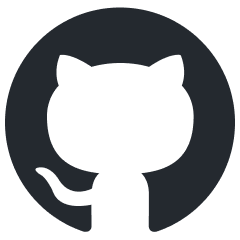
pinecone-ts-client
The official TypeScript/Node client for the Pinecone vector database
Stars: 209
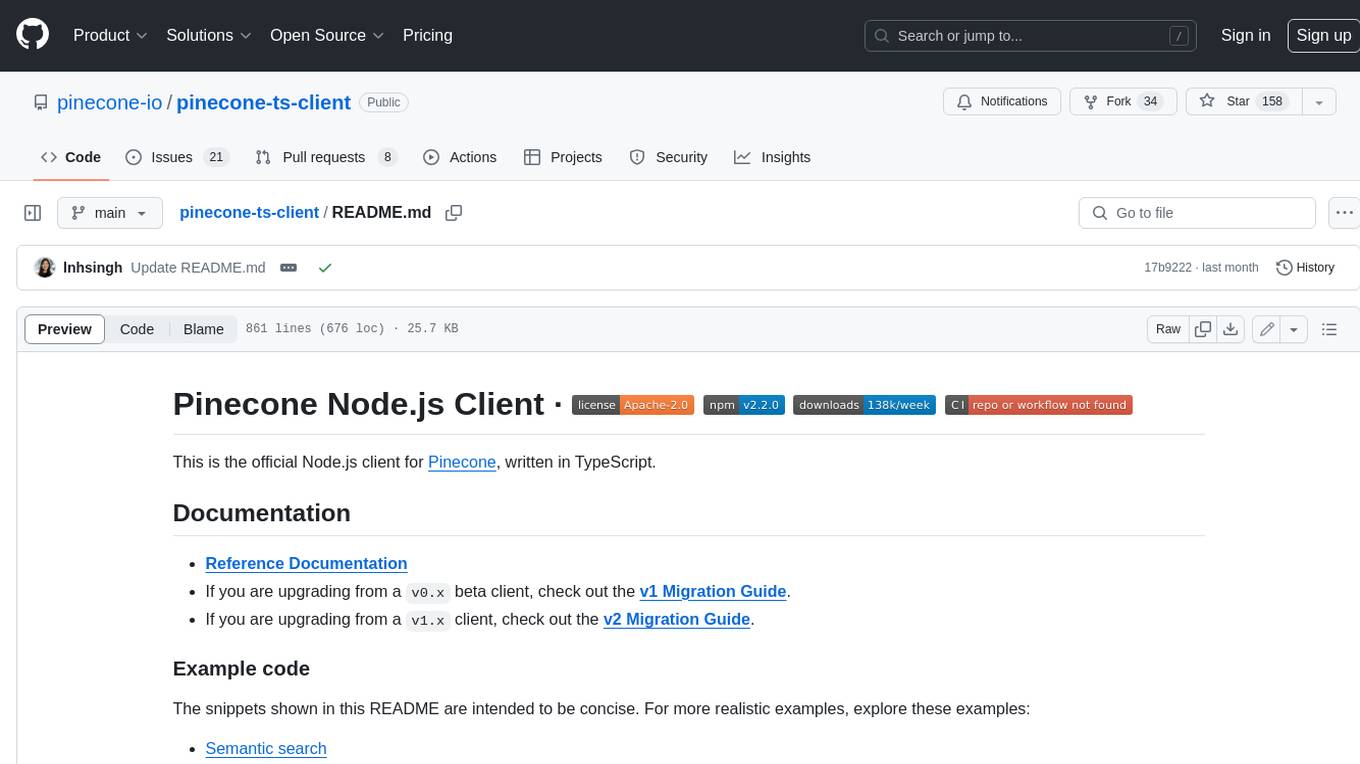
The official Node.js client for Pinecone, written in TypeScript. This client library provides a high-level interface for interacting with the Pinecone vector database service. With this client, you can create and manage indexes, upsert and query vector data, and perform other operations related to vector search and retrieval. The client is designed to be easy to use and provides a consistent and idiomatic experience for Node.js developers. It supports all the features and functionality of the Pinecone API, making it a comprehensive solution for building vector-powered applications in Node.js.
README:
This is the official Node.js SDK for Pinecone, written in TypeScript.
- Reference Documentation
- If you are upgrading from
v0.x
, check out the v1 Migration Guide. - If you are upgrading from
v1.x
, check out the v2 Migration Guide.
The snippets shown in this README are intended to be concise. For more realistic examples, explore these examples:
There is a breaking change involving the configureIndex
operation in this update. The structure of the object passed
when configuring an index has changed to include deletionProtection
. The podType
and replicas
fields can now be updated through the spec.pod
object. See Configure pod-based indexes for an example of the code.
-
Upgrading to
2.x
: There were many changes made in this release to support Pinecone's new Serverless index offering. The changes are covered in detail in the v2 Migration Guide. Serverless indexes are only available in2.x
release versions or greater. -
Upgrading to
1.x
: This release officially moved the SDK out of beta, and there are a number of breaking changes that need to be addressed when upgrading from a0.x
version. See the v1 Migration Guide for details.
The Pinecone TypeScript SDK is compatible with TypeScript >=4.1 and Node >=18.x.
npm install @pinecone-database/pinecone
The Pinecone Typescript SDK is intended for server-side use only. Using the SDK within a browser context can expose your API key(s). If you have deployed the SDK to production in a browser, please rotate your API keys.
An API key is required to initialize the client. It can be passed using an environment variable or in code through a configuration object. Get an API key in the console.
The environment variable used to configure the API key for the client is the following:
PINECONE_API_KEY="your_api_key"
PINECONE_API_KEY
is the only required variable. When this environment variable is set, the client constructor does not require any additional arguments.
import { Pinecone } from '@pinecone-database/pinecone';
const pc = new Pinecone();
If you prefer to pass configuration in code, the constructor accepts a config object containing the apiKey
value.
This is the object in which you would pass properties like maxRetries
(defaults to 3
) for retryable operations
(upsert
, update
, and configureIndex
).
import { Pinecone } from '@pinecone-database/pinecone';
const pc = new Pinecone({
apiKey: 'your_api_key',
maxRetries: 5,
});
If your network setup requires you to interact with Pinecone via a proxy, you can pass a custom ProxyAgent
from
the undici
library. Below is an example of how to
construct an undici
ProxyAgent
that routes network traffic through a mitm
proxy server while hitting Pinecone's /indexes
endpoint.
Note: The following strategy relies on Node's native fetch
implementation, released in Node v16 and
stabilized in Node v21. If you are running Node versions
18-21, you may experience issues stemming from the instability of the feature. There are currently no known issues
related to proxying in Node v18+.
import {
Pinecone,
type PineconeConfiguration,
} from '@pinecone-database/pinecone';
import { Dispatcher, ProxyAgent } from 'undici';
import * as fs from 'fs';
const cert = fs.readFileSync('path-to-your-mitm-proxy-cert-pem-file');
const client = new ProxyAgent({
uri: '<your proxy server URI>',
requestTls: {
port: '<your proxy server port>',
ca: cert,
host: '<your proxy server host>',
},
});
const customFetch = (
input: string | URL | Request,
init: RequestInit | undefined
) => {
return fetch(input, {
...init,
dispatcher: client as Dispatcher,
keepalive: true, # optional
});
};
const config: PineconeConfiguration = {
apiKey:
'<your Pinecone API key, available in your dashboard at app.pinecone.io>',
fetchApi: customFetch,
};
const pc = new Pinecone(config);
const indexes = async () => {
return await pc.listIndexes();
};
indexes().then((response) => {
console.log('My indexes: ', response);
});
At a minimum, to create a serverless index you must specify a name
, dimension
, and spec
. The dimension
indicates the size of the vectors you intend to store in the index. For example, if your intention was to store and
query embeddings (vectors) generated with OpenAI's textembedding-ada-002 model, you would need to create an index with dimension 1536
to match the output of that model. By default, serverless indexes will have a vectorType
of dense
.
The spec
configures how the index should be deployed. For serverless indexes, you define only the cloud and region where the index should be hosted. For pod-based indexes, you define the environment where the index should be hosted, the pod type and size to use, and other index characteristics. For more information on serverless and regional availability, see Understanding indexes.
import { Pinecone } from '@pinecone-database/pinecone';
const pc = new Pinecone();
await pc.createIndex({
name: 'sample-index',
dimension: 1536,
spec: {
serverless: {
cloud: 'aws',
region: 'us-west-2',
},
},
tags: { team: 'data-science' },
});
You can also use vectorType
to create sparse
serverless indexes. These indexes enable direct indexing and retrieval of sparse vectors, supporting traditional methods like BM25 and learned sparse models such as pinecone-sparse-english-v0. A sparse
index must have a distance metric
of dotproduct
and does not require a specified dimension. If no
metric is provided with a vectorType
of sparse
, it will default to dotproduct
:
import { Pinecone } from '@pinecone-database/pinecone';
const pc = new Pinecone();
await pc.createIndex({
name: 'sample-index',
metric: 'dotproduct',
spec: {
serverless: {
cloud: 'aws',
region: 'us-west-2',
},
},
tags: { team: 'data-science' },
vectorType: 'sparse',
});
Integrated inference requires a serverless index configured for a specific embedding model. You can either create a new index for a model or configure an existing index for a model. To create an index that accepts source text and converts it to vectors automatically using an embedding model hosted by Pinecone, use the createIndexForModel
method:
import { Pinecone } from '@pinecone-database/pinecone';
const pc = new Pinecone();
await pc.createIndexForModel({
name: 'integrated-index',
cloud: 'aws',
region: 'us-east-1',
embed: {
model: 'multilingual-e5-large',
fieldMap: { text: 'chunk_text' },
},
waitUntilReady: true,
});
To create a pod-based index, you define pod
in the spec
object which contains the environment
where the index should be hosted, and the podType
and pods
size to use. Many optional configuration fields allow greater control over hardware resources and availability. To learn more about the purpose of these fields, see Understanding indexes and Scale pod-based indexes.
import { Pinecone } from '@pinecone-database/pinecone';
const pc = new Pinecone();
await pc.createIndex({
name: 'sample-index-2',
dimension: 1536,
metric: 'dotproduct',
spec: {
pod: {
environment: 'us-east4-gcp',
pods: 2,
podType: 'p1.x2',
metadataConfig: {
indexed: ['product_type'],
},
},
tags: { team: 'data-science' },
},
// This option tells the client not to throw if the index already exists.
suppressConflicts: true,
// This option tells the client not to resolve the promise until the
// index is ready.
waitUntilReady: true,
});
The createIndex
method issues a create request to the API that returns quickly, but the resulting index is
not immediately ready for upserting, querying, or performing other data operations. You can use the
describeIndex
method to find out the status of an index and see whether it is ready for use.
import { Pinecone } from '@pinecone-database/pinecone';
const pc = new Pinecone();
await pc.describeIndex('serverless-index');
// {
// name: 'serverless-index',
// dimension: 1536,
// metric: 'cosine',
// host: 'serverless-index-4zo0ijk.svc.us-west2-aws.pinecone.io',
// deletionProtection: 'disabled',
// spec: {
// serverless: {
// cloud: 'aws',
// region: 'us-west-2'
// }
// },
// status: {
// ready: false,
// state: 'Initializing'
// }
// }
If you pass the waitUntilReady
option, the client will handle polling for status updates on a newly created index. The promise returned by createIndex
will not be resolved until the index status indicates it is ready to handle data operations. This can be especially useful for integration testing, where index creation in a setup step will be immediately followed by data operations.
import { Pinecone } from '@pinecone-database/pinecone';
const pc = new Pinecone();
await pc.createIndex({
name: 'serverless-index',
dimension: 1536,
spec: {
serverless: {
cloud: 'aws',
region: 'us-west-2',
},
},
waitUntilReady: true,
});
ℹ️ Note
Serverless and starter indexes do not support collections.
As you use Pinecone for more things, you may wish to explore different index configurations with the same vector data. Collections provide an easy way to do this. See other client methods for working with collections here.
Given that you have an existing collection:
import { Pinecone } from '@pinecone-database/pinecone';
const pc = new Pinecone();
await pc.describeCollection('product-description-embeddings');
// {
// name: 'product-description-embeddings',
// size: 543427063,
// status: 'Ready',
// dimension: 2,
// vectorCount: 10001498,
// environment: 'us-east4-gcp'
// }
Note: For pod-based indexes, you can specify a sourceCollection
from which to create an index. The
collection must be in the same environment as the index.
import { Pinecone } from '@pinecone-database/pinecone';
const pc = new Pinecone();
await pc.createIndex({
name: 'product-description-p1x1',
dimension: 256,
metric: 'cosine',
spec: {
pod: {
environment: 'us-east4-gcp',
pods: 1,
podType: 'p1.x1',
sourceCollection: 'product-description-embeddings',
},
},
});
When the new index is ready, it should contain all the data that was in the collection, ready to be queried.
import { Pinecone } from '@pinecone-database/pinecone';
const pc = new Pinecone();
await pc.index('product-description-p2x2').describeIndexStats();
// {
// namespaces: { '': { recordCount: 78000 } },
// dimension: 256,
// indexFullness: 0.9,
// totalRecordCount: 78000
// }
You can configure both serverless and pod indexes with deletionProtection
. Any index with this property set to 'enabled'
will be unable to be deleted. By default, deletionProtection
will be set to 'disabled'
if not provided as a part of the createIndex
request. To enable deletionProtection
you can pass the value while calling createIndex
.
import { Pinecone } from '@pinecone-database/pinecone';
const pc = new Pinecone();
await pc.createIndex({
name: 'deletion-protected-index',
dimension: 1536,
metric: 'cosine',
deletionProtection: 'enabled',
spec: {
serverless: {
cloud: 'aws',
region: 'us-west-2',
},
},
});
To disable deletion protection, you can use the configureIndex
operation.
import { Pinecone } from '@pinecone-database/pinecone';
const pc = new Pinecone();
await pc.configureIndex('deletion-protected-index', {
deletionProtection: 'disabled',
});
You can create or configure serverless and pod indexes with tags. Indexes can hold an arbitrary number of tags outlining metadata you would like attached to the index object itself, such as team ownership, project, or any other relevant information.
import { Pinecone } from '@pinecone-database/pinecone';
const pc = new Pinecone();
// Create index with tag
await pc.createIndex({
name: 'tag-index',
dimension: 1536,
metric: 'cosine',
spec: {
serverless: {
cloud: 'aws',
region: 'us-west-2',
},
},
tags: { team: 'data-science' }, // index tag
});
// Configure index with a new tag
await pc.configureIndex('tag-index', {
tags: { project: 'recommendation' }, // new index tag
});
// Delete an existing tag
await pc.configureIndex('tag-index', {
tags: { project: '' }, // Pass an empty string to an existing key to delete a tag; this will delete the `project` tag
});
You can fetch the description of any index by name using describeIndex
.
import { Pinecone } from '@pinecone-database/pinecone';
const pc = new Pinecone();
await pc.describeIndex('serverless-index');
// {
// name: 'serverless-index',
// dimension: 1536,
// metric: 'cosine',
// host: 'serverless-index-4zo0ijk.svc.us-west2-aws.pinecone.io',
// deletionProtection: 'disabled',
// spec: {
// serverless: {
// cloud: 'aws',
// region: 'us-west-2'
// },
// },
// status: {
// ready: true,
// state: 'Ready'
// }
// }
ℹ️ Note
This section applies to pod-based indexes only. With serverless indexes, you don't configure any compute or storage resources. Instead, serverless indexes scale automatically based on usage.
You can adjust the number of replicas or scale to a larger pod size (specified with podType
). See Scale pod-based indexes. You cannot downgrade pod size or change the base pod type.
import { Pinecone } from '@pinecone-database/pinecone';
const pc = new Pinecone();
await pc.configureIndex('pod-index', {
spec: {
pod: {
replicas: 2,
podType: 'p1.x4',
},
},
});
const config = await pc.describeIndex('pod-index');
// {
// name: 'pod-index',
// dimension: 1536,
// metric: 'cosine',
// host: 'pod-index-4zo0ijk.svc.us-east1-gcp.pinecone.io',
// deletionProtection: 'disabled',
// spec: {
// pod: {
// environment: 'us-east1-gcp',
// replicas: 2,
// shards: 2,
// podType: 'p1.x4',
// pods: 4,
// metadataConfig: [Object],
// sourceCollection: undefined
// }
// },
// status: {
// ready: true,
// state: 'ScalingUpPodSize'
// }
// }
Indexes are deleted by name.
import { Pinecone } from '@pinecone-database/pinecone';
const pc = new Pinecone();
await pc.deleteIndex('sample-index');
The listIndexes
command returns an object with an array of index models under indexes
.
import { Pinecone } from '@pinecone-database/pinecone';
const pc = new Pinecone();
await pc.listIndexes();
// {
// indexes: [
// {
// name: 'serverless-index',
// dimension: 1536,
// metric: 'cosine',
// host: 'serverless-index-4zo0ijk.svc.us-west2-aws.pinecone.io',
// deletionProtection: 'disabled',
// spec: {
// serverless: {
// cloud: 'aws',
// region: 'us-west-2',
// },
// },
// status: {
// ready: true,
// state: 'Ready',
// },
// },
// {
// name: 'pod-index',
// dimension: 1536,
// metric: 'cosine',
// host: 'pod-index-4zo0ijk.svc.us-west2-aws.pinecone.io',
// deletionProtection: 'disabled',
// spec: {
// pod: {
// environment: 'us-west2-aws',
// replicas: 1,
// shards: 1,
// podType: 'p1.x1',
// pods: 1,
// },
// },
// status: {
// ready: true,
// state: 'Ready',
// },
// },
// ],
// }
ℹ️ Note
Serverless and starter indexes do not support collections.
A collection is a static copy of a pod-based index that may be used to create backups, to create copies of indexes, or to perform experiments with different index configurations. To learn more about Pinecone collections, see Understanding collections.
import { Pinecone } from '@pinecone-database/pinecone';
const pc = new Pinecone();
await pc.createCollection({
name: 'collection-name',
source: 'index-name',
});
This API call should return quickly, but the creation of a collection can take from minutes to hours depending on the size of the source index and the index's configuration. Use describeCollection
to check the status of a collection.
import { Pinecone } from '@pinecone-database/pinecone';
const pc = new Pinecone();
await pc.deleteCollection('collection-name');
You can use listCollections
to confirm the deletion.
import { Pinecone } from '@pinecone-database/pinecone';
const pc = new Pinecone();
const describeCollection = await pc.describeCollection('collection3');
// {
// name: 'collection3',
// size: 3126700,
// status: 'Ready',
// dimension: 3,
// vectorCount: 1234,
// environment: 'us-east1-gcp',
// }
The listCollections
command returns an object with an array of collection models under collections
.
import { Pinecone } from '@pinecone-database/pinecone';
const pc = new Pinecone();
const list = await pc.listCollections();
// {
// collections: [
// {
// name: 'collection1',
// size: 3089687,
// status: 'Ready',
// dimension: 3,
// vectorCount: 17378,
// environment: 'us-west1-gcp',
// },
// {
// name: 'collection2',
// size: 208309,
// status: 'Ready',
// dimension: 3,
// vectorCount: 1000,
// environment: 'us-east4-gcp',
// },
// ];
// }
Pinecone indexes support operations for working with vector data using operations such as upsert, query, fetch, and delete.
To perform data operations on an index, you target it using the index
method.
import { Pinecone } from '@pinecone-database/pinecone';
const pc = new Pinecone();
const index = pc.index('test-index');
// Now perform index operations
await index.fetch(['1']);
The first argument is the name of the index you are targeting. There's an optional second argument for providing an
index host override. Providing this second argument allows you to bypass the SDK's default behavior of resolving
your index host via the provided index name. You can find your index host in the Pinecone console, or by using the describeIndex
or listIndexes
operations.
import { Pinecone } from '@pinecone-database/pinecone';
const pc = new Pinecone();
const index = pc.index('test-index', 'my-index-host-1532-svc.io');
// Now perform index operations against: https://my-index-host-1532-svc.io
await index.fetch(['1']);
If you are storing metadata alongside your vector values, you can pass a type parameter to index()
in order to get proper TypeScript typechecking.
import { Pinecone, PineconeRecord } from '@pinecone-database/pinecone';
const pc = new Pinecone();
type MovieMetadata = {
title: string,
runtime: number,
genre: 'comedy' | 'horror' | 'drama' | 'action'
}
// Specify a custom metadata type while targeting the index
const index = pc.index<MovieMetadata>('test-index');
// Now you get type errors if upserting malformed metadata
await index.upsert([{
id: '1234',
values: [
.... // embedding values
],
metadata: {
genre: 'Gone with the Wind',
runtime: 238,
genre: 'drama',
// @ts-expect-error because category property not in MovieMetadata
category: 'classic'
}
}])
const results = await index.query({
vector: [
... // query embedding
],
filter: { genre: { '$eq': 'drama' }}
})
const movie = results.matches[0];
if (movie.metadata) {
// Since we passed the MovieMetadata type parameter above,
// we can interact with metadata fields without having to
// do any typecasting.
const { title, runtime, genre } = movie.metadata;
console.log(`The best match in drama was ${title}`)
}
By default, all data operations take place inside the default namespace of ''
. If you are working with other non-default namespaces, you can target the namespace by chaining a call to namespace()
.
import { Pinecone } from '@pinecone-database/pinecone';
const pc = new Pinecone();
const index = pc.index('test-index').namespace('ns1');
// Now perform index operations in the targeted index and namespace
await index.fetch(['1']);
See Use namespaces for more information.
