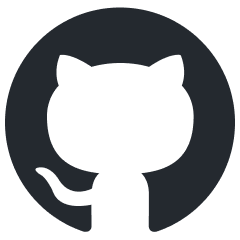
aiorun
A "run" function for asyncio-based apps that does all the boilerplate.
Stars: 445
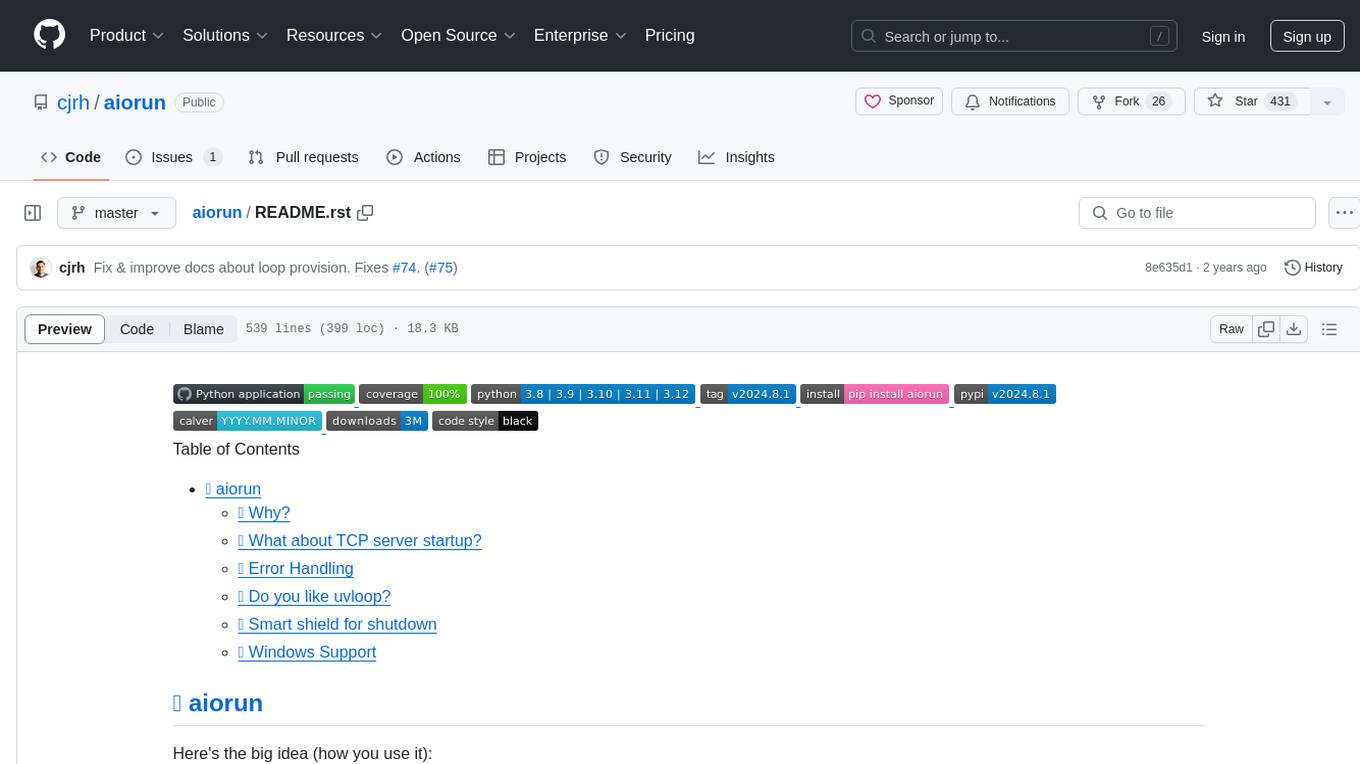
aiorun is a Python package that provides a `run()` function as the starting point of your `asyncio`-based application. The `run()` function handles everything needed during the shutdown sequence of the application, such as creating a `Task` for the given coroutine, running the event loop, adding signal handlers for `SIGINT` and `SIGTERM`, cancelling tasks, waiting for the executor to complete shutdown, and closing the loop. It automates standard actions for asyncio apps, eliminating the need to write boilerplate code. The package also offers error handling options and tools for specific scenarios like TCP server startup and smart shield for shutdown.
README:
.. image:: https://github.com/cjrh/aiorun/workflows/Python%20application/badge.svg :target: https://github.com/cjrh/aiorun/actions
.. image:: https://coveralls.io/repos/github/cjrh/aiorun/badge.svg?branch=master :target: https://coveralls.io/github/cjrh/aiorun?branch=master
.. image:: https://img.shields.io/pypi/pyversions/aiorun.svg :target: https://pypi.python.org/pypi/aiorun
.. image:: https://img.shields.io/github/tag/cjrh/aiorun.svg :target: https://img.shields.io/github/tag/cjrh/aiorun.svg
.. image:: https://img.shields.io/badge/install-pip%20install%20aiorun-ff69b4.svg :target: https://img.shields.io/badge/install-pip%20install%20aiorun-ff69b4.svg
.. image:: https://img.shields.io/pypi/v/aiorun.svg :target: https://pypi.org/project/aiorun/
.. image:: https://img.shields.io/badge/calver-YYYY.MM.MINOR-22bfda.svg :alt: This project uses calendar-based versioning scheme :target: http://calver.org/
.. image:: https://pepy.tech/badge/aiorun :alt: Downloads :target: https://pepy.tech/project/aiorun
.. image:: https://img.shields.io/badge/code%20style-black-000000.svg :alt: This project uses the "black" style formatter for Python code :target: https://github.com/python/black
.. contents:: Table of Contents
Here's the big idea (how you use it):
.. code-block:: python
import asyncio from aiorun import run
async def main(): # Put your application code here await asyncio.sleep(1.0)
if name == 'main': run(main())
This package provides a run()
function as the starting point
of your asyncio
-based application. The run()
function will
run forever. If you want to shut down when main()
completes, just
call loop.stop()
inside it: that will initiate shutdown.
.. warning::
Note that `aiorun.run(coro)` will run **forever**, unlike the standard
library's ``asyncio.run()`` helper. You can call `aiorun.run()`
without a coroutine parameter, and it will still run forever.
This is surprising to many people, because they sometimes expect that
unhandled exceptions should abort the program, with an exception and
a traceback. If you want this behaviour, please see the section on
*error handling* further down.
.. warning::
Note that `aiorun.run(coro)` will create a **new event loop instance**
every time it is invoked (same as `asyncio.run`). This might cause
confusing errors if your code interacts with the default event loop
instance provided by the stdlib `asyncio` library. For such situations
you can provide the actual loop you're using with
`aiorun.run(coro, loop=loop)`. There is more info about this further down.
However, generally speaking, configuring your own loop and providing
it in this way is a code smell. You will find it much easier to
reason about your code if you do all your task creation *inside*
an async context, such as within an `async def` function, because then
there will no ambiguity about which event loop is in play: it will
always be the one returned by `asyncio.get_running_loop()`.
The run()
function will handle everything that normally needs
to be done during the shutdown sequence of the application. All you
need to do is write your coroutines and run them.
So what the heck does run()
do exactly?? It does these standard,
idiomatic actions for asyncio apps:
- creates a
Task
for the given coroutine (schedules it on the event loop), - calls
loop.run_forever()
, - adds default (and smart) signal handlers for both
SIGINT
andSIGTERM
that will stop the loop; - and when the loop stops (either by signal or called directly), then it will...
- ...gather all outstanding tasks,
- cancel them using
task.cancel()
, - resume running the loop until all those tasks are done,
- wait for the executor to complete shutdown, and
- finally close the loop.
All of this stuff is boilerplate that you will never have to write
again. So, if you use aiorun
this is what you need to remember:
- Spawn all your work from a single, starting coroutine
- When a shutdown signal is received, all currently-pending tasks
will have
CancelledError
raised internally. It's up to you whether you want to handle this inside each coroutine with atry/except
or not. - If you want to protect coros from cancellation, see
shutdown_waits_for()
further down. - Try to have executor jobs be shortish, since the shutdown process will wait
for them to finish. If you need a long-running thread or process tasks, use
a dedicated thread/subprocess and set
daemon=True
instead.
There's not much else to know for general use. aiorun
has a few special
tools that you might need in unusual circumstances. These are discussed
next.
You will see in many examples online that for servers, startup happens in
several run_until_complete()
phases before the primary run_forever()
which is the "main" running part of the program. How do we handle that with
aiorun?
Let's recreate the echo client & server <https://docs.python.org/3/library/asyncio-stream.html#tcp-echo-client-using-streams>
_
examples from the Standard Library documentation:
Client:
.. code-block:: python
# echo_client.py
import asyncio
from aiorun import run
async def tcp_echo_client(message):
# Same as original!
reader, writer = await asyncio.open_connection('127.0.0.1', 8888)
print('Send: %r' % message)
writer.write(message.encode())
data = await reader.read(100)
print('Received: %r' % data.decode())
print('Close the socket')
writer.close()
asyncio.get_event_loop().stop() # Exit after one msg like original
message = 'Hello World!'
run(tcp_echo_client(message))
Server:
.. code-block:: python
import asyncio
from aiorun import run
async def handle_echo(reader, writer):
# Same as original!
data = await reader.read(100)
message = data.decode()
addr = writer.get_extra_info('peername')
print("Received %r from %r" % (message, addr))
print("Send: %r" % message)
writer.write(data)
await writer.drain()
print("Close the client socket")
writer.close()
async def main():
server = await asyncio.start_server(handle_echo, '127.0.0.1', 8888)
print('Serving on {}'.format(server.sockets[0].getsockname()))
async with server:
await server.serve_forever()
run(main())
It works the same as the original examples, except you see this
when you hit CTRL-C
on the server instance:
.. code-block:: bash
$ python echo_server.py
Running forever.
Serving on ('127.0.0.1', 8888)
Received 'Hello World!' from ('127.0.0.1', 57198)
Send: 'Hello World!'
Close the client socket
^CStopping the loop
Entering shutdown phase.
Cancelling pending tasks.
Cancelling task: <Task pending coro=[...snip...]>
Running pending tasks till complete
Waiting for executor shutdown.
Leaving. Bye!
Task gathering, cancellation, and executor shutdown all happen automatically.
Unlike the standard library's asyncio.run()
method, aiorun.run
will run forever, and does not stop on unhandled exceptions. This is partly
because we predate the standard library method, during the time in which
run_forever()
was actually the recommended API for servers, and partly
because it can make sense for long-lived servers to be resilient to
unhandled exceptions. For example, if 99% of your API works fine, but the
one new endpoint you just added has a bug: do you really want that one new
endpoint to crash-loop your deployed service?
Nevertheless, not all usages of aiorun
are long-lived servers, so some
users would prefer that aiorun.run()
crash on an unhandled exception,
just like any normal Python program. For this, we have an extra parameter
that enables it:
.. code-block:: python
from aiorun import run
async def main(): raise Exception('ouch')
if name == 'main': run(main(), stop_on_unhandled_errors=True)
This produces the following output:
.. code-block::
$ python stop_demo.py
Unhandled exception; stopping loop.
Traceback (most recent call last):
File "/opt/project/examples/stop_unhandled.py", line 9, in <module>
run(main(), stop_on_unhandled_errors=True)
File "/opt/project/aiorun.py", line 294, in run
raise pending_exception_to_raise
File "/opt/project/aiorun.py", line 206, in new_coro
await coro
File "/opt/project/examples/stop_unhandled.py", line 5, in main
raise Exception("ouch")
Exception: ouch
Error handling scenarios can get very complex, and I suggest that you try to keep your error handling as simple as possible. Nevertheless, sometimes people have special needs that require some complexity, so let's look at a few scenarios where error-handling considerations can be more challenging.
aiorun.run()
can also be started without an initial coroutine, in which
case any other created tasks still run as normal; in this case exceptions
still abort the program if the parameter is supplied:
.. code-block:: python
import asyncio
from aiorun import run
async def job():
raise Exception("ouch")
if __name__ == "__main__":
loop = asyncio.new_event_loop()
asyncio.set_event_loop(loop)
loop.create_task(job())
run(loop=loop, stop_on_unhandled_errors=True)
The output is the same as the previous program. In this second example,
we made a our own loop instance and passed that to run()
. It is also possible
to configure your exception handler on the loop, but if you do this the
stop_on_unhandled_errors
parameter is no longer allowed:
.. code-block:: python
import asyncio
from aiorun import run
async def job():
raise Exception("ouch")
if __name__ == "__main__":
loop = asyncio.new_event_loop()
asyncio.set_event_loop(loop)
loop.create_task(job())
loop.set_exception_handler(lambda loop, context: "Error")
run(loop=loop, stop_on_unhandled_errors=True)
But this is not allowed:
.. code-block::
Traceback (most recent call last):
File "/opt/project/examples/stop_unhandled_illegal.py", line 15, in <module>
run(loop=loop, stop_on_unhandled_errors=True)
File "/opt/project/aiorun.py", line 171, in run
raise Exception(
Exception: If you provide a loop instance, and you've configured a
custom exception handler on it, then the 'stop_on_unhandled_errors'
parameter is unavailable (all exceptions will be handled).
/usr/local/lib/python3.8/asyncio/base_events.py:633:
RuntimeWarning: coroutine 'job' was never awaited
Remember that the parameter stop_on_unhandled_errors
is just a convenience. If you're
going to go to the trouble of making your own loop instance anyway, you can
stop the loop yourself inside your own exception handler just fine, and
then you no longer need to set stop_on_unhandled_errors
:
.. code-block:: python
# custom_stop.py
import asyncio
from aiorun import run
async def job():
raise Exception("ouch")
async def other_job():
try:
await asyncio.sleep(10)
except asyncio.CancelledError:
print("other_job was cancelled!")
if __name__ == "__main__":
loop = asyncio.new_event_loop()
asyncio.set_event_loop(loop)
loop.create_task(job())
loop.create_task(other_job())
def handler(loop, context):
# https://docs.python.org/3/library/asyncio-eventloop.html#asyncio.loop.call_exception_handler
print(f'Stopping loop due to error: {context["exception"]} ')
loop.stop()
loop.set_exception_handler(handler=handler)
run(loop=loop)
In this example, we schedule two jobs on the loop. One of them raises an
exception, and you can see in the output that the other job was still
cancelled during shutdown as expected (which is what you expect aiorun
to do!):
.. code-block::
$ python custom_stop.py
Stopping loop due to error: ouch
other_job was cancelled!
Note however that in this situation the exception is being handled by
your custom exception handler, and does not bubble up out of the run()
like you saw in earlier examples. If you want to do something with that
exception, like reraise it or something, you need to capture it inside your
custom exception handler and then do something with it, like add it to a list
that you check after run()
completes, and then reraise there or something
similar.
.. code-block:: python
import asyncio from aiorun import run
async def main():
if name == 'main': run(main(), use_uvloop=True)
Note that you have to pip install uvloop
yourself.
It's unusual, but sometimes you're going to want a coroutine to not get
interrupted by cancellation during the shutdown sequence. You'll look in
the official docs and find asyncio.shield()
.
Unfortunately, shield()
doesn't work in shutdown scenarios because
the protection offered by shield()
only applies if the specific coroutine
inside which the shield()
is used, gets cancelled directly.
Let me explain: if you do a conventional shutdown sequence (like aiorun
is doing internally), this is the sequence of steps:
-
tasks = all_tasks()
, followed by -
[t.cancel() for t in tasks]
, and then run_until_complete(gather(*tasks))
The way shield()
works internally is it creates a secret, inner
taskβwhich also gets included in the all_tasks()
call above! Thus
it also receives a cancellation exception just like everything else.
Therefore, we have an alternative version of shield()
that works better for
us: shutdown_waits_for()
. If you've got a coroutine that must not be
cancelled during the shutdown sequence, just wrap it in
shutdown_waits_for()
!
Here's an example:
.. code-block:: python
import asyncio
from aiorun import run, shutdown_waits_for
async def corofn():
for i in range(10):
print(i)
await asyncio.sleep(1)
print('done!')
async def main():
try:
await shutdown_waits_for(corofn())
except asyncio.CancelledError:
print('oh noes!')
run(main())
If you hit CTRL-C
before 10 seconds has passed, you will see
oh noes!
printed immediately, and then after 10 seconds (since start),
done!
is printed, and thereafter the program exits.
Output:
.. code-block:: shell
$ python testshield.py
0
1
2
3
4
^CStopping the loop
oh noes!
5
6
7
8
9
done!
Behind the scenes, all_tasks()
would have been cancelled by CTRL-C
,
except ones wrapped in shutdown_waits_for()
calls. In this respect, it
is loosely similar to asyncio.shield()
, but with special applicability
to our shutdown scenario in aiorun()
.
Be careful with this: the coroutine should still finish up at some point. The main use case for this is short-lived tasks that you don't want to write explicit cancellation handling.
