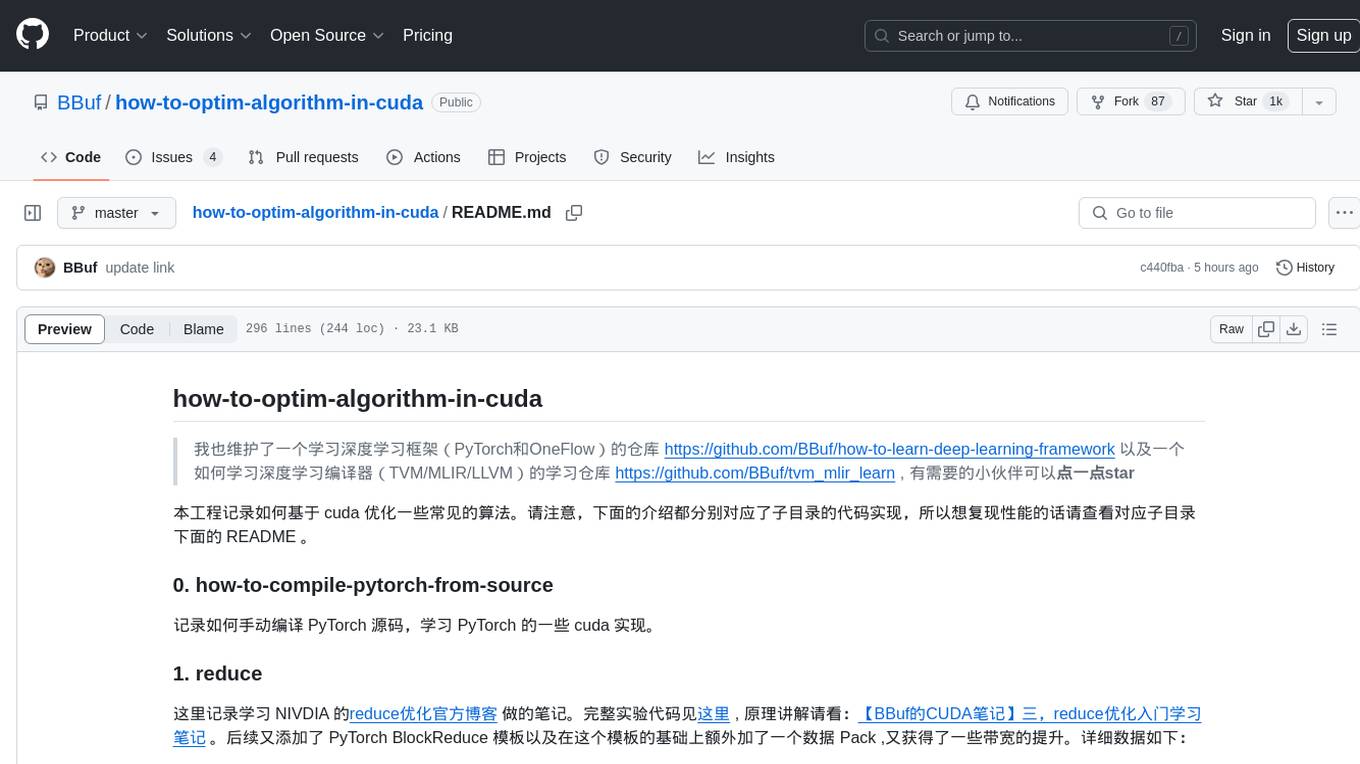
how-to-optim-algorithm-in-cuda
This repository documents how to optimize common algorithms based on CUDA. It includes subdirectories with code implementations for specific optimizations. The optimizations cover topics such as compiling PyTorch from source, NVIDIA's reduce optimization, OneFlow's elementwise template, fast atomic add for half data types, upsample nearest2d optimization in OneFlow, optimized indexing in PyTorch, OneFlow's softmax kernel, linear attention optimization, and more. The repository also includes learning resources related to deep learning frameworks, compilers, and optimization techniques.
