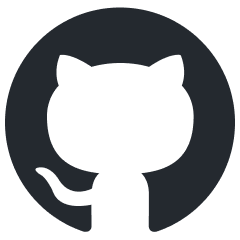
DeepMesh
Official code of DeepMesh: Auto-Regressive Artist-mesh Creation with Reinforcement Learning
Stars: 350
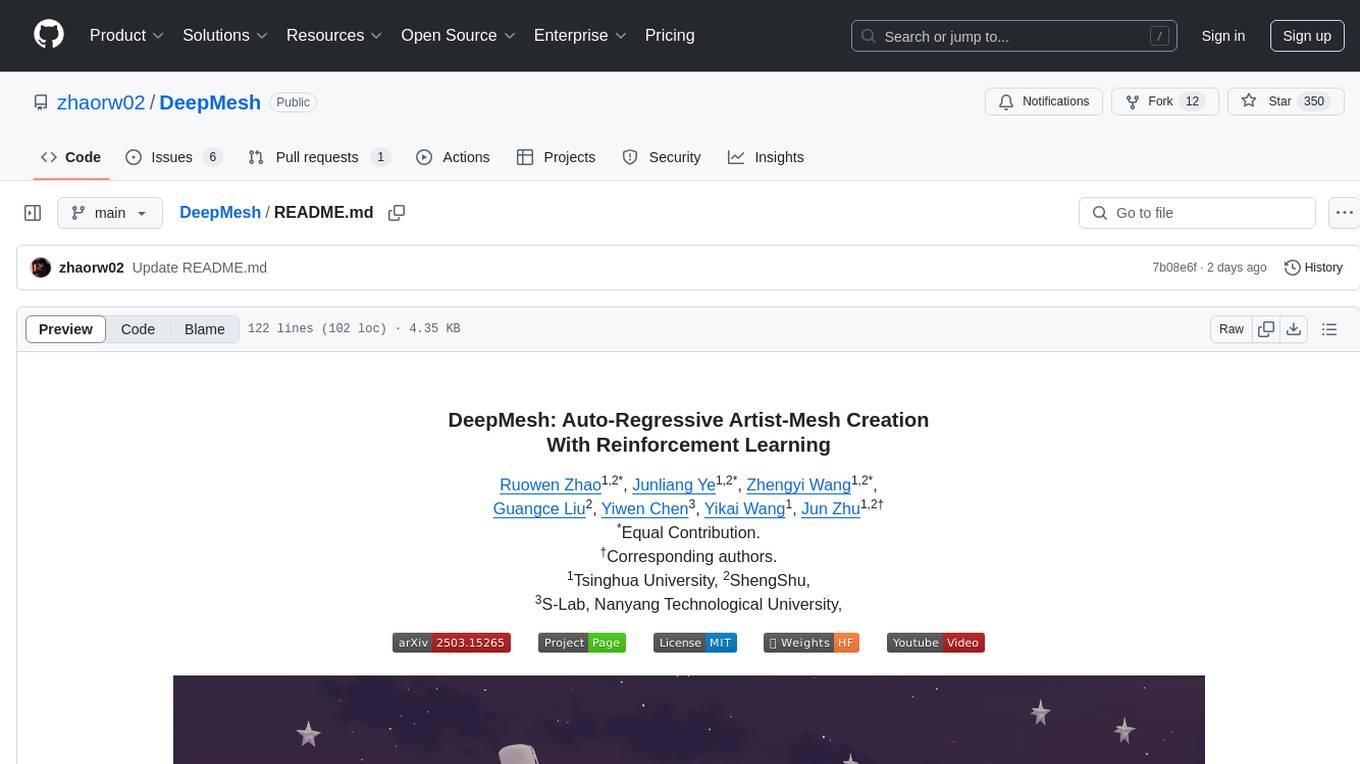
README:
Ruowen Zhao1,2*,
Junliang Ye1,2*,
Zhengyi Wang1,2*,
Guangce Liu2,
Yiwen Chen3,
Yikai Wang1,
Jun Zhu1,2†
*Equal Contribution.
†Corresponding authors.
1Tsinghua University,
2ShengShu,
3S-Lab, Nanyang Technological University,
All of the meshes above are generated by DeepMesh. DeepMesh can generate high-quality meshes conditioned on the given point cloud by auto-regressive transformer.
- [3/20] 🔥🔥We released the pretrained weight of DeepMesh (0.5 B).
Our environment has been tested on Ubuntu 22, CUDA 11.8 with A100, A800 and A6000.
- Clone our repo and create conda environment
git clone https://github.com/zhaorw02/DeepMesh.git && cd DeepMesh
conda env create -f environment.yaml
conda activate deepmesh
- Install the pretrained model weight
pip install -U "huggingface_hub[cli]"
huggingface-cli login
huggingface-cli download zzzrw/DeepMesh --local-dir ./
# Note: if you want to use your own point cloud, please make sure the normal is included.
# The file format should be a .npy file with shape (N, 6), where N is the number of points. The first 3 columns are the coordinates, and the last 3 columns are the normal.
# Generate all obj/ply in your folder
CUDA_VISIBLE_DEVICES=0 torchrun --nproc-per-node=1 --master_port=12345 sample.py \
--model_path "your_model_path" \
--steps 90000 \
--input_path examples \
--output_path mesh_output \
--repeat_num 4 \
--temperature 0.5 \
# Generate the specified obj/ply in your folder
CUDA_VISIBLE_DEVICES=0 torchrun --nproc-per-node=1 --master_port=22345.py \
--model_path "your_model_path" \
--steps 90000 \
--input_path examples \
--output_path mesh_output \
--repeat_num 4 \
--uid_list "wand1.obj,wand2.obj,wand3.ply" \
--temperature 0.5 \
# Or
bash sample.sh
- Please refer to our project_page for more examples.
- [ ] Release of pre-training code ( truncted sliding training ).
- [ ] Release of post-training code ( DPO ).
- [ ] Release of larger model ( 1b version ).