Oh, and you can use shutdown_waits_for()
as if it were asyncio.shield()
too. For that use-case it works the same. If you're using aiorun
, there
is no reason to use shield()
.
aiorun
also supports Windows! Kinda. Sorta. The root problem with Windows,
for a thing like aiorun
is that Windows doesn't support signal handling
the way Linux or Mac OS X does. Like, at all.
For Linux, aiorun
does "the right thing" out of the box for the
SIGINT
and SIGTERM
signals; i.e., it will catch them and initiate
a safe shutdown process as described earlier. However, on Windows, these
signals don't work.
There are two signals that work on Windows: the CTRL-C
signal (happens
when you press, unsurprisingly, CTRL-C
, and the CTRL-BREAK
signal
which happens when you...well, you get the picture.
The good news is that, for aiorun
, both of these will work. Yay! The bad
news is that for them to work, you have to run your code in a Console
window. Boo!
Fortunately, it turns out that you can run an asyncio-based process not
attached to a Console window, e.g. as a service or a subprocess, and have
it also receive a signal to safely shut down in a controlled way. It turns
out that it is possible to send a CTRL-BREAK
signal to another process,
with no console window involved, but only as long as that process was created
in a particular way and---here is the drop---this targetted process is a
child process of the one sending the signal. Yeah, I know, it's a downer.
There is an example of how to do this in the tests:
.. code-block:: python3
import subprocess as sp
proc = sp.Popen(
['python', 'app.py'],
stdout=sp.PIPE,
stderr=sp.STDOUT,
creationflags=sp.CREATE_NEW_PROCESS_GROUP
)
print(proc.pid)
Notice how we print out the process id (pid
). Then you can send that
process the signal from a completely different process, once you know
the pid
:
.. code-block:: python3
import os, signal
os.kill(pid, signal.CTRL_BREAK_EVENT)
(Remember, os.kill()
doesn't actually kill, it only sends a signal)
aiorun
supports this use-case above, although I'll be pretty surprised
if anyone actually uses it to manage microservices (does anyone do this?)
So to summarize: aiorun
will do a controlled shutdown if either
CTRL-C
or CTRL-BREAK
is entered via keyboard in a Console window
with a running instance, or if the CTRL-BREAK
signal is sent to
a subprocess that was created with the CREATE_NEW_PROCESS_GROUP
flag set. Here <https://stackoverflow.com/a/35792192>
_ is a much more
detailed explanation of these issues.
Finally, uvloop
is not yet supported on Windows so that won't work
either.
At the very least, aiorun
will, well, run on Windows Β―\(γ)/Β―
For Tasks:
Click tags to check more tools for each tasksFor Jobs:
Alternative AI tools for aiorun
Similar Open Source Tools
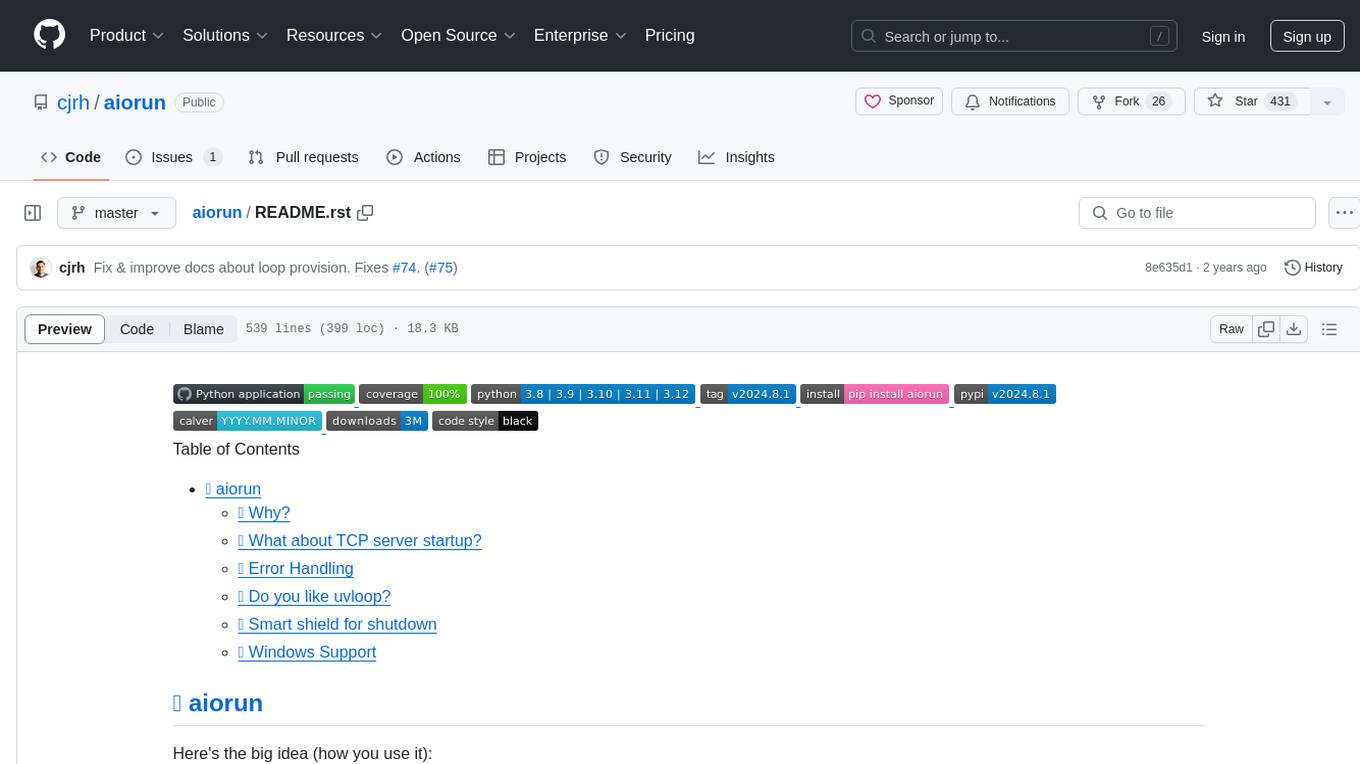
aiorun
aiorun is a Python package that provides a `run()` function as the starting point of your `asyncio`-based application. The `run()` function handles everything needed during the shutdown sequence of the application, such as creating a `Task` for the given coroutine, running the event loop, adding signal handlers for `SIGINT` and `SIGTERM`, cancelling tasks, waiting for the executor to complete shutdown, and closing the loop. It automates standard actions for asyncio apps, eliminating the need to write boilerplate code. The package also offers error handling options and tools for specific scenarios like TCP server startup and smart shield for shutdown.
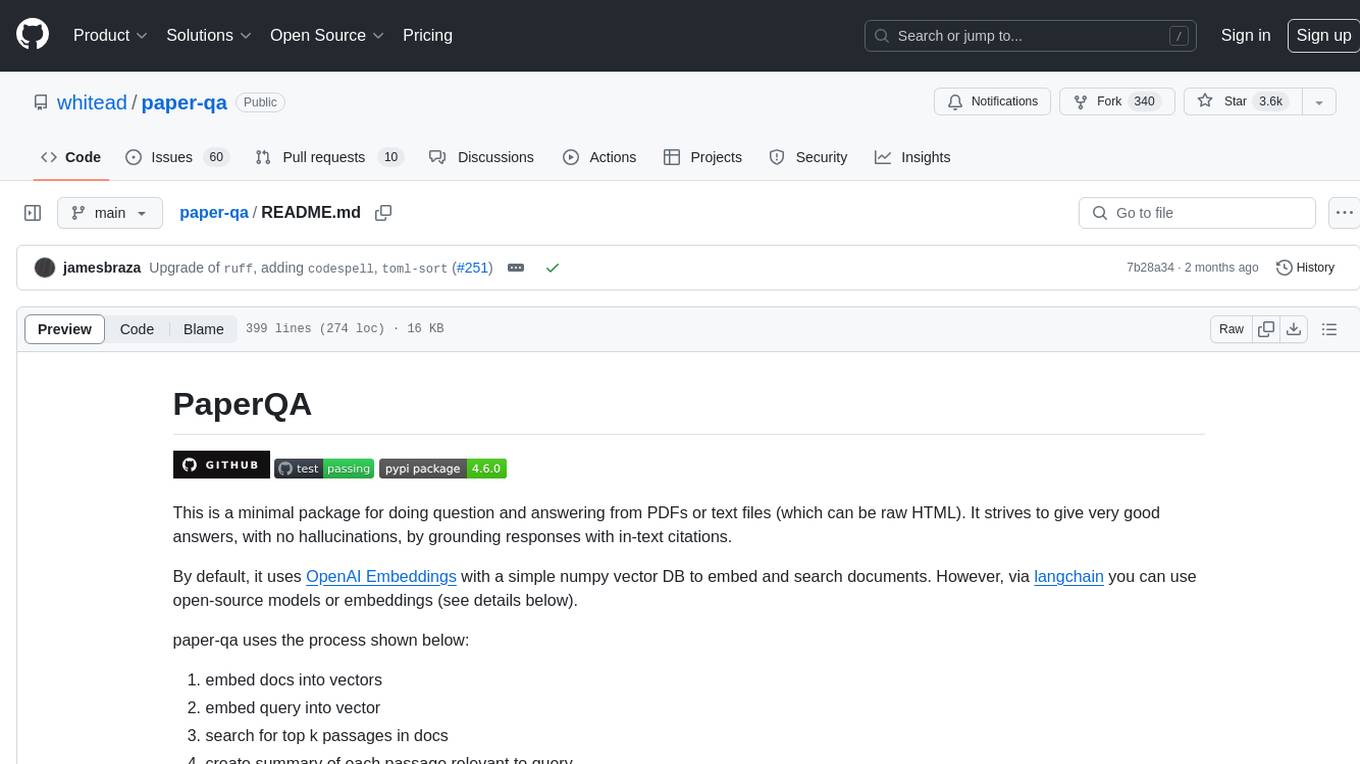
paper-qa
PaperQA is a minimal package for question and answering from PDFs or text files, providing very good answers with in-text citations. It uses OpenAI Embeddings to embed and search documents, and follows a process of embedding docs and queries, searching for top passages, creating summaries, scoring and selecting relevant summaries, putting summaries into prompt, and generating answers. Users can customize prompts and use various models for embeddings and LLMs. The tool can be used asynchronously and supports adding documents from paths, files, or URLs.
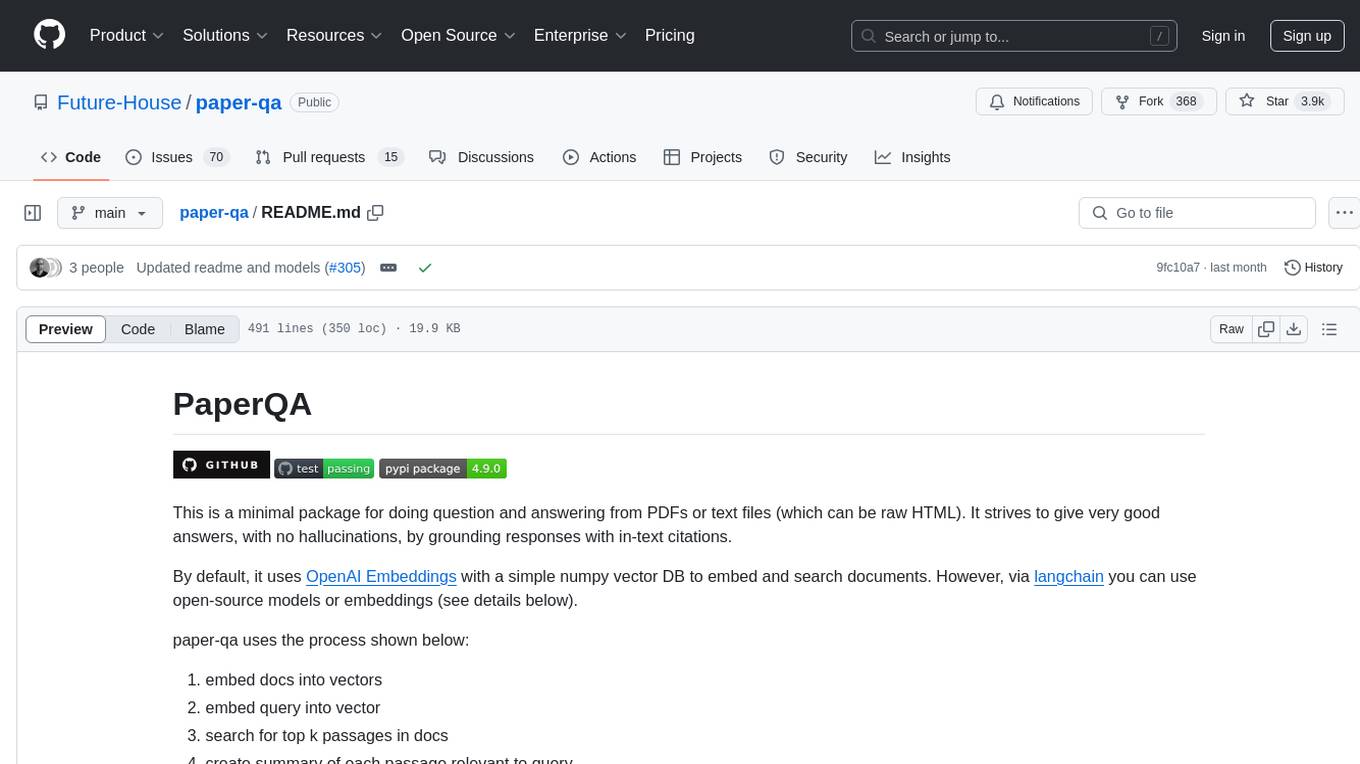
paper-qa
PaperQA is a minimal package for question and answering from PDFs or text files, providing very good answers with in-text citations. It uses OpenAI Embeddings to embed and search documents, and includes a process of embedding docs, queries, searching for top passages, creating summaries, using an LLM to re-score and select relevant summaries, putting summaries into prompt, and generating answers. The tool can be used to answer specific questions related to scientific research by leveraging citations and relevant passages from documents.
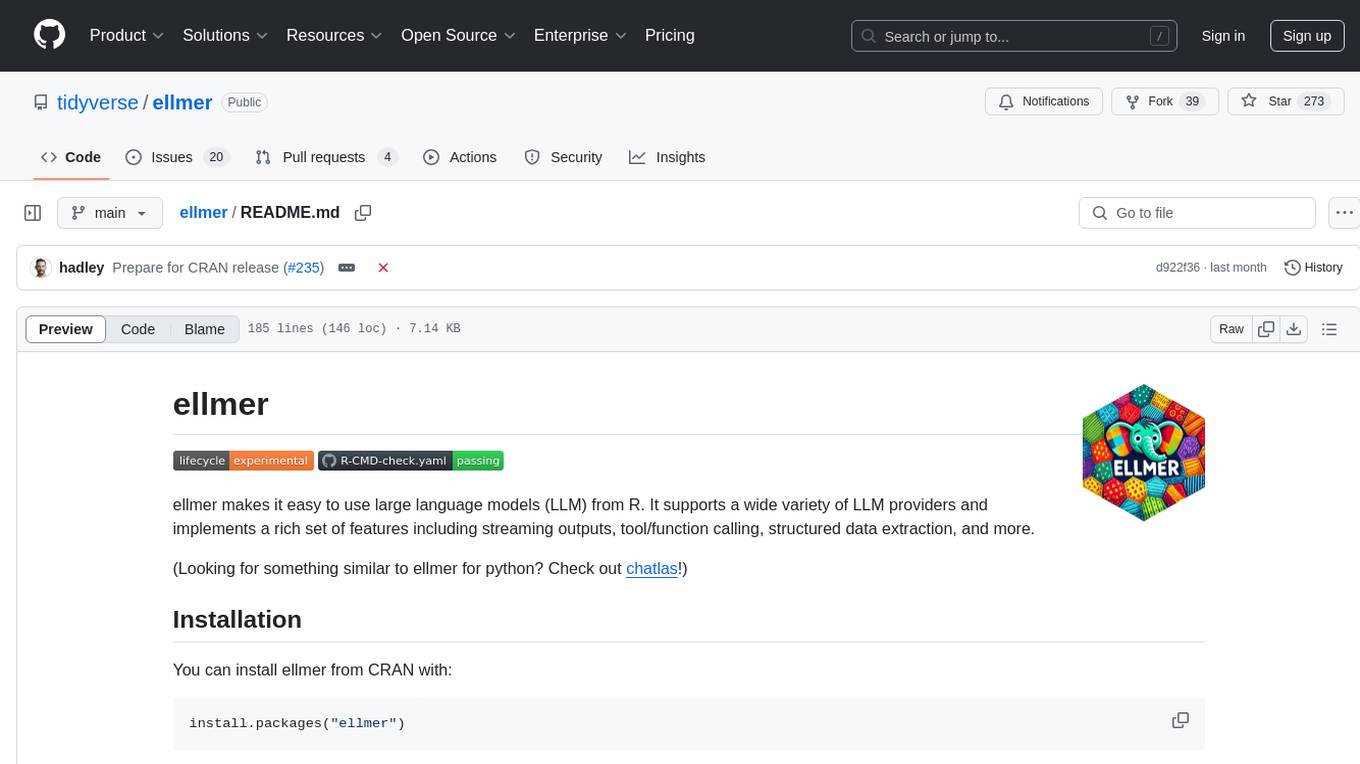
ellmer
ellmer is a tool that facilitates the use of large language models (LLM) from R. It supports various LLM providers and offers features such as streaming outputs, tool/function calling, and structured data extraction. Users can interact with ellmer in different ways, including interactive chat console, interactive method call, and programmatic chat. The tool provides support for multiple model providers and offers recommendations for different use cases, such as exploration or organizational use.
