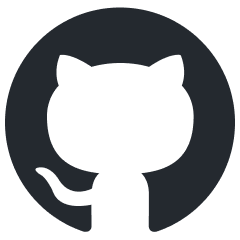
JittorLLMs
计图大模型推理库,具有高性能、配置要求低、中文支持好、可移植等特点
Stars: 2404
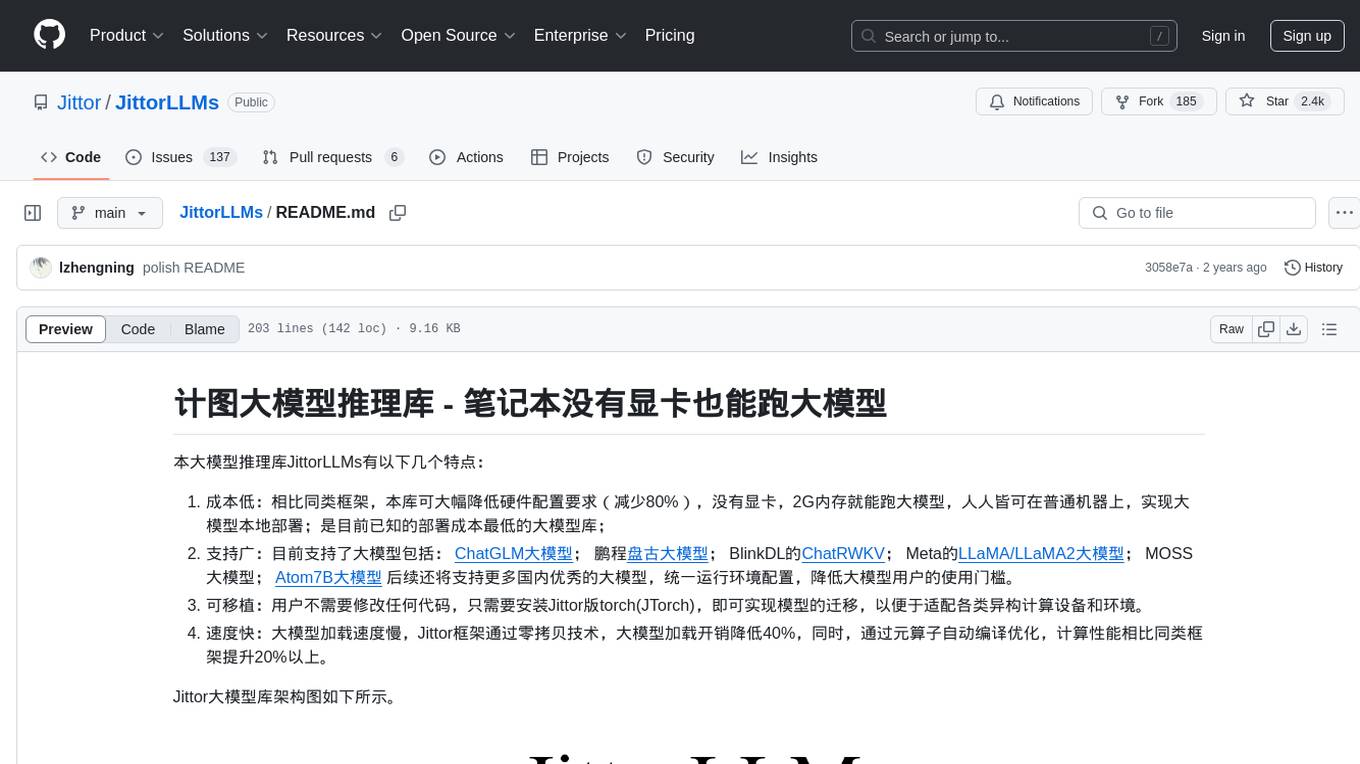
JittorLLMs is a large model inference library that allows running large models on machines with low hardware requirements. It significantly reduces hardware configuration demands, enabling deployment on ordinary machines with 2GB of memory. It supports various large models and provides a unified environment configuration for users. Users can easily migrate models without modifying any code by installing Jittor version of torch (JTorch). The framework offers fast model loading speed, optimized computation performance, and portability across different computing devices and environments.
README:
本大模型推理库JittorLLMs有以下几个特点:
- 成本低:相比同类框架,本库可大幅降低硬件配置要求(减少80%),没有显卡,2G内存就能跑大模型,人人皆可在普通机器上,实现大模型本地部署;是目前已知的部署成本最低的大模型库;
- 支持广:目前支持了大模型包括: ChatGLM大模型; 鹏程盘古大模型; BlinkDL的ChatRWKV; Meta的LLaMA/LLaMA2大模型; MOSS大模型; Atom7B大模型 后续还将支持更多国内优秀的大模型,统一运行环境配置,降低大模型用户的使用门槛。
- 可移植:用户不需要修改任何代码,只需要安装Jittor版torch(JTorch),即可实现模型的迁移,以便于适配各类异构计算设备和环境。
- 速度快:大模型加载速度慢,Jittor框架通过零拷贝技术,大模型加载开销降低40%,同时,通过元算子自动编译优化,计算性能相比同类框架提升20%以上。
Jittor大模型库架构图如下所示。
- 内存要求:至少2G,推荐32G
- 显存:可选, 推荐16G
- 操作系统:支持Windows,Mac,Linux全平台。
- 磁盘空间:至少40GB空闲磁盘空间,用于下载参数和存储交换文件。
- Python版本要求至少
3.8
(Linux的Python版本至少3.7
)。
磁盘空间不够时,可以通过环境变量JITTOR_HOME
指定缓存存放路径。
内存或者显存不够,出现进程被杀死的情况,请参考下方,限制内存消耗的方法。
可以通过下述指令安装依赖。(注意:此脚本会安装Jittor版torch,推荐用户新建环境运行)
# 国内使用 gitlink clone
git clone https://gitlink.org.cn/jittor/JittorLLMs.git --depth 1
# github: git clone https://github.com/Jittor/JittorLLMs.git --depth 1
cd JittorLLMs
# -i 指定用jittor的源, -I 强制重装Jittor版torch
pip install -r requirements.txt -i https://pypi.jittor.org/simple -I
如果出现找不到jittor版本的错误,可能是您使用的镜像还没有更新,使用如下命令更新最新版:pip install jittor -U -i https://pypi.org/simple
部署只需一行命令即可:
python cli_demo.py [chatglm|pangualpha|llama|chatrwkv|llama2|atom7b]
运行后会自动从服务器上下载模型文件到本地,会占用根目录下一定的硬盘空间。 例如对于盘古α约为 15G。最开始运行的时候会编译一些CUDA算子,这会花费一些时间进行加载。
下图是 ChatGLM 的实时对话截图:
下图是 盘古Alpha 的实时对话截图:
下图是 ChatRWKV 的实时对话截图:
下图是 LLaMA 的实时对话截图:
下图是 LLaMA2 的实时对话截图:
下图是 Atom7b 的实时对话截图:
目前支持了 ChatGLM
、Atom7B
和 盘古α 的中文对话,ChatRWKV
,LLaMA
和LLaMA2
支持英文对话,后续会持续更新最新的模型参数以及微调的结果。MOSS
大··模型使用方式请参考 MOSS 官方仓库。
内存或者显存不够,出现进程被杀死的情况,请参考下方,限制内存消耗的方法。
JittorLLM通过gradio库,允许用户在浏览器之中和大模型直接进行对话。
python web_demo.py chatglm
可以得到下图所示的结果。
JittorLLM在api.py文件之中,提供了一个架设后端服务的示例。
python api.py chatglm
接着可以使用如下代码进行直接访问
post_data = json.dumps({'prompt': 'Hello, solve 5x=13'})
print(json.loads(requests.post("http://0.0.0.0:8000", post_data).text)['response'])
针对大模型显存消耗大等痛点,Jittor团队研发了动态交换技术,根据我们调研,Jittor框架是世界上首个支持动态图变量自动交换功能的框架,区别于以往的基于静态图交换技术,用户不需要修改任何代码,原生的动态图代码即可直接支持张量交换,张量数据可以在显存-内存-硬盘之间自动交换,降低用户开发难度。
同时,根据我们调研,Jittor大模型推理库也是目前对配置门槛要求最低的框架,只需要参数磁盘空间和2G内存,无需显卡,也可以部署大模型,下面是在不同硬件配置条件下的资源消耗与速度对比。可以发现,JittorLLMs在显存充足的情况下,性能优于同类框架,而显存不足甚至没有显卡,JittorLLMs都能以一定速度运行。
节省内存方法,请安装Jittor版本大于1.3.7.8,并添加如下环境变量:
export JT_SAVE_MEM=1
# 限制cpu最多使用16G
export cpu_mem_limit=16000000000
# 限制device内存(如gpu、tpu等)最多使用8G
export device_mem_limit=8000000000
# windows 用户,请使用powershell
# $env:JT_SAVE_MEM="1"
# $env:cpu_mem_limit="16000000000"
# $env:device_mem_limit="8000000000"
用户可以自由设定cpu和设备内存的使用量,如果不希望对内存进行限制,可以设置为-1
。
# 限制cpu最多使用16G
export cpu_mem_limit=-1
# 限制device内存(如gpu、tpu等)最多使用8G
export device_mem_limit=-1
# windows 用户,请使用powershell
# $env:JT_SAVE_MEM="1"
# $env:cpu_mem_limit="-1"
# $env:device_mem_limit="-1"
如果想要清理磁盘交换文件,可以运行如下命令
python -m jittor_utils.clean_cache swap
大模型在推理过程中,常常碰到参数文件过大,模型加载效率低下等问题。Jittor框架通过内存直通读取,减少内存拷贝数量,大大提升模型加载效率。相比PyTorch框架,Jittor框架的模型加载效率提升了40%。
Jittor团队发布Jittor版PyTorch接口JTorch,用户无需修改任何代码,只需要按照如下方法安装,即可通过Jittor框架的优势节省显存、提高效率。
pip install torch -i https://pypi.jittor.org/simple
通过jtorch,即可适配各类异构大模型代码,如常见的Megatron、Hugging Face Transformers,均可直接移植。同时,通过计图底层元算子硬件适配能力,可以十分方便的迁移到各类国内外计算设备上。
欢迎各位大模型用户尝试、使用,并且给我们提出宝贵的意见,未来,非十科技和清华大学可视媒体研究中心将继续专注于大模型的支撑,服务好大模型用户,提供成本更低,效率更高的解决方案,同时,欢迎各位大模型用户提交代码到JittorLLMs,丰富Jittor大模型库的支持。
- Jittor文档:https://cg.cs.tsinghua.edu.cn/jittor/assets/docs/index.html
- Jittor论坛:https://discuss.jittor.org/
- Jittor开发者交流群:761222083
- 模型训练与微调
- 移植 MOSS 大模型
- 动态 swap 性能优化
- CPU 性能优化
- 添加更多国内外优秀大模型支持
- ......
- MOSS
- BELLE
欢迎各位向我们提交请求
欢迎各位向我们提出宝贵的意见,可加入计图开发者交流群实时交流。
本计图大模型推理库,由非十科技领衔,与清华大学可视媒体研究中心合作研发,希望为国内大模型的研究提供软硬件的支撑。
北京非十科技有限公司是国内专业从事人工智能服务的科技公司,在3D AIGC、深度学习框架以及大模型领域,具有领先的技术优势。技术上致力于加速人工智能算法从硬件到软件全流程的落地应用、提供各类计算加速硬件的适配、定制深度学习框架以及优化人工智能应用性能速度等服务。公司技术骨干毕业自清华大学,具有丰富的系统软件、图形学、编译技术和深度学习框架的研发经验。公司研发了基于计图深度学习框架的国产自主可控人工智能系统,完成了对近十个国产加速硬件厂商的适配,正积极促进于国产人工智能生态的发展。开源了的高性能的神经辐射场渲染库JNeRF,可生成高质量3D AIGC模型,开源的JittorLLMs是目前硬件配置要求最低的大模型推理库。
For Tasks:
Click tags to check more tools for each tasksFor Jobs:
Alternative AI tools for JittorLLMs
Similar Open Source Tools
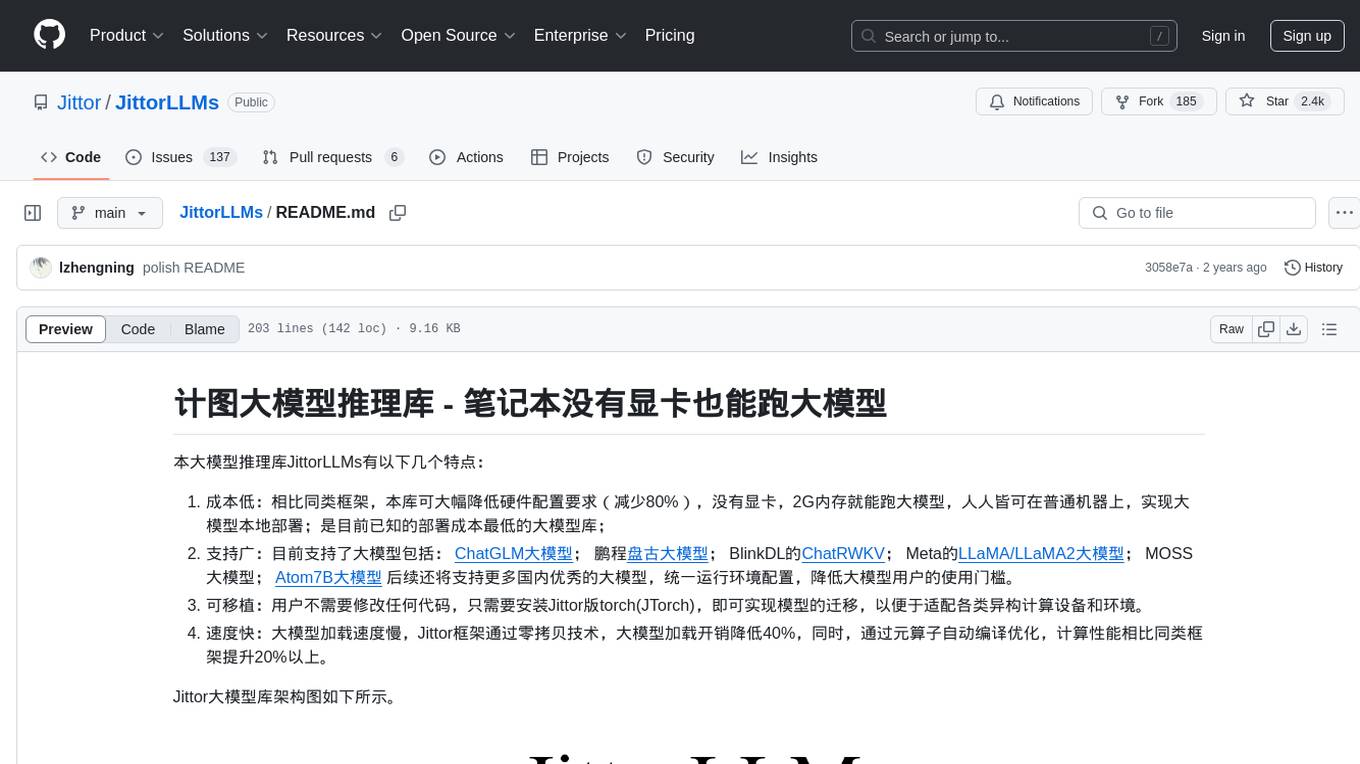
JittorLLMs
JittorLLMs is a large model inference library that allows running large models on machines with low hardware requirements. It significantly reduces hardware configuration demands, enabling deployment on ordinary machines with 2GB of memory. It supports various large models and provides a unified environment configuration for users. Users can easily migrate models without modifying any code by installing Jittor version of torch (JTorch). The framework offers fast model loading speed, optimized computation performance, and portability across different computing devices and environments.