Our code is based on these wonderful repos:
@article{zhao2025deepmesh,
title={DeepMesh: Auto-Regressive Artist-mesh Creation with Reinforcement Learning},
author={Zhao, Ruowen and Ye, Junliang and Wang, Zhengyi and Liu, Guangce and Chen, Yiwen and Wang, Yikai and Zhu, Jun},
journal={arXiv preprint arXiv:2503.15265},
year={2025}
}
For Tasks:
Click tags to check more tools for each tasksFor Jobs:
Alternative AI tools for DeepMesh
Similar Open Source Tools
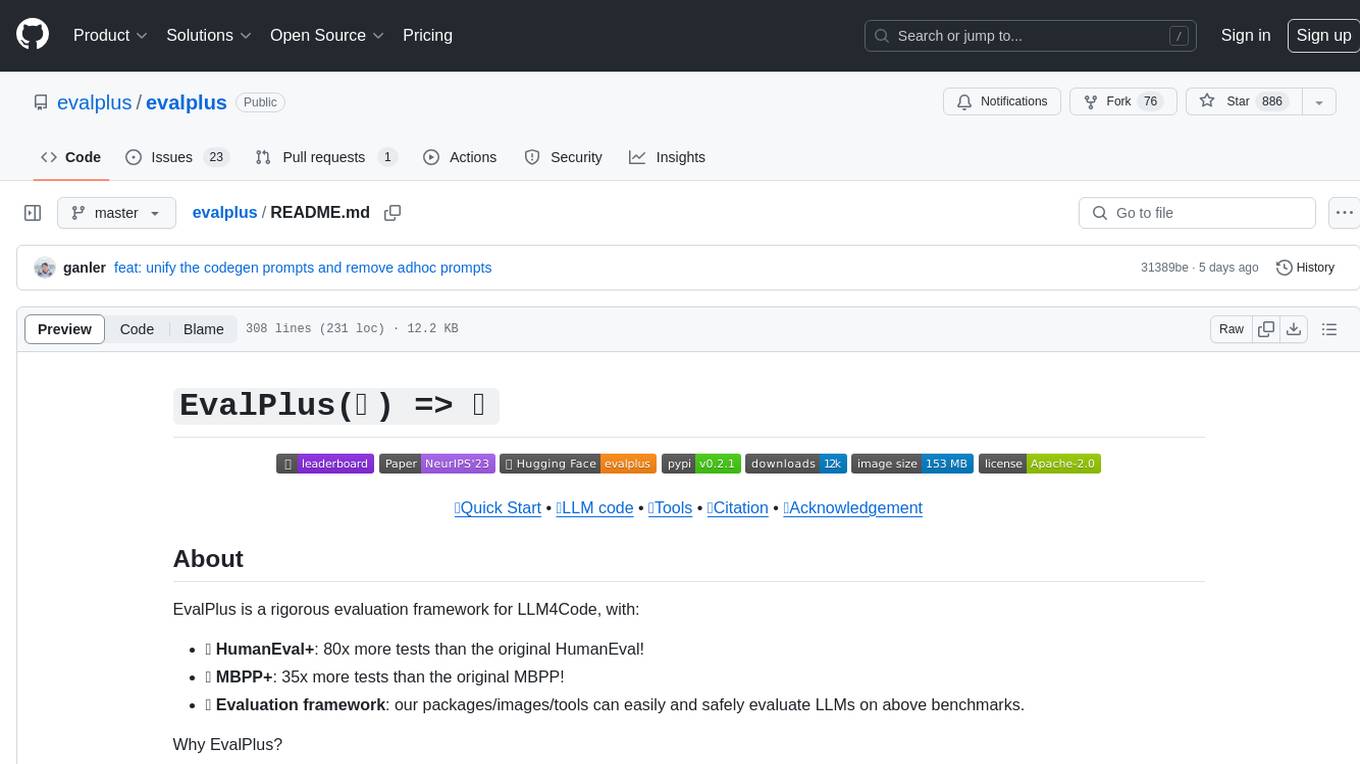
evalplus
EvalPlus is a rigorous evaluation framework for LLM4Code, providing HumanEval+ and MBPP+ tests to evaluate large language models on code generation tasks. It offers precise evaluation and ranking, coding rigorousness analysis, and pre-generated code samples. Users can use EvalPlus to generate code solutions, post-process code, and evaluate code quality. The tool includes tools for code generation and test input generation using various backends.
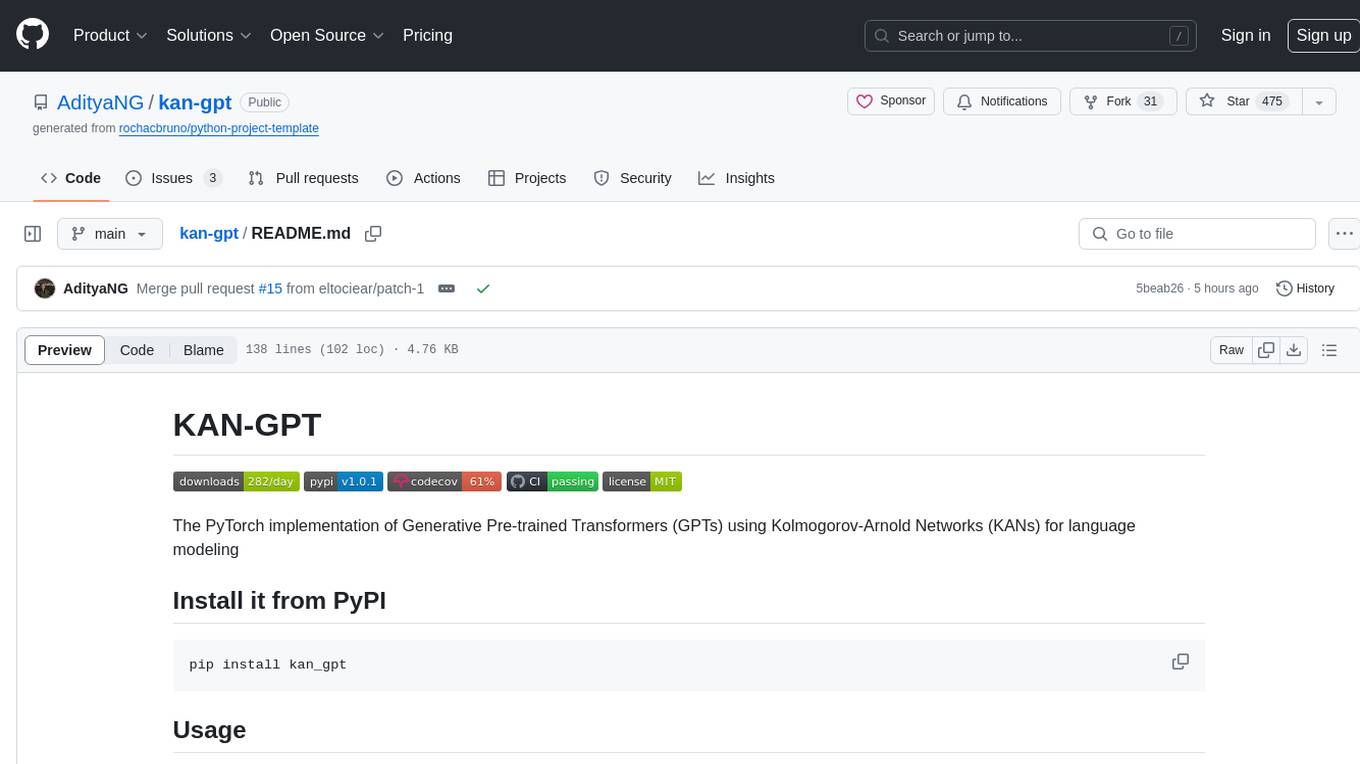
kan-gpt
The KAN-GPT repository is a PyTorch implementation of Generative Pre-trained Transformers (GPTs) using Kolmogorov-Arnold Networks (KANs) for language modeling. It provides a model for generating text based on prompts, with a focus on improving performance compared to traditional MLP-GPT models. The repository includes scripts for training the model, downloading datasets, and evaluating model performance. Development tasks include integrating with other libraries, testing, and documentation.
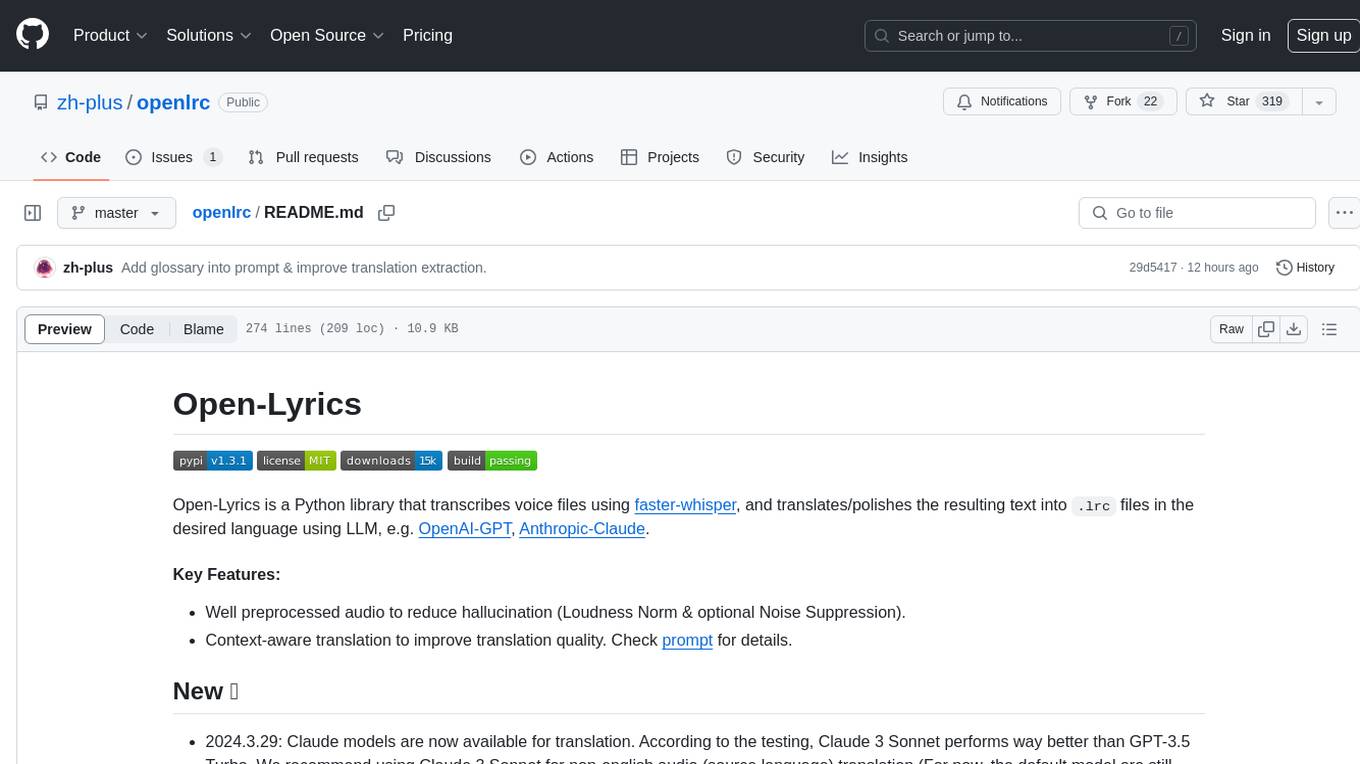
openlrc
Open-Lyrics is a Python library that transcribes voice files using faster-whisper and translates/polishes the resulting text into `.lrc` files in the desired language using LLM, e.g. OpenAI-GPT, Anthropic-Claude. It offers well preprocessed audio to reduce hallucination and context-aware translation to improve translation quality. Users can install the library from PyPI or GitHub and follow the installation steps to set up the environment. The tool supports GUI usage and provides Python code examples for transcription and translation tasks. It also includes features like utilizing context and glossary for translation enhancement, pricing information for different models, and a list of todo tasks for future improvements.
