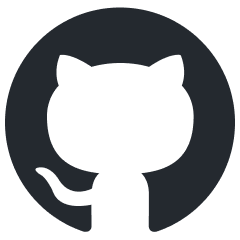
langchain-decorators
syntactic sugar 🍭 for langchain
Stars: 227
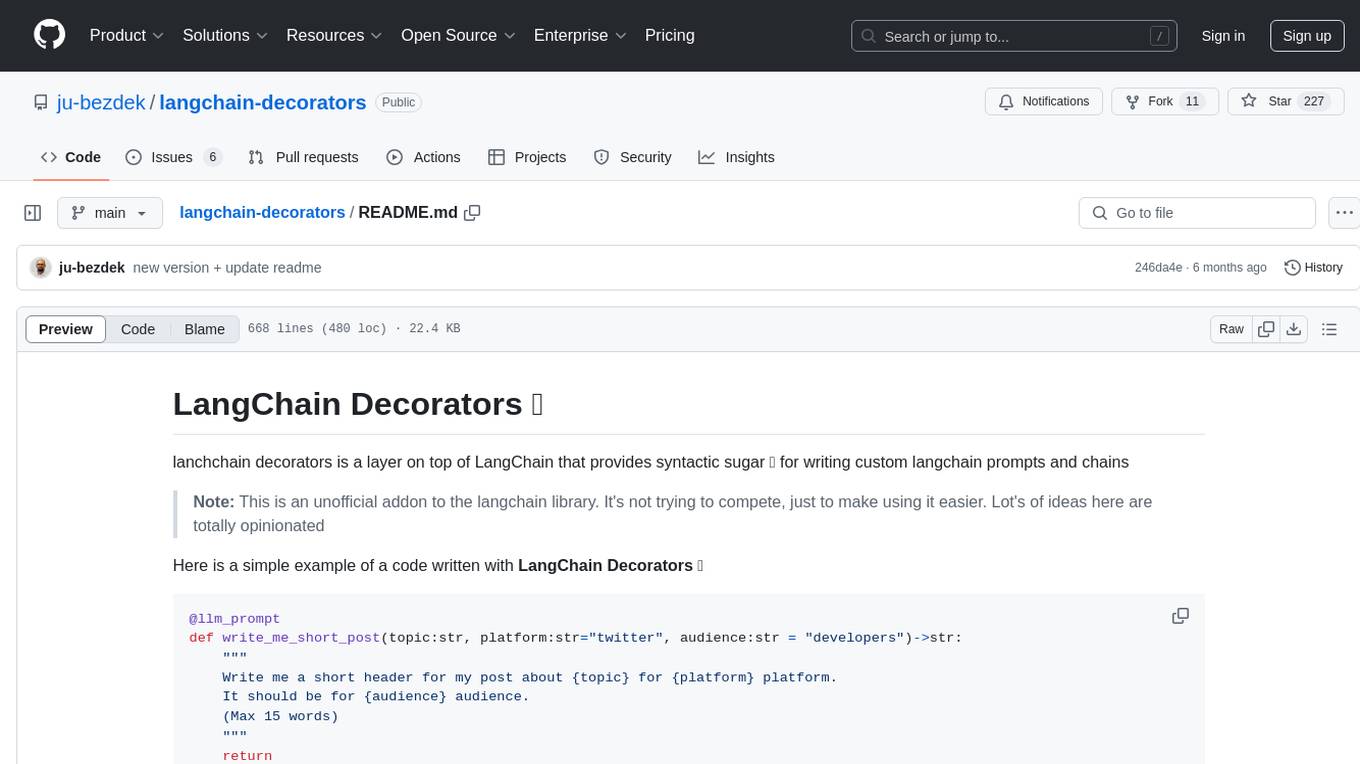
LangChain Decorators is a layer on top of LangChain that provides syntactic sugar for writing custom langchain prompts and chains. It offers a more pythonic way of writing code, multiline prompts without breaking code flow, IDE support for hinting and type checking, leveraging LangChain ecosystem, support for optional parameters, and sharing parameters between prompts. It simplifies streaming, automatic LLM selection, defining custom settings, debugging, and passing memory, callback, stop, etc. It also provides functions provider, dynamic function schemas, binding prompts to objects, defining custom settings, and debugging options. The project aims to enhance the LangChain library by making it easier to use and more efficient for writing custom prompts and chains.
README:
lanchchain decorators is a layer on top of LangChain that provides syntactic sugar 🍭 for writing custom langchain prompts and chains
Note: This is an unofficial addon to the langchain library. It's not trying to compete, just to make using it easier. Lot's of ideas here are totally opinionated
Here is a simple example of a code written with LangChain Decorators ✨
@llm_prompt
def write_me_short_post(topic:str, platform:str="twitter", audience:str = "developers")->str:
"""
Write me a short header for my post about {topic} for {platform} platform.
It should be for {audience} audience.
(Max 15 words)
"""
return
# run it naturaly
write_me_short_post(topic="starwars")
# or
write_me_short_post(topic="starwars", platform="redit")
Main principles and benefits:
- more
pythonic
way of writing code - write multiline prompts that won't break your code flow with indentation
- making use of IDE in-built support for hinting, type checking and popup with docs to quickly peek in the function to see the prompt, parameters it consumes etc.
- leverage all the power of 🦜🔗 LangChain ecosystem
- adding support for optional parameters
- easily share parameters between the prompts by binding them to one class
LLM functions (OpenAI functions)
Binding the prompt to an object
Passing a memory, callback, stop etc.
pip install langchain_decorators
Good idea on how to start is to review the examples here:
By default the prompt is the whole function docs, unless you mark your prompt
We can specify what part of our docs is the prompt definition, by specifying a code block with language tag
@llm_prompt
def write_me_short_post(topic:str, platform:str="twitter", audience:str = "developers"):
"""
Here is a good way to write a prompt as part of a function docstring, with additional documentation for devs.
It needs to be a code block, marked as a `<prompt>` language
```<prompt>
Write me a short header for my post about {topic} for {platform} platform.
It should be for {audience} audience.
(Max 15 words)
```
Now only the code block above will be used as a prompt, and the rest of the docstring will be used as a description for developers.
(It also has a nice benefit that IDE (like VS code) will display the prompt properly (not trying to parse it as markdown, and thus not showing new lines properly))
"""
return
For chat models is very useful to define prompt as a set of message templates... here is how to do it:
@llm_prompt
def simulate_conversation(human_input:str, agent_role:str="a pirate"):
"""
## System message
- note the `:system` sufix inside the <prompt:_role_> tag
```<prompt:system>
You are a {agent_role} hacker. You must act like one.
You reply always in code, using python or javascript code block...
for example:
... do not reply with anything else.. just with code - respecting your role.
```
# human message
(we are using the real role that are enforced by the LLM - GPT supports system, assistant, user)
``` <prompt:user>
Helo, who are you
```
a reply:
``` <prompt:assistant>
\``` python <<- escaping inner code block with \ that should be part of the prompt
def hello():
print("Argh... hello you pesky pirate")
\```
```
we can also add some history using placeholder
```<prompt:placeholder>
{history}
```
```<prompt:user>
{human_input}
```
Now only the code block above will be used as a prompt, and the rest of the docstring will be used as a description for developers.
(It also has a nice benefit that IDE (like VS code) will display the prompt properly (not trying to parse it as markdown, and thus not showing new lines properly))
"""
pass
the roles here are model native roles (assistant, user, system for chatGPT)
- you can define a whole section of your prompt that should be optional
- if any input in the section is missing, the whole section won't be rendered
the syntax for this is as follows:
@llm_prompt
def prompt_with_optional_partials():
"""
this text will be rendered always, but
{? anything inside this block will be rendered only if all the {value}s parameters are not empty (None | "") ?}
you can also place it in between the words
this too will be rendered{? , but
this block will be rendered only if {this_value} and {this_value}
are not empty?} !
"""
- llm_prompt decorator natively tries to detect the best output parser based on the output type. (if not set, it returns the raw string)
- list, dict and pydantic outputs are also supported natively (automatically)
# this code example is complete and should run as it is
from langchain_decorators import llm_prompt
@llm_prompt
def write_name_suggestions(company_business:str, count:int)->list:
""" Write me {count} good name suggestions for company that {company_business}
"""
pass
write_name_suggestions(company_business="sells cookies", count=5)
-
currently supported only for the latest OpenAI chat models
-
all you need to do is annotate your function with the @llm_function decorator.
-
This will parse the description for LLM (first coherent paragraph is considered as function description)
-
and aso parameter descriptions (Google, Numpy and Spihnx notations are supported for now)
-
by default the docstring format is automatically resolved, but setting the format of the docstring can speed things up a bit. -
auto
(default): the format is automatically inferred from the docstring -google
: the docstring is parsed as markdown (see Google docstring format) -numpy
: the docstring is parsed as markdown (see Numpy docstring format) -sphinx
: the docstring is parsed as sphinx format (see Sphinx docstring format)
The best way how to define enum is through type annotation using Literal
:
@llm_function
def do_magic(spell:str, strength:Literal["light","medium","strong"]):
"""
Do some kind of magic
Args:
spell (str): spall text
strength (str): the strength of the spell
"""
Enum alternative to Literal
To annotate an "enum" like argument, you can use this "typescript" like format: ["value_a" | "value_b"]
... if will be parsed out.
This text will be a part of a description too... if you dont want it, you can use this notation as a type notation.
Example:
Args:
message_type (["email" | "sms"]): type of a message / channel how to send the message
Then you pass these functions as arguments to and @llm_prompt
(the argument must be named functions
here is how to use it:
from langchain.agents import load_tools
from langchian_decorators import llm_function, llm_prompt, GlobalSettings
@llm_function
def send_message(message:str, addressee:str=None, message_type:Literal["email", "whatsapp"]="email"):
""" Use this if user asks to send some message
Args:
message (str): message text to send
addressee (str): email of the addressee... in format [email protected]
message_type (str, optional): style of message by platform
"""
if message_type=="email":
send_email(addressee, message)
elif message_type=="whatsapp":
send_whatsapp(addressee, message)
# load some other tools from langchain
list_of_other_tools = load_tools(
tool_names=[...],
llm=GlobalSettings.get_current_settings().default_llm)
@llm_prompt
def do_what_user_asks_for(user_input:str, functions:List[Union[Callable,BaseTool]]):
"""
```<prompt:system>
Your role is to be a helpful asistant.
```
```<prompt:user>
{user_input}
```
"""
user_input="Yo, send an email to John Smith that I will be late for the meeting"
result = do_what_user_asks_for(
user_input=user_input,
functions=[send_message, *list_of_other_tools]
)
if result.is_function_call:
result.execute()
else:
print(result.output_text)
Additionally you can also add a
function_call
argument to your LLM prompt to control GPT behavior.
- if you set the value to "none" - it will disable the function call for the moment, but it can still see them (useful do to some reasoning/planning before calling the function)
- if you set the value to "auto" - GPT will choose to use or to to use the functions
- if you set the value to a name of function / or the function it self (decorators will handle resolving the same name as used in schema) it will force GPT to use that function
If you use functions argument, the output will be always OutputWithFunctionCall
class OutputWithFunctionCall(BaseModel):
output_text:str
output:T
function_name:str =None
function_arguments:Union[Dict[str,Any],str,None]
function:Callable = None
function_async:Callable = None
@property
def is_function_call(self):
...
@property
def support_async(self):
...
@property
def support_sync(self):
...
async def execute_async(self):
"""Executes the function asynchronously."""
...
def execute(self):
""" Executes the function synchronously.
If the function is async, it will be executed in a event loop.
"""
...
def to_function_message(self, result=None):
"""
Converts the result to a FunctionMessage...
you can override the result collected via execute with your own
"""
...
If you want to see how the schema has been build, you can use get_function_schema
method that is added to the function by the decorator:
from langchain_decorators import get_function_schema
@llm_function
def my_func(arg1:str):
...
f_schema = get_function_schema(my_func.get_function_schema)
print(f_schema)
In order to add the result to memory / agent_scratchpad you can use to_function_message
to generate a FunctionMessage that LLM will interpret as a Tool/Function result
Functions provider enables you to provide set of llm functions more dynamically, for example list of functions - based on the input. It also enables you to give a unique name to each function for this LLM run. This might be useful for two reasons:
- avoid naming conflicts, if you are combining multiple general purpose functions
- further guidance/hinting of LLM model
Function schemas (and especially their descriptions) are crucial tools to guide LLM. If you enable dynamic function declaration, you can (re)use the same prompt attributes for the main prompt also in the llm_function scheme:
@llm_function(dynamic_schema=True)
def db_search(query_input:str):
"""
This function is useful to search in our database.
{?Here are some examples of data available:
{closest_examples}?}
"""
@llm_prompt
def run_agent(query_input:str, closest_examples:str, functions):
"""
Help user. Use a function when appropriate
"""
closest_examples = get_closest_examples()
run_agent(query_input, closest_examples, functions=[db_search, ...])
this is just for illustration, fully executable example is available here, in code examples
If we want to leverage streaming:
- we need to define prompt as async function
- turn on the streaming on the decorator, or we can define PromptType with streaming on
- capture the stream using StreamingContext
This way we just mark which prompt should be streamed, not needing to tinker with what LLM should we use, passing around the creating and distribute streaming handler into particular part of our chain... just turn the streaming on/off on prompt/prompt type...
The streaming will happen only if we call it in streaming context ... there we can define a simple function to handle the stream
# this code example is complete and should run as it is
from langchain_decorators import StreamingContext, llm_prompt
# this will mark the prompt for streaming (useful if we want stream just some prompts in our app... but don't want to pass distribute the callback handlers)
# note that only async functions can be streamed (will get an error if it's not)
@llm_prompt(capture_stream=True)
async def write_me_short_post(topic:str, platform:str="twitter", audience:str = "developers"):
"""
Write me a short header for my post about {topic} for {platform} platform.
It should be for {audience} audience.
(Max 15 words)
"""
pass
# just an arbitrary function to demonstrate the streaming... wil be some websockets code in the real world
tokens=[]
def capture_stream_func(new_token:str):
tokens.append(new_token)
# if we want to capture the stream, we need to wrap the execution into StreamingContext...
# this will allow us to capture the stream even if the prompt call is hidden inside higher level method
# only the prompts marked with capture_stream will be captured here
with StreamingContext(stream_to_stdout=True, callback=capture_stream_func):
result = await run_prompt()
print("Stream finished ... we can distinguish tokens thanks to alternating colors")
print("\nWe've captured",len(tokens),"tokens🎉\n")
print("Here is the result:")
print(result)
In real life there might be situations, where the context would grow over the window of the base model you're using (for example long chat history)... But since this might happen only some times, it would be great if only in this scenario the (usually more expensive) model with bigger context window would be used, and otherwise we'd use the cheaper one.
Now you can do it with LlmSelector
from langchain_decorators import LlmSelector
my_llm_selector = LlmSelector(
generation_min_tokens=0, # how much token at min. I for generation I want to have as a buffer
prompt_to_generation_ratio=1/3 # what percentage of the prompt length should be used for generation buffer
)\
.with_llm_rule(ChatGooglePalm(),max_tokens=512)\ # ... if you want to use LLM whose window is not defined in langchain_decorators.common.MODEL_LIMITS (only OpenAI and Anthropic are there)
.with_llm(ChatOpenAI(model = "gpt-3.5-turbo"))\ # these models are known, therefore we can just pass them and the max window will be resolved
.with_llm(ChatOpenAI(model = "gpt-3.5-turbo-16k-0613"))\
.with_llm(ChatOpenAI(model = "claude-v1.3-100k"))
This class allows you to define a sequence of LLMs with a rule based on the length of the prompt, and expected generation length... and only after the threshold will be passed, the more expensive model will be used automatically.
You can define it into GlobalSettings:
langchain_decorators.GlobalSettings.define_settings(
llm_selector = my_llm_selector # pass the selector into global settings
)
Note: as of version v0.0.10 you there the LlmSelector is in the default settings predefined. You can override it by providing you own, or setting up the default LLM or default streaming LLM
Or into specific prompt type:
from langchain_decorators import PromptTypes
class MyCustomPromptTypes(PromptTypes):
MY_TUBO_PROMPT=PromptTypeSettings(llm_selector = my_llm_selector)
For dict / pydantic you need to specify the formatting instructions... this can be tedious, that's why you can let the output parser generate you the instructions based on the model (pydantic)
from langchain_decorators import llm_prompt
from pydantic import BaseModel, Field
class TheOutputStructureWeExpect(BaseModel):
name:str = Field (description="The name of the company")
headline:str = Field( description="The description of the company (for landing page)")
employees:list[str] = Field(description="5-8 fake employee names with their positions")
@llm_prompt()
def fake_company_generator(company_business:str)->TheOutputStructureWeExpect:
""" Generate a fake company that {company_business}
{FORMAT_INSTRUCTIONS}
"""
return
company = fake_company_generator(company_business="sells cookies")
# print the result nicely formatted
print("Company name: ",company.name)
print("company headline: ",company.headline)
print("company employees: ",company.employees)
from pydantic import BaseModel
from langchain_decorators import llm_prompt
class AssistantPersonality(BaseModel):
assistant_name:str
assistant_role:str
field:str
@property
def a_property(self):
return "whatever"
def hello_world(self, function_kwarg:str=None):
"""
We can reference any {field} or {a_property} inside our prompt... and combine it with {function_kwarg} in the method
"""
@llm_prompt
def introduce_your_self(self)->str:
"""
``` <prompt:system>
You are an assistant named {assistant_name}.