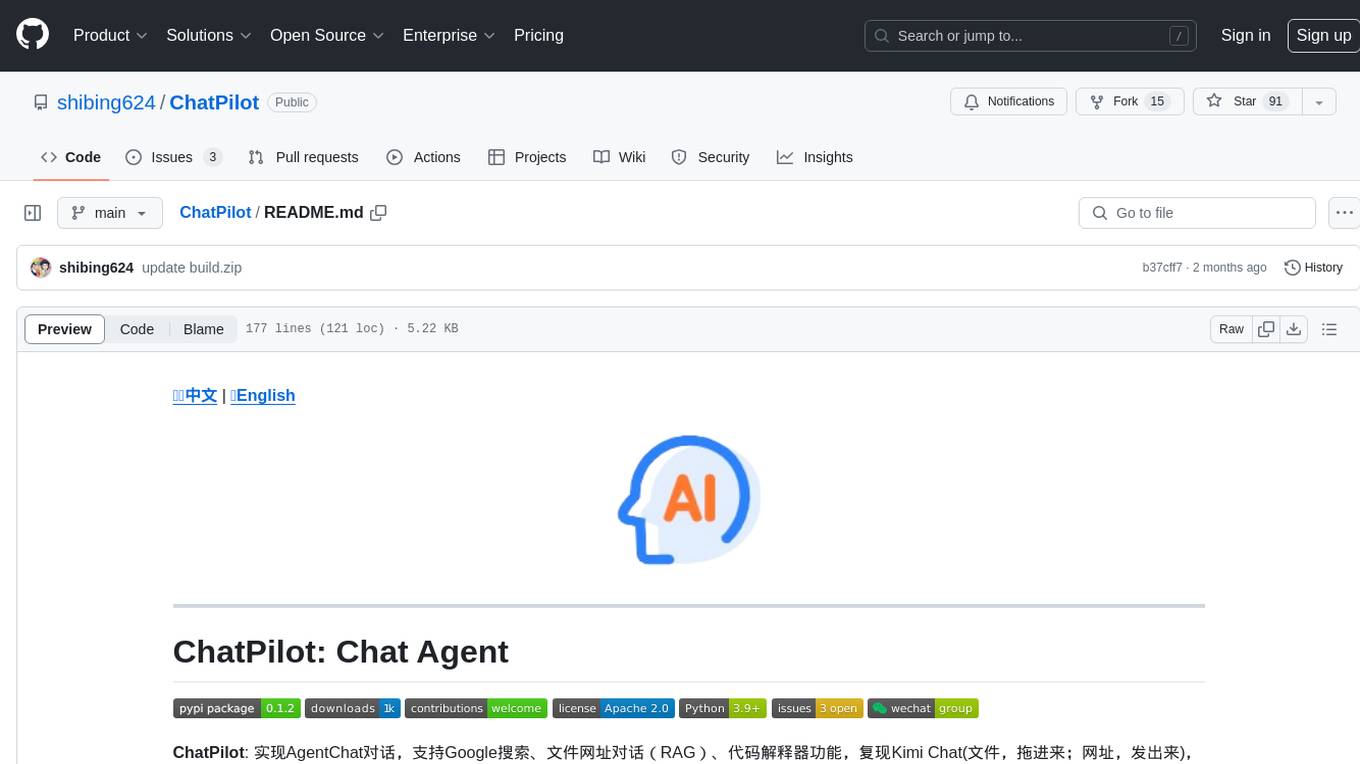
ChatPilot
ChatPilot is a chat agent tool that enables AgentChat conversations, supports Google search, URL conversation (RAG), and code interpreter functionality, replicates Kimi Chat (file, drag and drop; URL, send out), and supports OpenAI/Azure API. It is based on LangChain and implements ReAct and OpenAI Function Call for agent Q&A dialogue. The tool supports various automatic tools such as online search using Google Search API, URL parsing tool, Python code interpreter, and enhanced RAG file Q&A with query rewriting support. It also allows front-end and back-end service separation using Svelte and FastAPI, respectively. Additionally, it supports voice input/output, image generation, user management, permission control, and chat record import/export.
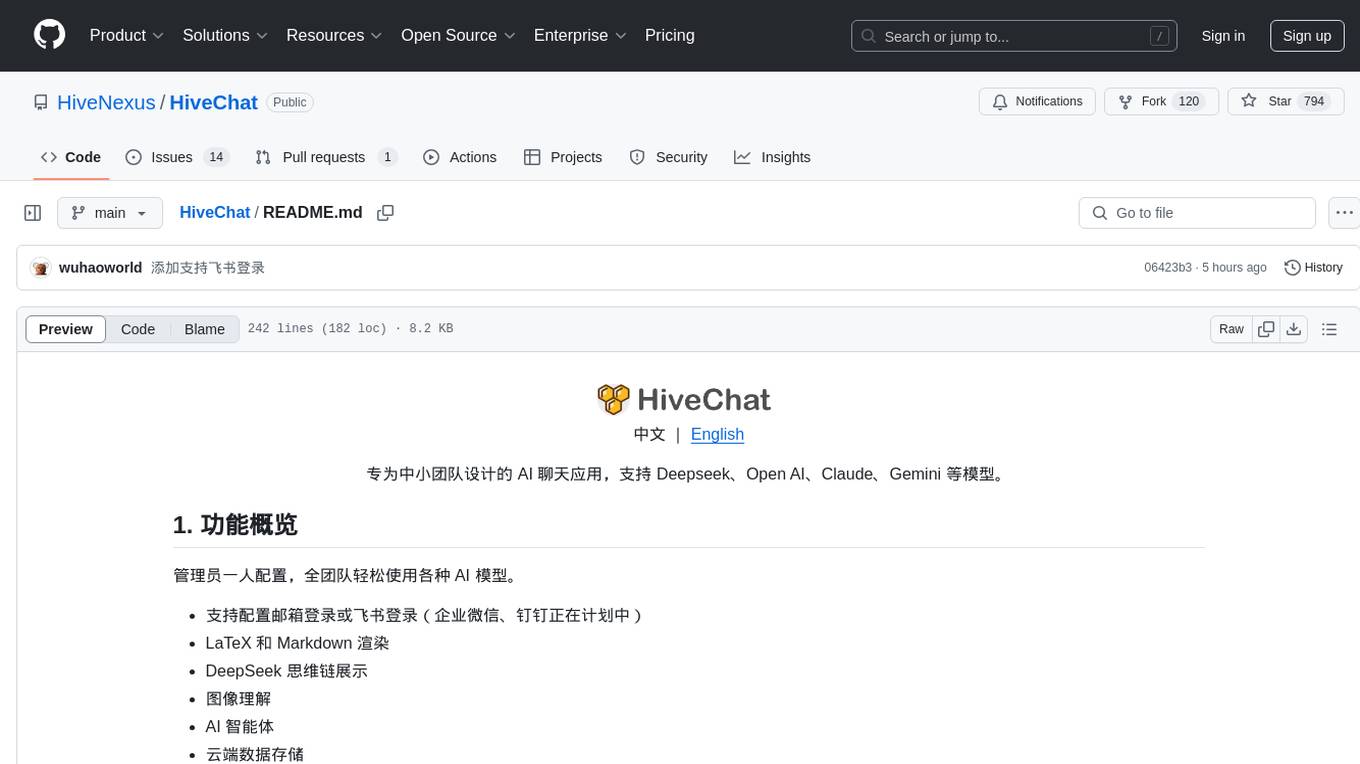
HiveChat
HiveChat is an AI chat application designed for small and medium teams. It supports various models such as DeepSeek, Open AI, Claude, and Gemini. The tool allows easy configuration by one administrator for the entire team to use different AI models. It supports features like email or Feishu login, LaTeX and Markdown rendering, DeepSeek mind map display, image understanding, AI agents, cloud data storage, and integration with multiple large model service providers. Users can engage in conversations by logging in, while administrators can configure AI service providers, manage users, and control account registration. The technology stack includes Next.js, Tailwindcss, Auth.js, PostgreSQL, Drizzle ORM, and Ant Design.
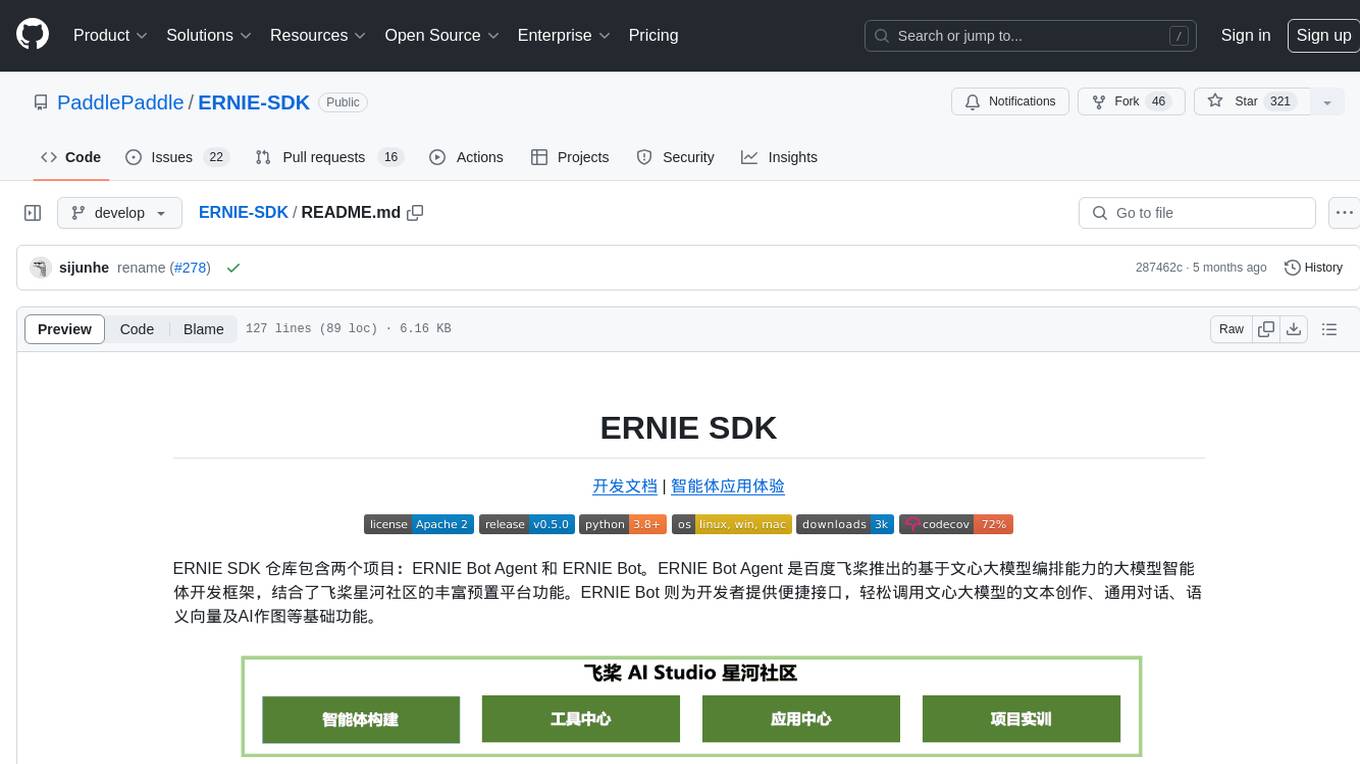
ERNIE-SDK
ERNIE SDK repository contains two projects: ERNIE Bot Agent and ERNIE Bot. ERNIE Bot Agent is a large model intelligent agent development framework based on the Wenxin large model orchestration capability introduced by Baidu PaddlePaddle, combined with the rich preset platform functions of the PaddlePaddle Star River community. ERNIE Bot provides developers with convenient interfaces to easily call the Wenxin large model for text creation, general conversation, semantic vectors, and AI drawing basic functions.
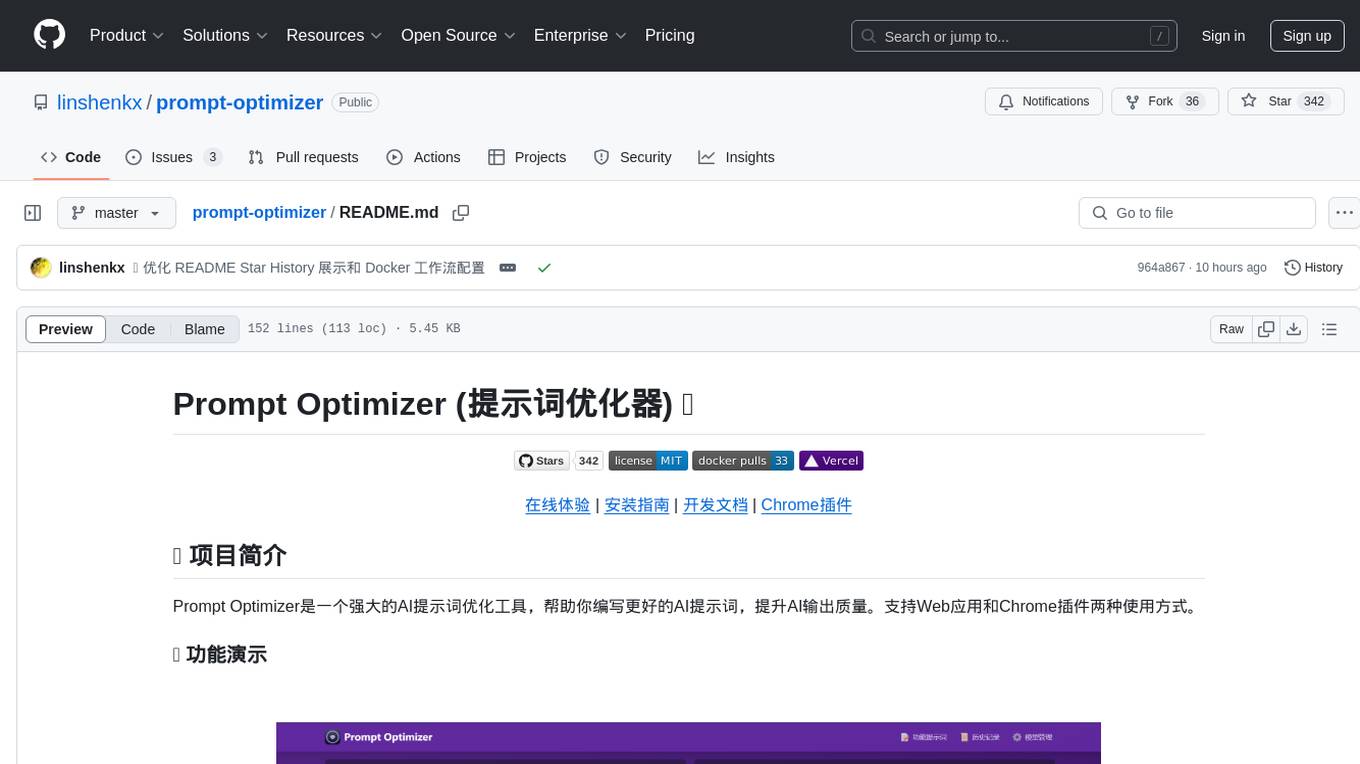
prompt-optimizer
Prompt Optimizer is a powerful AI prompt optimization tool that helps you write better AI prompts, improving AI output quality. It supports both web application and Chrome extension usage. The tool features intelligent optimization for prompt words, real-time testing to compare before and after optimization, integration with multiple mainstream AI models, client-side processing for security, encrypted local storage for data privacy, responsive design for user experience, and more.
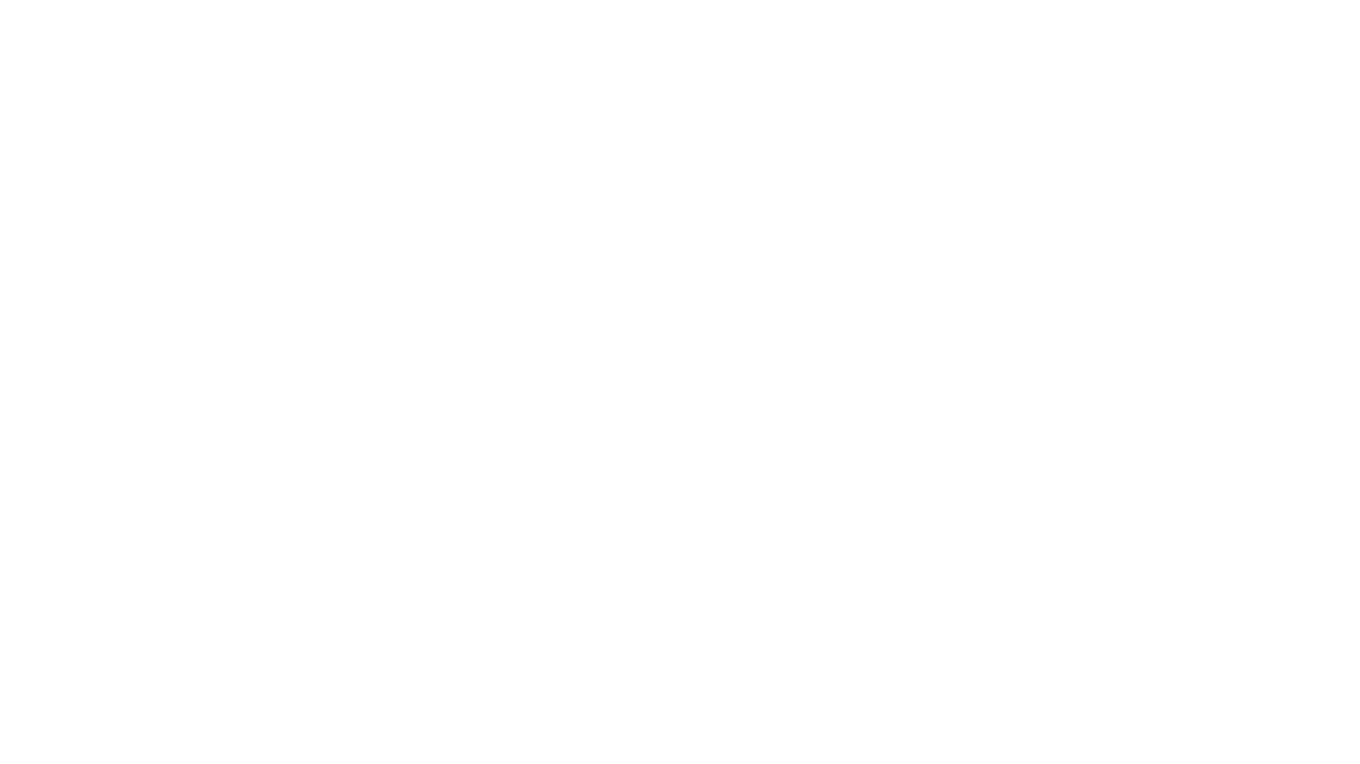
magic-resume
Magic Resume is a modern online resume editor that makes creating professional resumes simple and fun. Built on Next.js and Framer Motion, it supports real-time preview and custom themes. Features include Next.js 14+ based construction, smooth animation effects (Framer Motion), custom theme support, responsive design, dark mode, export to PDF, real-time preview, auto-save, and local storage. The technology stack includes Next.js 14+, TypeScript, Framer Motion, Tailwind CSS, Shadcn/ui, and Lucide Icons.