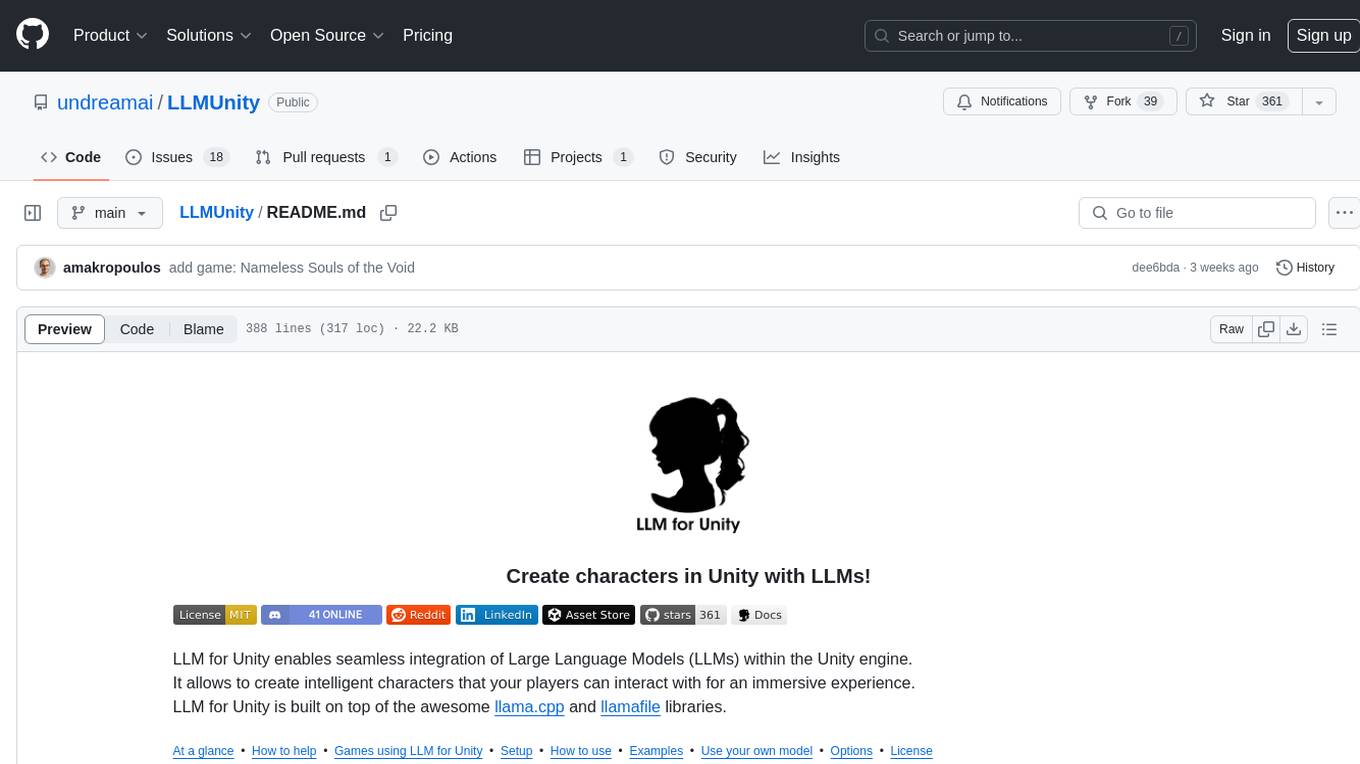
LLMUnity
LLM for Unity enables seamless integration of Large Language Models (LLMs) within the Unity engine, allowing users to create intelligent characters for immersive player interactions. The tool supports major LLM models, runs locally without internet access, offers fast inference on CPU and GPU, and is easy to set up with a single line of code. It is free for both personal and commercial use, tested on Unity 2021 LTS, 2022 LTS, and 2023. Users can build multiple AI characters efficiently, use remote servers for processing, and customize model settings for text generation.
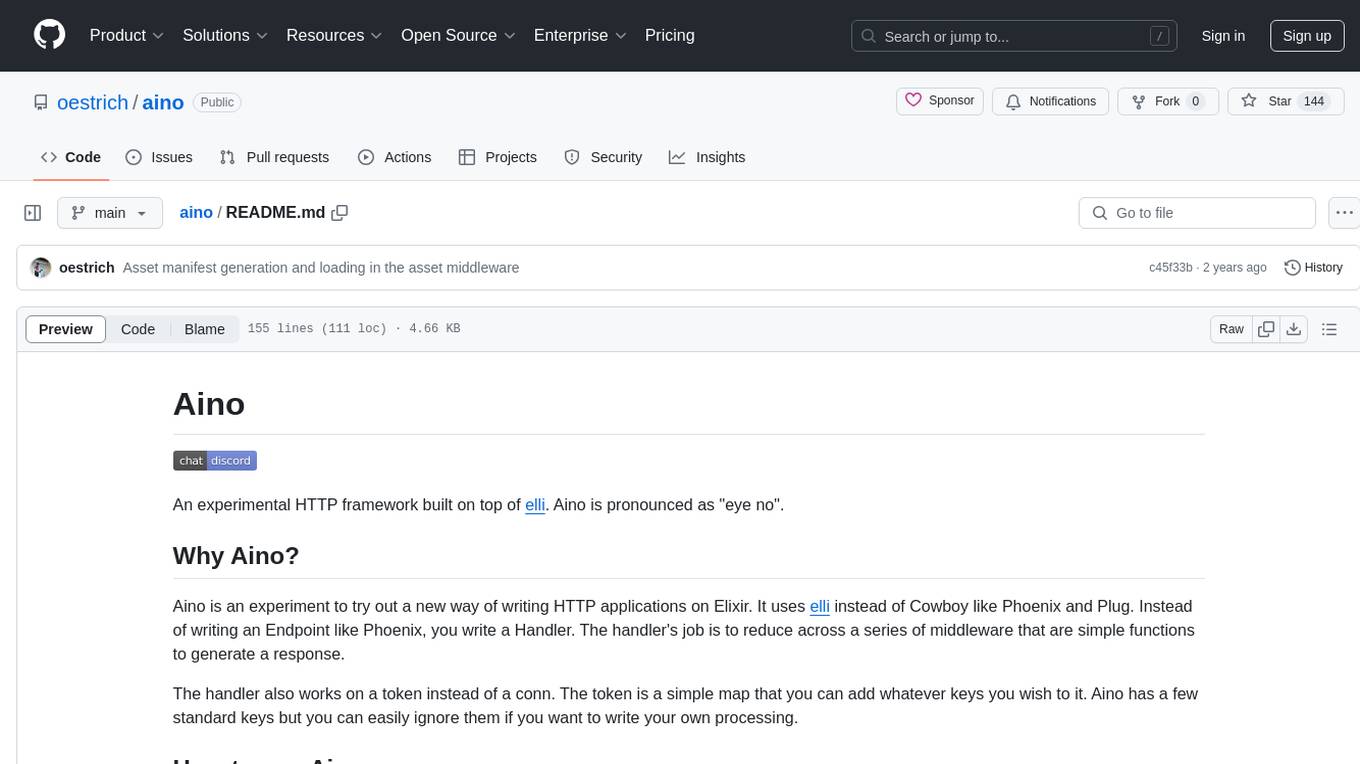
aino
Aino is an experimental HTTP framework for Elixir that uses elli instead of Cowboy like Phoenix and Plug. It focuses on writing handlers to process requests through middleware functions. Aino works on a token instead of a conn, allowing flexibility in adding custom keys. It includes built-in middleware for common tasks and a routing layer for defining routes. Handlers in Aino must return a token with specific keys for response rendering.
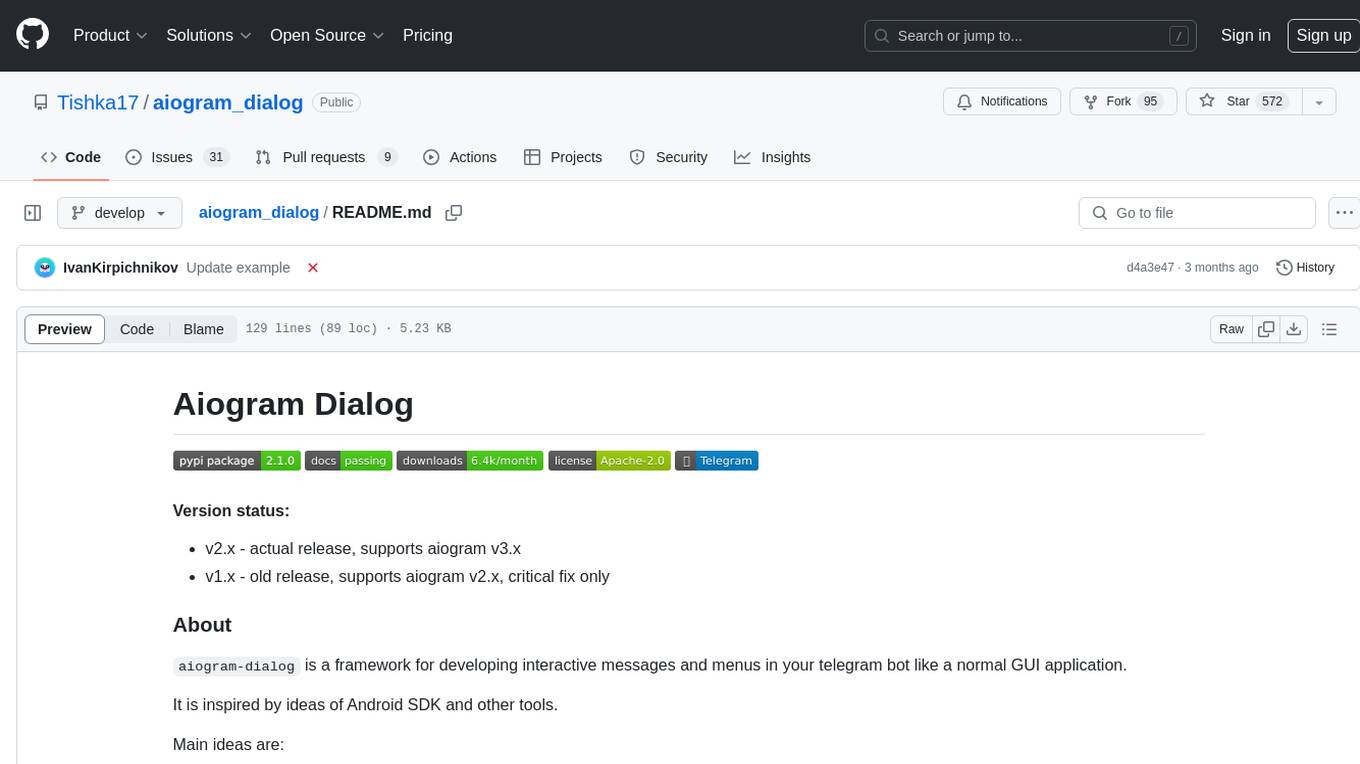
aiogram_dialog
Aiogram Dialog is a framework for developing interactive messages and menus in Telegram bots, inspired by Android SDK. It allows splitting data retrieval, rendering, and action processing, creating reusable widgets, and designing bots with a focus on user experience. The tool supports rich text rendering, automatic message updating, multiple dialog stacks, inline keyboard widgets, stateful widgets, various button layouts, media handling, transitions between windows, and offline HTML-preview for messages and transitions diagram.
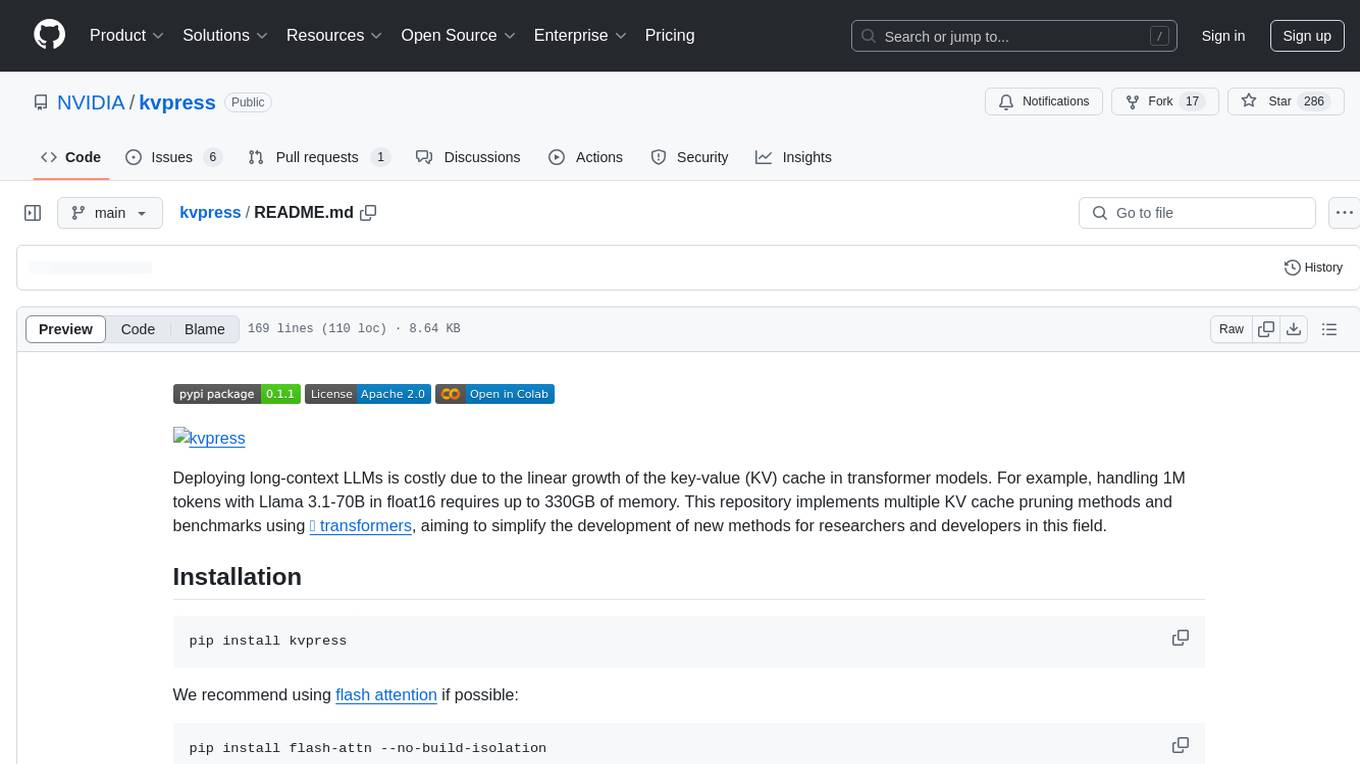
kvpress
This repository implements multiple key-value cache pruning methods and benchmarks using transformers, aiming to simplify the development of new methods for researchers and developers in the field of long-context language models. It provides a set of 'presses' that compress the cache during the pre-filling phase, with each press having a compression ratio attribute. The repository includes various training-free presses, special presses, and supports KV cache quantization. Users can contribute new presses and evaluate the performance of different presses on long-context datasets.