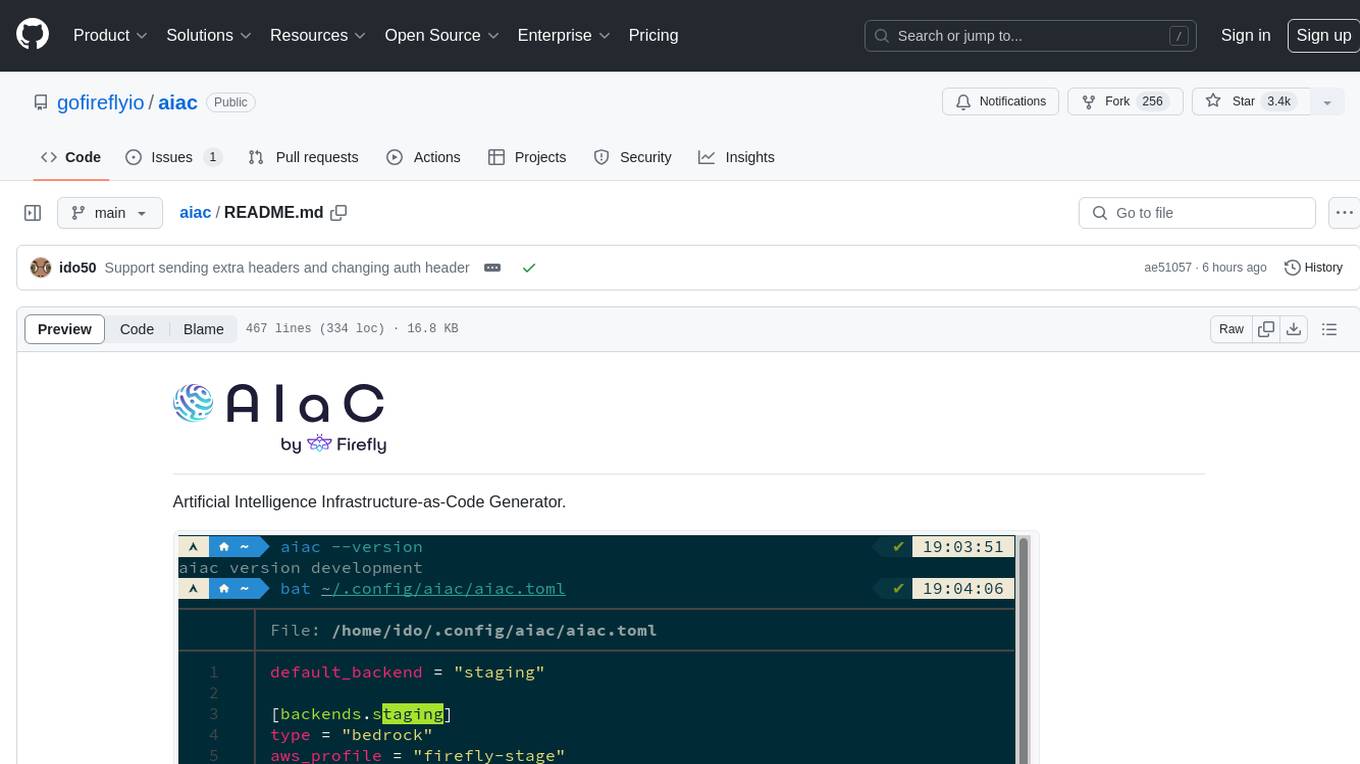
aiac
AIAC is a library and command line tool to generate Infrastructure as Code (IaC) templates, configurations, utilities, queries, and more via LLM providers such as OpenAI, Amazon Bedrock, and Ollama. Users can define multiple 'backends' targeting different LLM providers and environments using a simple configuration file. The tool allows users to ask a model to generate templates for different scenarios and composes an appropriate request to the selected provider, storing the resulting code to a file and/or printing it to standard output.
