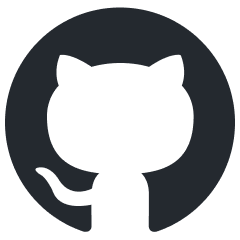
palimpzest
A System for (Optimized) Semantic Computation
Stars: 82
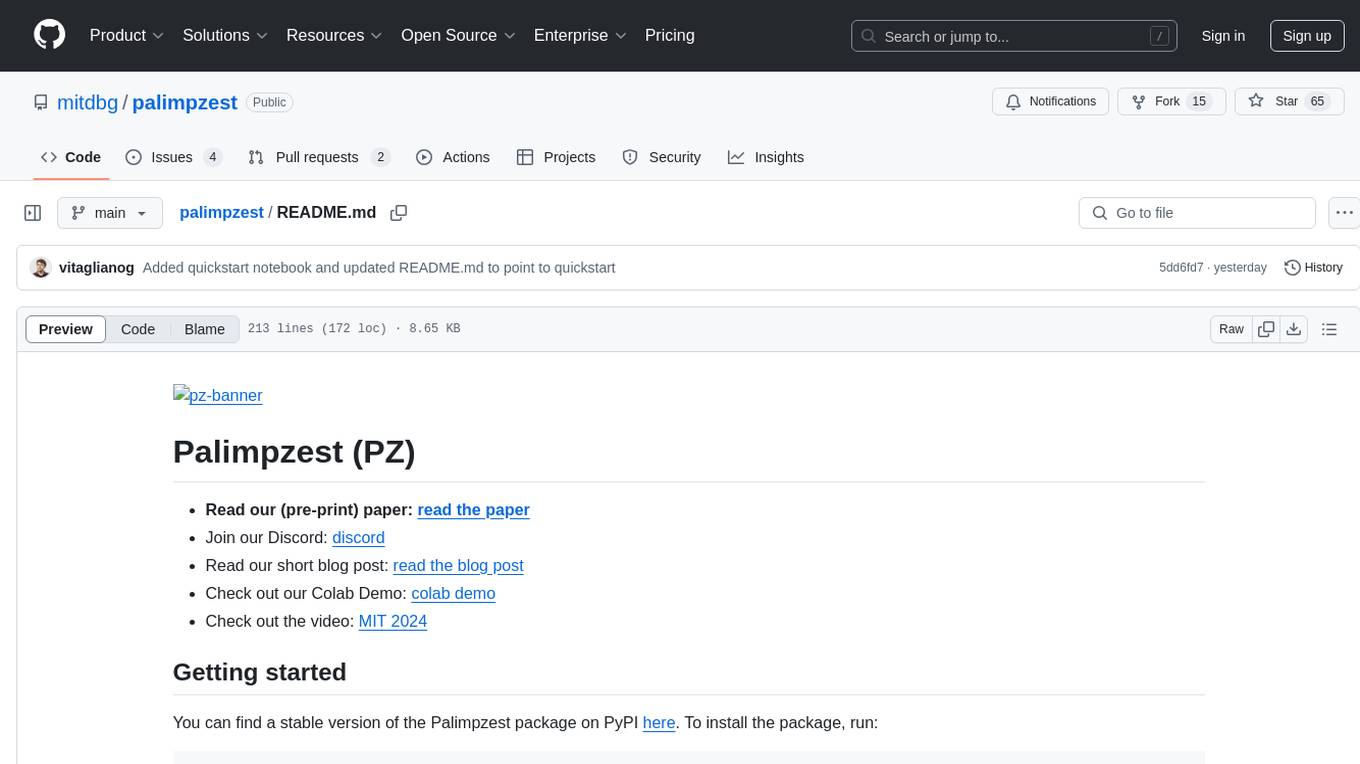
Palimpzest (PZ) is a tool for managing and optimizing workloads, particularly for data processing tasks. It provides a CLI tool and Python demos for users to register datasets, run workloads, and access results. Users can easily initialize their system, register datasets, and manage configurations using the CLI commands provided. Palimpzest also supports caching intermediate results and configuring for parallel execution with remote services like OpenAI and together.ai. The tool aims to streamline the workflow of working with datasets and optimizing performance for data extraction tasks.
README:
Our full documentation is the definitive resource for learning how to use PZ. It contains all of the installation and quickstart materials on this page, as well as user guides, full API documentation, and much more.
You can find a stable version of the PZ package on PyPI here. To install the package, run:
$ pip install palimpzest
Alternatively, to install the latest version of the package from this repository, you can clone this repository and run the following commands:
$ git clone [email protected]:mitdbg/palimpzest.git
$ cd palimpzest
$ pip install .
We are actively hacking on PZ and would love to have you join our community
Our Discord server is the best place to:
- Get help with your PZ program(s)
- Give feedback to the maintainers
- Discuss the future direction(s) of the project
- Discuss anything related to data processing with LLMs!
We are eager to learn more about your workloads and use cases, and will take them into consideration in planning our future roadmap.
The easiest way to get started with Palimpzest is to run the quickstart.ipynb
jupyter notebook. We demonstrate the full workflow of working with PZ, including registering a dataset, composing and executing a pipeline, and accessing the results.
To run the notebook, you can use the following command:
$ jupyter notebook
And then access the notebook from the jupyter interface in your browser at localhost:8888
.
For eager readers, the code in the notebook can be found in the following condensed snippet. However, we do suggest reading the notebook as it contains more insight into each element of the program.
import palimpzest as pz
# define the fields we wish to compute
email_cols = [
{"name": "sender", "type": str, "desc": "The email address of the sender"},
{"name": "subject", "type": str, "desc": "The subject of the email"},
{"name": "date", "type": str, "desc": "The date the email was sent"},
]
# lazily construct the computation to get emails about holidays sent in July
dataset = pz.Dataset("testdata/enron-tiny/")
dataset = dataset.sem_add_columns(email_cols)
dataset = dataset.sem_filter("The email was sent in July")
dataset = dataset.sem_filter("The email is about holidays")
# execute the computation w/the MinCost policy
config = pz.QueryProcessorConfig(policy=pz.MinCost(), verbose=True)
output = dataset.run(config)
# display output (if using Jupyter, otherwise use print(output_df))
output_df = output.to_df(cols=["date", "sender", "subject"])
display(output_df)
Below are simple instructions to run PZ on a test data set of enron emails that is included with the system.
To run the provided demos, you will need to download the test data. Due to the size of the data, we are unable to include it in the repository. You can download the test data by running the following command from a unix terminal (requires wget
and tar
):
chmod +x testdata/download-testdata.sh
./testdata/download-testdata.sh
Set your OpenAI (or Together.ai) api key at the command line:
# set one (or both) of the following:
export OPENAI_API_KEY=<your-api-key>
export TOGETHER_API_KEY=<your-api-key>
Now you can run the simple test program with:
$ python demos/simple-demo.py --task enron --dataset testdata/enron-eval-tiny --verbose
For Tasks:
Click tags to check more tools for each tasksFor Jobs:
Alternative AI tools for palimpzest
Similar Open Source Tools
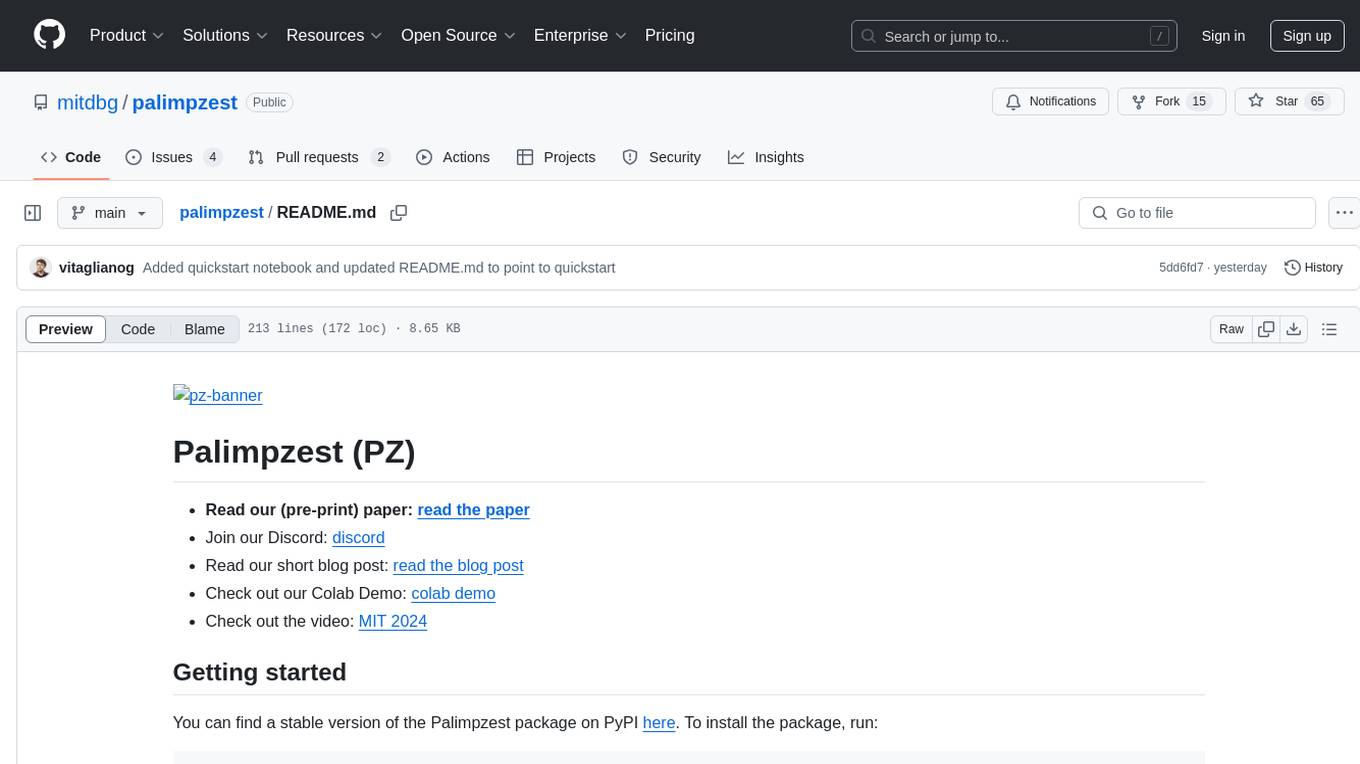
palimpzest
Palimpzest (PZ) is a tool for managing and optimizing workloads, particularly for data processing tasks. It provides a CLI tool and Python demos for users to register datasets, run workloads, and access results. Users can easily initialize their system, register datasets, and manage configurations using the CLI commands provided. Palimpzest also supports caching intermediate results and configuring for parallel execution with remote services like OpenAI and together.ai. The tool aims to streamline the workflow of working with datasets and optimizing performance for data extraction tasks.
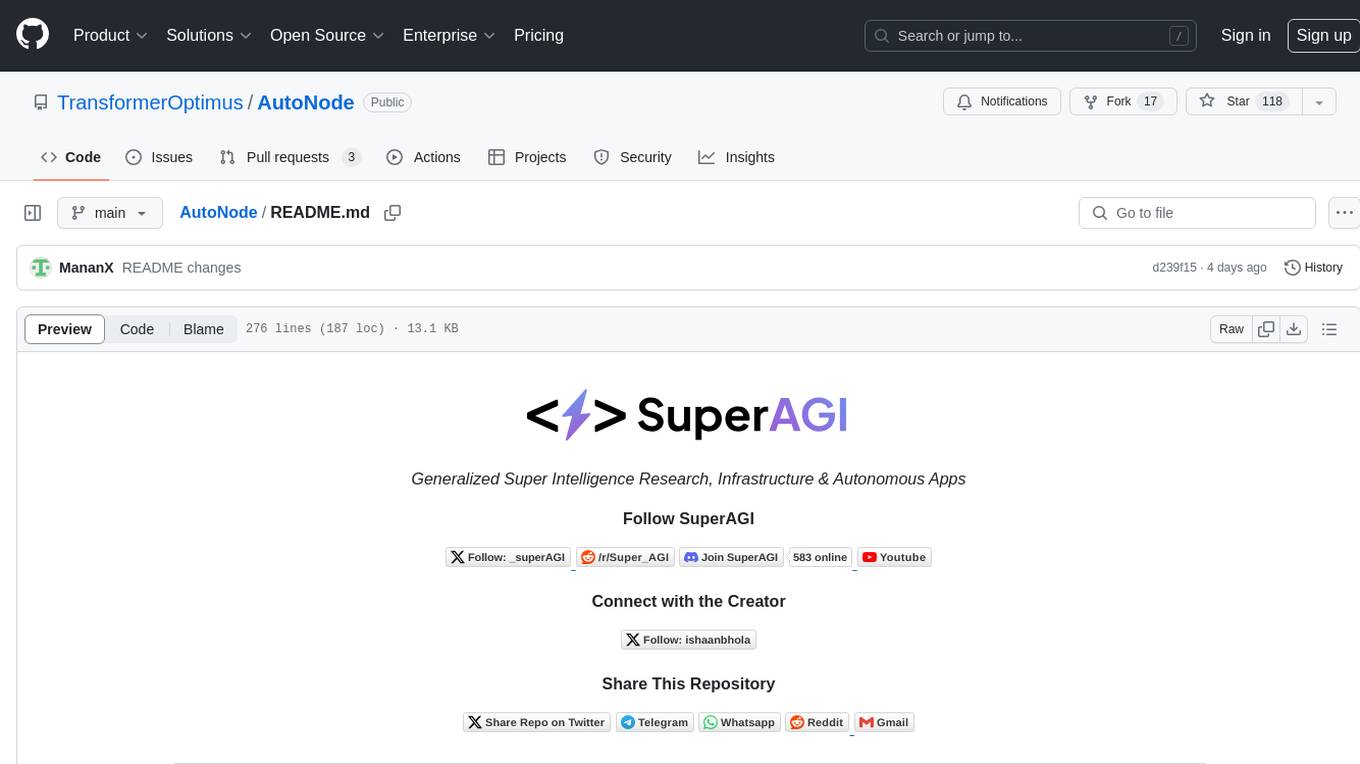
AutoNode
AutoNode is a self-operating computer system designed to automate web interactions and data extraction processes. It leverages advanced technologies like OCR (Optical Character Recognition), YOLO (You Only Look Once) models for object detection, and a custom site-graph to navigate and interact with web pages programmatically. Users can define objectives, create site-graphs, and utilize AutoNode via API to automate tasks on websites. The tool also supports training custom YOLO models for object detection and OCR for text recognition on web pages. AutoNode can be used for tasks such as extracting product details, automating web interactions, and more.