Pinecone expects records inserted into indexes to have the following form:
type PineconeRecord = {
id: string;
values: Array<number>;
sparseValues?: Array<number>;
metadata?: object;
};
To upsert some vectors, you can use the client like so:
import { Pinecone } from '@pinecone-database/pinecone';
const pc = new Pinecone();
// Target an index
const index = pc.index('sample-index');
// Prepare your data. The length of each array
// of vector values must match the dimension of
// the index where you plan to store them.
const vectors = [
{
id: '1',
values: [0.236, 0.971, 0.559],
sparseValues: { indices: [0, 1], values: [0.236, 0.34] }, // Optional; for hybrid search
},
{
id: '2',
values: [0.685, 0.111, 0.857],
sparseValues: { indices: [0, 1], values: [0.345, 0.98] }, // Optional; for hybrid search
},
];
// Upsert the data into your index
await index.upsert(vectors);
You can now import vectors en masse from object
storage. Import
is a long-running, asynchronous operation that imports large numbers of records into a Pinecone
serverless index.
In order to import vectors from object storage, they must be stored in Parquet files and adhere to the necessary file format. Your object storage must also adhere to the necessary directory structure.
The following example imports vectors from an Amazon S3 bucket into a Pinecone serverless index:
import { Pinecone } from '@pinecone-database/pinecone';
const pc = new Pinecone();
const indexName = 'sample-index';
await pc.createIndex({
name: indexName,
dimension: 10,
spec: {
serverless: {
cloud: 'aws',
region: 'eu-west-1',
},
},
});
const index = pc.Index(indexName);
const storageURI = 's3://my-bucket/my-directory/';
await index.startImport(storageURI, 'continue'); // "Continue" will avoid aborting the operation if errors are encountered.
// {
// "id": "import-id"
// }
You can start, cancel, and check the status of all or one import operation(s).
Notes:
-
Import
only works with Serverless indexes -
Import
is in public preview - The only object storage provider currently supported is Amazon S3
- Vectors will take at least 10 minutes to appear in your index upon completion of the import operation, since this operation is optimized for very large workloads
- See limits for further information
When experimenting with data operations, it's sometimes helpful to know how many records/vectors are stored in each
namespace. In that case, target the index and use the describeIndexStats()
command.
import { Pinecone } from '@pinecone-database/pinecone';
const pc = new Pinecone();
const index = pc.index('example-index');
await index.describeIndexStats();
// {
// namespaces: {
// '': { recordCount: 10 }
// foo: { recordCount: 2000 },
// bar: { recordCount: 2000 }
// },
// dimension: 1536,
// indexFullness: 0,
// totalRecordCount: 4010
// }
The query method accepts a large number of options. The dimension of the query vector must match the dimension of your index.
type QueryOptions = {
topK: number; // number of results desired
vector?: Array<number>; // must match dimension of index
sparseVector?: {
indices: Array<integer>; // indices must fall within index dimension
values: Array<number>; // indices and values arrays must have same length
};
id?: string;
includeMetadata: boolean;
includeValues: boolean;
};
For example, to query by vector values you would pass the vector
param in the options configuration. For brevity sake this example query vector is tiny (dimension 2), but in a more realistic use case this query vector would be an embedding outputted by a model. Look at the Example code to see more realistic examples of how to use query
.
import { Pinecone } from '@pinecone-database/pinecone';
const pc = new Pinecone();
const index = pc.index('my-index');
await index.query({ topK: 3, vector: [0.22, 0.66] });
// {
// matches: [
// {
// id: '556',
// score: 1.00000012,
// values: [],
// sparseValues: undefined,
// metadata: undefined
// },
// {
// id: '137',
// score: 1.00000012,
// values: [],
// sparseValues: undefined,
// metadata: undefined
// },
// {
// id: '129',
// score: 1.00000012,
// values: [],
// sparseValues: undefined,
// metadata: undefined
// }
// ],
// namespace: '',
// usage: {
// readUnits: 5
// }
// }
You include options to includeMetadata: true
or includeValues: true
if you need this information. By default,
these are not returned to keep the response payload small.
Remember that data operations take place within the context of a namespace
, so if you are working with namespaces and do not see expected results you should check that you are targeting the correct namespace with your query.
import { Pinecone } from '@pinecone-database/pinecone';
const pc = new Pinecone();
// Target the index and namespace
const index = pc.index('my-index').namespace('my-namespace');
const results = await index.query({ topK: 3, vector: [0.22, 0.66] });
You can query using the vector values of an existing record in the index by passing a record ID. Please note that the record with the specified ID may be in this operation's response.
import { Pinecone } from '@pinecone-database/pinecone';
const pc = new Pinecone();
const index = pc.index('my-index');
const results = await index.query({ topK: 10, id: '1' });
If you are working with sparse-dense vectors, you can add sparse vector values to perform a hybrid search.
import { Pinecone } from '@pinecone-database/pinecone';
const pc = new Pinecone();
await pc.createIndex({
name: 'hybrid-search-index',
metric: 'dotproduct', // Note: dot product is the only distance metric supported for hybrid search
dimension: 2,
spec: {
pod: {
environment: 'us-west4-gcp',
podType: 'p2.x1',
},
},
waitUntilReady: true,
});
const index = pc.index('hybrid-search-index');
const hybridRecords = [
{
id: '1',
values: [0.236, 0.971], // dense vectors
sparseValues: { indices: [0, 1], values: [0.236, 0.34] }, // sparse vectors
},
{
id: '2',
values: [0.685, 0.111],
sparseValues: { indices: [0, 1], values: [0.887, 0.243] },
},
];
await index.upsert(hybridRecords);
const query = 'What is the most popular red dress?';
// ... send query to dense vector embedding model and save those values in `denseQueryVector`
// ... send query to sparse vector embedding model and save those values in `sparseQueryVector`
const denseQueryVector = [0.236, 0.971];
const sparseQueryVector = { indices: [0, 1], values: [0.0, 0.34] };
// Execute a hybrid search
await index.query({
topK: 3,
vector: denseQueryVector,
sparseVector: sparseQueryVector,
});
You may want to update vector values
, sparseValues
, or metadata
. Specify the id and the attribute value you want to update.
import { Pinecone } from '@pinecone-database/pinecone';
const pc = new Pinecone();
const index = pc.index('imdb-movies');
await index.update({
id: '18593',
metadata: { genre: 'romance' },
});
The listPaginated
method can be used to list record IDs matching a particular ID prefix in a paginated format. With
clever assignment
of record ids, this can be used to help model hierarchical relationships between different records such as when there are embeddings for multiple chunks or fragments related to the same document.
Notes:
- When you do not specify a
prefix
, the default prefix is an empty string, which returns all vector IDs in your index - There is a hard limit of
100
vector IDs if nolimit
is specified. Consequently, if there are fewer than100
vector IDs that match a givenprefix
in your index, and you do not specify alimit
, yourpaginationToken
will beundefined
The following example shows how to fetch both pages of vector IDs for vectors whose IDs contain the prefix doc1#
,
assuming a limit
of 3
and doc1
document being chunked into 4
vectors.
const pc = new Pinecone();
const index = pc.index('my-index').namespace('my-namespace');
// Fetch the 1st 3 vector IDs matching prefix 'doc1#'
const results = await index.listPaginated({ limit: 3, prefix: 'doc1#' });
console.log(results);
// {
// vectors: [
// { id: 'doc1#01' }
// { id: 'doc1#02' }
// { id: 'doc1#03' }
// ...
// ],
// pagination: {
// next: 'eyJza2lwX3Bhc3QiOiJwcmVUZXN0LS04MCIsInByZWZpeCI6InByZVRlc3QifQ=='
// },
// namespace: 'my-namespace',
// usage: { readUnits: 1 }
// }
// Fetch the final vector ID matching prefix 'doc1#' using the paginationToken returned by the previous call
const nextResults = await index.listPaginated({
prefix: 'doc1#',
paginationToken: results.pagination?.next,
});
console.log(nextResults);
// {
// vectors: [
// { id: 'doc1#04' }
// ],
// pagination: undefined,
// namespace: 'my-namespace',
// usage: { readUnits: 1 }
// }
import { Pinecone } from '@pinecone-database/pinecone';
const pc = new Pinecone();
const index = pc.index('my-index');
const fetchResult = await index.fetch(['id-1', 'id-2']);
For convenience there are several delete-related methods. You can verify the results of a delete operation by trying to fetch()
a record or looking at the index summary with describeIndexStats()
import { Pinecone } from '@pinecone-database/pinecone';
const pc = new Pinecone();
const index = pc.index('my-index');
await index.deleteOne('id-to-delete');
import { Pinecone } from '@pinecone-database/pinecone';
const pc = new Pinecone();
const index = pc.index('my-index');
await index.deleteMany(['id-1', 'id-2', 'id-3']);
Note: deletion by metadata filter only applies to pod-based indexes.
import { Pinecone } from '@pinecone-database/pinecone';
const pc = new Pinecone();
const index = pc.index('albums-database');
await index.deleteMany({ genre: 'rock' });
ℹ️ NOTE
Indexes in the gcp-starter environment do not support namespaces.
To nuke everything in the targeted namespace, use the deleteAll
method.
import { Pinecone } from '@pinecone-database/pinecone';
const pc = new Pinecone();
const index = pc.index('my-index');
await index.namespace('foo-namespace').deleteAll();
If you do not specify a namespace, the records in the default namespace ''
will be deleted.
Use embedding and & reranking models hosted by Pinecone. Learn more about Inference in the docs.
To see the available models:
Generate embeddings for documents and queries.
import { Pinecone } from '@pinecone-database/pinecone';
const client = new Pinecone({ apiKey: '<Your API key from app.pinecone.io>' });
const embeddingModel = 'multilingual-e5-large';
const documents = [
'Turkey is a classic meat to eat at American Thanksgiving.',
'Many people enjoy the beautiful mosques in Turkey.',
];
const docParameters = {
inputType: 'passage',
truncate: 'END',
};
async function generateDocEmbeddings() {
try {
return await client.inference.embed(
embeddingModel,
documents,
docParameters
);
} catch (error) {
console.error('Error generating embeddings:', error);
}
}
generateDocEmbeddings().then((embeddingsResponse) => {
if (embeddingsResponse) {
console.log(embeddingsResponse);
}
});
// << Upsert documents into Pinecone >>
const userQuery = ['How should I prepare my turkey?'];
const queryParameters = {
inputType: 'query',
truncate: 'END',
};
async function generateQueryEmbeddings() {
try {
return await client.inference.embed(
embeddingModel,
userQuery,
queryParameters
);
} catch (error) {
console.error('Error generating embeddings:', error);
}
}
generateQueryEmbeddings().then((embeddingsResponse) => {
if (embeddingsResponse) {
console.log(embeddingsResponse);
}
});
// << Send query to Pinecone to retrieve similar documents >>
Rerank documents in descending relevance-order against a query.
Note: The score
represents the absolute measure of relevance of a given query and passage pair. Normalized
between [0, 1], the score
represents how closely relevant a specific item and query are, with scores closer to 1
indicating higher relevance.
import { Pinecone } from '@pinecone-database/pinecone';
const pc = new Pinecone();
const rerankingModel = 'bge-reranker-v2-m3';
const myQuery = 'What are some good Turkey dishes for Thanksgiving?';
// Option 1: Documents as an array of strings
const myDocsStrings = [
'I love turkey sandwiches with pastrami',
'A lemon brined Turkey with apple sausage stuffing is a classic Thanksgiving main',
'My favorite Thanksgiving dish is pumpkin pie',
'Turkey is a great source of protein',
];
// Option 1 response
const response = await pc.inference.rerank(
rerankingModel,
myQuery,
myDocsStrings
);
console.log(response);
// {
// model: 'bge-reranker-v2-m3',
// data: [
// { index: 1, score: 0.5633179, document: [Object] },
// { index: 2, score: 0.02013874, document: [Object] },
// { index: 3, score: 0.00035419367, document: [Object] },
// { index: 0, score: 0.00021485926, document: [Object] }
// ],
// usage: { rerankUnits: 1 }
// }
// Option 2: Documents as an array of objects
const myDocsObjs = [
{
title: 'Turkey Sandwiches',
body: 'I love turkey sandwiches with pastrami',
},
{
title: 'Lemon Turkey',
body: 'A lemon brined Turkey with apple sausage stuffing is a classic Thanksgiving main',
},
{
title: 'Thanksgiving',
body: 'My favorite Thanksgiving dish is pumpkin pie',
},
{
title: 'Protein Sources',
body: 'Turkey is a great source of protein',
},
];
// Option 2: Options object declaring which custom key to rerank on
// Note: If no custom key is passed via `rankFields`, each doc must contain a `text` key, and that will act as the default)
const rerankOptions = {
topN: 3,
returnDocuments: false,
rankFields: ['body'],
parameters: {
inputType: 'passage',
truncate: 'END',
},
};
// Option 2 response
const response = await pc.inference.rerank(
rerankingModel,
myQuery,
myDocsObjs,
rerankOptions
);
console.log(response);
// {
// model: 'bge-reranker-v2-m3',
// data: [
// { index: 1, score: 0.5633179, document: undefined },
// { index: 2, score: 0.02013874, document: undefined },
// { index: 3, score: 0.00035419367, document: undefined },
// ],
// usage: { rerankUnits: 1 }
//}
When using an index with integrated inference, embedding and reranking operations are tied to index operations and do not require extra steps. This allows working with an index that accepts source text and converts it to vectors automatically using an embedding model hosted by Pinecone.
Integrated inference requires a serverless index configured for a specific embedding model. You can either create a new index for a model or configure an existing index for a model. See Create an integrated index for specifics on creating these indexes.
Once you have an index configured for a specific embedding model, use the upsertRecords
operation on the Index
class to convert your source data to embeddings and upsert them into a namespace.
Note the following requirements for each record:
- Each record must contain a unique
id
, which will serve as the record identifier in the index namespace. - Each record must contain a field with the data for embedding. This field must match the field_map specified when creating the index.
- Any additional fields in the record will be stored in the index and can be returned in search results or used to filter search results.
import { Pinecone } from '@pinecone-database/pinecone';
const pc = new Pinecone();
// Target an integrated index
const namespace = pc.index('integrated-index').namespace('namespace1');
const records = [
{
id: 'rec1',
chunk_text:
"Apple's first product, the Apple I, was released in 1976 and was hand-built by co-founder Steve Wozniak.",
category: 'product',
},
{
id: 'rec2',
chunk_text:
'Apples are a great source of dietary fiber, which supports digestion and helps maintain a healthy gut.',
category: 'nutrition',
},
{
id: 'rec3',
chunk_text:
'Apples originated in Central Asia and have been cultivated for thousands of years, with over 7,500 varieties available today.',
category: 'cultivation',
},
{
id: 'rec4',
chunk_text:
'In 2001, Apple released the iPod, which transformed the music industry by making portable music widely accessible.',
category: 'product',
},
{
id: 'rec5',
chunk_text:
'Apple went public in 1980, making history with one of the largest IPOs at that time.',
category: 'milestone',
},
{
id: 'rec6',
chunk_text:
'Rich in vitamin C and other antioxidants, apples contribute to immune health and may reduce the risk of chronic diseases.',
category: 'nutrition',
},
{
id: 'rec7',
chunk_text:
"Known for its design-forward products, Apple's branding and market strategy have greatly influenced the technology sector and popularized minimalist design worldwide.",
category: 'influence',
},
{
id: 'rec8',
chunk_text:
'The high fiber content in apples can also help regulate blood sugar levels, making them a favorable snack for people with diabetes.',
category: 'nutrition',
},
];
// Upsert the data into your index
await namespace.upsertRecords(records);
Use the searchRecords
method to convert a query to a vector embedding and then search your namespace for the most semantically similar records, along with their similarity scores.
import { Pinecone } from '@pinecone-database/pinecone';
const pc = new Pinecone();
// Target an integrated index
const namespace = pc.index('integrated-index').namespace('namespace1');
// search for 4 records most semantically relevant to the query 'Disease prevention'
const response = await namespace.searchRecords({
query: { topK: 4, inputs: { text: 'Disease prevention' } },
});
To rerank initial search results based on relevance to the query, add the rerank parameter, including the reranking model you want to use, the number of reranked results to return, and the fields to use for reranking, if different than the main query.
For example, repeat the search for the 4 documents most semantically related to the query, “Disease prevention”, but this time rerank the results and return only the 2 most relevant documents:
const response = await namespace.searchRecords({
query: {
topK: 4,
inputs: { text: 'Disease prevention' },
},
rerank: {
model: 'bge-reranker-v2-m3',
topN: 2,
rankFields: ['chunk_text'],
},
fields: ['category', 'chunk_text'],
});
The Pinecone Assistant API enables you to create and manage AI assistants powered by Pinecone's vector database capabilities. These Assistants can be customized with specific instructions and metadata, and can interact with files and engage in chat conversations.
Creates a new Assistant with specified configurations. You can define the Assistant's name, provide instructions that guide its behavior, and attach metadata for organization and tracking purposes.
import { Pinecone } from '@pinecone-database/pinecone';
const pc = new Pinecone();
const assistant = await pc.createAssistant({
name: 'product-assistant',
instructions: 'You are a helpful product recommendation assistant.',
metadata: {
team: 'product',
version: '1.0',
},
});
Deletes an Assistant by name.
Note: Deleting an Assistant also deletes all associated files.
import { Pinecone } from '@pinecone-database/pinecone';
const pc = new Pinecone();
await pc.deleteAssistant('test1');
Retrieves information about an Assistant by name.
import { Pinecone } from '@pinecone-database/pinecone';
const pc = new Pinecone();
const test = await pc.describeAssistant('test1');
console.log(test);
// {
// name: 'test10',
// instructions: undefined,
// metadata: undefined,
// status: 'Ready',
// host: 'https://prod-1-data.ke.pinecone.io',
// createdAt: 2025-01-08T22:24:50.525Z,
// updatedAt: 2025-01-08T22:24:52.303Z
// }
Updates an Assistant by name. You can update the Assistant's name, instructions, and/or metadata.
import { Pinecone } from '@pinecone-database/pinecone';
const pc = new Pinecone();
await pc.updateAssistant('test1', {
instructions: 'some new instructions!',
});
Retrieves a list of all Assistants in your account. This method returns details about each Assistant including their names, instructions, metadata, status, and host.
import { Pinecone } from '@pinecone-database/pinecone';
const pc = new Pinecone();
const assistants = await pc.listAssistants();
console.log(assistants);
// {
// assistants: [{
// name: 'product-assistant',
// instructions: 'You are a helpful product recommendation assistant.',
// metadata: { team: 'product', version: '1.0' },
// status: 'Ready',
// host: 'product-assistant-abc123.svc.pinecone.io'
// }]
// }
You can chat with Assistants using either the chat
method or the chatCompletion
methods.
Note: Your Assistant must contain files in order for chat to work.
The following example shows how to chat with an Assistant using the chat
methods:
import { Pinecone } from '@pinecone-database/pinecone';
const pc = new Pinecone();
const assistantName = 'test1';
const assistant = pc.Assistant(assistantName);
const chatResp = await assistant.chat({
messages: [
{
role: 'user',
content: 'What is the capital of France?',
},
],
});
console.log(chatResp);
// {
// id: '000000000000000023e7fb015be9d0ad',
// finishReason: 'stop',
// message: {
// role: 'assistant',
// content: 'The capital of France is Paris.'
// },
// model: 'gpt-4o-2024-05-13',
// citations: [ { position: 209, references: [Array] } ],
// usage: { promptTokens: 493, completionTokens: 38, totalTokens: 531 }
// }
chatCompletion
is based on the OpenAI Chat Completion format, and is useful if OpenAI-compatible responses. However, it has limited functionality compared to the standard chat
method. Read more here.
import { Pinecone } from '@pinecone-database/pinecone';
const pc = new Pinecone();
const assistantName = 'test1';
const assistant = pc.Assistant(assistantName);
const chatResp = await assistant.chatCompletion({
messages: [
{
role: 'user',
content: 'What is the capital of France?',
},
],
});
console.log(chatResp);
// {
// id: '000000000000000023e7fb015be9d0ad',
// choices: [
// {
// finishReason: 'stop',
// index: 0,
// message: {
// role: 'assistant',
// content: 'The capital of France is Paris.'
// }
// }
// ],
// finishReason: 'stop',
// model: 'gpt-4o-2024-05-13',
// usage: { promptTokens: 493, completionTokens: 38, totalTokens: 531 }
// }
Assistant chat responses can also be streamed using the chatStream
and chatCompletionStream
methods on the Assistant
class. These methods return a ChatStream
which implements AsyncIterable
, returning an async iterator object allowing for manipulation of the stream. You can stream either the chat or chat completions operations.
Note: The shape of the JSON returned in each streamed chunk will be different depending which method is being used.