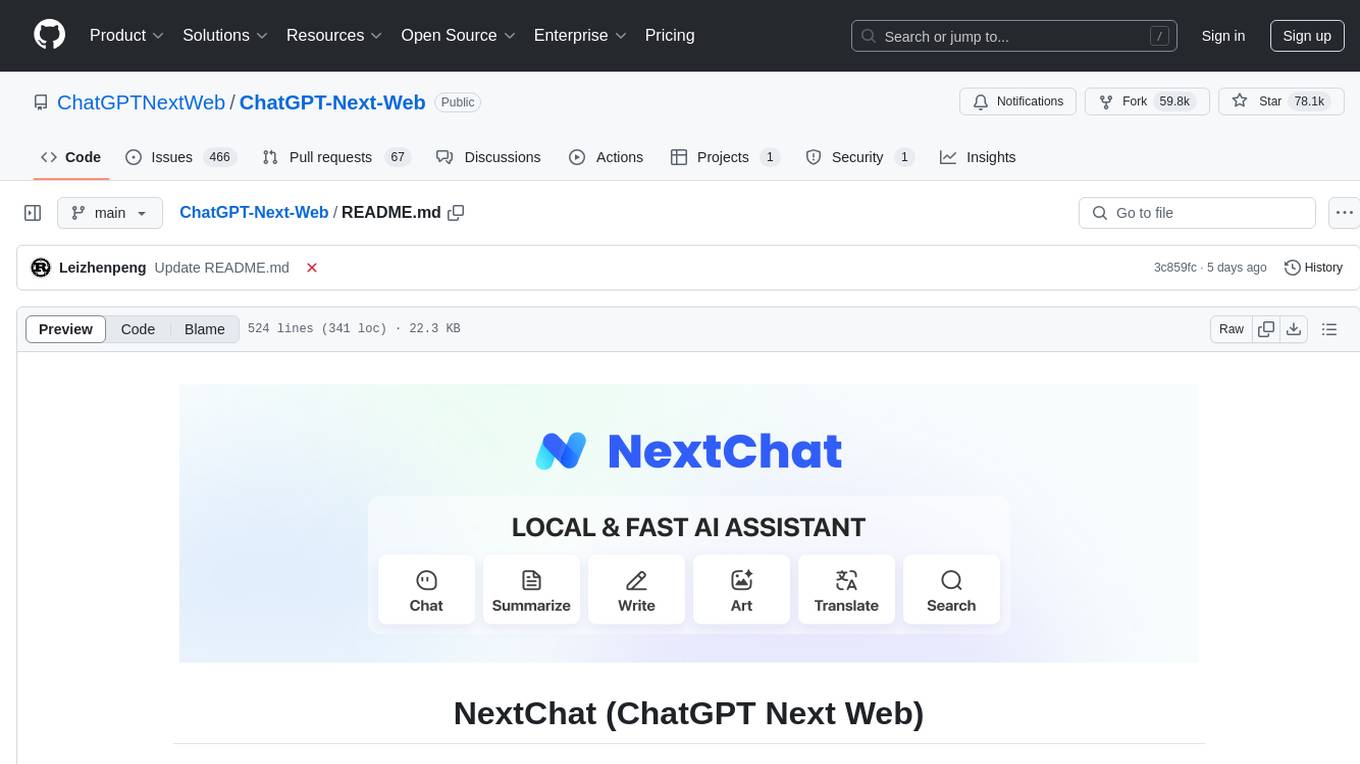
ChatGPT-Next-Web
ChatGPT Next Web is a well-designed cross-platform ChatGPT web UI tool that supports Claude, GPT4, and Gemini Pro models. It allows users to deploy their private ChatGPT applications with ease. The tool offers features like one-click deployment, compact client for Linux/Windows/MacOS, compatibility with self-deployed LLMs, privacy-first approach with local data storage, markdown support, responsive design, fast loading speed, prompt templates, awesome prompts, chat history compression, multilingual support, and more.
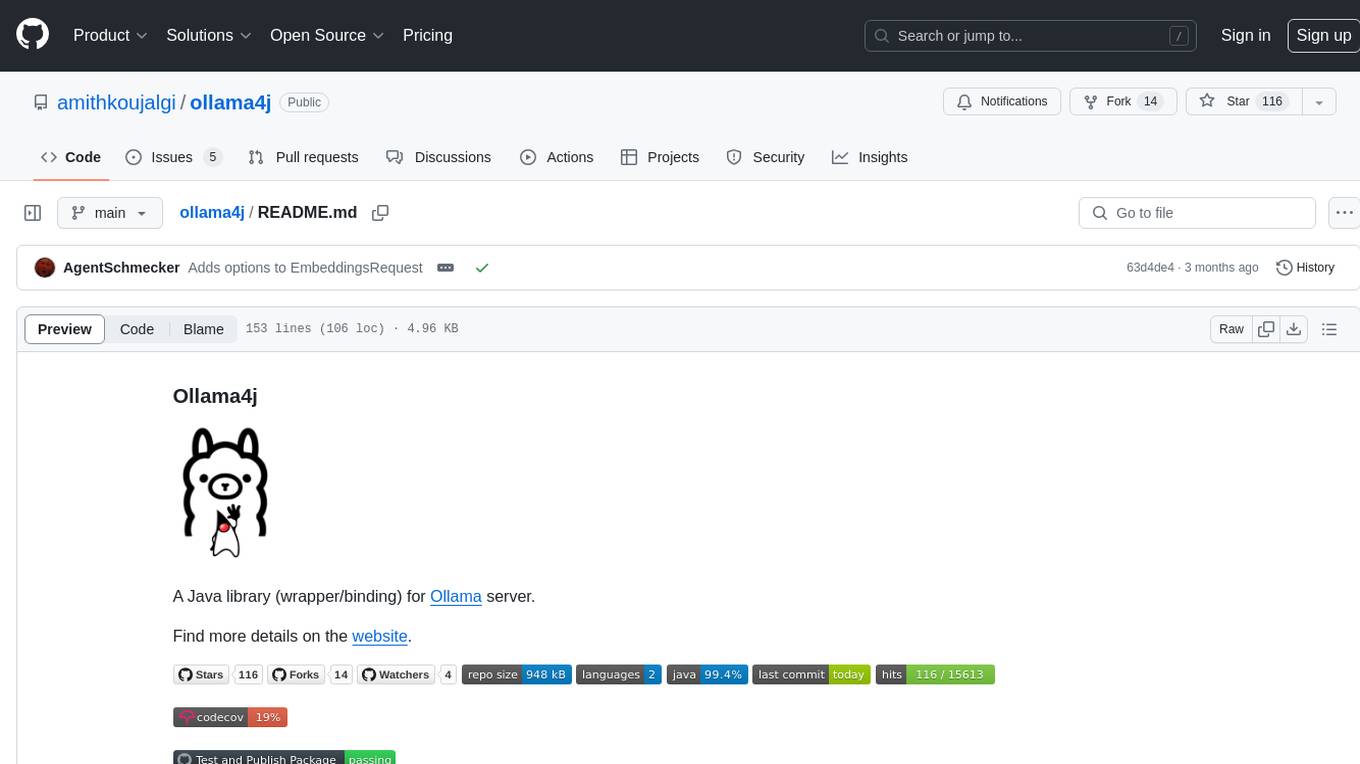
ollama4j
Ollama4j is a Java library that serves as a wrapper or binding for the Ollama server. It facilitates communication with the Ollama server and provides models for deployment. The tool requires Java 11 or higher and can be installed locally or via Docker. Users can integrate Ollama4j into Maven projects by adding the specified dependency. The tool offers API specifications and supports various development tasks such as building, running unit tests, and integration tests. Releases are automated through GitHub Actions CI workflow. Areas of improvement include adhering to Java naming conventions, updating deprecated code, implementing logging, using lombok, and enhancing request body creation. Contributions to the project are encouraged, whether reporting bugs, suggesting enhancements, or contributing code.