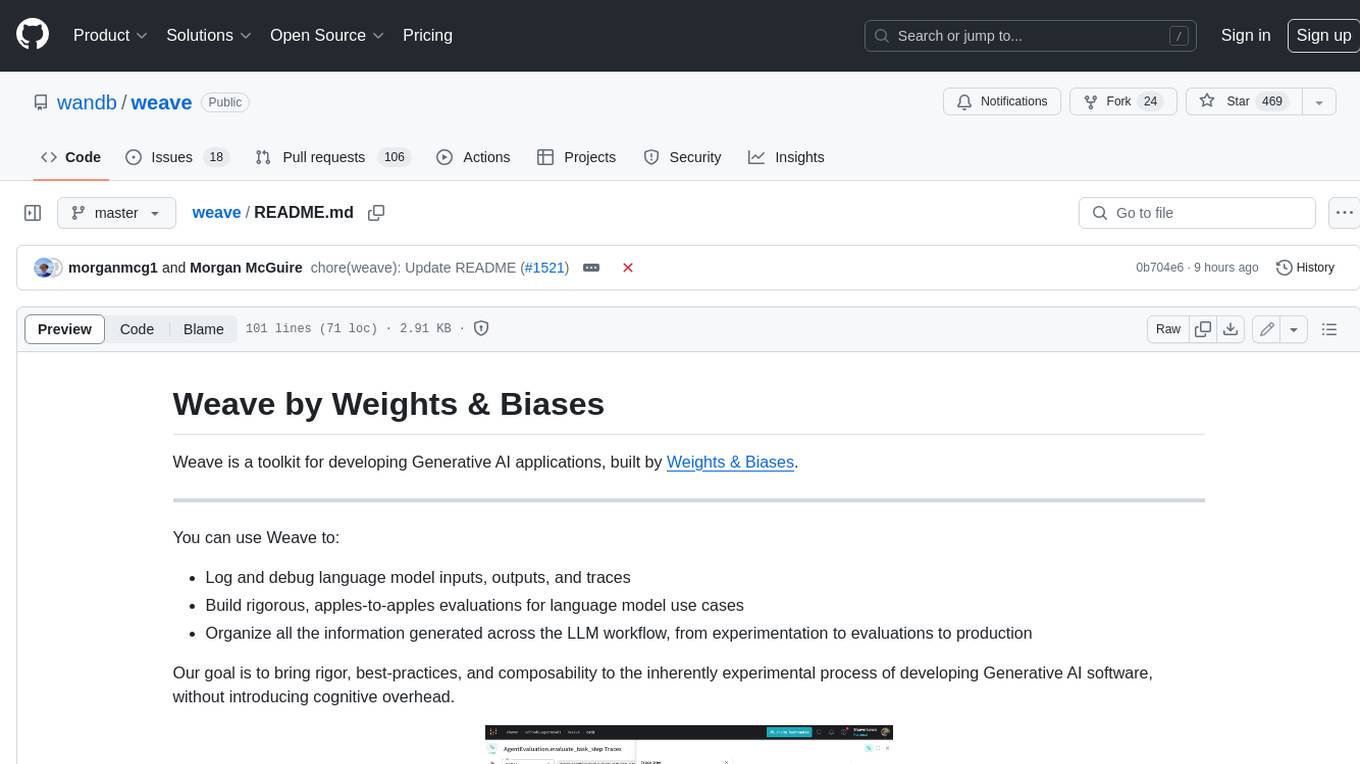
weave
Weave is a toolkit for developing Generative AI applications, built by Weights & Biases. With Weave, you can log and debug language model inputs, outputs, and traces; build rigorous, apples-to-apples evaluations for language model use cases; and organize all the information generated across the LLM workflow, from experimentation to evaluations to production. Weave aims to bring rigor, best-practices, and composability to the inherently experimental process of developing Generative AI software, without introducing cognitive overhead.
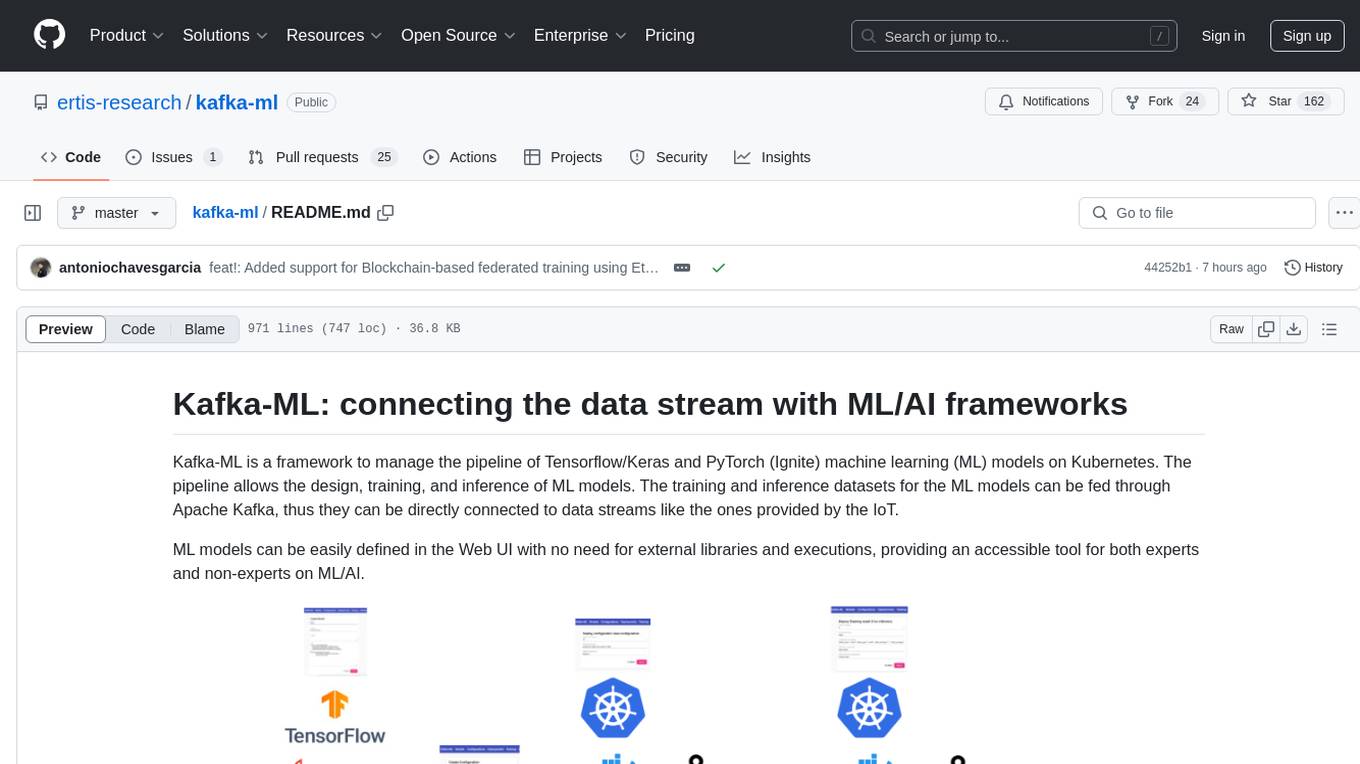
kafka-ml
Kafka-ML is a framework designed to manage the pipeline of Tensorflow/Keras and PyTorch machine learning models on Kubernetes. It enables the design, training, and inference of ML models with datasets fed through Apache Kafka, connecting them directly to data streams like those from IoT devices. The Web UI allows easy definition of ML models without external libraries, catering to both experts and non-experts in ML/AI.
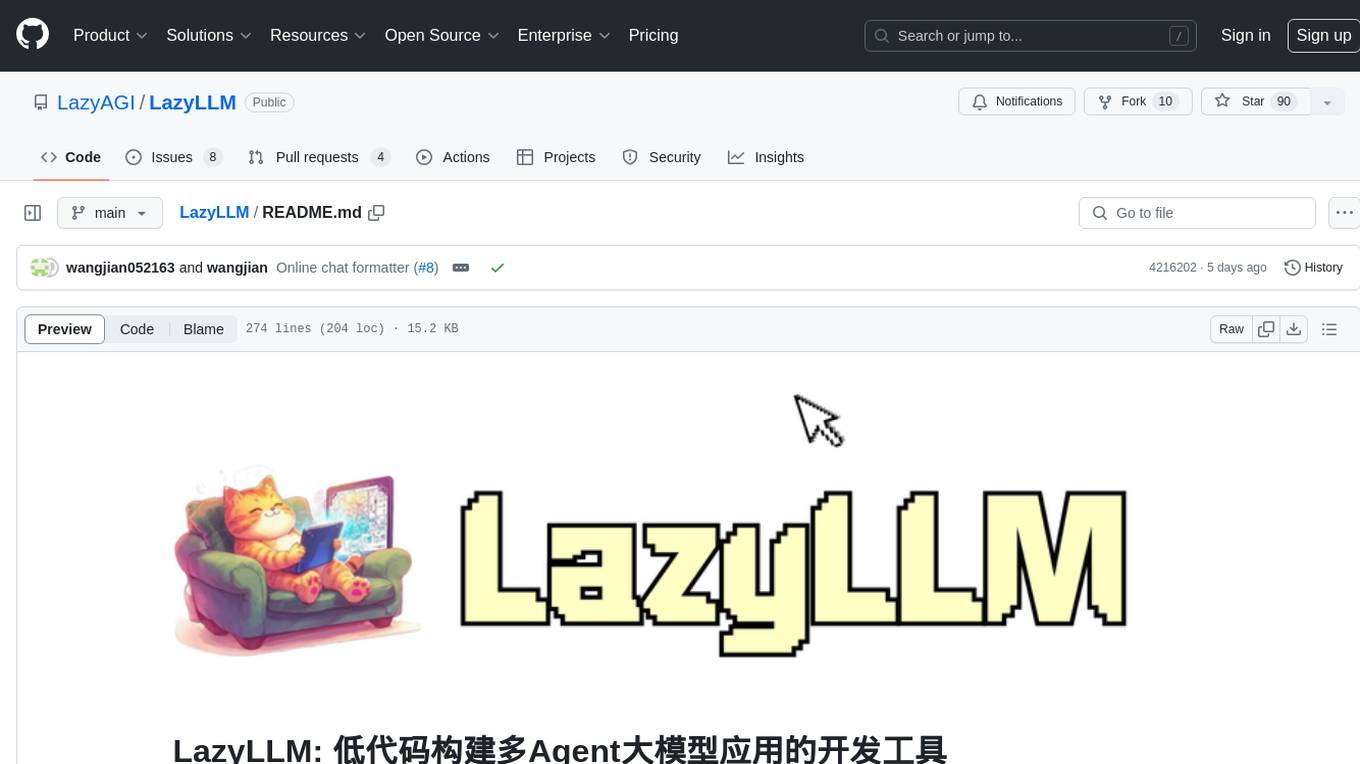
LazyLLM
LazyLLM is a low-code development tool for building complex AI applications with multiple agents. It assists developers in building AI applications at a low cost and continuously optimizing their performance. The tool provides a convenient workflow for application development and offers standard processes and tools for various stages of application development. Users can quickly prototype applications with LazyLLM, analyze bad cases with scenario task data, and iteratively optimize key components to enhance the overall application performance. LazyLLM aims to simplify the AI application development process and provide flexibility for both beginners and experts to create high-quality applications.
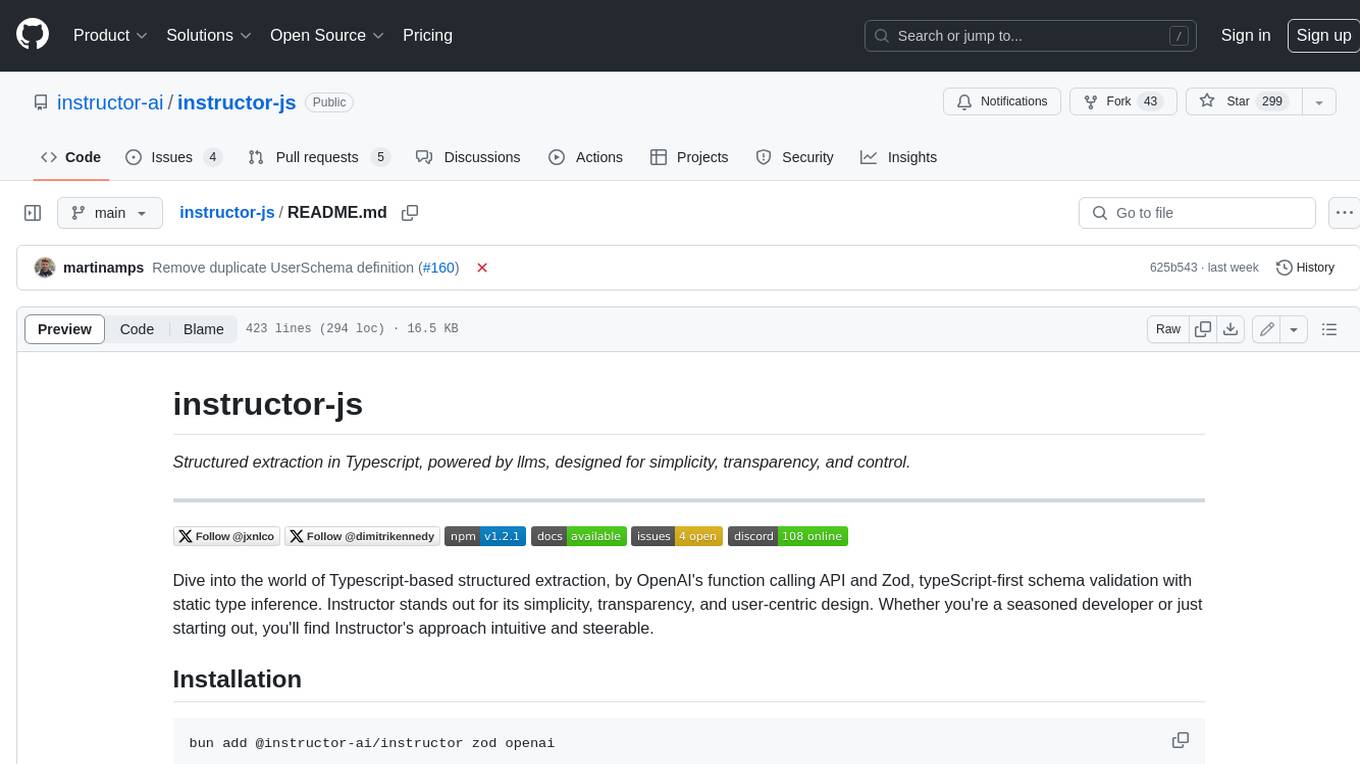
instructor-js
Instructor is a Typescript library for structured extraction in Typescript, powered by llms, designed for simplicity, transparency, and control. It stands out for its simplicity, transparency, and user-centric design. Whether you're a seasoned developer or just starting out, you'll find Instructor's approach intuitive and steerable.