Chat stream:
import { Pinecone } from '@pinecone-database/pinecone';
const pc = new Pinecone();
const assistantName = 'test1';
const assistant = pc.Assistant(assistantName);
const chatStream = await assistant.chatStream({
messages: [
{
role: 'user',
content: 'What is the capital of France?',
},
],
});
for await (const chunk of chatStream) {
console.log(chunk);
}
// Each chunk in the stream will have a different shape depending on the type:
//
// {
// type: 'message_start',
// id: 'response_id',
// model: 'gpt-4o-2024-05-13',
// role: 'assistant'
// }
// {
// type: 'content_chunk',
// id: 'response_id',
// model: 'gpt-4o-2024-05-13',
// delta: { content: 'The' }
// }
// {
// type: 'content_chunk',
// id: 'response_id',
// model: 'gpt-4o-2024-05-13',
// delta: { content: ' capital' }
// }
// {
// type: 'content_chunk',
// id: 'response_id',
// model: 'gpt-4o-2024-05-13',
// delta: { content: ' of' }
// }
// {
// type: 'content_chunk',
// id: 'response_id',
// model: 'gpt-4o-2024-05-13',
// delta: { content: ' France' }
// }
// {
// type: 'content_chunk',
// id: 'response_id',
// model: 'gpt-4o-2024-05-13',
// delta: { content: ' is Paris.' }
// }
// {
// type: 'citation',
// id: 'response_id',
// model: 'gpt-4o-2024-05-13',
// citation: { position: 1538, references: [ [Object] ] }
// }
// {
// type: 'message_end',
// id: '000000000000000002378669324ef087',
// model: 'gpt-4o-2024-05-13',
// finishReason: 'stop',
// usage: { promptTokens: 9080, completionTokens: 312, totalTokens: 9392 }
// }
Chat completion stream:
import { Pinecone } from '@pinecone-database/pinecone';
const pc = new Pinecone();
const assistantName = 'test1';
const assistant = pc.Assistant(assistantName);
const chatCompletionStream = await assistant.chatCompletionStream({
messages: [
{
role: 'user',
content: 'What is the capital of France?',
},
],
});
for await (const chunk of chatCompletionStream) {
console.log(chunk);
}
// Each chunk will have the same OpenAI compatible completion shape:
//
// {
// id: 'response-id',
// choices: [
// {
// index: 0,
// delta: {
// role: 'assistant'
// },
// finishReason: null
// }
// ],
// model: 'gpt-4o-2024-05-13',
// usage: null
// }
// {
// id: 'response-id',
// choices: [
// {
// index: 0,
// delta: {
// content: 'The capital'
// },
// finishReason: null
// }
// ],
// model: 'gpt-4o-2024-05-13',
// usage: null
// }
// ... rest of stream
// {
// id: 'response-id',
// choices: [],
// model: 'gpt-4o-2024-05-13',
// usage: {
// promptTokens: 9080,
// completionTokens: 338,
// totalTokens: 9418
// }
// }
Returns context snippets associated with a given query and an Assistant's response. This is useful for understanding how the Assistant arrived at its answer(s).
import { Pinecone } from '@pinecone-database/pinecone';
const pc = new Pinecone();
const assistantName = 'test1';
const assistant = pc.Assistant(assistantName);
const context = await assistant.context({
messages: ['What is the capital of France?'],
topK: 1,
});
console.log(context);
// {
// snippets: [
// {
// type: 'text',
// content: 'The capital of France is Paris.',
// score: 0.9978925,
// reference: [Object]
// },
// ],
// usage: { promptTokens: 527, completionTokens: 0, totalTokens: 527 }
// }
You can add files to an Assistant to enable it to interact with files during chat conversations. The following
example shows how to upload a local test-file.txt
file to an Assistant.
Note: You must upload at least 1 file in order to chat with an Assistant.
import { Pinecone } from '@pinecone-database/pinecone';
const pc = new Pinecone();
const assistantName = 'test1';
const assistant = pc.Assistant(assistantName);
await assistant.uploadFile({
path: 'test-file.txt',
metadata: { 'test-key': 'test-value' },
});
// {
// name: 'test-file.txt',
// id: '921ad74c-2421-413a-8c86-fca81ceabc5c',
// metadata: { 'test-key': 'test-value' },
// createdOn: 2025-01-06T19:14:21.969Z,
// updatedOn: 2025-01-06T19:14:21.969Z,
// status: 'Processing',
// percentDone: null,
// signedUrl: null,
// errorMessage: null
// }
Lists all files that have been uploaded to an Assistant. Optionally, you can pass a filter to list only files that meet certain criteria.
import { Pinecone } from '@pinecone-database/pinecone';
const pc = new Pinecone();
const assistantName = 'test1';
const assistant = pc.Assistant(assistantName);
const files = await assistant.listFiles({
filter: { metadata: { key: 'value' } },
});
console.log(files);
// {
// files: [
// {
// name: 'test-file.txt',
// id: '1a56ddd0-c6d8-4295-80c0-9bfd6f5cb87b',
// metadata: [Object],
// createdOn: 2025-01-06T19:14:21.969Z,
// updatedOn: 2025-01-06T19:14:36.925Z,
// status: 'Available',
// percentDone: 1,
// signedUrl: undefined,
// errorMessage: undefined
// }
// ]
// }
Retrieves information about a file in an Assistant by ID.
import { Pinecone } from '@pinecone-database/pinecone';
const pc = new Pinecone();
const assistantName = 'test1';
const assistant = pc.Assistant(assistantName);
const files = await assistant.listFiles();
let fileId: string;
if (files.files) {
fileId = files.files[0].id;
} else {
fileId = '';
}
const resp = await assistant.describeFile({ fileId: fileId });
console.log(resp);
// {
// name: 'test-file.txt',
// id: '1a56ddd0-c6d8-4295-80c0-9bfd6f5cb87b',
// metadata: undefined,
// createdOn: 2025-01-06T19:14:21.969Z,
// updatedOn: 2025-01-06T19:14:36.925Z,
// status: 'Available',
// percentDone: 1,
// signedUrl: undefined,
// errorMessage: undefined
// }
Deletes a file(s) from an Assistant by ID.
Note: Deleting files is a PERMANENT operation. Deleted files cannot be recovered.
import { Pinecone } from '@pinecone-database/pinecone';
const pc = new Pinecone();
const assistantName = 'test1';
const assistant = pc.Assistant(assistantName);
const files = await assistant.listFiles();
let fileId: string;
if (files.files) {
fileId = files.files[0].id;
await assistant.deleteFile({ fileId: fileId });
}
All testing takes place automatically in CI and is configured using Github actions
and workflows, located in the .github
directory of this repo.
See CONTRIBUTING.md for more information.
For Tasks:
Click tags to check more tools for each tasksFor Jobs:
Alternative AI tools for pinecone-ts-client
Similar Open Source Tools
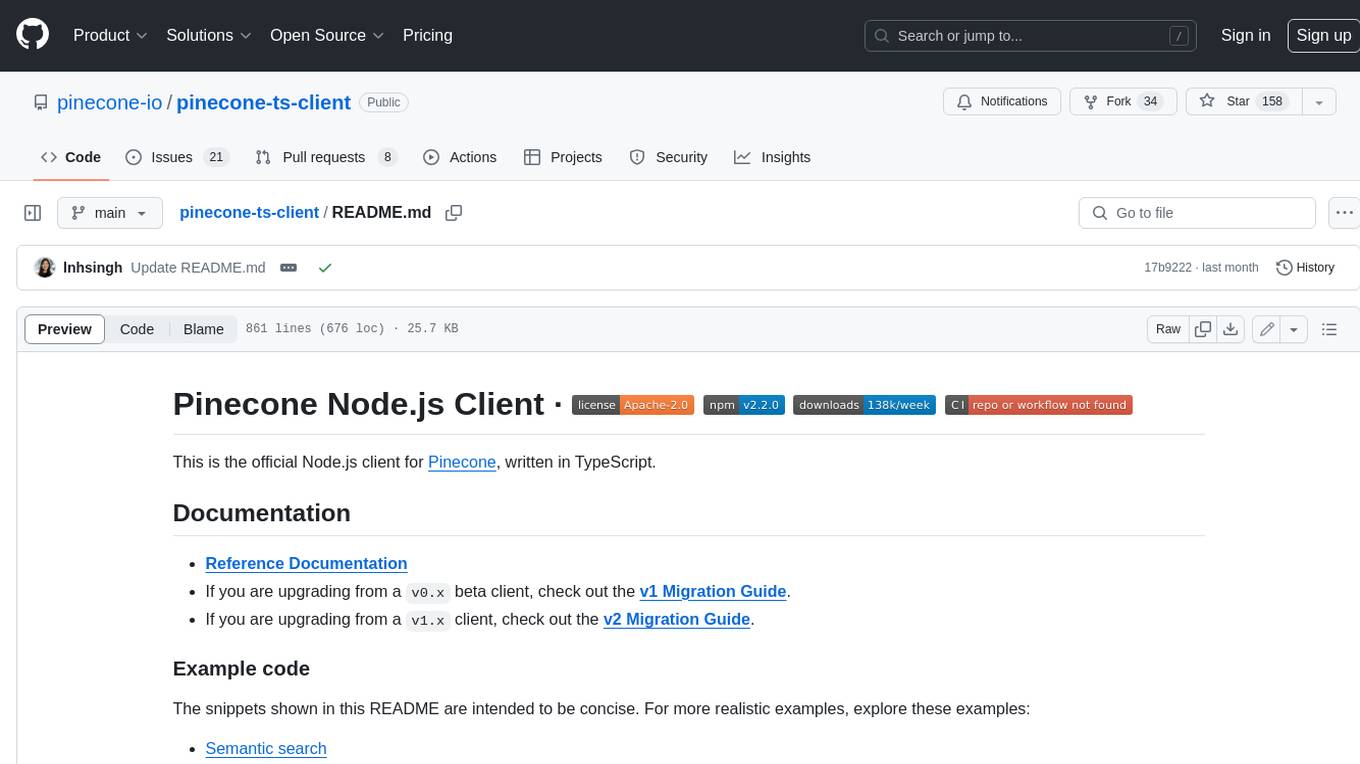
pinecone-ts-client
The official Node.js client for Pinecone, written in TypeScript. This client library provides a high-level interface for interacting with the Pinecone vector database service. With this client, you can create and manage indexes, upsert and query vector data, and perform other operations related to vector search and retrieval. The client is designed to be easy to use and provides a consistent and idiomatic experience for Node.js developers. It supports all the features and functionality of the Pinecone API, making it a comprehensive solution for building vector-powered applications in Node.js.
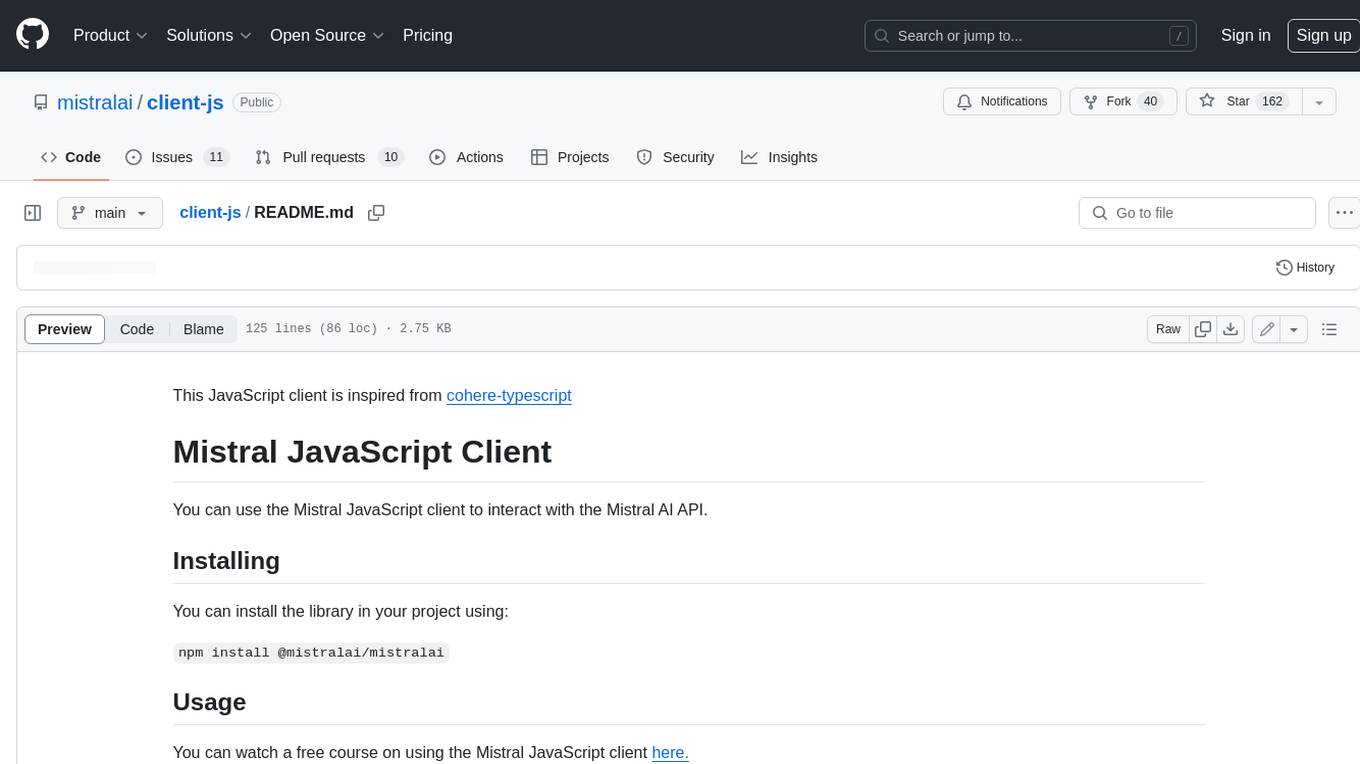
client-js
The Mistral JavaScript client is a library that allows you to interact with the Mistral AI API. With this client, you can perform various tasks such as listing models, chatting with streaming, chatting without streaming, and generating embeddings. To use the client, you can install it in your project using npm and then set up the client with your API key. Once the client is set up, you can use it to perform the desired tasks. For example, you can use the client to chat with a model by providing a list of messages. The client will then return the response from the model. You can also use the client to generate embeddings for a given input. The embeddings can then be used for various downstream tasks such as clustering or classification.
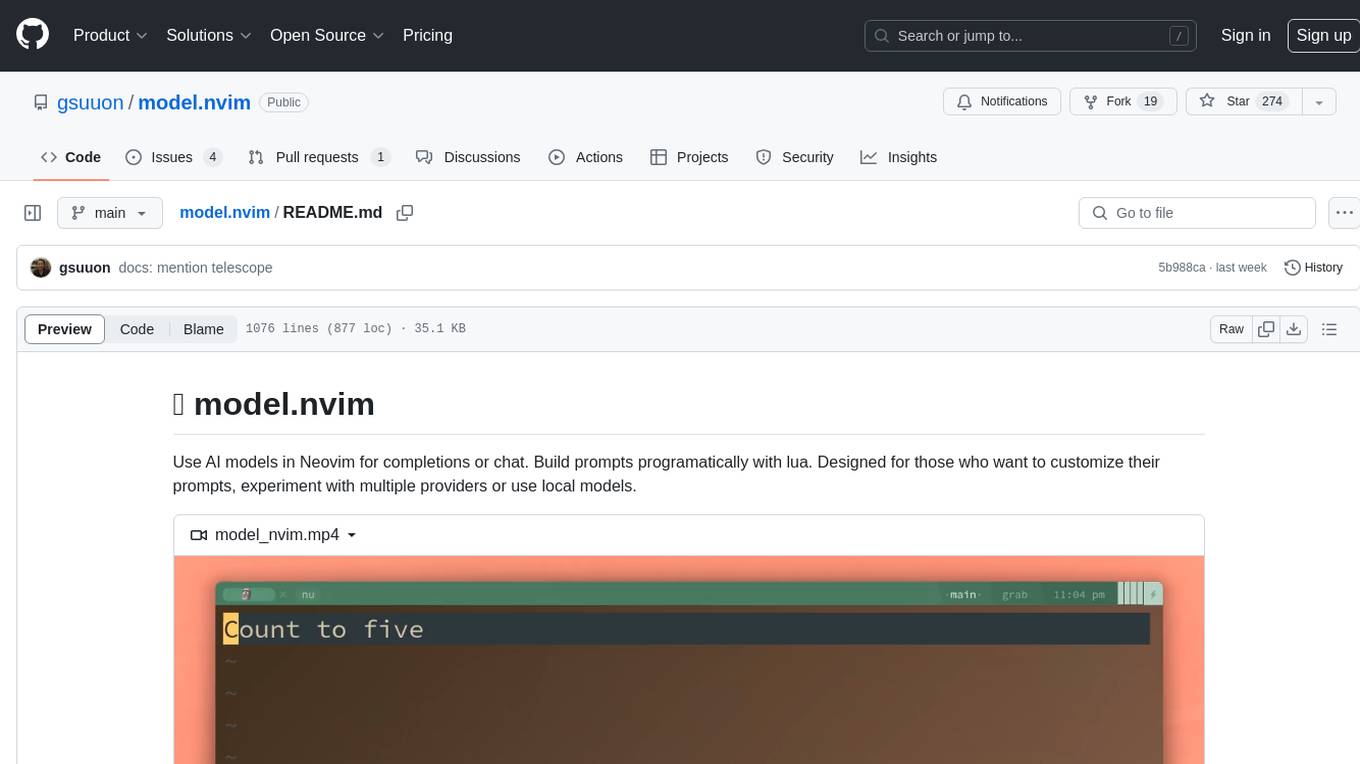
model.nvim
model.nvim is a tool designed for Neovim users who want to utilize AI models for completions or chat within their text editor. It allows users to build prompts programmatically with Lua, customize prompts, experiment with multiple providers, and use both hosted and local models. The tool supports features like provider agnosticism, programmatic prompts in Lua, async and multistep prompts, streaming completions, and chat functionality in 'mchat' filetype buffer. Users can customize prompts, manage responses, and context, and utilize various providers like OpenAI ChatGPT, Google PaLM, llama.cpp, ollama, and more. The tool also supports treesitter highlights and folds for chat buffers.
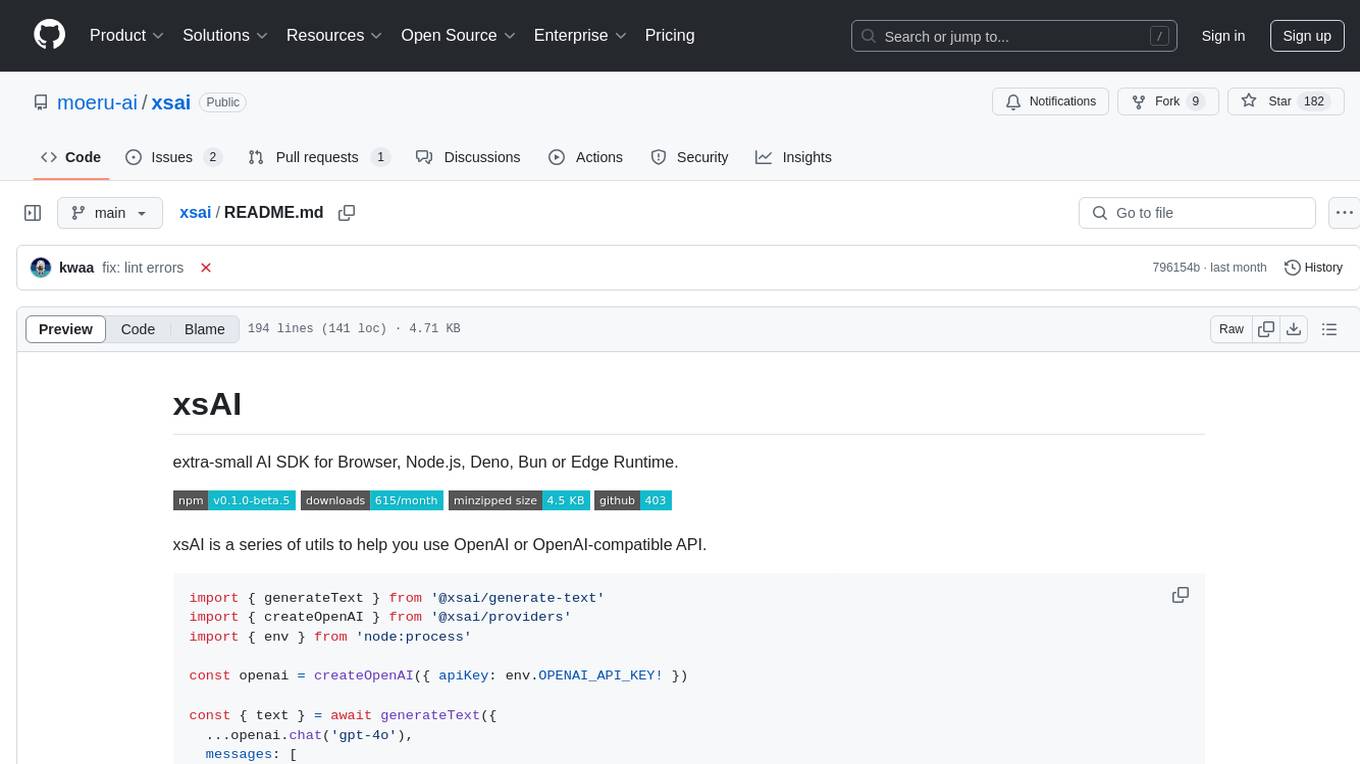
xsai
xsAI is an extra-small AI SDK designed for Browser, Node.js, Deno, Bun, or Edge Runtime. It provides a series of utils to help users utilize OpenAI or OpenAI-compatible APIs. The SDK is lightweight and efficient, using a variety of methods to minimize its size. It is runtime-agnostic, working seamlessly across different environments without depending on Node.js Built-in Modules. Users can easily install specific utils like generateText or streamText, and leverage tools like weather to perform tasks such as getting the weather in a location.