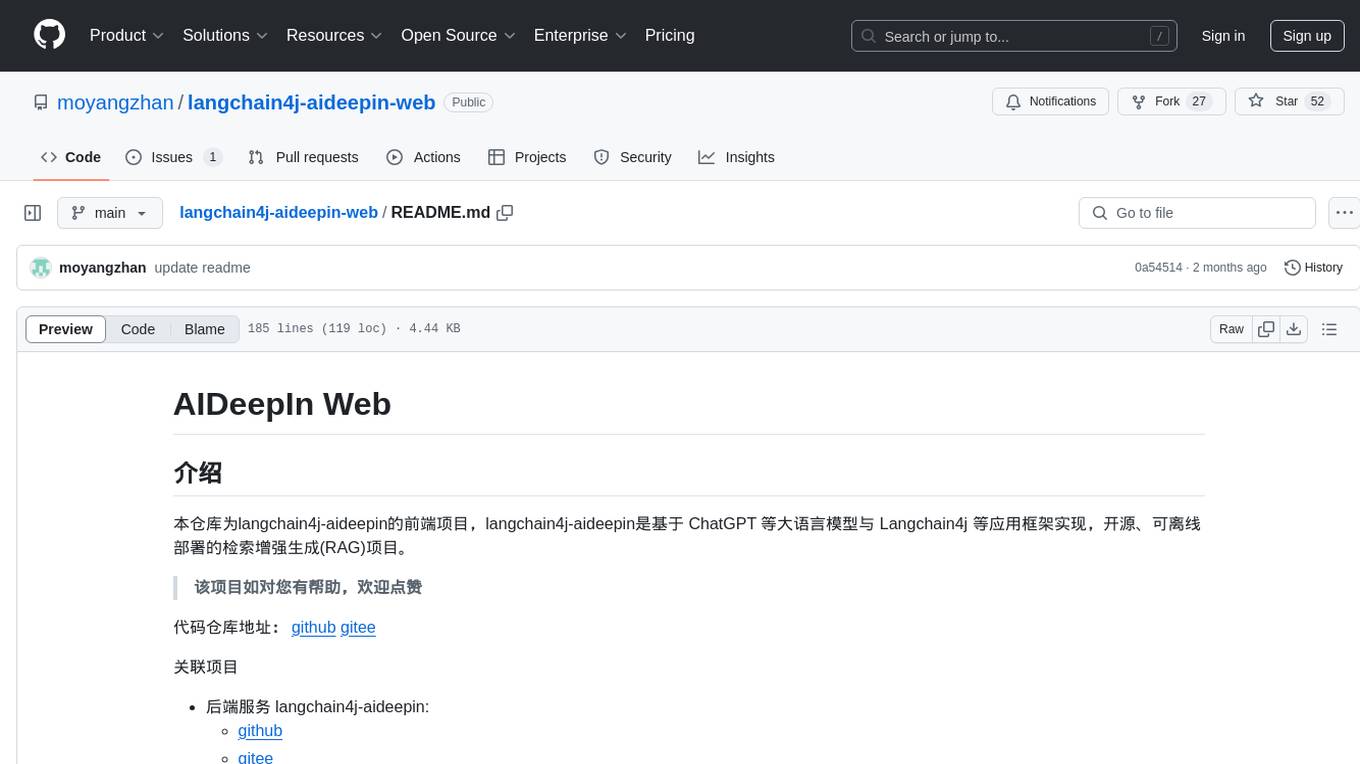
langchain4j-aideepin-web
The langchain4j-aideepin-web repository is the frontend project of langchain4j-aideepin, an open-source, offline deployable retrieval enhancement generation (RAG) project based on large language models such as ChatGPT and application frameworks such as Langchain4j. It includes features like registration & login, multi-sessions (multi-roles), image generation (text-to-image, image editing, image-to-image), suggestions, quota control, knowledge base (RAG) based on large models, model switching, and search engine switching.
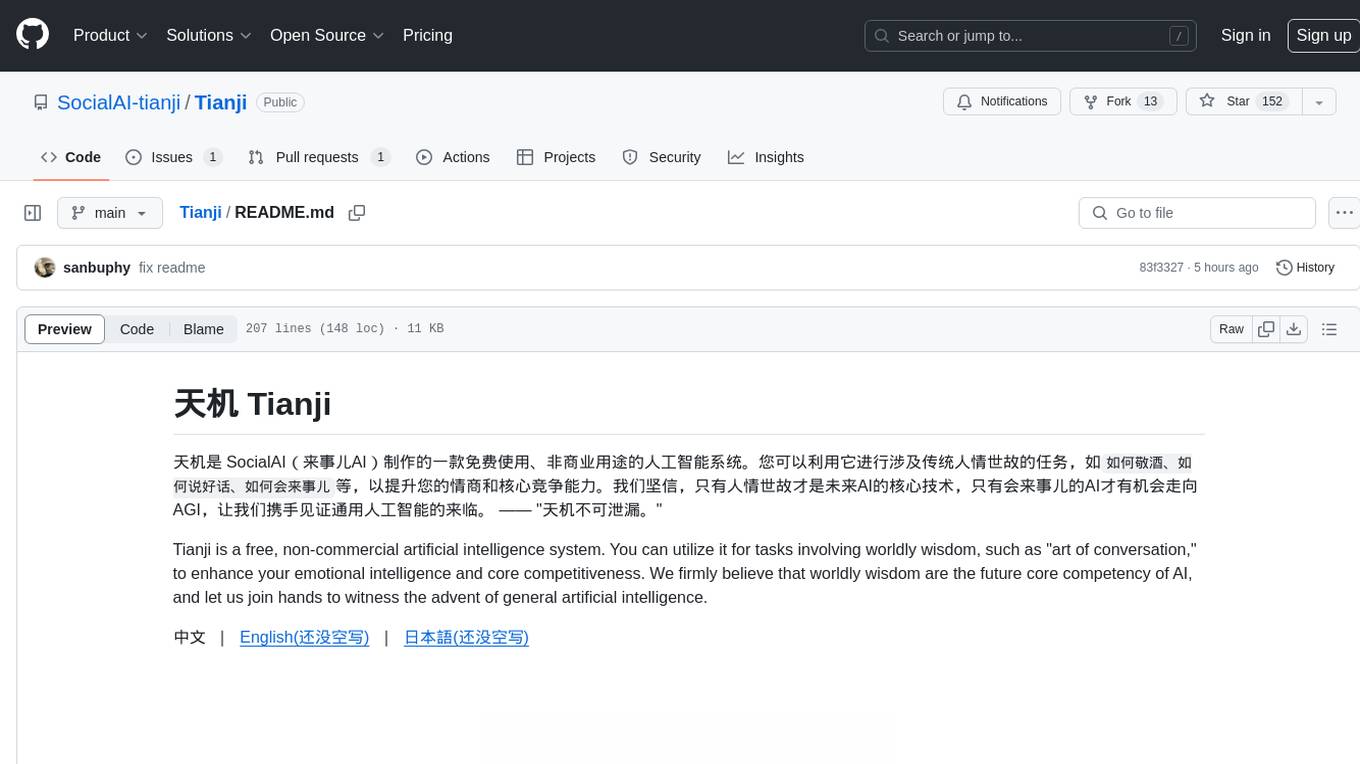
Tianji
Tianji is a free, non-commercial artificial intelligence system developed by SocialAI for tasks involving worldly wisdom, such as etiquette, hospitality, gifting, wishes, communication, awkwardness resolution, and conflict handling. It includes four main technical routes: pure prompt, Agent architecture, knowledge base, and model training. Users can find corresponding source code for these routes in the tianji directory to replicate their own vertical domain AI applications. The project aims to accelerate the penetration of AI into various fields and enhance AI's core competencies.
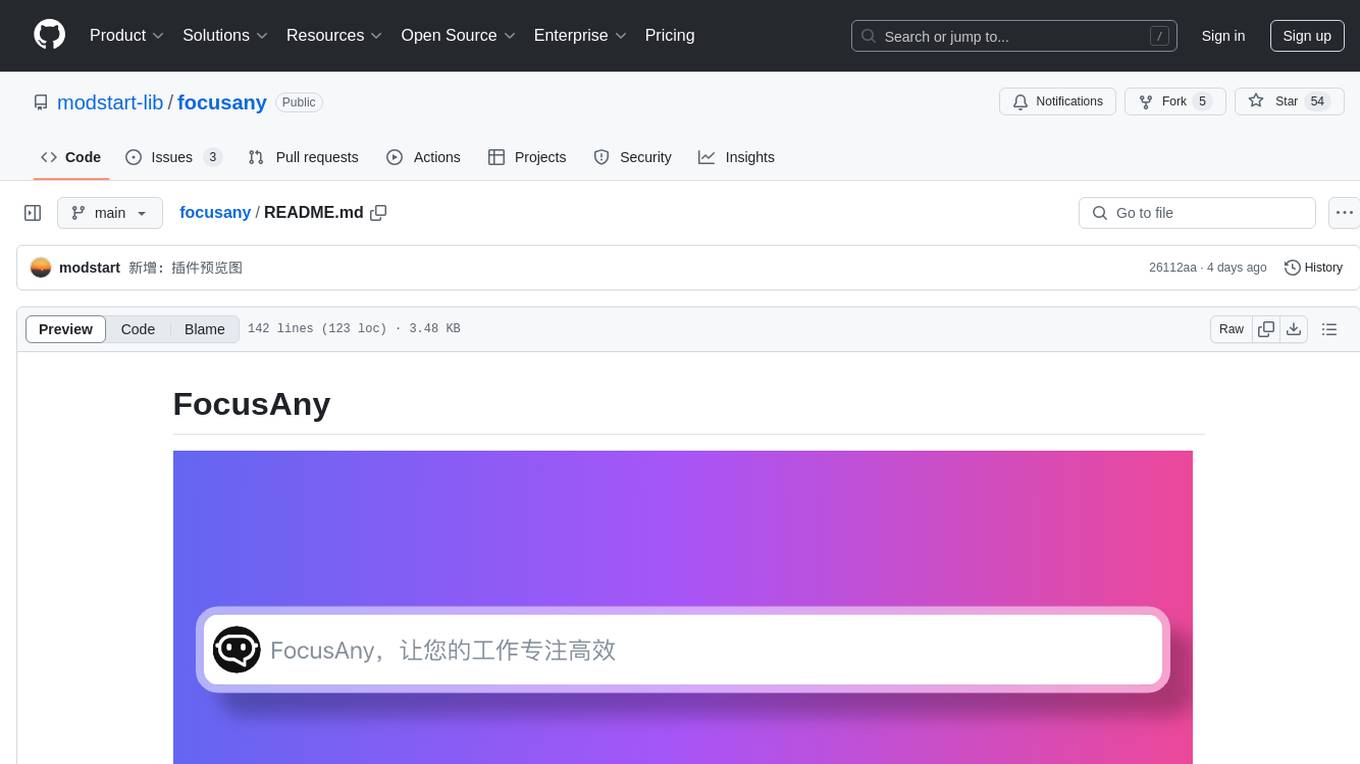
focusany
FocusAny is a desktop toolbar system that supports one-click startup of market plugins and local plugins, quickly expands functionality, and improves work efficiency. It features customizable keyboard shortcuts, plugin management, command management, quick file launching, global shortcut launching, data center for file synchronization, support for dark mode, and various plugins available in the market. The tool is built using Electron, Vue3, and TypeScript.
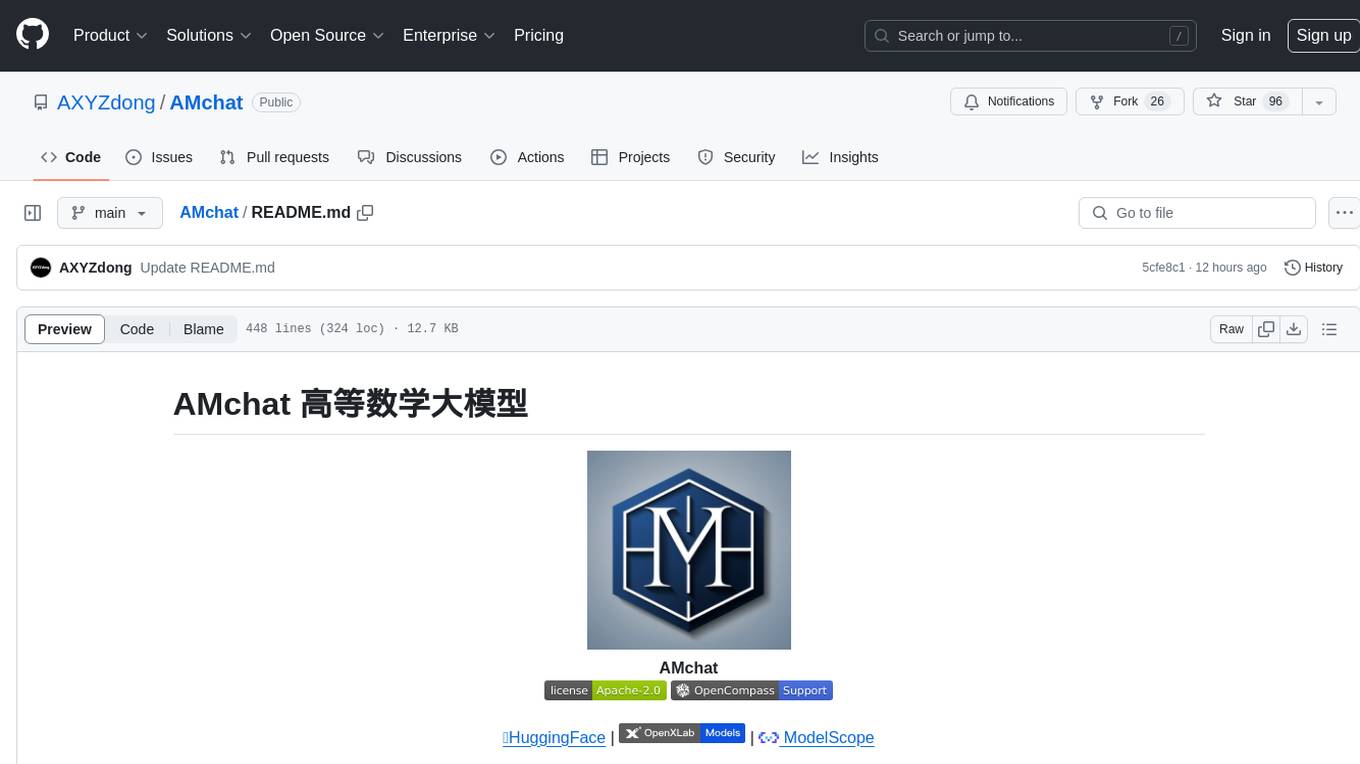
AMchat
AMchat is a large language model that integrates advanced math concepts, exercises, and solutions. The model is based on the InternLM2-Math-7B model and is specifically designed to answer advanced math problems. It provides a comprehensive dataset that combines Math and advanced math exercises and solutions. Users can download the model from ModelScope or OpenXLab, deploy it locally or using Docker, and even retrain it using XTuner for fine-tuning. The tool also supports LMDeploy for quantization, OpenCompass for evaluation, and various other features for model deployment and evaluation. The project contributors have provided detailed documentation and guides for users to utilize the tool effectively.