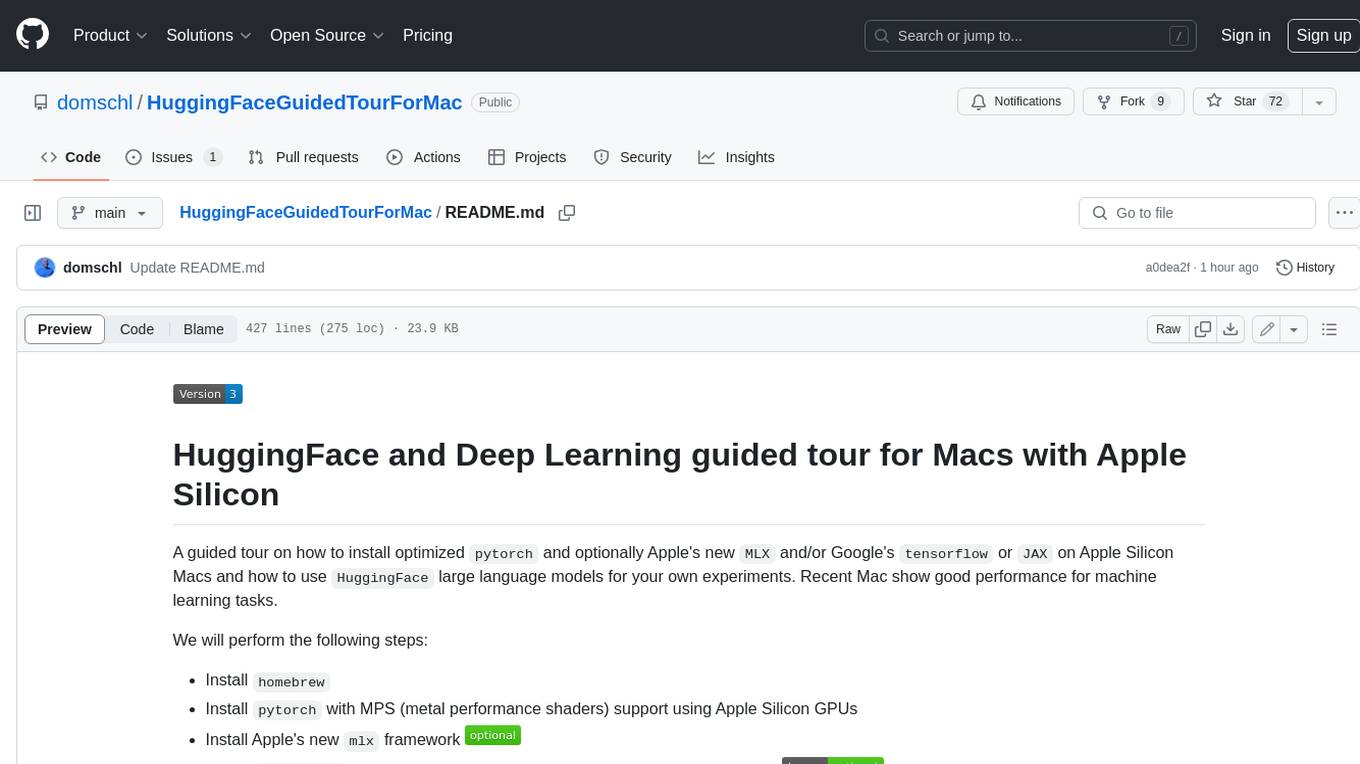
HuggingFaceGuidedTourForMac
HuggingFaceGuidedTourForMac is a guided tour on how to install optimized pytorch and optionally Apple's new MLX, JAX, and TensorFlow on Apple Silicon Macs. The repository provides steps to install homebrew, pytorch with MPS support, MLX, JAX, TensorFlow, and Jupyter lab. It also includes instructions on running large language models using HuggingFace transformers. The repository aims to help users set up their Macs for deep learning experiments with optimized performance.
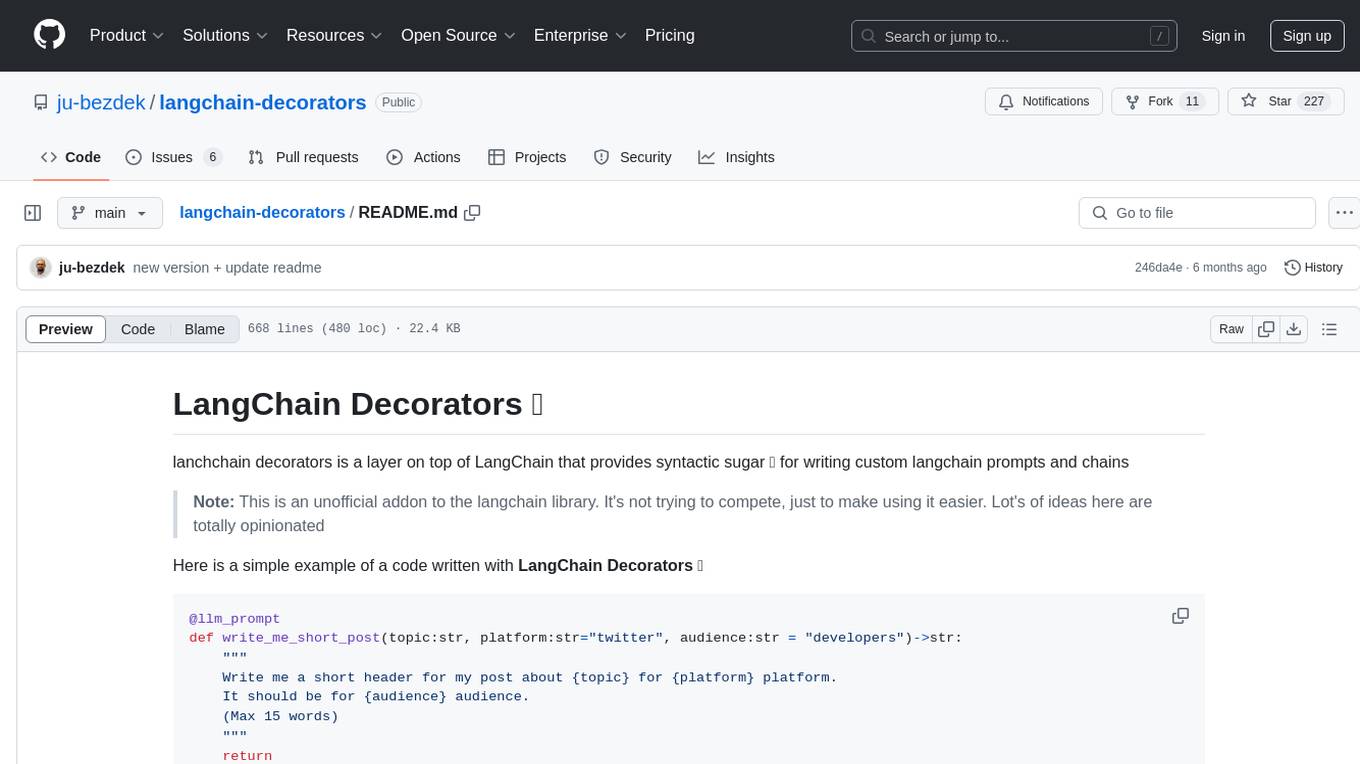
langchain-decorators
LangChain Decorators is a layer on top of LangChain that provides syntactic sugar for writing custom langchain prompts and chains. It offers a more pythonic way of writing code, multiline prompts without breaking code flow, IDE support for hinting and type checking, leveraging LangChain ecosystem, support for optional parameters, and sharing parameters between prompts. It simplifies streaming, automatic LLM selection, defining custom settings, debugging, and passing memory, callback, stop, etc. It also provides functions provider, dynamic function schemas, binding prompts to objects, defining custom settings, and debugging options. The project aims to enhance the LangChain library by making it easier to use and more efficient for writing custom prompts and chains.
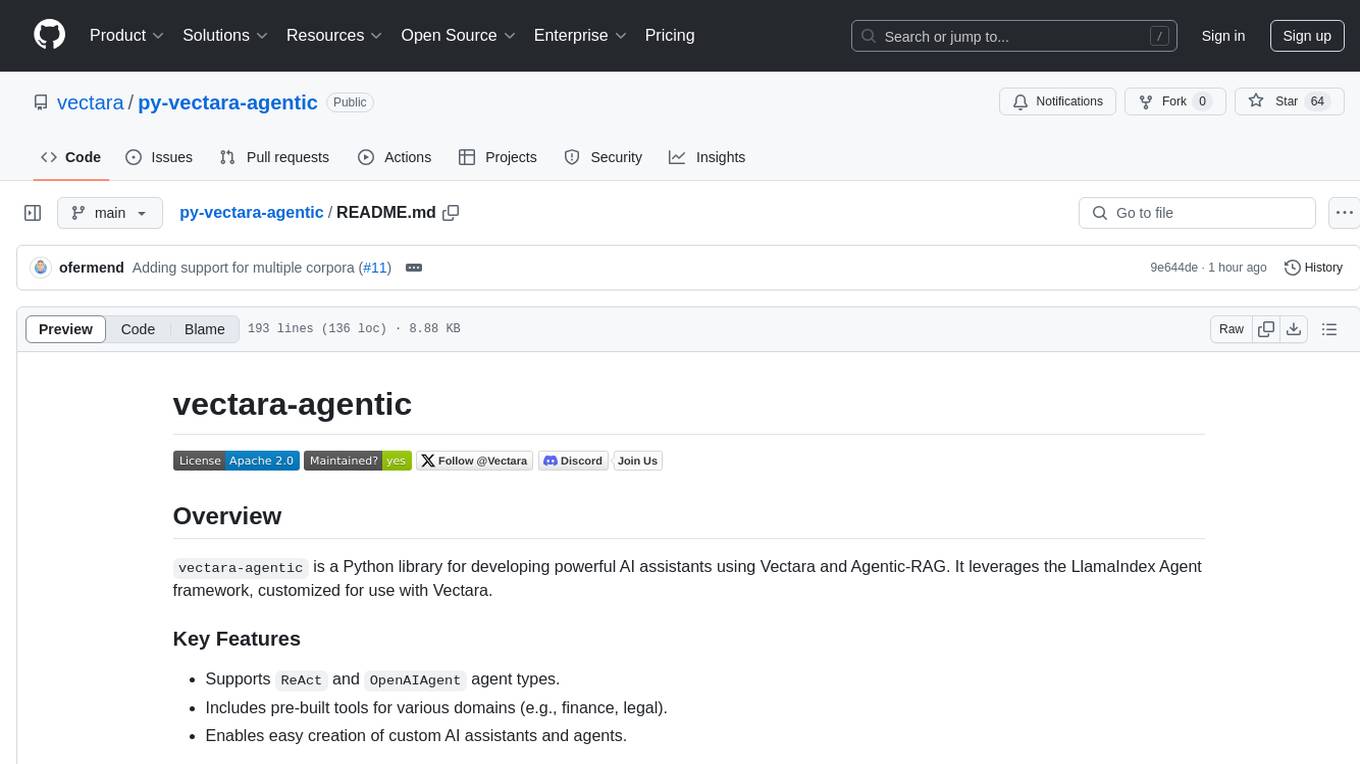
py-vectara-agentic
The `vectara-agentic` Python library is designed for developing powerful AI assistants using Vectara and Agentic-RAG. It supports various agent types, includes pre-built tools for domains like finance and legal, and enables easy creation of custom AI assistants and agents. The library provides tools for summarizing text, rephrasing text, legal tasks like summarizing legal text and critiquing as a judge, financial tasks like analyzing balance sheets and income statements, and database tools for inspecting and querying databases. It also supports observability via LlamaIndex and Arize Phoenix integration.
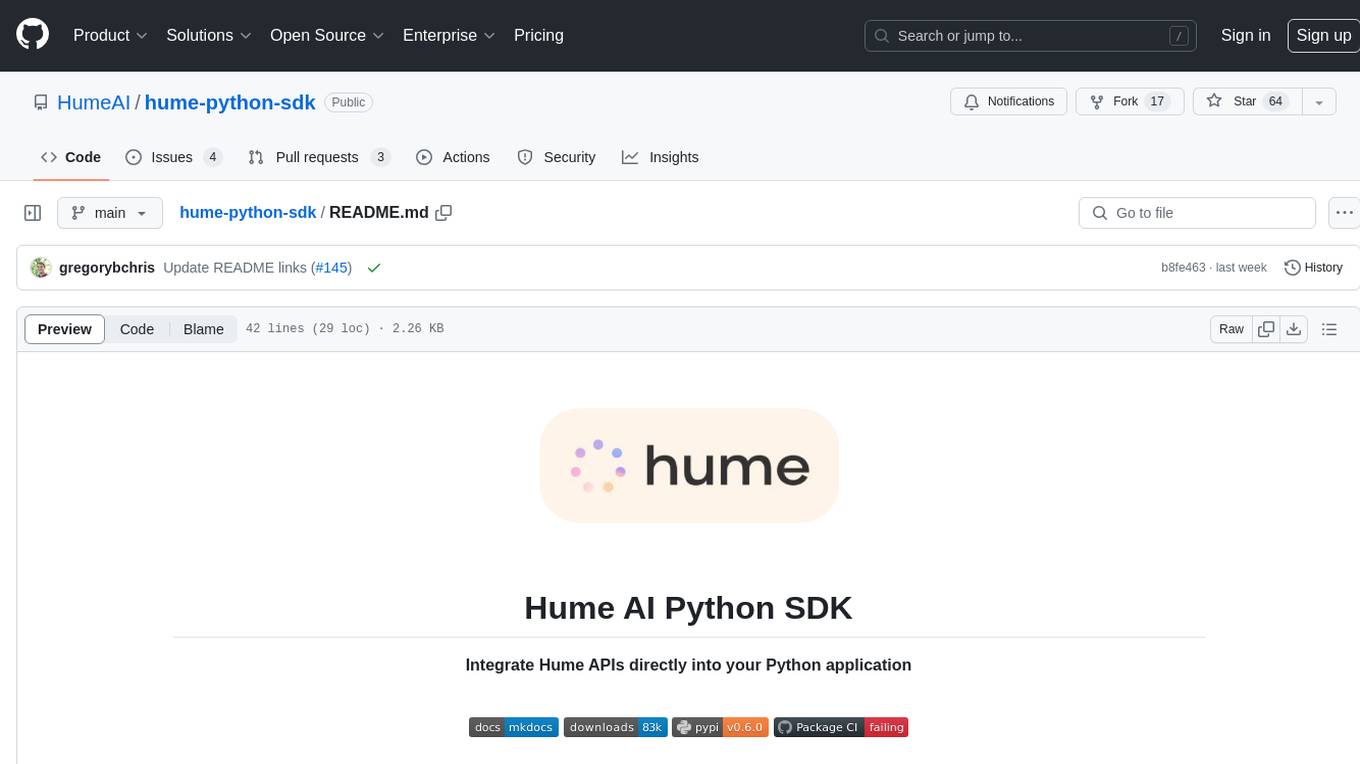
hume-python-sdk
The Hume AI Python SDK allows users to integrate Hume APIs directly into their Python applications. Users can access complete documentation, quickstart guides, and example notebooks to get started. The SDK is designed to provide support for Hume's expressive communication platform built on scientific research. Users are encouraged to create an account at beta.hume.ai and stay updated on changes through Discord. The SDK may undergo breaking changes to improve tooling and ensure reliable releases in the future.
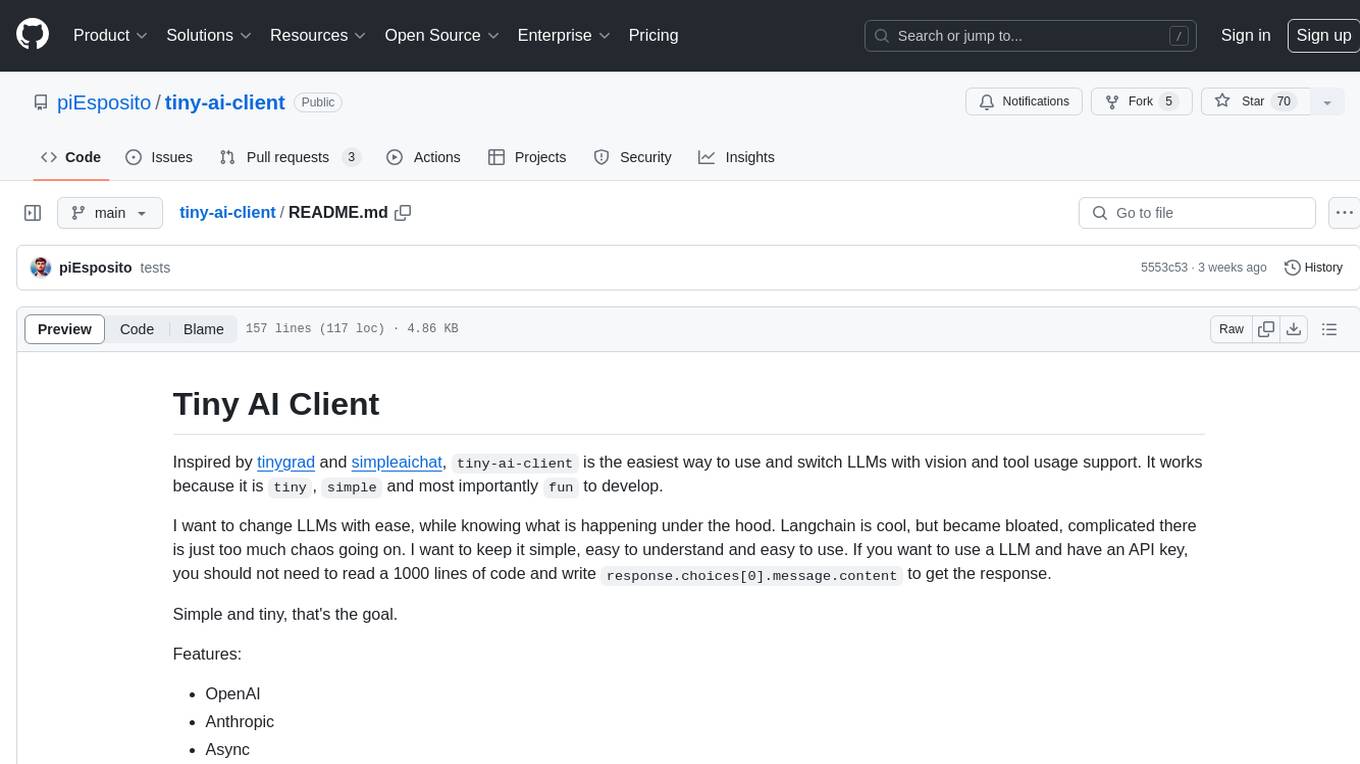
tiny-ai-client
Tiny AI Client is a lightweight tool designed for easy usage and switching of Language Model Models (LLMs) with support for vision and tool usage. It aims to provide a simple and intuitive interface for interacting with various LLMs, allowing users to easily set, change models, send messages, use tools, and handle vision tasks. The core logic of the tool is kept minimal and easy to understand, with separate modules for vision and tool usage utilities. Users can interact with the tool through simple Python scripts, passing model names, messages, tools, and images as required.