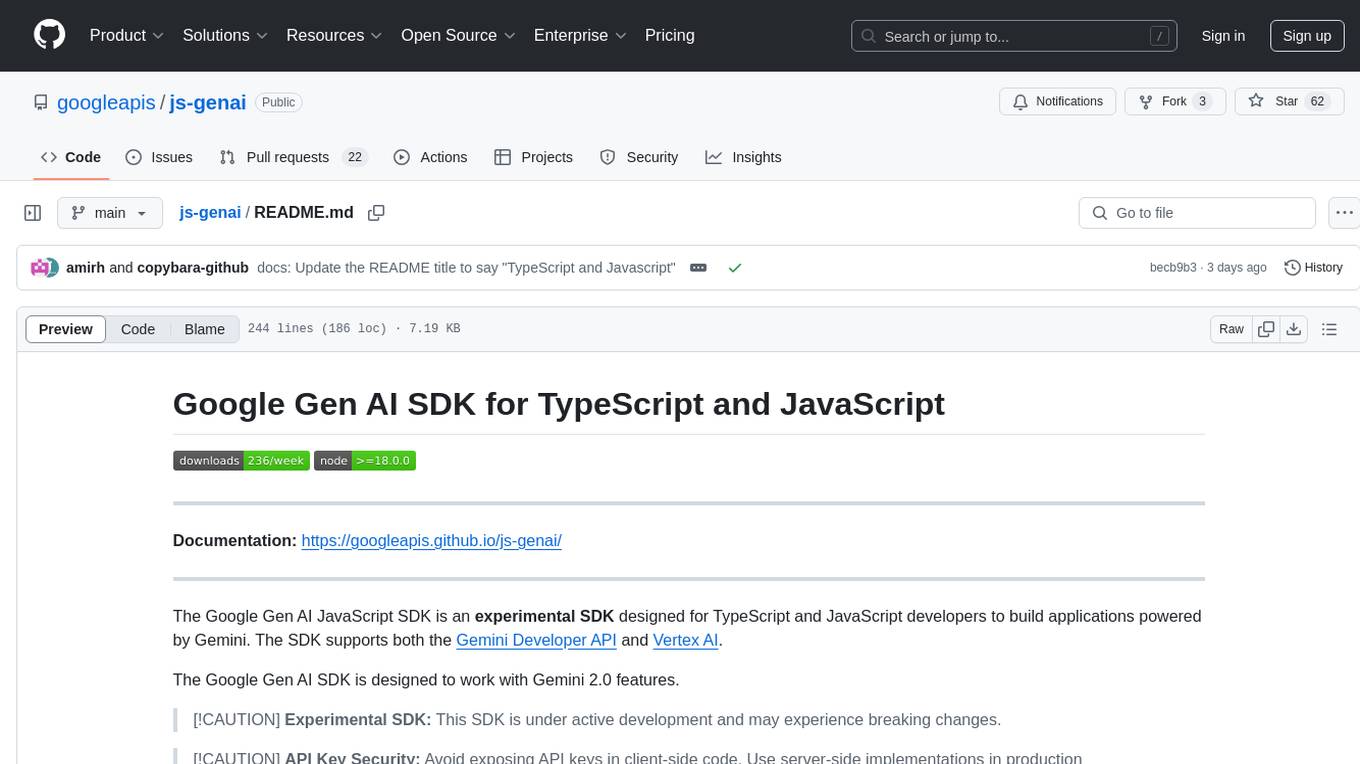
js-genai
The Google Gen AI JavaScript SDK is an experimental SDK for TypeScript and JavaScript developers to build applications powered by Gemini. It supports both the Gemini Developer API and Vertex AI. The SDK is designed to work with Gemini 2.0 features. Users can access API features through the GoogleGenAI classes, which provide submodules for querying models, managing caches, creating chats, uploading files, and starting live sessions. The SDK also allows for function calling to interact with external systems. Users can find more samples in the GitHub samples directory.
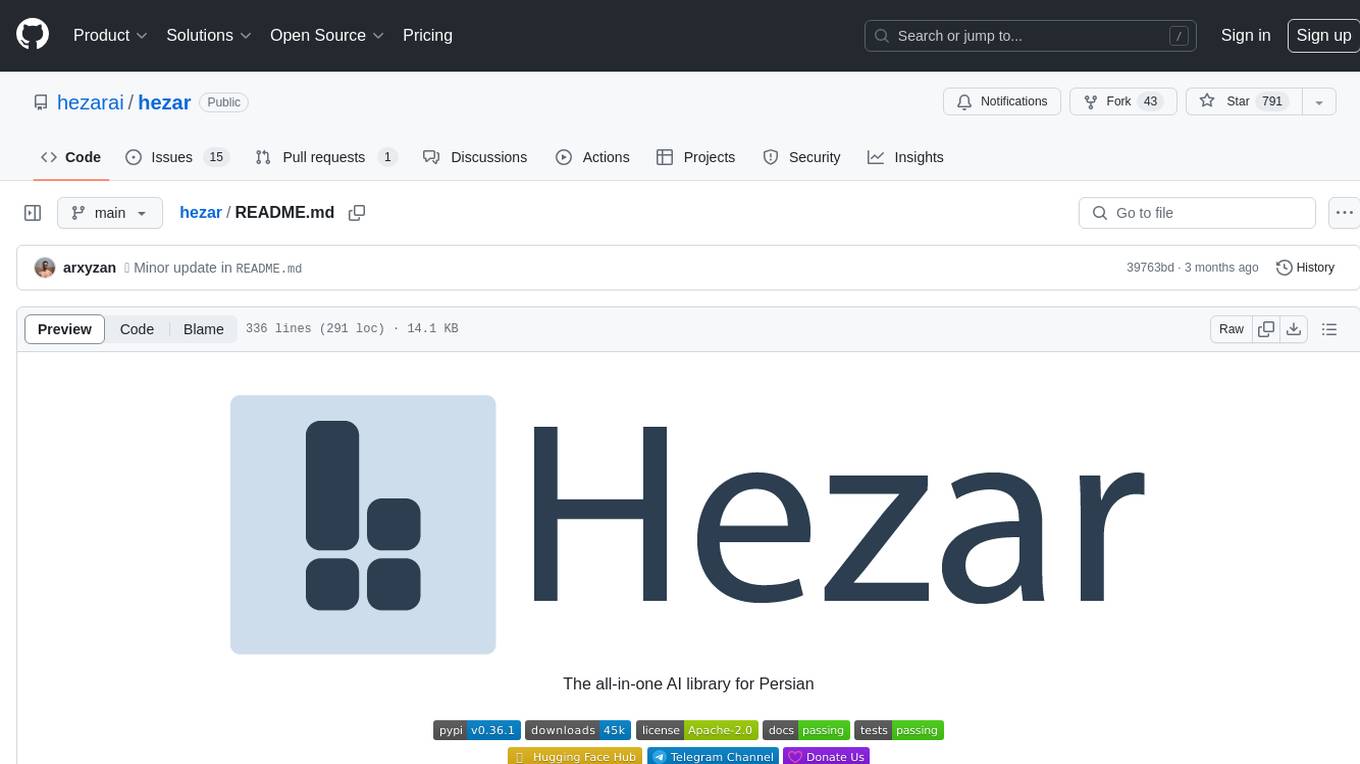
hezar
Hezar is an all-in-one AI library designed specifically for the Persian community. It brings together various AI models and tools, making it easy to use AI with just a few lines of code. The library seamlessly integrates with Hugging Face Hub, offering a developer-friendly interface and task-based model interface. In addition to models, Hezar provides tools like word embeddings, tokenizers, feature extractors, and more. It also includes supplementary ML tools for deployment, benchmarking, and optimization.
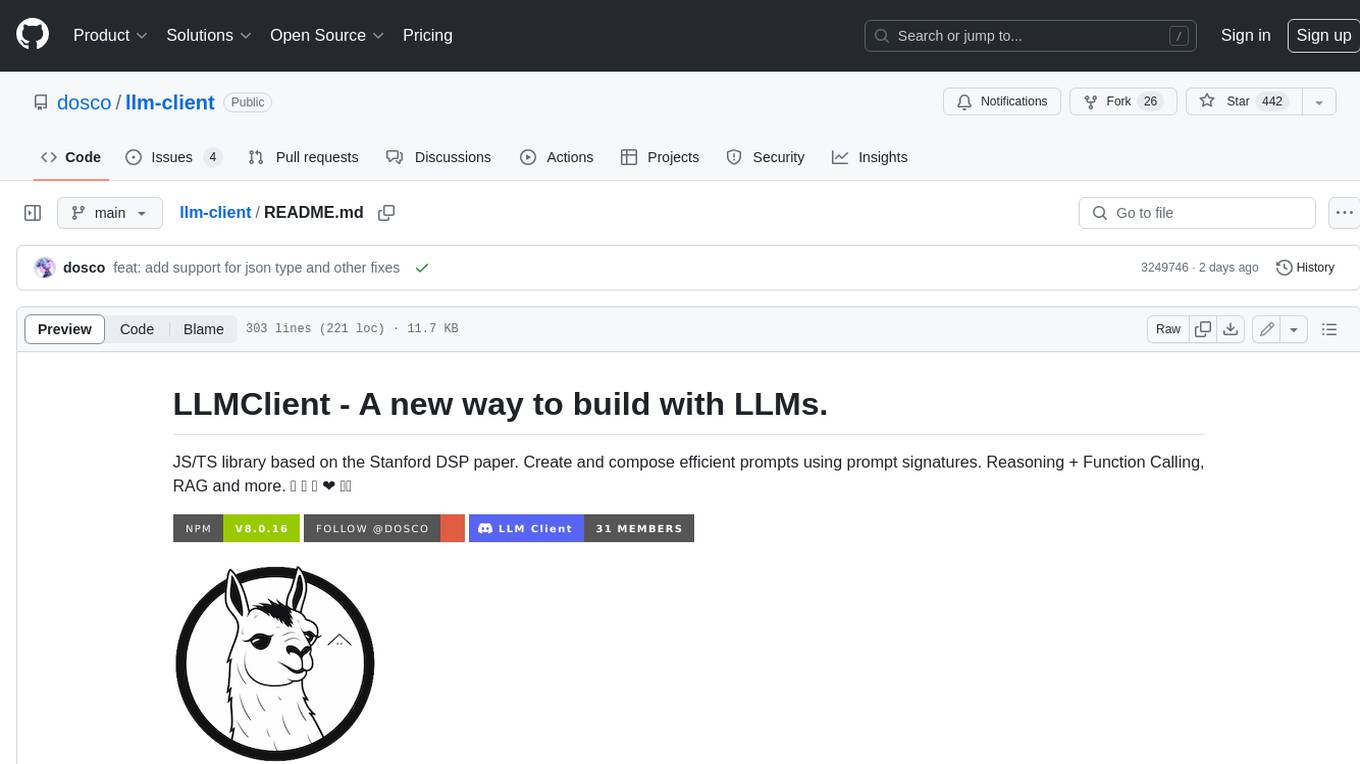
llm-client
LLMClient is a JavaScript/TypeScript library that simplifies working with large language models (LLMs) by providing an easy-to-use interface for building and composing efficient prompts using prompt signatures. These signatures enable the automatic generation of typed prompts, allowing developers to leverage advanced capabilities like reasoning, function calling, RAG, ReAcT, and Chain of Thought. The library supports various LLMs and vector databases, making it a versatile tool for a wide range of applications.
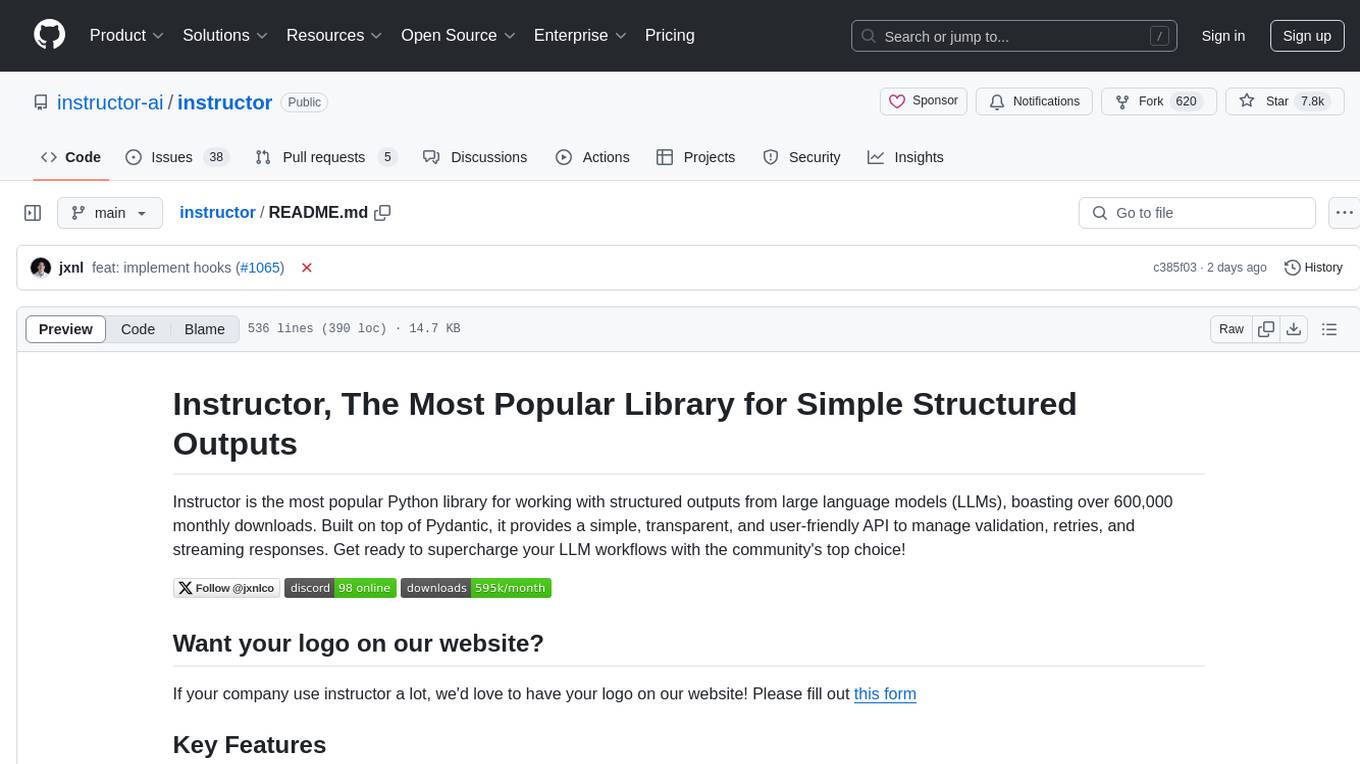
instructor
Instructor is a popular Python library for managing structured outputs from large language models (LLMs). It offers a user-friendly API for validation, retries, and streaming responses. With support for various LLM providers and multiple languages, Instructor simplifies working with LLM outputs. The library includes features like response models, retry management, validation, streaming support, and flexible backends. It also provides hooks for logging and monitoring LLM interactions, and supports integration with Anthropic, Cohere, Gemini, Litellm, and Google AI models. Instructor facilitates tasks such as extracting user data from natural language, creating fine-tuned models, managing uploaded files, and monitoring usage of OpenAI models.
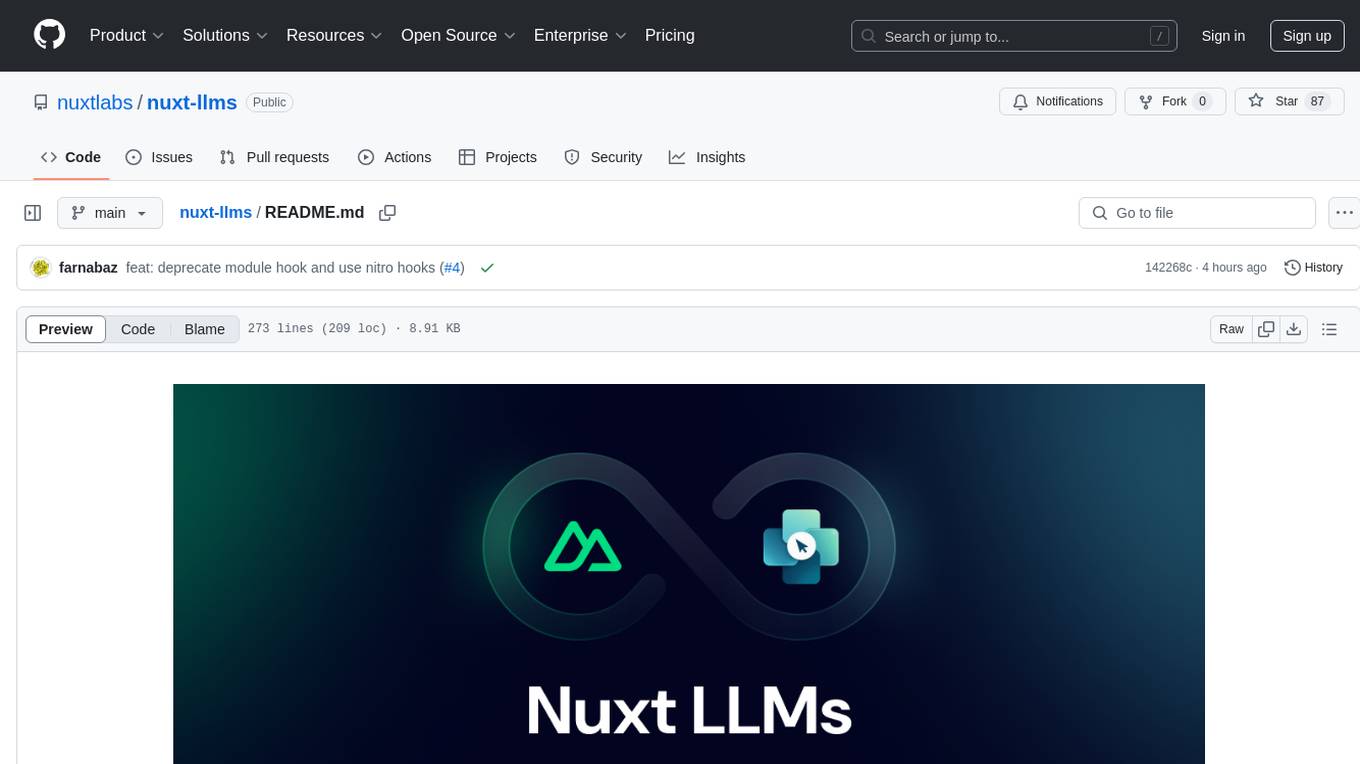
nuxt-llms
Nuxt LLMs automatically generates llms.txt markdown documentation for Nuxt applications. It provides runtime hooks to collect data from various sources and generate structured documentation. The tool allows customization of sections directly from nuxt.config.ts and integrates with Nuxt modules via the runtime hooks system. It generates two documentation formats: llms.txt for concise structured documentation and llms_full.txt for detailed documentation. Users can extend documentation using hooks to add sections, links, and metadata. The tool is suitable for developers looking to automate documentation generation for their Nuxt applications.
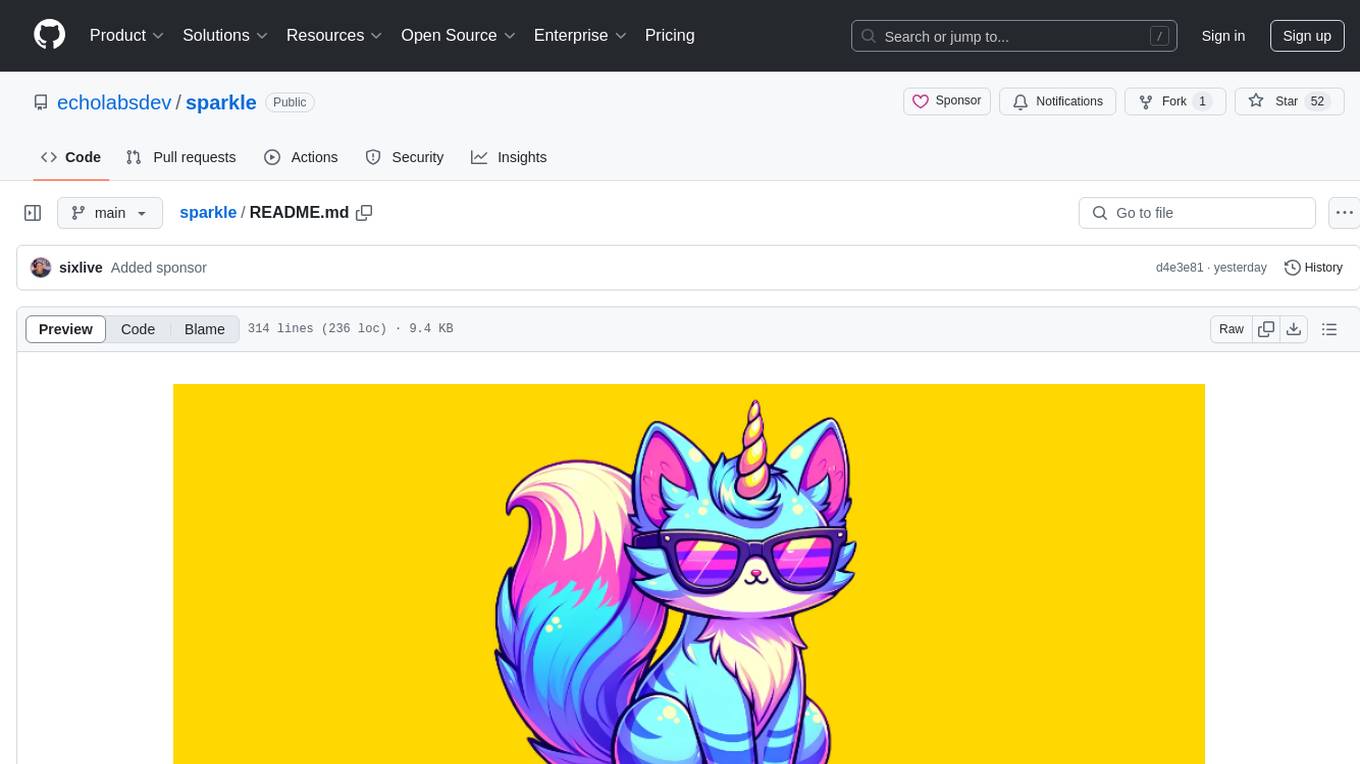
sparkle
Sparkle is a tool that streamlines the process of building AI-driven features in applications using Large Language Models (LLMs). It guides users through creating and managing agents, defining tools, and interacting with LLM providers like OpenAI. Sparkle allows customization of LLM provider settings, model configurations, and provides a seamless integration with Sparkle Server for exposing agents via an OpenAI-compatible chat API endpoint.