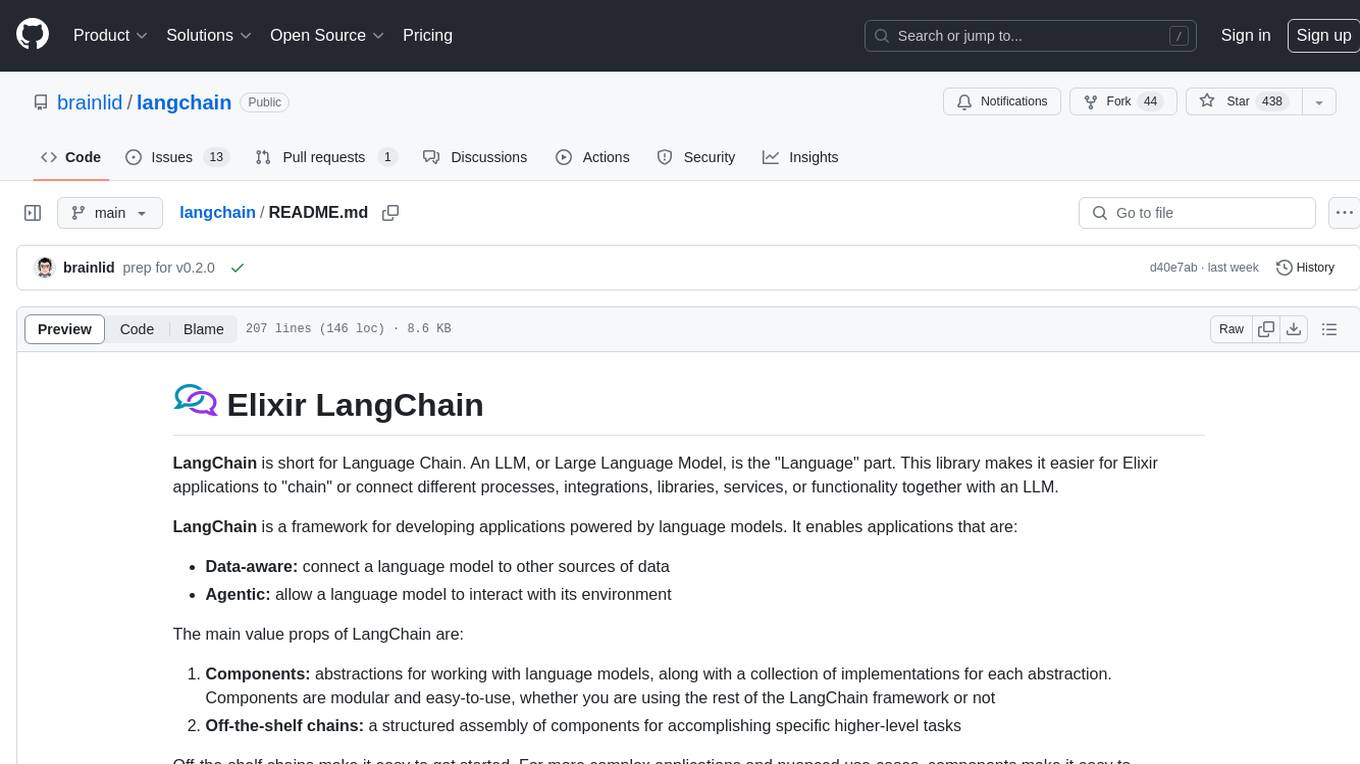
langchain
LangChain is a framework for developing Elixir applications powered by language models. It enables applications to connect language models to other data sources and interact with the environment. The library provides components for working with language models and off-the-shelf chains for specific tasks. It aims to assist in building applications that combine large language models with other sources of computation or knowledge. LangChain is written in Elixir and is not aimed for parity with the JavaScript and Python versions due to differences in programming paradigms and design choices. The library is designed to make it easy to integrate language models into applications and expose features, data, and functionality to the models.
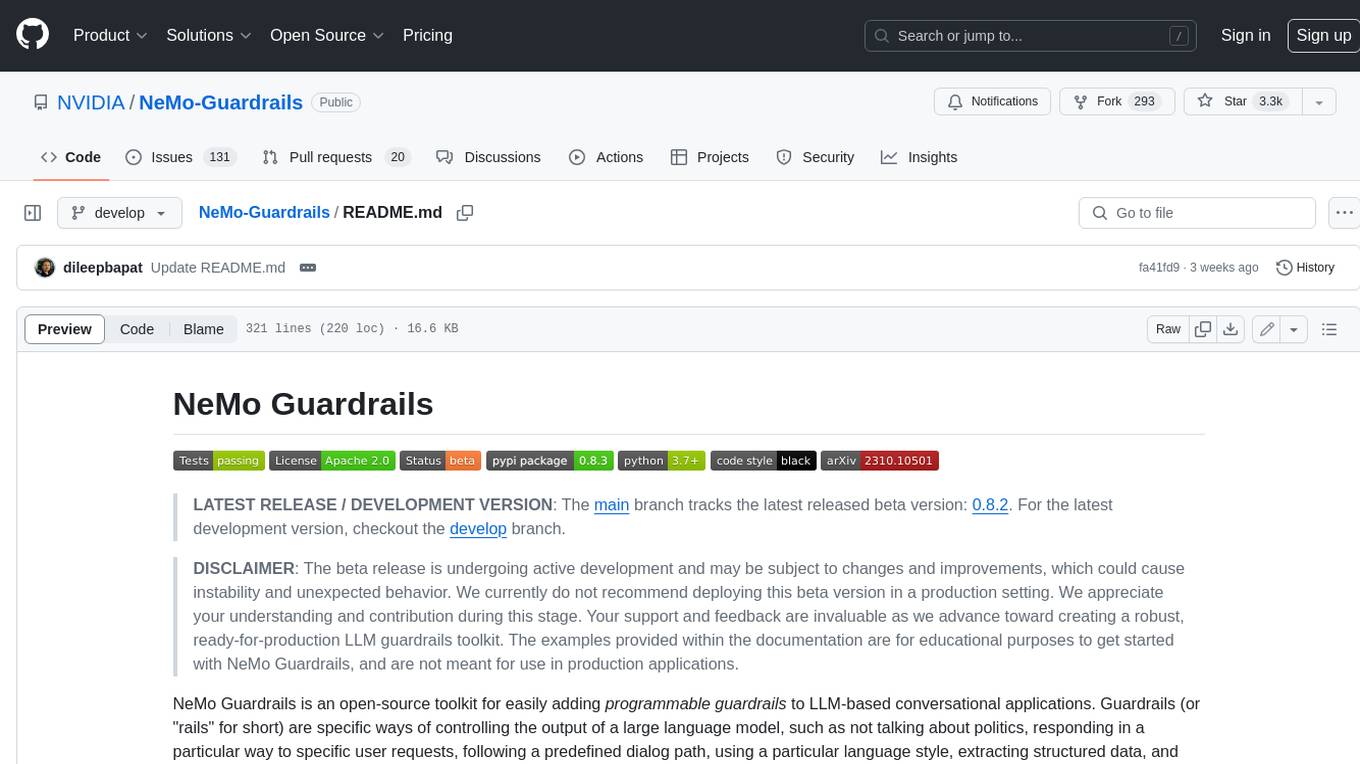
NeMo-Guardrails
NeMo Guardrails is an open-source toolkit for easily adding _programmable guardrails_ to LLM-based conversational applications. Guardrails (or "rails" for short) are specific ways of controlling the output of a large language model, such as not talking about politics, responding in a particular way to specific user requests, following a predefined dialog path, using a particular language style, extracting structured data, and more.
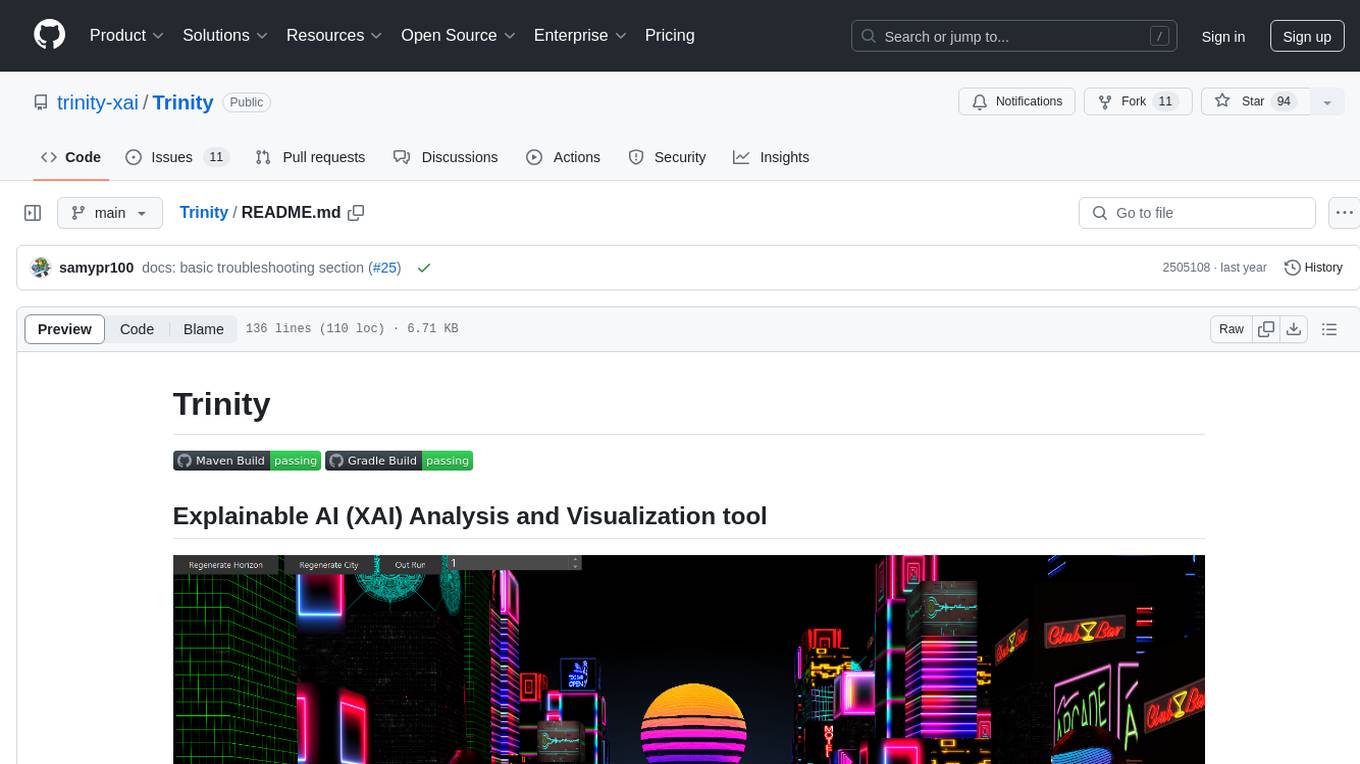
Trinity
Trinity is an Explainable AI (XAI) Analysis and Visualization tool designed for Deep Learning systems or other models performing complex classification or decoding. It provides performance analysis through interactive 3D projections that are hyper-dimensional aware, allowing users to explore hyperspace, hypersurface, projections, and manifolds. Trinity primarily works with JSON data formats and supports the visualization of FeatureVector objects. Users can analyze and visualize data points, correlate inputs with classification results, and create custom color maps for better data interpretation. Trinity has been successfully applied to various use cases including Deep Learning Object detection models, COVID gene/tissue classification, Brain Computer Interface decoders, and Large Language Model (ChatGPT) Embeddings Analysis.
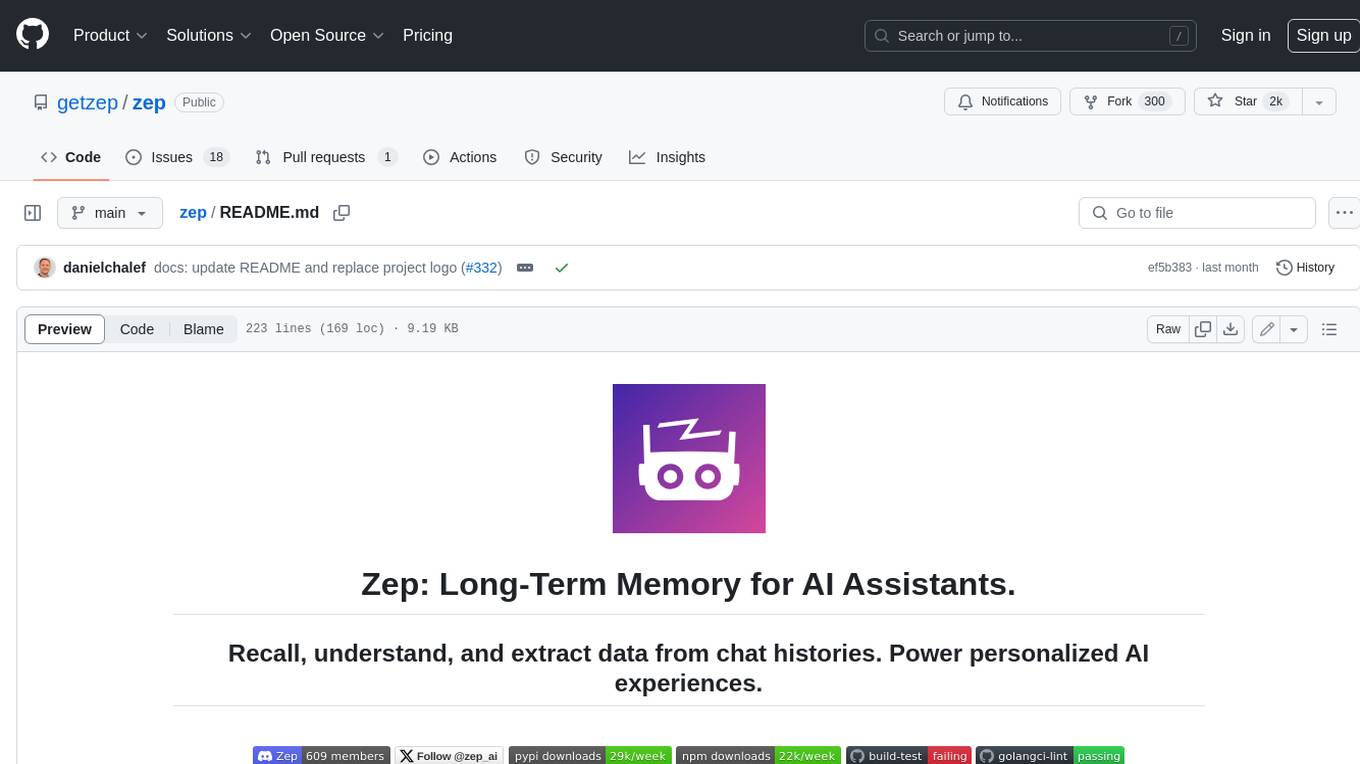
zep
Zep is a long-term memory service for AI Assistant apps. With Zep, you can provide AI assistants with the ability to recall past conversations, no matter how distant, while also reducing hallucinations, latency, and cost. Zep persists and recalls chat histories, and automatically generates summaries and other artifacts from these chat histories. It also embeds messages and summaries, enabling you to search Zep for relevant context from past conversations. Zep does all of this asyncronously, ensuring these operations don't impact your user's chat experience. Data is persisted to database, allowing you to scale out when growth demands. Zep also provides a simple, easy to use abstraction for document vector search called Document Collections. This is designed to complement Zep's core memory features, but is not designed to be a general purpose vector database. Zep allows you to be more intentional about constructing your prompt: 1. automatically adding a few recent messages, with the number customized for your app; 2. a summary of recent conversations prior to the messages above; 3. and/or contextually relevant summaries or messages surfaced from the entire chat session. 4. and/or relevant Business data from Zep Document Collections.