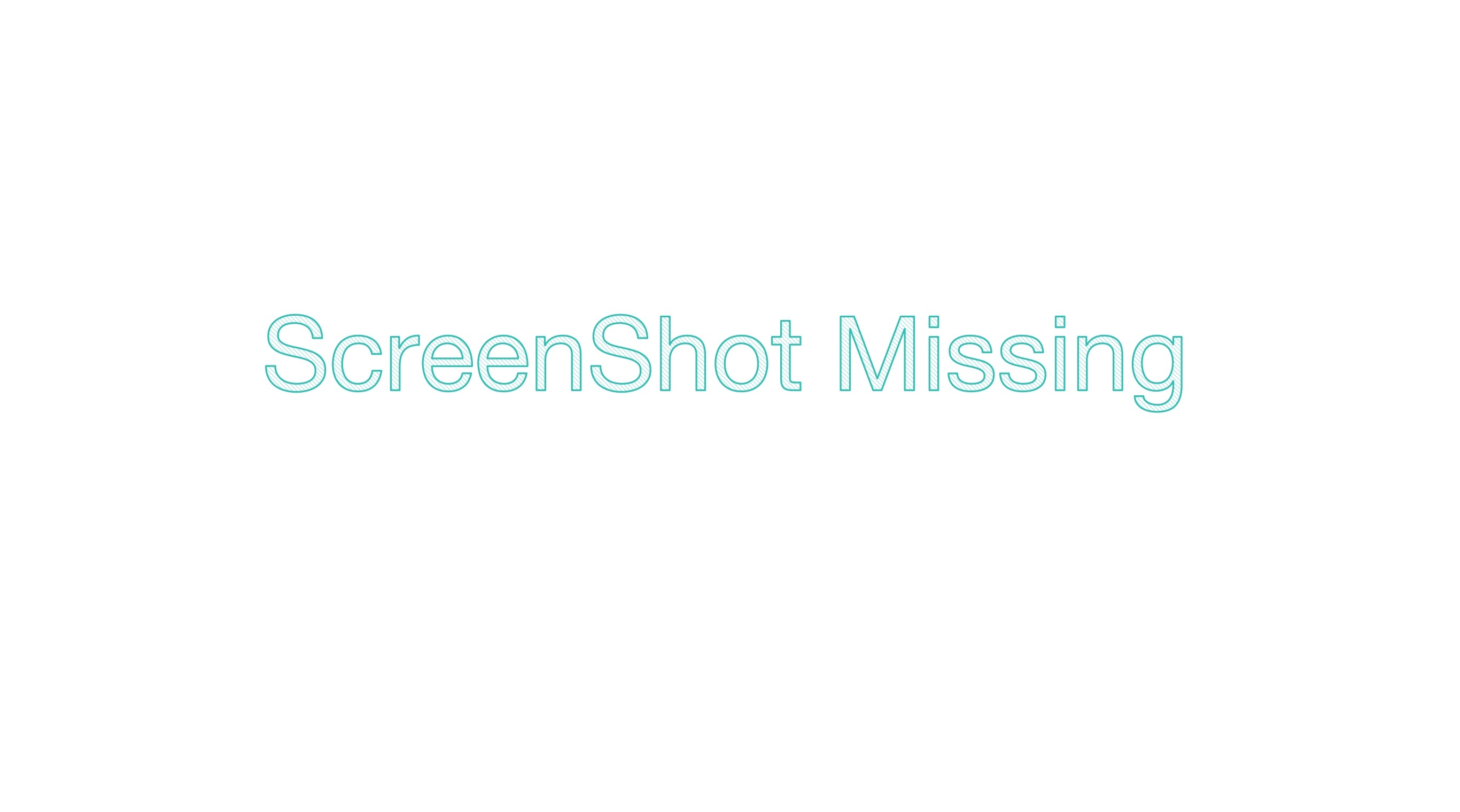
ENOVA
ENOVA is an open-source service for Large Language Model (LLM) deployment, monitoring, injection, and auto-scaling. It addresses challenges in deploying stable serverless LLM services on GPU clusters with auto-scaling by deconstructing the LLM service execution process and providing configuration recommendations and performance detection. Users can build and deploy LLM with few command lines, recommend optimal computing resources, experience LLM performance, observe operating status, achieve load balancing, and more. ENOVA ensures stable operation, cost-effectiveness, efficiency, and strong scalability of LLM services.