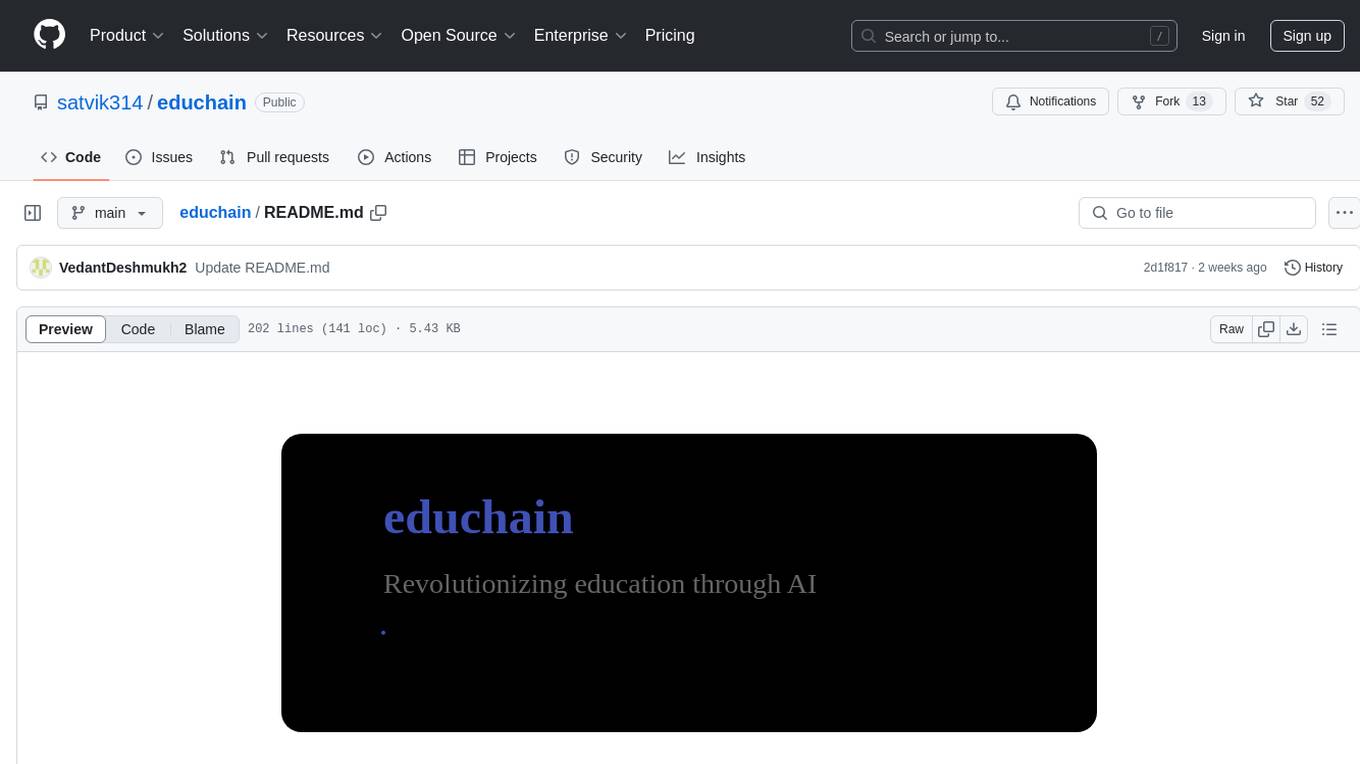
educhain
Educhain is a powerful Python package that leverages Generative AI to create engaging and personalized educational content. It enables users to generate multiple-choice questions, create lesson plans, and support various LLM models. Users can export questions to JSON, PDF, and CSV formats, customize prompt templates, and generate questions from text, PDF, URL files, youtube videos, and images. Educhain outperforms traditional methods in content generation speed and quality. It offers advanced configuration options and has a roadmap for future enhancements, including integration with popular Learning Management Systems and a mobile app for content generation on-the-go.
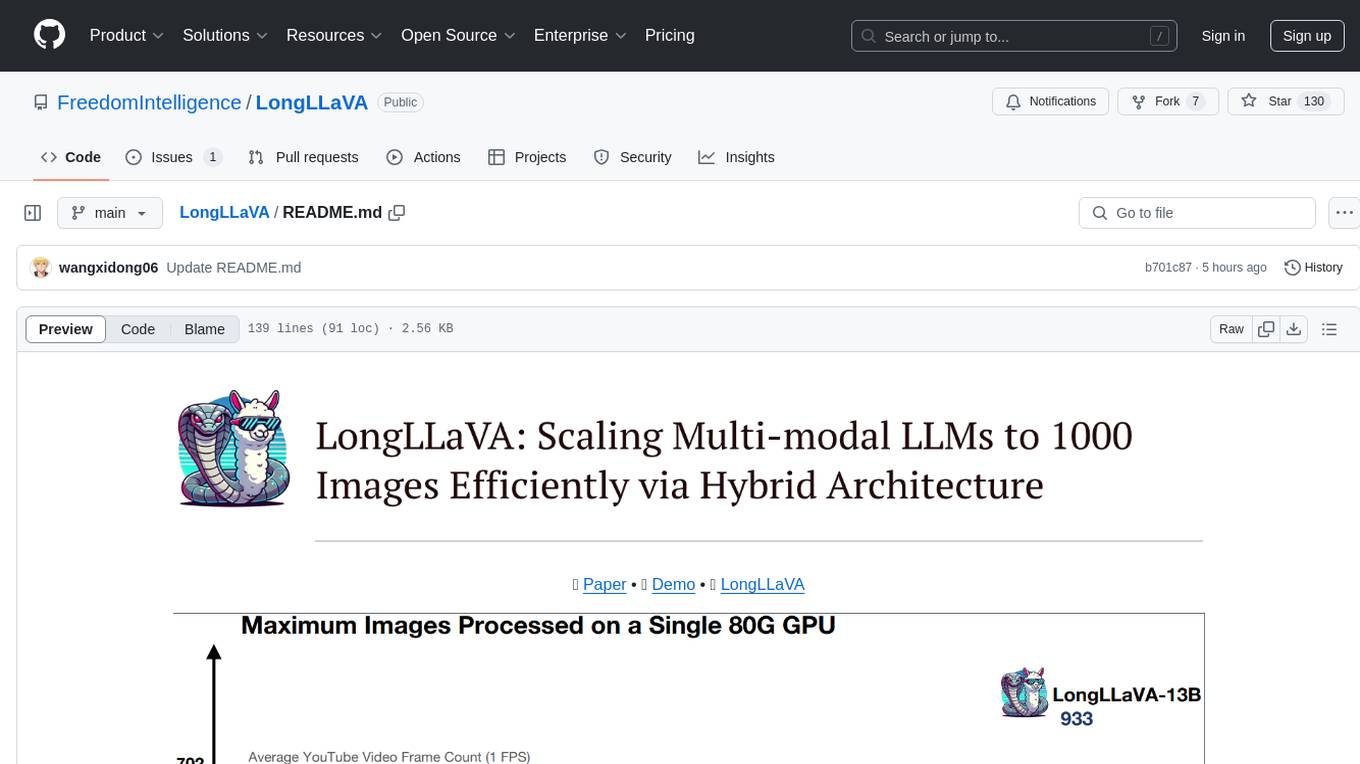
LongLLaVA
LongLLaVA is a tool for scaling multi-modal LLMs to 1000 images efficiently via hybrid architecture. It includes stages for single-image alignment, instruction-tuning, and multi-image instruction-tuning, with evaluation through a command line interface and model inference. The tool aims to achieve GPT-4V level capabilities and beyond, providing reproducibility of results and benchmarks for efficiency and performance.
