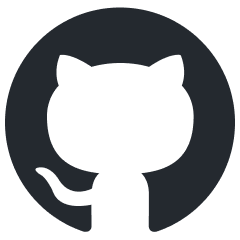
sglang
SGLang is a fast serving framework for large language models and vision language models.
Stars: 5638
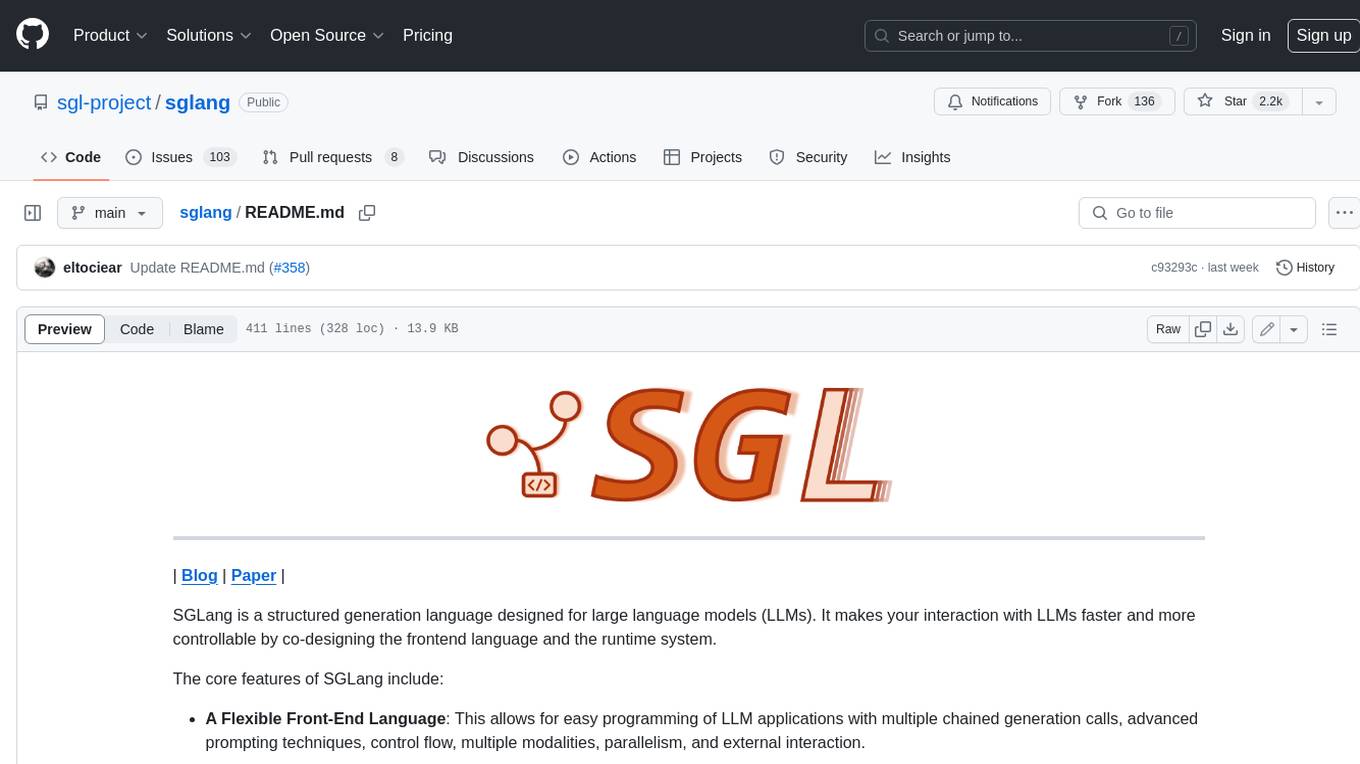
SGLang is a structured generation language designed for large language models (LLMs). It makes your interaction with LLMs faster and more controllable by co-designing the frontend language and the runtime system. The core features of SGLang include: - **A Flexible Front-End Language**: This allows for easy programming of LLM applications with multiple chained generation calls, advanced prompting techniques, control flow, multiple modalities, parallelism, and external interaction. - **A High-Performance Runtime with RadixAttention**: This feature significantly accelerates the execution of complex LLM programs by automatic KV cache reuse across multiple calls. It also supports other common techniques like continuous batching and tensor parallelism.
README:
| Blog | Paper | Slides | Join Slack | Join Bi-Weekly Development Meeting (Oct. 19) |
- [Oct. 16, 2024] Online meetup for efficient LLM deployment and serving, co-hosted by SGLang, FlashInfer, and MLC LLM! Fill out the Google form to receive the invite link. (Directly join the Meeting link if you are too late to register.)
- [2024/09] 🔥 SGLang v0.3 Release: 7x Faster DeepSeek MLA, 1.5x Faster torch.compile, Multi-Image/Video LLaVA-OneVision (blog).
- [2024/07] 🔥 Faster Llama3 Serving with SGLang Runtime (vs. TensorRT-LLM, vLLM) (blog).
- [2024/02] SGLang enables 3x faster JSON decoding with compressed finite state machine (blog).
More
SGLang is a fast serving framework for large language models and vision language models. It makes your interaction with models faster and more controllable by co-designing the backend runtime and frontend language. The core features include:
- Fast Backend Runtime: Provides efficient serving with RadixAttention for prefix caching, jump-forward constrained decoding, continuous batching, token attention (paged attention), tensor parallelism, FlashInfer kernels, chunked prefill, and quantization (INT4/FP8/AWQ/GPTQ).
- Flexible Frontend Language: Offers an intuitive interface for programming LLM applications, including chained generation calls, advanced prompting, control flow, multi-modal inputs, parallelism, and external interactions.
- Extensive Model Support: Supports a wide range of generative models (Llama, Gemma, Mistral, QWen, DeepSeek, LLaVA, etc.) and embedding models (e5-mistral), with easy extensibility for integrating new models.
- Active Community: SGLang is open-source and backed by an active community with industry adoption.
- Install
- Backend: SGLang Runtime (SRT)
- Frontend: Structured Generation Language (SGLang)
- Benchmark And Performance
- Roadmap
- Citation And Acknowledgment
You can install SGLang using any of the methods below.
pip install --upgrade pip
pip install "sglang[all]"
# Install FlashInfer CUDA kernels
pip install flashinfer -i https://flashinfer.ai/whl/cu121/torch2.4/
# Use the last release branch
git clone -b v0.3.3.post1 https://github.com/sgl-project/sglang.git
cd sglang
pip install --upgrade pip
pip install -e "python[all]"
# Install FlashInfer CUDA kernels
pip install flashinfer -i https://flashinfer.ai/whl/cu121/torch2.4/
The docker images are available on Docker Hub as lmsysorg/sglang, built from Dockerfile.
Replace <secret>
below with your huggingface hub token.
docker run --gpus all \
-p 30000:30000 \
-v ~/.cache/huggingface:/root/.cache/huggingface \
--env "HF_TOKEN=<secret>" \
--ipc=host \
lmsysorg/sglang:latest \
python3 -m sglang.launch_server --model-path meta-llama/Llama-3.1-8B-Instruct --host 0.0.0.0 --port 30000
More
This method is recommended if you plan to serve it as a service. A better approach is to use the k8s-sglang-service.yaml.
- Copy the compose.yml to your local machine
- Execute the command
docker compose up -d
in your terminal.
More
To deploy on Kubernetes or 12+ clouds, you can use SkyPilot.
- Install SkyPilot and set up Kubernetes cluster or cloud access: see SkyPilot's documentation.
- Deploy on your own infra with a single command and get the HTTP API endpoint:
SkyPilot YAML: sglang.yaml
# sglang.yaml
envs:
HF_TOKEN: null
resources:
image_id: docker:lmsysorg/sglang:latest
accelerators: A100
ports: 30000
run: |
conda deactivate
python3 -m sglang.launch_server \
--model-path meta-llama/Llama-3.1-8B-Instruct \
--host 0.0.0.0 \
--port 30000
# Deploy on any cloud or Kubernetes cluster. Use --cloud <cloud> to select a specific cloud provider.
HF_TOKEN=<secret> sky launch -c sglang --env HF_TOKEN sglang.yaml
# Get the HTTP API endpoint
sky status --endpoint 30000 sglang
- To further scale up your deployment with autoscaling and failure recovery, check out the SkyServe + SGLang guide.
-
FlashInfer is the default attention kernel backend. It only supports sm75 and above. If you encounter any FlashInfer-related issues on sm75+ devices (e.g., T4, A10, A100, L4, L40S, H100), please switch to other kernels by adding
--attention-backend triton --sampling-backend pytorch
and open an issue on GitHub. - If you only need to use the OpenAI backend, you can avoid installing other dependencies by using
pip install "sglang[openai]"
.
The SGLang Runtime (SRT) is an efficient serving engine.
Launch a server
python -m sglang.launch_server --model-path meta-llama/Meta-Llama-3-8B-Instruct --port 30000
Send a request
curl http://localhost:30000/generate \
-H "Content-Type: application/json" \
-d '{
"text": "Once upon a time,",
"sampling_params": {
"max_new_tokens": 16,
"temperature": 0
}
}'
Learn more about the argument specification, streaming, and multi-modal support here.
In addition, the server supports OpenAI-compatible APIs.
import openai
client = openai.Client(
base_url="http://127.0.0.1:30000/v1", api_key="EMPTY")
# Text completion
response = client.completions.create(
model="default",
prompt="The capital of France is",
temperature=0,
max_tokens=32,
)
print(response)
# Chat completion
response = client.chat.completions.create(
model="default",
messages=[
{"role": "system", "content": "You are a helpful AI assistant"},
{"role": "user", "content": "List 3 countries and their capitals."},
],
temperature=0,
max_tokens=64,
)
print(response)
# Text embedding
response = client.embeddings.create(
model="default",
input="How are you today",
)
print(response)
It supports streaming, vision, and almost all features of the Chat/Completions/Models/Batch endpoints specified by the OpenAI API Reference.
- To enable multi-GPU tensor parallelism, add
--tp 2
. If it reports the error "peer access is not supported between these two devices", add--enable-p2p-check
to the server launch command.
python -m sglang.launch_server --model-path meta-llama/Meta-Llama-3-8B-Instruct --tp 2
- To enable multi-GPU data parallelism, add
--dp 2
. Data parallelism is better for throughput if there is enough memory. It can also be used together with tensor parallelism. The following command uses 4 GPUs in total.
python -m sglang.launch_server --model-path meta-llama/Meta-Llama-3-8B-Instruct --dp 2 --tp 2
- If you see out-of-memory errors during serving, try to reduce the memory usage of the KV cache pool by setting a smaller value of
--mem-fraction-static
. The default value is0.9
.
python -m sglang.launch_server --model-path meta-llama/Meta-Llama-3-8B-Instruct --mem-fraction-static 0.7
- See hyperparameter_tuning.md on tuning hyperparameters for better performance.
- If you see out-of-memory errors during prefill for long prompts, try to set a smaller chunked prefill size.
python -m sglang.launch_server --model-path meta-llama/Meta-Llama-3-8B-Instruct --chunked-prefill-size 4096
- To enable torch.compile acceleration, add
--enable-torch-compile
. It accelerates small models on small batch sizes. - To enable torchao quantization, add
--torchao-config int4wo-128
. It supports various quantization strategies. - To enable fp8 weight quantization, add
--quantization fp8
on a fp16 checkpoint or directly load a fp8 checkpoint without specifying any arguments. - To enable fp8 kv cache quantization, add
--kv-cache-dtype fp8_e5m2
. - If the model does not have a chat template in the Hugging Face tokenizer, you can specify a custom chat template.
- To run tensor parallelism on multiple nodes, add
--nnodes 2
. If you have two nodes with two GPUs on each node and want to run TP=4, letsgl-dev-0
be the hostname of the first node and50000
be an available port, you can use the following commands. If you meet deadlock, please try to add--disable-cuda-graph
# Node 0
python -m sglang.launch_server --model-path meta-llama/Meta-Llama-3-8B-Instruct --tp 4 --nccl-init sgl-dev-0:50000 --nnodes 2 --node-rank 0
# Node 1
python -m sglang.launch_server --model-path meta-llama/Meta-Llama-3-8B-Instruct --tp 4 --nccl-init sgl-dev-0:50000 --nnodes 2 --node-rank 1
We also provide an inference engine without a HTTP server. For example,
import sglang as sgl
def main():
prompts = [
"Hello, my name is",
"The president of the United States is",
"The capital of France is",
"The future of AI is",
]
sampling_params = {"temperature": 0.8, "top_p": 0.95}
llm = sgl.Engine(model_path="meta-llama/Meta-Llama-3.1-8B-Instruct")
outputs = llm.generate(prompts, sampling_params)
for prompt, output in zip(prompts, outputs):
print("===============================")
print(f"Prompt: {prompt}\nGenerated text: {output['text']}")
if __name__ == "__main__":
main()
This can be used for:
- Offline Batch Inference
- Building Custom Servers
You can view the full example here
Generative Models
- Llama / Llama 2 / Llama 3 / Llama 3.1
- Mistral / Mixtral / Mistral NeMo
- Gemma / Gemma 2
- Qwen / Qwen 2 / Qwen 2 MoE
- DeepSeek / DeepSeek 2
- OLMoE
-
LLaVA-OneVision
python3 -m sglang.launch_server --model-path lmms-lab/llava-onevision-qwen2-7b-ov --port=30000 --chat-template=chatml-llava
python3 -m sglang.launch_server --model-path lmms-lab/llava-onevision-qwen2-72b-ov --port=30000 --tp-size=8 --chat-template=chatml-llava
- Query the server with the OpenAI Vision API. See examples at test/srt/test_vision_openai_server.py
- LLaVA 1.5 / 1.6 / NeXT
python -m sglang.launch_server --model-path lmms-lab/llama3-llava-next-8b --port=30000 --tp-size=1 --chat-template=llava_llama_3
python -m sglang.launch_server --model-path lmms-lab/llava-next-72b --port=30000 --tp-size=8 --chat-template=chatml-llava
- Query the server with the OpenAI Vision API. See examples at test/srt/test_vision_openai_server.py
- Yi-VL
- StableLM
- Command-R
- DBRX
- Grok
- ChatGLM
- InternLM 2
- Exaone 3
- BaiChuan2
- MiniCPM / MiniCPM 3
- XVERSE / XVERSE MoE
- SmolLM
Embedding Models
- e5-mistral
- gte-Qwen2
python -m sglang.launch_server --model-path Alibaba-NLP/gte-Qwen2-7B-instruct --is-embedding
Instructions for supporting a new model are here.
More
To use a model from ModelScope, set the environment variable SGLANG_USE_MODELSCOPE.
export SGLANG_USE_MODELSCOPE=true
Launch Qwen2-7B-Instruct Server
SGLANG_USE_MODELSCOPE=true python -m sglang.launch_server --model-path qwen/Qwen2-7B-Instruct --port 30000
Or start it by docker.
docker run --gpus all \
-p 30000:30000 \
-v ~/.cache/modelscope:/root/.cache/modelscope \
--env "SGLANG_USE_MODELSCOPE=true" \
--ipc=host \
lmsysorg/sglang:latest \
python3 -m sglang.launch_server --model-path Qwen/Qwen2.5-7B-Instruct --host 0.0.0.0 --port 30000
More
# Run 405B (fp8) on a single node
python -m sglang.launch_server --model-path meta-llama/Meta-Llama-3.1-405B-Instruct-FP8 --tp 8
# Run 405B (fp16) on two nodes
## on the first node, replace the `172.16.4.52:20000` with your own first node ip address and port
GLOO_SOCKET_IFNAME=eth0 python3 -m sglang.launch_server --model-path meta-llama/Meta-Llama-3.1-405B-Instruct --tp 16 --nccl-init-addr 172.16.4.52:20000 --nnodes 2 --node-rank 0 --disable-cuda-graph
## on the first node, replace the `172.16.4.52:20000` with your own first node ip address and port
GLOO_SOCKET_IFNAME=eth0 python3 -m sglang.launch_server --model-path meta-llama/Meta-Llama-3.1-405B-Instruct --tp 16 --nccl-init-addr 172.16.4.52:20000 --nnodes 2 --node-rank 1 --disable-cuda-graph
- Benchmark a single static batch by running the following command without launching a server. The arguments are the same as for
launch_server.py
. Note that this is not a dynamic batching server, so it may run out of memory for a batch size that a real server can handle. A real server truncates the prefill into several batches, while this unit test does not. For accurate large batch testing, please usesglang.bench_serving
instead.python -m sglang.bench_latency --model-path meta-llama/Meta-Llama-3-8B-Instruct --batch 32 --input-len 256 --output-len 32
- Benchmark online serving. Launch a server first and run the following command.
python3 -m sglang.bench_serving --backend sglang --num-prompt 10
The frontend language can be used with local models or API models. It is an alternative to the OpenAI API. You may found it easier to use for complex prompting workflow.
The example below shows how to use sglang to answer a multi-turn question.
First, launch a server with
python -m sglang.launch_server --model-path meta-llama/Meta-Llama-3-8B-Instruct --port 30000
Then, connect to the server and answer a multi-turn question.
from sglang import function, system, user, assistant, gen, set_default_backend, RuntimeEndpoint
@function
def multi_turn_question(s, question_1, question_2):
s += system("You are a helpful assistant.")
s += user(question_1)
s += assistant(gen("answer_1", max_tokens=256))
s += user(question_2)
s += assistant(gen("answer_2", max_tokens=256))
set_default_backend(RuntimeEndpoint("http://localhost:30000"))
state = multi_turn_question.run(
question_1="What is the capital of the United States?",
question_2="List two local attractions.",
)
for m in state.messages():
print(m["role"], ":", m["content"])
print(state["answer_1"])
Set the OpenAI API Key
export OPENAI_API_KEY=sk-******
Then, answer a multi-turn question.
from sglang import function, system, user, assistant, gen, set_default_backend, OpenAI
@function
def multi_turn_question(s, question_1, question_2):
s += system("You are a helpful assistant.")
s += user(question_1)
s += assistant(gen("answer_1", max_tokens=256))
s += user(question_2)
s += assistant(gen("answer_2", max_tokens=256))
set_default_backend(OpenAI("gpt-3.5-turbo"))
state = multi_turn_question.run(
question_1="What is the capital of the United States?",
question_2="List two local attractions.",
)
for m in state.messages():
print(m["role"], ":", m["content"])
print(state["answer_1"])
Anthropic and VertexAI (Gemini) models are also supported. You can find more examples at examples/quick_start.