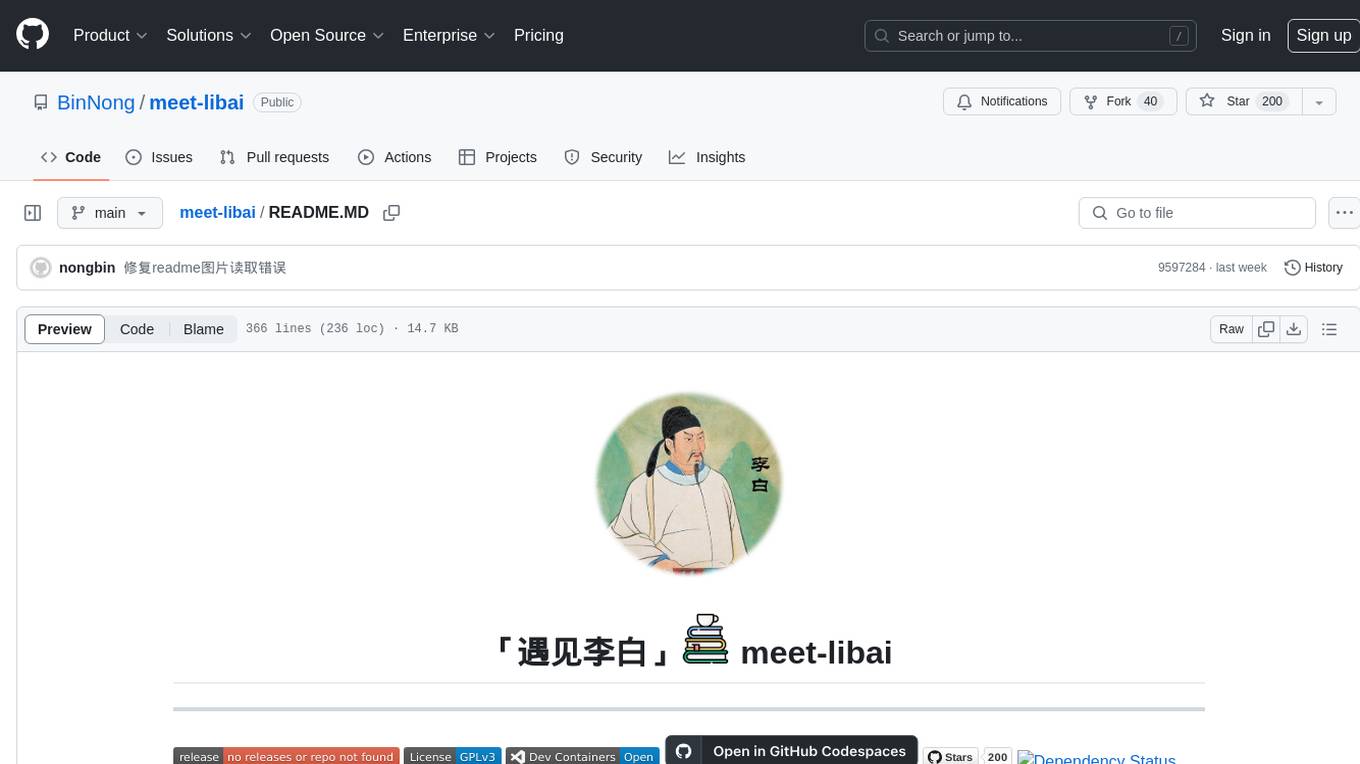
meet-libai
The 'meet-libai' project aims to promote and popularize the cultural heritage of the Chinese poet Li Bai by constructing a knowledge graph of Li Bai and training a professional AI intelligent body using large models. The project includes features such as data preprocessing, knowledge graph construction, question-answering system development, and visualization exploration of the graph structure. It also provides code implementations for large models and RAG retrieval enhancement.
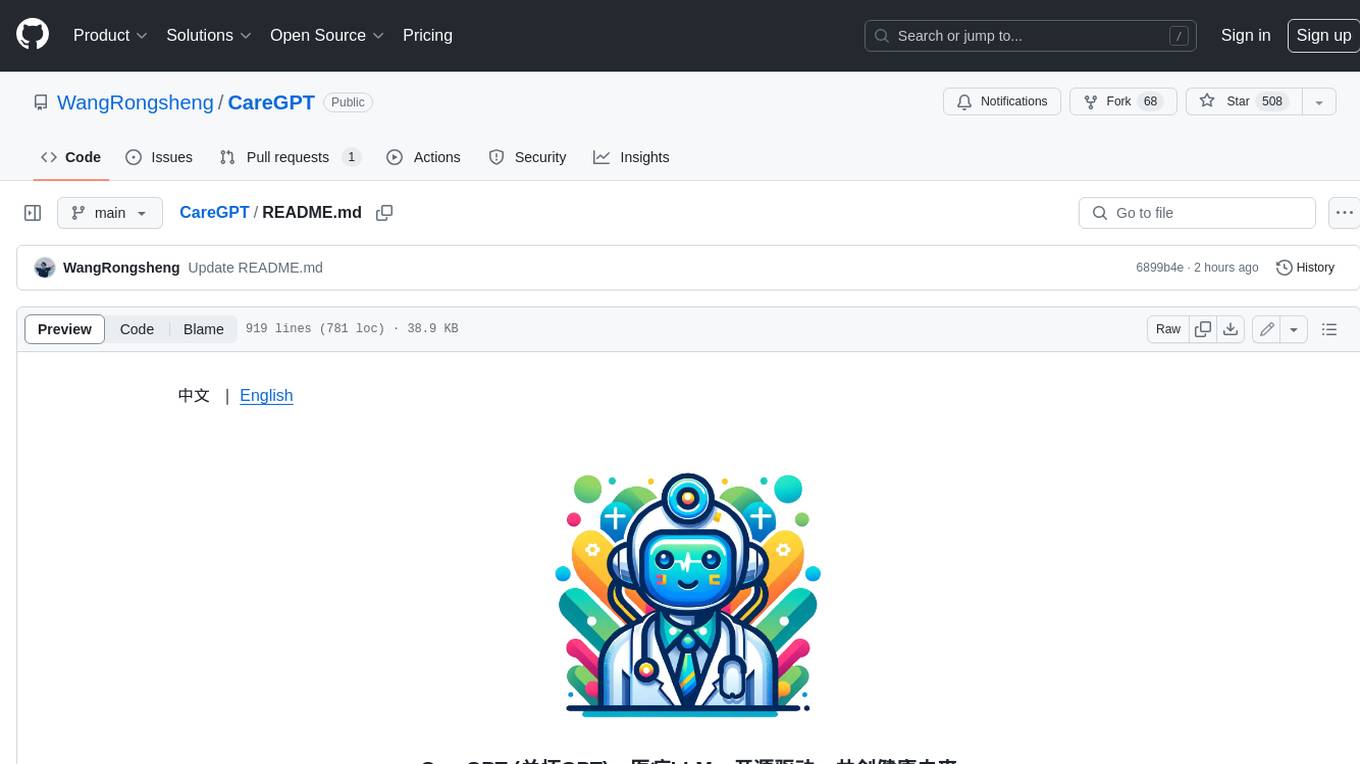
CareGPT
CareGPT is a medical large language model (LLM) that explores medical data, training, and deployment related research work. It integrates resources, open-source models, rich data, and efficient deployment methods. It supports various medical tasks, including patient diagnosis, medical dialogue, and medical knowledge integration. The model has been fine-tuned on diverse medical datasets to enhance its performance in the healthcare domain.
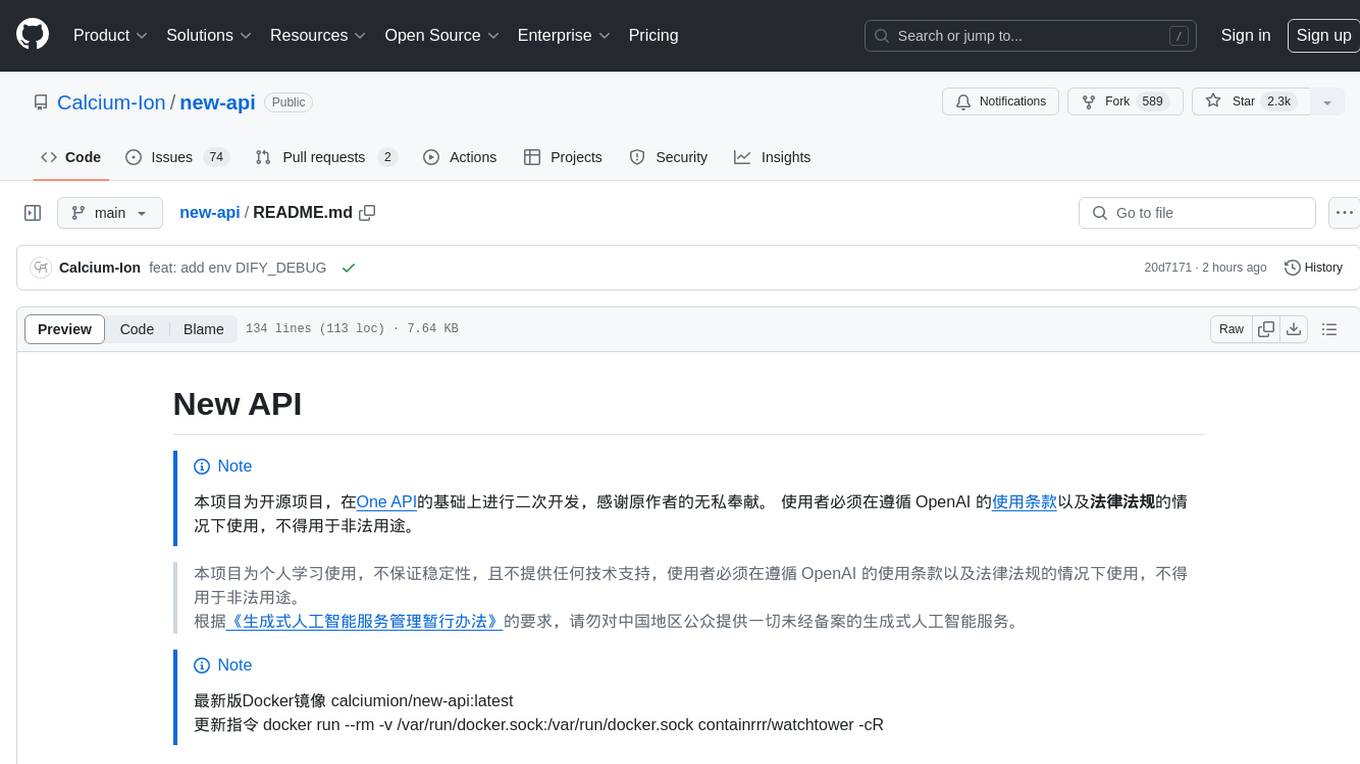
new-api
New API is an open-source project based on One API with additional features and improvements. It offers a new UI interface, supports Midjourney-Proxy(Plus) interface, online recharge functionality, model-based charging, channel weight randomization, data dashboard, token-controlled models, Telegram authorization login, Suno API support, Rerank model integration, and various third-party models. Users can customize models, retry channels, and configure caching settings. The deployment can be done using Docker with SQLite or MySQL databases. The project provides documentation for Midjourney and Suno interfaces, and it is suitable for AI enthusiasts and developers looking to enhance AI capabilities.
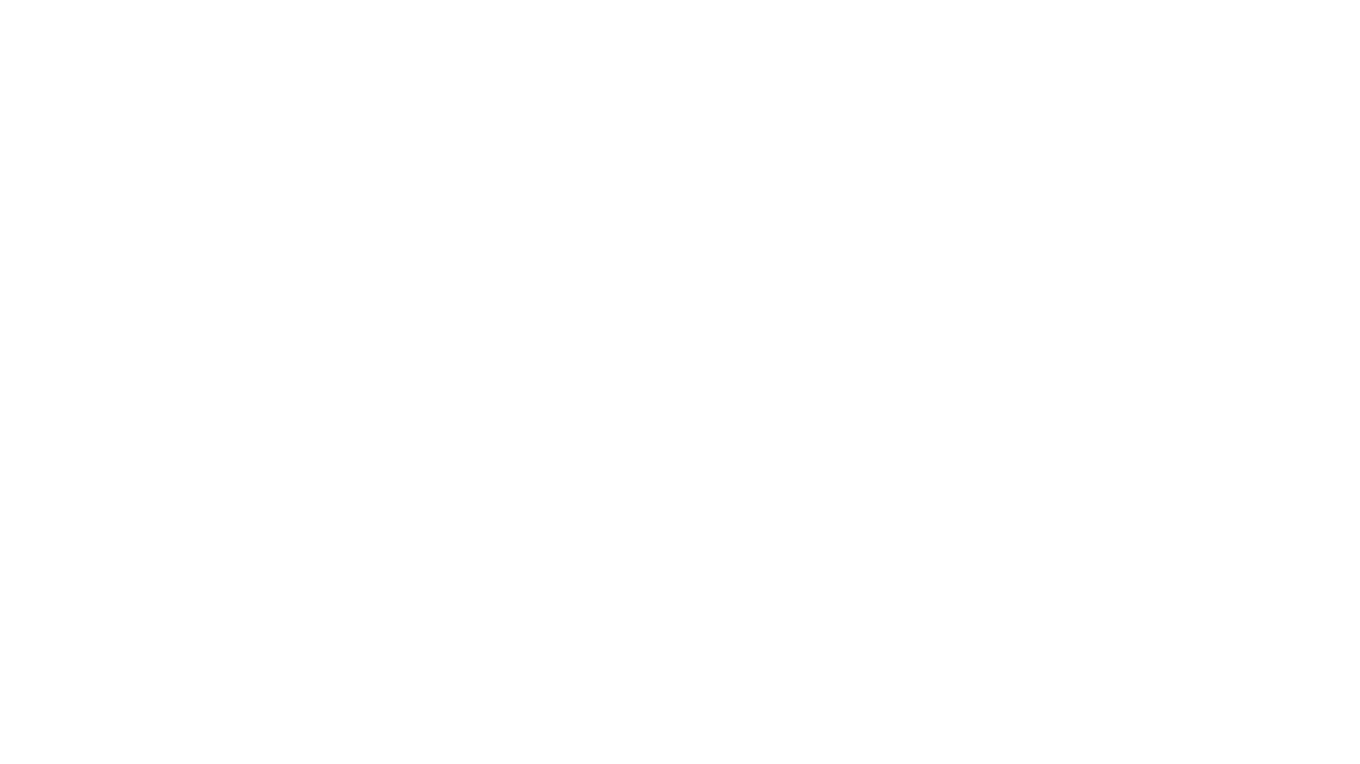
retro-aim-server
Retro AIM Server is an instant messaging server that revives AOL Instant Messenger clients from the 2000s. It supports Windows AIM client versions 5.0-5.9, away messages, buddy icons, buddy list, chat rooms, instant messaging, user profiles, blocking/visibility toggle/idle notification, and warning. The Management API provides functionality for administering the server, including listing users, creating users, changing passwords, and listing active sessions.