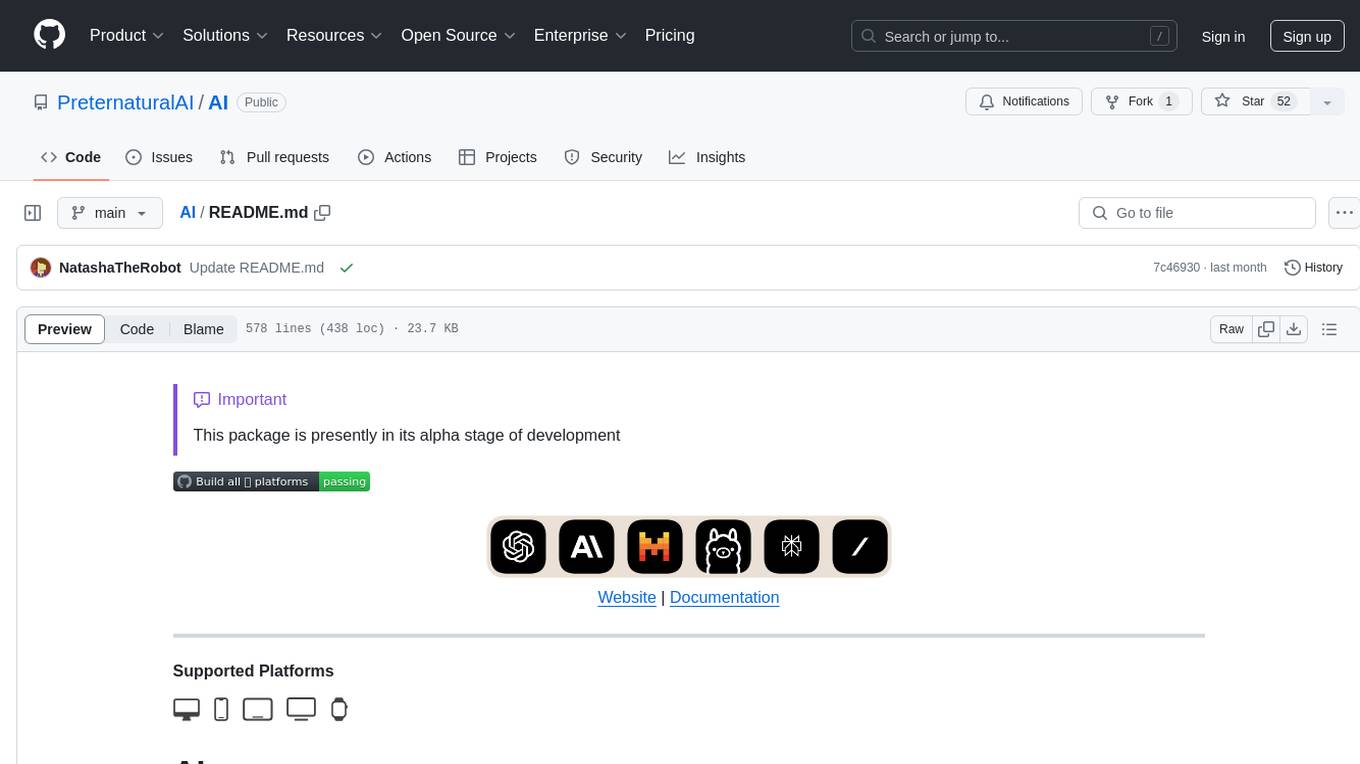
AI
AI is an open-source Swift framework for interfacing with generative AI. It provides functionalities for text completions, image-to-text vision, function calling, DALLE-3 image generation, audio transcription and generation, and text embeddings. The framework supports multiple AI models from providers like OpenAI, Anthropic, Mistral, Groq, and ElevenLabs. Users can easily integrate AI capabilities into their Swift projects using AI framework.
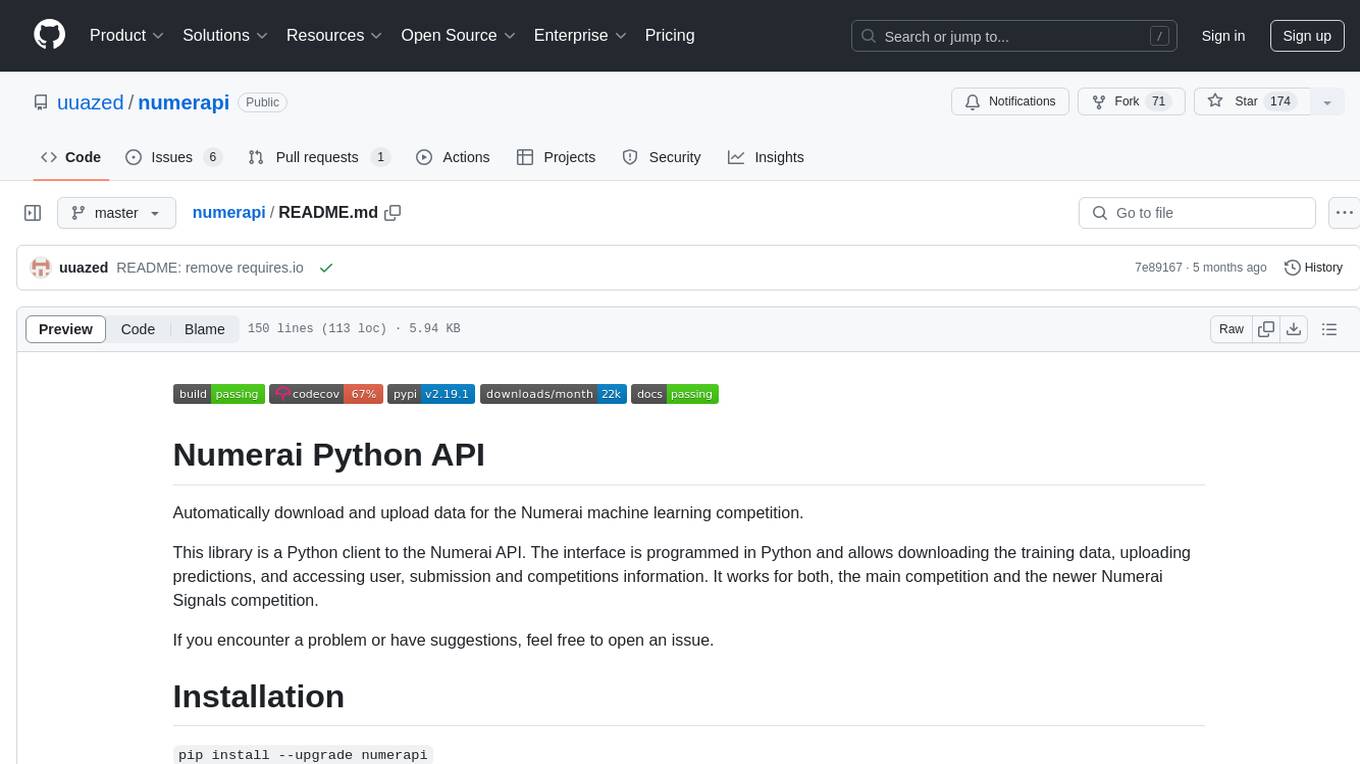
numerapi
Numerapi is a Python client to the Numerai API that allows users to automatically download and upload data for the Numerai machine learning competition. It provides functionalities for downloading training data, uploading predictions, and accessing user, submission, and competitions information for both the main competition and Numerai Signals competition. Users can interact with the API using Python modules or command line interface. Tokens are required for certain actions like uploading predictions or staking, which can be obtained from Numer.ai account settings. The tool also supports features like checking new rounds, getting leaderboards, and managing stakes.
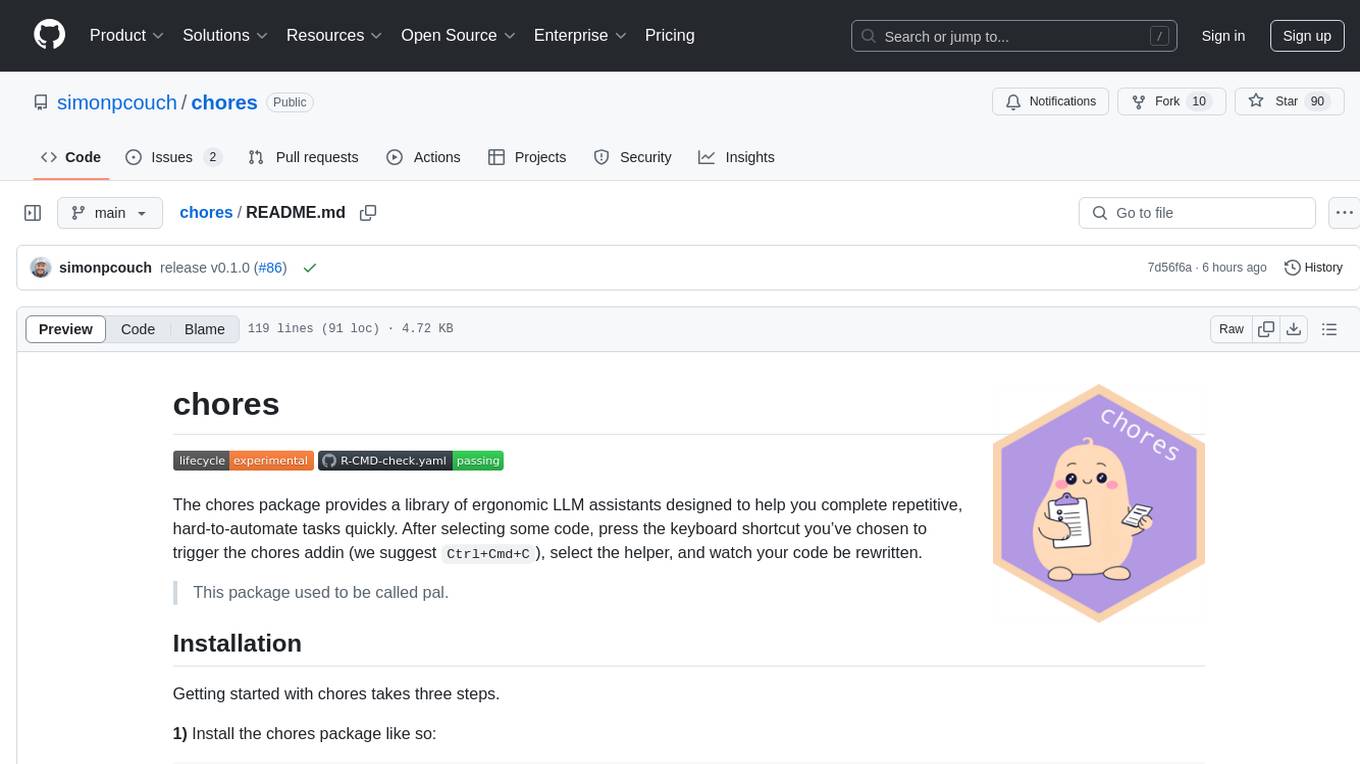
chores
The chores package provides a library of ergonomic LLM assistants designed to help users complete repetitive, hard-to-automate tasks quickly. Users can select code, trigger the chores addin, choose a helper, and watch their code be rewritten. The package offers chore helpers for tasks like converting to cli, testthat, and documenting functions with roxygen. Users can also create their own chore helpers by providing instructions in a markdown file. The cost of using helpers depends on the length of the prompt and the model chosen.
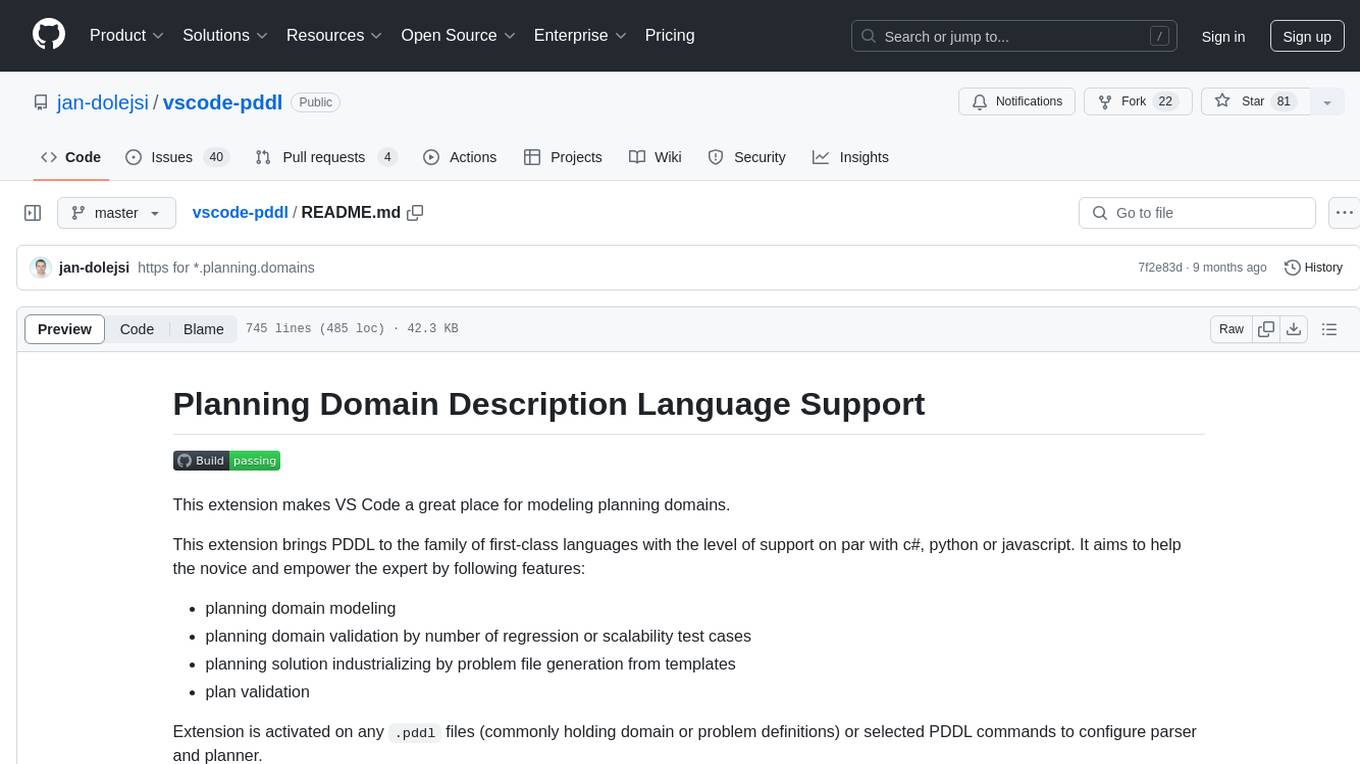
vscode-pddl
The vscode-pddl extension provides comprehensive support for Planning Domain Description Language (PDDL) in Visual Studio Code. It enables users to model planning domains, validate them, industrialize planning solutions, and run planners. The extension offers features like syntax highlighting, auto-completion, plan visualization, plan validation, plan happenings evaluation, search debugging, and integration with Planning.Domains. Users can create PDDL files, run planners, visualize plans, and debug search algorithms efficiently within VS Code.