To begin with, import sglang.
import sglang as sgl
sglang
provides some simple primitives such as gen
, select
, fork
, image
.
You can implement your prompt flow in a function decorated by sgl.function
.
You can then invoke the function with run
or run_batch
.
The system will manage the state, chat template, parallelism and batching for you.
The complete code for the examples below can be found at readme_examples.py
You can use any Python code within the function body, including control flow, nested function calls, and external libraries.
@sgl.function
def tool_use(s, question):
s += "To answer this question: " + question + ". "
s += "I need to use a " + sgl.gen("tool", choices=["calculator", "search engine"]) + ". "
if s["tool"] == "calculator":
s += "The math expression is" + sgl.gen("expression")
elif s["tool"] == "search engine":
s += "The key word to search is" + sgl.gen("word")
Use fork
to launch parallel prompts.
Because sgl.gen
is non-blocking, the for loop below issues two generation calls in parallel.
@sgl.function
def tip_suggestion(s):
s += (
"Here are two tips for staying healthy: "
"1. Balanced Diet. 2. Regular Exercise.\n\n"
)
forks = s.fork(2)
for i, f in enumerate(forks):
f += f"Now, expand tip {i+1} into a paragraph:\n"
f += sgl.gen(f"detailed_tip", max_tokens=256, stop="\n\n")
s += "Tip 1:" + forks[0]["detailed_tip"] + "\n"
s += "Tip 2:" + forks[1]["detailed_tip"] + "\n"
s += "In summary" + sgl.gen("summary")
Use sgl.image
to pass an image as input.
@sgl.function
def image_qa(s, image_file, question):
s += sgl.user(sgl.image(image_file) + question)
s += sgl.assistant(sgl.gen("answer", max_tokens=256)
See also srt_example_llava.py.
Use regex
to specify a regular expression as a decoding constraint.
This is only supported for local models.
@sgl.function
def regular_expression_gen(s):
s += "Q: What is the IP address of the Google DNS servers?\n"
s += "A: " + sgl.gen(
"answer",
temperature=0,
regex=r"((25[0-5]|2[0-4]\d|[01]?\d\d?).){3}(25[0-5]|2[0-4]\d|[01]?\d\d?)",
)
Use regex
to specify a JSON schema with a regular expression.
character_regex = (
r"""\{\n"""
+ r""" "name": "[\w\d\s]{1,16}",\n"""
+ r""" "house": "(Gryffindor|Slytherin|Ravenclaw|Hufflepuff)",\n"""
+ r""" "blood status": "(Pure-blood|Half-blood|Muggle-born)",\n"""
+ r""" "occupation": "(student|teacher|auror|ministry of magic|death eater|order of the phoenix)",\n"""
+ r""" "wand": \{\n"""
+ r""" "wood": "[\w\d\s]{1,16}",\n"""
+ r""" "core": "[\w\d\s]{1,16}",\n"""
+ r""" "length": [0-9]{1,2}\.[0-9]{0,2}\n"""
+ r""" \},\n"""
+ r""" "alive": "(Alive|Deceased)",\n"""
+ r""" "patronus": "[\w\d\s]{1,16}",\n"""
+ r""" "bogart": "[\w\d\s]{1,16}"\n"""
+ r"""\}"""
)
@sgl.function
def character_gen(s, name):
s += name + " is a character in Harry Potter. Please fill in the following information about this character.\n"
s += sgl.gen("json_output", max_tokens=256, regex=character_regex)
See also json_decode.py for an additional example of specifying formats with Pydantic models.
Use run_batch
to run a batch of requests with continuous batching.
@sgl.function
def text_qa(s, question):
s += "Q: " + question + "\n"
s += "A:" + sgl.gen("answer", stop="\n")
states = text_qa.run_batch(
[
{"question": "What is the capital of the United Kingdom?"},
{"question": "What is the capital of France?"},
{"question": "What is the capital of Japan?"},
],
progress_bar=True
)
Add stream=True
to enable streaming.
@sgl.function
def text_qa(s, question):
s += "Q: " + question + "\n"
s += "A:" + sgl.gen("answer", stop="\n")
state = text_qa.run(
question="What is the capital of France?",
temperature=0.1,
stream=True
)
for out in state.text_iter():
print(out, end="", flush=True)
Use sgl.system
, sgl.user
and sgl.assistant
to set roles when using Chat models. You can also define more complex role prompts using begin and end tokens.
@sgl.function
def chat_example(s):
s += sgl.system("You are a helpful assistant.")
# Same as: s += s.system("You are a helpful assistant.")
with s.user():
s += "Question: What is the capital of France?"
s += sgl.assistant_begin()
s += "Answer: " + sgl.gen(max_tokens=100, stop="\n")
s += sgl.assistant_end()
- The
choices
argument insgl.gen
is implemented by computing the token-length normalized log probabilities of all choices and selecting the one with the highest probability. - The
regex
argument insgl.gen
is implemented through autoregressive decoding with logit bias masking, according to the constraints set by the regex. It is compatible withtemperature=0
andtemperature != 0
.
Learn more at this blog.
Please cite our paper, SGLang: Efficient Execution of Structured Language Model Programs, if you find the project useful. We also learned from the design and reused code from the following projects: Guidance, vLLM, LightLLM, FlashInfer, Outlines, and LMQL.
For Tasks:
Click tags to check more tools for each tasksFor Jobs:
Alternative AI tools for sglang
Similar Open Source Tools
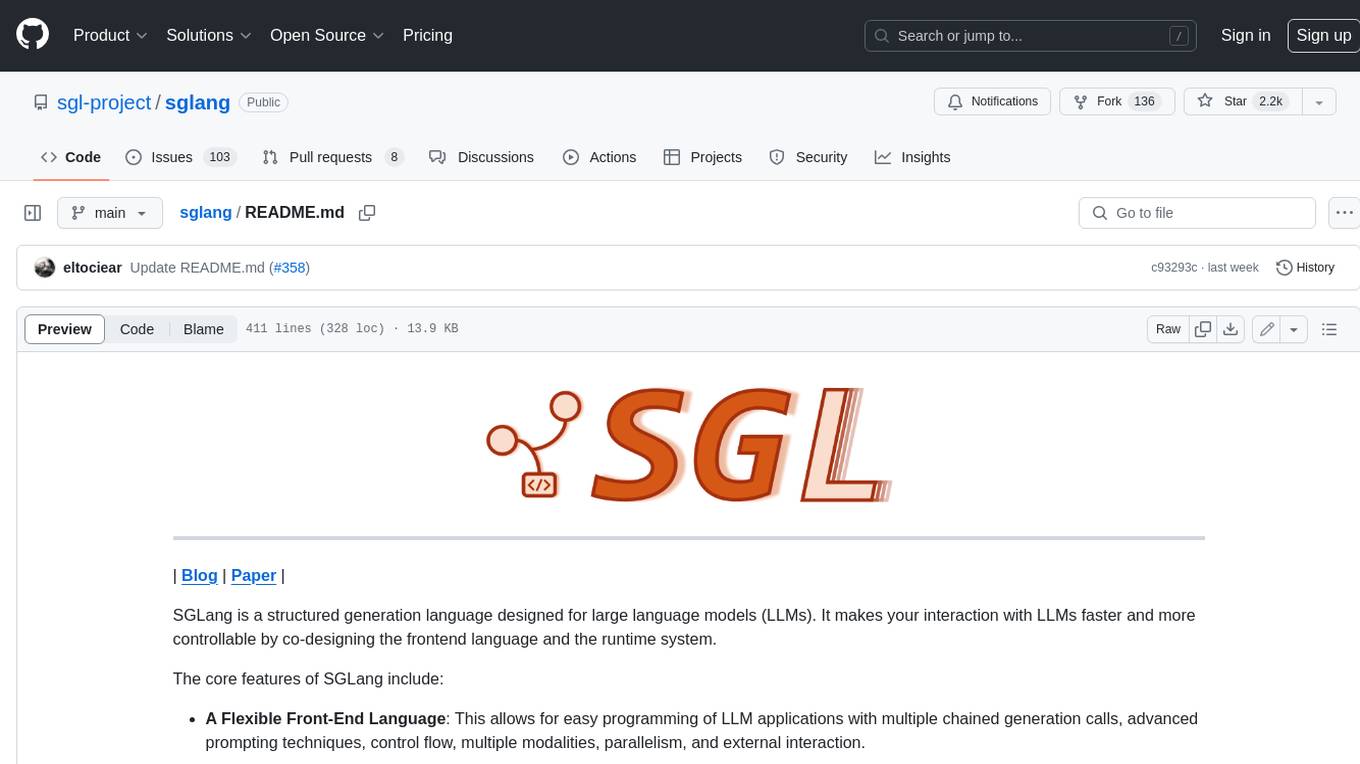
sglang
SGLang is a structured generation language designed for large language models (LLMs). It makes your interaction with LLMs faster and more controllable by co-designing the frontend language and the runtime system. The core features of SGLang include: - **A Flexible Front-End Language**: This allows for easy programming of LLM applications with multiple chained generation calls, advanced prompting techniques, control flow, multiple modalities, parallelism, and external interaction. - **A High-Performance Runtime with RadixAttention**: This feature significantly accelerates the execution of complex LLM programs by automatic KV cache reuse across multiple calls. It also supports other common techniques like continuous batching and tensor parallelism.
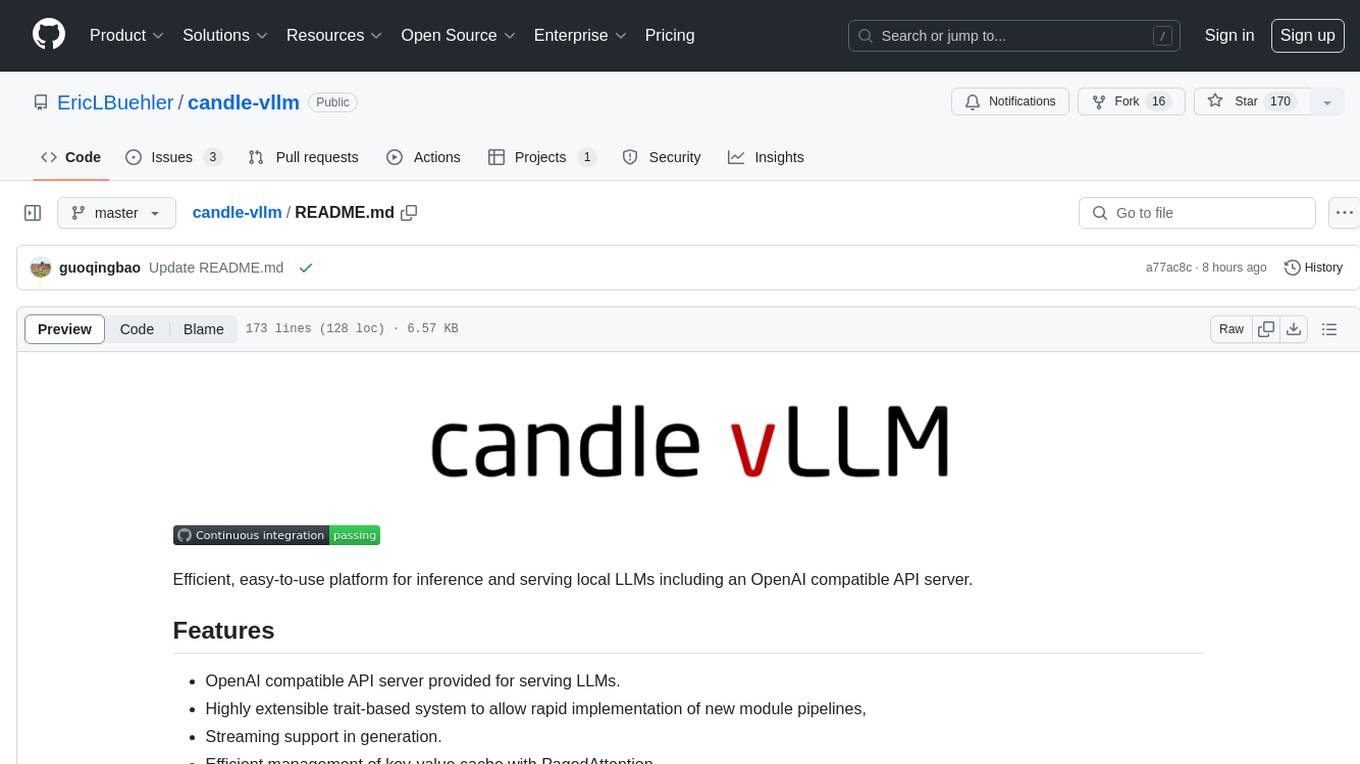
candle-vllm
Candle-vllm is an efficient and easy-to-use platform designed for inference and serving local LLMs, featuring an OpenAI compatible API server. It offers a highly extensible trait-based system for rapid implementation of new module pipelines, streaming support in generation, efficient management of key-value cache with PagedAttention, and continuous batching. The tool supports chat serving for various models and provides a seamless experience for users to interact with LLMs through different interfaces.
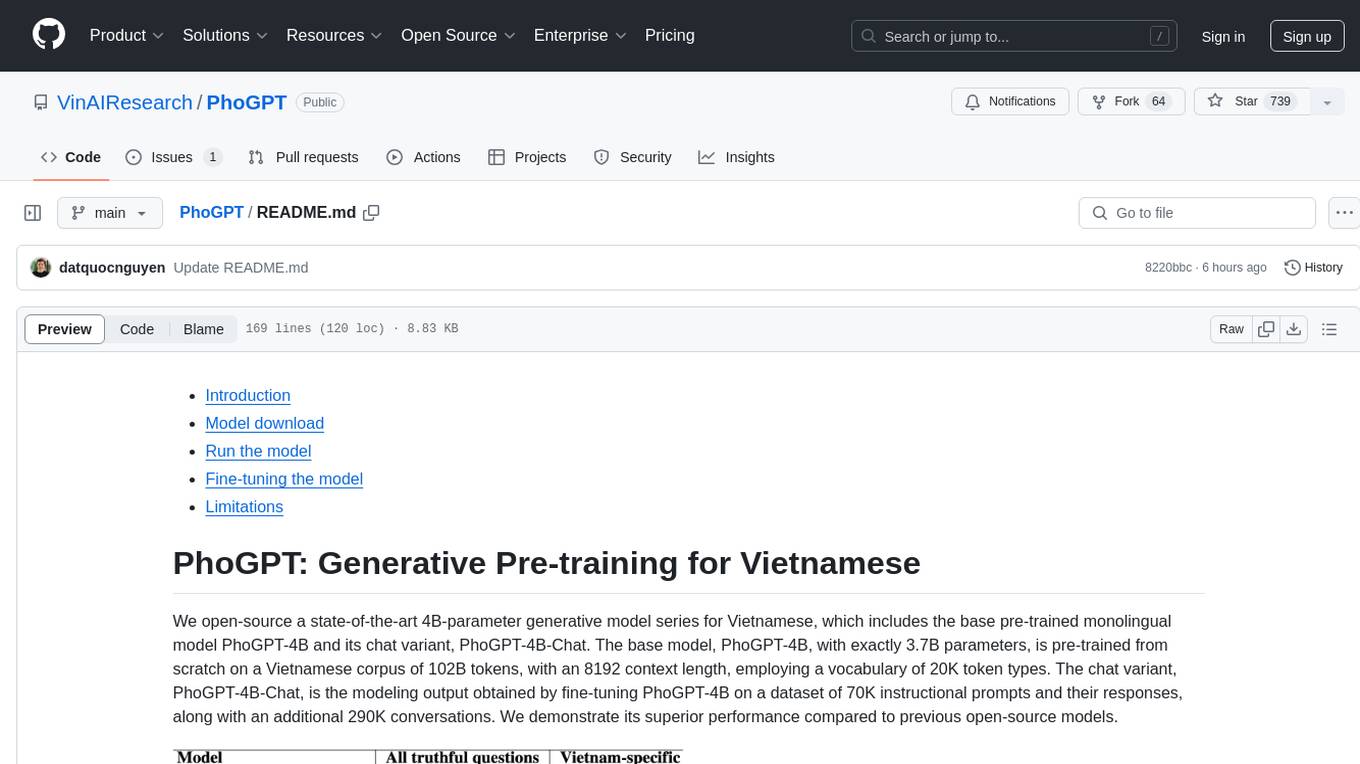
PhoGPT
PhoGPT is an open-source 4B-parameter generative model series for Vietnamese, including the base pre-trained monolingual model PhoGPT-4B and its chat variant, PhoGPT-4B-Chat. PhoGPT-4B is pre-trained from scratch on a Vietnamese corpus of 102B tokens, with an 8192 context length and a vocabulary of 20K token types. PhoGPT-4B-Chat is fine-tuned on instructional prompts and conversations, demonstrating superior performance. Users can run the model with inference engines like vLLM and Text Generation Inference, and fine-tune it using llm-foundry. However, PhoGPT has limitations in reasoning, coding, and mathematics tasks, and may generate harmful or biased responses.