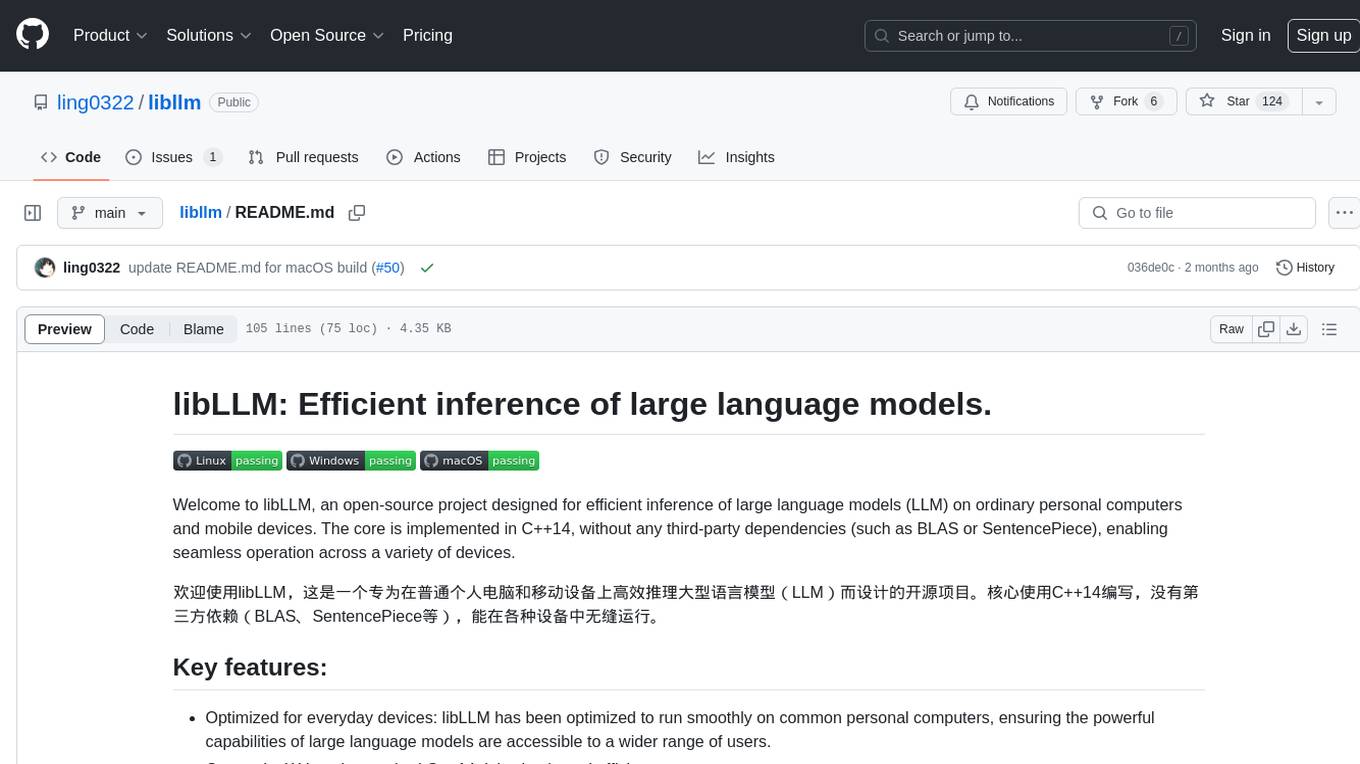
libllm
libLLM is an open-source project designed for efficient inference of large language models (LLM) on personal computers and mobile devices. It is optimized to run smoothly on common devices, written in C++14 without external dependencies, and supports CUDA for accelerated inference. Users can build the tool for CPU only or with CUDA support, and run libLLM from the command line. Additionally, there are API examples available for Python and the tool can export Huggingface models.
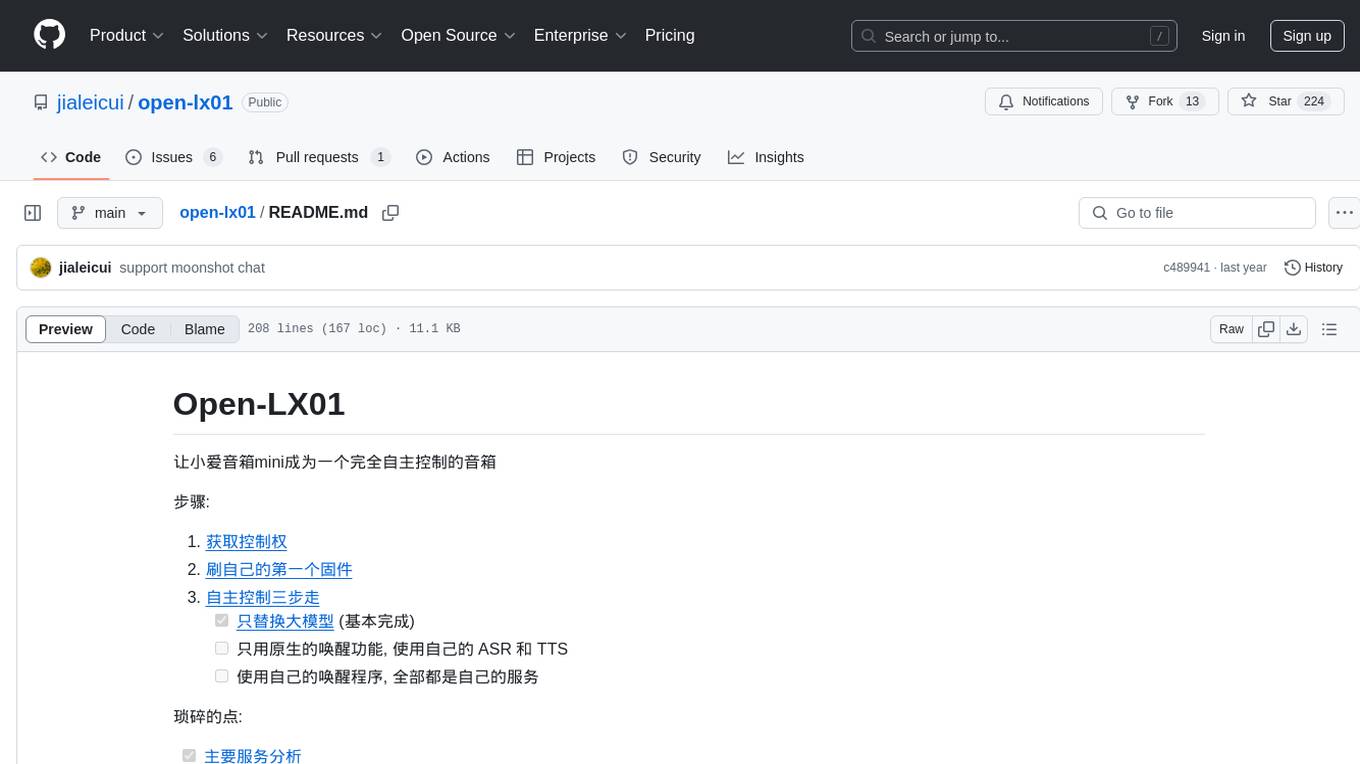
open-lx01
Open-LX01 is a project aimed at turning the Xiao Ai Mini smart speaker into a fully self-controlled device. The project involves steps such as gaining control, flashing custom firmware, and achieving autonomous control. It includes analysis of main services, reverse engineering methods, cross-compilation environment setup, customization of programs on the speaker, and setting up a web server. The project also covers topics like using custom ASR and TTS, developing a wake-up program, and creating a UI for various configurations. Additionally, it explores topics like gdb-server setup, open-mico-aivs-lab, and open-mipns-sai integration using Porcupine or Kaldi.