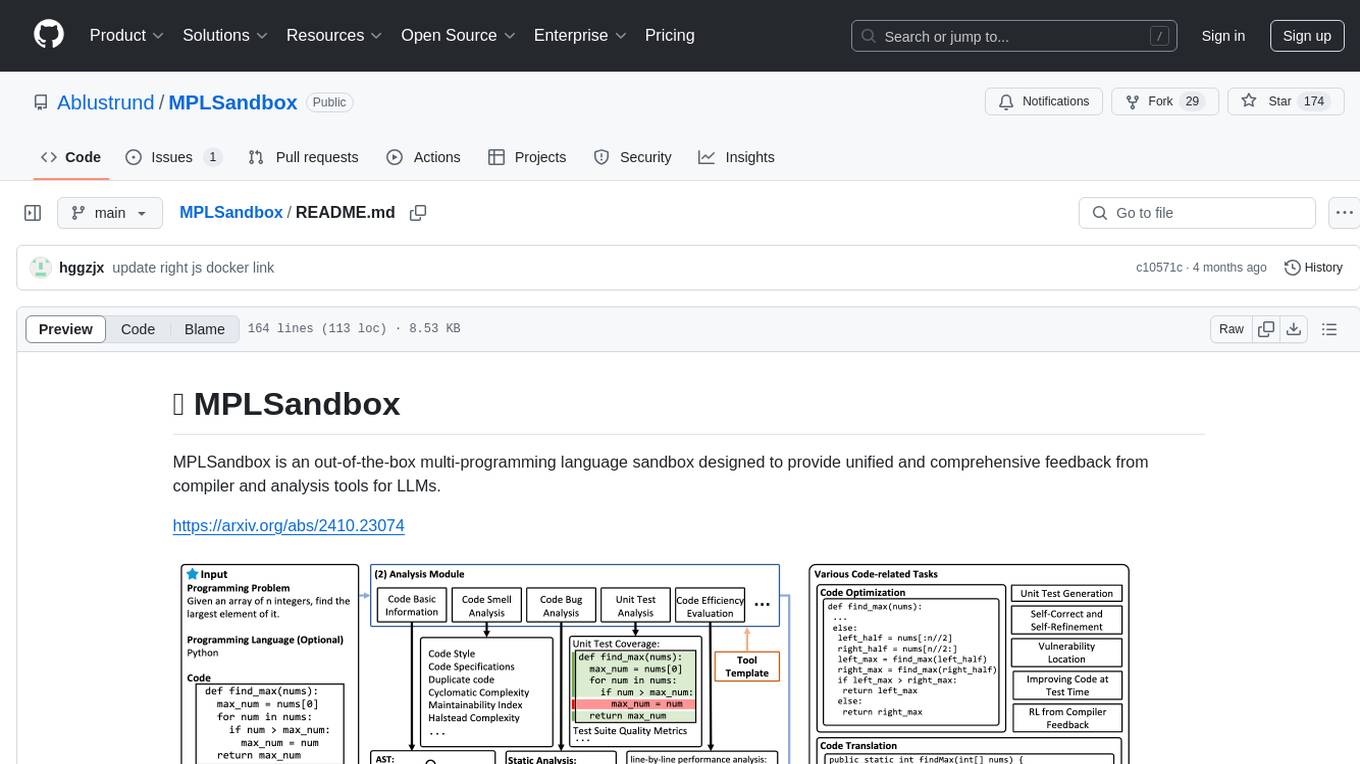
MPLSandbox
MPLSandbox is an out-of-the-box multi-programming language sandbox designed to provide unified and comprehensive feedback from compiler and analysis tools for LLMs. It simplifies code analysis for researchers and can be seamlessly integrated into LLM training and application processes to enhance performance in a range of code-related tasks. The sandbox environment ensures safe code execution, the code analysis module offers comprehensive analysis reports, and the information integration module combines compilation feedback and analysis results for complex code-related tasks.
For similar tasks
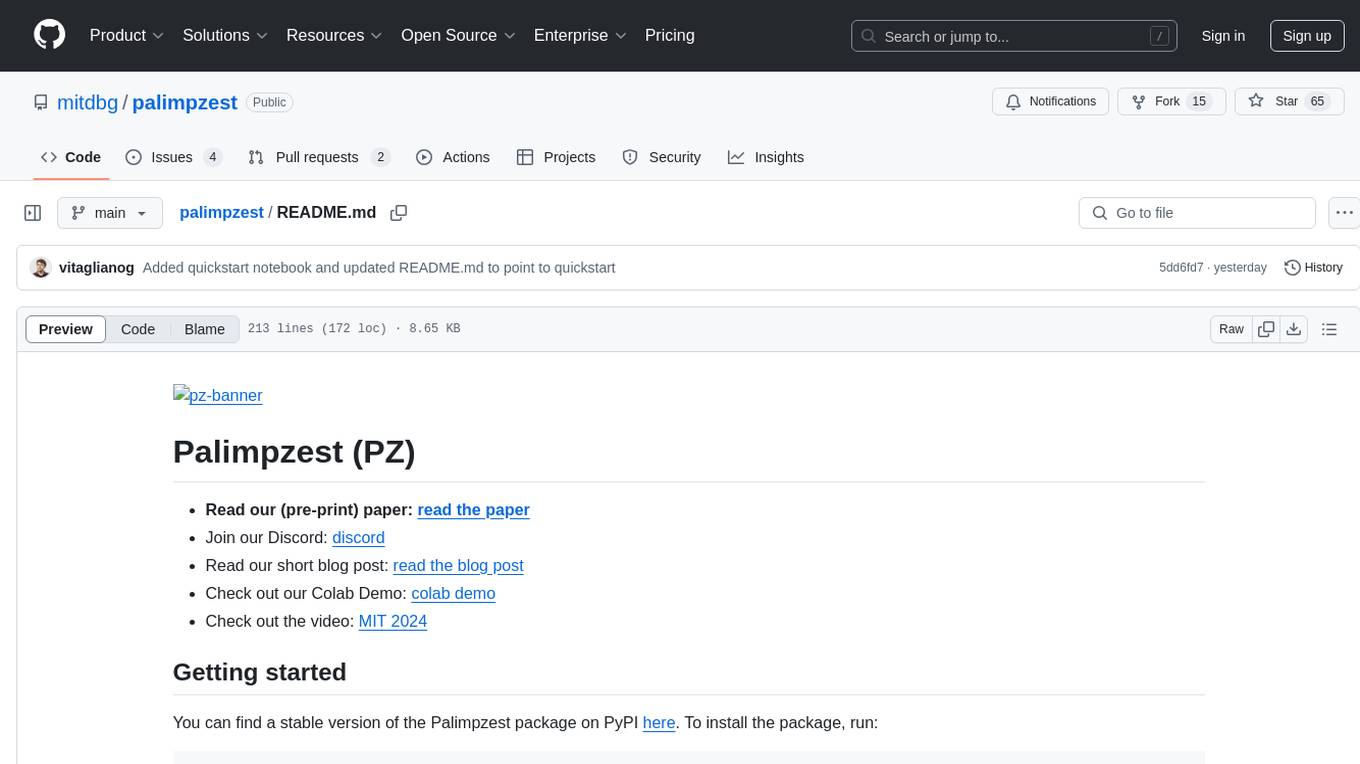
palimpzest
Palimpzest (PZ) is a tool for managing and optimizing workloads, particularly for data processing tasks. It provides a CLI tool and Python demos for users to register datasets, run workloads, and access results. Users can easily initialize their system, register datasets, and manage configurations using the CLI commands provided. Palimpzest also supports caching intermediate results and configuring for parallel execution with remote services like OpenAI and together.ai. The tool aims to streamline the workflow of working with datasets and optimizing performance for data extraction tasks.
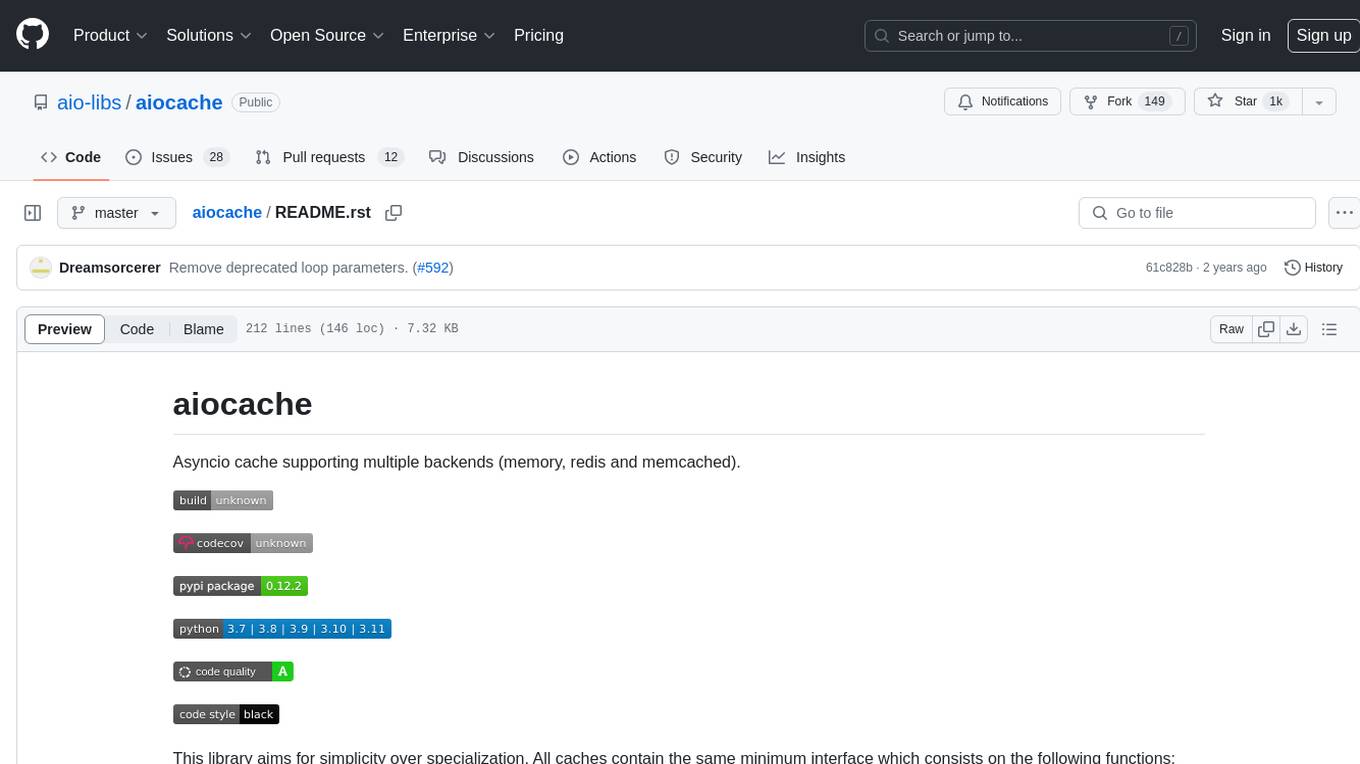
aiocache
Aiocache is an asyncio cache library that supports multiple backends such as memory, redis, and memcached. It provides a simple interface for functions like add, get, set, multi_get, multi_set, exists, increment, delete, clear, and raw. Users can easily install and use the library for caching data in Python applications. Aiocache allows for easy instantiation of caches and setup of cache aliases for reusing configurations. It also provides support for backends, serializers, and plugins to customize cache operations. The library offers detailed documentation and examples for different use cases and configurations.
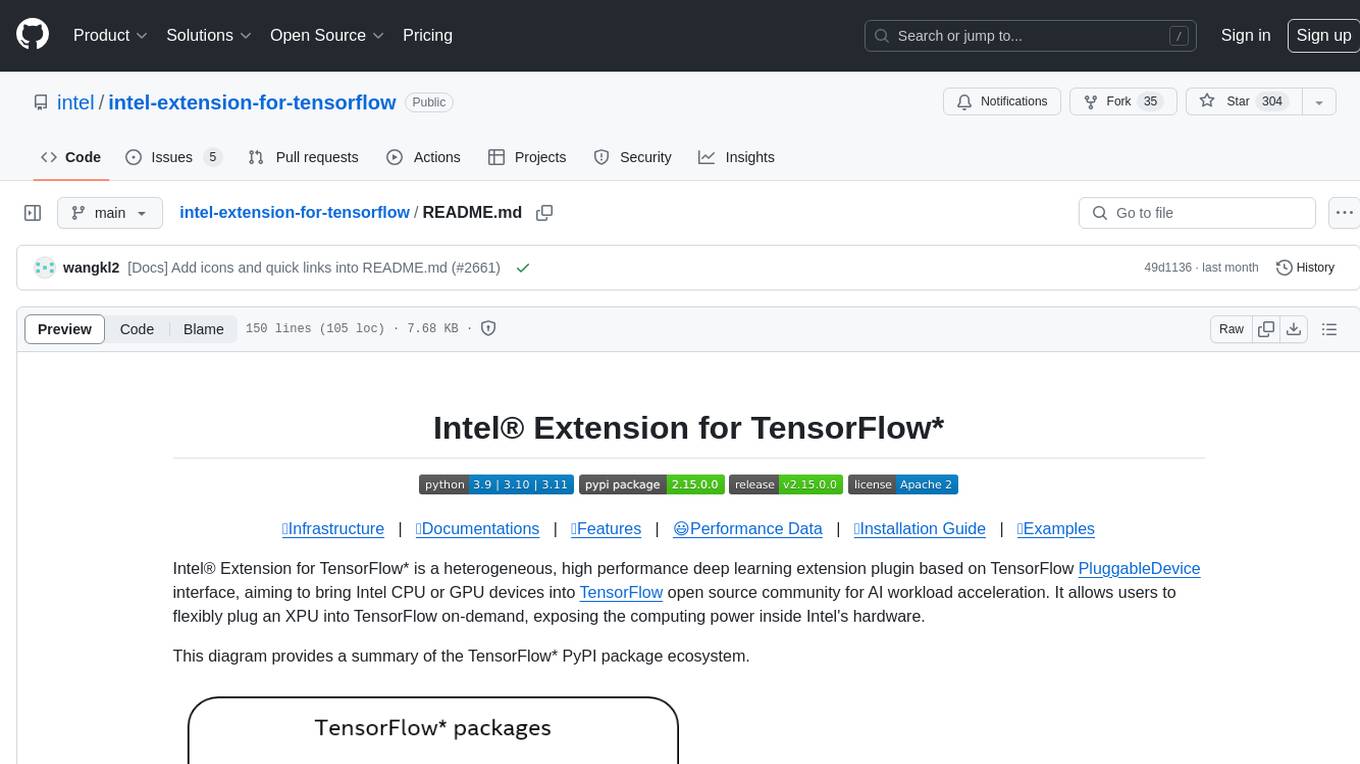
intel-extension-for-tensorflow
Intel® Extension for TensorFlow* is a high performance deep learning extension plugin based on TensorFlow PluggableDevice interface. It aims to accelerate AI workloads by allowing users to plug Intel CPU or GPU devices into TensorFlow on-demand, exposing the computing power inside Intel's hardware. The extension provides XPU specific implementation, kernels & operators, graph optimizer, device runtime, XPU configuration management, XPU backend selection, and options for turning on/off advanced features.
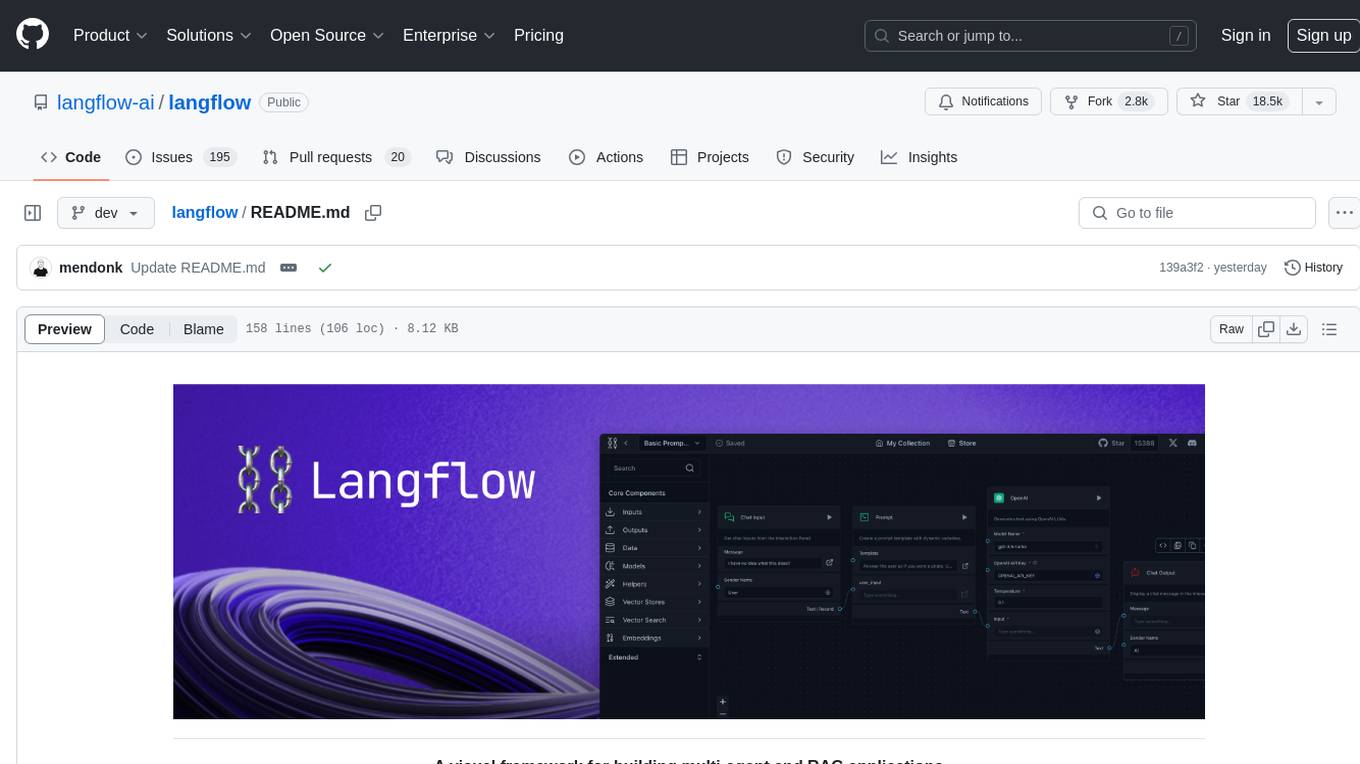
langflow
Langflow is an open-source Python-powered visual framework designed for building multi-agent and RAG applications. It is fully customizable, language model agnostic, and vector store agnostic. Users can easily create flows by dragging components onto the canvas, connect them, and export the flow as a JSON file. Langflow also provides a command-line interface (CLI) for easy management and configuration, allowing users to customize the behavior of Langflow for development or specialized deployment scenarios. The tool can be deployed on various platforms such as Google Cloud Platform, Railway, and Render. Contributors are welcome to enhance the project on GitHub by following the contributing guidelines.