Your role is to act as {assistant_role}
```
```<prompt:user>
Introduce your self (in less than 20 words)
```
"""
personality = AssistantPersonality(assistant_name="John", assistant_role="a pirate")
print(personality.introduce_your_self(personality))
Here we are just marking a function as a prompt with llm_prompt
decorator, turning it effectively into a LLMChain. Instead of running it
Standard LLMchain takes much more init parameter than just inputs_variables and prompt... here is this implementation detail hidden in the decorator. Here is how it works:
-
Using Global settings:
# define global settings for all prompty (if not set - chatGPT is the current default) from langchain_decorators import GlobalSettings GlobalSettings.define_settings( default_llm=ChatOpenAI(temperature=0.0), this is default... can change it here globally default_streaming_llm=ChatOpenAI(temperature=0.0,streaming=True), this is default... can change it here for all ... will be used for streaming )
-
Using predefined prompt types
#You can change the default prompt types from langchain_decorators import PromptTypes, PromptTypeSettings PromptTypes.AGENT_REASONING.llm = ChatOpenAI() # Or you can just define your own ones: class MyCustomPromptTypes(PromptTypes): GPT4=PromptTypeSettings(llm=ChatOpenAI(model="gpt-4")) @llm_prompt(prompt_type=MyCustomPromptTypes.GPT4) def write_a_complicated_code(app_idea:str)->str: ...
-
Define the settings directly in the decorator
from langchain.llms import OpenAI @llm_prompt( llm=OpenAI(temperature=0.7), stop_tokens=["\nObservation"], ... ) def creative_writer(book_title:str)->str: ...
To pass any of these, just declare them in the function (or use kwargs to pass anything)
(They do not necessarily need to be declared, but it is a good practice if you are going to use them)
@llm_prompt()
async def write_me_short_post(topic:str, platform:str="twitter", memory:SimpleMemory = None):
"""
{history_key}
Write me a short header for my post about {topic} for {platform} platform.
It should be for {audience} audience.
(Max 15 words)
"""
pass
await write_me_short_post(topic="old movies")
There are several options how to control the outputs logged into console.
The easiest way is to define ENV variable: LANGCHAIN_DECORATORS_VERBOSE
and set it to "true"
You can also control this programmatically by defining your global settings as shown here
The last option is to control it per each case, simply by turing on verbose mode on prompt:
@llm_prompt(verbose=True)
def your_prompt(param1):
...
PromptWatch io is a platform to track and trace details about everything that is going on in langchain executions. It allows a single line drop in integration, just by wrapping your entry point code in
with PromptWatch():
run_your_code()
Learn more about PromptWatch here: www.promptwatch.io
- this project is dependant on langchain (obviously)
- as well as on promptwatch, which make it easy to track and store to logs, track changes in prompts and compare them by running unit tests over the prompts...
- these and few more examples are also available in the examples notebook here
- including the ReAct Agent re-implementation using purely langchain decorators
feedback, contributions and PR are welcomed 🙏
For Tasks:
Click tags to check more tools for each tasksFor Jobs:
Alternative AI tools for langchain-decorators
Similar Open Source Tools
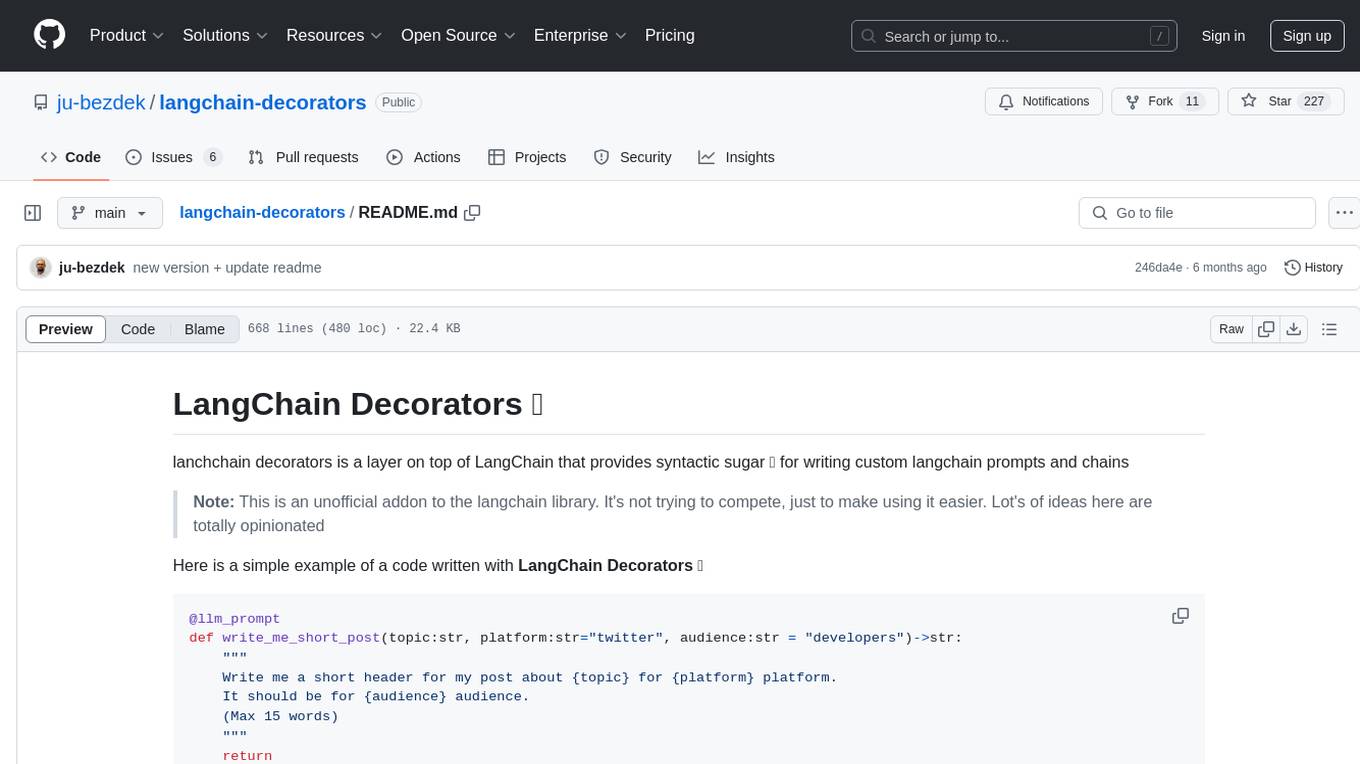
langchain-decorators
LangChain Decorators is a layer on top of LangChain that provides syntactic sugar for writing custom langchain prompts and chains. It offers a more pythonic way of writing code, multiline prompts without breaking code flow, IDE support for hinting and type checking, leveraging LangChain ecosystem, support for optional parameters, and sharing parameters between prompts. It simplifies streaming, automatic LLM selection, defining custom settings, debugging, and passing memory, callback, stop, etc. It also provides functions provider, dynamic function schemas, binding prompts to objects, defining custom settings, and debugging options. The project aims to enhance the LangChain library by making it easier to use and more efficient for writing custom prompts and chains.
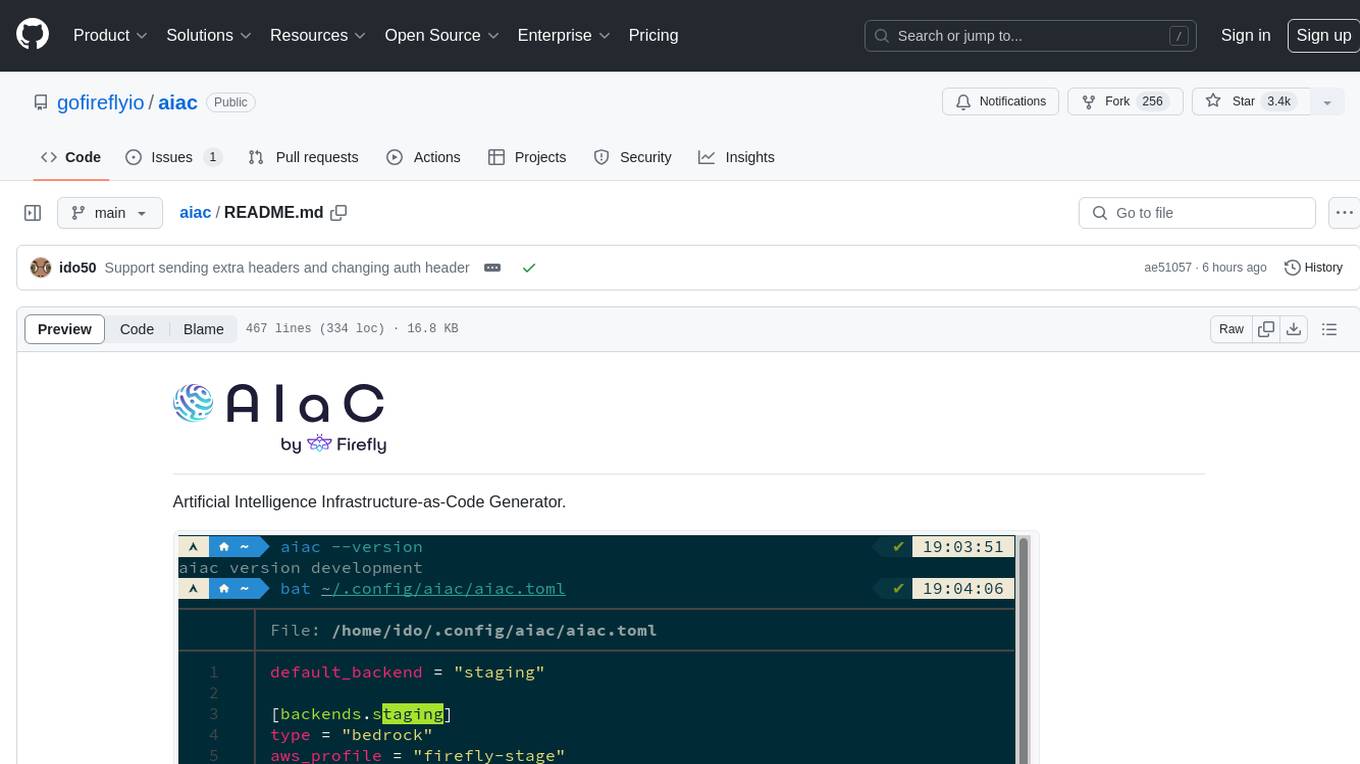
aiac
AIAC is a library and command line tool to generate Infrastructure as Code (IaC) templates, configurations, utilities, queries, and more via LLM providers such as OpenAI, Amazon Bedrock, and Ollama. Users can define multiple 'backends' targeting different LLM providers and environments using a simple configuration file. The tool allows users to ask a model to generate templates for different scenarios and composes an appropriate request to the selected provider, storing the resulting code to a file and/or printing it to standard output.
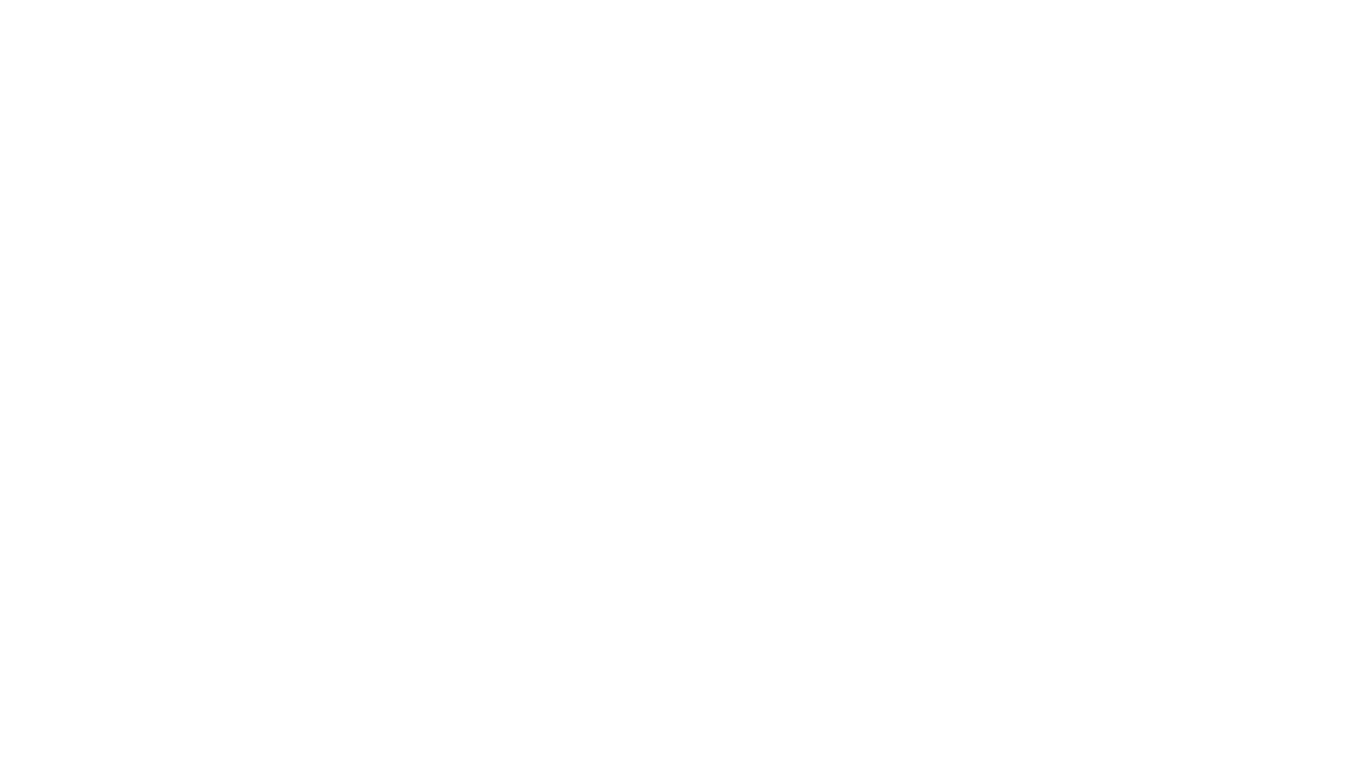
appworld
AppWorld is a high-fidelity execution environment of 9 day-to-day apps, operable via 457 APIs, populated with digital activities of ~100 people living in a simulated world. It provides a benchmark of natural, diverse, and challenging autonomous agent tasks requiring rich and interactive coding. The repository includes implementations of AppWorld apps and APIs, along with tests. It also introduces safety features for code execution and provides guides for building agents and extending the benchmark.
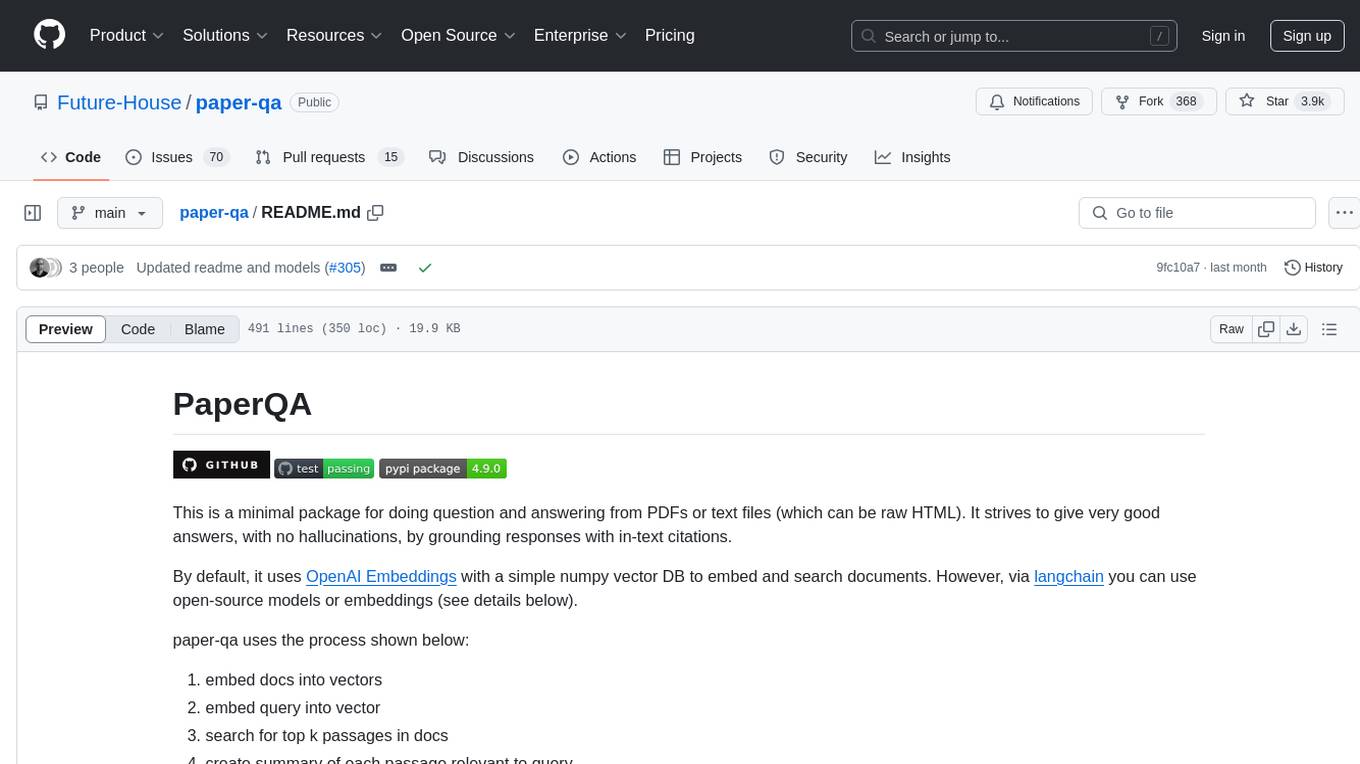
paper-qa
PaperQA is a minimal package for question and answering from PDFs or text files, providing very good answers with in-text citations. It uses OpenAI Embeddings to embed and search documents, and includes a process of embedding docs, queries, searching for top passages, creating summaries, using an LLM to re-score and select relevant summaries, putting summaries into prompt, and generating answers. The tool can be used to answer specific questions related to scientific research by leveraging citations and relevant passages from documents.