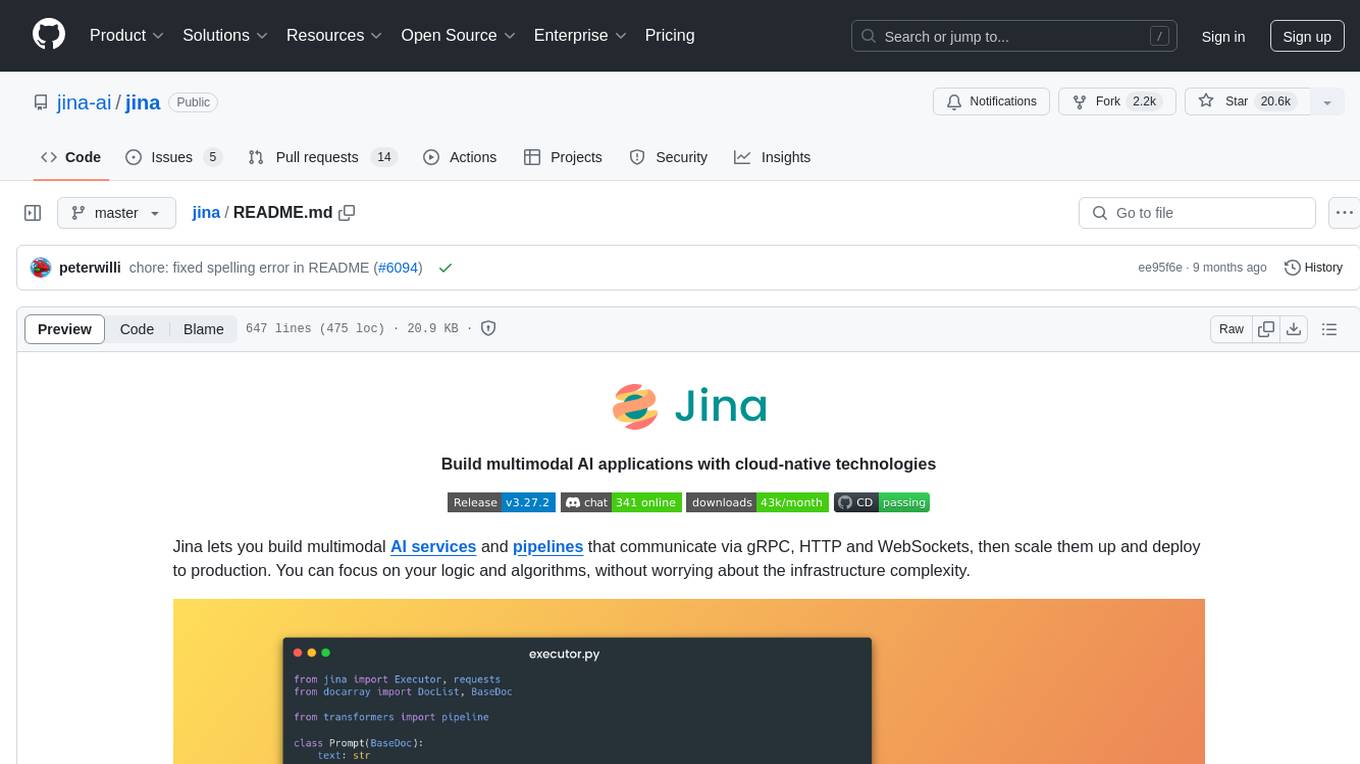
jina
Jina is a tool that allows users to build multimodal AI services and pipelines using cloud-native technologies. It provides a Pythonic experience for serving ML models and transitioning from local deployment to advanced orchestration frameworks like Docker-Compose, Kubernetes, or Jina AI Cloud. Users can build and serve models for any data type and deep learning framework, design high-performance services with easy scaling, serve LLM models while streaming their output, integrate with Docker containers via Executor Hub, and host on CPU/GPU using Jina AI Cloud. Jina also offers advanced orchestration and scaling capabilities, a smooth transition to the cloud, and easy scalability and concurrency features for applications. Users can deploy to their own cloud or system with Kubernetes and Docker Compose integration, and even deploy to JCloud for autoscaling and monitoring.