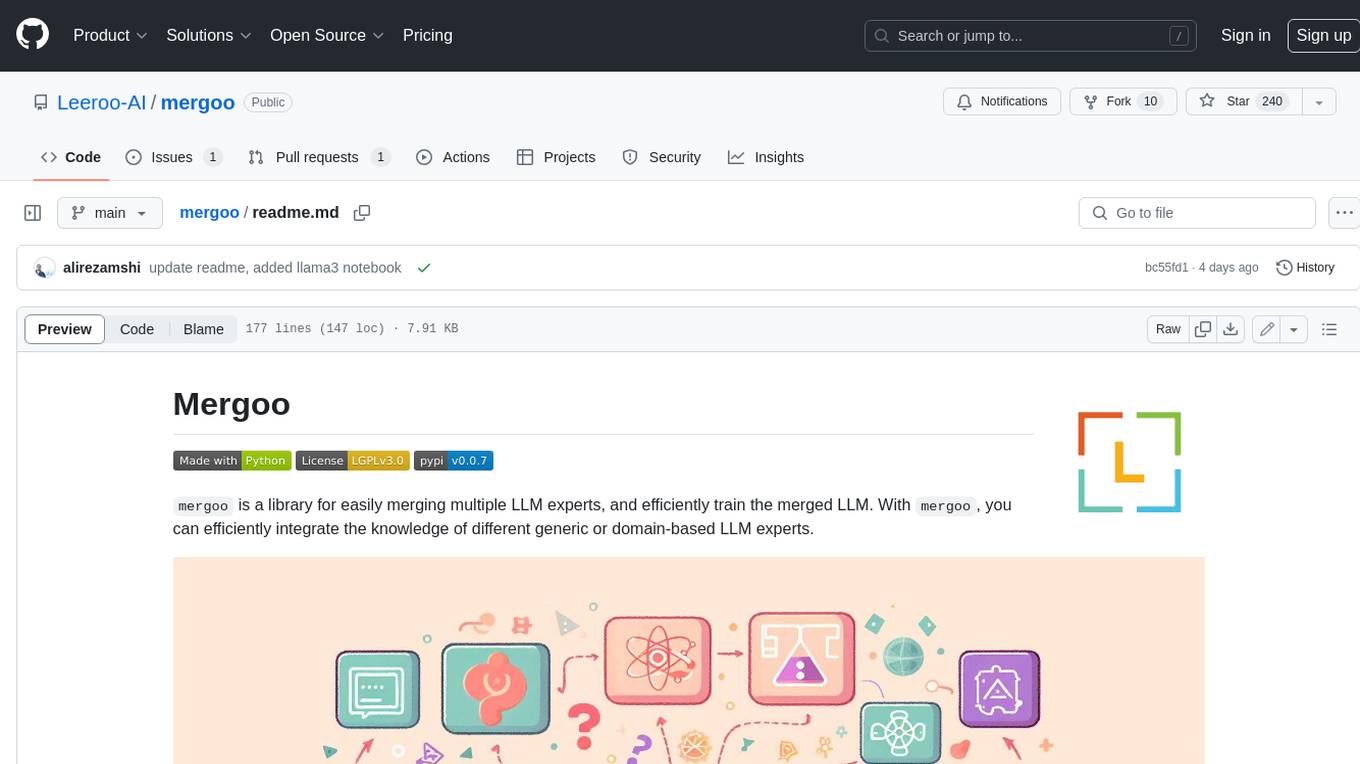
mergoo
Mergoo is a library for easily merging multiple LLM experts and efficiently training the merged LLM. With Mergoo, you can efficiently integrate the knowledge of different generic or domain-based LLM experts. Mergoo supports several merging methods, including Mixture-of-Experts, Mixture-of-Adapters, and Layer-wise merging. It also supports various base models, including LLaMa, Mistral, and BERT, and trainers, including Hugging Face Trainer, SFTrainer, and PEFT. Mergoo provides flexible merging for each layer and supports training choices such as only routing MoE layers or fully fine-tuning the merged LLM.
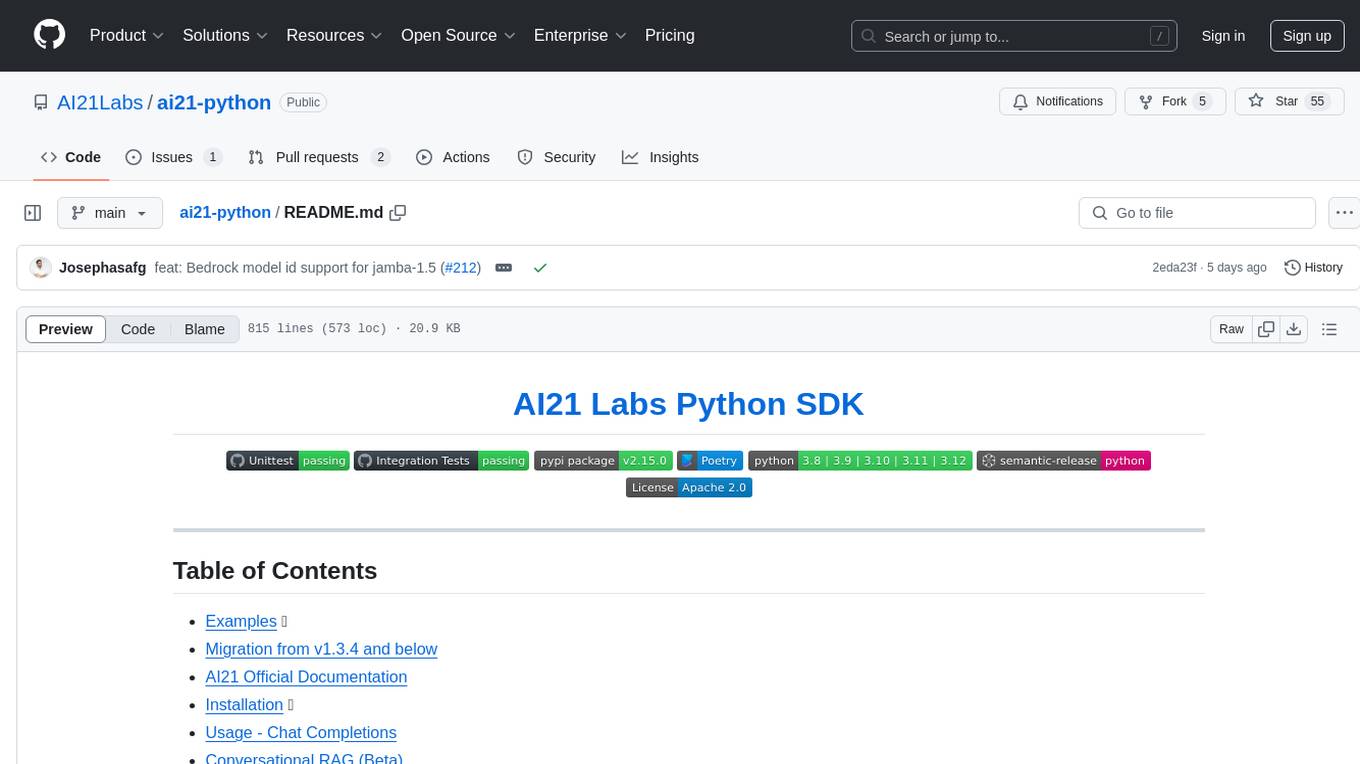
ai21-python
The AI21 Labs Python SDK is a comprehensive tool for interacting with the AI21 API. It provides functionalities for chat completions, conversational RAG, token counting, error handling, and support for various cloud providers like AWS, Azure, and Vertex. The SDK offers both synchronous and asynchronous usage, along with detailed examples and documentation. Users can quickly get started with the SDK to leverage AI21's powerful models for various natural language processing tasks.
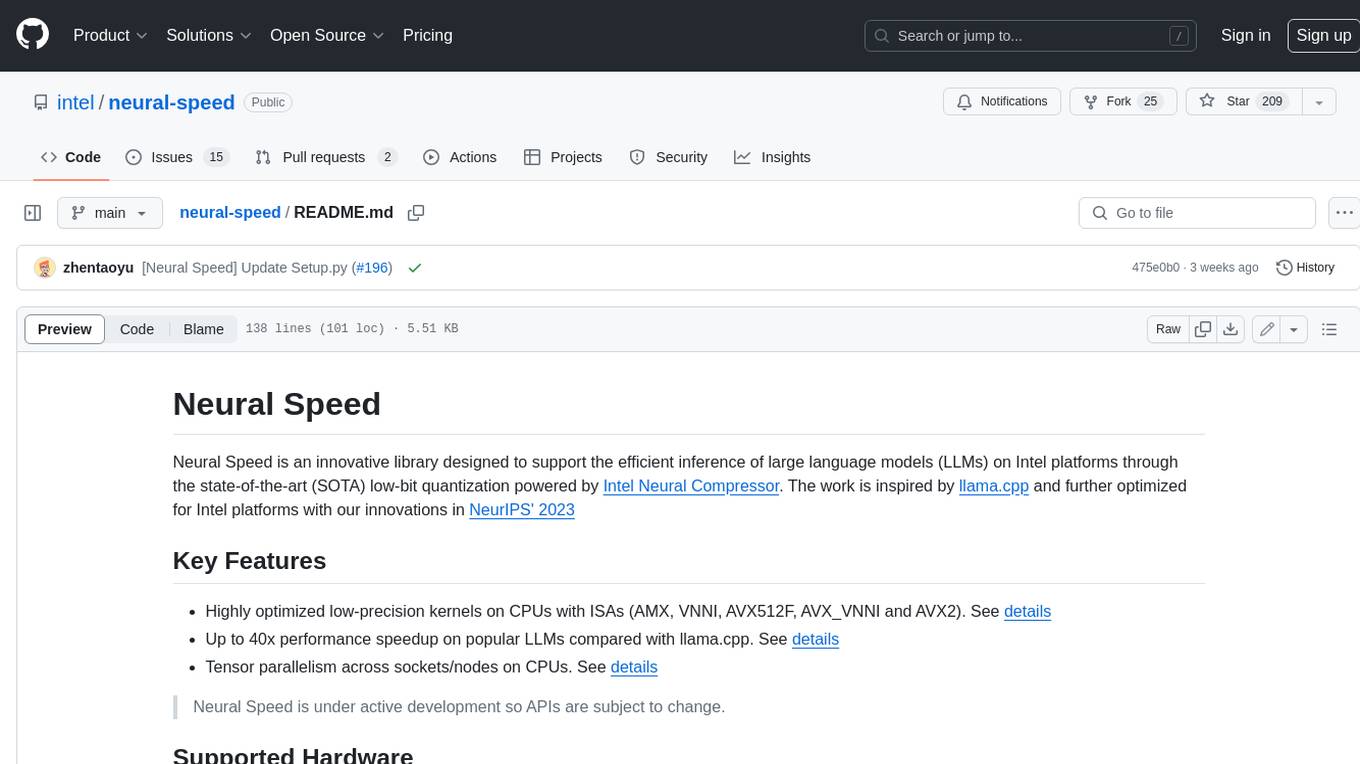
neural-speed
Neural Speed is an innovative library designed to support the efficient inference of large language models (LLMs) on Intel platforms through the state-of-the-art (SOTA) low-bit quantization powered by Intel Neural Compressor. The work is inspired by llama.cpp and further optimized for Intel platforms with our innovations in NeurIPS' 2023
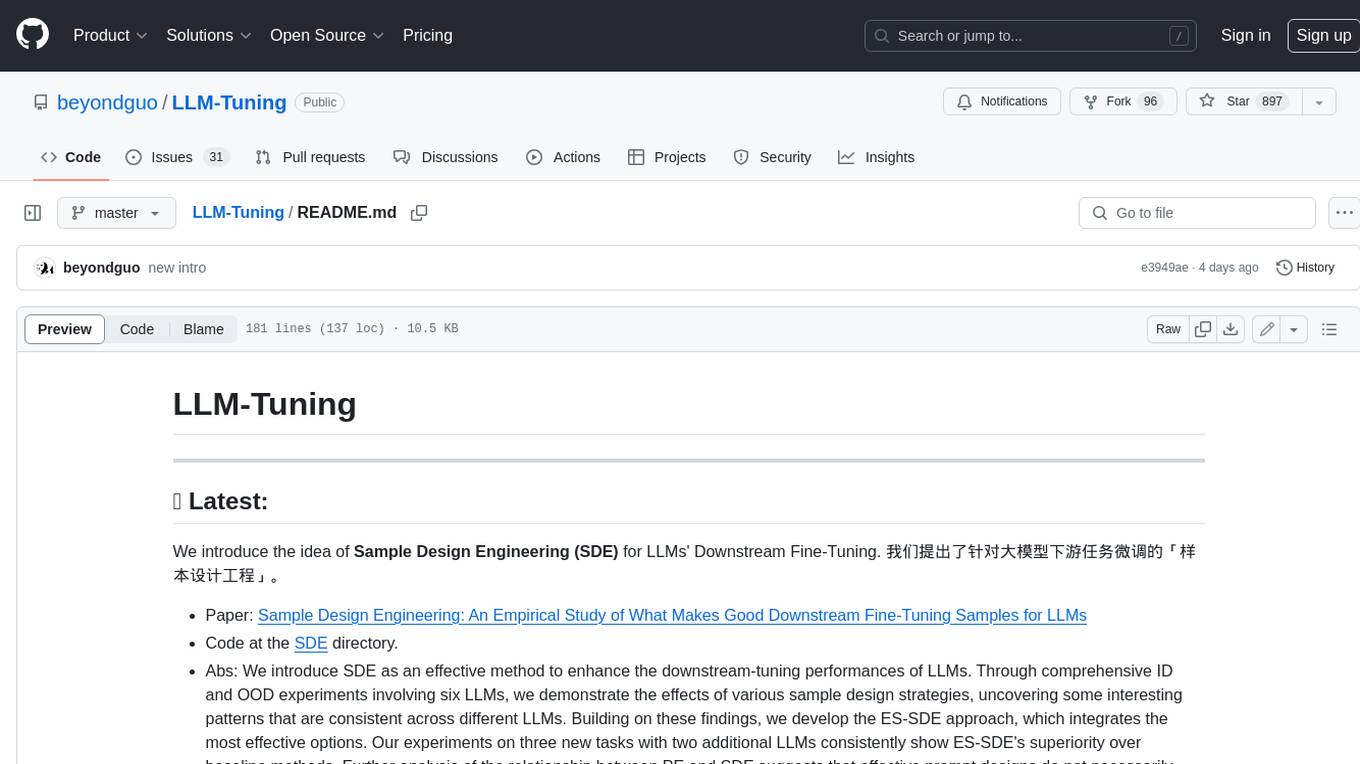
LLM-Tuning
LLM-Tuning is a collection of tools and resources for fine-tuning large language models (LLMs). It includes a library of pre-trained LoRA models, a set of tutorials and examples, and a community forum for discussion and support. LLM-Tuning makes it easy to fine-tune LLMs for a variety of tasks, including text classification, question answering, and dialogue generation. With LLM-Tuning, you can quickly and easily improve the performance of your LLMs on downstream tasks.
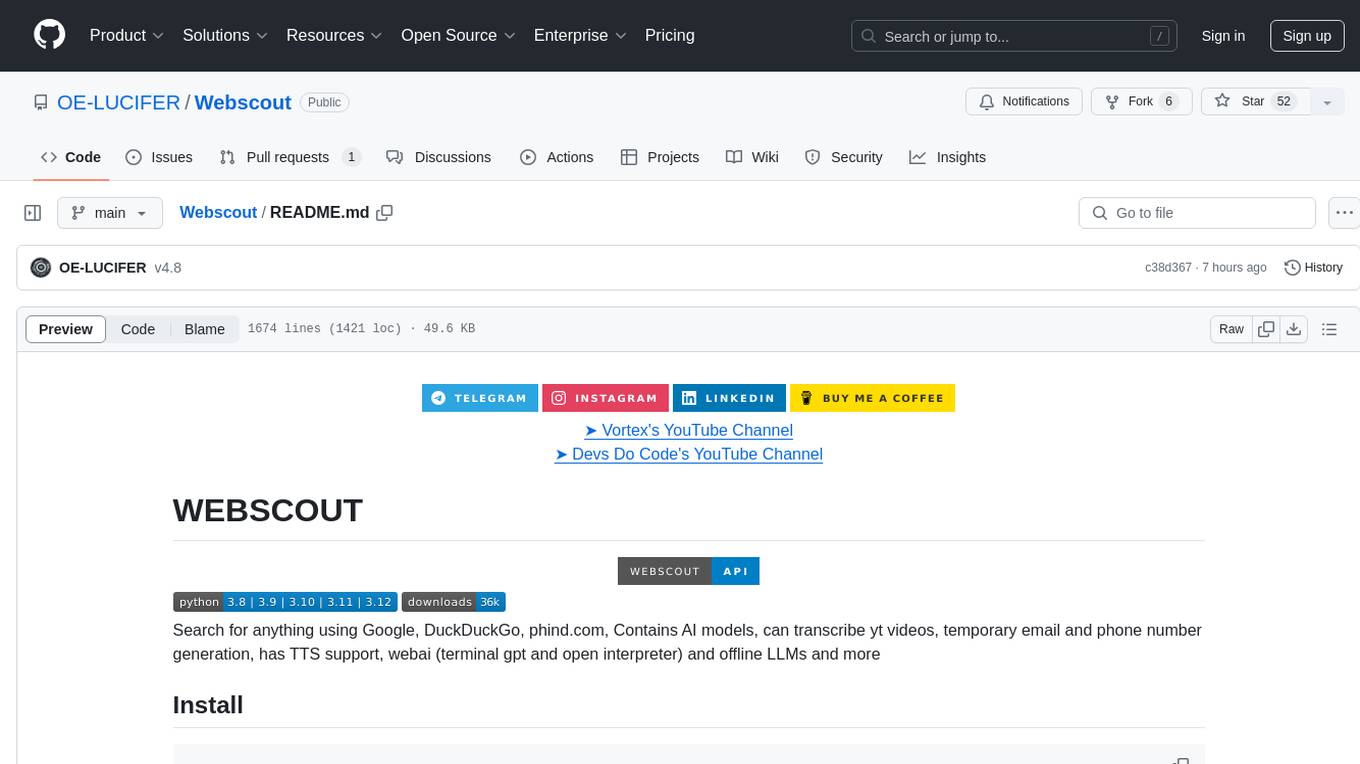
Webscout
WebScout is a versatile tool that allows users to search for anything using Google, DuckDuckGo, and phind.com. It contains AI models, can transcribe YouTube videos, generate temporary email and phone numbers, has TTS support, webai (terminal GPT and open interpreter), and offline LLMs. It also supports features like weather forecasting, YT video downloading, temp mail and number generation, text-to-speech, advanced web searches, and more.