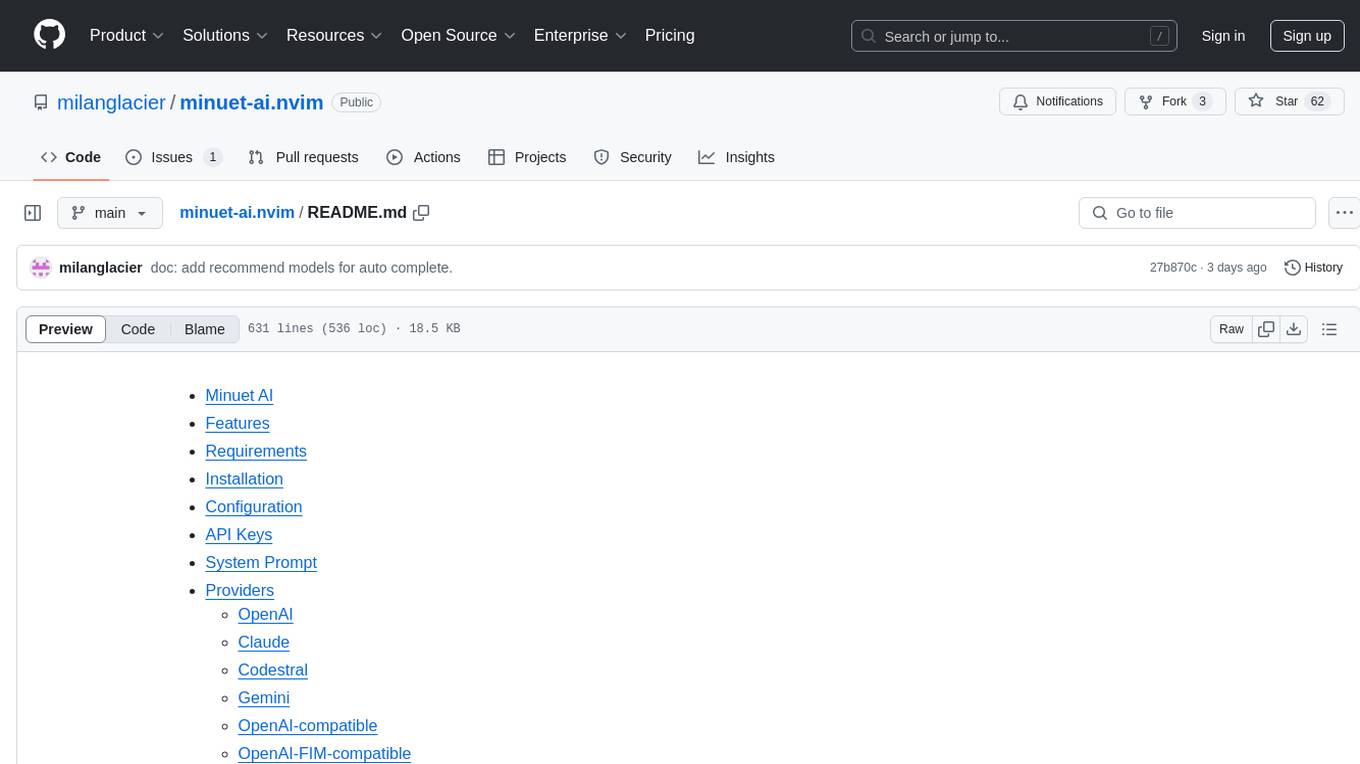
minuet-ai.nvim
Minuet AI is a Neovim plugin that integrates with nvim-cmp to provide AI-powered code completion using multiple AI providers such as OpenAI, Claude, Gemini, Codestral, and Huggingface. It offers customizable configuration options and streaming support for completion delivery. Users can manually invoke completion or use cost-effective models for auto-completion. The plugin requires API keys for supported AI providers and allows customization of system prompts. Minuet AI also supports changing providers, toggling auto-completion, and provides solutions for input delay issues. Integration with lazyvim is possible, and future plans include implementing RAG on the codebase and virtual text UI support.
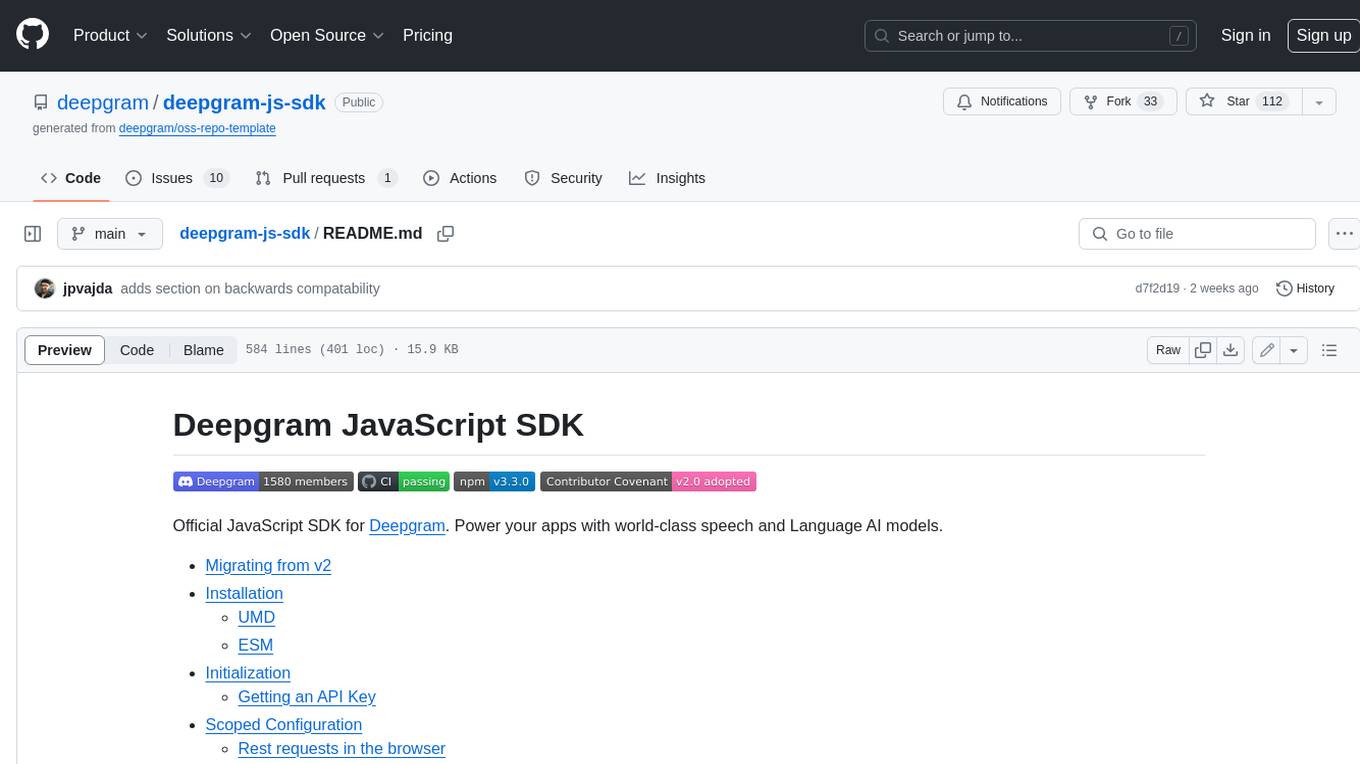
deepgram-js-sdk
Deepgram JavaScript SDK. Power your apps with world-class speech and Language AI models.
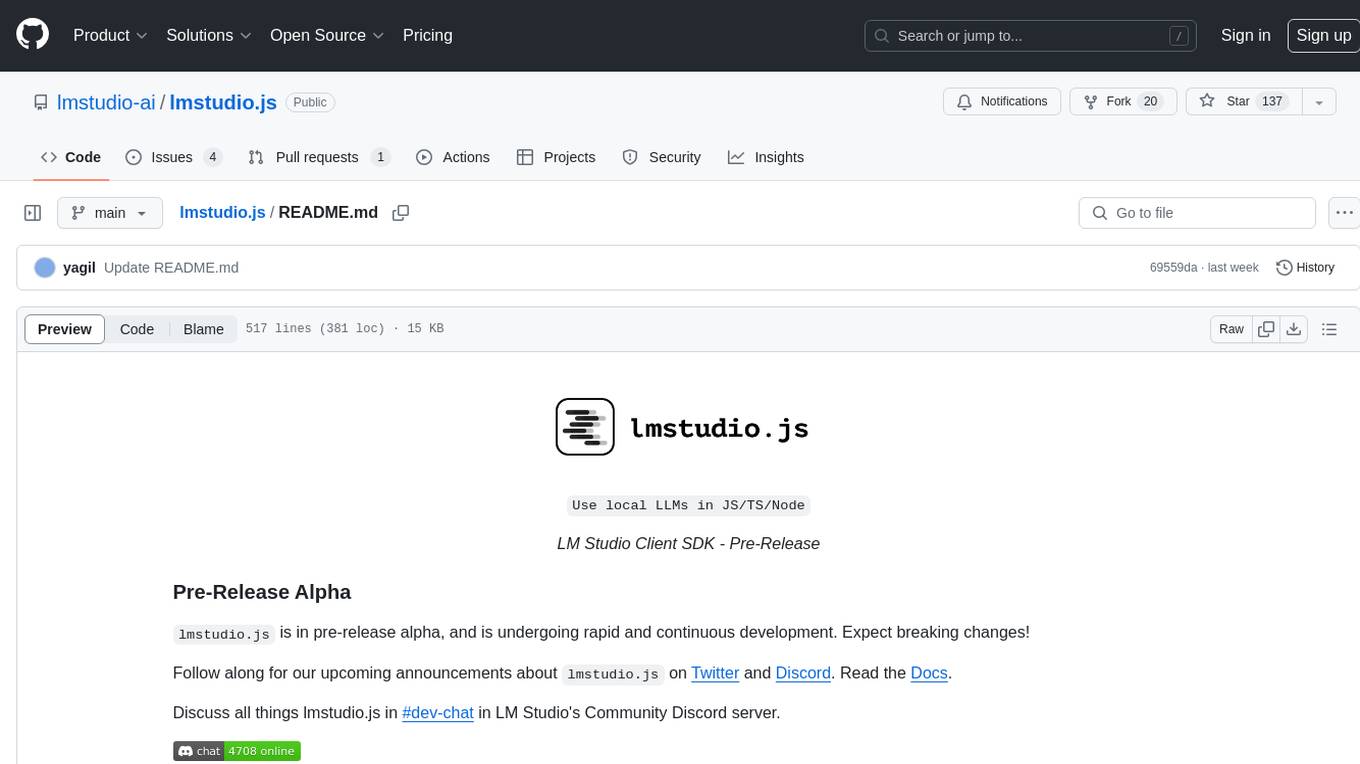
lmstudio.js
lmstudio.js is a pre-release alpha client SDK for LM Studio, allowing users to use local LLMs in JS/TS/Node. It is currently undergoing rapid development with breaking changes expected. Users can follow LM Studio's announcements on Twitter and Discord. The SDK provides API usage for loading models, predicting text, setting up the local LLM server, and more. It supports features like custom loading progress tracking, model unloading, structured output prediction, and cancellation of predictions. Users can interact with LM Studio through the CLI tool 'lms' and perform tasks like text completion, conversation, and getting prediction statistics.
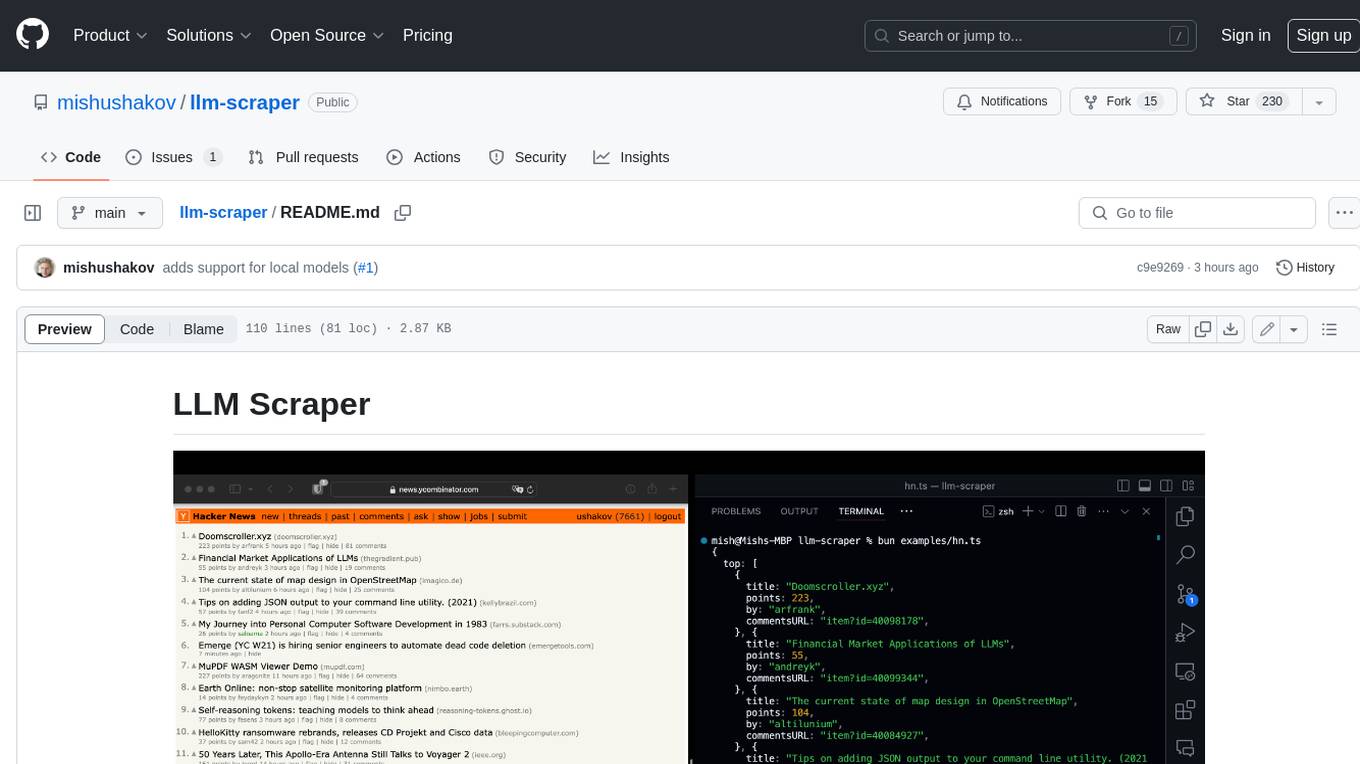
llm-scraper
LLM Scraper is a TypeScript library that allows you to convert any webpages into structured data using LLMs. It supports Local (GGUF), OpenAI, Groq chat models, and schemas defined with Zod. With full type-safety in TypeScript and based on the Playwright framework, it offers streaming when crawling multiple pages and supports four input modes: html, markdown, text, and image.
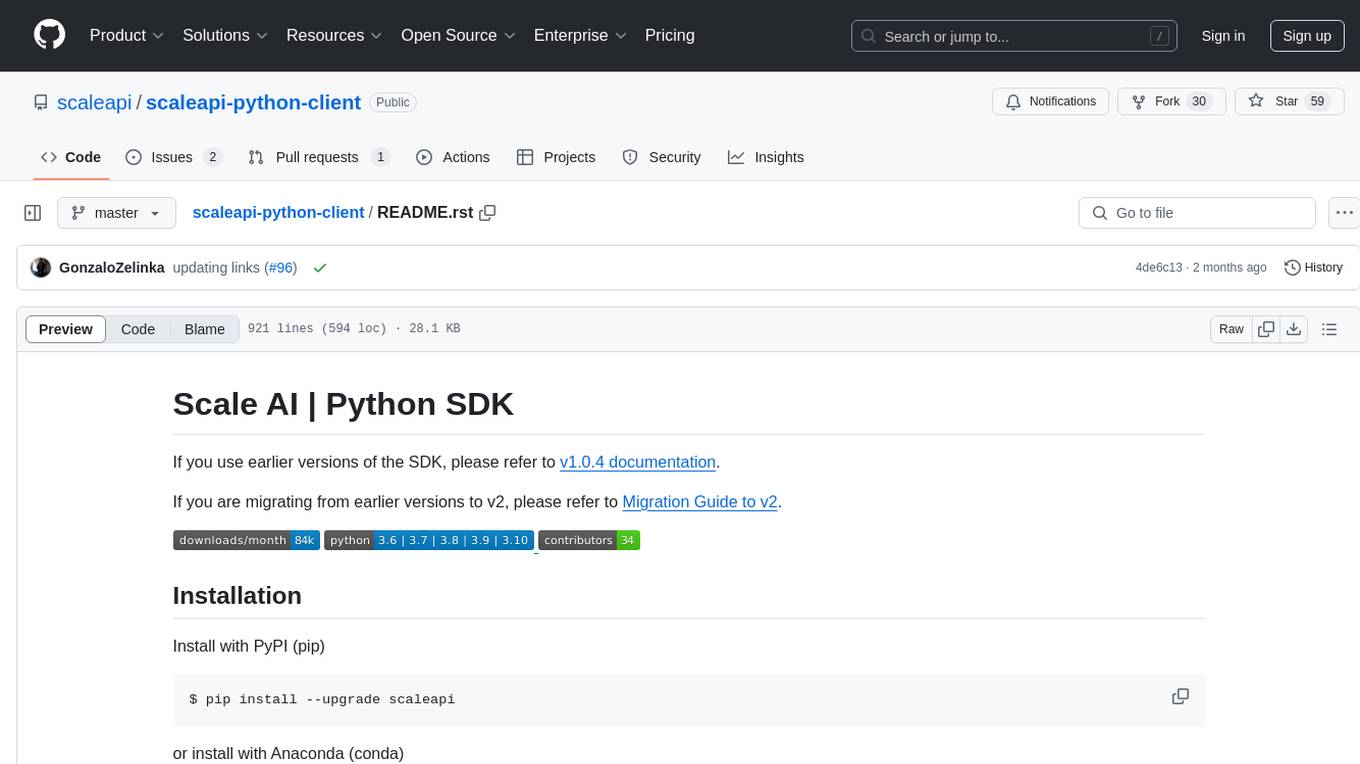
scaleapi-python-client
The Scale AI Python SDK is a tool that provides a Python interface for interacting with the Scale API. It allows users to easily create tasks, manage projects, upload files, and work with evaluation tasks, training tasks, and Studio assignments. The SDK handles error handling and provides detailed documentation for each method. Users can also manage teammates, project groups, and batches within the Scale Studio environment. The SDK supports various functionalities such as creating tasks, retrieving tasks, canceling tasks, auditing tasks, updating task attributes, managing files, managing team members, and working with evaluation and training tasks.