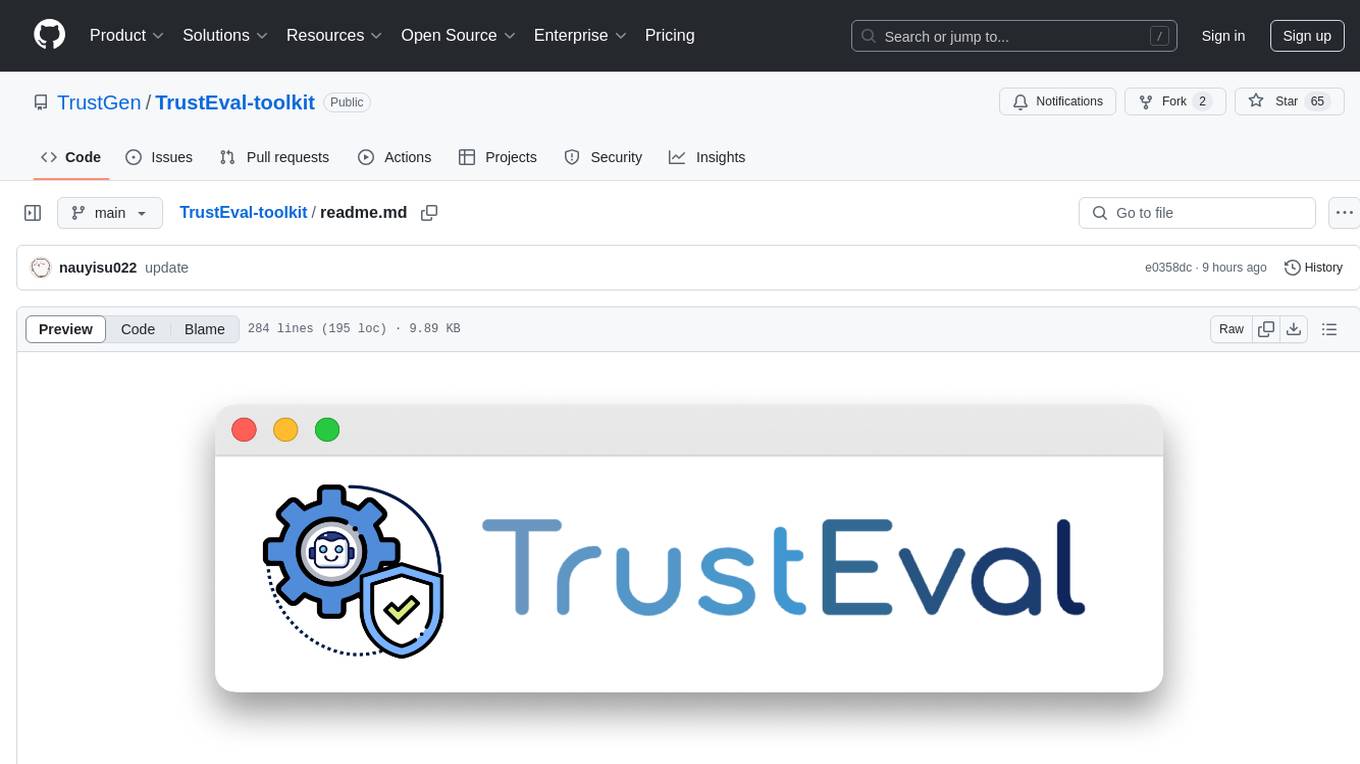
TrustEval-toolkit
TrustEval-toolkit is a dynamic and comprehensive framework for evaluating the trustworthiness of Generative Foundation Models (GenFMs) across dimensions such as safety, fairness, robustness, privacy, and more. It offers features like dynamic dataset generation, multi-model compatibility, customizable metrics, metadata-driven pipelines, comprehensive evaluation dimensions, optimized inference, and detailed reports.
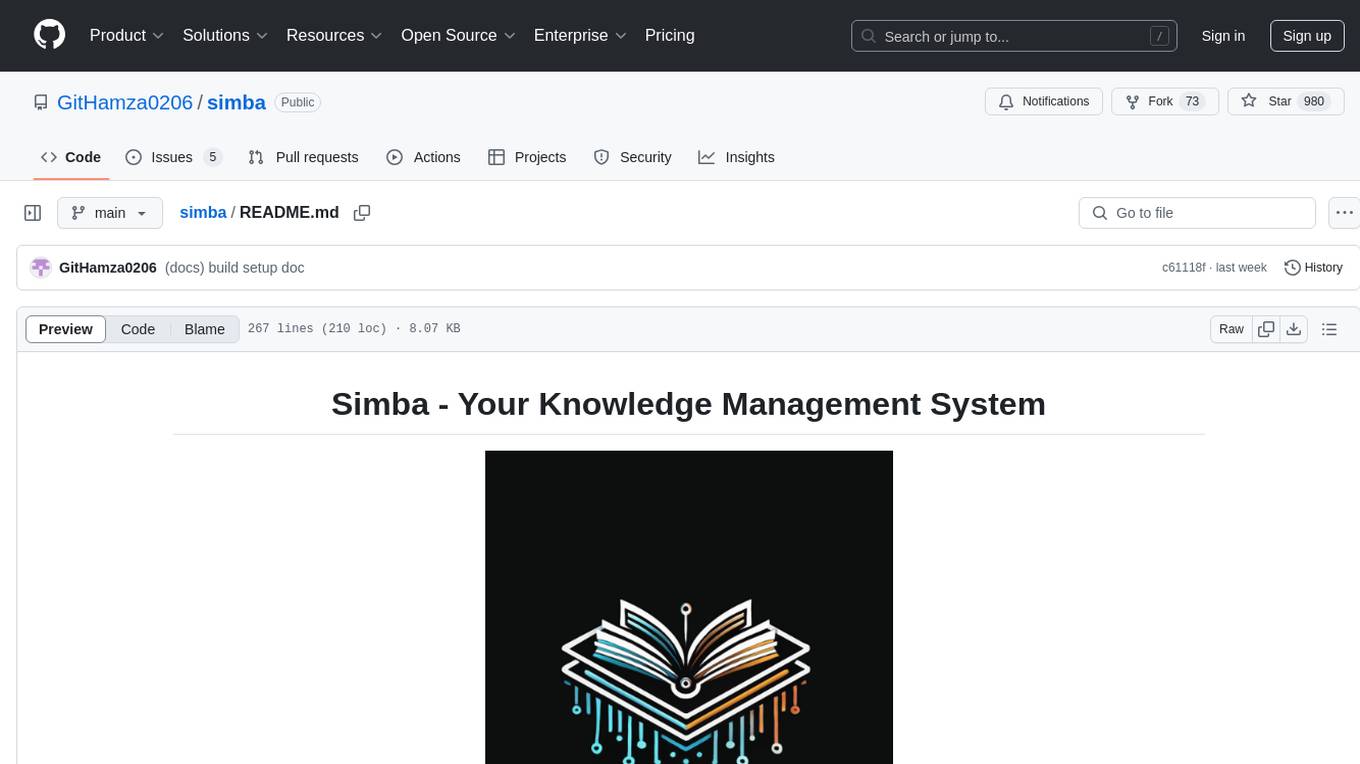
simba
Simba is an open source, portable Knowledge Management System (KMS) designed to seamlessly integrate with any Retrieval-Augmented Generation (RAG) system. It features a modern UI and modular architecture, allowing developers to focus on building advanced AI solutions without the complexities of knowledge management. Simba offers a user-friendly interface to visualize and modify document chunks, supports various vector stores and embedding models, and simplifies knowledge management for developers. It is community-driven, extensible, and aims to enhance AI functionality by providing a seamless integration with RAG-based systems.