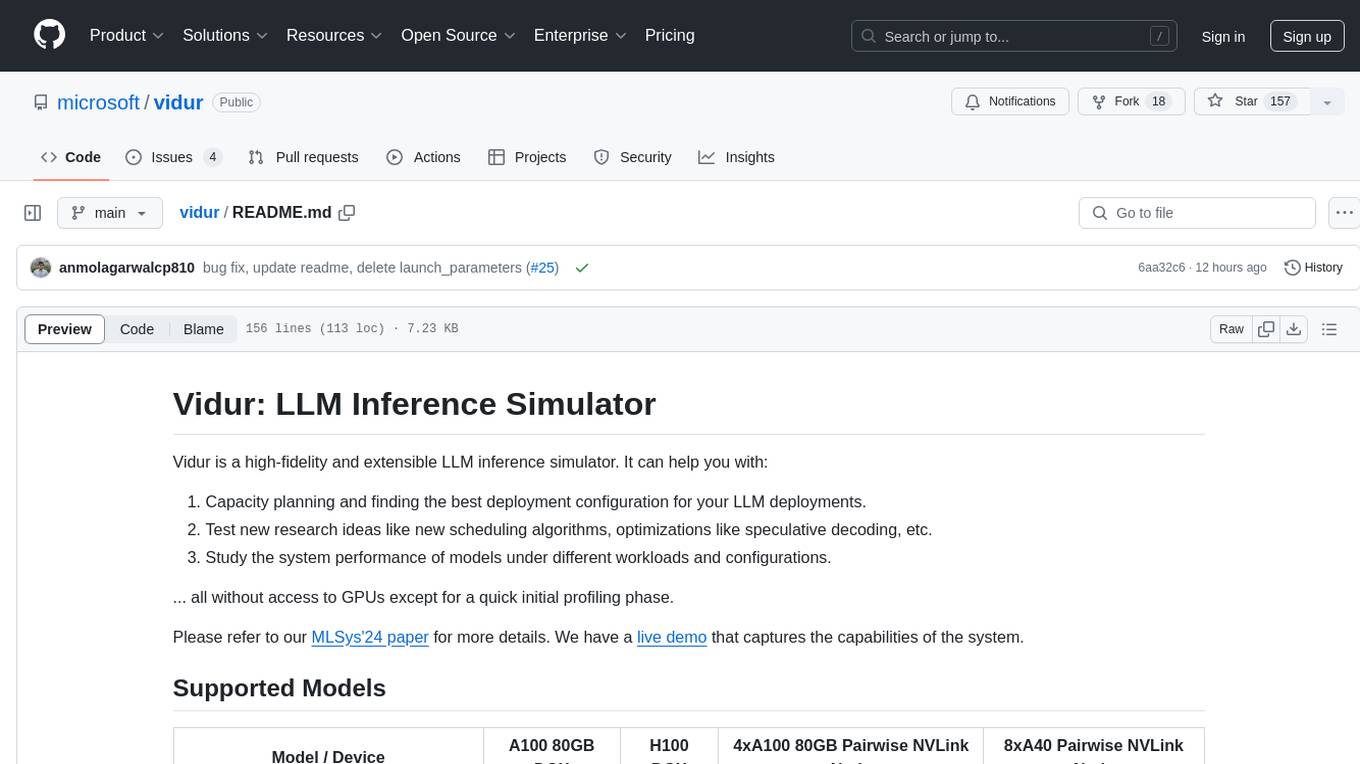
vidur
Vidur is a high-fidelity and extensible LLM inference simulator designed for capacity planning, deployment configuration optimization, testing new research ideas, and studying system performance of models under different workloads and configurations. It supports various models and devices, offers chrome trace exports, and can be set up using mamba, venv, or conda. Users can run the simulator with various parameters and monitor metrics using wandb. Contributions are welcome, subject to a Contributor License Agreement and adherence to the Microsoft Open Source Code of Conduct.