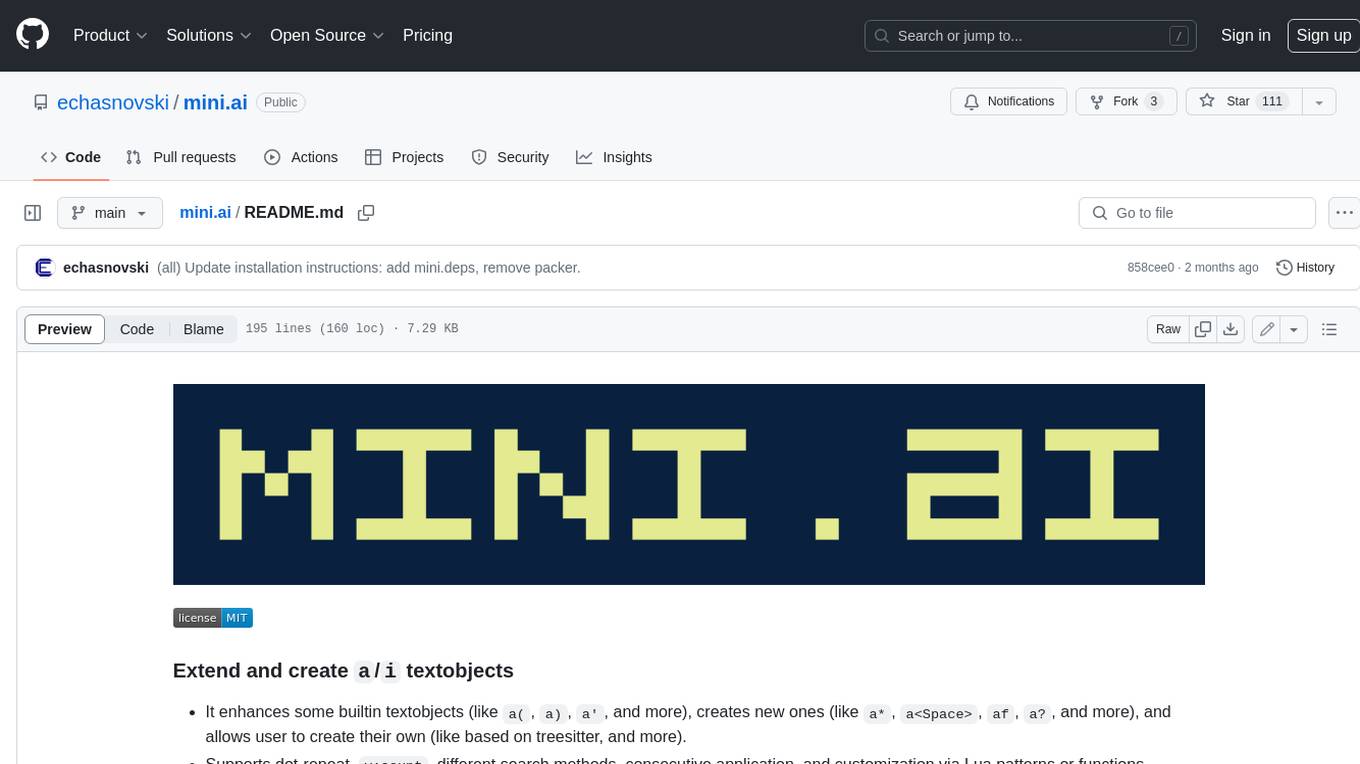
mini.ai
This plugin extends and creates `a`/`i` textobjects in Neovim. It enhances some builtin textobjects (like `a(`, `a)`, `a'`, and more), creates new ones (like `a*`, `a
For similar tasks
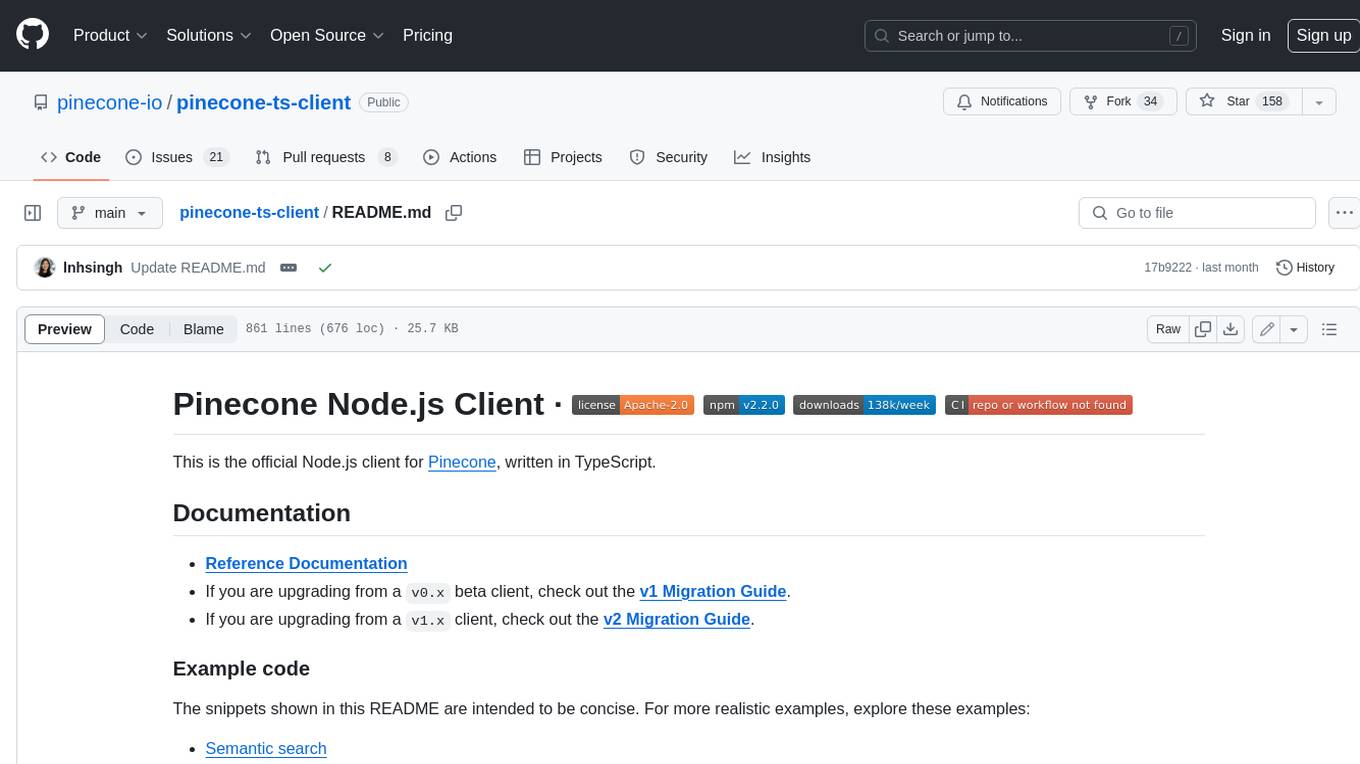
pinecone-ts-client
The official Node.js client for Pinecone, written in TypeScript. This client library provides a high-level interface for interacting with the Pinecone vector database service. With this client, you can create and manage indexes, upsert and query vector data, and perform other operations related to vector search and retrieval. The client is designed to be easy to use and provides a consistent and idiomatic experience for Node.js developers. It supports all the features and functionality of the Pinecone API, making it a comprehensive solution for building vector-powered applications in Node.js.
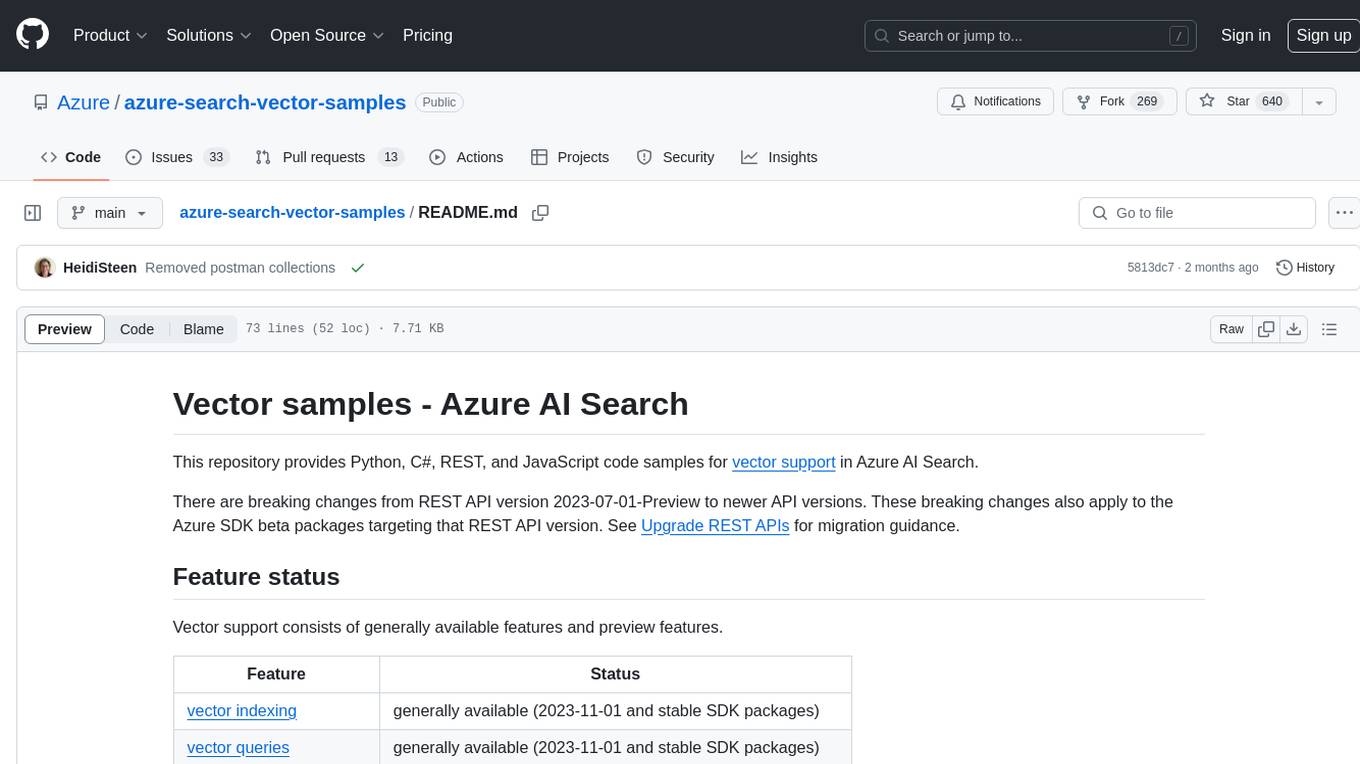
azure-search-vector-samples
This repository provides code samples in Python, C#, REST, and JavaScript for vector support in Azure AI Search. It includes demos for various languages showcasing vectorization of data, creating indexes, and querying vector data. Additionally, it offers tools like Azure AI Search Lab for experimenting with AI-enabled search scenarios in Azure and templates for deploying custom chat-with-your-data solutions. The repository also features documentation on vector search, hybrid search, creating and querying vector indexes, and REST API references for Azure AI Search and Azure OpenAI Service.
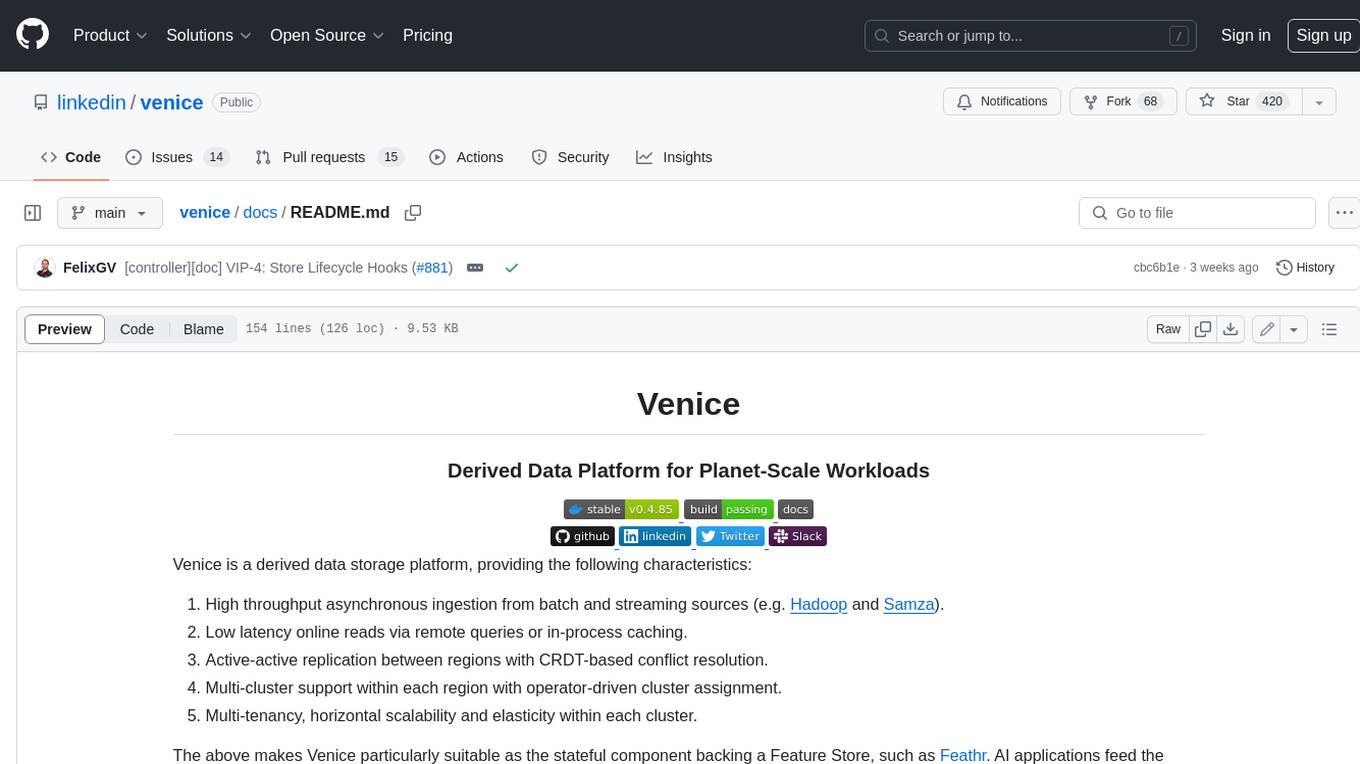
venice
Venice is a derived data storage platform, providing the following characteristics: 1. High throughput asynchronous ingestion from batch and streaming sources (e.g. Hadoop and Samza). 2. Low latency online reads via remote queries or in-process caching. 3. Active-active replication between regions with CRDT-based conflict resolution. 4. Multi-cluster support within each region with operator-driven cluster assignment. 5. Multi-tenancy, horizontal scalability and elasticity within each cluster. The above makes Venice particularly suitable as the stateful component backing a Feature Store, such as Feathr. AI applications feed the output of their ML training jobs into Venice and then query the data for use during online inference workloads.
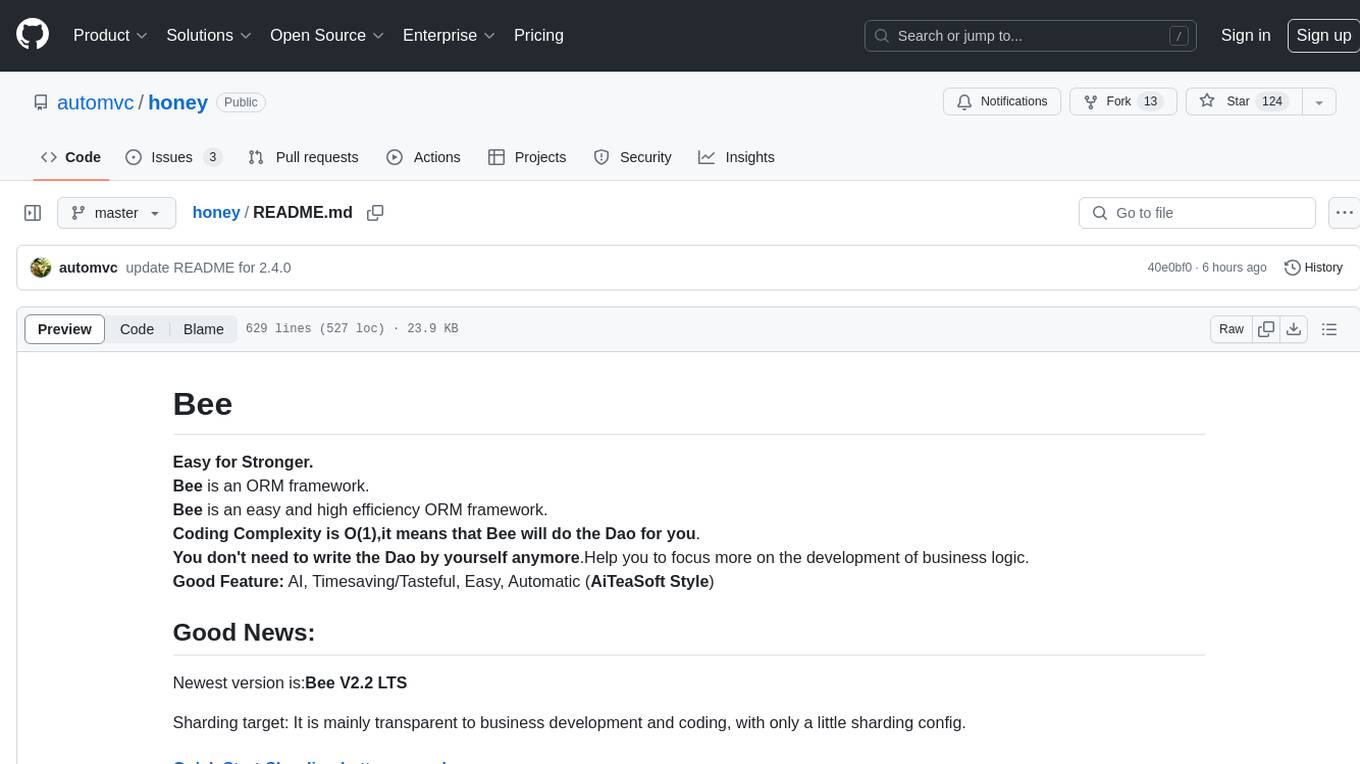
honey
Bee is an ORM framework that provides easy and high-efficiency database operations, allowing developers to focus on business logic development. It supports various databases and features like automatic filtering, partial field queries, pagination, and JSON format results. Bee also offers advanced functionalities like sharding, transactions, complex queries, and MongoDB ORM. The tool is designed for rapid application development in Java, offering faster development for Java Web and Spring Cloud microservices. The Enterprise Edition provides additional features like financial computing support, automatic value insertion, desensitization, dictionary value conversion, multi-tenancy, and more.
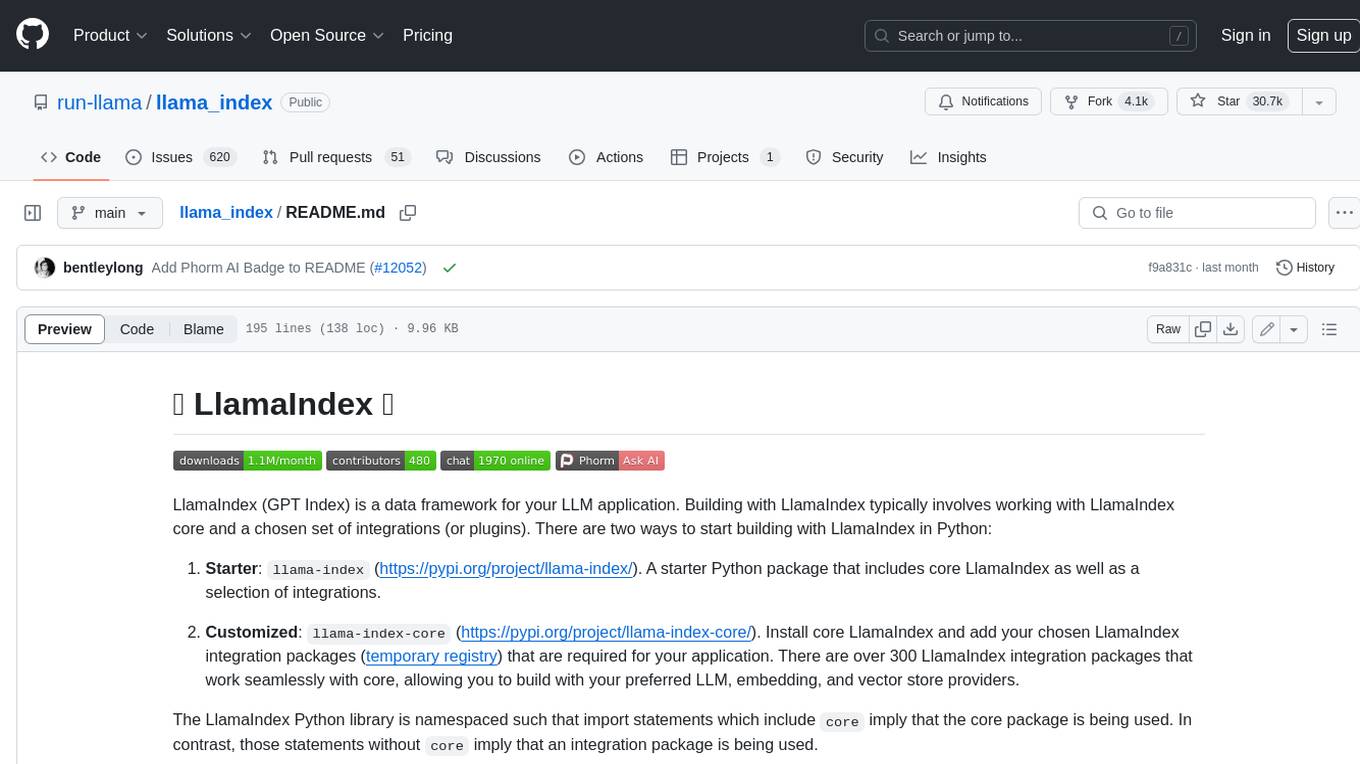
llama_index
LlamaIndex is a data framework for building LLM applications. It provides tools for ingesting, structuring, and querying data, as well as integrating with LLMs and other tools. LlamaIndex is designed to be easy to use for both beginner and advanced users, and it provides a comprehensive set of features for building LLM applications.