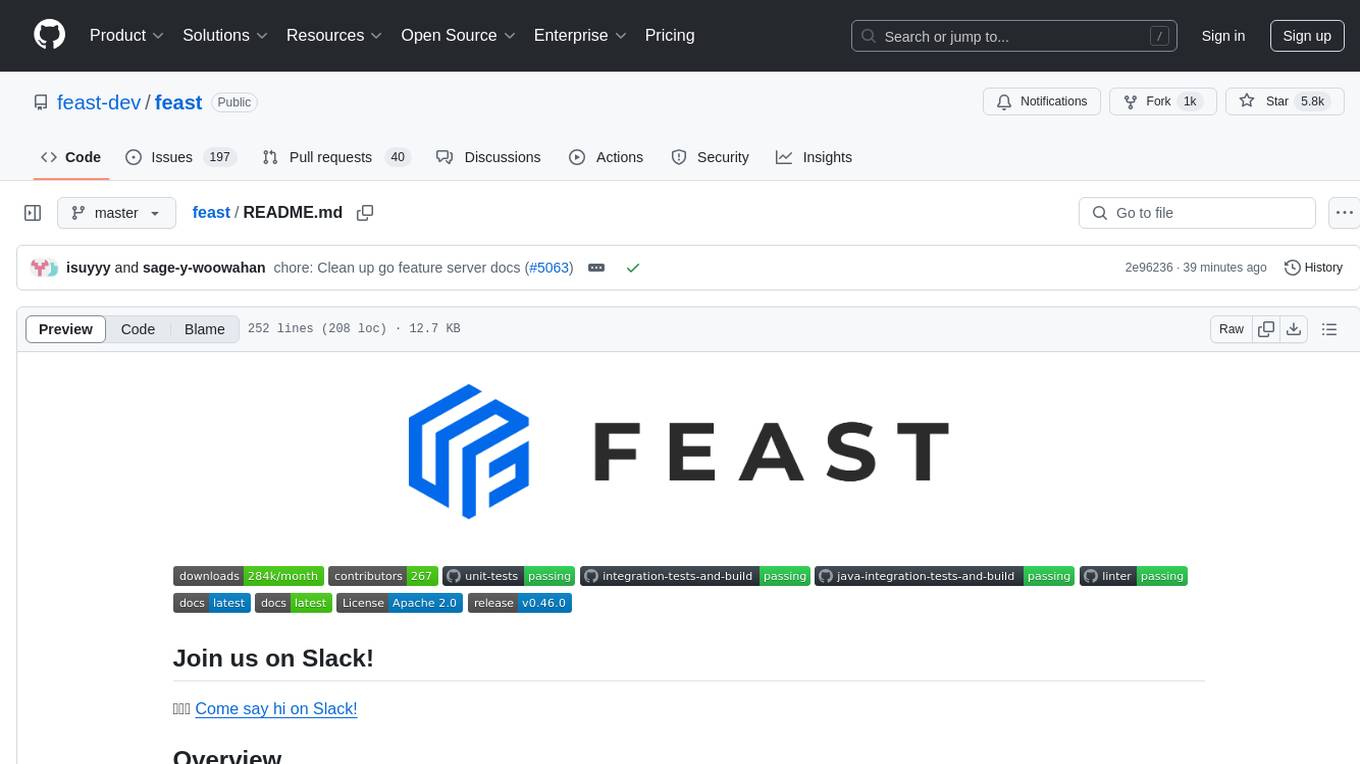
feast
Feast is an open source feature store for machine learning, providing a fast path to manage infrastructure for productionizing analytic data. It allows ML platform teams to make features consistently available, avoid data leakage, and decouple ML from data infrastructure. Feast abstracts feature storage from retrieval, ensuring portability across different model training and serving scenarios.
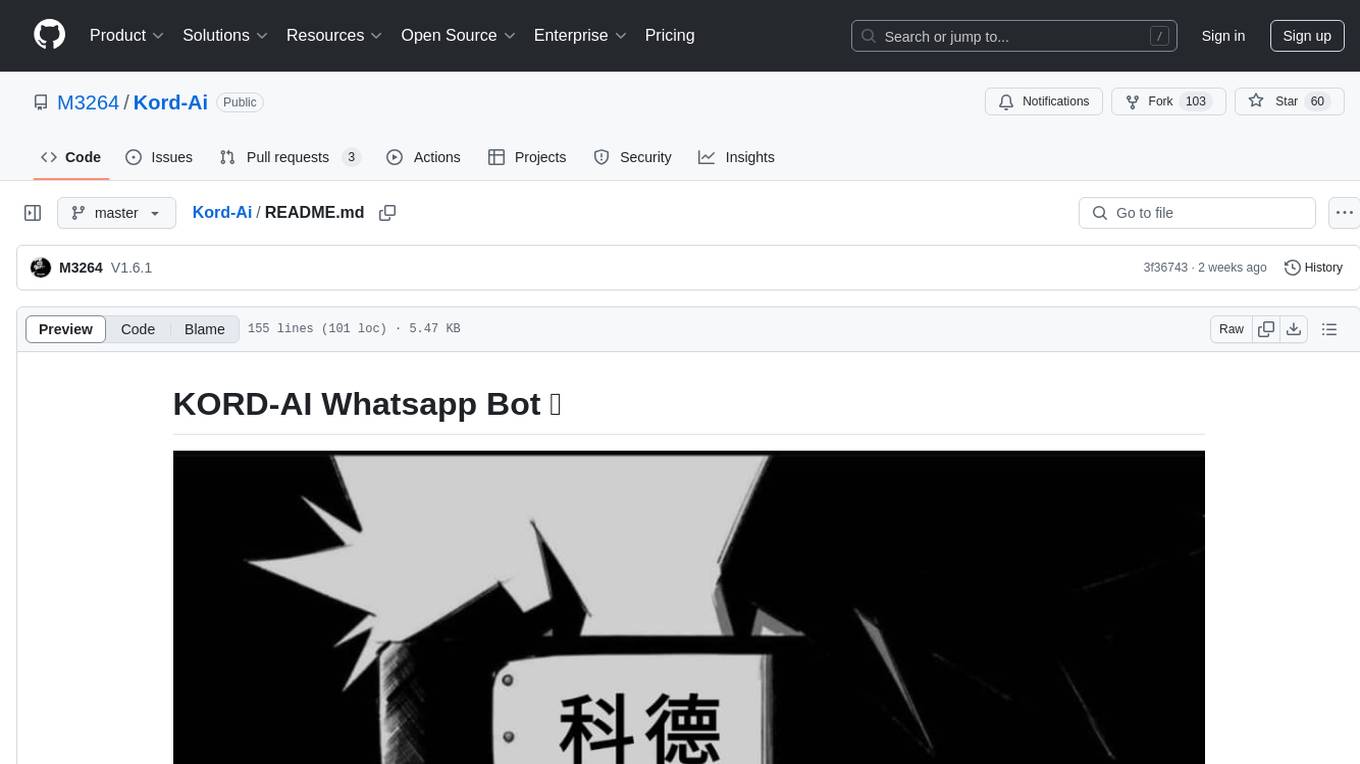
Kord-Ai
Kord-Ai is a WhatsApp bot designed to automate interactions on WhatsApp by executing predefined commands or responding to user inputs. It can handle tasks like sending messages, sharing media, and managing group activities, providing convenience and efficiency for users and businesses. The bot offers features for deployment on various platforms, including Heroku, Replit, Koyeb, Glitch, Codespace, Render, Railway, VPS, and PC. Users can deploy the bot by obtaining a session ID, forking the repository, setting configurations in the Config.js file, and starting/stopping the bot using npm commands. It is important to note that Kord-Ai is a bot created by M3264, not affiliated with WhatsApp, and users should be cautious in its usage.