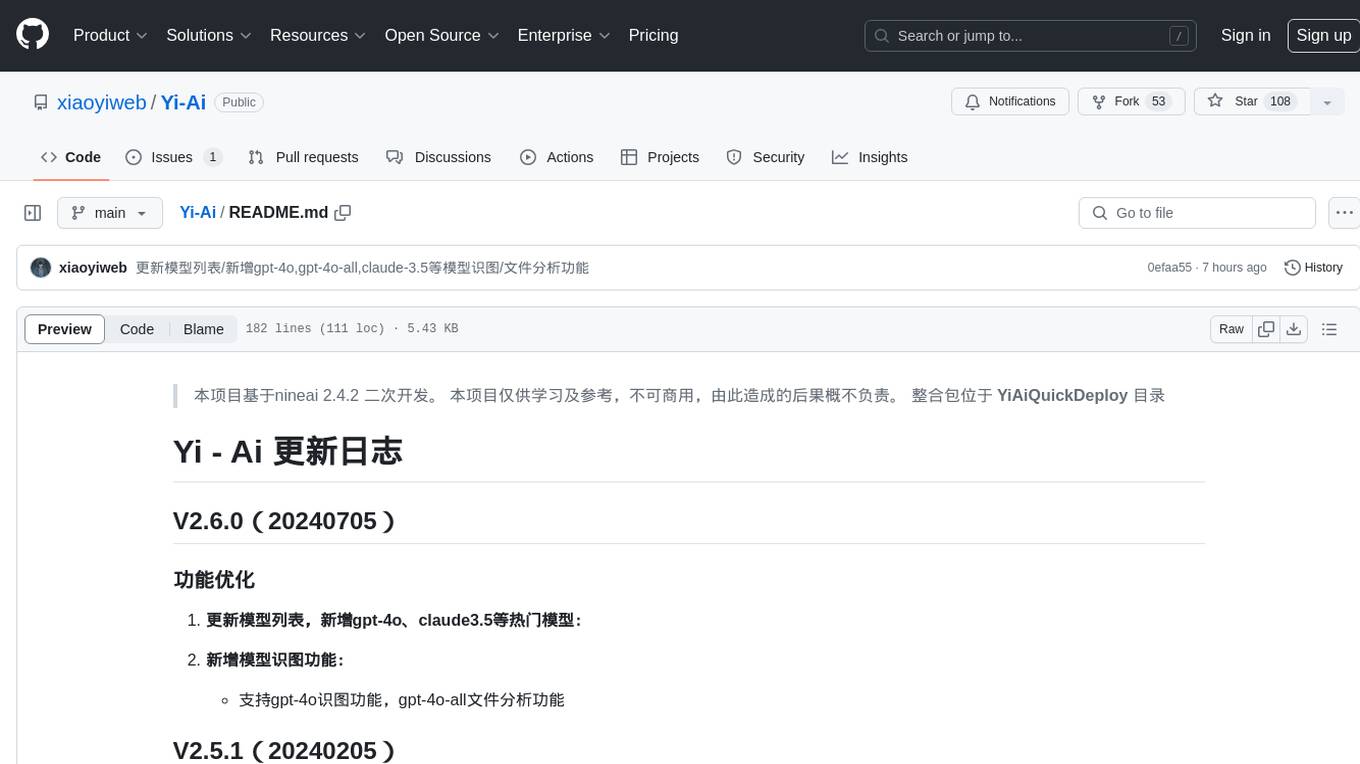
Yi-Ai
Yi-Ai is a project based on the development of nineai 2.4.2. It is for learning and reference purposes only, not for commercial use. The project includes updates to popular models like gpt-4o and claude3.5, as well as new features such as model image recognition. It also supports various functionalities like model sorting, file type extensions, and bug fixes. The project provides deployment tutorials for both integrated and compiled packages, with instructions for environment setup, configuration, dependency installation, and project startup. Additionally, it offers a management platform with different access levels and emphasizes the importance of following the steps for proper system operation.
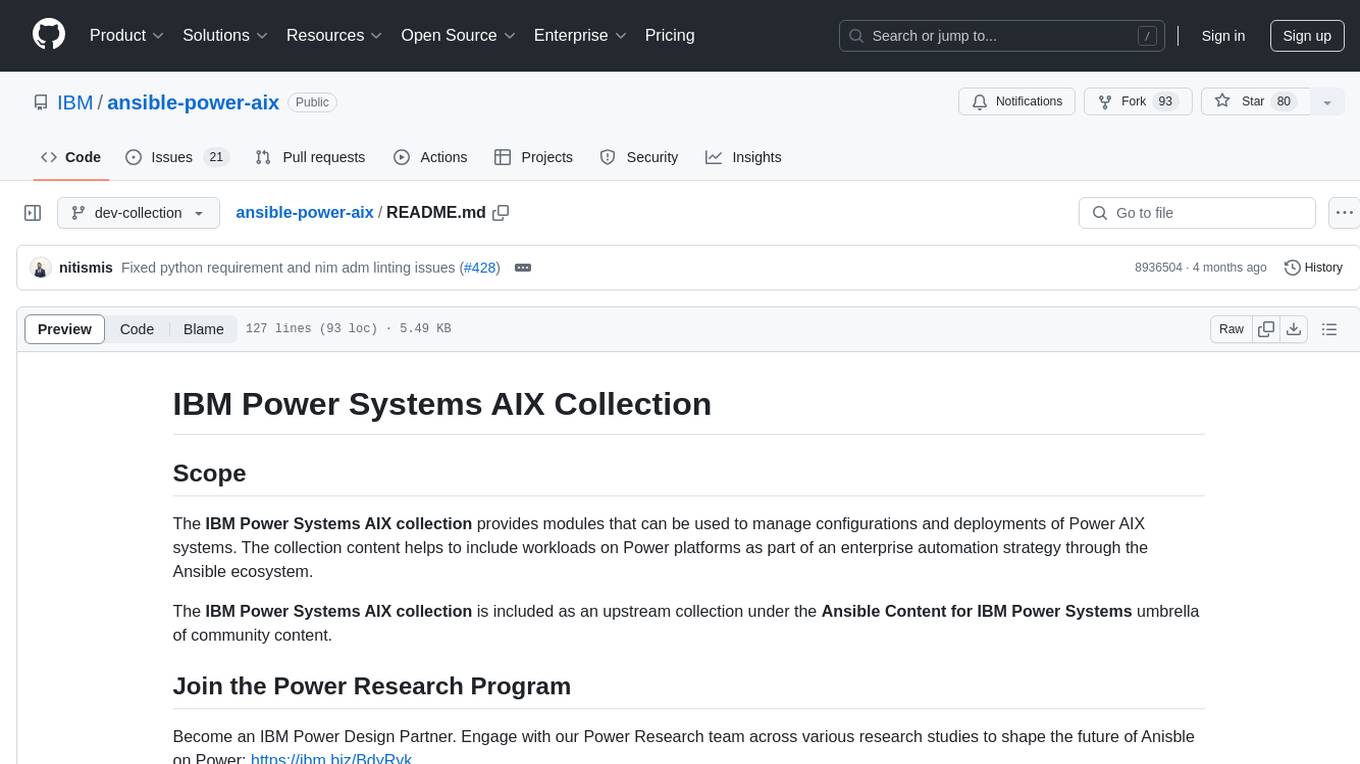
ansible-power-aix
The IBM Power Systems AIX Collection provides modules to manage configurations and deployments of Power AIX systems, enabling workloads on Power platforms as part of an enterprise automation strategy through the Ansible ecosystem. It includes example best practices, requirements for AIX versions, Ansible, and Python, along with resources for documentation and contribution.
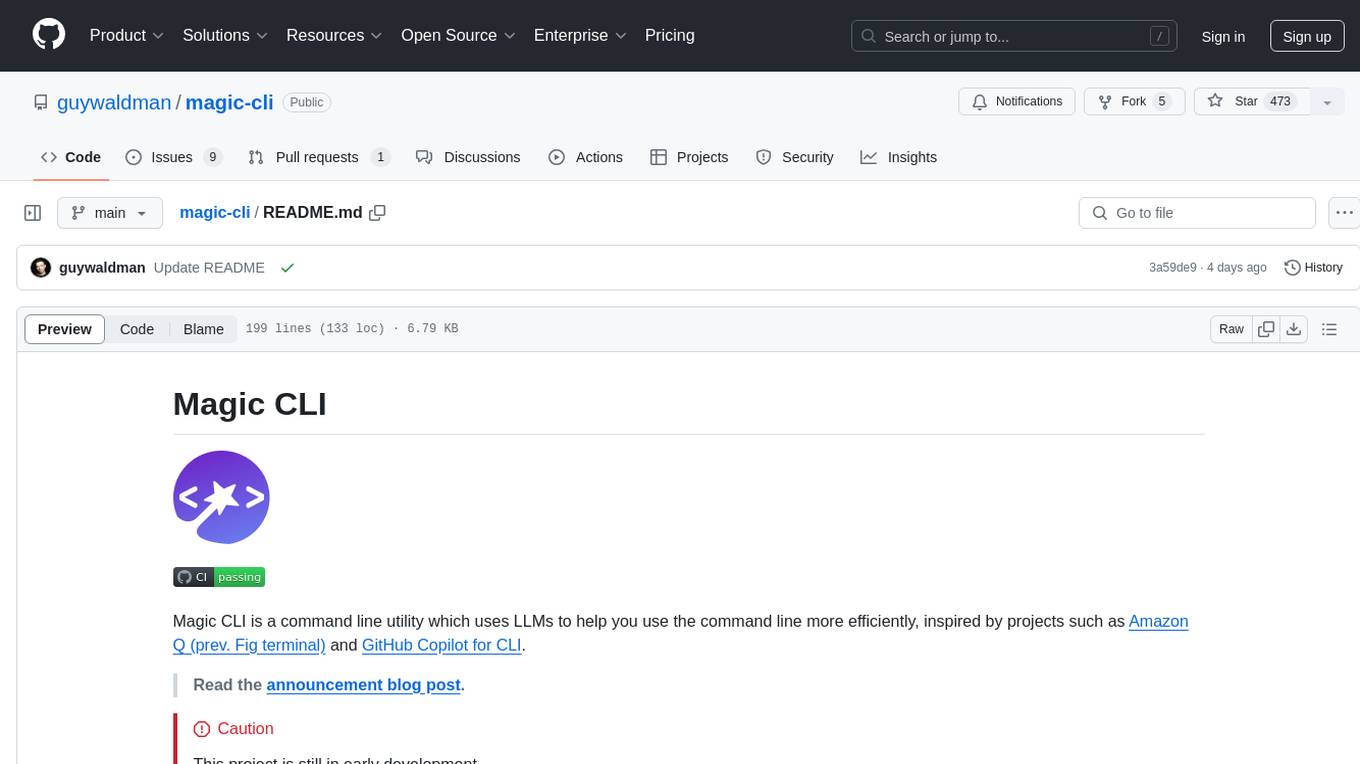
magic-cli
Magic CLI is a command line utility that leverages Large Language Models (LLMs) to enhance command line efficiency. It is inspired by projects like Amazon Q and GitHub Copilot for CLI. The tool allows users to suggest commands, search across command history, and generate commands for specific tasks using local or remote LLM providers. Magic CLI also provides configuration options for LLM selection and response generation. The project is still in early development, so users should expect breaking changes and bugs.
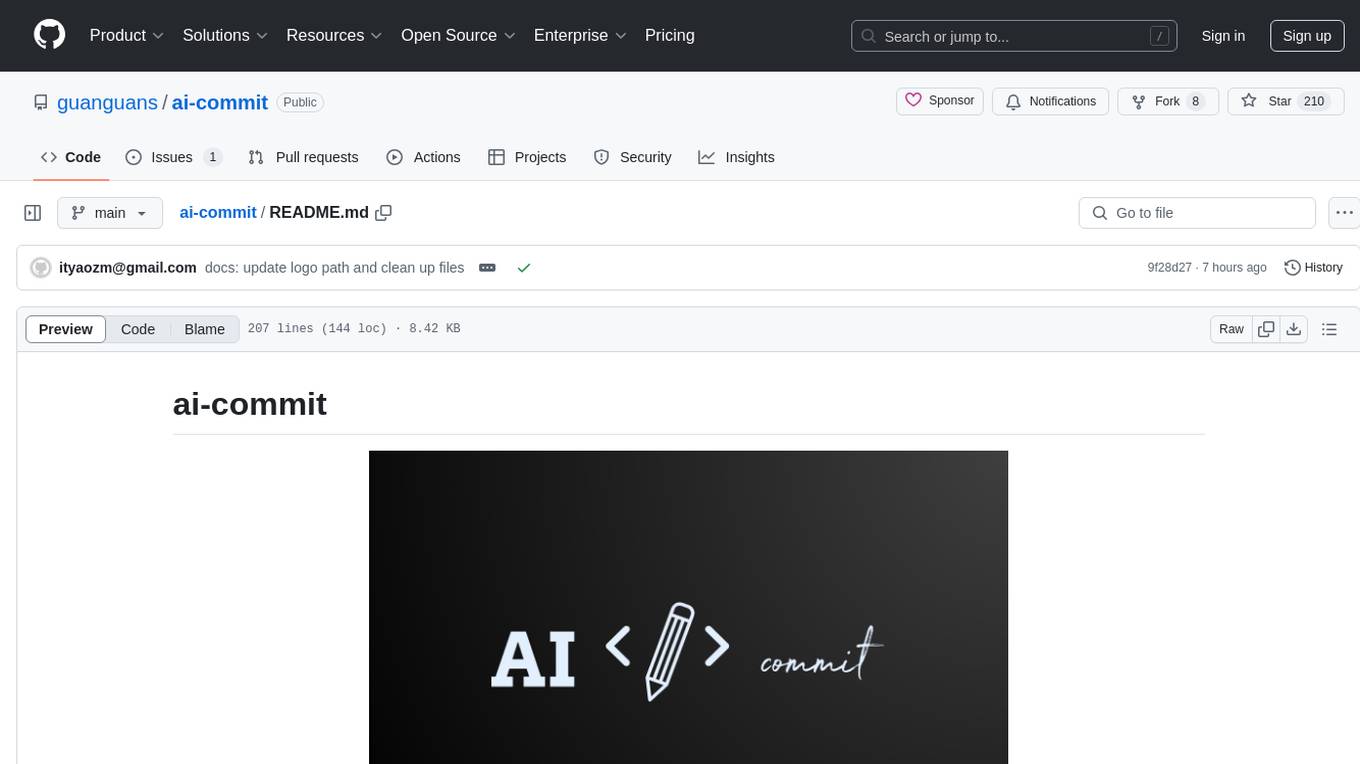
ai-commit
ai-commit is a tool that automagically generates conventional git commit messages using AI. It supports various generators like Bito Cli, ERNIE-Bot-turbo, ERNIE-Bot, Moonshot, and OpenAI Chat. The tool requires PHP version 7.3 or higher for installation. Users can configure generators, set API keys, and easily generate and commit messages with customizable options. Additionally, ai-commit provides commands for managing configurations, self-updating, and shell completion scripts.