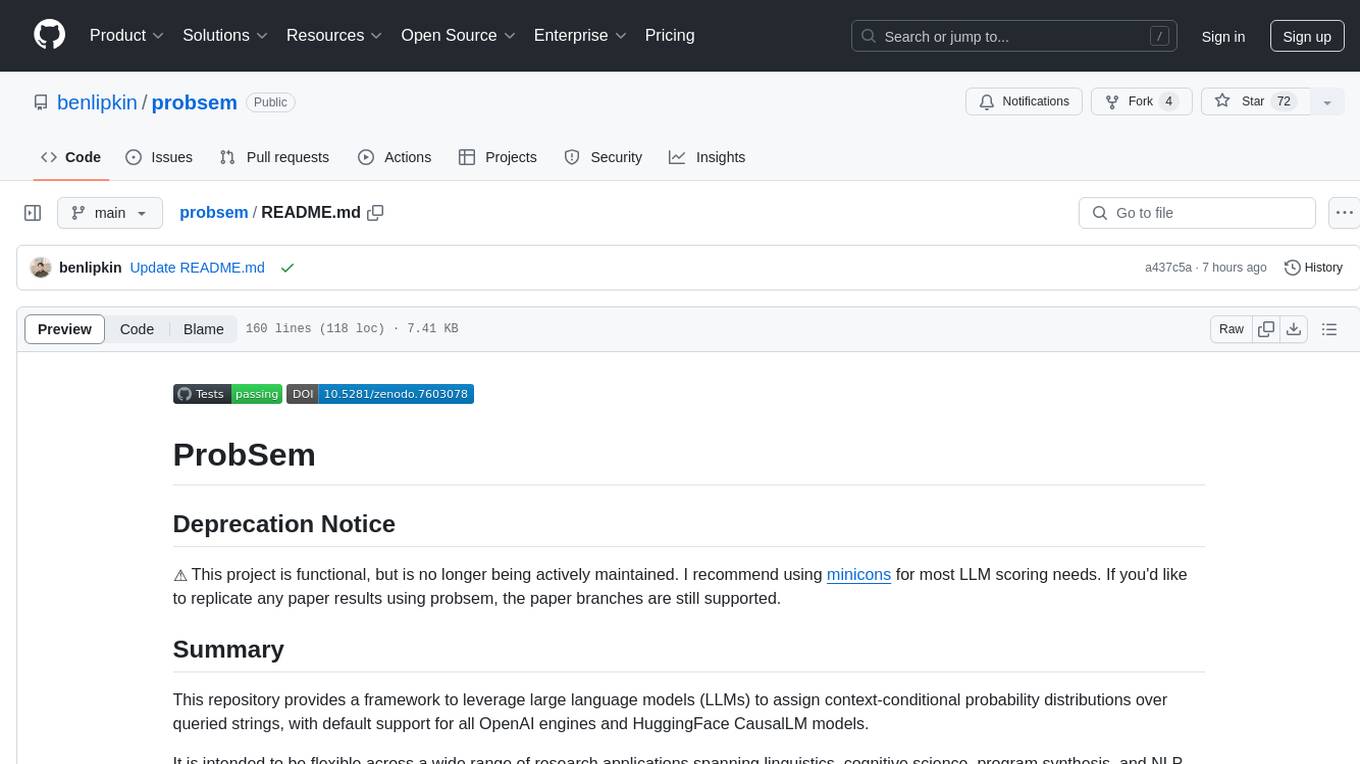
probsem
ProbSem is a repository that provides a framework to leverage large language models (LLMs) for assigning context-conditional probability distributions over queried strings. It supports OpenAI engines and HuggingFace CausalLM models, and is flexible for research applications in linguistics, cognitive science, program synthesis, and NLP. Users can define prompts, contexts, and queries to derive probability distributions over possible completions, enabling tasks like cloze completion, multiple-choice QA, semantic parsing, and code completion. The repository offers CLI and API interfaces for evaluation, with options to customize models, normalize scores, and adjust temperature for probability distributions.
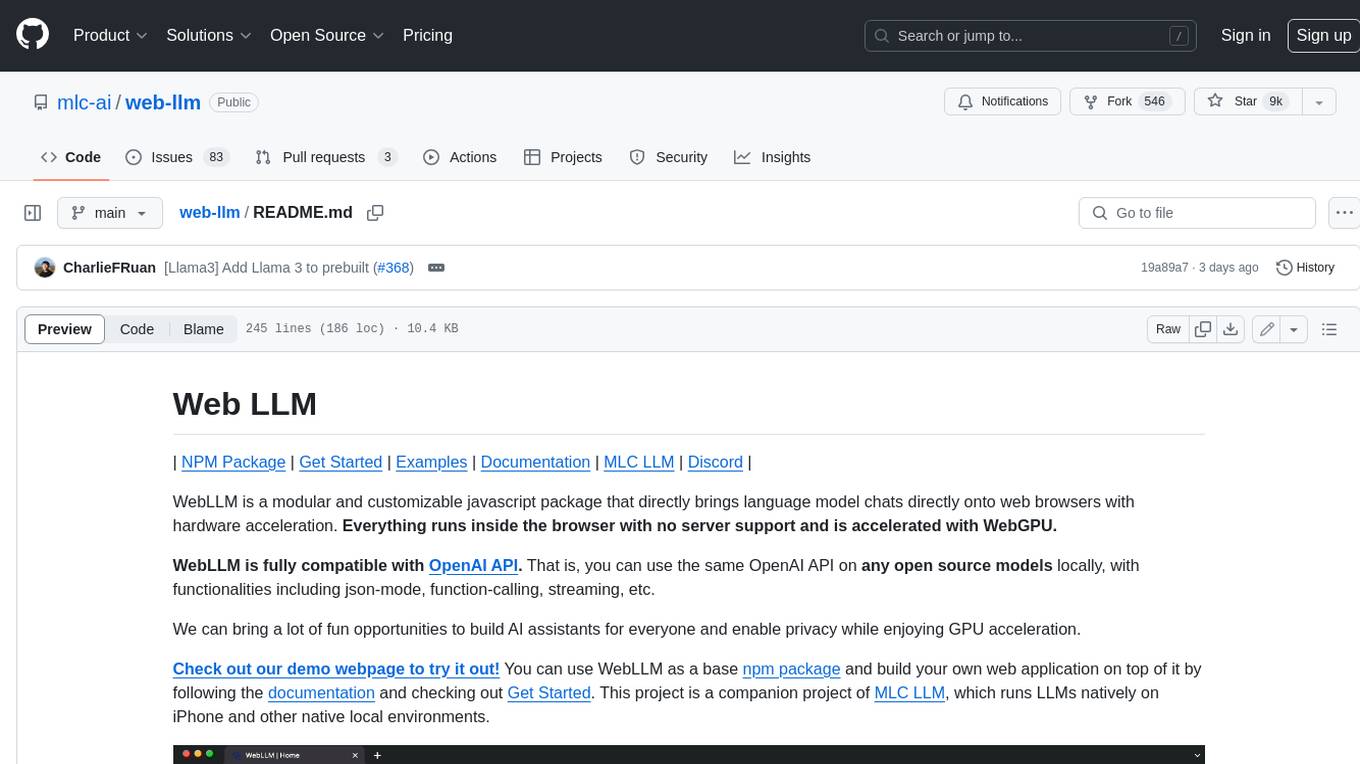
web-llm
WebLLM is a modular and customizable javascript package that directly brings language model chats directly onto web browsers with hardware acceleration. Everything runs inside the browser with no server support and is accelerated with WebGPU. WebLLM is fully compatible with OpenAI API. That is, you can use the same OpenAI API on any open source models locally, with functionalities including json-mode, function-calling, streaming, etc. We can bring a lot of fun opportunities to build AI assistants for everyone and enable privacy while enjoying GPU acceleration.
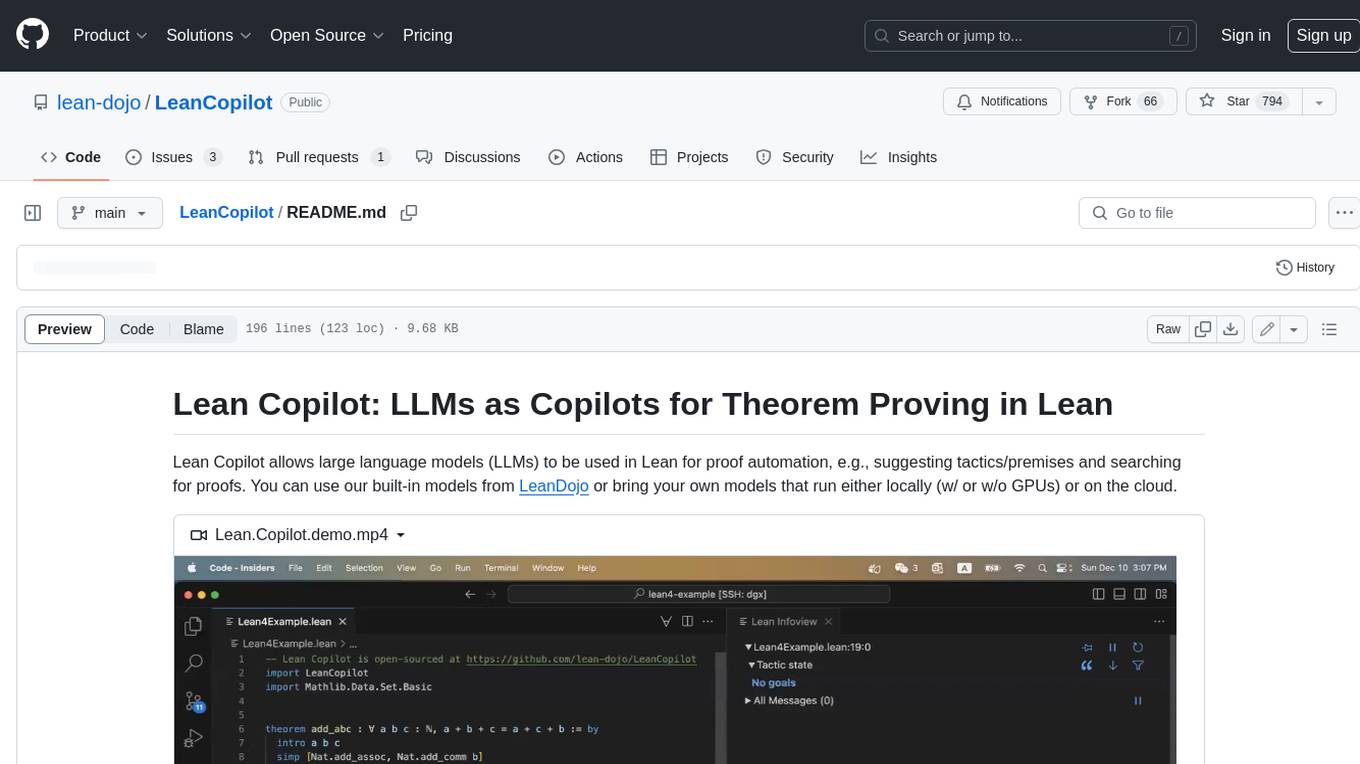
LeanCopilot
Lean Copilot is a tool that enables the use of large language models (LLMs) in Lean for proof automation. It provides features such as suggesting tactics/premises, searching for proofs, and running inference of LLMs. Users can utilize built-in models from LeanDojo or bring their own models to run locally or on the cloud. The tool supports platforms like Linux, macOS, and Windows WSL, with optional CUDA and cuDNN for GPU acceleration. Advanced users can customize behavior using Tactic APIs and Model APIs. Lean Copilot also allows users to bring their own models through ExternalGenerator or ExternalEncoder. The tool comes with caveats such as occasional crashes and issues with premise selection and proof search. Users can get in touch through GitHub Discussions for questions, bug reports, feature requests, and suggestions. The tool is designed to enhance theorem proving in Lean using LLMs.
For similar tasks
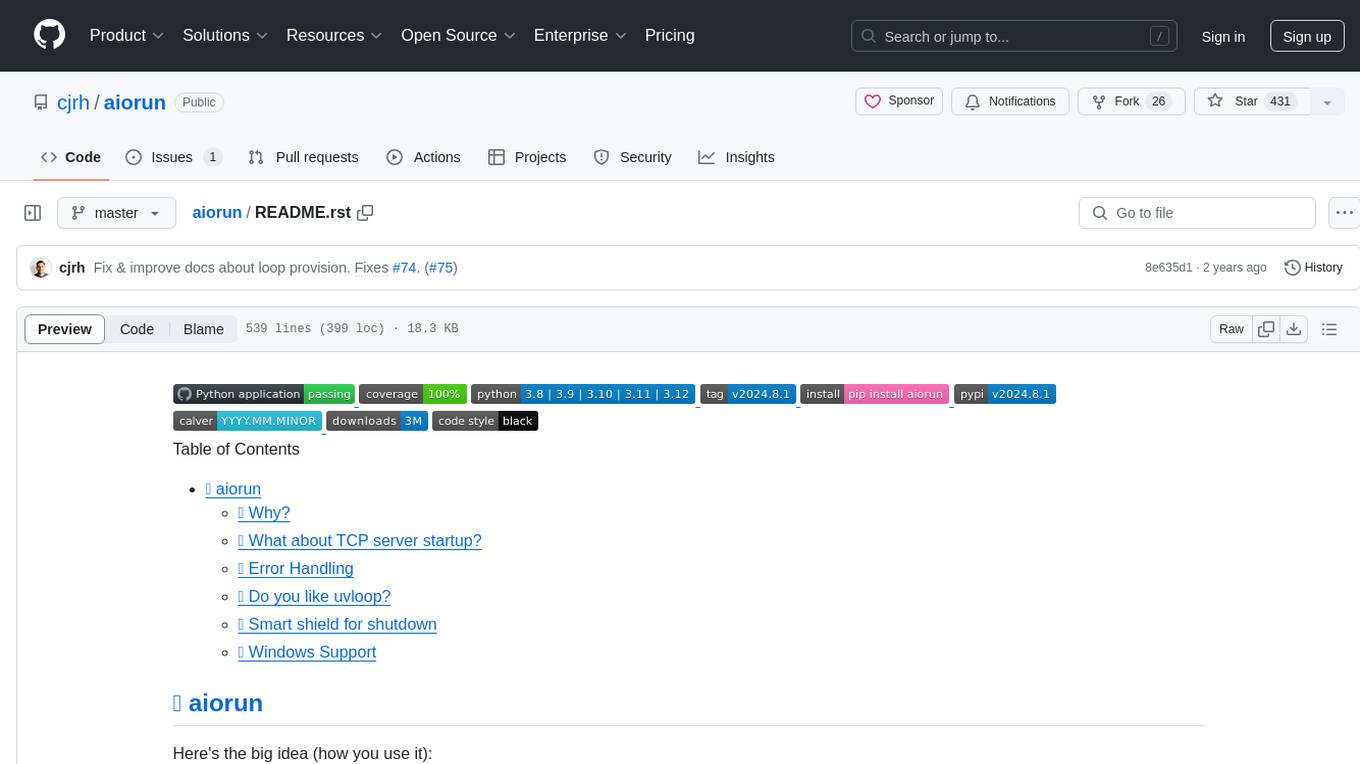
aiorun
aiorun is a Python package that provides a `run()` function as the starting point of your `asyncio`-based application. The `run()` function handles everything needed during the shutdown sequence of the application, such as creating a `Task` for the given coroutine, running the event loop, adding signal handlers for `SIGINT` and `SIGTERM`, cancelling tasks, waiting for the executor to complete shutdown, and closing the loop. It automates standard actions for asyncio apps, eliminating the need to write boilerplate code. The package also offers error handling options and tools for specific scenarios like TCP server startup and smart shield for shutdown.