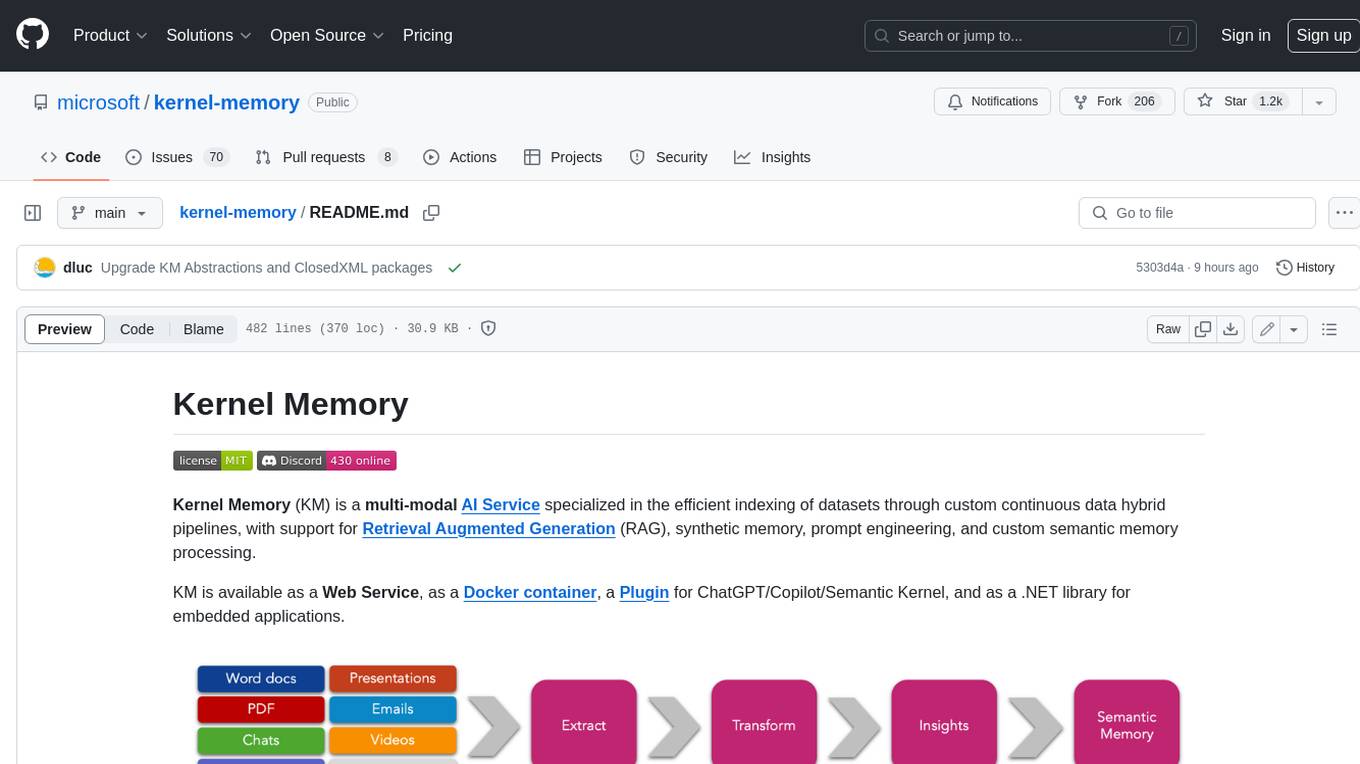
kernel-memory
Kernel Memory (KM) is a multi-modal AI Service specialized in the efficient indexing of datasets through custom continuous data hybrid pipelines, with support for Retrieval Augmented Generation (RAG), synthetic memory, prompt engineering, and custom semantic memory processing. KM is available as a Web Service, as a Docker container, a Plugin for ChatGPT/Copilot/Semantic Kernel, and as a .NET library for embedded applications. Utilizing advanced embeddings and LLMs, the system enables Natural Language querying for obtaining answers from the indexed data, complete with citations and links to the original sources. Designed for seamless integration as a Plugin with Semantic Kernel, Microsoft Copilot and ChatGPT, Kernel Memory enhances data-driven features in applications built for most popular AI platforms.
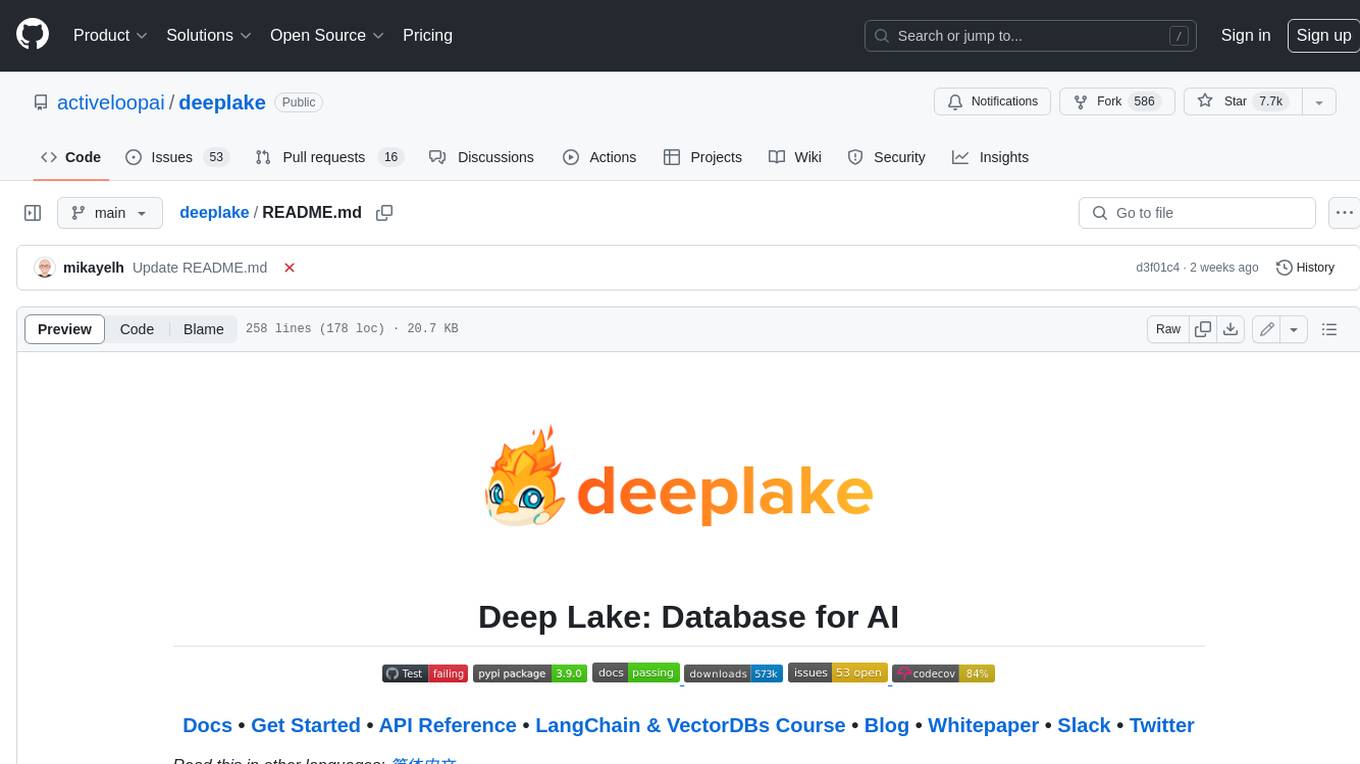
deeplake
Deep Lake is a Database for AI powered by a storage format optimized for deep-learning applications. Deep Lake can be used for: 1. Storing data and vectors while building LLM applications 2. Managing datasets while training deep learning models Deep Lake simplifies the deployment of enterprise-grade LLM-based products by offering storage for all data types (embeddings, audio, text, videos, images, pdfs, annotations, etc.), querying and vector search, data streaming while training models at scale, data versioning and lineage, and integrations with popular tools such as LangChain, LlamaIndex, Weights & Biases, and many more. Deep Lake works with data of any size, it is serverless, and it enables you to store all of your data in your own cloud and in one place. Deep Lake is used by Intel, Bayer Radiology, Matterport, ZERO Systems, Red Cross, Yale, & Oxford.
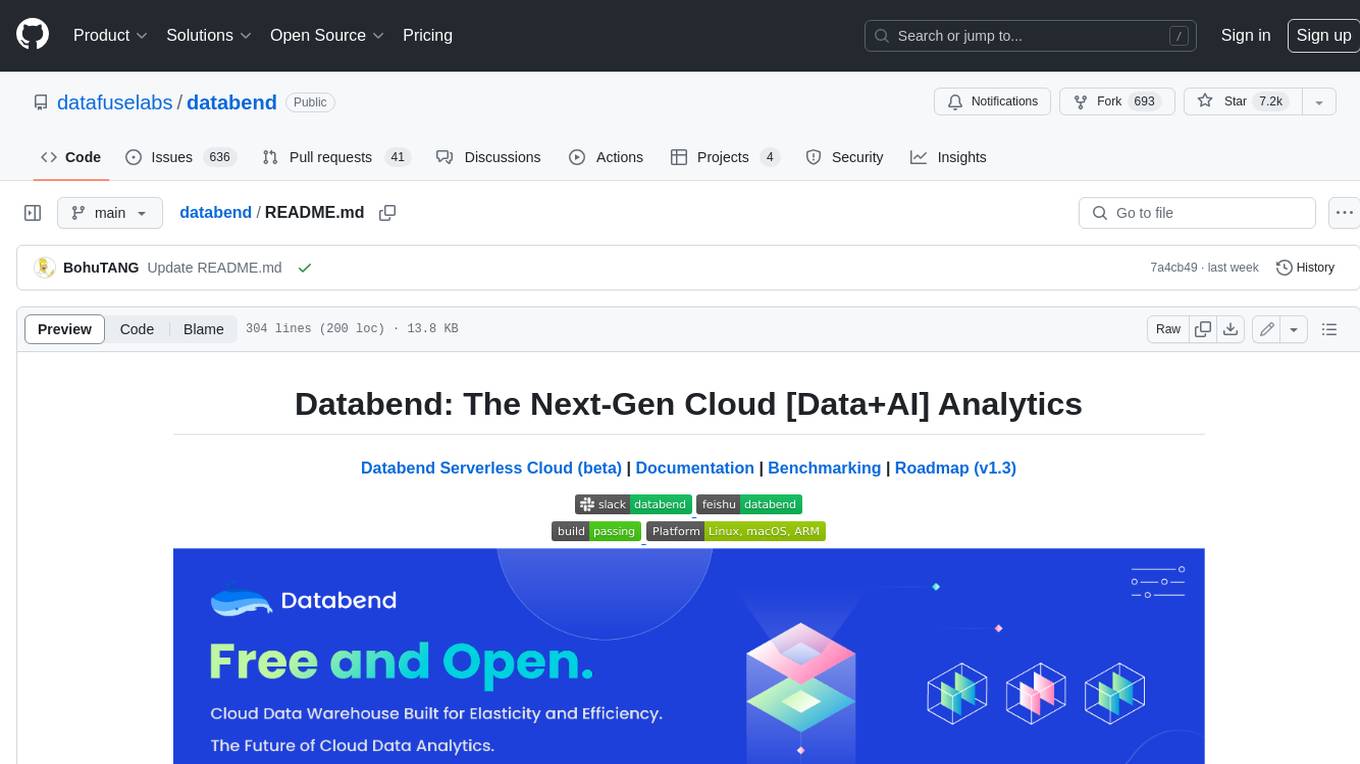
databend
Databend is an open-source cloud data warehouse that serves as a cost-effective alternative to Snowflake. With its focus on fast query execution and data ingestion, it's designed for complex analysis of the world's largest datasets.
For similar jobs
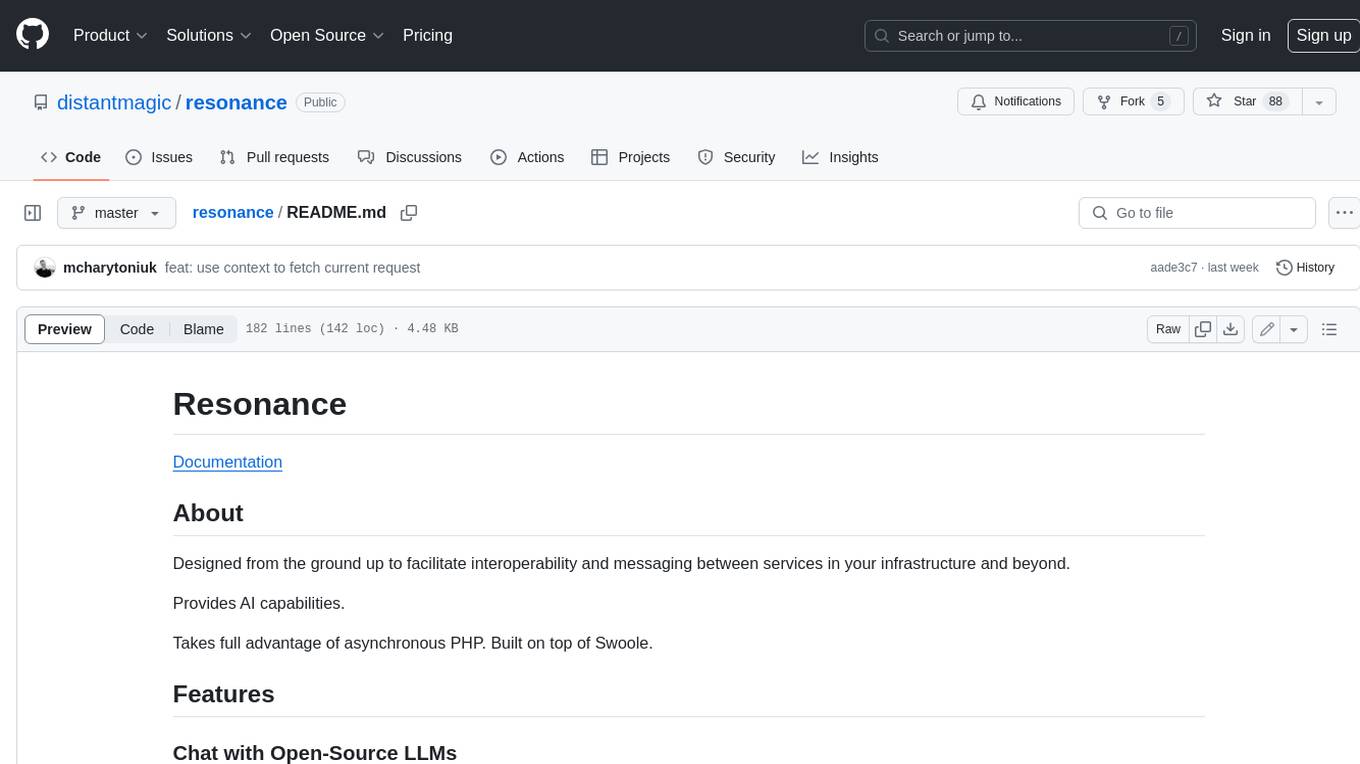
resonance
Resonance is a framework designed to facilitate interoperability and messaging between services in your infrastructure and beyond. It provides AI capabilities and takes full advantage of asynchronous PHP, built on top of Swoole. With Resonance, you can: * Chat with Open-Source LLMs: Create prompt controllers to directly answer user's prompts. LLM takes care of determining user's intention, so you can focus on taking appropriate action. * Asynchronous Where it Matters: Respond asynchronously to incoming RPC or WebSocket messages (or both combined) with little overhead. You can set up all the asynchronous features using attributes. No elaborate configuration is needed. * Simple Things Remain Simple: Writing HTTP controllers is similar to how it's done in the synchronous code. Controllers have new exciting features that take advantage of the asynchronous environment. * Consistency is Key: You can keep the same approach to writing software no matter the size of your project. There are no growing central configuration files or service dependencies registries. Every relation between code modules is local to those modules. * Promises in PHP: Resonance provides a partial implementation of Promise/A+ spec to handle various asynchronous tasks. * GraphQL Out of the Box: You can build elaborate GraphQL schemas by using just the PHP attributes. Resonance takes care of reusing SQL queries and optimizing the resources' usage. All fields can be resolved asynchronously.
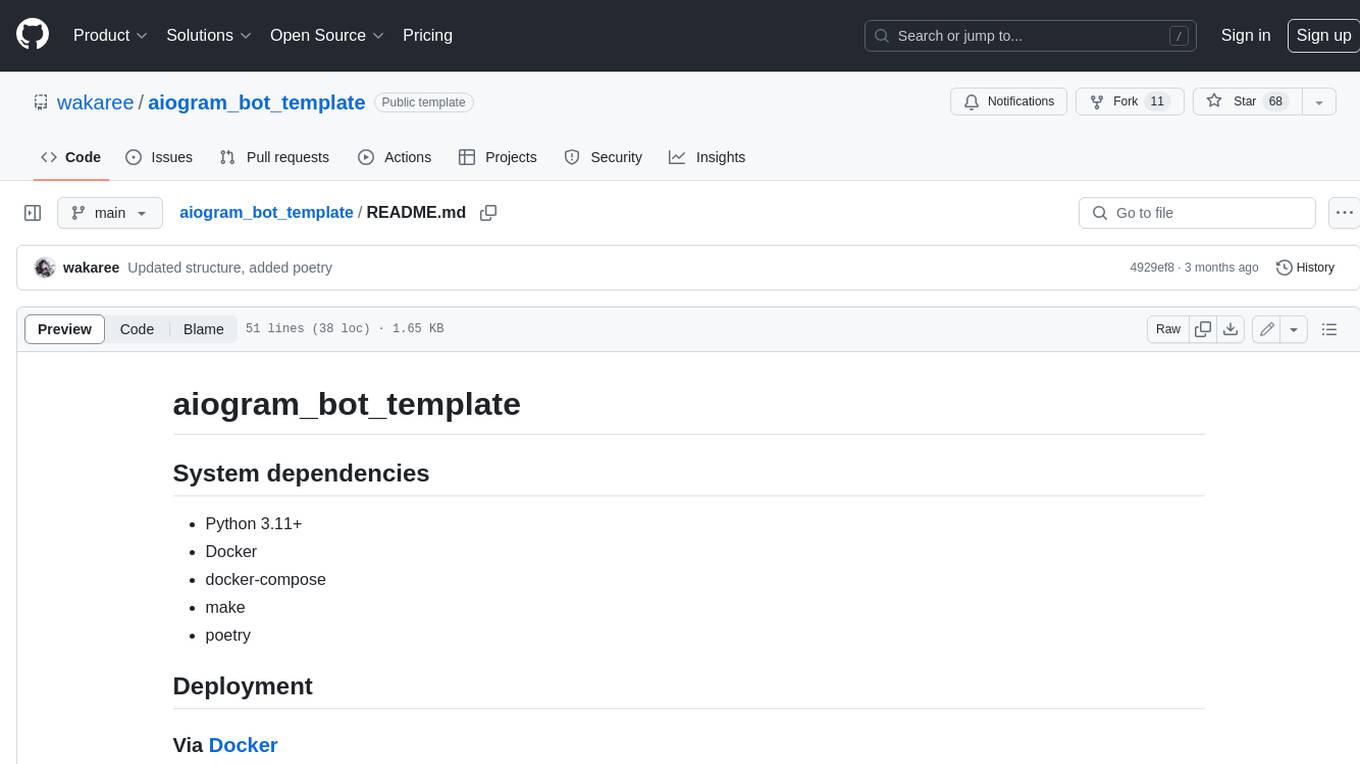
aiogram_bot_template
Aiogram bot template is a boilerplate for creating Telegram bots using Aiogram framework. It provides a solid foundation for building robust and scalable bots with a focus on code organization, database integration, and localization.