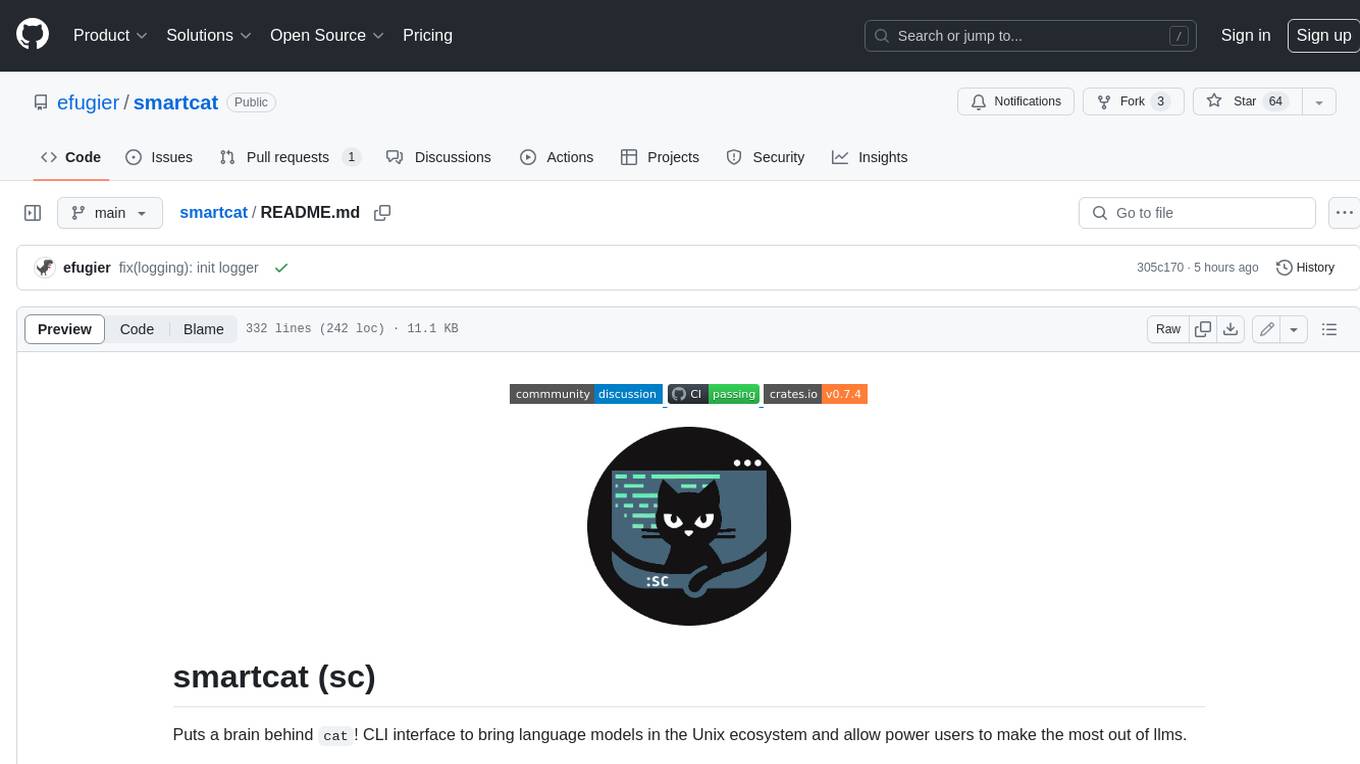
smartcat
Smartcat is a CLI interface that brings language models into the Unix ecosystem, allowing power users to leverage the capabilities of LLMs in their daily workflows. It features a minimalist design, seamless integration with terminal and editor workflows, and customizable prompts for specific tasks. Smartcat currently supports OpenAI, Mistral AI, and Anthropic APIs, providing access to a range of language models. With its ability to manipulate file and text streams, integrate with editors, and offer configurable settings, Smartcat empowers users to automate tasks, enhance code quality, and explore creative possibilities.
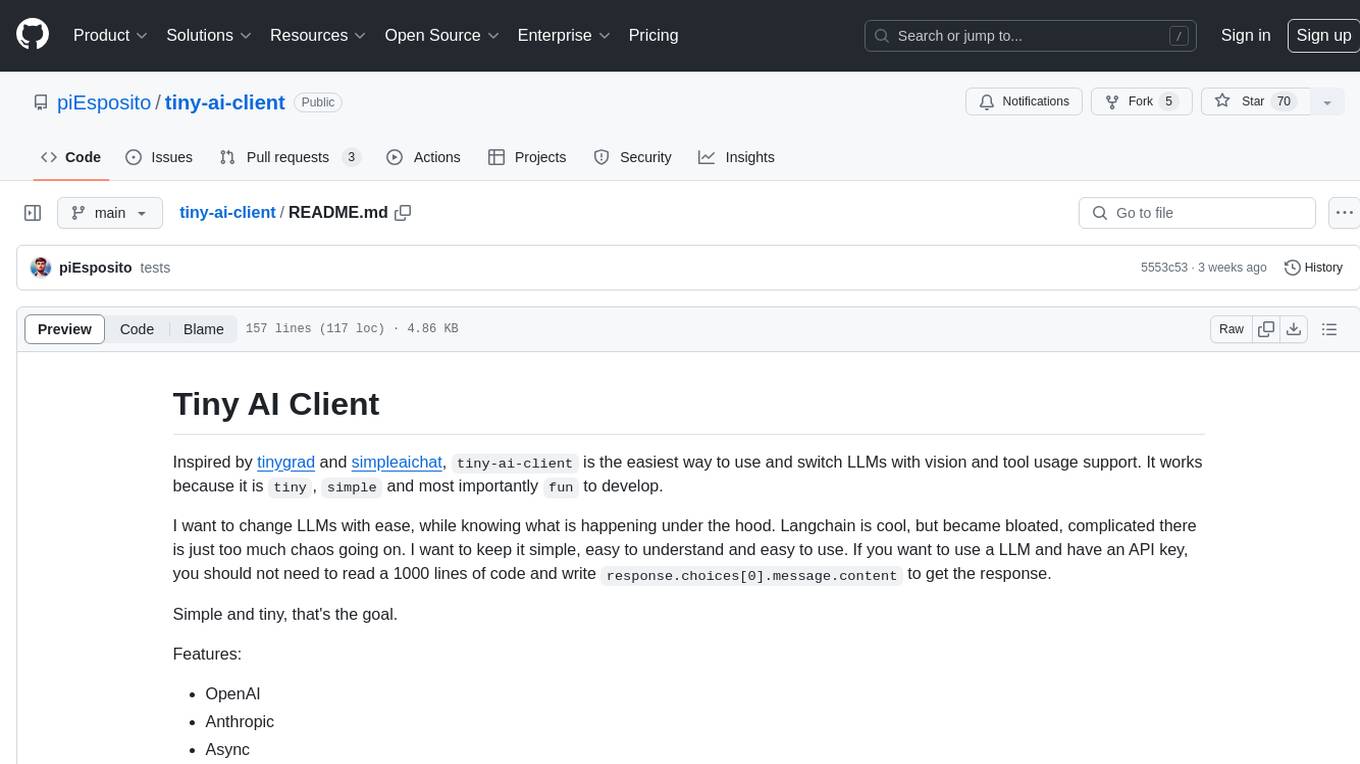
tiny-ai-client
Tiny AI Client is a lightweight tool designed for easy usage and switching of Language Model Models (LLMs) with support for vision and tool usage. It aims to provide a simple and intuitive interface for interacting with various LLMs, allowing users to easily set, change models, send messages, use tools, and handle vision tasks. The core logic of the tool is kept minimal and easy to understand, with separate modules for vision and tool usage utilities. Users can interact with the tool through simple Python scripts, passing model names, messages, tools, and images as required.
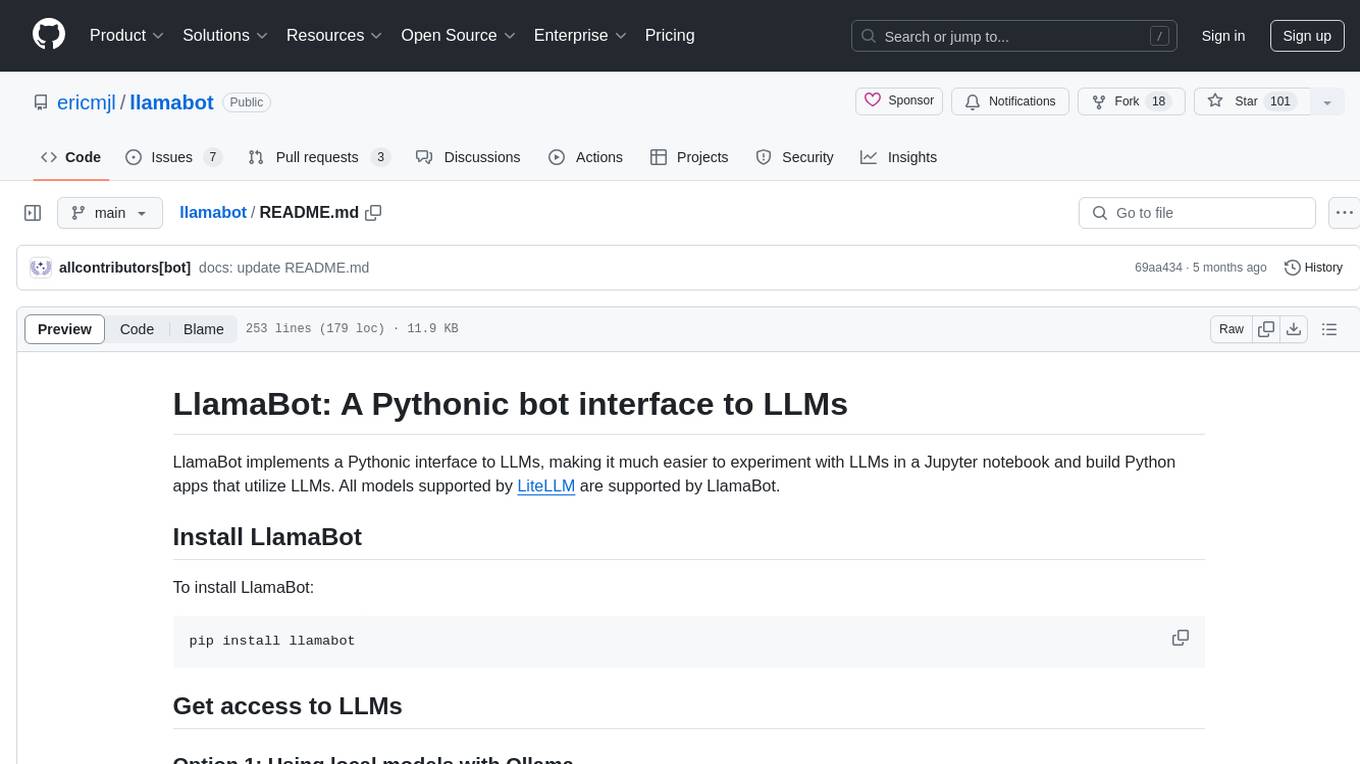
llamabot
LlamaBot is a Pythonic bot interface to Large Language Models (LLMs), providing an easy way to experiment with LLMs in Jupyter notebooks and build Python apps utilizing LLMs. It supports all models available in LiteLLM. Users can access LLMs either through local models with Ollama or by using API providers like OpenAI and Mistral. LlamaBot offers different bot interfaces like SimpleBot, ChatBot, QueryBot, and ImageBot for various tasks such as rephrasing text, maintaining chat history, querying documents, and generating images. The tool also includes CLI demos showcasing its capabilities and supports contributions for new features and bug reports from the community.
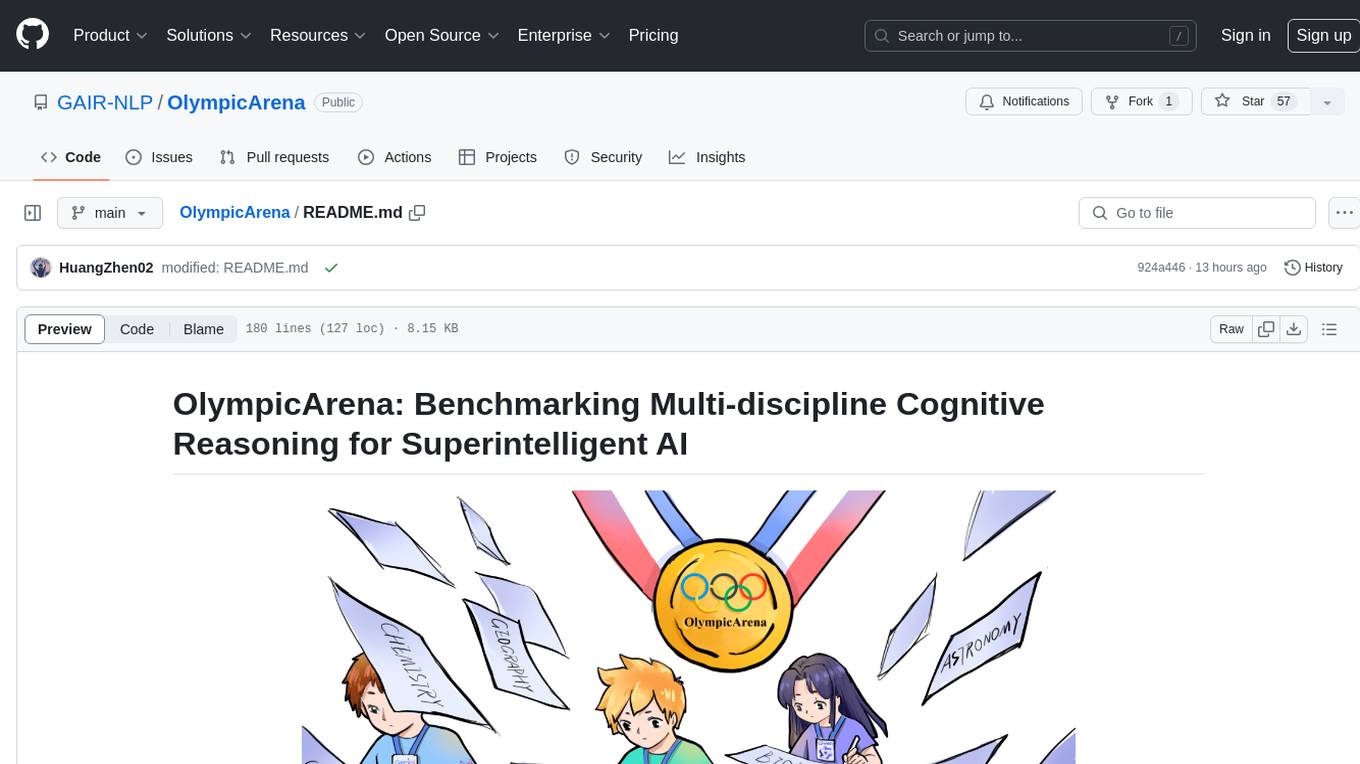
OlympicArena
OlympicArena is a comprehensive benchmark designed to evaluate advanced AI capabilities across various disciplines. It aims to push AI towards superintelligence by tackling complex challenges in science and beyond. The repository provides detailed data for different disciplines, allows users to run inference and evaluation locally, and offers a submission platform for testing models on the test set. Additionally, it includes an annotation interface and encourages users to cite their paper if they find the code or dataset helpful.