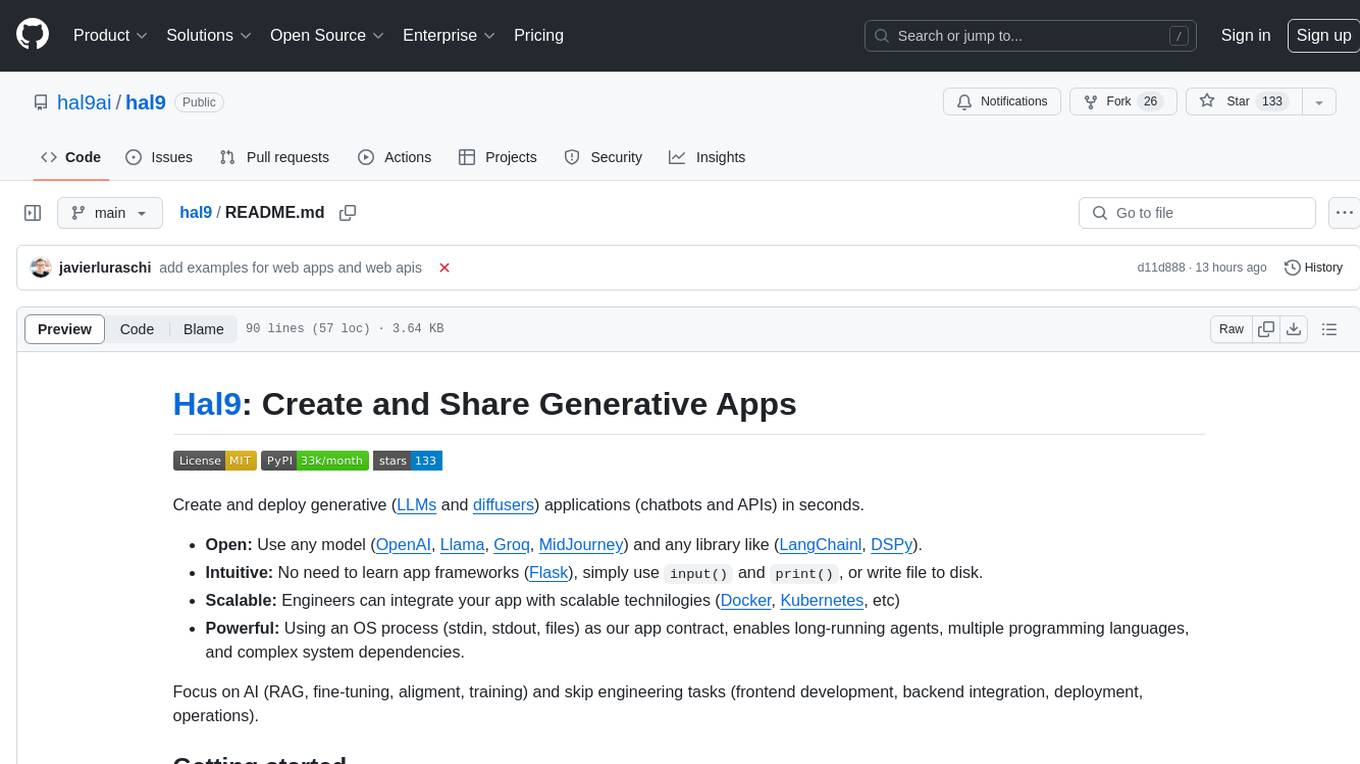
hal9
Hal9 is a tool that allows users to create and deploy generative applications such as chatbots and APIs quickly. It is open, intuitive, scalable, and powerful, enabling users to use various models and libraries without the need to learn complex app frameworks. With a focus on AI tasks like RAG, fine-tuning, alignment, and training, Hal9 simplifies the development process by skipping engineering tasks like frontend development, backend integration, deployment, and operations.
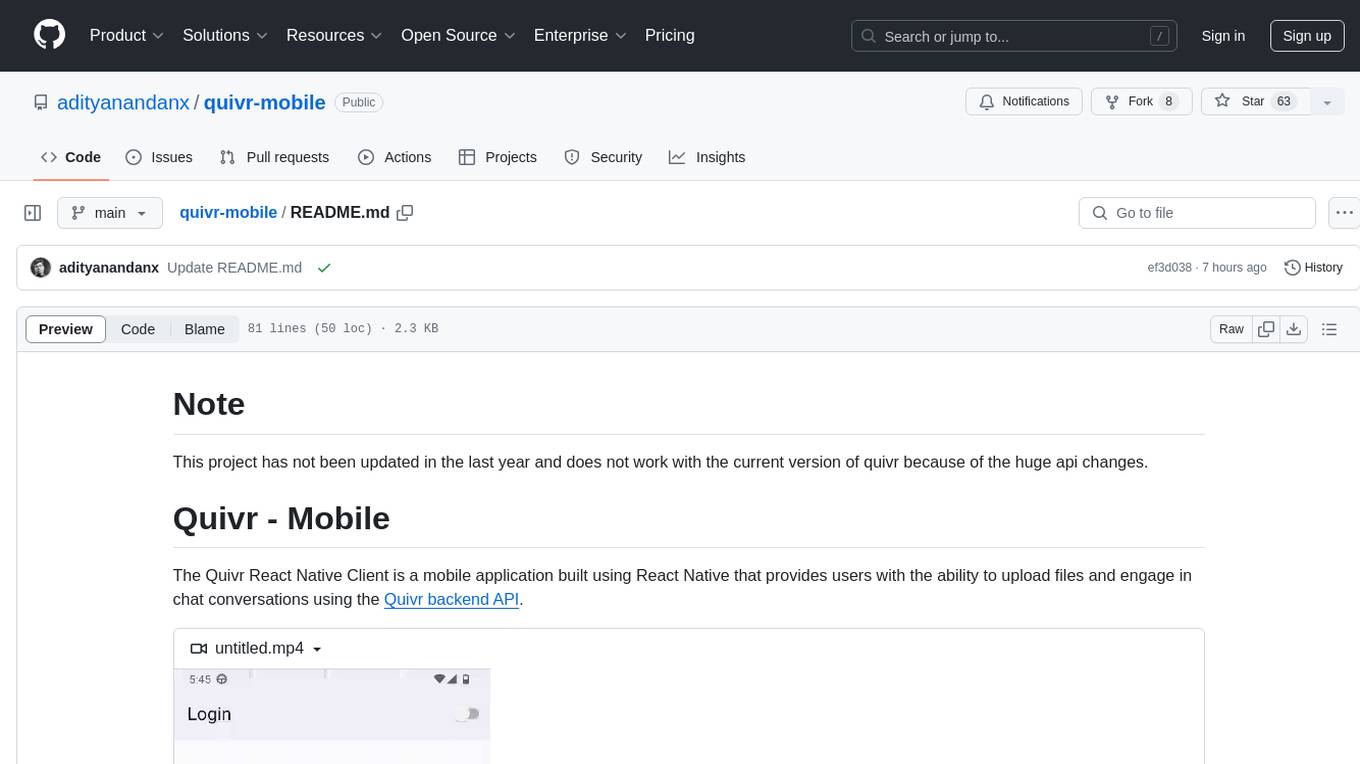
quivr-mobile
Quivr-Mobile is a React Native mobile application that allows users to upload files and engage in chat conversations using the Quivr backend API. It supports features like file upload and chatting with a language model about uploaded data. The project uses technologies like React Native, React Native Paper, and React Native Navigation. Users can follow the installation steps to set up the client and contribute to the project by opening issues or submitting pull requests following the existing coding style.
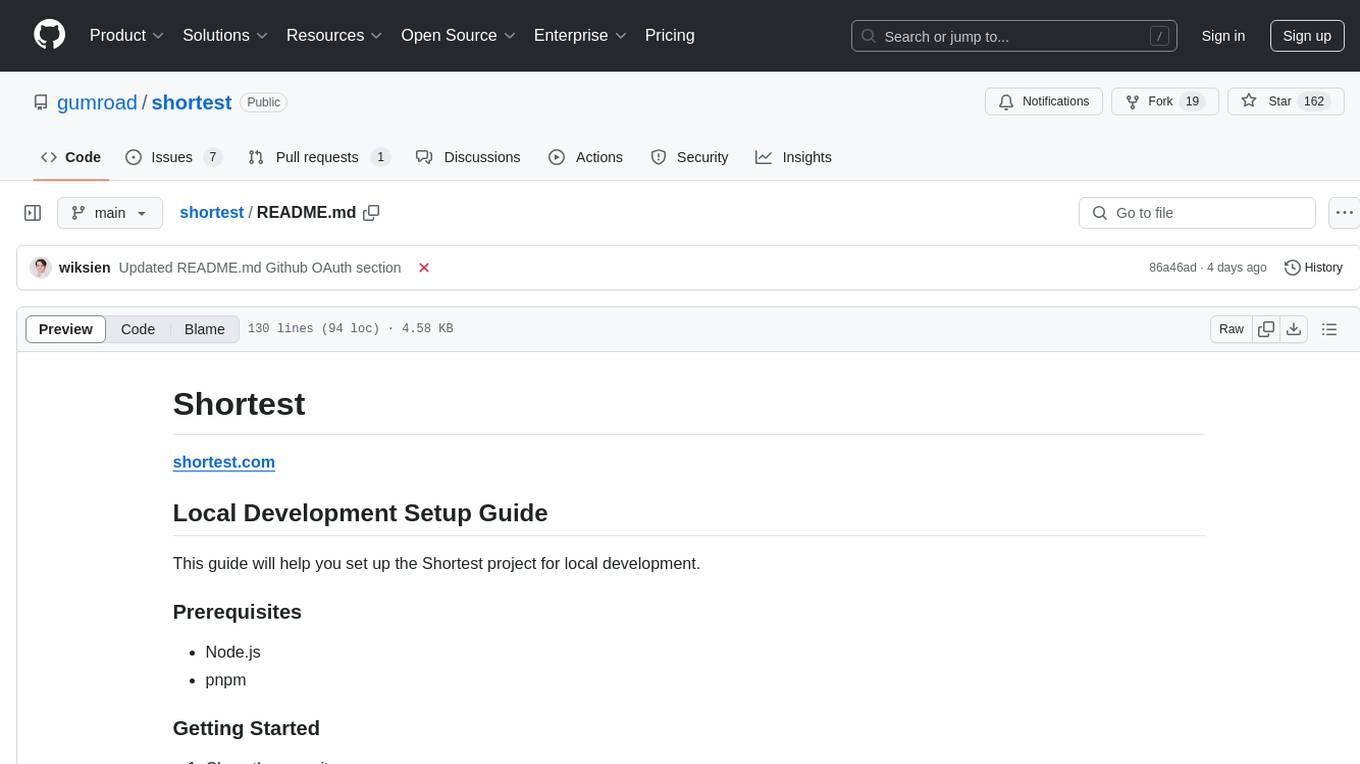
shortest
Shortest is a project for local development that helps set up environment variables and services for a web application. It provides a guide for setting up Node.js and pnpm dependencies, configuring services like Clerk, Vercel Postgres, Anthropic, Stripe, and GitHub OAuth, and running the application and tests locally.
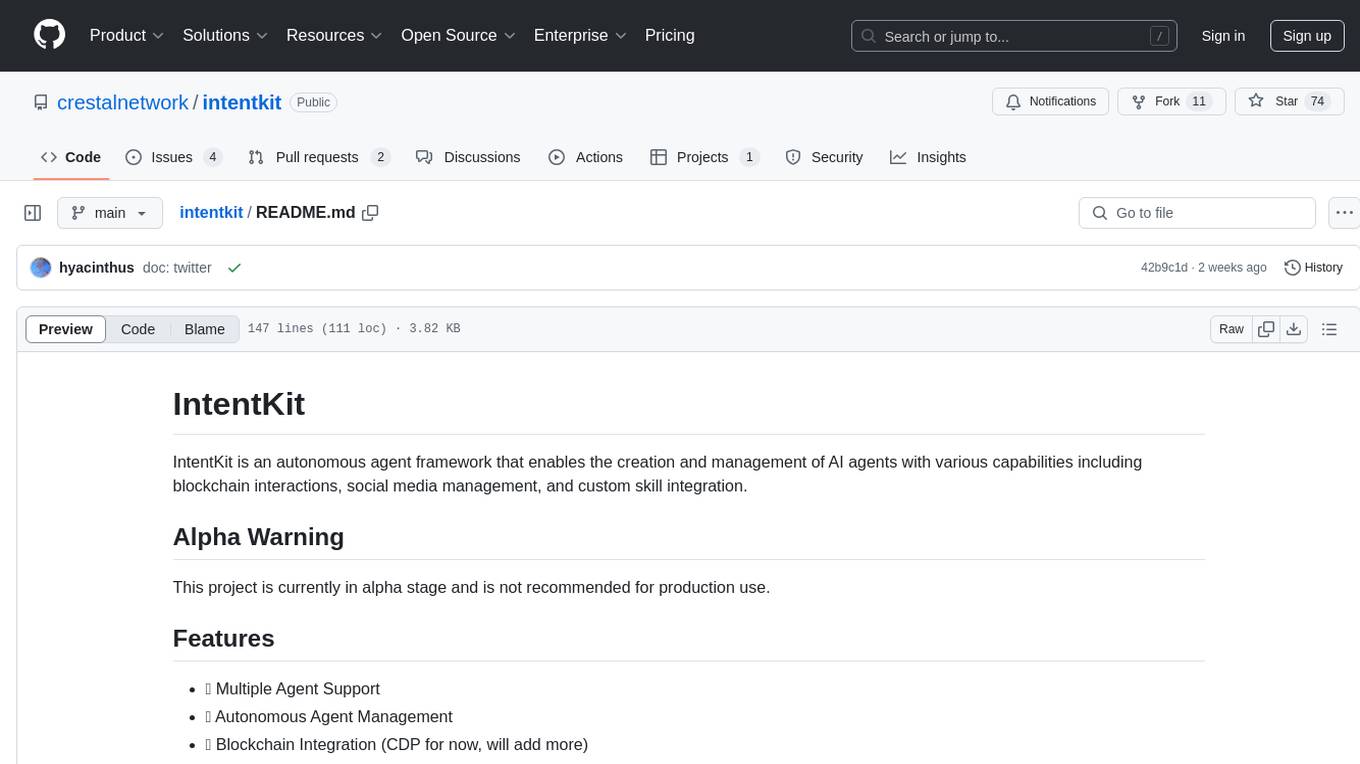
intentkit
IntentKit is an autonomous agent framework that enables the creation and management of AI agents with capabilities including blockchain interactions, social media management, and custom skill integration. It supports multiple agents, autonomous agent management, blockchain integration, social media integration, extensible skill system, and plugin system. The project is in alpha stage and not recommended for production use. It provides quick start guides for Docker and local development, integrations with Twitter and Coinbase, configuration options using environment variables or AWS Secrets Manager, project structure with core application code, entry points, configuration management, database models, skills, skill sets, and utility functions. Developers can add new skills by creating, implementing, and registering them in the skill directory.