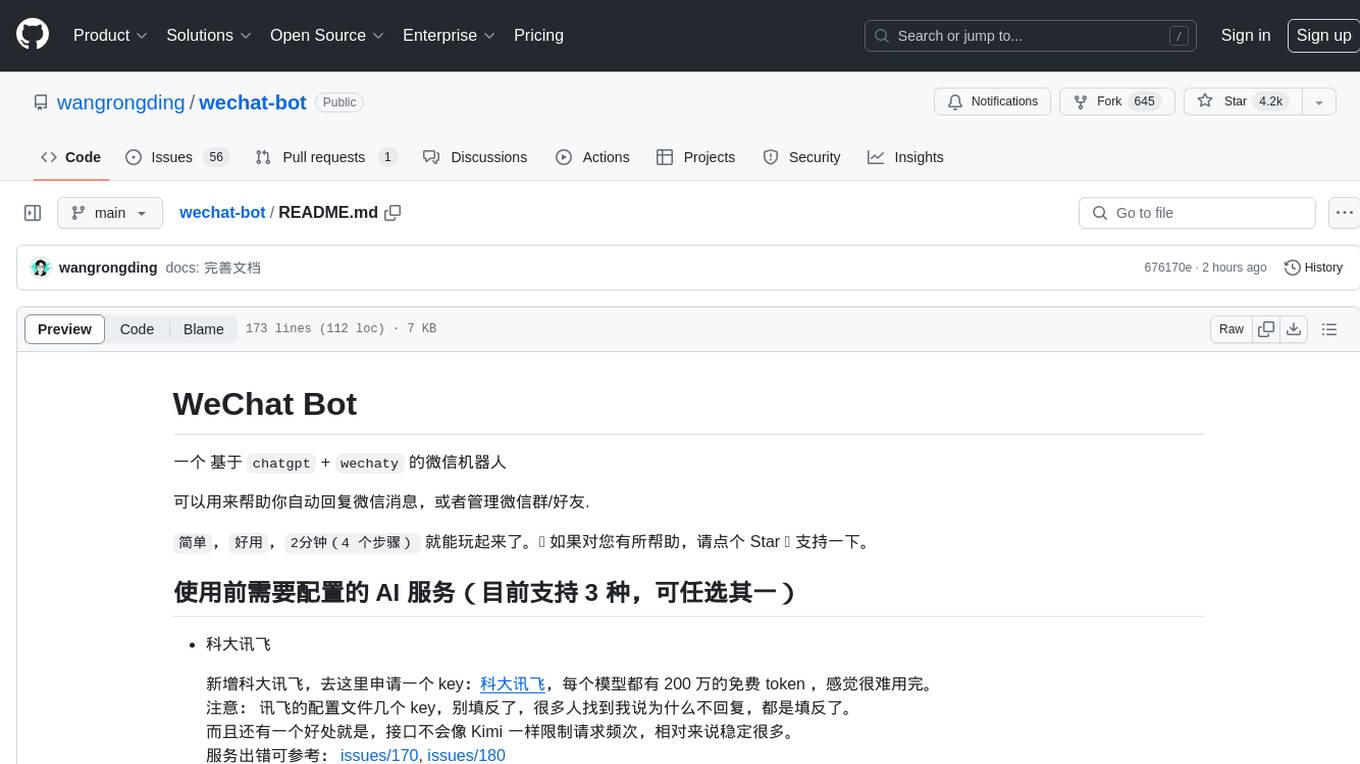
wechat-bot
WeChat Bot is a simple and easy-to-use WeChat robot based on chatgpt and wechaty. It can help you automatically reply to WeChat messages or manage WeChat groups/friends. The tool requires configuration of AI services such as Xunfei, Kimi, or ChatGPT. Users can customize the tool to automatically reply to group or private chat messages based on predefined conditions. The tool supports running in Docker for easy deployment and provides a convenient way to interact with various AI services for WeChat automation.
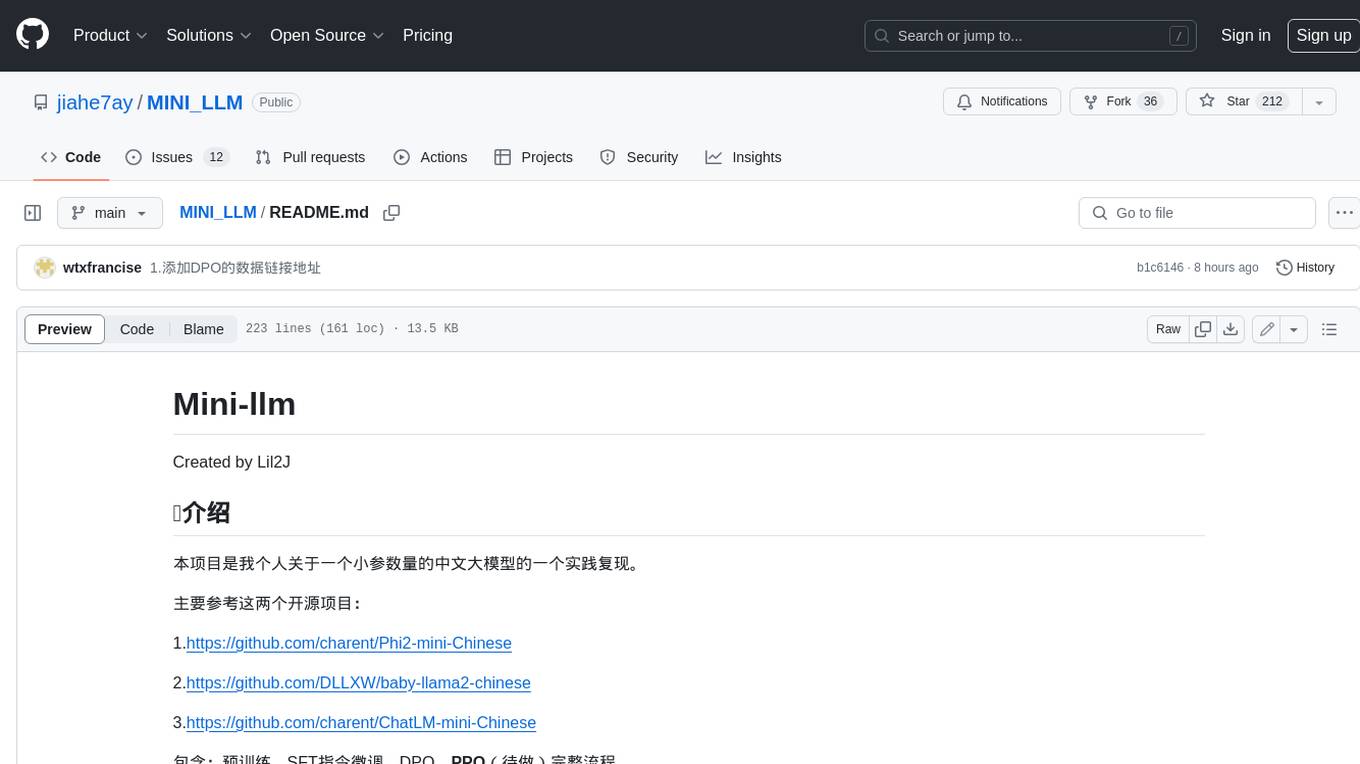
MINI_LLM
This project is a personal implementation and reproduction of a small-parameter Chinese LLM. It mainly refers to these two open source projects: https://github.com/charent/Phi2-mini-Chinese and https://github.com/DLLXW/baby-llama2-chinese. It includes the complete process of pre-training, SFT instruction fine-tuning, DPO, and PPO (to be done). I hope to share it with everyone and hope that everyone can work together to improve it!
For similar tasks
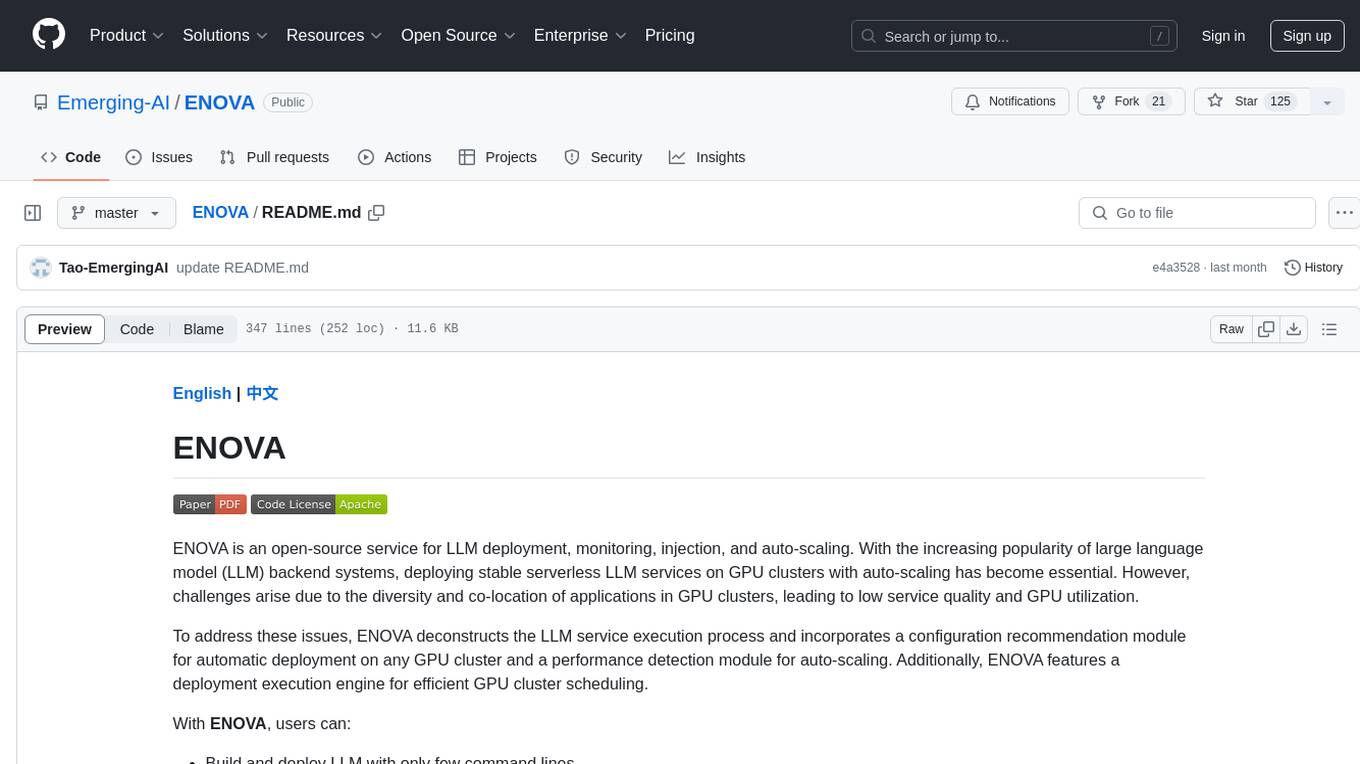
ENOVA
ENOVA is an open-source service for Large Language Model (LLM) deployment, monitoring, injection, and auto-scaling. It addresses challenges in deploying stable serverless LLM services on GPU clusters with auto-scaling by deconstructing the LLM service execution process and providing configuration recommendations and performance detection. Users can build and deploy LLM with few command lines, recommend optimal computing resources, experience LLM performance, observe operating status, achieve load balancing, and more. ENOVA ensures stable operation, cost-effectiveness, efficiency, and strong scalability of LLM services.
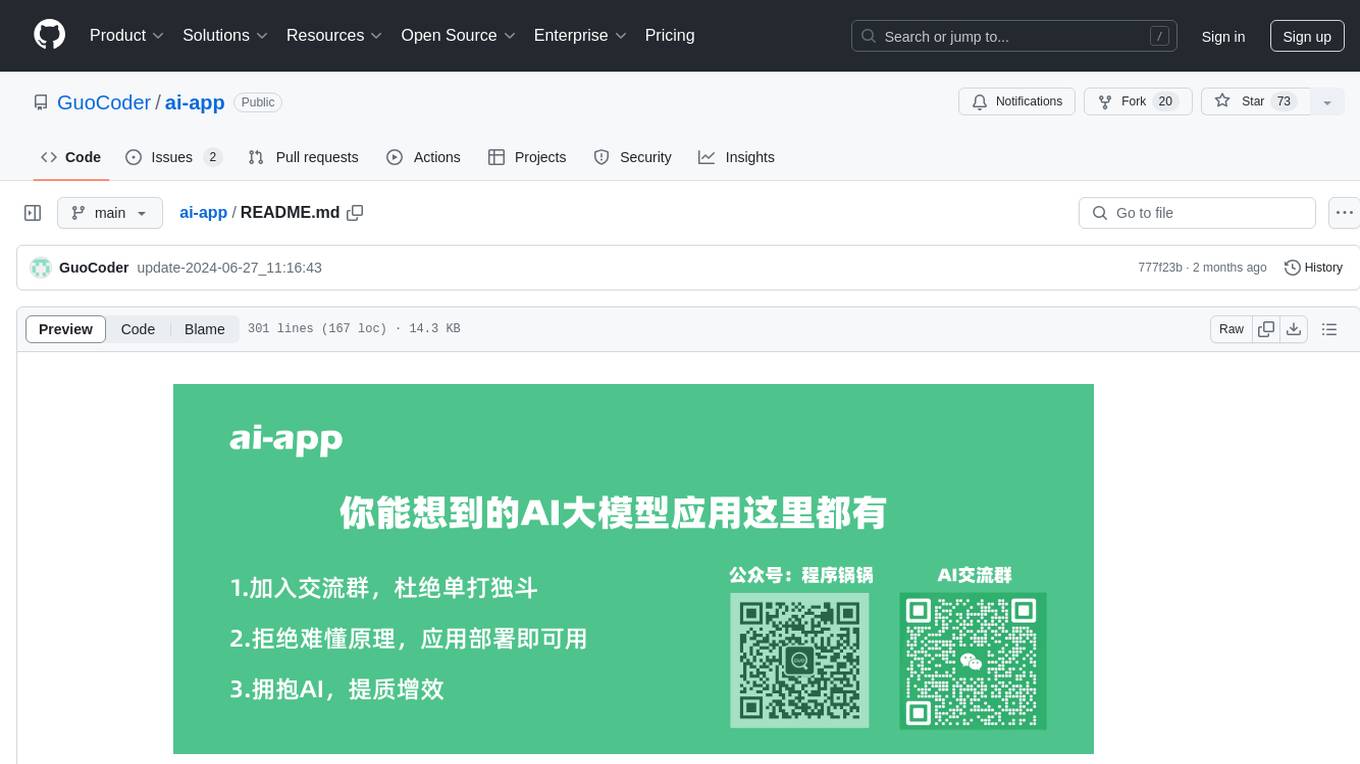
ai-app
The 'ai-app' repository is a comprehensive collection of tools and resources related to artificial intelligence, focusing on topics such as server environment setup, PyCharm and Anaconda installation, large model deployment and training, Transformer principles, RAG technology, vector databases, AI image, voice, and music generation, and AI Agent frameworks. It also includes practical guides and tutorials on implementing various AI applications. The repository serves as a valuable resource for individuals interested in exploring different aspects of AI technology.
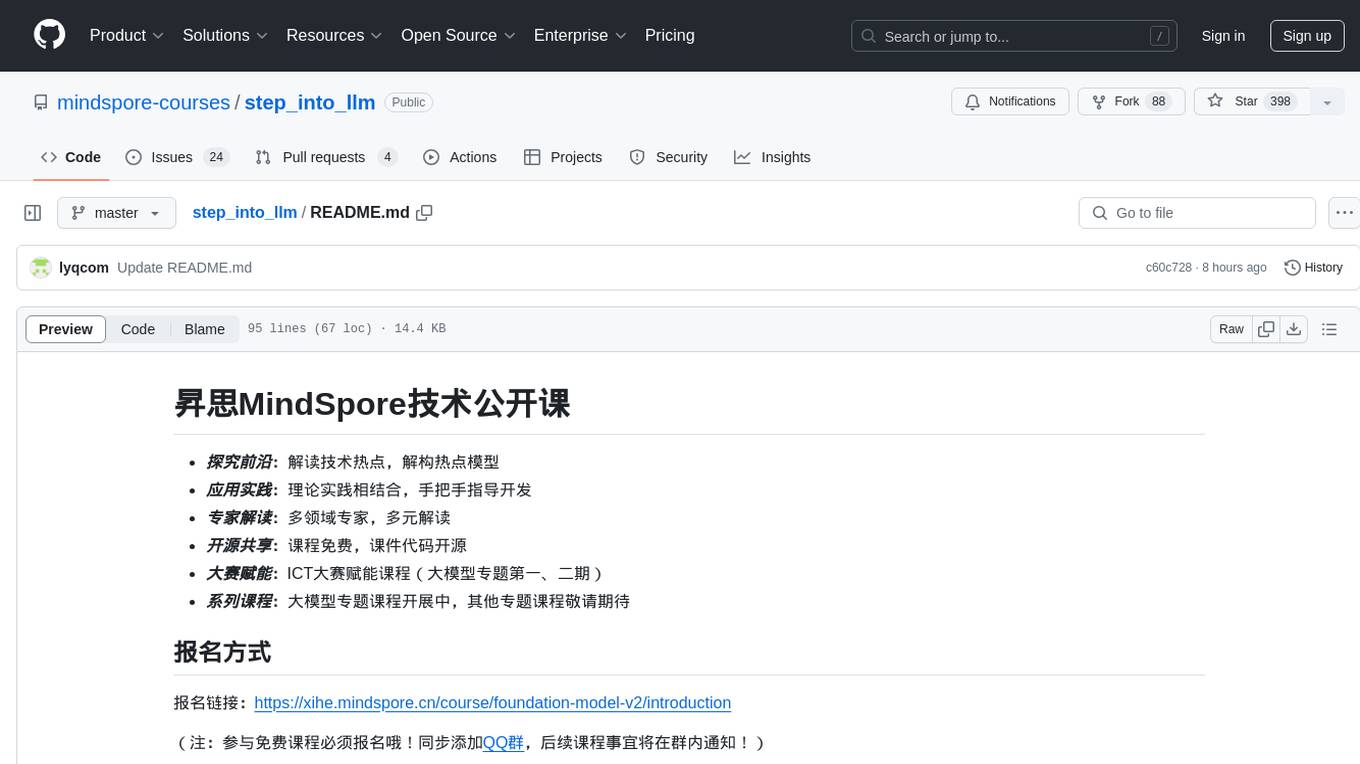
step_into_llm
The 'step_into_llm' repository is dedicated to the 昇思MindSpore technology open class, which focuses on exploring cutting-edge technologies, combining theory with practical applications, expert interpretations, open sharing, and empowering competitions. The repository contains course materials, including slides and code, for the ongoing second phase of the course. It covers various topics related to large language models (LLMs) such as Transformer, BERT, GPT, GPT2, and more. The course aims to guide developers interested in LLMs from theory to practical implementation, with a special emphasis on the development and application of large models.