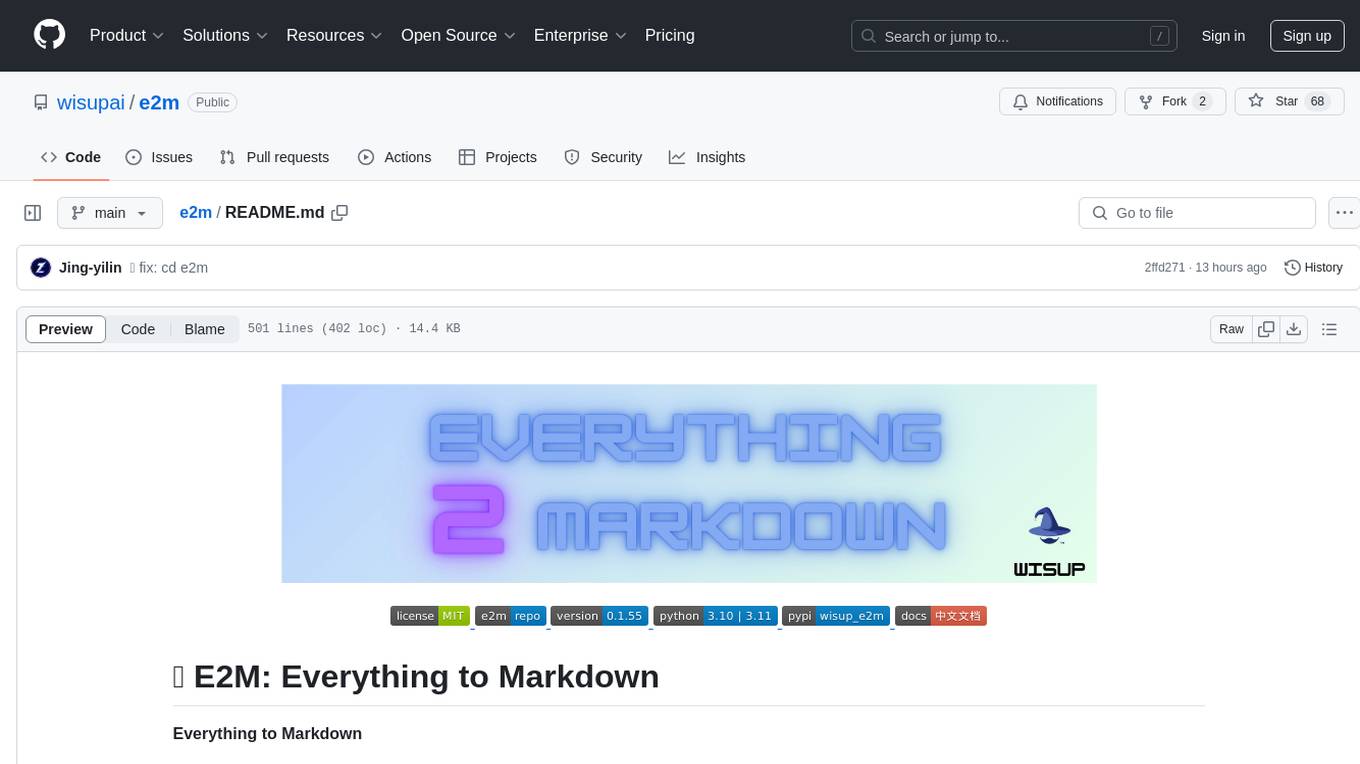
e2m
E2M is a Python library that can parse and convert various file types into Markdown format. It supports the conversion of multiple file formats, including doc, docx, epub, html, htm, url, pdf, ppt, pptx, mp3, and m4a. The ultimate goal of the E2M project is to provide high-quality data for Retrieval-Augmented Generation (RAG) and model training or fine-tuning. The core architecture consists of a Parser responsible for parsing various file types into text or image data, and a Converter responsible for converting text or image data into Markdown format.
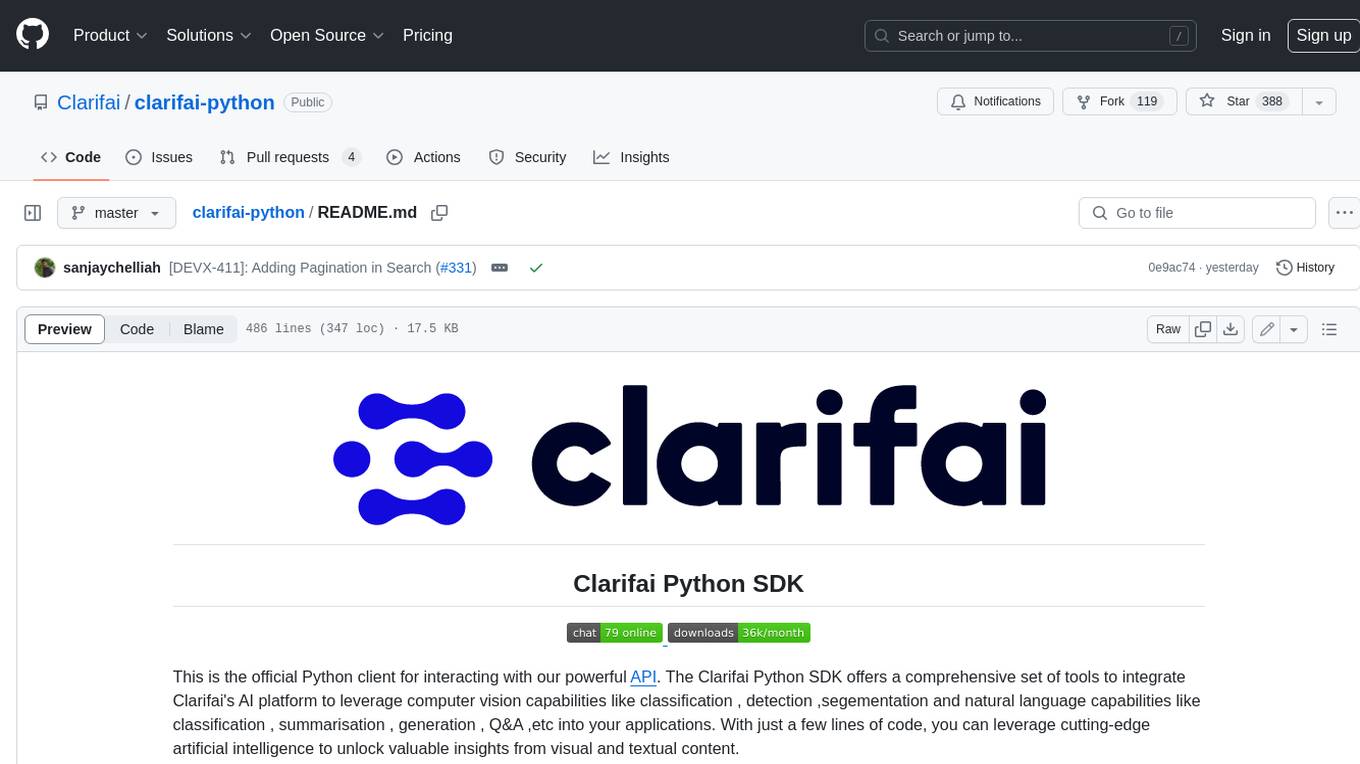
clarifai-python
The Clarifai Python SDK offers a comprehensive set of tools to integrate Clarifai's AI platform to leverage computer vision capabilities like classification , detection ,segementation and natural language capabilities like classification , summarisation , generation , Q&A ,etc into your applications. With just a few lines of code, you can leverage cutting-edge artificial intelligence to unlock valuable insights from visual and textual content.
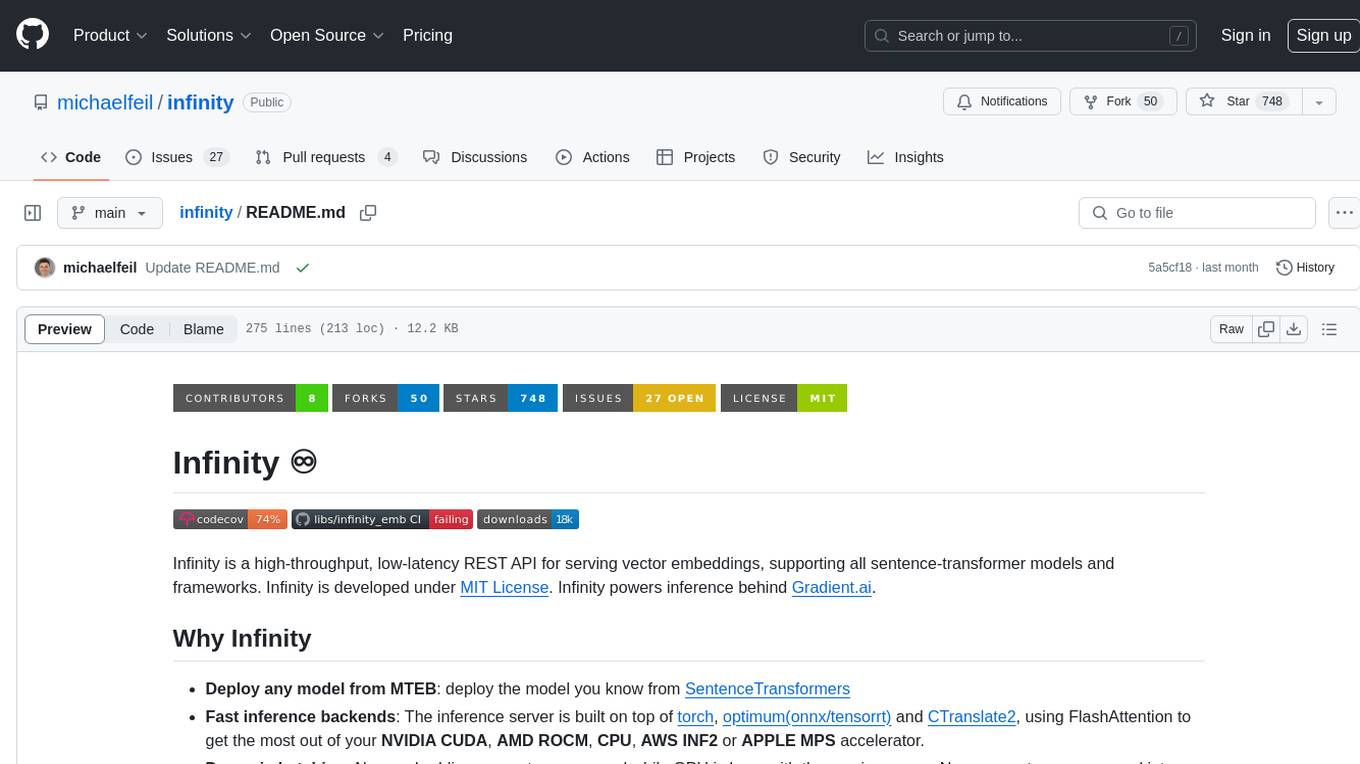
infinity
Infinity is a high-throughput, low-latency REST API for serving vector embeddings, supporting all sentence-transformer models and frameworks. It is developed under the MIT License and powers inference behind Gradient.ai. The API allows users to deploy models from SentenceTransformers, offers fast inference backends utilizing various accelerators, dynamic batching for efficient processing, correct and tested implementation, and easy-to-use API built on FastAPI with Swagger documentation. Users can embed text, rerank documents, and perform text classification tasks using the tool. Infinity supports various models from Huggingface and provides flexibility in deployment via CLI, Docker, Python API, and cloud services like dstack. The tool is suitable for tasks like embedding, reranking, and text classification.
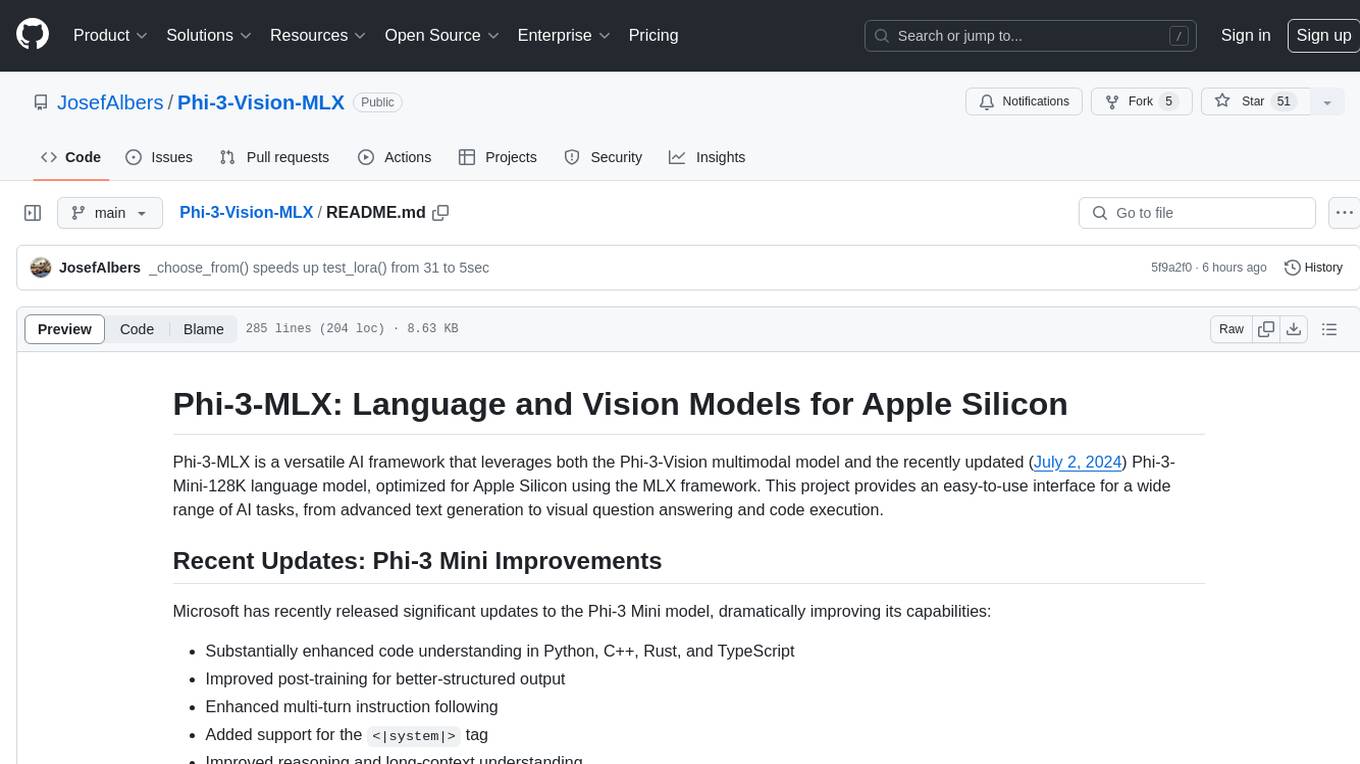
Phi-3-Vision-MLX
Phi-3-MLX is a versatile AI framework that leverages both the Phi-3-Vision multimodal model and the Phi-3-Mini-128K language model optimized for Apple Silicon using the MLX framework. It provides an easy-to-use interface for a wide range of AI tasks, from advanced text generation to visual question answering and code execution. The project features support for batched generation, flexible agent system, custom toolchains, model quantization, LoRA fine-tuning capabilities, and API integration for extended functionality.