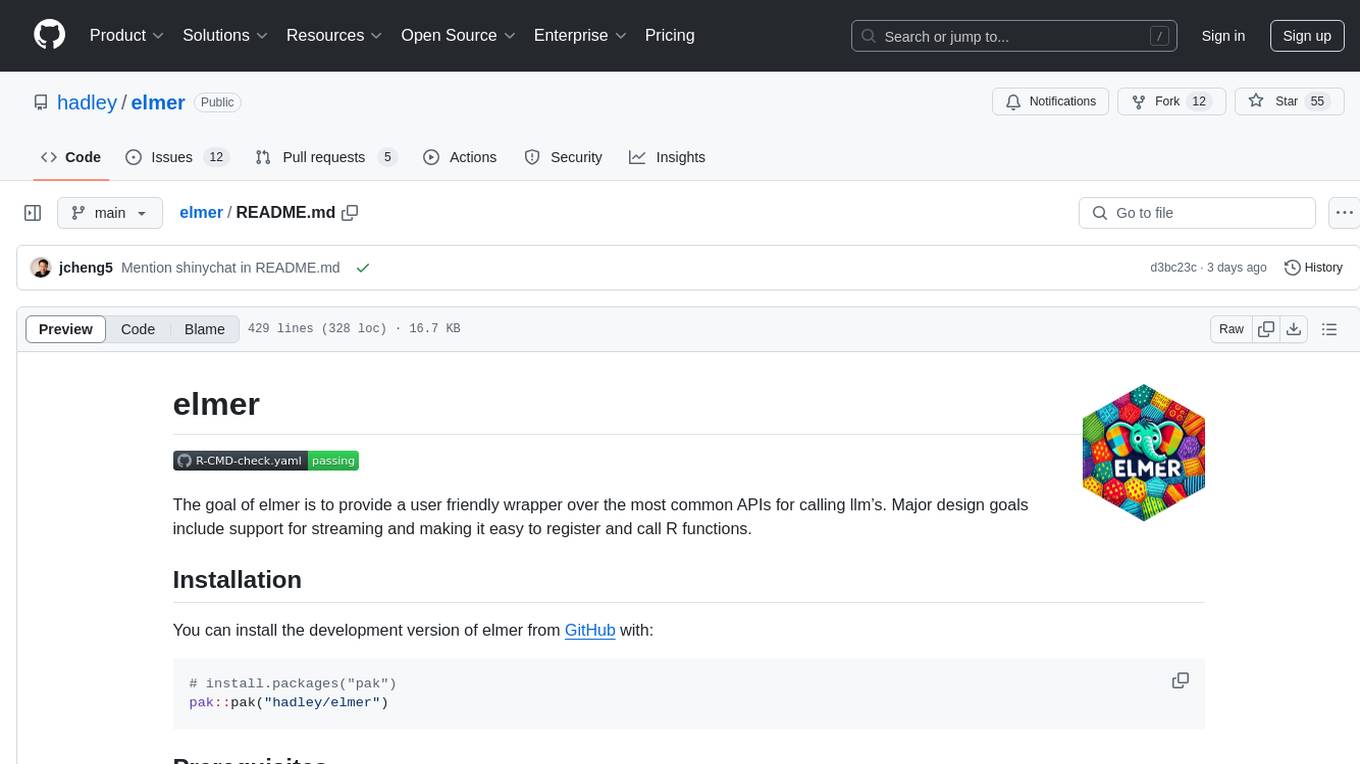
elmer
Elmer is a user-friendly wrapper over common APIs for calling llm’s, with support for streaming and easy registration and calling of R functions. Users can interact with Elmer in various ways, such as interactive chat console, interactive method call, programmatic chat, and streaming results. Elmer also supports async usage for running multiple chat sessions concurrently, useful for Shiny applications. The tool calling feature allows users to define external tools that Elmer can request to execute, enhancing the capabilities of the chat model.
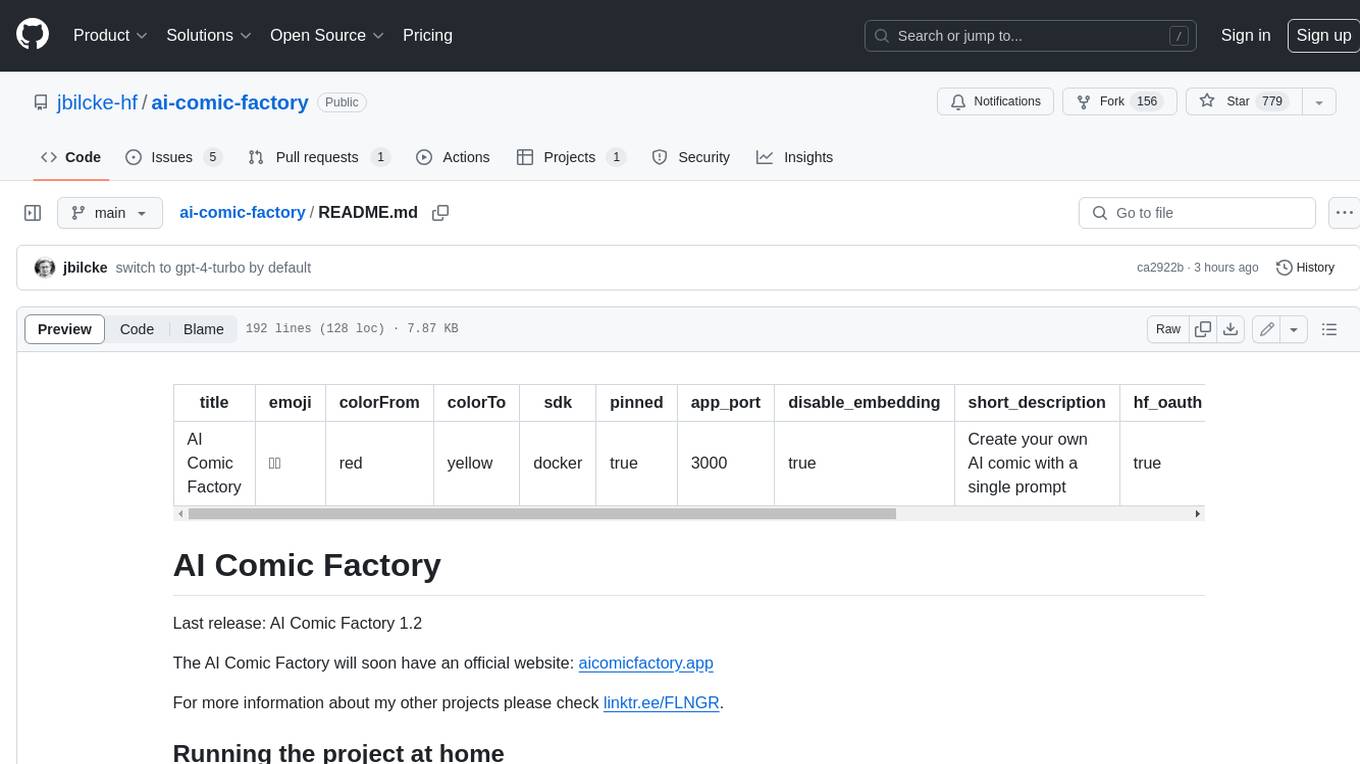
ai-comic-factory
The AI Comic Factory is a tool that allows you to create your own AI comics with a single prompt. It uses a large language model (LLM) to generate the story and dialogue, and a rendering API to generate the panel images. The AI Comic Factory is open-source and can be run on your own website or computer. It is a great tool for anyone who wants to create their own comics, or for anyone who is interested in the potential of AI for storytelling.
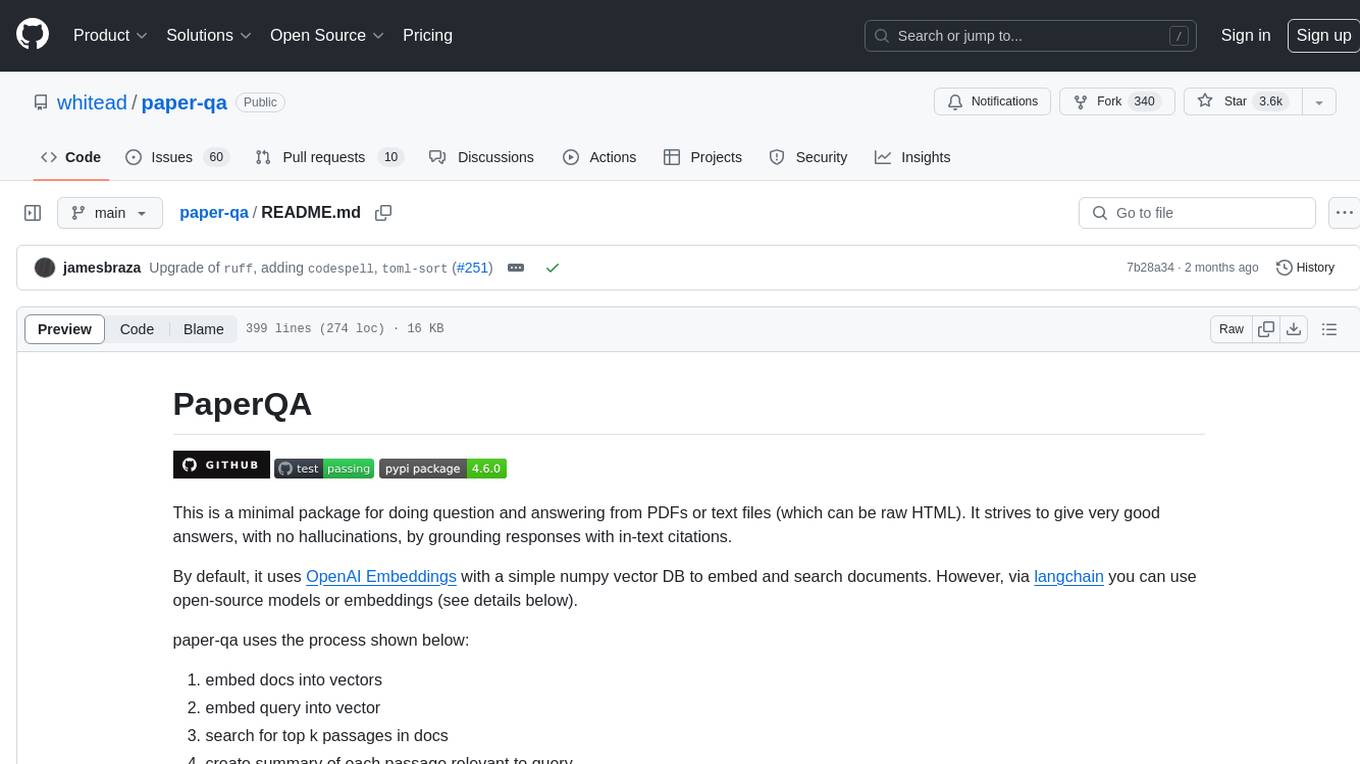
paper-qa
PaperQA is a minimal package for question and answering from PDFs or text files, providing very good answers with in-text citations. It uses OpenAI Embeddings to embed and search documents, and follows a process of embedding docs and queries, searching for top passages, creating summaries, scoring and selecting relevant summaries, putting summaries into prompt, and generating answers. Users can customize prompts and use various models for embeddings and LLMs. The tool can be used asynchronously and supports adding documents from paths, files, or URLs.
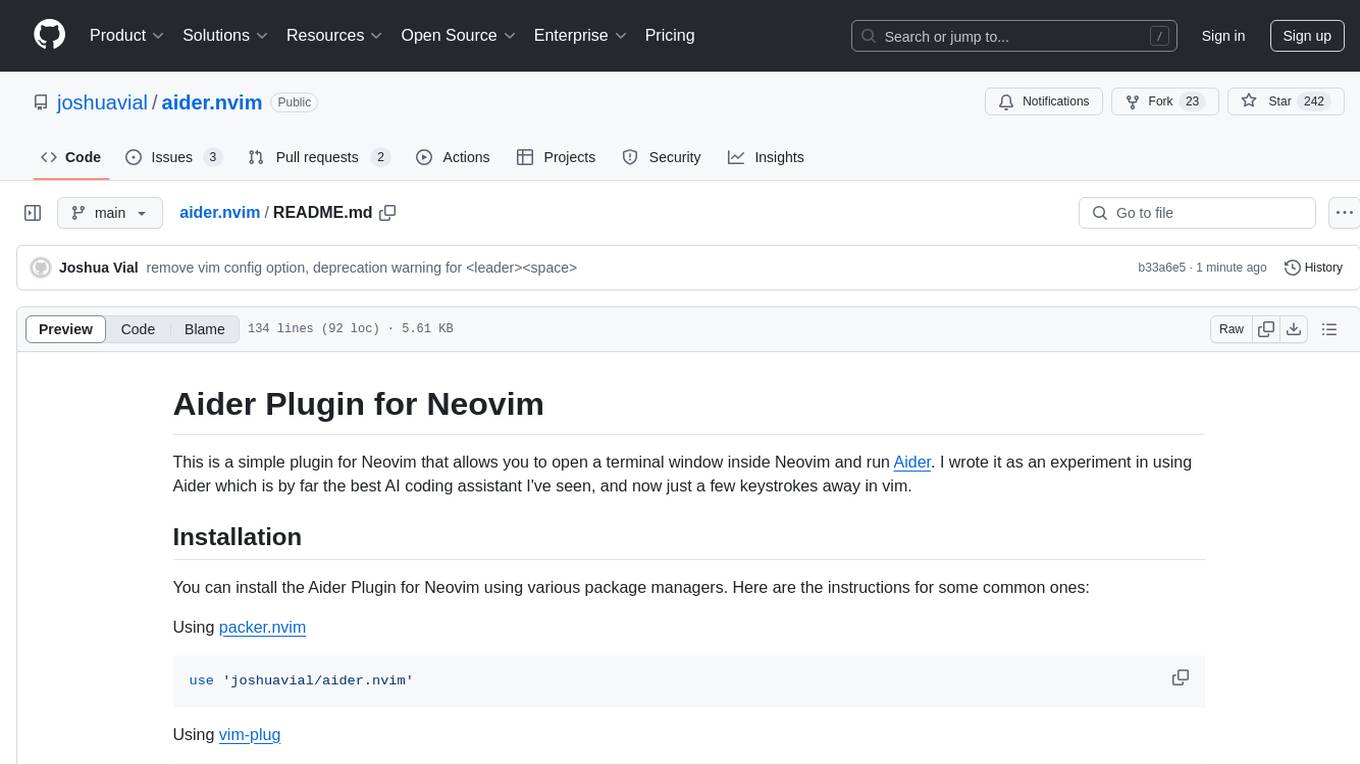
aider.nvim
Aider.nvim is a Neovim plugin that integrates the Aider AI coding assistant, allowing users to open a terminal window within Neovim to run Aider. It provides functions like AiderOpen to open the terminal window, AiderAddModifiedFiles to add git-modified files to the Aider chat, and customizable keybindings. Users can configure the plugin using the setup function to manage context, keybindings, debug logging, and ignore specific buffer names.
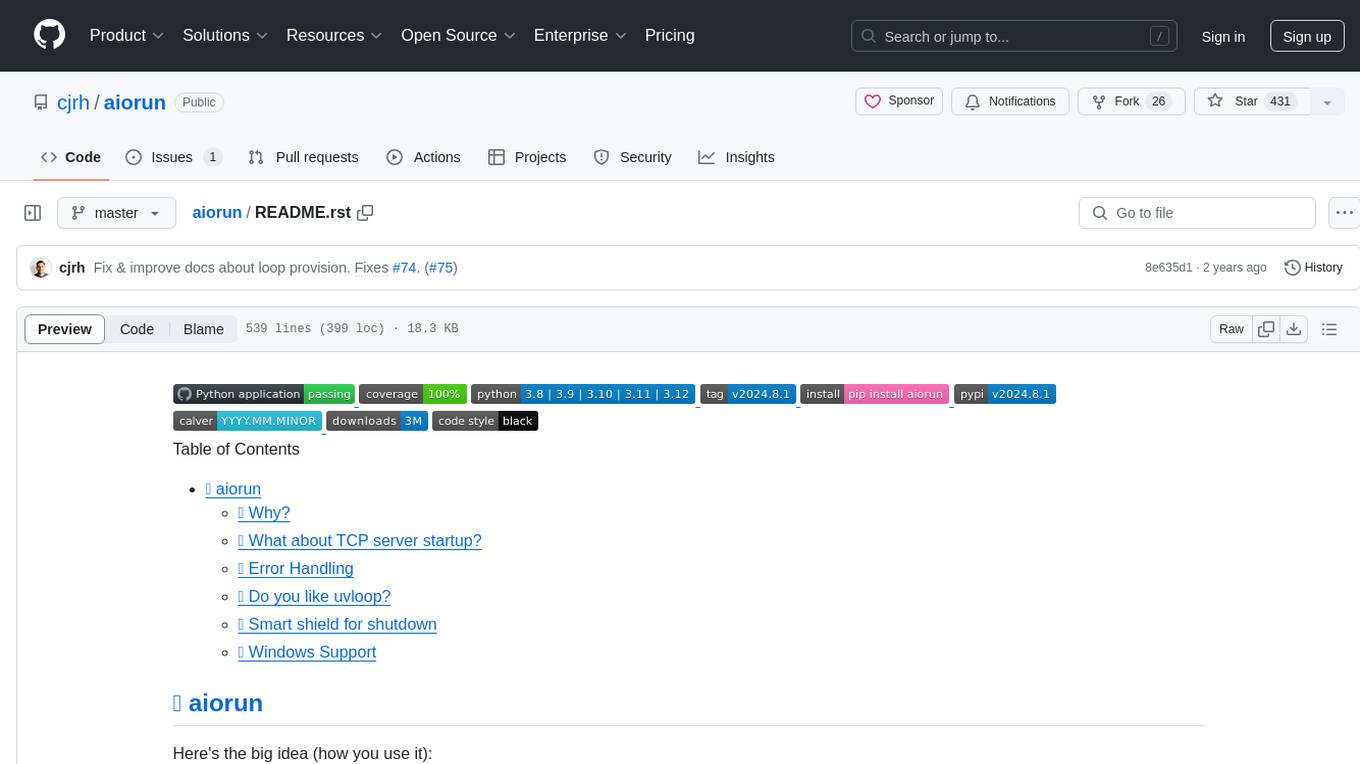
aiorun
aiorun is a Python package that provides a `run()` function as the starting point of your `asyncio`-based application. The `run()` function handles everything needed during the shutdown sequence of the application, such as creating a `Task` for the given coroutine, running the event loop, adding signal handlers for `SIGINT` and `SIGTERM`, cancelling tasks, waiting for the executor to complete shutdown, and closing the loop. It automates standard actions for asyncio apps, eliminating the need to write boilerplate code. The package also offers error handling options and tools for specific scenarios like TCP server startup and smart shield for shutdown.