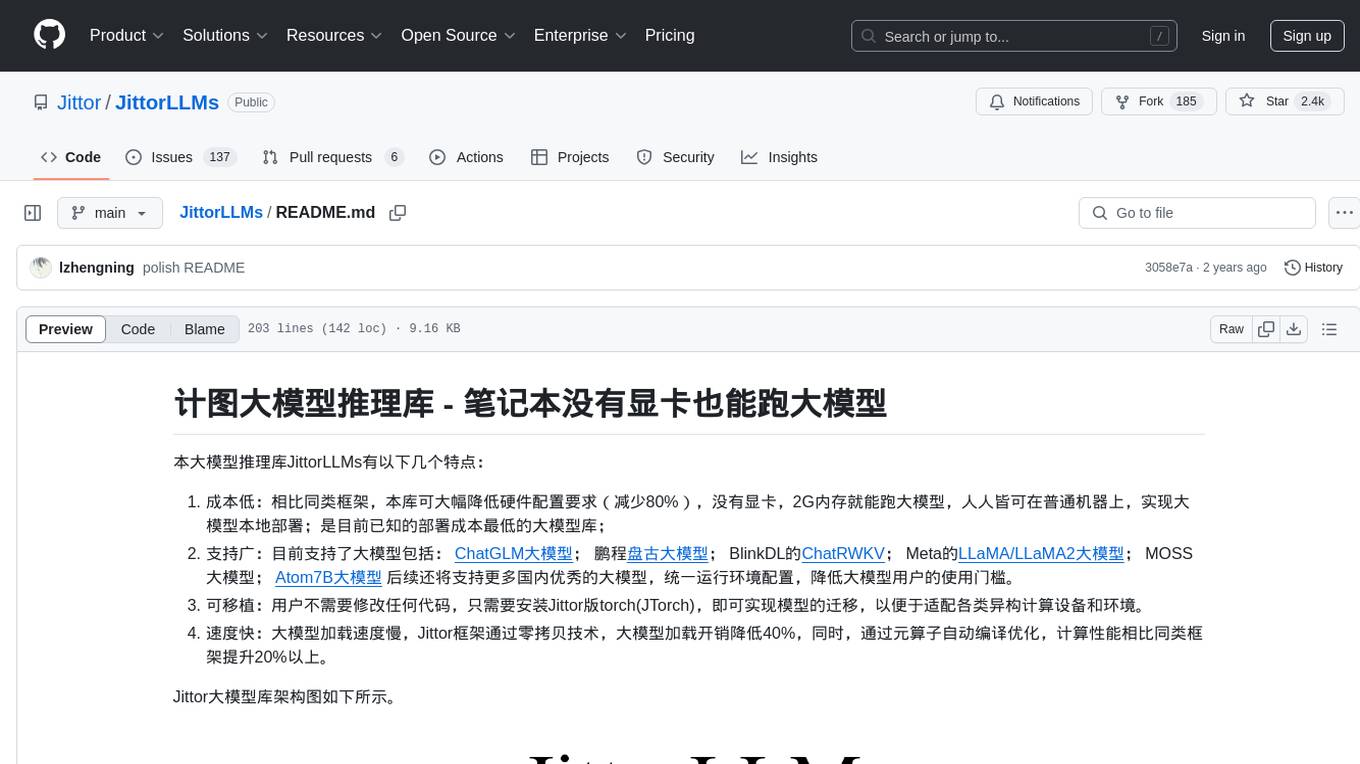
JittorLLMs
JittorLLMs is a large model inference library that allows running large models on machines with low hardware requirements. It significantly reduces hardware configuration demands, enabling deployment on ordinary machines with 2GB of memory. It supports various large models and provides a unified environment configuration for users. Users can easily migrate models without modifying any code by installing Jittor version of torch (JTorch). The framework offers fast model loading speed, optimized computation performance, and portability across different computing devices and environments.
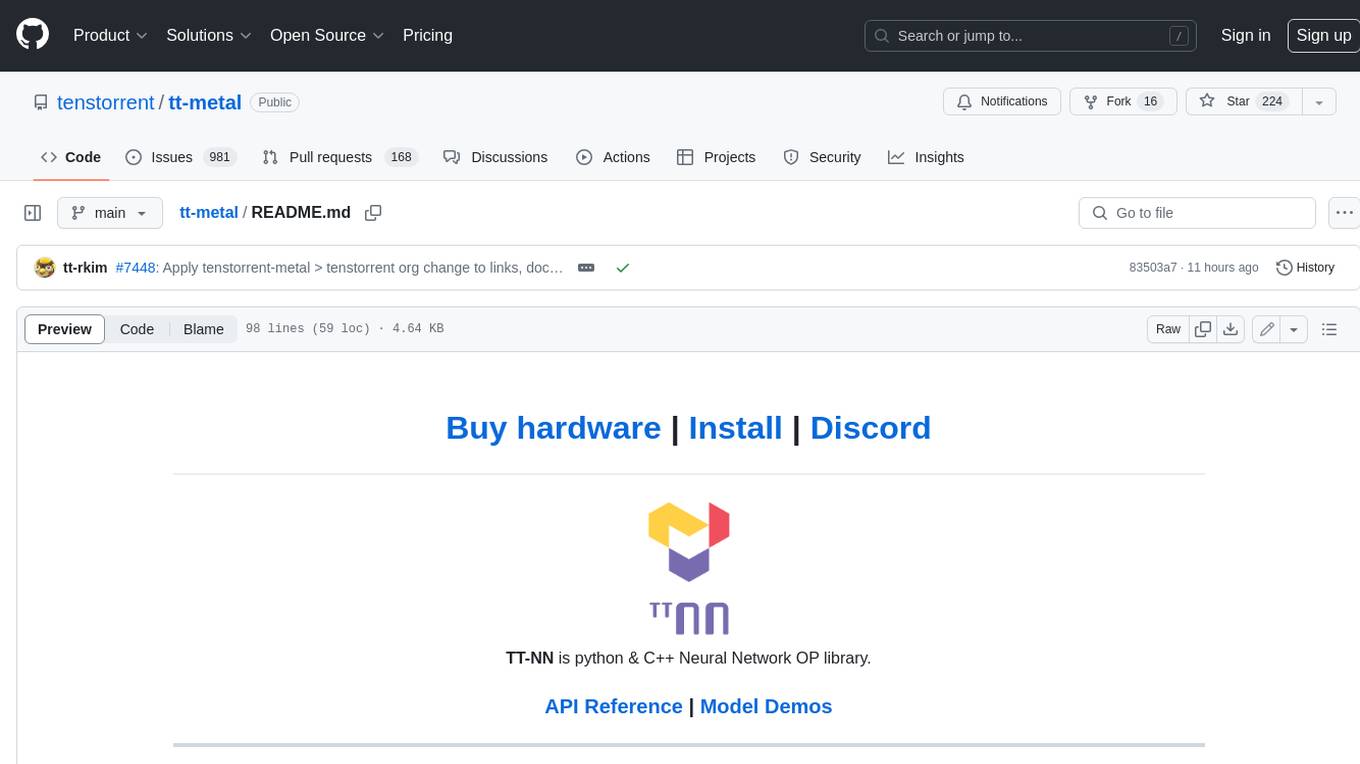
tt-metal
TT-NN is a python & C++ Neural Network OP library. It provides a low-level programming model, TT-Metalium, enabling kernel development for Tenstorrent hardware.
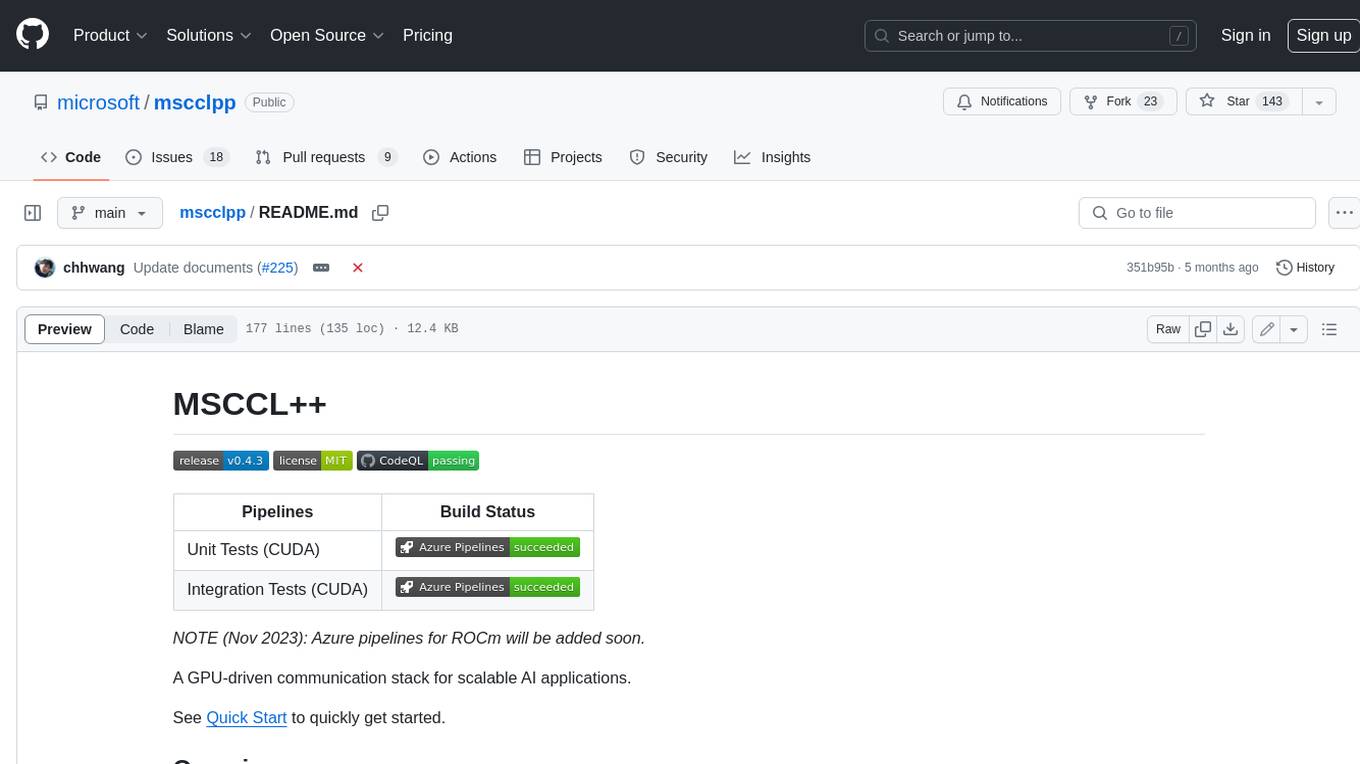
mscclpp
MSCCL++ is a GPU-driven communication stack for scalable AI applications. It provides a highly efficient and customizable communication stack for distributed GPU applications. MSCCL++ redefines inter-GPU communication interfaces, delivering a highly efficient and customizable communication stack for distributed GPU applications. Its design is specifically tailored to accommodate diverse performance optimization scenarios often encountered in state-of-the-art AI applications. MSCCL++ provides communication abstractions at the lowest level close to hardware and at the highest level close to application API. The lowest level of abstraction is ultra light weight which enables a user to implement logics of data movement for a collective operation such as AllReduce inside a GPU kernel extremely efficiently without worrying about memory ordering of different ops. The modularity of MSCCL++ enables a user to construct the building blocks of MSCCL++ in a high level abstraction in Python and feed them to a CUDA kernel in order to facilitate the user's productivity. MSCCL++ provides fine-grained synchronous and asynchronous 0-copy 1-sided abstracts for communication primitives such as `put()`, `get()`, `signal()`, `flush()`, and `wait()`. The 1-sided abstractions allows a user to asynchronously `put()` their data on the remote GPU as soon as it is ready without requiring the remote side to issue any receive instruction. This enables users to easily implement flexible communication logics, such as overlapping communication with computation, or implementing customized collective communication algorithms without worrying about potential deadlocks. Additionally, the 0-copy capability enables MSCCL++ to directly transfer data between user's buffers without using intermediate internal buffers which saves GPU bandwidth and memory capacity. MSCCL++ provides consistent abstractions regardless of the location of the remote GPU (either on the local node or on a remote node) or the underlying link (either NVLink/xGMI or InfiniBand). This simplifies the code for inter-GPU communication, which is often complex due to memory ordering of GPU/CPU read/writes and therefore, is error-prone.
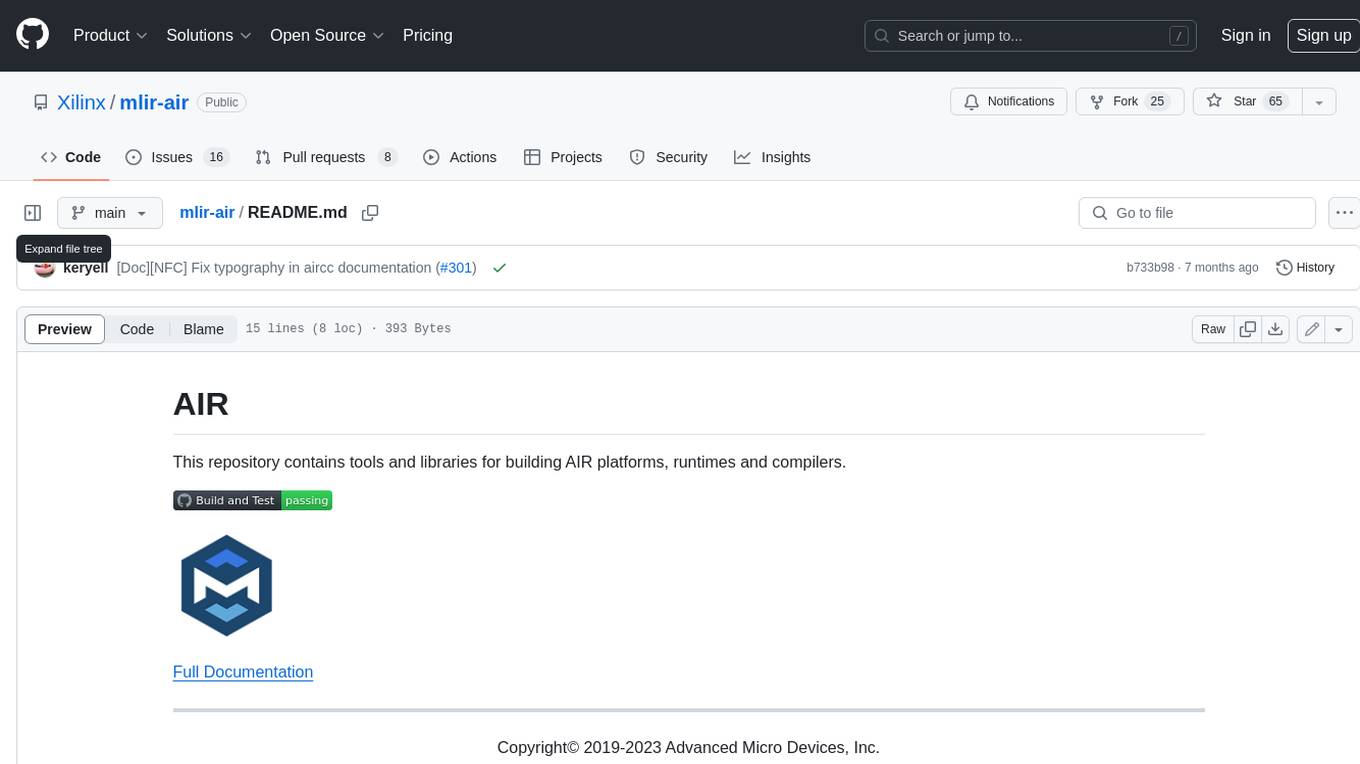
mlir-air
This repository contains tools and libraries for building AIR platforms, runtimes and compilers.