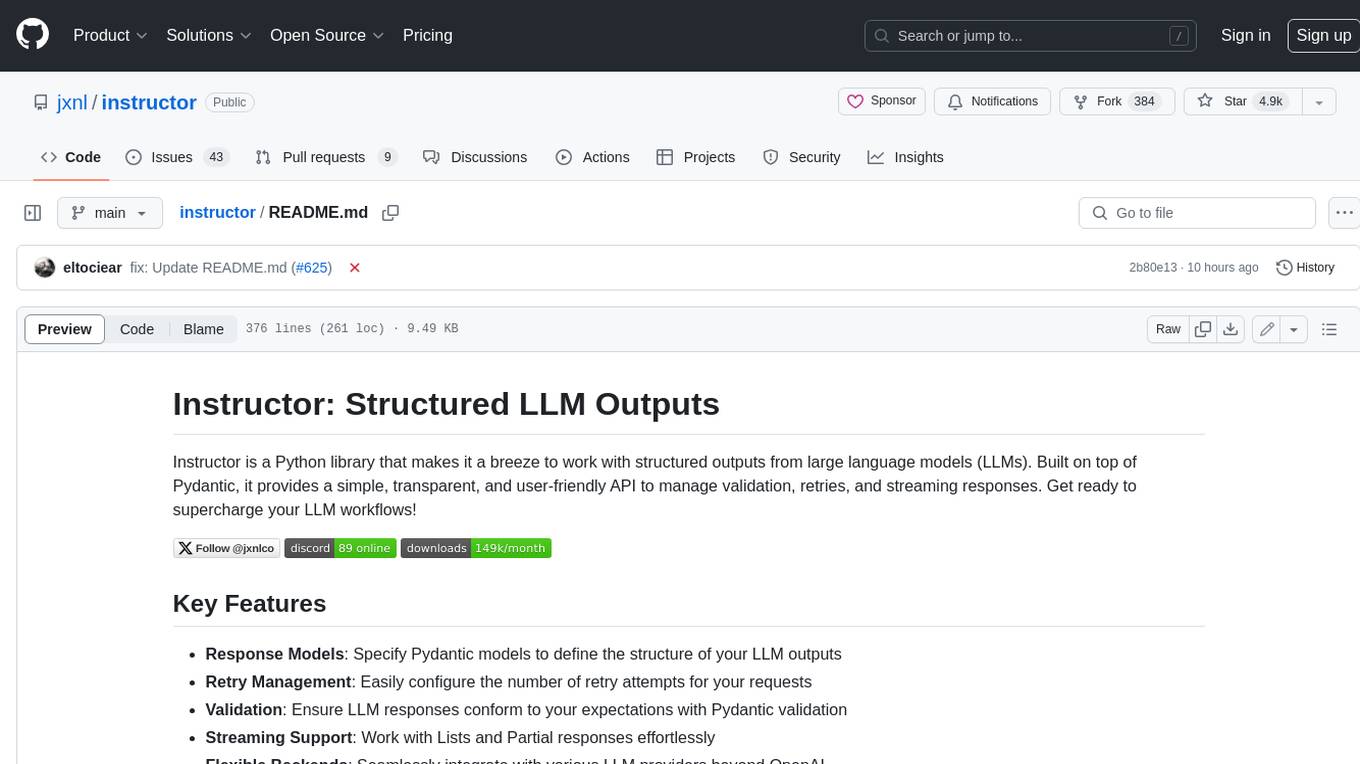
instructor
Instructor is a Python library that makes it a breeze to work with structured outputs from large language models (LLMs). Built on top of Pydantic, it provides a simple, transparent, and user-friendly API to manage validation, retries, and streaming responses. Get ready to supercharge your LLM workflows!
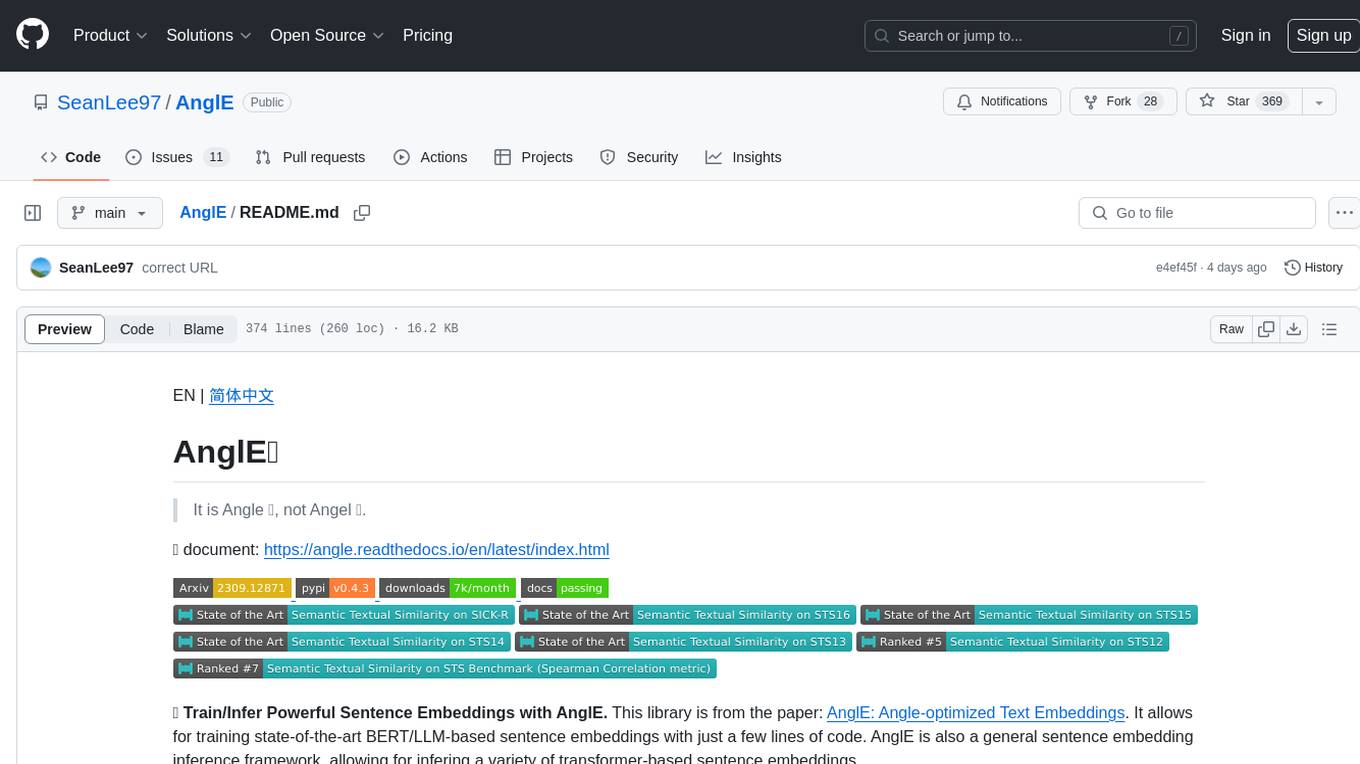
AnglE
AnglE is a library for training state-of-the-art BERT/LLM-based sentence embeddings with just a few lines of code. It also serves as a general sentence embedding inference framework, allowing for inferring a variety of transformer-based sentence embeddings. The library supports various loss functions such as AnglE loss, Contrastive loss, CoSENT loss, and Espresso loss. It provides backbones like BERT-based models, LLM-based models, and Bi-directional LLM-based models for training on single or multi-GPU setups. AnglE has achieved significant performance on various benchmarks and offers official pretrained models for both BERT-based and LLM-based models.
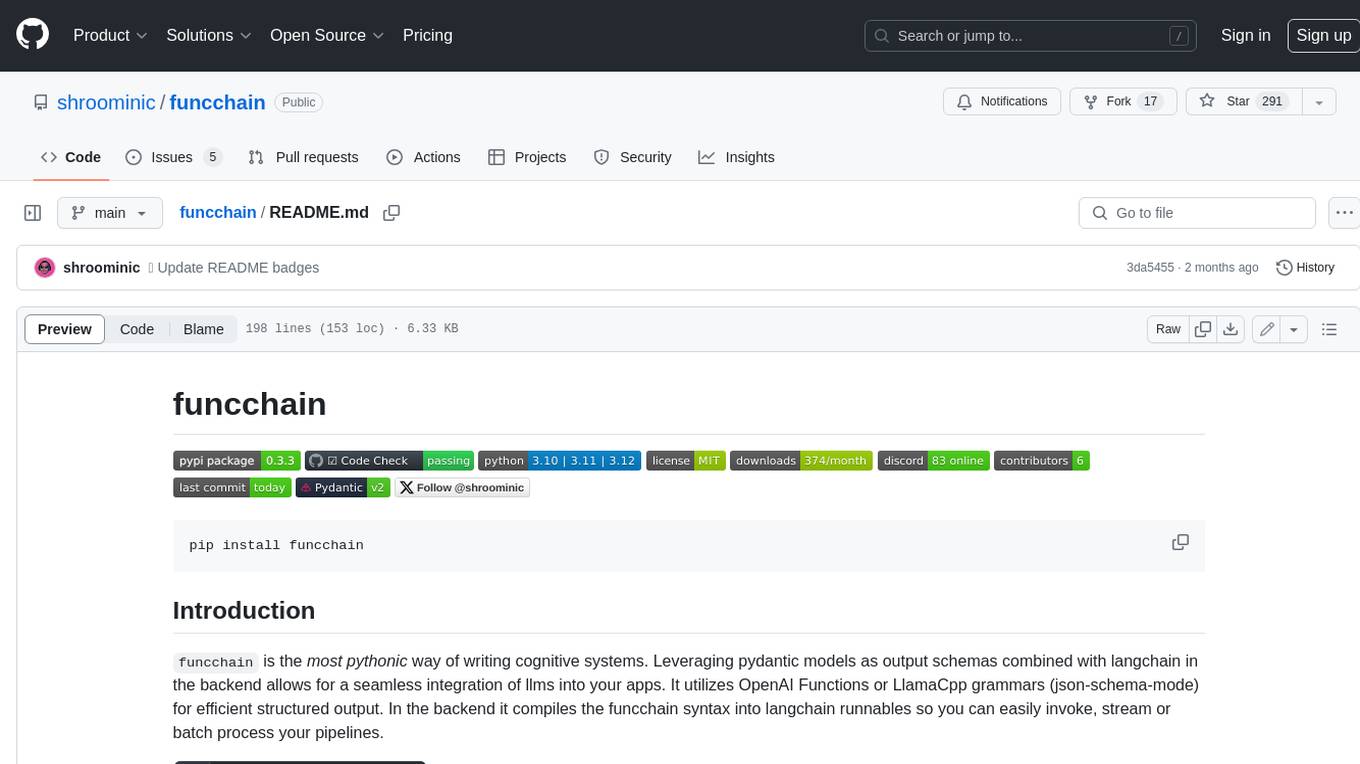
funcchain
Funcchain is a Python library that allows you to easily write cognitive systems by leveraging Pydantic models as output schemas and LangChain in the backend. It provides a seamless integration of LLMs into your apps, utilizing OpenAI Functions or LlamaCpp grammars (json-schema-mode) for efficient structured output. Funcchain compiles the Funcchain syntax into LangChain runnables, enabling you to invoke, stream, or batch process your pipelines effortlessly.
For similar tasks
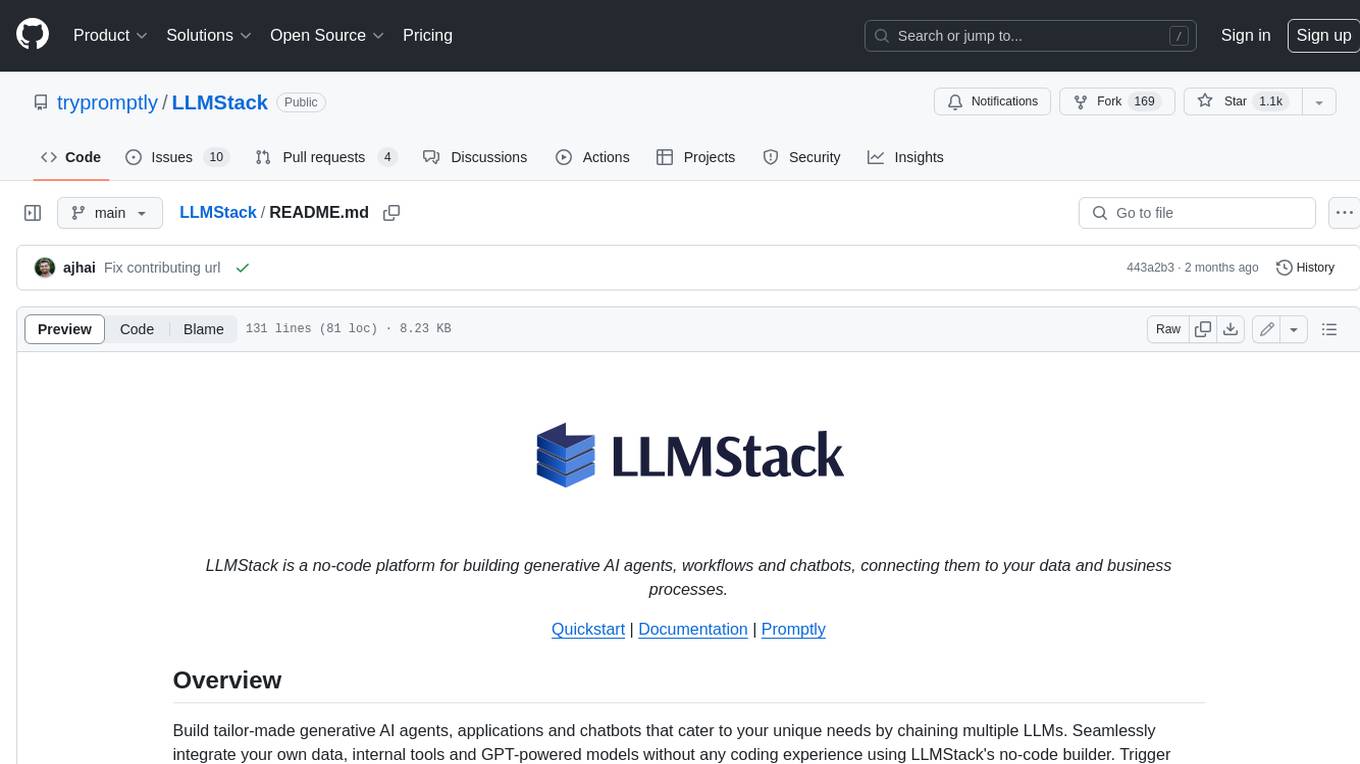
LLMStack
LLMStack is a no-code platform for building generative AI agents, workflows, and chatbots. It allows users to connect their own data, internal tools, and GPT-powered models without any coding experience. LLMStack can be deployed to the cloud or on-premise and can be accessed via HTTP API or triggered from Slack or Discord.
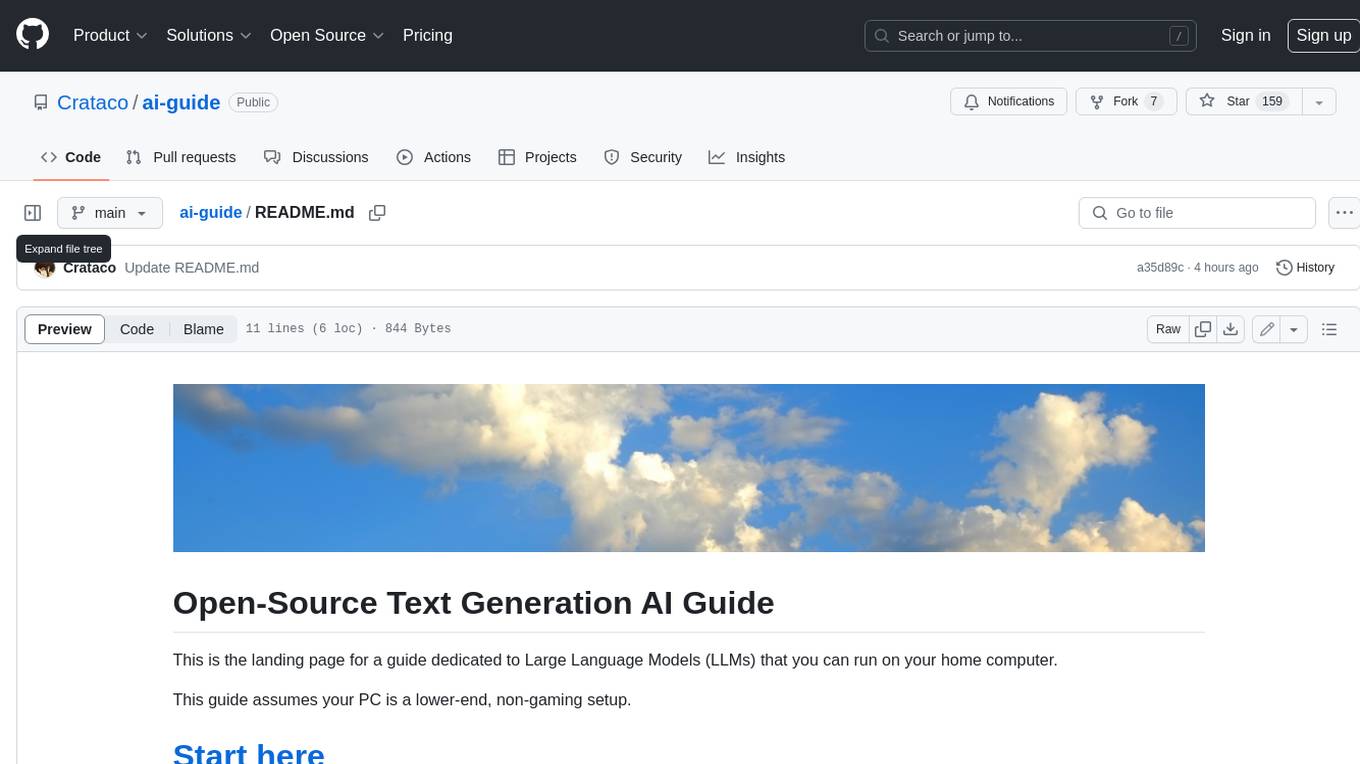
ai-guide
This guide is dedicated to Large Language Models (LLMs) that you can run on your home computer. It assumes your PC is a lower-end, non-gaming setup.