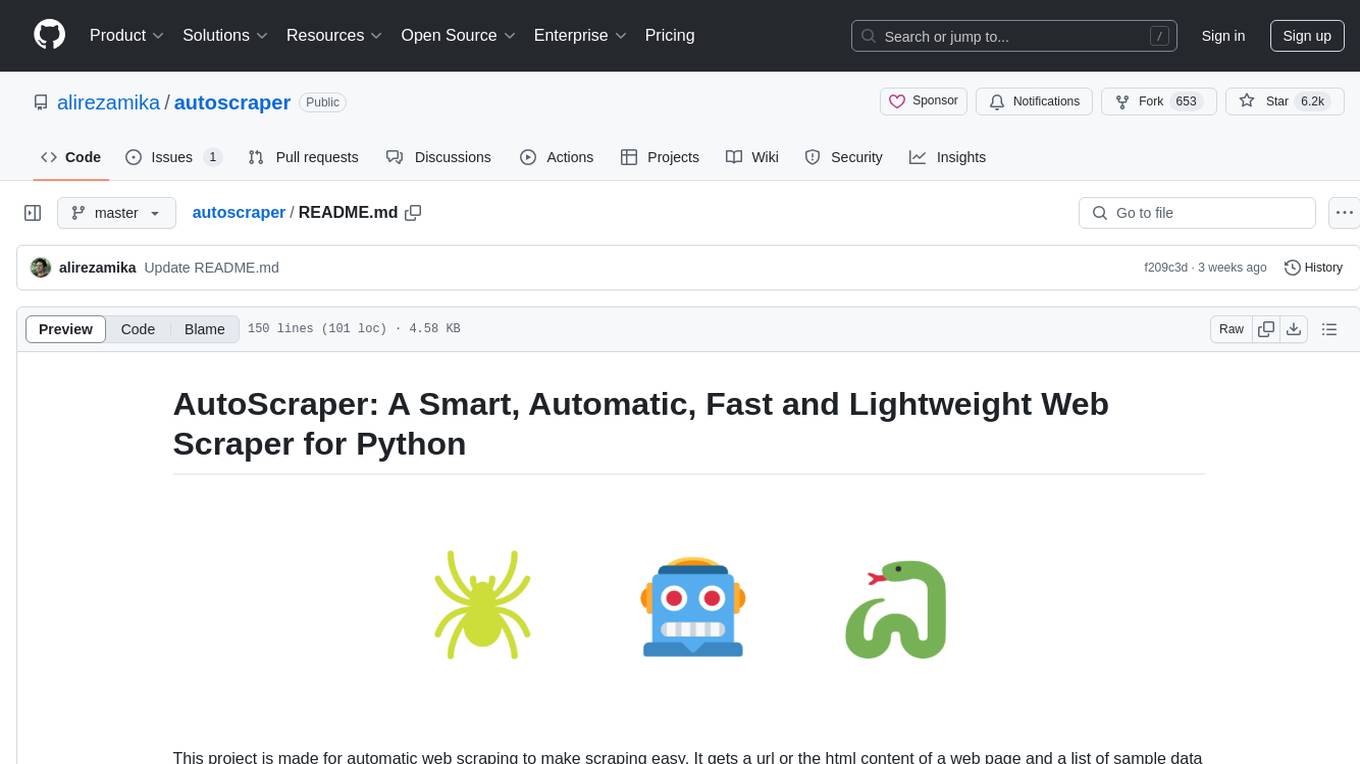
autoscraper
AutoScraper is a smart, automatic, fast, and lightweight web scraping tool for Python. It simplifies the process of web scraping by learning scraping rules based on sample data provided by the user. The tool can extract text, URLs, or HTML tag values from web pages and return similar elements. Users can utilize the learned object to scrape similar content or exact elements from new pages. AutoScraper is compatible with Python 3 and offers easy installation from various sources. It provides functionalities for fetching similar and exact results from web pages, such as extracting post titles from Stack Overflow or live stock prices from Yahoo Finance. The tool allows customization with custom requests module parameters like proxies or headers. Users can save and load models for future use and explore advanced usages through tutorials and examples.
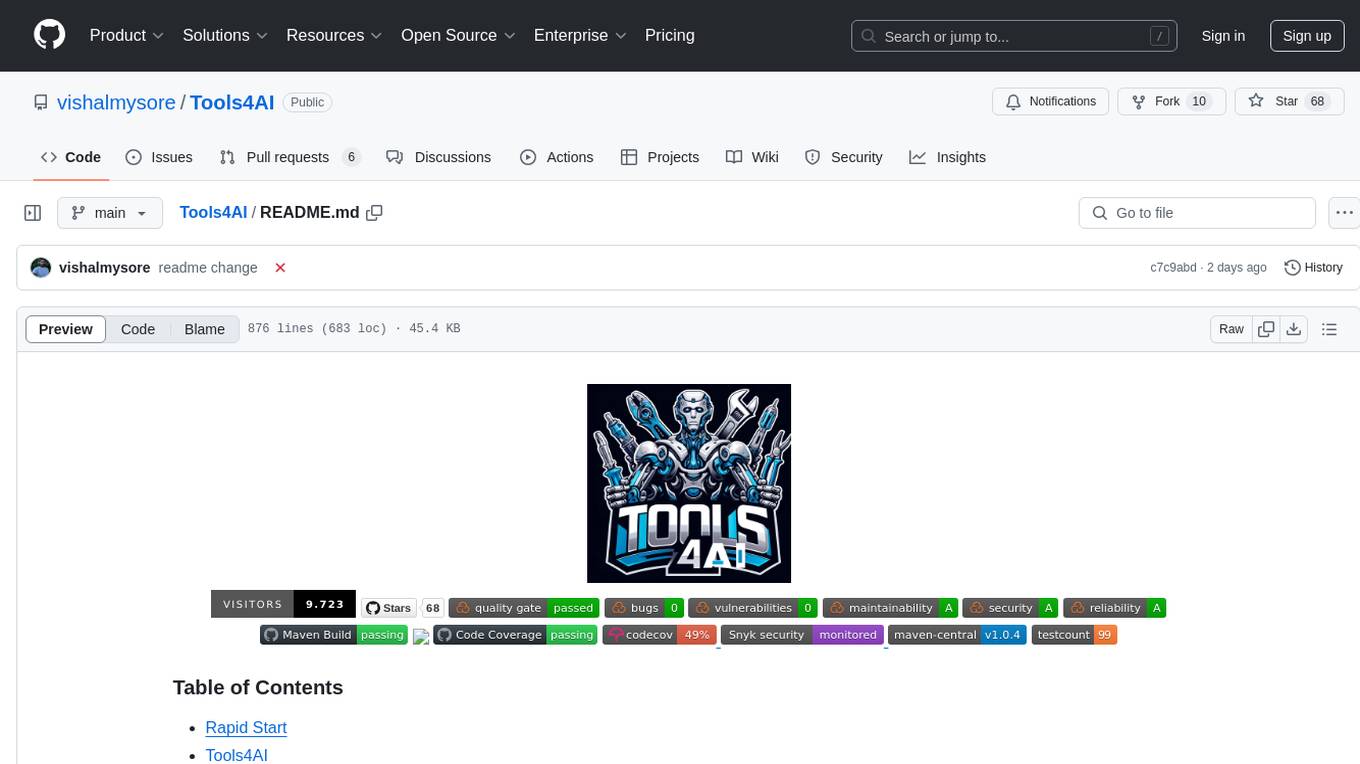
Tools4AI
Tools4AI is a Java-based Agentic Framework for building AI agents to integrate with enterprise Java applications. It enables the conversion of natural language prompts into actionable behaviors, streamlining user interactions with complex systems. By leveraging AI capabilities, it enhances productivity and innovation across diverse applications. The framework allows for seamless integration of AI with various systems, such as customer service applications, to interpret user requests, trigger actions, and streamline workflows. Prompt prediction anticipates user actions based on input prompts, enhancing user experience by proactively suggesting relevant actions or services based on context.
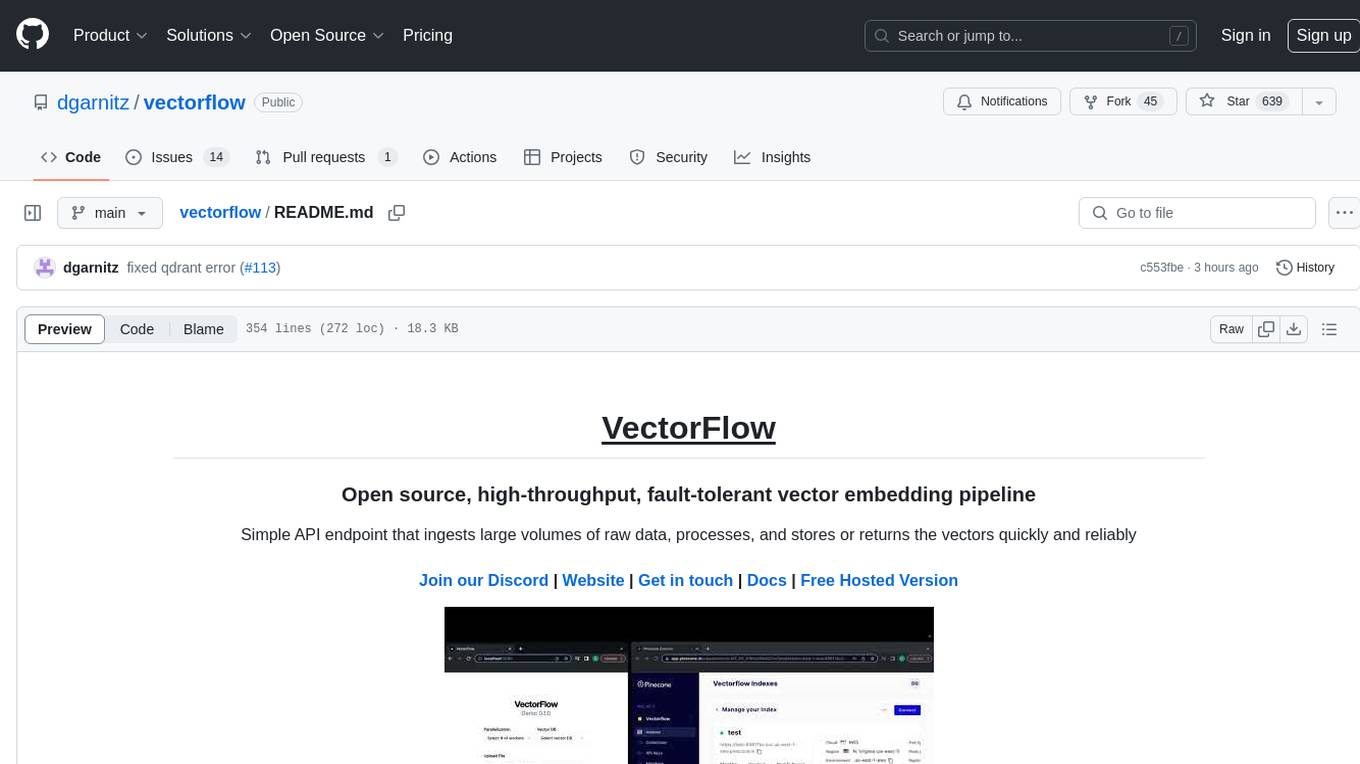
vectorflow
VectorFlow is an open source, high throughput, fault tolerant vector embedding pipeline. It provides a simple API endpoint for ingesting large volumes of raw data, processing, and storing or returning the vectors quickly and reliably. The tool supports text-based files like TXT, PDF, HTML, and DOCX, and can be run locally with Kubernetes in production. VectorFlow offers functionalities like embedding documents, running chunking schemas, custom chunking, and integrating with vector databases like Pinecone, Qdrant, and Weaviate. It enforces a standardized schema for uploading data to a vector store and supports features like raw embeddings webhook, chunk validation webhook, S3 endpoint, and telemetry. The tool can be used with the Python client and provides detailed instructions for running and testing the functionalities.
For similar tasks
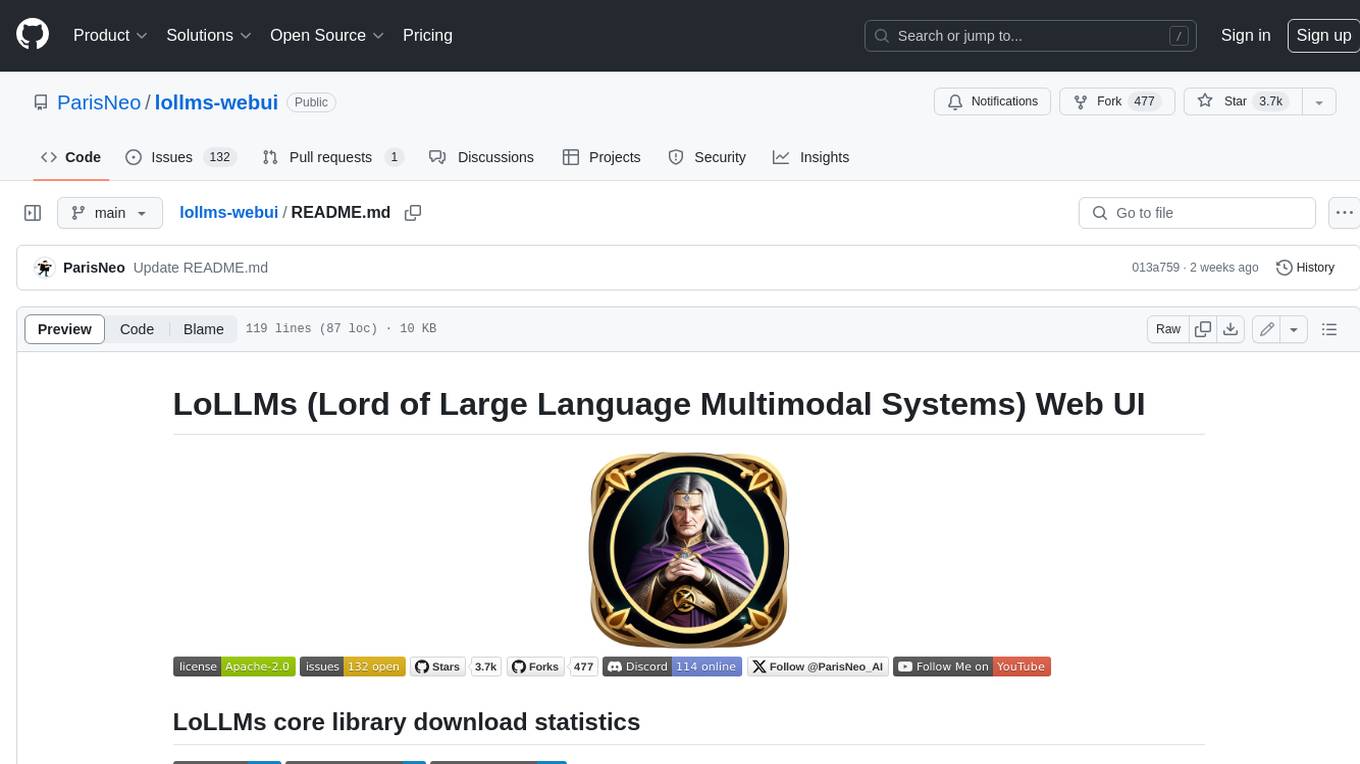
lollms-webui
LoLLMs WebUI (Lord of Large Language Multimodal Systems: One tool to rule them all) is a user-friendly interface to access and utilize various LLM (Large Language Models) and other AI models for a wide range of tasks. With over 500 AI expert conditionings across diverse domains and more than 2500 fine tuned models over multiple domains, LoLLMs WebUI provides an immediate resource for any problem, from car repair to coding assistance, legal matters, medical diagnosis, entertainment, and more. The easy-to-use UI with light and dark mode options, integration with GitHub repository, support for different personalities, and features like thumb up/down rating, copy, edit, and remove messages, local database storage, search, export, and delete multiple discussions, make LoLLMs WebUI a powerful and versatile tool.