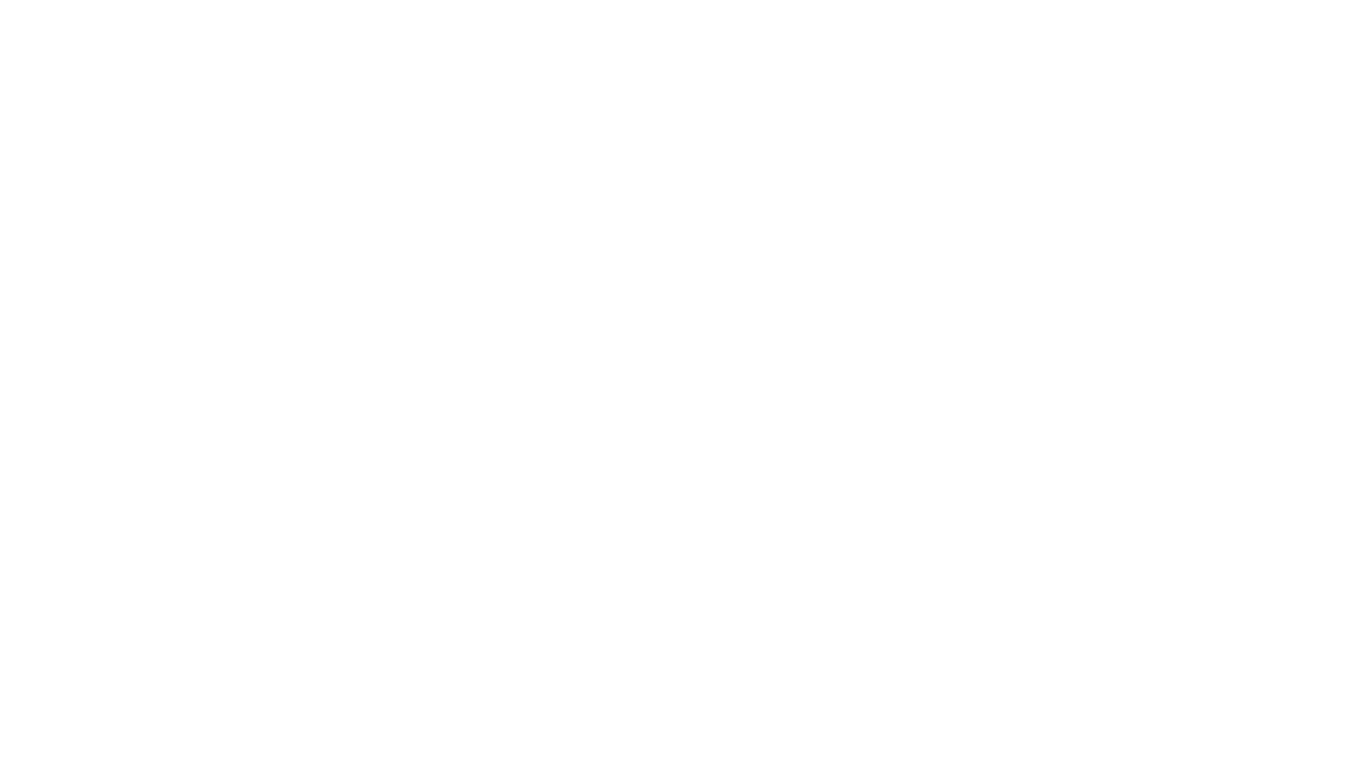
free-for-life
A massive list including a huge amount of products and services that are completely free! ⭐ Star on GitHub • 🤝 Contribute # Table of Contents * APIs, Data & ML * Artificial Intelligence * BaaS * Code Editors * Code Generation * DNS * Databases * Design & UI * Domains * Email * Font * For Students * Forms * Linux Distributions * Messaging & Streaming * PaaS * Payments & Billing * SSL
For similar jobs
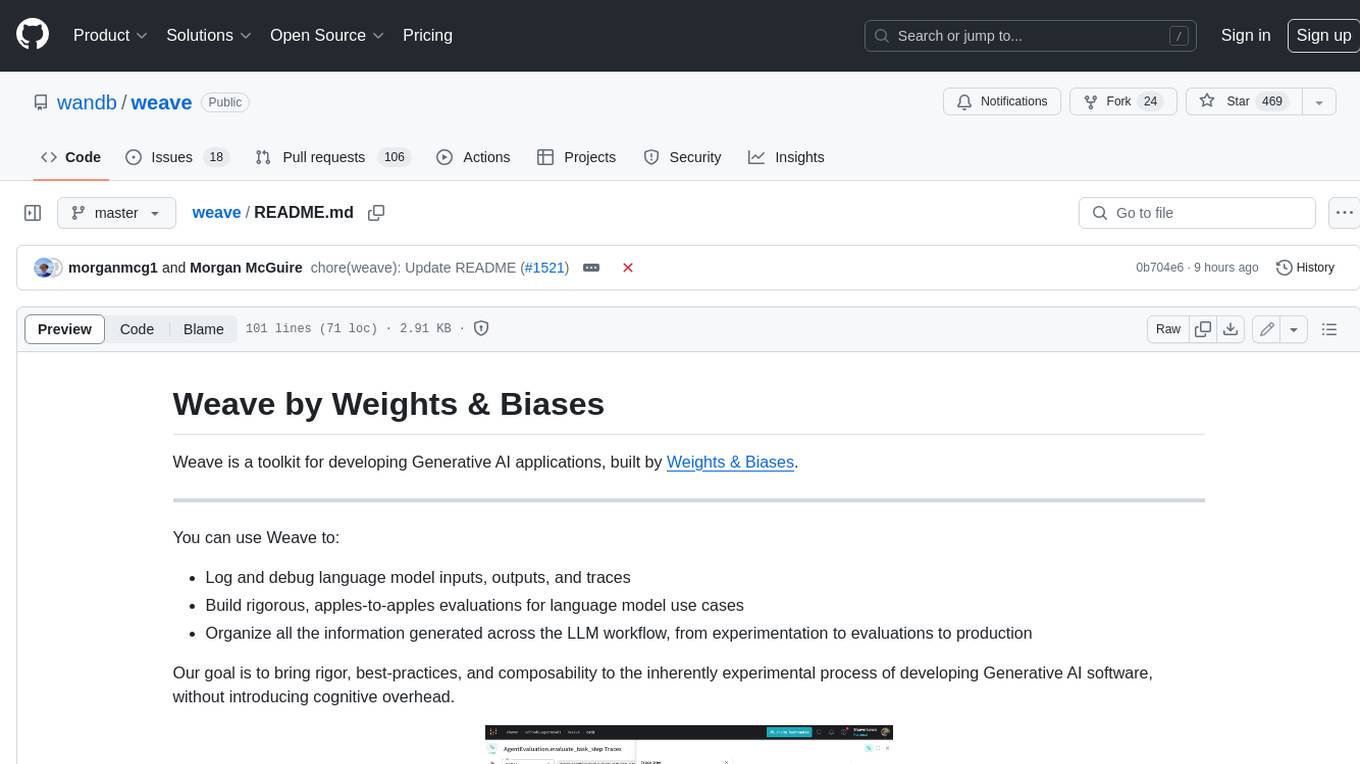
weave
Weave is a toolkit for developing Generative AI applications, built by Weights & Biases. With Weave, you can log and debug language model inputs, outputs, and traces; build rigorous, apples-to-apples evaluations for language model use cases; and organize all the information generated across the LLM workflow, from experimentation to evaluations to production. Weave aims to bring rigor, best-practices, and composability to the inherently experimental process of developing Generative AI software, without introducing cognitive overhead.
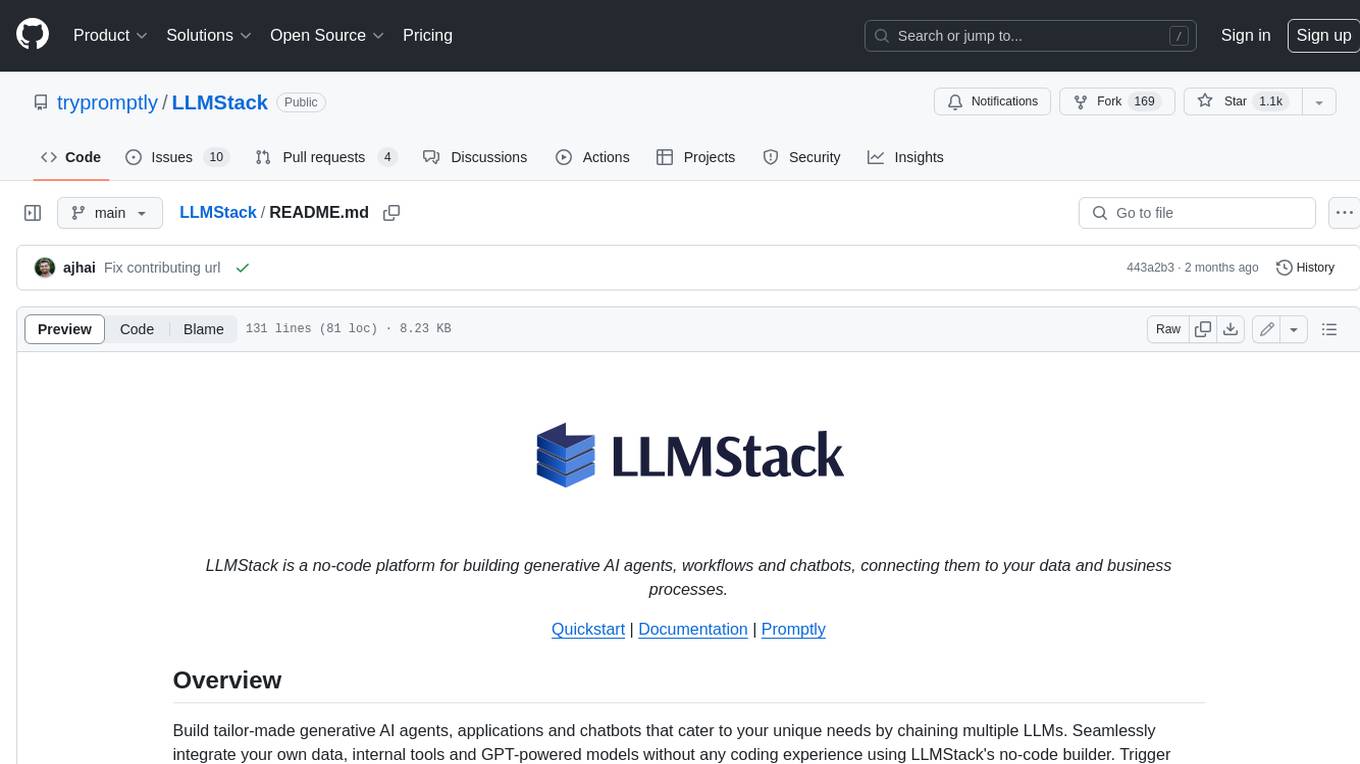
LLMStack
LLMStack is a no-code platform for building generative AI agents, workflows, and chatbots. It allows users to connect their own data, internal tools, and GPT-powered models without any coding experience. LLMStack can be deployed to the cloud or on-premise and can be accessed via HTTP API or triggered from Slack or Discord.
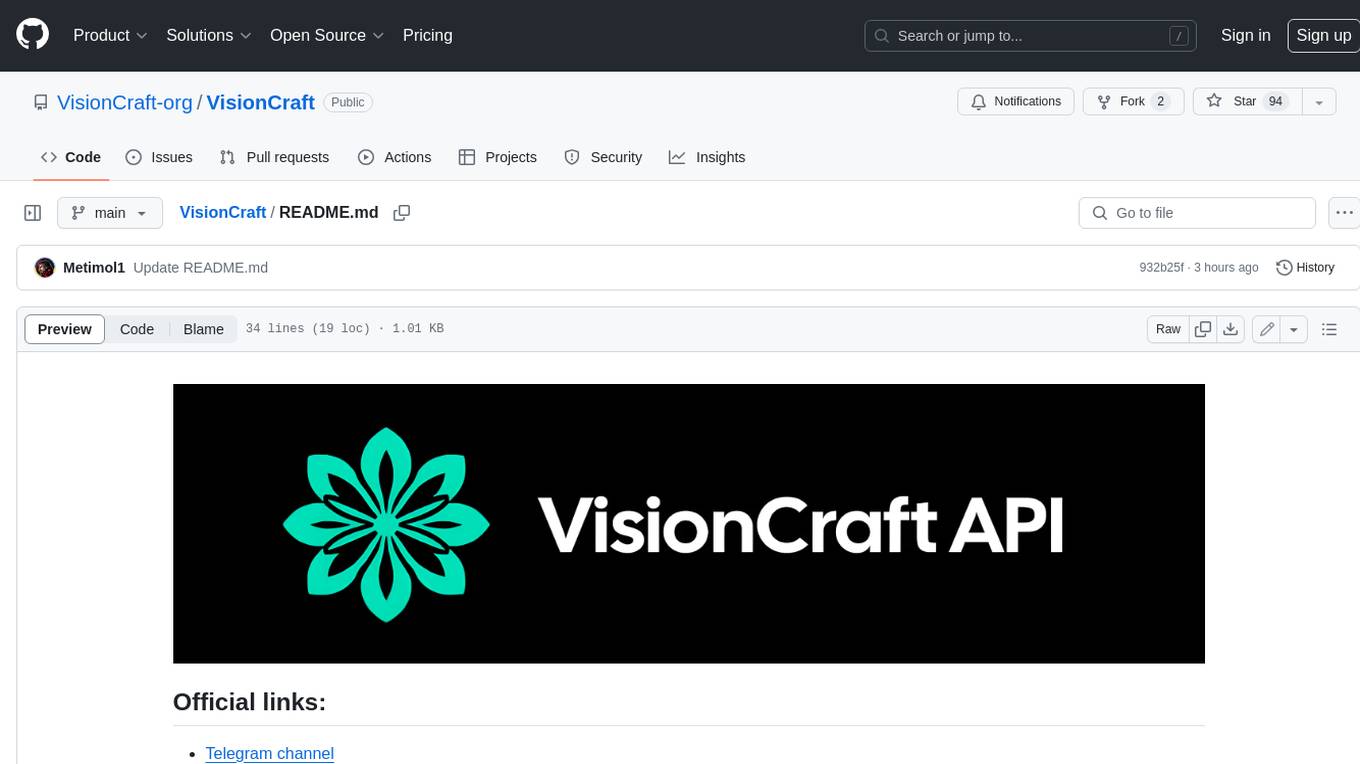
VisionCraft
The VisionCraft API is a free API for using over 100 different AI models. From images to sound.
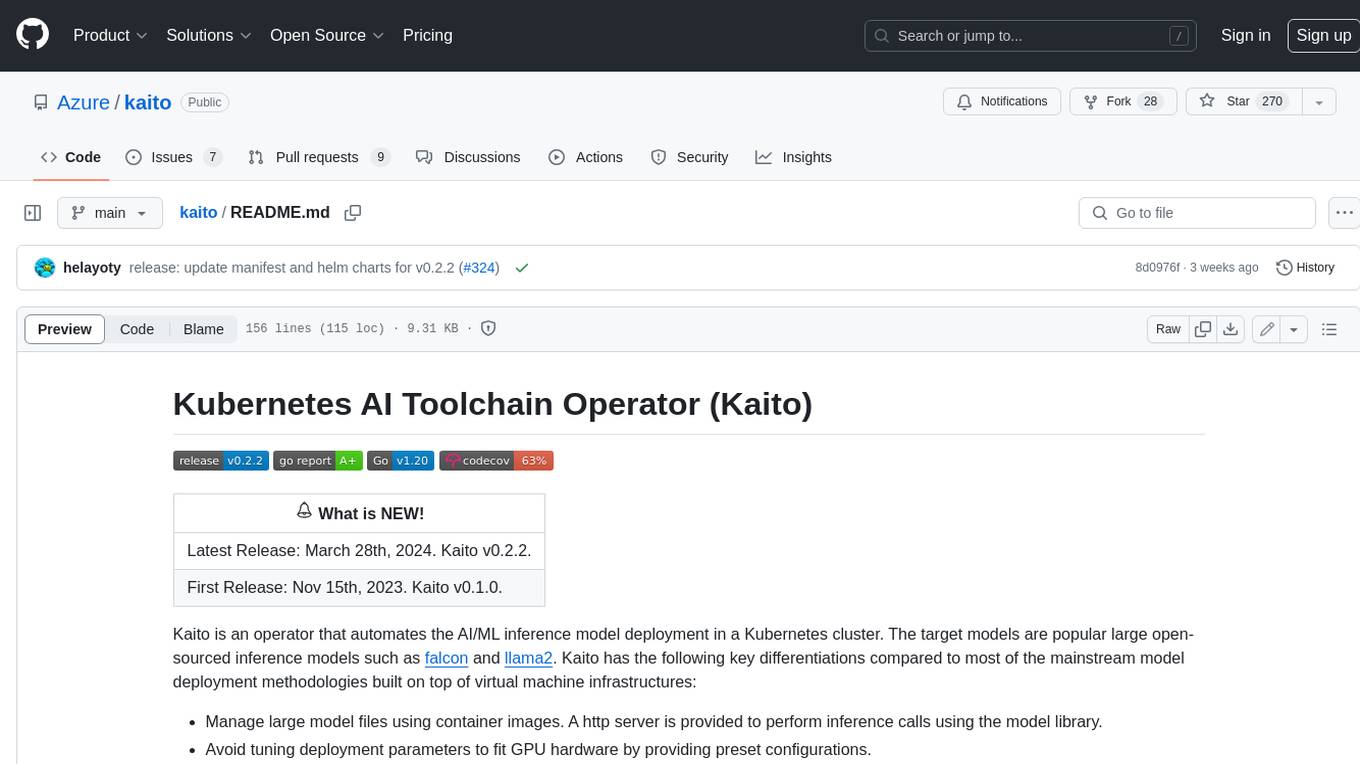
kaito
Kaito is an operator that automates the AI/ML inference model deployment in a Kubernetes cluster. It manages large model files using container images, avoids tuning deployment parameters to fit GPU hardware by providing preset configurations, auto-provisions GPU nodes based on model requirements, and hosts large model images in the public Microsoft Container Registry (MCR) if the license allows. Using Kaito, the workflow of onboarding large AI inference models in Kubernetes is largely simplified.