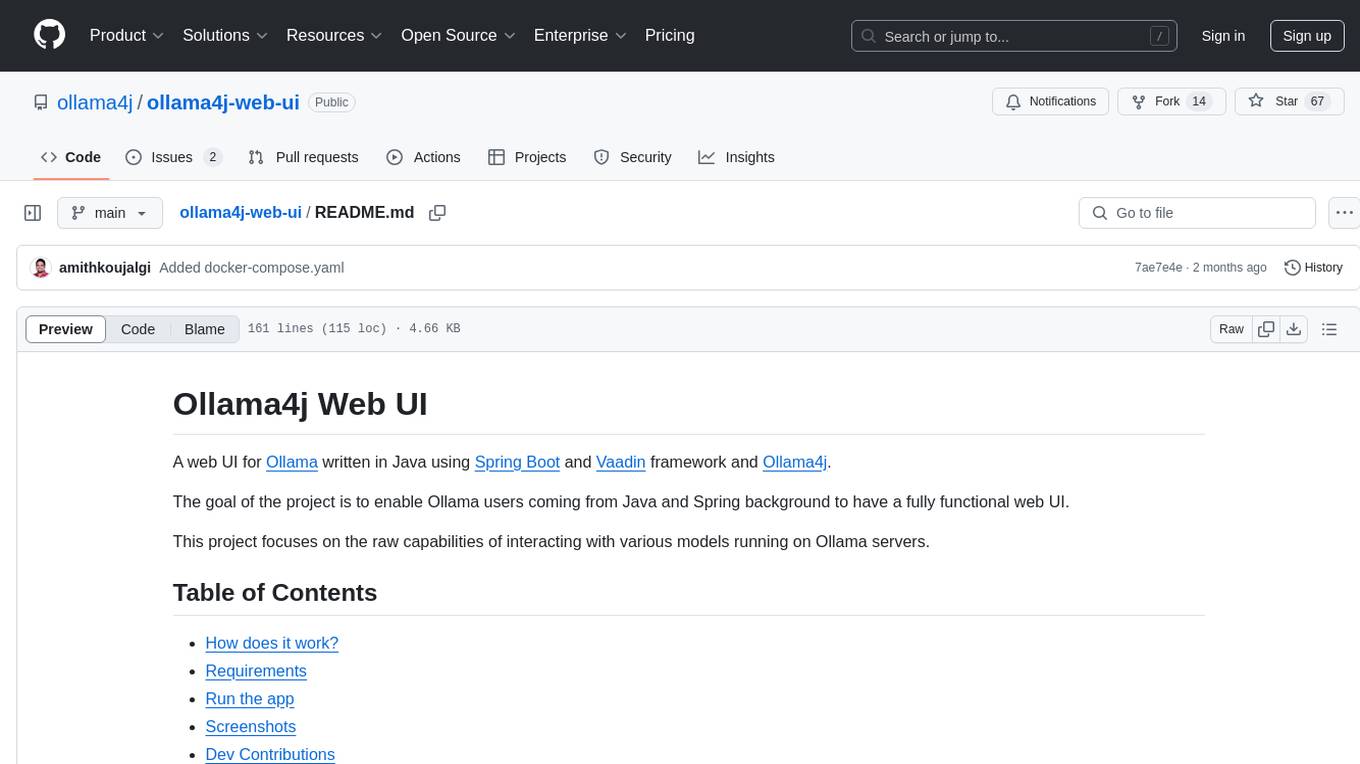
ollama4j-web-ui
Ollama4j Web UI is a Java-based web interface built using Spring Boot and Vaadin framework for Ollama users with Java and Spring background. It allows users to interact with various models running on Ollama servers, providing a fully functional web UI experience. The project offers multiple ways to run the application, including via Docker, Docker Compose, or as a standalone JAR. Users can configure the environment variables and access the web UI through a browser. The project also includes features for error handling on the UI and settings pane for customizing default parameters.
For similar jobs
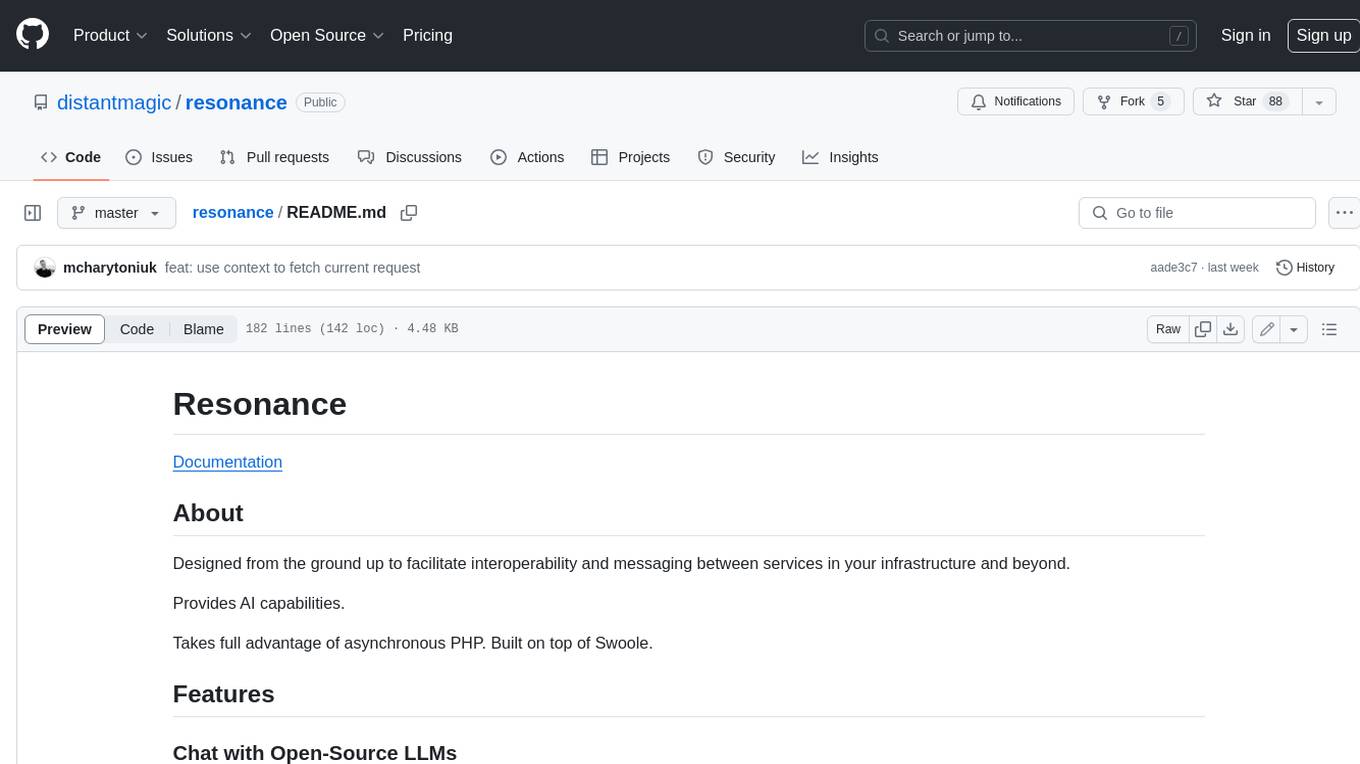
resonance
Resonance is a framework designed to facilitate interoperability and messaging between services in your infrastructure and beyond. It provides AI capabilities and takes full advantage of asynchronous PHP, built on top of Swoole. With Resonance, you can: * Chat with Open-Source LLMs: Create prompt controllers to directly answer user's prompts. LLM takes care of determining user's intention, so you can focus on taking appropriate action. * Asynchronous Where it Matters: Respond asynchronously to incoming RPC or WebSocket messages (or both combined) with little overhead. You can set up all the asynchronous features using attributes. No elaborate configuration is needed. * Simple Things Remain Simple: Writing HTTP controllers is similar to how it's done in the synchronous code. Controllers have new exciting features that take advantage of the asynchronous environment. * Consistency is Key: You can keep the same approach to writing software no matter the size of your project. There are no growing central configuration files or service dependencies registries. Every relation between code modules is local to those modules. * Promises in PHP: Resonance provides a partial implementation of Promise/A+ spec to handle various asynchronous tasks. * GraphQL Out of the Box: You can build elaborate GraphQL schemas by using just the PHP attributes. Resonance takes care of reusing SQL queries and optimizing the resources' usage. All fields can be resolved asynchronously.
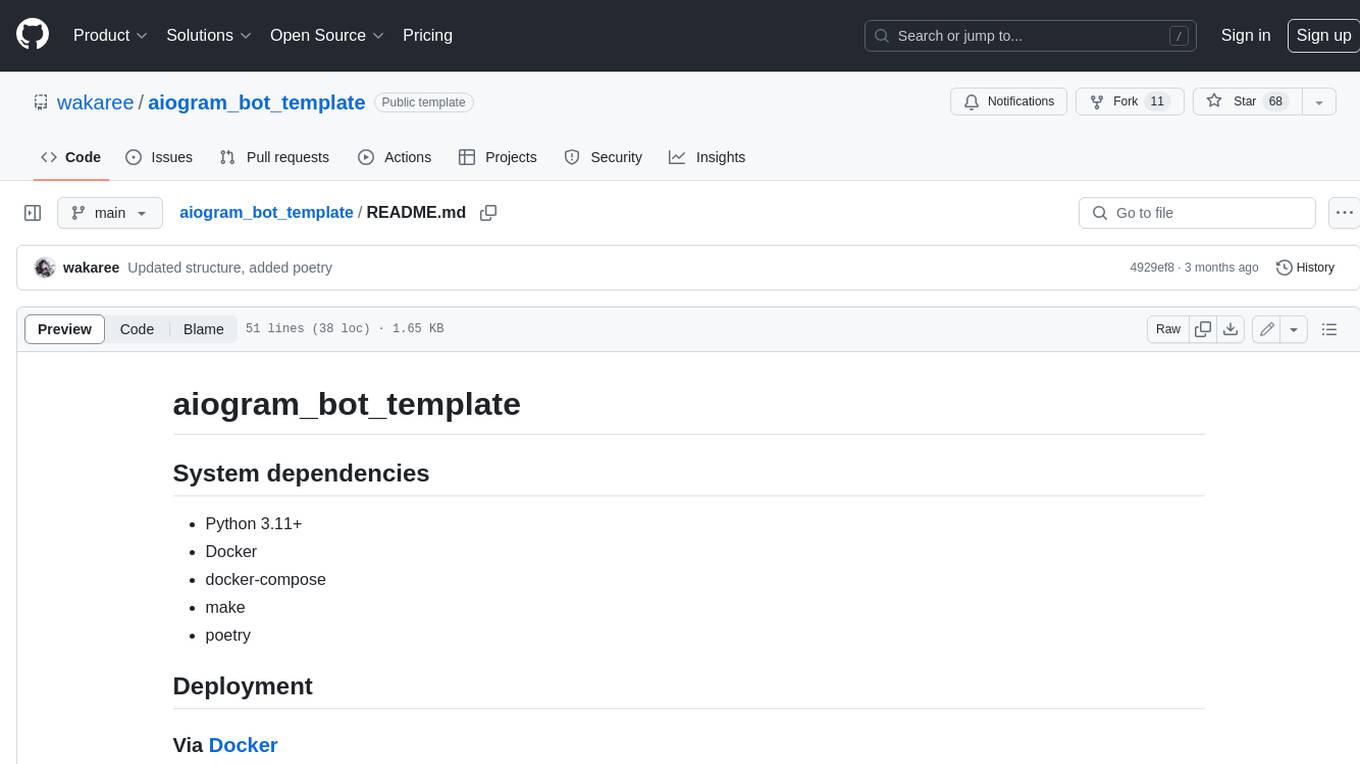
aiogram_bot_template
Aiogram bot template is a boilerplate for creating Telegram bots using Aiogram framework. It provides a solid foundation for building robust and scalable bots with a focus on code organization, database integration, and localization.
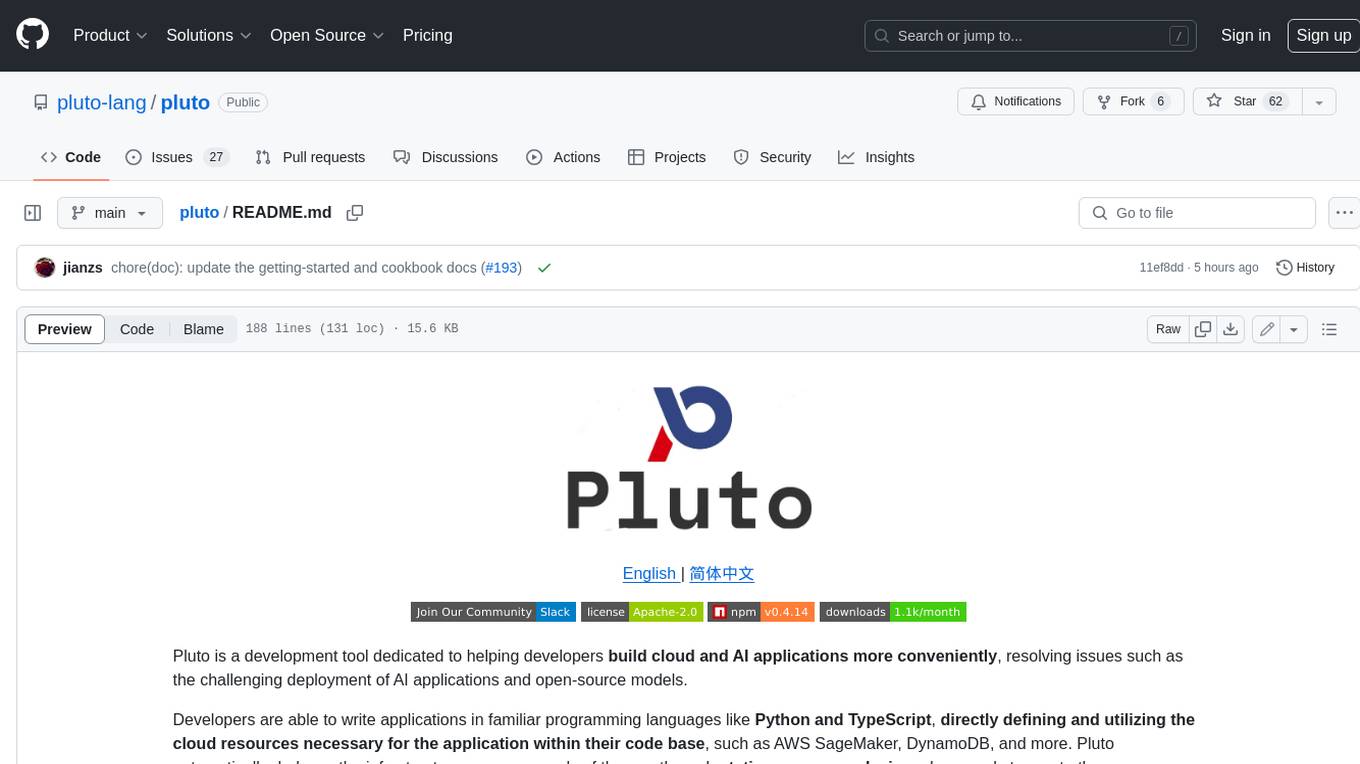
pluto
Pluto is a development tool dedicated to helping developers **build cloud and AI applications more conveniently** , resolving issues such as the challenging deployment of AI applications and open-source models. Developers are able to write applications in familiar programming languages like **Python and TypeScript** , **directly defining and utilizing the cloud resources necessary for the application within their code base** , such as AWS SageMaker, DynamoDB, and more. Pluto automatically deduces the infrastructure resource needs of the app through **static program analysis** and proceeds to create these resources on the specified cloud platform, **simplifying the resources creation and application deployment process**.
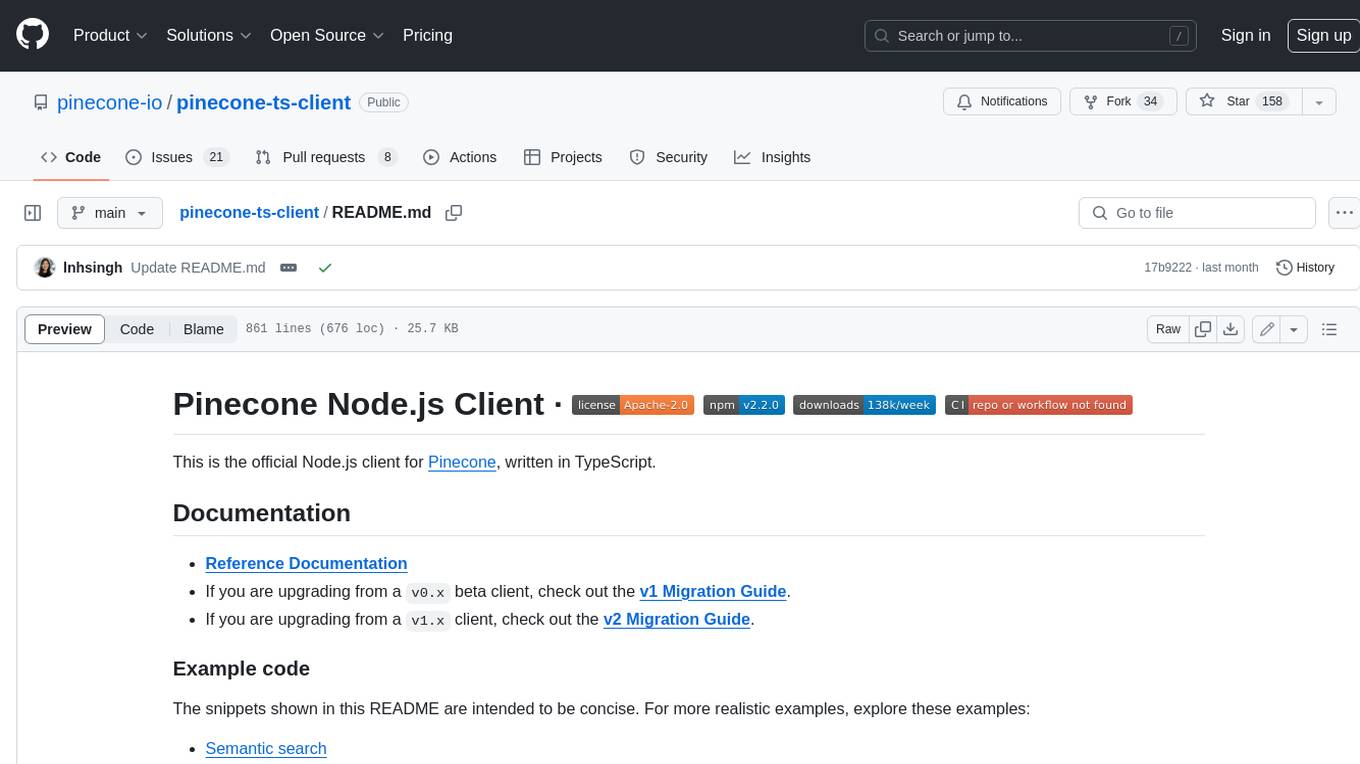
pinecone-ts-client
The official Node.js client for Pinecone, written in TypeScript. This client library provides a high-level interface for interacting with the Pinecone vector database service. With this client, you can create and manage indexes, upsert and query vector data, and perform other operations related to vector search and retrieval. The client is designed to be easy to use and provides a consistent and idiomatic experience for Node.js developers. It supports all the features and functionality of the Pinecone API, making it a comprehensive solution for building vector-powered applications in Node.js.