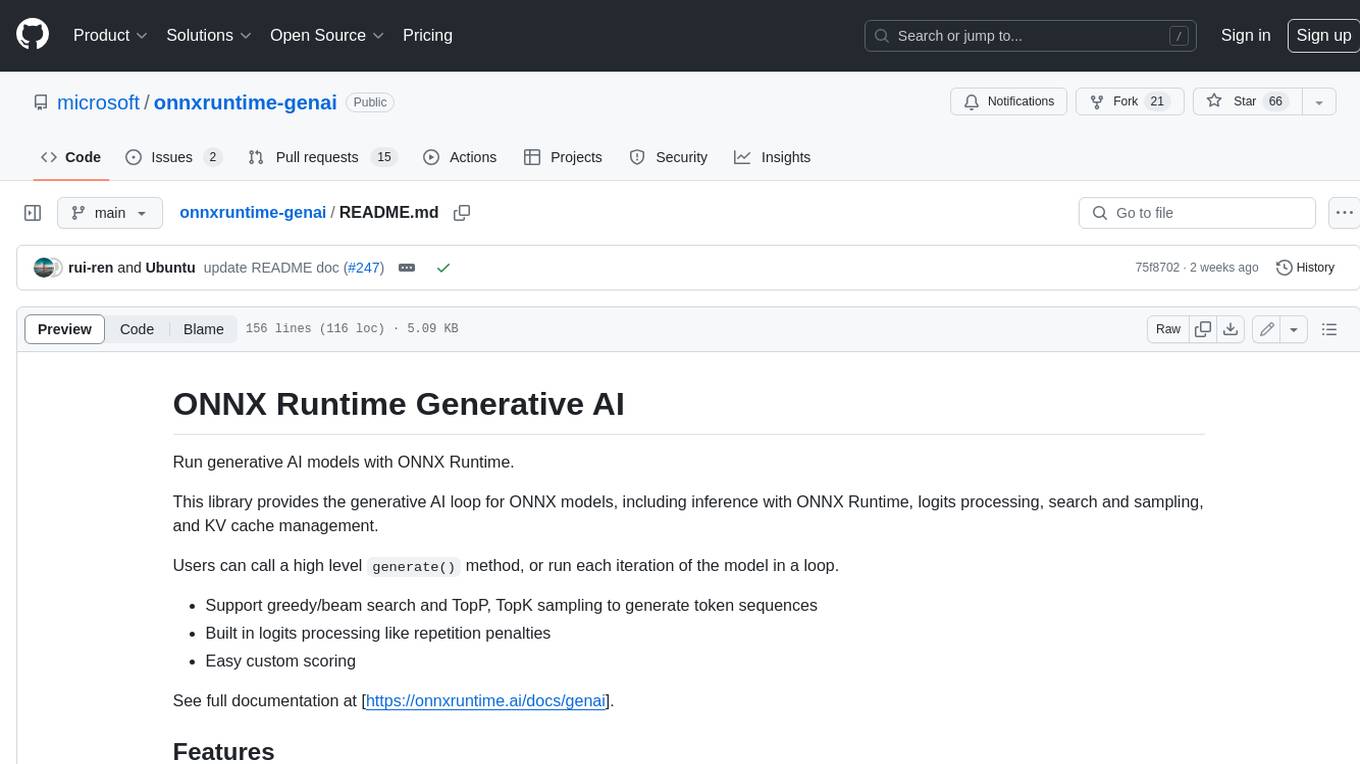
onnxruntime-genai
ONNX Runtime Generative AI is a library that provides the generative AI loop for ONNX models, including inference with ONNX Runtime, logits processing, search and sampling, and KV cache management. Users can call a high level `generate()` method, or run each iteration of the model in a loop. It supports greedy/beam search and TopP, TopK sampling to generate token sequences, has built in logits processing like repetition penalties, and allows for easy custom scoring.
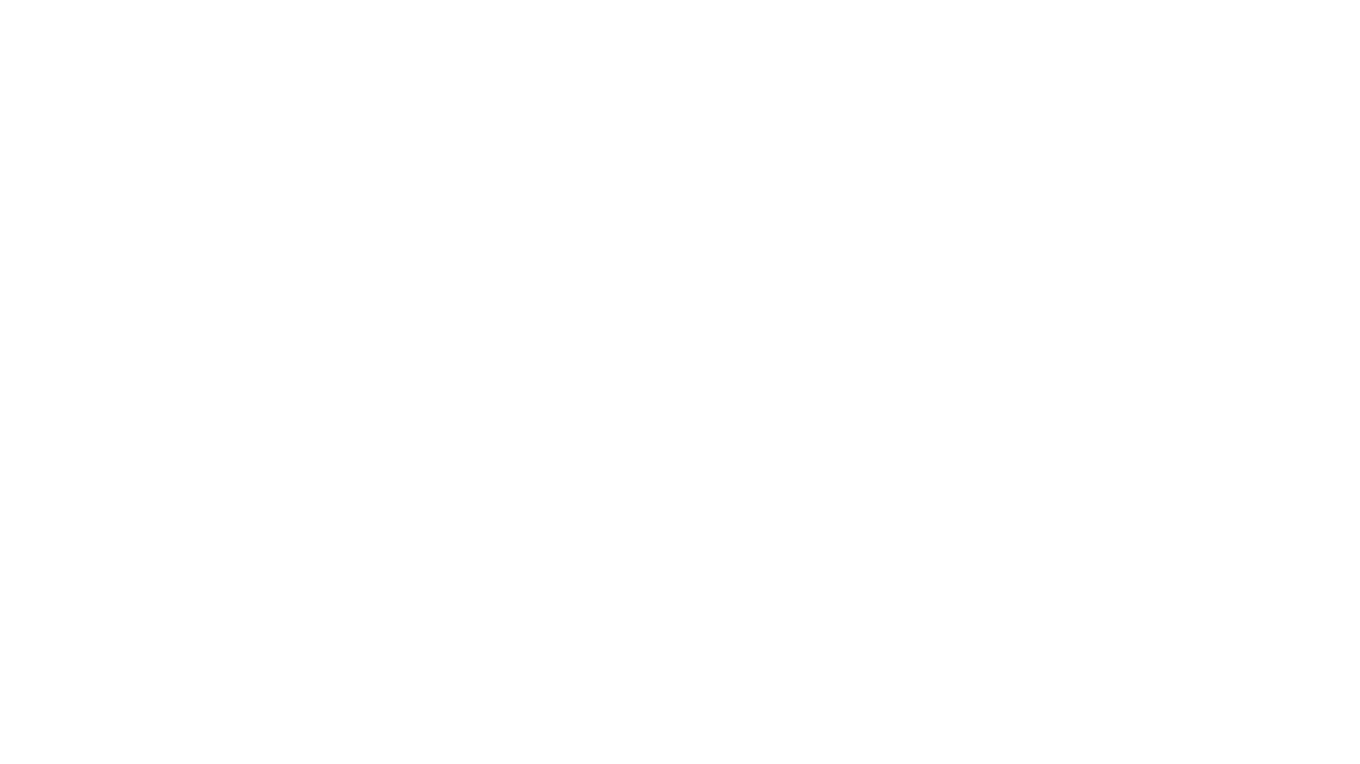
jupyter-ai
Jupyter AI connects generative AI with Jupyter notebooks. It provides a user-friendly and powerful way to explore generative AI models in notebooks and improve your productivity in JupyterLab and the Jupyter Notebook. Specifically, Jupyter AI offers: * An `%%ai` magic that turns the Jupyter notebook into a reproducible generative AI playground. This works anywhere the IPython kernel runs (JupyterLab, Jupyter Notebook, Google Colab, Kaggle, VSCode, etc.). * A native chat UI in JupyterLab that enables you to work with generative AI as a conversational assistant. * Support for a wide range of generative model providers, including AI21, Anthropic, AWS, Cohere, Gemini, Hugging Face, NVIDIA, and OpenAI. * Local model support through GPT4All, enabling use of generative AI models on consumer grade machines with ease and privacy.
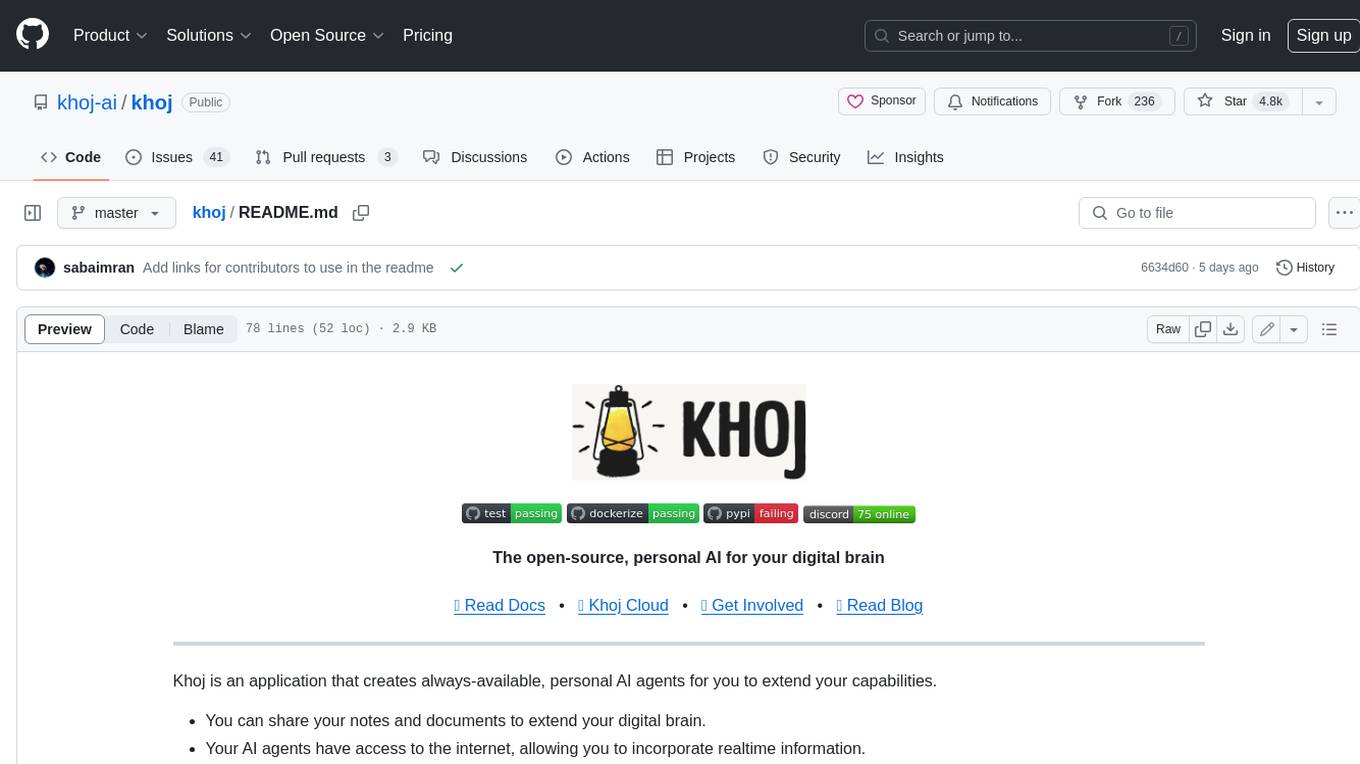
khoj
Khoj is an open-source, personal AI assistant that extends your capabilities by creating always-available AI agents. You can share your notes and documents to extend your digital brain, and your AI agents have access to the internet, allowing you to incorporate real-time information. Khoj is accessible on Desktop, Emacs, Obsidian, Web, and Whatsapp, and you can share PDF, markdown, org-mode, notion files, and GitHub repositories. You'll get fast, accurate semantic search on top of your docs, and your agents can create deeply personal images and understand your speech. Khoj is self-hostable and always will be.
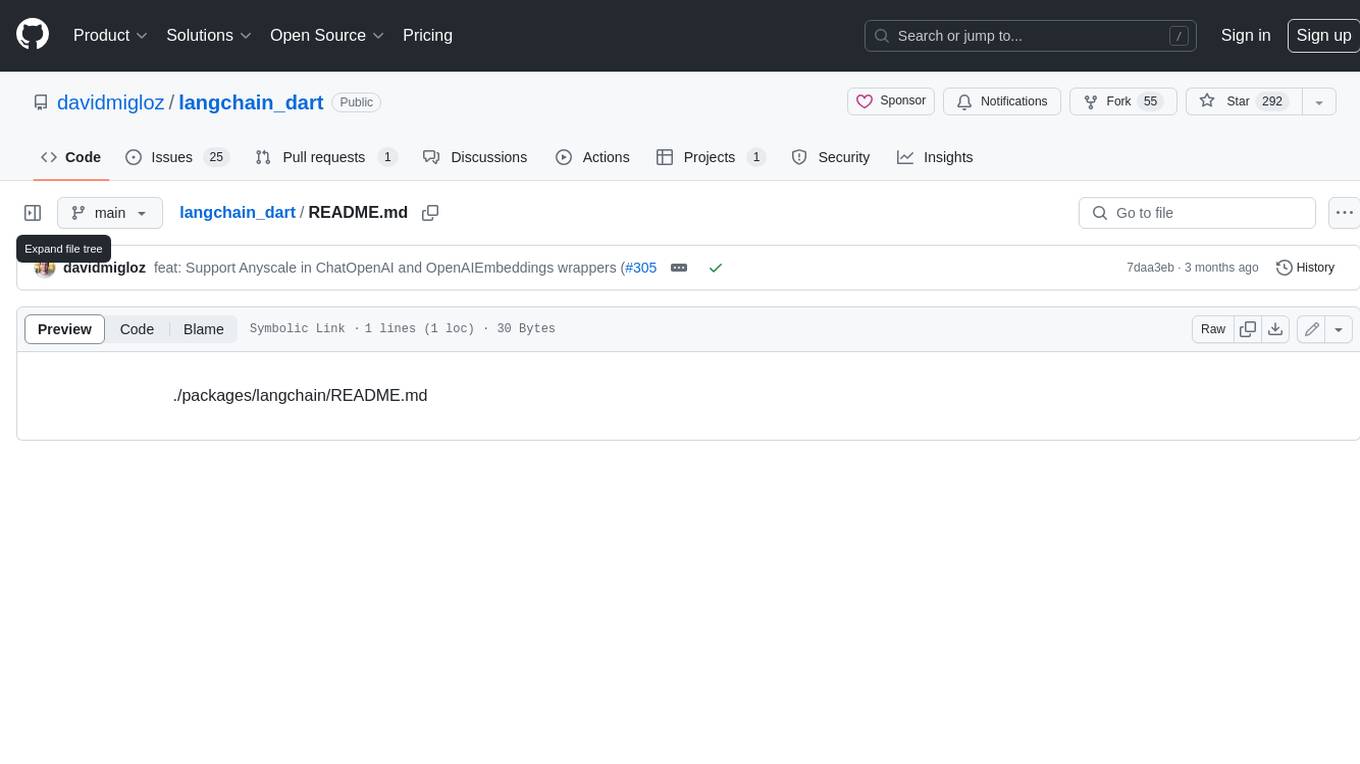
langchain_dart
LangChain.dart is a Dart port of the popular LangChain Python framework created by Harrison Chase. LangChain provides a set of ready-to-use components for working with language models and a standard interface for chaining them together to formulate more advanced use cases (e.g. chatbots, Q&A with RAG, agents, summarization, extraction, etc.). The components can be grouped into a few core modules: * **Model I/O:** LangChain offers a unified API for interacting with various LLM providers (e.g. OpenAI, Google, Mistral, Ollama, etc.), allowing developers to switch between them with ease. Additionally, it provides tools for managing model inputs (prompt templates and example selectors) and parsing the resulting model outputs (output parsers). * **Retrieval:** assists in loading user data (via document loaders), transforming it (with text splitters), extracting its meaning (using embedding models), storing (in vector stores) and retrieving it (through retrievers) so that it can be used to ground the model's responses (i.e. Retrieval-Augmented Generation or RAG). * **Agents:** "bots" that leverage LLMs to make informed decisions about which available tools (such as web search, calculators, database lookup, etc.) to use to accomplish the designated task. The different components can be composed together using the LangChain Expression Language (LCEL).
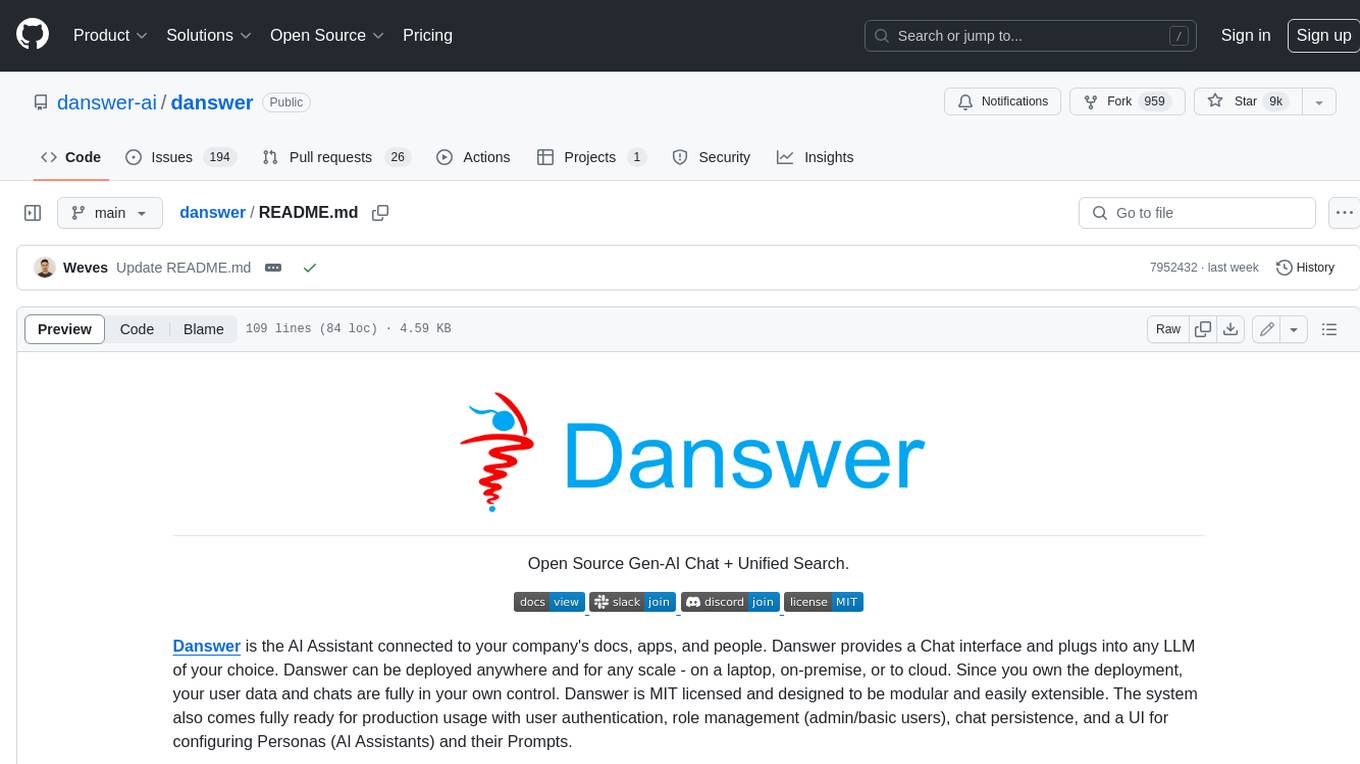
danswer
Danswer is an open-source Gen-AI Chat and Unified Search tool that connects to your company's docs, apps, and people. It provides a Chat interface and plugs into any LLM of your choice. Danswer can be deployed anywhere and for any scale - on a laptop, on-premise, or to cloud. Since you own the deployment, your user data and chats are fully in your own control. Danswer is MIT licensed and designed to be modular and easily extensible. The system also comes fully ready for production usage with user authentication, role management (admin/basic users), chat persistence, and a UI for configuring Personas (AI Assistants) and their Prompts. Danswer also serves as a Unified Search across all common workplace tools such as Slack, Google Drive, Confluence, etc. By combining LLMs and team specific knowledge, Danswer becomes a subject matter expert for the team. Imagine ChatGPT if it had access to your team's unique knowledge! It enables questions such as "A customer wants feature X, is this already supported?" or "Where's the pull request for feature Y?"