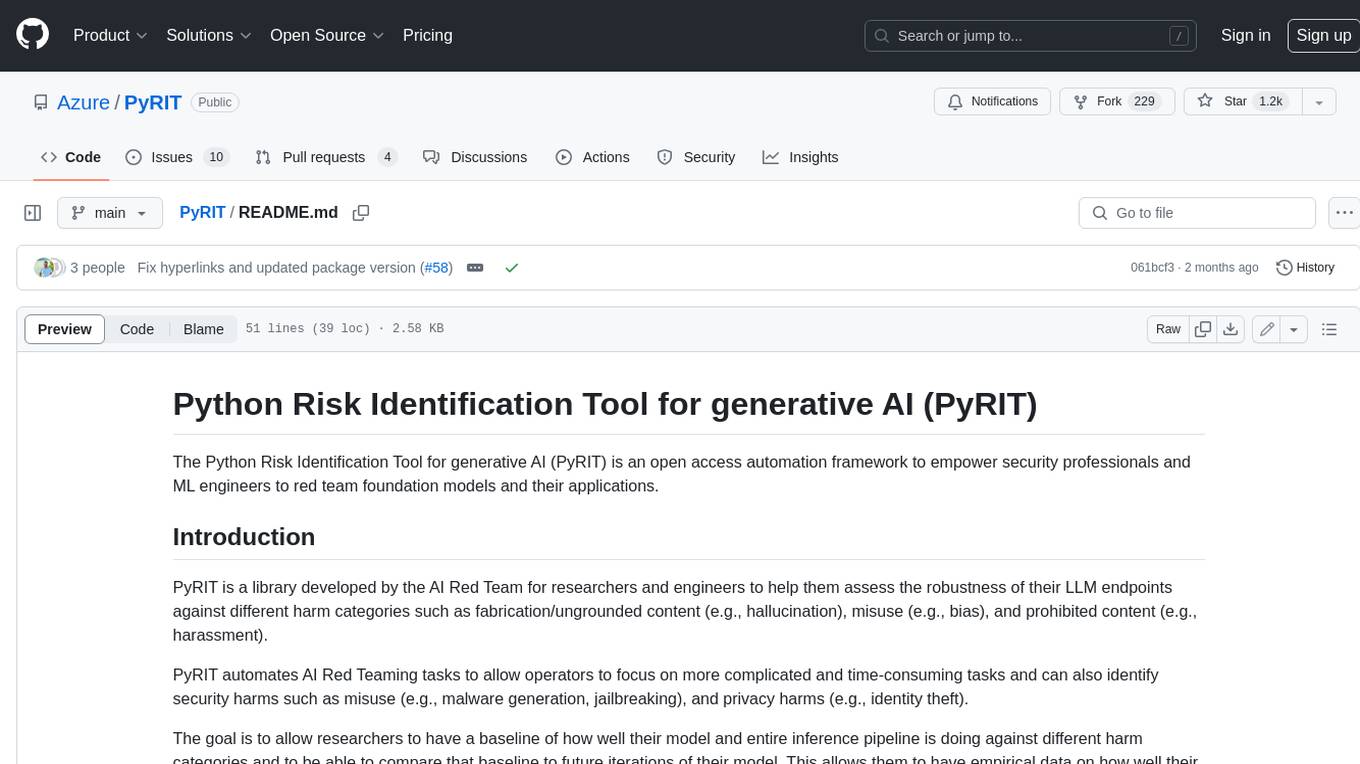
PyRIT
PyRIT is an open access automation framework designed to empower security professionals and ML engineers to red team foundation models and their applications. It automates AI Red Teaming tasks to allow operators to focus on more complicated and time-consuming tasks and can also identify security harms such as misuse (e.g., malware generation, jailbreaking), and privacy harms (e.g., identity theft). The goal is to allow researchers to have a baseline of how well their model and entire inference pipeline is doing against different harm categories and to be able to compare that baseline to future iterations of their model. This allows them to have empirical data on how well their model is doing today, and detect any degradation of performance based on future improvements.
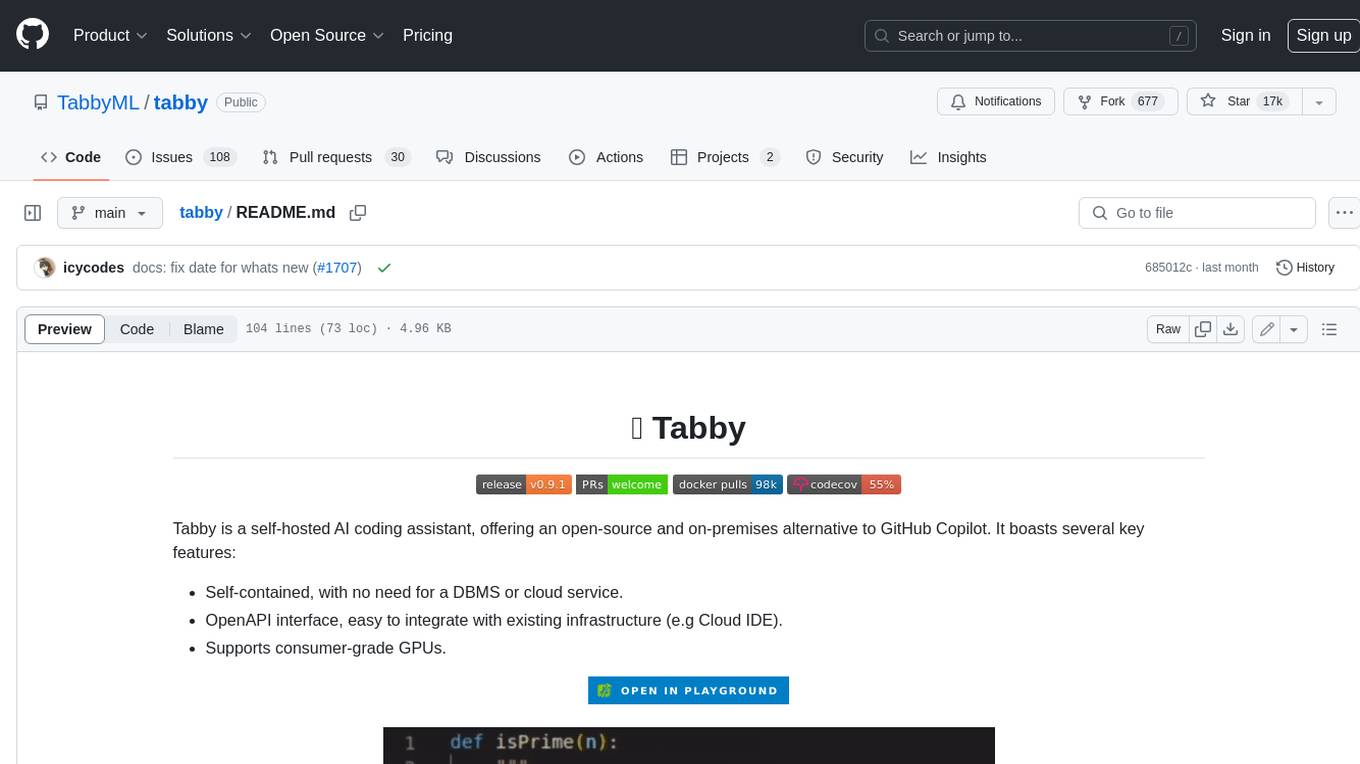
tabby
Tabby is a self-hosted AI coding assistant, offering an open-source and on-premises alternative to GitHub Copilot. It boasts several key features: * Self-contained, with no need for a DBMS or cloud service. * OpenAPI interface, easy to integrate with existing infrastructure (e.g Cloud IDE). * Supports consumer-grade GPUs.
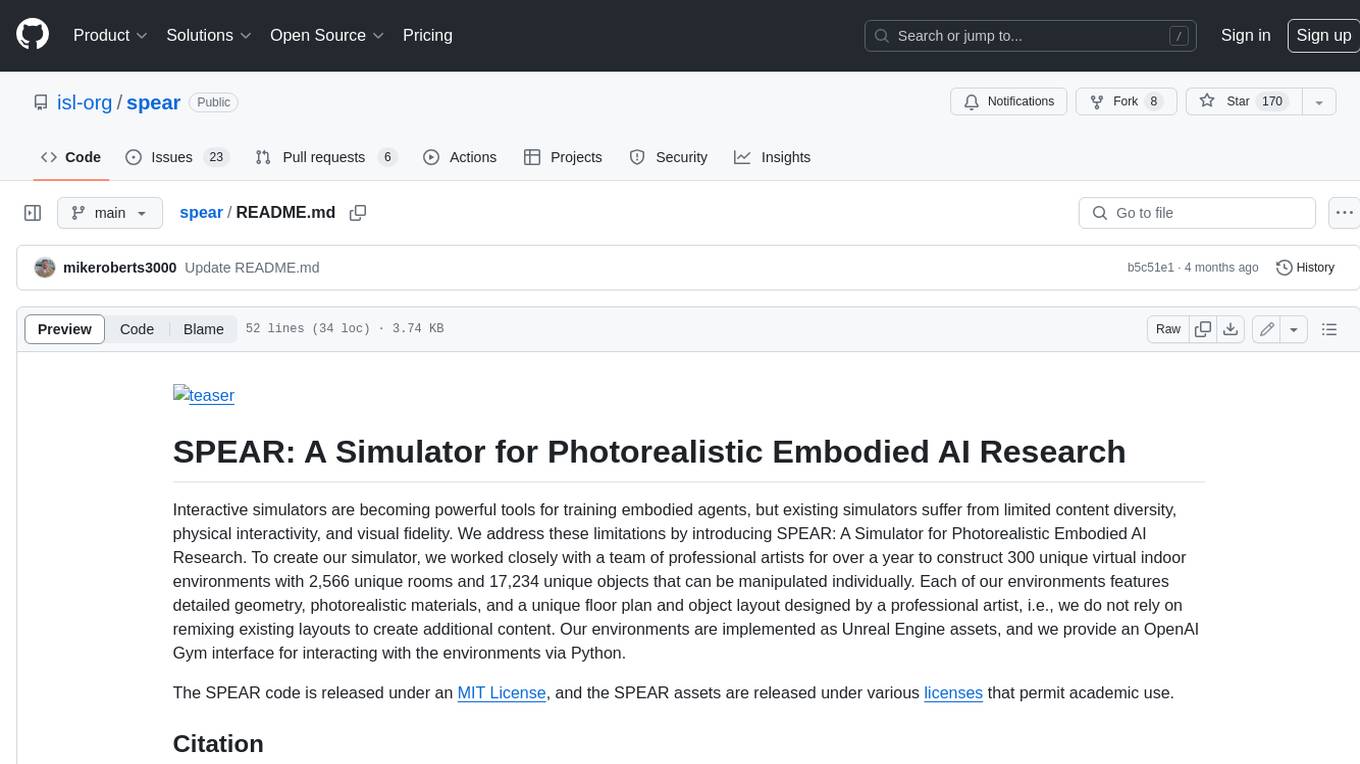
spear
SPEAR (Simulator for Photorealistic Embodied AI Research) is a powerful tool for training embodied agents. It features 300 unique virtual indoor environments with 2,566 unique rooms and 17,234 unique objects that can be manipulated individually. Each environment is designed by a professional artist and features detailed geometry, photorealistic materials, and a unique floor plan and object layout. SPEAR is implemented as Unreal Engine assets and provides an OpenAI Gym interface for interacting with the environments via Python.
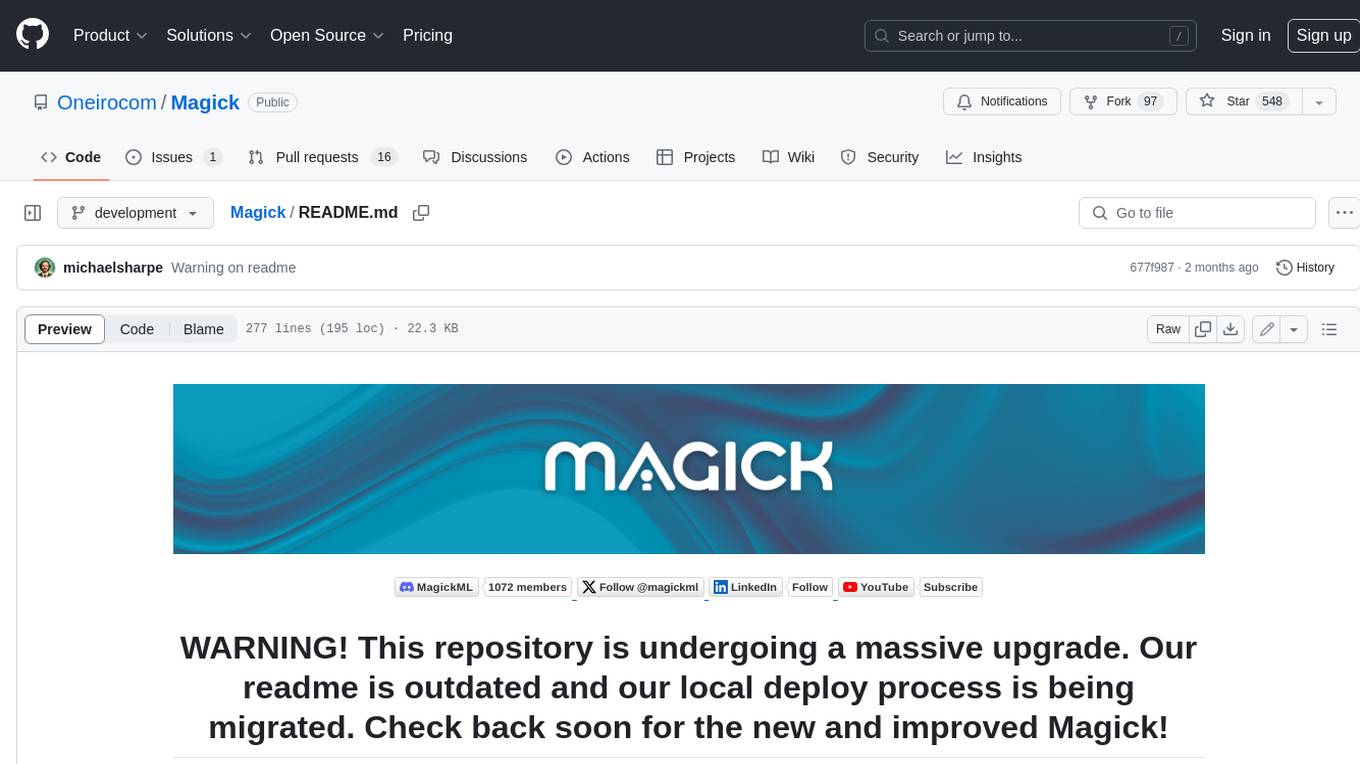
Magick
Magick is a groundbreaking visual AIDE (Artificial Intelligence Development Environment) for no-code data pipelines and multimodal agents. Magick can connect to other services and comes with nodes and templates well-suited for intelligent agents, chatbots, complex reasoning systems and realistic characters.