For similar jobs
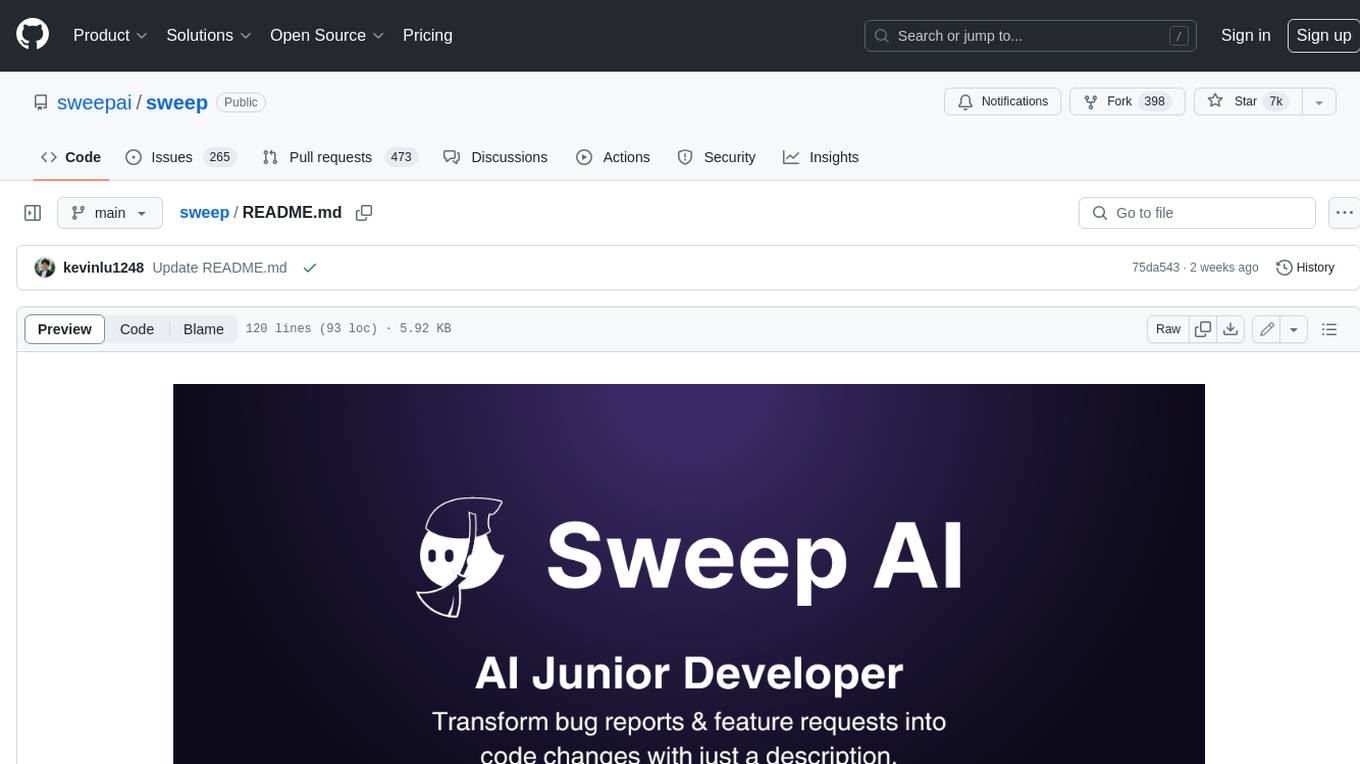
sweep
Sweep is an AI junior developer that turns bugs and feature requests into code changes. It automatically handles developer experience improvements like adding type hints and improving test coverage.
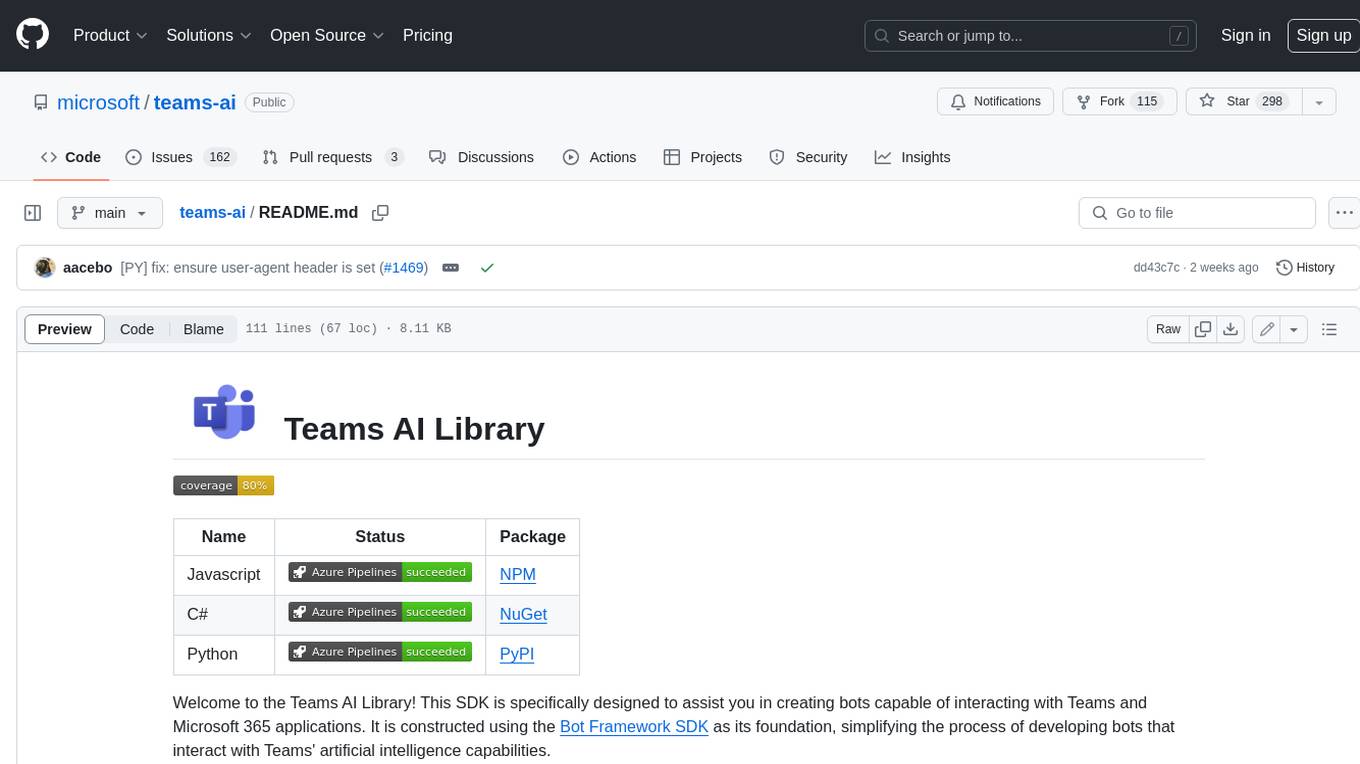
teams-ai
The Teams AI Library is a software development kit (SDK) that helps developers create bots that can interact with Teams and Microsoft 365 applications. It is built on top of the Bot Framework SDK and simplifies the process of developing bots that interact with Teams' artificial intelligence capabilities. The SDK is available for JavaScript/TypeScript, .NET, and Python.
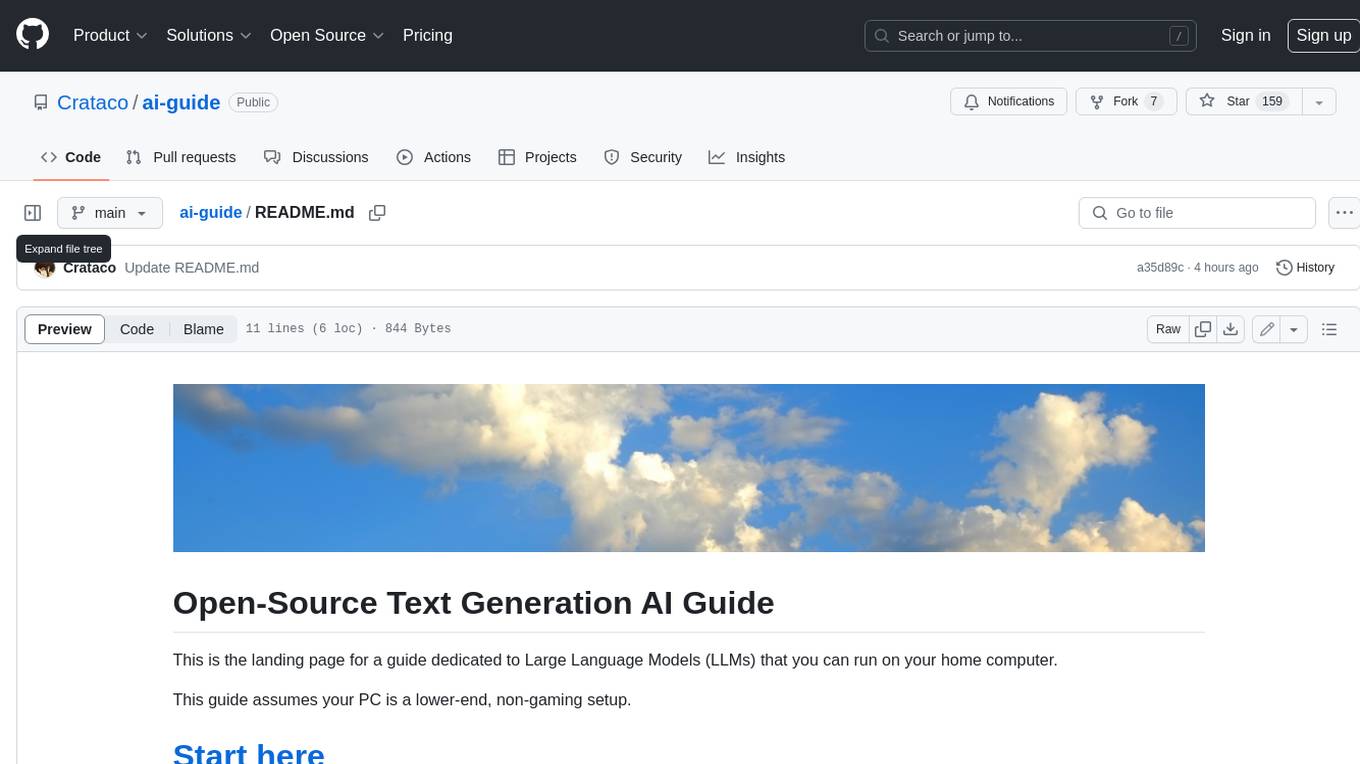
ai-guide
This guide is dedicated to Large Language Models (LLMs) that you can run on your home computer. It assumes your PC is a lower-end, non-gaming setup.
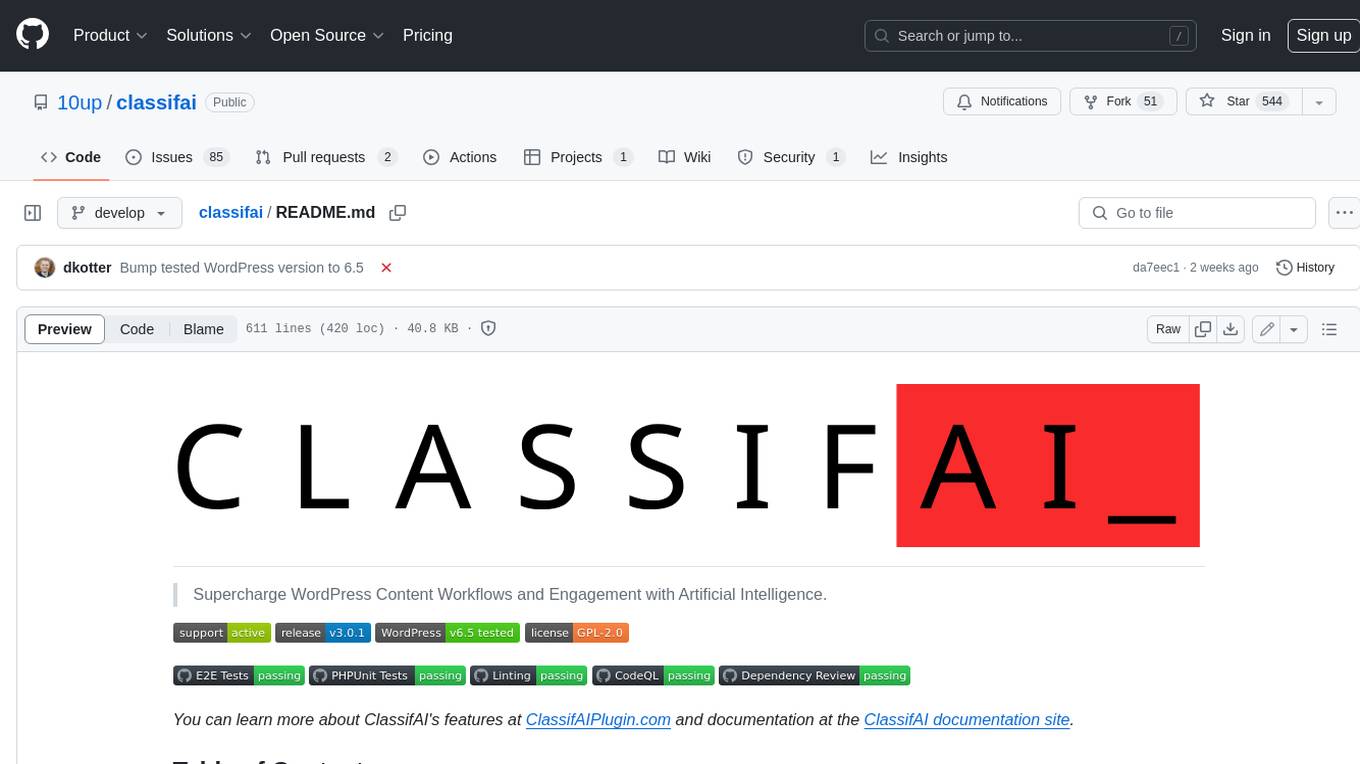
classifai
Supercharge WordPress Content Workflows and Engagement with Artificial Intelligence. Tap into leading cloud-based services like OpenAI, Microsoft Azure AI, Google Gemini and IBM Watson to augment your WordPress-powered websites. Publish content faster while improving SEO performance and increasing audience engagement. ClassifAI integrates Artificial Intelligence and Machine Learning technologies to lighten your workload and eliminate tedious tasks, giving you more time to create original content that matters.