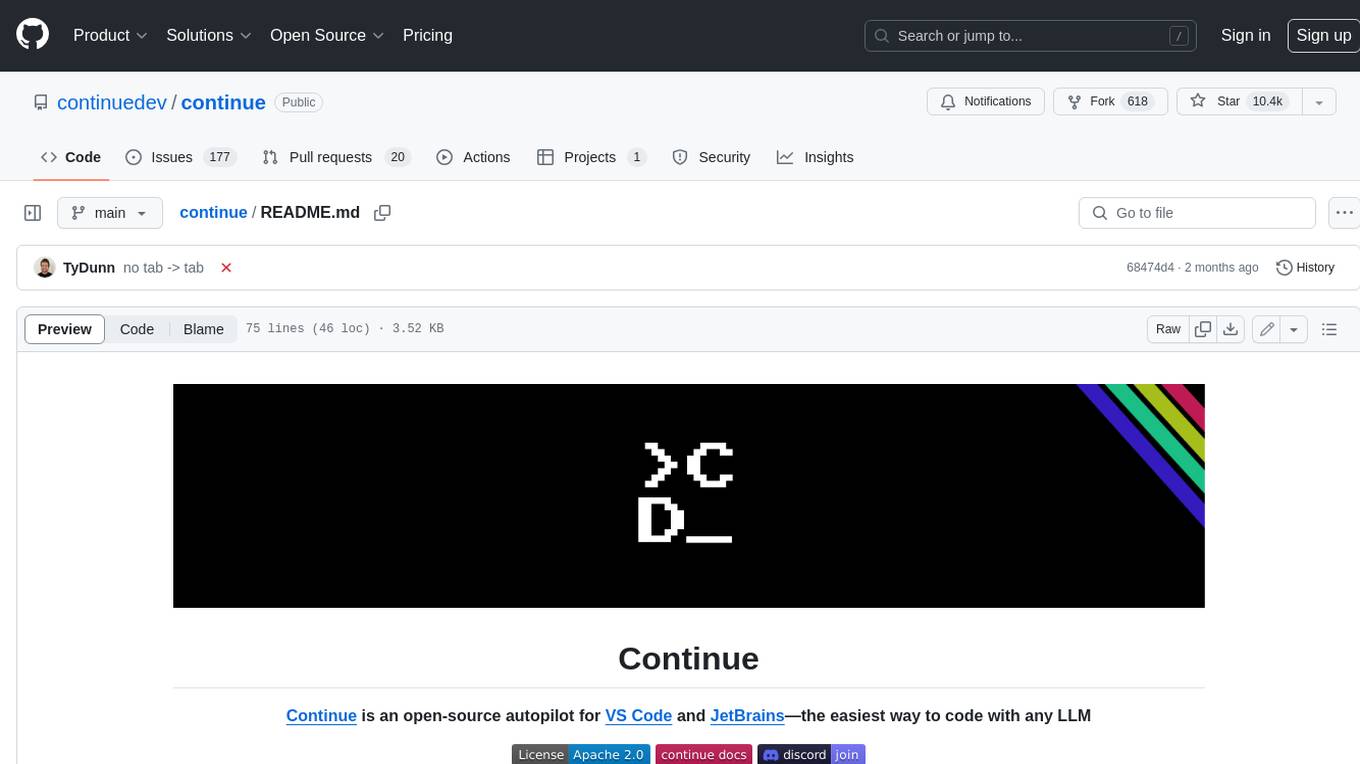
continue
Continue is an open-source autopilot for VS Code and JetBrains that allows you to code with any LLM. With Continue, you can ask coding questions, edit code in natural language, generate files from scratch, and more. Continue is easy to use and can help you save time and improve your coding skills.
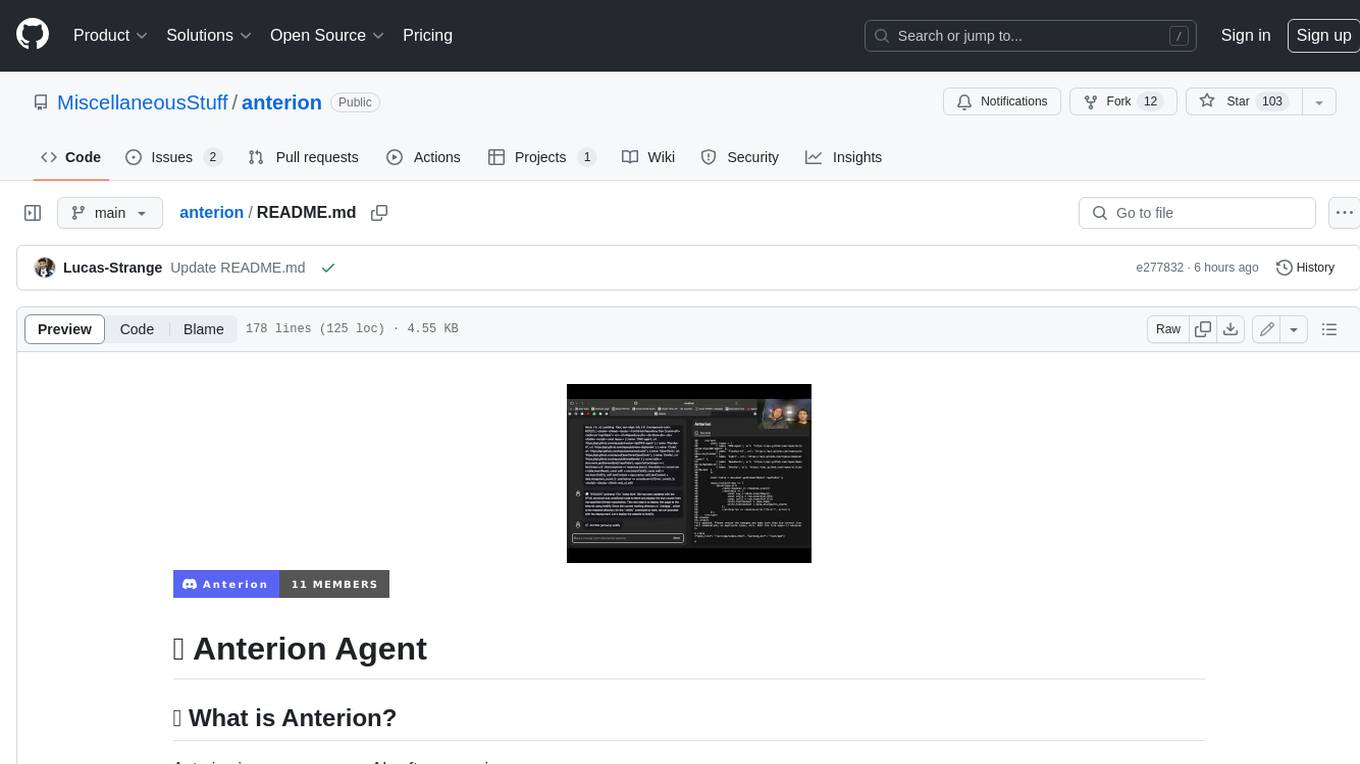
anterion
Anterion is an open-source AI software engineer that extends the capabilities of `SWE-agent` to plan and execute open-ended engineering tasks, with a frontend inspired by `OpenDevin`. It is designed to help users fix bugs and prototype ideas with ease. Anterion is equipped with easy deployment and a user-friendly interface, making it accessible to users of all skill levels.
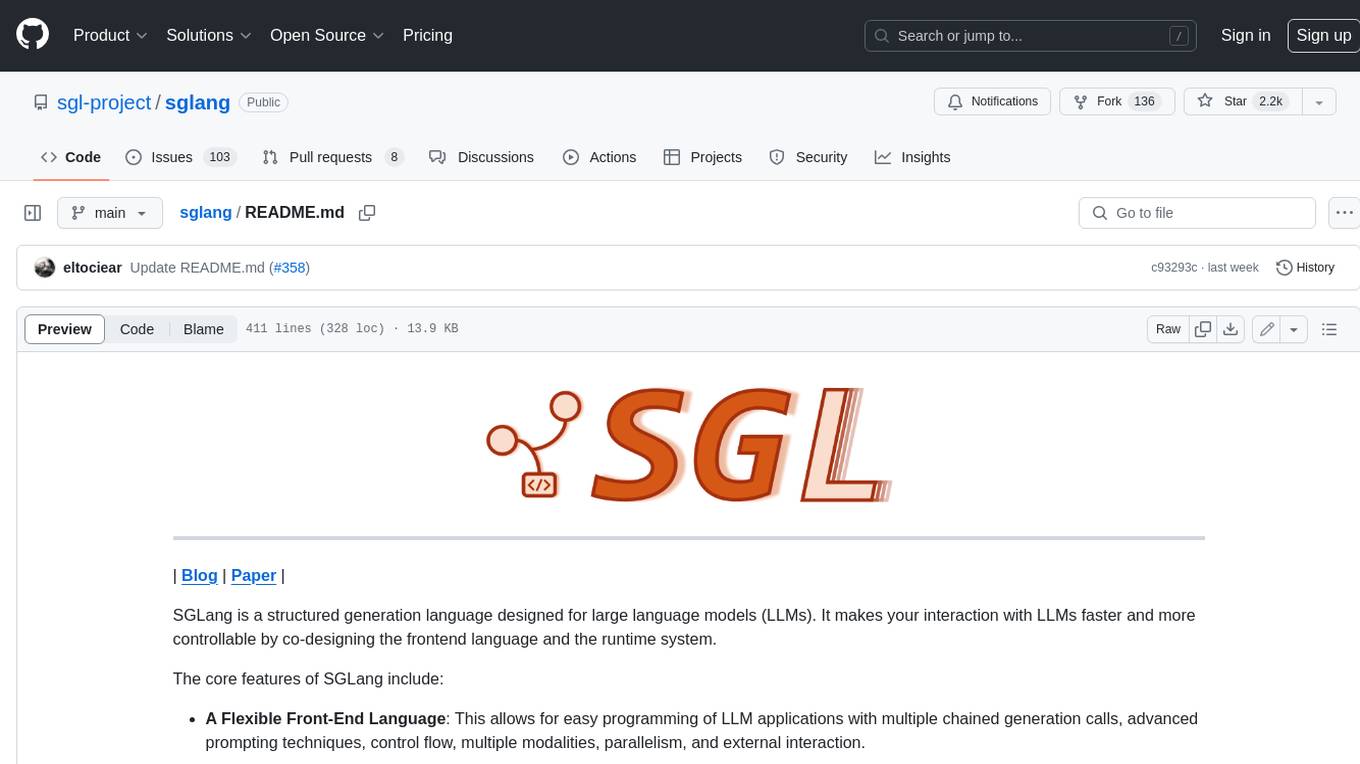
sglang
SGLang is a structured generation language designed for large language models (LLMs). It makes your interaction with LLMs faster and more controllable by co-designing the frontend language and the runtime system. The core features of SGLang include: - **A Flexible Front-End Language**: This allows for easy programming of LLM applications with multiple chained generation calls, advanced prompting techniques, control flow, multiple modalities, parallelism, and external interaction. - **A High-Performance Runtime with RadixAttention**: This feature significantly accelerates the execution of complex LLM programs by automatic KV cache reuse across multiple calls. It also supports other common techniques like continuous batching and tensor parallelism.
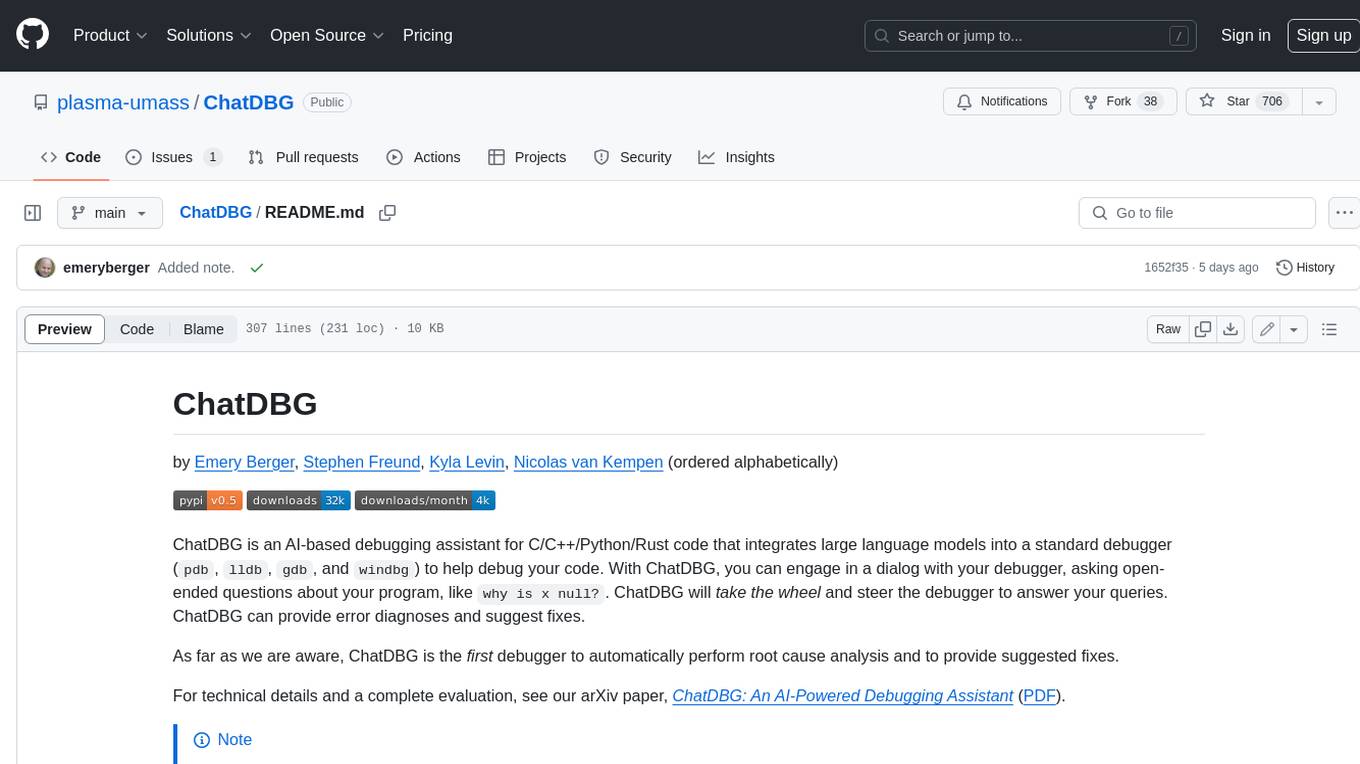
ChatDBG
ChatDBG is an AI-based debugging assistant for C/C++/Python/Rust code that integrates large language models into a standard debugger (`pdb`, `lldb`, `gdb`, and `windbg`) to help debug your code. With ChatDBG, you can engage in a dialog with your debugger, asking open-ended questions about your program, like `why is x null?`. ChatDBG will _take the wheel_ and steer the debugger to answer your queries. ChatDBG can provide error diagnoses and suggest fixes. As far as we are aware, ChatDBG is the _first_ debugger to automatically perform root cause analysis and to provide suggested fixes.
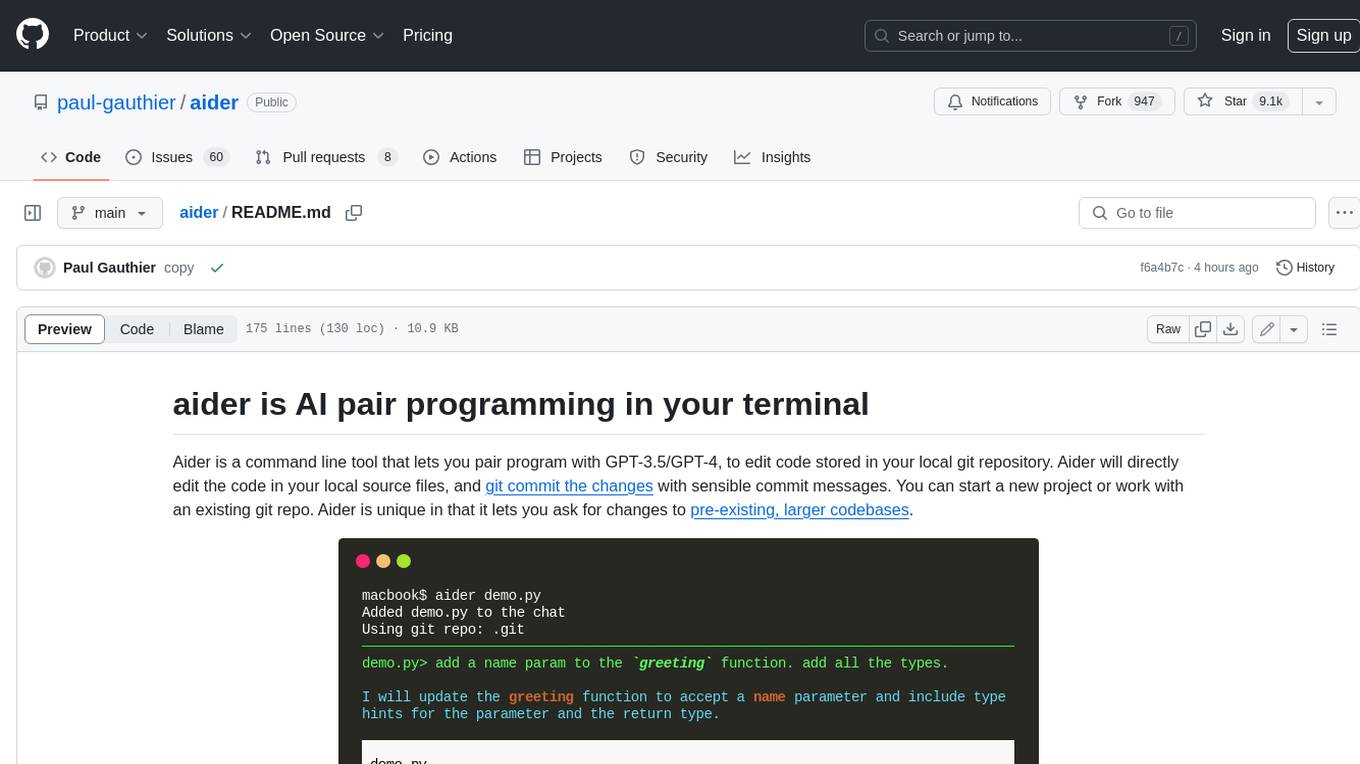
aider
Aider is a command-line tool that lets you pair program with GPT-3.5/GPT-4 to edit code stored in your local git repository. Aider will directly edit the code in your local source files and git commit the changes with sensible commit messages. You can start a new project or work with an existing git repo. Aider is unique in that it lets you ask for changes to pre-existing, larger codebases.