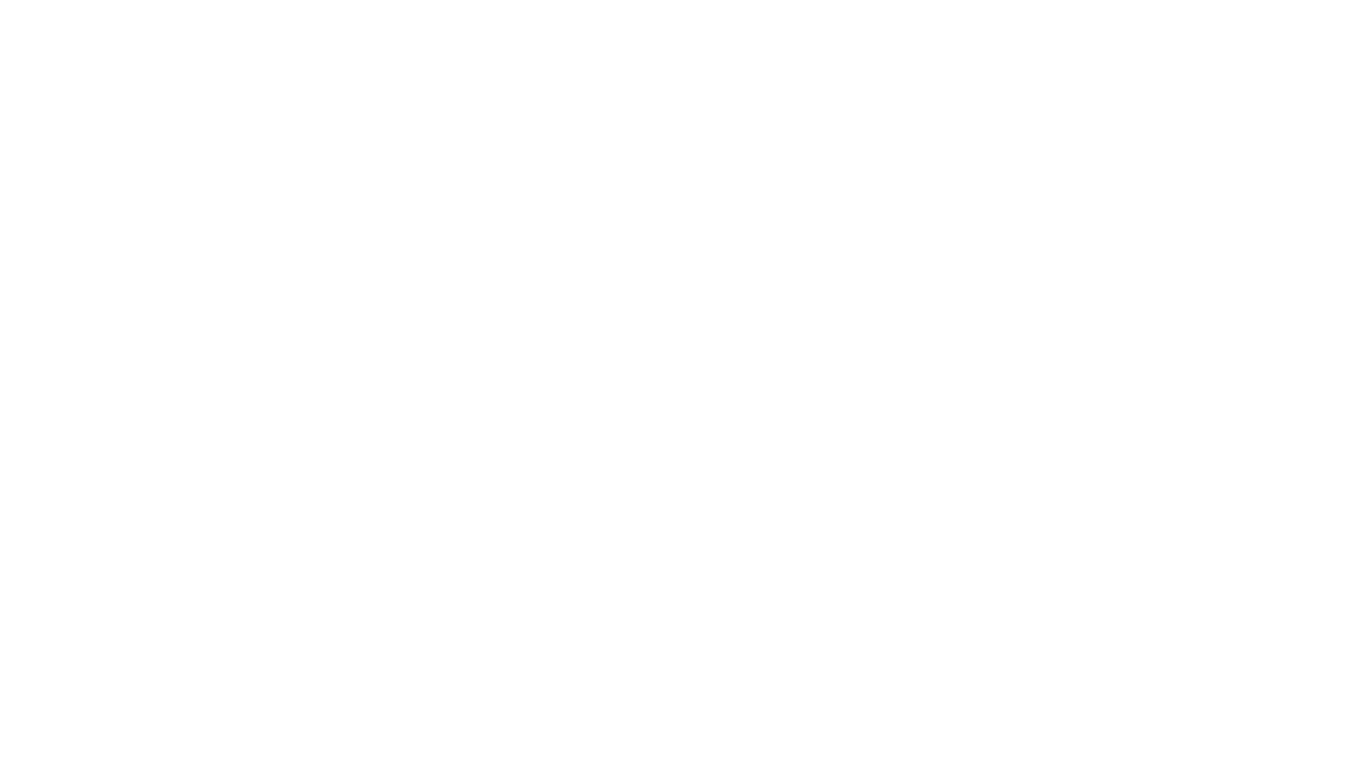
infinity
Infinity is an AI-native database designed for LLM applications, providing incredibly fast full-text and vector search capabilities. It supports a wide range of data types, including vectors, full-text, and structured data, and offers a fused search feature that combines multiple embeddings and full text. Infinity is easy to use, with an intuitive Python API and a single-binary architecture that simplifies deployment. It achieves high performance, with 0.1 milliseconds query latency on million-scale vector datasets and up to 15K QPS.
For similar jobs
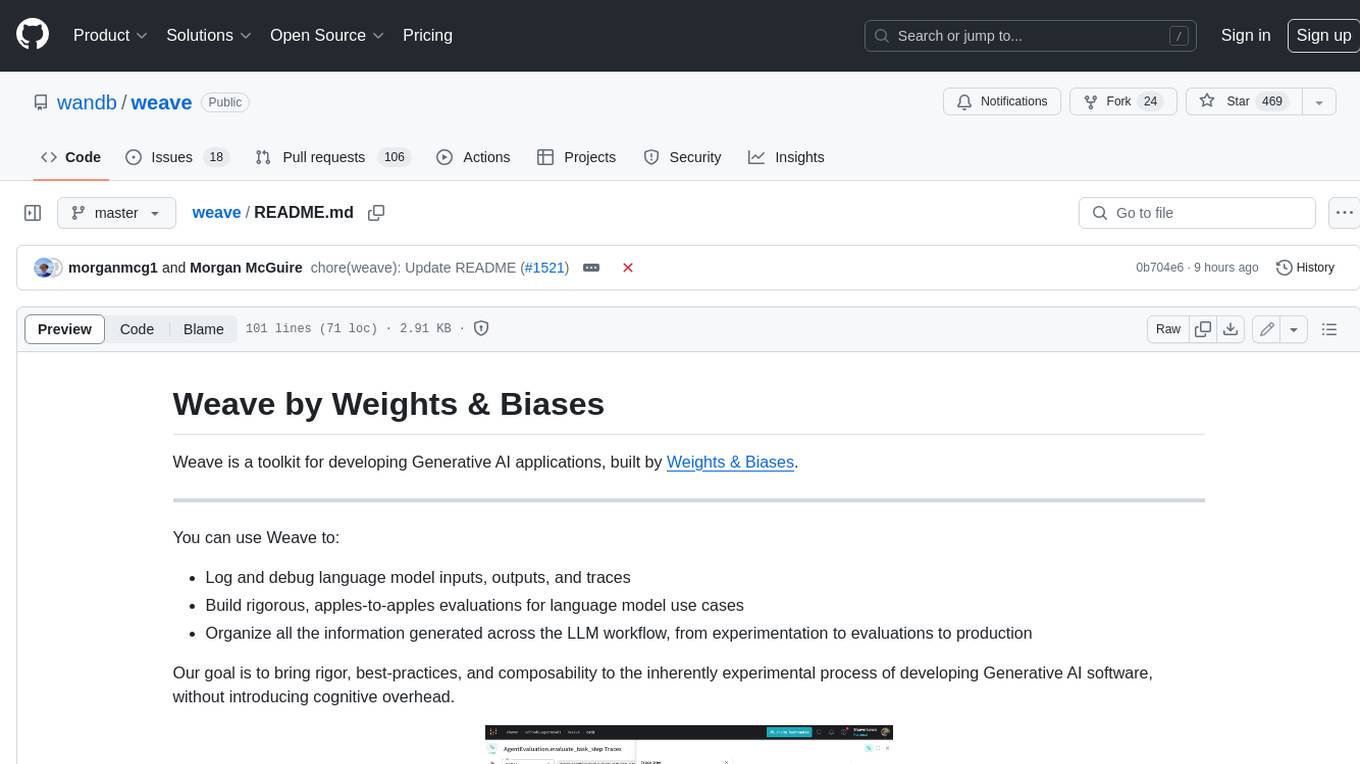
weave
Weave is a toolkit for developing Generative AI applications, built by Weights & Biases. With Weave, you can log and debug language model inputs, outputs, and traces; build rigorous, apples-to-apples evaluations for language model use cases; and organize all the information generated across the LLM workflow, from experimentation to evaluations to production. Weave aims to bring rigor, best-practices, and composability to the inherently experimental process of developing Generative AI software, without introducing cognitive overhead.
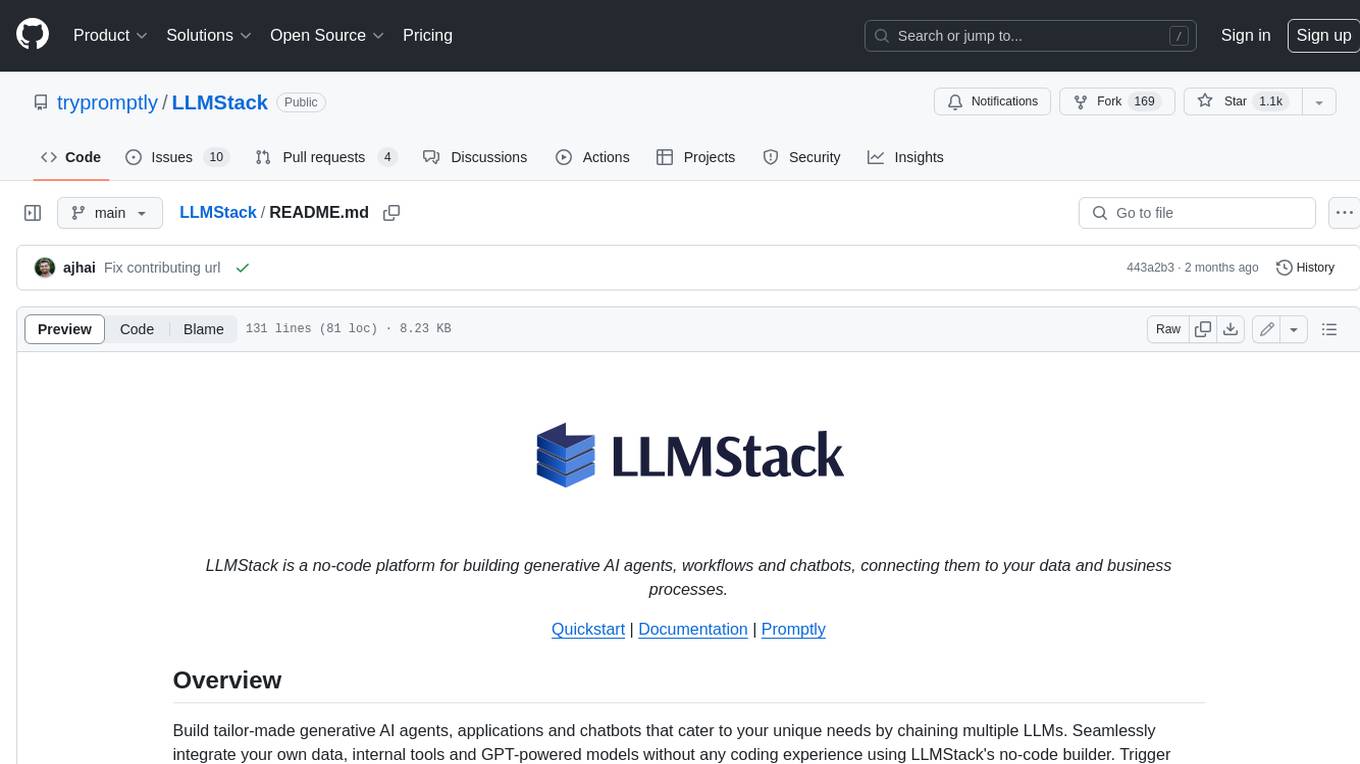
LLMStack
LLMStack is a no-code platform for building generative AI agents, workflows, and chatbots. It allows users to connect their own data, internal tools, and GPT-powered models without any coding experience. LLMStack can be deployed to the cloud or on-premise and can be accessed via HTTP API or triggered from Slack or Discord.
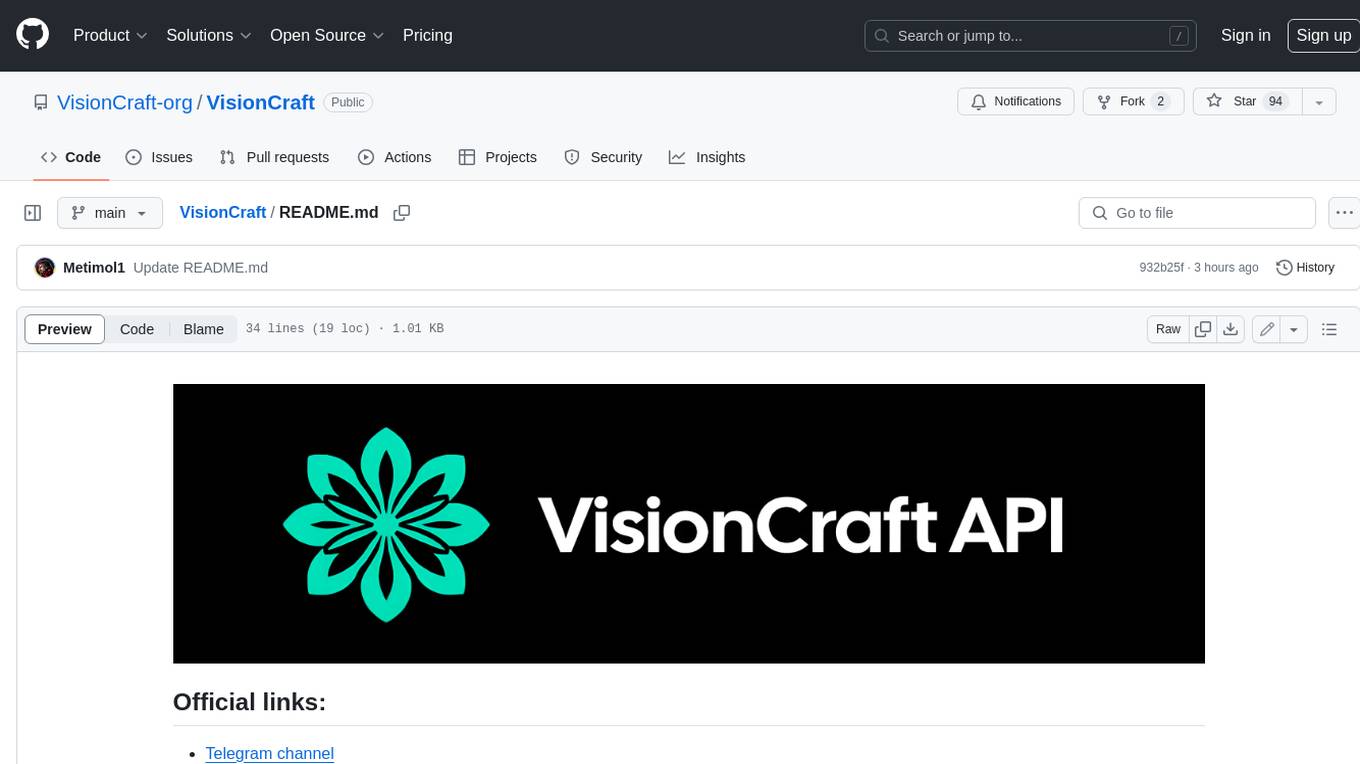
VisionCraft
The VisionCraft API is a free API for using over 100 different AI models. From images to sound.