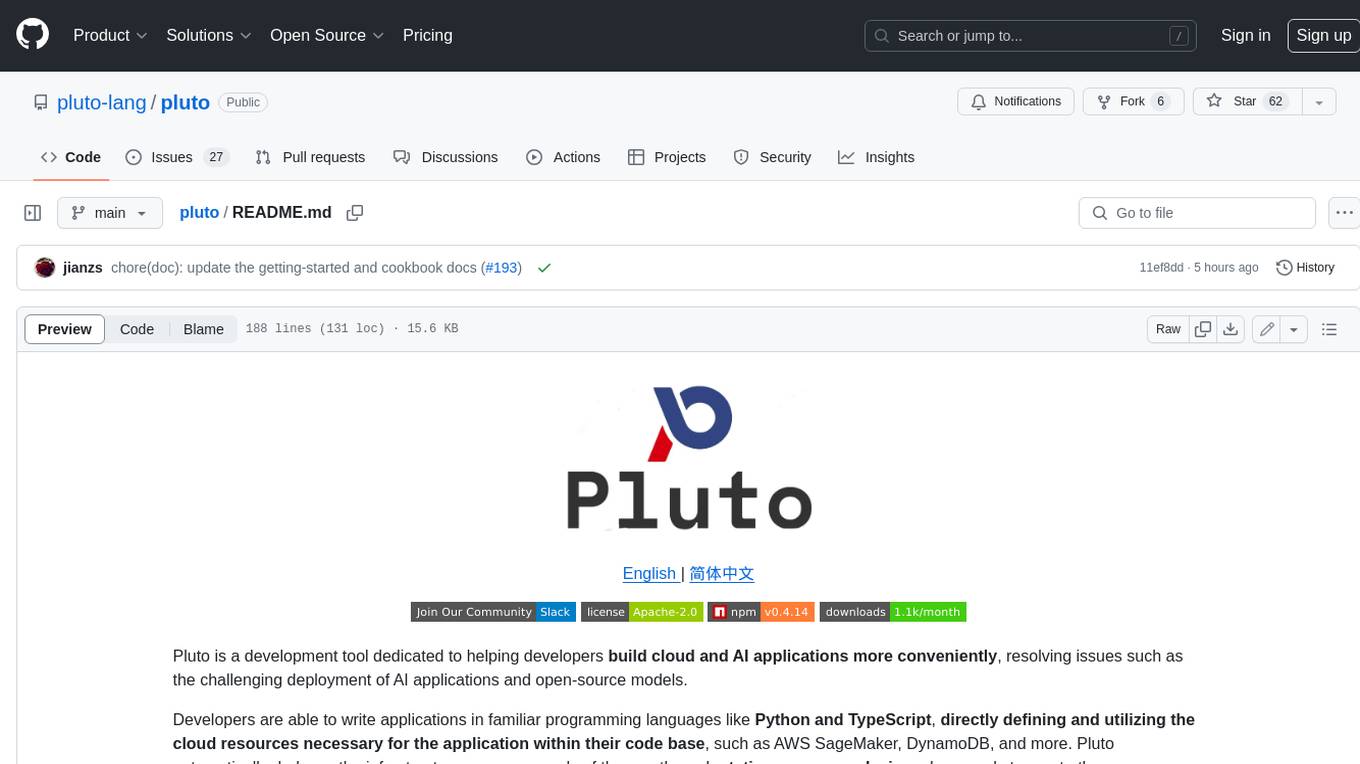
pluto
Pluto is a development tool dedicated to helping developers **build cloud and AI applications more conveniently** , resolving issues such as the challenging deployment of AI applications and open-source models. Developers are able to write applications in familiar programming languages like **Python and TypeScript** , **directly defining and utilizing the cloud resources necessary for the application within their code base** , such as AWS SageMaker, DynamoDB, and more. Pluto automatically deduces the infrastructure resource needs of the app through **static program analysis** and proceeds to create these resources on the specified cloud platform, **simplifying the resources creation and application deployment process**.
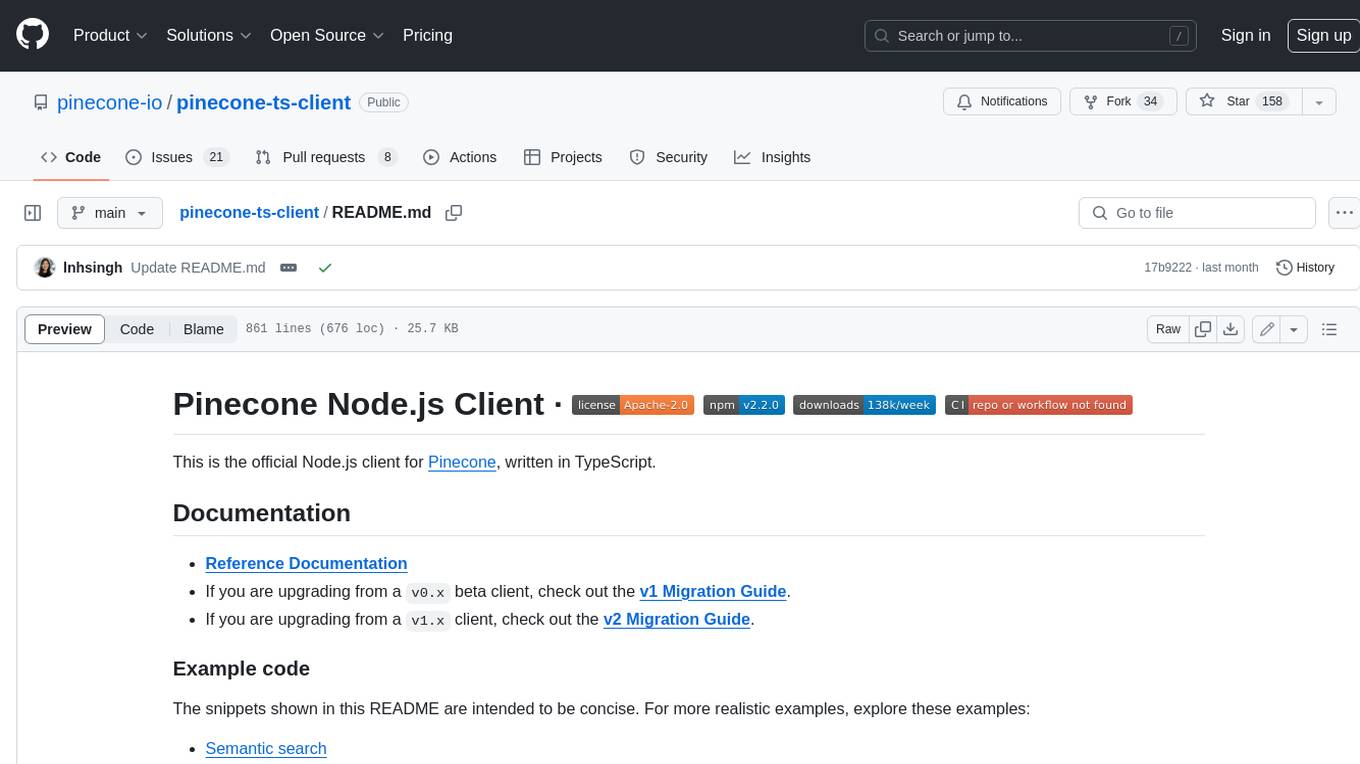
pinecone-ts-client
The official Node.js client for Pinecone, written in TypeScript. This client library provides a high-level interface for interacting with the Pinecone vector database service. With this client, you can create and manage indexes, upsert and query vector data, and perform other operations related to vector search and retrieval. The client is designed to be easy to use and provides a consistent and idiomatic experience for Node.js developers. It supports all the features and functionality of the Pinecone API, making it a comprehensive solution for building vector-powered applications in Node.js.
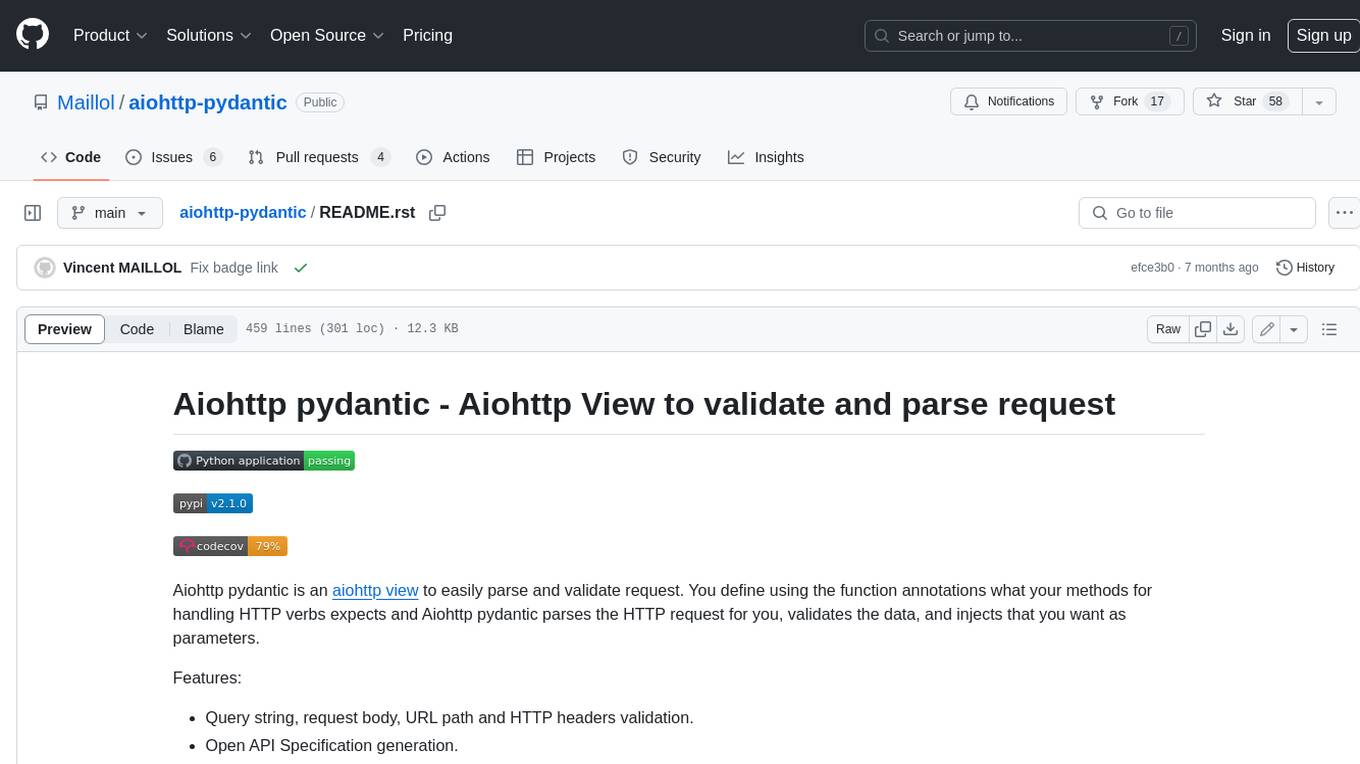
aiohttp-pydantic
Aiohttp pydantic is an aiohttp view to easily parse and validate requests. You define using function annotations what your methods for handling HTTP verbs expect, and Aiohttp pydantic parses the HTTP request for you, validates the data, and injects the parameters you want. It provides features like query string, request body, URL path, and HTTP headers validation, as well as Open API Specification generation.
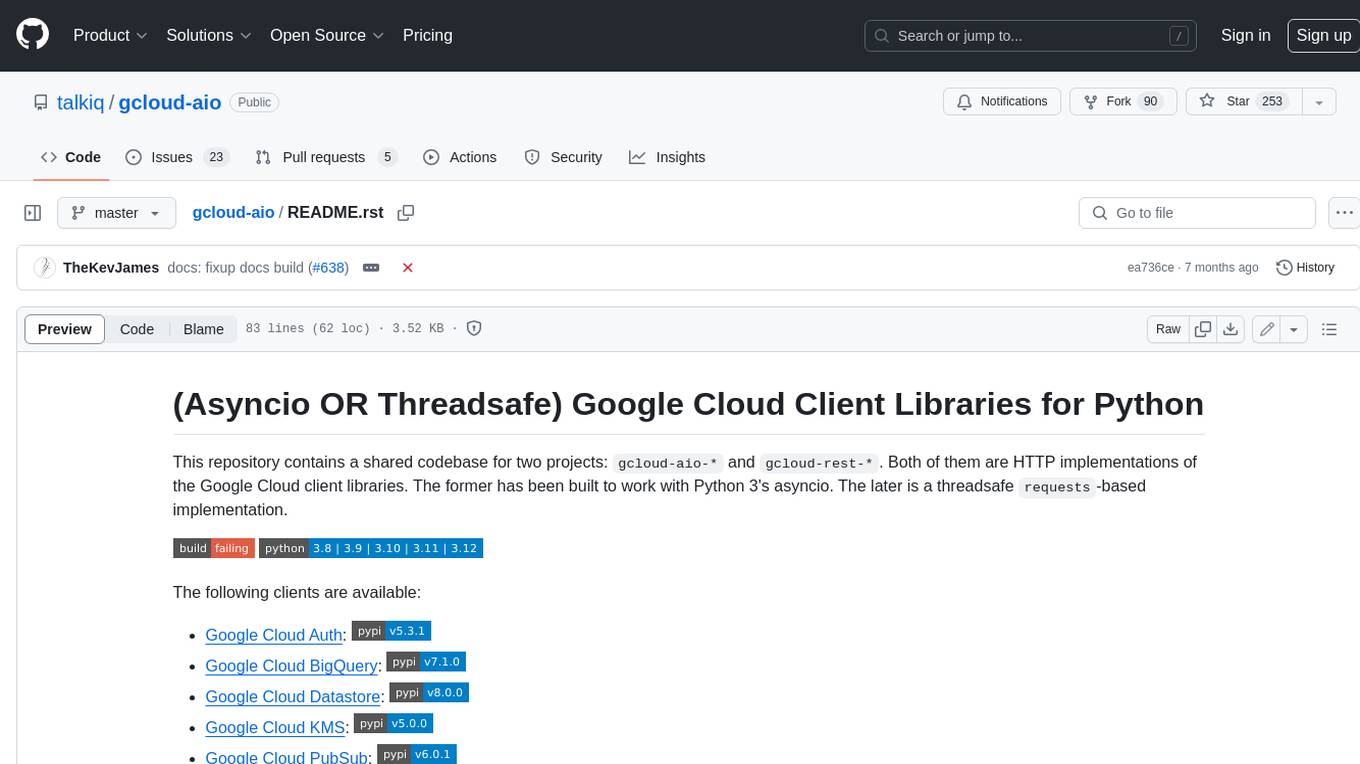
gcloud-aio
This repository contains shared codebase for two projects: gcloud-aio and gcloud-rest. gcloud-aio is built for Python 3's asyncio, while gcloud-rest is a threadsafe requests-based implementation. It provides clients for Google Cloud services like Auth, BigQuery, Datastore, KMS, PubSub, Storage, and Task Queue. Users can install the library using pip and refer to the documentation for usage details. Developers can contribute to the project by following the contribution guide.
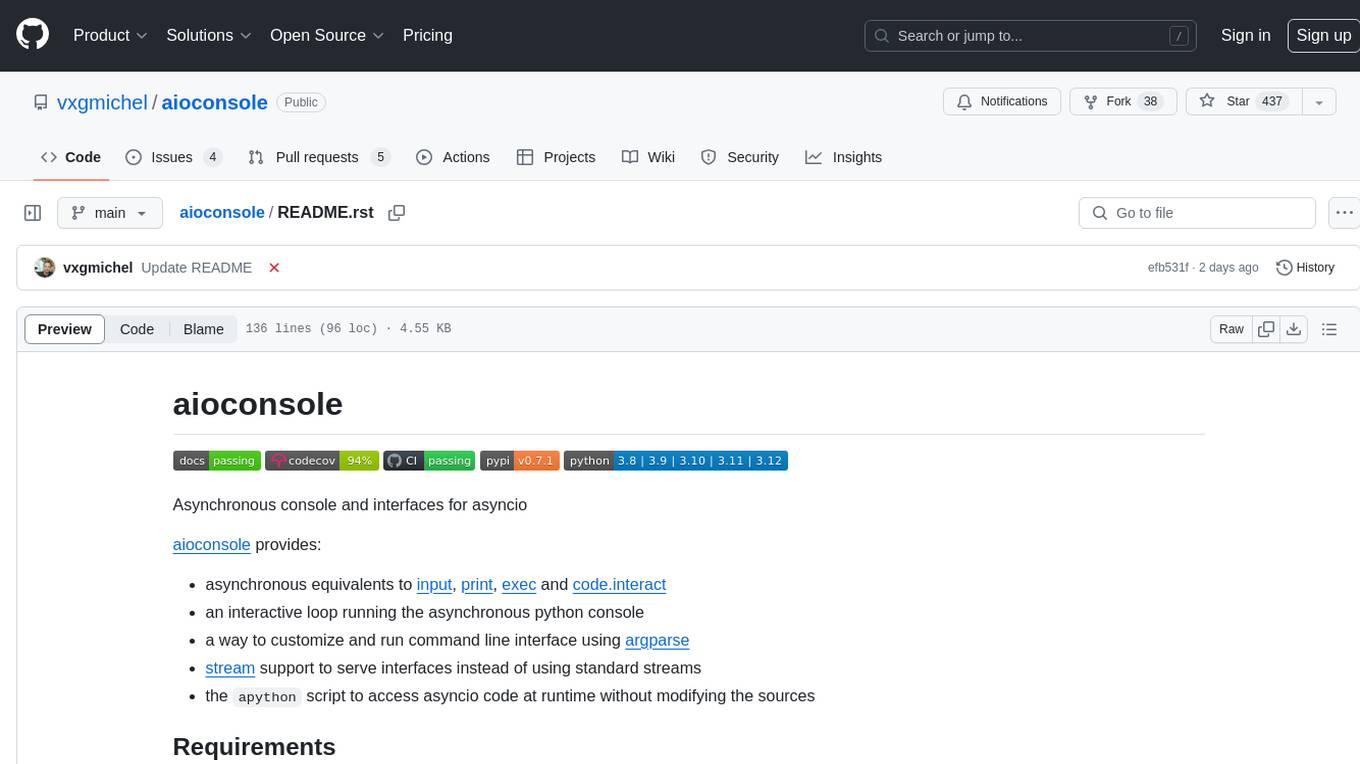
aioconsole
aioconsole is a Python package that provides asynchronous console and interfaces for asyncio. It offers asynchronous equivalents to input, print, exec, and code.interact, an interactive loop running the asynchronous Python console, customization and running of command line interfaces using argparse, stream support to serve interfaces instead of using standard streams, and the apython script to access asyncio code at runtime without modifying the sources. The package requires Python version 3.8 or higher and can be installed from PyPI or GitHub. It allows users to run Python files or modules with a modified asyncio policy, replacing the default event loop with an interactive loop. aioconsole is useful for scenarios where users need to interact with asyncio code in a console environment.
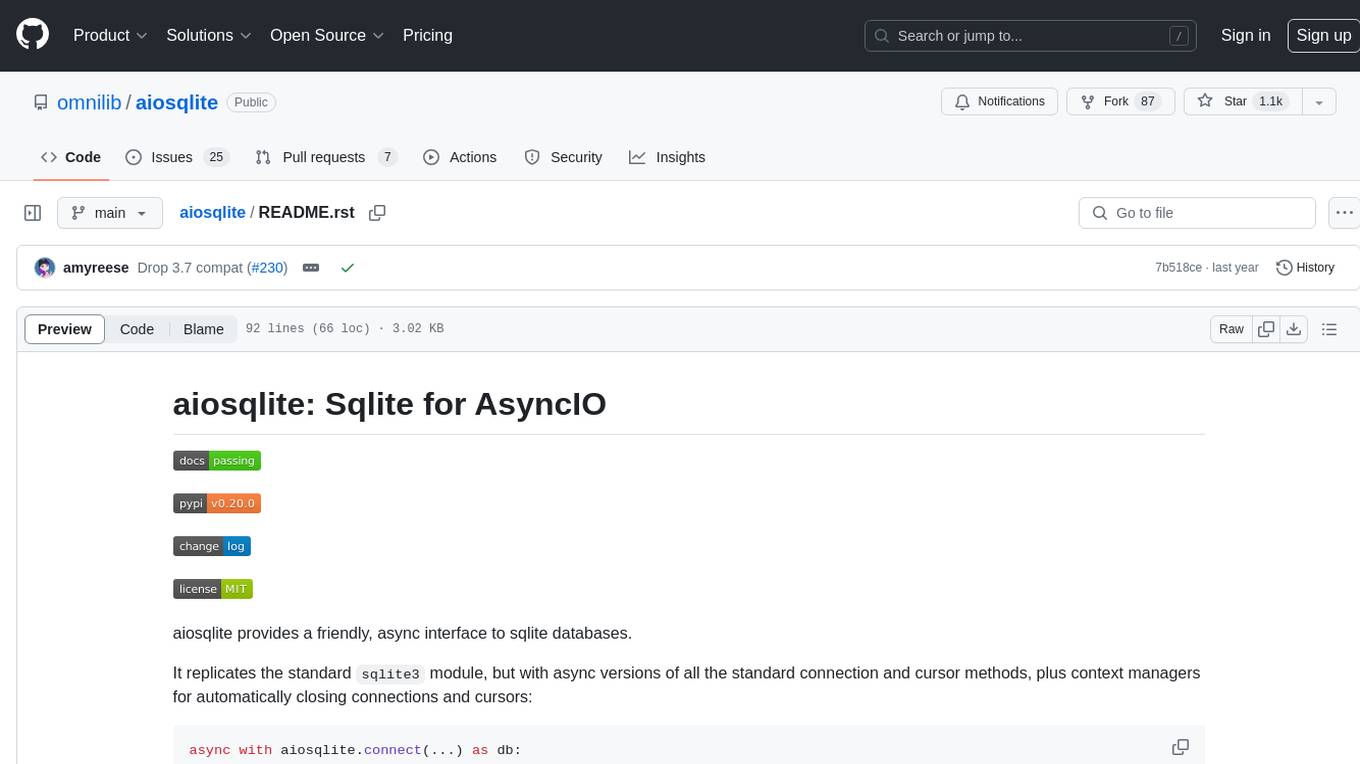
aiosqlite
aiosqlite is a Python library that provides a friendly, async interface to SQLite databases. It replicates the standard sqlite3 module but with async versions of all the standard connection and cursor methods, along with context managers for automatically closing connections and cursors. It allows interaction with SQLite databases on the main AsyncIO event loop without blocking execution of other coroutines while waiting for queries or data fetches. The library also replicates most of the advanced features of sqlite3, such as row factories and total changes tracking.