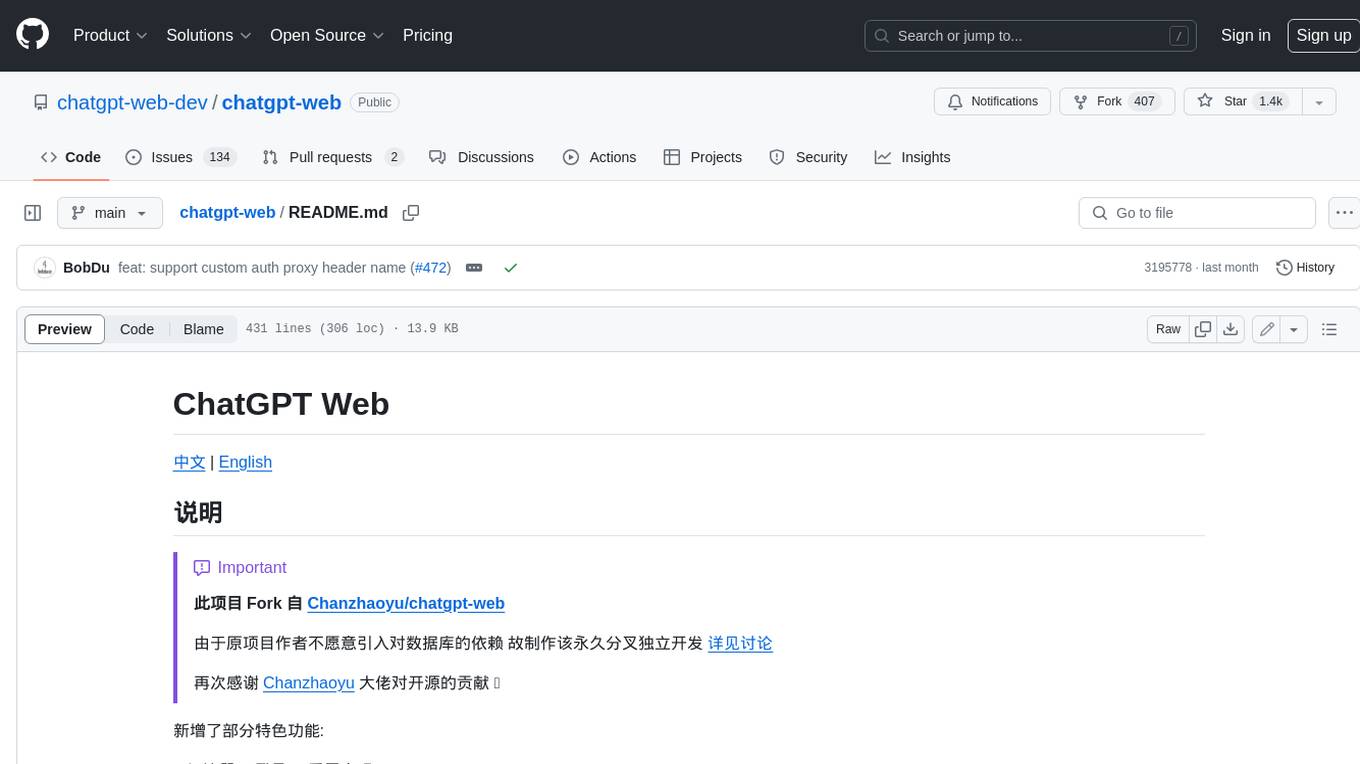
chatgpt-web
ChatGPT Web is a web application that provides access to the ChatGPT API. It offers two non-official methods to interact with ChatGPT: through the ChatGPTAPI (using the `gpt-3.5-turbo-0301` model) or through the ChatGPTUnofficialProxyAPI (using a web access token). The ChatGPTAPI method is more reliable but requires an OpenAI API key, while the ChatGPTUnofficialProxyAPI method is free but less reliable. The application includes features such as user registration and login, synchronization of conversation history, customization of API keys and sensitive words, and management of users and keys. It also provides a user interface for interacting with ChatGPT and supports multiple languages and themes.
For similar jobs
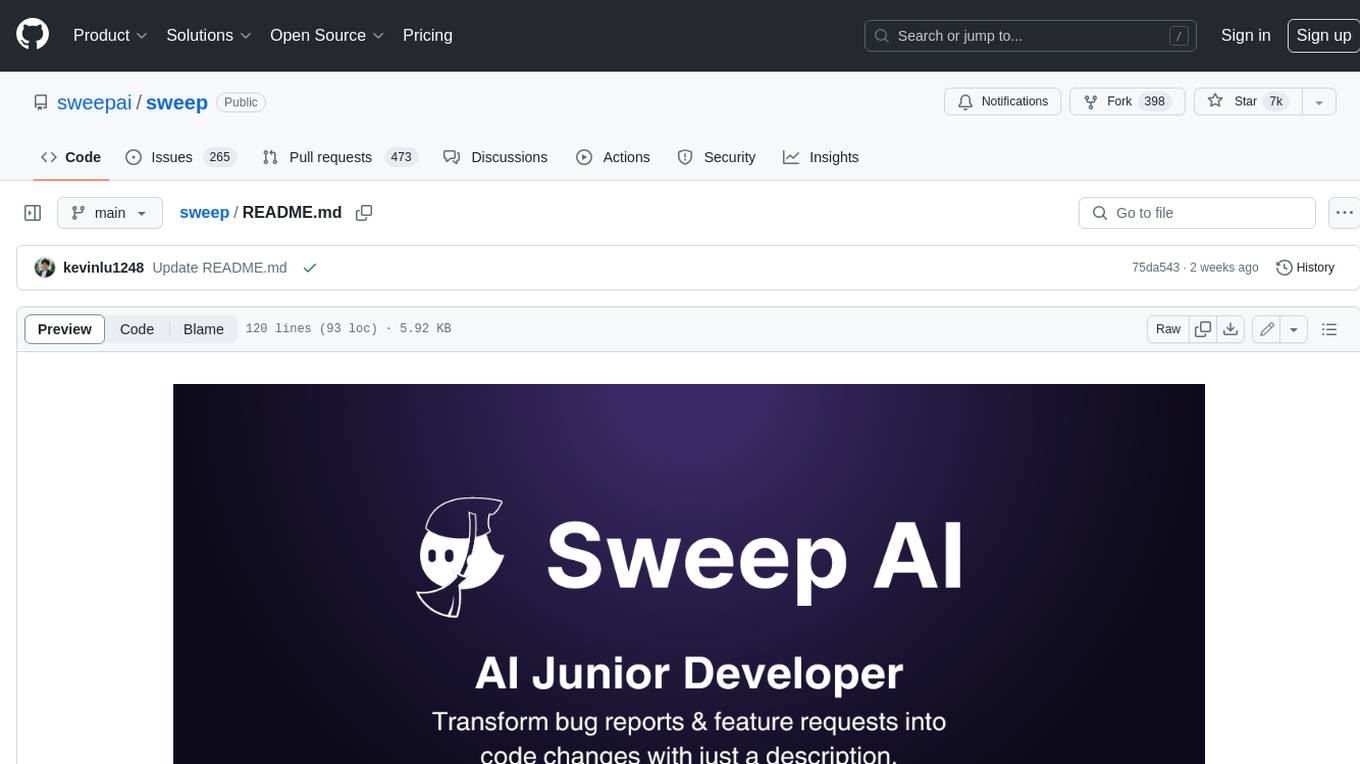
sweep
Sweep is an AI junior developer that turns bugs and feature requests into code changes. It automatically handles developer experience improvements like adding type hints and improving test coverage.
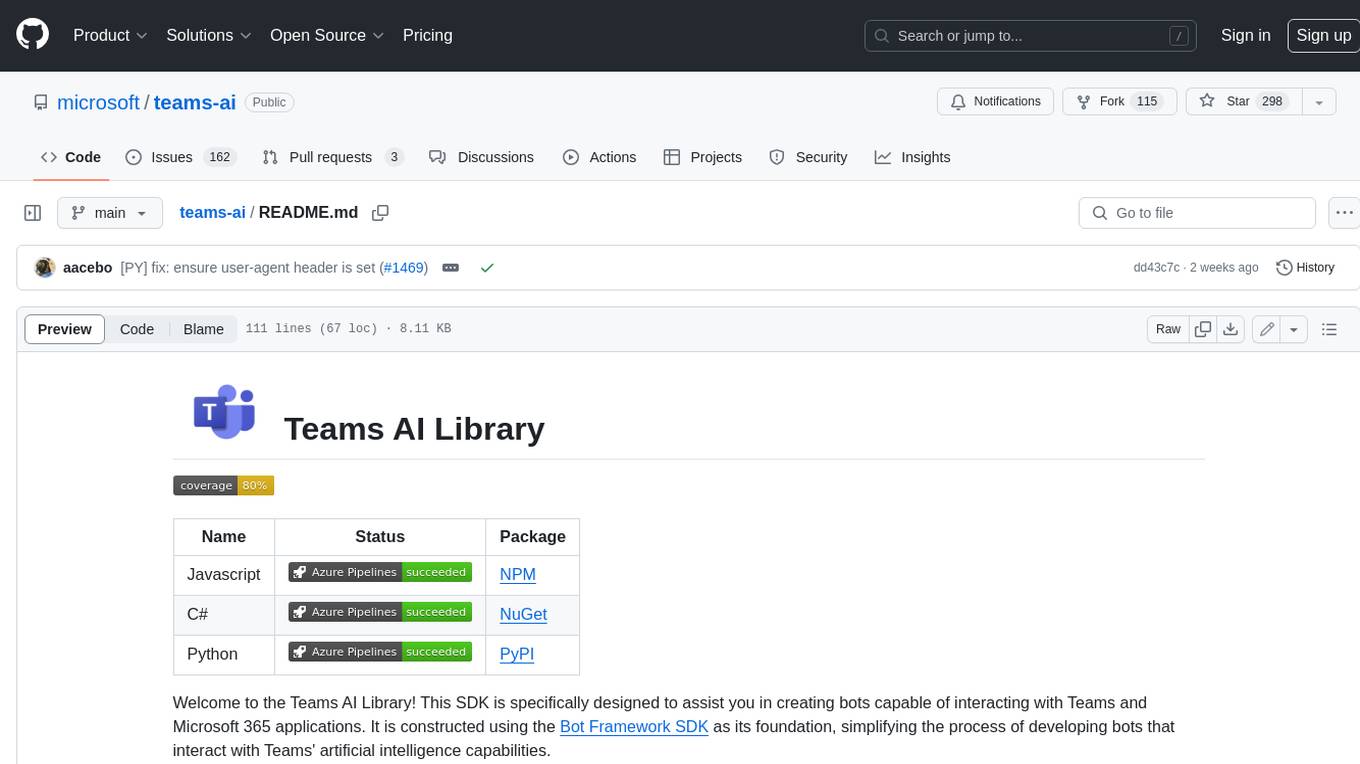
teams-ai
The Teams AI Library is a software development kit (SDK) that helps developers create bots that can interact with Teams and Microsoft 365 applications. It is built on top of the Bot Framework SDK and simplifies the process of developing bots that interact with Teams' artificial intelligence capabilities. The SDK is available for JavaScript/TypeScript, .NET, and Python.
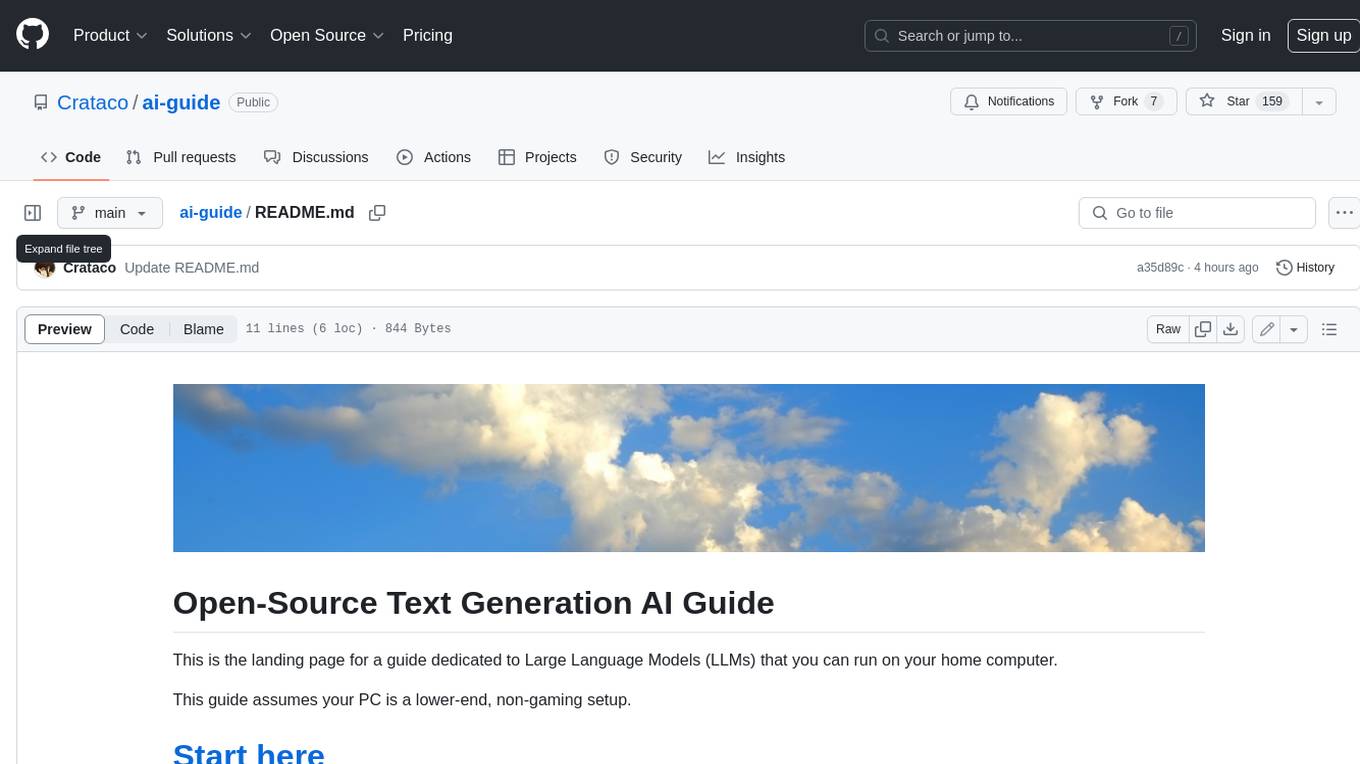
ai-guide
This guide is dedicated to Large Language Models (LLMs) that you can run on your home computer. It assumes your PC is a lower-end, non-gaming setup.