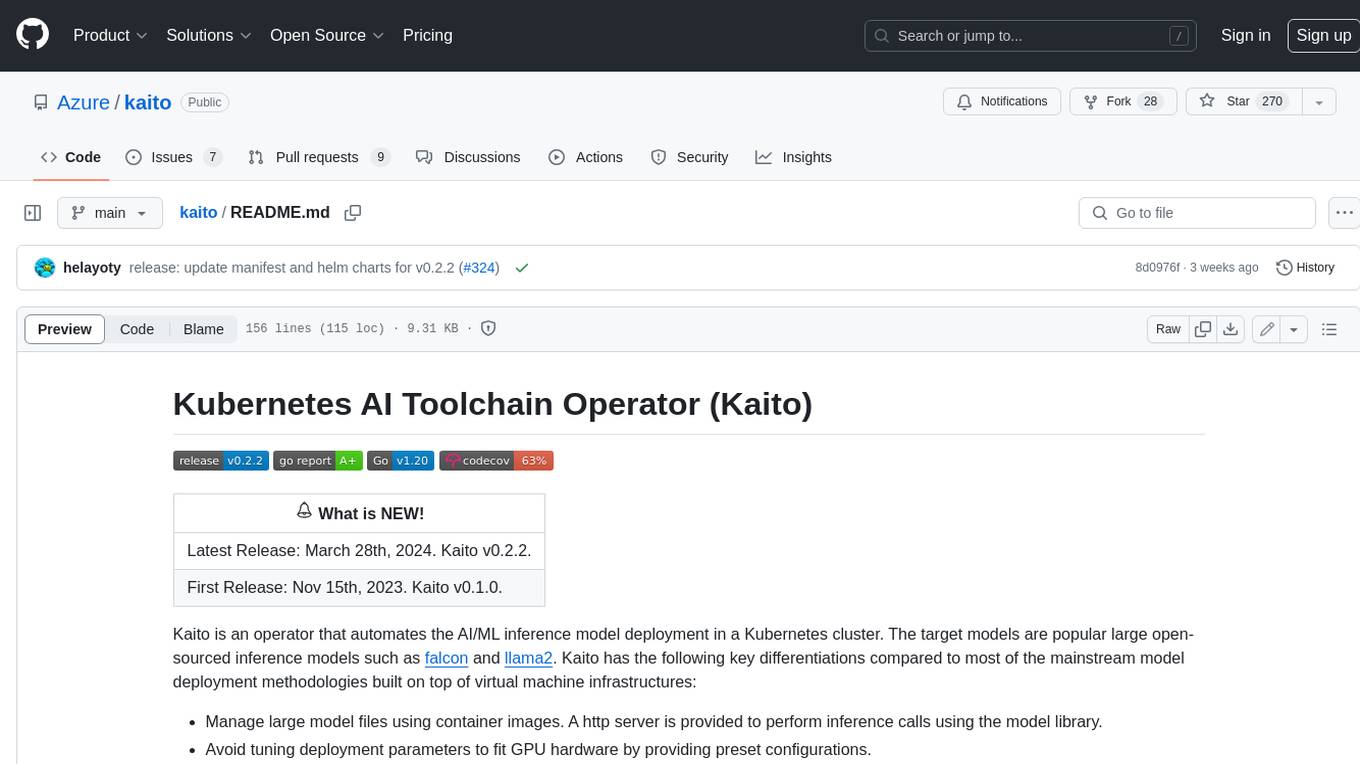
kaito
Kaito is an operator that automates the AI/ML inference model deployment in a Kubernetes cluster. It manages large model files using container images, avoids tuning deployment parameters to fit GPU hardware by providing preset configurations, auto-provisions GPU nodes based on model requirements, and hosts large model images in the public Microsoft Container Registry (MCR) if the license allows. Using Kaito, the workflow of onboarding large AI inference models in Kubernetes is largely simplified.
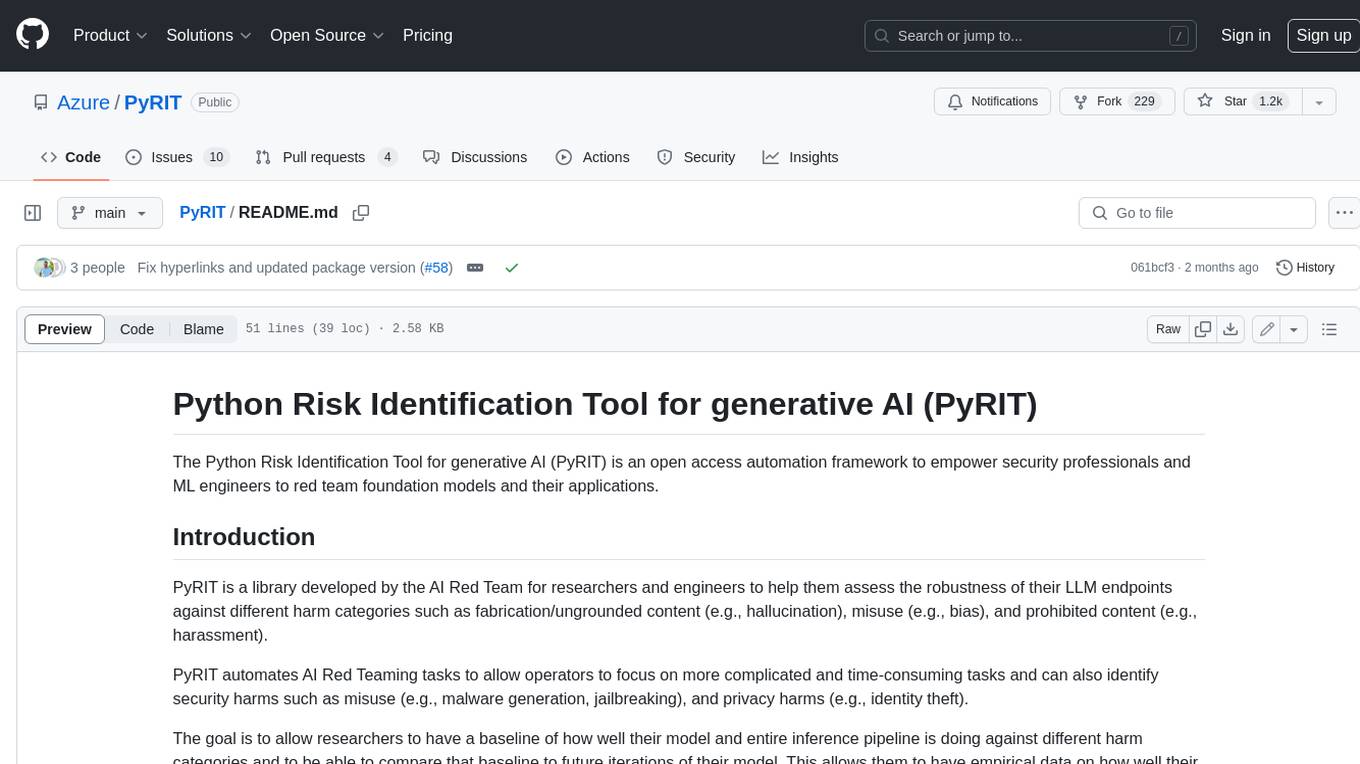
PyRIT
PyRIT is an open access automation framework designed to empower security professionals and ML engineers to red team foundation models and their applications. It automates AI Red Teaming tasks to allow operators to focus on more complicated and time-consuming tasks and can also identify security harms such as misuse (e.g., malware generation, jailbreaking), and privacy harms (e.g., identity theft). The goal is to allow researchers to have a baseline of how well their model and entire inference pipeline is doing against different harm categories and to be able to compare that baseline to future iterations of their model. This allows them to have empirical data on how well their model is doing today, and detect any degradation of performance based on future improvements.
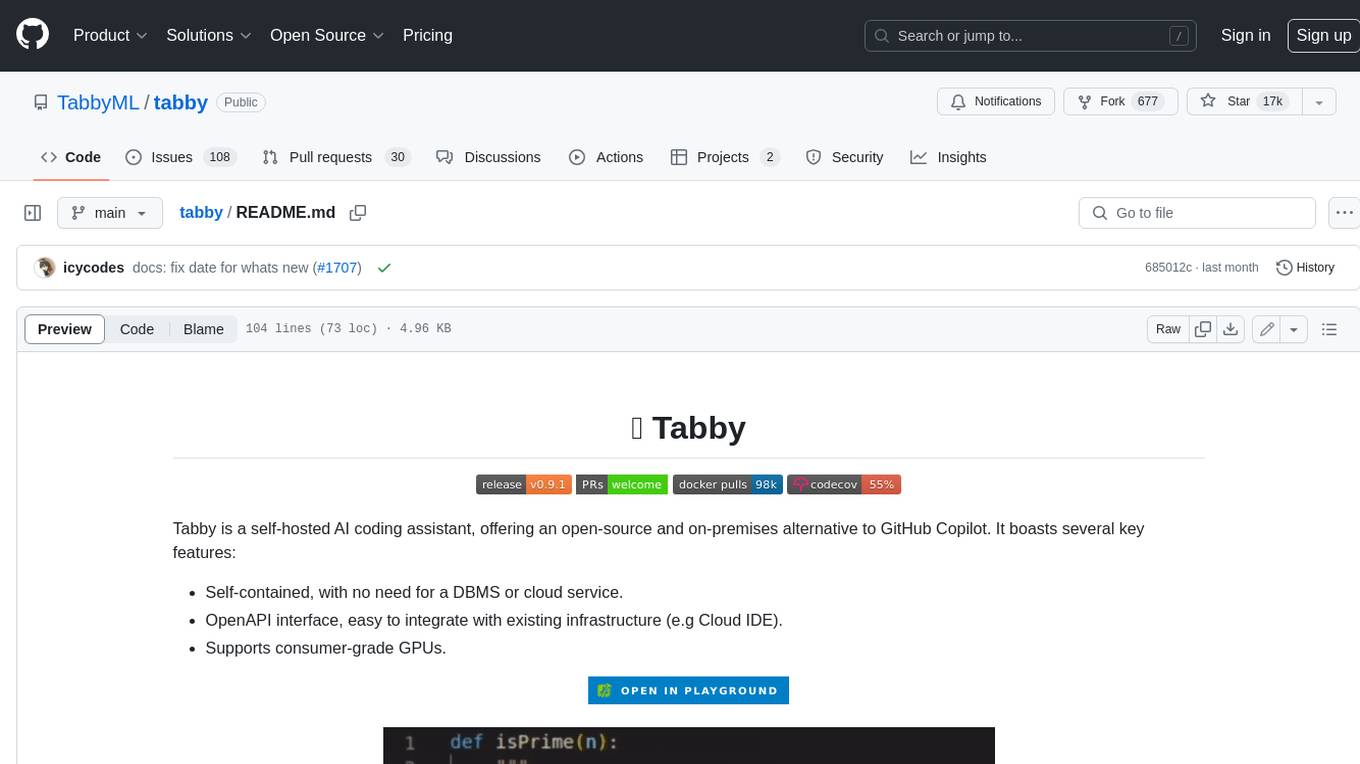
tabby
Tabby is a self-hosted AI coding assistant, offering an open-source and on-premises alternative to GitHub Copilot. It boasts several key features: * Self-contained, with no need for a DBMS or cloud service. * OpenAPI interface, easy to integrate with existing infrastructure (e.g Cloud IDE). * Supports consumer-grade GPUs.
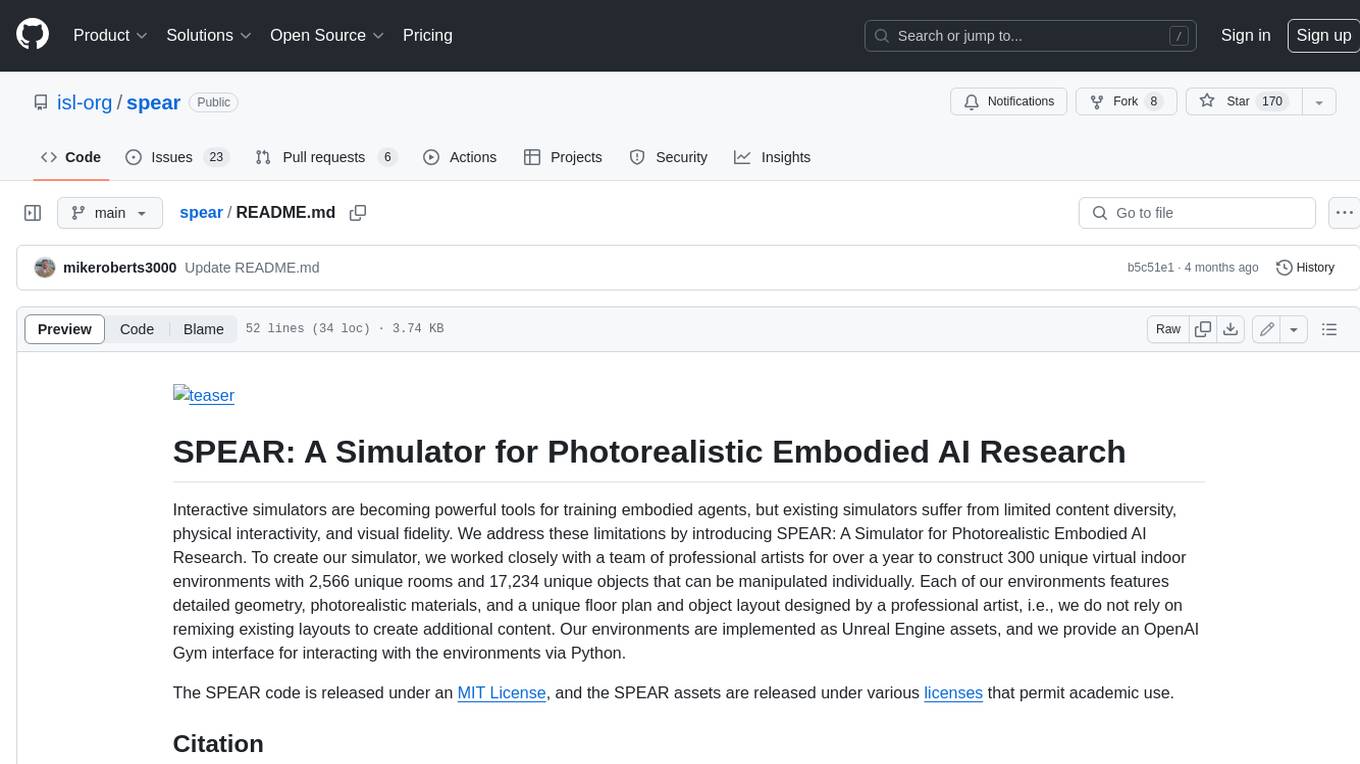
spear
SPEAR (Simulator for Photorealistic Embodied AI Research) is a powerful tool for training embodied agents. It features 300 unique virtual indoor environments with 2,566 unique rooms and 17,234 unique objects that can be manipulated individually. Each environment is designed by a professional artist and features detailed geometry, photorealistic materials, and a unique floor plan and object layout. SPEAR is implemented as Unreal Engine assets and provides an OpenAI Gym interface for interacting with the environments via Python.
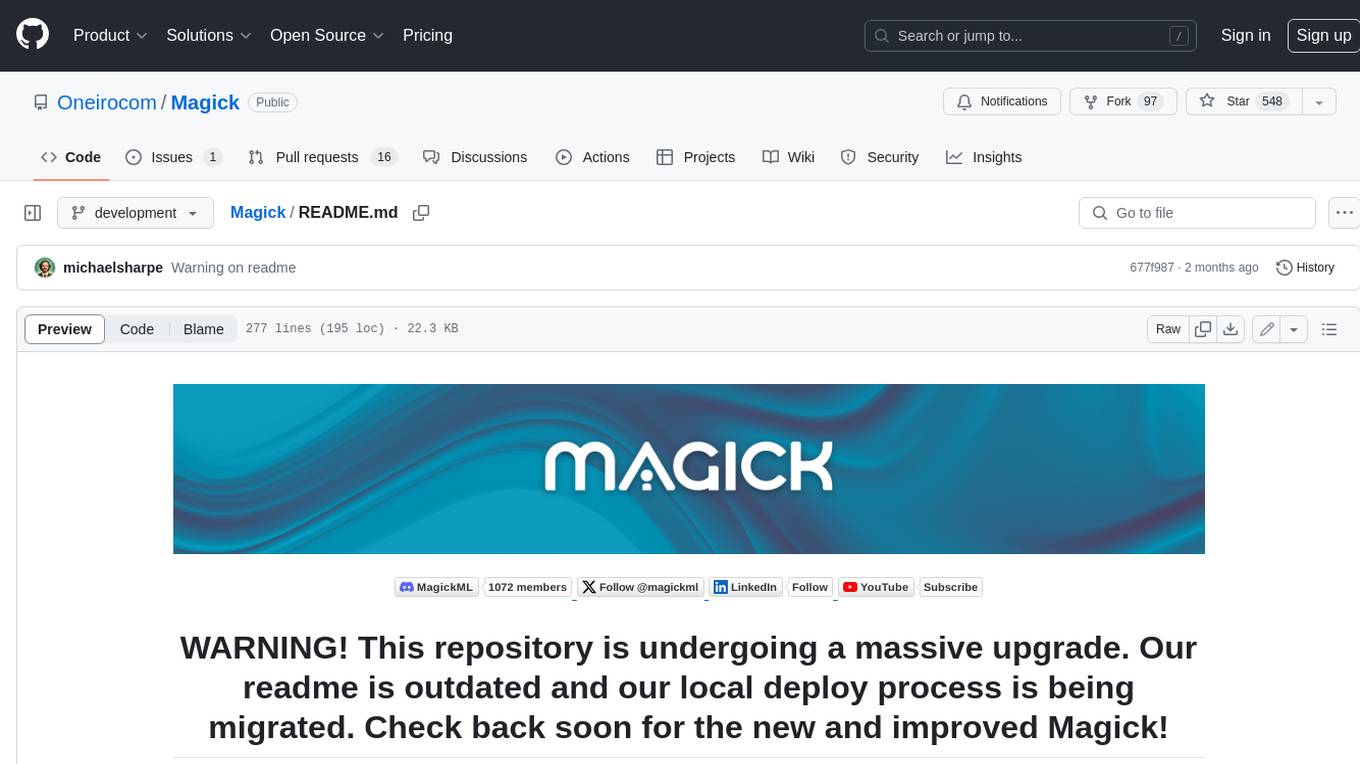
Magick
Magick is a groundbreaking visual AIDE (Artificial Intelligence Development Environment) for no-code data pipelines and multimodal agents. Magick can connect to other services and comes with nodes and templates well-suited for intelligent agents, chatbots, complex reasoning systems and realistic characters.