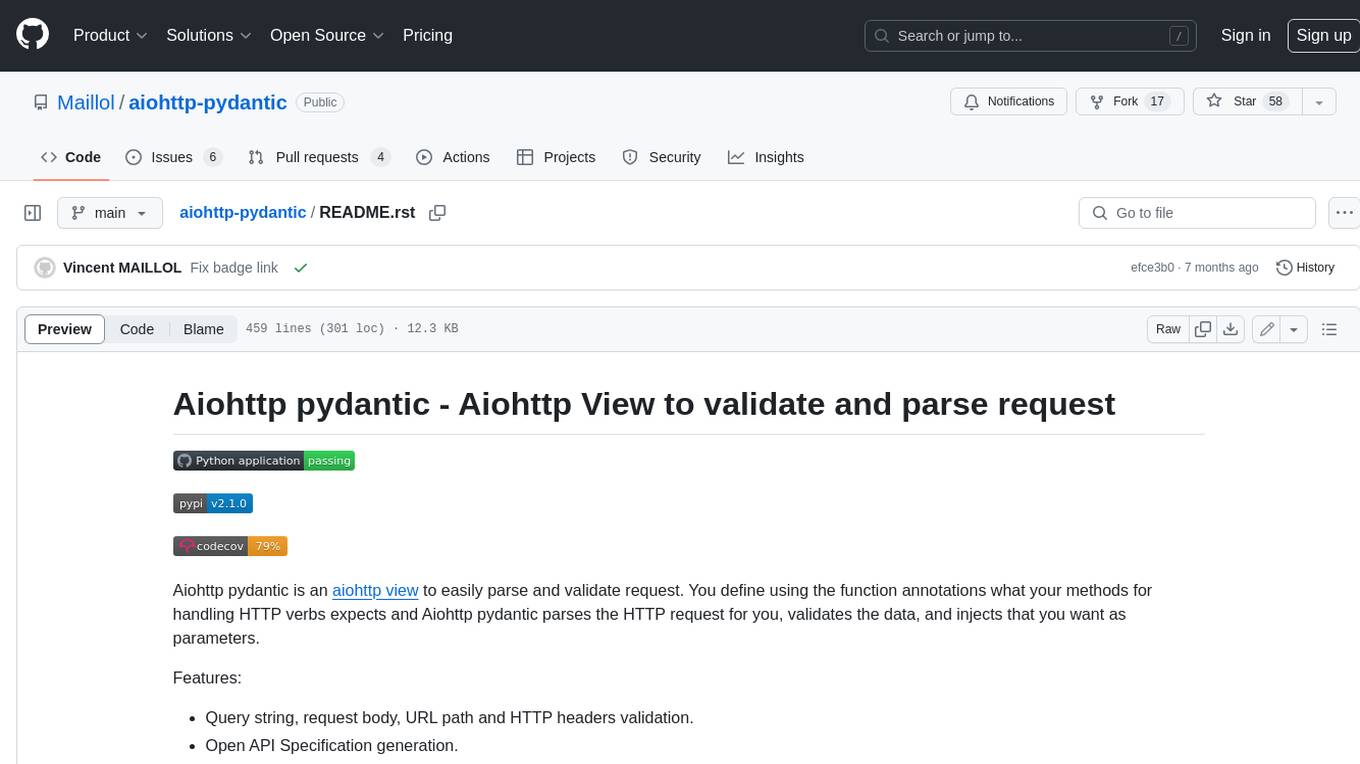
aiohttp-pydantic
Aiohttp pydantic is an aiohttp view to easily parse and validate requests. You define using function annotations what your methods for handling HTTP verbs expect, and Aiohttp pydantic parses the HTTP request for you, validates the data, and injects the parameters you want. It provides features like query string, request body, URL path, and HTTP headers validation, as well as Open API Specification generation.
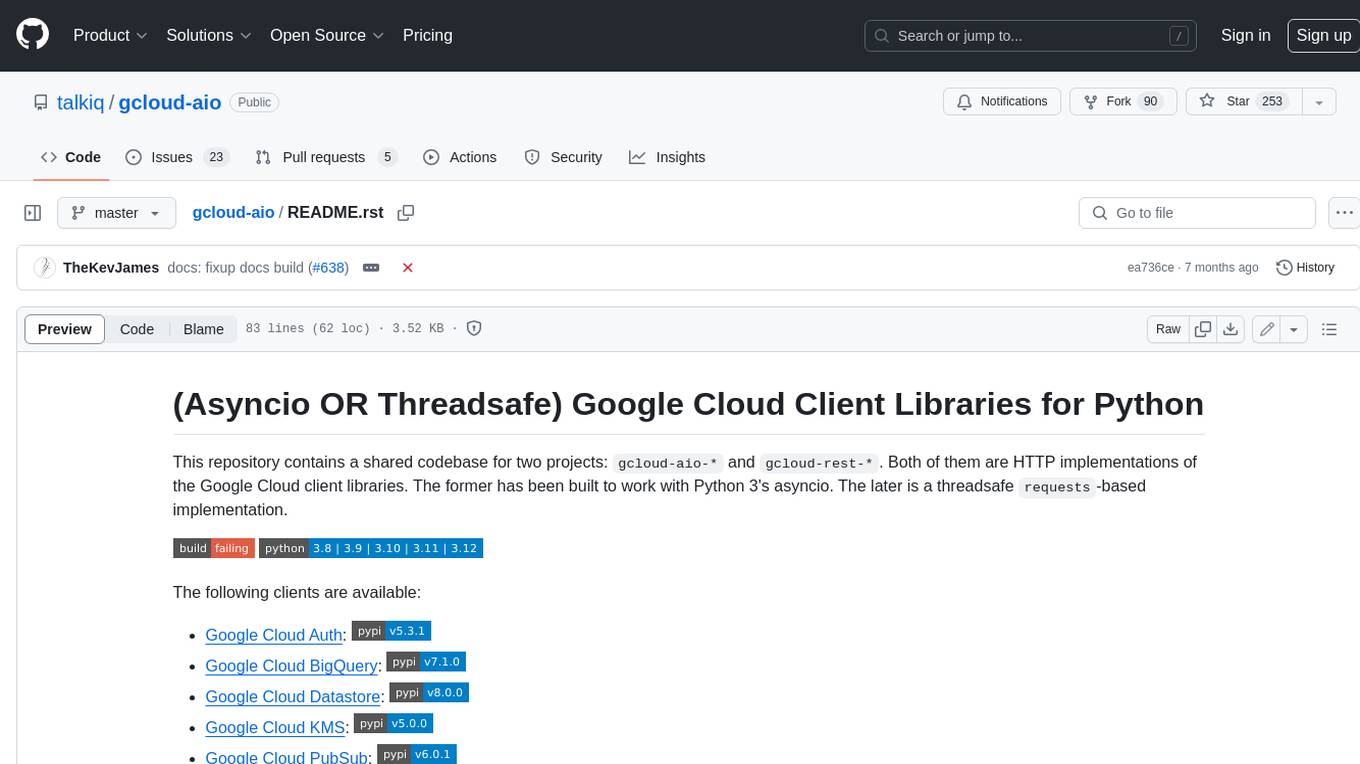
gcloud-aio
This repository contains shared codebase for two projects: gcloud-aio and gcloud-rest. gcloud-aio is built for Python 3's asyncio, while gcloud-rest is a threadsafe requests-based implementation. It provides clients for Google Cloud services like Auth, BigQuery, Datastore, KMS, PubSub, Storage, and Task Queue. Users can install the library using pip and refer to the documentation for usage details. Developers can contribute to the project by following the contribution guide.
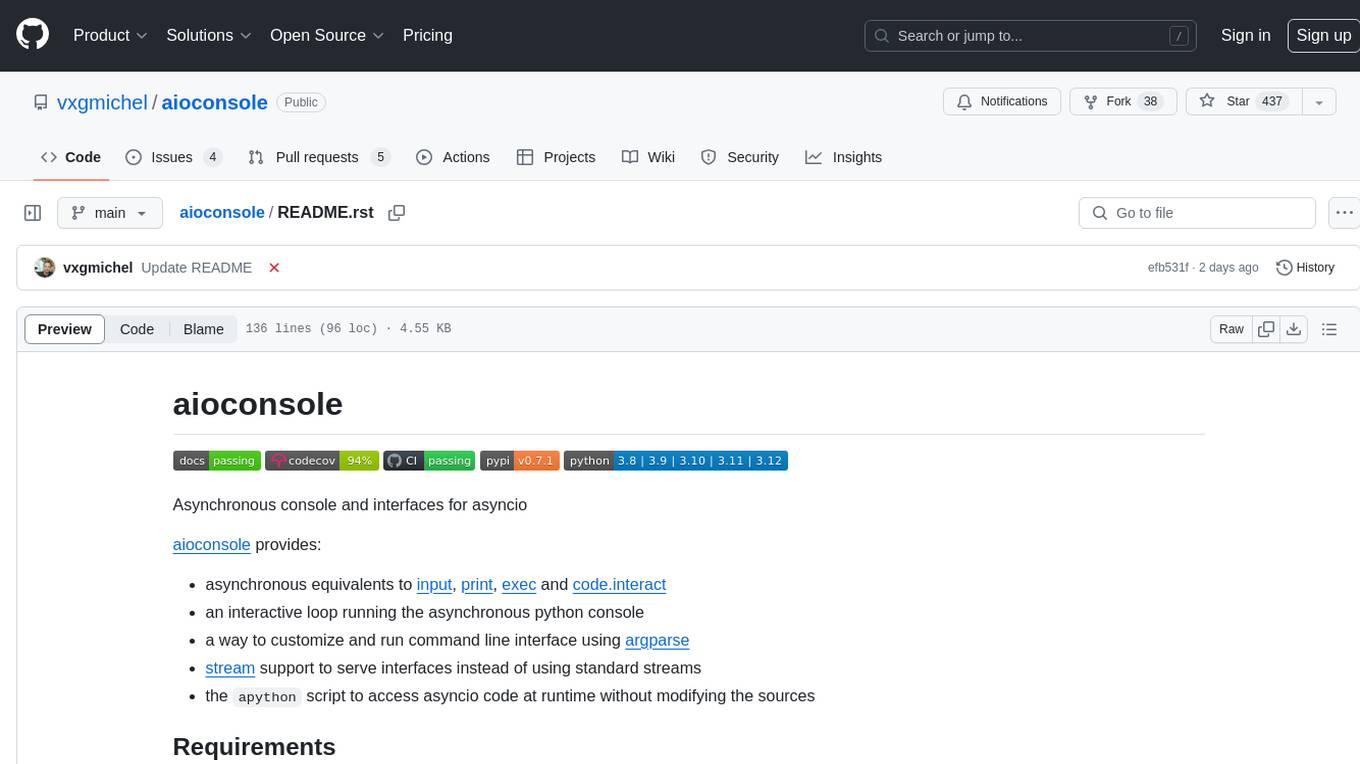
aioconsole
aioconsole is a Python package that provides asynchronous console and interfaces for asyncio. It offers asynchronous equivalents to input, print, exec, and code.interact, an interactive loop running the asynchronous Python console, customization and running of command line interfaces using argparse, stream support to serve interfaces instead of using standard streams, and the apython script to access asyncio code at runtime without modifying the sources. The package requires Python version 3.8 or higher and can be installed from PyPI or GitHub. It allows users to run Python files or modules with a modified asyncio policy, replacing the default event loop with an interactive loop. aioconsole is useful for scenarios where users need to interact with asyncio code in a console environment.
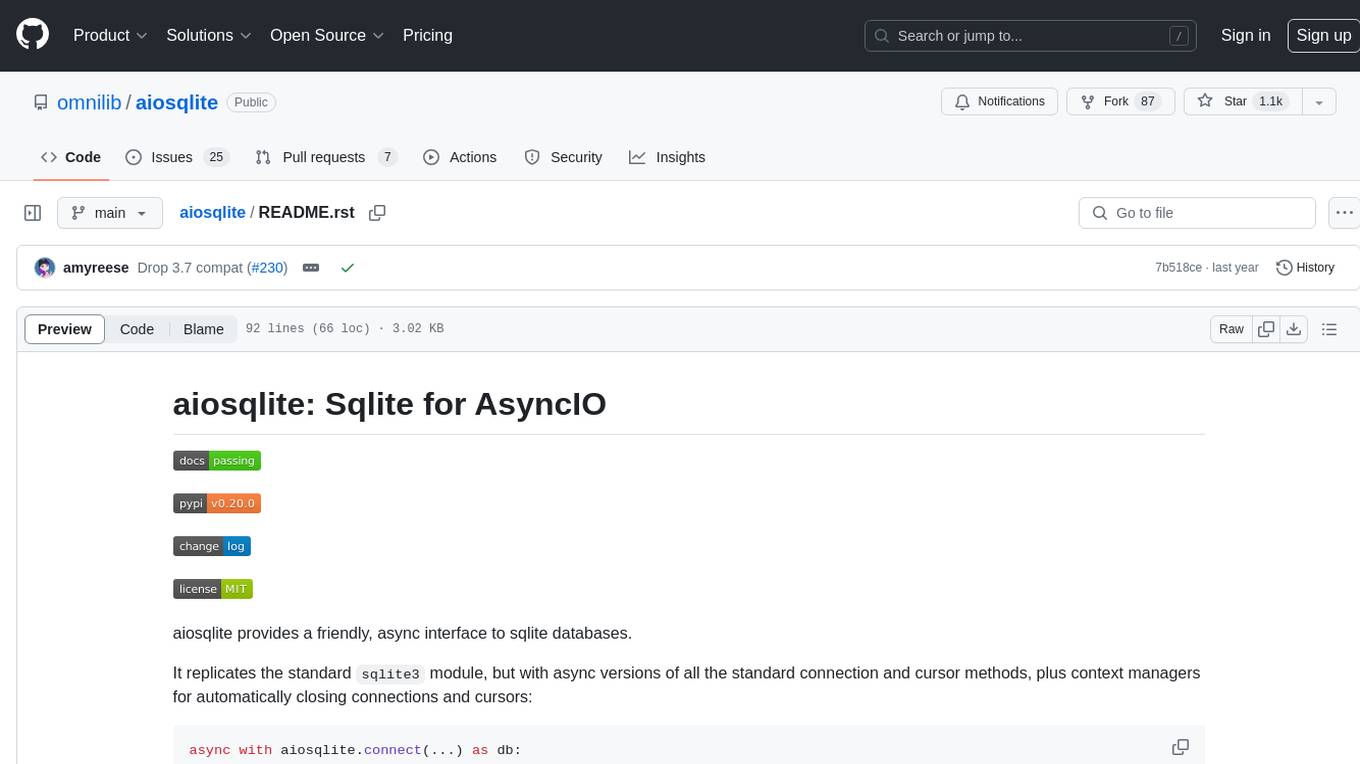
aiosqlite
aiosqlite is a Python library that provides a friendly, async interface to SQLite databases. It replicates the standard sqlite3 module but with async versions of all the standard connection and cursor methods, along with context managers for automatically closing connections and cursors. It allows interaction with SQLite databases on the main AsyncIO event loop without blocking execution of other coroutines while waiting for queries or data fetches. The library also replicates most of the advanced features of sqlite3, such as row factories and total changes tracking.