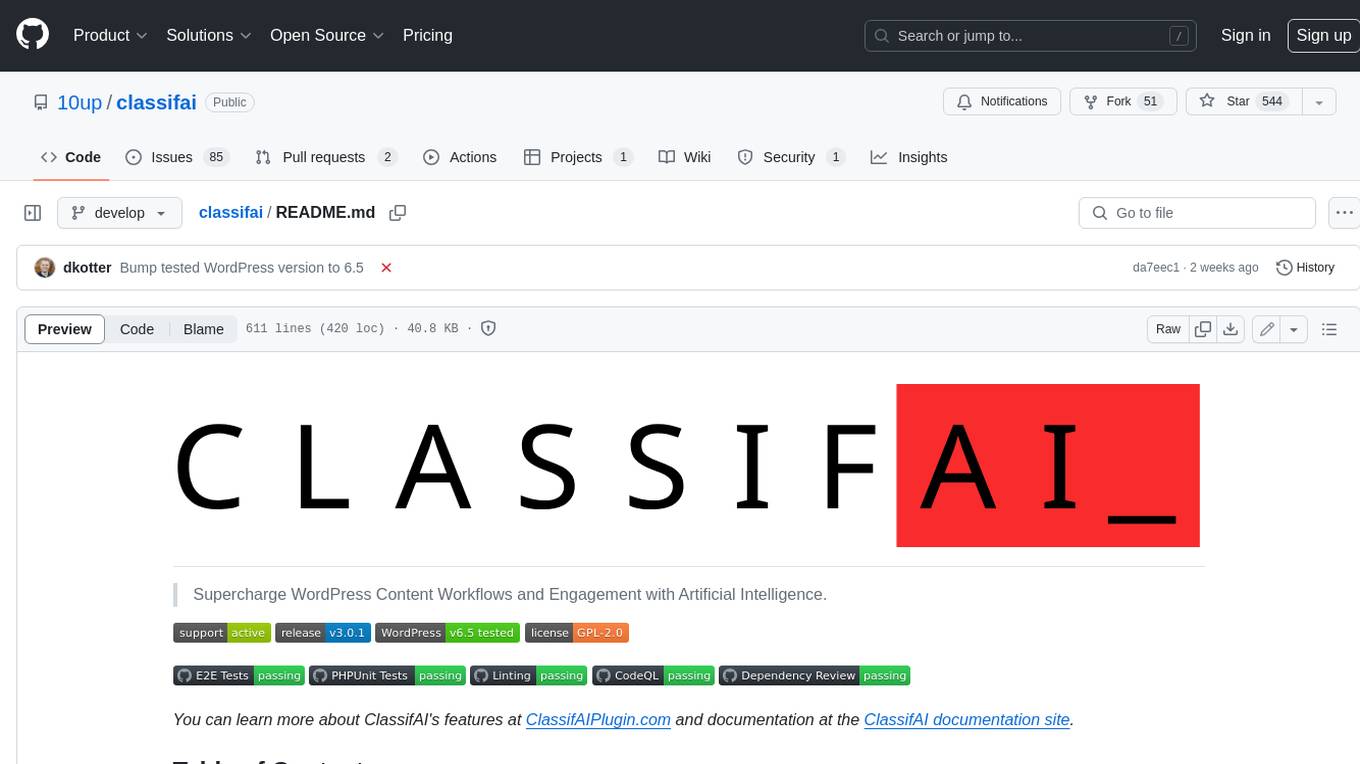
classifai
Supercharge WordPress Content Workflows and Engagement with Artificial Intelligence. Tap into leading cloud-based services like OpenAI, Microsoft Azure AI, Google Gemini and IBM Watson to augment your WordPress-powered websites. Publish content faster while improving SEO performance and increasing audience engagement. ClassifAI integrates Artificial Intelligence and Machine Learning technologies to lighten your workload and eliminate tedious tasks, giving you more time to create original content that matters.
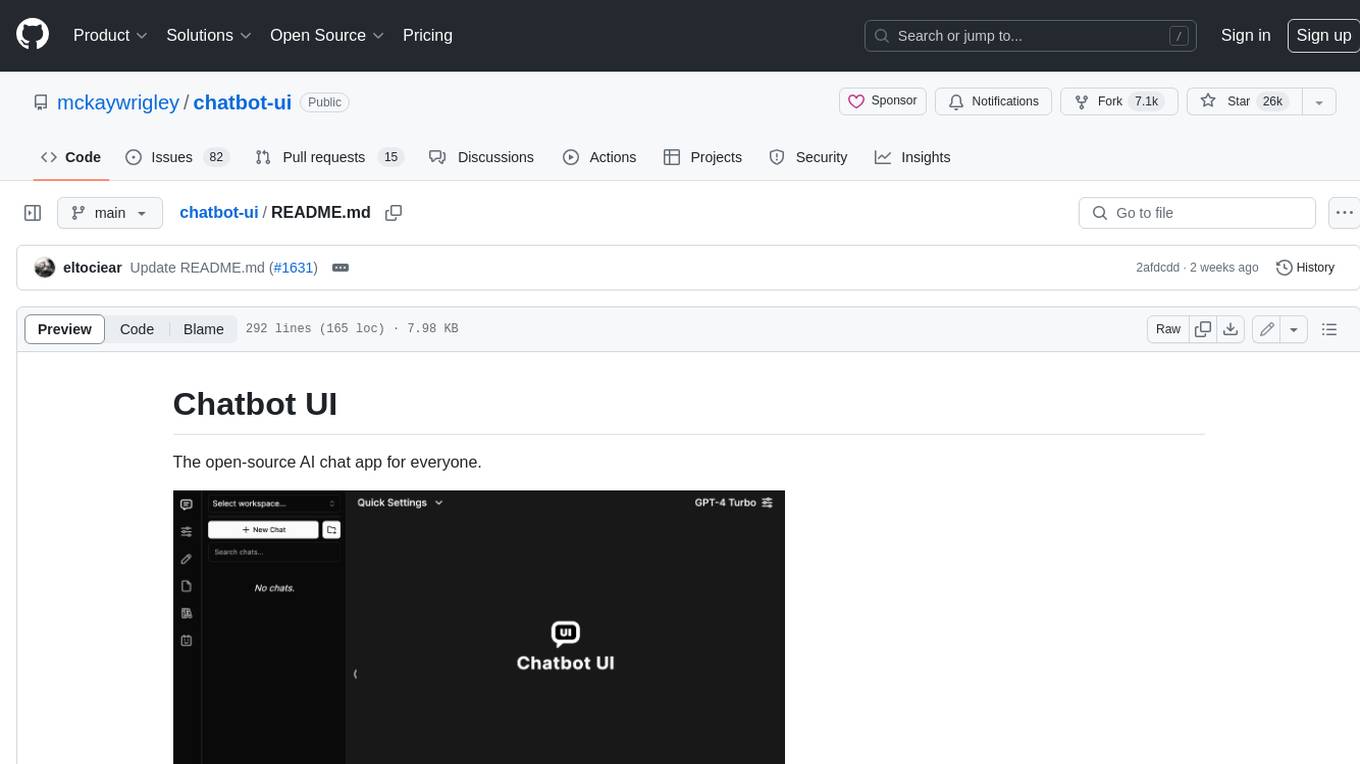
chatbot-ui
Chatbot UI is an open-source AI chat app that allows users to create and deploy their own AI chatbots. It is easy to use and can be customized to fit any need. Chatbot UI is perfect for businesses, developers, and anyone who wants to create a chatbot.
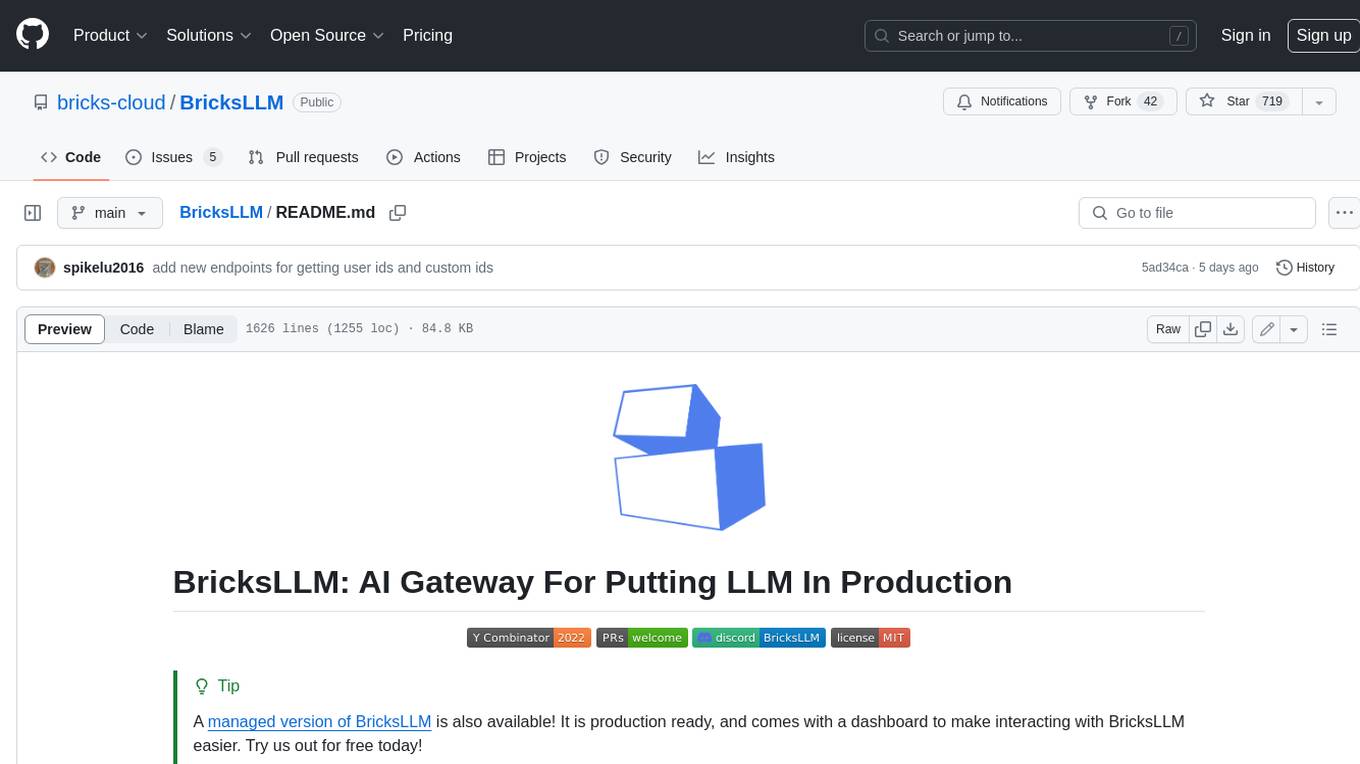
BricksLLM
BricksLLM is a cloud native AI gateway written in Go. Currently, it provides native support for OpenAI, Anthropic, Azure OpenAI and vLLM. BricksLLM aims to provide enterprise level infrastructure that can power any LLM production use cases. Here are some use cases for BricksLLM: * Set LLM usage limits for users on different pricing tiers * Track LLM usage on a per user and per organization basis * Block or redact requests containing PIIs * Improve LLM reliability with failovers, retries and caching * Distribute API keys with rate limits and cost limits for internal development/production use cases * Distribute API keys with rate limits and cost limits for students
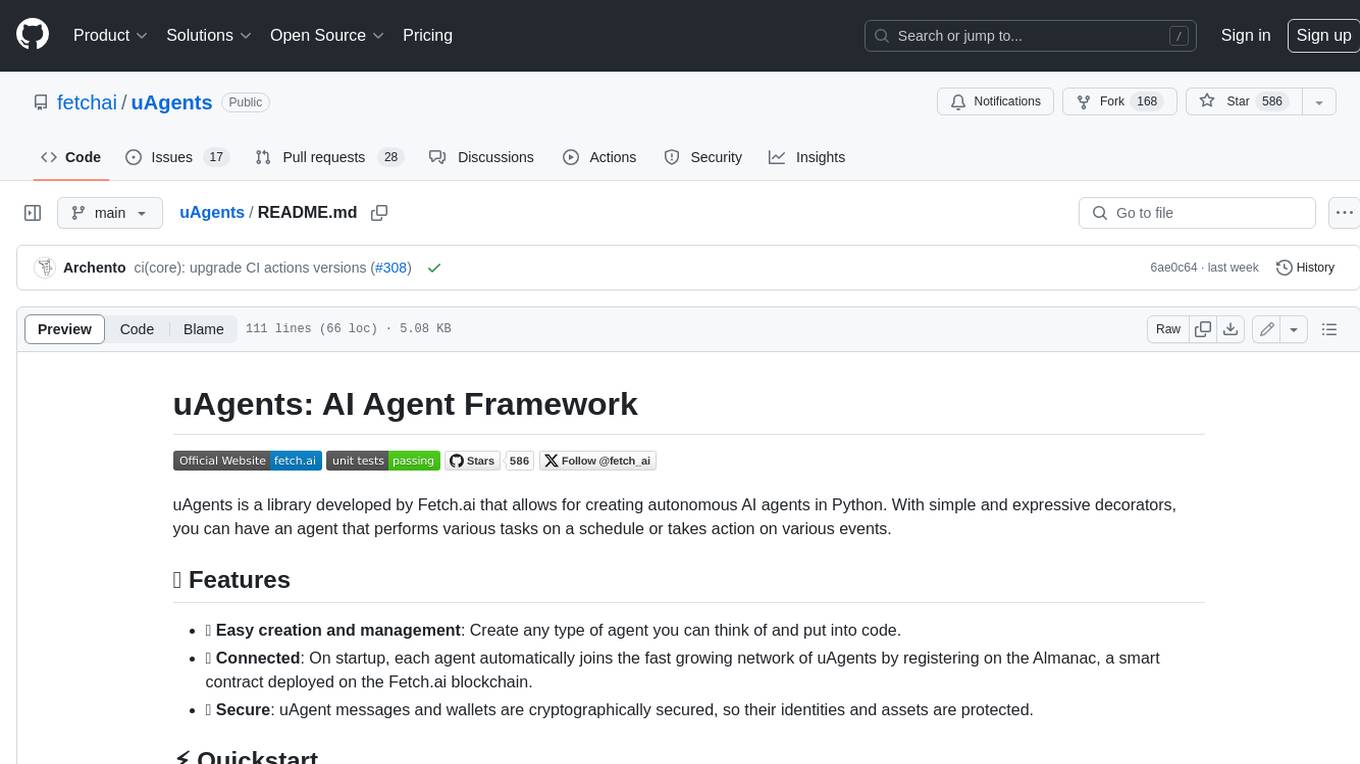
uAgents
uAgents is a Python library developed by Fetch.ai that allows for the creation of autonomous AI agents. These agents can perform various tasks on a schedule or take action on various events. uAgents are easy to create and manage, and they are connected to a fast-growing network of other uAgents. They are also secure, with cryptographically secured messages and wallets.
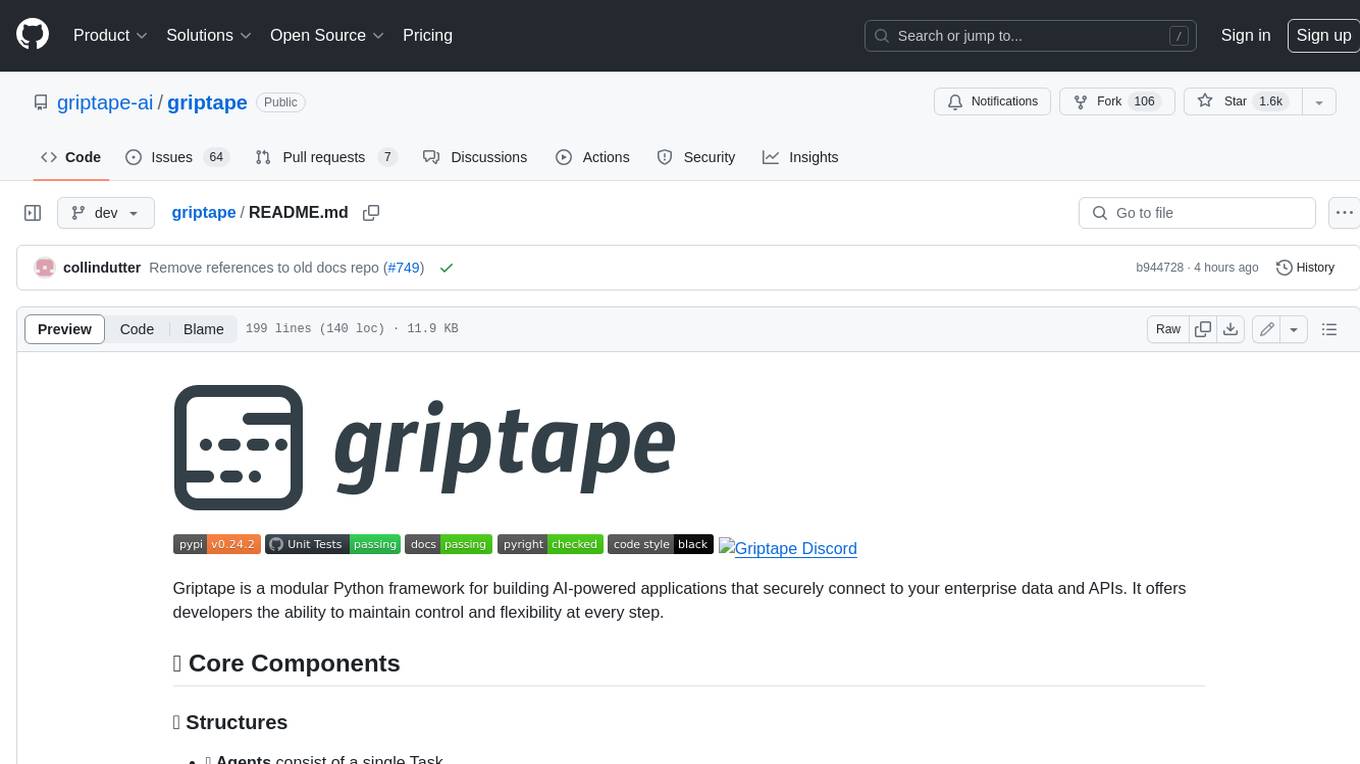
griptape
Griptape is a modular Python framework for building AI-powered applications that securely connect to your enterprise data and APIs. It offers developers the ability to maintain control and flexibility at every step. Griptape's core components include Structures (Agents, Pipelines, and Workflows), Tasks, Tools, Memory (Conversation Memory, Task Memory, and Meta Memory), Drivers (Prompt and Embedding Drivers, Vector Store Drivers, Image Generation Drivers, Image Query Drivers, SQL Drivers, Web Scraper Drivers, and Conversation Memory Drivers), Engines (Query Engines, Extraction Engines, Summary Engines, Image Generation Engines, and Image Query Engines), and additional components (Rulesets, Loaders, Artifacts, Chunkers, and Tokenizers). Griptape enables developers to create AI-powered applications with ease and efficiency.