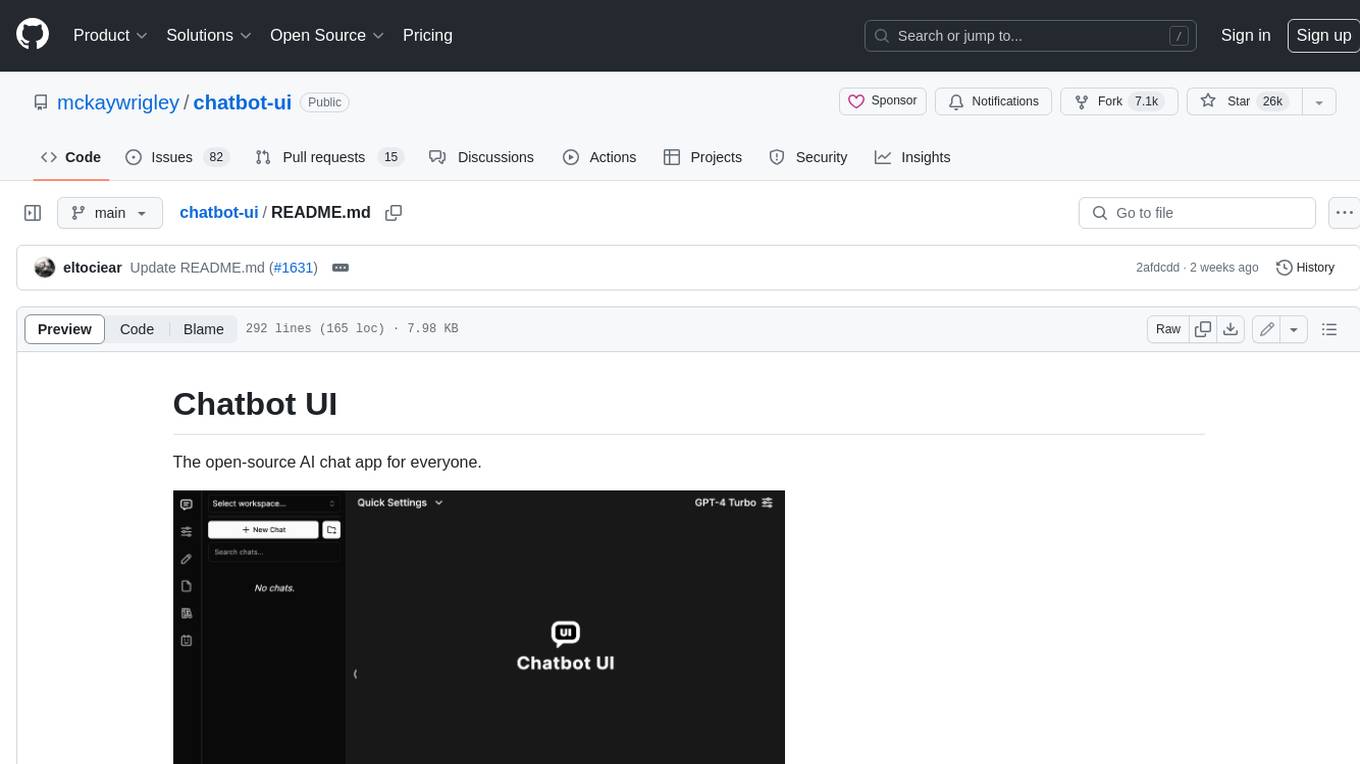
chatbot-ui
Chatbot UI is an open-source AI chat app that allows users to create and deploy their own AI chatbots. It is easy to use and can be customized to fit any need. Chatbot UI is perfect for businesses, developers, and anyone who wants to create a chatbot.
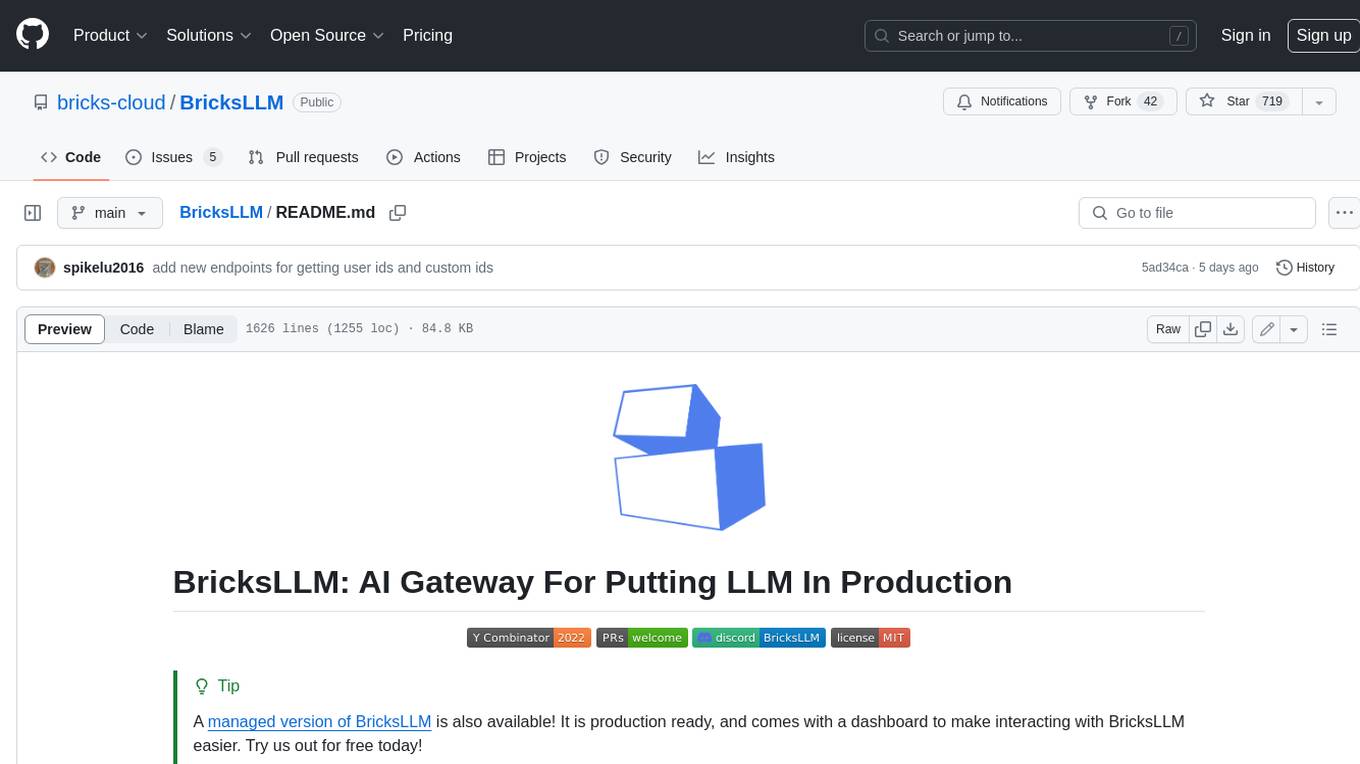
BricksLLM
BricksLLM is a cloud native AI gateway written in Go. Currently, it provides native support for OpenAI, Anthropic, Azure OpenAI and vLLM. BricksLLM aims to provide enterprise level infrastructure that can power any LLM production use cases. Here are some use cases for BricksLLM: * Set LLM usage limits for users on different pricing tiers * Track LLM usage on a per user and per organization basis * Block or redact requests containing PIIs * Improve LLM reliability with failovers, retries and caching * Distribute API keys with rate limits and cost limits for internal development/production use cases * Distribute API keys with rate limits and cost limits for students
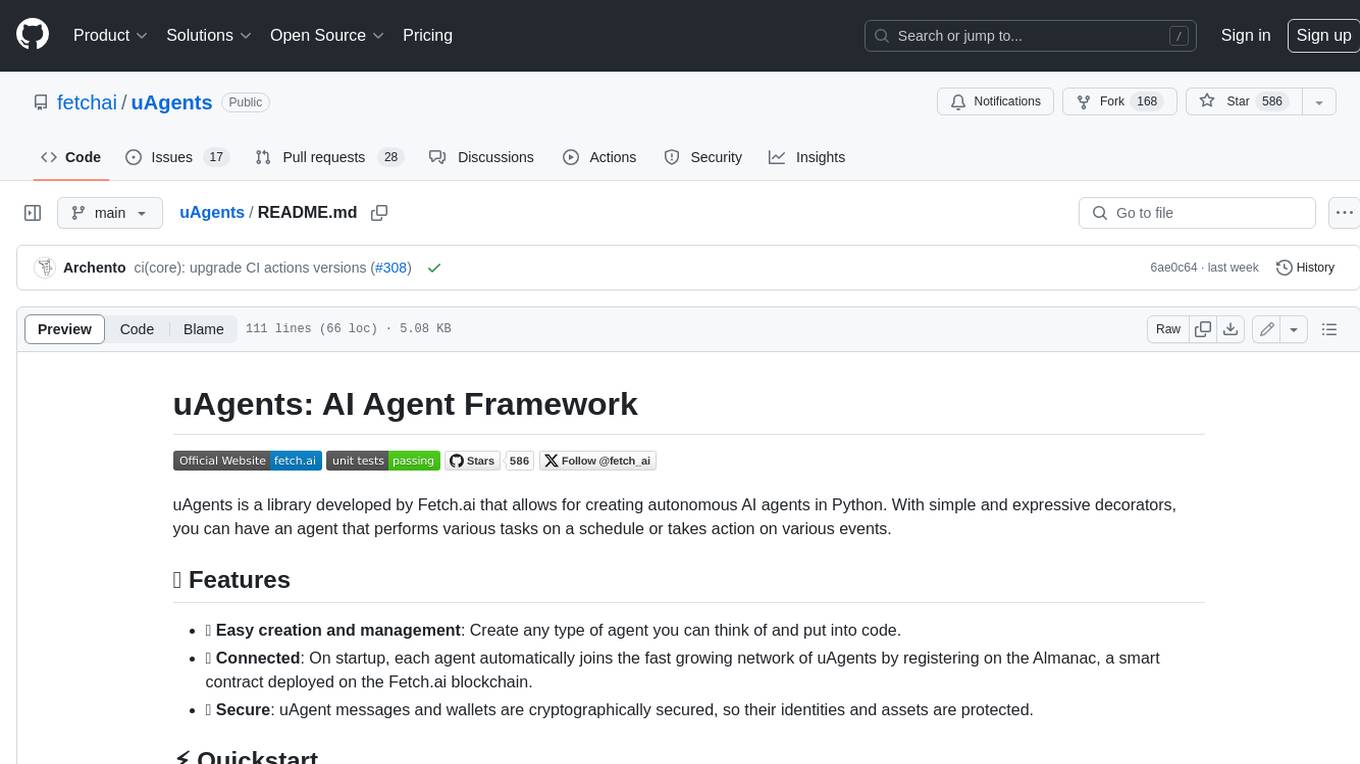
uAgents
uAgents is a Python library developed by Fetch.ai that allows for the creation of autonomous AI agents. These agents can perform various tasks on a schedule or take action on various events. uAgents are easy to create and manage, and they are connected to a fast-growing network of other uAgents. They are also secure, with cryptographically secured messages and wallets.
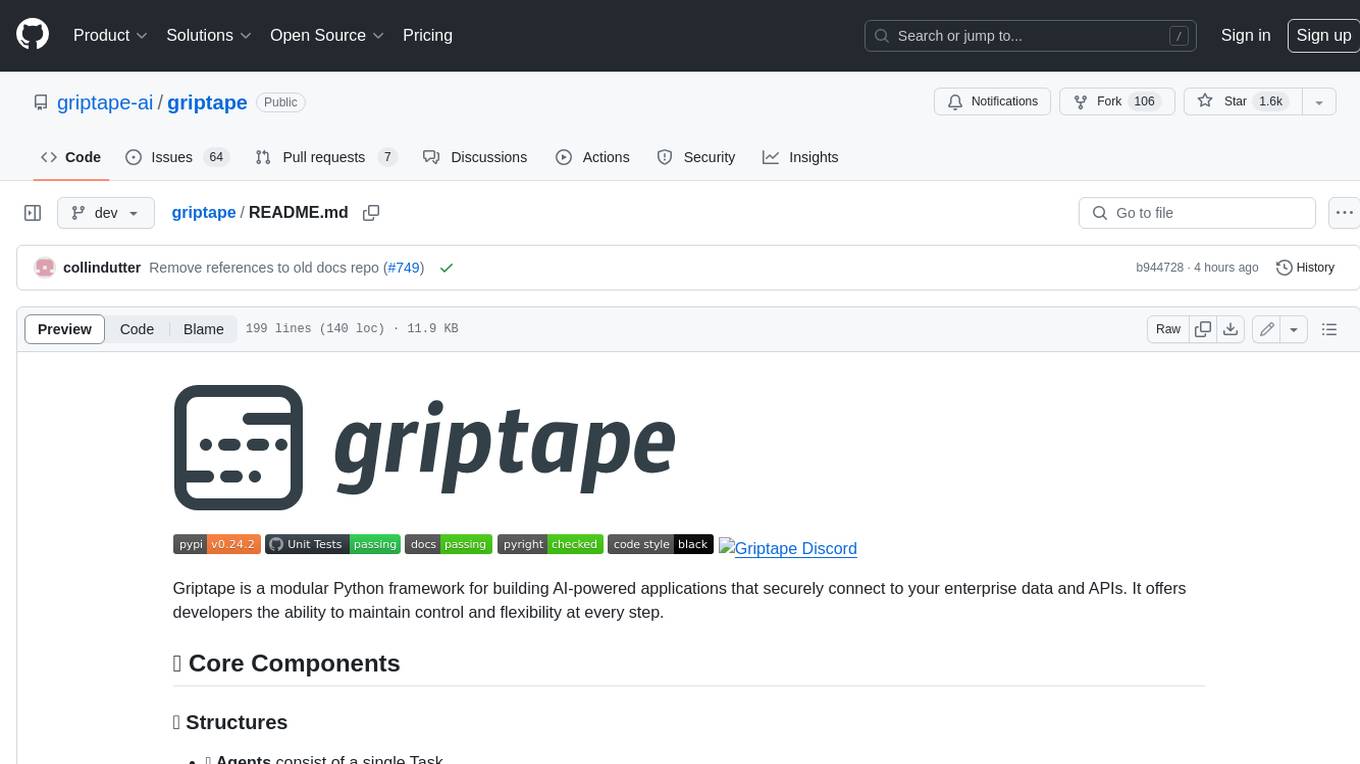
griptape
Griptape is a modular Python framework for building AI-powered applications that securely connect to your enterprise data and APIs. It offers developers the ability to maintain control and flexibility at every step. Griptape's core components include Structures (Agents, Pipelines, and Workflows), Tasks, Tools, Memory (Conversation Memory, Task Memory, and Meta Memory), Drivers (Prompt and Embedding Drivers, Vector Store Drivers, Image Generation Drivers, Image Query Drivers, SQL Drivers, Web Scraper Drivers, and Conversation Memory Drivers), Engines (Query Engines, Extraction Engines, Summary Engines, Image Generation Engines, and Image Query Engines), and additional components (Rulesets, Loaders, Artifacts, Chunkers, and Tokenizers). Griptape enables developers to create AI-powered applications with ease and efficiency.