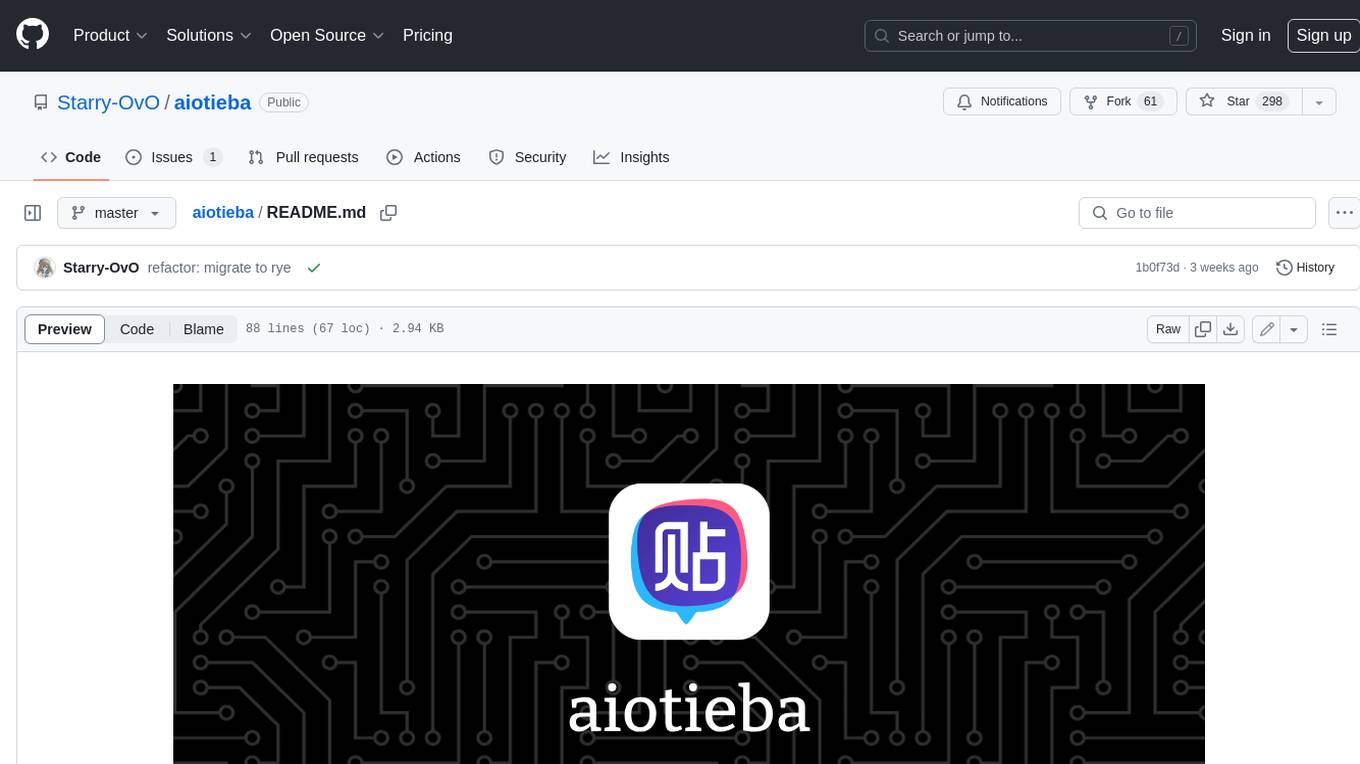
aiotieba
Aiotieba is an asynchronous Python library for interacting with the Tieba API. It provides a comprehensive set of features for working with Tieba, including support for authentication, thread and post management, and image and file uploading. Aiotieba is well-documented and easy to use, making it a great choice for developers who want to build applications that interact with Tieba.
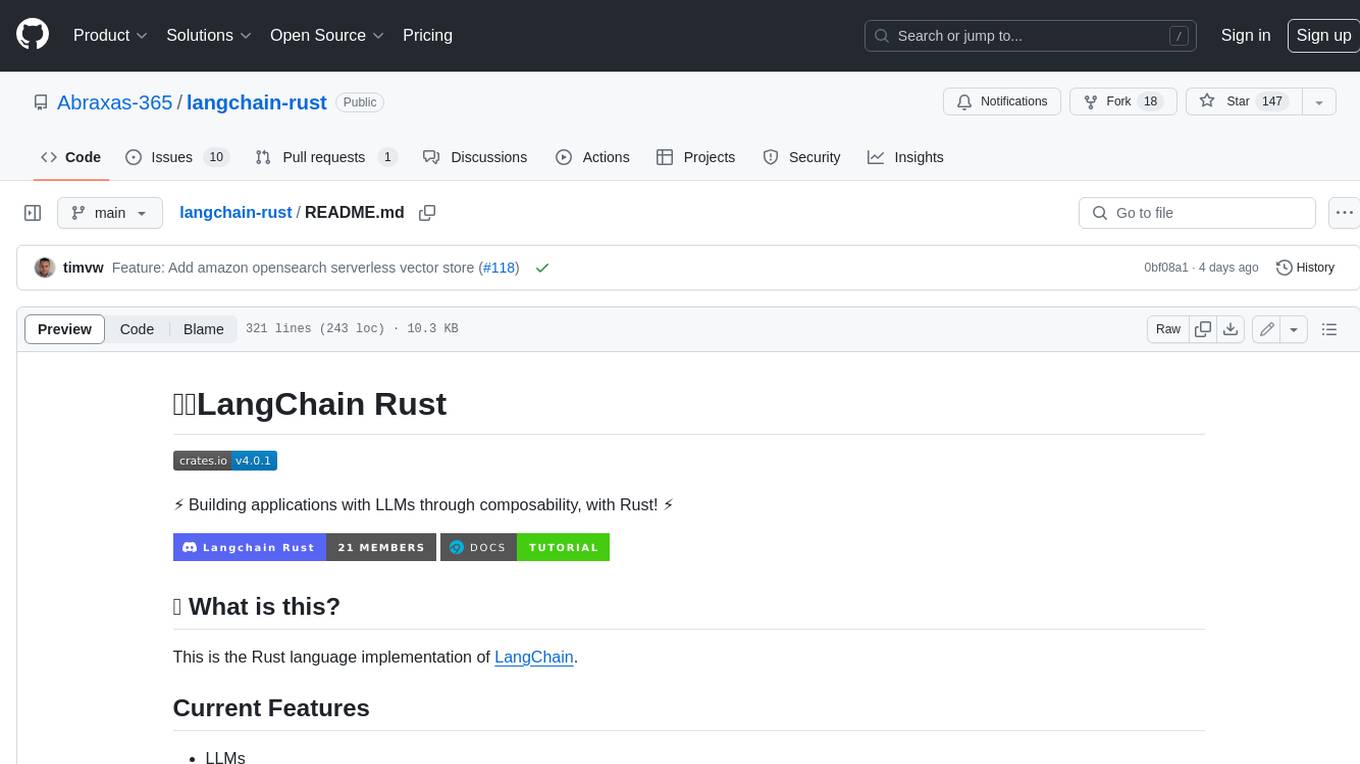
langchain-rust
LangChain Rust is a library for building applications with Large Language Models (LLMs) through composability. It provides a set of tools and components that can be used to create conversational agents, document loaders, and other applications that leverage LLMs. LangChain Rust supports a variety of LLMs, including OpenAI, Azure OpenAI, Ollama, and Anthropic Claude. It also supports a variety of embeddings, vector stores, and document loaders. LangChain Rust is designed to be easy to use and extensible, making it a great choice for developers who want to build applications with LLMs.