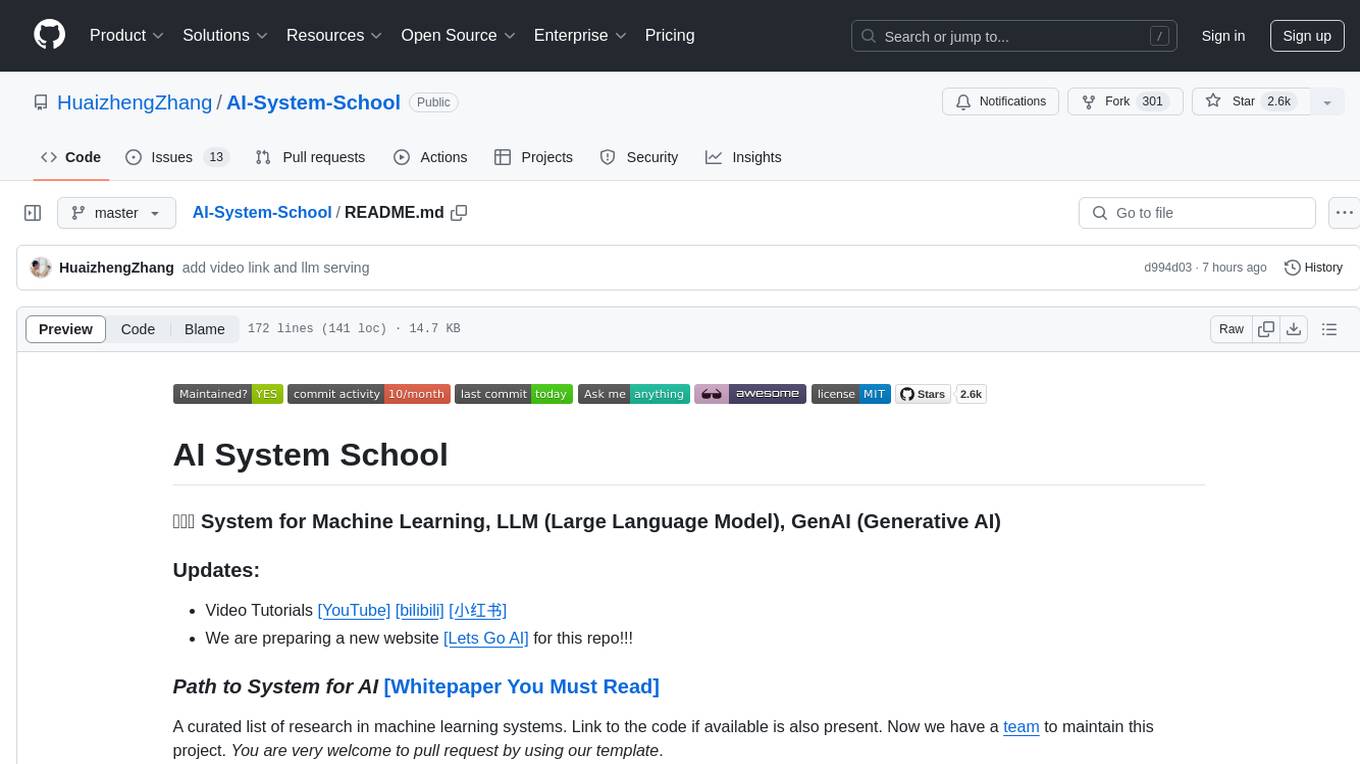
AI-System-School
AI System School is a curated list of research in machine learning systems, focusing on ML/DL infra, LLM infra, domain-specific infra, ML/LLM conferences, and general resources. It provides resources such as data processing, training systems, video systems, autoML systems, and more. The repository aims to help users navigate the landscape of AI systems and machine learning infrastructure, offering insights into conferences, surveys, books, videos, courses, and blogs related to the field.